diff --git a/spaces/1acneusushi/gradio-2dmoleculeeditor/data/Archicad 22 The Ultimate Guide for Architectural Design.md b/spaces/1acneusushi/gradio-2dmoleculeeditor/data/Archicad 22 The Ultimate Guide for Architectural Design.md deleted file mode 100644 index 5751f3d279e5a9c81aa31daafc9fa4e961430a63..0000000000000000000000000000000000000000 --- a/spaces/1acneusushi/gradio-2dmoleculeeditor/data/Archicad 22 The Ultimate Guide for Architectural Design.md +++ /dev/null @@ -1,53 +0,0 @@ - -
Archicad 22 is a powerful software for architectural design and documentation. It allows you to create 3D models, 2D drawings, and BIM data for your projects. Archicad 22 also has many features and tools to help you optimize your workflow and enhance your creativity.
-Download Zip ———>>> https://byltly.com/2uKyA2
In this article, we will show you some of the basics of using Archicad 22 for architectural design. We will cover how to:
-By the end of this article, you should have a good understanding of how to use Archicad 22 for architectural design. Let's get started!
- -The first step in using Archicad 22 is to set up a new project. To do this, you need to:
- -You have now set up a new project in Archicad 22. You can start designing your building in the next step.
- -The next step in using Archicad 22 is to create the building elements that make up your structure. You can use the tools in the Toolbox palette to draw walls, slabs, roofs, columns, beams, stairs, railings, and other elements. To create a building element, you need to:
-You have now created some building elements in Archicad 22. You can add more details and objects to your model in the next step.
- -The next step in using Archicad 22 is to add objects to your model. Objects are predefined or custom-made components that represent doors, windows, furniture, fixtures, appliances, plants, cars, people, and other items. You can use the Object tool or the Library Manager palette to insert objects into your model. To add an object, you need to:
-You have now added some objects to your model in Archicad 22. You can apply materials and colors to your model in the next step.
- -The next step in using
ddb901b051GTA 5 is one of the most popular and exciting games in the world. It is an open-world action-adventure game that lets you explore the city of Los Santos and its surrounding areas. You can play as three different characters: Michael, Franklin and Trevor, each with their own story and skills. You can also switch between them at any time and experience different missions, activities and challenges.
-Download ===== https://byltly.com/2uKykq
However, if you live in Myanmar, you may have some difficulties in downloading GTA 5. This is because the game is not officially available in the country due to some legal issues. Moreover, the internet speed and bandwidth in Myanmar are not very reliable, which can make the download process very slow and frustrating.
-But don't worry, there are still some ways to download GTA 5 in Myanmar. In this article, we will show you how to do it step by step.
-A VPN (Virtual Private Network) is a service that allows you to connect to a server in another country and access the internet from there. This way, you can bypass the geo-restrictions and censorship that may prevent you from downloading GTA 5 in Myanmar.
-Here are the steps to use a VPN to download GTA 5 in Myanmar:
-A torrent is a file that contains information about other files that are shared by users on a peer-to-peer network. You can use a torrent client such as BitTorrent or uTorrent to download these files on your device.
- -However, using a torrent to download GTA 5 in Myanmar is not recommended for several reasons. First of all, it is illegal and may violate the copyright laws of both Myanmar and the country where GTA 5 is produced. Secondly, it may expose you to malware and viruses that can harm your device and data. Thirdly, it may not guarantee the quality and performance of the game.
-If you still want to use a torrent to download GTA 5 in Myanmar, do it at your own risk. Here are the steps to do it:
-If you are a fan of role-playing games (RPGs) created with RPG Maker MV, you may have encountered some image files with the extension .rpgmvp. These are encrypted PNG files that are used by the game engine to protect the assets from modification. However, sometimes you may want to convert these files to JPG format for various purposes, such as viewing, editing, sharing, or printing.
-In this article, we will show you how to convert rpgmvp to jpg using different methods, both online and offline. We will also explain the advantages and disadvantages of each method, and how to use an encryption key if you have one. By the end of this article, you will be able to choose the best rpgmvp to jpg converter software for your needs.
-Download File 🔗 https://urlin.us/2uSTSd
One of the easiest ways to convert rpgmvp to jpg is to use an online converter tool. There are many websites that offer this service for free, but we recommend using Docpose RPGMVP Converter, which is fast, secure, and simple. Here are the steps to follow:
-The pros of using an online converter are:
-The cons of using an online converter are:
-rpgmvp to jpg converter online free
-rpgmvp to jpg converter github
-rpgmvp to jpg converter mac
-rpgmvp to jpg converter windows
-rpgmvp to jpg converter linux
-rpgmvp to jpg converter software
-rpgmvp to jpg converter tool
-rpgmvp to jpg converter app
-rpgmvp to jpg converter apk
-rpgmvp to jpg converter exe
-rpgmvp to jpg converter python
-rpgmvp to jpg converter rust
-rpgmvp to jpg converter petschko
-rpgmvp to jpg converter catink123
-rpgmvp to jpg converter fileproinfo
-rpgmvp to jpg converter batch
-rpgmvp to jpg converter command line
-rpgmvp to jpg converter drag and drop
-rpgmvp to jpg converter open source
-rpgmvp to jpg converter no watermark
-rpgmvp to jpg converter without encryption key
-rpgmvp to jpg converter decrypter
-rpgmvp to jpg converter encryption key finder
-rpgmvp to jpg converter for android
-rpgmvp to jpg converter for ios
-rpgmvp to jpg converter for windows 10
-rpgmvp to jpg converter for mac os x
-rpgmvp to jpg converter for linux ubuntu
-rpgmvp to jpg converter for chromebook
-rpgmvp to jpg converter for web browser
-rpgmvp to jpg converter for RPG Maker MV games
-rpgmvp to jpg converter for RPG Maker MZ games
-rpgmvp to jpg converter for RPG Maker VX Ace games
-rpgmvp to jpg converter for RPG Maker XP games
-rpgmvp to jpg converter for RPG Maker 2003 games
-how to convert rpgmvp to jpg online free
-how to convert rpgmvp to jpg on mac
-how to convert rpgmvp to jpg on windows
-how to convert rpgmvp to jpg on linux
-how to convert rpgmvp to jpg using github tools
-how to convert rpgmvp to jpg using software tools
-how to convert rpgmvp to jpg using command line tools
-how to convert rpgmvp files into JPG files easily and quickly
If you prefer to convert rpgmvp to jpg offline, you can use a program that runs on your device. One of the best options is rpgmvp_converter, which is a simple program that can convert an (almost) proprietary picture format from the RPG Maker V game engine. Here are the steps to follow:
-rpgmvp_converter path_to_your_file
, replacing path_to_your_file
with the actual path to your file. For example, if your file is called picture.rpgmvp, you would enter this in terminal: < code>rpgmvp_converter picture.rpgmvp.The pros of using an offline converter are:
-The cons of using an offline converter are:
-Some rpgmvp files may be encrypted with a key that prevents them from being converted by normal methods. This is usually done by the game developers to protect their intellectual property. However, if you have the permission and the key to decrypt these files, you can use a tool called Petschko RPG-Maker MV-Decrypter, which is a web-based application that can decrypt and encrypt RPG Maker MV files. Here are the steps to follow:
-var encryptionKey = 'xxxxxxxxxxxxxxxxxxxxxxxxxxxxxxxx';
, where xxxxxxxxxxxxxxxxxxxxxxxxxxxxxxxx is the key.The pros of using a decrypter with key are:
-The cons of using a decrypter with key are:
-In this article, we have shown you how to convert rpgmvp to jpg using different methods, both online and offline. We have also explained the advantages and disadvantages of each method, and how to use an encryption key if you have one. We hope that this article has helped you choose the best rpgmvp to jpg converter software for your needs.
-If you want to learn more about rpgmvp files and how they work, you can check out this [article] by Petschko, which explains the technical details of the encryption process. You can also visit [RPG Maker Web], which is the official website of RPG Maker MV, where you can find more resources, tutorials, and community support for creating your own RPGs.
-Thank you for reading this article. If you have any questions or feedback, please leave a comment below. Happy gaming!
-RPGMVP files are encrypted PNG files that are used by RPG Maker MV game engine. PNG files are standard image files that can be opened by any image viewer or editor. RPGMVP files need to be decrypted before they can be converted to other formats, such as JPG.
-The easiest way to open rpgmvp files without converting them is to play the RPG Maker MV game that contains them. You can also use a program called [RPG Maker MV Player], which is a free application that allows you to play any RPG Maker MV game without installing it on your device.
-The best settings for rpgmvp to jpg conversion depend on your purpose and preference. Generally, you want to balance between image quality and file size. JPG files use lossy compression, which means that some data is lost when converting from png to jpg. Therefore, you may notice some loss of detail, sharpness, or color accuracy in the converted jpg files. To minimize this, you can choose a higher quality setting for the jpg output, such as 80% or 90%. However, this will also increase the file size, which may affect the loading speed or storage space of your device. To reduce the file size, you can choose a lower quality setting, such as 50% or 60%, but this will also reduce the image quality. You can experiment with different settings until you find the optimal balance for your needs.
-If you want to publish your converted jpg files on the web, such as on a blog, a website, or a social media platform, you may want to optimize them for faster loading and better performance. There are several ways to do this, such as:
-You can use various online tools or programs to perform these optimization tasks, such as [TinyJPG], [Image Resizer], [Crop Image], [Progressive JPEG Converter], or [Cloudflare].
-If you are a game developer who uses RPG Maker MV to create your own RPGs, you may want to protect your rpgmvp files from unauthorized use, such as copying, modifying, or distributing them without your permission. There are several ways to do this, such as:
-However, you should also be aware that none of these methods are foolproof, and there may be ways to bypass or break them. Therefore, you should always keep a backup of your original rpgmvp files and monitor their usage and distribution. You should also respect the rights and wishes of other game developers who use RPG Maker MV and do not use their rpgmvp files without their permission.
197e85843dIf you are looking for a way to spice up your online entertainment, you might want to check out APK Bar Bar. This is an app that lets you watch live streams of various hosts from different countries, such as Indonesia, Vietnam, Thailand, Russia, Korea, America, and more. You can interact with them, send gifts, chat with other viewers, and enjoy a variety of content.
-In this article, we will show you how to download APK Bar Bar safely and easily on your Android device. We will also explain what APK Bar Bar is, why you might want to download it, how to install and use it, and answer some frequently asked questions. So, let's get started!
-Download ★★★★★ https://urlin.us/2uSS5M
APK Bar Bar is an app that allows you to watch live broadcasts of various hosts from different countries. You can choose from a wide range of categories, such as music, dance, comedy, gaming, beauty, lifestyle, education, and more. You can also filter by language, region, gender, age, popularity, and other criteria.
-Some of the features and benefits of using APK Bar Bar are:
-You might be wondering why you should download APK Bar Bar when there are so many other live streaming apps available. Well, here are some of the reasons why APK Bar Bar stands out from the crowd:
-So, if you are looking for a live streaming app that offers you more than just entertainment, APK Bar Bar is the one for you!
-Now that you know what APK Bar Bar is and why you should download it, let's see how you can do it safely and easily on your Android device. Here are the steps you need to follow:
-Note: Downloading APK files from unknown sources can be risky and dangerous for your device's security and performance. Make sure you have a reliable antivirus or anti-malware app installed on your device before downloading any APK files. Also, scan the APK file before installing it to make sure it is safe and clean.
-Installing APK Bar Bar is easy, but how do you use it effectively and efficiently? Here are some tips on how to use APK Bar Bar:
-download apk navigation bar
-download apk live bar bar indonesia
-download apk status bar
-download apk sound bar
-download apk live bar bar vietnam
-download apk action bar
-download apk live bar bar thailand
-download apk bottom bar
-download apk live bar bar rusia
-download apk volume bar
-download apk live bar bar korea
-download apk swipe bar
-download apk live bar bar amerika
-download apk notification bar
-download apk live bar bar bebas parah
-download apk search bar
-download apk live bar bar no sensor
-download apk gesture bar
-download apk live bar bar 2023
-download apk control center ios 14 - screen recorder, assistive touch, night mode, screen capture, video recorder, screenshot, screen recorder with audio, virtual home button, touch screen anywhere with one hand mode, lock screen, quick settings, smart control - iphone x control center, music control, brightness control, volume control, auto rotation, flashlight, do not disturb mode, calculator, camera, alarm clock and more.
-download apk live streaming hot - watch live stream videos of talented stars from all over the world on your phone. You can chat with them and send gifts to show your support. You can also join the fun by broadcasting your own talents and skills. Whether you like singing, dancing, gaming, cooking, or anything else, you can find your audience here. You can also follow your favorite streamers and get notified when they go live. Join the community and enjoy the best live entertainment on your phone.
-download apk floating toolbar - a handy tool that allows you to access various functions and apps from anywhere on your screen. You can customize the toolbar with your favorite apps and shortcuts. You can also adjust the size, position, and transparency of the toolbar. You can use the floating toolbar to launch apps, switch tasks, take screenshots, record videos, adjust settings, and more. You can also hide the toolbar when you don't need it.
-download apk video editor - a powerful and easy-to-use video editing app that lets you create amazing videos with your photos and clips. You can trim, crop, rotate, merge, split, add music, apply filters, stickers, texts, transitions, effects, and more. You can also adjust the speed, brightness, contrast, saturation, and other parameters of your videos. You can export your videos in HD quality and share them on social media platforms.
-download apk music player - a stylish and feature-rich music player app that lets you enjoy your favorite songs on your phone. You can browse and play music by albums, artists, genres, playlists, folders, and more. You can also create and edit your own playlists. You can customize the sound quality with the equalizer and bass booster. You can also change the theme and appearance of the music player according to your preference.
-download apk photo editor - a fun and creative photo editing app that lets you enhance your pictures with various tools and effects. You can crop, resize, rotate, flip, adjust color, brightness,
Using APK Bar Bar is simple and fun, but make sure you follow the rules and guidelines of the app and respect the hosts and other users. Do not engage in any inappropriate or abusive behavior, such as spamming, trolling, harassing, bullying, or violating any laws or regulations. If you encounter any such behavior, report it immediately and block the user.
-APK Bar Bar is an app that lets you watch live streams of various hosts from different countries and interact with them. It offers you a unique and diverse online entertainment experience that you can enjoy for free. You can also send gifts, follow your favorite hosts, discover new content, and join a vibrant and multicultural community.
-If you are interested in trying out APK Bar Bar, you can download it safely and easily from its official website at https://apkbarbar.com/. You can also follow the steps we have provided in this article to install and use it on your Android device. We hope you have fun with APK Bar Bar and share your feedback with us!
-Thank you for reading this article. We hope you found it helpful and informative. If you have any questions or comments, please feel free to leave them below. We would love to hear from you!
-To use APK Bar Bar, you need an Android device that runs on Android 4.4 or higher and has at least 1 GB of RAM and 100 MB of free storage space. You also need a stable internet connection and a valid email address or phone number to create an account.
-APK Bar Bar is safe and legal as long as you download it from its official website at https://apkbarbar.com/. Do not download it from any other websites or sources, as they might contain viruses, malware, or other harmful elements. Also, make sure you scan the APK file before installing it to make sure it is safe and clean.
-You can earn coins or diamonds on APK Bar Bar by completing tasks or watching ads. You can also buy them with real money through various payment methods. Coins or diamonds are used to send gifts to your favorite hosts or exchange them for cash or other rewards.
-You can contact APK Bar Bar customer service by tapping on the "Settings" icon at the top left corner of the screen and then tapping on "Feedback". You can also email them at apkbarbar@gmail.com or visit their Facebook page at https://www.facebook.com/apkbarbar.
-You can delete your APK Bar Bar account by tapping on the "Settings" icon at the top left corner of the screen and then tapping on "Account". You will see an option that says "Delete Account". Tap on it and confirm your decision. Once you delete your account, all your data and history will be erased and you will not be able to recover them.
197e85843dIf you are a fan of Standoff 2, the dynamic first-person shooter game with realistic graphics and animation, you might have wondered what it would be like to open more cases and boxes and get rare and powerful skins for your weapons. Well, wonder no more, because there is a game that lets you do just that: Case Simulator 2 Standoff.
-Case Simulator 2 Standoff is a simulation game that mimics the case opening mechanics of Standoff 2. You can open all kinds of cases and boxes, from the basic ones to the newest collections, and get a chance to win skins, stickers, charms, and even knives. You can also simulate battles in the background to earn gold and other items, and try your luck in various game modes such as Upgrade, Jackpot, Crash, Quiz, Tower, Bomb Defuse, and more.
-Download Zip … https://jinyurl.com/2uNKnu
In this article, we will give you a brief overview of what Case Simulator 2 Standoff is, what features it has, how to play it, and some tips and tricks to help you get the most out of it. We will also answer some frequently asked questions about the game. So, let's get started!
-Case Simulator 2 Standoff is a game created by fans of Standoff 2 for fans of Standoff 2. It is not an official game by the developers of Standoff 2, nor is it affiliated with them in any way. It is simply a fun and entertaining way to experience the thrill of opening cases and boxes without spending real money or affecting your progress in the original game.
-скачать кейс симулятор 2 стандофф на пк
-скачать кейс симулятор 2 стандофф мод много денег
-скачать кейс симулятор 2 стандофф последняя версия
-скачать кейс симулятор 2 стандофф бесплатно
-скачать кейс симулятор 2 стандофф взлом
-скачать кейс симулятор 2 стандофф на андроид
-скачать кейс симулятор 2 стандофф на ios
-скачать кейс симулятор 2 стандофф на компьютер
-скачать кейс симулятор 2 стандофф на телефон
-скачать кейс симулятор 2 стандофф на windows
-как скачать кейс симулятор 2 стандофф
-где скачать кейс симулятор 2 стандофф
-отзывы о кейс симулятор 2 стандофф
-обзор кейс симулятор 2 стандофф
-видео про кейс симулятор 2 стандофф
-играть в кейс симулятор 2 стандофф онлайн
-играть в кейс симулятор 2 стандофф без интернета
-играть в кейс симулятор 2 стандофф без регистрации
-играть в кейс симулятор 2 стандофф бесплатно
-играть в кейс симулятор 2 стандофф на пк
-как играть в кейс симулятор 2 стандофф
-как выигрывать в кейс симулятор 2 стандофф
-как получить легендарное оружие в кейс симулятор 2 стандофф
-как открыть все кейсы в кейс симулятор 2 стандофф
-как заработать голду в кейс симулятор 2 стандофф
-коды для кейс симулятор 2 стандофф
-читы для кейс симулятор 2 стандофф
-хаки для кейс симулятор 2 стандофф
-трюки для кейс симулятор 2 стандофф
-подсказки для кейс симулятор 2 стандофф
-руководство по кейс симулятор 2 стандофф
-инструкция по кейс симулятор 2 стандофвв
-установить кейс симулятор 2 стандоввв
-обновить кейс симулятор 2 становвв
-удалить кейс симулятор 2 становвв
-переустановить кейс симулятор 2 становвв
-запустить кейс симулятор 2 становвв
-остановить кейс симулятор 2 становвв
-выйти из кейс симулятор 2 становвв
-перезапустить кейс симулятор 2 становвв
The game is available for Android devices on Google Play Store , and for iOS devices on App Store . You can also play it online on Yandex Games . The game is free to download and play, but it contains ads and offers in-app purchases.
-Case Simulator 2 Standoff has many features that make it an enjoyable and addictive game for anyone who loves Standoff 2. Here are some of them:
-Playing Case Simulator 2 Standoff is very easy and intuitive. Here are the basic steps to get you started:
-To help you get the most out of Case Simulator 2 Standoff, here are some tips and tricks that you might find useful:
-Here are some frequently asked questions about Case Simulator 2 Standoff and their answers:
-Case Simulator 2 Standoff is a game that simulates opening cases and boxes from the popular first-person shooter game Standoff 2. You can get various skins, stickers, charms, and knives for your weapons and test your luck in different game modes. You can also simulate battles in the background to earn gold and other items, and buy, sell, trade, or exchange items with other players in the marketplace. The game is free to play, but it contains ads and offers in-app purchases.
-If you are a fan of Standoff 2, you might enjoy playing Case Simulator 2 Standoff as a way to experience the thrill of opening cases and boxes without spending real money or affecting your progress in the original game. You can also challenge yourself in different game modes and compete with other players. You can also share your results with your friends on social media.
-We hope that this article has given you a brief overview of what Case Simulator 2 Standoff is, what features it has, how to play it, and some tips and tricks to help you get the most out of it. We also hope that we have answered some of your questions about the game. If you have any more questions or feedback, feel free to leave a comment below. Thank you for reading!
197e85843dIf you are looking for a fun and smart sci-fi show to watch, you might want to check out Eureka. This is a series that follows the adventures of a U.S. Marshal who becomes the sheriff of a secret town where the best minds in America work on cutting-edge inventions for the government. In this article, we will tell you what Eureka is, why you should watch it, and where you can download season 1 legally and safely.
-Download ->>> https://jinyurl.com/2uNPHn
Eureka is an American science fiction comedy drama that aired on Syfy from 2006 to 2012. The show is set in a fictional town called Eureka, located in Oregon, where the most brilliant scientists and engineers live and work on various projects for the Department of Defense. The town is also home to Global Dynamics, a top-secret research facility that houses some of the most advanced technologies in the world.
-The show's protagonist is Jack Carter, a U.S. Marshal who stumbles upon Eureka while transporting his rebellious daughter Zoe back to Los Angeles. He soon discovers that the town is not as normal as it seems, and that he has to deal with strange phenomena, rogue experiments, and eccentric residents on a daily basis. He also develops a friendship with Henry Deacon, a jack-of-all-trades who knows everything about Eureka, and a romantic interest in Allison Blake, a Department of Defense agent who oversees Global Dynamics.
-Other main characters include Jo Lupo, Carter's deputy sheriff who is a former Army Ranger; Douglas Fargo, a clumsy but brilliant scientist who works at Global Dynamics; Nathan Stark, a Nobel Prize-winning physicist who is Allison's ex-husband and rival; Zoe Carter, Jack's teenage daughter who attends Eureka High School; Jim Taggart, an Australian zoologist who specializes in capturing dangerous creatures; and Beverly Barlowe, a psychiatrist who has ulterior motives.
-The first season of Eureka consists of 12 episodes that were aired from July to October in 2006. Here is the list of the episodes and their titles:
-Eureka is not your typical sci-fi show. It is not only about futuristic gadgets and scientific concepts, but also about humor, drama, and human relationships. It is a show that can make you laugh, think, and feel at the same time. It is a show that can appeal to a wide range of audiences, from sci-fi fans to comedy lovers, from adults to kids, from casual viewers to binge-watchers.
-Some of the benefits of watching a sci-fi comedy drama like Eureka are:
-download eureka season 1 episodes
-download eureka season 1 free online
-download eureka season 1 full hd
-download eureka season 1 amazon prime
-download eureka season 1 peacock premium
-download eureka season 1 vudu
-download eureka season 1 apple tv
-download eureka season 1 google play movies
-download eureka season 1 microsoft store
-download eureka season 1 torrent
-download eureka season 1 netflix
-download eureka season 1 hulu
-download eureka season 1 dvd
-download eureka season 1 blu-ray
-download eureka season 1 pilot episode
-download eureka season 1 finale episode
-download eureka season 1 extended version
-download eureka season 1 webisodes
-download eureka season 1 behind the scenes
-download eureka season 1 cast interviews
-download eureka season 1 subtitles
-download eureka season 1 english subtitles
-download eureka season 1 spanish subtitles
-download eureka season 1 french subtitles
-download eureka season 1 german subtitles
-download eureka season 1 trailer
-download eureka season 1 promo
-download eureka season 1 sneak peek
-download eureka season 1 recap
-download eureka season 1 review
-download eureka season 1 ratings
-download eureka season 1 soundtrack
-download eureka season 1 theme song
-download eureka season 1 bloopers
-download eureka season 1 deleted scenes
-download eureka season 1 fan art
-download eureka season 1 fan fiction
-download eureka season 1 trivia quiz
-download eureka season 1 script pdf
-download eureka season 1 wallpaper hd
Eureka is not only a fan-favorite show, but also a critically acclaimed one. The show has received positive reviews and ratings from both critics and viewers alike. Here are some of the accolades that the show has earned:
-Eureka is not only a popular show, but also a prestigious one. The show has been recognized by various organizations and institutions for its excellence in different aspects of television production. Here are some of the awards and nominations that the show has received:
-Award | Category | Year | Result |
---|---|---|---|
Primetime Emmy Award | Outstanding Special Visual Effects for a Series | 2007 | Nominated |
Primetime Emmy Award | Outstanding Special Visual Effects for a Series | 2008 | Nominated |
Primetime Emmy Award | Outstanding Special Visual Effects for a Series | 2010 | Nominated |
Primetime Emmy Award | Outstanding Original Main Title Theme Music | 2011 | Nominated |
Saturn Award | Best Syndicated/Cable Television Series | 2008 | Nominated |
Saturn Award | Best Syndicated/Cable Television Series | 2010 | Nominated |
Visual Effects Society Award | Outstanding Visual Effects in a Broadcast Series | 2007 | Nominated |
Visual Effects Society Award | Outstanding Visual Effects in a Broadcast Series | 2008 | Nominated |
Hugo Award | Best Dramatic Presentation, Short Form (for episode "Your Face or Mine") | 2008 | Nominated |
Leo Award | Best Visual Effects in a Dramatic Series | 2009 | Won |
If you are interested in watching Eureka season 1, you might be wondering where you can download it legally and safely. The good news is that there are several options available for you to choose from, depending on your preferences and budget. Here are some of the legal and safe options for streaming or buying the show online:
-To help you decide which option is best for you, here is a comparison of the prices and features of different platforms that offer Eureka season 1:
-Platform | Price | Features |
---|---|---|
Amazon Prime Video | $19.99 (buy) / $9.99 (rent) / $0 (stream) | - Stream with Prime membership ($12.99/month or $119/year) - Download or watch online - HD quality - Closed captions - Watch on multiple devices - No ads |
iTunes | $19.99 (buy) / N/A (rent) / N/A (stream) | - Download or watch online - HD quality - Closed captions - Watch on multiple devices - No ads |
Google Play | $19.99 (buy) / N/A (rent) / N/A (stream) | - Download or watch online - HD quality - Closed captions - Watch on multiple devices - No ads |
Vudu | $19.99 (buy) / $9.99 (rent) / N/A (stream) | - Download or watch online - HD quality - Closed captions - Watch on multiple devices - No ads |
Here is a table of the download options and their links for Eureka season 1:
-Platform | Link |
---|---|
Amazon Prime Video | [Download Eureka Season 1 on Amazon Prime Video] |
iTunes | [Download Eureka Season 1 on iTunes] |
Google Play | [Download Eureka Season 1 on Google Play] |
Vudu | [Download Eureka Season 1 on Vudu] |
Eureka is a sci-fi comedy drama that you don't want to miss. It is a show that combines science, humor, and heart in a unique and entertaining way. It is a show that features a talented cast, a creative plot, and a stunning visual effects. It is a show that has received rave reviews and awards from critics and fans alike.
-If you are interested in watching Eureka season 1, you have several options to download it legally and safely. You can stream it on Amazon Prime Video, or buy or rent it on iTunes, Google Play, or Vudu. You can compare the prices and features of different platforms, and choose the one that suits you best. You can also click on the links provided in the table above to access the download options directly.
-So what are you waiting for? Download Eureka season 1 today, and enjoy the amazing adventures of Jack Carter and his friends in the town of Eureka. You will not regret it!
-A1: No, Eureka is not based on a true story. It is a fictional show that was created by Andrew Cosby and Jaime Paglia. However, some of the scientific concepts and inventions that are featured in the show are inspired by real-life research and technology.
-A2: There are five seasons in Eureka, with a total of 77 episodes. The show ran from 2006 to 2012, and ended with a special Christmas episode.
-A3: The creators of Eureka are Andrew Cosby and Jaime Paglia, who also served as executive producers and writers for the show. The stars of Eureka are Colin Ferguson as Jack Carter, Salli Richardson-Whitfield as Allison Blake, Joe Morton as Henry Deacon, Erica Cerra as Jo Lupo, Neil Grayston as Douglas Fargo, Ed Quinn as Nathan Stark, Jordan Hinson as Zoe Carter, Matt Frewer as Jim Taggart, and Debrah Farentino as Beverly Barlowe.
-A4: The spin-off series of Eureka is Warehouse 13, which is another sci-fi comedy drama that aired on Syfy from 2009 to 2014. Warehouse 13 is about a secret government facility that stores supernatural artifacts collected from around the world. The show has crossed over with Eureka several times, featuring guest appearances from some of the characters.
-A5: Eureka was filmed mostly in Vancouver, British Columbia, Canada. Some of the locations that were used for filming include Burnaby Village Museum, Riverview Hospital, Lynn Canyon Park, Britannia Beach, and Fort Langley.
197e85843dFinal Thoughts is a Punjabi song by AP Dhillon, Shinda Kahlon, and Gminxr, released in 2022. The song is a heartfelt expression of love, regret, and hope, as the singer reflects on his past relationship and wishes for a better future. The song is part of AP Dhillon's album Hidden Gems, which showcases his versatility and talent as a singer, rapper, songwriter, and record producer.
-The song has gained immense popularity among Punjabi music lovers, as well as listeners from other regions and countries. The song has over 100 million views on YouTube, and has also been featured on various music streaming services, such as Spotify, Apple Music, Amazon Music, and more. You can listen to the song on any of these platforms, or download it legally from websites that offer free music downloads, such as [SoundCloud](^1^), [Amazon](^2^), or [Spotify](^3^).
-Download ❤ https://jinyurl.com/2uNJNP
The lyrics of Final Thoughts are written by Shinda Kahlon, who is also a singer and rapper. The lyrics are in Punjabi, with some English words mixed in. The lyrics convey a mix of emotions, such as sadness, nostalgia, anger, guilt, forgiveness, and optimism. The singer addresses his ex-girlfriend, who has left him for someone else. He tells her that he still loves her, but he also blames her for breaking his heart. He admits that he made some mistakes, but he also asks her to remember the good times they had together. He hopes that she will come back to him someday, but he also wishes her happiness with her new partner. He says that these are his final thoughts before he moves on with his life.
-Some of the key lines and verses from the song are:
-The music of Final Thoughts is composed by Gminxr, who is also a record producer and DJ. The music is in the genre of hip hop, with elements of trap, R&B, and pop. The music is catchy, upbeat, and melodic, with a smooth and soothing vocal delivery by AP Dhillon. The music also features some samples and effects, such as sirens, gunshots, and echoes, that add to the mood and intensity of the song. The music is well-produced and mixed, with a clear and balanced sound quality.
-final thoughts ap dhillon lyrics
-final thoughts ap dhillon mp3 download
-final thoughts ap dhillon shazam
-final thoughts ap dhillon spotify
-final thoughts ap dhillon youtube
-final thoughts ap dhillon shinda kahlon gminxr
-final thoughts ap dhillon apple music
-final thoughts ap dhillon run-up records
-final thoughts ap dhillon video
-final thoughts ap dhillon soundcloud
-final thoughts ap dhillon genius
-final thoughts ap dhillon ringtone
-final thoughts ap dhillon instrumental
-final thoughts ap dhillon reaction
-final thoughts ap dhillon remix
-final thoughts ap dhillon chords
-final thoughts ap dhillon karaoke
-final thoughts ap dhillon meaning
-final thoughts ap dhillon 320kbps
-final thoughts ap dhillon mr jatt
-final thoughts ap dhillon punjabi song
-final thoughts ap dhillon two hearts never break the same
-final thoughts ap dhillon tiktok
-final thoughts ap dhillon instagram
-final thoughts ap dhillon whatsapp status
-final thoughts ap dhillon english translation
-final thoughts ap dhillon amazon music
-final thoughts ap dhillon gaana
-final thoughts ap dhillon hungama
-final thoughts ap dhillon wynk music
-final thoughts ap dhillon jiosaavn
-final thoughts ap dhillon pandora
-final thoughts ap dhillon deezer
-final thoughts ap dhillon tidal
-final thoughts ap dhillon napster
-final thoughts ap dhillon iheartradio
-final thoughts ap dhillon last.fm
-final thoughts ap dhillon musixmatch
-final thoughts ap dhillon audiomack
-final thoughts ap dhillon bandcamp
-final thoughts ap dhillon vimeo
-final thoughts ap dhillon vevo
-final thoughts ap dhillon facebook
-final thoughts ap dhillon twitter
-final thoughts ap dhillon snapchat
-final thoughts ap dhillon reddit
-final thoughts ap dhillon quora
-final thoughts ap dhillon pinterest
-final thoughts ap dhillon tumblr
The video of Final Thoughts is directed by Sukh Sanghera, who is also a filmmaker and photographer. The video is shot in various locations, such as a beach, a park, a street, and a house. The video shows AP Dhillon singing and rapping the song, while also acting out some scenes from his past relationship with his ex-girlfriend. The video also features some other actors and models, who play the roles of his friends, his new girlfriend, and his ex-girlfriend's new boyfriend. The video is well-edited and cinematographed, with a vibrant and colorful visual style.
-The impact and reception of Final Thoughts have been phenomenal and positive. The song has influenced the Punjabi music industry and culture by bringing a fresh and unique perspective to the genre of hip hop. The song has also showcased the talent and potential of AP Dhillon and his collaborators, who have been praised for their creativity and originality. The song has also inspired many young and aspiring artists to pursue their passion and dreams in music.
-The song has been received by critics and fans with admiration and appreciation. The song has been hailed as one of the best Punjabi songs of 2022, and one of the most emotional and relatable songs ever. The song has also been lauded for its lyrics, music, video, and performance, which have been described as captivating, powerful, authentic, and impressive. The song has also been nominated for several awards and honors, such as the BritAsia TV Music Awards, the PTC Punjabi Music Awards, the Mirchi Music Awards, and more.
-The song has performed well on various charts and platforms, both nationally and internationally. The song has topped the charts in India, Canada, UK, Australia, New Zealand, and more. The song has also been featured on several playlists and radio stations around the world. The song has also broken several records and milestones, such as the most viewed Punjabi song on YouTube in 24 hours, the most streamed Punjabi song on Spotify in a week, the most liked Punjabi song on Instagram in a month, and more.
-In conclusion, Final Thoughts by AP Dhillon is a Punjabi song that will make you feel a range of emotions, from sadness to happiness. The song is a masterpiece of artistry and expression, that showcases the skills and talents of AP Dhillon and his collaborators. The song is also a hit among listeners and critics alike, who have praised it for its quality and impact. The song is definitely worth listening to and downloading, as it will touch your heart and soul.
-If you are looking for a Punjabi song that will make you feel something deep and real, then you should definitely check out Final Thoughts by AP Dhillon. You can download the song from any of the links below, or listen to it on your favorite music streaming service. You will not regret it, as it will make you appreciate the beauty and complexity of love and life.
-A: AP Dhillon is a Punjabi singer, rapper, songwriter, and record producer, based in Canada. He is known for his fusion of hip hop, R&B, and Punjabi music, and his collaborations with other artists, such as Gurinder Gill, Money Musik, Shinda Kahlon, and Gminxr. He is also the founder of Run-Up Records, an independent music label.
-A: The title Final Thoughts refers to the last thoughts that the singer has about his ex-girlfriend, before he decides to move on with his life. The title also implies that this is the final song that he will make about her, as he closes this chapter of his life.
-A: You can download Final Thoughts by AP Dhillon for free from websites that offer free music downloads, such as [SoundCloud], [Amazon], or [Spotify]. You can also use a YouTube to MP3 converter to download the song from YouTube. However, you should always respect the rights and wishes of the artists and support them by buying their music legally.
-A: Some other songs by AP Dhillon that you should listen to are:
-Song | Album | Year |
---|---|---|
Majhail | Hidden Gems | 2022 |
Brown Munde | Brown Munde | 2021 |
All Good | All Good | 2021 |
Excuses | Excuses | 2020 |
Tinted Windows | Tinted Windows | 2020 |
A: You can find more information about AP Dhillon and his music on his official website [apdhillon.com], or on his social media accounts, such as [Instagram], [Twitter], [Facebook], or [YouTube]. You can also follow his music label Run-Up Records on [Instagram] or [YouTube].
401be4b1e0Los modelos 3D son representaciones digitales de objetos que se pueden ver, manipular y representar en tres dimensiones. Se utilizan para diversos fines, como diseño, ingeniería, entretenimiento, educación, arte y más. Los modelos 3D pueden ayudarte a visualizar tus ideas, crear simulaciones realistas, probar tus productos y mostrar tu trabajo.
-Download Zip ✶ https://bltlly.com/2v6JB6
Pero ¿cómo puedes acceder a modelos 3D? ¿Y cómo puedes usarlos para tus propios proyectos? En este artículo, le mostraremos cómo descargar modelos 3D desde la web, cómo usarlos para diferentes aplicaciones y cuáles son los beneficios del modelado 3D.
-Una de las formas más fáciles de obtener modelos 3D es descargarlos desde la web. Hay muchos sitios web que ofrecen modelos 3D gratuitos o de pago que puede descargar y usar para fines personales o comerciales. Sin embargo, antes de descargar cualquier modelo 3D, debe considerar dos cosas: el formato de archivo y la licencia.
-Hay cientos de formatos de archivo 3D diferentes que almacenan información sobre modelos 3D, como geometría, textura, color, animación, etc. Algunos de los formatos de archivo 3D más populares son:
-Hay muchos sitios web que ofrecen modelos 3D gratuitos o de pago que puedes descargar y usar para tus proyectos. Algunos de los mejores son:
- -Estos son solo algunos ejemplos de los muchos sitios web que ofrecen modelos 3D para descargar. También puedes buscar modelos 3D en Google u otros motores de búsqueda usando palabras clave como "descarga de modelos 3D", "modelo 3D gratis", "sitio web de modelos 3D", etc.
-Una vez que haya descargado modelos 3D de la web, puede utilizarlos para diferentes aplicaciones dependiendo de sus necesidades y objetivos. Algunas de las aplicaciones más comunes son:
- -Si quieres crear tus propios modelos 3D o editar los que has descargado, necesitas usar un software de diseño 3D que te permita manipular la geometría, textura, color y otros aspectos de un modelo 3D. Algunos de los software de diseño 3D más populares son:
-Estos son solo algunos ejemplos de los muchos software de diseño 3D que puede utilizar para crear y editar modelos 3D. También puede encontrar más opciones en Google u otros motores de búsqueda mediante el uso de palabras clave como "software de diseño 3d", "software de diseño 3d gratuito", "mejor software de diseño 3d", etc.
-Si quieres convertir tus modelos 3D en objetos físicos que puedas tocar y sostener, necesitas usar una impresora 3D o un escáner 3D que te permita imprimir o escanear tus modelos 3D en un material de tu elección. Algunos de los dispositivos de impresión y escaneo 3D más populares son:
-Estos son solo algunos ejemplos de los muchos dispositivos de impresión y escaneo 3D que se pueden usar para prototipado físico y fabricación. También puedes encontrar más opciones en Google u otros motores de búsqueda usando palabras clave como "impresora 3d", "mejor impresora 3d", "escáner 3d", "mejor escáner 3d", etc.
-Si quieres crear experiencias inmersivas e interactivas con tus modelos 3D, necesitas usar un software de visualización y animación 3D que te permita representar, animar e interactuar con tus modelos 3D en tiempo real. Algunos de los software de visualización y animación 3D más populares son:
-Como puedes ver, el modelado 3D es una habilidad útil y divertida que puede ayudarte a crear cosas increíbles con tu imaginación. Puede descargar modelos 3D desde la web o crear su propio software de diseño 3D. También puede utilizarlos para diferentes aplicaciones, como impresión 3D, escaneo 3D, visualización 3D y animación 3D. También puede compartir sus modelos 3D con otros o venderlos en línea.
-El modelado 3D tiene muchos beneficios, como:
-Si quieres comenzar con el modelado 3D, necesitas tener una computadora, un software de diseño 3D y un sitio web de modelos 3D. También puede obtener una impresora 3D o un escáner 3D si desea imprimir o escanear sus modelos 3D. También puede encontrar muchos recursos en línea, como tutoriales, cursos, libros, blogs, foros, etc., que pueden ayudarlo a aprender los conceptos básicos y las habilidades avanzadas de modelado 3D.
-Aquí hay algunas preguntas frecuentes sobre el modelado 3D:
-El modelado 3D es un término general que se refiere a la creación y manipulación de representaciones digitales de objetos en tres dimensiones. CAD (Computer-Aided Design) es un tipo específico de modelado 3D que se utiliza para fines técnicos y de ingeniería, como el diseño de máquinas, edificios, circuitos, etc.
-Algunos de los mejores software de modelado 3D para principiantes son SketchUp, Tinkercad, Blender y Unity. Son gratuitos, fáciles de usar y ofrecen muchas características y funciones para crear y editar modelos 3D.
-El tiempo que toma aprender modelado 3D depende de muchos factores, como su experiencia anterior, su estilo de aprendizaje, sus objetivos, su elección de software, etc. Sin embargo, puede esperar aprender los fundamentos del modelado 3D en unas pocas semanas o meses si practica regularmente y sigue algunos tutoriales o cursos en línea.
-El costo de descargar o comprar modelos 3D varía dependiendo del sitio web, la licencia, la calidad, la complejidad y la popularidad del modelo 3D. Algunos sitios web ofrecen modelos 3D gratuitos bajo licencias de Creative Commons, lo que significa que puede usarlos para fines personales o comerciales, siempre y cuando dé crédito al creador original. Algunos sitios web ofrecen modelos 3D pagados bajo licencias libres de derechos o editoriales, lo que significa que puede usarlos para fines personales o comerciales sin dar crédito, pero con algunas restricciones dependiendo de los términos de la licencia. El precio de los modelos 3D pagados puede variar desde unos pocos dólares hasta cientos de dólares.
-Hay muchas maneras de ganar dinero con el modelado 3D, como:
-Estos son solo algunos ejemplos de las muchas maneras de hacer dinero con el modelado 3D. También puede encontrar más oportunidades en Google u otros motores de búsqueda mediante el uso de palabras clave como "cómo hacer dinero con el modelado 3D", "trabajos de modelado 3D", "carreras de modelado 3D", etc.
-Espero que este artículo te haya ayudado a entender cómo descargar y usar modelos 3D para tus proyectos. Si usted tiene alguna pregunta o comentario, por favor siéntase libre de dejarlos abajo. Gracias por leer y feliz modelado 3D!
64aa2da5cf¿Te gusta jugar y usar aplicaciones en tu dispositivo móvil? ¿Desea acceder a más características, funciones y contenido que las versiones regulares? ¿Quieres ahorrar dinero y evitar anuncios mientras disfrutas de tus aplicaciones y juegos favoritos? Si respondiste sí a cualquiera de estas preguntas, entonces deberías echar un vistazo a appking io, una plataforma donde puedes descargar juegos de mod y aplicaciones modificadas de forma gratuita. En este artículo, le diremos todo lo que necesita saber sobre appking io, incluyendo qué es, cómo usarlo y qué beneficios ofrece. También te presentaremos a AppKing APK, una aplicación que te permite jugar y ganar dinero al mismo tiempo. Sigue leyendo para saber más.
-Download >>>>> https://bltlly.com/2v6LMx
Appking io es un sitio web que proporciona juegos de mod y aplicaciones modificadas para dispositivos Android e iOS. Los juegos mod son juegos que han sido modificados por desarrolladores de terceros para desbloquear funciones premium, eliminar anuncios, agregar recursos ilimitados o cambiar el juego. Las aplicaciones modificadas son aplicaciones que se han modificado para mejorar su rendimiento, funcionalidad o apariencia. Por ejemplo, puedes descargar una versión modificada de Spotify que te permite escuchar música sin conexión, saltar anuncios y disfrutar de saltos ilimitados. Otro software que puede encontrar en appking io incluye VPN, emuladores, grabadoras de pantalla, editores de video y más.
-Appking io tiene muchas características que lo convierten en una gran plataforma para descargar juegos de mod y aplicaciones modificadas. Aquí están algunas de ellas:
-Appking io también tiene una amplia gama de aplicaciones modificadas que mejoran su experiencia con redes sociales, música, transmisión de video, mensajería, productividad, educación, salud, fitness y más. Puede descargar versiones modificadas de Facebook, Instagram, TikTok, YouTube, Netflix, WhatsApp, Spotify, Duolingo, Fitbit y muchos otros. Todas las aplicaciones modificadas son gratuitas de descargar y usar, y vienen con características mejoradas que las hacen más útiles y convenientes.
-Appking io no es solo sobre juegos de mod y aplicaciones modificadas. También ofrece otro software que puede ayudarlo con el rendimiento, la seguridad, la personalización, el entretenimiento y la creatividad de su dispositivo. Puede encontrar VPN que protegen su privacidad y seguridad en línea; emuladores que le permiten ejecutar aplicaciones Android o iOS en su PC; grabadoras de pantalla que le permiten capturar la actividad de la pantalla; editores de video que le permiten crear videos increíbles; y más.
- -Usar appking io es muy fácil y simple. Aquí están los requisitos y pasos:
-Para usar appking io, necesita tener un dispositivo Android o iOS con una conexión a Internet. También debe habilitar la instalación de aplicaciones de fuentes desconocidas en la configuración del dispositivo. Esto se debe a que appking io no está disponible en las tiendas de aplicaciones oficiales, y debe descargarlo desde su sitio web. Puede encontrar las instrucciones sobre cómo hacerlo en el sitio web appking io.
-Una vez que haya cumplido con los requisitos, puede seguir estos pasos para usar appking io:
-Appking io tiene muchos beneficios que lo convierten en una gran plataforma para descargar juegos de mod y aplicaciones modificadas. Estos son algunos de ellos:
-Todo el software que puedes descargar de appking io es gratuito. No tienes que pagar ninguna tarifa o suscripción para acceder a ellos. Tampoco tienes que preocuparte por los anuncios o las compras en la aplicación que pueden interrumpir tu experiencia o costarte dinero. Además, todo el software es seguro y seguro de usar. El equipo de appking io los prueba y verifica antes de subirlos al sitio web. No contienen ningún virus, malware o spyware que pueda dañar tu dispositivo o datos.
-Appking io tiene una gran variedad de software que puede adaptarse a sus preferencias y necesidades. Puedes encontrar juegos de mod y aplicaciones modificadas para diferentes categorías, géneros y propósitos. También puede encontrar otro software que le puede ayudar con diversas tareas y actividades. Todo el software es de alta calidad y rendimiento. Se actualizan regularmente para corregir cualquier error o errores y para agregar nuevas características y contenido. También son compatibles con la mayoría de dispositivos y versiones Android e iOS.
-Appking io proporciona actualizaciones y soporte para sus usuarios. Puede recibir notificaciones cuando hay nuevas versiones o actualizaciones disponibles para el software que ha descargado. También puede ponerse en contacto con el equipo de appking io si tiene preguntas, comentarios o problemas con respecto a su software. Ellos le responderán lo antes posible y le ayudarán a resolver sus problemas. También puede unirse a la comunidad de usuarios de appking io y compartir sus opiniones, sugerencias o experiencias con su software.
-AppKing APK es una aplicación que le permite jugar juegos y ganar dinero en línea. Es desarrollado por AppKing Studio, una compañía que se especializa en crear aplicaciones de juegos con recompensas reales. AppKing APK tiene una variedad de juegos que usted puede elegir, tales como trivia, rompecabezas, árcade, casino, deportes, y más. Puedes jugar estos juegos gratis o con una pequeña cuota de inscripción. Puedes ganar premios en efectivo en función de tu puntuación, rango o suerte. Puede retirar sus ganancias a través de PayPal u otros métodos.
-AppKing APK tiene muchas características que lo convierten en una gran aplicación para jugar y ganar dinero. Estos son algunos de ellos:
-AppKing APK tiene un montón de juegos divertidos y fáciles que se pueden jugar en cualquier momento y en cualquier lugar. No necesitas ninguna habilidad o conocimiento especial para jugar a estos juegos. Solo necesitas seguir las instrucciones y usar tu lógica, memoria, reflejos o intuición. También puede elegir entre diferentes niveles de dificultad y modos de juego para adaptarse a sus preferencias y estado de ánimo.
-AppKing APK le da recompensas reales e instantáneas para jugar. Usted puede ganar premios en efectivo
AppKing APK le da recompensas reales e instantáneas para jugar juegos. Usted puede ganar premios en efectivo que van desde $0.01 a $1000 dependiendo del juego y el resultado. También puede ganar monedas que puede cambiar por dinero en efectivo o tarjetas de regalo. Puede retirar sus ganancias a través de PayPal u otros métodos en 24 horas. No tienes que preocuparte por cargos ocultos.
-Usar AppKing APK es muy fácil y simple. Aquí están los requisitos y pasos:
-Para utilizar AppKing APK, es necesario tener un dispositivo Android con una conexión a Internet. También necesitas tener una cuenta PayPal u otros métodos de pago para retirar tus ganancias. Puede descargar AppKing APK desde su sitio web oficial o de otras fuentes. Sin embargo, debe asegurarse de que la fuente sea confiable y confiable.
-Una vez que haya cumplido con los requisitos, puede seguir estos pasos para usar AppKing APK:
-AppKing APK tiene muchos beneficios que lo convierten en una gran aplicación para jugar y ganar dinero. Estos son algunos de ellos:
-AppKing APK le proporciona entretenimiento e ingresos al mismo tiempo. Puede jugar juegos divertidos y fáciles que se adapten a su gusto y estado de ánimo. También puedes ganar premios en efectivo reales y monedas que puedes usar para tus necesidades o deseos. Puedes disfrutar jugando y ganando dinero sin problemas ni estrés.
-AppKing APK garantiza su seguridad y transparencia cuando se trata de jugar y ganar dinero. Protege su información personal y detalles de pago de cualquier acceso no autorizado o mal uso. También te proporciona información clara y precisa sobre los juegos, las recompensas, las reglas y los términos y condiciones. No oculta ninguna tarifa o cargo.
-En conclusión, appking io es una plataforma donde puedes descargar juegos de mod y aplicaciones modificadas de forma gratuita. Tiene muchas características, como juegos de mod, aplicaciones modificadas, otro software, descargas gratuitas y seguras, variedad y calidad de software, actualizaciones y soporte, etc. Es fácil de usar, ya que solo necesita visitar su sitio web, elegir el software que desea descargar, instalarlo en su dispositivo, y disfrutar de sus características. AppKing APK es una aplicación que le permite jugar juegos y ganar dinero en línea. Tiene muchas características, como juegos divertidos y fáciles, recompensas reales e instantáneas, programas de referencia y bonos, etc. Es fácil de usar, ya que solo necesita descargar e instalar la aplicación, registrarse con su correo electrónico o cuenta de Facebook, jugar juegos, retirar sus ganancias, invitar a tus amigos, y unirse a programas de bonificación. Tanto appking io y AppKing APK son excelentes plataformas para descargar juegos de mod y aplicaciones modificadas y jugar juegos y ganar dinero. Tienen muchos beneficios, como entretenimiento e ingresos, flexibilidad y conveniencia, seguridad y transparencia, etc. También son gratuitos y seguros de usar. Si estás interesado en probarlos, puedes visitar sus sitios web y descargarlos desde allí. También puede encontrar más información y comentarios sobre ellos en línea. Esperamos que este artículo te haya ayudado a aprender más sobre appking io y AppKing APK. Si tiene alguna pregunta o comentario, no dude en ponerse en contacto con nosotros o dejar un comentario a continuación. Gracias por leer y tener un gran día!
Appking io es un sitio web que proporciona juegos de mod y aplicaciones modificadas para dispositivos Android e iOS. AppKing APK es una aplicación que le permite jugar juegos y ganar dinero en línea. Ambos son desarrollados por AppKing Studio, pero tienen diferentes propósitos y características.
-Appking io y AppKing APK son legales y seguros de usar siempre y cuando siga sus términos y condiciones y respete los derechos de propiedad intelectual de los desarrolladores originales del software que proporcionan. No contienen ningún virus, malware o spyware que pueda dañar su dispositivo o datos. Sin embargo, debes ser consciente de los riesgos que implica el uso de juegos mod y aplicaciones modificadas, como problemas de compatibilidad, errores, errores, prohibiciones o acciones legales.
-Puede descargar appking io y AppKing APK desde sus sitios web oficiales o de otras fuentes. Sin embargo, debe asegurarse de que la fuente sea confiable y confiable. También debe habilitar la instalación de aplicaciones de fuentes desconocidas en la configuración del dispositivo antes de instalarlas.
-Puede retirar sus ganancias de AppKing APK a través de PayPal u otros métodos en 24 horas. Usted necesita tener un saldo mínimo de $10 para solicitar un retiro. También necesitas verificar tu identidad y detalles de pago antes de recibir tu dinero.
-Puede ponerse en contacto con appking io o AppKing APK enviando un correo electrónico a su equipo de soporte en support@appking.io o llenando el formulario de contacto en su sitio web. También puedes seguirlos en sus cuentas de redes sociales o unirte a su comunidad de usuarios.
-Si usted es un suscriptor de Azercell, es posible que haya escuchado o experimentado un servicio llamado Simurq. Este servicio pretende ofrecerle actualizaciones sobre las últimas noticias, música, moda, celebridades, tendencias y mucho más. Sin embargo, es posible que no sea consciente de lo que realmente es Simurq, cómo funciona, cuánto cuesta y cómo puede afectar su teléfono y su billetera. En este artículo te explicaremos todo lo que necesitas saber sobre Simurq y por qué debes detenerlo lo antes posible.
-Simurq es un servicio que Azercell ofrece a sus clientes que tienen planes de prepago o pospago. Puede activar el servicio en la sección Azercell del menú del teléfono. Una vez que lo active, comenzará a recibir mensajes interactivos en la pantalla del teléfono que le ofrecerán diferentes tipos de contenido. El contenido es proporcionado por agencias de información conocidas, como Reuters, BBC, CNN, etc. El contenido incluye noticias, consejos, extractos de música, fotos, canciones populares y mucha otra información. Puede optar por ver o ignorar los mensajes, o responder con un código para obtener más detalles o descargar el contenido.
-Download Zip ⚡ https://bltlly.com/2v6L3r
Las transmisiones de Simurq son gratuitas, lo que significa que no se le cobrará por recibir los mensajes. Sin embargo, el costo del contenido ofrecido se indica en cada mensaje que reciba en su teléfono. Por ejemplo, si quieres descargar una canción o un video, tendrás que pagar una cierta cantidad de dinero que se deducirá de tu saldo. Los precios varían dependiendo del tipo y tamaño del contenido. Para más información, puede llamar al 6045. El primer minuto de la llamada es gratuito, pero la tarifa de servicio para cada minuto posterior es de 0,02 AZN. También puede comprobar su saldo marcando *100#YES.
- -Uno de los principales problemas con Simurq es que puede enviarte demasiados mensajes que quizás no quieras o no necesites. Los mensajes pueden ser intrusivos y molestos, especialmente si aparecen en su pantalla cuando está ocupado o espera una notificación importante. Los mensajes también pueden interferir con el rendimiento y la funcionalidad de su teléfono, ya que pueden ralentizar su dispositivo o causar problemas técnicos. Además, los mensajes pueden ser engañosos o inexactos, ya que pueden no reflejar sus preferencias o intereses, o pueden contener información obsoleta o falsa.
-Otro problema con Simurq es que puede hacerte pagar por contenido que no quieres o no necesitas. Los mensajes que Simurq te envía pueden no coincidir con tus gustos o preferencias, y puedes terminar descargando algo que no te gusta o que no usas. Además, es posible que el contenido que ofrece Simurq no sea exclusivo ni original, y que puedas encontrarlo de forma gratuita en otras plataformas o fuentes. Por ejemplo, puedes escuchar música o ver videos en YouTube, Spotify, Netflix, etc. sin pagar nada. Por lo tanto, Simurq puede ser un desperdicio de dinero y una fuente de frustración.
-Si desea detener Simurq y darse de baja del servicio, hay dos formas de hacerlo. La primera forma es desactivar Simurq desde el menú del teléfono. Para ello, debe ir a la sección Azercell del menú del teléfono y seleccionar la opción "Simurq". Luego, debe elegir la opción "Desactivar" y confirmar su elección. Recibirá un mensaje de confirmación de que Simurq ha sido desactivado. La segunda forma es enviar un mensaje de texto con la palabra "STOP" a 6045. También recibirá un mensaje de confirmación de que Simurq ha sido desactivado.
-Si tiene algún problema o queja sobre Simurq, como que le cobren por el contenido que no solicitó o descargó, o que no pueda desactivar el servicio, puede ponerse en contacto con el servicio de atención al cliente de Azercell para obtener ayuda. Puede llamar al 111 desde su número de Azercell o al 012 490 11 11 desde cualquier otro número. También puede enviar un correo electrónico a customer.care@azercell.com o visitar una de las oficinas o distribuidores de Azercell. Puede encontrar más información en el sitio web de Azercell: [Azercell].
- -Simurq es un servicio ofrecido por Azercell que le envía mensajes interactivos con varios contenidos. Sin embargo, Simurq no es un servicio beneficioso y puede ser molesto y caro. Simurq puede enviar spam a tu teléfono con mensajes no deseados y distraerte de las notificaciones importantes. Simurq puede cobrarte por contenido que quizás no te interese o que puedas encontrar gratis en otro sitio. Simurq puede consumir sus datos y batería sin su consentimiento. Por lo tanto, le recomendamos que deje de Simurq y se dé de baja del servicio lo antes posible. Puede desactivar Simurq desde el menú de su teléfono o enviando un mensaje de texto con la palabra "STOP" a 6045. También puede ponerse en contacto con el servicio de atención al cliente de Azercell si tiene algún problema o queja. Puede evitar suscribirse a Simurq o servicios similares en el futuro leyendo los términos y condiciones de cualquier servicio que active en su teléfono, comprobando su saldo y monitoreando sus datos y uso de la batería regularmente, y tener cuidado con los mensajes que abre o responde en su teléfono.
-Simurq es un servicio ofrecido por Azercell que le envía mensajes interactivos con varios contenidos, como noticias, música, moda, celebridades, tendencias y mucho más. Puede activar el servicio en la sección Azercell del menú de su teléfono y optar por ver o ignorar los mensajes, o responder con un código para obtener más detalles o descargar el contenido.
-Puede detener Simurq y darse de baja del servicio mediante la desactivación de su menú de teléfono o enviando un mensaje de texto con la palabra "STOP" a 6045. Recibirás un mensaje de confirmación de que Simurq ha sido desactivado. También puede ponerse en contacto con el servicio de atención al cliente de Azercell si tiene algún problema o queja.
-Simurq no es una estafa, pero tampoco es un servicio beneficioso. Simurq puede enviar spam a tu teléfono con mensajes no deseados y distraerte de las notificaciones importantes. Simurq puede cobrarte por contenido que quizás no te interese o que puedas encontrar gratis en otro sitio. Simurq puede consumir sus datos y batería sin su consentimiento. Por lo tanto, le recomendamos que detenga Simurq y se dé de baja del servicio lo antes posible.
-Si estás buscando alternativas a Simurq, puedes encontrar muchas otras fuentes o plataformas que ofrecen contenido similar o mejor de forma gratuita o por un precio más bajo. Por ejemplo, puedes usar aplicaciones de redes sociales, como Facebook, Instagram, Twitter, etc., para seguir las últimas noticias, tendencias, celebridades y más. También puede utilizar servicios de streaming, como YouTube, Spotify, Netflix, etc., para escuchar música o ver videos de su elección. También puedes usar motores de búsqueda, como Bing, Google, etc., para encontrar cualquier información que necesites.
64aa2da5cfSi usted es un fan de los juegos de carreras de coches, es posible que haya oído hablar de CarX Drift Racing Highway Mod APK. Esta es una versión modificada del juego original CarX Drift Racing 2 que ofrece dinero ilimitado, coches desbloqueados y más características. Pero, ¿vale la pena descargar y jugar? En este artículo, vamos a revisar CarX Drift Racing Highway Mod APK y decirle todo lo que necesita saber al respecto.
-CarX Drift Racing Highway Mod APK es un juego para Android que le permite experimentar la emoción de la deriva en las carreteras. Es una mezcla de física realista, gráficos llamativos y conducción extrema en carreteras llenas de tráfico. Puedes elegir entre una variedad de coches, personalizarlos a tu gusto, y competir en diferentes modos y desafíos.
-Download Zip --->>> https://bltlly.com/2v6KQe
CarX Drift Racing Highway Mod APK tiene muchas características que lo hacen destacar de otros juegos de carreras de coches. Aquí están algunos de ellos:
-El juego utiliza un motor de física realista que simula el comportamiento de los coches reales en diferentes superficies y condiciones. Puedes sentir la diferencia entre asfalto, hierba, arena y nieve. También puede ajustar la suspensión, la presión de los neumáticos, el ángulo de curvatura y otros parámetros para adaptarse a su estilo de conducción.
-El juego cuenta con gráficos impresionantes que crean un entorno realista e inmersivo. Puedes ver los detalles de los coches, los reflejos de las luces, las sombras de los objetos y los efectos de humo. También puede cambiar la hora del día, el clima y el ángulo de la cámara para obtener diferentes vistas.
-El juego te desafía a conducir en carreteras ocupadas con otros coches y camiones. Usted tiene que evitar colisiones, adelantar a otros vehículos, y la deriva alrededor de las esquinas. También puede utilizar el impulso nitro para acelerar y realizar acrobacias espectaculares.
- -El juego tiene un modo de campaña que te sumerge en el mundo de las carreras callejeras. Usted tiene que completar varias misiones, ganar dinero, mejorar sus coches, y desbloquear nuevos. También puedes competir contra otros corredores y jefes en diferentes lugares.
-El juego le permite personalizar sus coches a su gusto. Puede cambiar el color, el trabajo de pintura, calcomanías, llantas, alerones, escapes, y más. También puede ajustar el motor, la transmisión, los frenos, el turbo y el nitro para mejorar el rendimiento.
-Si desea descargar e instalar CarX Drift Racing Highway Mod APK en su dispositivo Android, es necesario seguir estos pasos:
-CarX Drift Racing Highway Mod APK tiene algunos pros y contras que usted debe considerar antes de jugar. Aquí hay una tabla que los resume:
- -Si estás buscando otros juegos de carreras de coches que ofrecen características similares y jugabilidad, puedes probar estas alternativas:
- -CarX Drift Racing Highway Mod APK es un divertido y emocionante juego de carreras de coches que le permite la deriva en carreteras de carretera con la física realista y gráficos. También ofrece dinero ilimitado, coches desbloqueados, coches personalizables, modo de campaña y más características. Sin embargo, también tiene algunos inconvenientes, como requerir mucho espacio de almacenamiento y RAM, agotar la batería rápidamente, contener anuncios y errores, y no ser compatible con algunos dispositivos. Por lo tanto, debe sopesar los pros y los contras antes de descargarlo y reproducirlo. También puedes probar algunas alternativas si quieres explorar otros juegos de carreras de coches.
-Si eres un fan de la moda y los juegos de rol, es posible que hayas oído hablar de Love Nikki, un popular juego móvil que combina ambos géneros. Love Nikki es un juego que te permite vestir a tu avatar en miles de trajes, accesorios, peinados y maquillaje. También puedes explorar un mundo de fantasía lleno de historias, misiones, eventos y competiciones. Pero lo que si quieres disfrutar del juego sin gastar dinero real o ver anuncios? Ahí es donde el amor Nikki Mod APK entra en juego. En este artículo, le diremos qué es Love Nikki Mod APK, por qué debe descargarlo, y cómo descargarlo de forma segura y fácil.
-Love Nikki es un juego para móviles que fue lanzado en 2017 por Elex Technology. Está disponible para dispositivos Android e iOS. El juego ha sido descargado más de 100 millones de veces y ha recibido críticas positivas de críticos y jugadores por igual. Estas son algunas de las características que hacen de Love Nikki un juego único y divertido.
-DOWNLOAD ››››› https://bltlly.com/2v6MkO
Love Nikki no es solo un juego de disfraces. También es un juego de rol que te permite crear tu propio personaje y personalizar su apariencia. Puedes elegir entre diferentes estilos, como elegante, lindo, sexy, cool o deportivo. También puede mezclar y combinar diferentes elementos para crear sus propios trajes. El juego tiene más de 20,000 artículos para elegir, incluyendo ropa, zapatos, bolsos, joyas, sombreros, gafas, tatuajes y más.
-Pero vestirte no es lo único que puedes hacer en Love Nikki. También puede participar en diversos desafíos y concursos que ponen a prueba su sentido de la moda y habilidades. Puedes competir con otros jugadores o PNJ en diferentes temas y escenarios. También puede unirse a asociaciones y cooperar con otros estilistas para completar tareas y ganar recompensas. -El juego tiene más de 600 capítulos para explorar, cada uno con su propia trama y diálogo. También encontrarás varios eventos e historias paralelas que añaden más profundidad y diversión al juego. Descubrirás secretos, misterios, romance y drama mientras juegas a Love Nikki.
-Love Nikki no es solo un juego para un jugador. También es un juego social que te permite interactuar con otros jugadores de todo el mundo. Puede unirse o crear asociaciones y chatear con otros miembros. También puede visitar las casas de otros jugadores y dejar comentarios sobre sus diseños. También puedes compartir tu ropa e ideas en plataformas de redes sociales como Facebook, Instagram o Twitter.
-Pero eso no es todo. Love Nikki también te da la oportunidad de convertirte en un diseñador. Puedes usar la función Design Studio para crear tu propia ropa usando diferentes materiales, patrones, colores y formas. También puedes enviar tus diseños al Corredor Estrellado y obtener comentarios de otros jugadores. También puedes votar por tus diseños favoritos y apoyar a otros creadores.
- -Love Nikki es un juego gratuito, pero también tiene algunas compras en el juego que pueden mejorar tu experiencia de juego. Por ejemplo, puedes comprar dinero y diamantes, las dos monedas principales del juego, para desbloquear más atuendos y objetos. También puede comprar resistencia, que se requiere para jugar los capítulos y eventos. También puedes comprar niveles VIP, que te dan acceso a beneficios y funciones exclusivas.
-Sin embargo, no todo el mundo puede permitirse el lujo de gastar dinero real en el juego. Algunos jugadores también pueden encontrar los anuncios molestos o intrusivos. Es por eso que algunos jugadores optan por descargar Love Nikki Mod APK, una versión modificada del juego que le da algunas ventajas y beneficios. Estos son algunos de los beneficios de descargar Love Nikki Mod APK.
-Otra razón por la que los jugadores descargar Love Nikki Mod APK es obtener acceso gratuito a todos los trajes y artículos en el juego. Como mencionamos anteriormente, Love Nikki tiene más de 20.000 artículos para elegir, pero no todos están disponibles de forma gratuita. Algunos de ellos están bloqueados detrás de niveles VIP, eventos, misiones o sorteos gacha. Con Love Nikki Mod APK, puede desbloquear todos los trajes y artículos sin gastar dinero o diamantes. También puede utilizarlos en cualquier desafío o concurso sin restricciones. De esta manera, podrás disfrutar del máximo potencial del juego y expresar tu creatividad y estilo.
-Una razón final por la descarga de los jugadores Love Nikki Mod APK es deshacerse de los anuncios y los requisitos de raíz. Los anuncios pueden ser molestos y distraer, especialmente cuando aparecen en el medio del juego o cuando estás tratando de ver un video. También pueden ralentizar su dispositivo o consumir sus datos. Con Love Nikki Mod APK, puede jugar el juego sin anuncios o interrupciones.
-Además, algunos juegos modded requieren que raíz de su dispositivo, lo que significa alterar sus ajustes de software y darse control total sobre él. Esto puede ser arriesgado y complicado, ya que puede anular su garantía, exponer su dispositivo a malware o causar un mal funcionamiento. Con Love Nikki Mod APK, usted no necesita raíz de su dispositivo para instalar o jugar el juego. Solo tienes que seguir unos sencillos pasos que explicaremos más adelante.
-Ahora que sabes lo que es Love Nikki Mod APK y por qué debe descargarlo, es posible que se pregunte cómo hacerlo. No te preocupes, le guiará a través del proceso paso a paso. Aquí están las cosas que necesita hacer para descargar Love Nikki Mod APK de forma segura y fácil.
-Para evitar estos riesgos, es necesario hacer una investigación antes de descargar nada de Internet. Es necesario comprobar la reputación y los comentarios del sitio web que ofrece Love Nikki Mod APK. Necesitas buscar comentarios positivos de otros usuarios que han descargado y usado el juego modificado. También debe buscar signos de legitimidad y seguridad, como cifrado HTTPS, certificados, insignias o sellos.
-Uno de los sitios web que recomendamos para descargar Love Nikki Mod APK es [ModAPKStore]. Este sitio web es una de las fuentes más populares y confiables para juegos y aplicaciones modificadas. Tiene una gran colección de juegos y aplicaciones que se actualizan regularmente y se prueban para la calidad y el rendimiento. También tiene una interfaz fácil de usar y una velocidad de descarga rápida. Puede descargar Love Nikki Mod APK desde este sitio web haciendo clic en este [enlace].
-Lo siguiente que debe hacer es habilitar fuentes desconocidas en la configuración del dispositivo. Esto es necesario porque el amor Nikki Mod APK no es de la tienda oficial de Google Play o App Store. Es a partir de una fuente de terceros que el dispositivo podría no reconocer o confiar. Para permitir que su dispositivo instale y ejecute Love Nikki Mod APK, debe darle permiso para hacerlo.
-Para habilitar fuentes desconocidas en su dispositivo, debe seguir estos pasos:
-Una vez que haya habilitado fuentes desconocidas, puede pasar al siguiente paso.
-Para instalar el archivo APK de Love Nikki Mod APK, debe seguir estos pasos:
-Una vez realizada la instalación, puede pasar al paso final.
-Lo último que tienes que hacer es disfrutar del juego. Ahora puedes lanzar Love Nikki Mod APK desde el cajón de aplicaciones de tu dispositivo o la pantalla de inicio. También puede crear un acceso directo o widget para facilitar el acceso. Ahora puedes jugar Love Nikki con dinero y diamantes ilimitados, acceso gratuito a todos los atuendos y artículos, sin anuncios y sin necesidad de raíz. También puedes disfrutar de todas las características y contenidos del juego original, como la historia, los desafíos, los eventos y la comunidad.
-Felicidades, que ha descargado con éxito e instalado Love Nikki Mod APK en su dispositivo. Ahora puedes divertirte vistiendo a tu avatar, explorando el mundo de fantasía, compitiendo con otros jugadores y creando tus propios diseños. También puede compartir sus trajes e ideas con otros amantes de la moda en línea. También puedes actualizar el juego regularmente para obtener nuevas características y mejoras.
-Si usted tiene alguna pregunta o comentario sobre Love Nikki Mod APK, no dude en dejar un comentario a continuación. Nos encantaría saber de ti y ayudarte. ¡Gracias por leer este artículo y feliz juego!
-Aquí están algunas de las preguntas más frecuentes sobre Love Nikki Mod APK:
-Sí, Love Nikki Mod APK es seguro, siempre y cuando lo descargue de una fuente confiable como [ModAPKStore]. Este sitio web tiene una buena reputación y comentarios de otros usuarios que han descargado y utilizado el juego modificado. También tiene cifrado HTTPS, certificados, insignias o sellos que indican su legitimidad y seguridad. Sin embargo, siempre debe tener cuidado al descargar algo de Internet y escanearlo con un antivirus o un detector de malware antes de instalarlo en su dispositivo.
-No, Love Nikki Mod APK no es legal porque viola los términos y condiciones del desarrollador de juegos original Elex Technology. Al usar un juego modificado, estás eludiendo sus reglas y regulaciones con respecto a las compras, anuncios y contenido dentro del juego. También estás infringiendo sus derechos de propiedad intelectual al modificar su juego sin su permiso. Por lo tanto, el uso de Love Nikki Mod APK es ilegal y poco ético.
-Posiblemente, sí . Hay una posibilidad de que se le prohibió el uso de Love Nikki Mod APK, especialmente si se utiliza en línea o en modos multijugador. El desarrollador de juegos Elex Technology tiene el derecho de monitorear y detectar cualquier actividad sospechosa o fraudulenta en sus servidores. También pueden prohibir o suspender cualquier cuenta que viole sus términos y condiciones o perjudique la integridad y reputación de su juego. Por lo tanto, el uso de Love Nikki Mod APK es arriesgado y no se recomienda.
-No, no se puede utilizar Love Nikki Mod APK con el juego original. El juego modificado y el juego original son dos aplicaciones separadas que tienen diferentes datos y código. No pueden coexistir o interactuar entre sí en su dispositivo. Si intenta instalar ambas aplicaciones en su dispositivo, es posible que encuentre errores, fallos o conflictos. También puede perder su progreso o datos en cualquiera de las aplicaciones. Por lo tanto, solo debe usar una aplicación a la vez en su dispositivo.
64aa2da5cfSi usted está buscando una forma confiable y conveniente para disfrutar de los juegos de azar en línea en su dispositivo Android, es posible que desee considerar la descarga de la aplicación Betway. Betway es una de las plataformas de apuestas online más populares y confiables del mundo, ofreciendo una amplia gama de juegos y deportes para apostar. Si usted es un fan de los juegos de casino, casino en vivo, esports, o apuestas deportivas, usted encontrará algo para satisfacer su gusto y presupuesto en Betway.
-Download ✪ https://bltlly.com/2v6Kqp
En este artículo, le mostraremos cómo descargar e instalar la aplicación Betway para Android, así como cómo usarla para realizar sus apuestas y divertirse. También compararemos la aplicación Betway con el sitio móvil de Betway y le proporcionaremos información sobre la compatibilidad y los requisitos de la aplicación. Así que, sin más preámbulos, empecemos.
-Betway es una plataforma de juego en línea que se lanzó en 2006 y desde entonces ha crecido hasta convertirse en uno de los principales jugadores de la industria. Betway opera en varios mercados en todo el mundo, incluyendo África, Europa, Asia y América Latina. Betway está autorizado y regulado por varias autoridades, como la Comisión de Juego del Reino Unido, la Autoridad de Juego de Malta y la Autoridad de Juego de Suecia.
-Algunas de las características y beneficios que hacen que Betway se destaque de otras plataformas de juego en línea son:
- -Si quieres acceder a Betway en tu dispositivo Android, tienes dos opciones: usar la aplicación Betway o usar el sitio móvil de Betway. Ambas opciones tienen sus pros y sus contras, dependiendo de tus preferencias y necesidades. Estas son algunas de las principales diferencias entre ellas:
-Como puedes ver, la aplicación Betway tiene algunas ventajas sobre el sitio móvil de Betway, como un rendimiento más rápido, un menor consumo de datos y más características. Sin embargo, el sitio móvil de Betway también es una buena opción si no desea descargar o instalar nada en su dispositivo, o si tiene un dispositivo anterior o incompatible. En última instancia, la elección es suya.
-Dado que la aplicación Betway no está disponible en Google Play Store, tendrá que permitir que su dispositivo instale aplicaciones de fuentes desconocidas. Para hacer esto, vaya a la configuración del dispositivo y busque la opción de seguridad o privacidad. Luego, active la opción que dice "permitir fuentes desconocidas" o "permitir la instalación desde fuentes desconocidas". Esto le permitirá instalar el archivo apk Betway en su dispositivo.
-Siguiente, tendrá que descargar el archivo apk Betway de una fuente de confianza. Puede hacer esto visitando el sitio web oficial de Betway y haciendo clic en el botón "descargar aplicación". Alternativamente, puede utilizar este enlace para descargar el archivo apk Betway directamente. El tamaño del archivo es de aproximadamente 10 MB y debería tomar unos segundos o minutos para descargar dependiendo de su velocidad de Internet.
-Una vez que haya descargado el archivo apk Betway, puede proceder a instalar la aplicación Betway en su dispositivo. Para hacer esto, busque el archivo en su carpeta de descargas o barra de notificaciones y toque en él. Verá una ventana emergente pidiéndole que confirme la instalación. Toque en "instalar" y espere a que se complete el proceso. También puede ver un mensaje de advertencia diciendo que la aplicación puede dañar su dispositivo. Esto es normal y puede ignorarlo tocando en "instalar de todos modos". La aplicación Betway se instalará en su dispositivo y verá un icono en la pantalla de inicio o en el cajón de aplicaciones.
-Ahora que ha instalado la aplicación Betway en su dispositivo, puede comenzar a usarla para realizar sus apuestas y disfrutar del juego en línea. Aquí está cómo usarlo:
-Si ya tiene una cuenta de Betway, puede simplemente iniciar sesión en la aplicación usando sus credenciales existentes. Para ello, abra la aplicación y toque en "iniciar sesión". Introduzca su nombre de usuario o dirección de correo electrónico y contraseña y toque en "iniciar sesión". Estarás conectado a tu cuenta y podrás acceder a todas las características y funciones de la aplicación.
-Una vez que haya iniciado sesión en su cuenta, puede elegir entre una variedad de juegos y deportes para apostar. Puedes usar la barra de menú en la parte inferior de la pantalla para navegar entre diferentes categorías, como casino, casino en vivo, esports, deportes, promociones y más. También puedes usar la barra de búsqueda en la parte superior de la pantalla para encontrar un juego o deporte específico por nombre o palabra clave.
-Cuando encuentres un juego o deporte que te interese, toca en él para ver más detalles y opciones. Verá información como probabilidades, mercados, estadísticas, resultados en vivo y más. También puede ver transmisiones en vivo de algunos eventos si están disponibles.
Cuando se ha decidido por un juego o deporte para apostar, puede realizar sus apuestas y disfrutar de la emoción de los juegos de azar en línea. Para hacer una apuesta, toque en las probabilidades o el mercado que desea apostar y se añadirá a su boleto de apuesta. Puede agregar varias apuestas a su boleto de apuesta si desea crear una apuesta de combinación o acumuladora. También puedes ajustar tu apuesta y ver tu posible pago en tu boleta de apuesta.
-Cuando esté satisfecho con su apuesta, toque en "realizar apuestas" y confirme su apuesta. Verá un mensaje de confirmación y su apuesta será procesada. Puede rastrear el estado de su apuesta en su historial de apuestas o en la sección de puntuación en vivo. También puede retirar su apuesta antes de que el evento termine si desea asegurar una ganancia o minimizar una pérdida.
- -La aplicación Betway para Android es compatible con la mayoría de los dispositivos Android que se ejecutan en Android 4.4 o superior. Sin embargo, algunos dispositivos más antiguos o de gama baja pueden no ser compatibles con la aplicación o algunas de sus características. Para asegurarse de que tiene la mejor experiencia con la aplicación Betway, debe tener un dispositivo que cumpla con las siguientes especificaciones mínimas:
-Tu dispositivo debe tener Android 4.4 o superior instalado. Puede comprobar su versión de Android yendo a la configuración del dispositivo y buscando la opción de información sobre el teléfono o el software.
-Su dispositivo debe tener al menos 1 GB de RAM, 100 MB de espacio de almacenamiento libre y una resolución de pantalla de 800 x 480 píxeles o más. Puede comprobar estas especificaciones yendo a la configuración del dispositivo y buscando la opción de almacenamiento, memoria o visualización.
-La aplicación Betway para Android es una gran manera de disfrutar de los juegos de azar en línea en su dispositivo. Ofrece una amplia gama de juegos y deportes para apostar, así como una interfaz fácil de usar y segura. Puede descargar e instalar la aplicación Betway para Android siguiendo los pasos que hemos descrito en este artículo. También puede utilizar el sitio móvil de Betway si prefiere no descargar nada en su dispositivo.
-Esperamos que este artículo te haya ayudado a aprender más sobre la aplicación Betway para Android y cómo usarla. Si tiene alguna pregunta o comentario, no dude en contactarnos o dejar un comentario a continuación. Nos encantaría saber de usted.
-Aquí están algunas de las preguntas más frecuentes sobre la aplicación Betway para Android:
-%%capture
-!pip install transformers wandb torchmetrics lightning
-
Requirement already satisfied: transformers in c:\users\froro\onedrive\escritorio\unal\rna\financia\env\lib\site-packages (4.27.4) -Requirement already satisfied: wandb in c:\users\froro\onedrive\escritorio\unal\rna\financia\env\lib\site-packages (0.14.2) -Collecting torchmetrics - Using cached torchmetrics-0.11.4-py3-none-any.whl (519 kB) -Collecting lightning - Downloading lightning-2.0.3-py3-none-any.whl (1.8 MB) - ---------------------------------------- 0.0/1.8 MB ? eta -:--:-- - - -------------------------------------- 0.1/1.8 MB 2.6 MB/s eta 0:00:01 - ----- ---------------------------------- 0.2/1.8 MB 2.9 MB/s eta 0:00:01 - ----------- ---------------------------- 0.5/1.8 MB 4.6 MB/s eta 0:00:01 - ------------------------ --------------- 1.1/1.8 MB 6.5 MB/s eta 0:00:01 - ---------------------------------------- 1.8/1.8 MB 8.4 MB/s eta 0:00:00 -Requirement already satisfied: tqdm>=4.27 in c:\users\froro\onedrive\escritorio\unal\rna\financia\env\lib\site-packages (from transformers) (4.65.0) -Requirement already satisfied: huggingface-hub<1.0,>=0.11.0 in c:\users\froro\onedrive\escritorio\unal\rna\financia\env\lib\site-packages (from transformers) (0.13.4) -Requirement already satisfied: numpy>=1.17 in c:\users\froro\onedrive\escritorio\unal\rna\financia\env\lib\site-packages (from transformers) (1.24.2) -Requirement already satisfied: regex!=2019.12.17 in c:\users\froro\onedrive\escritorio\unal\rna\financia\env\lib\site-packages (from transformers) (2023.3.23) -Requirement already satisfied: tokenizers!=0.11.3,<0.14,>=0.11.1 in c:\users\froro\onedrive\escritorio\unal\rna\financia\env\lib\site-packages (from transformers) (0.13.3) -Requirement already satisfied: filelock in c:\users\froro\onedrive\escritorio\unal\rna\financia\env\lib\site-packages (from transformers) (3.11.0) -Requirement already satisfied: requests in c:\users\froro\onedrive\escritorio\unal\rna\financia\env\lib\site-packages (from transformers) (2.28.2) -Requirement already satisfied: packaging>=20.0 in c:\users\froro\onedrive\escritorio\unal\rna\financia\env\lib\site-packages (from transformers) (23.0) -Requirement already satisfied: pyyaml>=5.1 in c:\users\froro\onedrive\escritorio\unal\rna\financia\env\lib\site-packages (from transformers) (6.0) -Requirement already satisfied: GitPython!=3.1.29,>=1.0.0 in c:\users\froro\onedrive\escritorio\unal\rna\financia\env\lib\site-packages (from wandb) (3.1.31) -Requirement already satisfied: pathtools in c:\users\froro\onedrive\escritorio\unal\rna\financia\env\lib\site-packages (from wandb) (0.1.2) -Requirement already satisfied: appdirs>=1.4.3 in c:\users\froro\onedrive\escritorio\unal\rna\financia\env\lib\site-packages (from wandb) (1.4.4) -Requirement already satisfied: docker-pycreds>=0.4.0 in c:\users\froro\onedrive\escritorio\unal\rna\financia\env\lib\site-packages (from wandb) (0.4.0) -Requirement already satisfied: Click!=8.0.0,>=7.0 in c:\users\froro\onedrive\escritorio\unal\rna\financia\env\lib\site-packages (from wandb) (8.1.3) -Requirement already satisfied: setproctitle in c:\users\froro\onedrive\escritorio\unal\rna\financia\env\lib\site-packages (from wandb) (1.3.2) -Requirement already satisfied: protobuf!=4.21.0,<5,>=3.19.0 in c:\users\froro\onedrive\escritorio\unal\rna\financia\env\lib\site-packages (from wandb) (4.22.3) -Requirement already satisfied: sentry-sdk>=1.0.0 in c:\users\froro\onedrive\escritorio\unal\rna\financia\env\lib\site-packages (from wandb) (1.19.1) -Requirement already satisfied: psutil>=5.0.0 in c:\users\froro\onedrive\escritorio\unal\rna\financia\env\lib\site-packages (from wandb) (5.9.4) -Requirement already satisfied: setuptools in c:\users\froro\onedrive\escritorio\unal\rna\financia\env\lib\site-packages (from wandb) (67.6.1) -Requirement already satisfied: torch>=1.8.1 in c:\users\froro\onedrive\escritorio\unal\rna\financia\env\lib\site-packages (from torchmetrics) (1.13.1) -Collecting lightning-utilities<2.0,>=0.7.0 - Using cached lightning_utilities-0.8.0-py3-none-any.whl (20 kB) -Collecting arrow<3.0,>=1.2.0 - Using cached arrow-1.2.3-py3-none-any.whl (66 kB) -Collecting fastapi<0.89.0,>=0.69.0 - Using cached fastapi-0.88.0-py3-none-any.whl (55 kB) -Collecting pytorch-lightning - Downloading pytorch_lightning-2.0.3-py3-none-any.whl (720 kB) - ---------------------------------------- 0.0/720.6 kB ? eta -:--:-- - ------------------------------------- 720.6/720.6 kB 44.4 MB/s eta 0:00:00 -Requirement already satisfied: traitlets<7.0,>=5.3.0 in c:\users\froro\onedrive\escritorio\unal\rna\financia\env\lib\site-packages (from lightning) (5.9.0) -Requirement already satisfied: Jinja2<5.0 in c:\users\froro\onedrive\escritorio\unal\rna\financia\env\lib\site-packages (from lightning) (3.1.2) -Collecting starsessions<2.0,>=1.2.1 - Using cached starsessions-1.3.0-py3-none-any.whl (10 kB) -Collecting deepdiff<8.0,>=5.7.0 - Using cached deepdiff-6.3.0-py3-none-any.whl (69 kB) -Collecting croniter<1.4.0,>=1.3.0 - Using cached croniter-1.3.15-py2.py3-none-any.whl (19 kB) -Requirement already satisfied: urllib3<3.0 in c:\users\froro\onedrive\escritorio\unal\rna\financia\env\lib\site-packages (from lightning) (1.26.15) -Collecting python-multipart<2.0,>=0.0.5 - Using cached python_multipart-0.0.6-py3-none-any.whl (45 kB) -Requirement already satisfied: typing-extensions<6.0,>=4.0.0 in c:\users\froro\onedrive\escritorio\unal\rna\financia\env\lib\site-packages (from lightning) (4.5.0) -Requirement already satisfied: fsspec<2024.0,>=2022.5.0 in c:\users\froro\onedrive\escritorio\unal\rna\financia\env\lib\site-packages (from lightning) (2023.4.0) -Collecting starlette - Downloading starlette-0.28.0-py3-none-any.whl (68 kB) - ---------------------------------------- 0.0/68.9 kB ? eta -:--:-- - ---------------------------------------- 68.9/68.9 kB 3.7 MB/s eta 0:00:00 -Collecting lightning-cloud>=0.5.34 - Using cached lightning_cloud-0.5.36-py3-none-any.whl (562 kB) -Collecting websockets<12.0 - Using cached websockets-11.0.3-cp310-cp310-win_amd64.whl (124 kB) -Collecting dateutils<2.0 - Using cached dateutils-0.6.12-py2.py3-none-any.whl (5.7 kB) -Requirement already satisfied: pydantic<4.0,>=1.7.4 in c:\users\froro\onedrive\escritorio\unal\rna\financia\env\lib\site-packages (from lightning) (1.10.7) -Collecting beautifulsoup4<6.0,>=4.8.0 - Using cached beautifulsoup4-4.12.2-py3-none-any.whl (142 kB) -Collecting rich<15.0,>=12.3.0 - Using cached rich-13.4.1-py3-none-any.whl (239 kB) -Collecting inquirer<5.0,>=2.10.0 - Using cached inquirer-3.1.3-py3-none-any.whl (18 kB) -Collecting uvicorn<2.0 - Using cached uvicorn-0.22.0-py3-none-any.whl (58 kB) -Collecting websocket-client<3.0 - Using cached websocket_client-1.5.2-py3-none-any.whl (56 kB) -Requirement already satisfied: python-dateutil>=2.7.0 in c:\users\froro\onedrive\escritorio\unal\rna\financia\env\lib\site-packages (from arrow<3.0,>=1.2.0->lightning) (2.8.2) -Collecting soupsieve>1.2 - Using cached soupsieve-2.4.1-py3-none-any.whl (36 kB) -Requirement already satisfied: colorama in c:\users\froro\onedrive\escritorio\unal\rna\financia\env\lib\site-packages (from Click!=8.0.0,>=7.0->wandb) (0.4.6) -Requirement already satisfied: pytz in c:\users\froro\onedrive\escritorio\unal\rna\financia\env\lib\site-packages (from dateutils<2.0->lightning) (2023.3) -Collecting ordered-set<4.2.0,>=4.0.2 - Using cached ordered_set-4.1.0-py3-none-any.whl (7.6 kB) -Requirement already satisfied: six>=1.4.0 in c:\users\froro\onedrive\escritorio\unal\rna\financia\env\lib\site-packages (from docker-pycreds>=0.4.0->wandb) (1.16.0) -Collecting starlette - Using cached starlette-0.22.0-py3-none-any.whl (64 kB) -Collecting anyio<5,>=3.4.0 - Using cached anyio-3.7.0-py3-none-any.whl (80 kB) -Requirement already satisfied: aiohttp!=4.0.0a0,!=4.0.0a1 in c:\users\froro\onedrive\escritorio\unal\rna\financia\env\lib\site-packages (from fsspec<2024.0,>=2022.5.0->lightning) (3.8.4) -Requirement already satisfied: gitdb<5,>=4.0.1 in c:\users\froro\onedrive\escritorio\unal\rna\financia\env\lib\site-packages (from GitPython!=3.1.29,>=1.0.0->wandb) (4.0.10) -Collecting readchar>=3.0.6 - Using cached readchar-4.0.5-py3-none-any.whl (8.5 kB) -Collecting python-editor>=1.0.4 - Using cached python_editor-1.0.4-py3-none-any.whl (4.9 kB) -Collecting blessed>=1.19.0 - Using cached blessed-1.20.0-py2.py3-none-any.whl (58 kB) -Requirement already satisfied: MarkupSafe>=2.0 in c:\users\froro\onedrive\escritorio\unal\rna\financia\env\lib\site-packages (from Jinja2<5.0->lightning) (2.1.2) -Collecting pyjwt - Using cached PyJWT-2.7.0-py3-none-any.whl (22 kB) -Requirement already satisfied: idna<4,>=2.5 in c:\users\froro\onedrive\escritorio\unal\rna\financia\env\lib\site-packages (from requests->transformers) (3.4) -Requirement already satisfied: charset-normalizer<4,>=2 in c:\users\froro\onedrive\escritorio\unal\rna\financia\env\lib\site-packages (from requests->transformers) (3.1.0) -Requirement already satisfied: certifi>=2017.4.17 in c:\users\froro\onedrive\escritorio\unal\rna\financia\env\lib\site-packages (from requests->transformers) (2022.12.7) -Collecting markdown-it-py<3.0.0,>=2.2.0 - Using cached markdown_it_py-2.2.0-py3-none-any.whl (84 kB) -Requirement already satisfied: pygments<3.0.0,>=2.13.0 in c:\users\froro\onedrive\escritorio\unal\rna\financia\env\lib\site-packages (from rich<15.0,>=12.3.0->lightning) (2.15.0) -Collecting itsdangerous<3.0.0,>=2.0.1 - Using cached itsdangerous-2.1.2-py3-none-any.whl (15 kB) -Collecting h11>=0.8 - Using cached h11-0.14.0-py3-none-any.whl (58 kB) -Requirement already satisfied: async-timeout<5.0,>=4.0.0a3 in c:\users\froro\onedrive\escritorio\unal\rna\financia\env\lib\site-packages (from aiohttp!=4.0.0a0,!=4.0.0a1->fsspec<2024.0,>=2022.5.0->lightning) (4.0.2) -Requirement already satisfied: aiosignal>=1.1.2 in c:\users\froro\onedrive\escritorio\unal\rna\financia\env\lib\site-packages (from aiohttp!=4.0.0a0,!=4.0.0a1->fsspec<2024.0,>=2022.5.0->lightning) (1.3.1) -Requirement already satisfied: yarl<2.0,>=1.0 in c:\users\froro\onedrive\escritorio\unal\rna\financia\env\lib\site-packages (from aiohttp!=4.0.0a0,!=4.0.0a1->fsspec<2024.0,>=2022.5.0->lightning) (1.8.2) -Requirement already satisfied: frozenlist>=1.1.1 in c:\users\froro\onedrive\escritorio\unal\rna\financia\env\lib\site-packages (from aiohttp!=4.0.0a0,!=4.0.0a1->fsspec<2024.0,>=2022.5.0->lightning) (1.3.3) -Requirement already satisfied: multidict<7.0,>=4.5 in c:\users\froro\onedrive\escritorio\unal\rna\financia\env\lib\site-packages (from aiohttp!=4.0.0a0,!=4.0.0a1->fsspec<2024.0,>=2022.5.0->lightning) (6.0.4) -Requirement already satisfied: attrs>=17.3.0 in c:\users\froro\onedrive\escritorio\unal\rna\financia\env\lib\site-packages (from aiohttp!=4.0.0a0,!=4.0.0a1->fsspec<2024.0,>=2022.5.0->lightning) (22.2.0) -Collecting sniffio>=1.1 - Using cached sniffio-1.3.0-py3-none-any.whl (10 kB) -Collecting exceptiongroup - Using cached exceptiongroup-1.1.1-py3-none-any.whl (14 kB) -Collecting jinxed>=1.1.0 - Using cached jinxed-1.2.0-py2.py3-none-any.whl (33 kB) -Requirement already satisfied: wcwidth>=0.1.4 in c:\users\froro\onedrive\escritorio\unal\rna\financia\env\lib\site-packages (from blessed>=1.19.0->inquirer<5.0,>=2.10.0->lightning) (0.2.6) -Requirement already satisfied: smmap<6,>=3.0.1 in c:\users\froro\onedrive\escritorio\unal\rna\financia\env\lib\site-packages (from gitdb<5,>=4.0.1->GitPython!=3.1.29,>=1.0.0->wandb) (5.0.0) -Collecting mdurl~=0.1 - Using cached mdurl-0.1.2-py3-none-any.whl (10.0 kB) -Collecting ansicon - Using cached ansicon-1.89.0-py2.py3-none-any.whl (63 kB) -Installing collected packages: python-editor, ansicon, websockets, websocket-client, soupsieve, sniffio, readchar, python-multipart, pyjwt, ordered-set, mdurl, lightning-utilities, jinxed, itsdangerous, h11, exceptiongroup, uvicorn, torchmetrics, markdown-it-py, deepdiff, dateutils, croniter, blessed, beautifulsoup4, arrow, anyio, starlette, rich, inquirer, starsessions, pytorch-lightning, fastapi, lightning-cloud, lightning -Successfully installed ansicon-1.89.0 anyio-3.7.0 arrow-1.2.3 beautifulsoup4-4.12.2 blessed-1.20.0 croniter-1.3.15 dateutils-0.6.12 deepdiff-6.3.0 exceptiongroup-1.1.1 fastapi-0.88.0 h11-0.14.0 inquirer-3.1.3 itsdangerous-2.1.2 jinxed-1.2.0 lightning-2.0.3 lightning-cloud-0.5.36 lightning-utilities-0.8.0 markdown-it-py-2.2.0 mdurl-0.1.2 ordered-set-4.1.0 pyjwt-2.7.0 python-editor-1.0.4 python-multipart-0.0.6 pytorch-lightning-2.0.3 readchar-4.0.5 rich-13.4.1 sniffio-1.3.0 soupsieve-2.4.1 starlette-0.22.0 starsessions-1.3.0 torchmetrics-0.11.4 uvicorn-0.22.0 websocket-client-1.5.2 websockets-11.0.3 --
-[notice] A new release of pip is available: 23.0.1 -> 23.1.2 -[notice] To update, run: python.exe -m pip install --upgrade pip --
Instalamos las librerias que se neceista para el entrenamiento
- -import numpy as np
-import pandas as pd
-from sklearn.model_selection import train_test_split
-from sklearn.metrics import accuracy_score, f1_score
-import wandb
-import pandas as pd
-import torch
-from torch.utils.data import Dataset, DataLoader
-from transformers import set_seed
-from torch import nn
-
-def SEED(seed):
- torch.manual_seed(seed)
- np.random.seed(seed)
- set_seed(seed)
-
-
-SEED(42)
-
-
-# Configuration by model
-NUM_VARAIBLES = 3
-NUM_LABELS = 3
-num_labels = NUM_LABELS * NUM_VARAIBLES
-divice = torch.device('cuda' if torch.cuda.is_available() else 'cpu')
-
Importamos las librerias necesarias y fijamos las semillas para iniciar el entrenamiento
- -El modelo pre-entrenado de Robertuito para realizar fine-tuning en la detección de sentimientos en español. Al modificar el enfoque de clasificación a multilabel, el modelo será capaz de retornar un vector de 9 posiciones, donde cada posición representa la probabilidad o incertidumbre de pertenecer a una etiqueta específica.
-El fine-tuning del modelo de Robertuito implica ajustar los pesos y parámetros del modelo utilizando tus datos específicos y el nuevo enfoque de clasificación multilabel. Durante este proceso, puedes utilizar técnicas como el descenso de gradiente estocástico (SGD) o el algoritmo Adam para optimizar la función de pérdida y lograr un modelo más preciso.
-Una vez completado el fine-tuning, podrás utilizar el modelo para realizar inferencias en nuevos textos. Al proporcionar un texto de entrada, el modelo calculará las probabilidades de pertenencia a cada una de las 9 etiquetas de sentimiento. Estas probabilidades reflejarán la confianza o incertidumbre del modelo en relación con cada etiqueta.
-Es importante destacar que durante el proceso de fine-tuning, es necesario contar con un conjunto de datos etiquetados correctamente para entrenar el modelo y ajustar los pesos de manera adecuada. Además, la cantidad y calidad de los datos utilizados en el fine-tuning pueden influir en el rendimiento del modelo final.
-Con el modelo pre-entrenado de Robertuito y el enfoque de fine-tuning para clasificación multilabel, estarás en condiciones de realizar detección de sentimientos más precisa y obtener información sobre las probabilidades asociadas a cada etiqueta de sentimiento en tus datos de entrada en español.
- -from transformers import (
- AutoModelForSequenceClassification,
- AutoTokenizer,
- get_constant_schedule_with_warmup,
-)
-
-# Configuring the model
-num_labels = NUM_LABELS * NUM_VARAIBLES
-model_name = "pysentimiento/robertuito-sentiment-analysis"
-auto_tokenizer = AutoTokenizer.from_pretrained(model_name)
-model_hugginface = AutoModelForSequenceClassification.from_pretrained(model_name, num_labels=num_labels, ignore_mismatched_sizes=True)
-
Downloading (…)okenizer_config.json: 0%| | 0.00/384 [00:00<?, ?B/s]-
Downloading (…)/main/tokenizer.json: 0%| | 0.00/1.31M [00:00<?, ?B/s]-
Downloading (…)cial_tokens_map.json: 0%| | 0.00/167 [00:00<?, ?B/s]-
Downloading (…)lve/main/config.json: 0%| | 0.00/925 [00:00<?, ?B/s]-
Downloading pytorch_model.bin: 0%| | 0.00/435M [00:00<?, ?B/s]-
Some weights of RobertaForSequenceClassification were not initialized from the model checkpoint at pysentimiento/robertuito-sentiment-analysis and are newly initialized because the shapes did not match: -- classifier.out_proj.weight: found shape torch.Size([3, 768]) in the checkpoint and torch.Size([9, 768]) in the model instantiated -- classifier.out_proj.bias: found shape torch.Size([3]) in the checkpoint and torch.Size([9]) in the model instantiated -You should probably TRAIN this model on a down-stream task to be able to use it for predictions and inference. --
La configuración del modelo se realiza en base a las siguientes consideraciones:
-Número de etiquetas: Se define la variable num_labels como el producto de NUM_LABELS y NUM_VARIABLES. Esto se debe a que se está trabajando con un enfoque multilabel, donde se tienen múltiples etiquetas para cada instancia de datos. El número de etiquetas se obtiene multiplicando el número de categorías posibles en cada variable (como se mencionó anteriormente, 3 categorías para cada una de las 3 variables).
-Nombre del modelo: Se especifica el nombre del modelo pre-entrenado utilizado, que en este caso es "pysentimiento/robertuito-sentiment-analysis". Este modelo ya ha sido entrenado en una tarea similar de detección de sentimientos en español y se utilizará como punto de partida para el fine-tuning.
-Tokenizador automático: Se crea una instancia de AutoTokenizer a partir del modelo pre-entrenado. Esto permite convertir los textos de entrada en secuencias numéricas que el modelo puede procesar. El tokenizador se adapta automáticamente al modelo utilizado, en este caso, Robertuito, y maneja la tokenización adecuada para el idioma español.
-Modelo de clasificación de secuencia: Se crea una instancia de AutoModelForSequenceClassification a partir del modelo pre-entrenado. Este modelo es específico para tareas de clasificación de secuencias y se adapta automáticamente al modelo pre-entrenado especificado. Se le proporciona el número de etiquetas (num_labels) que se definió previamente y se establece ignore_mismatched_sizes=True para manejar casos en los que las dimensiones
-A continuación se muestra el esqueleto de la clase FinanciaSentimental que hereda de Dataset y se encarga de cargar los datos para entrenar el modelo. Se realiza el procesamiento de los textos mediante el tokenizador y las etiquetas se convierten en tensores para su posterior uso en el entrenamiento del modelo:
- -class FinanciaSentimental(Dataset):
- """This class is used to load the data and tokenize it"""
- def __init__(self, tokenizer, dataframe, columns, max_len=512):
- self.tokenizer = tokenizer
- self.dataframe = dataframe
- ## Columns to target
- self._columns = columns
- self.max_len = max_len
-
- @property
- def columns(self):
- """Return the columns to target"""
- return self._columns
-
- def __len__(self):
- """Return the length of the dataset"""
- return len(self.dataframe)
-
- def __getitem__(self, index):
- """Get the data at the index"""
- values = self.dataframe.iloc[index]
- text = values['text']
- label = values[self._columns].values.astype(np.float32)
- inputs = self.tokenizer.encode_plus(text, max_length=130, pad_to_max_length=True, padding='max_length', truncation=True, return_tensors='pt')
- label = torch.tensor(label, dtype=torch.float)
- input_ids = inputs["input_ids"].squeeze().to(dtype=torch.long)
- attention_mask = inputs["attention_mask"].squeeze().to(dtype=torch.long)
- token_type_ids = inputs["token_type_ids"].squeeze().to(dtype=torch.long)
-
- inputs_dict = {
- "input_ids": input_ids,
- "attention_mask": attention_mask,
- "token_type_ids": token_type_ids,
- "labels":label
- }
-
- return inputs_dict
-
la lógica de entrenamiento utilizando la librería PyTorch Lightning, junto con el optimizador AdamW y un scheduler que reduce la tasa de aprendizaje a medida que se acerca al objetivo:
- -import torch
-import lightning.pytorch as pl
-from tqdm import tqdm
-from sklearn.metrics import f1_score, accuracy_score
-from torch.nn import BCEWithLogitsLoss
-
-class FinanciaMultilabel(pl.LightningModule):
-
- def __init__(self, model, num_labels):
- super().__init__()
- self.model = model
- self.num_labels = num_labels
- self.loss = BCEWithLogitsLoss()
- self.validation_step_outputs = []
-
- def forward(self, input_ids, attention_mask, token_type_ids):
- return self.model(input_ids, attention_mask, token_type_ids).logits
-
- def training_step(self, batch, batch_idx):
- input_ids = batch["input_ids"]
- attention_mask = batch["attention_mask"]
- labels = batch["labels"]
- token_type_ids = batch["token_type_ids"]
- outputs = self(input_ids, attention_mask, token_type_ids)
- loss = self.loss(outputs.view(-1,self.num_labels), labels.type_as(outputs).view(-1,self.num_labels))
- self.log('train_loss', loss)
- return loss
-
- def validation_step(self, batch, batch_idx):
- input_ids = batch["input_ids"]
- attention_mask = batch["attention_mask"]
- labels = batch["labels"]
- token_type_ids = batch["token_type_ids"]
- outputs = self(input_ids, attention_mask, token_type_ids)
- loss = self.loss(outputs.view(-1,self.num_labels), labels.type_as(outputs).view(-1,self.num_labels))
- pred_labels = torch.sigmoid(outputs)
- info = {'val_loss': loss, 'pred_labels': pred_labels, 'labels': labels}
- self.validation_step_outputs.append(info)
- return
-
- def on_validation_epoch_end(self):
- outputs = self.validation_step_outputs
- avg_loss = torch.stack([x['val_loss'] for x in outputs]).mean()
- pred_labels = torch.cat([x['pred_labels'] for x in outputs])
- labels = torch.cat([x['labels'] for x in outputs])
- threshold = 0.50
- pred_bools = pred_labels > threshold
- true_bools = labels == 1
- val_f1_accuracy = f1_score(true_bools.cpu(), pred_bools.cpu(), average='micro')*100
- val_flat_accuracy = accuracy_score(true_bools.cpu(), pred_bools.cpu())*100
- self.log('val_loss', avg_loss)
- self.log('val_f1_accuracy', val_f1_accuracy, prog_bar=True)
- self.log('val_flat_accuracy', val_flat_accuracy, prog_bar=True)
- self.validation_step_outputs.clear()
-
- def configure_optimizers(self):
- optimizer = torch.optim.AdamW(self.parameters(), lr=2e-5)
- scheduler = torch.optim.lr_scheduler.ReduceLROnPlateau(optimizer, mode='max', factor=0.1, patience=2, verbose=True, min_lr=1e-6)
- return {
- 'optimizer': optimizer,
- 'lr_scheduler': {
- 'scheduler': scheduler,
- 'monitor': 'val_loss'
- }
- }
-
El modelo de referencia utilizado en el proyecto es el "robertuito-sentiment-analysis" de la biblioteca "pysentimiento". Puedes encontrar más información sobre este modelo en el siguiente enlace: pysentimiento/robertuito-sentiment-analysis.
-Este modelo representa el estado del arte en la detección de sentimientos en español y ha sido pre-entrenado en un corpus extenso de textos en español. Utiliza la arquitectura de modelo basada en Transformers, que ha demostrado ser altamente efectiva en tareas de procesamiento del lenguaje natural.
-El modelo está diseñado para realizar la clasificación de sentimientos en textos en español, proporcionando predicciones para tres categorías principales: positivo, negativo y neutro. Es importante destacar que el modelo fue ajustado para adaptarse a un problema de múltiples etiquetas, donde se espera que la salida sea un vector de nueve componentes que representan las diferentes combinaciones de sentimientos para las variables objetivo, compañías y consumidores.
-El uso de este modelo pre-entrenado ofrece la ventaja de aprovechar el conocimiento y las características lingüísticas aprendidas en grandes cantidades de datos, lo que puede mejorar el rendimiento y la precisión de la detección de sentimientos en noticias financieras.
- -train_df = "/content/drive/Shareddrives/Redes neuronales/Datasets/df_with_sentiment.csv"
-df = pd.read_csv(train_df)
-df = df[["id", "text", "target", "target_sentiment", "companies_sentiment", "consumers_sentiment", "tag"]]
-df = pd.get_dummies(df, columns = ["target_sentiment", "companies_sentiment","consumers_sentiment"])
-df_train = df[df.tag == "train"]
-df_test = df[df.tag == "test"]
-df_valid, df_test = train_test_split(df_test, test_size=0.5)
-
columns_varaibles = ["target_sentiment_negative", "target_sentiment_neutral", "target_sentiment_positive" ,"companies_sentiment_negative" ,"companies_sentiment_neutral", "companies_sentiment_positive", 'consumers_sentiment_negative',
- 'consumers_sentiment_neutral', 'consumers_sentiment_positive']
-
A continuación, se muestra cómo instanciar los conjuntos de datos y crear los dataloaders con un tamaño de lote (batch_size) de 16
- -train_dataset = FinanciaSentimental(auto_tokenizer, df_train, columns_varaibles)
-valid_dataset = FinanciaSentimental(auto_tokenizer, df_valid, columns_varaibles)
-test_dataset = FinanciaSentimental(auto_tokenizer, df_test, columns_varaibles)
-
En el código proporcionado se están creando los dataloaders para el conjunto de entrenamiento (train_dataloader), validación (valid_dataloader) y prueba (test_dataloader).
-La clase DataLoader de PyTorch se utiliza para cargar los conjuntos de datos y generar lotes de datos para el entrenamiento del modelo.
-Cada dataloader se crea con los siguientes parámetros:
-train_dataset, valid_dataset, test_dataset: Son los conjuntos de datos correspondientes al entrenamiento, validación y prueba, respectivamente. -batch_size: Especifica el número de ejemplos que se procesarán en paralelo en cada iteración del entrenamiento. En este caso, se establece en 16, lo que significa que se procesarán 16 ejemplos a la vez. -shuffle=True: Indica si los ejemplos se deben mezclar aleatoriamente antes de cada iteración. En este caso, se establece en True para los dataloaders de entrenamiento, validación y prueba, lo que garantiza que los ejemplos se mezclen en cada época de entrenamiento y evaluación.
- -train_dataloader = DataLoader(train_dataset, batch_size=16, shuffle=True)
-valid_dataloader = DataLoader(valid_dataset, batch_size=16, shuffle=True)
-test_dataloader = DataLoader(test_dataset, batch_size=16, shuffle=True)
-
Todas las métricas se llevan un registro en wandb, para comparar los modelos
- -from pytorch_lightning.loggers import WandbLogger
-wandb_logger = WandbLogger(project='FinancIA', name='#1', save_code=True, log_model=False, sync_tensorboard=True, save_dir="./logs")
-
wandb: Logging into wandb.ai. (Learn how to deploy a W&B server locally: https://wandb.me/wandb-server) -wandb: You can find your API key in your browser here: https://wandb.ai/authorize -wandb: Paste an API key from your profile and hit enter, or press ctrl+c to quit:-
·········· --
wandb: Appending key for api.wandb.ai to your netrc file: /root/.netrc -wandb: WARNING Path ./logs/wandb/ wasn't writable, using system temp directory. --
/tmp/wandb/run-20230608_031230-1y2tfqnr
-Realizamos el respectivo entrenameinto del modelo. Se va a guardar el ultimo epoch y el que tenga la mejor -métricas
- -from lightning.pytorch import Trainer
-from lightning.pytorch.callbacks import ModelCheckpoint, EarlyStopping
-from lightning.pytorch.callbacks.progress import TQDMProgressBar
-from lightning.pytorch.loggers import WandbLogger
-from torch.utils.data import DataLoader, WeightedRandomSampler
-
-checkpoint_callback = ModelCheckpoint(monitor="val_loss", mode="max", save_last=True, save_weights_only=True)
-tqdm_callback = TQDMProgressBar(refresh_rate=1)
-trainer = pl.Trainer( accelerator="cuda", max_epochs=10, logger=wandb_logger, callbacks=[checkpoint_callback, tqdm_callback], precision=16,)
-model = FinanciaMultilabel(model_hugginface,9)
-wandb_logger.watch(model, log="all")
-trainer.fit(model,train_dataloader, valid_dataloader)
-
/usr/local/lib/python3.10/dist-packages/lightning/fabric/connector.py:555: UserWarning: 16 is supported for historical reasons but its usage is discouraged. Please set your precision to 16-mixed instead!
- rank_zero_warn(
-INFO:pytorch_lightning.utilities.rank_zero:Using 16bit Automatic Mixed Precision (AMP)
-INFO:pytorch_lightning.utilities.rank_zero:GPU available: True (cuda), used: True
-INFO:pytorch_lightning.utilities.rank_zero:TPU available: False, using: 0 TPU cores
-INFO:pytorch_lightning.utilities.rank_zero:IPU available: False, using: 0 IPUs
-INFO:pytorch_lightning.utilities.rank_zero:HPU available: False, using: 0 HPUs
-wandb: logging graph, to disable use `wandb.watch(log_graph=False)`
-INFO: LOCAL_RANK: 0 - CUDA_VISIBLE_DEVICES: [0]
-INFO:lightning.pytorch.accelerators.cuda:LOCAL_RANK: 0 - CUDA_VISIBLE_DEVICES: [0]
-INFO:
- | Name | Type | Params
------------------------------------------------------------
-0 | model | RobertaForSequenceClassification | 108 M
-1 | loss | BCEWithLogitsLoss | 0
------------------------------------------------------------
-108 M Trainable params
-0 Non-trainable params
-108 M Total params
-435.183 Total estimated model params size (MB)
-INFO:lightning.pytorch.callbacks.model_summary:
- | Name | Type | Params
------------------------------------------------------------
-0 | model | RobertaForSequenceClassification | 108 M
-1 | loss | BCEWithLogitsLoss | 0
------------------------------------------------------------
-108 M Trainable params
-0 Non-trainable params
-108 M Total params
-435.183 Total estimated model params size (MB)
-
-Sanity Checking: 0it [00:00, ?it/s]-
/usr/local/lib/python3.10/dist-packages/lightning/pytorch/trainer/connectors/data_connector.py:480: PossibleUserWarning: Your `val_dataloader`'s sampler has shuffling enabled, it is strongly recommended that you turn shuffling off for val/test dataloaders. - rank_zero_warn( -/usr/local/lib/python3.10/dist-packages/lightning/pytorch/loops/fit_loop.py:280: PossibleUserWarning: The number of training batches (46) is smaller than the logging interval Trainer(log_every_n_steps=50). Set a lower value for log_every_n_steps if you want to see logs for the training epoch. - rank_zero_warn( --
Training: 0it [00:00, ?it/s]-
Validation: 0it [00:00, ?it/s]-
Validation: 0it [00:00, ?it/s]-
Validation: 0it [00:00, ?it/s]-
Validation: 0it [00:00, ?it/s]-
Validation: 0it [00:00, ?it/s]-
Validation: 0it [00:00, ?it/s]-
Validation: 0it [00:00, ?it/s]-
Validation: 0it [00:00, ?it/s]-
Validation: 0it [00:00, ?it/s]-
Validation: 0it [00:00, ?it/s]-
INFO:pytorch_lightning.utilities.rank_zero:`Trainer.fit` stopped: `max_epochs=10` reached. --
!zip -r logs.zip logs
-
adding: logs/ (stored 0%) - adding: logs/wandb/ (stored 0%) - adding: logs/FinancIA/ (stored 0%) - adding: logs/FinancIA/1y2tfqnr/ (stored 0%) - adding: logs/FinancIA/1y2tfqnr/checkpoints/ (stored 0%) - adding: logs/FinancIA/1y2tfqnr/checkpoints/last.ckpt (deflated 7%) - adding: logs/FinancIA/1y2tfqnr/checkpoints/epoch=7-step=368.ckpt (deflated 7%) --
Cargamos el mdoelo con las mejores métricas.
- -device = "cuda"
-model = FinanciaMultilabel.load_from_checkpoint(
- "/content/logs/FinancIA/1y2tfqnr/checkpoints/epoch=7-step=368.ckpt",
- model=model_hugginface,
- num_labels=num_labels,
- map_location=device
- )
-
Cargamos las 9 etiquetas de neustro mdoelo.
- -RETURN_VALUES = [
- "target_sentiment_negative",
- "target_sentiment_neutral",
- "target_sentiment_positive",
- "companies_sentiment_negative",
- "companies_sentiment_neutral",
- "companies_sentiment_positive",
- "consumers_sentiment_negative",
- "consumers_sentiment_neutral",
- "consumers_sentiment_positive"
-]
-
Corremos y gaurdamos las métricas para el módulo de testo
- -from sklearn.metrics import f1_score, accuracy_score, precision_score, recall_score
-model.eval()
-device = "cuda"
-# Paso 3: procesar los datos de entrada y obtener las predicciones
-all_preds = []
-labels = []
-with torch.no_grad():
- for batch in test_dataloader:
- b_input_ids = batch["input_ids"].to(device)
- b_input_mask = batch["attention_mask"].to(device)
- b_labels = batch["labels"]
- token_type_ids = batch["token_type_ids"]
- outputs = model(b_input_ids, token_type_ids=None, attention_mask=b_input_mask)
- preds = torch.sigmoid(outputs).detach().cpu().numpy()
- labels.append(b_labels.numpy())
- all_preds.append(preds)
-
-# Paso 4: procesar las predicciones
-all_preds = np.concatenate(all_preds)
-binary_preds = (all_preds > 0.5).astype(int)
-
-# Paso 5: evaluar el rendimiento del modelo
-test_labels = np.concatenate(labels)
-
Al imprimir el reporte, se observa que al modelo le resulta más difícil predecir las etiquetas neutrales en general. Sin embargo, en general se obtiene un puntaje F1 de alrededor del 60%. Aunque no es una métrica muy alta, es lo suficientemente buena para tener un conjunto de datos inicial y poder mejorarlo a medida que se disponga de más noticias.
- -from sklearn.metrics import classification_report, multilabel_confusion_matrix
-print(classification_report(test_labels, binary_preds, target_names=RETURN_VALUES))
-
precision recall f1-score support - - target_sentiment_negative 0.60 0.96 0.74 27 - target_sentiment_neutral 0.50 0.50 0.50 4 - target_sentiment_positive 0.91 0.59 0.72 54 -companies_sentiment_negative 0.56 0.54 0.55 28 - companies_sentiment_neutral 0.57 0.50 0.53 40 -companies_sentiment_positive 0.71 0.29 0.42 17 -consumers_sentiment_negative 0.59 0.59 0.59 17 - consumers_sentiment_neutral 0.74 0.81 0.77 48 -consumers_sentiment_positive 0.61 0.55 0.58 20 - - micro avg 0.67 0.63 0.65 255 - macro avg 0.64 0.59 0.60 255 - weighted avg 0.69 0.63 0.64 255 - samples avg 0.68 0.63 0.65 255 - --
A continuación, se presentan las matrices de confusión donde las filas representan las etiquetas verdaderas y las columnas representan las etiquetas predichas por el modelo. Los valores en la matriz indican la cantidad de casos en los que se predijo correctamente (verdaderos positivos y verdaderos negativos) y en los que se predijo incorrectamente (falsos positivos y falsos negativos).
-Estas matrices de confusión nos permiten visualizar cómo el modelo está clasificando las etiquetas y proporcionan una visión más detallada del rendimiento del modelo para cada variable de sentimiento.
- -import seaborn as sns
-import matplotlib.pyplot as plt
-
-values = multilabel_confusion_matrix(test_labels, binary_preds)
-heatmap = sns.heatmap(values[0],annot=True)
-plt.title(RETURN_VALUES[0] )
-
-# Mostrar el heatmap
-plt.show()
-
|
Se puede observar que el modelo tiene un buen desempeño al detectar correctamente las muestras que no pertenecen a su clase. Además, también logra diferenciar en su mayoría las muestras que sí pertenecen a su clase. Sin embargo, hay 17 casos en los que se produce un filtrado incorrecto, es decir, se predice que no pertenecen a la clase correcta cuando en realidad sí pertenecen.
- -heatmap = sns.heatmap(values[1],annot=True)
-plt.title(RETURN_VALUES[1])
-
-# Mostrar el heatmap
-plt.show()
-
|
Se puede observar que el modelo muestra un desequilibrio en los datos, lo cual puede afectar su capacidad de generalización y confiabilidad en la detección de las clases minoritarias. Aunque logra detectar correctamente los textos que no pertenecen a su clase, es importante tener en cuenta que el desbalance puede generar una tendencia hacia la clasificación de la clase mayoritaria.
-Este desbalance puede afectar la precisión y la capacidad del modelo para detectar correctamente las clases minoritarias, lo que implica que los resultados obtenidos podrían no ser completamente confiables. Para abordar este problema, se recomienda considerar técnicas de manejo de desequilibrio de datos, como la submuestreo o sobremuestreo de las clases minoritarias, o el uso de técnicas de ponderación de clases durante el entrenamiento del modelo. Esto ayudará a mejorar el rendimiento del modelo en la detección de todas las clases de manera equilibrada.
- -heatmap = sns.heatmap(values[2],annot=True)
-plt.title(RETURN_VALUES[2] )
-
-# Mostrar el heatmap
-plt.show()
-
|
Se puede observar que el modelo tiene un buen desempeño al predecir correctamente las muestras que pertenecen a su clase. Sin embargo, también se observa un alto número de falsos positivos, es decir, casos en los que el modelo predice incorrectamente que una muestra pertenece a su clase cuando en realidad no lo hace.
-Este resultado indica que el modelo puede tener una tendencia a sobreestimar la pertenencia a su clase, lo que lleva a un mayor número de falsos positivos. Es importante tener en cuenta este aspecto, ya que puede afectar la confiabilidad de las predicciones del modelo.
-Para abordar este problema, se pueden considerar diferentes enfoques, como ajustar el umbral de clasificación del modelo para equilibrar la precisión y el recall, o utilizar técnicas de ajuste de pesos de clase para penalizar los falsos positivos de manera más significativa durante el entrenamiento.
- -heatmap = sns.heatmap(values[3],annot=True)
-plt.title(RETURN_VALUES[3] )
-
-# Mostrar el heatmap
-plt.show()
-
|
Se puede observar que el modelo muestra una buena capacidad para detectar correctamente las muestras negativas, lo cual es un aspecto positivo. Sin embargo, también se evidencia una tendencia a tener falsos positivos, es decir, predicciones incorrectas de muestras positivas.
- -heatmap = sns.heatmap(values[4],annot=True)
-plt.title(RETURN_VALUES[4])
-
-# Mostrar el heatmap
-plt.show()
-
|
Se puede observar que el modelo muestra una capacidad limitada para detectar correctamente las muestras positivas, ya que se encuentran altos números de falsos positivos. Esto significa que el modelo tiende a clasificar erróneamente muestras que no pertenecen a la clase positiva como positivas.
- -heatmap = sns.heatmap(values[5],annot=True)
-plt.title(RETURN_VALUES[5] )
-
-# Mostrar el heatmap
-plt.show()
-
|
Se puede observar claramente un desbalance en los datos, donde la clase negativa está representada en mayor medida que la clase positiva. Esto puede ser un factor que contribuye a la capacidad limitada del modelo para detectar correctamente la clase positiva.
- -heatmap = sns.heatmap(values[6],annot=True)
-plt.title(RETURN_VALUES[6] )
-
-# Mostrar el heatmap
-plt.show()
-
|
Se puede observar que el modelo muestra un buen desempeño en la detección de la clase negativa, lo cual es un aspecto positivo. Sin embargo, se presentan dificultades en la detección de la clase positiva.
-El hecho de que el modelo logre detectar correctamente la clase negativa indica que puede capturar las características distintivas de las muestras negativas y realizar predicciones precisas en esa categoría. Esto es alentador y muestra que el modelo puede identificar eficazmente los textos que no pertenecen a la clase negativa.
- -heatmap = sns.heatmap(values[7],annot=True)
-plt.title(RETURN_VALUES[7] )
-
-# Mostrar el heatmap
-plt.show()
-
|
Según la información proporcionada, se puede observar que el modelo muestra dificultades en la detección de la clase negativa, mientras que logra detectar la clase positiva aunque con errores.
-El hecho de que el modelo se equivoque constantemente en la detección de la clase negativa indica que hay características o patrones específicos en las muestras negativas que no están siendo capturados adecuadamente por el modelo. Esto puede llevar a un alto número de falsos positivos en la clasificación de muestras que no pertenecen a la clase negativa.
- -heatmap = sns.heatmap(values[8],annot=True)
-plt.title(RETURN_VALUES[8])
-
-# Mostrar el heatmap
-plt.show()
-
|
El modelo muestra un buen desempeño en la detección de la clase negativa, lo cual es alentador. Sin embargo, su rendimiento en la detección de la clase positiva es calificado como regular.
-El hecho de que el modelo logre identificar correctamente la clase negativa indica que puede capturar las características distintivas de las muestras negativas y realizar predicciones precisas en esa categoría. Esto es positivo, ya que el modelo es capaz de discernir textos que no pertenecen a la clase negativa con una buena precisión.
-Por otro lado, el rendimiento regular en la detección de la clase positiva indica que el modelo tiene dificultades para identificar correctamente las muestras que pertenecen a esta categoría. Esto se refleja en una menor precisión y recall para la clase positiva, así como un mayor número de falsos negativos.
-Para mejorar el rendimiento en la detección de la clase positiva, se pueden considerar diferentes estrategias. Esto incluye ajustar los umbrales de clasificación, utilizar técnicas de aumento de datos para equilibrar la clase positiva y explorar arquitecturas de modelos más complejas o métodos de aprendizaje más avanzados.
- -import numpy as np
-import matplotlib.pyplot as plt
-from sklearn.metrics import roc_curve, auc
-
-# test_labels: etiquetas verdaderas del conjunto de prueba
-# binary_preds: predicciones binarias del modelo para el conjunto de prueba
-# RETURN_VALUES: nombres de las clases
-
-# Calcular las curvas ROC y AUC para cada clase
-fpr = dict()
-tpr = dict()
-roc_auc = dict()
-n_classes = len(RETURN_VALUES)
-for i in range(n_classes):
- fpr[i], tpr[i], _ = roc_curve(test_labels[:, i], binary_preds[:, i])
- roc_auc[i] = auc(fpr[i], tpr[i])
-
-# Calcular la curva ROC micro
-fpr_micro, tpr_micro, _ = roc_curve(test_labels.ravel(), binary_preds.ravel())
-roc_auc_micro = auc(fpr_micro, tpr_micro)
-
-# Calcular la curva ROC macro
-all_fpr = np.unique(np.concatenate(list(fpr.values())))
-mean_tpr = np.zeros_like(all_fpr)
-for i in range(n_classes):
- mean_tpr += np.interp(all_fpr, fpr[i], tpr[i])
-mean_tpr /= n_classes
-fpr_macro = all_fpr
-tpr_macro = mean_tpr
-roc_auc_macro = auc(fpr_macro, tpr_macro)
-
-# Plotear las curvas ROC
-plt.figure(figsize=(10, 8))
-
-# Plotear las curvas ROC para cada clase
-for i in range(n_classes):
- plt.plot(fpr[i], tpr[i], label='Curva ROC {} (AUC = {:.2f})'.format(RETURN_VALUES[i], roc_auc[i]))
-
-# Plotear la curva ROC micro
-plt.plot(fpr_micro, tpr_micro, label='Curva ROC micro (AUC = {:.2f})'.format(roc_auc_micro), color='deeppink', linestyle=':', linewidth=4)
-
-# Plotear la curva ROC macro
-plt.plot(fpr_macro, tpr_macro, label='Curva ROC macro (AUC = {:.2f})'.format(roc_auc_macro), color='navy', linestyle=':', linewidth=4)
-
-plt.plot([0, 1], [0, 1], 'k--', linewidth=2)
-plt.xlim([0.0, 1.0])
-plt.ylim([0.0, 1.05])
-plt.xlabel('Tasa de Falsos Positivos')
-plt.ylabel('Tasa de Verdaderos Positivos')
-plt.title('Curvas AUC-ROC para Clasificación Multietiqueta')
-plt.legend(loc="lower right")
-plt.show()
-
|
En conclusión, al analizar las métricas y los resultados obtenidos, se puede observar lo siguiente:
-La detección de la clase "companies_sentiment_neutral" presenta dificultades tanto para los etiquetadores como para el modelo. Esto se refleja en un AUC bajo, lo que indica que el modelo está adivinando en lugar de hacer predicciones precisas. La neutralidad es un concepto subjetivo y puede ser difícil de definir y clasificar correctamente.
-En general, las métricas para las otras clases son aceptables, aunque se puede mejorar el modelo. Una recomendación es etiquetar más datos para la clase "companies_sentiment" y aumentar el tamaño del conjunto de datos para incluir más ejemplos de noticias relacionadas con América Latina. Esto ayudará a fortalecer el desempeño del modelo en el contexto empresarial.
-El siguiente paso sería evaluar la incertidumbre de los nuevos datos utilizando la filosofía del active learning. Esto implica seleccionar cuidadosamente las muestras que el modelo encuentra más difíciles de clasificar y agregarlas al conjunto de entrenamiento para mejorar el rendimiento general.
-Dado que siempre habrá un desequilibrio en los datos debido a la naturaleza de cómo se modela el problema, es importante penalizar con más peso las clases positivas. Esto puede lograrse mediante técnicas de ponderación de clases o ajustando los umbrales de clasificación para tener en cuenta la importancia relativa de cada clase.
-Baseline
", - AutoEvalColumn.revision.name: "N/A", - AutoEvalColumn.precision.name: None, - AutoEvalColumn.average.name: 25.0, - AutoEvalColumn.arc.name: 25.0, - AutoEvalColumn.hellaswag.name: 25.0, - AutoEvalColumn.mmlu.name: 25.0, - AutoEvalColumn.truthfulqa.name: 25.0, - AutoEvalColumn.winogrande.name: 50.0, - AutoEvalColumn.gsm8k.name: 0.21, - AutoEvalColumn.drop.name: 0.47, - AutoEvalColumn.dummy.name: "baseline", - AutoEvalColumn.model_type.name: "", -} diff --git a/spaces/ICML2022/OFA/fairseq/examples/criss/mining/mine.py b/spaces/ICML2022/OFA/fairseq/examples/criss/mining/mine.py deleted file mode 100644 index c872da196fe0df776622365748ad7963fee1f0a0..0000000000000000000000000000000000000000 --- a/spaces/ICML2022/OFA/fairseq/examples/criss/mining/mine.py +++ /dev/null @@ -1,240 +0,0 @@ -#!/usr/bin/env python3 -u -# Copyright (c) Facebook, Inc. and its affiliates. -# -# This source code is licensed under the MIT license found in the -# LICENSE file in the root directory of this source tree. -import argparse -import glob -from subprocess import check_call - -try: - import faiss - - has_faiss = True -except ImportError: - has_faiss = False -import numpy as np - - -GB = 1024 * 1024 * 1024 - - -def call(cmd): - print(cmd) - check_call(cmd, shell=True) - - -def get_batches(directory, lang, prefix="all_avg_pool"): - print(f"Finding in {directory}/{prefix}.{lang}*") - files = glob.glob(f"{directory}/{prefix}.{lang}*") - emb_files = [] - txt_files = [] - for emb_fi in files: - emb_files.append(emb_fi) - txt_fi = emb_fi.replace(prefix, "sentences") - txt_files.append(txt_fi) - return emb_files, txt_files - - -def load_batch(emb_file, dim): - embeddings = np.fromfile(emb_file, dtype=np.float32) - num_rows = int(embeddings.shape[0] / dim) - embeddings = embeddings.reshape((num_rows, dim)) - faiss.normalize_L2(embeddings) - return embeddings - - -def knnGPU_sharded(x_batches_f, y_batches_f, dim, k, direction="x2y"): - if not has_faiss: - raise ImportError("Please install Faiss") - sims = [] - inds = [] - xfrom = 0 - xto = 0 - for x_batch_f in x_batches_f: - yfrom = 0 - yto = 0 - x_batch = load_batch(x_batch_f, dim) - xto = xfrom + x_batch.shape[0] - bsims, binds = [], [] - for y_batch_f in y_batches_f: - y_batch = load_batch(y_batch_f, dim) - neighbor_size = min(k, y_batch.shape[0]) - yto = yfrom + y_batch.shape[0] - print("{}-{} -> {}-{}".format(xfrom, xto, yfrom, yto)) - idx = faiss.IndexFlatIP(dim) - idx = faiss.index_cpu_to_all_gpus(idx) - idx.add(y_batch) - bsim, bind = idx.search(x_batch, neighbor_size) - - bsims.append(bsim) - binds.append(bind + yfrom) - yfrom += y_batch.shape[0] - del idx - del y_batch - bsims = np.concatenate(bsims, axis=1) - binds = np.concatenate(binds, axis=1) - aux = np.argsort(-bsims, axis=1) - sim_batch = np.zeros((x_batch.shape[0], k), dtype=np.float32) - ind_batch = np.zeros((x_batch.shape[0], k), dtype=np.int64) - for i in range(x_batch.shape[0]): - for j in range(k): - sim_batch[i, j] = bsims[i, aux[i, j]] - ind_batch[i, j] = binds[i, aux[i, j]] - sims.append(sim_batch) - inds.append(ind_batch) - xfrom += x_batch.shape[0] - del x_batch - sim = np.concatenate(sims, axis=0) - ind = np.concatenate(inds, axis=0) - return sim, ind - - -def score(sim, fwd_mean, bwd_mean, margin): - return margin(sim, (fwd_mean + bwd_mean) / 2) - - -def score_candidates( - sim_mat, candidate_inds, fwd_mean, bwd_mean, margin, verbose=False -): - print(" - scoring {:d} candidates".format(sim_mat.shape[0])) - scores = np.zeros(candidate_inds.shape) - for i in range(scores.shape[0]): - for j in range(scores.shape[1]): - k = int(candidate_inds[i, j]) - scores[i, j] = score(sim_mat[i, j], fwd_mean[i], bwd_mean[k], margin) - return scores - - -def load_text(files): - all_sentences = [] - for fi in files: - with open(fi) as sentence_fi: - for line in sentence_fi: - all_sentences.append(line.strip()) - print(f"Read {len(all_sentences)} sentences") - return all_sentences - - -if __name__ == "__main__": - parser = argparse.ArgumentParser(description="Mine bitext") - parser.add_argument("--src-lang", help="Source language") - parser.add_argument("--tgt-lang", help="Target language") - parser.add_argument( - "--dict-path", help="Path to dictionary file", default="dict.txt" - ) - parser.add_argument( - "--spm-path", help="Path to SPM model file", default="sentence.bpe.model" - ) - parser.add_argument("--dim", type=int, default=1024, help="Embedding dimension") - parser.add_argument("--mem", type=int, default=5, help="Memory in GB") - parser.add_argument("--src-dir", help="Source directory") - parser.add_argument("--tgt-dir", help="Target directory") - parser.add_argument("--output", help="Output path") - parser.add_argument( - "--neighborhood", type=int, default=4, help="Embedding dimension" - ) - parser.add_argument( - "--threshold", type=float, default=1.06, help="Threshold on mined bitext" - ) - parser.add_argument( - "--valid-size", - type=int, - default=2000, - help="Number of sentences used for validation set", - ) - parser.add_argument( - "--min-count", - type=int, - default=50000, - help="Min num sentences used for each language", - ) - args = parser.parse_args() - - x_batches_f, x_sents_f = get_batches(args.src_dir, args.src_lang) - y_batches_f, y_sents_f = get_batches(args.tgt_dir, args.tgt_lang) - margin = lambda a, b: a / b - y2x_sim, y2x_ind = knnGPU_sharded( - y_batches_f, x_batches_f, args.dim, args.neighborhood, direction="y2x" - ) - x2y_sim, x2y_ind = knnGPU_sharded( - x_batches_f, y_batches_f, args.dim, args.neighborhood, direction="x2y" - ) - - x2y_mean = x2y_sim.mean(axis=1) - y2x_mean = y2x_sim.mean(axis=1) - fwd_scores = score_candidates(x2y_sim, x2y_ind, x2y_mean, y2x_mean, margin) - bwd_scores = score_candidates(y2x_sim, y2x_ind, y2x_mean, x2y_mean, margin) - fwd_best = x2y_ind[np.arange(x2y_sim.shape[0]), fwd_scores.argmax(axis=1)] - bwd_best = y2x_ind[np.arange(y2x_sim.shape[0]), bwd_scores.argmax(axis=1)] - indices = np.stack( - ( - np.concatenate((np.arange(x2y_ind.shape[0]), bwd_best)), - np.concatenate((fwd_best, np.arange(y2x_ind.shape[0]))), - ), - axis=1, - ) - scores = np.concatenate((fwd_scores.max(axis=1), bwd_scores.max(axis=1))) - - x_sentences = load_text(x_sents_f) - y_sentences = load_text(y_sents_f) - - threshold = args.threshold - min_count = args.min_count - seen_src, seen_trg = set(), set() - directory = args.output - call(f"mkdir -p {directory}") - src_out = open( - f"{directory}/all.{args.src_lang}", - mode="w", - encoding="utf-8", - errors="surrogateescape", - ) - tgt_out = open( - f"{directory}/all.{args.tgt_lang}", - mode="w", - encoding="utf-8", - errors="surrogateescape", - ) - scores_out = open( - f"{directory}/all.scores", mode="w", encoding="utf-8", errors="surrogateescape" - ) - count = 0 - for i in np.argsort(-scores): - src_ind, trg_ind = indices[i] - if src_ind not in seen_src and trg_ind not in seen_trg: - seen_src.add(src_ind) - seen_trg.add(trg_ind) - if scores[i] > threshold or count < min_count: - if x_sentences[src_ind]: - print(scores[i], file=scores_out) - print(x_sentences[src_ind], file=src_out) - print(y_sentences[trg_ind], file=tgt_out) - count += 1 - else: - print(f"Ignoring sentence: {x_sentences[src_ind]}") - src_out.close() - tgt_out.close() - scores_out.close() - - print(f"Found {count} pairs for threshold={threshold}") - with open(f"{directory}/all.{args.src_lang}") as all_s, open( - f"{directory}/all.{args.tgt_lang}" - ) as all_t, open(f"{directory}/valid.{args.src_lang}", "w") as valid_s, open( - f"{directory}/valid.{args.tgt_lang}", "w" - ) as valid_t, open( - f"{directory}/train.{args.src_lang}", "w" - ) as train_s, open( - f"{directory}/train.{args.tgt_lang}", "w" - ) as train_t: - count = 0 - for s_line, t_line in zip(all_s, all_t): - s_line = s_line.split("\t")[1] - t_line = t_line.split("\t")[1] - if count >= args.valid_size: - train_s.write(s_line) - train_t.write(t_line) - else: - valid_s.write(s_line) - valid_t.write(t_line) - count += 1 diff --git a/spaces/ICML2022/OFA/fairseq/examples/m2m_100/tokenizers/tokenize_thai.py b/spaces/ICML2022/OFA/fairseq/examples/m2m_100/tokenizers/tokenize_thai.py deleted file mode 100644 index 9c72cb89056f6fc92a8963415e5f3a1e61b33a5b..0000000000000000000000000000000000000000 --- a/spaces/ICML2022/OFA/fairseq/examples/m2m_100/tokenizers/tokenize_thai.py +++ /dev/null @@ -1,13 +0,0 @@ -#!/usr/bin/env python3 -# Copyright (c) Facebook, Inc. and its affiliates. -# -# This source code is licensed under the MIT license found in the -# LICENSE file in the root directory of this source tree. - -import sys - -from pythainlp import word_tokenize - - -for line in sys.stdin: - print(" ".join(word_tokenize(line.strip()))) diff --git a/spaces/ICML2022/OFA/fairseq/examples/wav2vec/unsupervised/data/__init__.py b/spaces/ICML2022/OFA/fairseq/examples/wav2vec/unsupervised/data/__init__.py deleted file mode 100644 index d0545627efc9a6f9bb180e351ead519a2cb6dea7..0000000000000000000000000000000000000000 --- a/spaces/ICML2022/OFA/fairseq/examples/wav2vec/unsupervised/data/__init__.py +++ /dev/null @@ -1,13 +0,0 @@ -# Copyright (c) Facebook, Inc. and its affiliates. -# -# This source code is licensed under the MIT license found in the -# LICENSE file in the root directory of this source tree. - -from .extracted_features_dataset import ExtractedFeaturesDataset -from .random_input_dataset import RandomInputDataset - - -__all__ = [ - "ExtractedFeaturesDataset", - "RandomInputDataset", -] diff --git a/spaces/IDEA-Research/Grounded-SAM/GroundingDINO/groundingdino/util/time_counter.py b/spaces/IDEA-Research/Grounded-SAM/GroundingDINO/groundingdino/util/time_counter.py deleted file mode 100644 index 0aedb2e4d61bfbe7571dca9d50053f0fedaa1359..0000000000000000000000000000000000000000 --- a/spaces/IDEA-Research/Grounded-SAM/GroundingDINO/groundingdino/util/time_counter.py +++ /dev/null @@ -1,62 +0,0 @@ -import json -import time - - -class TimeCounter: - def __init__(self) -> None: - pass - - def clear(self): - self.timedict = {} - self.basetime = time.perf_counter() - - def timeit(self, name): - nowtime = time.perf_counter() - self.basetime - self.timedict[name] = nowtime - self.basetime = time.perf_counter() - - -class TimeHolder: - def __init__(self) -> None: - self.timedict = {} - - def update(self, _timedict: dict): - for k, v in _timedict.items(): - if k not in self.timedict: - self.timedict[k] = AverageMeter(name=k, val_only=True) - self.timedict[k].update(val=v) - - def final_res(self): - return {k: v.avg for k, v in self.timedict.items()} - - def __str__(self): - return json.dumps(self.final_res(), indent=2) - - -class AverageMeter(object): - """Computes and stores the average and current value""" - - def __init__(self, name, fmt=":f", val_only=False): - self.name = name - self.fmt = fmt - self.val_only = val_only - self.reset() - - def reset(self): - self.val = 0 - self.avg = 0 - self.sum = 0 - self.count = 0 - - def update(self, val, n=1): - self.val = val - self.sum += val * n - self.count += n - self.avg = self.sum / self.count - - def __str__(self): - if self.val_only: - fmtstr = "{name} {val" + self.fmt + "}" - else: - fmtstr = "{name} {val" + self.fmt + "} ({avg" + self.fmt + "})" - return fmtstr.format(**self.__dict__) diff --git a/spaces/Ikaros521/so-vits-svc-4.0-ikaros2/resample.py b/spaces/Ikaros521/so-vits-svc-4.0-ikaros2/resample.py deleted file mode 100644 index 5e96106c9a066e6d73652c544322d029dd98f746..0000000000000000000000000000000000000000 --- a/spaces/Ikaros521/so-vits-svc-4.0-ikaros2/resample.py +++ /dev/null @@ -1,48 +0,0 @@ -import os -import argparse -import librosa -import numpy as np -from multiprocessing import Pool, cpu_count -from scipy.io import wavfile -from tqdm import tqdm - - -def process(item): - spkdir, wav_name, args = item - # speaker 's5', 'p280', 'p315' are excluded, - speaker = spkdir.replace("\\", "/").split("/")[-1] - wav_path = os.path.join(args.in_dir, speaker, wav_name) - if os.path.exists(wav_path) and '.wav' in wav_path: - os.makedirs(os.path.join(args.out_dir2, speaker), exist_ok=True) - wav, sr = librosa.load(wav_path, None) - wav, _ = librosa.effects.trim(wav, top_db=20) - peak = np.abs(wav).max() - if peak > 1.0: - wav = 0.98 * wav / peak - wav2 = librosa.resample(wav, orig_sr=sr, target_sr=args.sr2) - wav2 /= max(wav2.max(), -wav2.min()) - save_name = wav_name - save_path2 = os.path.join(args.out_dir2, speaker, save_name) - wavfile.write( - save_path2, - args.sr2, - (wav2 * np.iinfo(np.int16).max).astype(np.int16) - ) - - - -if __name__ == "__main__": - parser = argparse.ArgumentParser() - parser.add_argument("--sr2", type=int, default=44100, help="sampling rate") - parser.add_argument("--in_dir", type=str, default="./dataset_raw", help="path to source dir") - parser.add_argument("--out_dir2", type=str, default="./dataset/44k", help="path to target dir") - args = parser.parse_args() - processs = cpu_count()-2 if cpu_count() >4 else 1 - pool = Pool(processes=processs) - - for speaker in os.listdir(args.in_dir): - spk_dir = os.path.join(args.in_dir, speaker) - if os.path.isdir(spk_dir): - print(spk_dir) - for _ in tqdm(pool.imap_unordered(process, [(spk_dir, i, args) for i in os.listdir(spk_dir) if i.endswith("wav")])): - pass diff --git a/spaces/InpaintAI/Inpaint-Anything/third_party/lama/bin/to_jit.py b/spaces/InpaintAI/Inpaint-Anything/third_party/lama/bin/to_jit.py deleted file mode 100644 index 8acea396545cdadbc004618a23c78d60c0ed6e95..0000000000000000000000000000000000000000 --- a/spaces/InpaintAI/Inpaint-Anything/third_party/lama/bin/to_jit.py +++ /dev/null @@ -1,75 +0,0 @@ -import os -from pathlib import Path - -import hydra -import torch -import yaml -from omegaconf import OmegaConf -from torch import nn - -from saicinpainting.training.trainers import load_checkpoint -from saicinpainting.utils import register_debug_signal_handlers - - -class JITWrapper(nn.Module): - def __init__(self, model): - super().__init__() - self.model = model - - def forward(self, image, mask): - batch = { - "image": image, - "mask": mask - } - out = self.model(batch) - return out["inpainted"] - - -@hydra.main(config_path="../configs/prediction", config_name="default.yaml") -def main(predict_config: OmegaConf): - register_debug_signal_handlers() # kill -10Your ratings have been saved! -You have been moved to the next random seed, if you want -to keep rating more samples.
-{% endif %} -{% if already_filled %} -You already rated those samples in the past, - filling this form will override your previous ratings. -
-{% endif %} -Welcome {{session['user']}} to the survey #{{signature}}. -Go to the result page to check the results. Go to the home page to start a new survey. -
- -{% for error in errors %} -{{error}}
-{% endfor %} - -{% if not blind %} -Base config is: {{ref_name}}
-The following experiments are compared:
-This is a blind experiment, the order of all XPs is shuffled with every sample.
-{% endif %} -The current random seed is {{seed}}. You can change it with the following form, and also update blind/non blind. -
- - - 元素,则不添加按钮
- }
- var firstChild = code.firstChild;
- if (!firstChild) {
- return; // 如果 元素没有子节点,则不添加按钮
- }
- var button = document.createElement('button');
- button.textContent = '\uD83D\uDCCE'; // 使用 📎 符号作为“复制”按钮的文本
- button.style.position = 'relative';
- button.style.float = 'right';
- button.style.fontSize = '1em'; // 可选:调整按钮大小
- button.style.background = 'none'; // 可选:去掉背景颜色
- button.style.border = 'none'; // 可选:去掉边框
- button.style.cursor = 'pointer'; // 可选:显示指针样式
- button.addEventListener('click', function () {
- var range = document.createRange();
- range.selectNodeContents(code);
- range.setStartBefore(firstChild); // 将范围设置为第一个子节点之前
- var selection = window.getSelection();
- selection.removeAllRanges();
- selection.addRange(range);
-
- try {
- var success = document.execCommand('copy');
- if (success) {
- button.textContent = '\u2714';
- setTimeout(function () {
- button.textContent = '\uD83D\uDCCE'; // 恢复按钮为“复制”
- }, 2000);
- } else {
- button.textContent = '\u2716';
- }
- } catch (e) {
- console.error(e);
- button.textContent = '\u2716';
- }
-
- selection.removeAllRanges();
- });
- code.insertBefore(button, firstChild); // 将按钮插入到第一个子元素之前
- }
-
- function handleNewElements(mutationsList, observer) {
- for (var mutation of mutationsList) {
- if (mutation.type === 'childList') {
- for (var node of mutation.addedNodes) {
- if (node.nodeName === 'PRE') {
- addCopyButton(node);
- }
- }
- }
- }
- }
-
- var observer = new MutationObserver(handleNewElements);
- observer.observe(document.documentElement, { childList: true, subtree: true });
-
- document.querySelectorAll('pre').forEach(addCopyButton);
-})();
diff --git a/spaces/XzJosh/LittleTaffy-Bert-VITS2/transforms.py b/spaces/XzJosh/LittleTaffy-Bert-VITS2/transforms.py
deleted file mode 100644
index 4793d67ca5a5630e0ffe0f9fb29445c949e64dae..0000000000000000000000000000000000000000
--- a/spaces/XzJosh/LittleTaffy-Bert-VITS2/transforms.py
+++ /dev/null
@@ -1,193 +0,0 @@
-import torch
-from torch.nn import functional as F
-
-import numpy as np
-
-
-DEFAULT_MIN_BIN_WIDTH = 1e-3
-DEFAULT_MIN_BIN_HEIGHT = 1e-3
-DEFAULT_MIN_DERIVATIVE = 1e-3
-
-
-def piecewise_rational_quadratic_transform(inputs,
- unnormalized_widths,
- unnormalized_heights,
- unnormalized_derivatives,
- inverse=False,
- tails=None,
- tail_bound=1.,
- min_bin_width=DEFAULT_MIN_BIN_WIDTH,
- min_bin_height=DEFAULT_MIN_BIN_HEIGHT,
- min_derivative=DEFAULT_MIN_DERIVATIVE):
-
- if tails is None:
- spline_fn = rational_quadratic_spline
- spline_kwargs = {}
- else:
- spline_fn = unconstrained_rational_quadratic_spline
- spline_kwargs = {
- 'tails': tails,
- 'tail_bound': tail_bound
- }
-
- outputs, logabsdet = spline_fn(
- inputs=inputs,
- unnormalized_widths=unnormalized_widths,
- unnormalized_heights=unnormalized_heights,
- unnormalized_derivatives=unnormalized_derivatives,
- inverse=inverse,
- min_bin_width=min_bin_width,
- min_bin_height=min_bin_height,
- min_derivative=min_derivative,
- **spline_kwargs
- )
- return outputs, logabsdet
-
-
-def searchsorted(bin_locations, inputs, eps=1e-6):
- bin_locations[..., -1] += eps
- return torch.sum(
- inputs[..., None] >= bin_locations,
- dim=-1
- ) - 1
-
-
-def unconstrained_rational_quadratic_spline(inputs,
- unnormalized_widths,
- unnormalized_heights,
- unnormalized_derivatives,
- inverse=False,
- tails='linear',
- tail_bound=1.,
- min_bin_width=DEFAULT_MIN_BIN_WIDTH,
- min_bin_height=DEFAULT_MIN_BIN_HEIGHT,
- min_derivative=DEFAULT_MIN_DERIVATIVE):
- inside_interval_mask = (inputs >= -tail_bound) & (inputs <= tail_bound)
- outside_interval_mask = ~inside_interval_mask
-
- outputs = torch.zeros_like(inputs)
- logabsdet = torch.zeros_like(inputs)
-
- if tails == 'linear':
- unnormalized_derivatives = F.pad(unnormalized_derivatives, pad=(1, 1))
- constant = np.log(np.exp(1 - min_derivative) - 1)
- unnormalized_derivatives[..., 0] = constant
- unnormalized_derivatives[..., -1] = constant
-
- outputs[outside_interval_mask] = inputs[outside_interval_mask]
- logabsdet[outside_interval_mask] = 0
- else:
- raise RuntimeError('{} tails are not implemented.'.format(tails))
-
- outputs[inside_interval_mask], logabsdet[inside_interval_mask] = rational_quadratic_spline(
- inputs=inputs[inside_interval_mask],
- unnormalized_widths=unnormalized_widths[inside_interval_mask, :],
- unnormalized_heights=unnormalized_heights[inside_interval_mask, :],
- unnormalized_derivatives=unnormalized_derivatives[inside_interval_mask, :],
- inverse=inverse,
- left=-tail_bound, right=tail_bound, bottom=-tail_bound, top=tail_bound,
- min_bin_width=min_bin_width,
- min_bin_height=min_bin_height,
- min_derivative=min_derivative
- )
-
- return outputs, logabsdet
-
-def rational_quadratic_spline(inputs,
- unnormalized_widths,
- unnormalized_heights,
- unnormalized_derivatives,
- inverse=False,
- left=0., right=1., bottom=0., top=1.,
- min_bin_width=DEFAULT_MIN_BIN_WIDTH,
- min_bin_height=DEFAULT_MIN_BIN_HEIGHT,
- min_derivative=DEFAULT_MIN_DERIVATIVE):
- if torch.min(inputs) < left or torch.max(inputs) > right:
- raise ValueError('Input to a transform is not within its domain')
-
- num_bins = unnormalized_widths.shape[-1]
-
- if min_bin_width * num_bins > 1.0:
- raise ValueError('Minimal bin width too large for the number of bins')
- if min_bin_height * num_bins > 1.0:
- raise ValueError('Minimal bin height too large for the number of bins')
-
- widths = F.softmax(unnormalized_widths, dim=-1)
- widths = min_bin_width + (1 - min_bin_width * num_bins) * widths
- cumwidths = torch.cumsum(widths, dim=-1)
- cumwidths = F.pad(cumwidths, pad=(1, 0), mode='constant', value=0.0)
- cumwidths = (right - left) * cumwidths + left
- cumwidths[..., 0] = left
- cumwidths[..., -1] = right
- widths = cumwidths[..., 1:] - cumwidths[..., :-1]
-
- derivatives = min_derivative + F.softplus(unnormalized_derivatives)
-
- heights = F.softmax(unnormalized_heights, dim=-1)
- heights = min_bin_height + (1 - min_bin_height * num_bins) * heights
- cumheights = torch.cumsum(heights, dim=-1)
- cumheights = F.pad(cumheights, pad=(1, 0), mode='constant', value=0.0)
- cumheights = (top - bottom) * cumheights + bottom
- cumheights[..., 0] = bottom
- cumheights[..., -1] = top
- heights = cumheights[..., 1:] - cumheights[..., :-1]
-
- if inverse:
- bin_idx = searchsorted(cumheights, inputs)[..., None]
- else:
- bin_idx = searchsorted(cumwidths, inputs)[..., None]
-
- input_cumwidths = cumwidths.gather(-1, bin_idx)[..., 0]
- input_bin_widths = widths.gather(-1, bin_idx)[..., 0]
-
- input_cumheights = cumheights.gather(-1, bin_idx)[..., 0]
- delta = heights / widths
- input_delta = delta.gather(-1, bin_idx)[..., 0]
-
- input_derivatives = derivatives.gather(-1, bin_idx)[..., 0]
- input_derivatives_plus_one = derivatives[..., 1:].gather(-1, bin_idx)[..., 0]
-
- input_heights = heights.gather(-1, bin_idx)[..., 0]
-
- if inverse:
- a = (((inputs - input_cumheights) * (input_derivatives
- + input_derivatives_plus_one
- - 2 * input_delta)
- + input_heights * (input_delta - input_derivatives)))
- b = (input_heights * input_derivatives
- - (inputs - input_cumheights) * (input_derivatives
- + input_derivatives_plus_one
- - 2 * input_delta))
- c = - input_delta * (inputs - input_cumheights)
-
- discriminant = b.pow(2) - 4 * a * c
- assert (discriminant >= 0).all()
-
- root = (2 * c) / (-b - torch.sqrt(discriminant))
- outputs = root * input_bin_widths + input_cumwidths
-
- theta_one_minus_theta = root * (1 - root)
- denominator = input_delta + ((input_derivatives + input_derivatives_plus_one - 2 * input_delta)
- * theta_one_minus_theta)
- derivative_numerator = input_delta.pow(2) * (input_derivatives_plus_one * root.pow(2)
- + 2 * input_delta * theta_one_minus_theta
- + input_derivatives * (1 - root).pow(2))
- logabsdet = torch.log(derivative_numerator) - 2 * torch.log(denominator)
-
- return outputs, -logabsdet
- else:
- theta = (inputs - input_cumwidths) / input_bin_widths
- theta_one_minus_theta = theta * (1 - theta)
-
- numerator = input_heights * (input_delta * theta.pow(2)
- + input_derivatives * theta_one_minus_theta)
- denominator = input_delta + ((input_derivatives + input_derivatives_plus_one - 2 * input_delta)
- * theta_one_minus_theta)
- outputs = input_cumheights + numerator / denominator
-
- derivative_numerator = input_delta.pow(2) * (input_derivatives_plus_one * theta.pow(2)
- + 2 * input_delta * theta_one_minus_theta
- + input_derivatives * (1 - theta).pow(2))
- logabsdet = torch.log(derivative_numerator) - 2 * torch.log(denominator)
-
- return outputs, logabsdet
diff --git a/spaces/XzJosh/TianDou-Bert-VITS2/server.py b/spaces/XzJosh/TianDou-Bert-VITS2/server.py
deleted file mode 100644
index c736ca4f95fec853950eef6654ef79856beffc0a..0000000000000000000000000000000000000000
--- a/spaces/XzJosh/TianDou-Bert-VITS2/server.py
+++ /dev/null
@@ -1,123 +0,0 @@
-from flask import Flask, request, Response
-from io import BytesIO
-import torch
-from av import open as avopen
-
-import commons
-import utils
-from models import SynthesizerTrn
-from text.symbols import symbols
-from text import cleaned_text_to_sequence, get_bert
-from text.cleaner import clean_text
-from scipy.io import wavfile
-
-# Flask Init
-app = Flask(__name__)
-app.config['JSON_AS_ASCII'] = False
-def get_text(text, language_str, hps):
- norm_text, phone, tone, word2ph = clean_text(text, language_str)
- print([f"{p}{t}" for p, t in zip(phone, tone)])
- phone, tone, language = cleaned_text_to_sequence(phone, tone, language_str)
-
- if hps.data.add_blank:
- phone = commons.intersperse(phone, 0)
- tone = commons.intersperse(tone, 0)
- language = commons.intersperse(language, 0)
- for i in range(len(word2ph)):
- word2ph[i] = word2ph[i] * 2
- word2ph[0] += 1
- bert = get_bert(norm_text, word2ph, language_str)
-
- assert bert.shape[-1] == len(phone)
-
- phone = torch.LongTensor(phone)
- tone = torch.LongTensor(tone)
- language = torch.LongTensor(language)
-
- return bert, phone, tone, language
-
-def infer(text, sdp_ratio, noise_scale, noise_scale_w,length_scale,sid):
- bert, phones, tones, lang_ids = get_text(text,"ZH", hps,)
- with torch.no_grad():
- x_tst=phones.to(dev).unsqueeze(0)
- tones=tones.to(dev).unsqueeze(0)
- lang_ids=lang_ids.to(dev).unsqueeze(0)
- bert = bert.to(dev).unsqueeze(0)
- x_tst_lengths = torch.LongTensor([phones.size(0)]).to(dev)
- speakers = torch.LongTensor([hps.data.spk2id[sid]]).to(dev)
- audio = net_g.infer(x_tst, x_tst_lengths, speakers, tones, lang_ids,bert, sdp_ratio=sdp_ratio
- , noise_scale=noise_scale, noise_scale_w=noise_scale_w, length_scale=length_scale)[0][0,0].data.cpu().float().numpy()
- return audio
-
-def replace_punctuation(text, i=2):
- punctuation = ",。?!"
- for char in punctuation:
- text = text.replace(char, char * i)
- return text
-
-def wav2(i, o, format):
- inp = avopen(i, 'rb')
- out = avopen(o, 'wb', format=format)
- if format == "ogg": format = "libvorbis"
-
- ostream = out.add_stream(format)
-
- for frame in inp.decode(audio=0):
- for p in ostream.encode(frame): out.mux(p)
-
- for p in ostream.encode(None): out.mux(p)
-
- out.close()
- inp.close()
-
-# Load Generator
-hps = utils.get_hparams_from_file("./configs/config.json")
-
-dev='cuda'
-net_g = SynthesizerTrn(
- len(symbols),
- hps.data.filter_length // 2 + 1,
- hps.train.segment_size // hps.data.hop_length,
- n_speakers=hps.data.n_speakers,
- **hps.model).to(dev)
-_ = net_g.eval()
-
-_ = utils.load_checkpoint("logs/G_649000.pth", net_g, None,skip_optimizer=True)
-
-@app.route("/",methods=['GET','POST'])
-def main():
- if request.method == 'GET':
- try:
- speaker = request.args.get('speaker')
- text = request.args.get('text').replace("/n","")
- sdp_ratio = float(request.args.get("sdp_ratio", 0.2))
- noise = float(request.args.get("noise", 0.5))
- noisew = float(request.args.get("noisew", 0.6))
- length = float(request.args.get("length", 1.2))
- if length >= 2:
- return "Too big length"
- if len(text) >=200:
- return "Too long text"
- fmt = request.args.get("format", "wav")
- if None in (speaker, text):
- return "Missing Parameter"
- if fmt not in ("mp3", "wav", "ogg"):
- return "Invalid Format"
- except:
- return "Invalid Parameter"
-
- with torch.no_grad():
- audio = infer(text, sdp_ratio=sdp_ratio, noise_scale=noise, noise_scale_w=noisew, length_scale=length, sid=speaker)
-
- with BytesIO() as wav:
- wavfile.write(wav, hps.data.sampling_rate, audio)
- torch.cuda.empty_cache()
- if fmt == "wav":
- return Response(wav.getvalue(), mimetype="audio/wav")
- wav.seek(0, 0)
- with BytesIO() as ofp:
- wav2(wav, ofp, fmt)
- return Response(
- ofp.getvalue(),
- mimetype="audio/mpeg" if fmt == "mp3" else "audio/ogg"
- )
diff --git a/spaces/XzJosh/TianDou-Bert-VITS2/transforms.py b/spaces/XzJosh/TianDou-Bert-VITS2/transforms.py
deleted file mode 100644
index 4793d67ca5a5630e0ffe0f9fb29445c949e64dae..0000000000000000000000000000000000000000
--- a/spaces/XzJosh/TianDou-Bert-VITS2/transforms.py
+++ /dev/null
@@ -1,193 +0,0 @@
-import torch
-from torch.nn import functional as F
-
-import numpy as np
-
-
-DEFAULT_MIN_BIN_WIDTH = 1e-3
-DEFAULT_MIN_BIN_HEIGHT = 1e-3
-DEFAULT_MIN_DERIVATIVE = 1e-3
-
-
-def piecewise_rational_quadratic_transform(inputs,
- unnormalized_widths,
- unnormalized_heights,
- unnormalized_derivatives,
- inverse=False,
- tails=None,
- tail_bound=1.,
- min_bin_width=DEFAULT_MIN_BIN_WIDTH,
- min_bin_height=DEFAULT_MIN_BIN_HEIGHT,
- min_derivative=DEFAULT_MIN_DERIVATIVE):
-
- if tails is None:
- spline_fn = rational_quadratic_spline
- spline_kwargs = {}
- else:
- spline_fn = unconstrained_rational_quadratic_spline
- spline_kwargs = {
- 'tails': tails,
- 'tail_bound': tail_bound
- }
-
- outputs, logabsdet = spline_fn(
- inputs=inputs,
- unnormalized_widths=unnormalized_widths,
- unnormalized_heights=unnormalized_heights,
- unnormalized_derivatives=unnormalized_derivatives,
- inverse=inverse,
- min_bin_width=min_bin_width,
- min_bin_height=min_bin_height,
- min_derivative=min_derivative,
- **spline_kwargs
- )
- return outputs, logabsdet
-
-
-def searchsorted(bin_locations, inputs, eps=1e-6):
- bin_locations[..., -1] += eps
- return torch.sum(
- inputs[..., None] >= bin_locations,
- dim=-1
- ) - 1
-
-
-def unconstrained_rational_quadratic_spline(inputs,
- unnormalized_widths,
- unnormalized_heights,
- unnormalized_derivatives,
- inverse=False,
- tails='linear',
- tail_bound=1.,
- min_bin_width=DEFAULT_MIN_BIN_WIDTH,
- min_bin_height=DEFAULT_MIN_BIN_HEIGHT,
- min_derivative=DEFAULT_MIN_DERIVATIVE):
- inside_interval_mask = (inputs >= -tail_bound) & (inputs <= tail_bound)
- outside_interval_mask = ~inside_interval_mask
-
- outputs = torch.zeros_like(inputs)
- logabsdet = torch.zeros_like(inputs)
-
- if tails == 'linear':
- unnormalized_derivatives = F.pad(unnormalized_derivatives, pad=(1, 1))
- constant = np.log(np.exp(1 - min_derivative) - 1)
- unnormalized_derivatives[..., 0] = constant
- unnormalized_derivatives[..., -1] = constant
-
- outputs[outside_interval_mask] = inputs[outside_interval_mask]
- logabsdet[outside_interval_mask] = 0
- else:
- raise RuntimeError('{} tails are not implemented.'.format(tails))
-
- outputs[inside_interval_mask], logabsdet[inside_interval_mask] = rational_quadratic_spline(
- inputs=inputs[inside_interval_mask],
- unnormalized_widths=unnormalized_widths[inside_interval_mask, :],
- unnormalized_heights=unnormalized_heights[inside_interval_mask, :],
- unnormalized_derivatives=unnormalized_derivatives[inside_interval_mask, :],
- inverse=inverse,
- left=-tail_bound, right=tail_bound, bottom=-tail_bound, top=tail_bound,
- min_bin_width=min_bin_width,
- min_bin_height=min_bin_height,
- min_derivative=min_derivative
- )
-
- return outputs, logabsdet
-
-def rational_quadratic_spline(inputs,
- unnormalized_widths,
- unnormalized_heights,
- unnormalized_derivatives,
- inverse=False,
- left=0., right=1., bottom=0., top=1.,
- min_bin_width=DEFAULT_MIN_BIN_WIDTH,
- min_bin_height=DEFAULT_MIN_BIN_HEIGHT,
- min_derivative=DEFAULT_MIN_DERIVATIVE):
- if torch.min(inputs) < left or torch.max(inputs) > right:
- raise ValueError('Input to a transform is not within its domain')
-
- num_bins = unnormalized_widths.shape[-1]
-
- if min_bin_width * num_bins > 1.0:
- raise ValueError('Minimal bin width too large for the number of bins')
- if min_bin_height * num_bins > 1.0:
- raise ValueError('Minimal bin height too large for the number of bins')
-
- widths = F.softmax(unnormalized_widths, dim=-1)
- widths = min_bin_width + (1 - min_bin_width * num_bins) * widths
- cumwidths = torch.cumsum(widths, dim=-1)
- cumwidths = F.pad(cumwidths, pad=(1, 0), mode='constant', value=0.0)
- cumwidths = (right - left) * cumwidths + left
- cumwidths[..., 0] = left
- cumwidths[..., -1] = right
- widths = cumwidths[..., 1:] - cumwidths[..., :-1]
-
- derivatives = min_derivative + F.softplus(unnormalized_derivatives)
-
- heights = F.softmax(unnormalized_heights, dim=-1)
- heights = min_bin_height + (1 - min_bin_height * num_bins) * heights
- cumheights = torch.cumsum(heights, dim=-1)
- cumheights = F.pad(cumheights, pad=(1, 0), mode='constant', value=0.0)
- cumheights = (top - bottom) * cumheights + bottom
- cumheights[..., 0] = bottom
- cumheights[..., -1] = top
- heights = cumheights[..., 1:] - cumheights[..., :-1]
-
- if inverse:
- bin_idx = searchsorted(cumheights, inputs)[..., None]
- else:
- bin_idx = searchsorted(cumwidths, inputs)[..., None]
-
- input_cumwidths = cumwidths.gather(-1, bin_idx)[..., 0]
- input_bin_widths = widths.gather(-1, bin_idx)[..., 0]
-
- input_cumheights = cumheights.gather(-1, bin_idx)[..., 0]
- delta = heights / widths
- input_delta = delta.gather(-1, bin_idx)[..., 0]
-
- input_derivatives = derivatives.gather(-1, bin_idx)[..., 0]
- input_derivatives_plus_one = derivatives[..., 1:].gather(-1, bin_idx)[..., 0]
-
- input_heights = heights.gather(-1, bin_idx)[..., 0]
-
- if inverse:
- a = (((inputs - input_cumheights) * (input_derivatives
- + input_derivatives_plus_one
- - 2 * input_delta)
- + input_heights * (input_delta - input_derivatives)))
- b = (input_heights * input_derivatives
- - (inputs - input_cumheights) * (input_derivatives
- + input_derivatives_plus_one
- - 2 * input_delta))
- c = - input_delta * (inputs - input_cumheights)
-
- discriminant = b.pow(2) - 4 * a * c
- assert (discriminant >= 0).all()
-
- root = (2 * c) / (-b - torch.sqrt(discriminant))
- outputs = root * input_bin_widths + input_cumwidths
-
- theta_one_minus_theta = root * (1 - root)
- denominator = input_delta + ((input_derivatives + input_derivatives_plus_one - 2 * input_delta)
- * theta_one_minus_theta)
- derivative_numerator = input_delta.pow(2) * (input_derivatives_plus_one * root.pow(2)
- + 2 * input_delta * theta_one_minus_theta
- + input_derivatives * (1 - root).pow(2))
- logabsdet = torch.log(derivative_numerator) - 2 * torch.log(denominator)
-
- return outputs, -logabsdet
- else:
- theta = (inputs - input_cumwidths) / input_bin_widths
- theta_one_minus_theta = theta * (1 - theta)
-
- numerator = input_heights * (input_delta * theta.pow(2)
- + input_derivatives * theta_one_minus_theta)
- denominator = input_delta + ((input_derivatives + input_derivatives_plus_one - 2 * input_delta)
- * theta_one_minus_theta)
- outputs = input_cumheights + numerator / denominator
-
- derivative_numerator = input_delta.pow(2) * (input_derivatives_plus_one * theta.pow(2)
- + 2 * input_delta * theta_one_minus_theta
- + input_derivatives * (1 - theta).pow(2))
- logabsdet = torch.log(derivative_numerator) - 2 * torch.log(denominator)
-
- return outputs, logabsdet
diff --git a/spaces/YeOldHermit/Super-Resolution-Anime-Diffusion/diffusers/schedulers/scheduling_k_dpm_2_ancestral_discrete.py b/spaces/YeOldHermit/Super-Resolution-Anime-Diffusion/diffusers/schedulers/scheduling_k_dpm_2_ancestral_discrete.py
deleted file mode 100644
index b7d2175f027a6e83d5b77824ea1edd309ae76128..0000000000000000000000000000000000000000
--- a/spaces/YeOldHermit/Super-Resolution-Anime-Diffusion/diffusers/schedulers/scheduling_k_dpm_2_ancestral_discrete.py
+++ /dev/null
@@ -1,324 +0,0 @@
-# Copyright 2022 Katherine Crowson, The HuggingFace Team and hlky. All rights reserved.
-#
-# Licensed under the Apache License, Version 2.0 (the "License");
-# you may not use this file except in compliance with the License.
-# You may obtain a copy of the License at
-#
-# http://www.apache.org/licenses/LICENSE-2.0
-#
-# Unless required by applicable law or agreed to in writing, software
-# distributed under the License is distributed on an "AS IS" BASIS,
-# WITHOUT WARRANTIES OR CONDITIONS OF ANY KIND, either express or implied.
-# See the License for the specific language governing permissions and
-# limitations under the License.
-
-from typing import List, Optional, Tuple, Union
-
-import numpy as np
-import torch
-
-from ..configuration_utils import ConfigMixin, register_to_config
-from ..utils import _COMPATIBLE_STABLE_DIFFUSION_SCHEDULERS
-from .scheduling_utils import SchedulerMixin, SchedulerOutput
-
-
-class KDPM2AncestralDiscreteScheduler(SchedulerMixin, ConfigMixin):
- """
- Scheduler created by @crowsonkb in [k_diffusion](https://github.com/crowsonkb/k-diffusion), see:
- https://github.com/crowsonkb/k-diffusion/blob/5b3af030dd83e0297272d861c19477735d0317ec/k_diffusion/sampling.py#L188
-
- Scheduler inspired by DPM-Solver-2 and Algorthim 2 from Karras et al. (2022).
-
- [`~ConfigMixin`] takes care of storing all config attributes that are passed in the scheduler's `__init__`
- function, such as `num_train_timesteps`. They can be accessed via `scheduler.config.num_train_timesteps`.
- [`SchedulerMixin`] provides general loading and saving functionality via the [`SchedulerMixin.save_pretrained`] and
- [`~SchedulerMixin.from_pretrained`] functions.
-
- Args:
- num_train_timesteps (`int`): number of diffusion steps used to train the model. beta_start (`float`): the
- starting `beta` value of inference. beta_end (`float`): the final `beta` value. beta_schedule (`str`):
- the beta schedule, a mapping from a beta range to a sequence of betas for stepping the model. Choose from
- `linear` or `scaled_linear`.
- trained_betas (`np.ndarray`, optional):
- option to pass an array of betas directly to the constructor to bypass `beta_start`, `beta_end` etc.
- options to clip the variance used when adding noise to the denoised sample. Choose from `fixed_small`,
- `fixed_small_log`, `fixed_large`, `fixed_large_log`, `learned` or `learned_range`.
- prediction_type (`str`, default `epsilon`, optional):
- prediction type of the scheduler function, one of `epsilon` (predicting the noise of the diffusion
- process), `sample` (directly predicting the noisy sample`) or `v_prediction` (see section 2.4
- https://imagen.research.google/video/paper.pdf)
- """
-
- _compatibles = _COMPATIBLE_STABLE_DIFFUSION_SCHEDULERS.copy()
- order = 2
-
- @register_to_config
- def __init__(
- self,
- num_train_timesteps: int = 1000,
- beta_start: float = 0.00085, # sensible defaults
- beta_end: float = 0.012,
- beta_schedule: str = "linear",
- trained_betas: Optional[Union[np.ndarray, List[float]]] = None,
- prediction_type: str = "epsilon",
- ):
- if trained_betas is not None:
- self.betas = torch.tensor(trained_betas, dtype=torch.float32)
- elif beta_schedule == "linear":
- self.betas = torch.linspace(beta_start, beta_end, num_train_timesteps, dtype=torch.float32)
- elif beta_schedule == "scaled_linear":
- # this schedule is very specific to the latent diffusion model.
- self.betas = (
- torch.linspace(beta_start**0.5, beta_end**0.5, num_train_timesteps, dtype=torch.float32) ** 2
- )
- else:
- raise NotImplementedError(f"{beta_schedule} does is not implemented for {self.__class__}")
-
- self.alphas = 1.0 - self.betas
- self.alphas_cumprod = torch.cumprod(self.alphas, dim=0)
-
- # set all values
- self.set_timesteps(num_train_timesteps, None, num_train_timesteps)
-
- def index_for_timestep(self, timestep):
- indices = (self.timesteps == timestep).nonzero()
- if self.state_in_first_order:
- pos = -1
- else:
- pos = 0
- return indices[pos].item()
-
- def scale_model_input(
- self,
- sample: torch.FloatTensor,
- timestep: Union[float, torch.FloatTensor],
- ) -> torch.FloatTensor:
- """
- Args:
- Ensures interchangeability with schedulers that need to scale the denoising model input depending on the
- current timestep.
- sample (`torch.FloatTensor`): input sample timestep (`int`, optional): current timestep
- Returns:
- `torch.FloatTensor`: scaled input sample
- """
- step_index = self.index_for_timestep(timestep)
-
- if self.state_in_first_order:
- sigma = self.sigmas[step_index]
- else:
- sigma = self.sigmas_interpol[step_index - 1]
-
- sample = sample / ((sigma**2 + 1) ** 0.5)
- return sample
-
- def set_timesteps(
- self,
- num_inference_steps: int,
- device: Union[str, torch.device] = None,
- num_train_timesteps: Optional[int] = None,
- ):
- """
- Sets the timesteps used for the diffusion chain. Supporting function to be run before inference.
-
- Args:
- num_inference_steps (`int`):
- the number of diffusion steps used when generating samples with a pre-trained model.
- device (`str` or `torch.device`, optional):
- the device to which the timesteps should be moved to. If `None`, the timesteps are not moved.
- """
- self.num_inference_steps = num_inference_steps
-
- num_train_timesteps = num_train_timesteps or self.config.num_train_timesteps
-
- timesteps = np.linspace(0, num_train_timesteps - 1, num_inference_steps, dtype=float)[::-1].copy()
-
- sigmas = np.array(((1 - self.alphas_cumprod) / self.alphas_cumprod) ** 0.5)
- self.log_sigmas = torch.from_numpy(np.log(sigmas)).to(device)
-
- sigmas = np.interp(timesteps, np.arange(0, len(sigmas)), sigmas)
- sigmas = np.concatenate([sigmas, [0.0]]).astype(np.float32)
- sigmas = torch.from_numpy(sigmas).to(device=device)
-
- # compute up and down sigmas
- sigmas_next = sigmas.roll(-1)
- sigmas_next[-1] = 0.0
- sigmas_up = (sigmas_next**2 * (sigmas**2 - sigmas_next**2) / sigmas**2) ** 0.5
- sigmas_down = (sigmas_next**2 - sigmas_up**2) ** 0.5
- sigmas_down[-1] = 0.0
-
- # compute interpolated sigmas
- sigmas_interpol = sigmas.log().lerp(sigmas_down.log(), 0.5).exp()
- sigmas_interpol[-2:] = 0.0
-
- # set sigmas
- self.sigmas = torch.cat([sigmas[:1], sigmas[1:].repeat_interleave(2), sigmas[-1:]])
- self.sigmas_interpol = torch.cat(
- [sigmas_interpol[:1], sigmas_interpol[1:].repeat_interleave(2), sigmas_interpol[-1:]]
- )
- self.sigmas_up = torch.cat([sigmas_up[:1], sigmas_up[1:].repeat_interleave(2), sigmas_up[-1:]])
- self.sigmas_down = torch.cat([sigmas_down[:1], sigmas_down[1:].repeat_interleave(2), sigmas_down[-1:]])
-
- # standard deviation of the initial noise distribution
- self.init_noise_sigma = self.sigmas.max()
-
- timesteps = torch.from_numpy(timesteps).to(device)
- timesteps_interpol = self.sigma_to_t(sigmas_interpol).to(device)
- interleaved_timesteps = torch.stack((timesteps_interpol[:-2, None], timesteps[1:, None]), dim=-1).flatten()
- timesteps = torch.cat([timesteps[:1], interleaved_timesteps])
-
- if str(device).startswith("mps"):
- # mps does not support float64
- self.timesteps = timesteps.to(device, dtype=torch.float32)
- else:
- self.timesteps = timesteps
-
- self.sample = None
-
- def sigma_to_t(self, sigma):
- # get log sigma
- log_sigma = sigma.log()
-
- # get distribution
- dists = log_sigma - self.log_sigmas[:, None]
-
- # get sigmas range
- low_idx = dists.ge(0).cumsum(dim=0).argmax(dim=0).clamp(max=self.log_sigmas.shape[0] - 2)
- high_idx = low_idx + 1
-
- low = self.log_sigmas[low_idx]
- high = self.log_sigmas[high_idx]
-
- # interpolate sigmas
- w = (low - log_sigma) / (low - high)
- w = w.clamp(0, 1)
-
- # transform interpolation to time range
- t = (1 - w) * low_idx + w * high_idx
- t = t.view(sigma.shape)
- return t
-
- @property
- def state_in_first_order(self):
- return self.sample is None
-
- def step(
- self,
- model_output: Union[torch.FloatTensor, np.ndarray],
- timestep: Union[float, torch.FloatTensor],
- sample: Union[torch.FloatTensor, np.ndarray],
- generator: Optional[torch.Generator] = None,
- return_dict: bool = True,
- ) -> Union[SchedulerOutput, Tuple]:
- """
- Args:
- Predict the sample at the previous timestep by reversing the SDE. Core function to propagate the diffusion
- process from the learned model outputs (most often the predicted noise).
- model_output (`torch.FloatTensor` or `np.ndarray`): direct output from learned diffusion model. timestep
- (`int`): current discrete timestep in the diffusion chain. sample (`torch.FloatTensor` or `np.ndarray`):
- current instance of sample being created by diffusion process.
- return_dict (`bool`): option for returning tuple rather than SchedulerOutput class
- Returns:
- [`~schedulers.scheduling_utils.SchedulerOutput`] or `tuple`:
- [`~schedulers.scheduling_utils.SchedulerOutput`] if `return_dict` is True, otherwise a `tuple`. When
- returning a tuple, the first element is the sample tensor.
- """
- step_index = self.index_for_timestep(timestep)
-
- if self.state_in_first_order:
- sigma = self.sigmas[step_index]
- sigma_interpol = self.sigmas_interpol[step_index]
- sigma_up = self.sigmas_up[step_index]
- sigma_down = self.sigmas_down[step_index - 1]
- else:
- # 2nd order / KPDM2's method
- sigma = self.sigmas[step_index - 1]
- sigma_interpol = self.sigmas_interpol[step_index - 1]
- sigma_up = self.sigmas_up[step_index - 1]
- sigma_down = self.sigmas_down[step_index - 1]
-
- # currently only gamma=0 is supported. This usually works best anyways.
- # We can support gamma in the future but then need to scale the timestep before
- # passing it to the model which requires a change in API
- gamma = 0
- sigma_hat = sigma * (gamma + 1) # Note: sigma_hat == sigma for now
-
- device = model_output.device
- if device.type == "mps":
- # randn does not work reproducibly on mps
- noise = torch.randn(model_output.shape, dtype=model_output.dtype, device="cpu", generator=generator).to(
- device
- )
- else:
- noise = torch.randn(model_output.shape, dtype=model_output.dtype, device=device, generator=generator).to(
- device
- )
-
- # 1. compute predicted original sample (x_0) from sigma-scaled predicted noise
- if self.config.prediction_type == "epsilon":
- sigma_input = sigma_hat if self.state_in_first_order else sigma_interpol
- pred_original_sample = sample - sigma_input * model_output
- elif self.config.prediction_type == "v_prediction":
- sigma_input = sigma_hat if self.state_in_first_order else sigma_interpol
- pred_original_sample = model_output * (-sigma_input / (sigma_input**2 + 1) ** 0.5) + (
- sample / (sigma_input**2 + 1)
- )
- else:
- raise ValueError(
- f"prediction_type given as {self.config.prediction_type} must be one of `epsilon`, or `v_prediction`"
- )
-
- if self.state_in_first_order:
- # 2. Convert to an ODE derivative for 1st order
- derivative = (sample - pred_original_sample) / sigma_hat
- # 3. delta timestep
- dt = sigma_interpol - sigma_hat
-
- # store for 2nd order step
- self.sample = sample
- self.dt = dt
- prev_sample = sample + derivative * dt
- else:
- # DPM-Solver-2
- # 2. Convert to an ODE derivative for 2nd order
- derivative = (sample - pred_original_sample) / sigma_interpol
- # 3. delta timestep
- dt = sigma_down - sigma_hat
-
- sample = self.sample
- self.sample = None
-
- prev_sample = sample + derivative * dt
- prev_sample = prev_sample + noise * sigma_up
-
- if not return_dict:
- return (prev_sample,)
-
- return SchedulerOutput(prev_sample=prev_sample)
-
- def add_noise(
- self,
- original_samples: torch.FloatTensor,
- noise: torch.FloatTensor,
- timesteps: torch.FloatTensor,
- ) -> torch.FloatTensor:
- # Make sure sigmas and timesteps have the same device and dtype as original_samples
- self.sigmas = self.sigmas.to(device=original_samples.device, dtype=original_samples.dtype)
- if original_samples.device.type == "mps" and torch.is_floating_point(timesteps):
- # mps does not support float64
- self.timesteps = self.timesteps.to(original_samples.device, dtype=torch.float32)
- timesteps = timesteps.to(original_samples.device, dtype=torch.float32)
- else:
- self.timesteps = self.timesteps.to(original_samples.device)
- timesteps = timesteps.to(original_samples.device)
-
- step_indices = [self.index_for_timestep(t) for t in timesteps]
-
- sigma = self.sigmas[step_indices].flatten()
- while len(sigma.shape) < len(original_samples.shape):
- sigma = sigma.unsqueeze(-1)
-
- noisy_samples = original_samples + noise * sigma
- return noisy_samples
-
- def __len__(self):
- return self.config.num_train_timesteps
diff --git a/spaces/YouLiXiya/Mobile-SAM/GroundingDINO/groundingdino/models/GroundingDINO/csrc/MsDeformAttn/ms_deform_attn_cuda.h b/spaces/YouLiXiya/Mobile-SAM/GroundingDINO/groundingdino/models/GroundingDINO/csrc/MsDeformAttn/ms_deform_attn_cuda.h
deleted file mode 100644
index ad1311a78f61303616504eb991aaa9c4a93d9948..0000000000000000000000000000000000000000
--- a/spaces/YouLiXiya/Mobile-SAM/GroundingDINO/groundingdino/models/GroundingDINO/csrc/MsDeformAttn/ms_deform_attn_cuda.h
+++ /dev/null
@@ -1,33 +0,0 @@
-/*!
-**************************************************************************************************
-* Deformable DETR
-* Copyright (c) 2020 SenseTime. All Rights Reserved.
-* Licensed under the Apache License, Version 2.0 [see LICENSE for details]
-**************************************************************************************************
-* Modified from https://github.com/chengdazhi/Deformable-Convolution-V2-PyTorch/tree/pytorch_1.0.0
-**************************************************************************************************
-*/
-
-#pragma once
-#include
-
-namespace groundingdino {
-
-at::Tensor ms_deform_attn_cuda_forward(
- const at::Tensor &value,
- const at::Tensor &spatial_shapes,
- const at::Tensor &level_start_index,
- const at::Tensor &sampling_loc,
- const at::Tensor &attn_weight,
- const int im2col_step);
-
-std::vector ms_deform_attn_cuda_backward(
- const at::Tensor &value,
- const at::Tensor &spatial_shapes,
- const at::Tensor &level_start_index,
- const at::Tensor &sampling_loc,
- const at::Tensor &attn_weight,
- const at::Tensor &grad_output,
- const int im2col_step);
-
-} // namespace groundingdino
\ No newline at end of file
diff --git a/spaces/Yudha515/Rvc-Models/audiocraft/utils/utils.py b/spaces/Yudha515/Rvc-Models/audiocraft/utils/utils.py
deleted file mode 100644
index 86e1448d065fa182ca69aae00d2f2a7eea55d8a4..0000000000000000000000000000000000000000
--- a/spaces/Yudha515/Rvc-Models/audiocraft/utils/utils.py
+++ /dev/null
@@ -1,234 +0,0 @@
-# Copyright (c) Meta Platforms, Inc. and affiliates.
-# All rights reserved.
-#
-# This source code is licensed under the license found in the
-# LICENSE file in the root directory of this source tree.
-
-from concurrent.futures import ProcessPoolExecutor
-from functools import wraps
-import hashlib
-import logging
-import typing as tp
-
-import flashy
-import flashy.distrib
-import omegaconf
-import torch
-from torch.nn.utils.rnn import pad_sequence
-
-
-logger = logging.getLogger(__name__)
-
-
-def dict_from_config(cfg: omegaconf.DictConfig) -> dict:
- """Convenience function to map an omegaconf configuration to a dictionary.
-
- Args:
- cfg (omegaconf.DictConfig): Original configuration to map to dict.
- Returns:
- dict: Config as dictionary object.
- """
- dct = omegaconf.OmegaConf.to_container(cfg, resolve=True)
- assert isinstance(dct, dict)
- return dct
-
-
-def random_subset(dataset, max_samples: int, seed: int = 42) -> torch.utils.data.Subset:
- if max_samples >= len(dataset):
- return dataset
-
- generator = torch.Generator().manual_seed(seed)
- perm = torch.randperm(len(dataset), generator=generator)
- return torch.utils.data.Subset(dataset, perm[:max_samples].tolist())
-
-
-def get_loader(dataset, num_samples: tp.Optional[int], batch_size: int,
- num_workers: int, seed: int, **kwargs) -> torch.utils.data.DataLoader:
- """Convenience function to load dataset into a dataloader with optional subset sampling.
-
- Args:
- dataset: Dataset to load.
- num_samples (Optional[int]): Number of samples to limit subset size.
- batch_size (int): Batch size.
- num_workers (int): Number of workers for data loading.
- seed (int): Random seed.
- """
- if num_samples is not None:
- dataset = random_subset(dataset, num_samples, seed)
-
- dataloader = flashy.distrib.loader(
- dataset,
- batch_size=batch_size,
- num_workers=num_workers,
- **kwargs
- )
- return dataloader
-
-
-def get_dataset_from_loader(dataloader):
- dataset = dataloader.dataset
- if isinstance(dataset, torch.utils.data.Subset):
- return dataset.dataset
- else:
- return dataset
-
-
-def multinomial(input: torch.Tensor, num_samples: int, replacement=False, *, generator=None):
- """torch.multinomial with arbitrary number of dimensions, and number of candidates on the last dimension.
-
- Args:
- input (torch.Tensor): The input tensor containing probabilities.
- num_samples (int): Number of samples to draw.
- replacement (bool): Whether to draw with replacement or not.
- Keywords args:
- generator (torch.Generator): A pseudorandom number generator for sampling.
- Returns:
- torch.Tensor: Last dimension contains num_samples indices
- sampled from the multinomial probability distribution
- located in the last dimension of tensor input.
- """
- input_ = input.reshape(-1, input.shape[-1])
- output_ = torch.multinomial(input_, num_samples=num_samples, replacement=replacement, generator=generator)
- output = output_.reshape(*list(input.shape[:-1]), -1)
- return output
-
-
-def sample_top_k(probs: torch.Tensor, k: int) -> torch.Tensor:
- """Sample next token from top K values along the last dimension of the input probs tensor.
-
- Args:
- probs (torch.Tensor): Input probabilities with token candidates on the last dimension.
- k (int): The k in “top-k”.
- Returns:
- torch.Tensor: Sampled tokens.
- """
- top_k_value, _ = torch.topk(probs, k, dim=-1)
- min_value_top_k = top_k_value[..., [-1]]
- probs *= (probs >= min_value_top_k).float()
- probs.div_(probs.sum(dim=-1, keepdim=True))
- next_token = multinomial(probs, num_samples=1)
- return next_token
-
-
-def sample_top_p(probs: torch.Tensor, p: float) -> torch.Tensor:
- """Sample next token from top P probabilities along the last dimension of the input probs tensor.
-
- Args:
- probs (torch.Tensor): Input probabilities with token candidates on the last dimension.
- p (int): The p in “top-p”.
- Returns:
- torch.Tensor: Sampled tokens.
- """
- probs_sort, probs_idx = torch.sort(probs, dim=-1, descending=True)
- probs_sum = torch.cumsum(probs_sort, dim=-1)
- mask = probs_sum - probs_sort > p
- probs_sort *= (~mask).float()
- probs_sort.div_(probs_sort.sum(dim=-1, keepdim=True))
- next_token = multinomial(probs_sort, num_samples=1)
- next_token = torch.gather(probs_idx, -1, next_token)
- return next_token
-
-
-class DummyPoolExecutor:
- """Dummy pool executor to use when we actually have only 1 worker.
- (e.g. instead of ProcessPoolExecutor).
- """
- class DummyResult:
- def __init__(self, func, *args, **kwargs):
- self.func = func
- self.args = args
- self.kwargs = kwargs
-
- def result(self):
- return self.func(*self.args, **self.kwargs)
-
- def __init__(self, workers, mp_context=None):
- pass
-
- def submit(self, func, *args, **kwargs):
- return DummyPoolExecutor.DummyResult(func, *args, **kwargs)
-
- def __enter__(self):
- return self
-
- def __exit__(self, exc_type, exc_value, exc_tb):
- return
-
-
-def get_pool_executor(num_workers: int, mp_context=None):
- return ProcessPoolExecutor(num_workers, mp_context) if num_workers > 1 else DummyPoolExecutor(1)
-
-
-def length_to_mask(lengths: torch.Tensor, max_len: tp.Optional[int] = None) -> torch.Tensor:
- """Utility function to convert a tensor of sequence lengths to a mask (useful when working on padded sequences).
- For example: [3, 5] => [[1, 1, 1, 0, 0], [1, 1, 1, 1, 1]]
-
- Args:
- lengths (torch.Tensor): tensor with lengths
- max_len (int): can set the max length manually. Defaults to None.
- Returns:
- torch.Tensor: mask with 0s where there is pad tokens else 1s
- """
- assert len(lengths.shape) == 1, "Length shape should be 1 dimensional."
- final_length = lengths.max().item() if not max_len else max_len
- final_length = max(final_length, 1) # if all seqs are of len zero we don't want a zero-size tensor
- return torch.arange(final_length)[None, :].to(lengths.device) < lengths[:, None]
-
-
-def hash_trick(word: str, vocab_size: int) -> int:
- """Hash trick to pair each word with an index
-
- Args:
- word (str): word we wish to convert to an index
- vocab_size (int): size of the vocabulary
- Returns:
- int: index of the word in the embedding LUT
- """
- hash = int(hashlib.sha256(word.encode("utf-8")).hexdigest(), 16)
- return hash % vocab_size
-
-
-def with_rank_rng(base_seed: int = 1234):
- """Decorator for a function so that the function will use a Random Number Generator
- whose state depend on the GPU rank. The original RNG state is restored upon returning.
-
- Args:
- base_seed (int): Random seed.
- """
- def _decorator(fun: tp.Callable):
- @wraps(fun)
- def _decorated(*args, **kwargs):
- state = torch.get_rng_state()
- seed = base_seed ^ flashy.distrib.rank()
- torch.manual_seed(seed)
- logger.debug('Rank dependent seed set to %d', seed)
- try:
- return fun(*args, **kwargs)
- finally:
- torch.set_rng_state(state)
- logger.debug('RNG state restored.')
- return _decorated
- return _decorator
-
-
-def collate(tensors: tp.List[torch.Tensor], dim: int = 0) -> tp.Tuple[torch.Tensor, torch.Tensor]:
- """Get a list of tensors and collate them to a single tensor. according to the following logic:
- - `dim` specifies the time dimension which will be stacked and padded.
- - The output will contain 1 new dimension (dimension index 0) which will be the size of
- of the original list.
-
- Args:
- tensors (tp.List[torch.Tensor]): List of tensors to collate.
- dim (int): Dimension which will be stacked and padded.
- Returns:
- tp.Tuple[torch.Tensor, torch.Tensor]:
- torch.Tensor: Stacked and padded tensor. The output will contain 1 new dimension
- (dimension index 0) which will be the size of the original list.
- torch.Tensor: Tensor containing length of original tensor sizes (without padding).
- """
- tensors = [x.transpose(0, dim) for x in tensors]
- lens = torch.LongTensor([len(x) for x in tensors])
- padded_tensors = pad_sequence(tensors)
- padded_tensors = padded_tensors.transpose(0, 1)
- padded_tensors = padded_tensors.transpose(1, dim + 1)
- return padded_tensors, lens
diff --git a/spaces/Yuliang/ECON/lib/pymafx/utils/renderer.py b/spaces/Yuliang/ECON/lib/pymafx/utils/renderer.py
deleted file mode 100644
index 5b201badc2c6da232d0d753c9303b7c62361ca7f..0000000000000000000000000000000000000000
--- a/spaces/Yuliang/ECON/lib/pymafx/utils/renderer.py
+++ /dev/null
@@ -1,656 +0,0 @@
-import imp
-import json
-import os
-from pickle import NONE
-
-import numpy as np
-# os.environ['PYOPENGL_PLATFORM'] = 'osmesa'
-import torch
-import torch.nn.functional as F
-import trimesh
-from core import constants, path_config
-from models.smpl import get_model_faces, get_model_tpose, get_smpl_faces
-# import neural_renderer as nr
-from skimage.transform import resize
-from torchvision.utils import make_grid
-from utils.densepose_methods import DensePoseMethods
-from utils.imutils import crop
-
-from .geometry import convert_to_full_img_cam
-
-try:
- import math
-
- import pyrender
- from pyrender.constants import RenderFlags
-except:
- pass
-try:
- from opendr.camera import ProjectPoints
- from opendr.lighting import LambertianPointLight, SphericalHarmonics
- from opendr.renderer import ColoredRenderer
-except:
- pass
-
-import logging
-
-from pytorch3d.renderer import (
- AmbientLights,
- BlendParams,
- FoVPerspectiveCameras,
- HardFlatShader,
- HardGouraudShader,
- HardPhongShader,
- MeshRasterizer,
- MeshRenderer,
- PerspectiveCameras,
- PointLights,
- RasterizationSettings,
- SoftPhongShader,
- SoftSilhouetteShader,
- TexturesVertex,
- look_at_view_transform,
-)
-from pytorch3d.structures.meshes import Meshes
-
-# from pytorch3d.renderer.mesh.renderer import MeshRendererWithFragments
-
-logger = logging.getLogger(__name__)
-
-
-class WeakPerspectiveCamera(pyrender.Camera):
- def __init__(
- self, scale, translation, znear=pyrender.camera.DEFAULT_Z_NEAR, zfar=None, name=None
- ):
- super(WeakPerspectiveCamera, self).__init__(
- znear=znear,
- zfar=zfar,
- name=name,
- )
- self.scale = scale
- self.translation = translation
-
- def get_projection_matrix(self, width=None, height=None):
- P = np.eye(4)
- P[0, 0] = self.scale[0]
- P[1, 1] = self.scale[1]
- P[0, 3] = self.translation[0] * self.scale[0]
- P[1, 3] = -self.translation[1] * self.scale[1]
- P[2, 2] = -1
- return P
-
-
-class PyRenderer:
- def __init__(
- self, resolution=(224, 224), orig_img=False, wireframe=False, scale_ratio=1., vis_ratio=1.
- ):
- self.resolution = (resolution[0] * scale_ratio, resolution[1] * scale_ratio)
- # self.scale_ratio = scale_ratio
-
- self.faces = {
- 'smplx': get_model_faces('smplx'),
- 'smpl': get_model_faces('smpl'),
- # 'mano': get_model_faces('mano'),
- # 'flame': get_model_faces('flame'),
- }
- self.orig_img = orig_img
- self.wireframe = wireframe
- self.renderer = pyrender.OffscreenRenderer(
- viewport_width=self.resolution[0], viewport_height=self.resolution[1], point_size=1.0
- )
-
- self.vis_ratio = vis_ratio
-
- # set the scene
- self.scene = pyrender.Scene(bg_color=[0.0, 0.0, 0.0, 0.0], ambient_light=(0.3, 0.3, 0.3))
-
- light = pyrender.PointLight(color=np.array([1.0, 1.0, 1.0]) * 0.2, intensity=1)
-
- yrot = np.radians(120) # angle of lights
-
- light_pose = np.eye(4)
- light_pose[:3, 3] = [0, -1, 1]
- self.scene.add(light, pose=light_pose)
-
- light_pose[:3, 3] = [0, 1, 1]
- self.scene.add(light, pose=light_pose)
-
- light_pose[:3, 3] = [1, 1, 2]
- self.scene.add(light, pose=light_pose)
-
- spot_l = pyrender.SpotLight(
- color=np.ones(3), intensity=15.0, innerConeAngle=np.pi / 3, outerConeAngle=np.pi / 2
- )
-
- light_pose[:3, 3] = [1, 2, 2]
- self.scene.add(spot_l, pose=light_pose)
-
- light_pose[:3, 3] = [-1, 2, 2]
- self.scene.add(spot_l, pose=light_pose)
-
- # light_pose[:3, 3] = [-2, 2, 0]
- # self.scene.add(spot_l, pose=light_pose)
-
- # light_pose[:3, 3] = [-2, 2, 0]
- # self.scene.add(spot_l, pose=light_pose)
-
- self.colors_dict = {
- 'red': np.array([0.5, 0.2, 0.2]),
- 'pink': np.array([0.7, 0.5, 0.5]),
- 'neutral': np.array([0.7, 0.7, 0.6]),
- # 'purple': np.array([0.5, 0.5, 0.7]),
- 'purple': np.array([0.55, 0.4, 0.9]),
- 'green': np.array([0.5, 0.55, 0.3]),
- 'sky': np.array([0.3, 0.5, 0.55]),
- 'white': np.array([1.0, 0.98, 0.94]),
- }
-
- def __call__(
- self,
- verts,
- faces=None,
- img=np.zeros((224, 224, 3)),
- cam=np.array([1, 0, 0]),
- focal_length=[5000, 5000],
- camera_rotation=np.eye(3),
- crop_info=None,
- angle=None,
- axis=None,
- mesh_filename=None,
- color_type=None,
- color=[1.0, 1.0, 0.9],
- iwp_mode=True,
- crop_img=True,
- mesh_type='smpl',
- scale_ratio=1.,
- rgba_mode=False
- ):
-
- if faces is None:
- faces = self.faces[mesh_type]
- mesh = trimesh.Trimesh(vertices=verts, faces=faces, process=False)
-
- Rx = trimesh.transformations.rotation_matrix(math.radians(180), [1, 0, 0])
- mesh.apply_transform(Rx)
-
- if mesh_filename is not None:
- mesh.export(mesh_filename)
-
- if angle and axis:
- R = trimesh.transformations.rotation_matrix(math.radians(angle), axis)
- mesh.apply_transform(R)
-
- cam = cam.copy()
- if iwp_mode:
- resolution = np.array(img.shape[:2]) * scale_ratio
- if len(cam) == 4:
- sx, sy, tx, ty = cam
- # sy = sx
- camera_translation = np.array([
- tx, ty, 2 * focal_length[0] / (resolution[0] * sy + 1e-9)
- ])
- elif len(cam) == 3:
- sx, tx, ty = cam
- sy = sx
- camera_translation = np.array([
- -tx, ty, 2 * focal_length[0] / (resolution[0] * sy + 1e-9)
- ])
- render_res = resolution
- self.renderer.viewport_width = render_res[1]
- self.renderer.viewport_height = render_res[0]
- else:
- if crop_info['opt_cam_t'] is None:
- camera_translation = convert_to_full_img_cam(
- pare_cam=cam[None],
- bbox_height=crop_info['bbox_scale'] * 200.,
- bbox_center=crop_info['bbox_center'],
- img_w=crop_info['img_w'],
- img_h=crop_info['img_h'],
- focal_length=focal_length[0],
- )
- else:
- camera_translation = crop_info['opt_cam_t']
- if torch.is_tensor(camera_translation):
- camera_translation = camera_translation[0].cpu().numpy()
- camera_translation = camera_translation.copy()
- camera_translation[0] *= -1
- if 'img_h' in crop_info and 'img_w' in crop_info:
- render_res = (int(crop_info['img_h'][0]), int(crop_info['img_w'][0]))
- else:
- render_res = img.shape[:2] if type(img) is not list else img[0].shape[:2]
- self.renderer.viewport_width = render_res[1]
- self.renderer.viewport_height = render_res[0]
- camera_rotation = camera_rotation.T
- camera = pyrender.IntrinsicsCamera(
- fx=focal_length[0], fy=focal_length[1], cx=render_res[1] / 2., cy=render_res[0] / 2.
- )
-
- if color_type != None:
- color = self.colors_dict[color_type]
-
- material = pyrender.MetallicRoughnessMaterial(
- metallicFactor=0.2,
- roughnessFactor=0.6,
- alphaMode='OPAQUE',
- baseColorFactor=(color[0], color[1], color[2], 1.0)
- )
-
- mesh = pyrender.Mesh.from_trimesh(mesh, material=material)
-
- mesh_node = self.scene.add(mesh, 'mesh')
-
- camera_pose = np.eye(4)
- camera_pose[:3, :3] = camera_rotation
- camera_pose[:3, 3] = camera_rotation @ camera_translation
- cam_node = self.scene.add(camera, pose=camera_pose)
-
- if self.wireframe:
- render_flags = RenderFlags.RGBA | RenderFlags.ALL_WIREFRAME | RenderFlags.SHADOWS_SPOT
- else:
- render_flags = RenderFlags.RGBA | RenderFlags.SHADOWS_SPOT
-
- rgb, _ = self.renderer.render(self.scene, flags=render_flags)
- if crop_info is not None and crop_img:
- crop_res = img.shape[:2]
- rgb, _, _ = crop(rgb, crop_info['bbox_center'][0], crop_info['bbox_scale'][0], crop_res)
-
- valid_mask = (rgb[:, :, -1] > 0)[:, :, np.newaxis]
-
- image_list = [img] if type(img) is not list else img
-
- return_img = []
- for item in image_list:
- if scale_ratio != 1:
- orig_size = item.shape[:2]
- item = resize(
- item, (orig_size[0] * scale_ratio, orig_size[1] * scale_ratio),
- anti_aliasing=True
- )
- item = (item * 255).astype(np.uint8)
- output_img = rgb[:, :, :-1] * valid_mask * self.vis_ratio + (
- 1 - valid_mask * self.vis_ratio
- ) * item
- # output_img[valid_mask < 0.5] = item[valid_mask < 0.5]
- # if scale_ratio != 1:
- # output_img = resize(output_img, (orig_size[0], orig_size[1]), anti_aliasing=True)
- if rgba_mode:
- output_img_rgba = np.zeros((output_img.shape[0], output_img.shape[1], 4))
- output_img_rgba[:, :, :3] = output_img
- output_img_rgba[:, :, 3][valid_mask[:, :, 0]] = 255
- output_img = output_img_rgba.astype(np.uint8)
- image = output_img.astype(np.uint8)
- return_img.append(image)
- return_img.append(item)
-
- if type(img) is not list:
- # if scale_ratio == 1:
- return_img = return_img[0]
-
- self.scene.remove_node(mesh_node)
- self.scene.remove_node(cam_node)
-
- return return_img
-
-
-class OpenDRenderer:
- def __init__(self, resolution=(224, 224), ratio=1):
- self.resolution = (resolution[0] * ratio, resolution[1] * ratio)
- self.ratio = ratio
- self.focal_length = 5000.
- self.K = np.array([[self.focal_length, 0., self.resolution[1] / 2.],
- [0., self.focal_length, self.resolution[0] / 2.], [0., 0., 1.]])
- self.colors_dict = {
- 'red': np.array([0.5, 0.2, 0.2]),
- 'pink': np.array([0.7, 0.5, 0.5]),
- 'neutral': np.array([0.7, 0.7, 0.6]),
- 'purple': np.array([0.5, 0.5, 0.7]),
- 'green': np.array([0.5, 0.55, 0.3]),
- 'sky': np.array([0.3, 0.5, 0.55]),
- 'white': np.array([1.0, 0.98, 0.94]),
- }
- self.renderer = ColoredRenderer()
- self.faces = get_smpl_faces()
-
- def reset_res(self, resolution):
- self.resolution = (resolution[0] * self.ratio, resolution[1] * self.ratio)
- self.K = np.array([[self.focal_length, 0., self.resolution[1] / 2.],
- [0., self.focal_length, self.resolution[0] / 2.], [0., 0., 1.]])
-
- def __call__(
- self,
- verts,
- faces=None,
- color=None,
- color_type='white',
- R=None,
- mesh_filename=None,
- img=np.zeros((224, 224, 3)),
- cam=np.array([1, 0, 0]),
- rgba=False,
- addlight=True
- ):
- '''Render mesh using OpenDR
- verts: shape - (V, 3)
- faces: shape - (F, 3)
- img: shape - (224, 224, 3), range - [0, 255] (np.uint8)
- axis: rotate along with X/Y/Z axis (by angle)
- R: rotation matrix (used to manipulate verts) shape - [3, 3]
- Return:
- rendered img: shape - (224, 224, 3), range - [0, 255] (np.uint8)
- '''
- ## Create OpenDR renderer
- rn = self.renderer
- h, w = self.resolution
- K = self.K
-
- f = np.array([K[0, 0], K[1, 1]])
- c = np.array([K[0, 2], K[1, 2]])
-
- if faces is None:
- faces = self.faces
- if len(cam) == 4:
- t = np.array([cam[2], cam[3], 2 * K[0, 0] / (w * cam[0] + 1e-9)])
- elif len(cam) == 3:
- t = np.array([cam[1], cam[2], 2 * K[0, 0] / (w * cam[0] + 1e-9)])
-
- rn.camera = ProjectPoints(rt=np.array([0, 0, 0]), t=t, f=f, c=c, k=np.zeros(5))
- rn.frustum = {'near': 1., 'far': 1000., 'width': w, 'height': h}
-
- albedo = np.ones_like(verts) * .9
-
- if color is not None:
- color0 = np.array(color)
- color1 = np.array(color)
- color2 = np.array(color)
- elif color_type == 'white':
- color0 = np.array([1., 1., 1.])
- color1 = np.array([1., 1., 1.])
- color2 = np.array([0.7, 0.7, 0.7])
- color = np.ones_like(verts) * self.colors_dict[color_type][None, :]
- else:
- color0 = self.colors_dict[color_type] * 1.2
- color1 = self.colors_dict[color_type] * 1.2
- color2 = self.colors_dict[color_type] * 1.2
- color = np.ones_like(verts) * self.colors_dict[color_type][None, :]
-
- # render_smpl = rn.r
- if R is not None:
- assert R.shape == (3, 3), "Shape of rotation matrix should be (3, 3)"
- verts = np.dot(verts, R)
-
- rn.set(v=verts, f=faces, vc=color, bgcolor=np.zeros(3))
-
- if addlight:
- yrot = np.radians(120) # angle of lights
- # # 1. 1. 0.7
- rn.vc = LambertianPointLight(
- f=rn.f,
- v=rn.v,
- num_verts=len(rn.v),
- light_pos=rotateY(np.array([-200, -100, -100]), yrot),
- vc=albedo,
- light_color=color0
- )
-
- # Construct Left Light
- rn.vc += LambertianPointLight(
- f=rn.f,
- v=rn.v,
- num_verts=len(rn.v),
- light_pos=rotateY(np.array([800, 10, 300]), yrot),
- vc=albedo,
- light_color=color1
- )
-
- # Construct Right Light
- rn.vc += LambertianPointLight(
- f=rn.f,
- v=rn.v,
- num_verts=len(rn.v),
- light_pos=rotateY(np.array([-500, 500, 1000]), yrot),
- vc=albedo,
- light_color=color2
- )
-
- rendered_image = rn.r
- visibility_image = rn.visibility_image
-
- image_list = [img] if type(img) is not list else img
-
- return_img = []
- for item in image_list:
- if self.ratio != 1:
- img_resized = resize(
- item, (item.shape[0] * self.ratio, item.shape[1] * self.ratio),
- anti_aliasing=True
- )
- else:
- img_resized = item / 255.
-
- try:
- img_resized[visibility_image != (2**32 - 1)
- ] = rendered_image[visibility_image != (2**32 - 1)]
- except:
- logger.warning('Can not render mesh.')
-
- img_resized = (img_resized * 255).astype(np.uint8)
- res = img_resized
-
- if rgba:
- img_resized_rgba = np.zeros((img_resized.shape[0], img_resized.shape[1], 4))
- img_resized_rgba[:, :, :3] = img_resized
- img_resized_rgba[:, :, 3][visibility_image != (2**32 - 1)] = 255
- res = img_resized_rgba.astype(np.uint8)
- return_img.append(res)
-
- if type(img) is not list:
- return_img = return_img[0]
-
- return return_img
-
-
-# https://github.com/classner/up/blob/master/up_tools/camera.py
-def rotateY(points, angle):
- """Rotate all points in a 2D array around the y axis."""
- ry = np.array([[np.cos(angle), 0., np.sin(angle)], [0., 1., 0.],
- [-np.sin(angle), 0., np.cos(angle)]])
- return np.dot(points, ry)
-
-
-def rotateX(points, angle):
- """Rotate all points in a 2D array around the x axis."""
- rx = np.array([[1., 0., 0.], [0., np.cos(angle), -np.sin(angle)],
- [0., np.sin(angle), np.cos(angle)]])
- return np.dot(points, rx)
-
-
-def rotateZ(points, angle):
- """Rotate all points in a 2D array around the z axis."""
- rz = np.array([[np.cos(angle), -np.sin(angle), 0.], [np.sin(angle),
- np.cos(angle), 0.], [0., 0., 1.]])
- return np.dot(points, rz)
-
-
-class IUV_Renderer(object):
- def __init__(
- self,
- focal_length=5000.,
- orig_size=224,
- output_size=56,
- mode='iuv',
- device=torch.device('cuda'),
- mesh_type='smpl'
- ):
-
- self.focal_length = focal_length
- self.orig_size = orig_size
- self.output_size = output_size
-
- if mode in ['iuv']:
- if mesh_type == 'smpl':
- DP = DensePoseMethods()
-
- vert_mapping = DP.All_vertices.astype('int64') - 1
- self.vert_mapping = torch.from_numpy(vert_mapping)
-
- faces = DP.FacesDensePose
- faces = faces[None, :, :]
- self.faces = torch.from_numpy(
- faces.astype(np.int32)
- ) # [1, 13774, 3], torch.int32
-
- num_part = float(np.max(DP.FaceIndices))
- self.num_part = num_part
-
- dp_vert_pid_fname = 'data/dp_vert_pid.npy'
- if os.path.exists(dp_vert_pid_fname):
- dp_vert_pid = list(np.load(dp_vert_pid_fname))
- else:
- print('creating data/dp_vert_pid.npy')
- dp_vert_pid = []
- for v in range(len(vert_mapping)):
- for i, f in enumerate(DP.FacesDensePose):
- if v in f:
- dp_vert_pid.append(DP.FaceIndices[i])
- break
- np.save(dp_vert_pid_fname, np.array(dp_vert_pid))
-
- textures_vts = np.array([(dp_vert_pid[i] / num_part, DP.U_norm[i], DP.V_norm[i])
- for i in range(len(vert_mapping))])
- self.textures_vts = torch.from_numpy(
- textures_vts[None].astype(np.float32)
- ) # (1, 7829, 3)
- elif mode == 'pncc':
- self.vert_mapping = None
- self.faces = torch.from_numpy(
- get_model_faces(mesh_type)[None].astype(np.int32)
- ) # mano: torch.Size([1, 1538, 3])
- textures_vts = get_model_tpose(mesh_type).unsqueeze(
- 0
- ) # mano: torch.Size([1, 778, 3])
-
- texture_min = torch.min(textures_vts) - 0.001
- texture_range = torch.max(textures_vts) - texture_min + 0.001
- self.textures_vts = (textures_vts - texture_min) / texture_range
- elif mode in ['seg']:
- self.vert_mapping = None
- body_model = 'smpl'
-
- self.faces = torch.from_numpy(get_smpl_faces().astype(np.int32)[None])
-
- with open(
- os.path.join(
- path_config.SMPL_MODEL_DIR, '{}_vert_segmentation.json'.format(body_model)
- ), 'rb'
- ) as json_file:
- smpl_part_id = json.load(json_file)
-
- v_id = []
- for k in smpl_part_id.keys():
- v_id.extend(smpl_part_id[k])
-
- v_id = torch.tensor(v_id)
- n_verts = len(torch.unique(v_id))
- num_part = len(constants.SMPL_PART_ID.keys())
- self.num_part = num_part
-
- seg_vert_pid = np.zeros(n_verts)
- for k in smpl_part_id.keys():
- seg_vert_pid[smpl_part_id[k]] = constants.SMPL_PART_ID[k]
-
- print('seg_vert_pid', seg_vert_pid.shape)
- textures_vts = seg_vert_pid[:, None].repeat(3, axis=1) / num_part
- print('textures_vts', textures_vts.shape)
- # textures_vts = np.array(
- # [(seg_vert_pid[i] / num_part,) * 3 for i in
- # range(n_verts)])
- self.textures_vts = torch.from_numpy(textures_vts[None].astype(np.float32))
-
- K = np.array([[self.focal_length, 0., self.orig_size / 2.],
- [0., self.focal_length, self.orig_size / 2.], [0., 0., 1.]])
-
- R = np.array([[-1., 0., 0.], [0., -1., 0.], [0., 0., 1.]])
-
- t = np.array([0, 0, 5])
-
- if self.orig_size != 224:
- rander_scale = self.orig_size / float(224)
- K[0, 0] *= rander_scale
- K[1, 1] *= rander_scale
- K[0, 2] *= rander_scale
- K[1, 2] *= rander_scale
-
- self.K = torch.FloatTensor(K[None, :, :])
- self.R = torch.FloatTensor(R[None, :, :])
- self.t = torch.FloatTensor(t[None, None, :])
-
- camK = F.pad(self.K, (0, 1, 0, 1), "constant", 0)
- camK[:, 2, 2] = 0
- camK[:, 3, 2] = 1
- camK[:, 2, 3] = 1
-
- self.K = camK
-
- self.device = device
- lights = AmbientLights(device=self.device)
-
- raster_settings = RasterizationSettings(
- image_size=output_size,
- blur_radius=0,
- faces_per_pixel=1,
- )
- self.renderer = MeshRenderer(
- rasterizer=MeshRasterizer(raster_settings=raster_settings),
- shader=HardFlatShader(
- device=self.device,
- lights=lights,
- blend_params=BlendParams(background_color=[0, 0, 0], sigma=0.0, gamma=0.0)
- )
- )
-
- def camera_matrix(self, cam):
- batch_size = cam.size(0)
-
- K = self.K.repeat(batch_size, 1, 1)
- R = self.R.repeat(batch_size, 1, 1)
- t = torch.stack([
- -cam[:, 1], -cam[:, 2], 2 * self.focal_length / (self.orig_size * cam[:, 0] + 1e-9)
- ],
- dim=-1)
-
- if cam.is_cuda:
- # device_id = cam.get_device()
- K = K.to(cam.device)
- R = R.to(cam.device)
- t = t.to(cam.device)
-
- return K, R, t
-
- def verts2iuvimg(self, verts, cam, iwp_mode=True):
- batch_size = verts.size(0)
-
- K, R, t = self.camera_matrix(cam)
-
- if self.vert_mapping is None:
- vertices = verts
- else:
- vertices = verts[:, self.vert_mapping, :]
-
- mesh = Meshes(vertices, self.faces.to(verts.device).expand(batch_size, -1, -1))
- mesh.textures = TexturesVertex(
- verts_features=self.textures_vts.to(verts.device).expand(batch_size, -1, -1)
- )
-
- cameras = PerspectiveCameras(
- device=verts.device,
- R=R,
- T=t,
- K=K,
- in_ndc=False,
- image_size=[(self.orig_size, self.orig_size)]
- )
-
- iuv_image = self.renderer(mesh, cameras=cameras)
- iuv_image = iuv_image[..., :3].permute(0, 3, 1, 2)
-
- return iuv_image
diff --git a/spaces/ZeroGPT/GPTZero/app.py b/spaces/ZeroGPT/GPTZero/app.py
deleted file mode 100644
index 6a124fb32f93614508653e4c0049e661fd2e6ce5..0000000000000000000000000000000000000000
--- a/spaces/ZeroGPT/GPTZero/app.py
+++ /dev/null
@@ -1,12 +0,0 @@
-import gradio as gr
-
-def say_hello():
- return "Welcome to ZeroGPT.cc. Check out our site for more details: https://zerogpt.cc"
-
-iface = gr.Interface(fn=say_hello,
- inputs=None,
- outputs="text",
- title="GPTZero Alternative - AI Content Detector - ZeroGPT.CC",
- description="ZeroGPT.cc: Use the Best Free AI Text Detector for Accurate Results
ZeroGPT.cc is a powerful platform that can help you identify whether a text was generated by AI tools such as Open AI ChatGPT, Google Bard, and Bing AI. This free tool leverages advanced language models and sophisticated algorithms to accurately detect and analyze content. With ZeroGPT, you can quickly and easily verify the authenticity of any text, giving you the confidence to use and share content that meets your high standards of quality and originality.
Check our website for more details: Zerogpt.cc
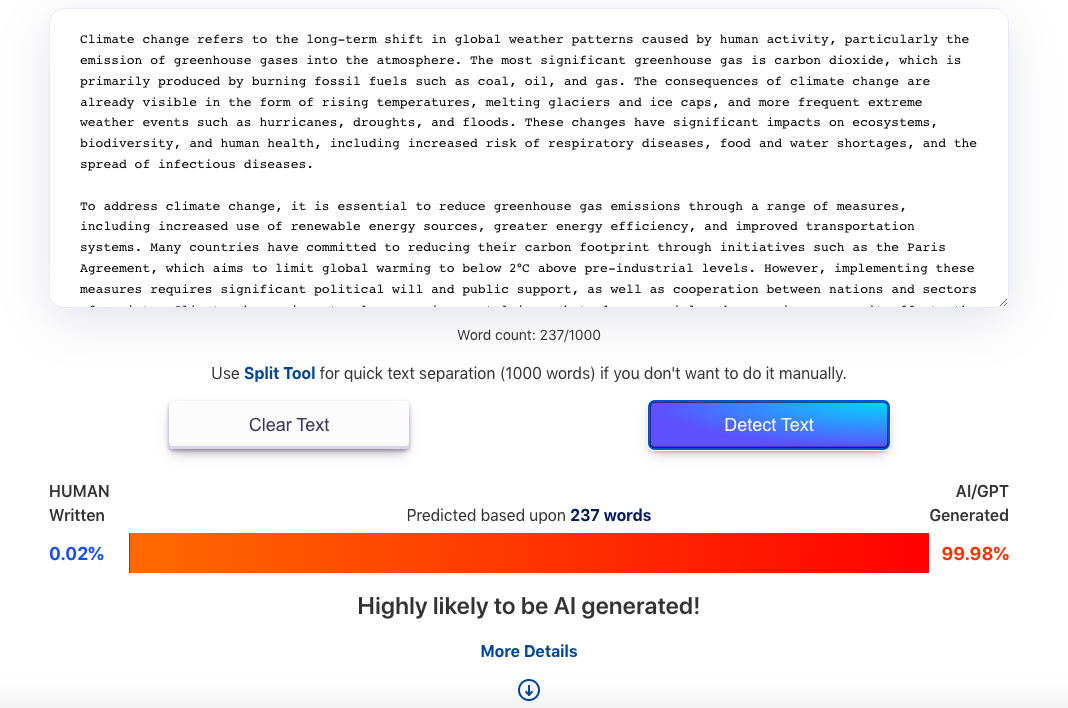
Why Should You Choose Our AI Text Detector - ZeroGPT.cc?
- Accurate Results
- Fast Results
- Easy to Operate
")
-
-iface.launch()
diff --git a/spaces/a-v-bely/spanish-task-generator/utilities_option_menu/frontend/dist/js/chunk-vendors.1b4b40f9.js b/spaces/a-v-bely/spanish-task-generator/utilities_option_menu/frontend/dist/js/chunk-vendors.1b4b40f9.js
deleted file mode 100644
index f6a646483aa5d4b157c13fdc05f258952522e127..0000000000000000000000000000000000000000
--- a/spaces/a-v-bely/spanish-task-generator/utilities_option_menu/frontend/dist/js/chunk-vendors.1b4b40f9.js
+++ /dev/null
@@ -1,72 +0,0 @@
-(window["webpackJsonp"]=window["webpackJsonp"]||[]).push([["chunk-vendors"],{"00ee":function(t,e,n){var r=n("b622"),i=r("toStringTag"),s={};s[i]="z",t.exports="[object z]"===String(s)},"0366":function(t,e,n){var r=n("1c0b");t.exports=function(t,e,n){if(r(t),void 0===e)return t;switch(n){case 0:return function(){return t.call(e)};case 1:return function(n){return t.call(e,n)};case 2:return function(n,r){return t.call(e,n,r)};case 3:return function(n,r,i){return t.call(e,n,r,i)}}return function(){return t.apply(e,arguments)}}},"06cf":function(t,e,n){var r=n("83ab"),i=n("d1e7"),s=n("5c6c"),o=n("fc6a"),a=n("c04e"),c=n("5135"),u=n("0cfb"),l=Object.getOwnPropertyDescriptor;e.f=r?l:function(t,e){if(t=o(t),e=a(e,!0),u)try{return l(t,e)}catch(n){}if(c(t,e))return s(!i.f.call(t,e),t[e])}},"0cfb":function(t,e,n){var r=n("83ab"),i=n("d039"),s=n("cc12");t.exports=!r&&!i((function(){return 7!=Object.defineProperty(s("div"),"a",{get:function(){return 7}}).a}))},"19aa":function(t,e){t.exports=function(t,e,n){if(!(t instanceof e))throw TypeError("Incorrect "+(n?n+" ":"")+"invocation");return t}},"1be4":function(t,e,n){var r=n("d066");t.exports=r("document","documentElement")},"1c0b":function(t,e){t.exports=function(t){if("function"!=typeof t)throw TypeError(String(t)+" is not a function");return t}},"1c7e":function(t,e,n){var r=n("b622"),i=r("iterator"),s=!1;try{var o=0,a={next:function(){return{done:!!o++}},return:function(){s=!0}};a[i]=function(){return this},Array.from(a,(function(){throw 2}))}catch(c){}t.exports=function(t,e){if(!e&&!s)return!1;var n=!1;try{var r={};r[i]=function(){return{next:function(){return{done:n=!0}}}},t(r)}catch(c){}return n}},"1cdc":function(t,e,n){var r=n("342f");t.exports=/(iphone|ipod|ipad).*applewebkit/i.test(r)},"1d80":function(t,e){t.exports=function(t){if(void 0==t)throw TypeError("Can't call method on "+t);return t}},"1dde":function(t,e,n){var r=n("d039"),i=n("b622"),s=n("2d00"),o=i("species");t.exports=function(t){return s>=51||!r((function(){var e=[],n=e.constructor={};return n[o]=function(){return{foo:1}},1!==e[t](Boolean).foo}))}},2266:function(t,e,n){var r=n("825a"),i=n("e95a"),s=n("50c4"),o=n("0366"),a=n("35a1"),c=n("9bdd"),u=function(t,e){this.stopped=t,this.result=e},l=t.exports=function(t,e,n,l,h){var f,d,p,y,b,g,m,v=o(e,n,l?2:1);if(h)f=t;else{if(d=a(t),"function"!=typeof d)throw TypeError("Target is not iterable");if(i(d)){for(p=0,y=s(t.length);y>p;p++)if(b=l?v(r(m=t[p])[0],m[1]):v(t[p]),b&&b instanceof u)return b;return new u(!1)}f=d.call(t)}g=f.next;while(!(m=g.call(f)).done)if(b=c(f,v,m.value,l),"object"==typeof b&&b&&b instanceof u)return b;return new u(!1)};l.stop=function(t){return new u(!0,t)}},"23cb":function(t,e,n){var r=n("a691"),i=Math.max,s=Math.min;t.exports=function(t,e){var n=r(t);return n<0?i(n+e,0):s(n,e)}},"23e7":function(t,e,n){var r=n("da84"),i=n("06cf").f,s=n("9112"),o=n("6eeb"),a=n("ce4e"),c=n("e893"),u=n("94ca");t.exports=function(t,e){var n,l,h,f,d,p,y=t.target,b=t.global,g=t.stat;if(l=b?r:g?r[y]||a(y,{}):(r[y]||{}).prototype,l)for(h in e){if(d=e[h],t.noTargetGet?(p=i(l,h),f=p&&p.value):f=l[h],n=u(b?h:y+(g?".":"#")+h,t.forced),!n&&void 0!==f){if(typeof d===typeof f)continue;c(d,f)}(t.sham||f&&f.sham)&&s(d,"sham",!0),o(l,h,d,t)}}},"241c":function(t,e,n){var r=n("ca84"),i=n("7839"),s=i.concat("length","prototype");e.f=Object.getOwnPropertyNames||function(t){return r(t,s)}},2626:function(t,e,n){"use strict";var r=n("d066"),i=n("9bf2"),s=n("b622"),o=n("83ab"),a=s("species");t.exports=function(t){var e=r(t),n=i.f;o&&e&&!e[a]&&n(e,a,{configurable:!0,get:function(){return this}})}},"2cf4":function(t,e,n){var r,i,s,o=n("da84"),a=n("d039"),c=n("c6b6"),u=n("0366"),l=n("1be4"),h=n("cc12"),f=n("1cdc"),d=o.location,p=o.setImmediate,y=o.clearImmediate,b=o.process,g=o.MessageChannel,m=o.Dispatch,v=0,_={},w="onreadystatechange",I=function(t){if(_.hasOwnProperty(t)){var e=_[t];delete _[t],e()}},S=function(t){return function(){I(t)}},O=function(t){I(t.data)},T=function(t){o.postMessage(t+"",d.protocol+"//"+d.host)};p&&y||(p=function(t){var e=[],n=1;while(arguments.length>n)e.push(arguments[n++]);return _[++v]=function(){("function"==typeof t?t:Function(t)).apply(void 0,e)},r(v),v},y=function(t){delete _[t]},"process"==c(b)?r=function(t){b.nextTick(S(t))}:m&&m.now?r=function(t){m.now(S(t))}:g&&!f?(i=new g,s=i.port2,i.port1.onmessage=O,r=u(s.postMessage,s,1)):!o.addEventListener||"function"!=typeof postMessage||o.importScripts||a(T)||"file:"===d.protocol?r=w in h("script")?function(t){l.appendChild(h("script"))[w]=function(){l.removeChild(this),I(t)}}:function(t){setTimeout(S(t),0)}:(r=T,o.addEventListener("message",O,!1))),t.exports={set:p,clear:y}},"2d00":function(t,e,n){var r,i,s=n("da84"),o=n("342f"),a=s.process,c=a&&a.versions,u=c&&c.v8;u?(r=u.split("."),i=r[0]+r[1]):o&&(r=o.match(/Edge\/(\d+)/),(!r||r[1]>=74)&&(r=o.match(/Chrome\/(\d+)/),r&&(i=r[1]))),t.exports=i&&+i},"320c":function(t,e,n){"use strict";
-/*
-object-assign
-(c) Sindre Sorhus
-@license MIT
-*/var r=Object.getOwnPropertySymbols,i=Object.prototype.hasOwnProperty,s=Object.prototype.propertyIsEnumerable;function o(t){if(null===t||void 0===t)throw new TypeError("Object.assign cannot be called with null or undefined");return Object(t)}function a(){try{if(!Object.assign)return!1;var t=new String("abc");if(t[5]="de","5"===Object.getOwnPropertyNames(t)[0])return!1;for(var e={},n=0;n<10;n++)e["_"+String.fromCharCode(n)]=n;var r=Object.getOwnPropertyNames(e).map((function(t){return e[t]}));if("0123456789"!==r.join(""))return!1;var i={};return"abcdefghijklmnopqrst".split("").forEach((function(t){i[t]=t})),"abcdefghijklmnopqrst"===Object.keys(Object.assign({},i)).join("")}catch(s){return!1}}t.exports=a()?Object.assign:function(t,e){for(var n,a,c=o(t),u=1;uc)i.f(t,n=r[c++],e[n]);return t}},"3bbe":function(t,e,n){var r=n("861d");t.exports=function(t){if(!r(t)&&null!==t)throw TypeError("Can't set "+String(t)+" as a prototype");return t}},"3f8c":function(t,e){t.exports={}},"428f":function(t,e,n){var r=n("da84");t.exports=r},"44ad":function(t,e,n){var r=n("d039"),i=n("c6b6"),s="".split;t.exports=r((function(){return!Object("z").propertyIsEnumerable(0)}))?function(t){return"String"==i(t)?s.call(t,""):Object(t)}:Object},"44d2":function(t,e,n){var r=n("b622"),i=n("7c73"),s=n("9bf2"),o=r("unscopables"),a=Array.prototype;void 0==a[o]&&s.f(a,o,{configurable:!0,value:i(null)}),t.exports=function(t){a[o][t]=!0}},"44de":function(t,e,n){var r=n("da84");t.exports=function(t,e){var n=r.console;n&&n.error&&(1===arguments.length?n.error(t):n.error(t,e))}},4840:function(t,e,n){var r=n("825a"),i=n("1c0b"),s=n("b622"),o=s("species");t.exports=function(t,e){var n,s=r(t).constructor;return void 0===s||void 0==(n=r(s)[o])?e:i(n)}},4930:function(t,e,n){var r=n("d039");t.exports=!!Object.getOwnPropertySymbols&&!r((function(){return!String(Symbol())}))},"4cec":function(t,e,n){"use strict";t.exports=n("a93d")},"4d64":function(t,e,n){var r=n("fc6a"),i=n("50c4"),s=n("23cb"),o=function(t){return function(e,n,o){var a,c=r(e),u=i(c.length),l=s(o,u);if(t&&n!=n){while(u>l)if(a=c[l++],a!=a)return!0}else for(;u>l;l++)if((t||l in c)&&c[l]===n)return t||l||0;return!t&&-1}};t.exports={includes:o(!0),indexOf:o(!1)}},"50c4":function(t,e,n){var r=n("a691"),i=Math.min;t.exports=function(t){return t>0?i(r(t),9007199254740991):0}},5135:function(t,e){var n={}.hasOwnProperty;t.exports=function(t,e){return n.call(t,e)}},5692:function(t,e,n){var r=n("c430"),i=n("c6cd");(t.exports=function(t,e){return i[t]||(i[t]=void 0!==e?e:{})})("versions",[]).push({version:"3.6.5",mode:r?"pure":"global",copyright:"© 2020 Denis Pushkarev (zloirock.ru)"})},"56ef":function(t,e,n){var r=n("d066"),i=n("241c"),s=n("7418"),o=n("825a");t.exports=r("Reflect","ownKeys")||function(t){var e=i.f(o(t)),n=s.f;return n?e.concat(n(t)):e}},"5c40":function(t,e,n){"use strict";n.d(e,"a",(function(){return qt})),n.d(e,"b",(function(){return et})),n.d(e,"c",(function(){return d})),n.d(e,"d",(function(){return ut})),n.d(e,"e",(function(){return vt})),n.d(e,"f",(function(){return Fe})),n.d(e,"g",(function(){return De})),n.d(e,"h",(function(){return mt})),n.d(e,"i",(function(){return yt})),n.d(e,"j",(function(){return vn})),n.d(e,"k",(function(){return sn})),n.d(e,"l",(function(){return ee})),n.d(e,"m",(function(){return _n})),n.d(e,"n",(function(){return Ht})),n.d(e,"o",(function(){return Vt})),n.d(e,"p",(function(){return $t})),n.d(e,"q",(function(){return Pt})),n.d(e,"r",(function(){return at})),n.d(e,"s",(function(){return K})),n.d(e,"t",(function(){return H})),n.d(e,"u",(function(){return wn})),n.d(e,"v",(function(){return In})),n.d(e,"w",(function(){return Z})),n.d(e,"x",(function(){return Zt})),n.d(e,"y",(function(){return te})),n.d(e,"z",(function(){return Kt})),n.d(e,"A",(function(){return o})),n.d(e,"B",(function(){return Ue})),n.d(e,"C",(function(){return $})),n.d(e,"D",(function(){return G}));var r=n("a1e9"),i=n("9ff4");const s=[];function o(t,...e){Object(r["f"])();const n=s.length?s[s.length-1].component:null,i=n&&n.appContext.config.warnHandler,o=a();if(i)f(i,n,11,[t+e.join(""),n&&n.proxy,o.map(({vnode:t})=>`at <${gn(n,t.type)}>`).join("\n"),o]);else{const n=["[Vue warn]: "+t,...e];o.length&&n.push("\n",...c(o)),console.warn(...n)}Object(r["j"])()}function a(){let t=s[s.length-1];if(!t)return[];const e=[];while(t){const n=e[0];n&&n.vnode===t?n.recurseCount++:e.push({vnode:t,recurseCount:0});const r=t.component&&t.component.parent;t=r&&r.vnode}return e}function c(t){const e=[];return t.forEach((t,n)=>{e.push(...0===n?[]:["\n"],...u(t))}),e}function u({vnode:t,recurseCount:e}){const n=e>0?`... (${e} recursive calls)`:"",r=!!t.component&&null==t.component.parent,i=" at <"+gn(t.component,t.type,r),s=">"+n;return t.props?[i,...l(t.props),s]:[i+s]}function l(t){const e=[],n=Object.keys(t);return n.slice(0,3).forEach(n=>{e.push(...h(n,t[n]))}),n.length>3&&e.push(" ..."),e}function h(t,e,n){return Object(i["x"])(e)?(e=JSON.stringify(e),n?e:[`${t}=${e}`]):"number"===typeof e||"boolean"===typeof e||null==e?n?e:[`${t}=${e}`]:Object(r["e"])(e)?(e=h(t,Object(r["m"])(e.value),!0),n?e:[t+"=Ref<",e,">"]):Object(i["n"])(e)?[`${t}=fn${e.name?`<${e.name}>`:""}`]:(e=Object(r["m"])(e),n?e:[t+"=",e])}function f(t,e,n,r){let i;try{i=r?t(...r):t()}catch(s){p(s,e,n)}return i}function d(t,e,n,r){if(Object(i["n"])(t)){const s=f(t,e,n,r);return s&&Object(i["t"])(s)&&s.catch(t=>{p(t,e,n)}),s}const s=[];for(let i=0;i-1&&(b[e]=null)}function B(t){Object(i["m"])(t)?g.push(...t):S&&S.includes(t,O+1)||g.push(t),j()}function j(){_||w||(w=!0,v=m.then(L))}function D(t){if(g.length){for(S=[...new Set(g)],g.length=0,O=0;Onull==t.id?1/0:t.id;function L(t){for(w=!1,_=!0,b.sort((t,e)=>F(t)-F(e)),I=0;I1?n(a,{attrs:u,slots:c,emit:l}):n(a,null)),t=e.props?u:C(u)}let p=g;if(!1!==e.inheritAttrs&&t){const e=Object.keys(t),{shapeFlag:n}=p;e.length&&(1&n||6&n)&&(1&n&&e.some(i["q"])&&(t=N(t)),p=gt(p,t))}const m=r.scopeId,v=m&&p.scopeId!==m,_=n&&n.type.__scopeId,w=_&&_!==m?_+"-s":null;if(v||w){const t={};v&&(t[m]=""),w&&(t[w]=""),p=gt(p,t)}r.dirs&&(p.dirs=r.dirs),r.transition&&(p.transition=r.transition),g=p}catch(m){p(m,t,1),g=yt(rt)}return E=null,g}const C=t=>{let e;for(const n in t)("class"===n||"style"===n||Object(i["s"])(n))&&((e||(e={}))[n]=t[n]);return e},N=t=>{const e={};for(const n in t)Object(i["q"])(n)||(e[n]=t[n]);return e};function k(t,e,n){const{props:r,children:i}=t,{props:s,children:o,patchFlag:a}=e;if(e.dirs||e.transition)return!0;if(!(n&&a>0))return!(!i&&!o||o&&o.$stable)||r!==s&&(r?!s||V(r,s):!!s);if(1024&a)return!0;if(16&a)return r?V(r,s):!!s;if(8&a){const t=e.dynamicProps;for(let e=0;et.__isSuspense;function z(t,e){e&&!e.isResolved?Object(i["m"])(t)?e.effects.push(...t):e.effects.push(t):B(t)}function $(t,e=E){return e?function(){const n=E;M(e);const r=t.apply(null,arguments);return M(n),r}:t}let Y=null;const W=[];function H(t){W.push(Y=t)}function K(){W.pop(),Y=W[W.length-1]||null}function G(t){return e=>$((function(){H(t);const n=e.apply(this,arguments);return K(),n}))}const q=t=>t.__isTeleport;const J="components";function Z(t){return Q(J,t)||t}const X=Symbol();function Q(t,e,n=!0){const r=E||rn;if(r){const n=r.type;if(t===J){const t=n.displayName||n.name;if(t&&(t===e||t===Object(i["e"])(e)||t===Object(i["f"])(Object(i["e"])(e))))return n}const s=tt(n[t],e)||tt(r.appContext[t],e);return s}}function tt(t,e){return t&&(t[e]||t[Object(i["e"])(e)]||t[Object(i["f"])(Object(i["e"])(e))])}const et=Symbol(void 0),nt=Symbol(void 0),rt=Symbol(void 0),it=Symbol(void 0),st=[];let ot=null;function at(t=!1){st.push(ot=t?null:[])}let ct=1;function ut(t,e,n,r,s){const o=yt(t,e,n,r,s,!0);return o.dynamicChildren=ot||i["a"],st.pop(),ot=st[st.length-1]||null,ot&&ot.push(o),o}function lt(t){return!!t&&!0===t.__v_isVNode}function ht(t,e){return t.type===e.type&&t.key===e.key}const ft="__vInternal",dt=({key:t})=>null!=t?t:null,pt=({ref:t})=>null!=t?Object(i["m"])(t)?t:[E,t]:null,yt=bt;function bt(t,e=null,n=null,s=0,o=null,a=!1){if(t&&t!==X||(t=rt),lt(t)){const r=gt(t,e);return n&&It(r,n),r}if(Object(i["n"])(t)&&"__vccOpts"in t&&(t=t.__vccOpts),e){(Object(r["c"])(e)||ft in e)&&(e=Object(i["h"])({},e));let{class:t,style:n}=e;t&&!Object(i["x"])(t)&&(e.class=Object(i["C"])(t)),Object(i["r"])(n)&&(Object(r["c"])(n)&&!Object(i["m"])(n)&&(n=Object(i["h"])({},n)),e.style=Object(i["D"])(n))}const c=Object(i["x"])(t)?1:P(t)?128:q(t)?64:Object(i["r"])(t)?4:Object(i["n"])(t)?2:0;const u={__v_isVNode:!0,__v_skip:!0,type:t,props:e,key:e&&dt(e),ref:e&&pt(e),scopeId:Y,children:null,component:null,suspense:null,dirs:null,transition:null,el:null,anchor:null,target:null,targetAnchor:null,staticCount:0,shapeFlag:c,patchFlag:s,dynamicProps:o,dynamicChildren:null,appContext:null};return It(u,n),ct>0&&!a&&ot&&32!==s&&(s>0||6&c)&&ot.push(u),u}function gt(t,e){const{props:n,patchFlag:r}=t,s=e?n?St(n,e):Object(i["h"])({},e):n;return{__v_isVNode:!0,__v_skip:!0,type:t.type,props:s,key:s&&dt(s),ref:e&&e.ref?pt(e):t.ref,scopeId:t.scopeId,children:t.children,target:t.target,targetAnchor:t.targetAnchor,staticCount:t.staticCount,shapeFlag:t.shapeFlag,patchFlag:e&&t.type!==et?-1===r?16:16|r:r,dynamicProps:t.dynamicProps,dynamicChildren:t.dynamicChildren,appContext:t.appContext,dirs:t.dirs,transition:t.transition,component:t.component,suspense:t.suspense,el:t.el,anchor:t.anchor}}function mt(t=" ",e=0){return yt(nt,null,t,e)}function vt(t="",e=!1){return e?(at(),ut(rt,null,t)):yt(rt,null,t)}function _t(t){return null==t||"boolean"===typeof t?yt(rt):Object(i["m"])(t)?yt(et,null,t):"object"===typeof t?null===t.el?t:gt(t):yt(nt,null,String(t))}function wt(t){return null===t.el?t:gt(t)}function It(t,e){let n=0;const{shapeFlag:r}=t;if(null==e)e=null;else if(Object(i["m"])(e))n=16;else if("object"===typeof e){if((1&r||64&r)&&e.default)return void It(t,e.default());{n=32;const r=e._;r||ft in e?3===r&&E&&(1024&E.vnode.patchFlag?(e._=2,t.patchFlag|=1024):e._=1):e._ctx=E}}else Object(i["n"])(e)?(e={default:e,_ctx:E},n=32):(e=String(e),64&r?(n=16,e=[mt(e)]):n=8);t.children=e,t.shapeFlag|=n}function St(...t){const e=Object(i["h"])({},t[0]);for(let n=1;nObject(i["h"])(n,Tt(t))))),e||r?(Object(i["m"])(e)?e.forEach(t=>n[t]=null):Object(i["h"])(n,e),t.__emits=n):t.__emits=void 0}function At(t,e){let n;return!(!Object(i["s"])(e)||!(n=Tt(t)))&&(e=e.replace(/Once$/,""),Object(i["j"])(n,e[2].toLowerCase()+e.slice(3))||Object(i["j"])(n,e.slice(2)))}function xt(t,e,n,s=!1){const o={},a={};Object(i["g"])(a,ft,1),jt(t,e,o,a),n?t.props=s?o:Object(r["k"])(o):t.type.props?t.props=o:t.props=a,t.attrs=a}function Bt(t,e,n,s){const{props:o,attrs:a,vnode:{patchFlag:c}}=t,u=Object(r["m"])(o),[l]=Ft(t.type);if(!(s||c>0)||16&c){let r;jt(t,e,o,a);for(const t in u)e&&(Object(i["j"])(e,t)||(r=Object(i["k"])(t))!==t&&Object(i["j"])(e,r))||(l?!n||void 0===n[t]&&void 0===n[r]||(o[t]=Dt(l,e||i["b"],t,void 0)):delete o[t]);if(a!==u)for(const t in a)e&&Object(i["j"])(e,t)||delete a[t]}else if(8&c){const n=t.vnode.dynamicProps;for(let t=0;t{const[e,s]=Ft(t);Object(i["h"])(n,e),s&&r.push(...s)};t.extends&&(s=!0,e(t.extends)),t.mixins&&(s=!0,t.mixins.forEach(e))}if(!e&&!s)return t.__props=i["a"];if(Object(i["m"])(e))for(let a=0;a-1,a[1]=e<0||t-1||Object(i["j"])(a,"default"))&&r.push(s)}}}}const o=[n,r];return t.__props=o,o}function Lt(t){const e=t&&t.toString().match(/^\s*function (\w+)/);return e?e[1]:""}function Et(t,e){return Lt(t)===Lt(e)}function Mt(t,e){if(Object(i["m"])(e)){for(let n=0,r=e.length;n{if(n.isUnmounted)return;Object(r["f"])(),on(n);const s=d(e,n,t,i);return on(null),Object(r["j"])(),s});i?s.unshift(o):s.push(o)}else 0}const Nt=t=>(e,n=rn)=>!cn&&Ct(t,e,n),kt=Nt("bm"),Vt=Nt("m"),Rt=Nt("bu"),Pt=Nt("u"),zt=Nt("bum"),$t=Nt("um"),Yt=Nt("rtg"),Wt=Nt("rtc"),Ht=(t,e=rn)=>{Ct("ec",t,e)};function Kt(){const t={isMounted:!1,isLeaving:!1,isUnmounting:!1,leavingVNodes:new Map};return Vt(()=>{t.isMounted=!0}),zt(()=>{t.isUnmounting=!0}),t}const Gt={name:"BaseTransition",props:{mode:String,appear:Boolean,persisted:Boolean,onBeforeEnter:Function,onEnter:Function,onAfterEnter:Function,onEnterCancelled:Function,onBeforeLeave:Function,onLeave:Function,onAfterLeave:Function,onLeaveCancelled:Function,onBeforeAppear:Function,onAppear:Function,onAfterAppear:Function,onAppearCancelled:Function},setup(t,{slots:e}){const n=sn(),i=Kt();let s;return()=>{const o=e.default&&ee(e.default(),!0);if(!o||!o.length)return;const a=Object(r["m"])(t),{mode:c}=a;const u=o[0];if(i.isLeaving)return Xt(u);const l=Qt(u);if(!l)return Xt(u);const h=l.transition=Zt(l,a,i,n),f=n.subTree,d=f&&Qt(f);let p=!1;const{getTransitionKey:y}=l.type;if(y){const t=y();void 0===s?s=t:t!==s&&(s=t,p=!0)}if(d&&d.type!==rt&&(!ht(l,d)||p)){const t=Zt(d,a,i,n);if(te(d,t),"out-in"===c)return i.isLeaving=!0,t.afterLeave=()=>{i.isLeaving=!1,n.update()},Xt(u);"in-out"===c&&(t.delayLeave=(t,e,n)=>{const r=Jt(i,d);r[String(d.key)]=d,t._leaveCb=()=>{e(),t._leaveCb=void 0,delete h.delayedLeave},h.delayedLeave=n})}return u}}},qt=Gt;function Jt(t,e){const{leavingVNodes:n}=t;let r=n.get(e.type);return r||(r=Object.create(null),n.set(e.type,r)),r}function Zt(t,{appear:e,persisted:n=!1,onBeforeEnter:r,onEnter:i,onAfterEnter:s,onEnterCancelled:o,onBeforeLeave:a,onLeave:c,onAfterLeave:u,onLeaveCancelled:l,onBeforeAppear:h,onAppear:f,onAfterAppear:p,onAppearCancelled:y},b,g){const m=String(t.key),v=Jt(b,t),_=(t,e)=>{t&&d(t,g,9,e)},w={persisted:n,beforeEnter(n){let i=r;if(!b.isMounted){if(!e)return;i=h||r}n._leaveCb&&n._leaveCb(!0);const s=v[m];s&&ht(t,s)&&s.el._leaveCb&&s.el._leaveCb(),_(i,[n])},enter(t){let n=i,r=s,a=o;if(!b.isMounted){if(!e)return;n=f||i,r=p||s,a=y||o}let c=!1;const u=t._enterCb=e=>{c||(c=!0,_(e?a:r,[t]),w.delayedLeave&&w.delayedLeave(),t._enterCb=void 0)};n?(n(t,u),n.length<=1&&u()):u()},leave(e,n){const r=String(t.key);if(e._enterCb&&e._enterCb(!0),b.isUnmounting)return n();_(a,[e]);let i=!1;const s=e._leaveCb=s=>{i||(i=!0,n(),_(s?l:u,[e]),e._leaveCb=void 0,v[r]===t&&delete v[r])};v[r]=t,c?(c(e,s),c.length<=1&&s()):s()}};return w}function Xt(t){if(ne(t))return t=gt(t),t.children=null,t}function Qt(t){return ne(t)?t.children?t.children[0]:void 0:t}function te(t,e){6&t.shapeFlag&&t.component?te(t.component.subTree,e):t.transition=e}function ee(t,e=!1){let n=[],r=0;for(let i=0;i1)for(let i=0;it.type.__isKeepAlive;RegExp,RegExp;function re(t){return t.displayName||t.name}function ie(t,e){return Object(i["m"])(t)?t.some(t=>ie(t,e)):Object(i["x"])(t)?t.split(",").indexOf(e)>-1:!!t.test&&t.test(e)}function se(t,e){ae(t,"a",e)}function oe(t,e){ae(t,"da",e)}function ae(t,e,n=rn){const r=t.__wdc||(t.__wdc=()=>{let e=n;while(e){if(e.isDeactivated)return;e=e.parent}t()});if(Ct(e,r,n),n){let t=n.parent;while(t&&t.parent)ne(t.parent.vnode)&&ce(r,e,n,t),t=t.parent}}function ce(t,e,n,r){Ct(e,t,r,!0),$t(()=>{Object(i["E"])(r[e],t)},n)}function ue(t){let e=t.shapeFlag;256&e&&(e-=256),512&e&&(e-=512),t.shapeFlag=e}const le=t=>"_"===t[0]||"$stable"===t,he=t=>Object(i["m"])(t)?t.map(_t):[_t(t)],fe=(t,e,n)=>$(t=>he(e(t)),n),de=(t,e)=>{const n=t._ctx;for(const r in t){if(le(r))continue;const s=t[r];if(Object(i["n"])(s))e[r]=fe(r,s,n);else if(null!=s){0;const t=he(s);e[r]=()=>t}}},pe=(t,e)=>{const n=he(e);t.slots.default=()=>n},ye=(t,e)=>{if(32&t.vnode.shapeFlag){const n=e._;n?(t.slots=e,Object(i["g"])(e,"_",n)):de(e,t.slots={})}else t.slots={},e&&pe(t,e);Object(i["g"])(t.slots,ft,1)},be=(t,e)=>{const{vnode:n,slots:r}=t;let s=!0,o=i["b"];if(32&n.shapeFlag){const t=e._;t?1===t?s=!1:Object(i["h"])(r,e):(s=!e.$stable,de(e,r)),o=e}else e&&(pe(t,e),o={default:1});if(s)for(const i in r)le(i)||i in o||delete r[i]};function ge(t,e,n,r){const i=t.dirs,s=e&&e.dirs;for(let o=0;o/svg/.test(t.namespaceURI)&&"foreignObject"!==t.tagName,Oe=t=>8===t.nodeType;function Te(t){const{mt:e,p:n,o:{patchProp:r,nextSibling:s,parentNode:o,remove:a,insert:c,createComment:u}}=t,l=(t,e)=>{Ie=!1,h(e.firstChild,t,null,null),D(),Ie&&console.error("Hydration completed but contains mismatches.")},h=(n,r,i,a,c=!1)=>{const u=Oe(n)&&"["===n.data,l=()=>y(n,r,i,a,u),{type:g,ref:m,shapeFlag:v}=r,_=n.nodeType;r.el=n;let w=null;switch(g){case nt:3!==_?w=l():(n.data!==r.children&&(Ie=!0,n.data=r.children),w=s(n));break;case rt:w=8!==_||u?l():s(n);break;case it:if(1===_){w=n;const t=!r.children.length;for(let e=0;e{e(r,t,null,i,a,Se(t),c)},h=r.type.__asyncLoader;h?h().then(l):l(),w=u?b(n):s(n)}else 64&v?w=8!==_?l():r.type.hydrate(n,r,i,a,c,t,d):128&v&&(w=r.type.hydrate(n,r,i,a,Se(o(n)),c,t,h))}return null!=m&&i&&je(m,null,i,a,r),w},f=(t,e,n,s,o)=>{o=o||!!e.dynamicChildren;const{props:c,patchFlag:u,shapeFlag:l,dirs:h}=e;if(-1!==u){if(c)if(!o||16&u||32&u)for(const e in c)!Object(i["u"])(e)&&Object(i["s"])(e)&&r(t,e,null,c[e]);else c.onClick&&r(t,"onClick",null,c.onClick);let f;if((f=c&&c.onVnodeBeforeMount)&&Ee(f,n,e),h&&ge(e,null,n,"beforeMount"),((f=c&&c.onVnodeMounted)||h)&&z(()=>{f&&Ee(f,n,e),h&&ge(e,null,n,"mounted")},s),16&l&&(!c||!c.innerHTML&&!c.textContent)){let r=d(t.firstChild,e,t,n,s,o);while(r){Ie=!0;const t=r;r=r.nextSibling,a(t)}}else 8&l&&t.textContent!==e.children&&(Ie=!0,t.textContent=e.children)}return t.nextSibling},d=(t,e,r,i,s,o)=>{o=o||!!e.dynamicChildren;const a=e.children,c=a.length;for(let u=0;u{const a=o(t),l=d(s(t),e,a,n,r,i);return l&&Oe(l)&&"]"===l.data?s(e.anchor=l):(Ie=!0,c(e.anchor=u("]"),a,l),l)},y=(t,e,r,i,c)=>{if(Ie=!0,e.el=null,c){const e=b(t);while(1){const n=s(t);if(!n||n===e)break;a(n)}}const u=s(t),l=o(t);return a(t),n(null,e,l,u,r,i,Se(l)),u},b=t=>{let e=0;while(t)if(t=s(t),t&&Oe(t)&&("["===t.data&&e++,"]"===t.data)){if(0===e)return s(t);e--}return t};return[l,h]}function Ae(){}const xe={scheduler:A};const Be=z,je=(t,e,n,s,o)=>{let a;a=o?4&o.shapeFlag?o.component.proxy:o.el:null;const[c,u]=t;const l=e&&e[1],h=c.refs===i["b"]?c.refs={}:c.refs,d=c.setupState;null!=l&&l!==u&&(Object(i["x"])(l)?(h[l]=null,Object(i["j"])(d,l)&&Be(()=>{d[l]=null},s)):Object(r["e"])(l)&&(l.value=null)),Object(i["x"])(u)?(h[u]=a,Object(i["j"])(d,u)&&Be(()=>{d[u]=a},s)):Object(r["e"])(u)?u.value=a:Object(i["n"])(u)&&f(u,n,12,[a,h])};function De(t){return Le(t)}function Fe(t){return Le(t,Te)}function Le(t,e){Ae();const{insert:n,remove:s,patchProp:o,forcePatchProp:a,createElement:c,createText:u,createComment:l,setText:h,setElementText:f,parentNode:d,nextSibling:p,setScopeId:y=i["d"],cloneNode:b,insertStaticContent:g}=t,m=(t,e,n,r=null,i=null,s=null,o=!1,a=!1)=>{t&&!ht(t,e)&&(r=G(t),$(t,i,s,!0),t=null),-2===e.patchFlag&&(a=!1,e.dynamicChildren=null);const{type:c,ref:u,shapeFlag:l}=e;switch(c){case nt:v(t,e,n,r);break;case rt:_(t,e,n,r);break;case it:null==t&&w(e,n,r,o);break;case et:j(t,e,n,r,i,s,o,a);break;default:1&l?I(t,e,n,r,i,s,o,a):6&l?F(t,e,n,r,i,s,o,a):(64&l||128&l)&&c.process(t,e,n,r,i,s,o,a,J)}null!=u&&i&&je(u,t&&t.ref,i,s,e)},v=(t,e,r,i)=>{if(null==t)n(e.el=u(e.children),r,i);else{const n=e.el=t.el;e.children!==t.children&&h(n,e.children)}},_=(t,e,r,i)=>{null==t?n(e.el=l(e.children||""),r,i):e.el=t.el},w=(t,e,n,r)=>{[t.el,t.anchor]=g(t.children,e,n,r)},I=(t,e,n,r,i,s,o,a)=>{o=o||"svg"===e.type,null==t?S(e,n,r,i,s,o,a):T(t,e,i,s,o,a)},S=(t,e,r,s,a,u,l)=>{let h,d;const{type:p,props:g,shapeFlag:m,transition:v,scopeId:_,patchFlag:w,dirs:I}=t;if(t.el&&void 0!==b&&-1===w)h=t.el=b(t.el);else{if(h=t.el=c(t.type,u,g&&g.is),8&m?f(h,t.children):16&m&&O(t.children,h,null,s,a,u&&"foreignObject"!==p,l||!!t.dynamicChildren),g){for(const e in g)Object(i["u"])(e)||o(h,e,null,g[e],u,t.children,s,a,K);(d=g.onVnodeBeforeMount)&&Ee(d,s,t)}I&&ge(t,null,s,"beforeMount"),_&&y(h,_);const e=s&&s.type.__scopeId;e&&e!==_&&y(h,e+"-s"),v&&!v.persisted&&v.beforeEnter(h)}n(h,e,r);const S=(!a||a&&a.isResolved)&&v&&!v.persisted;((d=g&&g.onVnodeMounted)||S||I)&&Be(()=>{d&&Ee(d,s,t),S&&v.enter(h),I&&ge(t,null,s,"mounted")},a)},O=(t,e,n,r,i,s,o,a=0)=>{for(let c=a;c{const u=e.el=t.el;let{patchFlag:l,dynamicChildren:h,dirs:d}=e;l|=16&t.patchFlag;const p=t.props||i["b"],y=e.props||i["b"];let b;if((b=y.onVnodeBeforeUpdate)&&Ee(b,n,e,t),d&&ge(e,t,n,"beforeUpdate"),l>0){if(16&l)B(u,e,p,y,n,r,s);else if(2&l&&p.class!==y.class&&o(u,"class",null,y.class,s),4&l&&o(u,"style",p.style,y.style,s),8&l){const i=e.dynamicProps;for(let e=0;e{b&&Ee(b,n,e,t),d&&ge(e,t,n,"updated")},r)},A=(t,e,n,r,i,s)=>{for(let o=0;o{if(n!==r){for(const l in r){if(Object(i["u"])(l))continue;const h=r[l],f=n[l];(h!==f||a&&a(t,l))&&o(t,l,f,h,u,e.children,s,c,K)}if(n!==i["b"])for(const a in n)Object(i["u"])(a)||a in r||o(t,a,n[a],null,u,e.children,s,c,K)}},j=(t,e,r,i,s,o,a,c)=>{const l=e.el=t?t.el:u(""),h=e.anchor=t?t.anchor:u("");let{patchFlag:f,dynamicChildren:d}=e;f>0&&(c=!0),null==t?(n(l,r,i),n(h,r,i),O(e.children,r,h,s,o,a,c)):f>0&&64&f&&d?A(t.dynamicChildren,d,r,s,o,a):N(t,e,r,h,s,o,a,c)},F=(t,e,n,r,i,s,o,a)=>{null==t?512&e.shapeFlag?i.ctx.activate(e,n,r,o,a):L(e,n,r,i,s,o,a):E(t,e,a)},L=(t,e,n,r,i,s,o)=>{const a=t.component=nn(t,r,i);if(ne(t)&&(a.ctx.renderer=J),un(a),a.asyncDep){if(!i)return void 0;if(i.registerDep(a,M),!t.el){const t=a.subTree=yt(rt);_(null,t,e,n)}}else M(a,t,e,n,i,s,o)},E=(t,e,n)=>{const r=e.component=t.component;if(k(t,e,n)){if(r.asyncDep&&!r.asyncResolved)return void C(r,e,n);r.next=e,x(r.update),r.update()}else e.component=t.component,e.el=t.el,r.vnode=e},M=(t,e,n,s,o,a,c)=>{t.update=Object(r["b"])((function(){if(t.isMounted){let e,{next:n,bu:r,u:s,parent:u,vnode:l}=t,h=n;0,n?C(t,n,c):n=l;const f=U(t);0;const p=t.subTree;t.subTree=f,n.el=l.el,r&&Object(i["l"])(r),(e=n.props&&n.props.onVnodeBeforeUpdate)&&Ee(e,u,n,l),t.refs!==i["b"]&&(t.refs={}),m(p,f,d(p.el),G(p),t,o,a),n.el=f.el,null===h&&R(t,f.el),s&&Be(s,o),(e=n.props&&n.props.onVnodeUpdated)&&Be(()=>{Ee(e,u,n,l)},o)}else{let r;const{el:c,props:u}=e,{bm:l,m:h,a:f,parent:d}=t;0;const p=t.subTree=U(t);0,l&&Object(i["l"])(l),(r=u&&u.onVnodeBeforeMount)&&Ee(r,d,e),c&&X?X(e.el,p,t,o):(m(null,p,n,s,t,o,a),e.el=p.el),h&&Be(h,o),(r=u&&u.onVnodeMounted)&&Be(()=>{Ee(r,d,e)},o),f&&256&e.shapeFlag&&Be(f,o),t.isMounted=!0}}),xe)},C=(t,e,n)=>{e.component=t;const r=t.vnode.props;t.vnode=e,t.next=null,Bt(t,e.props,r,n),be(t,e.children)},N=(t,e,n,r,i,s,o,a=!1)=>{const c=t&&t.children,u=t?t.shapeFlag:0,l=e.children,{patchFlag:h,shapeFlag:d}=e;if(h>0){if(128&h)return void P(c,l,n,r,i,s,o,a);if(256&h)return void V(c,l,n,r,i,s,o,a)}8&d?(16&u&&K(c,i,s),l!==c&&f(n,l)):16&u?16&d?P(c,l,n,r,i,s,o,a):K(c,i,s,!0):(8&u&&f(n,""),16&d&&O(l,n,r,i,s,o,a))},V=(t,e,n,r,s,o,a,c)=>{t=t||i["a"],e=e||i["a"];const u=t.length,l=e.length,h=Math.min(u,l);let f;for(f=0;fl?K(t,s,o,!0,h):O(e,n,r,s,o,a,c,h)},P=(t,e,n,r,s,o,a,c)=>{let u=0;const l=e.length;let h=t.length-1,f=l-1;while(u<=h&&u<=f){const r=t[u],i=e[u]=c?wt(e[u]):_t(e[u]);if(!ht(r,i))break;m(r,i,n,null,s,o,a,c),u++}while(u<=h&&u<=f){const r=t[h],i=e[f]=c?wt(e[f]):_t(e[f]);if(!ht(r,i))break;m(r,i,n,null,s,o,a,c),h--,f--}if(u>h){if(u<=f){const t=f+1,i=tf)while(u<=h)$(t[u],s,o,!0),u++;else{const d=u,p=u,y=new Map;for(u=p;u<=f;u++){const t=e[u]=c?wt(e[u]):_t(e[u]);null!=t.key&&y.set(t.key,u)}let b,g=0;const v=f-p+1;let _=!1,w=0;const I=new Array(v);for(u=0;u=v){$(r,s,o,!0);continue}let i;if(null!=r.key)i=y.get(r.key);else for(b=p;b<=f;b++)if(0===I[b-p]&&ht(r,e[b])){i=b;break}void 0===i?$(r,s,o,!0):(I[i-p]=u+1,i>=w?w=i:_=!0,m(r,e[i],n,null,s,o,a,c),g++)}const S=_?Me(I):i["a"];for(b=S.length-1,u=v-1;u>=0;u--){const t=p+u,i=e[t],c=t+1{const{el:o,type:a,transition:c,children:u,shapeFlag:l}=t;if(6&l)return void z(t.component.subTree,e,r,i);if(128&l)return void t.suspense.move(e,r,i);if(64&l)return void a.move(t,e,r,J);if(a===et){n(o,e,r);for(let t=0;tc.enter(o),s);else{const{leave:t,delayLeave:i,afterLeave:s}=c,a=()=>n(o,e,r),u=()=>{t(o,()=>{a(),s&&s()})};i?i(o,a,u):u()}else n(o,e,r)},$=(t,e,n,r=!1)=>{const{type:i,props:s,ref:o,children:a,dynamicChildren:c,shapeFlag:u,patchFlag:l,dirs:h}=t;if(null!=o&&e&&je(o,null,e,n,null),256&u)return void e.ctx.deactivate(t);const f=1&u&&h;let d;if((d=s&&s.onVnodeBeforeUnmount)&&Ee(d,e,t),6&u)H(t.component,n,r);else{if(128&u)return void t.suspense.unmount(n,r);f&&ge(t,null,e,"beforeUnmount"),c&&(i!==et||l>0&&64&l)?K(c,e,n):16&u&&K(a,e,n),64&u&&t.type.remove(t,J),r&&Y(t)}((d=s&&s.onVnodeUnmounted)||f)&&Be(()=>{d&&Ee(d,e,t),f&&ge(t,null,e,"unmounted")},n)},Y=t=>{const{type:e,el:n,anchor:r,transition:i}=t;if(e===et)return void W(n,r);const o=()=>{s(n),i&&!i.persisted&&i.afterLeave&&i.afterLeave()};if(1&t.shapeFlag&&i&&!i.persisted){const{leave:e,delayLeave:r}=i,s=()=>e(n,o);r?r(t.el,o,s):s()}else o()},W=(t,e)=>{let n;while(t!==e)n=p(t),s(t),t=n;s(e)},H=(t,e,n)=>{const{bum:s,effects:o,update:a,subTree:c,um:u,da:l,isDeactivated:h}=t;if(s&&Object(i["l"])(s),o)for(let i=0;i{t.isUnmounted=!0},e),!e||e.isResolved||e.isUnmounted||!t.asyncDep||t.asyncResolved||(e.deps--,0===e.deps&&e.resolve())},K=(t,e,n,r=!1,i=0)=>{for(let s=i;s6&t.shapeFlag?G(t.component.subTree):128&t.shapeFlag?t.suspense.next():p(t.anchor||t.el),q=(t,e)=>{null==t?e._vnode&&$(e._vnode,null,null,!0):m(e._vnode||null,t,e),D(),e._vnode=t},J={p:m,um:$,m:z,r:Y,mt:L,mc:O,pc:N,pbc:A,n:G,o:t};let Z,X;return e&&([Z,X]=e(J)),{render:q,hydrate:Z,createApp:we(q,Z)}}function Ee(t,e,n,r=null){d(t,e,7,[n,r])}function Me(t){const e=t.slice(),n=[0];let r,i,s,o,a;const c=t.length;for(r=0;r0&&(e[r]=n[s-1]),n[s]=r)}}s=n.length,o=n[s-1];while(s-- >0)n[s]=o,o=e[o];return n}function Ue(t,e){return ke(t,null,e)}const Ce={};function Ne(t,e,n){return ke(t,e,n)}function ke(t,e,{immediate:n,deep:s,flush:o,onTrack:a,onTrigger:c}=i["b"],u=rn){let l,h;if(Object(r["e"])(t)?l=()=>t.value:Object(r["d"])(t)?(l=()=>t,s=!0):l=Object(i["m"])(t)?()=>t.map(t=>Object(r["e"])(t)?t.value:Object(r["d"])(t)?Re(t):Object(i["n"])(t)?f(t,u,2):void 0):Object(i["n"])(t)?e?()=>f(t,u,2):()=>{if(!u||!u.isUnmounted)return h&&h(),f(t,u,3,[p])}:i["d"],e&&s){const t=l;l=()=>Re(t())}const p=t=>{h=m.options.onStop=()=>{f(t,u,4)}};let y=Object(i["m"])(t)?[]:Ce;const b=()=>{if(m.active)if(e){const t=m();(s||Object(i["i"])(t,y))&&(h&&h(),d(e,u,3,[t,y===Ce?void 0:y,p]),y=t)}else m()};let g;"sync"===o?g=b:"pre"===o?(b.id=-1,g=()=>{!u||u.isMounted?A(b):b()}):g=()=>Be(b,u&&u.suspense);const m=Object(r["b"])(l,{lazy:!0,onTrack:a,onTrigger:c,scheduler:g});return pn(m),e?n?b():y=m():m(),()=>{Object(r["l"])(m),u&&Object(i["E"])(u.effects,m)}}function Ve(t,e,n){const r=this.proxy,s=Object(i["x"])(t)?()=>r[t]:t.bind(r);return ke(s,e.bind(r),n,this)}function Re(t,e=new Set){if(!Object(i["r"])(t)||e.has(t))return t;if(e.add(t),Object(i["m"])(t))for(let n=0;n{Re(t.get(r),e)});else if(t instanceof Set)t.forEach(t=>{Re(t,e)});else for(const n in t)Re(t[n],e);return t}function Pe(t,e){if(rn){let n=rn.provides;const r=rn.parent&&rn.parent.provides;r===n&&(n=rn.provides=Object.create(r)),n[t]=e}else 0}function ze(t,e){const n=rn||E;if(n){const r=n.provides;if(t in r)return r[t];if(arguments.length>1)return e}else 0}function $e(t,e,n=[],r=[],s=!1){const{mixins:o,extends:a,data:c,computed:u,methods:l,watch:h,provide:f,inject:d,beforeMount:p,mounted:y,beforeUpdate:b,updated:g,activated:m,deactivated:v,beforeUnmount:_,unmounted:w,render:I,renderTracked:S,renderTriggered:O,errorCaptured:T}=e,A=t.proxy,x=t.ctx,B=t.appContext.mixins;s&&I&&t.render===i["d"]&&(t.render=I),s||(Ye("beforeCreate",e,A,B),He(t,B,n,r)),a&&$e(t,a,n,r,!0),o&&He(t,o,n,r);if(d)if(Object(i["m"])(d))for(let i=0;iKe(t,e,A)),u)for(const j in u){const t=u[j],e=Object(i["n"])(t)?t.bind(A,A):Object(i["n"])(t.get)?t.get.bind(A,A):i["d"];0;const n=!Object(i["n"])(t)&&Object(i["n"])(t.set)?t.set.bind(A):i["d"],r=mn({get:e,set:n});Object.defineProperty(x,j,{enumerable:!0,configurable:!0,get:()=>r.value,set:t=>r.value=t})}if(h&&r.push(h),!s&&r.length&&r.forEach(t=>{for(const e in t)Ge(t[e],x,A,e)}),f){const t=Object(i["n"])(f)?f.call(A):f;for(const e in t)Pe(e,t[e])}s||Ye("created",e,A,B),p&&kt(p.bind(A)),y&&Vt(y.bind(A)),b&&Rt(b.bind(A)),g&&Pt(g.bind(A)),m&&se(m.bind(A)),v&&oe(v.bind(A)),T&&Ht(T.bind(A)),S&&Wt(S.bind(A)),O&&Yt(O.bind(A)),_&&zt(_.bind(A)),w&&$t(w.bind(A))}function Ye(t,e,n,r){We(t,r,n);const i=e.extends&&e.extends[t];i&&i.call(n);const s=e.mixins;s&&We(t,s,n);const o=e[t];o&&o.call(n)}function We(t,e,n){for(let r=0;rn[r];if(Object(i["x"])(t)){const n=e[t];Object(i["n"])(n)&&Ne(s,n)}else Object(i["n"])(t)?Ne(s,t.bind(n)):Object(i["r"])(t)&&(Object(i["m"])(t)?t.forEach(t=>Ge(t,e,n,r)):Ne(s,t.handler.bind(n),t))}function qe(t){const e=t.type,{__merged:n,mixins:r,extends:i}=e;if(n)return n;const s=t.appContext.mixins;if(!s.length&&!r&&!i)return e;const o={};return s.forEach(e=>Je(o,e,t)),i&&Je(o,i,t),r&&r.forEach(e=>Je(o,e,t)),Je(o,e,t),e.__merged=o}function Je(t,e,n){const r=n.appContext.config.optionMergeStrategies;for(const s in e)r&&Object(i["j"])(r,s)?t[s]=r[s](t[s],e[s],n.proxy,s):Object(i["j"])(t,s)||(t[s]=e[s])}const Ze=Object(i["h"])(Object.create(null),{$:t=>t,$el:t=>t.vnode.el,$data:t=>t.data,$props:t=>t.props,$attrs:t=>t.attrs,$slots:t=>t.slots,$refs:t=>t.refs,$parent:t=>t.parent&&t.parent.proxy,$root:t=>t.root&&t.root.proxy,$emit:t=>t.emit,$options:t=>qe(t),$forceUpdate:t=>()=>A(t.update),$nextTick:()=>T,$watch:t=>Ve.bind(t)}),Xe={get({_:t},e){const{ctx:n,setupState:s,data:o,props:a,accessCache:c,type:u,appContext:l}=t;if("__v_skip"===e)return!0;let h;if("$"!==e[0]){const t=c[e];if(void 0!==t)switch(t){case 0:return s[e];case 1:return o[e];case 3:return n[e];case 2:return a[e]}else{if(s!==i["b"]&&Object(i["j"])(s,e))return c[e]=0,s[e];if(o!==i["b"]&&Object(i["j"])(o,e))return c[e]=1,o[e];if((h=Ft(u)[0])&&Object(i["j"])(h,e))return c[e]=2,a[e];if(n!==i["b"]&&Object(i["j"])(n,e))return c[e]=3,n[e];c[e]=4}}const f=Ze[e];let d,p;return f?("$attrs"===e&&Object(r["n"])(t,"get",e),f(t)):(d=u.__cssModules)&&(d=d[e])?d:n!==i["b"]&&Object(i["j"])(n,e)?(c[e]=3,n[e]):(p=l.config.globalProperties,Object(i["j"])(p,e)?p[e]:void 0)},set({_:t},e,n){const{data:r,setupState:s,ctx:o}=t;if(s!==i["b"]&&Object(i["j"])(s,e))s[e]=n;else if(r!==i["b"]&&Object(i["j"])(r,e))r[e]=n;else if(e in t.props)return!1;return("$"!==e[0]||!(e.slice(1)in t))&&(o[e]=n,!0)},has({_:{data:t,setupState:e,accessCache:n,ctx:r,type:s,appContext:o}},a){let c;return void 0!==n[a]||t!==i["b"]&&Object(i["j"])(t,a)||e!==i["b"]&&Object(i["j"])(e,a)||(c=Ft(s)[0])&&Object(i["j"])(c,a)||Object(i["j"])(r,a)||Object(i["j"])(Ze,a)||Object(i["j"])(o.config.globalProperties,a)}};const Qe=Object(i["h"])({},Xe,{get(t,e){if(e!==Symbol.unscopables)return Xe.get(t,e,t)},has(t,e){const n="_"!==e[0]&&!Object(i["o"])(e);return n}});const tn=_e();let en=0;function nn(t,e,n){const r=t.type,s=(e?e.appContext:t.appContext)||tn,o={uid:en++,vnode:t,type:r,parent:e,appContext:s,root:null,next:null,subTree:null,update:null,render:null,proxy:null,withProxy:null,effects:null,provides:e?e.provides:Object.create(s.provides),accessCache:null,renderCache:[],ctx:i["b"],data:i["b"],props:i["b"],attrs:i["b"],slots:i["b"],refs:i["b"],setupState:i["b"],setupContext:null,suspense:n,asyncDep:null,asyncResolved:!1,isMounted:!1,isUnmounted:!1,isDeactivated:!1,bc:null,c:null,bm:null,m:null,bu:null,u:null,um:null,bum:null,da:null,a:null,rtg:null,rtc:null,ec:null,emit:null,emitted:null};return o.ctx={_:o},o.root=e?e.root:o,o.emit=Ot.bind(null,o),o}let rn=null;const sn=()=>rn||E,on=t=>{rn=t};let an,cn=!1;function un(t,e=!1){cn=e;const{props:n,children:r,shapeFlag:i}=t.vnode,s=4&i;xt(t,n,s,e),ye(t,r);const o=s?ln(t,e):void 0;return cn=!1,o}function ln(t,e){const n=t.type;t.accessCache={},t.proxy=new Proxy(t.ctx,Xe);const{setup:s}=n;if(s){const n=t.setupContext=s.length>1?dn(t):null;rn=t,Object(r["f"])();const o=f(s,t,0,[t.props,n]);if(Object(r["j"])(),rn=null,Object(i["t"])(o)){if(e)return o.then(e=>{hn(t,e)});t.asyncDep=o}else hn(t,o)}else fn(t)}function hn(t,e,n){Object(i["n"])(e)?t.render=e:Object(i["r"])(e)&&(t.setupState=Object(r["g"])(e)),fn(t)}function fn(t,e){const n=t.type;t.render||(an&&n.template&&!n.render&&(n.render=an(n.template,{isCustomElement:t.appContext.config.isCustomElement,delimiters:n.delimiters})),t.render=n.render||i["d"],t.render._rc&&(t.withProxy=new Proxy(t.ctx,Qe))),rn=t,$e(t,n),rn=null}function dn(t){return{attrs:t.attrs,slots:t.slots,emit:t.emit}}function pn(t){rn&&(rn.effects||(rn.effects=[])).push(t)}const yn=/(?:^|[-_])(\w)/g,bn=t=>t.replace(yn,t=>t.toUpperCase()).replace(/[-_]/g,"");function gn(t,e,n=!1){let r=Object(i["n"])(e)&&e.displayName||e.name;if(!r&&e.__file){const t=e.__file.match(/([^/\\]+)\.vue$/);t&&(r=t[1])}if(!r&&t&&t.parent){const n=t=>{for(const n in t)if(t[n]===e)return n};r=n(t.parent.type.components)||n(t.appContext.components)}return r?bn(r):n?"App":"Anonymous"}function mn(t){const e=Object(r["a"])(t);return pn(e.effect),e}function vn(t){return Object(i["n"])(t)?{setup:t,name:t.name}:t}function _n(t,e,n){return 2===arguments.length?Object(i["r"])(e)&&!Object(i["m"])(e)?lt(e)?yt(t,null,[e]):yt(t,e):yt(t,null,e):(lt(n)&&(n=[n]),yt(t,e,n))}Symbol("");function wn(t,e){let n;if(Object(i["m"])(t)||Object(i["x"])(t)){n=new Array(t.length);for(let r=0,i=t.length;rl){var d,p=u(arguments[l++]),y=h?s(p).concat(h(p)):s(p),b=y.length,g=0;while(b>g)d=y[g++],r&&!f.call(p,d)||(n[d]=p[d])}return n}:l},"65f0":function(t,e,n){var r=n("861d"),i=n("e8b5"),s=n("b622"),o=s("species");t.exports=function(t,e){var n;return i(t)&&(n=t.constructor,"function"!=typeof n||n!==Array&&!i(n.prototype)?r(n)&&(n=n[o],null===n&&(n=void 0)):n=void 0),new(void 0===n?Array:n)(0===e?0:e)}},"69f3":function(t,e,n){var r,i,s,o=n("7f9a"),a=n("da84"),c=n("861d"),u=n("9112"),l=n("5135"),h=n("f772"),f=n("d012"),d=a.WeakMap,p=function(t){return s(t)?i(t):r(t,{})},y=function(t){return function(e){var n;if(!c(e)||(n=i(e)).type!==t)throw TypeError("Incompatible receiver, "+t+" required");return n}};if(o){var b=new d,g=b.get,m=b.has,v=b.set;r=function(t,e){return v.call(b,t,e),e},i=function(t){return g.call(b,t)||{}},s=function(t){return m.call(b,t)}}else{var _=h("state");f[_]=!0,r=function(t,e){return u(t,_,e),e},i=function(t){return l(t,_)?t[_]:{}},s=function(t){return l(t,_)}}t.exports={set:r,get:i,has:s,enforce:p,getterFor:y}},"6eeb":function(t,e,n){var r=n("da84"),i=n("9112"),s=n("5135"),o=n("ce4e"),a=n("8925"),c=n("69f3"),u=c.get,l=c.enforce,h=String(String).split("String");(t.exports=function(t,e,n,a){var c=!!a&&!!a.unsafe,u=!!a&&!!a.enumerable,f=!!a&&!!a.noTargetGet;"function"==typeof n&&("string"!=typeof e||s(n,"name")||i(n,"name",e),l(n).source=h.join("string"==typeof e?e:"")),t!==r?(c?!f&&t[e]&&(u=!0):delete t[e],u?t[e]=n:i(t,e,n)):u?t[e]=n:o(e,n)})(Function.prototype,"toString",(function(){return"function"==typeof this&&u(this).source||a(this)}))},7418:function(t,e){e.f=Object.getOwnPropertySymbols},7839:function(t,e){t.exports=["constructor","hasOwnProperty","isPrototypeOf","propertyIsEnumerable","toLocaleString","toString","valueOf"]},"7b0b":function(t,e,n){var r=n("1d80");t.exports=function(t){return Object(r(t))}},"7c73":function(t,e,n){var r,i=n("825a"),s=n("37e8"),o=n("7839"),a=n("d012"),c=n("1be4"),u=n("cc12"),l=n("f772"),h=">",f="<",d="prototype",p="script",y=l("IE_PROTO"),b=function(){},g=function(t){return f+p+h+t+f+"/"+p+h},m=function(t){t.write(g("")),t.close();var e=t.parentWindow.Object;return t=null,e},v=function(){var t,e=u("iframe"),n="java"+p+":";return e.style.display="none",c.appendChild(e),e.src=String(n),t=e.contentWindow.document,t.open(),t.write(g("document.F=Object")),t.close(),t.F},_=function(){try{r=document.domain&&new ActiveXObject("htmlfile")}catch(e){}_=r?m(r):v();var t=o.length;while(t--)delete _[d][o[t]];return _()};a[y]=!0,t.exports=Object.create||function(t,e){var n;return null!==t?(b[d]=i(t),n=new b,b[d]=null,n[y]=t):n=_(),void 0===e?n:s(n,e)}},"7dd0":function(t,e,n){"use strict";var r=n("23e7"),i=n("9ed3"),s=n("e163"),o=n("d2bb"),a=n("d44e"),c=n("9112"),u=n("6eeb"),l=n("b622"),h=n("c430"),f=n("3f8c"),d=n("ae93"),p=d.IteratorPrototype,y=d.BUGGY_SAFARI_ITERATORS,b=l("iterator"),g="keys",m="values",v="entries",_=function(){return this};t.exports=function(t,e,n,l,d,w,I){i(n,e,l);var S,O,T,A=function(t){if(t===d&&F)return F;if(!y&&t in j)return j[t];switch(t){case g:return function(){return new n(this,t)};case m:return function(){return new n(this,t)};case v:return function(){return new n(this,t)}}return function(){return new n(this)}},x=e+" Iterator",B=!1,j=t.prototype,D=j[b]||j["@@iterator"]||d&&j[d],F=!y&&D||A(d),L="Array"==e&&j.entries||D;if(L&&(S=s(L.call(new t)),p!==Object.prototype&&S.next&&(h||s(S)===p||(o?o(S,p):"function"!=typeof S[b]&&c(S,b,_)),a(S,x,!0,!0),h&&(f[x]=_))),d==m&&D&&D.name!==m&&(B=!0,F=function(){return D.call(this)}),h&&!I||j[b]===F||c(j,b,F),f[e]=F,d)if(O={values:A(m),keys:w?F:A(g),entries:A(v)},I)for(T in O)(y||B||!(T in j))&&u(j,T,O[T]);else r({target:e,proto:!0,forced:y||B},O);return O}},"7f9a":function(t,e,n){var r=n("da84"),i=n("8925"),s=r.WeakMap;t.exports="function"===typeof s&&/native code/.test(i(s))},"825a":function(t,e,n){var r=n("861d");t.exports=function(t){if(!r(t))throw TypeError(String(t)+" is not an object");return t}},"830f":function(t,e,n){"use strict";n.d(e,"a",(function(){return Q}));var r=n("9ff4"),i=n("5c40");n("a1e9");const s="http://www.w3.org/2000/svg",o="undefined"!==typeof document?document:null;let a,c;const u={insert:(t,e,n)=>{e.insertBefore(t,n||null)},remove:t=>{const e=t.parentNode;e&&e.removeChild(t)},createElement:(t,e,n)=>e?o.createElementNS(s,t):o.createElement(t,n?{is:n}:void 0),createText:t=>o.createTextNode(t),createComment:t=>o.createComment(t),setText:(t,e)=>{t.nodeValue=e},setElementText:(t,e)=>{t.textContent=e},parentNode:t=>t.parentNode,nextSibling:t=>t.nextSibling,querySelector:t=>o.querySelector(t),setScopeId(t,e){t.setAttribute(e,"")},cloneNode(t){return t.cloneNode(!0)},insertStaticContent(t,e,n,r){const i=r?c||(c=o.createElementNS(s,"svg")):a||(a=o.createElement("div"));i.innerHTML=t;const l=i.firstChild;let h=l,f=h;while(h)f=h,u.insert(h,e,n),h=i.firstChild;return[l,f]}};function l(t,e,n){if(null==e&&(e=""),n)t.setAttribute("class",e);else{const n=t._vtc;n&&(e=(e?[e,...n]:[...n]).join(" ")),t.className=e}}function h(t,e,n){const i=t.style;if(n)if(Object(r["x"])(n))e!==n&&(i.cssText=n);else{for(const t in n)d(i,t,n[t]);if(e&&!Object(r["x"])(e))for(const t in e)null==n[t]&&d(i,t,"")}else t.removeAttribute("style")}const f=/\s*!important$/;function d(t,e,n){if(e.startsWith("--"))t.setProperty(e,n);else{const i=b(t,e);f.test(n)?t.setProperty(Object(r["k"])(i),n.replace(f,""),"important"):t[i]=n}}const p=["Webkit","Moz","ms"],y={};function b(t,e){const n=y[e];if(n)return n;let i=Object(r["e"])(e);if("filter"!==i&&i in t)return y[e]=i;i=Object(r["f"])(i);for(let r=0;rdocument.createEvent("Event").timeStamp&&(_=()=>performance.now());let w=0;const I=Promise.resolve(),S=()=>{w=0},O=()=>w||(I.then(S),w=_());function T(t,e,n,r){t.addEventListener(e,n,r)}function A(t,e,n,r){t.removeEventListener(e,n,r)}function x(t,e,n,r,i=null){const s=n&&n.invoker;if(r&&s)n.invoker=null,s.value=r,r.invoker=s;else{const[n,o]=j(e);r?T(t,n,D(r,i),o):s&&A(t,n,s,o)}}const B=/(?:Once|Passive|Capture)$/;function j(t){let e;if(B.test(t)){let n;e={};while(n=t.match(B))t=t.slice(0,t.length-n[0].length),e[n[0].toLowerCase()]=!0}return[t.slice(2).toLowerCase(),e]}function D(t,e){const n=t=>{const r=t.timeStamp||_();r>=n.attached-1&&Object(i["c"])(F(t,n.value),e,5,[t])};return n.value=t,t.invoker=n,n.attached=O(),n}function F(t,e){if(Object(r["m"])(e)){const n=t.stopImmediatePropagation;return t.stopImmediatePropagation=()=>{n.call(t),t._stopped=!0},e.map(t=>e=>!e._stopped&&t(e))}return e}const L=/^on[a-z]/,E=(t,e)=>"value"===e,M=(t,e,n,i,s=!1,o,a,c,u)=>{switch(e){case"class":l(t,i,s);break;case"style":h(t,n,i);break;default:Object(r["s"])(e)?Object(r["q"])(e)||x(t,e,n,i,a):U(t,e,i,s)?v(t,e,i,o,a,c,u):("true-value"===e?t._trueValue=i:"false-value"===e&&(t._falseValue=i),m(t,e,i,s));break}};function U(t,e,n,i){return i?"innerHTML"===e||!!(e in t&&L.test(e)&&Object(r["n"])(n)):"spellcheck"!==e&&"draggable"!==e&&(("list"!==e||"INPUT"!==t.tagName)&&((!L.test(e)||!Object(r["x"])(n))&&e in t))}const C="transition",N="animation",k=(t,{slots:e})=>Object(i["m"])(i["a"],R(t),e);k.displayName="Transition";const V={name:String,type:String,css:{type:Boolean,default:!0},duration:[String,Number,Object],enterFromClass:String,enterActiveClass:String,enterToClass:String,appearFromClass:String,appearActiveClass:String,appearToClass:String,leaveFromClass:String,leaveActiveClass:String,leaveToClass:String};k.props=Object(r["h"])({},i["a"].props,V);function R(t){let{name:e="v",type:n,css:i=!0,duration:s,enterFromClass:o=e+"-enter-from",enterActiveClass:a=e+"-enter-active",enterToClass:c=e+"-enter-to",appearFromClass:u=o,appearActiveClass:l=a,appearToClass:h=c,leaveFromClass:f=e+"-leave-from",leaveActiveClass:d=e+"-leave-active",leaveToClass:p=e+"-leave-to"}=t;const y={};for(const r in t)r in V||(y[r]=t[r]);if(!i)return y;const b=P(s),g=b&&b[0],m=b&&b[1],{onBeforeEnter:v,onEnter:_,onEnterCancelled:w,onLeave:I,onLeaveCancelled:S,onBeforeAppear:O=v,onAppear:T=_,onAppearCancelled:A=w}=y,x=(t,e,n)=>{Y(t,e?h:c),Y(t,e?l:a),n&&n()},B=(t,e)=>{Y(t,p),Y(t,d),e&&e()},j=t=>(e,r)=>{const i=t?T:_,s=()=>x(e,t,r);i&&i(e,s),W(()=>{Y(e,t?u:o),$(e,t?h:c),i&&i.length>1||(g?setTimeout(s,g):H(e,n,s))})};return Object(r["h"])(y,{onBeforeEnter(t){v&&v(t),$(t,a),$(t,o)},onBeforeAppear(t){O&&O(t),$(t,l),$(t,u)},onEnter:j(!1),onAppear:j(!0),onLeave(t,e){const r=()=>B(t,e);$(t,d),$(t,f),W(()=>{Y(t,f),$(t,p),I&&I.length>1||(m?setTimeout(r,m):H(t,n,r))}),I&&I(t,r)},onEnterCancelled(t){x(t,!1),w&&w(t)},onAppearCancelled(t){x(t,!0),A&&A(t)},onLeaveCancelled(t){B(t),S&&S(t)}})}function P(t){if(null==t)return null;if(Object(r["r"])(t))return[z(t.enter),z(t.leave)];{const e=z(t);return[e,e]}}function z(t){const e=Object(r["G"])(t);return e}function $(t,e){e.split(/\s+/).forEach(e=>e&&t.classList.add(e)),(t._vtc||(t._vtc=new Set)).add(e)}function Y(t,e){e.split(/\s+/).forEach(e=>e&&t.classList.remove(e));const{_vtc:n}=t;n&&(n.delete(e),n.size||(t._vtc=void 0))}function W(t){requestAnimationFrame(()=>{requestAnimationFrame(t)})}function H(t,e,n){const{type:r,timeout:i,propCount:s}=K(t,e);if(!r)return n();const o=r+"end";let a=0;const c=()=>{t.removeEventListener(o,u),n()},u=e=>{e.target===t&&++a>=s&&c()};setTimeout(()=>{a(n[t]||"").split(", "),i=r(C+"Delay"),s=r(C+"Duration"),o=G(i,s),a=r(N+"Delay"),c=r(N+"Duration"),u=G(a,c);let l=null,h=0,f=0;e===C?o>0&&(l=C,h=o,f=s.length):e===N?u>0&&(l=N,h=u,f=c.length):(h=Math.max(o,u),l=h>0?o>u?C:N:null,f=l?l===C?s.length:c.length:0);const d=l===C&&/\b(transform|all)(,|$)/.test(n[C+"Property"]);return{type:l,timeout:h,propCount:f,hasTransform:d}}function G(t,e){while(t.lengthq(e)+q(t[n])))}function q(t){return 1e3*Number(t.slice(0,-1).replace(",","."))}new WeakMap,new WeakMap;const J=Object(r["h"])({patchProp:M,forcePatchProp:E},u);let Z;function X(){return Z||(Z=Object(i["g"])(J))}const Q=(...t)=>{const e=X().createApp(...t);const{mount:n}=e;return e.mount=t=>{const i=tt(t);if(!i)return;const s=e._component;Object(r["n"])(s)||s.render||s.template||(s.template=i.innerHTML),i.innerHTML="";const o=n(i);return i.removeAttribute("v-cloak"),i.setAttribute("data-v-app",""),o},e};function tt(t){if(Object(r["x"])(t)){const e=document.querySelector(t);return e}return t}},"83ab":function(t,e,n){var r=n("d039");t.exports=!r((function(){return 7!=Object.defineProperty({},1,{get:function(){return 7}})[1]}))},8418:function(t,e,n){"use strict";var r=n("c04e"),i=n("9bf2"),s=n("5c6c");t.exports=function(t,e,n){var o=r(e);o in t?i.f(t,o,s(0,n)):t[o]=n}},"861d":function(t,e){t.exports=function(t){return"object"===typeof t?null!==t:"function"===typeof t}},8925:function(t,e,n){var r=n("c6cd"),i=Function.toString;"function"!=typeof r.inspectSource&&(r.inspectSource=function(t){return i.call(t)}),t.exports=r.inspectSource},"90e3":function(t,e){var n=0,r=Math.random();t.exports=function(t){return"Symbol("+String(void 0===t?"":t)+")_"+(++n+r).toString(36)}},9112:function(t,e,n){var r=n("83ab"),i=n("9bf2"),s=n("5c6c");t.exports=r?function(t,e,n){return i.f(t,e,s(1,n))}:function(t,e,n){return t[e]=n,t}},"94ca":function(t,e,n){var r=n("d039"),i=/#|\.prototype\./,s=function(t,e){var n=a[o(t)];return n==u||n!=c&&("function"==typeof e?r(e):!!e)},o=s.normalize=function(t){return String(t).replace(i,".").toLowerCase()},a=s.data={},c=s.NATIVE="N",u=s.POLYFILL="P";t.exports=s},"99af":function(t,e,n){"use strict";var r=n("23e7"),i=n("d039"),s=n("e8b5"),o=n("861d"),a=n("7b0b"),c=n("50c4"),u=n("8418"),l=n("65f0"),h=n("1dde"),f=n("b622"),d=n("2d00"),p=f("isConcatSpreadable"),y=9007199254740991,b="Maximum allowed index exceeded",g=d>=51||!i((function(){var t=[];return t[p]=!1,t.concat()[0]!==t})),m=h("concat"),v=function(t){if(!o(t))return!1;var e=t[p];return void 0!==e?!!e:s(t)},_=!g||!m;r({target:"Array",proto:!0,forced:_},{concat:function(t){var e,n,r,i,s,o=a(this),h=l(o,0),f=0;for(e=-1,r=arguments.length;ey)throw TypeError(b);for(n=0;n=y)throw TypeError(b);u(h,f++,s)}return h.length=f,h}})},"9bdd":function(t,e,n){var r=n("825a");t.exports=function(t,e,n,i){try{return i?e(r(n)[0],n[1]):e(n)}catch(o){var s=t["return"];throw void 0!==s&&r(s.call(t)),o}}},"9bf2":function(t,e,n){var r=n("83ab"),i=n("0cfb"),s=n("825a"),o=n("c04e"),a=Object.defineProperty;e.f=r?a:function(t,e,n){if(s(t),e=o(e,!0),s(n),i)try{return a(t,e,n)}catch(r){}if("get"in n||"set"in n)throw TypeError("Accessors not supported");return"value"in n&&(t[e]=n.value),t}},"9ed3":function(t,e,n){"use strict";var r=n("ae93").IteratorPrototype,i=n("7c73"),s=n("5c6c"),o=n("d44e"),a=n("3f8c"),c=function(){return this};t.exports=function(t,e,n){var u=e+" Iterator";return t.prototype=i(r,{next:s(1,n)}),o(t,u,!1,!0),a[u]=c,t}},"9ff4":function(t,e,n){"use strict";(function(t){function r(t,e){const n=Object.create(null),r=t.split(",");for(let i=0;i!!n[t.toLowerCase()]:t=>!!n[t]}n.d(e,"a",(function(){return S})),n.d(e,"b",(function(){return I})),n.d(e,"c",(function(){return T})),n.d(e,"d",(function(){return O})),n.d(e,"e",(function(){return K})),n.d(e,"f",(function(){return J})),n.d(e,"g",(function(){return Q})),n.d(e,"h",(function(){return j})),n.d(e,"i",(function(){return Z})),n.d(e,"j",(function(){return L})),n.d(e,"k",(function(){return q})),n.d(e,"l",(function(){return X})),n.d(e,"m",(function(){return E})),n.d(e,"n",(function(){return U})),n.d(e,"o",(function(){return s})),n.d(e,"p",(function(){return y})),n.d(e,"q",(function(){return B})),n.d(e,"r",(function(){return k})),n.d(e,"s",(function(){return x})),n.d(e,"t",(function(){return V})),n.d(e,"u",(function(){return Y})),n.d(e,"v",(function(){return b})),n.d(e,"w",(function(){return a})),n.d(e,"x",(function(){return C})),n.d(e,"y",(function(){return N})),n.d(e,"z",(function(){return m})),n.d(e,"A",(function(){return v})),n.d(e,"B",(function(){return r})),n.d(e,"C",(function(){return f})),n.d(e,"D",(function(){return c})),n.d(e,"E",(function(){return D})),n.d(e,"F",(function(){return _})),n.d(e,"G",(function(){return tt})),n.d(e,"H",(function(){return z}));const i="Infinity,undefined,NaN,isFinite,isNaN,parseFloat,parseInt,decodeURI,decodeURIComponent,encodeURI,encodeURIComponent,Math,Number,Date,Array,Object,Boolean,String,RegExp,Map,Set,JSON,Intl",s=r(i);const o="itemscope,allowfullscreen,formnovalidate,ismap,nomodule,novalidate,readonly",a=r(o);function c(t){if(E(t)){const e={};for(let n=0;n{if(t){const n=t.split(l);n.length>1&&(e[n[0].trim()]=n[1].trim())}}),e}function f(t){let e="";if(C(t))e=t;else if(E(t))for(let n=0;nm(t,e))}const _=t=>null==t?"":k(t)?JSON.stringify(t,w,2):String(t),w=(t,e)=>e instanceof Map?{[`Map(${e.size})`]:[...e.entries()].reduce((t,[e,n])=>(t[e+" =>"]=n,t),{})}:e instanceof Set?{[`Set(${e.size})`]:[...e.values()]}:!k(e)||E(e)||$(e)?e:String(e),I={},S=[],O=()=>{},T=()=>!1,A=/^on[^a-z]/,x=t=>A.test(t),B=t=>t.startsWith("onUpdate:"),j=Object.assign,D=(t,e)=>{const n=t.indexOf(e);n>-1&&t.splice(n,1)},F=Object.prototype.hasOwnProperty,L=(t,e)=>F.call(t,e),E=Array.isArray,M=t=>t instanceof Date,U=t=>"function"===typeof t,C=t=>"string"===typeof t,N=t=>"symbol"===typeof t,k=t=>null!==t&&"object"===typeof t,V=t=>k(t)&&U(t.then)&&U(t.catch),R=Object.prototype.toString,P=t=>R.call(t),z=t=>P(t).slice(8,-1),$=t=>"[object Object]"===P(t),Y=r("key,ref,onVnodeBeforeMount,onVnodeMounted,onVnodeBeforeUpdate,onVnodeUpdated,onVnodeBeforeUnmount,onVnodeUnmounted"),W=t=>{const e=Object.create(null);return n=>{const r=e[n];return r||(e[n]=t(n))}},H=/-(\w)/g,K=W(t=>t.replace(H,(t,e)=>e?e.toUpperCase():"")),G=/\B([A-Z])/g,q=W(t=>t.replace(G,"-$1").toLowerCase()),J=W(t=>t.charAt(0).toUpperCase()+t.slice(1)),Z=(t,e)=>t!==e&&(t===t||e===e),X=(t,e)=>{for(let n=0;n{Object.defineProperty(t,e,{configurable:!0,enumerable:!1,value:n})},tt=t=>{const e=parseFloat(t);return isNaN(e)?t:e}}).call(this,n("c8ba"))},a1e9:function(t,e,n){"use strict";n.d(e,"a",(function(){return Ot})),n.d(e,"b",(function(){return l})),n.d(e,"c",(function(){return yt})),n.d(e,"d",(function(){return dt})),n.d(e,"e",(function(){return mt})),n.d(e,"f",(function(){return g})),n.d(e,"g",(function(){return St})),n.d(e,"h",(function(){return ut})),n.d(e,"i",(function(){return vt})),n.d(e,"j",(function(){return v})),n.d(e,"k",(function(){return lt})),n.d(e,"l",(function(){return h})),n.d(e,"m",(function(){return bt})),n.d(e,"n",(function(){return _})),n.d(e,"o",(function(){return w})),n.d(e,"p",(function(){return wt}));var r=n("9ff4");const i=new WeakMap,s=[];let o;const a=Symbol(""),c=Symbol("");function u(t){return t&&!0===t._isEffect}function l(t,e=r["b"]){u(t)&&(t=t.raw);const n=d(t,e);return e.lazy||n(),n}function h(t){t.active&&(p(t),t.options.onStop&&t.options.onStop(),t.active=!1)}let f=0;function d(t,e){const n=function(){if(!n.active)return e.scheduler?void 0:t();if(!s.includes(n)){p(n);try{return m(),s.push(n),o=n,t()}finally{s.pop(),v(),o=s[s.length-1]}}};return n.id=f++,n._isEffect=!0,n.active=!0,n.raw=t,n.deps=[],n.options=e,n}function p(t){const{deps:e}=t;if(e.length){for(let n=0;n{t&&t.forEach(t=>h.add(t))};if("clear"===e)l.forEach(f);else if("length"===n&&Object(r["m"])(t))l.forEach((t,e)=>{("length"===e||e>=s)&&f(t)});else{void 0!==n&&f(l.get(n));const i="add"===e||"delete"===e&&!Object(r["m"])(t);(i||"set"===e&&t instanceof Map)&&f(l.get(Object(r["m"])(t)?"length":a)),i&&t instanceof Map&&f(l.get(c))}const d=t=>{t.options.scheduler?t.options.scheduler(t):t()};h.forEach(d)}const I=new Set(Object.getOwnPropertyNames(Symbol).map(t=>Symbol[t]).filter(r["y"])),S=B(),O=B(!1,!0),T=B(!0),A=B(!0,!0),x={};function B(t=!1,e=!1){return function(n,i,s){if("__v_isReactive"===i)return!t;if("__v_isReadonly"===i)return t;if("__v_raw"===i&&s===(t?n["__v_readonly"]:n["__v_reactive"]))return n;const o=Object(r["m"])(n);if(o&&Object(r["j"])(x,i))return Reflect.get(x,i,s);const a=Reflect.get(n,i,s);return(Object(r["y"])(i)?I.has(i):"__proto__"===i||"__v_isRef"===i)?a:(t||_(n,"get",i),e?a:mt(a)?o?a:a.value:Object(r["r"])(a)?t?ht(a):ut(a):a)}}["includes","indexOf","lastIndexOf"].forEach(t=>{x[t]=function(...e){const n=bt(this);for(let t=0,i=this.length;tObject(r["r"])(t)?ut(t):t),V=t=>Object(r["r"])(t)?ht(t):t,R=t=>t,P=t=>Reflect.getPrototypeOf(t);function z(t,e,n){t=bt(t);const r=bt(e);e!==r&&_(t,"get",e),_(t,"get",r);const{has:i,get:s}=P(t);return i.call(t,e)?n(s.call(t,e)):i.call(t,r)?n(s.call(t,r)):void 0}function $(t){const e=bt(this),n=bt(t);t!==n&&_(e,"has",t),_(e,"has",n);const r=P(e).has;return r.call(e,t)||r.call(e,n)}function Y(t){return t=bt(t),_(t,"iterate",a),Reflect.get(P(t),"size",t)}function W(t){t=bt(t);const e=bt(this),n=P(e),r=n.has.call(e,t),i=n.add.call(e,t);return r||w(e,"add",t,t),i}function H(t,e){e=bt(e);const n=bt(this),{has:i,get:s,set:o}=P(n);let a=i.call(n,t);a||(t=bt(t),a=i.call(n,t));const c=s.call(n,t),u=o.call(n,t,e);return a?Object(r["i"])(e,c)&&w(n,"set",t,e,c):w(n,"add",t,e),u}function K(t){const e=bt(this),{has:n,get:r,delete:i}=P(e);let s=n.call(e,t);s||(t=bt(t),s=n.call(e,t));const o=r?r.call(e,t):void 0,a=i.call(e,t);return s&&w(e,"delete",t,void 0,o),a}function G(){const t=bt(this),e=0!==t.size,n=void 0,r=P(t).clear.call(t);return e&&w(t,"clear",void 0,void 0,n),r}function q(t,e){return function(n,r){const i=this,s=bt(i),o=t?V:e?R:k;function c(t,e){return n.call(r,o(t),o(e),i)}return!t&&_(s,"iterate",a),P(s).forEach.call(s,c)}}function J(t,e,n){return function(...r){const i=bt(this),s=i instanceof Map,o="entries"===t||t===Symbol.iterator&&s,u="keys"===t&&s,l=P(i)[t].apply(i,r),h=e?V:n?R:k;return!e&&_(i,"iterate",u?c:a),{next(){const{value:t,done:e}=l.next();return e?{value:t,done:e}:{value:o?[h(t[0]),h(t[1])]:h(t),done:e}},[Symbol.iterator](){return this}}}}function Z(t){return function(...e){return"delete"!==t&&this}}const X={get(t){return z(this,t,k)},get size(){return Y(this)},has:$,add:W,set:H,delete:K,clear:G,forEach:q(!1,!1)},Q={get(t){return z(this,t,R)},get size(){return Y(this)},has:$,add:W,set:H,delete:K,clear:G,forEach:q(!1,!0)},tt={get(t){return z(this,t,V)},get size(){return Y(this)},has:$,add:Z("add"),set:Z("set"),delete:Z("delete"),clear:Z("clear"),forEach:q(!0,!1)},et=["keys","values","entries",Symbol.iterator];function nt(t,e){const n=e?Q:t?tt:X;return(e,i,s)=>"__v_isReactive"===i?!t:"__v_isReadonly"===i?t:"__v_raw"===i?e:Reflect.get(Object(r["j"])(n,i)&&i in e?n:e,i,s)}et.forEach(t=>{X[t]=J(t,!1,!1),tt[t]=J(t,!0,!1),Q[t]=J(t,!1,!0)});const rt={get:nt(!1,!1)},it={get:nt(!1,!0)},st={get:nt(!0,!1)};const ot=new Set([Set,Map,WeakMap,WeakSet]),at=Object(r["B"])("Object,Array,Map,Set,WeakMap,WeakSet"),ct=t=>!t["__v_skip"]&&at(Object(r["H"])(t))&&!Object.isFrozen(t);function ut(t){return t&&t["__v_isReadonly"]?t:ft(t,!1,U,rt)}function lt(t){return ft(t,!1,N,it)}function ht(t){return ft(t,!0,C,st)}function ft(t,e,n,i){if(!Object(r["r"])(t))return t;if(t["__v_raw"]&&(!e||!t["__v_isReactive"]))return t;const s=e?"__v_readonly":"__v_reactive";if(Object(r["j"])(t,s))return t[s];if(!ct(t))return t;const o=new Proxy(t,ot.has(t.constructor)?i:n);return Object(r["g"])(t,s,o),o}function dt(t){return pt(t)?dt(t["__v_raw"]):!(!t||!t["__v_isReactive"])}function pt(t){return!(!t||!t["__v_isReadonly"])}function yt(t){return dt(t)||pt(t)}function bt(t){return t&&bt(t["__v_raw"])||t}const gt=t=>Object(r["r"])(t)?ut(t):t;function mt(t){return!!t&&!0===t.__v_isRef}function vt(t){return _t(t)}function _t(t,e=!1){if(mt(t))return t;let n=e?t:gt(t);const i={__v_isRef:!0,get value(){return _(i,"get","value"),n},set value(s){Object(r["i"])(bt(s),t)&&(t=s,n=e?s:gt(s),w(i,"set","value",s))}};return i}function wt(t){return mt(t)?t.value:t}const It={get:(t,e,n)=>wt(Reflect.get(t,e,n)),set:(t,e,n,r)=>{const i=t[e];return mt(i)&&!mt(n)?(i.value=n,!0):Reflect.set(t,e,n,r)}};function St(t){return dt(t)?t:new Proxy(t,It)}function Ot(t){let e,n;Object(r["n"])(t)?(e=t,n=r["d"]):(e=t.get,n=t.set);let i,s,o=!0;const a=l(e,{lazy:!0,scheduler:()=>{o||(o=!0,w(s,"set","value"))}});return s={__v_isRef:!0,["__v_isReadonly"]:Object(r["n"])(t)||!t.set,effect:a,get value(){return o&&(i=a(),o=!1),_(s,"get","value"),i},set value(t){n(t)}},s}},a691:function(t,e){var n=Math.ceil,r=Math.floor;t.exports=function(t){return isNaN(t=+t)?0:(t>0?r:n)(t)}},a79d:function(t,e,n){"use strict";var r=n("23e7"),i=n("c430"),s=n("fea9"),o=n("d039"),a=n("d066"),c=n("4840"),u=n("cdf9"),l=n("6eeb"),h=!!s&&o((function(){s.prototype["finally"].call({then:function(){}},(function(){}))}));r({target:"Promise",proto:!0,real:!0,forced:h},{finally:function(t){var e=c(this,a("Promise")),n="function"==typeof t;return this.then(n?function(n){return u(e,t()).then((function(){return n}))}:t,n?function(n){return u(e,t()).then((function(){throw n}))}:t)}}),i||"function"!=typeof s||s.prototype["finally"]||l(s.prototype,"finally",a("Promise").prototype["finally"])},a93d:function(t,e,n){"use strict";
-/** @license React v16.13.1
- * react-is.production.min.js
- *
- * Copyright (c) Facebook, Inc. and its affiliates.
- *
- * This source code is licensed under the MIT license found in the
- * LICENSE file in the root directory of this source tree.
- */var r="function"===typeof Symbol&&Symbol.for,i=r?Symbol.for("react.element"):60103,s=r?Symbol.for("react.portal"):60106,o=r?Symbol.for("react.fragment"):60107,a=r?Symbol.for("react.strict_mode"):60108,c=r?Symbol.for("react.profiler"):60114,u=r?Symbol.for("react.provider"):60109,l=r?Symbol.for("react.context"):60110,h=r?Symbol.for("react.async_mode"):60111,f=r?Symbol.for("react.concurrent_mode"):60111,d=r?Symbol.for("react.forward_ref"):60112,p=r?Symbol.for("react.suspense"):60113,y=r?Symbol.for("react.suspense_list"):60120,b=r?Symbol.for("react.memo"):60115,g=r?Symbol.for("react.lazy"):60116,m=r?Symbol.for("react.block"):60121,v=r?Symbol.for("react.fundamental"):60117,_=r?Symbol.for("react.responder"):60118,w=r?Symbol.for("react.scope"):60119;function I(t){if("object"===typeof t&&null!==t){var e=t.$$typeof;switch(e){case i:switch(t=t.type,t){case h:case f:case o:case c:case a:case p:return t;default:switch(t=t&&t.$$typeof,t){case l:case d:case g:case b:case u:return t;default:return e}}case s:return e}}}function S(t){return I(t)===f}e.AsyncMode=h,e.ConcurrentMode=f,e.ContextConsumer=l,e.ContextProvider=u,e.Element=i,e.ForwardRef=d,e.Fragment=o,e.Lazy=g,e.Memo=b,e.Portal=s,e.Profiler=c,e.StrictMode=a,e.Suspense=p,e.isAsyncMode=function(t){return S(t)||I(t)===h},e.isConcurrentMode=S,e.isContextConsumer=function(t){return I(t)===l},e.isContextProvider=function(t){return I(t)===u},e.isElement=function(t){return"object"===typeof t&&null!==t&&t.$$typeof===i},e.isForwardRef=function(t){return I(t)===d},e.isFragment=function(t){return I(t)===o},e.isLazy=function(t){return I(t)===g},e.isMemo=function(t){return I(t)===b},e.isPortal=function(t){return I(t)===s},e.isProfiler=function(t){return I(t)===c},e.isStrictMode=function(t){return I(t)===a},e.isSuspense=function(t){return I(t)===p},e.isValidElementType=function(t){return"string"===typeof t||"function"===typeof t||t===o||t===f||t===c||t===a||t===p||t===y||"object"===typeof t&&null!==t&&(t.$$typeof===g||t.$$typeof===b||t.$$typeof===u||t.$$typeof===l||t.$$typeof===d||t.$$typeof===v||t.$$typeof===_||t.$$typeof===w||t.$$typeof===m)},e.typeOf=I},ab5b:function(t,e,n){"use strict";t.exports=n("be24")},ab8b:function(t,e,n){},ae40:function(t,e,n){var r=n("83ab"),i=n("d039"),s=n("5135"),o=Object.defineProperty,a={},c=function(t){throw t};t.exports=function(t,e){if(s(a,t))return a[t];e||(e={});var n=[][t],u=!!s(e,"ACCESSORS")&&e.ACCESSORS,l=s(e,0)?e[0]:c,h=s(e,1)?e[1]:void 0;return a[t]=!!n&&!i((function(){if(u&&!r)return!0;var t={length:-1};u?o(t,1,{enumerable:!0,get:c}):t[1]=1,n.call(t,l,h)}))}},ae93:function(t,e,n){"use strict";var r,i,s,o=n("e163"),a=n("9112"),c=n("5135"),u=n("b622"),l=n("c430"),h=u("iterator"),f=!1,d=function(){return this};[].keys&&(s=[].keys(),"next"in s?(i=o(o(s)),i!==Object.prototype&&(r=i)):f=!0),void 0==r&&(r={}),l||c(r,h)||a(r,h,d),t.exports={IteratorPrototype:r,BUGGY_SAFARI_ITERATORS:f}},b575:function(t,e,n){var r,i,s,o,a,c,u,l,h=n("da84"),f=n("06cf").f,d=n("c6b6"),p=n("2cf4").set,y=n("1cdc"),b=h.MutationObserver||h.WebKitMutationObserver,g=h.process,m=h.Promise,v="process"==d(g),_=f(h,"queueMicrotask"),w=_&&_.value;w||(r=function(){var t,e;v&&(t=g.domain)&&t.exit();while(i){e=i.fn,i=i.next;try{e()}catch(n){throw i?o():s=void 0,n}}s=void 0,t&&t.enter()},v?o=function(){g.nextTick(r)}:b&&!y?(a=!0,c=document.createTextNode(""),new b(r).observe(c,{characterData:!0}),o=function(){c.data=a=!a}):m&&m.resolve?(u=m.resolve(void 0),l=u.then,o=function(){l.call(u,r)}):o=function(){p.call(h,r)}),t.exports=w||function(t){var e={fn:t,next:void 0};s&&(s.next=e),i||(i=e,o()),s=e}},b622:function(t,e,n){var r=n("da84"),i=n("5692"),s=n("5135"),o=n("90e3"),a=n("4930"),c=n("fdbf"),u=i("wks"),l=r.Symbol,h=c?l:l&&l.withoutSetter||o;t.exports=function(t){return s(u,t)||(a&&s(l,t)?u[t]=l[t]:u[t]=h("Symbol."+t)),u[t]}},be24:function(t,e,n){"use strict";
-/** @license React v16.13.1
- * react.production.min.js
- *
- * Copyright (c) Facebook, Inc. and its affiliates.
- *
- * This source code is licensed under the MIT license found in the
- * LICENSE file in the root directory of this source tree.
- */var r=n("320c"),i="function"===typeof Symbol&&Symbol.for,s=i?Symbol.for("react.element"):60103,o=i?Symbol.for("react.portal"):60106,a=i?Symbol.for("react.fragment"):60107,c=i?Symbol.for("react.strict_mode"):60108,u=i?Symbol.for("react.profiler"):60114,l=i?Symbol.for("react.provider"):60109,h=i?Symbol.for("react.context"):60110,f=i?Symbol.for("react.forward_ref"):60112,d=i?Symbol.for("react.suspense"):60113,p=i?Symbol.for("react.memo"):60115,y=i?Symbol.for("react.lazy"):60116,b="function"===typeof Symbol&&Symbol.iterator;function g(t){for(var e="https://reactjs.org/docs/error-decoder.html?invariant="+t,n=1;nL.length&&L.push(t)}function U(t,e,n,r){var i=typeof t;"undefined"!==i&&"boolean"!==i||(t=null);var a=!1;if(null===t)a=!0;else switch(i){case"string":case"number":a=!0;break;case"object":switch(t.$$typeof){case s:case o:a=!0}}if(a)return n(r,t,""===e?"."+N(t,0):e),1;if(a=0,e=""===e?".":e+":",Array.isArray(t))for(var c=0;cc)r(a,n=e[c++])&&(~s(u,n)||u.push(n));return u}},cc12:function(t,e,n){var r=n("da84"),i=n("861d"),s=r.document,o=i(s)&&i(s.createElement);t.exports=function(t){return o?s.createElement(t):{}}},cca6:function(t,e,n){var r=n("23e7"),i=n("60da");r({target:"Object",stat:!0,forced:Object.assign!==i},{assign:i})},cd74:function(t,e,n){},cdf9:function(t,e,n){var r=n("825a"),i=n("861d"),s=n("f069");t.exports=function(t,e){if(r(t),i(e)&&e.constructor===t)return e;var n=s.f(t),o=n.resolve;return o(e),n.promise}},ce4e:function(t,e,n){var r=n("da84"),i=n("9112");t.exports=function(t,e){try{i(r,t,e)}catch(n){r[t]=e}return e}},d012:function(t,e){t.exports={}},d039:function(t,e){t.exports=function(t){try{return!!t()}catch(e){return!0}}},d066:function(t,e,n){var r=n("428f"),i=n("da84"),s=function(t){return"function"==typeof t?t:void 0};t.exports=function(t,e){return arguments.length<2?s(r[t])||s(i[t]):r[t]&&r[t][e]||i[t]&&i[t][e]}},d092:function(t,e,n){"use strict";n.d(e,"a",(function(){return Vh}));var r={};n.r(r),n.d(r,"memcpy",(function(){return jt})),n.d(r,"joinUint8Arrays",(function(){return Dt})),n.d(r,"toArrayBufferView",(function(){return Ft})),n.d(r,"toInt8Array",(function(){return Lt})),n.d(r,"toInt16Array",(function(){return Et})),n.d(r,"toInt32Array",(function(){return Mt})),n.d(r,"toBigInt64Array",(function(){return Ut})),n.d(r,"toUint8Array",(function(){return Ct})),n.d(r,"toUint16Array",(function(){return Nt})),n.d(r,"toUint32Array",(function(){return kt})),n.d(r,"toBigUint64Array",(function(){return Vt})),n.d(r,"toFloat32Array",(function(){return Rt})),n.d(r,"toFloat64Array",(function(){return Pt})),n.d(r,"toUint8ClampedArray",(function(){return zt})),n.d(r,"toArrayBufferViewIterator",(function(){return Yt})),n.d(r,"toInt8ArrayIterator",(function(){return Wt})),n.d(r,"toInt16ArrayIterator",(function(){return Ht})),n.d(r,"toInt32ArrayIterator",(function(){return Kt})),n.d(r,"toUint8ArrayIterator",(function(){return Gt})),n.d(r,"toUint16ArrayIterator",(function(){return qt})),n.d(r,"toUint32ArrayIterator",(function(){return Jt})),n.d(r,"toFloat32ArrayIterator",(function(){return Zt})),n.d(r,"toFloat64ArrayIterator",(function(){return Xt})),n.d(r,"toUint8ClampedArrayIterator",(function(){return Qt})),n.d(r,"toArrayBufferViewAsyncIterator",(function(){return te})),n.d(r,"toInt8ArrayAsyncIterator",(function(){return ee})),n.d(r,"toInt16ArrayAsyncIterator",(function(){return ne})),n.d(r,"toInt32ArrayAsyncIterator",(function(){return re})),n.d(r,"toUint8ArrayAsyncIterator",(function(){return ie})),n.d(r,"toUint16ArrayAsyncIterator",(function(){return se})),n.d(r,"toUint32ArrayAsyncIterator",(function(){return oe})),n.d(r,"toFloat32ArrayAsyncIterator",(function(){return ae})),n.d(r,"toFloat64ArrayAsyncIterator",(function(){return ce})),n.d(r,"toUint8ClampedArrayAsyncIterator",(function(){return ue})),n.d(r,"rebaseValueOffsets",(function(){return le})),n.d(r,"compareArrayLike",(function(){return he}));var i={};n.r(i),n.d(i,"getBool",(function(){return Ee})),n.d(i,"getBit",(function(){return Me})),n.d(i,"setBool",(function(){return Ue})),n.d(i,"truncateBitmap",(function(){return Ce})),n.d(i,"packBools",(function(){return Ne})),n.d(i,"iterateBits",(function(){return ke})),n.d(i,"popcnt_bit_range",(function(){return Ve})),n.d(i,"popcnt_array",(function(){return Re})),n.d(i,"popcnt_uint32",(function(){return Pe}));var s={};n.r(s),n.d(s,"uint16ToFloat64",(function(){return gr})),n.d(s,"float64ToUint16",(function(){return mr}));var o={};n.r(o),n.d(o,"isArrowBigNumSymbol",(function(){return Sr})),n.d(o,"bignumToString",(function(){return jr})),n.d(o,"bignumToBigInt",(function(){return Dr})),n.d(o,"BN",(function(){return Lr}));var a={};n.r(a),n.d(a,"clampIndex",(function(){return yi})),n.d(a,"clampRange",(function(){return gi})),n.d(a,"createElementComparator",(function(){return _i}));var c={};n.r(c),n.d(c,"BaseInt64",(function(){return mo})),n.d(c,"Uint64",(function(){return vo})),n.d(c,"Int64",(function(){return _o})),n.d(c,"Int128",(function(){return wo}));n("da6a");var u=n("ab5b"),l=n.n(u);const h=new WeakMap,f=new WeakMap;function d(t){const e=h.get(t);return console.assert(null!=e,"'this' is expected an Event object, but got",t),e}function p(t){null==t.passiveListener?t.event.cancelable&&(t.canceled=!0,"function"===typeof t.event.preventDefault&&t.event.preventDefault()):"undefined"!==typeof console&&"function"===typeof console.error&&console.error("Unable to preventDefault inside passive event listener invocation.",t.passiveListener)}function y(t,e){h.set(this,{eventTarget:t,event:e,eventPhase:2,currentTarget:t,canceled:!1,stopped:!1,immediateStopped:!1,passiveListener:null,timeStamp:e.timeStamp||Date.now()}),Object.defineProperty(this,"isTrusted",{value:!1,enumerable:!0});const n=Object.keys(e);for(let r=0;r0){const t=new Array(arguments.length);for(let e=0;e57343)i.push(s);else if(56320<=s&&s<=57343)i.push(65533);else if(55296<=s&&s<=56319)if(r===n-1)i.push(65533);else{var o=t.charCodeAt(r+1);if(56320<=o&&o<=57343){var a=1023&s,c=1023&o;i.push(65536+(a<<10)+c),r+=1}else i.push(65533)}r+=1}return i}function V(t){for(var e="",n=0;n>10),56320+(1023&r)))}return e}U.Offset,U.Table,U.SIZEOF_SHORT=2,U.SIZEOF_INT=4,U.FILE_IDENTIFIER_LENGTH=4,U.Encoding={UTF8_BYTES:1,UTF16_STRING:2},U.int32=new Int32Array(2),U.float32=new Float32Array(U.int32.buffer),U.float64=new Float64Array(U.int32.buffer),U.isLittleEndian=1===new Uint16Array(new Uint8Array([1,0]).buffer)[0],U.Long=function(t,e){this.low=0|t,this.high=0|e},U.Long.create=function(t,e){return 0==t&&0==e?U.Long.ZERO:new U.Long(t,e)},U.Long.prototype.toFloat64=function(){return(this.low>>>0)+4294967296*this.high},U.Long.prototype.equals=function(t){return this.low==t.low&&this.high==t.high},U.Long.ZERO=new U.Long(0,0),U.Builder=function(t){if(t)e=t;else var e=1024;this.bb=U.ByteBuffer.allocate(e),this.space=e,this.minalign=1,this.vtable=null,this.vtable_in_use=0,this.isNested=!1,this.object_start=0,this.vtables=[],this.vector_num_elems=0,this.force_defaults=!1},U.Builder.prototype.clear=function(){this.bb.clear(),this.space=this.bb.capacity(),this.minalign=1,this.vtable=null,this.vtable_in_use=0,this.isNested=!1,this.object_start=0,this.vtables=[],this.vector_num_elems=0,this.force_defaults=!1},U.Builder.prototype.forceDefaults=function(t){this.force_defaults=t},U.Builder.prototype.dataBuffer=function(){return this.bb},U.Builder.prototype.asUint8Array=function(){return this.bb.bytes().subarray(this.bb.position(),this.bb.position()+this.offset())},U.Builder.prototype.prep=function(t,e){t>this.minalign&&(this.minalign=t);var n=1+~(this.bb.capacity()-this.space+e)&t-1;while(this.space=0&&0==this.vtable[e];e--);for(var n=e+1;e>=0;e--)this.addInt16(0!=this.vtable[e]?t-this.vtable[e]:0);var r=2;this.addInt16(t-this.object_start);var i=(n+r)*U.SIZEOF_SHORT;this.addInt16(i);var s=0,o=this.space;t:for(e=0;e=0;r--)this.writeInt8(n.charCodeAt(r))}this.prep(this.minalign,U.SIZEOF_INT),this.addOffset(t),this.bb.setPosition(this.space)},U.Builder.prototype.requiredField=function(t,e){var n=this.bb.capacity()-t,r=n-this.bb.readInt32(n),i=0!=this.bb.readInt16(r+e);if(!i)throw new Error("FlatBuffers: field "+e+" must be set")},U.Builder.prototype.startVector=function(t,e,n){this.notNested(),this.vector_num_elems=e,this.prep(U.SIZEOF_INT,t*e),this.prep(n,t*e)},U.Builder.prototype.endVector=function(){return this.writeInt32(this.vector_num_elems),this.offset()},U.Builder.prototype.createString=function(t){if(t instanceof Uint8Array)var e=t;else{e=[];var n=0;while(n=56320)r=i;else{var s=t.charCodeAt(n++);r=(i<<10)+s+-56613888}r<128?e.push(r):(r<2048?e.push(r>>6&31|192):(r<65536?e.push(r>>12&15|224):e.push(r>>18&7|240,r>>12&63|128),e.push(r>>6&63|128)),e.push(63&r|128))}}this.addInt8(0),this.startVector(1,e.length,1),this.bb.setPosition(this.space-=e.length);n=0;for(var o=this.space,a=this.bb.bytes();n>24},U.ByteBuffer.prototype.readUint8=function(t){return this.bytes_[t]},U.ByteBuffer.prototype.readInt16=function(t){return this.readUint16(t)<<16>>16},U.ByteBuffer.prototype.readUint16=function(t){return this.bytes_[t]|this.bytes_[t+1]<<8},U.ByteBuffer.prototype.readInt32=function(t){return this.bytes_[t]|this.bytes_[t+1]<<8|this.bytes_[t+2]<<16|this.bytes_[t+3]<<24},U.ByteBuffer.prototype.readUint32=function(t){return this.readInt32(t)>>>0},U.ByteBuffer.prototype.readInt64=function(t){return new U.Long(this.readInt32(t),this.readInt32(t+4))},U.ByteBuffer.prototype.readUint64=function(t){return new U.Long(this.readUint32(t),this.readUint32(t+4))},U.ByteBuffer.prototype.readFloat32=function(t){return U.int32[0]=this.readInt32(t),U.float32[0]},U.ByteBuffer.prototype.readFloat64=function(t){return U.int32[U.isLittleEndian?0:1]=this.readInt32(t),U.int32[U.isLittleEndian?1:0]=this.readInt32(t+4),U.float64[0]},U.ByteBuffer.prototype.writeInt8=function(t,e){this.bytes_[t]=e},U.ByteBuffer.prototype.writeUint8=function(t,e){this.bytes_[t]=e},U.ByteBuffer.prototype.writeInt16=function(t,e){this.bytes_[t]=e,this.bytes_[t+1]=e>>8},U.ByteBuffer.prototype.writeUint16=function(t,e){this.bytes_[t]=e,this.bytes_[t+1]=e>>8},U.ByteBuffer.prototype.writeInt32=function(t,e){this.bytes_[t]=e,this.bytes_[t+1]=e>>8,this.bytes_[t+2]=e>>16,this.bytes_[t+3]=e>>24},U.ByteBuffer.prototype.writeUint32=function(t,e){this.bytes_[t]=e,this.bytes_[t+1]=e>>8,this.bytes_[t+2]=e>>16,this.bytes_[t+3]=e>>24},U.ByteBuffer.prototype.writeInt64=function(t,e){this.writeInt32(t,e.low),this.writeInt32(t+4,e.high)},U.ByteBuffer.prototype.writeUint64=function(t,e){this.writeUint32(t,e.low),this.writeUint32(t+4,e.high)},U.ByteBuffer.prototype.writeFloat32=function(t,e){U.float32[0]=e,this.writeInt32(t,U.int32[0])},U.ByteBuffer.prototype.writeFloat64=function(t,e){U.float64[0]=e,this.writeInt32(t,U.int32[U.isLittleEndian?0:1]),this.writeInt32(t+4,U.int32[U.isLittleEndian?1:0])},U.ByteBuffer.prototype.getBufferIdentifier=function(){if(this.bytes_.length>10),56320+(1023&s)))}return r},U.ByteBuffer.prototype.__indirect=function(t){return t+this.readInt32(t)},U.ByteBuffer.prototype.__vector=function(t){return t+this.readInt32(t)+U.SIZEOF_INT},U.ByteBuffer.prototype.__vector_len=function(t){return this.readInt32(t+this.readInt32(t))},U.ByteBuffer.prototype.__has_identifier=function(t){if(t.length!=U.FILE_IDENTIFIER_LENGTH)throw new Error("FlatBuffers: file identifier must be length "+U.FILE_IDENTIFIER_LENGTH);for(var e=0;e>6*n)+r];while(n>0){var s=e>>6*(n-1);i.push(128|63&s),n-=1}return i}}K.prototype={decode:function(t,e){var n;n="object"===typeof t&&t instanceof ArrayBuffer?new Uint8Array(t):"object"===typeof t&&"buffer"in t&&t.buffer instanceof ArrayBuffer?new Uint8Array(t.buffer,t.byteOffset,t.byteLength):new Uint8Array(0),e=N(e),this._streaming||(this._decoder=new q({fatal:this._fatal}),this._BOMseen=!1),this._streaming=Boolean(e["stream"]);var r,i=new P(n),s=[];while(!i.endOfStream()){if(r=this._decoder.handler(i,i.read()),r===z)break;null!==r&&(Array.isArray(r)?s.push.apply(s,r):s.push(r))}if(!this._streaming){do{if(r=this._decoder.handler(i,i.read()),r===z)break;null!==r&&(Array.isArray(r)?s.push.apply(s,r):s.push(r))}while(!i.endOfStream());this._decoder=null}return s.length&&(-1===["utf-8"].indexOf(this.encoding)||this._ignoreBOM||this._BOMseen||(65279===s[0]?(this._BOMseen=!0,s.shift()):this._BOMseen=!0)),V(s)}},G.prototype={encode:function(t,e){t=t?String(t):"",e=N(e),this._streaming||(this._encoder=new J(this._options)),this._streaming=Boolean(e["stream"]);var n,r=[],i=new P(k(t));while(!i.endOfStream()){if(n=this._encoder.handler(i,i.read()),n===z)break;Array.isArray(n)?r.push.apply(r,n):r.push(n)}if(!this._streaming){while(1){if(n=this._encoder.handler(i,i.read()),n===z)break;Array.isArray(n)?r.push.apply(r,n):r.push(n)}this._encoder=null}return new Uint8Array(r)}};const Z="function"===typeof Buffer?Buffer:null,X="function"===typeof TextDecoder&&"function"===typeof TextEncoder,Q=(t=>{if(X||!Z){const e=new t("utf-8");return t=>e.decode(t)}return t=>{const{buffer:e,byteOffset:n,length:r}=Ct(t);return Z.from(e,n,r).toString()}})("undefined"!==typeof TextDecoder?TextDecoder:K),tt=(t=>{if(X||!Z){const e=new t;return t=>e.encode(t)}return(t="")=>Ct(Z.from(t,"utf8"))})("undefined"!==typeof TextEncoder?TextEncoder:G),et=Object.freeze({done:!0,value:void 0});class nt{constructor(t){this._json=t}get schema(){return this._json["schema"]}get batches(){return this._json["batches"]||[]}get dictionaries(){return this._json["dictionaries"]||[]}}class rt{tee(){return this._getDOMStream().tee()}pipe(t,e){return this._getNodeStream().pipe(t,e)}pipeTo(t,e){return this._getDOMStream().pipeTo(t,e)}pipeThrough(t,e){return this._getDOMStream().pipeThrough(t,e)}_getDOMStream(){return this._DOMStream||(this._DOMStream=this.toDOMStream())}_getNodeStream(){return this._nodeStream||(this._nodeStream=this.toNodeStream())}}class it extends rt{constructor(){super(),this._values=[],this.resolvers=[],this._closedPromise=new Promise(t=>this._closedPromiseResolve=t)}get closed(){return this._closedPromise}async cancel(t){await this.return(t)}write(t){this._ensureOpen()&&(this.resolvers.length<=0?this._values.push(t):this.resolvers.shift().resolve({done:!1,value:t}))}abort(t){this._closedPromiseResolve&&(this.resolvers.length<=0?this._error={error:t}:this.resolvers.shift().reject({done:!0,value:t}))}close(){if(this._closedPromiseResolve){const{resolvers:t}=this;while(t.length>0)t.shift().resolve(et);this._closedPromiseResolve(),this._closedPromiseResolve=void 0}}[Symbol.asyncIterator](){return this}toDOMStream(t){return fe.toDOMStream(this._closedPromiseResolve||this._error?this:this._values,t)}toNodeStream(t){return fe.toNodeStream(this._closedPromiseResolve||this._error?this:this._values,t)}async throw(t){return await this.abort(t),et}async return(t){return await this.close(),et}async read(t){return(await this.next(t,"read")).value}async peek(t){return(await this.next(t,"peek")).value}next(...t){return this._values.length>0?Promise.resolve({done:!1,value:this._values.shift()}):this._error?Promise.reject({done:!0,value:this._error.error}):this._closedPromiseResolve?new Promise((t,e)=>{this.resolvers.push({resolve:t,reject:e})}):Promise.resolve(et)}_ensureOpen(){if(this._closedPromiseResolve)return!0;throw new Error(this+" is closed")}}const[st,ot]=(()=>{const t=()=>{throw new Error("BigInt is not available in this environment")};function e(){throw t()}return e.asIntN=()=>{throw t()},e.asUintN=()=>{throw t()},"undefined"!==typeof BigInt?[BigInt,!0]:[e,!1]})(),[at,ct]=(()=>{const t=()=>{throw new Error("BigInt64Array is not available in this environment")};class e{static get BYTES_PER_ELEMENT(){return 8}static of(){throw t()}static from(){throw t()}constructor(){throw t()}}return"undefined"!==typeof BigInt64Array?[BigInt64Array,!0]:[e,!1]})(),[ut,lt]=(()=>{const t=()=>{throw new Error("BigUint64Array is not available in this environment")};class e{static get BYTES_PER_ELEMENT(){return 8}static of(){throw t()}static from(){throw t()}constructor(){throw t()}}return"undefined"!==typeof BigUint64Array?[BigUint64Array,!0]:[e,!1]})(),ht=t=>"number"===typeof t,ft=t=>"boolean"===typeof t,dt=t=>"function"===typeof t,pt=t=>null!=t&&Object(t)===t,yt=t=>pt(t)&&dt(t.then),bt=t=>pt(t)&&dt(t[Symbol.iterator]),gt=t=>pt(t)&&dt(t[Symbol.asyncIterator]),mt=t=>pt(t)&&pt(t["schema"]),vt=t=>pt(t)&&"done"in t&&"value"in t,_t=t=>pt(t)&&dt(t["stat"])&&ht(t["fd"]),wt=t=>pt(t)&&St(t["body"]),It=t=>pt(t)&&dt(t["abort"])&&dt(t["getWriter"])&&!(t instanceof rt),St=t=>pt(t)&&dt(t["cancel"])&&dt(t["getReader"])&&!(t instanceof rt),Ot=t=>pt(t)&&dt(t["end"])&&dt(t["write"])&&ft(t["writable"])&&!(t instanceof rt),Tt=t=>pt(t)&&dt(t["read"])&&dt(t["pipe"])&&ft(t["readable"])&&!(t instanceof rt);var At=U.ByteBuffer;const xt="undefined"!==typeof SharedArrayBuffer?SharedArrayBuffer:ArrayBuffer;function Bt(t){let e,n,r,i,s=t[0]?[t[0]]:[];for(let o,a,c=0,u=0,l=t.length;++ct+e.byteLength,0),a=0,c=-1,u=Math.min(e||1/0,o);for(let l=s.length;++cFt(Int8Array,t),Et=t=>Ft(Int16Array,t),Mt=t=>Ft(Int32Array,t),Ut=t=>Ft(at,t),Ct=t=>Ft(Uint8Array,t),Nt=t=>Ft(Uint16Array,t),kt=t=>Ft(Uint32Array,t),Vt=t=>Ft(ut,t),Rt=t=>Ft(Float32Array,t),Pt=t=>Ft(Float64Array,t),zt=t=>Ft(Uint8ClampedArray,t),$t=t=>(t.next(),t);function*Yt(t,e){const n=function*(t){yield t},r="string"===typeof e||ArrayBuffer.isView(e)||e instanceof ArrayBuffer||e instanceof xt?n(e):bt(e)?e:n(e);yield*$t(function*(e){let n=null;do{n=e.next(yield Ft(t,n))}while(!n.done)}(r[Symbol.iterator]()))}const Wt=t=>Yt(Int8Array,t),Ht=t=>Yt(Int16Array,t),Kt=t=>Yt(Int32Array,t),Gt=t=>Yt(Uint8Array,t),qt=t=>Yt(Uint16Array,t),Jt=t=>Yt(Uint32Array,t),Zt=t=>Yt(Float32Array,t),Xt=t=>Yt(Float64Array,t),Qt=t=>Yt(Uint8ClampedArray,t);async function*te(t,e){if(yt(e))return yield*te(t,await e);const n=async function*(t){yield await t},r=async function*(t){yield*$t(function*(t){let e=null;do{e=t.next(yield e&&e.value)}while(!e.done)}(t[Symbol.iterator]()))},i="string"===typeof e||ArrayBuffer.isView(e)||e instanceof ArrayBuffer||e instanceof xt?n(e):bt(e)?r(e):gt(e)?e:n(e);yield*$t(async function*(e){let n=null;do{n=await e.next(yield Ft(t,n))}while(!n.done)}(i[Symbol.asyncIterator]()))}const ee=t=>te(Int8Array,t),ne=t=>te(Int16Array,t),re=t=>te(Int32Array,t),ie=t=>te(Uint8Array,t),se=t=>te(Uint16Array,t),oe=t=>te(Uint32Array,t),ae=t=>te(Float32Array,t),ce=t=>te(Float64Array,t),ue=t=>te(Uint8ClampedArray,t);function le(t,e,n){if(0!==t){n=n.slice(0,e+1);for(let r=-1;++r<=e;)n[r]+=t}return n}function he(t,e){let n=0,r=t.length;if(r!==e.length)return!1;if(r>0)do{if(t[n]!==e[n])return!1}while(++n(t.next(),t);function*pe(t){let e,n,r,i,s=!1,o=[],a=0;function c(){return"peek"===r?Dt(o,i)[0]:([n,o,a]=Dt(o,i),n)}({cmd:r,size:i}=yield null);let u=Gt(t)[Symbol.iterator]();try{do{if(({done:e,value:n}=isNaN(i-a)?u.next(void 0):u.next(i-a)),!e&&n.byteLength>0&&(o.push(n),a+=n.byteLength),e||i<=a)do{({cmd:r,size:i}=yield c())}while(i0&&(o.push(n),a+=n.byteLength),e||i<=a)do{({cmd:r,size:i}=yield c())}while(i0&&(o.push(Ct(e)),a+=e.byteLength),i||r<=a)do{({cmd:n,size:r}=yield c())}while(r{}):Promise.resolve()}releaseLock(){this.reader&&this.reader.releaseLock(),this.reader=this.byobReader=this.defaultReader=null}async cancel(t){const{reader:e,source:n}=this;e&&await e["cancel"](t).catch(()=>{}),n&&n["locked"]&&this.releaseLock()}async read(t){if(0===t)return{done:null==this.reader,value:new Uint8Array(0)};const e=this.supportsBYOB&&"number"===typeof t?await this.readFromBYOBReader(t):await this.getDefaultReader().read();return!e.done&&(e.value=Ct(e)),e}getDefaultReader(){return this.byobReader&&this.releaseLock(),this.defaultReader||(this.defaultReader=this.source["getReader"](),this.defaultReader["closed"].catch(()=>{})),this.reader=this.defaultReader}getBYOBReader(){return this.defaultReader&&this.releaseLock(),this.byobReader||(this.byobReader=this.source["getReader"]({mode:"byob"}),this.byobReader["closed"].catch(()=>{})),this.reader=this.byobReader}async readFromBYOBReader(t){return await me(this.getBYOBReader(),new ArrayBuffer(t),0,t)}}async function me(t,e,n,r){if(n>=r)return{done:!1,value:new Uint8Array(e,0,r)};const{done:i,value:s}=await t.read(new Uint8Array(e,n,r-n));return(n+=s.byteLength){let n,r=t=>n([e,t]);return[e,r,new Promise(i=>(n=i)&&t["once"](e,r))]};async function*_e(t){let e,n,r,i=[],s="error",o=!1,a=null,c=0,u=[];function l(){return"peek"===e?Dt(u,n)[0]:([r,u,c]=Dt(u,n),r)}if(({cmd:e,size:n}=yield null),t["isTTY"])return yield new Uint8Array(0);try{i[0]=ve(t,"end"),i[1]=ve(t,"error");do{if(i[2]=ve(t,"readable"),[s,a]=await Promise.race(i.map(t=>t[2])),"error"===s)break;if((o="end"===s)||(isFinite(n-c)?(r=Ct(t["read"](n-c)),r.byteLength0&&(u.push(r),c+=r.byteLength)),o||n<=c)do{({cmd:e,size:n}=yield l())}while(n{for(const[n,o]of e)t["off"](n,o);try{const e=t["destroy"];e&&e.call(t,n),n=void 0}catch(s){n=s||n}finally{null!=n?i(n):r()}})}}class we{}var Ie,Se;(function(t){(function(t){(function(t){(function(t){let e;(function(t){t[t["V1"]=0]="V1",t[t["V2"]=1]="V2",t[t["V3"]=2]="V3",t[t["V4"]=3]="V4"})(e=t.MetadataVersion||(t.MetadataVersion={}))})(t.flatbuf||(t.flatbuf={}))})(t.arrow||(t.arrow={}))})(t.apache||(t.apache={}))})(Ie||(Ie={})),function(t){(function(t){(function(t){(function(t){let e;(function(t){t[t["Sparse"]=0]="Sparse",t[t["Dense"]=1]="Dense"})(e=t.UnionMode||(t.UnionMode={}))})(t.flatbuf||(t.flatbuf={}))})(t.arrow||(t.arrow={}))})(t.apache||(t.apache={}))}(Ie||(Ie={})),function(t){(function(t){(function(t){(function(t){let e;(function(t){t[t["HALF"]=0]="HALF",t[t["SINGLE"]=1]="SINGLE",t[t["DOUBLE"]=2]="DOUBLE"})(e=t.Precision||(t.Precision={}))})(t.flatbuf||(t.flatbuf={}))})(t.arrow||(t.arrow={}))})(t.apache||(t.apache={}))}(Ie||(Ie={})),function(t){(function(t){(function(t){(function(t){let e;(function(t){t[t["DAY"]=0]="DAY",t[t["MILLISECOND"]=1]="MILLISECOND"})(e=t.DateUnit||(t.DateUnit={}))})(t.flatbuf||(t.flatbuf={}))})(t.arrow||(t.arrow={}))})(t.apache||(t.apache={}))}(Ie||(Ie={})),function(t){(function(t){(function(t){(function(t){let e;(function(t){t[t["SECOND"]=0]="SECOND",t[t["MILLISECOND"]=1]="MILLISECOND",t[t["MICROSECOND"]=2]="MICROSECOND",t[t["NANOSECOND"]=3]="NANOSECOND"})(e=t.TimeUnit||(t.TimeUnit={}))})(t.flatbuf||(t.flatbuf={}))})(t.arrow||(t.arrow={}))})(t.apache||(t.apache={}))}(Ie||(Ie={})),function(t){(function(t){(function(t){(function(t){let e;(function(t){t[t["YEAR_MONTH"]=0]="YEAR_MONTH",t[t["DAY_TIME"]=1]="DAY_TIME"})(e=t.IntervalUnit||(t.IntervalUnit={}))})(t.flatbuf||(t.flatbuf={}))})(t.arrow||(t.arrow={}))})(t.apache||(t.apache={}))}(Ie||(Ie={})),function(t){(function(t){(function(t){(function(t){let e;(function(t){t[t["NONE"]=0]="NONE",t[t["Null"]=1]="Null",t[t["Int"]=2]="Int",t[t["FloatingPoint"]=3]="FloatingPoint",t[t["Binary"]=4]="Binary",t[t["Utf8"]=5]="Utf8",t[t["Bool"]=6]="Bool",t[t["Decimal"]=7]="Decimal",t[t["Date"]=8]="Date",t[t["Time"]=9]="Time",t[t["Timestamp"]=10]="Timestamp",t[t["Interval"]=11]="Interval",t[t["List"]=12]="List",t[t["Struct_"]=13]="Struct_",t[t["Union"]=14]="Union",t[t["FixedSizeBinary"]=15]="FixedSizeBinary",t[t["FixedSizeList"]=16]="FixedSizeList",t[t["Map"]=17]="Map",t[t["Duration"]=18]="Duration",t[t["LargeBinary"]=19]="LargeBinary",t[t["LargeUtf8"]=20]="LargeUtf8",t[t["LargeList"]=21]="LargeList"})(e=t.Type||(t.Type={}))})(t.flatbuf||(t.flatbuf={}))})(t.arrow||(t.arrow={}))})(t.apache||(t.apache={}))}(Ie||(Ie={})),function(t){(function(t){(function(t){(function(t){let e;(function(t){t[t["Little"]=0]="Little",t[t["Big"]=1]="Big"})(e=t.Endianness||(t.Endianness={}))})(t.flatbuf||(t.flatbuf={}))})(t.arrow||(t.arrow={}))})(t.apache||(t.apache={}))}(Ie||(Ie={})),function(t){(function(t){(function(t){(function(t){class e{constructor(){this.bb=null,this.bb_pos=0}__init(t,e){return this.bb_pos=t,this.bb=e,this}static getRootAsNull(t,n){return(n||new e).__init(t.readInt32(t.position())+t.position(),t)}static startNull(t){t.startObject(0)}static endNull(t){let e=t.endObject();return e}static createNull(t){return e.startNull(t),e.endNull(t)}}t.Null=e})(t.flatbuf||(t.flatbuf={}))})(t.arrow||(t.arrow={}))})(t.apache||(t.apache={}))}(Ie||(Ie={})),function(t){(function(t){(function(t){(function(t){class e{constructor(){this.bb=null,this.bb_pos=0}__init(t,e){return this.bb_pos=t,this.bb=e,this}static getRootAsStruct_(t,n){return(n||new e).__init(t.readInt32(t.position())+t.position(),t)}static startStruct_(t){t.startObject(0)}static endStruct_(t){let e=t.endObject();return e}static createStruct_(t){return e.startStruct_(t),e.endStruct_(t)}}t.Struct_=e})(t.flatbuf||(t.flatbuf={}))})(t.arrow||(t.arrow={}))})(t.apache||(t.apache={}))}(Ie||(Ie={})),function(t){(function(t){(function(t){(function(t){class e{constructor(){this.bb=null,this.bb_pos=0}__init(t,e){return this.bb_pos=t,this.bb=e,this}static getRootAsList(t,n){return(n||new e).__init(t.readInt32(t.position())+t.position(),t)}static startList(t){t.startObject(0)}static endList(t){let e=t.endObject();return e}static createList(t){return e.startList(t),e.endList(t)}}t.List=e})(t.flatbuf||(t.flatbuf={}))})(t.arrow||(t.arrow={}))})(t.apache||(t.apache={}))}(Ie||(Ie={})),function(t){(function(t){(function(t){(function(t){class e{constructor(){this.bb=null,this.bb_pos=0}__init(t,e){return this.bb_pos=t,this.bb=e,this}static getRootAsLargeList(t,n){return(n||new e).__init(t.readInt32(t.position())+t.position(),t)}static startLargeList(t){t.startObject(0)}static endLargeList(t){let e=t.endObject();return e}static createLargeList(t){return e.startLargeList(t),e.endLargeList(t)}}t.LargeList=e})(t.flatbuf||(t.flatbuf={}))})(t.arrow||(t.arrow={}))})(t.apache||(t.apache={}))}(Ie||(Ie={})),function(t){(function(t){(function(t){(function(t){class e{constructor(){this.bb=null,this.bb_pos=0}__init(t,e){return this.bb_pos=t,this.bb=e,this}static getRootAsFixedSizeList(t,n){return(n||new e).__init(t.readInt32(t.position())+t.position(),t)}listSize(){let t=this.bb.__offset(this.bb_pos,4);return t?this.bb.readInt32(this.bb_pos+t):0}static startFixedSizeList(t){t.startObject(1)}static addListSize(t,e){t.addFieldInt32(0,e,0)}static endFixedSizeList(t){let e=t.endObject();return e}static createFixedSizeList(t,n){return e.startFixedSizeList(t),e.addListSize(t,n),e.endFixedSizeList(t)}}t.FixedSizeList=e})(t.flatbuf||(t.flatbuf={}))})(t.arrow||(t.arrow={}))})(t.apache||(t.apache={}))}(Ie||(Ie={})),function(t){(function(t){(function(t){(function(t){class e{constructor(){this.bb=null,this.bb_pos=0}__init(t,e){return this.bb_pos=t,this.bb=e,this}static getRootAsMap(t,n){return(n||new e).__init(t.readInt32(t.position())+t.position(),t)}keysSorted(){let t=this.bb.__offset(this.bb_pos,4);return!!t&&!!this.bb.readInt8(this.bb_pos+t)}static startMap(t){t.startObject(1)}static addKeysSorted(t,e){t.addFieldInt8(0,+e,0)}static endMap(t){let e=t.endObject();return e}static createMap(t,n){return e.startMap(t),e.addKeysSorted(t,n),e.endMap(t)}}t.Map=e})(t.flatbuf||(t.flatbuf={}))})(t.arrow||(t.arrow={}))})(t.apache||(t.apache={}))}(Ie||(Ie={})),function(t){(function(e){(function(e){(function(e){class n{constructor(){this.bb=null,this.bb_pos=0}__init(t,e){return this.bb_pos=t,this.bb=e,this}static getRootAsUnion(t,e){return(e||new n).__init(t.readInt32(t.position())+t.position(),t)}mode(){let e=this.bb.__offset(this.bb_pos,4);return e?this.bb.readInt16(this.bb_pos+e):t.apache.arrow.flatbuf.UnionMode.Sparse}typeIds(t){let e=this.bb.__offset(this.bb_pos,6);return e?this.bb.readInt32(this.bb.__vector(this.bb_pos+e)+4*t):0}typeIdsLength(){let t=this.bb.__offset(this.bb_pos,6);return t?this.bb.__vector_len(this.bb_pos+t):0}typeIdsArray(){let t=this.bb.__offset(this.bb_pos,6);return t?new Int32Array(this.bb.bytes().buffer,this.bb.bytes().byteOffset+this.bb.__vector(this.bb_pos+t),this.bb.__vector_len(this.bb_pos+t)):null}static startUnion(t){t.startObject(2)}static addMode(e,n){e.addFieldInt16(0,n,t.apache.arrow.flatbuf.UnionMode.Sparse)}static addTypeIds(t,e){t.addFieldOffset(1,e,0)}static createTypeIdsVector(t,e){t.startVector(4,e.length,4);for(let n=e.length-1;n>=0;n--)t.addInt32(e[n]);return t.endVector()}static startTypeIdsVector(t,e){t.startVector(4,e,4)}static endUnion(t){let e=t.endObject();return e}static createUnion(t,e,r){return n.startUnion(t),n.addMode(t,e),n.addTypeIds(t,r),n.endUnion(t)}}e.Union=n})(e.flatbuf||(e.flatbuf={}))})(e.arrow||(e.arrow={}))})(t.apache||(t.apache={}))}(Ie||(Ie={})),function(t){(function(t){(function(t){(function(t){class e{constructor(){this.bb=null,this.bb_pos=0}__init(t,e){return this.bb_pos=t,this.bb=e,this}static getRootAsInt(t,n){return(n||new e).__init(t.readInt32(t.position())+t.position(),t)}bitWidth(){let t=this.bb.__offset(this.bb_pos,4);return t?this.bb.readInt32(this.bb_pos+t):0}isSigned(){let t=this.bb.__offset(this.bb_pos,6);return!!t&&!!this.bb.readInt8(this.bb_pos+t)}static startInt(t){t.startObject(2)}static addBitWidth(t,e){t.addFieldInt32(0,e,0)}static addIsSigned(t,e){t.addFieldInt8(1,+e,0)}static endInt(t){let e=t.endObject();return e}static createInt(t,n,r){return e.startInt(t),e.addBitWidth(t,n),e.addIsSigned(t,r),e.endInt(t)}}t.Int=e})(t.flatbuf||(t.flatbuf={}))})(t.arrow||(t.arrow={}))})(t.apache||(t.apache={}))}(Ie||(Ie={})),function(t){(function(e){(function(e){(function(e){class n{constructor(){this.bb=null,this.bb_pos=0}__init(t,e){return this.bb_pos=t,this.bb=e,this}static getRootAsFloatingPoint(t,e){return(e||new n).__init(t.readInt32(t.position())+t.position(),t)}precision(){let e=this.bb.__offset(this.bb_pos,4);return e?this.bb.readInt16(this.bb_pos+e):t.apache.arrow.flatbuf.Precision.HALF}static startFloatingPoint(t){t.startObject(1)}static addPrecision(e,n){e.addFieldInt16(0,n,t.apache.arrow.flatbuf.Precision.HALF)}static endFloatingPoint(t){let e=t.endObject();return e}static createFloatingPoint(t,e){return n.startFloatingPoint(t),n.addPrecision(t,e),n.endFloatingPoint(t)}}e.FloatingPoint=n})(e.flatbuf||(e.flatbuf={}))})(e.arrow||(e.arrow={}))})(t.apache||(t.apache={}))}(Ie||(Ie={})),function(t){(function(t){(function(t){(function(t){class e{constructor(){this.bb=null,this.bb_pos=0}__init(t,e){return this.bb_pos=t,this.bb=e,this}static getRootAsUtf8(t,n){return(n||new e).__init(t.readInt32(t.position())+t.position(),t)}static startUtf8(t){t.startObject(0)}static endUtf8(t){let e=t.endObject();return e}static createUtf8(t){return e.startUtf8(t),e.endUtf8(t)}}t.Utf8=e})(t.flatbuf||(t.flatbuf={}))})(t.arrow||(t.arrow={}))})(t.apache||(t.apache={}))}(Ie||(Ie={})),function(t){(function(t){(function(t){(function(t){class e{constructor(){this.bb=null,this.bb_pos=0}__init(t,e){return this.bb_pos=t,this.bb=e,this}static getRootAsBinary(t,n){return(n||new e).__init(t.readInt32(t.position())+t.position(),t)}static startBinary(t){t.startObject(0)}static endBinary(t){let e=t.endObject();return e}static createBinary(t){return e.startBinary(t),e.endBinary(t)}}t.Binary=e})(t.flatbuf||(t.flatbuf={}))})(t.arrow||(t.arrow={}))})(t.apache||(t.apache={}))}(Ie||(Ie={})),function(t){(function(t){(function(t){(function(t){class e{constructor(){this.bb=null,this.bb_pos=0}__init(t,e){return this.bb_pos=t,this.bb=e,this}static getRootAsLargeUtf8(t,n){return(n||new e).__init(t.readInt32(t.position())+t.position(),t)}static startLargeUtf8(t){t.startObject(0)}static endLargeUtf8(t){let e=t.endObject();return e}static createLargeUtf8(t){return e.startLargeUtf8(t),e.endLargeUtf8(t)}}t.LargeUtf8=e})(t.flatbuf||(t.flatbuf={}))})(t.arrow||(t.arrow={}))})(t.apache||(t.apache={}))}(Ie||(Ie={})),function(t){(function(t){(function(t){(function(t){class e{constructor(){this.bb=null,this.bb_pos=0}__init(t,e){return this.bb_pos=t,this.bb=e,this}static getRootAsLargeBinary(t,n){return(n||new e).__init(t.readInt32(t.position())+t.position(),t)}static startLargeBinary(t){t.startObject(0)}static endLargeBinary(t){let e=t.endObject();return e}static createLargeBinary(t){return e.startLargeBinary(t),e.endLargeBinary(t)}}t.LargeBinary=e})(t.flatbuf||(t.flatbuf={}))})(t.arrow||(t.arrow={}))})(t.apache||(t.apache={}))}(Ie||(Ie={})),function(t){(function(t){(function(t){(function(t){class e{constructor(){this.bb=null,this.bb_pos=0}__init(t,e){return this.bb_pos=t,this.bb=e,this}static getRootAsFixedSizeBinary(t,n){return(n||new e).__init(t.readInt32(t.position())+t.position(),t)}byteWidth(){let t=this.bb.__offset(this.bb_pos,4);return t?this.bb.readInt32(this.bb_pos+t):0}static startFixedSizeBinary(t){t.startObject(1)}static addByteWidth(t,e){t.addFieldInt32(0,e,0)}static endFixedSizeBinary(t){let e=t.endObject();return e}static createFixedSizeBinary(t,n){return e.startFixedSizeBinary(t),e.addByteWidth(t,n),e.endFixedSizeBinary(t)}}t.FixedSizeBinary=e})(t.flatbuf||(t.flatbuf={}))})(t.arrow||(t.arrow={}))})(t.apache||(t.apache={}))}(Ie||(Ie={})),function(t){(function(t){(function(t){(function(t){class e{constructor(){this.bb=null,this.bb_pos=0}__init(t,e){return this.bb_pos=t,this.bb=e,this}static getRootAsBool(t,n){return(n||new e).__init(t.readInt32(t.position())+t.position(),t)}static startBool(t){t.startObject(0)}static endBool(t){let e=t.endObject();return e}static createBool(t){return e.startBool(t),e.endBool(t)}}t.Bool=e})(t.flatbuf||(t.flatbuf={}))})(t.arrow||(t.arrow={}))})(t.apache||(t.apache={}))}(Ie||(Ie={})),function(t){(function(t){(function(t){(function(t){class e{constructor(){this.bb=null,this.bb_pos=0}__init(t,e){return this.bb_pos=t,this.bb=e,this}static getRootAsDecimal(t,n){return(n||new e).__init(t.readInt32(t.position())+t.position(),t)}precision(){let t=this.bb.__offset(this.bb_pos,4);return t?this.bb.readInt32(this.bb_pos+t):0}scale(){let t=this.bb.__offset(this.bb_pos,6);return t?this.bb.readInt32(this.bb_pos+t):0}static startDecimal(t){t.startObject(2)}static addPrecision(t,e){t.addFieldInt32(0,e,0)}static addScale(t,e){t.addFieldInt32(1,e,0)}static endDecimal(t){let e=t.endObject();return e}static createDecimal(t,n,r){return e.startDecimal(t),e.addPrecision(t,n),e.addScale(t,r),e.endDecimal(t)}}t.Decimal=e})(t.flatbuf||(t.flatbuf={}))})(t.arrow||(t.arrow={}))})(t.apache||(t.apache={}))}(Ie||(Ie={})),function(t){(function(e){(function(e){(function(e){class n{constructor(){this.bb=null,this.bb_pos=0}__init(t,e){return this.bb_pos=t,this.bb=e,this}static getRootAsDate(t,e){return(e||new n).__init(t.readInt32(t.position())+t.position(),t)}unit(){let e=this.bb.__offset(this.bb_pos,4);return e?this.bb.readInt16(this.bb_pos+e):t.apache.arrow.flatbuf.DateUnit.MILLISECOND}static startDate(t){t.startObject(1)}static addUnit(e,n){e.addFieldInt16(0,n,t.apache.arrow.flatbuf.DateUnit.MILLISECOND)}static endDate(t){let e=t.endObject();return e}static createDate(t,e){return n.startDate(t),n.addUnit(t,e),n.endDate(t)}}e.Date=n})(e.flatbuf||(e.flatbuf={}))})(e.arrow||(e.arrow={}))})(t.apache||(t.apache={}))}(Ie||(Ie={})),function(t){(function(e){(function(e){(function(e){class n{constructor(){this.bb=null,this.bb_pos=0}__init(t,e){return this.bb_pos=t,this.bb=e,this}static getRootAsTime(t,e){return(e||new n).__init(t.readInt32(t.position())+t.position(),t)}unit(){let e=this.bb.__offset(this.bb_pos,4);return e?this.bb.readInt16(this.bb_pos+e):t.apache.arrow.flatbuf.TimeUnit.MILLISECOND}bitWidth(){let t=this.bb.__offset(this.bb_pos,6);return t?this.bb.readInt32(this.bb_pos+t):32}static startTime(t){t.startObject(2)}static addUnit(e,n){e.addFieldInt16(0,n,t.apache.arrow.flatbuf.TimeUnit.MILLISECOND)}static addBitWidth(t,e){t.addFieldInt32(1,e,32)}static endTime(t){let e=t.endObject();return e}static createTime(t,e,r){return n.startTime(t),n.addUnit(t,e),n.addBitWidth(t,r),n.endTime(t)}}e.Time=n})(e.flatbuf||(e.flatbuf={}))})(e.arrow||(e.arrow={}))})(t.apache||(t.apache={}))}(Ie||(Ie={})),function(t){(function(e){(function(e){(function(e){class n{constructor(){this.bb=null,this.bb_pos=0}__init(t,e){return this.bb_pos=t,this.bb=e,this}static getRootAsTimestamp(t,e){return(e||new n).__init(t.readInt32(t.position())+t.position(),t)}unit(){let e=this.bb.__offset(this.bb_pos,4);return e?this.bb.readInt16(this.bb_pos+e):t.apache.arrow.flatbuf.TimeUnit.SECOND}timezone(t){let e=this.bb.__offset(this.bb_pos,6);return e?this.bb.__string(this.bb_pos+e,t):null}static startTimestamp(t){t.startObject(2)}static addUnit(e,n){e.addFieldInt16(0,n,t.apache.arrow.flatbuf.TimeUnit.SECOND)}static addTimezone(t,e){t.addFieldOffset(1,e,0)}static endTimestamp(t){let e=t.endObject();return e}static createTimestamp(t,e,r){return n.startTimestamp(t),n.addUnit(t,e),n.addTimezone(t,r),n.endTimestamp(t)}}e.Timestamp=n})(e.flatbuf||(e.flatbuf={}))})(e.arrow||(e.arrow={}))})(t.apache||(t.apache={}))}(Ie||(Ie={})),function(t){(function(e){(function(e){(function(e){class n{constructor(){this.bb=null,this.bb_pos=0}__init(t,e){return this.bb_pos=t,this.bb=e,this}static getRootAsInterval(t,e){return(e||new n).__init(t.readInt32(t.position())+t.position(),t)}unit(){let e=this.bb.__offset(this.bb_pos,4);return e?this.bb.readInt16(this.bb_pos+e):t.apache.arrow.flatbuf.IntervalUnit.YEAR_MONTH}static startInterval(t){t.startObject(1)}static addUnit(e,n){e.addFieldInt16(0,n,t.apache.arrow.flatbuf.IntervalUnit.YEAR_MONTH)}static endInterval(t){let e=t.endObject();return e}static createInterval(t,e){return n.startInterval(t),n.addUnit(t,e),n.endInterval(t)}}e.Interval=n})(e.flatbuf||(e.flatbuf={}))})(e.arrow||(e.arrow={}))})(t.apache||(t.apache={}))}(Ie||(Ie={})),function(t){(function(e){(function(e){(function(e){class n{constructor(){this.bb=null,this.bb_pos=0}__init(t,e){return this.bb_pos=t,this.bb=e,this}static getRootAsDuration(t,e){return(e||new n).__init(t.readInt32(t.position())+t.position(),t)}unit(){let e=this.bb.__offset(this.bb_pos,4);return e?this.bb.readInt16(this.bb_pos+e):t.apache.arrow.flatbuf.TimeUnit.MILLISECOND}static startDuration(t){t.startObject(1)}static addUnit(e,n){e.addFieldInt16(0,n,t.apache.arrow.flatbuf.TimeUnit.MILLISECOND)}static endDuration(t){let e=t.endObject();return e}static createDuration(t,e){return n.startDuration(t),n.addUnit(t,e),n.endDuration(t)}}e.Duration=n})(e.flatbuf||(e.flatbuf={}))})(e.arrow||(e.arrow={}))})(t.apache||(t.apache={}))}(Ie||(Ie={})),function(t){(function(t){(function(t){(function(t){class e{constructor(){this.bb=null,this.bb_pos=0}__init(t,e){return this.bb_pos=t,this.bb=e,this}static getRootAsKeyValue(t,n){return(n||new e).__init(t.readInt32(t.position())+t.position(),t)}key(t){let e=this.bb.__offset(this.bb_pos,4);return e?this.bb.__string(this.bb_pos+e,t):null}value(t){let e=this.bb.__offset(this.bb_pos,6);return e?this.bb.__string(this.bb_pos+e,t):null}static startKeyValue(t){t.startObject(2)}static addKey(t,e){t.addFieldOffset(0,e,0)}static addValue(t,e){t.addFieldOffset(1,e,0)}static endKeyValue(t){let e=t.endObject();return e}static createKeyValue(t,n,r){return e.startKeyValue(t),e.addKey(t,n),e.addValue(t,r),e.endKeyValue(t)}}t.KeyValue=e})(t.flatbuf||(t.flatbuf={}))})(t.arrow||(t.arrow={}))})(t.apache||(t.apache={}))}(Ie||(Ie={})),function(t){(function(e){(function(e){(function(e){class n{constructor(){this.bb=null,this.bb_pos=0}__init(t,e){return this.bb_pos=t,this.bb=e,this}static getRootAsDictionaryEncoding(t,e){return(e||new n).__init(t.readInt32(t.position())+t.position(),t)}id(){let t=this.bb.__offset(this.bb_pos,4);return t?this.bb.readInt64(this.bb_pos+t):this.bb.createLong(0,0)}indexType(e){let n=this.bb.__offset(this.bb_pos,6);return n?(e||new t.apache.arrow.flatbuf.Int).__init(this.bb.__indirect(this.bb_pos+n),this.bb):null}isOrdered(){let t=this.bb.__offset(this.bb_pos,8);return!!t&&!!this.bb.readInt8(this.bb_pos+t)}static startDictionaryEncoding(t){t.startObject(3)}static addId(t,e){t.addFieldInt64(0,e,t.createLong(0,0))}static addIndexType(t,e){t.addFieldOffset(1,e,0)}static addIsOrdered(t,e){t.addFieldInt8(2,+e,0)}static endDictionaryEncoding(t){let e=t.endObject();return e}static createDictionaryEncoding(t,e,r,i){return n.startDictionaryEncoding(t),n.addId(t,e),n.addIndexType(t,r),n.addIsOrdered(t,i),n.endDictionaryEncoding(t)}}e.DictionaryEncoding=n})(e.flatbuf||(e.flatbuf={}))})(e.arrow||(e.arrow={}))})(t.apache||(t.apache={}))}(Ie||(Ie={})),function(t){(function(e){(function(e){(function(e){class n{constructor(){this.bb=null,this.bb_pos=0}__init(t,e){return this.bb_pos=t,this.bb=e,this}static getRootAsField(t,e){return(e||new n).__init(t.readInt32(t.position())+t.position(),t)}name(t){let e=this.bb.__offset(this.bb_pos,4);return e?this.bb.__string(this.bb_pos+e,t):null}nullable(){let t=this.bb.__offset(this.bb_pos,6);return!!t&&!!this.bb.readInt8(this.bb_pos+t)}typeType(){let e=this.bb.__offset(this.bb_pos,8);return e?this.bb.readUint8(this.bb_pos+e):t.apache.arrow.flatbuf.Type.NONE}type(t){let e=this.bb.__offset(this.bb_pos,10);return e?this.bb.__union(t,this.bb_pos+e):null}dictionary(e){let n=this.bb.__offset(this.bb_pos,12);return n?(e||new t.apache.arrow.flatbuf.DictionaryEncoding).__init(this.bb.__indirect(this.bb_pos+n),this.bb):null}children(e,n){let r=this.bb.__offset(this.bb_pos,14);return r?(n||new t.apache.arrow.flatbuf.Field).__init(this.bb.__indirect(this.bb.__vector(this.bb_pos+r)+4*e),this.bb):null}childrenLength(){let t=this.bb.__offset(this.bb_pos,14);return t?this.bb.__vector_len(this.bb_pos+t):0}customMetadata(e,n){let r=this.bb.__offset(this.bb_pos,16);return r?(n||new t.apache.arrow.flatbuf.KeyValue).__init(this.bb.__indirect(this.bb.__vector(this.bb_pos+r)+4*e),this.bb):null}customMetadataLength(){let t=this.bb.__offset(this.bb_pos,16);return t?this.bb.__vector_len(this.bb_pos+t):0}static startField(t){t.startObject(7)}static addName(t,e){t.addFieldOffset(0,e,0)}static addNullable(t,e){t.addFieldInt8(1,+e,0)}static addTypeType(e,n){e.addFieldInt8(2,n,t.apache.arrow.flatbuf.Type.NONE)}static addType(t,e){t.addFieldOffset(3,e,0)}static addDictionary(t,e){t.addFieldOffset(4,e,0)}static addChildren(t,e){t.addFieldOffset(5,e,0)}static createChildrenVector(t,e){t.startVector(4,e.length,4);for(let n=e.length-1;n>=0;n--)t.addOffset(e[n]);return t.endVector()}static startChildrenVector(t,e){t.startVector(4,e,4)}static addCustomMetadata(t,e){t.addFieldOffset(6,e,0)}static createCustomMetadataVector(t,e){t.startVector(4,e.length,4);for(let n=e.length-1;n>=0;n--)t.addOffset(e[n]);return t.endVector()}static startCustomMetadataVector(t,e){t.startVector(4,e,4)}static endField(t){let e=t.endObject();return e}static createField(t,e,r,i,s,o,a,c){return n.startField(t),n.addName(t,e),n.addNullable(t,r),n.addTypeType(t,i),n.addType(t,s),n.addDictionary(t,o),n.addChildren(t,a),n.addCustomMetadata(t,c),n.endField(t)}}e.Field=n})(e.flatbuf||(e.flatbuf={}))})(e.arrow||(e.arrow={}))})(t.apache||(t.apache={}))}(Ie||(Ie={})),function(t){(function(t){(function(t){(function(t){class e{constructor(){this.bb=null,this.bb_pos=0}__init(t,e){return this.bb_pos=t,this.bb=e,this}offset(){return this.bb.readInt64(this.bb_pos)}length(){return this.bb.readInt64(this.bb_pos+8)}static createBuffer(t,e,n){return t.prep(8,16),t.writeInt64(n),t.writeInt64(e),t.offset()}}t.Buffer=e})(t.flatbuf||(t.flatbuf={}))})(t.arrow||(t.arrow={}))})(t.apache||(t.apache={}))}(Ie||(Ie={})),function(t){(function(e){(function(e){(function(e){class n{constructor(){this.bb=null,this.bb_pos=0}__init(t,e){return this.bb_pos=t,this.bb=e,this}static getRootAsSchema(t,e){return(e||new n).__init(t.readInt32(t.position())+t.position(),t)}endianness(){let e=this.bb.__offset(this.bb_pos,4);return e?this.bb.readInt16(this.bb_pos+e):t.apache.arrow.flatbuf.Endianness.Little}fields(e,n){let r=this.bb.__offset(this.bb_pos,6);return r?(n||new t.apache.arrow.flatbuf.Field).__init(this.bb.__indirect(this.bb.__vector(this.bb_pos+r)+4*e),this.bb):null}fieldsLength(){let t=this.bb.__offset(this.bb_pos,6);return t?this.bb.__vector_len(this.bb_pos+t):0}customMetadata(e,n){let r=this.bb.__offset(this.bb_pos,8);return r?(n||new t.apache.arrow.flatbuf.KeyValue).__init(this.bb.__indirect(this.bb.__vector(this.bb_pos+r)+4*e),this.bb):null}customMetadataLength(){let t=this.bb.__offset(this.bb_pos,8);return t?this.bb.__vector_len(this.bb_pos+t):0}static startSchema(t){t.startObject(3)}static addEndianness(e,n){e.addFieldInt16(0,n,t.apache.arrow.flatbuf.Endianness.Little)}static addFields(t,e){t.addFieldOffset(1,e,0)}static createFieldsVector(t,e){t.startVector(4,e.length,4);for(let n=e.length-1;n>=0;n--)t.addOffset(e[n]);return t.endVector()}static startFieldsVector(t,e){t.startVector(4,e,4)}static addCustomMetadata(t,e){t.addFieldOffset(2,e,0)}static createCustomMetadataVector(t,e){t.startVector(4,e.length,4);for(let n=e.length-1;n>=0;n--)t.addOffset(e[n]);return t.endVector()}static startCustomMetadataVector(t,e){t.startVector(4,e,4)}static endSchema(t){let e=t.endObject();return e}static finishSchemaBuffer(t,e){t.finish(e)}static createSchema(t,e,r,i){return n.startSchema(t),n.addEndianness(t,e),n.addFields(t,r),n.addCustomMetadata(t,i),n.endSchema(t)}}e.Schema=n})(e.flatbuf||(e.flatbuf={}))})(e.arrow||(e.arrow={}))})(t.apache||(t.apache={}))}(Ie||(Ie={})),function(t){(function(t){(function(t){(function(t){t.Schema=Ie.apache.arrow.flatbuf.Schema})(t.flatbuf||(t.flatbuf={}))})(t.arrow||(t.arrow={}))})(t.apache||(t.apache={}))}(Se||(Se={})),function(t){(function(t){(function(t){(function(t){let e;(function(t){t[t["NONE"]=0]="NONE",t[t["Schema"]=1]="Schema",t[t["DictionaryBatch"]=2]="DictionaryBatch",t[t["RecordBatch"]=3]="RecordBatch",t[t["Tensor"]=4]="Tensor",t[t["SparseTensor"]=5]="SparseTensor"})(e=t.MessageHeader||(t.MessageHeader={}))})(t.flatbuf||(t.flatbuf={}))})(t.arrow||(t.arrow={}))})(t.apache||(t.apache={}))}(Se||(Se={})),function(t){(function(t){(function(t){(function(t){class e{constructor(){this.bb=null,this.bb_pos=0}__init(t,e){return this.bb_pos=t,this.bb=e,this}length(){return this.bb.readInt64(this.bb_pos)}nullCount(){return this.bb.readInt64(this.bb_pos+8)}static createFieldNode(t,e,n){return t.prep(8,16),t.writeInt64(n),t.writeInt64(e),t.offset()}}t.FieldNode=e})(t.flatbuf||(t.flatbuf={}))})(t.arrow||(t.arrow={}))})(t.apache||(t.apache={}))}(Se||(Se={})),function(t){(function(e){(function(e){(function(e){class n{constructor(){this.bb=null,this.bb_pos=0}__init(t,e){return this.bb_pos=t,this.bb=e,this}static getRootAsRecordBatch(t,e){return(e||new n).__init(t.readInt32(t.position())+t.position(),t)}length(){let t=this.bb.__offset(this.bb_pos,4);return t?this.bb.readInt64(this.bb_pos+t):this.bb.createLong(0,0)}nodes(e,n){let r=this.bb.__offset(this.bb_pos,6);return r?(n||new t.apache.arrow.flatbuf.FieldNode).__init(this.bb.__vector(this.bb_pos+r)+16*e,this.bb):null}nodesLength(){let t=this.bb.__offset(this.bb_pos,6);return t?this.bb.__vector_len(this.bb_pos+t):0}buffers(t,e){let n=this.bb.__offset(this.bb_pos,8);return n?(e||new Ie.apache.arrow.flatbuf.Buffer).__init(this.bb.__vector(this.bb_pos+n)+16*t,this.bb):null}buffersLength(){let t=this.bb.__offset(this.bb_pos,8);return t?this.bb.__vector_len(this.bb_pos+t):0}static startRecordBatch(t){t.startObject(3)}static addLength(t,e){t.addFieldInt64(0,e,t.createLong(0,0))}static addNodes(t,e){t.addFieldOffset(1,e,0)}static startNodesVector(t,e){t.startVector(16,e,8)}static addBuffers(t,e){t.addFieldOffset(2,e,0)}static startBuffersVector(t,e){t.startVector(16,e,8)}static endRecordBatch(t){let e=t.endObject();return e}static createRecordBatch(t,e,r,i){return n.startRecordBatch(t),n.addLength(t,e),n.addNodes(t,r),n.addBuffers(t,i),n.endRecordBatch(t)}}e.RecordBatch=n})(e.flatbuf||(e.flatbuf={}))})(e.arrow||(e.arrow={}))})(t.apache||(t.apache={}))}(Se||(Se={})),function(t){(function(e){(function(e){(function(e){class n{constructor(){this.bb=null,this.bb_pos=0}__init(t,e){return this.bb_pos=t,this.bb=e,this}static getRootAsDictionaryBatch(t,e){return(e||new n).__init(t.readInt32(t.position())+t.position(),t)}id(){let t=this.bb.__offset(this.bb_pos,4);return t?this.bb.readInt64(this.bb_pos+t):this.bb.createLong(0,0)}data(e){let n=this.bb.__offset(this.bb_pos,6);return n?(e||new t.apache.arrow.flatbuf.RecordBatch).__init(this.bb.__indirect(this.bb_pos+n),this.bb):null}isDelta(){let t=this.bb.__offset(this.bb_pos,8);return!!t&&!!this.bb.readInt8(this.bb_pos+t)}static startDictionaryBatch(t){t.startObject(3)}static addId(t,e){t.addFieldInt64(0,e,t.createLong(0,0))}static addData(t,e){t.addFieldOffset(1,e,0)}static addIsDelta(t,e){t.addFieldInt8(2,+e,0)}static endDictionaryBatch(t){let e=t.endObject();return e}static createDictionaryBatch(t,e,r,i){return n.startDictionaryBatch(t),n.addId(t,e),n.addData(t,r),n.addIsDelta(t,i),n.endDictionaryBatch(t)}}e.DictionaryBatch=n})(e.flatbuf||(e.flatbuf={}))})(e.arrow||(e.arrow={}))})(t.apache||(t.apache={}))}(Se||(Se={})),function(t){(function(e){(function(e){(function(e){class n{constructor(){this.bb=null,this.bb_pos=0}__init(t,e){return this.bb_pos=t,this.bb=e,this}static getRootAsMessage(t,e){return(e||new n).__init(t.readInt32(t.position())+t.position(),t)}version(){let t=this.bb.__offset(this.bb_pos,4);return t?this.bb.readInt16(this.bb_pos+t):Ie.apache.arrow.flatbuf.MetadataVersion.V1}headerType(){let e=this.bb.__offset(this.bb_pos,6);return e?this.bb.readUint8(this.bb_pos+e):t.apache.arrow.flatbuf.MessageHeader.NONE}header(t){let e=this.bb.__offset(this.bb_pos,8);return e?this.bb.__union(t,this.bb_pos+e):null}bodyLength(){let t=this.bb.__offset(this.bb_pos,10);return t?this.bb.readInt64(this.bb_pos+t):this.bb.createLong(0,0)}customMetadata(t,e){let n=this.bb.__offset(this.bb_pos,12);return n?(e||new Ie.apache.arrow.flatbuf.KeyValue).__init(this.bb.__indirect(this.bb.__vector(this.bb_pos+n)+4*t),this.bb):null}customMetadataLength(){let t=this.bb.__offset(this.bb_pos,12);return t?this.bb.__vector_len(this.bb_pos+t):0}static startMessage(t){t.startObject(5)}static addVersion(t,e){t.addFieldInt16(0,e,Ie.apache.arrow.flatbuf.MetadataVersion.V1)}static addHeaderType(e,n){e.addFieldInt8(1,n,t.apache.arrow.flatbuf.MessageHeader.NONE)}static addHeader(t,e){t.addFieldOffset(2,e,0)}static addBodyLength(t,e){t.addFieldInt64(3,e,t.createLong(0,0))}static addCustomMetadata(t,e){t.addFieldOffset(4,e,0)}static createCustomMetadataVector(t,e){t.startVector(4,e.length,4);for(let n=e.length-1;n>=0;n--)t.addOffset(e[n]);return t.endVector()}static startCustomMetadataVector(t,e){t.startVector(4,e,4)}static endMessage(t){let e=t.endObject();return e}static finishMessageBuffer(t,e){t.finish(e)}static createMessage(t,e,r,i,s,o){return n.startMessage(t),n.addVersion(t,e),n.addHeaderType(t,r),n.addHeader(t,i),n.addBodyLength(t,s),n.addCustomMetadata(t,o),n.endMessage(t)}}e.Message=n})(e.flatbuf||(e.flatbuf={}))})(e.arrow||(e.arrow={}))})(t.apache||(t.apache={}))}(Se||(Se={}));Ie.apache.arrow.flatbuf.Type;var Oe,Te,Ae=Ie.apache.arrow.flatbuf.DateUnit,xe=Ie.apache.arrow.flatbuf.TimeUnit,Be=Ie.apache.arrow.flatbuf.Precision,je=Ie.apache.arrow.flatbuf.UnionMode,De=Ie.apache.arrow.flatbuf.IntervalUnit,Fe=Se.apache.arrow.flatbuf.MessageHeader,Le=Ie.apache.arrow.flatbuf.MetadataVersion;function Ee(t,e,n,r){return 0!==(n&1<>r}function Ue(t,e,n){return n?!!(t[e>>3]|=1<>3]&=~(1<0||n.byteLength>3):Ne(ke(n,t,e,null,Ee)).subarray(0,r)),i}return n}function Ne(t){let e=[],n=0,r=0,i=0;for(const o of t)o&&(i|=1<0)&&(e[n++]=i);let s=new Uint8Array(e.length+7&-8);return s.set(e),s}function*ke(t,e,n,r,i){let s=e%8,o=e>>3,a=0,c=n;for(;c>0;s=0){let e=t[o++];do{yield i(r,a++,e,s)}while(--c>0&&++s<8)}}function Ve(t,e,n){if(n-e<=0)return 0;if(n-e<8){let r=0;for(const i of ke(t,e,n-e,t,Me))r+=i;return r}const r=n>>3<<3,i=e+(e%8===0?0:8-e%8);return Ve(t,e,i)+Ve(t,r,n)+Re(t,i>>3,r-i>>3)}function Re(t,e,n){let r=0,i=0|e;const s=new DataView(t.buffer,t.byteOffset,t.byteLength),o=void 0===n?t.byteLength:i+n;while(o-i>=4)r+=Pe(s.getUint32(i)),i+=4;while(o-i>=2)r+=Pe(s.getUint16(i)),i+=2;while(o-i>=1)r+=Pe(s.getUint8(i)),i+=1;return r}function Pe(t){let e=0|t;return e-=e>>>1&1431655765,e=(858993459&e)+(e>>>2&858993459),16843009*(e+(e>>>4)&252645135)>>>24}(function(t){t[t["NONE"]=0]="NONE",t[t["Null"]=1]="Null",t[t["Int"]=2]="Int",t[t["Float"]=3]="Float",t[t["Binary"]=4]="Binary",t[t["Utf8"]=5]="Utf8",t[t["Bool"]=6]="Bool",t[t["Decimal"]=7]="Decimal",t[t["Date"]=8]="Date",t[t["Time"]=9]="Time",t[t["Timestamp"]=10]="Timestamp",t[t["Interval"]=11]="Interval",t[t["List"]=12]="List",t[t["Struct"]=13]="Struct",t[t["Union"]=14]="Union",t[t["FixedSizeBinary"]=15]="FixedSizeBinary",t[t["FixedSizeList"]=16]="FixedSizeList",t[t["Map"]=17]="Map",t[t["Dictionary"]=-1]="Dictionary",t[t["Int8"]=-2]="Int8",t[t["Int16"]=-3]="Int16",t[t["Int32"]=-4]="Int32",t[t["Int64"]=-5]="Int64",t[t["Uint8"]=-6]="Uint8",t[t["Uint16"]=-7]="Uint16",t[t["Uint32"]=-8]="Uint32",t[t["Uint64"]=-9]="Uint64",t[t["Float16"]=-10]="Float16",t[t["Float32"]=-11]="Float32",t[t["Float64"]=-12]="Float64",t[t["DateDay"]=-13]="DateDay",t[t["DateMillisecond"]=-14]="DateMillisecond",t[t["TimestampSecond"]=-15]="TimestampSecond",t[t["TimestampMillisecond"]=-16]="TimestampMillisecond",t[t["TimestampMicrosecond"]=-17]="TimestampMicrosecond",t[t["TimestampNanosecond"]=-18]="TimestampNanosecond",t[t["TimeSecond"]=-19]="TimeSecond",t[t["TimeMillisecond"]=-20]="TimeMillisecond",t[t["TimeMicrosecond"]=-21]="TimeMicrosecond",t[t["TimeNanosecond"]=-22]="TimeNanosecond",t[t["DenseUnion"]=-23]="DenseUnion",t[t["SparseUnion"]=-24]="SparseUnion",t[t["IntervalDayTime"]=-25]="IntervalDayTime",t[t["IntervalYearMonth"]=-26]="IntervalYearMonth"})(Oe||(Oe={})),function(t){t[t["OFFSET"]=0]="OFFSET",t[t["DATA"]=1]="DATA",t[t["VALIDITY"]=2]="VALIDITY",t[t["TYPE"]=3]="TYPE"}(Te||(Te={}));class ze{visitMany(t,...e){return t.map((t,n)=>this.visit(t,...e.map(t=>t[n])))}visit(...t){return this.getVisitFn(t[0],!1).apply(this,t)}getVisitFn(t,e=!0){return $e(this,t,e)}visitNull(t,...e){return null}visitBool(t,...e){return null}visitInt(t,...e){return null}visitFloat(t,...e){return null}visitUtf8(t,...e){return null}visitBinary(t,...e){return null}visitFixedSizeBinary(t,...e){return null}visitDate(t,...e){return null}visitTimestamp(t,...e){return null}visitTime(t,...e){return null}visitDecimal(t,...e){return null}visitList(t,...e){return null}visitStruct(t,...e){return null}visitUnion(t,...e){return null}visitDictionary(t,...e){return null}visitInterval(t,...e){return null}visitFixedSizeList(t,...e){return null}visitMap(t,...e){return null}}function $e(t,e,n=!0){let r=null,i=Oe.NONE;switch(e instanceof Yn||e instanceof we?i=Ye(e.type):e instanceof un?i=Ye(e):"number"!==typeof(i=e)&&(i=Oe[e]),i){case Oe.Null:r=t.visitNull;break;case Oe.Bool:r=t.visitBool;break;case Oe.Int:r=t.visitInt;break;case Oe.Int8:r=t.visitInt8||t.visitInt;break;case Oe.Int16:r=t.visitInt16||t.visitInt;break;case Oe.Int32:r=t.visitInt32||t.visitInt;break;case Oe.Int64:r=t.visitInt64||t.visitInt;break;case Oe.Uint8:r=t.visitUint8||t.visitInt;break;case Oe.Uint16:r=t.visitUint16||t.visitInt;break;case Oe.Uint32:r=t.visitUint32||t.visitInt;break;case Oe.Uint64:r=t.visitUint64||t.visitInt;break;case Oe.Float:r=t.visitFloat;break;case Oe.Float16:r=t.visitFloat16||t.visitFloat;break;case Oe.Float32:r=t.visitFloat32||t.visitFloat;break;case Oe.Float64:r=t.visitFloat64||t.visitFloat;break;case Oe.Utf8:r=t.visitUtf8;break;case Oe.Binary:r=t.visitBinary;break;case Oe.FixedSizeBinary:r=t.visitFixedSizeBinary;break;case Oe.Date:r=t.visitDate;break;case Oe.DateDay:r=t.visitDateDay||t.visitDate;break;case Oe.DateMillisecond:r=t.visitDateMillisecond||t.visitDate;break;case Oe.Timestamp:r=t.visitTimestamp;break;case Oe.TimestampSecond:r=t.visitTimestampSecond||t.visitTimestamp;break;case Oe.TimestampMillisecond:r=t.visitTimestampMillisecond||t.visitTimestamp;break;case Oe.TimestampMicrosecond:r=t.visitTimestampMicrosecond||t.visitTimestamp;break;case Oe.TimestampNanosecond:r=t.visitTimestampNanosecond||t.visitTimestamp;break;case Oe.Time:r=t.visitTime;break;case Oe.TimeSecond:r=t.visitTimeSecond||t.visitTime;break;case Oe.TimeMillisecond:r=t.visitTimeMillisecond||t.visitTime;break;case Oe.TimeMicrosecond:r=t.visitTimeMicrosecond||t.visitTime;break;case Oe.TimeNanosecond:r=t.visitTimeNanosecond||t.visitTime;break;case Oe.Decimal:r=t.visitDecimal;break;case Oe.List:r=t.visitList;break;case Oe.Struct:r=t.visitStruct;break;case Oe.Union:r=t.visitUnion;break;case Oe.DenseUnion:r=t.visitDenseUnion||t.visitUnion;break;case Oe.SparseUnion:r=t.visitSparseUnion||t.visitUnion;break;case Oe.Dictionary:r=t.visitDictionary;break;case Oe.Interval:r=t.visitInterval;break;case Oe.IntervalDayTime:r=t.visitIntervalDayTime||t.visitInterval;break;case Oe.IntervalYearMonth:r=t.visitIntervalYearMonth||t.visitInterval;break;case Oe.FixedSizeList:r=t.visitFixedSizeList;break;case Oe.Map:r=t.visitMap;break}if("function"===typeof r)return r;if(!n)return()=>null;throw new Error(`Unrecognized type '${Oe[i]}'`)}function Ye(t){switch(t.typeId){case Oe.Null:return Oe.Null;case Oe.Int:const{bitWidth:e,isSigned:n}=t;switch(e){case 8:return n?Oe.Int8:Oe.Uint8;case 16:return n?Oe.Int16:Oe.Uint16;case 32:return n?Oe.Int32:Oe.Uint32;case 64:return n?Oe.Int64:Oe.Uint64}return Oe.Int;case Oe.Float:switch(t.precision){case Be.HALF:return Oe.Float16;case Be.SINGLE:return Oe.Float32;case Be.DOUBLE:return Oe.Float64}return Oe.Float;case Oe.Binary:return Oe.Binary;case Oe.Utf8:return Oe.Utf8;case Oe.Bool:return Oe.Bool;case Oe.Decimal:return Oe.Decimal;case Oe.Time:switch(t.unit){case xe.SECOND:return Oe.TimeSecond;case xe.MILLISECOND:return Oe.TimeMillisecond;case xe.MICROSECOND:return Oe.TimeMicrosecond;case xe.NANOSECOND:return Oe.TimeNanosecond}return Oe.Time;case Oe.Timestamp:switch(t.unit){case xe.SECOND:return Oe.TimestampSecond;case xe.MILLISECOND:return Oe.TimestampMillisecond;case xe.MICROSECOND:return Oe.TimestampMicrosecond;case xe.NANOSECOND:return Oe.TimestampNanosecond}return Oe.Timestamp;case Oe.Date:switch(t.unit){case Ae.DAY:return Oe.DateDay;case Ae.MILLISECOND:return Oe.DateMillisecond}return Oe.Date;case Oe.Interval:switch(t.unit){case De.DAY_TIME:return Oe.IntervalDayTime;case De.YEAR_MONTH:return Oe.IntervalYearMonth}return Oe.Interval;case Oe.Map:return Oe.Map;case Oe.List:return Oe.List;case Oe.Struct:return Oe.Struct;case Oe.Union:switch(t.mode){case je.Dense:return Oe.DenseUnion;case je.Sparse:return Oe.SparseUnion}return Oe.Union;case Oe.FixedSizeBinary:return Oe.FixedSizeBinary;case Oe.FixedSizeList:return Oe.FixedSizeList;case Oe.Dictionary:return Oe.Dictionary}throw new Error(`Unrecognized type '${Oe[t.typeId]}'`)}ze.prototype.visitInt8=null,ze.prototype.visitInt16=null,ze.prototype.visitInt32=null,ze.prototype.visitInt64=null,ze.prototype.visitUint8=null,ze.prototype.visitUint16=null,ze.prototype.visitUint32=null,ze.prototype.visitUint64=null,ze.prototype.visitFloat16=null,ze.prototype.visitFloat32=null,ze.prototype.visitFloat64=null,ze.prototype.visitDateDay=null,ze.prototype.visitDateMillisecond=null,ze.prototype.visitTimestampSecond=null,ze.prototype.visitTimestampMillisecond=null,ze.prototype.visitTimestampMicrosecond=null,ze.prototype.visitTimestampNanosecond=null,ze.prototype.visitTimeSecond=null,ze.prototype.visitTimeMillisecond=null,ze.prototype.visitTimeMicrosecond=null,ze.prototype.visitTimeNanosecond=null,ze.prototype.visitDenseUnion=null,ze.prototype.visitSparseUnion=null,ze.prototype.visitIntervalDayTime=null,ze.prototype.visitIntervalYearMonth=null;class We extends ze{compareSchemas(t,e){return t===e||e instanceof t.constructor&&cn.compareFields(t.fields,e.fields)}compareFields(t,e){return t===e||Array.isArray(t)&&Array.isArray(e)&&t.length===e.length&&t.every((t,n)=>cn.compareField(t,e[n]))}compareField(t,e){return t===e||e instanceof t.constructor&&t.name===e.name&&t.nullable===e.nullable&&cn.visit(t.type,e.type)}}function He(t,e){return e instanceof t.constructor}function Ke(t,e){return t===e||He(t,e)}function Ge(t,e){return t===e||He(t,e)&&t.bitWidth===e.bitWidth&&t.isSigned===e.isSigned}function qe(t,e){return t===e||He(t,e)&&t.precision===e.precision}function Je(t,e){return t===e||He(t,e)&&t.byteWidth===e.byteWidth}function Ze(t,e){return t===e||He(t,e)&&t.unit===e.unit}function Xe(t,e){return t===e||He(t,e)&&t.unit===e.unit&&t.timezone===e.timezone}function Qe(t,e){return t===e||He(t,e)&&t.unit===e.unit&&t.bitWidth===e.bitWidth}function tn(t,e){return t===e||He(t,e)&&t.children.length===e.children.length&&cn.compareFields(t.children,e.children)}function en(t,e){return t===e||He(t,e)&&t.children.length===e.children.length&&cn.compareFields(t.children,e.children)}function nn(t,e){return t===e||He(t,e)&&t.mode===e.mode&&t.typeIds.every((t,n)=>t===e.typeIds[n])&&cn.compareFields(t.children,e.children)}function rn(t,e){return t===e||He(t,e)&&t.id===e.id&&t.isOrdered===e.isOrdered&&cn.visit(t.indices,e.indices)&&cn.visit(t.dictionary,e.dictionary)}function sn(t,e){return t===e||He(t,e)&&t.unit===e.unit}function on(t,e){return t===e||He(t,e)&&t.listSize===e.listSize&&t.children.length===e.children.length&&cn.compareFields(t.children,e.children)}function an(t,e){return t===e||He(t,e)&&t.keysSorted===e.keysSorted&&t.children.length===e.children.length&&cn.compareFields(t.children,e.children)}We.prototype.visitNull=Ke,We.prototype.visitBool=Ke,We.prototype.visitInt=Ge,We.prototype.visitInt8=Ge,We.prototype.visitInt16=Ge,We.prototype.visitInt32=Ge,We.prototype.visitInt64=Ge,We.prototype.visitUint8=Ge,We.prototype.visitUint16=Ge,We.prototype.visitUint32=Ge,We.prototype.visitUint64=Ge,We.prototype.visitFloat=qe,We.prototype.visitFloat16=qe,We.prototype.visitFloat32=qe,We.prototype.visitFloat64=qe,We.prototype.visitUtf8=Ke,We.prototype.visitBinary=Ke,We.prototype.visitFixedSizeBinary=Je,We.prototype.visitDate=Ze,We.prototype.visitDateDay=Ze,We.prototype.visitDateMillisecond=Ze,We.prototype.visitTimestamp=Xe,We.prototype.visitTimestampSecond=Xe,We.prototype.visitTimestampMillisecond=Xe,We.prototype.visitTimestampMicrosecond=Xe,We.prototype.visitTimestampNanosecond=Xe,We.prototype.visitTime=Qe,We.prototype.visitTimeSecond=Qe,We.prototype.visitTimeMillisecond=Qe,We.prototype.visitTimeMicrosecond=Qe,We.prototype.visitTimeNanosecond=Qe,We.prototype.visitDecimal=Ke,We.prototype.visitList=tn,We.prototype.visitStruct=en,We.prototype.visitUnion=nn,We.prototype.visitDenseUnion=nn,We.prototype.visitSparseUnion=nn,We.prototype.visitDictionary=rn,We.prototype.visitInterval=sn,We.prototype.visitIntervalDayTime=sn,We.prototype.visitIntervalYearMonth=sn,We.prototype.visitFixedSizeList=on,We.prototype.visitMap=an;const cn=new We;class un{static isNull(t){return t&&t.typeId===Oe.Null}static isInt(t){return t&&t.typeId===Oe.Int}static isFloat(t){return t&&t.typeId===Oe.Float}static isBinary(t){return t&&t.typeId===Oe.Binary}static isUtf8(t){return t&&t.typeId===Oe.Utf8}static isBool(t){return t&&t.typeId===Oe.Bool}static isDecimal(t){return t&&t.typeId===Oe.Decimal}static isDate(t){return t&&t.typeId===Oe.Date}static isTime(t){return t&&t.typeId===Oe.Time}static isTimestamp(t){return t&&t.typeId===Oe.Timestamp}static isInterval(t){return t&&t.typeId===Oe.Interval}static isList(t){return t&&t.typeId===Oe.List}static isStruct(t){return t&&t.typeId===Oe.Struct}static isUnion(t){return t&&t.typeId===Oe.Union}static isFixedSizeBinary(t){return t&&t.typeId===Oe.FixedSizeBinary}static isFixedSizeList(t){return t&&t.typeId===Oe.FixedSizeList}static isMap(t){return t&&t.typeId===Oe.Map}static isDictionary(t){return t&&t.typeId===Oe.Dictionary}get typeId(){return Oe.NONE}compareTo(t){return cn.visit(this,t)}}un[Symbol.toStringTag]=(t=>(t.children=null,t.ArrayType=Array,t[Symbol.toStringTag]="DataType"))(un.prototype);class ln extends un{toString(){return"Null"}get typeId(){return Oe.Null}}ln[Symbol.toStringTag]=(t=>t[Symbol.toStringTag]="Null")(ln.prototype);class hn extends un{constructor(t,e){super(),this.isSigned=t,this.bitWidth=e}get typeId(){return Oe.Int}get ArrayType(){switch(this.bitWidth){case 8:return this.isSigned?Int8Array:Uint8Array;case 16:return this.isSigned?Int16Array:Uint16Array;case 32:return this.isSigned?Int32Array:Uint32Array;case 64:return this.isSigned?Int32Array:Uint32Array}throw new Error(`Unrecognized ${this[Symbol.toStringTag]} type`)}toString(){return`${this.isSigned?"I":"Ui"}nt${this.bitWidth}`}}hn[Symbol.toStringTag]=(t=>(t.isSigned=null,t.bitWidth=null,t[Symbol.toStringTag]="Int"))(hn.prototype);class fn extends hn{constructor(){super(!0,8)}}class dn extends hn{constructor(){super(!0,16)}}class pn extends hn{constructor(){super(!0,32)}}class yn extends hn{constructor(){super(!0,64)}}class bn extends hn{constructor(){super(!1,8)}}class gn extends hn{constructor(){super(!1,16)}}class mn extends hn{constructor(){super(!1,32)}}class vn extends hn{constructor(){super(!1,64)}}Object.defineProperty(fn.prototype,"ArrayType",{value:Int8Array}),Object.defineProperty(dn.prototype,"ArrayType",{value:Int16Array}),Object.defineProperty(pn.prototype,"ArrayType",{value:Int32Array}),Object.defineProperty(yn.prototype,"ArrayType",{value:Int32Array}),Object.defineProperty(bn.prototype,"ArrayType",{value:Uint8Array}),Object.defineProperty(gn.prototype,"ArrayType",{value:Uint16Array}),Object.defineProperty(mn.prototype,"ArrayType",{value:Uint32Array}),Object.defineProperty(vn.prototype,"ArrayType",{value:Uint32Array});class _n extends un{constructor(t){super(),this.precision=t}get typeId(){return Oe.Float}get ArrayType(){switch(this.precision){case Be.HALF:return Uint16Array;case Be.SINGLE:return Float32Array;case Be.DOUBLE:return Float64Array}throw new Error(`Unrecognized ${this[Symbol.toStringTag]} type`)}toString(){return"Float"+(this.precision<<5||16)}}_n[Symbol.toStringTag]=(t=>(t.precision=null,t[Symbol.toStringTag]="Float"))(_n.prototype);class wn extends _n{constructor(){super(Be.HALF)}}class In extends _n{constructor(){super(Be.SINGLE)}}class Sn extends _n{constructor(){super(Be.DOUBLE)}}Object.defineProperty(wn.prototype,"ArrayType",{value:Uint16Array}),Object.defineProperty(In.prototype,"ArrayType",{value:Float32Array}),Object.defineProperty(Sn.prototype,"ArrayType",{value:Float64Array});class On extends un{constructor(){super()}get typeId(){return Oe.Binary}toString(){return"Binary"}}On[Symbol.toStringTag]=(t=>(t.ArrayType=Uint8Array,t[Symbol.toStringTag]="Binary"))(On.prototype);class Tn extends un{constructor(){super()}get typeId(){return Oe.Utf8}toString(){return"Utf8"}}Tn[Symbol.toStringTag]=(t=>(t.ArrayType=Uint8Array,t[Symbol.toStringTag]="Utf8"))(Tn.prototype);class An extends un{constructor(){super()}get typeId(){return Oe.Bool}toString(){return"Bool"}}An[Symbol.toStringTag]=(t=>(t.ArrayType=Uint8Array,t[Symbol.toStringTag]="Bool"))(An.prototype);class xn extends un{constructor(t,e){super(),this.scale=t,this.precision=e}get typeId(){return Oe.Decimal}toString(){return`Decimal[${this.precision}e${this.scale>0?"+":""}${this.scale}]`}}xn[Symbol.toStringTag]=(t=>(t.scale=null,t.precision=null,t.ArrayType=Uint32Array,t[Symbol.toStringTag]="Decimal"))(xn.prototype);class Bn extends un{constructor(t){super(),this.unit=t}get typeId(){return Oe.Date}toString(){return`Date${32*(this.unit+1)}<${Ae[this.unit]}>`}}Bn[Symbol.toStringTag]=(t=>(t.unit=null,t.ArrayType=Int32Array,t[Symbol.toStringTag]="Date"))(Bn.prototype);class jn extends Bn{constructor(){super(Ae.DAY)}}class Dn extends Bn{constructor(){super(Ae.MILLISECOND)}}class Fn extends un{constructor(t,e){super(),this.unit=t,this.bitWidth=e}get typeId(){return Oe.Time}toString(){return`Time${this.bitWidth}<${xe[this.unit]}>`}}Fn[Symbol.toStringTag]=(t=>(t.unit=null,t.bitWidth=null,t.ArrayType=Int32Array,t[Symbol.toStringTag]="Time"))(Fn.prototype);class Ln extends un{constructor(t,e){super(),this.unit=t,this.timezone=e}get typeId(){return Oe.Timestamp}toString(){return`Timestamp<${xe[this.unit]}${this.timezone?", "+this.timezone:""}>`}}Ln[Symbol.toStringTag]=(t=>(t.unit=null,t.timezone=null,t.ArrayType=Int32Array,t[Symbol.toStringTag]="Timestamp"))(Ln.prototype);class En extends un{constructor(t){super(),this.unit=t}get typeId(){return Oe.Interval}toString(){return`Interval<${De[this.unit]}>`}}En[Symbol.toStringTag]=(t=>(t.unit=null,t.ArrayType=Int32Array,t[Symbol.toStringTag]="Interval"))(En.prototype);class Mn extends un{constructor(t){super(),this.children=[t]}get typeId(){return Oe.List}toString(){return`List<${this.valueType}>`}get valueType(){return this.children[0].type}get valueField(){return this.children[0]}get ArrayType(){return this.valueType.ArrayType}}Mn[Symbol.toStringTag]=(t=>(t.children=null,t[Symbol.toStringTag]="List"))(Mn.prototype);class Un extends un{constructor(t){super(),this.children=t}get typeId(){return Oe.Struct}toString(){return`Struct<{${this.children.map(t=>`${t.name}:${t.type}`).join(", ")}}>`}}Un[Symbol.toStringTag]=(t=>(t.children=null,t[Symbol.toStringTag]="Struct"))(Un.prototype);class Cn extends un{constructor(t,e,n){super(),this.mode=t,this.children=n,this.typeIds=e=Int32Array.from(e),this.typeIdToChildIndex=e.reduce((t,e,n)=>(t[e]=n)&&t||t,Object.create(null))}get typeId(){return Oe.Union}toString(){return`${this[Symbol.toStringTag]}<${this.children.map(t=>""+t.type).join(" | ")}>`}}Cn[Symbol.toStringTag]=(t=>(t.mode=null,t.typeIds=null,t.children=null,t.typeIdToChildIndex=null,t.ArrayType=Int8Array,t[Symbol.toStringTag]="Union"))(Cn.prototype);class Nn extends un{constructor(t){super(),this.byteWidth=t}get typeId(){return Oe.FixedSizeBinary}toString(){return`FixedSizeBinary[${this.byteWidth}]`}}Nn[Symbol.toStringTag]=(t=>(t.byteWidth=null,t.ArrayType=Uint8Array,t[Symbol.toStringTag]="FixedSizeBinary"))(Nn.prototype);class kn extends un{constructor(t,e){super(),this.listSize=t,this.children=[e]}get typeId(){return Oe.FixedSizeList}get valueType(){return this.children[0].type}get valueField(){return this.children[0]}get ArrayType(){return this.valueType.ArrayType}toString(){return`FixedSizeList[${this.listSize}]<${this.valueType}>`}}kn[Symbol.toStringTag]=(t=>(t.children=null,t.listSize=null,t[Symbol.toStringTag]="FixedSizeList"))(kn.prototype);class Vn extends un{constructor(t,e=!1){super(),this.children=[t],this.keysSorted=e}get typeId(){return Oe.Map}get keyType(){return this.children[0].type.children[0].type}get valueType(){return this.children[0].type.children[1].type}toString(){return`Map<{${this.children[0].type.children.map(t=>`${t.name}:${t.type}`).join(", ")}}>`}}Vn[Symbol.toStringTag]=(t=>(t.children=null,t.keysSorted=null,t[Symbol.toStringTag]="Map_"))(Vn.prototype);const Rn=(t=>()=>++t)(-1);class Pn extends un{constructor(t,e,n,r){super(),this.indices=e,this.dictionary=t,this.isOrdered=r||!1,this.id=null==n?Rn():"number"===typeof n?n:n.low}get typeId(){return Oe.Dictionary}get children(){return this.dictionary.children}get valueType(){return this.dictionary}get ArrayType(){return this.dictionary.ArrayType}toString(){return`Dictionary<${this.indices}, ${this.dictionary}>`}}function zn(t){let e=t;switch(t.typeId){case Oe.Decimal:return 4;case Oe.Timestamp:return 2;case Oe.Date:return 1+e.unit;case Oe.Interval:return 1+e.unit;case Oe.Int:return+(e.bitWidth>32)+1;case Oe.Time:return+(e.bitWidth>32)+1;case Oe.FixedSizeList:return e.listSize;case Oe.FixedSizeBinary:return e.byteWidth;default:return 1}}Pn[Symbol.toStringTag]=(t=>(t.id=null,t.indices=null,t.isOrdered=null,t.dictionary=null,t[Symbol.toStringTag]="Dictionary"))(Pn.prototype);const $n=-1;class Yn{constructor(t,e,n,r,i,s,o){let a;this.type=t,this.dictionary=o,this.offset=Math.floor(Math.max(e||0,0)),this.length=Math.floor(Math.max(n||0,0)),this._nullCount=Math.floor(Math.max(r||0,-1)),this.childData=(s||[]).map(t=>t instanceof Yn?t:t.data),i instanceof Yn?(this.stride=i.stride,this.values=i.values,this.typeIds=i.typeIds,this.nullBitmap=i.nullBitmap,this.valueOffsets=i.valueOffsets):(this.stride=zn(t),i&&((a=i[0])&&(this.valueOffsets=a),(a=i[1])&&(this.values=a),(a=i[2])&&(this.nullBitmap=a),(a=i[3])&&(this.typeIds=a)))}get typeId(){return this.type.typeId}get ArrayType(){return this.type.ArrayType}get buffers(){return[this.valueOffsets,this.values,this.nullBitmap,this.typeIds]}get byteLength(){let t=0,{valueOffsets:e,values:n,nullBitmap:r,typeIds:i}=this;return e&&(t+=e.byteLength),n&&(t+=n.byteLength),r&&(t+=r.byteLength),i&&(t+=i.byteLength),this.childData.reduce((t,e)=>t+e.byteLength,t)}get nullCount(){let t,e=this._nullCount;return e<=$n&&(t=this.nullBitmap)&&(this._nullCount=e=this.length-Ve(t,this.offset,this.offset+this.length)),e}clone(t,e=this.offset,n=this.length,r=this._nullCount,i=this,s=this.childData){return new Yn(t,e,n,r,i,s,this.dictionary)}slice(t,e){const{stride:n,typeId:r,childData:i}=this,s=+(0===this._nullCount)-1,o=16===r?n:1,a=this._sliceBuffers(t,e,n,r);return this.clone(this.type,this.offset+t,e,s,a,!i.length||this.valueOffsets?i:this._sliceChildren(i,o*t,o*e))}_changeLengthAndBackfillNullBitmap(t){if(this.typeId===Oe.Null)return this.clone(this.type,0,t,0);const{length:e,nullCount:n}=this,r=new Uint8Array((t+63&-64)>>3).fill(255,0,e>>3);r[e>>3]=(1<0&&r.set(Ce(this.offset,e,this.nullBitmap),0);const i=this.buffers;return i[Te.VALIDITY]=r,this.clone(this.type,0,t,n+(t-e),i)}_sliceBuffers(t,e,n,r){let i,{buffers:s}=this;return(i=s[Te.TYPE])&&(s[Te.TYPE]=i.subarray(t,t+e)),(i=s[Te.OFFSET])&&(s[Te.OFFSET]=i.subarray(t,t+e+1))||(i=s[Te.DATA])&&(s[Te.DATA]=6===r?i:i.subarray(n*t,n*(t+e))),s}_sliceChildren(t,e,n){return t.map(t=>t.slice(e,n))}static new(t,e,n,r,i,s,o){switch(i instanceof Yn?i=i.buffers:i||(i=[]),t.typeId){case Oe.Null:return Yn.Null(t,e,n);case Oe.Int:return Yn.Int(t,e,n,r||0,i[Te.VALIDITY],i[Te.DATA]||[]);case Oe.Dictionary:return Yn.Dictionary(t,e,n,r||0,i[Te.VALIDITY],i[Te.DATA]||[],o);case Oe.Float:return Yn.Float(t,e,n,r||0,i[Te.VALIDITY],i[Te.DATA]||[]);case Oe.Bool:return Yn.Bool(t,e,n,r||0,i[Te.VALIDITY],i[Te.DATA]||[]);case Oe.Decimal:return Yn.Decimal(t,e,n,r||0,i[Te.VALIDITY],i[Te.DATA]||[]);case Oe.Date:return Yn.Date(t,e,n,r||0,i[Te.VALIDITY],i[Te.DATA]||[]);case Oe.Time:return Yn.Time(t,e,n,r||0,i[Te.VALIDITY],i[Te.DATA]||[]);case Oe.Timestamp:return Yn.Timestamp(t,e,n,r||0,i[Te.VALIDITY],i[Te.DATA]||[]);case Oe.Interval:return Yn.Interval(t,e,n,r||0,i[Te.VALIDITY],i[Te.DATA]||[]);case Oe.FixedSizeBinary:return Yn.FixedSizeBinary(t,e,n,r||0,i[Te.VALIDITY],i[Te.DATA]||[]);case Oe.Binary:return Yn.Binary(t,e,n,r||0,i[Te.VALIDITY],i[Te.OFFSET]||[],i[Te.DATA]||[]);case Oe.Utf8:return Yn.Utf8(t,e,n,r||0,i[Te.VALIDITY],i[Te.OFFSET]||[],i[Te.DATA]||[]);case Oe.List:return Yn.List(t,e,n,r||0,i[Te.VALIDITY],i[Te.OFFSET]||[],(s||[])[0]);case Oe.FixedSizeList:return Yn.FixedSizeList(t,e,n,r||0,i[Te.VALIDITY],(s||[])[0]);case Oe.Struct:return Yn.Struct(t,e,n,r||0,i[Te.VALIDITY],s||[]);case Oe.Map:return Yn.Map(t,e,n,r||0,i[Te.VALIDITY],i[Te.OFFSET]||[],(s||[])[0]);case Oe.Union:return Yn.Union(t,e,n,r||0,i[Te.VALIDITY],i[Te.TYPE]||[],i[Te.OFFSET]||s,s)}throw new Error("Unrecognized typeId "+t.typeId)}static Null(t,e,n){return new Yn(t,e,n,0)}static Int(t,e,n,r,i,s){return new Yn(t,e,n,r,[void 0,Ft(t.ArrayType,s),Ct(i)])}static Dictionary(t,e,n,r,i,s,o){return new Yn(t,e,n,r,[void 0,Ft(t.indices.ArrayType,s),Ct(i)],[],o)}static Float(t,e,n,r,i,s){return new Yn(t,e,n,r,[void 0,Ft(t.ArrayType,s),Ct(i)])}static Bool(t,e,n,r,i,s){return new Yn(t,e,n,r,[void 0,Ft(t.ArrayType,s),Ct(i)])}static Decimal(t,e,n,r,i,s){return new Yn(t,e,n,r,[void 0,Ft(t.ArrayType,s),Ct(i)])}static Date(t,e,n,r,i,s){return new Yn(t,e,n,r,[void 0,Ft(t.ArrayType,s),Ct(i)])}static Time(t,e,n,r,i,s){return new Yn(t,e,n,r,[void 0,Ft(t.ArrayType,s),Ct(i)])}static Timestamp(t,e,n,r,i,s){return new Yn(t,e,n,r,[void 0,Ft(t.ArrayType,s),Ct(i)])}static Interval(t,e,n,r,i,s){return new Yn(t,e,n,r,[void 0,Ft(t.ArrayType,s),Ct(i)])}static FixedSizeBinary(t,e,n,r,i,s){return new Yn(t,e,n,r,[void 0,Ft(t.ArrayType,s),Ct(i)])}static Binary(t,e,n,r,i,s,o){return new Yn(t,e,n,r,[Mt(s),Ct(o),Ct(i)])}static Utf8(t,e,n,r,i,s,o){return new Yn(t,e,n,r,[Mt(s),Ct(o),Ct(i)])}static List(t,e,n,r,i,s,o){return new Yn(t,e,n,r,[Mt(s),void 0,Ct(i)],[o])}static FixedSizeList(t,e,n,r,i,s){return new Yn(t,e,n,r,[void 0,void 0,Ct(i)],[s])}static Struct(t,e,n,r,i,s){return new Yn(t,e,n,r,[void 0,void 0,Ct(i)],s)}static Map(t,e,n,r,i,s,o){return new Yn(t,e,n,r,[Mt(s),void 0,Ct(i)],[o])}static Union(t,e,n,r,i,s,o,a){const c=[void 0,void 0,Ct(i),Ft(t.ArrayType,s)];return t.mode===je.Sparse?new Yn(t,e,n,r,c,o):(c[Te.OFFSET]=Mt(o),new Yn(t,e,n,r,c,a))}}Yn.prototype.childData=Object.freeze([]);const Wn=void 0;function Hn(t){if(null===t)return"null";if(t===Wn)return"undefined";switch(typeof t){case"number":return""+t;case"bigint":return""+t;case"string":return`"${t}"`}return"function"===typeof t[Symbol.toPrimitive]?t[Symbol.toPrimitive]("string"):ArrayBuffer.isView(t)?`[${t}]`:JSON.stringify(t)}function Kn(t){if(!t||t.length<=0)return function(t){return!0};let e="",n=t.filter(t=>t===t);return n.length>0&&(e=`\n switch (x) {${n.map(t=>`\n case ${Gn(t)}:`).join("")}\n return false;\n }`),t.length!==n.length&&(e="if (x !== x) return false;\n"+e),new Function("x",e+"\nreturn true;")}function Gn(t){return"bigint"!==typeof t?Hn(t):ot?Hn(t)+"n":`"${Hn(t)}"`}const qn=(t,e)=>(t*e+63&-64||64)/e,Jn=(t,e=0)=>t.length>=e?t.subarray(0,e):jt(new t.constructor(e),t,0);class Zn{constructor(t,e=1){this.buffer=t,this.stride=e,this.BYTES_PER_ELEMENT=t.BYTES_PER_ELEMENT,this.ArrayType=t.constructor,this._resize(this.length=t.length/e|0)}get byteLength(){return this.length*this.stride*this.BYTES_PER_ELEMENT|0}get reservedLength(){return this.buffer.length/this.stride}get reservedByteLength(){return this.buffer.byteLength}set(t,e){return this}append(t){return this.set(this.length,t)}reserve(t){if(t>0){this.length+=t;const e=this.stride,n=this.length*e,r=this.buffer.length;n>=r&&this._resize(qn(0===r?1*n:2*n,this.BYTES_PER_ELEMENT))}return this}flush(t=this.length){t=qn(t*this.stride,this.BYTES_PER_ELEMENT);const e=Jn(this.buffer,t);return this.clear(),e}clear(){return this.length=0,this._resize(0),this}_resize(t){return this.buffer=jt(new this.ArrayType(t),this.buffer)}}Zn.prototype.offset=0;class Xn extends Zn{last(){return this.get(this.length-1)}get(t){return this.buffer[t]}set(t,e){return this.reserve(t-this.length+1),this.buffer[t*this.stride]=e,this}}class Qn extends Xn{constructor(t=new Uint8Array(0)){super(t,1/8),this.numValid=0}get numInvalid(){return this.length-this.numValid}get(t){return this.buffer[t>>3]>>t%8&1}set(t,e){const{buffer:n}=this.reserve(t-this.length+1),r=t>>3,i=t%8,s=n[r]>>i&1;return e?0===s&&(n[r]|=1<this.length&&this.set(t-1,0),super.flush(t+1)}}class er extends Zn{get ArrayType64(){return this._ArrayType64||(this._ArrayType64=this.buffer instanceof Int32Array?at:ut)}set(t,e){switch(this.reserve(t-this.length+1),typeof e){case"bigint":this.buffer64[t]=e;break;case"number":this.buffer[t*this.stride]=e;break;default:this.buffer.set(e,t*this.stride)}return this}_resize(t){const e=super._resize(t),n=e.byteLength/(this.BYTES_PER_ELEMENT*this.stride);return ot&&(this.buffer64=new this.ArrayType64(e.buffer,e.byteOffset,n)),e}}class nr{constructor({type:t,nullValues:e}){this.length=0,this.finished=!1,this.type=t,this.children=[],this.nullValues=e,this.stride=zn(t),this._nulls=new Qn,e&&e.length>0&&(this._isValid=Kn(e))}static new(t){}static throughNode(t){throw new Error('"throughNode" not available in this environment')}static throughDOM(t){throw new Error('"throughDOM" not available in this environment')}static throughIterable(t){return sr(t)}static throughAsyncIterable(t){return or(t)}toVector(){return we.new(this.flush())}get ArrayType(){return this.type.ArrayType}get nullCount(){return this._nulls.numInvalid}get numChildren(){return this.children.length}get byteLength(){let t=0;return this._offsets&&(t+=this._offsets.byteLength),this._values&&(t+=this._values.byteLength),this._nulls&&(t+=this._nulls.byteLength),this._typeIds&&(t+=this._typeIds.byteLength),this.children.reduce((t,e)=>t+e.byteLength,t)}get reservedLength(){return this._nulls.reservedLength}get reservedByteLength(){let t=0;return this._offsets&&(t+=this._offsets.reservedByteLength),this._values&&(t+=this._values.reservedByteLength),this._nulls&&(t+=this._nulls.reservedByteLength),this._typeIds&&(t+=this._typeIds.reservedByteLength),this.children.reduce((t,e)=>t+e.reservedByteLength,t)}get valueOffsets(){return this._offsets?this._offsets.buffer:null}get values(){return this._values?this._values.buffer:null}get nullBitmap(){return this._nulls?this._nulls.buffer:null}get typeIds(){return this._typeIds?this._typeIds.buffer:null}append(t){return this.set(this.length,t)}isValid(t){return this._isValid(t)}set(t,e){return this.setValid(t,this.isValid(e))&&this.setValue(t,e),this}setValue(t,e){this._setValue(this,t,e)}setValid(t,e){return this.length=this._nulls.set(t,+e).length,e}addChild(t,e=""+this.numChildren){throw new Error(`Cannot append children to non-nested type "${this.type}"`)}getChildAt(t){return this.children[t]||null}flush(){const t=[],e=this._values,n=this._offsets,r=this._typeIds,{length:i,nullCount:s}=this;r?(t[Te.TYPE]=r.flush(i),n&&(t[Te.OFFSET]=n.flush(i))):n?(e&&(t[Te.DATA]=e.flush(n.last())),t[Te.OFFSET]=n.flush(i)):e&&(t[Te.DATA]=e.flush(i)),s>0&&(t[Te.VALIDITY]=this._nulls.flush(i));const o=Yn.new(this.type,0,i,s,t,this.children.map(t=>t.flush()));return this.clear(),o}finish(){return this.finished=!0,this.children.forEach(t=>t.finish()),this}clear(){return this.length=0,this._offsets&&this._offsets.clear(),this._values&&this._values.clear(),this._nulls&&this._nulls.clear(),this._typeIds&&this._typeIds.clear(),this.children.forEach(t=>t.clear()),this}}nr.prototype.length=1,nr.prototype.stride=1,nr.prototype.children=null,nr.prototype.finished=!1,nr.prototype.nullValues=null,nr.prototype._isValid=()=>!0;class rr extends nr{constructor(t){super(t),this._values=new Xn(new this.ArrayType(0),this.stride)}setValue(t,e){const n=this._values;return n.reserve(t-n.length+1),super.setValue(t,e)}}class ir extends nr{constructor(t){super(t),this._pendingLength=0,this._offsets=new tr}setValue(t,e){const n=this._pending||(this._pending=new Map),r=n.get(t);r&&(this._pendingLength-=r.length),this._pendingLength+=e.length,n.set(t,e)}setValid(t,e){return!!super.setValid(t,e)||((this._pending||(this._pending=new Map)).set(t,void 0),!1)}clear(){return this._pendingLength=0,this._pending=void 0,super.clear()}flush(){return this._flush(),super.flush()}finish(){return this._flush(),super.finish()}_flush(){const t=this._pending,e=this._pendingLength;return this._pendingLength=0,this._pending=void 0,t&&t.size>0&&this._flushPending(t,e),this}}function sr(t){const{["queueingStrategy"]:e="count"}=t,{["highWaterMark"]:n=("bytes"!==e?1e3:16384)}=t,r="bytes"!==e?"length":"byteLength";return function*(e){let i=0,s=nr.new(t);for(const t of e)s.append(t)[r]>=n&&++i&&(yield s.toVector());(s.finish().length>0||0===i)&&(yield s.toVector())}}function or(t){const{["queueingStrategy"]:e="count"}=t,{["highWaterMark"]:n=("bytes"!==e?1e3:16384)}=t,r="bytes"!==e?"length":"byteLength";return async function*(e){let i=0,s=nr.new(t);for await(const t of e)s.append(t)[r]>=n&&++i&&(yield s.toVector());(s.finish().length>0||0===i)&&(yield s.toVector())}}class ar extends nr{constructor(t){super(t),this._values=new Qn}setValue(t,e){this._values.set(t,+e)}}class cr extends nr{setValue(t,e){}setValid(t,e){return this.length=Math.max(t+1,this.length),e}}class ur extends rr{}class lr extends ur{}class hr extends ur{}class fr extends rr{}class dr extends nr{constructor({type:t,nullValues:e,dictionaryHashFunction:n}){super({type:new Pn(t.dictionary,t.indices,t.id,t.isOrdered)}),this._nulls=null,this._dictionaryOffset=0,this._keysToIndices=Object.create(null),this.indices=nr.new({type:this.type.indices,nullValues:e}),this.dictionary=nr.new({type:this.type.dictionary,nullValues:null}),"function"===typeof n&&(this.valueToKey=n)}get values(){return this.indices.values}get nullCount(){return this.indices.nullCount}get nullBitmap(){return this.indices.nullBitmap}get byteLength(){return this.indices.byteLength+this.dictionary.byteLength}get reservedLength(){return this.indices.reservedLength+this.dictionary.reservedLength}get reservedByteLength(){return this.indices.reservedByteLength+this.dictionary.reservedByteLength}isValid(t){return this.indices.isValid(t)}setValid(t,e){const n=this.indices;return e=n.setValid(t,e),this.length=n.length,e}setValue(t,e){let n=this._keysToIndices,r=this.valueToKey(e),i=n[r];return void 0===i&&(n[r]=i=this._dictionaryOffset+this.dictionary.append(e).length-1),this.indices.setValue(t,i)}flush(){const t=this.type,e=this._dictionary,n=this.dictionary.toVector(),r=this.indices.flush().clone(t);return r.dictionary=e?e.concat(n):n,this.finished||(this._dictionaryOffset+=n.length),this._dictionary=r.dictionary,this.clear(),r}finish(){return this.indices.finish(),this.dictionary.finish(),this._dictionaryOffset=0,this._keysToIndices=Object.create(null),super.finish()}clear(){return this.indices.clear(),this.dictionary.clear(),super.clear()}valueToKey(t){return"string"===typeof t?t:""+t}}class pr extends rr{}const yr=new Float64Array(1),br=new Uint32Array(yr.buffer);function gr(t){let e=(31744&t)>>10,n=(1023&t)/1024,r=(-1)**((32768&t)>>15);switch(e){case 31:return r*(n?NaN:1/0);case 0:return r*(n?6103515625e-14*n:0)}return r*2**(e-15)*(1+n)}function mr(t){if(t!==t)return 32256;yr[0]=t;let e=(2147483648&br[1])>>16&65535,n=2146435072&br[1],r=0;return n>=1089470464?br[0]>0?n=31744:(n=(2080374784&n)>>16,r=(1048575&br[1])>>10):n<=1056964608?(r=1048576+(1048575&br[1]),r=1048576+(r<<(n>>20)-998)>>21,n=0):(n=n-1056964608>>10,r=512+(1048575&br[1])>>10),e|n|65535&r}class vr extends rr{}class _r extends vr{setValue(t,e){this._values.set(t,mr(e))}}class wr extends vr{setValue(t,e){this._values.set(t,e)}}class Ir extends vr{setValue(t,e){this._values.set(t,e)}}const Sr=Symbol.for("isArrowBigNum");function Or(t,...e){return 0===e.length?Object.setPrototypeOf(Ft(this["TypedArray"],t),this.constructor.prototype):Object.setPrototypeOf(new this["TypedArray"](t,...e),this.constructor.prototype)}function Tr(...t){return Or.apply(this,t)}function Ar(...t){return Or.apply(this,t)}function xr(...t){return Or.apply(this,t)}function Br(t){let e,n,{buffer:r,byteOffset:i,length:s,signed:o}=t,a=new Int32Array(r,i,s),c=0,u=0,l=a.length;while(u>>=0),c+=(n>>>0)+e*u**32;return c}let jr,Dr;function Fr(t){let e="",n=new Uint32Array(2),r=new Uint16Array(t.buffer,t.byteOffset,t.byteLength/2),i=new Uint32Array((r=new Uint16Array(r).reverse()).buffer),s=-1,o=r.length-1;do{for(n[0]=r[s=0];s8===t.byteLength?new t["BigIntArray"](t.buffer,t.byteOffset,1)[0]:Fr(t),jr=t=>8===t.byteLength?""+new t["BigIntArray"](t.buffer,t.byteOffset,1)[0]:Fr(t)):(jr=Fr,Dr=jr);class Lr{constructor(t,e){return Lr.new(t,e)}static new(t,e){switch(e){case!0:return new Tr(t);case!1:return new Ar(t)}switch(t.constructor){case Int8Array:case Int16Array:case Int32Array:case at:return new Tr(t)}return 16===t.byteLength?new xr(t):new Ar(t)}static signed(t){return new Tr(t)}static unsigned(t){return new Ar(t)}static decimal(t){return new xr(t)}}class Er extends rr{setValue(t,e){this._values.set(t,e)}}class Mr extends Er{}class Ur extends Er{}class Cr extends Er{}class Nr extends Er{constructor(t){t["nullValues"]&&(t["nullValues"]=t["nullValues"].map(zr)),super(t),this._values=new er(new Int32Array(0),2)}get values64(){return this._values.buffer64}isValid(t){return super.isValid(zr(t))}}class kr extends Er{}class Vr extends Er{}class Rr extends Er{}class Pr extends Er{constructor(t){t["nullValues"]&&(t["nullValues"]=t["nullValues"].map(zr)),super(t),this._values=new er(new Uint32Array(0),2)}get values64(){return this._values.buffer64}isValid(t){return super.isValid(zr(t))}}const zr=(t=>e=>(ArrayBuffer.isView(e)&&(t.buffer=e.buffer,t.byteOffset=e.byteOffset,t.byteLength=e.byteLength,e=Dr(t),t.buffer=null),e))({BigIntArray:at});class $r extends rr{}class Yr extends $r{}class Wr extends $r{}class Hr extends $r{}class Kr extends $r{}class Gr extends rr{}class qr extends Gr{}class Jr extends Gr{}class Zr extends Gr{}class Xr extends Gr{}class Qr extends rr{}class ti extends Qr{}class ei extends Qr{}class ni extends ir{constructor(t){super(t),this._values=new Zn(new Uint8Array(0))}get byteLength(){let t=this._pendingLength+4*this.length;return this._offsets&&(t+=this._offsets.byteLength),this._values&&(t+=this._values.byteLength),this._nulls&&(t+=this._nulls.byteLength),t}setValue(t,e){return super.setValue(t,Ct(e))}_flushPending(t,e){const n=this._offsets,r=this._values.reserve(e).buffer;let i,s=0,o=0,a=0;for([s,i]of t)void 0===i?n.set(s,0):(o=i.length,r.set(i,a),n.set(s,o),a+=o)}}class ri extends ir{constructor(t){super(t),this._values=new Zn(new Uint8Array(0))}get byteLength(){let t=this._pendingLength+4*this.length;return this._offsets&&(t+=this._offsets.byteLength),this._values&&(t+=this._values.byteLength),this._nulls&&(t+=this._nulls.byteLength),t}setValue(t,e){return super.setValue(t,tt(e))}_flushPending(t,e){}}ri.prototype._flushPending=ni.prototype._flushPending;class ii{get length(){return this._values.length}get(t){return this._values[t]}clear(){return this._values=null,this}bind(t){return t instanceof we?t:(this._values=t,this)}}const si=Symbol.for("parent"),oi=Symbol.for("rowIndex"),ai=Symbol.for("keyToIdx"),ci=Symbol.for("idxToVal"),ui=Symbol.for("nodejs.util.inspect.custom");class li{constructor(t,e){this[si]=t,this.size=e}entries(){return this[Symbol.iterator]()}has(t){return void 0!==this.get(t)}get(t){let e=void 0;if(null!==t&&void 0!==t){const n=this[ai]||(this[ai]=new Map);let r=n.get(t);if(void 0!==r){const t=this[ci]||(this[ci]=new Array(this.size));void 0!==(e=t[r])||(t[r]=e=this.getValue(r))}else if((r=this.getIndex(t))>-1){n.set(t,r);const i=this[ci]||(this[ci]=new Array(this.size));void 0!==(e=i[r])||(i[r]=e=this.getValue(r))}}return e}set(t,e){if(null!==t&&void 0!==t){const n=this[ai]||(this[ai]=new Map);let r=n.get(t);if(void 0===r&&n.set(t,r=this.getIndex(t)),r>-1){const t=this[ci]||(this[ci]=new Array(this.size));t[r]=this.setValue(r,e)}}return this}clear(){throw new Error(`Clearing ${this[Symbol.toStringTag]} not supported.`)}delete(t){throw new Error(`Deleting ${this[Symbol.toStringTag]} values not supported.`)}*[Symbol.iterator](){const t=this.keys(),e=this.values(),n=this[ai]||(this[ai]=new Map),r=this[ci]||(this[ci]=new Array(this.size));for(let i,s,o,a,c=0;!(o=t.next()).done&&!(a=e.next()).done;++c)i=o.value,s=a.value,r[c]=s,n.has(i)||n.set(i,c),yield[i,s]}forEach(t,e){const n=this.keys(),r=this.values(),i=void 0===e?t:(n,r,i)=>t.call(e,n,r,i),s=this[ai]||(this[ai]=new Map),o=this[ci]||(this[ci]=new Array(this.size));for(let a,c,u,l,h=0;!(u=n.next()).done&&!(l=r.next()).done;++h)a=u.value,c=l.value,o[h]=c,s.has(a)||s.set(a,h),i(c,a,this)}toArray(){return[...this.values()]}toJSON(){const t={};return this.forEach((e,n)=>t[n]=e),t}inspect(){return this.toString()}[ui](){return this.toString()}toString(){const t=[];return this.forEach((e,n)=>{n=Hn(n),e=Hn(e),t.push(`${n}: ${e}`)}),`{ ${t.join(", ")} }`}}li[Symbol.toStringTag]=(t=>(Object.defineProperties(t,{size:{writable:!0,enumerable:!1,configurable:!1,value:0},[si]:{writable:!0,enumerable:!1,configurable:!1,value:null},[oi]:{writable:!0,enumerable:!1,configurable:!1,value:-1}}),t[Symbol.toStringTag]="Row"))(li.prototype);class hi extends li{constructor(t){return super(t,t.length),pi(this)}keys(){return this[si].getChildAt(0)[Symbol.iterator]()}values(){return this[si].getChildAt(1)[Symbol.iterator]()}getKey(t){return this[si].getChildAt(0).get(t)}getIndex(t){return this[si].getChildAt(0).indexOf(t)}getValue(t){return this[si].getChildAt(1).get(t)}setValue(t,e){this[si].getChildAt(1).set(t,e)}}class fi extends li{constructor(t){return super(t,t.type.children.length),di(this)}*keys(){for(const t of this[si].type.children)yield t.name}*values(){for(const t of this[si].type.children)yield this[t.name]}getKey(t){return this[si].type.children[t].name}getIndex(t){return this[si].type.children.findIndex(e=>e.name===t)}getValue(t){return this[si].getChildAt(t).get(this[oi])}setValue(t,e){return this[si].getChildAt(t).set(this[oi],e)}}Object.setPrototypeOf(li.prototype,Map.prototype);const di=(()=>{const t={enumerable:!0,configurable:!1,get:null,set:null};return e=>{let n=-1,r=e[ai]||(e[ai]=new Map);const i=t=>function(){return this.get(t)},s=t=>function(e){return this.set(t,e)};for(const o of e.keys())r.set(o,++n),t.get=i(o),t.set=s(o),e.hasOwnProperty(o)||(t.enumerable=!0,Object.defineProperty(e,o,t)),e.hasOwnProperty(n)||(t.enumerable=!1,Object.defineProperty(e,n,t));return t.get=t.set=null,e}})(),pi=(()=>{if("undefined"===typeof Proxy)return di;const t=li.prototype.has,e=li.prototype.get,n=li.prototype.set,r=li.prototype.getKey,i={isExtensible(){return!1},deleteProperty(){return!1},preventExtensions(){return!0},ownKeys(t){return[...t.keys()].map(t=>""+t)},has(t,e){switch(e){case"getKey":case"getIndex":case"getValue":case"setValue":case"toArray":case"toJSON":case"inspect":case"constructor":case"isPrototypeOf":case"propertyIsEnumerable":case"toString":case"toLocaleString":case"valueOf":case"size":case"has":case"get":case"set":case"clear":case"delete":case"keys":case"values":case"entries":case"forEach":case"__proto__":case"__defineGetter__":case"__defineSetter__":case"hasOwnProperty":case"__lookupGetter__":case"__lookupSetter__":case Symbol.iterator:case Symbol.toStringTag:case si:case oi:case ci:case ai:case ui:return!0}return"number"!==typeof e||t.has(e)||(e=t.getKey(e)),t.has(e)},get(n,i,s){switch(i){case"getKey":case"getIndex":case"getValue":case"setValue":case"toArray":case"toJSON":case"inspect":case"constructor":case"isPrototypeOf":case"propertyIsEnumerable":case"toString":case"toLocaleString":case"valueOf":case"size":case"has":case"get":case"set":case"clear":case"delete":case"keys":case"values":case"entries":case"forEach":case"__proto__":case"__defineGetter__":case"__defineSetter__":case"hasOwnProperty":case"__lookupGetter__":case"__lookupSetter__":case Symbol.iterator:case Symbol.toStringTag:case si:case oi:case ci:case ai:case ui:return Reflect.get(n,i,s)}return"number"!==typeof i||t.call(s,i)||(i=r.call(s,i)),e.call(s,i)},set(e,i,s,o){switch(i){case si:case oi:case ci:case ai:return Reflect.set(e,i,s,o);case"getKey":case"getIndex":case"getValue":case"setValue":case"toArray":case"toJSON":case"inspect":case"constructor":case"isPrototypeOf":case"propertyIsEnumerable":case"toString":case"toLocaleString":case"valueOf":case"size":case"has":case"get":case"set":case"clear":case"delete":case"keys":case"values":case"entries":case"forEach":case"__proto__":case"__defineGetter__":case"__defineSetter__":case"hasOwnProperty":case"__lookupGetter__":case"__lookupSetter__":case Symbol.iterator:case Symbol.toStringTag:return!1}return"number"!==typeof i||t.call(o,i)||(i=r.call(o,i)),!!t.call(o,i)&&!!n.call(o,i,s)}};return t=>new Proxy(t,i)})();function yi(t,e,n){const r=t.length,i=e>-1?e:r+e%r;return n?n(t,i):i}let bi;function gi(t,e,n,r){let{length:i=0}=t,s="number"!==typeof e?0:e,o="number"!==typeof n?i:n;return s<0&&(s=(s%i+i)%i),o<0&&(o=(o%i+i)%i),oi&&(o=i),r?r(t,s,o):[s,o]}const mi=ot?st(0):0,vi=t=>t!==t;function _i(t){let e=typeof t;if("object"!==e||null===t)return vi(t)?vi:"bigint"!==e?e=>e===t:e=>mi+e===t;if(t instanceof Date){const e=t.valueOf();return t=>t instanceof Date&&t.valueOf()===e}return ArrayBuffer.isView(t)?e=>!!e&&he(t,e):t instanceof Map?Ii(t):Array.isArray(t)?wi(t):t instanceof we?Si(t):Oi(t)}function wi(t){const e=[];for(let n=-1,r=t.length;++nn[++e]=_i(t)),Ti(n)}function Si(t){const e=[];for(let n=-1,r=t.length;++n!1;const n=[];for(let r=-1,i=e.length;++r{if(!n||"object"!==typeof n)return!1;switch(n.constructor){case Array:return Ai(t,n);case Map:case hi:case fi:return Bi(t,n,n.keys());case Object:case void 0:return Bi(t,n,e||Object.keys(n))}return n instanceof we&&xi(t,n)}}function Ai(t,e){const n=t.length;if(e.length!==n)return!1;for(let r=-1;++r`}get data(){return this._chunks[0]?this._chunks[0].data:null}get ArrayType(){return this._type.ArrayType}get numChildren(){return this._numChildren}get stride(){return this._chunks[0]?this._chunks[0].stride:1}get byteLength(){return this._chunks.reduce((t,e)=>t+e.byteLength,0)}get nullCount(){let t=this._nullCount;return t<0&&(this._nullCount=t=this._chunks.reduce((t,{nullCount:e})=>t+e,0)),t}get indices(){if(un.isDictionary(this._type)){if(!this._indices){const t=this._chunks;this._indices=1===t.length?t[0].indices:ji.concat(...t.map(t=>t.indices))}return this._indices}return null}get dictionary(){return un.isDictionary(this._type)?this._chunks[this._chunks.length-1].data.dictionary:null}*[Symbol.iterator](){for(const t of this._chunks)yield*t}clone(t=this._chunks){return new ji(this._type,t)}concat(...t){return this.clone(ji.flatten(this,...t))}slice(t,e){return gi(this,t,e,this._sliceInternal)}getChildAt(t){if(t<0||t>=this._numChildren)return null;let e,n,r,i=this._children||(this._children=[]);return(e=i[t])?e:(n=(this._type.children||[])[t])&&(r=this._chunks.map(e=>e.getChildAt(t)).filter(t=>null!=t),r.length>0)?i[t]=new ji(n.type,r):null}search(t,e){let n=t,r=this._chunkOffsets,i=r.length-1;if(n<0)return null;if(n>=r[i])return null;if(i<=1)return e?e(this,0,n):[0,n];let s=0,o=0,a=0;do{if(s+1===i)return e?e(this,s,n-o):[s,n-o];a=s+(i-s)/2|0,n>=r[a]?s=a:i=a}while(n=(o=r[s]));return null}isValid(t){return!!this.search(t,this.isValidInternal)}get(t){return this.search(t,this.getInternal)}set(t,e){this.search(t,({chunks:t},n,r)=>t[n].set(r,e))}indexOf(t,e){return e&&"number"===typeof e?this.search(e,(e,n,r)=>this.indexOfInternal(e,n,r,t)):this.indexOfInternal(this,0,Math.max(0,e||0),t)}toArray(){const{chunks:t}=this,e=t.length;let n=this._type.ArrayType;if(e<=0)return new n(0);if(e<=1)return t[0].toArray();let r=0,i=new Array(e);for(let a=-1;++a=n)break;if(e>=c+a)continue;if(c>=e&&c+a<=n){r.push(t);continue}const u=Math.max(0,e-c),l=Math.min(n-c,a);r.push(t.slice(u,l))}return t.clone(r)}}function Di(t){let e=new Uint32Array((t||[]).length+1),n=e[0]=0,r=e.length;for(let i=0;++i(e.set(t,n),n+t.length),Li=(t,e,n)=>{let r=n;for(let i=-1,s=t.length;++it>0)&&(t=t.clone({nullable:!0}));return new Ei(t,r)}get field(){return this._field}get name(){return this._field.name}get nullable(){return this._field.nullable}get metadata(){return this._field.metadata}clone(t=this._chunks){return new Ei(this._field,t)}getChildAt(t){if(t<0||t>=this.numChildren)return null;let e,n,r,i=this._children||(this._children=[]);return(e=i[t])?e:(n=(this.type.children||[])[t])&&(r=this._chunks.map(e=>e.getChildAt(t)).filter(t=>null!=t),r.length>0)?i[t]=new Ei(n,r):null}}class Mi extends Ei{constructor(t,e,n){super(t,[e],n),this._chunk=e}search(t,e){return e?e(this,0,t):[0,t]}isValid(t){return this._chunk.isValid(t)}get(t){return this._chunk.get(t)}set(t,e){this._chunk.set(t,e)}indexOf(t,e){return this._chunk.indexOf(t,e)}}const Ui=Array.isArray,Ci=(t,e)=>Pi(t,e,[],0),Ni=t=>{const[e,n]=Wi(t,[[],[]]);return n.map((t,n)=>t instanceof Ei?Ei.new(t.field.clone(e[n]),t):t instanceof we?Ei.new(e[n],t):Ei.new(e[n],[]))},ki=t=>Wi(t,[[],[]]),Vi=(t,e)=>zi(t,e,[],0),Ri=(t,e)=>$i(t,e,[],0);function Pi(t,e,n,r){let i,s=r,o=-1,a=e.length;while(++oi.getChildAt(e)),n,s).length:i instanceof we&&(n[s++]=i);return n}const Yi=(t,[e,n],r)=>(t[0][r]=e,t[1][r]=n,t);function Wi(t,e){let n,r;switch(r=t.length){case 0:return e;case 1:if(n=e[0],!t[0])return e;if(Ui(t[0]))return Wi(t[0],e);t[0]instanceof Yn||t[0]instanceof we||t[0]instanceof un||([n,t]=Object.entries(t[0]).reduce(Yi,e));break;default:Ui(n=t[r-1])?t=Ui(t[0])?t[0]:t.slice(0,r-1):(t=Ui(t[0])?t[0]:t,n=[])}let i,s,o=-1,a=-1,c=-1,u=t.length,[l,h]=e;while(++c`${e}: ${t}`).join(", ")} }>`}compareTo(t){return cn.compareSchemas(this,t)}select(...t){const e=t.reduce((t,e)=>(t[e]=!0)&&t,Object.create(null));return new Hi(this.fields.filter(t=>e[t.name]),this.metadata)}selectAt(...t){return new Hi(t.map(t=>this.fields[t]).filter(Boolean),this.metadata)}assign(...t){const e=t[0]instanceof Hi?t[0]:new Hi(Ci(Ki,t)),n=[...this.fields],r=Gi(Gi(new Map,this.metadata),e.metadata),i=e.fields.filter(t=>{const e=n.findIndex(e=>e.name===t.name);return!~e||(n[e]=t.clone({metadata:Gi(Gi(new Map,n[e].metadata),t.metadata)}))&&!1}),s=qi(i,new Map);return new Hi([...n,...i],r,new Map([...this.dictionaries,...s]))}}class Ki{constructor(t,e,n=!1,r){this.name=t,this.type=e,this.nullable=n,this.metadata=r||new Map}static new(...t){let[e,n,r,i]=t;return t[0]&&"object"===typeof t[0]&&(({name:e}=t[0]),void 0===n&&(n=t[0].type),void 0===r&&(r=t[0].nullable),void 0===i&&(i=t[0].metadata)),new Ki(""+e,n,r,i)}get typeId(){return this.type.typeId}get[Symbol.toStringTag](){return"Field"}toString(){return`${this.name}: ${this.type}`}compareTo(t){return cn.compareField(this,t)}clone(...t){let[e,n,r,i]=t;return t[0]&&"object"===typeof t[0]?({name:e=this.name,type:n=this.type,nullable:r=this.nullable,metadata:i=this.metadata}=t[0]):[e=this.name,n=this.type,r=this.nullable,i=this.metadata]=t,Ki.new(e,n,r,i)}}function Gi(t,e){return new Map([...t||new Map,...e||new Map])}function qi(t,e=new Map){for(let n=-1,r=t.length;++n0&&qi(i.children,e)}return e}Hi.prototype.fields=null,Hi.prototype.metadata=null,Hi.prototype.dictionaries=null,Ki.prototype.type=null,Ki.prototype.name=null,Ki.prototype.nullable=null,Ki.prototype.metadata=null;class Ji extends ir{constructor(t){super(t),this._run=new ii,this._offsets=new tr}addChild(t,e="0"){if(this.numChildren>0)throw new Error("ListBuilder can only have one child.");return this.children[this.numChildren]=t,this.type=new Mn(new Ki(e,t.type,!0)),this.numChildren-1}clear(){return this._run.clear(),super.clear()}_flushPending(t){const e=this._run,n=this._offsets,r=this._setValue;let i,s=0;for([s,i]of t)void 0===i?n.set(s,0):(n.set(s,i.length),r(this,s,e.bind(i)))}}class Zi extends nr{constructor(){super(...arguments),this._run=new ii}setValue(t,e){super.setValue(t,this._run.bind(e))}addChild(t,e="0"){if(this.numChildren>0)throw new Error("FixedSizeListBuilder can only have one child.");const n=this.children.push(t);return this.type=new kn(this.type.listSize,new Ki(e,t.type,!0)),n}clear(){return this._run.clear(),super.clear()}}class Xi extends ir{set(t,e){return super.set(t,e)}setValue(t,e){e=e instanceof Map?e:new Map(Object.entries(e));const n=this._pending||(this._pending=new Map),r=n.get(t);r&&(this._pendingLength-=r.size),this._pendingLength+=e.size,n.set(t,e)}addChild(t,e=""+this.numChildren){if(this.numChildren>0)throw new Error("ListBuilder can only have one child.");return this.children[this.numChildren]=t,this.type=new Vn(new Ki(e,t.type,!0),this.type.keysSorted),this.numChildren-1}_flushPending(t){const e=this._offsets,n=this._setValue;t.forEach((t,r)=>{void 0===t?e.set(r,0):(e.set(r,t.size),n(this,r,t))})}}class Qi extends nr{addChild(t,e=""+this.numChildren){const n=this.children.push(t);return this.type=new Un([...this.type.children,new Ki(e,t.type,!0)]),n}}class ts extends nr{constructor(t){super(t),this._typeIds=new Xn(new Int8Array(0),1),"function"===typeof t["valueToChildTypeId"]&&(this._valueToChildTypeId=t["valueToChildTypeId"])}get typeIdToChildIndex(){return this.type.typeIdToChildIndex}append(t,e){return this.set(this.length,t,e)}set(t,e,n){return void 0===n&&(n=this._valueToChildTypeId(this,e,t)),this.setValid(t,this.isValid(e))&&this.setValue(t,e,n),this}setValue(t,e,n){this._typeIds.set(t,n),super.setValue(t,e)}addChild(t,e=""+this.children.length){const n=this.children.push(t),{type:{children:r,mode:i,typeIds:s}}=this,o=[...r,new Ki(e,t.type)];return this.type=new Cn(i,[...s,n],o),n}_valueToChildTypeId(t,e,n){throw new Error("Cannot map UnionBuilder value to child typeId. Pass the `childTypeId` as the second argument to unionBuilder.append(), or supply a `valueToChildTypeId` function as part of the UnionBuilder constructor options.")}}class es extends ts{}class ns extends ts{constructor(t){super(t),this._offsets=new Xn(new Int32Array(0))}setValue(t,e,n){const r=this.type.typeIdToChildIndex[n];return this._offsets.set(t,this.getChildAt(r).length),super.setValue(t,e,n)}}class rs extends ze{}const is=(t,e,n)=>{t[e]=n/864e5|0},ss=(t,e,n)=>{t[e]=n%4294967296|0,t[e+1]=n/4294967296|0},os=(t,e,n)=>{t[e]=1e3*n%4294967296|0,t[e+1]=1e3*n/4294967296|0},as=(t,e,n)=>{t[e]=1e6*n%4294967296|0,t[e+1]=1e6*n/4294967296|0},cs=(t,e,n,r)=>{const{[n]:i,[n+1]:s}=e;null!=i&&null!=s&&t.set(r.subarray(0,s-i),i)},us=({offset:t,values:e},n,r)=>{const i=t+n;r?e[i>>3]|=1<>3]&=~(1<{is(t,e,n.valueOf())},hs=({values:t},e,n)=>{ss(t,2*e,n.valueOf())},fs=({stride:t,values:e},n,r)=>{e[t*n]=r},ds=({stride:t,values:e},n,r)=>{e[t*n]=mr(r)},ps=(t,e,n)=>{switch(typeof n){case"bigint":t.values64[e]=n;break;case"number":t.values[e*t.stride]=n;break;default:const r=n,{stride:i,ArrayType:s}=t,o=Ft(s,r);t.values.set(o.subarray(0,i),i*e)}},ys=({stride:t,values:e},n,r)=>{e.set(r.subarray(0,t),t*n)},bs=({values:t,valueOffsets:e},n,r)=>cs(t,e,n,r),gs=({values:t,valueOffsets:e},n,r)=>{cs(t,e,n,tt(r))},ms=(t,e,n)=>{t.type.bitWidth<64?fs(t,e,n):ps(t,e,n)},vs=(t,e,n)=>{t.type.precision!==Be.HALF?fs(t,e,n):ds(t,e,n)},_s=(t,e,n)=>{t.type.unit===Ae.DAY?ls(t,e,n):hs(t,e,n)},ws=({values:t},e,n)=>ss(t,2*e,n/1e3),Is=({values:t},e,n)=>ss(t,2*e,n),Ss=({values:t},e,n)=>os(t,2*e,n),Os=({values:t},e,n)=>as(t,2*e,n),Ts=(t,e,n)=>{switch(t.type.unit){case xe.SECOND:return ws(t,e,n);case xe.MILLISECOND:return Is(t,e,n);case xe.MICROSECOND:return Ss(t,e,n);case xe.NANOSECOND:return Os(t,e,n)}},As=({values:t,stride:e},n,r)=>{t[e*n]=r},xs=({values:t,stride:e},n,r)=>{t[e*n]=r},Bs=({values:t},e,n)=>{t.set(n.subarray(0,2),2*e)},js=({values:t},e,n)=>{t.set(n.subarray(0,2),2*e)},Ds=(t,e,n)=>{switch(t.type.unit){case xe.SECOND:return As(t,e,n);case xe.MILLISECOND:return xs(t,e,n);case xe.MICROSECOND:return Bs(t,e,n);case xe.NANOSECOND:return js(t,e,n)}},Fs=({values:t},e,n)=>{t.set(n.subarray(0,4),4*e)},Ls=(t,e,n)=>{const r=t.getChildAt(0),i=t.valueOffsets;for(let s=-1,o=i[e],a=i[e+1];o{const r=t.getChildAt(0),i=t.valueOffsets,s=n instanceof Map?[...n]:Object.entries(n);for(let o=-1,a=i[e],c=i[e+1];a(n,r,i)=>n&&n.set(t,e[i]),Us=(t,e)=>(n,r,i)=>n&&n.set(t,e.get(i)),Cs=(t,e)=>(n,r,i)=>n&&n.set(t,e.get(r.name)),Ns=(t,e)=>(n,r,i)=>n&&n.set(t,e[r.name]),ks=(t,e,n)=>{const r=n instanceof Map?Cs(e,n):n instanceof we?Us(e,n):Array.isArray(n)?Ms(e,n):Ns(e,n);t.type.children.forEach((e,n)=>r(t.getChildAt(n),e,n))},Vs=(t,e,n)=>{t.type.mode===je.Dense?Rs(t,e,n):Ps(t,e,n)},Rs=(t,e,n)=>{const r=t.typeIdToChildIndex[t.typeIds[e]],i=t.getChildAt(r);i&&i.set(t.valueOffsets[e],n)},Ps=(t,e,n)=>{const r=t.typeIdToChildIndex[t.typeIds[e]],i=t.getChildAt(r);i&&i.set(e,n)},zs=(t,e,n)=>{const r=t.getKey(e);null!==r&&t.setValue(r,n)},$s=(t,e,n)=>{t.type.unit===De.DAY_TIME?Ys(t,e,n):Ws(t,e,n)},Ys=({values:t},e,n)=>{t.set(n.subarray(0,2),2*e)},Ws=({values:t},e,n)=>{t[e]=12*n[0]+n[1]%12},Hs=(t,e,n)=>{const r=t.getChildAt(0),{stride:i}=t;for(let s=-1,o=e*i;++s0){const r=t["children"]||[],i={nullValues:t["nullValues"]},s=Array.isArray(r)?(t,e)=>r[e]||i:({name:t})=>r[t]||i;e.children.forEach((t,e)=>{const{type:r}=t,i=s(t,e);n.children.push(Js({...i,type:r}))})}return n}var Zs;nr.new=Js,Object.keys(Oe).map(t=>Oe[t]).filter(t=>"number"===typeof t&&t!==Oe.NONE).forEach(t=>{const e=qs.visit(t);e.prototype._setValue=Ks.getVisitFn(t)}),ri.prototype._setValue=Ks.visitBinary,function(t){(function(e){(function(e){(function(e){class n{constructor(){this.bb=null,this.bb_pos=0}__init(t,e){return this.bb_pos=t,this.bb=e,this}static getRootAsFooter(t,e){return(e||new n).__init(t.readInt32(t.position())+t.position(),t)}version(){let t=this.bb.__offset(this.bb_pos,4);return t?this.bb.readInt16(this.bb_pos+t):Ie.apache.arrow.flatbuf.MetadataVersion.V1}schema(t){let e=this.bb.__offset(this.bb_pos,6);return e?(t||new Ie.apache.arrow.flatbuf.Schema).__init(this.bb.__indirect(this.bb_pos+e),this.bb):null}dictionaries(e,n){let r=this.bb.__offset(this.bb_pos,8);return r?(n||new t.apache.arrow.flatbuf.Block).__init(this.bb.__vector(this.bb_pos+r)+24*e,this.bb):null}dictionariesLength(){let t=this.bb.__offset(this.bb_pos,8);return t?this.bb.__vector_len(this.bb_pos+t):0}recordBatches(e,n){let r=this.bb.__offset(this.bb_pos,10);return r?(n||new t.apache.arrow.flatbuf.Block).__init(this.bb.__vector(this.bb_pos+r)+24*e,this.bb):null}recordBatchesLength(){let t=this.bb.__offset(this.bb_pos,10);return t?this.bb.__vector_len(this.bb_pos+t):0}static startFooter(t){t.startObject(4)}static addVersion(t,e){t.addFieldInt16(0,e,Ie.apache.arrow.flatbuf.MetadataVersion.V1)}static addSchema(t,e){t.addFieldOffset(1,e,0)}static addDictionaries(t,e){t.addFieldOffset(2,e,0)}static startDictionariesVector(t,e){t.startVector(24,e,8)}static addRecordBatches(t,e){t.addFieldOffset(3,e,0)}static startRecordBatchesVector(t,e){t.startVector(24,e,8)}static endFooter(t){let e=t.endObject();return e}static finishFooterBuffer(t,e){t.finish(e)}static createFooter(t,e,r,i,s){return n.startFooter(t),n.addVersion(t,e),n.addSchema(t,r),n.addDictionaries(t,i),n.addRecordBatches(t,s),n.endFooter(t)}}e.Footer=n})(e.flatbuf||(e.flatbuf={}))})(e.arrow||(e.arrow={}))})(t.apache||(t.apache={}))}(Zs||(Zs={})),function(t){(function(t){(function(t){(function(t){class e{constructor(){this.bb=null,this.bb_pos=0}__init(t,e){return this.bb_pos=t,this.bb=e,this}offset(){return this.bb.readInt64(this.bb_pos)}metaDataLength(){return this.bb.readInt32(this.bb_pos+8)}bodyLength(){return this.bb.readInt64(this.bb_pos+16)}static createBlock(t,e,n,r){return t.prep(8,24),t.writeInt64(r),t.pad(4),t.writeInt32(n),t.writeInt64(e),t.offset()}}t.Block=e})(t.flatbuf||(t.flatbuf={}))})(t.arrow||(t.arrow={}))})(t.apache||(t.apache={}))}(Zs||(Zs={}));var Xs=U.Long,Qs=U.Builder,to=U.ByteBuffer,eo=Zs.apache.arrow.flatbuf.Block,no=Zs.apache.arrow.flatbuf.Footer;class ro{constructor(t,e=Le.V4,n,r){this.schema=t,this.version=e,n&&(this._recordBatches=n),r&&(this._dictionaryBatches=r)}static decode(t){t=new to(Ct(t));const e=no.getRootAsFooter(t),n=Hi.decode(e.schema());return new io(n,e)}static encode(t){const e=new Qs,n=Hi.encode(e,t.schema);no.startRecordBatchesVector(e,t.numRecordBatches),[...t.recordBatches()].slice().reverse().forEach(t=>so.encode(e,t));const r=e.endVector();no.startDictionariesVector(e,t.numDictionaries),[...t.dictionaryBatches()].slice().reverse().forEach(t=>so.encode(e,t));const i=e.endVector();return no.startFooter(e),no.addSchema(e,n),no.addVersion(e,Le.V4),no.addRecordBatches(e,r),no.addDictionaries(e,i),no.finishFooterBuffer(e,no.endFooter(e)),e.asUint8Array()}get numRecordBatches(){return this._recordBatches.length}get numDictionaries(){return this._dictionaryBatches.length}*recordBatches(){for(let t,e=-1,n=this.numRecordBatches;++e=0&&t=0&&t=0&&t=0&&t0)return super.write(t)}toString(t=!1){return t?Q(this.toUint8Array(!0)):this.toUint8Array(!1).then(Q)}toUint8Array(t=!1){return t?Dt(this._values)[0]:(async()=>{let t=[],e=0;for await(const n of this)t.push(n),e+=n.byteLength;return Dt(t,e)[0]})()}}class ao{constructor(t){t&&(this.source=new uo(fe.fromIterable(t)))}[Symbol.iterator](){return this}next(t){return this.source.next(t)}throw(t){return this.source.throw(t)}return(t){return this.source.return(t)}peek(t){return this.source.peek(t)}read(t){return this.source.read(t)}}class co{constructor(t){t instanceof co?this.source=t.source:t instanceof oo?this.source=new lo(fe.fromAsyncIterable(t)):Tt(t)?this.source=new lo(fe.fromNodeStream(t)):St(t)?this.source=new lo(fe.fromDOMStream(t)):wt(t)?this.source=new lo(fe.fromDOMStream(t.body)):bt(t)?this.source=new lo(fe.fromIterable(t)):(yt(t)||gt(t))&&(this.source=new lo(fe.fromAsyncIterable(t)))}[Symbol.asyncIterator](){return this}next(t){return this.source.next(t)}throw(t){return this.source.throw(t)}return(t){return this.source.return(t)}get closed(){return this.source.closed}cancel(t){return this.source.cancel(t)}peek(t){return this.source.peek(t)}read(t){return this.source.read(t)}}class uo{constructor(t){this.source=t}cancel(t){this.return(t)}peek(t){return this.next(t,"peek").value}read(t){return this.next(t,"read").value}next(t,e="read"){return this.source.next({cmd:e,size:t})}throw(t){return Object.create(this.source.throw&&this.source.throw(t)||et)}return(t){return Object.create(this.source.return&&this.source.return(t)||et)}}class lo{constructor(t){this.source=t,this._closedPromise=new Promise(t=>this._closedPromiseResolve=t)}async cancel(t){await this.return(t)}get closed(){return this._closedPromise}async read(t){return(await this.next(t,"read")).value}async peek(t){return(await this.next(t,"peek")).value}async next(t,e="read"){return await this.source.next({cmd:e,size:t})}async throw(t){const e=this.source.throw&&await this.source.throw(t)||et;return this._closedPromiseResolve&&this._closedPromiseResolve(),this._closedPromiseResolve=void 0,Object.create(e)}async return(t){const e=this.source.return&&await this.source.return(t)||et;return this._closedPromiseResolve&&this._closedPromiseResolve(),this._closedPromiseResolve=void 0,Object.create(e)}}class ho extends ao{constructor(t,e){super(),this.position=0,this.buffer=Ct(t),this.size="undefined"===typeof e?this.buffer.byteLength:e}readInt32(t){const{buffer:e,byteOffset:n}=this.readAt(t,4);return new DataView(e,n).getInt32(0,!0)}seek(t){return this.position=Math.min(t,this.size),t{this.size=(await t.stat()).size,delete this._pending})()}async readInt32(t){const{buffer:e,byteOffset:n}=await this.readAt(t,4);return new DataView(e,n).getInt32(0,!0)}async seek(t){return this._pending&&await this._pending,this.position=Math.min(t,this.size),t>>16,65535&this.buffer[1],this.buffer[0]>>>16,65535&this.buffer[0]]),n=new Uint32Array([t.buffer[1]>>>16,65535&t.buffer[1],t.buffer[0]>>>16,65535&t.buffer[0]]);let r=e[3]*n[3];this.buffer[0]=65535&r;let i=r>>>16;return r=e[2]*n[3],i+=r,r=e[3]*n[2]>>>0,i+=r,this.buffer[0]+=i<<16,this.buffer[1]=i>>>0>>16,this.buffer[1]+=e[1]*n[3]+e[2]*n[2]+e[3]*n[1],this.buffer[1]+=e[0]*n[3]+e[1]*n[2]+e[2]*n[1]+e[3]*n[0]<<16,this}_plus(t){const e=this.buffer[0]+t.buffer[0]>>>0;this.buffer[1]+=t.buffer[1],e>>0&&++this.buffer[1],this.buffer[0]=e}lessThan(t){return this.buffer[1]>>0,e[2]=this.buffer[2]+t.buffer[2]>>>0,e[1]=this.buffer[1]+t.buffer[1]>>>0,e[0]=this.buffer[0]+t.buffer[0]>>>0,e[0]>>0&&++e[1],e[1]>>0&&++e[2],e[2]>>0&&++e[3],this.buffer[3]=e[3],this.buffer[2]=e[2],this.buffer[1]=e[1],this.buffer[0]=e[0],this}hex(){return`${yo(this.buffer[3])} ${yo(this.buffer[2])} ${yo(this.buffer[1])} ${yo(this.buffer[0])}`}static multiply(t,e){let n=new wo(new Uint32Array(t.buffer));return n.times(e)}static add(t,e){let n=new wo(new Uint32Array(t.buffer));return n.plus(e)}static from(t,e=new Uint32Array(4)){return wo.fromString("string"===typeof t?t:t.toString(),e)}static fromNumber(t,e=new Uint32Array(4)){return wo.fromString(t.toString(),e)}static fromString(t,e=new Uint32Array(4)){const n=t.startsWith("-"),r=t.length;let i=new wo(e);for(let s=n?1:0;s0&&this.readData(t,n)||new Uint8Array(0)}readOffsets(t,e){return this.readData(t,e)}readTypeIds(t,e){return this.readData(t,e)}readData(t,{length:e,offset:n}=this.nextBufferRange()){return this.bytes.subarray(n,n+e)}readDictionary(t){return this.dictionaries.get(t.id)}}class So extends Io{constructor(t,e,n,r){super(new Uint8Array(0),e,n,r),this.sources=t}readNullBitmap(t,e,{offset:n}=this.nextBufferRange()){return e<=0?new Uint8Array(0):Ne(this.sources[n])}readOffsets(t,{offset:e}=this.nextBufferRange()){return Ft(Uint8Array,Ft(Int32Array,this.sources[e]))}readTypeIds(t,{offset:e}=this.nextBufferRange()){return Ft(Uint8Array,Ft(t.ArrayType,this.sources[e]))}readData(t,{offset:e}=this.nextBufferRange()){const{sources:n}=this;return un.isTimestamp(t)||(un.isInt(t)||un.isTime(t))&&64===t.bitWidth||un.isDate(t)&&t.unit===Ae.MILLISECOND?Ft(Uint8Array,_o.convertArray(n[e])):un.isDecimal(t)?Ft(Uint8Array,wo.convertArray(n[e])):un.isBinary(t)||un.isFixedSizeBinary(t)?Oo(n[e]):un.isBool(t)?Ne(n[e]):un.isUtf8(t)?tt(n[e].join("")):Ft(Uint8Array,Ft(t.ArrayType,n[e].map(t=>+t)))}}function Oo(t){const e=t.join(""),n=new Uint8Array(e.length/2);for(let r=0;r>1]=parseInt(e.substr(r,2),16);return n}var To=U.Long,Ao=Ie.apache.arrow.flatbuf.Null,xo=Ie.apache.arrow.flatbuf.Int,Bo=Ie.apache.arrow.flatbuf.FloatingPoint,jo=Ie.apache.arrow.flatbuf.Binary,Do=Ie.apache.arrow.flatbuf.Bool,Fo=Ie.apache.arrow.flatbuf.Utf8,Lo=Ie.apache.arrow.flatbuf.Decimal,Eo=Ie.apache.arrow.flatbuf.Date,Mo=Ie.apache.arrow.flatbuf.Time,Uo=Ie.apache.arrow.flatbuf.Timestamp,Co=Ie.apache.arrow.flatbuf.Interval,No=Ie.apache.arrow.flatbuf.List,ko=Ie.apache.arrow.flatbuf.Struct_,Vo=Ie.apache.arrow.flatbuf.Union,Ro=Ie.apache.arrow.flatbuf.DictionaryEncoding,Po=Ie.apache.arrow.flatbuf.FixedSizeBinary,zo=Ie.apache.arrow.flatbuf.FixedSizeList,$o=Ie.apache.arrow.flatbuf.Map;class Yo extends ze{visit(t,e){return null==t||null==e?void 0:super.visit(t,e)}visitNull(t,e){return Ao.startNull(e),Ao.endNull(e)}visitInt(t,e){return xo.startInt(e),xo.addBitWidth(e,t.bitWidth),xo.addIsSigned(e,t.isSigned),xo.endInt(e)}visitFloat(t,e){return Bo.startFloatingPoint(e),Bo.addPrecision(e,t.precision),Bo.endFloatingPoint(e)}visitBinary(t,e){return jo.startBinary(e),jo.endBinary(e)}visitBool(t,e){return Do.startBool(e),Do.endBool(e)}visitUtf8(t,e){return Fo.startUtf8(e),Fo.endUtf8(e)}visitDecimal(t,e){return Lo.startDecimal(e),Lo.addScale(e,t.scale),Lo.addPrecision(e,t.precision),Lo.endDecimal(e)}visitDate(t,e){return Eo.startDate(e),Eo.addUnit(e,t.unit),Eo.endDate(e)}visitTime(t,e){return Mo.startTime(e),Mo.addUnit(e,t.unit),Mo.addBitWidth(e,t.bitWidth),Mo.endTime(e)}visitTimestamp(t,e){const n=t.timezone&&e.createString(t.timezone)||void 0;return Uo.startTimestamp(e),Uo.addUnit(e,t.unit),void 0!==n&&Uo.addTimezone(e,n),Uo.endTimestamp(e)}visitInterval(t,e){return Co.startInterval(e),Co.addUnit(e,t.unit),Co.endInterval(e)}visitList(t,e){return No.startList(e),No.endList(e)}visitStruct(t,e){return ko.startStruct_(e),ko.endStruct_(e)}visitUnion(t,e){Vo.startTypeIdsVector(e,t.typeIds.length);const n=Vo.createTypeIdsVector(e,t.typeIds);return Vo.startUnion(e),Vo.addMode(e,t.mode),Vo.addTypeIds(e,n),Vo.endUnion(e)}visitDictionary(t,e){const n=this.visit(t.indices,e);return Ro.startDictionaryEncoding(e),Ro.addId(e,new To(t.id,0)),Ro.addIsOrdered(e,t.isOrdered),void 0!==n&&Ro.addIndexType(e,n),Ro.endDictionaryEncoding(e)}visitFixedSizeBinary(t,e){return Po.startFixedSizeBinary(e),Po.addByteWidth(e,t.byteWidth),Po.endFixedSizeBinary(e)}visitFixedSizeList(t,e){return zo.startFixedSizeList(e),zo.addListSize(e,t.listSize),zo.endFixedSizeList(e)}visitMap(t,e){return $o.startMap(e),$o.addKeysSorted(e,t.keysSorted),$o.endMap(e)}}const Wo=new Yo;function Ho(t,e=new Map){return new Hi(qo(t,e),ea(t["customMetadata"]),e)}function Ko(t){return new ma(t["count"],Zo(t["columns"]),Xo(t["columns"]))}function Go(t){return new va(Ko(t["data"]),t["id"],t["isDelta"])}function qo(t,e){return(t["fields"]||[]).filter(Boolean).map(t=>Ki.fromJSON(t,e))}function Jo(t,e){return(t["children"]||[]).filter(Boolean).map(t=>Ki.fromJSON(t,e))}function Zo(t){return(t||[]).reduce((t,e)=>[...t,new wa(e["count"],Qo(e["VALIDITY"])),...Zo(e["children"])],[])}function Xo(t,e=[]){for(let n=-1,r=(t||[]).length;++nt+ +(0===e),0)}function ta(t,e){let n,r,i,s,o,a;return e&&(s=t["dictionary"])?e.has(n=s["id"])?(r=(r=s["indexType"])?na(r):new pn,a=new Pn(e.get(n),r,n,s["isOrdered"]),i=new Ki(t["name"],a,t["nullable"],ea(t["customMetadata"]))):(r=(r=s["indexType"])?na(r):new pn,e.set(n,o=ra(t,Jo(t,e))),a=new Pn(o,r,n,s["isOrdered"]),i=new Ki(t["name"],a,t["nullable"],ea(t["customMetadata"]))):(o=ra(t,Jo(t,e)),i=new Ki(t["name"],o,t["nullable"],ea(t["customMetadata"]))),i||null}function ea(t){return new Map(Object.entries(t||{}))}function na(t){return new hn(t["isSigned"],t["bitWidth"])}function ra(t,e){const n=t["type"]["name"];switch(n){case"NONE":return new ln;case"null":return new ln;case"binary":return new On;case"utf8":return new Tn;case"bool":return new An;case"list":return new Mn((e||[])[0]);case"struct":return new Un(e||[]);case"struct_":return new Un(e||[])}switch(n){case"int":{const e=t["type"];return new hn(e["isSigned"],e["bitWidth"])}case"floatingpoint":{const e=t["type"];return new _n(Be[e["precision"]])}case"decimal":{const e=t["type"];return new xn(e["scale"],e["precision"])}case"date":{const e=t["type"];return new Bn(Ae[e["unit"]])}case"time":{const e=t["type"];return new Fn(xe[e["unit"]],e["bitWidth"])}case"timestamp":{const e=t["type"];return new Ln(xe[e["unit"]],e["timezone"])}case"interval":{const e=t["type"];return new En(De[e["unit"]])}case"union":{const n=t["type"];return new Cn(je[n["mode"]],n["typeIds"]||[],e||[])}case"fixedsizebinary":{const e=t["type"];return new Nn(e["byteWidth"])}case"fixedsizelist":{const n=t["type"];return new kn(n["listSize"],(e||[])[0])}case"map":{const n=t["type"];return new Vn((e||[])[0],n["keysSorted"])}}throw new Error(`Unrecognized type: "${n}"`)}var ia=U.Long,sa=U.Builder,oa=U.ByteBuffer,aa=Ie.apache.arrow.flatbuf.Type,ca=Ie.apache.arrow.flatbuf.Field,ua=Ie.apache.arrow.flatbuf.Schema,la=Ie.apache.arrow.flatbuf.Buffer,ha=Se.apache.arrow.flatbuf.Message,fa=Ie.apache.arrow.flatbuf.KeyValue,da=Se.apache.arrow.flatbuf.FieldNode,pa=Ie.apache.arrow.flatbuf.Endianness,ya=Se.apache.arrow.flatbuf.RecordBatch,ba=Se.apache.arrow.flatbuf.DictionaryBatch;class ga{constructor(t,e,n,r){this._version=e,this._headerType=n,this.body=new Uint8Array(0),r&&(this._createHeader=()=>r),this._bodyLength="number"===typeof t?t:t.low}static fromJSON(t,e){const n=new ga(0,Le.V4,e);return n._createHeader=Ia(t,e),n}static decode(t){t=new oa(Ct(t));const e=ha.getRootAsMessage(t),n=e.bodyLength(),r=e.version(),i=e.headerType(),s=new ga(n,r,i);return s._createHeader=Sa(e,i),s}static encode(t){let e=new sa,n=-1;return t.isSchema()?n=Hi.encode(e,t.header()):t.isRecordBatch()?n=ma.encode(e,t.header()):t.isDictionaryBatch()&&(n=va.encode(e,t.header())),ha.startMessage(e),ha.addVersion(e,Le.V4),ha.addHeader(e,n),ha.addHeaderType(e,t.headerType),ha.addBodyLength(e,new ia(t.bodyLength,0)),ha.finishMessageBuffer(e,ha.endMessage(e)),e.asUint8Array()}static from(t,e=0){if(t instanceof Hi)return new ga(0,Le.V4,Fe.Schema,t);if(t instanceof ma)return new ga(e,Le.V4,Fe.RecordBatch,t);if(t instanceof va)return new ga(e,Le.V4,Fe.DictionaryBatch,t);throw new Error("Unrecognized Message header: "+t)}get type(){return this.headerType}get version(){return this._version}get headerType(){return this._headerType}get bodyLength(){return this._bodyLength}header(){return this._createHeader()}isSchema(){return this.headerType===Fe.Schema}isRecordBatch(){return this.headerType===Fe.RecordBatch}isDictionaryBatch(){return this.headerType===Fe.DictionaryBatch}}class ma{get nodes(){return this._nodes}get length(){return this._length}get buffers(){return this._buffers}constructor(t,e,n){this._nodes=e,this._buffers=n,this._length="number"===typeof t?t:t.low}}class va{get id(){return this._id}get data(){return this._data}get isDelta(){return this._isDelta}get length(){return this.data.length}get nodes(){return this.data.nodes}get buffers(){return this.data.buffers}constructor(t,e,n=!1){this._data=t,this._isDelta=n,this._id="number"===typeof e?e:e.low}}class _a{constructor(t,e){this.offset="number"===typeof t?t:t.low,this.length="number"===typeof e?e:e.low}}class wa{constructor(t,e){this.length="number"===typeof t?t:t.low,this.nullCount="number"===typeof e?e:e.low}}function Ia(t,e){return()=>{switch(e){case Fe.Schema:return Hi.fromJSON(t);case Fe.RecordBatch:return ma.fromJSON(t);case Fe.DictionaryBatch:return va.fromJSON(t)}throw new Error(`Unrecognized Message type: { name: ${Fe[e]}, type: ${e} }`)}}function Sa(t,e){return()=>{switch(e){case Fe.Schema:return Hi.decode(t.header(new ua));case Fe.RecordBatch:return ma.decode(t.header(new ya),t.version());case Fe.DictionaryBatch:return va.decode(t.header(new ba),t.version())}throw new Error(`Unrecognized Message type: { name: ${Fe[e]}, type: ${e} }`)}}function Oa(t,e=new Map){const n=Fa(t,e);return new Hi(n,Ma(t),e)}function Ta(t,e=Le.V4){return new ma(t.length(),ja(t),Da(t,e))}function Aa(t,e=Le.V4){return new va(ma.decode(t.data(),e),t.id(),t.isDelta())}function xa(t){return new _a(t.offset(),t.length())}function Ba(t){return new wa(t.length(),t.nullCount())}function ja(t){const e=[];for(let n,r=-1,i=-1,s=t.nodesLength();++rKi.encode(t,e));ua.startFieldsVector(t,n.length);const r=ua.createFieldsVector(t,n),i=e.metadata&&e.metadata.size>0?ua.createCustomMetadataVector(t,[...e.metadata].map(([e,n])=>{const r=t.createString(""+e),i=t.createString(""+n);return fa.startKeyValue(t),fa.addKey(t,r),fa.addValue(t,i),fa.endKeyValue(t)})):-1;return ua.startSchema(t),ua.addFields(t,r),ua.addEndianness(t,$a?pa.Little:pa.Big),-1!==i&&ua.addCustomMetadata(t,i),ua.endSchema(t)}function ka(t,e){let n=-1,r=-1,i=-1,s=e.type,o=e.typeId;un.isDictionary(s)?(o=s.dictionary.typeId,i=Wo.visit(s,t),r=Wo.visit(s.dictionary,t)):r=Wo.visit(s,t);const a=(s.children||[]).map(e=>Ki.encode(t,e)),c=ca.createChildrenVector(t,a),u=e.metadata&&e.metadata.size>0?ca.createCustomMetadataVector(t,[...e.metadata].map(([e,n])=>{const r=t.createString(""+e),i=t.createString(""+n);return fa.startKeyValue(t),fa.addKey(t,r),fa.addValue(t,i),fa.endKeyValue(t)})):-1;return e.name&&(n=t.createString(e.name)),ca.startField(t),ca.addType(t,r),ca.addTypeType(t,o),ca.addChildren(t,c),ca.addNullable(t,!!e.nullable),-1!==n&&ca.addName(t,n),-1!==i&&ca.addDictionary(t,i),-1!==u&&ca.addCustomMetadata(t,u),ca.endField(t)}function Va(t,e){const n=e.nodes||[],r=e.buffers||[];ya.startNodesVector(t,n.length),n.slice().reverse().forEach(e=>wa.encode(t,e));const i=t.endVector();ya.startBuffersVector(t,r.length),r.slice().reverse().forEach(e=>_a.encode(t,e));const s=t.endVector();return ya.startRecordBatch(t),ya.addLength(t,new ia(e.length,0)),ya.addNodes(t,i),ya.addBuffers(t,s),ya.endRecordBatch(t)}function Ra(t,e){const n=ma.encode(t,e.data);return ba.startDictionaryBatch(t),ba.addId(t,new ia(e.id,0)),ba.addIsDelta(t,e.isDelta),ba.addData(t,n),ba.endDictionaryBatch(t)}function Pa(t,e){return da.createFieldNode(t,new ia(e.length,0),new ia(e.nullCount,0))}function za(t,e){return la.createBuffer(t,new ia(e.offset,0),new ia(e.length,0))}Ki["encode"]=ka,Ki["decode"]=Ea,Ki["fromJSON"]=ta,Hi["encode"]=Na,Hi["decode"]=Oa,Hi["fromJSON"]=Ho,ma["encode"]=Va,ma["decode"]=Ta,ma["fromJSON"]=Ko,va["encode"]=Ra,va["decode"]=Aa,va["fromJSON"]=Go,wa["encode"]=Pa,wa["decode"]=Ba,_a["encode"]=za,_a["decode"]=xa;const $a=function(){const t=new ArrayBuffer(2);return new DataView(t).setInt16(0,256,!0),256===new Int16Array(t)[0]}();var Ya=U.ByteBuffer;const Wa=t=>`Expected ${Fe[t]} Message in stream, but was null or length 0.`,Ha=t=>`Header pointer of flatbuffer-encoded ${Fe[t]} Message is null or length 0.`,Ka=(t,e)=>`Expected to read ${t} metadata bytes, but only read ${e}.`,Ga=(t,e)=>`Expected to read ${t} bytes for message body, but only read ${e}.`;class qa{constructor(t){this.source=t instanceof ao?t:new ao(t)}[Symbol.iterator](){return this}next(){let t;return(t=this.readMetadataLength()).done||-1===t.value&&(t=this.readMetadataLength()).done||(t=this.readMetadata(t.value)).done?et:t}throw(t){return this.source.throw(t)}return(t){return this.source.return(t)}readMessage(t){let e;if((e=this.next()).done)return null;if(null!=t&&e.value.headerType!==t)throw new Error(Wa(t));return e.value}readMessageBody(t){if(t<=0)return new Uint8Array(0);const e=Ct(this.source.read(t));if(e.byteLength[...t,...n["VALIDITY"]&&[n["VALIDITY"]]||[],...n["TYPE"]&&[n["TYPE"]]||[],...n["OFFSET"]&&[n["OFFSET"]]||[],...n["DATA"]&&[n["DATA"]]||[],...e(n["children"])],[])}}readMessage(t){let e;if((e=this.next()).done)return null;if(null!=t&&e.value.headerType!==t)throw new Error(Wa(t));return e.value}readSchema(){const t=Fe.Schema,e=this.readMessage(t),n=e&&e.header();if(!e||!n)throw new Error(Ha(t));return n}}const Xa=4,Qa="ARROW1",tc=new Uint8Array(Qa.length);for(let $h=0;$h2147483647)throw new RangeError("Cannot write arrays larger than 2^31 - 1 in length");un.isNull(t.type)||oc.call(this,r<=0?new Uint8Array(0):Ce(e.offset,n,e.nullBitmap)),this.nodes.push(new wa(n,r))}return super.visit(t)}visitNull(t){return this}visitDictionary(t){return this.visit(t.indices)}get nodes(){return this._nodes}get buffers(){return this._buffers}get byteLength(){return this._byteLength}get bufferRegions(){return this._bufferRegions}}function oc(t){const e=t.byteLength+7&-8;return this.buffers.push(t),this.bufferRegions.push(new _a(this._byteLength,e)),this._byteLength+=e,this}function ac(t){const{type:e,length:n,typeIds:r,valueOffsets:i}=t;if(oc.call(this,r),e.mode===je.Sparse)return fc.call(this,t);if(e.mode===je.Dense){if(t.offset<=0)return oc.call(this,i),fc.call(this,t);{const s=r.reduce((t,e)=>Math.max(t,e),r[0]),o=new Int32Array(s+1),a=new Int32Array(s+1).fill(-1),c=new Int32Array(n),u=le(-i[0],n,i);for(let t,e,i=-1;++i=t.length?oc.call(this,new Uint8Array(0)):(e=t.values)instanceof Uint8Array?oc.call(this,Ce(t.offset,t.length,e)):oc.call(this,Ne(t))}function uc(t){return oc.call(this,t.values.subarray(0,t.length*t.stride))}function lc(t){const{length:e,values:n,valueOffsets:r}=t,i=r[0],s=r[e],o=Math.min(s-i,n.byteLength-i);return oc.call(this,le(-r[0],e,r)),oc.call(this,n.subarray(i,i+o)),this}function hc(t){const{length:e,valueOffsets:n}=t;return n&&oc.call(this,le(n[0],e,n)),this.visit(t.getChildAt(0))}function fc(t){return this.visitMany(t.type.children.map((e,n)=>t.getChildAt(n)).filter(Boolean))[0]}sc.prototype.visitBool=cc,sc.prototype.visitInt=uc,sc.prototype.visitFloat=uc,sc.prototype.visitUtf8=lc,sc.prototype.visitBinary=lc,sc.prototype.visitFixedSizeBinary=uc,sc.prototype.visitDate=uc,sc.prototype.visitTimestamp=uc,sc.prototype.visitTime=uc,sc.prototype.visitDecimal=uc,sc.prototype.visitList=hc,sc.prototype.visitStruct=fc,sc.prototype.visitUnion=ac,sc.prototype.visitInterval=uc,sc.prototype.visitFixedSizeList=hc,sc.prototype.visitMap=hc;class dc extends rt{constructor(t){super(),this._position=0,this._started=!1,this._sink=new oo,this._schema=null,this._dictionaryBlocks=[],this._recordBatchBlocks=[],this._dictionaryDeltaOffsets=new Map,pt(t)||(t={autoDestroy:!0,writeLegacyIpcFormat:!1}),this._autoDestroy="boolean"!==typeof t.autoDestroy||t.autoDestroy,this._writeLegacyIpcFormat="boolean"===typeof t.writeLegacyIpcFormat&&t.writeLegacyIpcFormat}static throughNode(t){throw new Error('"throughNode" not available in this environment')}static throughDOM(t,e){throw new Error('"throughDOM" not available in this environment')}toString(t=!1){return this._sink.toString(t)}toUint8Array(t=!1){return this._sink.toUint8Array(t)}writeAll(t){return yt(t)?t.then(t=>this.writeAll(t)):gt(t)?gc(this,t):bc(this,t)}get closed(){return this._sink.closed}[Symbol.asyncIterator](){return this._sink[Symbol.asyncIterator]()}toDOMStream(t){return this._sink.toDOMStream(t)}toNodeStream(t){return this._sink.toNodeStream(t)}close(){return this.reset()._sink.close()}abort(t){return this.reset()._sink.abort(t)}finish(){return this._autoDestroy?this.close():this.reset(this._sink,this._schema),this}reset(t=this._sink,e=null){return t===this._sink||t instanceof oo?this._sink=t:(this._sink=new oo,t&&It(t)?this.toDOMStream({type:"bytes"}).pipeTo(t):t&&Ot(t)&&this.toNodeStream({objectMode:!1}).pipe(t)),this._started&&this._schema&&this._writeFooter(this._schema),this._started=!1,this._dictionaryBlocks=[],this._recordBatchBlocks=[],this._dictionaryDeltaOffsets=new Map,e&&e.compareTo(this._schema)||(null===e?(this._position=0,this._schema=null):(this._started=!0,this._schema=e,this._writeSchema(e))),this}write(t){let e=null;if(!this._sink)throw new Error("RecordBatchWriter is closed");if(null===t||void 0===t)return this.finish()&&void 0;if(t instanceof zl&&!(e=t.schema))return this.finish()&&void 0;if(t instanceof Wl&&!(e=t.schema))return this.finish()&&void 0;if(e&&!e.compareTo(this._schema)){if(this._started&&this._autoDestroy)return this.close();this.reset(this._sink,e)}t instanceof Wl?t instanceof Hl||this._writeRecordBatch(t):t instanceof zl?this.writeAll(t.chunks):bt(t)&&this.writeAll(t)}_writeMessage(t,e=8){const n=e-1,r=ga.encode(t),i=r.byteLength,s=this._writeLegacyIpcFormat?4:8,o=i+s+n&~n,a=o-i-s;return t.headerType===Fe.RecordBatch?this._recordBatchBlocks.push(new so(o,t.bodyLength,this._position)):t.headerType===Fe.DictionaryBatch&&this._dictionaryBlocks.push(new so(o,t.bodyLength,this._position)),this._writeLegacyIpcFormat||this._write(Int32Array.of(-1)),this._write(Int32Array.of(o-s)),i>0&&this._write(r),this._writePadding(a)}_write(t){if(this._started){const e=Ct(t);e&&e.byteLength>0&&(this._sink.write(e),this._position+=e.byteLength)}return this}_writeSchema(t){return this._writeMessage(ga.from(t))}_writeFooter(t){return this._writeLegacyIpcFormat?this._write(Int32Array.of(0)):this._write(Int32Array.of(-1,0))}_writeMagic(){return this._write(tc)}_writePadding(t){return t>0?this._write(new Uint8Array(t)):this}_writeRecordBatch(t){const{byteLength:e,nodes:n,bufferRegions:r,buffers:i}=sc.assemble(t),s=new ma(t.length,n,r),o=ga.from(s,e);return this._writeDictionaries(t)._writeMessage(o)._writeBodyBuffers(i)}_writeDictionaryBatch(t,e,n=!1){this._dictionaryDeltaOffsets.set(e,t.length+(this._dictionaryDeltaOffsets.get(e)||0));const{byteLength:r,nodes:i,bufferRegions:s,buffers:o}=sc.assemble(t),a=new ma(t.length,i,s),c=new va(a,e,n),u=ga.from(c,r);return this._writeMessage(u)._writeBodyBuffers(o)}_writeBodyBuffers(t){let e,n,r;for(let i=-1,s=t.length;++i0&&(this._write(e),(r=(n+7&-8)-n)>0&&this._writePadding(r));return this}_writeDictionaries(t){for(let[e,n]of t.dictionaries){let t=this._dictionaryDeltaOffsets.get(e)||0;if(0===t||(n=n.slice(t)).length>0){const r="chunks"in n?n.chunks:[n];for(const n of r)this._writeDictionaryBatch(n,e,t>0),t+=n.length}}return this}}class pc extends dc{static writeAll(t,e){const n=new pc(e);return yt(t)?t.then(t=>n.writeAll(t)):gt(t)?gc(n,t):bc(n,t)}}class yc extends dc{constructor(){super(),this._autoDestroy=!0}static writeAll(t){const e=new yc;return yt(t)?t.then(t=>e.writeAll(t)):gt(t)?gc(e,t):bc(e,t)}_writeSchema(t){return this._writeMagic()._writePadding(2)}_writeFooter(t){const e=ro.encode(new ro(t,Le.V4,this._recordBatchBlocks,this._dictionaryBlocks));return super._writeFooter(t)._write(e)._write(Int32Array.of(e.byteLength))._writeMagic()}}function bc(t,e){let n=e;e instanceof zl&&(n=e.chunks,t.reset(void 0,e.schema));for(const r of n)t.write(r);return t.finish()}async function gc(t,e){for await(const n of e)t.write(n);return t.finish()}const mc=new Uint8Array(0),vc=t=>[mc,mc,new Uint8Array(t),mc];function _c(t,e,n=e.reduce((t,e)=>Math.max(t,e.length),0)){let r,i,s=-1,o=e.length;const a=[...t.fields],c=[],u=(n+63&-64)>>3;while(++st)),t)}function Ic(t,e){return Sc(t,e.map(t=>t instanceof ji?t.chunks.map(t=>t.data):[t.data]))}function Sc(t,e){const n=[...t.fields],r=[],i={numBatches:e.reduce((t,e)=>Math.max(t,e.length),0)};let s,o=0,a=0,c=-1,u=e.length,l=[];while(i.numBatches-- >0){for(a=Number.POSITIVE_INFINITY,c=-1;++c0&&(r[o++]=[a,l.slice()]))}return[t=new Hi(n,t.metadata),r.map(e=>new Wl(t,...e))]}function Oc(t,e,n,r,i){let s,o,a=0,c=-1,u=r.length;const l=(e+63&-64)>>3;while(++c=e?a===e?n[c]=s:(n[c]=s.slice(0,e),s=s.slice(e,a-e),i.numBatches=Math.max(i.numBatches,r[c].unshift(s))):((o=t[c]).nullable||(t[c]=o.clone({nullable:!0})),n[c]=s?s._changeLengthAndBackfillNullBitmap(e):Yn.new(o.type,0,e,e,vc(l)));return n}class Tc extends we{constructor(t,e){super(),this._children=e,this.numChildren=t.childData.length,this._bindDataAccessors(this.data=t)}get type(){return this.data.type}get typeId(){return this.data.typeId}get length(){return this.data.length}get offset(){return this.data.offset}get stride(){return this.data.stride}get nullCount(){return this.data.nullCount}get byteLength(){return this.data.byteLength}get VectorName(){return Oe[this.typeId]+"Vector"}get ArrayType(){return this.type.ArrayType}get values(){return this.data.values}get typeIds(){return this.data.typeIds}get nullBitmap(){return this.data.nullBitmap}get valueOffsets(){return this.data.valueOffsets}get[Symbol.toStringTag](){return`${this.VectorName}<${this.type[Symbol.toStringTag]}>`}clone(t,e=this._children){return we.new(t,e)}concat(...t){return ji.concat(this,...t)}slice(t,e){return gi(this,t,e,this._sliceInternal)}isValid(t){if(this.nullCount>0){const e=this.offset+t,n=this.nullBitmap[e>>3],r=n&1<=this.numChildren?null:(this._children||(this._children=[]))[t]||(this._children[t]=we.new(this.data.childData[t]))}toJSON(){return[...this]}_sliceInternal(t,e,n){return t.clone(t.data.slice(e,n-e),null)}_bindDataAccessors(t){}}Tc.prototype[Symbol.isConcatSpreadable]=!0;class Ac extends Tc{asUtf8(){return we.new(this.data.clone(new Tn))}}class xc extends Tc{static from(t){return Cl(()=>new An,t)}}class Bc extends Tc{static from(...t){return 2===t.length?Cl(()=>t[1]===Ae.DAY?new jn:new Dn,t[0]):Cl(()=>new Dn,t[0])}}class jc extends Bc{}class Dc extends Bc{}class Fc extends Tc{}class Lc extends Tc{constructor(t){super(t),this.indices=we.new(t.clone(this.type.indices))}static from(...t){if(3===t.length){const[e,n,r]=t,i=new Pn(e.type,n,null,null);return we.new(Yn.Dictionary(i,0,r.length,0,null,r,e))}return Cl(()=>t[0].type,t[0])}get dictionary(){return this.data.dictionary}reverseLookup(t){return this.dictionary.indexOf(t)}getKey(t){return this.indices.get(t)}getValue(t){return this.dictionary.get(t)}setKey(t,e){return this.indices.set(t,e)}setValue(t,e){return this.dictionary.set(t,e)}}Lc.prototype.indices=null;class Ec extends Tc{}class Mc extends Tc{}class Uc extends Tc{static from(t){let e=Pc(this);if(t instanceof ArrayBuffer||ArrayBuffer.isView(t)){let n=Rc(t.constructor)||e;if(null===e&&(e=n),e&&e===n){let n=new e,r=t.byteLength/n.ArrayType.BYTES_PER_ELEMENT;if(!Vc(e,t.constructor))return we.new(Yn.Float(n,0,r,0,null,t))}}if(e)return Cl(()=>new e,t);if(t instanceof DataView||t instanceof ArrayBuffer)throw new TypeError("Cannot infer float type from instance of "+t.constructor.name);throw new TypeError("Unrecognized FloatVector input")}}class Cc extends Uc{toFloat32Array(){return new Float32Array(this)}toFloat64Array(){return new Float64Array(this)}}class Nc extends Uc{}class kc extends Uc{}const Vc=(t,e)=>t===wn&&e!==Uint16Array,Rc=t=>{switch(t){case Uint16Array:return wn;case Float32Array:return In;case Float64Array:return Sn;default:return null}},Pc=t=>{switch(t){case Cc:return wn;case Nc:return In;case kc:return Sn;default:return null}};class zc extends Tc{}class $c extends zc{}class Yc extends zc{}class Wc extends Tc{static from(...t){let[e,n=!1]=t,r=nu(this,n);if(e instanceof ArrayBuffer||ArrayBuffer.isView(e)){let t=eu(e.constructor,n)||r;if(null===r&&(r=t),r&&r===t){let t=new r,n=e.byteLength/t.ArrayType.BYTES_PER_ELEMENT;return tu(r,e.constructor)&&(n*=.5),we.new(Yn.Int(t,0,n,0,null,e))}}if(r)return Cl(()=>new r,e);if(e instanceof DataView||e instanceof ArrayBuffer)throw new TypeError("Cannot infer integer type from instance of "+e.constructor.name);throw new TypeError("Unrecognized IntVector input")}}class Hc extends Wc{}class Kc extends Wc{}class Gc extends Wc{}class qc extends Wc{toBigInt64Array(){return Ut(this.values)}get values64(){return this._values64||(this._values64=this.toBigInt64Array())}}class Jc extends Wc{}class Zc extends Wc{}class Xc extends Wc{}class Qc extends Wc{toBigUint64Array(){return Vt(this.values)}get values64(){return this._values64||(this._values64=this.toBigUint64Array())}}const tu=(t,e)=>(t===yn||t===vn)&&(e===Int32Array||e===Uint32Array),eu=(t,e)=>{switch(t){case Int8Array:return fn;case Int16Array:return dn;case Int32Array:return e?yn:pn;case at:return yn;case Uint8Array:return bn;case Uint16Array:return gn;case Uint32Array:return e?vn:mn;case ut:return vn;default:return null}},nu=(t,e)=>{switch(t){case Hc:return fn;case Kc:return dn;case Gc:return e?yn:pn;case qc:return yn;case Jc:return bn;case Zc:return gn;case Xc:return e?vn:mn;case Qc:return vn;default:return null}};class ru extends Tc{}class iu extends Tc{asList(){const t=this.type.children[0];return we.new(this.data.clone(new Mn(t)))}bind(t){const e=this.getChildAt(0),{[t]:n,[t+1]:r}=this.valueOffsets;return new hi(e.slice(n,r))}}class su extends Tc{}const ou=Symbol.for("rowIndex");class au extends Tc{bind(t){const e=this._row||(this._row=new fi(this)),n=Object.create(e);return n[ou]=t,n}}class cu extends Tc{}class uu extends cu{}class lu extends cu{}class hu extends cu{}class fu extends cu{}class du extends Tc{}class pu extends du{}class yu extends du{}class bu extends du{}class gu extends du{}class mu extends Tc{get typeIdToChildIndex(){return this.data.type.typeIdToChildIndex}}class vu extends mu{get valueOffsets(){return this.data.valueOffsets}}class _u extends mu{}class wu extends Tc{static from(t){return Cl(()=>new Tn,t)}asBinary(){return we.new(this.data.clone(new On))}}function Iu(t){return function(){return t(this)}}function Su(t){return function(e){return t(this,e)}}function Ou(t){return function(e,n){return t(this,e,n)}}class Tu extends ze{}const Au=(t,e)=>864e5*t[e],xu=(t,e)=>4294967296*t[e+1]+(t[e]>>>0),Bu=(t,e)=>t[e+1]/1e3*4294967296+(t[e]>>>0)/1e3,ju=(t,e)=>t[e+1]/1e6*4294967296+(t[e]>>>0)/1e6,Du=t=>new Date(t),Fu=(t,e)=>Du(Au(t,e)),Lu=(t,e)=>Du(xu(t,e)),Eu=(t,e)=>null,Mu=(t,e,n)=>{const{[n]:r,[n+1]:i}=e;return null!=r&&null!=i?t.subarray(r,i):null},Uu=({offset:t,values:e},n)=>{const r=t+n,i=e[r>>3];return 0!==(i&1<Fu(t,e),Nu=({values:t},e)=>Lu(t,2*e),ku=({stride:t,values:e},n)=>e[t*n],Vu=({stride:t,values:e},n)=>gr(e[t*n]),Ru=({stride:t,values:e,type:n},r)=>Lr.new(e.subarray(t*r,t*(r+1)),n.isSigned),Pu=({stride:t,values:e},n)=>e.subarray(t*n,t*(n+1)),zu=({values:t,valueOffsets:e},n)=>Mu(t,e,n),$u=({values:t,valueOffsets:e},n)=>{const r=Mu(t,e,n);return null!==r?Q(r):null},Yu=(t,e)=>t.type.bitWidth<64?ku(t,e):Ru(t,e),Wu=(t,e)=>t.type.precision!==Be.HALF?ku(t,e):Vu(t,e),Hu=(t,e)=>t.type.unit===Ae.DAY?Cu(t,e):Nu(t,e),Ku=({values:t},e)=>1e3*xu(t,2*e),Gu=({values:t},e)=>xu(t,2*e),qu=({values:t},e)=>Bu(t,2*e),Ju=({values:t},e)=>ju(t,2*e),Zu=(t,e)=>{switch(t.type.unit){case xe.SECOND:return Ku(t,e);case xe.MILLISECOND:return Gu(t,e);case xe.MICROSECOND:return qu(t,e);case xe.NANOSECOND:return Ju(t,e)}},Xu=({values:t,stride:e},n)=>t[e*n],Qu=({values:t,stride:e},n)=>t[e*n],tl=({values:t},e)=>Lr.signed(t.subarray(2*e,2*(e+1))),el=({values:t},e)=>Lr.signed(t.subarray(2*e,2*(e+1))),nl=(t,e)=>{switch(t.type.unit){case xe.SECOND:return Xu(t,e);case xe.MILLISECOND:return Qu(t,e);case xe.MICROSECOND:return tl(t,e);case xe.NANOSECOND:return el(t,e)}},rl=({values:t},e)=>Lr.decimal(t.subarray(4*e,4*(e+1))),il=(t,e)=>{const n=t.getChildAt(0),{valueOffsets:r,stride:i}=t;return n.slice(r[e*i],r[e*i+1])},sl=(t,e)=>t.bind(e),ol=(t,e)=>t.bind(e),al=(t,e)=>t.type.mode===je.Dense?cl(t,e):ul(t,e),cl=(t,e)=>{const n=t.typeIdToChildIndex[t.typeIds[e]],r=t.getChildAt(n);return r?r.get(t.valueOffsets[e]):null},ul=(t,e)=>{const n=t.typeIdToChildIndex[t.typeIds[e]],r=t.getChildAt(n);return r?r.get(e):null},ll=(t,e)=>t.getValue(t.getKey(e)),hl=(t,e)=>t.type.unit===De.DAY_TIME?fl(t,e):dl(t,e),fl=({values:t},e)=>t.subarray(2*e,2*(e+1)),dl=({values:t},e)=>{const n=t[e],r=new Int32Array(2);return r[0]=n/12|0,r[1]=n%12|0,r},pl=(t,e)=>{const n=t.getChildAt(0),{stride:r}=t;return n.slice(e*r,(e+1)*r)};Tu.prototype.visitNull=Eu,Tu.prototype.visitBool=Uu,Tu.prototype.visitInt=Yu,Tu.prototype.visitInt8=ku,Tu.prototype.visitInt16=ku,Tu.prototype.visitInt32=ku,Tu.prototype.visitInt64=Ru,Tu.prototype.visitUint8=ku,Tu.prototype.visitUint16=ku,Tu.prototype.visitUint32=ku,Tu.prototype.visitUint64=Ru,Tu.prototype.visitFloat=Wu,Tu.prototype.visitFloat16=Vu,Tu.prototype.visitFloat32=ku,Tu.prototype.visitFloat64=ku,Tu.prototype.visitUtf8=$u,Tu.prototype.visitBinary=zu,Tu.prototype.visitFixedSizeBinary=Pu,Tu.prototype.visitDate=Hu,Tu.prototype.visitDateDay=Cu,Tu.prototype.visitDateMillisecond=Nu,Tu.prototype.visitTimestamp=Zu,Tu.prototype.visitTimestampSecond=Ku,Tu.prototype.visitTimestampMillisecond=Gu,Tu.prototype.visitTimestampMicrosecond=qu,Tu.prototype.visitTimestampNanosecond=Ju,Tu.prototype.visitTime=nl,Tu.prototype.visitTimeSecond=Xu,Tu.prototype.visitTimeMillisecond=Qu,Tu.prototype.visitTimeMicrosecond=tl,Tu.prototype.visitTimeNanosecond=el,Tu.prototype.visitDecimal=rl,Tu.prototype.visitList=il,Tu.prototype.visitStruct=ol,Tu.prototype.visitUnion=al,Tu.prototype.visitDenseUnion=cl,Tu.prototype.visitSparseUnion=ul,Tu.prototype.visitDictionary=ll,Tu.prototype.visitInterval=hl,Tu.prototype.visitIntervalDayTime=fl,Tu.prototype.visitIntervalYearMonth=dl,Tu.prototype.visitFixedSizeList=pl,Tu.prototype.visitMap=sl;const yl=new Tu;class bl extends ze{}function gl(t,e){return null===e&&t.length>0?0:-1}function ml(t,e){const{nullBitmap:n}=t;if(!n||t.nullCount<=0)return-1;let r=0;for(const i of ke(n,t.data.offset+(e||0),t.length,n,Ee)){if(!i)return r;++r}return-1}function vl(t,e,n){if(void 0===e)return-1;if(null===e)return ml(t,n);const r=_i(e);for(let i=(n||0)-1,s=t.length;++i0!==(r&1<0)return Sl(t);const{type:e,typeId:n,length:r}=t;return 1===t.stride&&(n===Oe.Timestamp||n===Oe.Int&&64!==e.bitWidth||n===Oe.Time&&64!==e.bitWidth||n===Oe.Float&&e.precision>0)?t.values.subarray(0,r)[Symbol.iterator]():function*(e){for(let n=-1;++nt+e,Dl=t=>"Cannot compute the byte width of variable-width column "+t;class Fl extends ze{visitNull(t){return 0}visitInt(t){return t.bitWidth/8}visitFloat(t){return t.ArrayType.BYTES_PER_ELEMENT}visitBinary(t){throw new Error(Dl(t))}visitUtf8(t){throw new Error(Dl(t))}visitBool(t){return 1/8}visitDecimal(t){return 16}visitDate(t){return 4*(t.unit+1)}visitTime(t){return t.bitWidth/8}visitTimestamp(t){return t.unit===xe.SECOND?4:8}visitInterval(t){return 4*(t.unit+1)}visitList(t){throw new Error(Dl(t))}visitStruct(t){return this.visitFields(t.children).reduce(jl,0)}visitUnion(t){return this.visitFields(t.children).reduce(jl,0)}visitFixedSizeBinary(t){return t.byteWidth}visitFixedSizeList(t){return t.listSize*this.visitFields(t.children).reduce(jl,0)}visitMap(t){return this.visitFields(t.children).reduce(jl,0)}visitDictionary(t){return this.visit(t.indices)}visitFields(t){return(t||[]).map(t=>this.visit(t.type))}visitSchema(t){return this.visitFields(t.fields).reduce(jl,0)}}const Ll=new Fl;class El extends ze{visitNull(){return su}visitBool(){return xc}visitInt(){return Wc}visitInt8(){return Hc}visitInt16(){return Kc}visitInt32(){return Gc}visitInt64(){return qc}visitUint8(){return Jc}visitUint16(){return Zc}visitUint32(){return Xc}visitUint64(){return Qc}visitFloat(){return Uc}visitFloat16(){return Cc}visitFloat32(){return Nc}visitFloat64(){return kc}visitUtf8(){return wu}visitBinary(){return Ac}visitFixedSizeBinary(){return Ec}visitDate(){return Bc}visitDateDay(){return jc}visitDateMillisecond(){return Dc}visitTimestamp(){return cu}visitTimestampSecond(){return uu}visitTimestampMillisecond(){return lu}visitTimestampMicrosecond(){return hu}visitTimestampNanosecond(){return fu}visitTime(){return du}visitTimeSecond(){return pu}visitTimeMillisecond(){return yu}visitTimeMicrosecond(){return bu}visitTimeNanosecond(){return gu}visitDecimal(){return Fc}visitList(){return ru}visitStruct(){return au}visitUnion(){return mu}visitDenseUnion(){return vu}visitSparseUnion(){return _u}visitDictionary(){return Lc}visitInterval(){return zc}visitIntervalDayTime(){return $c}visitIntervalYearMonth(){return Yc}visitFixedSizeList(){return Mc}visitMap(){return iu}}const Ml=new El;function Ul(t,...e){return new(Ml.getVisitFn(t)())(t,...e)}function Cl(t,e){if(bt(e))return we.from({nullValues:[null,void 0],type:t(),values:e});if(gt(e))return we.from({nullValues:[null,void 0],type:t(),values:e});const{values:n=[],type:r=t(),nullValues:i=[null,void 0]}={...e};return bt(n),we.from({nullValues:i,...e,type:r})}function Nl(t){const{values:e=[],...n}={nullValues:[null,void 0],...t};if(bt(e)){const t=[...nr.throughIterable(n)(e)];return 1===t.length?t[0]:ji.concat(t)}return(async t=>{const r=nr.throughAsyncIterable(n);for await(const n of r(e))t.push(n);return 1===t.length?t[0]:ji.concat(t)})([])}function kl(t){return function(){return t(this.type)}}function Vl(t){return function(e){return this.isValid(e)?t.call(this,e):null}}function Rl(t){return function(e,n){Ue(this.nullBitmap,this.offset+e,!(null===n||void 0===n))&&t.call(this,e,n)}}function Pl(){const t=this.nullBitmap;t&&t.byteLength>0&&(this.get=Vl(this.get),this.set=Rl(this.set))}we.new=Ul,we.from=Nl,Tc.prototype.get=function(t){return yl.visit(this,t)},Tc.prototype.set=function(t,e){return Ks.visit(this,t,e)},Tc.prototype.indexOf=function(t,e){return wl.visit(this,t,e)},Tc.prototype.toArray=function(){return Bl.visit(this)},Tc.prototype.getByteWidth=function(){return Ll.visit(this.type)},Tc.prototype[Symbol.iterator]=function(){return Tl.visit(this)},Tc.prototype._bindDataAccessors=Pl,Object.keys(Oe).map(t=>Oe[t]).filter(t=>"number"===typeof t).filter(t=>t!==Oe.NONE).forEach(t=>{const e=Ml.visit(t);e.prototype["get"]=Su(yl.getVisitFn(t)),e.prototype["set"]=Ou(Ks.getVisitFn(t)),e.prototype["indexOf"]=Ou(wl.getVisitFn(t)),e.prototype["toArray"]=Iu(Bl.getVisitFn(t)),e.prototype["getByteWidth"]=kl(Ll.getVisitFn(t)),e.prototype[Symbol.iterator]=Iu(Tl.getVisitFn(t))});class zl extends ji{constructor(...t){let e=null;t[0]instanceof Hi&&(e=t.shift());let n=Ci(Wl,t);if(!e&&!(e=n[0]&&n[0].schema))throw new TypeError("Table must be initialized with a Schema or at least one RecordBatch");n[0]||(n[0]=new Hl(e)),super(new Un(e.fields),n),this._schema=e,this._chunks=n}static empty(t=new Hi([])){return new zl(t,[])}static from(t){if(!t)return zl.empty();if("object"===typeof t){let e=bt(t["values"])?$l(t):gt(t["values"])?Yl(t):null;if(null!==e)return e}let e=Gl.from(t);return yt(e)?(async()=>await zl.from(await e))():e.isSync()&&(e=e.open())?e.schema?new zl(e.schema,[...e]):zl.empty():(async t=>{const e=await t,n=e.schema,r=[];if(n){for await(let t of e)r.push(t);return new zl(n,r)}return zl.empty()})(e.open())}static async fromAsync(t){return await zl.from(t)}static fromStruct(t){return zl.new(t.data.childData,t.type.children)}static new(...t){return new zl(...wc(Ni(t)))}get schema(){return this._schema}get length(){return this._length}get chunks(){return this._chunks}get numCols(){return this._numChildren}clone(t=this._chunks){return new zl(this._schema,t)}getColumn(t){return this.getColumnAt(this.getColumnIndex(t))}getColumnAt(t){return this.getChildAt(t)}getColumnIndex(t){return this._schema.fields.findIndex(e=>e.name===t)}getChildAt(t){if(t<0||t>=this.numChildren)return null;let e,n;const r=this._schema.fields,i=this._children||(this._children=[]);if(n=i[t])return n;if(e=r[t]){const n=this._chunks.map(e=>e.getChildAt(t)).filter(t=>null!=t);if(n.length>0)return i[t]=new Ei(e,n)}return null}serialize(t="binary",e=!0){const n=e?pc:yc;return n.writeAll(this).toUint8Array(!0)}count(){return this._length}select(...t){const e=this._schema.fields.reduce((t,e,n)=>t.set(e.name,n),new Map);return this.selectAt(...t.map(t=>e.get(t)).filter(t=>t>-1))}selectAt(...t){const e=this._schema.selectAt(...t);return new zl(e,this._chunks.map(({length:n,data:{childData:r}})=>new Wl(e,n,t.map(t=>r[t]).filter(Boolean))))}assign(t){const e=this._schema.fields,[n,r]=t.schema.fields.reduce((t,n,r)=>{const[i,s]=t,o=e.findIndex(t=>t.name===n.name);return~o?s[o]=r:i.push(r),t},[[],[]]),i=this._schema.assign(t.schema),s=[...e.map((e,n,i,s=r[n])=>void 0===s?this.getColumnAt(n):t.getColumnAt(s)),...n.map(e=>t.getColumnAt(e))].filter(Boolean);return new zl(...Ic(i,s))}}function $l(t){const{type:e}=t;return e instanceof Un?zl.fromStruct(au.from(t)):null}function Yl(t){const{type:e}=t;return e instanceof Un?au.from(t).then(t=>zl.fromStruct(t)):null}class Wl extends au{constructor(...t){let e,n,r=t[0];if(t[1]instanceof Yn)[,e,n]=t;else{const n=r.fields,[,i,s]=t;e=Yn.Struct(new Un(n),0,i,0,null,s)}super(e,n),this._schema=r}static from(t){return bt(t["values"]),zl.from(t)}static new(...t){const[e,n]=ki(t),r=n.filter(t=>t instanceof we);return new Wl(..._c(new Hi(e),r.map(t=>t.data)))}clone(t,e=this._children){return new Wl(this._schema,t,e)}concat(...t){const e=this._schema,n=ji.flatten(this,...t);return new zl(e,n.map(({data:t})=>new Wl(e,t)))}get schema(){return this._schema}get numCols(){return this._schema.fields.length}get dictionaries(){return this._dictionaries||(this._dictionaries=Kl.collect(this))}select(...t){const e=this._schema.fields.reduce((t,e,n)=>t.set(e.name,n),new Map);return this.selectAt(...t.map(t=>e.get(t)).filter(t=>t>-1))}selectAt(...t){const e=this._schema.selectAt(...t),n=t.map(t=>this.data.childData[t]).filter(Boolean);return new Wl(e,this.length,n)}}class Hl extends Wl{constructor(t){super(t,0,t.fields.map(t=>Yn.new(t.type,0,0,0)))}}class Kl extends ze{constructor(){super(...arguments),this.dictionaries=new Map}static collect(t){return(new Kl).visit(t.data,new Un(t.schema.fields)).dictionaries}visit(t,e){return un.isDictionary(e)?this.visitDictionary(t,e):(t.childData.forEach((t,n)=>this.visit(t,e.children[n].type)),this)}visitDictionary(t,e){const n=t.dictionary;return n&&n.length>0&&this.dictionaries.set(e.id,n),this}}class Gl extends rt{constructor(t){super(),this._impl=t}get closed(){return this._impl.closed}get schema(){return this._impl.schema}get autoDestroy(){return this._impl.autoDestroy}get dictionaries(){return this._impl.dictionaries}get numDictionaries(){return this._impl.numDictionaries}get numRecordBatches(){return this._impl.numRecordBatches}get footer(){return this._impl.isFile()?this._impl.footer:null}isSync(){return this._impl.isSync()}isAsync(){return this._impl.isAsync()}isFile(){return this._impl.isFile()}isStream(){return this._impl.isStream()}next(){return this._impl.next()}throw(t){return this._impl.throw(t)}return(t){return this._impl.return(t)}cancel(){return this._impl.cancel()}reset(t){return this._impl.reset(t),this._DOMStream=void 0,this._nodeStream=void 0,this}open(t){const e=this._impl.open(t);return yt(e)?e.then(()=>this):this}readRecordBatch(t){return this._impl.isFile()?this._impl.readRecordBatch(t):null}[Symbol.iterator](){return this._impl[Symbol.iterator]()}[Symbol.asyncIterator](){return this._impl[Symbol.asyncIterator]()}toDOMStream(){return fe.toDOMStream(this.isSync()?{[Symbol.iterator]:()=>this}:{[Symbol.asyncIterator]:()=>this})}toNodeStream(){return fe.toNodeStream(this.isSync()?{[Symbol.iterator]:()=>this}:{[Symbol.asyncIterator]:()=>this},{objectMode:!0})}static throughNode(t){throw new Error('"throughNode" not available in this environment')}static throughDOM(t,e){throw new Error('"throughDOM" not available in this environment')}static from(t){return t instanceof Gl?t:mt(t)?ch(t):_t(t)?hh(t):yt(t)?(async()=>await Gl.from(await t))():wt(t)||St(t)||Tt(t)||gt(t)?lh(new co(t)):uh(new ao(t))}static readAll(t){return t instanceof Gl?t.isSync()?oh(t):ah(t):mt(t)||ArrayBuffer.isView(t)||bt(t)||vt(t)?oh(t):ah(t)}}class ql extends Gl{constructor(t){super(t),this._impl=t}[Symbol.iterator](){return this._impl[Symbol.iterator]()}async*[Symbol.asyncIterator](){yield*this[Symbol.iterator]()}}class Jl extends Gl{constructor(t){super(t),this._impl=t}[Symbol.iterator](){throw new Error("AsyncRecordBatchStreamReader is not Iterable")}[Symbol.asyncIterator](){return this._impl[Symbol.asyncIterator]()}}class Zl extends ql{constructor(t){super(t),this._impl=t}}class Xl extends Jl{constructor(t){super(t),this._impl=t}}class Ql{constructor(t=new Map){this.closed=!1,this.autoDestroy=!0,this._dictionaryIndex=0,this._recordBatchIndex=0,this.dictionaries=t}get numDictionaries(){return this._dictionaryIndex}get numRecordBatches(){return this._recordBatchIndex}isSync(){return!1}isAsync(){return!1}isFile(){return!1}isStream(){return!1}reset(t){return this._dictionaryIndex=0,this._recordBatchIndex=0,this.schema=t,this.dictionaries=new Map,this}_loadRecordBatch(t,e){return new Wl(this.schema,t.length,this._loadVectors(t,e,this.schema.fields))}_loadDictionaryBatch(t,e){const{id:n,isDelta:r,data:i}=t,{dictionaries:s,schema:o}=this,a=s.get(n);if(r||!a){const t=o.dictionaries.get(n);return a&&r?a.concat(we.new(this._loadVectors(i,e,[t])[0])):we.new(this._loadVectors(i,e,[t])[0])}return a}_loadVectors(t,e,n){return new Io(e,t.nodes,t.buffers,this.dictionaries).visitMany(n)}}class th extends Ql{constructor(t,e){super(e),this._reader=mt(t)?new Za(this._handle=t):new qa(this._handle=t)}isSync(){return!0}isStream(){return!0}[Symbol.iterator](){return this}cancel(){!this.closed&&(this.closed=!0)&&(this.reset()._reader.return(),this._reader=null,this.dictionaries=null)}open(t){return this.closed||(this.autoDestroy=sh(this,t),this.schema||(this.schema=this._reader.readSchema())||this.cancel()),this}throw(t){return!this.closed&&this.autoDestroy&&(this.closed=!0)?this.reset()._reader.throw(t):et}return(t){return!this.closed&&this.autoDestroy&&(this.closed=!0)?this.reset()._reader.return(t):et}next(){if(this.closed)return et;let t,{_reader:e}=this;while(t=this._readNextMessageAndValidate())if(t.isSchema())this.reset(t.header());else{if(t.isRecordBatch()){this._recordBatchIndex++;const n=t.header(),r=e.readMessageBody(t.bodyLength),i=this._loadRecordBatch(n,r);return{done:!1,value:i}}if(t.isDictionaryBatch()){this._dictionaryIndex++;const n=t.header(),r=e.readMessageBody(t.bodyLength),i=this._loadDictionaryBatch(n,r);this.dictionaries.set(n.id,i)}}return this.schema&&0===this._recordBatchIndex?(this._recordBatchIndex++,{done:!1,value:new Hl(this.schema)}):this.return()}_readNextMessageAndValidate(t){return this._reader.readMessage(t)}}class eh extends Ql{constructor(t,e){super(e),this._reader=new Ja(this._handle=t)}isAsync(){return!0}isStream(){return!0}[Symbol.asyncIterator](){return this}async cancel(){!this.closed&&(this.closed=!0)&&(await this.reset()._reader.return(),this._reader=null,this.dictionaries=null)}async open(t){return this.closed||(this.autoDestroy=sh(this,t),this.schema||(this.schema=await this._reader.readSchema())||await this.cancel()),this}async throw(t){return!this.closed&&this.autoDestroy&&(this.closed=!0)?await this.reset()._reader.throw(t):et}async return(t){return!this.closed&&this.autoDestroy&&(this.closed=!0)?await this.reset()._reader.return(t):et}async next(){if(this.closed)return et;let t,{_reader:e}=this;while(t=await this._readNextMessageAndValidate())if(t.isSchema())await this.reset(t.header());else{if(t.isRecordBatch()){this._recordBatchIndex++;const n=t.header(),r=await e.readMessageBody(t.bodyLength),i=this._loadRecordBatch(n,r);return{done:!1,value:i}}if(t.isDictionaryBatch()){this._dictionaryIndex++;const n=t.header(),r=await e.readMessageBody(t.bodyLength),i=this._loadDictionaryBatch(n,r);this.dictionaries.set(n.id,i)}}return this.schema&&0===this._recordBatchIndex?(this._recordBatchIndex++,{done:!1,value:new Hl(this.schema)}):await this.return()}async _readNextMessageAndValidate(t){return await this._reader.readMessage(t)}}class nh extends th{constructor(t,e){super(t instanceof ho?t:new ho(t),e)}get footer(){return this._footer}get numDictionaries(){return this._footer?this._footer.numDictionaries:0}get numRecordBatches(){return this._footer?this._footer.numRecordBatches:0}isSync(){return!0}isFile(){return!0}open(t){if(!this.closed&&!this._footer){this.schema=(this._footer=this._readFooter()).schema;for(const t of this._footer.dictionaryBatches())t&&this._readDictionaryBatch(this._dictionaryIndex++)}return super.open(t)}readRecordBatch(t){if(this.closed)return null;this._footer||this.open();const e=this._footer&&this._footer.getRecordBatch(t);if(e&&this._handle.seek(e.offset)){const t=this._reader.readMessage(Fe.RecordBatch);if(t&&t.isRecordBatch()){const e=t.header(),n=this._reader.readMessageBody(t.bodyLength),r=this._loadRecordBatch(e,n);return r}}return null}_readDictionaryBatch(t){const e=this._footer&&this._footer.getDictionaryBatch(t);if(e&&this._handle.seek(e.offset)){const t=this._reader.readMessage(Fe.DictionaryBatch);if(t&&t.isDictionaryBatch()){const e=t.header(),n=this._reader.readMessageBody(t.bodyLength),r=this._loadDictionaryBatch(e,n);this.dictionaries.set(e.id,r)}}}_readFooter(){const{_handle:t}=this,e=t.size-rc,n=t.readInt32(e),r=t.readAt(e-n,n);return ro.decode(r)}_readNextMessageAndValidate(t){if(this._footer||this.open(),this._footer&&this._recordBatchIndex=4?ec(e)?new Zl(new nh(t.read())):new ql(new th(t)):new ql(new th(function*(){}()))}async function lh(t){const e=await t.peek(nc+7&-8);return e&&e.byteLength>=4?ec(e)?new Zl(new nh(await t.read())):new Jl(new eh(t)):new Jl(new eh(async function*(){}()))}async function hh(t){const{size:e}=await t.stat(),n=new fo(t,e);return e>=ic&&ec(await n.readAt(0,nc+7&-8))?new Xl(new rh(n)):new Jl(new eh(n))}function fh(t,e){if(gt(t))return ph(t,e);if(bt(t))return dh(t,e);throw new Error("toDOMStream() must be called with an Iterable or AsyncIterable")}function dh(t,e){let n=null;const r=e&&"bytes"===e.type||!1,i=e&&e.highWaterMark||2**24;return new ReadableStream({...e,start(e){s(e,n||(n=t[Symbol.iterator]()))},pull(t){n?s(t,n):t.close()},cancel(){n&&n.return&&n.return(),n=null}},{highWaterMark:r?i:void 0,...e});function s(t,e){let n,i=null,s=t.desiredSize||null;while(!(i=e.next(r?s:null)).done)if(ArrayBuffer.isView(i.value)&&(n=Ct(i.value))&&(null!=s&&r&&(s=s-n.byteLength+1),i.value=n),t.enqueue(i.value),null!=s&&--s<=0)return;t.close()}}function ph(t,e){let n=null;const r=e&&"bytes"===e.type||!1,i=e&&e.highWaterMark||2**24;return new ReadableStream({...e,async start(e){await s(e,n||(n=t[Symbol.asyncIterator]()))},async pull(t){n?await s(t,n):t.close()},async cancel(){n&&n.return&&await n.return(),n=null}},{highWaterMark:r?i:void 0,...e});async function s(t,e){let n,i=null,s=t.desiredSize||null;while(!(i=await e.next(r?s:null)).done)if(ArrayBuffer.isView(i.value)&&(n=Ct(i.value))&&(null!=s&&r&&(s=s-n.byteLength+1),i.value=n),t.enqueue(i.value),null!=s&&--s<=0)return;t.close()}}function yh(t){return new bh(t)}class bh{constructor(t){this._numChunks=0,this._finished=!1,this._bufferedSize=0;const{["readableStrategy"]:e,["writableStrategy"]:n,["queueingStrategy"]:r="count",...i}=t;this._controller=null,this._builder=nr.new(i),this._getSize="bytes"!==r?gh:mh;const{["highWaterMark"]:s=("bytes"===r?16384:1e3)}={...e},{["highWaterMark"]:o=("bytes"===r?16384:1e3)}={...n};this["readable"]=new ReadableStream({["cancel"]:()=>{this._builder.clear()},["pull"]:t=>{this._maybeFlush(this._builder,this._controller=t)},["start"]:t=>{this._maybeFlush(this._builder,this._controller=t)}},{highWaterMark:s,size:"bytes"!==r?gh:mh}),this["writable"]=new WritableStream({["abort"]:()=>{this._builder.clear()},["write"]:()=>{this._maybeFlush(this._builder,this._controller)},["close"]:()=>{this._maybeFlush(this._builder.finish(),this._controller)}},{highWaterMark:o,size:t=>this._writeValueAndReturnChunkSize(t)})}_writeValueAndReturnChunkSize(t){const e=this._bufferedSize;return this._bufferedSize=this._getSize(this._builder.append(t)),this._bufferedSize-e}_maybeFlush(t,e){null!==e&&(this._bufferedSize>=e.desiredSize&&++this._numChunks&&this._enqueue(e,t.toVector()),t.finished&&((t.length>0||0===this._numChunks)&&++this._numChunks&&this._enqueue(e,t.toVector()),!this._finished&&(this._finished=!0)&&this._enqueue(e,null)))}_enqueue(t,e){this._bufferedSize=0,this._controller=null,null===e?t.close():t.enqueue(e)}}const gh=t=>t.length,mh=t=>t.byteLength;function vh(t,e){const n=new oo;let r=null;const i=new ReadableStream({async cancel(){await n.close()},async start(t){await o(t,r||(r=await s()))},async pull(t){r?await o(t,r):t.close()}});return{writable:new WritableStream(n,{highWaterMark:16384,...t}),readable:i};async function s(){return await(await Gl.from(n)).open(e)}async function o(t,e){let n=t.desiredSize,r=null;while(!(r=await e.next()).done)if(t.enqueue(r.value),null!=n&&--n<=0)return;t.close()}}function _h(t,e){const n=new this(t),r=new co(n),i=new ReadableStream({type:"bytes",async cancel(){await r.cancel()},async pull(t){await s(t)},async start(t){await s(t)}},{highWaterMark:16384,...e});return{writable:new WritableStream(n,t),readable:i};async function s(t){let e=null,n=t.desiredSize;while(e=await r.read(n||null))if(t.enqueue(e),null!=n&&(n-=e.byteLength)<=0)return;t.close()}}class wh{eq(t){return t instanceof wh||(t=new Ih(t)),new jh(this,t)}le(t){return t instanceof wh||(t=new Ih(t)),new Dh(this,t)}ge(t){return t instanceof wh||(t=new Ih(t)),new Fh(this,t)}lt(t){return new Lh(this.ge(t))}gt(t){return new Lh(this.le(t))}ne(t){return new Lh(this.eq(t))}}class Ih extends wh{constructor(t){super(),this.v=t}}class Sh extends wh{constructor(t){super(),this.name=t}bind(t){if(!this.colidx){this.colidx=-1;const e=t.schema.fields;for(let t=-1;++te.get(t)}}class Oh{and(...t){return new xh(this,...t)}or(...t){return new Bh(this,...t)}not(){return new Lh(this)}}class Th extends Oh{constructor(t,e){super(),this.left=t,this.right=e}bind(t){return this.left instanceof Ih?this.right instanceof Ih?this._bindLitLit(t,this.left,this.right):this._bindLitCol(t,this.left,this.right):this.right instanceof Ih?this._bindColLit(t,this.left,this.right):this._bindColCol(t,this.left,this.right)}}class Ah extends Oh{constructor(...t){super(),this.children=t}}Ah.prototype.children=Object.freeze([]);class xh extends Ah{constructor(...t){t=t.reduce((t,e)=>t.concat(e instanceof xh?e.children:e),[]),super(...t)}bind(t){const e=this.children.map(e=>e.bind(t));return(t,n)=>e.every(e=>e(t,n))}}class Bh extends Ah{constructor(...t){t=t.reduce((t,e)=>t.concat(e instanceof Bh?e.children:e),[]),super(...t)}bind(t){const e=this.children.map(e=>e.bind(t));return(t,n)=>e.some(e=>e(t,n))}}class jh extends Th{_bindLitLit(t,e,n){const r=e.v==n.v;return()=>r}_bindColCol(t,e,n){const r=e.bind(t),i=n.bind(t);return(t,e)=>r(t,e)==i(t,e)}_bindColLit(t,e,n){const r=e.bind(t);if(e.vector instanceof Lc){let t;const r=e.vector;return r.dictionary!==this.lastDictionary?(t=r.reverseLookup(n.v),this.lastDictionary=r.dictionary,this.lastKey=t):t=this.lastKey,-1===t?()=>!1:e=>r.getKey(e)===t}return(t,e)=>r(t,e)==n.v}_bindLitCol(t,e,n){return this._bindColLit(t,n,e)}}class Dh extends Th{_bindLitLit(t,e,n){const r=e.v<=n.v;return()=>r}_bindColCol(t,e,n){const r=e.bind(t),i=n.bind(t);return(t,e)=>r(t,e)<=i(t,e)}_bindColLit(t,e,n){const r=e.bind(t);return(t,e)=>r(t,e)<=n.v}_bindLitCol(t,e,n){const r=n.bind(t);return(t,n)=>e.v<=r(t,n)}}class Fh extends Th{_bindLitLit(t,e,n){const r=e.v>=n.v;return()=>r}_bindColCol(t,e,n){const r=e.bind(t),i=n.bind(t);return(t,e)=>r(t,e)>=i(t,e)}_bindColLit(t,e,n){const r=e.bind(t);return(t,e)=>r(t,e)>=n.v}_bindLitCol(t,e,n){const r=n.bind(t);return(t,n)=>e.v>=r(t,n)}}class Lh extends Oh{constructor(t){super(),this.child=t}bind(t){const e=this.child.bind(t);return(t,n)=>!e(t,n)}}zl.prototype.countBy=function(t){return new Eh(this.chunks).countBy(t)},zl.prototype.scan=function(t,e){return new Eh(this.chunks).scan(t,e)},zl.prototype.scanReverse=function(t,e){return new Eh(this.chunks).scanReverse(t,e)},zl.prototype.filter=function(t){return new Eh(this.chunks).filter(t)};class Eh extends zl{filter(t){return new Uh(this.chunks,t)}scan(t,e){const n=this.chunks,r=n.length;for(let i=-1;++i=0;){const r=n[i];e&&e(r);for(let e=r.length;--e>=0;)t(e,r)}}countBy(t){const e=this.chunks,n=e.length,r="string"===typeof t?new Sh(t):t;r.bind(e[n-1]);const i=r.vector;if(!un.isDictionary(i.type))throw new Error("countBy currently only supports dictionary-encoded columns");const s=Math.ceil(Math.log(i.length)/Math.log(256)),o=4==s?Uint32Array:s>=2?Uint16Array:Uint8Array,a=new o(i.dictionary.length);for(let c=-1;++c=0;){const r=n[i],s=this._predicate.bind(r);let o=!1;for(let n=r.length;--n>=0;)s(n,r)&&(e&&!o&&(e(r),o=!0),t(n,r))}}count(){let t=0;const e=this._chunks,n=e.length;for(let r=-1;++r=2?Uint16Array:Uint8Array,a=new o(i.dictionary.length);for(let c=-1;++c=i.headerRows&&e=i.headerColumns;if(n){var o=["blank"];return e>0&&o.push("level"+t),{type:"blank",classNames:o.join(" "),content:""}}if(s){var a=e-i.headerColumns;o=["col_heading","level"+t,"col"+a];return{type:"columns",classNames:o.join(" "),content:i.getContent(i.columnsTable,a,t)}}if(r){var c=t-i.headerRows;o=["row_heading","level"+e,"row"+c];return{type:"index",id:"T_"+i.uuid+"level"+e+"_row"+c,classNames:o.join(" "),content:i.getContent(i.indexTable,c,e)}}c=t-i.headerRows,a=e-i.headerColumns,o=["data","row"+c,"col"+a];var u=i.styler?i.getContent(i.styler.displayValuesTable,c,a):i.getContent(i.dataTable,c,a);return{type:"data",id:"T_"+i.uuid+"row"+c+"_col"+a,classNames:o.join(" "),content:u}},this.getContent=function(t,e,n){var r=t.getColumnAt(n);if(null===r)return"";var s=i.getColumnTypeId(t,n);switch(s){case Oe.Timestamp:return i.nanosToDate(r.get(e));default:return r.get(e)}},this.dataTable=zl.from(t),this.indexTable=zl.from(e),this.columnsTable=zl.from(n),this.styler=r?{caption:r.caption,displayValuesTable:zl.from(r.displayValues),styles:r.styles,uuid:r.uuid}:void 0}return Object.defineProperty(t.prototype,"rows",{get:function(){return this.indexTable.length+this.columnsTable.numCols},enumerable:!0,configurable:!0}),Object.defineProperty(t.prototype,"columns",{get:function(){return this.indexTable.numCols+this.columnsTable.length},enumerable:!0,configurable:!0}),Object.defineProperty(t.prototype,"headerRows",{get:function(){return this.rows-this.dataRows},enumerable:!0,configurable:!0}),Object.defineProperty(t.prototype,"headerColumns",{get:function(){return this.columns-this.dataColumns},enumerable:!0,configurable:!0}),Object.defineProperty(t.prototype,"dataRows",{get:function(){return this.dataTable.length},enumerable:!0,configurable:!0}),Object.defineProperty(t.prototype,"dataColumns",{get:function(){return this.dataTable.numCols},enumerable:!0,configurable:!0}),Object.defineProperty(t.prototype,"uuid",{get:function(){return this.styler&&this.styler.uuid},enumerable:!0,configurable:!0}),Object.defineProperty(t.prototype,"caption",{get:function(){return this.styler&&this.styler.caption},enumerable:!0,configurable:!0}),Object.defineProperty(t.prototype,"styles",{get:function(){return this.styler&&this.styler.styles},enumerable:!0,configurable:!0}),Object.defineProperty(t.prototype,"table",{get:function(){return this.dataTable},enumerable:!0,configurable:!0}),Object.defineProperty(t.prototype,"index",{get:function(){return this.indexTable},enumerable:!0,configurable:!0}),Object.defineProperty(t.prototype,"columnTable",{get:function(){return this.columnsTable},enumerable:!0,configurable:!0}),t.prototype.serialize=function(){return{data:this.dataTable.serialize(),index:this.indexTable.serialize(),columns:this.columnsTable.serialize()}},t.prototype.getColumnTypeId=function(t,e){return t.schema.fields[e].type.typeId},t.prototype.nanosToDate=function(t){return new Date(t/1e6)},t}(),kh=function(){return kh=Object.assign||function(t){for(var e,n=1,r=arguments.length;n0?t.argsDataframeToObject(e["dfs"]):{};n=kh(kh({},n),r);var i=Boolean(e["disabled"]),s=e["theme"];s&&Rh(s);var o={disabled:i,args:n,theme:s},a=new CustomEvent(t.RENDER_EVENT,{detail:o});t.events.dispatchEvent(a)},t.argsDataframeToObject=function(e){var n=e.map((function(e){var n=e.key,r=e.value;return[n,t.toArrowTable(r)]}));return Object.fromEntries(n)},t.toArrowTable=function(t){var e=t.data,n=e.data,r=e.index,i=e.columns,s=e.styler;return new Nh(n,r,i,s)},t.sendBackMsg=function(t,e){window.parent.postMessage(kh({isStreamlitMessage:!0,type:t},e),"*")},t}(),Rh=function(t){var e=document.createElement("style");document.head.appendChild(e),e.innerHTML="\n :root {\n --primary-color: "+t.primaryColor+";\n --background-color: "+t.backgroundColor+";\n --secondary-background-color: "+t.secondaryBackgroundColor+";\n --text-color: "+t.textColor+";\n --font: "+t.font+";\n }\n\n body {\n background-color: var(--background-color);\n color: var(--text-color);\n }\n "};function Ph(t){var e=!1;try{e=t instanceof BigInt64Array||t instanceof BigUint64Array}catch(n){}return t instanceof Int8Array||t instanceof Uint8Array||t instanceof Uint8ClampedArray||t instanceof Int16Array||t instanceof Uint16Array||t instanceof Int32Array||t instanceof Uint32Array||t instanceof Float32Array||t instanceof Float64Array||e}
-/**
- * @license
- * Copyright 2018-2021 Streamlit Inc.
- *
- * Licensed under the Apache License, Version 2.0 (the "License");
- * you may not use this file except in compliance with the License.
- * You may obtain a copy of the License at
- *
- * http://www.apache.org/licenses/LICENSE-2.0
- *
- * Unless required by applicable law or agreed to in writing, software
- * distributed under the License is distributed on an "AS IS" BASIS,
- * WITHOUT WARRANTIES OR CONDITIONS OF ANY KIND, either express or implied.
- * See the License for the specific language governing permissions and
- * limitations under the License.
- */var zh=function(){var t=function(e,n){return t=Object.setPrototypeOf||{__proto__:[]}instanceof Array&&function(t,e){t.__proto__=e}||function(t,e){for(var n in e)e.hasOwnProperty(n)&&(t[n]=e[n])},t(e,n)};return function(e,n){function r(){this.constructor=e}t(e,n),e.prototype=null===n?Object.create(n):(r.prototype=n.prototype,new r)}}();(function(t){function e(){return null!==t&&t.apply(this,arguments)||this}zh(e,t),e.prototype.componentDidMount=function(){Vh.setFrameHeight()},e.prototype.componentDidUpdate=function(){Vh.setFrameHeight()}})(l.a.PureComponent)},d1e7:function(t,e,n){"use strict";var r={}.propertyIsEnumerable,i=Object.getOwnPropertyDescriptor,s=i&&!r.call({1:2},1);e.f=s?function(t){var e=i(this,t);return!!e&&e.enumerable}:r},d2bb:function(t,e,n){var r=n("825a"),i=n("3bbe");t.exports=Object.setPrototypeOf||("__proto__"in{}?function(){var t,e=!1,n={};try{t=Object.getOwnPropertyDescriptor(Object.prototype,"__proto__").set,t.call(n,[]),e=n instanceof Array}catch(s){}return function(n,s){return r(n),i(s),e?t.call(n,s):n.__proto__=s,n}}():void 0)},d44e:function(t,e,n){var r=n("9bf2").f,i=n("5135"),s=n("b622"),o=s("toStringTag");t.exports=function(t,e,n){t&&!i(t=n?t:t.prototype,o)&&r(t,o,{configurable:!0,value:e})}},da6a:function(t,e,n){"use strict";var r=n("4cec"),i={childContextTypes:!0,contextType:!0,contextTypes:!0,defaultProps:!0,displayName:!0,getDefaultProps:!0,getDerivedStateFromError:!0,getDerivedStateFromProps:!0,mixins:!0,propTypes:!0,type:!0},s={name:!0,length:!0,prototype:!0,caller:!0,callee:!0,arguments:!0,arity:!0},o={$$typeof:!0,render:!0,defaultProps:!0,displayName:!0,propTypes:!0},a={$$typeof:!0,compare:!0,defaultProps:!0,displayName:!0,propTypes:!0,type:!0},c={};function u(t){return r.isMemo(t)?a:c[t["$$typeof"]]||i}c[r.ForwardRef]=o,c[r.Memo]=a;var l=Object.defineProperty,h=Object.getOwnPropertyNames,f=Object.getOwnPropertySymbols,d=Object.getOwnPropertyDescriptor,p=Object.getPrototypeOf,y=Object.prototype;function b(t,e,n){if("string"!==typeof e){if(y){var r=p(e);r&&r!==y&&b(t,r,n)}var i=h(e);f&&(i=i.concat(f(e)));for(var o=u(t),a=u(e),c=0;c=e.length?(t.target=void 0,{value:void 0,done:!0}):"keys"==n?{value:r,done:!1}:"values"==n?{value:e[r],done:!1}:{value:[r,e[r]],done:!1}}),"values"),s.Arguments=s.Array,i("keys"),i("values"),i("entries")},e2cc:function(t,e,n){var r=n("6eeb");t.exports=function(t,e,n){for(var i in e)r(t,i,e[i],n);return t}},e667:function(t,e){t.exports=function(t){try{return{error:!1,value:t()}}catch(e){return{error:!0,value:e}}}},e6cf:function(t,e,n){"use strict";var r,i,s,o,a=n("23e7"),c=n("c430"),u=n("da84"),l=n("d066"),h=n("fea9"),f=n("6eeb"),d=n("e2cc"),p=n("d44e"),y=n("2626"),b=n("861d"),g=n("1c0b"),m=n("19aa"),v=n("c6b6"),_=n("8925"),w=n("2266"),I=n("1c7e"),S=n("4840"),O=n("2cf4").set,T=n("b575"),A=n("cdf9"),x=n("44de"),B=n("f069"),j=n("e667"),D=n("69f3"),F=n("94ca"),L=n("b622"),E=n("2d00"),M=L("species"),U="Promise",C=D.get,N=D.set,k=D.getterFor(U),V=h,R=u.TypeError,P=u.document,z=u.process,$=l("fetch"),Y=B.f,W=Y,H="process"==v(z),K=!!(P&&P.createEvent&&u.dispatchEvent),G="unhandledrejection",q="rejectionhandled",J=0,Z=1,X=2,Q=1,tt=2,et=F(U,(function(){var t=_(V)!==String(V);if(!t){if(66===E)return!0;if(!H&&"function"!=typeof PromiseRejectionEvent)return!0}if(c&&!V.prototype["finally"])return!0;if(E>=51&&/native code/.test(V))return!1;var e=V.resolve(1),n=function(t){t((function(){}),(function(){}))},r=e.constructor={};return r[M]=n,!(e.then((function(){}))instanceof n)})),nt=et||!I((function(t){V.all(t)["catch"]((function(){}))})),rt=function(t){var e;return!(!b(t)||"function"!=typeof(e=t.then))&&e},it=function(t,e,n){if(!e.notified){e.notified=!0;var r=e.reactions;T((function(){var i=e.value,s=e.state==Z,o=0;while(r.length>o){var a,c,u,l=r[o++],h=s?l.ok:l.fail,f=l.resolve,d=l.reject,p=l.domain;try{h?(s||(e.rejection===tt&&ct(t,e),e.rejection=Q),!0===h?a=i:(p&&p.enter(),a=h(i),p&&(p.exit(),u=!0)),a===l.promise?d(R("Promise-chain cycle")):(c=rt(a))?c.call(a,f,d):f(a)):d(i)}catch(y){p&&!u&&p.exit(),d(y)}}e.reactions=[],e.notified=!1,n&&!e.rejection&&ot(t,e)}))}},st=function(t,e,n){var r,i;K?(r=P.createEvent("Event"),r.promise=e,r.reason=n,r.initEvent(t,!1,!0),u.dispatchEvent(r)):r={promise:e,reason:n},(i=u["on"+t])?i(r):t===G&&x("Unhandled promise rejection",n)},ot=function(t,e){O.call(u,(function(){var n,r=e.value,i=at(e);if(i&&(n=j((function(){H?z.emit("unhandledRejection",r,t):st(G,t,r)})),e.rejection=H||at(e)?tt:Q,n.error))throw n.value}))},at=function(t){return t.rejection!==Q&&!t.parent},ct=function(t,e){O.call(u,(function(){H?z.emit("rejectionHandled",t):st(q,t,e.value)}))},ut=function(t,e,n,r){return function(i){t(e,n,i,r)}},lt=function(t,e,n,r){e.done||(e.done=!0,r&&(e=r),e.value=n,e.state=X,it(t,e,!0))},ht=function(t,e,n,r){if(!e.done){e.done=!0,r&&(e=r);try{if(t===n)throw R("Promise can't be resolved itself");var i=rt(n);i?T((function(){var r={done:!1};try{i.call(n,ut(ht,t,r,e),ut(lt,t,r,e))}catch(s){lt(t,r,s,e)}})):(e.value=n,e.state=Z,it(t,e,!1))}catch(s){lt(t,{done:!1},s,e)}}};et&&(V=function(t){m(this,V,U),g(t),r.call(this);var e=C(this);try{t(ut(ht,this,e),ut(lt,this,e))}catch(n){lt(this,e,n)}},r=function(t){N(this,{type:U,done:!1,notified:!1,parent:!1,reactions:[],rejection:!1,state:J,value:void 0})},r.prototype=d(V.prototype,{then:function(t,e){var n=k(this),r=Y(S(this,V));return r.ok="function"!=typeof t||t,r.fail="function"==typeof e&&e,r.domain=H?z.domain:void 0,n.parent=!0,n.reactions.push(r),n.state!=J&&it(this,n,!1),r.promise},catch:function(t){return this.then(void 0,t)}}),i=function(){var t=new r,e=C(t);this.promise=t,this.resolve=ut(ht,t,e),this.reject=ut(lt,t,e)},B.f=Y=function(t){return t===V||t===s?new i(t):W(t)},c||"function"!=typeof h||(o=h.prototype.then,f(h.prototype,"then",(function(t,e){var n=this;return new V((function(t,e){o.call(n,t,e)})).then(t,e)}),{unsafe:!0}),"function"==typeof $&&a({global:!0,enumerable:!0,forced:!0},{fetch:function(t){return A(V,$.apply(u,arguments))}}))),a({global:!0,wrap:!0,forced:et},{Promise:V}),p(V,U,!1,!0),y(U),s=l(U),a({target:U,stat:!0,forced:et},{reject:function(t){var e=Y(this);return e.reject.call(void 0,t),e.promise}}),a({target:U,stat:!0,forced:c||et},{resolve:function(t){return A(c&&this===s?V:this,t)}}),a({target:U,stat:!0,forced:nt},{all:function(t){var e=this,n=Y(e),r=n.resolve,i=n.reject,s=j((function(){var n=g(e.resolve),s=[],o=0,a=1;w(t,(function(t){var c=o++,u=!1;s.push(void 0),a++,n.call(e,t).then((function(t){u||(u=!0,s[c]=t,--a||r(s))}),i)})),--a||r(s)}));return s.error&&i(s.value),n.promise},race:function(t){var e=this,n=Y(e),r=n.reject,i=j((function(){var i=g(e.resolve);w(t,(function(t){i.call(e,t).then(n.resolve,r)}))}));return i.error&&r(i.value),n.promise}})},e893:function(t,e,n){var r=n("5135"),i=n("56ef"),s=n("06cf"),o=n("9bf2");t.exports=function(t,e){for(var n=i(e),a=o.f,c=s.f,u=0;u List[str]:
- try:
- return _perform_download(url, maxDuration=maxDuration, outputTemplate=None, destinationDirectory=destinationDirectory, playlistItems=playlistItems)
- except yt_dlp.utils.DownloadError as e:
- # In case of an OS error, try again with a different output template
- if e.msg and e.msg.find("[Errno 36] File name too long") >= 0:
- return _perform_download(url, maxDuration=maxDuration, outputTemplate="%(title).10s %(id)s.%(ext)s")
- pass
-
-def _perform_download(url: str, maxDuration: int = None, outputTemplate: str = None, destinationDirectory: str = None, playlistItems: str = "1"):
- # Create a temporary directory to store the downloaded files
- if destinationDirectory is None:
- destinationDirectory = mkdtemp()
-
- ydl_opts = {
- "format": "bestaudio/best",
- 'paths': {
- 'home': destinationDirectory
- }
- }
- if (playlistItems):
- ydl_opts['playlist_items'] = playlistItems
-
- # Add output template if specified
- if outputTemplate:
- ydl_opts['outtmpl'] = outputTemplate
-
- filename_collector = FilenameCollectorPP()
-
- with YoutubeDL(ydl_opts) as ydl:
- if maxDuration and maxDuration > 0:
- info = ydl.extract_info(url, download=False)
- entries = "entries" in info and info["entries"] or [info]
-
- total_duration = 0
-
- # Compute total duration
- for entry in entries:
- total_duration += float(entry["duration"])
-
- if total_duration >= maxDuration:
- raise ExceededMaximumDuration(videoDuration=total_duration, maxDuration=maxDuration, message="Video is too long")
-
- ydl.add_post_processor(filename_collector)
- ydl.download([url])
-
- if len(filename_collector.filenames) <= 0:
- raise Exception("Cannot download " + url)
-
- result = []
-
- for filename in filename_collector.filenames:
- result.append(filename)
- print("Downloaded " + filename)
-
- return result
-
-class ExceededMaximumDuration(Exception):
- def __init__(self, videoDuration, maxDuration, message):
- self.videoDuration = videoDuration
- self.maxDuration = maxDuration
- super().__init__(message)
\ No newline at end of file
diff --git a/spaces/abhishek/sketch-to-image/annotator/uniformer/mmcv/runner/hooks/logger/mlflow.py b/spaces/abhishek/sketch-to-image/annotator/uniformer/mmcv/runner/hooks/logger/mlflow.py
deleted file mode 100644
index f9a72592be47b534ce22573775fd5a7e8e86d72d..0000000000000000000000000000000000000000
--- a/spaces/abhishek/sketch-to-image/annotator/uniformer/mmcv/runner/hooks/logger/mlflow.py
+++ /dev/null
@@ -1,78 +0,0 @@
-# Copyright (c) OpenMMLab. All rights reserved.
-from ...dist_utils import master_only
-from ..hook import HOOKS
-from .base import LoggerHook
-
-
-@HOOKS.register_module()
-class MlflowLoggerHook(LoggerHook):
-
- def __init__(self,
- exp_name=None,
- tags=None,
- log_model=True,
- interval=10,
- ignore_last=True,
- reset_flag=False,
- by_epoch=True):
- """Class to log metrics and (optionally) a trained model to MLflow.
-
- It requires `MLflow`_ to be installed.
-
- Args:
- exp_name (str, optional): Name of the experiment to be used.
- Default None.
- If not None, set the active experiment.
- If experiment does not exist, an experiment with provided name
- will be created.
- tags (dict of str: str, optional): Tags for the current run.
- Default None.
- If not None, set tags for the current run.
- log_model (bool, optional): Whether to log an MLflow artifact.
- Default True.
- If True, log runner.model as an MLflow artifact
- for the current run.
- interval (int): Logging interval (every k iterations).
- ignore_last (bool): Ignore the log of last iterations in each epoch
- if less than `interval`.
- reset_flag (bool): Whether to clear the output buffer after logging
- by_epoch (bool): Whether EpochBasedRunner is used.
-
- .. _MLflow:
- https://www.mlflow.org/docs/latest/index.html
- """
- super(MlflowLoggerHook, self).__init__(interval, ignore_last,
- reset_flag, by_epoch)
- self.import_mlflow()
- self.exp_name = exp_name
- self.tags = tags
- self.log_model = log_model
-
- def import_mlflow(self):
- try:
- import mlflow
- import mlflow.pytorch as mlflow_pytorch
- except ImportError:
- raise ImportError(
- 'Please run "pip install mlflow" to install mlflow')
- self.mlflow = mlflow
- self.mlflow_pytorch = mlflow_pytorch
-
- @master_only
- def before_run(self, runner):
- super(MlflowLoggerHook, self).before_run(runner)
- if self.exp_name is not None:
- self.mlflow.set_experiment(self.exp_name)
- if self.tags is not None:
- self.mlflow.set_tags(self.tags)
-
- @master_only
- def log(self, runner):
- tags = self.get_loggable_tags(runner)
- if tags:
- self.mlflow.log_metrics(tags, step=self.get_iter(runner))
-
- @master_only
- def after_run(self, runner):
- if self.log_model:
- self.mlflow_pytorch.log_model(runner.model, 'models')
diff --git a/spaces/abhishek/sketch-to-image/annotator/uniformer/mmseg/models/backbones/hrnet.py b/spaces/abhishek/sketch-to-image/annotator/uniformer/mmseg/models/backbones/hrnet.py
deleted file mode 100644
index 331ebf3ccb8597b3f507670753789073fc3c946d..0000000000000000000000000000000000000000
--- a/spaces/abhishek/sketch-to-image/annotator/uniformer/mmseg/models/backbones/hrnet.py
+++ /dev/null
@@ -1,555 +0,0 @@
-import torch.nn as nn
-from annotator.uniformer.mmcv.cnn import (build_conv_layer, build_norm_layer, constant_init,
- kaiming_init)
-from annotator.uniformer.mmcv.runner import load_checkpoint
-from annotator.uniformer.mmcv.utils.parrots_wrapper import _BatchNorm
-
-from annotator.uniformer.mmseg.ops import Upsample, resize
-from annotator.uniformer.mmseg.utils import get_root_logger
-from ..builder import BACKBONES
-from .resnet import BasicBlock, Bottleneck
-
-
-class HRModule(nn.Module):
- """High-Resolution Module for HRNet.
-
- In this module, every branch has 4 BasicBlocks/Bottlenecks. Fusion/Exchange
- is in this module.
- """
-
- def __init__(self,
- num_branches,
- blocks,
- num_blocks,
- in_channels,
- num_channels,
- multiscale_output=True,
- with_cp=False,
- conv_cfg=None,
- norm_cfg=dict(type='BN', requires_grad=True)):
- super(HRModule, self).__init__()
- self._check_branches(num_branches, num_blocks, in_channels,
- num_channels)
-
- self.in_channels = in_channels
- self.num_branches = num_branches
-
- self.multiscale_output = multiscale_output
- self.norm_cfg = norm_cfg
- self.conv_cfg = conv_cfg
- self.with_cp = with_cp
- self.branches = self._make_branches(num_branches, blocks, num_blocks,
- num_channels)
- self.fuse_layers = self._make_fuse_layers()
- self.relu = nn.ReLU(inplace=False)
-
- def _check_branches(self, num_branches, num_blocks, in_channels,
- num_channels):
- """Check branches configuration."""
- if num_branches != len(num_blocks):
- error_msg = f'NUM_BRANCHES({num_branches}) <> NUM_BLOCKS(' \
- f'{len(num_blocks)})'
- raise ValueError(error_msg)
-
- if num_branches != len(num_channels):
- error_msg = f'NUM_BRANCHES({num_branches}) <> NUM_CHANNELS(' \
- f'{len(num_channels)})'
- raise ValueError(error_msg)
-
- if num_branches != len(in_channels):
- error_msg = f'NUM_BRANCHES({num_branches}) <> NUM_INCHANNELS(' \
- f'{len(in_channels)})'
- raise ValueError(error_msg)
-
- def _make_one_branch(self,
- branch_index,
- block,
- num_blocks,
- num_channels,
- stride=1):
- """Build one branch."""
- downsample = None
- if stride != 1 or \
- self.in_channels[branch_index] != \
- num_channels[branch_index] * block.expansion:
- downsample = nn.Sequential(
- build_conv_layer(
- self.conv_cfg,
- self.in_channels[branch_index],
- num_channels[branch_index] * block.expansion,
- kernel_size=1,
- stride=stride,
- bias=False),
- build_norm_layer(self.norm_cfg, num_channels[branch_index] *
- block.expansion)[1])
-
- layers = []
- layers.append(
- block(
- self.in_channels[branch_index],
- num_channels[branch_index],
- stride,
- downsample=downsample,
- with_cp=self.with_cp,
- norm_cfg=self.norm_cfg,
- conv_cfg=self.conv_cfg))
- self.in_channels[branch_index] = \
- num_channels[branch_index] * block.expansion
- for i in range(1, num_blocks[branch_index]):
- layers.append(
- block(
- self.in_channels[branch_index],
- num_channels[branch_index],
- with_cp=self.with_cp,
- norm_cfg=self.norm_cfg,
- conv_cfg=self.conv_cfg))
-
- return nn.Sequential(*layers)
-
- def _make_branches(self, num_branches, block, num_blocks, num_channels):
- """Build multiple branch."""
- branches = []
-
- for i in range(num_branches):
- branches.append(
- self._make_one_branch(i, block, num_blocks, num_channels))
-
- return nn.ModuleList(branches)
-
- def _make_fuse_layers(self):
- """Build fuse layer."""
- if self.num_branches == 1:
- return None
-
- num_branches = self.num_branches
- in_channels = self.in_channels
- fuse_layers = []
- num_out_branches = num_branches if self.multiscale_output else 1
- for i in range(num_out_branches):
- fuse_layer = []
- for j in range(num_branches):
- if j > i:
- fuse_layer.append(
- nn.Sequential(
- build_conv_layer(
- self.conv_cfg,
- in_channels[j],
- in_channels[i],
- kernel_size=1,
- stride=1,
- padding=0,
- bias=False),
- build_norm_layer(self.norm_cfg, in_channels[i])[1],
- # we set align_corners=False for HRNet
- Upsample(
- scale_factor=2**(j - i),
- mode='bilinear',
- align_corners=False)))
- elif j == i:
- fuse_layer.append(None)
- else:
- conv_downsamples = []
- for k in range(i - j):
- if k == i - j - 1:
- conv_downsamples.append(
- nn.Sequential(
- build_conv_layer(
- self.conv_cfg,
- in_channels[j],
- in_channels[i],
- kernel_size=3,
- stride=2,
- padding=1,
- bias=False),
- build_norm_layer(self.norm_cfg,
- in_channels[i])[1]))
- else:
- conv_downsamples.append(
- nn.Sequential(
- build_conv_layer(
- self.conv_cfg,
- in_channels[j],
- in_channels[j],
- kernel_size=3,
- stride=2,
- padding=1,
- bias=False),
- build_norm_layer(self.norm_cfg,
- in_channels[j])[1],
- nn.ReLU(inplace=False)))
- fuse_layer.append(nn.Sequential(*conv_downsamples))
- fuse_layers.append(nn.ModuleList(fuse_layer))
-
- return nn.ModuleList(fuse_layers)
-
- def forward(self, x):
- """Forward function."""
- if self.num_branches == 1:
- return [self.branches[0](x[0])]
-
- for i in range(self.num_branches):
- x[i] = self.branches[i](x[i])
-
- x_fuse = []
- for i in range(len(self.fuse_layers)):
- y = 0
- for j in range(self.num_branches):
- if i == j:
- y += x[j]
- elif j > i:
- y = y + resize(
- self.fuse_layers[i][j](x[j]),
- size=x[i].shape[2:],
- mode='bilinear',
- align_corners=False)
- else:
- y += self.fuse_layers[i][j](x[j])
- x_fuse.append(self.relu(y))
- return x_fuse
-
-
-@BACKBONES.register_module()
-class HRNet(nn.Module):
- """HRNet backbone.
-
- High-Resolution Representations for Labeling Pixels and Regions
- arXiv: https://arxiv.org/abs/1904.04514
-
- Args:
- extra (dict): detailed configuration for each stage of HRNet.
- in_channels (int): Number of input image channels. Normally 3.
- conv_cfg (dict): dictionary to construct and config conv layer.
- norm_cfg (dict): dictionary to construct and config norm layer.
- norm_eval (bool): Whether to set norm layers to eval mode, namely,
- freeze running stats (mean and var). Note: Effect on Batch Norm
- and its variants only.
- with_cp (bool): Use checkpoint or not. Using checkpoint will save some
- memory while slowing down the training speed.
- zero_init_residual (bool): whether to use zero init for last norm layer
- in resblocks to let them behave as identity.
-
- Example:
- >>> from annotator.uniformer.mmseg.models import HRNet
- >>> import torch
- >>> extra = dict(
- >>> stage1=dict(
- >>> num_modules=1,
- >>> num_branches=1,
- >>> block='BOTTLENECK',
- >>> num_blocks=(4, ),
- >>> num_channels=(64, )),
- >>> stage2=dict(
- >>> num_modules=1,
- >>> num_branches=2,
- >>> block='BASIC',
- >>> num_blocks=(4, 4),
- >>> num_channels=(32, 64)),
- >>> stage3=dict(
- >>> num_modules=4,
- >>> num_branches=3,
- >>> block='BASIC',
- >>> num_blocks=(4, 4, 4),
- >>> num_channels=(32, 64, 128)),
- >>> stage4=dict(
- >>> num_modules=3,
- >>> num_branches=4,
- >>> block='BASIC',
- >>> num_blocks=(4, 4, 4, 4),
- >>> num_channels=(32, 64, 128, 256)))
- >>> self = HRNet(extra, in_channels=1)
- >>> self.eval()
- >>> inputs = torch.rand(1, 1, 32, 32)
- >>> level_outputs = self.forward(inputs)
- >>> for level_out in level_outputs:
- ... print(tuple(level_out.shape))
- (1, 32, 8, 8)
- (1, 64, 4, 4)
- (1, 128, 2, 2)
- (1, 256, 1, 1)
- """
-
- blocks_dict = {'BASIC': BasicBlock, 'BOTTLENECK': Bottleneck}
-
- def __init__(self,
- extra,
- in_channels=3,
- conv_cfg=None,
- norm_cfg=dict(type='BN', requires_grad=True),
- norm_eval=False,
- with_cp=False,
- zero_init_residual=False):
- super(HRNet, self).__init__()
- self.extra = extra
- self.conv_cfg = conv_cfg
- self.norm_cfg = norm_cfg
- self.norm_eval = norm_eval
- self.with_cp = with_cp
- self.zero_init_residual = zero_init_residual
-
- # stem net
- self.norm1_name, norm1 = build_norm_layer(self.norm_cfg, 64, postfix=1)
- self.norm2_name, norm2 = build_norm_layer(self.norm_cfg, 64, postfix=2)
-
- self.conv1 = build_conv_layer(
- self.conv_cfg,
- in_channels,
- 64,
- kernel_size=3,
- stride=2,
- padding=1,
- bias=False)
-
- self.add_module(self.norm1_name, norm1)
- self.conv2 = build_conv_layer(
- self.conv_cfg,
- 64,
- 64,
- kernel_size=3,
- stride=2,
- padding=1,
- bias=False)
-
- self.add_module(self.norm2_name, norm2)
- self.relu = nn.ReLU(inplace=True)
-
- # stage 1
- self.stage1_cfg = self.extra['stage1']
- num_channels = self.stage1_cfg['num_channels'][0]
- block_type = self.stage1_cfg['block']
- num_blocks = self.stage1_cfg['num_blocks'][0]
-
- block = self.blocks_dict[block_type]
- stage1_out_channels = num_channels * block.expansion
- self.layer1 = self._make_layer(block, 64, num_channels, num_blocks)
-
- # stage 2
- self.stage2_cfg = self.extra['stage2']
- num_channels = self.stage2_cfg['num_channels']
- block_type = self.stage2_cfg['block']
-
- block = self.blocks_dict[block_type]
- num_channels = [channel * block.expansion for channel in num_channels]
- self.transition1 = self._make_transition_layer([stage1_out_channels],
- num_channels)
- self.stage2, pre_stage_channels = self._make_stage(
- self.stage2_cfg, num_channels)
-
- # stage 3
- self.stage3_cfg = self.extra['stage3']
- num_channels = self.stage3_cfg['num_channels']
- block_type = self.stage3_cfg['block']
-
- block = self.blocks_dict[block_type]
- num_channels = [channel * block.expansion for channel in num_channels]
- self.transition2 = self._make_transition_layer(pre_stage_channels,
- num_channels)
- self.stage3, pre_stage_channels = self._make_stage(
- self.stage3_cfg, num_channels)
-
- # stage 4
- self.stage4_cfg = self.extra['stage4']
- num_channels = self.stage4_cfg['num_channels']
- block_type = self.stage4_cfg['block']
-
- block = self.blocks_dict[block_type]
- num_channels = [channel * block.expansion for channel in num_channels]
- self.transition3 = self._make_transition_layer(pre_stage_channels,
- num_channels)
- self.stage4, pre_stage_channels = self._make_stage(
- self.stage4_cfg, num_channels)
-
- @property
- def norm1(self):
- """nn.Module: the normalization layer named "norm1" """
- return getattr(self, self.norm1_name)
-
- @property
- def norm2(self):
- """nn.Module: the normalization layer named "norm2" """
- return getattr(self, self.norm2_name)
-
- def _make_transition_layer(self, num_channels_pre_layer,
- num_channels_cur_layer):
- """Make transition layer."""
- num_branches_cur = len(num_channels_cur_layer)
- num_branches_pre = len(num_channels_pre_layer)
-
- transition_layers = []
- for i in range(num_branches_cur):
- if i < num_branches_pre:
- if num_channels_cur_layer[i] != num_channels_pre_layer[i]:
- transition_layers.append(
- nn.Sequential(
- build_conv_layer(
- self.conv_cfg,
- num_channels_pre_layer[i],
- num_channels_cur_layer[i],
- kernel_size=3,
- stride=1,
- padding=1,
- bias=False),
- build_norm_layer(self.norm_cfg,
- num_channels_cur_layer[i])[1],
- nn.ReLU(inplace=True)))
- else:
- transition_layers.append(None)
- else:
- conv_downsamples = []
- for j in range(i + 1 - num_branches_pre):
- in_channels = num_channels_pre_layer[-1]
- out_channels = num_channels_cur_layer[i] \
- if j == i - num_branches_pre else in_channels
- conv_downsamples.append(
- nn.Sequential(
- build_conv_layer(
- self.conv_cfg,
- in_channels,
- out_channels,
- kernel_size=3,
- stride=2,
- padding=1,
- bias=False),
- build_norm_layer(self.norm_cfg, out_channels)[1],
- nn.ReLU(inplace=True)))
- transition_layers.append(nn.Sequential(*conv_downsamples))
-
- return nn.ModuleList(transition_layers)
-
- def _make_layer(self, block, inplanes, planes, blocks, stride=1):
- """Make each layer."""
- downsample = None
- if stride != 1 or inplanes != planes * block.expansion:
- downsample = nn.Sequential(
- build_conv_layer(
- self.conv_cfg,
- inplanes,
- planes * block.expansion,
- kernel_size=1,
- stride=stride,
- bias=False),
- build_norm_layer(self.norm_cfg, planes * block.expansion)[1])
-
- layers = []
- layers.append(
- block(
- inplanes,
- planes,
- stride,
- downsample=downsample,
- with_cp=self.with_cp,
- norm_cfg=self.norm_cfg,
- conv_cfg=self.conv_cfg))
- inplanes = planes * block.expansion
- for i in range(1, blocks):
- layers.append(
- block(
- inplanes,
- planes,
- with_cp=self.with_cp,
- norm_cfg=self.norm_cfg,
- conv_cfg=self.conv_cfg))
-
- return nn.Sequential(*layers)
-
- def _make_stage(self, layer_config, in_channels, multiscale_output=True):
- """Make each stage."""
- num_modules = layer_config['num_modules']
- num_branches = layer_config['num_branches']
- num_blocks = layer_config['num_blocks']
- num_channels = layer_config['num_channels']
- block = self.blocks_dict[layer_config['block']]
-
- hr_modules = []
- for i in range(num_modules):
- # multi_scale_output is only used for the last module
- if not multiscale_output and i == num_modules - 1:
- reset_multiscale_output = False
- else:
- reset_multiscale_output = True
-
- hr_modules.append(
- HRModule(
- num_branches,
- block,
- num_blocks,
- in_channels,
- num_channels,
- reset_multiscale_output,
- with_cp=self.with_cp,
- norm_cfg=self.norm_cfg,
- conv_cfg=self.conv_cfg))
-
- return nn.Sequential(*hr_modules), in_channels
-
- def init_weights(self, pretrained=None):
- """Initialize the weights in backbone.
-
- Args:
- pretrained (str, optional): Path to pre-trained weights.
- Defaults to None.
- """
- if isinstance(pretrained, str):
- logger = get_root_logger()
- load_checkpoint(self, pretrained, strict=False, logger=logger)
- elif pretrained is None:
- for m in self.modules():
- if isinstance(m, nn.Conv2d):
- kaiming_init(m)
- elif isinstance(m, (_BatchNorm, nn.GroupNorm)):
- constant_init(m, 1)
-
- if self.zero_init_residual:
- for m in self.modules():
- if isinstance(m, Bottleneck):
- constant_init(m.norm3, 0)
- elif isinstance(m, BasicBlock):
- constant_init(m.norm2, 0)
- else:
- raise TypeError('pretrained must be a str or None')
-
- def forward(self, x):
- """Forward function."""
-
- x = self.conv1(x)
- x = self.norm1(x)
- x = self.relu(x)
- x = self.conv2(x)
- x = self.norm2(x)
- x = self.relu(x)
- x = self.layer1(x)
-
- x_list = []
- for i in range(self.stage2_cfg['num_branches']):
- if self.transition1[i] is not None:
- x_list.append(self.transition1[i](x))
- else:
- x_list.append(x)
- y_list = self.stage2(x_list)
-
- x_list = []
- for i in range(self.stage3_cfg['num_branches']):
- if self.transition2[i] is not None:
- x_list.append(self.transition2[i](y_list[-1]))
- else:
- x_list.append(y_list[i])
- y_list = self.stage3(x_list)
-
- x_list = []
- for i in range(self.stage4_cfg['num_branches']):
- if self.transition3[i] is not None:
- x_list.append(self.transition3[i](y_list[-1]))
- else:
- x_list.append(y_list[i])
- y_list = self.stage4(x_list)
-
- return y_list
-
- def train(self, mode=True):
- """Convert the model into training mode will keeping the normalization
- layer freezed."""
- super(HRNet, self).train(mode)
- if mode and self.norm_eval:
- for m in self.modules():
- # trick: eval have effect on BatchNorm only
- if isinstance(m, _BatchNorm):
- m.eval()
diff --git a/spaces/adirik/stylemc-demo/encoder4editing/utils/model_utils.py b/spaces/adirik/stylemc-demo/encoder4editing/utils/model_utils.py
deleted file mode 100644
index e51e95578f72b3218d6d832e3b604193cb68c1d7..0000000000000000000000000000000000000000
--- a/spaces/adirik/stylemc-demo/encoder4editing/utils/model_utils.py
+++ /dev/null
@@ -1,35 +0,0 @@
-import torch
-import argparse
-from models.psp import pSp
-from models.encoders.psp_encoders import Encoder4Editing
-
-
-def setup_model(checkpoint_path, device='cuda'):
- ckpt = torch.load(checkpoint_path, map_location='cpu')
- opts = ckpt['opts']
-
- opts['checkpoint_path'] = checkpoint_path
- opts['device'] = device
- opts = argparse.Namespace(**opts)
-
- net = pSp(opts)
- net.eval()
- net = net.to(device)
- return net, opts
-
-
-def load_e4e_standalone(checkpoint_path, device='cuda'):
- ckpt = torch.load(checkpoint_path, map_location='cpu')
- opts = argparse.Namespace(**ckpt['opts'])
- e4e = Encoder4Editing(50, 'ir_se', opts)
- e4e_dict = {k.replace('encoder.', ''): v for k, v in ckpt['state_dict'].items() if k.startswith('encoder.')}
- e4e.load_state_dict(e4e_dict)
- e4e.eval()
- e4e = e4e.to(device)
- latent_avg = ckpt['latent_avg'].to(device)
-
- def add_latent_avg(model, inputs, outputs):
- return outputs + latent_avg.repeat(outputs.shape[0], 1, 1)
-
- e4e.register_forward_hook(add_latent_avg)
- return e4e
diff --git a/spaces/ai4bharat/IndicNLG/app.py b/spaces/ai4bharat/IndicNLG/app.py
deleted file mode 100644
index e780ed16ccd7200a512f027fa1b17e618b317bc3..0000000000000000000000000000000000000000
--- a/spaces/ai4bharat/IndicNLG/app.py
+++ /dev/null
@@ -1,100 +0,0 @@
-import gradio as gr
-from transformers import AutoModelForSeq2SeqLM
-from transformers import AlbertTokenizer
-
-
-tokenizer = AlbertTokenizer.from_pretrained(
- "ai4bharat/MultiIndicWikiBioSS", do_lower_case=False, use_fast=False, keep_accents=True)
-qgmodel = AutoModelForSeq2SeqLM.from_pretrained(
- "ai4bharat/MultiIndicQuestionGenerationSS").eval()
-hgmodel = AutoModelForSeq2SeqLM.from_pretrained(
- "ai4bharat/MultiIndicHeadlineGenerationSS").eval()
-ssmodel = AutoModelForSeq2SeqLM.from_pretrained(
- "ai4bharat/MultiIndicSentenceSummarizationSS").eval()
-ppmodel = AutoModelForSeq2SeqLM.from_pretrained(
- "ai4bharat/MultiIndicParaphraseGenerationSS").eval()
-wbmodel = AutoModelForSeq2SeqLM.from_pretrained(
- "ai4bharat/MultiIndicWikiBioSS").eval()
-
-bos_id = tokenizer._convert_token_to_id_with_added_voc("")
-eos_id = tokenizer._convert_token_to_id_with_added_voc("")
-pad_id = tokenizer._convert_token_to_id_with_added_voc("")
-
-INDIC = {"Assamese": "as", "Bengali": "bn", "Gujarati": "gu", "Hindi": "hi", "Kannada": "kn",
- "Malayalam": "ml", "Marathi": "mr", "Odia": "or", "Punjabi": "pa", "Tamil": "ta", "Telugu": "te"}
-
-
-def generate(input, task, lang):
- lang = INDIC[lang]
- if task == "IndicWikiBio":
- model = wbmodel
- elif task == "IndicHeadlineGeneration":
- model = hgmodel
- elif task == "IndicParaphrasing":
- model = ppmodel
- elif task == "IndicSentenceSummarization":
- model = ssmodel
- elif task == "IndicQuestionGeneration":
- model = qgmodel
-
- inp = tokenizer(input.strip() + " <2" + lang + ">",
- add_special_tokens=False, return_tensors="pt", padding=True).input_ids
- model_output = model.generate(inp, use_cache=True, num_beams=1, max_length=100, min_length=1, early_stopping=True, pad_token_id=pad_id,
- bos_token_id=bos_id, eos_token_id=eos_id, decoder_start_token_id=tokenizer._convert_token_to_id_with_added_voc("<2"+lang+">"))
- decoded_output = tokenizer.decode(
- model_output[0], skip_special_tokens=True, clean_up_tokenization_spaces=False)
-
- return decoded_output
-
-
-tasks = ["IndicWikiBio", "IndicHeadlineGeneration", "IndicParaphrasing",
- "IndicSentenceSummarization", "IndicQuestionGeneration"]
-languages = list(INDIC.keys())
-
-language_drop_down = gr.inputs.Dropdown(
- languages, type="value", default="Hindi", label="Select Target Language")
-task_drop_down = gr.inputs.Dropdown(
- tasks, type="value", default="IndicSentenceSummarization", label="Select Task")
-text = gr.inputs.Textbox(lines=5, placeholder="Enter Indic Text here...",
- default="", label="Enter Text in corresponding Indic Language")
-text_ouptut = gr.outputs.Textbox(
- type="auto", label="View Generated Text in the corresponding Indic Language")
-
-supported_lang = ', '.join(languages)
-
-examples = [
- [u" name राम नरेश पांडेय office विधायक - 205 - कुशीनगर विधान सभा निर्वाचन क्षेत्र , उत्तर प्रदेश term 1967 से 1968 nationality भारतीय", "IndicWikiBio", "Hindi"],
- [u"वैश्विक व्यापार युद्ध की शिकार हुई तुर्की की मुद्रा लीरा के डूबने से अमेरिकी डॉलर के मुकाबले रुपया अब तक के न्यूनतम स्तर पर पहुंच गया। रुपये में रिकॉर्ड गिरावट से सोने की चमक में निखार नहीं आ सकी। वैश्विक बाजार में सोना करीब आठ महीने के निचले स्तर पर पहुंच गया तो घरेलू बाजार में यह करीब नौ महीने के निचले स्तर पर चला गया। वैश्विक मंदी की आशंका से वैश्विक बाजार में चांदी करीब ढाई साल और घरेलू बाजार में तकरीबन नौ महीने के निचले स्तर पर पहुंच गई। तुर्की की आर्थिक चिंता के कारण अमेरिकी डॉलर के मुकाबले रुपया कारोबार के दौरान 70.80 के स्तर तक गिर गया। यह इसका ऐतिहासिक रिकॉर्ड निम्न स्तर है। कमजोर रुपये से सोने की चमक बढऩे की उम्मीद की जा रही थी लेकिन वैश्विक बाजार में सोने की कीमत गिरकर 1,193.50 डॉलर प्रति औंस पहुंचने के कारण घरेलू बाजार में भी सोने की चमक फीकी पड़ गई। घरेलू बाजार में सोना गिरकर 29,655 रुपये प्रति 10 ग्राम पहुंच गया। घरेलू वायदा बाजार यानी एमसीएक्स पर सोना 29,700 के आस-पास कारोबार कर रहा है। देश में इस साल सोने की मांग में लगातार गिरावट देखने को मिल रही थी। अप्रैल-जून तिमाही में सोने का आयात 25 फीसदी से भी कम हुआ है। चालू महीने में सोने की मांग बढऩे की उम्मीद जगी थी लेकिन यह उम्मीद टूट सकती है क्योंकि दुनिया के सबसे बड़े गोल्ड फंड एसपीडीआर गोल्ड की होल्डिंग अप्रैल के बाद 10 फीसदी गिर चुकी है। इस समय यह पिछले ढाई साल के निचले स्तर पर है। इस साल वैश्विक बाजार में सोना करीब 8.5 फीसदी और घरेलू बाजार में 1.5 फीसदी टूट चुका है। सराफा मामलों के जानकार अनिल अग्रवाल कहते हैं कि वैश्विक हालात ऐसे हैं कि इस समय निवेशक डॉलर में पैसा लगा रहे हैं। इस कारण दूसरी मुद्रा और जिंस दबाव में हैं। हालांकि हालात यही रहे तो सोने में तेज सुधार भी देखने को मिलेगा। वैश्विक मंदी की बढ़ती आशंका का सबसे ज्यादा असर चांदी पर पड़ रहा है। वैश्विक बाजार में चांदी के दाम ढाई साल के निचले स्तर पर पहुंच चुके हैं। वैश्विक बाजार में चांदी की कीमत 15 डॉलर प्रति औंस के करीब चल रही है। इसके पहले अप्रैल 2016 में चांदी इस स्तर पर थी। वैश्विक बाजार में चांदी के दाम दो महीने पहले 18.13 डॉलर प्रति औंस पर चल रहे थे। चांदी कारोबारी राहुल मेहता कहते हैं कि सोना और मूल धातु में कमजोरी से चांदी पर दोहरा दबाव पड़ रहा है। वैश्विक बाजार का व्यापार युद्ध अब मुद्रा युद्ध में बदल गया है। वैश्विक अर्थव्यवस्था एक बार फिर मंदी की गिरफ्त में आ सकती है जिसके कारण औद्योगिक विकास भी प्रभावित होगा। यही वजह है कि चांदी की कीमतें लगातार लुढक़ रही हैं क्योंकि मांग में कमी आने की आशंका बढ़ती जा रही है। फिलहाल घरेलू बाजार में चांदी 37,825 रुपये प्रति किलोग्राम पर बिक रही है। तुर्की के आर्थिक संकट से एक बार फिर वैश्विक मंदी का डर है जिसका असर दुनियाभर के बाजारों पर देखा जा सकता है। इसने विश्व स्तर पर निवेशकों के रुख को प्रभावित किया है और वे डॉलर को एक सुरक्षित निवेश के तौर पर देख रहे हैं। आनंद राठी शेयर्स ऐंड स्टाक ब्रोकर्स में शोध विश्लेषक आर मारू ने कहा कि आयातकों की अधिक मांग से रुपये की विनिमय दर में गिरावट आई। उन्होंने कहा, तुर्की संकट को लेकर अनिश्चितता तथा डॉलर सूचकांक में तेजी को देखते हुए आयातक आक्रमक तरीके से डॉलर की लिवाली कर रहे हैं। दूसरी तरफ आरबीआई की तरफ से आक्रमक हस्तक्षेप न होने से भी रुपया नीचे आया। सरकार ने अमेरिकी डॉलर के मुकाबले रुपये के अब तक के न्यूनतम स्तर पर पहुंचने के लिए बाह्य कारकों को जिम्मेदार ठहराते हुए कहा कि इसमें चिंता की कोई बात नहीं है।", "IndicHeadlineGeneration", "Hindi"],
- [u"दिल्ली यूनिवर्सिटी देश की प्रसिद्ध यूनिवर्सिटी में से एक है.",
- "IndicParaphrasing", "Hindi"],
- [u"जम्मू एवं कश्मीर के अनंतनाग जिले में शनिवार को सुरक्षाबलों के साथ मुठभेड़ में दो आतंकवादियों को मार गिराया गया।",
- "IndicSentenceSummarization", "Hindi"],
- [u"7 फरवरी, 2016 [SEP] खेल 7 फरवरी, 2016 को कैलिफोर्निया के सांता क्लारा में सैन फ्रांसिस्को खाड़ी क्षेत्र में लेवी स्टेडियम में खेला गया था।",
- "IndicQuestionGeneration", "Hindi"]
-]
-
-iface = gr.Interface(fn=generate, inputs=[text, task_drop_down, language_drop_down], outputs=text_ouptut, title='IndicNLG System',
- description='Currently the model supports ' + supported_lang, article='More information can be found [here](https://ai4bharat.org/language-generation)', examples=examples)
-iface.launch(enable_queue=True)
-
-# with gr.blocks.Blocks() as block:
-# input = gr.Textbox(label="Input")
-# task = gr.Dropdown(["IndicWikiBio", "IndicHeadlineGeneration", "IndicParaphrasing",
-# "IndicSentenceSummarization", "IndicQuestionGeneration"], label="Task")
-# lang = gr.Dropdown(["as", "bn", "gu", "hi", "kn", "ml",
-# "mr", "or", "pa", "ta", "te"], label="Language")
-# generate = gr.Button("Generate")
-# output = gr.Textbox()
-# instructions = gr.HTML("How to use:
\
-# 1. This space supports 5 tasks and 11 Indic languages.
\
-# 2. First select the task from the dropdown box and it will show you an example of Input for Hindi. This default example display will be automated for each language in the future. Choose your language, give your input and then press the generate button. Note the formats for IndicWikiBio and Question generation when testing your own inputs. Also note that if you choose another task then the input will be replaced with the default example for that task.
\
-# 3. The tasks are:
\
-# 3.1 IndicWikiBio where the input is a Wikipedia table and the output will be a one sentence biograpy. You should pass the input in the following format: <TAG> key1 </TAG> value1 <TAG> key2 </TAG> value2.
\
-# 3.2 IndicHeadlineGeneration where the input is a document or paragraph the output will be a short title. Copy a paragraph from your favorite news site and get a headline. Dont paste extemely long paragraphs. You have been warned.
\
-# 3.3 IndicParaphrasing where the input is a sentence and the output is its paraphrase.
\
-# 3.4 IndicSentenceSummarization where the input is a long sentence and the output is a compact version of that sentence.
\
-# 3.5 IndicQuestionGeneration where the input is an answer and context and the output is the question that should be asked to get the answer. You should pass the input in the following format: ANSWER [SEP] CONTEXT.\
-# ")
-# task.change(set_example, inputs=[task, lang], outputs=[input, lang])
-# generate.click(greet, inputs=[input, task, lang], outputs=output)
-# block.launch()
diff --git a/spaces/akhaliq/PaintTransformer/inference.py b/spaces/akhaliq/PaintTransformer/inference.py
deleted file mode 100644
index a3b879d64011b80dec16a13c112f575d208a435c..0000000000000000000000000000000000000000
--- a/spaces/akhaliq/PaintTransformer/inference.py
+++ /dev/null
@@ -1,496 +0,0 @@
-import torch
-import torch.nn.functional as F
-import numpy as np
-from PIL import Image
-import network
-import morphology
-import os
-import math
-
-idx = 0
-
-
-def save_img(img, output_path):
- result = Image.fromarray((img.data.cpu().numpy().transpose((1, 2, 0)) * 255).astype(np.uint8))
- result.save(output_path)
-
-
-def param2stroke(param, H, W, meta_brushes):
- """
- Input a set of stroke parameters and output its corresponding foregrounds and alpha maps.
- Args:
- param: a tensor with shape n_strokes x n_param_per_stroke. Here, param_per_stroke is 8:
- x_center, y_center, width, height, theta, R, G, and B.
- H: output height.
- W: output width.
- meta_brushes: a tensor with shape 2 x 3 x meta_brush_height x meta_brush_width.
- The first slice on the batch dimension denotes vertical brush and the second one denotes horizontal brush.
-
- Returns:
- foregrounds: a tensor with shape n_strokes x 3 x H x W, containing color information.
- alphas: a tensor with shape n_strokes x 3 x H x W,
- containing binary information of whether a pixel is belonging to the stroke (alpha mat), for painting process.
- """
- # Firstly, resize the meta brushes to the required shape,
- # in order to decrease GPU memory especially when the required shape is small.
- meta_brushes_resize = F.interpolate(meta_brushes, (H, W))
- b = param.shape[0]
- # Extract shape parameters and color parameters.
- param_list = torch.split(param, 1, dim=1)
- x0, y0, w, h, theta = [item.squeeze(-1) for item in param_list[:5]]
- R, G, B = param_list[5:]
- # Pre-compute sin theta and cos theta
- sin_theta = torch.sin(torch.acos(torch.tensor(-1., device=param.device)) * theta)
- cos_theta = torch.cos(torch.acos(torch.tensor(-1., device=param.device)) * theta)
- # index means each stroke should use which meta stroke? Vertical meta stroke or horizontal meta stroke.
- # When h > w, vertical stroke should be used. When h <= w, horizontal stroke should be used.
- index = torch.full((b,), -1, device=param.device, dtype=torch.long)
- index[h > w] = 0
- index[h <= w] = 1
- brush = meta_brushes_resize[index.long()]
-
- # Calculate warp matrix according to the rules defined by pytorch, in order for warping.
- warp_00 = cos_theta / w
- warp_01 = sin_theta * H / (W * w)
- warp_02 = (1 - 2 * x0) * cos_theta / w + (1 - 2 * y0) * sin_theta * H / (W * w)
- warp_10 = -sin_theta * W / (H * h)
- warp_11 = cos_theta / h
- warp_12 = (1 - 2 * y0) * cos_theta / h - (1 - 2 * x0) * sin_theta * W / (H * h)
- warp_0 = torch.stack([warp_00, warp_01, warp_02], dim=1)
- warp_1 = torch.stack([warp_10, warp_11, warp_12], dim=1)
- warp = torch.stack([warp_0, warp_1], dim=1)
- # Conduct warping.
- grid = F.affine_grid(warp, [b, 3, H, W], align_corners=False)
- brush = F.grid_sample(brush, grid, align_corners=False)
- # alphas is the binary information suggesting whether a pixel is belonging to the stroke.
- alphas = (brush > 0).float()
- brush = brush.repeat(1, 3, 1, 1)
- alphas = alphas.repeat(1, 3, 1, 1)
- # Give color to foreground strokes.
- color_map = torch.cat([R, G, B], dim=1)
- color_map = color_map.unsqueeze(-1).unsqueeze(-1).repeat(1, 1, H, W)
- foreground = brush * color_map
- # Dilation and erosion are used for foregrounds and alphas respectively to prevent artifacts on stroke borders.
- foreground = morphology.dilation(foreground)
- alphas = morphology.erosion(alphas)
- return foreground, alphas
-
-
-def param2img_serial(
- param, decision, meta_brushes, cur_canvas, frame_dir, has_border=False, original_h=None, original_w=None):
- """
- Input stroke parameters and decisions for each patch, meta brushes, current canvas, frame directory,
- and whether there is a border (if intermediate painting results are required).
- Output the painting results of adding the corresponding strokes on the current canvas.
- Args:
- param: a tensor with shape batch size x patch along height dimension x patch along width dimension
- x n_stroke_per_patch x n_param_per_stroke
- decision: a 01 tensor with shape batch size x patch along height dimension x patch along width dimension
- x n_stroke_per_patch
- meta_brushes: a tensor with shape 2 x 3 x meta_brush_height x meta_brush_width.
- The first slice on the batch dimension denotes vertical brush and the second one denotes horizontal brush.
- cur_canvas: a tensor with shape batch size x 3 x H x W,
- where H and W denote height and width of padded results of original images.
- frame_dir: directory to save intermediate painting results. None means intermediate results are not required.
- has_border: on the last painting layer, in order to make sure that the painting results do not miss
- any important detail, we choose to paint again on this layer but shift patch_size // 2 pixels when
- cutting patches. In this case, if intermediate results are required, we need to cut the shifted length
- on the border before saving, or there would be a black border.
- original_h: to indicate the original height for cropping when saving intermediate results.
- original_w: to indicate the original width for cropping when saving intermediate results.
-
- Returns:
- cur_canvas: a tensor with shape batch size x 3 x H x W, denoting painting results.
- """
- # param: b, h, w, stroke_per_patch, param_per_stroke
- # decision: b, h, w, stroke_per_patch
- b, h, w, s, p = param.shape
- H, W = cur_canvas.shape[-2:]
- is_odd_y = h % 2 == 1
- is_odd_x = w % 2 == 1
- patch_size_y = 2 * H // h
- patch_size_x = 2 * W // w
- even_idx_y = torch.arange(0, h, 2, device=cur_canvas.device)
- even_idx_x = torch.arange(0, w, 2, device=cur_canvas.device)
- odd_idx_y = torch.arange(1, h, 2, device=cur_canvas.device)
- odd_idx_x = torch.arange(1, w, 2, device=cur_canvas.device)
- even_y_even_x_coord_y, even_y_even_x_coord_x = torch.meshgrid([even_idx_y, even_idx_x])
- odd_y_odd_x_coord_y, odd_y_odd_x_coord_x = torch.meshgrid([odd_idx_y, odd_idx_x])
- even_y_odd_x_coord_y, even_y_odd_x_coord_x = torch.meshgrid([even_idx_y, odd_idx_x])
- odd_y_even_x_coord_y, odd_y_even_x_coord_x = torch.meshgrid([odd_idx_y, even_idx_x])
- cur_canvas = F.pad(cur_canvas, [patch_size_x // 4, patch_size_x // 4,
- patch_size_y // 4, patch_size_y // 4, 0, 0, 0, 0])
-
- def partial_render(this_canvas, patch_coord_y, patch_coord_x, stroke_id):
- canvas_patch = F.unfold(this_canvas, (patch_size_y, patch_size_x),
- stride=(patch_size_y // 2, patch_size_x // 2))
- # canvas_patch: b, 3 * py * px, h * w
- canvas_patch = canvas_patch.view(b, 3, patch_size_y, patch_size_x, h, w).contiguous()
- canvas_patch = canvas_patch.permute(0, 4, 5, 1, 2, 3).contiguous()
- # canvas_patch: b, h, w, 3, py, px
- selected_canvas_patch = canvas_patch[:, patch_coord_y, patch_coord_x, :, :, :]
- selected_h, selected_w = selected_canvas_patch.shape[1:3]
- selected_param = param[:, patch_coord_y, patch_coord_x, stroke_id, :].view(-1, p).contiguous()
- selected_decision = decision[:, patch_coord_y, patch_coord_x, stroke_id].view(-1).contiguous()
- selected_foregrounds = torch.zeros(selected_param.shape[0], 3, patch_size_y, patch_size_x,
- device=this_canvas.device)
- selected_alphas = torch.zeros(selected_param.shape[0], 3, patch_size_y, patch_size_x, device=this_canvas.device)
- if selected_param[selected_decision, :].shape[0] > 0:
- selected_foregrounds[selected_decision, :, :, :], selected_alphas[selected_decision, :, :, :] = \
- param2stroke(selected_param[selected_decision, :], patch_size_y, patch_size_x, meta_brushes)
- selected_foregrounds = selected_foregrounds.view(
- b, selected_h, selected_w, 3, patch_size_y, patch_size_x).contiguous()
- selected_alphas = selected_alphas.view(b, selected_h, selected_w, 3, patch_size_y, patch_size_x).contiguous()
- selected_decision = selected_decision.view(b, selected_h, selected_w, 1, 1, 1).contiguous()
- selected_canvas_patch = selected_foregrounds * selected_alphas * selected_decision + selected_canvas_patch * (
- 1 - selected_alphas * selected_decision)
- this_canvas = selected_canvas_patch.permute(0, 3, 1, 4, 2, 5).contiguous()
- # this_canvas: b, 3, selected_h, py, selected_w, px
- this_canvas = this_canvas.view(b, 3, selected_h * patch_size_y, selected_w * patch_size_x).contiguous()
- # this_canvas: b, 3, selected_h * py, selected_w * px
- return this_canvas
-
- global idx
- if has_border:
- factor = 2
- else:
- factor = 4
- if even_idx_y.shape[0] > 0 and even_idx_x.shape[0] > 0:
- for i in range(s):
- canvas = partial_render(cur_canvas, even_y_even_x_coord_y, even_y_even_x_coord_x, i)
- if not is_odd_y:
- canvas = torch.cat([canvas, cur_canvas[:, :, -patch_size_y // 2:, :canvas.shape[3]]], dim=2)
- if not is_odd_x:
- canvas = torch.cat([canvas, cur_canvas[:, :, :canvas.shape[2], -patch_size_x // 2:]], dim=3)
- cur_canvas = canvas
- idx += 1
- if frame_dir is not None:
- frame = crop(cur_canvas[:, :, patch_size_y // factor:-patch_size_y // factor,
- patch_size_x // factor:-patch_size_x // factor], original_h, original_w)
- save_img(frame[0], os.path.join(frame_dir, '%03d.jpg' % idx))
-
- if odd_idx_y.shape[0] > 0 and odd_idx_x.shape[0] > 0:
- for i in range(s):
- canvas = partial_render(cur_canvas, odd_y_odd_x_coord_y, odd_y_odd_x_coord_x, i)
- canvas = torch.cat([cur_canvas[:, :, :patch_size_y // 2, -canvas.shape[3]:], canvas], dim=2)
- canvas = torch.cat([cur_canvas[:, :, -canvas.shape[2]:, :patch_size_x // 2], canvas], dim=3)
- if is_odd_y:
- canvas = torch.cat([canvas, cur_canvas[:, :, -patch_size_y // 2:, :canvas.shape[3]]], dim=2)
- if is_odd_x:
- canvas = torch.cat([canvas, cur_canvas[:, :, :canvas.shape[2], -patch_size_x // 2:]], dim=3)
- cur_canvas = canvas
- idx += 1
- if frame_dir is not None:
- frame = crop(cur_canvas[:, :, patch_size_y // factor:-patch_size_y // factor,
- patch_size_x // factor:-patch_size_x // factor], original_h, original_w)
- save_img(frame[0], os.path.join(frame_dir, '%03d.jpg' % idx))
-
- if odd_idx_y.shape[0] > 0 and even_idx_x.shape[0] > 0:
- for i in range(s):
- canvas = partial_render(cur_canvas, odd_y_even_x_coord_y, odd_y_even_x_coord_x, i)
- canvas = torch.cat([cur_canvas[:, :, :patch_size_y // 2, :canvas.shape[3]], canvas], dim=2)
- if is_odd_y:
- canvas = torch.cat([canvas, cur_canvas[:, :, -patch_size_y // 2:, :canvas.shape[3]]], dim=2)
- if not is_odd_x:
- canvas = torch.cat([canvas, cur_canvas[:, :, :canvas.shape[2], -patch_size_x // 2:]], dim=3)
- cur_canvas = canvas
- idx += 1
- if frame_dir is not None:
- frame = crop(cur_canvas[:, :, patch_size_y // factor:-patch_size_y // factor,
- patch_size_x // factor:-patch_size_x // factor], original_h, original_w)
- save_img(frame[0], os.path.join(frame_dir, '%03d.jpg' % idx))
-
- if even_idx_y.shape[0] > 0 and odd_idx_x.shape[0] > 0:
- for i in range(s):
- canvas = partial_render(cur_canvas, even_y_odd_x_coord_y, even_y_odd_x_coord_x, i)
- canvas = torch.cat([cur_canvas[:, :, :canvas.shape[2], :patch_size_x // 2], canvas], dim=3)
- if not is_odd_y:
- canvas = torch.cat([canvas, cur_canvas[:, :, -patch_size_y // 2:, -canvas.shape[3]:]], dim=2)
- if is_odd_x:
- canvas = torch.cat([canvas, cur_canvas[:, :, :canvas.shape[2], -patch_size_x // 2:]], dim=3)
- cur_canvas = canvas
- idx += 1
- if frame_dir is not None:
- frame = crop(cur_canvas[:, :, patch_size_y // factor:-patch_size_y // factor,
- patch_size_x // factor:-patch_size_x // factor], original_h, original_w)
- save_img(frame[0], os.path.join(frame_dir, '%03d.jpg' % idx))
-
- cur_canvas = cur_canvas[:, :, patch_size_y // 4:-patch_size_y // 4, patch_size_x // 4:-patch_size_x // 4]
-
- return cur_canvas
-
-
-def param2img_parallel(param, decision, meta_brushes, cur_canvas):
- """
- Input stroke parameters and decisions for each patch, meta brushes, current canvas, frame directory,
- and whether there is a border (if intermediate painting results are required).
- Output the painting results of adding the corresponding strokes on the current canvas.
- Args:
- param: a tensor with shape batch size x patch along height dimension x patch along width dimension
- x n_stroke_per_patch x n_param_per_stroke
- decision: a 01 tensor with shape batch size x patch along height dimension x patch along width dimension
- x n_stroke_per_patch
- meta_brushes: a tensor with shape 2 x 3 x meta_brush_height x meta_brush_width.
- The first slice on the batch dimension denotes vertical brush and the second one denotes horizontal brush.
- cur_canvas: a tensor with shape batch size x 3 x H x W,
- where H and W denote height and width of padded results of original images.
-
- Returns:
- cur_canvas: a tensor with shape batch size x 3 x H x W, denoting painting results.
- """
- # param: b, h, w, stroke_per_patch, param_per_stroke
- # decision: b, h, w, stroke_per_patch
- b, h, w, s, p = param.shape
- param = param.view(-1, 8).contiguous()
- decision = decision.view(-1).contiguous().bool()
- H, W = cur_canvas.shape[-2:]
- is_odd_y = h % 2 == 1
- is_odd_x = w % 2 == 1
- patch_size_y = 2 * H // h
- patch_size_x = 2 * W // w
- even_idx_y = torch.arange(0, h, 2, device=cur_canvas.device)
- even_idx_x = torch.arange(0, w, 2, device=cur_canvas.device)
- odd_idx_y = torch.arange(1, h, 2, device=cur_canvas.device)
- odd_idx_x = torch.arange(1, w, 2, device=cur_canvas.device)
- even_y_even_x_coord_y, even_y_even_x_coord_x = torch.meshgrid([even_idx_y, even_idx_x])
- odd_y_odd_x_coord_y, odd_y_odd_x_coord_x = torch.meshgrid([odd_idx_y, odd_idx_x])
- even_y_odd_x_coord_y, even_y_odd_x_coord_x = torch.meshgrid([even_idx_y, odd_idx_x])
- odd_y_even_x_coord_y, odd_y_even_x_coord_x = torch.meshgrid([odd_idx_y, even_idx_x])
- cur_canvas = F.pad(cur_canvas, [patch_size_x // 4, patch_size_x // 4,
- patch_size_y // 4, patch_size_y // 4, 0, 0, 0, 0])
- foregrounds = torch.zeros(param.shape[0], 3, patch_size_y, patch_size_x, device=cur_canvas.device)
- alphas = torch.zeros(param.shape[0], 3, patch_size_y, patch_size_x, device=cur_canvas.device)
- valid_foregrounds, valid_alphas = param2stroke(param[decision, :], patch_size_y, patch_size_x, meta_brushes)
- foregrounds[decision, :, :, :] = valid_foregrounds
- alphas[decision, :, :, :] = valid_alphas
- # foreground, alpha: b * h * w * stroke_per_patch, 3, patch_size_y, patch_size_x
- foregrounds = foregrounds.view(-1, h, w, s, 3, patch_size_y, patch_size_x).contiguous()
- alphas = alphas.view(-1, h, w, s, 3, patch_size_y, patch_size_x).contiguous()
- # foreground, alpha: b, h, w, stroke_per_patch, 3, render_size_y, render_size_x
- decision = decision.view(-1, h, w, s, 1, 1, 1).contiguous()
-
- # decision: b, h, w, stroke_per_patch, 1, 1, 1
-
- def partial_render(this_canvas, patch_coord_y, patch_coord_x):
-
- canvas_patch = F.unfold(this_canvas, (patch_size_y, patch_size_x),
- stride=(patch_size_y // 2, patch_size_x // 2))
- # canvas_patch: b, 3 * py * px, h * w
- canvas_patch = canvas_patch.view(b, 3, patch_size_y, patch_size_x, h, w).contiguous()
- canvas_patch = canvas_patch.permute(0, 4, 5, 1, 2, 3).contiguous()
- # canvas_patch: b, h, w, 3, py, px
- selected_canvas_patch = canvas_patch[:, patch_coord_y, patch_coord_x, :, :, :]
- selected_foregrounds = foregrounds[:, patch_coord_y, patch_coord_x, :, :, :, :]
- selected_alphas = alphas[:, patch_coord_y, patch_coord_x, :, :, :, :]
- selected_decisions = decision[:, patch_coord_y, patch_coord_x, :, :, :, :]
- for i in range(s):
- cur_foreground = selected_foregrounds[:, :, :, i, :, :, :]
- cur_alpha = selected_alphas[:, :, :, i, :, :, :]
- cur_decision = selected_decisions[:, :, :, i, :, :, :]
- selected_canvas_patch = cur_foreground * cur_alpha * cur_decision + selected_canvas_patch * (
- 1 - cur_alpha * cur_decision)
- this_canvas = selected_canvas_patch.permute(0, 3, 1, 4, 2, 5).contiguous()
- # this_canvas: b, 3, h_half, py, w_half, px
- h_half = this_canvas.shape[2]
- w_half = this_canvas.shape[4]
- this_canvas = this_canvas.view(b, 3, h_half * patch_size_y, w_half * patch_size_x).contiguous()
- # this_canvas: b, 3, h_half * py, w_half * px
- return this_canvas
-
- if even_idx_y.shape[0] > 0 and even_idx_x.shape[0] > 0:
- canvas = partial_render(cur_canvas, even_y_even_x_coord_y, even_y_even_x_coord_x)
- if not is_odd_y:
- canvas = torch.cat([canvas, cur_canvas[:, :, -patch_size_y // 2:, :canvas.shape[3]]], dim=2)
- if not is_odd_x:
- canvas = torch.cat([canvas, cur_canvas[:, :, :canvas.shape[2], -patch_size_x // 2:]], dim=3)
- cur_canvas = canvas
-
- if odd_idx_y.shape[0] > 0 and odd_idx_x.shape[0] > 0:
- canvas = partial_render(cur_canvas, odd_y_odd_x_coord_y, odd_y_odd_x_coord_x)
- canvas = torch.cat([cur_canvas[:, :, :patch_size_y // 2, -canvas.shape[3]:], canvas], dim=2)
- canvas = torch.cat([cur_canvas[:, :, -canvas.shape[2]:, :patch_size_x // 2], canvas], dim=3)
- if is_odd_y:
- canvas = torch.cat([canvas, cur_canvas[:, :, -patch_size_y // 2:, :canvas.shape[3]]], dim=2)
- if is_odd_x:
- canvas = torch.cat([canvas, cur_canvas[:, :, :canvas.shape[2], -patch_size_x // 2:]], dim=3)
- cur_canvas = canvas
-
- if odd_idx_y.shape[0] > 0 and even_idx_x.shape[0] > 0:
- canvas = partial_render(cur_canvas, odd_y_even_x_coord_y, odd_y_even_x_coord_x)
- canvas = torch.cat([cur_canvas[:, :, :patch_size_y // 2, :canvas.shape[3]], canvas], dim=2)
- if is_odd_y:
- canvas = torch.cat([canvas, cur_canvas[:, :, -patch_size_y // 2:, :canvas.shape[3]]], dim=2)
- if not is_odd_x:
- canvas = torch.cat([canvas, cur_canvas[:, :, :canvas.shape[2], -patch_size_x // 2:]], dim=3)
- cur_canvas = canvas
-
- if even_idx_y.shape[0] > 0 and odd_idx_x.shape[0] > 0:
- canvas = partial_render(cur_canvas, even_y_odd_x_coord_y, even_y_odd_x_coord_x)
- canvas = torch.cat([cur_canvas[:, :, :canvas.shape[2], :patch_size_x // 2], canvas], dim=3)
- if not is_odd_y:
- canvas = torch.cat([canvas, cur_canvas[:, :, -patch_size_y // 2:, -canvas.shape[3]:]], dim=2)
- if is_odd_x:
- canvas = torch.cat([canvas, cur_canvas[:, :, :canvas.shape[2], -patch_size_x // 2:]], dim=3)
- cur_canvas = canvas
-
- cur_canvas = cur_canvas[:, :, patch_size_y // 4:-patch_size_y // 4, patch_size_x // 4:-patch_size_x // 4]
-
- return cur_canvas
-
-
-def read_img(img_path, img_type='RGB', h=None, w=None):
- img = Image.open(img_path).convert(img_type)
- if h is not None and w is not None:
- img = img.resize((w, h), resample=Image.NEAREST)
- img = np.array(img)
- if img.ndim == 2:
- img = np.expand_dims(img, axis=-1)
- img = img.transpose((2, 0, 1))
- img = torch.from_numpy(img).unsqueeze(0).float() / 255.
- return img
-
-
-def pad(img, H, W):
- b, c, h, w = img.shape
- pad_h = (H - h) // 2
- pad_w = (W - w) // 2
- remainder_h = (H - h) % 2
- remainder_w = (W - w) % 2
- img = torch.cat([torch.zeros((b, c, pad_h, w), device=img.device), img,
- torch.zeros((b, c, pad_h + remainder_h, w), device=img.device)], dim=-2)
- img = torch.cat([torch.zeros((b, c, H, pad_w), device=img.device), img,
- torch.zeros((b, c, H, pad_w + remainder_w), device=img.device)], dim=-1)
- return img
-
-
-def crop(img, h, w):
- H, W = img.shape[-2:]
- pad_h = (H - h) // 2
- pad_w = (W - w) // 2
- remainder_h = (H - h) % 2
- remainder_w = (W - w) % 2
- img = img[:, :, pad_h:H - pad_h - remainder_h, pad_w:W - pad_w - remainder_w]
- return img
-
-
-def main(input_path, model_path, output_dir, need_animation=False, resize_h=None, resize_w=None, serial=False):
- if not os.path.exists(output_dir):
- os.mkdir(output_dir)
- input_name = os.path.basename(input_path)
- output_path = os.path.join(output_dir, input_name)
- frame_dir = None
- if need_animation:
- if not serial:
- print('It must be under serial mode if animation results are required, so serial flag is set to True!')
- serial = True
- frame_dir = os.path.join(output_dir, input_name[:input_name.find('.')])
- if not os.path.exists(frame_dir):
- os.mkdir(frame_dir)
- patch_size = 32
- stroke_num = 8
- device = torch.device("cuda" if torch.cuda.is_available() else "cpu")
- net_g = network.Painter(5, stroke_num, 256, 8, 3, 3).to(device)
- net_g.load_state_dict(torch.load(model_path))
- net_g.eval()
- for param in net_g.parameters():
- param.requires_grad = False
-
- brush_large_vertical = read_img('brush/brush_large_vertical.png', 'L').to(device)
- brush_large_horizontal = read_img('brush/brush_large_horizontal.png', 'L').to(device)
- meta_brushes = torch.cat(
- [brush_large_vertical, brush_large_horizontal], dim=0)
-
- with torch.no_grad():
- original_img = read_img(input_path, 'RGB', resize_h, resize_w).to(device)
- original_h, original_w = original_img.shape[-2:]
- K = max(math.ceil(math.log2(max(original_h, original_w) / patch_size)), 0)
- original_img_pad_size = patch_size * (2 ** K)
- original_img_pad = pad(original_img, original_img_pad_size, original_img_pad_size)
- final_result = torch.zeros_like(original_img_pad).to(device)
- for layer in range(0, K + 1):
- layer_size = patch_size * (2 ** layer)
- img = F.interpolate(original_img_pad, (layer_size, layer_size))
- result = F.interpolate(final_result, (patch_size * (2 ** layer), patch_size * (2 ** layer)))
- img_patch = F.unfold(img, (patch_size, patch_size), stride=(patch_size, patch_size))
- result_patch = F.unfold(result, (patch_size, patch_size),
- stride=(patch_size, patch_size))
- # There are patch_num * patch_num patches in total
- patch_num = (layer_size - patch_size) // patch_size + 1
-
- # img_patch, result_patch: b, 3 * output_size * output_size, h * w
- img_patch = img_patch.permute(0, 2, 1).contiguous().view(-1, 3, patch_size, patch_size).contiguous()
- result_patch = result_patch.permute(0, 2, 1).contiguous().view(
- -1, 3, patch_size, patch_size).contiguous()
- shape_param, stroke_decision = net_g(img_patch, result_patch)
- stroke_decision = network.SignWithSigmoidGrad.apply(stroke_decision)
-
- grid = shape_param[:, :, :2].view(img_patch.shape[0] * stroke_num, 1, 1, 2).contiguous()
- img_temp = img_patch.unsqueeze(1).contiguous().repeat(1, stroke_num, 1, 1, 1).view(
- img_patch.shape[0] * stroke_num, 3, patch_size, patch_size).contiguous()
- color = F.grid_sample(img_temp, 2 * grid - 1, align_corners=False).view(
- img_patch.shape[0], stroke_num, 3).contiguous()
- stroke_param = torch.cat([shape_param, color], dim=-1)
- # stroke_param: b * h * w, stroke_per_patch, param_per_stroke
- # stroke_decision: b * h * w, stroke_per_patch, 1
- param = stroke_param.view(1, patch_num, patch_num, stroke_num, 8).contiguous()
- decision = stroke_decision.view(1, patch_num, patch_num, stroke_num).contiguous().bool()
- # param: b, h, w, stroke_per_patch, 8
- # decision: b, h, w, stroke_per_patch
- param[..., :2] = param[..., :2] / 2 + 0.25
- param[..., 2:4] = param[..., 2:4] / 2
- if serial:
- final_result = param2img_serial(param, decision, meta_brushes, final_result,
- frame_dir, False, original_h, original_w)
- else:
- final_result = param2img_parallel(param, decision, meta_brushes, final_result)
-
- border_size = original_img_pad_size // (2 * patch_num)
- img = F.interpolate(original_img_pad, (patch_size * (2 ** layer), patch_size * (2 ** layer)))
- result = F.interpolate(final_result, (patch_size * (2 ** layer), patch_size * (2 ** layer)))
- img = F.pad(img, [patch_size // 2, patch_size // 2, patch_size // 2, patch_size // 2,
- 0, 0, 0, 0])
- result = F.pad(result, [patch_size // 2, patch_size // 2, patch_size // 2, patch_size // 2,
- 0, 0, 0, 0])
- img_patch = F.unfold(img, (patch_size, patch_size), stride=(patch_size, patch_size))
- result_patch = F.unfold(result, (patch_size, patch_size), stride=(patch_size, patch_size))
- final_result = F.pad(final_result, [border_size, border_size, border_size, border_size, 0, 0, 0, 0])
- h = (img.shape[2] - patch_size) // patch_size + 1
- w = (img.shape[3] - patch_size) // patch_size + 1
- # img_patch, result_patch: b, 3 * output_size * output_size, h * w
- img_patch = img_patch.permute(0, 2, 1).contiguous().view(-1, 3, patch_size, patch_size).contiguous()
- result_patch = result_patch.permute(0, 2, 1).contiguous().view(-1, 3, patch_size, patch_size).contiguous()
- shape_param, stroke_decision = net_g(img_patch, result_patch)
-
- grid = shape_param[:, :, :2].view(img_patch.shape[0] * stroke_num, 1, 1, 2).contiguous()
- img_temp = img_patch.unsqueeze(1).contiguous().repeat(1, stroke_num, 1, 1, 1).view(
- img_patch.shape[0] * stroke_num, 3, patch_size, patch_size).contiguous()
- color = F.grid_sample(img_temp, 2 * grid - 1, align_corners=False).view(
- img_patch.shape[0], stroke_num, 3).contiguous()
- stroke_param = torch.cat([shape_param, color], dim=-1)
- # stroke_param: b * h * w, stroke_per_patch, param_per_stroke
- # stroke_decision: b * h * w, stroke_per_patch, 1
- param = stroke_param.view(1, h, w, stroke_num, 8).contiguous()
- decision = stroke_decision.view(1, h, w, stroke_num).contiguous().bool()
- # param: b, h, w, stroke_per_patch, 8
- # decision: b, h, w, stroke_per_patch
- param[..., :2] = param[..., :2] / 2 + 0.25
- param[..., 2:4] = param[..., 2:4] / 2
- if serial:
- final_result = param2img_serial(param, decision, meta_brushes, final_result,
- frame_dir, True, original_h, original_w)
- else:
- final_result = param2img_parallel(param, decision, meta_brushes, final_result)
- final_result = final_result[:, :, border_size:-border_size, border_size:-border_size]
-
- final_result = crop(final_result, original_h, original_w)
- save_img(final_result[0], output_path)
-
-
-if __name__ == '__main__':
- main(input_path='input/chicago.jpg',
- model_path='model.pth',
- output_dir='output/',
- need_animation=False, # whether need intermediate results for animation.
- resize_h=None, # resize original input to this size. None means do not resize.
- resize_w=None, # resize original input to this size. None means do not resize.
- serial=False) # if need animation, serial must be True.
diff --git a/spaces/akhaliq/stylegan3_clip/torch_utils/misc.py b/spaces/akhaliq/stylegan3_clip/torch_utils/misc.py
deleted file mode 100644
index 02f97e276e756bb1c4140ac16e7e4bcc63da628a..0000000000000000000000000000000000000000
--- a/spaces/akhaliq/stylegan3_clip/torch_utils/misc.py
+++ /dev/null
@@ -1,266 +0,0 @@
-# Copyright (c) 2021, NVIDIA CORPORATION & AFFILIATES. All rights reserved.
-#
-# NVIDIA CORPORATION and its licensors retain all intellectual property
-# and proprietary rights in and to this software, related documentation
-# and any modifications thereto. Any use, reproduction, disclosure or
-# distribution of this software and related documentation without an express
-# license agreement from NVIDIA CORPORATION is strictly prohibited.
-
-import re
-import contextlib
-import numpy as np
-import torch
-import warnings
-import dnnlib
-
-#----------------------------------------------------------------------------
-# Cached construction of constant tensors. Avoids CPU=>GPU copy when the
-# same constant is used multiple times.
-
-_constant_cache = dict()
-
-def constant(value, shape=None, dtype=None, device=None, memory_format=None):
- value = np.asarray(value)
- if shape is not None:
- shape = tuple(shape)
- if dtype is None:
- dtype = torch.get_default_dtype()
- if device is None:
- device = torch.device('cpu')
- if memory_format is None:
- memory_format = torch.contiguous_format
-
- key = (value.shape, value.dtype, value.tobytes(), shape, dtype, device, memory_format)
- tensor = _constant_cache.get(key, None)
- if tensor is None:
- tensor = torch.as_tensor(value.copy(), dtype=dtype, device=device)
- if shape is not None:
- tensor, _ = torch.broadcast_tensors(tensor, torch.empty(shape))
- tensor = tensor.contiguous(memory_format=memory_format)
- _constant_cache[key] = tensor
- return tensor
-
-#----------------------------------------------------------------------------
-# Replace NaN/Inf with specified numerical values.
-
-try:
- nan_to_num = torch.nan_to_num # 1.8.0a0
-except AttributeError:
- def nan_to_num(input, nan=0.0, posinf=None, neginf=None, *, out=None): # pylint: disable=redefined-builtin
- assert isinstance(input, torch.Tensor)
- if posinf is None:
- posinf = torch.finfo(input.dtype).max
- if neginf is None:
- neginf = torch.finfo(input.dtype).min
- assert nan == 0
- return torch.clamp(input.unsqueeze(0).nansum(0), min=neginf, max=posinf, out=out)
-
-#----------------------------------------------------------------------------
-# Symbolic assert.
-
-try:
- symbolic_assert = torch._assert # 1.8.0a0 # pylint: disable=protected-access
-except AttributeError:
- symbolic_assert = torch.Assert # 1.7.0
-
-#----------------------------------------------------------------------------
-# Context manager to temporarily suppress known warnings in torch.jit.trace().
-# Note: Cannot use catch_warnings because of https://bugs.python.org/issue29672
-
-@contextlib.contextmanager
-def suppress_tracer_warnings():
- flt = ('ignore', None, torch.jit.TracerWarning, None, 0)
- warnings.filters.insert(0, flt)
- yield
- warnings.filters.remove(flt)
-
-#----------------------------------------------------------------------------
-# Assert that the shape of a tensor matches the given list of integers.
-# None indicates that the size of a dimension is allowed to vary.
-# Performs symbolic assertion when used in torch.jit.trace().
-
-def assert_shape(tensor, ref_shape):
- if tensor.ndim != len(ref_shape):
- raise AssertionError(f'Wrong number of dimensions: got {tensor.ndim}, expected {len(ref_shape)}')
- for idx, (size, ref_size) in enumerate(zip(tensor.shape, ref_shape)):
- if ref_size is None:
- pass
- elif isinstance(ref_size, torch.Tensor):
- with suppress_tracer_warnings(): # as_tensor results are registered as constants
- symbolic_assert(torch.equal(torch.as_tensor(size), ref_size), f'Wrong size for dimension {idx}')
- elif isinstance(size, torch.Tensor):
- with suppress_tracer_warnings(): # as_tensor results are registered as constants
- symbolic_assert(torch.equal(size, torch.as_tensor(ref_size)), f'Wrong size for dimension {idx}: expected {ref_size}')
- elif size != ref_size:
- raise AssertionError(f'Wrong size for dimension {idx}: got {size}, expected {ref_size}')
-
-#----------------------------------------------------------------------------
-# Function decorator that calls torch.autograd.profiler.record_function().
-
-def profiled_function(fn):
- def decorator(*args, **kwargs):
- with torch.autograd.profiler.record_function(fn.__name__):
- return fn(*args, **kwargs)
- decorator.__name__ = fn.__name__
- return decorator
-
-#----------------------------------------------------------------------------
-# Sampler for torch.utils.data.DataLoader that loops over the dataset
-# indefinitely, shuffling items as it goes.
-
-class InfiniteSampler(torch.utils.data.Sampler):
- def __init__(self, dataset, rank=0, num_replicas=1, shuffle=True, seed=0, window_size=0.5):
- assert len(dataset) > 0
- assert num_replicas > 0
- assert 0 <= rank < num_replicas
- assert 0 <= window_size <= 1
- super().__init__(dataset)
- self.dataset = dataset
- self.rank = rank
- self.num_replicas = num_replicas
- self.shuffle = shuffle
- self.seed = seed
- self.window_size = window_size
-
- def __iter__(self):
- order = np.arange(len(self.dataset))
- rnd = None
- window = 0
- if self.shuffle:
- rnd = np.random.RandomState(self.seed)
- rnd.shuffle(order)
- window = int(np.rint(order.size * self.window_size))
-
- idx = 0
- while True:
- i = idx % order.size
- if idx % self.num_replicas == self.rank:
- yield order[i]
- if window >= 2:
- j = (i - rnd.randint(window)) % order.size
- order[i], order[j] = order[j], order[i]
- idx += 1
-
-#----------------------------------------------------------------------------
-# Utilities for operating with torch.nn.Module parameters and buffers.
-
-def params_and_buffers(module):
- assert isinstance(module, torch.nn.Module)
- return list(module.parameters()) + list(module.buffers())
-
-def named_params_and_buffers(module):
- assert isinstance(module, torch.nn.Module)
- return list(module.named_parameters()) + list(module.named_buffers())
-
-def copy_params_and_buffers(src_module, dst_module, require_all=False):
- assert isinstance(src_module, torch.nn.Module)
- assert isinstance(dst_module, torch.nn.Module)
- src_tensors = dict(named_params_and_buffers(src_module))
- for name, tensor in named_params_and_buffers(dst_module):
- assert (name in src_tensors) or (not require_all)
- if name in src_tensors:
- tensor.copy_(src_tensors[name].detach()).requires_grad_(tensor.requires_grad)
-
-#----------------------------------------------------------------------------
-# Context manager for easily enabling/disabling DistributedDataParallel
-# synchronization.
-
-@contextlib.contextmanager
-def ddp_sync(module, sync):
- assert isinstance(module, torch.nn.Module)
- if sync or not isinstance(module, torch.nn.parallel.DistributedDataParallel):
- yield
- else:
- with module.no_sync():
- yield
-
-#----------------------------------------------------------------------------
-# Check DistributedDataParallel consistency across processes.
-
-def check_ddp_consistency(module, ignore_regex=None):
- assert isinstance(module, torch.nn.Module)
- for name, tensor in named_params_and_buffers(module):
- fullname = type(module).__name__ + '.' + name
- if ignore_regex is not None and re.fullmatch(ignore_regex, fullname):
- continue
- tensor = tensor.detach()
- if tensor.is_floating_point():
- tensor = nan_to_num(tensor)
- other = tensor.clone()
- torch.distributed.broadcast(tensor=other, src=0)
- assert (tensor == other).all(), fullname
-
-#----------------------------------------------------------------------------
-# Print summary table of module hierarchy.
-
-def print_module_summary(module, inputs, max_nesting=3, skip_redundant=True):
- assert isinstance(module, torch.nn.Module)
- assert not isinstance(module, torch.jit.ScriptModule)
- assert isinstance(inputs, (tuple, list))
-
- # Register hooks.
- entries = []
- nesting = [0]
- def pre_hook(_mod, _inputs):
- nesting[0] += 1
- def post_hook(mod, _inputs, outputs):
- nesting[0] -= 1
- if nesting[0] <= max_nesting:
- outputs = list(outputs) if isinstance(outputs, (tuple, list)) else [outputs]
- outputs = [t for t in outputs if isinstance(t, torch.Tensor)]
- entries.append(dnnlib.EasyDict(mod=mod, outputs=outputs))
- hooks = [mod.register_forward_pre_hook(pre_hook) for mod in module.modules()]
- hooks += [mod.register_forward_hook(post_hook) for mod in module.modules()]
-
- # Run module.
- outputs = module(*inputs)
- for hook in hooks:
- hook.remove()
-
- # Identify unique outputs, parameters, and buffers.
- tensors_seen = set()
- for e in entries:
- e.unique_params = [t for t in e.mod.parameters() if id(t) not in tensors_seen]
- e.unique_buffers = [t for t in e.mod.buffers() if id(t) not in tensors_seen]
- e.unique_outputs = [t for t in e.outputs if id(t) not in tensors_seen]
- tensors_seen |= {id(t) for t in e.unique_params + e.unique_buffers + e.unique_outputs}
-
- # Filter out redundant entries.
- if skip_redundant:
- entries = [e for e in entries if len(e.unique_params) or len(e.unique_buffers) or len(e.unique_outputs)]
-
- # Construct table.
- rows = [[type(module).__name__, 'Parameters', 'Buffers', 'Output shape', 'Datatype']]
- rows += [['---'] * len(rows[0])]
- param_total = 0
- buffer_total = 0
- submodule_names = {mod: name for name, mod in module.named_modules()}
- for e in entries:
- name = '' if e.mod is module else submodule_names[e.mod]
- param_size = sum(t.numel() for t in e.unique_params)
- buffer_size = sum(t.numel() for t in e.unique_buffers)
- output_shapes = [str(list(t.shape)) for t in e.outputs]
- output_dtypes = [str(t.dtype).split('.')[-1] for t in e.outputs]
- rows += [[
- name + (':0' if len(e.outputs) >= 2 else ''),
- str(param_size) if param_size else '-',
- str(buffer_size) if buffer_size else '-',
- (output_shapes + ['-'])[0],
- (output_dtypes + ['-'])[0],
- ]]
- for idx in range(1, len(e.outputs)):
- rows += [[name + f':{idx}', '-', '-', output_shapes[idx], output_dtypes[idx]]]
- param_total += param_size
- buffer_total += buffer_size
- rows += [['---'] * len(rows[0])]
- rows += [['Total', str(param_total), str(buffer_total), '-', '-']]
-
- # Print table.
- widths = [max(len(cell) for cell in column) for column in zip(*rows)]
- print()
- for row in rows:
- print(' '.join(cell + ' ' * (width - len(cell)) for cell, width in zip(row, widths)))
- print()
- return outputs
-
-#----------------------------------------------------------------------------
diff --git a/spaces/alexray/btc_predictor/venv/lib/python3.10/site-packages/pip/_vendor/chardet/langturkishmodel.py b/spaces/alexray/btc_predictor/venv/lib/python3.10/site-packages/pip/_vendor/chardet/langturkishmodel.py
deleted file mode 100644
index 43f4230aead2fd8c9f685bd0a726cf0723a9d98d..0000000000000000000000000000000000000000
--- a/spaces/alexray/btc_predictor/venv/lib/python3.10/site-packages/pip/_vendor/chardet/langturkishmodel.py
+++ /dev/null
@@ -1,4383 +0,0 @@
-#!/usr/bin/env python
-# -*- coding: utf-8 -*-
-
-from pip._vendor.chardet.sbcharsetprober import SingleByteCharSetModel
-
-
-# 3: Positive
-# 2: Likely
-# 1: Unlikely
-# 0: Negative
-
-TURKISH_LANG_MODEL = {
- 23: { # 'A'
- 23: 0, # 'A'
- 37: 0, # 'B'
- 47: 0, # 'C'
- 39: 0, # 'D'
- 29: 0, # 'E'
- 52: 0, # 'F'
- 36: 0, # 'G'
- 45: 0, # 'H'
- 53: 0, # 'I'
- 60: 0, # 'J'
- 16: 0, # 'K'
- 49: 0, # 'L'
- 20: 0, # 'M'
- 46: 0, # 'N'
- 42: 0, # 'O'
- 48: 0, # 'P'
- 44: 0, # 'R'
- 35: 0, # 'S'
- 31: 0, # 'T'
- 51: 0, # 'U'
- 38: 0, # 'V'
- 62: 0, # 'W'
- 43: 0, # 'Y'
- 56: 0, # 'Z'
- 1: 3, # 'a'
- 21: 0, # 'b'
- 28: 0, # 'c'
- 12: 2, # 'd'
- 2: 3, # 'e'
- 18: 0, # 'f'
- 27: 1, # 'g'
- 25: 1, # 'h'
- 3: 1, # 'i'
- 24: 0, # 'j'
- 10: 2, # 'k'
- 5: 1, # 'l'
- 13: 1, # 'm'
- 4: 1, # 'n'
- 15: 0, # 'o'
- 26: 0, # 'p'
- 7: 1, # 'r'
- 8: 1, # 's'
- 9: 1, # 't'
- 14: 1, # 'u'
- 32: 0, # 'v'
- 57: 0, # 'w'
- 58: 0, # 'x'
- 11: 3, # 'y'
- 22: 0, # 'z'
- 63: 0, # '·'
- 54: 0, # 'Ç'
- 50: 0, # 'Ö'
- 55: 0, # 'Ü'
- 59: 0, # 'â'
- 33: 1, # 'ç'
- 61: 0, # 'î'
- 34: 0, # 'ö'
- 17: 0, # 'ü'
- 30: 0, # 'ğ'
- 41: 0, # 'İ'
- 6: 0, # 'ı'
- 40: 0, # 'Ş'
- 19: 0, # 'ş'
- },
- 37: { # 'B'
- 23: 0, # 'A'
- 37: 0, # 'B'
- 47: 2, # 'C'
- 39: 0, # 'D'
- 29: 0, # 'E'
- 52: 2, # 'F'
- 36: 0, # 'G'
- 45: 0, # 'H'
- 53: 0, # 'I'
- 60: 0, # 'J'
- 16: 1, # 'K'
- 49: 0, # 'L'
- 20: 0, # 'M'
- 46: 0, # 'N'
- 42: 0, # 'O'
- 48: 1, # 'P'
- 44: 0, # 'R'
- 35: 1, # 'S'
- 31: 0, # 'T'
- 51: 0, # 'U'
- 38: 1, # 'V'
- 62: 0, # 'W'
- 43: 1, # 'Y'
- 56: 0, # 'Z'
- 1: 2, # 'a'
- 21: 0, # 'b'
- 28: 2, # 'c'
- 12: 0, # 'd'
- 2: 3, # 'e'
- 18: 0, # 'f'
- 27: 0, # 'g'
- 25: 0, # 'h'
- 3: 0, # 'i'
- 24: 0, # 'j'
- 10: 0, # 'k'
- 5: 0, # 'l'
- 13: 1, # 'm'
- 4: 1, # 'n'
- 15: 0, # 'o'
- 26: 0, # 'p'
- 7: 0, # 'r'
- 8: 0, # 's'
- 9: 0, # 't'
- 14: 2, # 'u'
- 32: 0, # 'v'
- 57: 0, # 'w'
- 58: 0, # 'x'
- 11: 0, # 'y'
- 22: 1, # 'z'
- 63: 0, # '·'
- 54: 0, # 'Ç'
- 50: 1, # 'Ö'
- 55: 0, # 'Ü'
- 59: 0, # 'â'
- 33: 0, # 'ç'
- 61: 0, # 'î'
- 34: 1, # 'ö'
- 17: 0, # 'ü'
- 30: 0, # 'ğ'
- 41: 0, # 'İ'
- 6: 0, # 'ı'
- 40: 1, # 'Ş'
- 19: 1, # 'ş'
- },
- 47: { # 'C'
- 23: 0, # 'A'
- 37: 0, # 'B'
- 47: 0, # 'C'
- 39: 0, # 'D'
- 29: 0, # 'E'
- 52: 1, # 'F'
- 36: 0, # 'G'
- 45: 0, # 'H'
- 53: 0, # 'I'
- 60: 0, # 'J'
- 16: 0, # 'K'
- 49: 1, # 'L'
- 20: 0, # 'M'
- 46: 1, # 'N'
- 42: 0, # 'O'
- 48: 1, # 'P'
- 44: 1, # 'R'
- 35: 0, # 'S'
- 31: 0, # 'T'
- 51: 0, # 'U'
- 38: 1, # 'V'
- 62: 0, # 'W'
- 43: 1, # 'Y'
- 56: 0, # 'Z'
- 1: 3, # 'a'
- 21: 0, # 'b'
- 28: 2, # 'c'
- 12: 0, # 'd'
- 2: 3, # 'e'
- 18: 0, # 'f'
- 27: 0, # 'g'
- 25: 0, # 'h'
- 3: 0, # 'i'
- 24: 2, # 'j'
- 10: 1, # 'k'
- 5: 2, # 'l'
- 13: 2, # 'm'
- 4: 2, # 'n'
- 15: 1, # 'o'
- 26: 0, # 'p'
- 7: 2, # 'r'
- 8: 0, # 's'
- 9: 0, # 't'
- 14: 3, # 'u'
- 32: 0, # 'v'
- 57: 0, # 'w'
- 58: 0, # 'x'
- 11: 0, # 'y'
- 22: 2, # 'z'
- 63: 0, # '·'
- 54: 0, # 'Ç'
- 50: 1, # 'Ö'
- 55: 0, # 'Ü'
- 59: 0, # 'â'
- 33: 1, # 'ç'
- 61: 0, # 'î'
- 34: 1, # 'ö'
- 17: 0, # 'ü'
- 30: 0, # 'ğ'
- 41: 1, # 'İ'
- 6: 3, # 'ı'
- 40: 0, # 'Ş'
- 19: 0, # 'ş'
- },
- 39: { # 'D'
- 23: 0, # 'A'
- 37: 0, # 'B'
- 47: 0, # 'C'
- 39: 0, # 'D'
- 29: 0, # 'E'
- 52: 1, # 'F'
- 36: 0, # 'G'
- 45: 0, # 'H'
- 53: 0, # 'I'
- 60: 0, # 'J'
- 16: 1, # 'K'
- 49: 0, # 'L'
- 20: 0, # 'M'
- 46: 0, # 'N'
- 42: 0, # 'O'
- 48: 1, # 'P'
- 44: 0, # 'R'
- 35: 0, # 'S'
- 31: 0, # 'T'
- 51: 0, # 'U'
- 38: 0, # 'V'
- 62: 0, # 'W'
- 43: 0, # 'Y'
- 56: 0, # 'Z'
- 1: 2, # 'a'
- 21: 0, # 'b'
- 28: 2, # 'c'
- 12: 0, # 'd'
- 2: 2, # 'e'
- 18: 0, # 'f'
- 27: 0, # 'g'
- 25: 0, # 'h'
- 3: 0, # 'i'
- 24: 0, # 'j'
- 10: 0, # 'k'
- 5: 1, # 'l'
- 13: 3, # 'm'
- 4: 0, # 'n'
- 15: 1, # 'o'
- 26: 0, # 'p'
- 7: 0, # 'r'
- 8: 0, # 's'
- 9: 0, # 't'
- 14: 1, # 'u'
- 32: 0, # 'v'
- 57: 0, # 'w'
- 58: 0, # 'x'
- 11: 0, # 'y'
- 22: 1, # 'z'
- 63: 0, # '·'
- 54: 1, # 'Ç'
- 50: 0, # 'Ö'
- 55: 0, # 'Ü'
- 59: 0, # 'â'
- 33: 1, # 'ç'
- 61: 0, # 'î'
- 34: 0, # 'ö'
- 17: 0, # 'ü'
- 30: 1, # 'ğ'
- 41: 0, # 'İ'
- 6: 1, # 'ı'
- 40: 1, # 'Ş'
- 19: 0, # 'ş'
- },
- 29: { # 'E'
- 23: 0, # 'A'
- 37: 0, # 'B'
- 47: 0, # 'C'
- 39: 0, # 'D'
- 29: 1, # 'E'
- 52: 0, # 'F'
- 36: 0, # 'G'
- 45: 0, # 'H'
- 53: 0, # 'I'
- 60: 0, # 'J'
- 16: 3, # 'K'
- 49: 0, # 'L'
- 20: 1, # 'M'
- 46: 0, # 'N'
- 42: 0, # 'O'
- 48: 0, # 'P'
- 44: 0, # 'R'
- 35: 0, # 'S'
- 31: 0, # 'T'
- 51: 0, # 'U'
- 38: 0, # 'V'
- 62: 0, # 'W'
- 43: 0, # 'Y'
- 56: 0, # 'Z'
- 1: 3, # 'a'
- 21: 0, # 'b'
- 28: 0, # 'c'
- 12: 2, # 'd'
- 2: 3, # 'e'
- 18: 0, # 'f'
- 27: 1, # 'g'
- 25: 0, # 'h'
- 3: 1, # 'i'
- 24: 1, # 'j'
- 10: 0, # 'k'
- 5: 3, # 'l'
- 13: 3, # 'm'
- 4: 3, # 'n'
- 15: 0, # 'o'
- 26: 0, # 'p'
- 7: 0, # 'r'
- 8: 1, # 's'
- 9: 1, # 't'
- 14: 1, # 'u'
- 32: 1, # 'v'
- 57: 0, # 'w'
- 58: 0, # 'x'
- 11: 2, # 'y'
- 22: 0, # 'z'
- 63: 0, # '·'
- 54: 0, # 'Ç'
- 50: 0, # 'Ö'
- 55: 0, # 'Ü'
- 59: 0, # 'â'
- 33: 0, # 'ç'
- 61: 0, # 'î'
- 34: 0, # 'ö'
- 17: 0, # 'ü'
- 30: 0, # 'ğ'
- 41: 0, # 'İ'
- 6: 3, # 'ı'
- 40: 0, # 'Ş'
- 19: 0, # 'ş'
- },
- 52: { # 'F'
- 23: 0, # 'A'
- 37: 1, # 'B'
- 47: 1, # 'C'
- 39: 1, # 'D'
- 29: 1, # 'E'
- 52: 2, # 'F'
- 36: 0, # 'G'
- 45: 2, # 'H'
- 53: 1, # 'I'
- 60: 0, # 'J'
- 16: 0, # 'K'
- 49: 0, # 'L'
- 20: 1, # 'M'
- 46: 1, # 'N'
- 42: 1, # 'O'
- 48: 2, # 'P'
- 44: 1, # 'R'
- 35: 1, # 'S'
- 31: 1, # 'T'
- 51: 1, # 'U'
- 38: 1, # 'V'
- 62: 0, # 'W'
- 43: 2, # 'Y'
- 56: 0, # 'Z'
- 1: 0, # 'a'
- 21: 1, # 'b'
- 28: 1, # 'c'
- 12: 1, # 'd'
- 2: 0, # 'e'
- 18: 1, # 'f'
- 27: 0, # 'g'
- 25: 0, # 'h'
- 3: 2, # 'i'
- 24: 1, # 'j'
- 10: 0, # 'k'
- 5: 0, # 'l'
- 13: 1, # 'm'
- 4: 2, # 'n'
- 15: 1, # 'o'
- 26: 0, # 'p'
- 7: 2, # 'r'
- 8: 1, # 's'
- 9: 1, # 't'
- 14: 1, # 'u'
- 32: 0, # 'v'
- 57: 0, # 'w'
- 58: 0, # 'x'
- 11: 1, # 'y'
- 22: 1, # 'z'
- 63: 0, # '·'
- 54: 0, # 'Ç'
- 50: 1, # 'Ö'
- 55: 2, # 'Ü'
- 59: 0, # 'â'
- 33: 0, # 'ç'
- 61: 0, # 'î'
- 34: 2, # 'ö'
- 17: 0, # 'ü'
- 30: 1, # 'ğ'
- 41: 1, # 'İ'
- 6: 2, # 'ı'
- 40: 0, # 'Ş'
- 19: 2, # 'ş'
- },
- 36: { # 'G'
- 23: 1, # 'A'
- 37: 0, # 'B'
- 47: 1, # 'C'
- 39: 0, # 'D'
- 29: 0, # 'E'
- 52: 1, # 'F'
- 36: 2, # 'G'
- 45: 0, # 'H'
- 53: 0, # 'I'
- 60: 0, # 'J'
- 16: 2, # 'K'
- 49: 0, # 'L'
- 20: 0, # 'M'
- 46: 2, # 'N'
- 42: 1, # 'O'
- 48: 1, # 'P'
- 44: 1, # 'R'
- 35: 1, # 'S'
- 31: 0, # 'T'
- 51: 1, # 'U'
- 38: 2, # 'V'
- 62: 0, # 'W'
- 43: 0, # 'Y'
- 56: 0, # 'Z'
- 1: 3, # 'a'
- 21: 0, # 'b'
- 28: 1, # 'c'
- 12: 0, # 'd'
- 2: 3, # 'e'
- 18: 0, # 'f'
- 27: 0, # 'g'
- 25: 0, # 'h'
- 3: 0, # 'i'
- 24: 1, # 'j'
- 10: 1, # 'k'
- 5: 0, # 'l'
- 13: 3, # 'm'
- 4: 2, # 'n'
- 15: 0, # 'o'
- 26: 1, # 'p'
- 7: 0, # 'r'
- 8: 1, # 's'
- 9: 1, # 't'
- 14: 3, # 'u'
- 32: 0, # 'v'
- 57: 0, # 'w'
- 58: 1, # 'x'
- 11: 0, # 'y'
- 22: 2, # 'z'
- 63: 0, # '·'
- 54: 1, # 'Ç'
- 50: 2, # 'Ö'
- 55: 0, # 'Ü'
- 59: 1, # 'â'
- 33: 2, # 'ç'
- 61: 0, # 'î'
- 34: 0, # 'ö'
- 17: 0, # 'ü'
- 30: 1, # 'ğ'
- 41: 1, # 'İ'
- 6: 2, # 'ı'
- 40: 2, # 'Ş'
- 19: 1, # 'ş'
- },
- 45: { # 'H'
- 23: 0, # 'A'
- 37: 1, # 'B'
- 47: 0, # 'C'
- 39: 0, # 'D'
- 29: 0, # 'E'
- 52: 2, # 'F'
- 36: 2, # 'G'
- 45: 1, # 'H'
- 53: 1, # 'I'
- 60: 0, # 'J'
- 16: 2, # 'K'
- 49: 1, # 'L'
- 20: 0, # 'M'
- 46: 1, # 'N'
- 42: 1, # 'O'
- 48: 1, # 'P'
- 44: 0, # 'R'
- 35: 2, # 'S'
- 31: 0, # 'T'
- 51: 1, # 'U'
- 38: 2, # 'V'
- 62: 0, # 'W'
- 43: 0, # 'Y'
- 56: 0, # 'Z'
- 1: 3, # 'a'
- 21: 0, # 'b'
- 28: 2, # 'c'
- 12: 0, # 'd'
- 2: 3, # 'e'
- 18: 0, # 'f'
- 27: 0, # 'g'
- 25: 0, # 'h'
- 3: 2, # 'i'
- 24: 0, # 'j'
- 10: 1, # 'k'
- 5: 0, # 'l'
- 13: 2, # 'm'
- 4: 0, # 'n'
- 15: 1, # 'o'
- 26: 1, # 'p'
- 7: 1, # 'r'
- 8: 0, # 's'
- 9: 0, # 't'
- 14: 3, # 'u'
- 32: 0, # 'v'
- 57: 0, # 'w'
- 58: 0, # 'x'
- 11: 0, # 'y'
- 22: 2, # 'z'
- 63: 0, # '·'
- 54: 1, # 'Ç'
- 50: 1, # 'Ö'
- 55: 0, # 'Ü'
- 59: 0, # 'â'
- 33: 1, # 'ç'
- 61: 0, # 'î'
- 34: 1, # 'ö'
- 17: 0, # 'ü'
- 30: 2, # 'ğ'
- 41: 1, # 'İ'
- 6: 0, # 'ı'
- 40: 2, # 'Ş'
- 19: 1, # 'ş'
- },
- 53: { # 'I'
- 23: 0, # 'A'
- 37: 0, # 'B'
- 47: 0, # 'C'
- 39: 0, # 'D'
- 29: 0, # 'E'
- 52: 1, # 'F'
- 36: 0, # 'G'
- 45: 0, # 'H'
- 53: 0, # 'I'
- 60: 0, # 'J'
- 16: 2, # 'K'
- 49: 0, # 'L'
- 20: 0, # 'M'
- 46: 0, # 'N'
- 42: 0, # 'O'
- 48: 1, # 'P'
- 44: 0, # 'R'
- 35: 0, # 'S'
- 31: 0, # 'T'
- 51: 0, # 'U'
- 38: 0, # 'V'
- 62: 0, # 'W'
- 43: 0, # 'Y'
- 56: 0, # 'Z'
- 1: 2, # 'a'
- 21: 0, # 'b'
- 28: 2, # 'c'
- 12: 0, # 'd'
- 2: 2, # 'e'
- 18: 0, # 'f'
- 27: 0, # 'g'
- 25: 0, # 'h'
- 3: 0, # 'i'
- 24: 0, # 'j'
- 10: 0, # 'k'
- 5: 2, # 'l'
- 13: 2, # 'm'
- 4: 0, # 'n'
- 15: 0, # 'o'
- 26: 0, # 'p'
- 7: 0, # 'r'
- 8: 0, # 's'
- 9: 0, # 't'
- 14: 2, # 'u'
- 32: 0, # 'v'
- 57: 0, # 'w'
- 58: 0, # 'x'
- 11: 0, # 'y'
- 22: 2, # 'z'
- 63: 0, # '·'
- 54: 1, # 'Ç'
- 50: 0, # 'Ö'
- 55: 0, # 'Ü'
- 59: 0, # 'â'
- 33: 2, # 'ç'
- 61: 0, # 'î'
- 34: 1, # 'ö'
- 17: 0, # 'ü'
- 30: 0, # 'ğ'
- 41: 0, # 'İ'
- 6: 0, # 'ı'
- 40: 1, # 'Ş'
- 19: 1, # 'ş'
- },
- 60: { # 'J'
- 23: 0, # 'A'
- 37: 0, # 'B'
- 47: 0, # 'C'
- 39: 0, # 'D'
- 29: 0, # 'E'
- 52: 0, # 'F'
- 36: 0, # 'G'
- 45: 0, # 'H'
- 53: 0, # 'I'
- 60: 0, # 'J'
- 16: 0, # 'K'
- 49: 0, # 'L'
- 20: 1, # 'M'
- 46: 0, # 'N'
- 42: 0, # 'O'
- 48: 0, # 'P'
- 44: 0, # 'R'
- 35: 0, # 'S'
- 31: 0, # 'T'
- 51: 0, # 'U'
- 38: 0, # 'V'
- 62: 0, # 'W'
- 43: 0, # 'Y'
- 56: 0, # 'Z'
- 1: 0, # 'a'
- 21: 1, # 'b'
- 28: 0, # 'c'
- 12: 1, # 'd'
- 2: 0, # 'e'
- 18: 0, # 'f'
- 27: 0, # 'g'
- 25: 0, # 'h'
- 3: 1, # 'i'
- 24: 0, # 'j'
- 10: 0, # 'k'
- 5: 0, # 'l'
- 13: 0, # 'm'
- 4: 1, # 'n'
- 15: 0, # 'o'
- 26: 0, # 'p'
- 7: 0, # 'r'
- 8: 1, # 's'
- 9: 0, # 't'
- 14: 0, # 'u'
- 32: 0, # 'v'
- 57: 0, # 'w'
- 58: 0, # 'x'
- 11: 0, # 'y'
- 22: 0, # 'z'
- 63: 0, # '·'
- 54: 0, # 'Ç'
- 50: 0, # 'Ö'
- 55: 0, # 'Ü'
- 59: 0, # 'â'
- 33: 0, # 'ç'
- 61: 0, # 'î'
- 34: 0, # 'ö'
- 17: 0, # 'ü'
- 30: 0, # 'ğ'
- 41: 0, # 'İ'
- 6: 0, # 'ı'
- 40: 0, # 'Ş'
- 19: 0, # 'ş'
- },
- 16: { # 'K'
- 23: 0, # 'A'
- 37: 0, # 'B'
- 47: 0, # 'C'
- 39: 0, # 'D'
- 29: 3, # 'E'
- 52: 0, # 'F'
- 36: 0, # 'G'
- 45: 0, # 'H'
- 53: 0, # 'I'
- 60: 0, # 'J'
- 16: 0, # 'K'
- 49: 0, # 'L'
- 20: 2, # 'M'
- 46: 0, # 'N'
- 42: 0, # 'O'
- 48: 0, # 'P'
- 44: 0, # 'R'
- 35: 0, # 'S'
- 31: 2, # 'T'
- 51: 0, # 'U'
- 38: 0, # 'V'
- 62: 0, # 'W'
- 43: 0, # 'Y'
- 56: 0, # 'Z'
- 1: 2, # 'a'
- 21: 3, # 'b'
- 28: 0, # 'c'
- 12: 3, # 'd'
- 2: 1, # 'e'
- 18: 3, # 'f'
- 27: 3, # 'g'
- 25: 3, # 'h'
- 3: 3, # 'i'
- 24: 2, # 'j'
- 10: 3, # 'k'
- 5: 0, # 'l'
- 13: 0, # 'm'
- 4: 3, # 'n'
- 15: 0, # 'o'
- 26: 1, # 'p'
- 7: 3, # 'r'
- 8: 3, # 's'
- 9: 3, # 't'
- 14: 0, # 'u'
- 32: 3, # 'v'
- 57: 0, # 'w'
- 58: 0, # 'x'
- 11: 2, # 'y'
- 22: 1, # 'z'
- 63: 0, # '·'
- 54: 0, # 'Ç'
- 50: 0, # 'Ö'
- 55: 0, # 'Ü'
- 59: 0, # 'â'
- 33: 0, # 'ç'
- 61: 0, # 'î'
- 34: 0, # 'ö'
- 17: 2, # 'ü'
- 30: 0, # 'ğ'
- 41: 1, # 'İ'
- 6: 3, # 'ı'
- 40: 0, # 'Ş'
- 19: 0, # 'ş'
- },
- 49: { # 'L'
- 23: 0, # 'A'
- 37: 0, # 'B'
- 47: 0, # 'C'
- 39: 0, # 'D'
- 29: 2, # 'E'
- 52: 0, # 'F'
- 36: 1, # 'G'
- 45: 1, # 'H'
- 53: 0, # 'I'
- 60: 0, # 'J'
- 16: 0, # 'K'
- 49: 0, # 'L'
- 20: 1, # 'M'
- 46: 0, # 'N'
- 42: 2, # 'O'
- 48: 0, # 'P'
- 44: 0, # 'R'
- 35: 0, # 'S'
- 31: 0, # 'T'
- 51: 0, # 'U'
- 38: 0, # 'V'
- 62: 0, # 'W'
- 43: 1, # 'Y'
- 56: 0, # 'Z'
- 1: 0, # 'a'
- 21: 3, # 'b'
- 28: 0, # 'c'
- 12: 2, # 'd'
- 2: 0, # 'e'
- 18: 0, # 'f'
- 27: 0, # 'g'
- 25: 0, # 'h'
- 3: 2, # 'i'
- 24: 0, # 'j'
- 10: 1, # 'k'
- 5: 0, # 'l'
- 13: 0, # 'm'
- 4: 2, # 'n'
- 15: 1, # 'o'
- 26: 1, # 'p'
- 7: 1, # 'r'
- 8: 1, # 's'
- 9: 1, # 't'
- 14: 0, # 'u'
- 32: 0, # 'v'
- 57: 0, # 'w'
- 58: 0, # 'x'
- 11: 2, # 'y'
- 22: 0, # 'z'
- 63: 0, # '·'
- 54: 0, # 'Ç'
- 50: 0, # 'Ö'
- 55: 2, # 'Ü'
- 59: 0, # 'â'
- 33: 0, # 'ç'
- 61: 0, # 'î'
- 34: 1, # 'ö'
- 17: 1, # 'ü'
- 30: 1, # 'ğ'
- 41: 0, # 'İ'
- 6: 2, # 'ı'
- 40: 0, # 'Ş'
- 19: 0, # 'ş'
- },
- 20: { # 'M'
- 23: 1, # 'A'
- 37: 0, # 'B'
- 47: 0, # 'C'
- 39: 0, # 'D'
- 29: 0, # 'E'
- 52: 0, # 'F'
- 36: 0, # 'G'
- 45: 0, # 'H'
- 53: 0, # 'I'
- 60: 1, # 'J'
- 16: 3, # 'K'
- 49: 0, # 'L'
- 20: 2, # 'M'
- 46: 0, # 'N'
- 42: 0, # 'O'
- 48: 0, # 'P'
- 44: 0, # 'R'
- 35: 0, # 'S'
- 31: 1, # 'T'
- 51: 0, # 'U'
- 38: 0, # 'V'
- 62: 0, # 'W'
- 43: 0, # 'Y'
- 56: 0, # 'Z'
- 1: 3, # 'a'
- 21: 2, # 'b'
- 28: 0, # 'c'
- 12: 3, # 'd'
- 2: 3, # 'e'
- 18: 0, # 'f'
- 27: 1, # 'g'
- 25: 1, # 'h'
- 3: 2, # 'i'
- 24: 2, # 'j'
- 10: 2, # 'k'
- 5: 2, # 'l'
- 13: 3, # 'm'
- 4: 3, # 'n'
- 15: 0, # 'o'
- 26: 1, # 'p'
- 7: 3, # 'r'
- 8: 0, # 's'
- 9: 2, # 't'
- 14: 3, # 'u'
- 32: 0, # 'v'
- 57: 0, # 'w'
- 58: 0, # 'x'
- 11: 2, # 'y'
- 22: 0, # 'z'
- 63: 0, # '·'
- 54: 0, # 'Ç'
- 50: 0, # 'Ö'
- 55: 0, # 'Ü'
- 59: 0, # 'â'
- 33: 3, # 'ç'
- 61: 0, # 'î'
- 34: 0, # 'ö'
- 17: 0, # 'ü'
- 30: 0, # 'ğ'
- 41: 0, # 'İ'
- 6: 3, # 'ı'
- 40: 0, # 'Ş'
- 19: 0, # 'ş'
- },
- 46: { # 'N'
- 23: 0, # 'A'
- 37: 1, # 'B'
- 47: 0, # 'C'
- 39: 0, # 'D'
- 29: 0, # 'E'
- 52: 1, # 'F'
- 36: 1, # 'G'
- 45: 1, # 'H'
- 53: 0, # 'I'
- 60: 0, # 'J'
- 16: 2, # 'K'
- 49: 0, # 'L'
- 20: 0, # 'M'
- 46: 1, # 'N'
- 42: 0, # 'O'
- 48: 0, # 'P'
- 44: 1, # 'R'
- 35: 1, # 'S'
- 31: 0, # 'T'
- 51: 1, # 'U'
- 38: 2, # 'V'
- 62: 0, # 'W'
- 43: 1, # 'Y'
- 56: 0, # 'Z'
- 1: 3, # 'a'
- 21: 0, # 'b'
- 28: 2, # 'c'
- 12: 0, # 'd'
- 2: 3, # 'e'
- 18: 0, # 'f'
- 27: 1, # 'g'
- 25: 0, # 'h'
- 3: 0, # 'i'
- 24: 2, # 'j'
- 10: 1, # 'k'
- 5: 1, # 'l'
- 13: 3, # 'm'
- 4: 2, # 'n'
- 15: 1, # 'o'
- 26: 1, # 'p'
- 7: 1, # 'r'
- 8: 0, # 's'
- 9: 0, # 't'
- 14: 3, # 'u'
- 32: 0, # 'v'
- 57: 0, # 'w'
- 58: 1, # 'x'
- 11: 1, # 'y'
- 22: 2, # 'z'
- 63: 0, # '·'
- 54: 1, # 'Ç'
- 50: 1, # 'Ö'
- 55: 0, # 'Ü'
- 59: 0, # 'â'
- 33: 0, # 'ç'
- 61: 0, # 'î'
- 34: 1, # 'ö'
- 17: 0, # 'ü'
- 30: 0, # 'ğ'
- 41: 1, # 'İ'
- 6: 2, # 'ı'
- 40: 1, # 'Ş'
- 19: 1, # 'ş'
- },
- 42: { # 'O'
- 23: 0, # 'A'
- 37: 0, # 'B'
- 47: 0, # 'C'
- 39: 0, # 'D'
- 29: 0, # 'E'
- 52: 1, # 'F'
- 36: 0, # 'G'
- 45: 1, # 'H'
- 53: 0, # 'I'
- 60: 0, # 'J'
- 16: 2, # 'K'
- 49: 1, # 'L'
- 20: 0, # 'M'
- 46: 0, # 'N'
- 42: 0, # 'O'
- 48: 2, # 'P'
- 44: 1, # 'R'
- 35: 1, # 'S'
- 31: 0, # 'T'
- 51: 1, # 'U'
- 38: 1, # 'V'
- 62: 0, # 'W'
- 43: 0, # 'Y'
- 56: 0, # 'Z'
- 1: 3, # 'a'
- 21: 0, # 'b'
- 28: 2, # 'c'
- 12: 0, # 'd'
- 2: 2, # 'e'
- 18: 0, # 'f'
- 27: 0, # 'g'
- 25: 0, # 'h'
- 3: 0, # 'i'
- 24: 0, # 'j'
- 10: 0, # 'k'
- 5: 3, # 'l'
- 13: 3, # 'm'
- 4: 0, # 'n'
- 15: 1, # 'o'
- 26: 0, # 'p'
- 7: 0, # 'r'
- 8: 0, # 's'
- 9: 0, # 't'
- 14: 2, # 'u'
- 32: 0, # 'v'
- 57: 0, # 'w'
- 58: 0, # 'x'
- 11: 0, # 'y'
- 22: 2, # 'z'
- 63: 0, # '·'
- 54: 2, # 'Ç'
- 50: 1, # 'Ö'
- 55: 0, # 'Ü'
- 59: 0, # 'â'
- 33: 2, # 'ç'
- 61: 0, # 'î'
- 34: 1, # 'ö'
- 17: 0, # 'ü'
- 30: 1, # 'ğ'
- 41: 2, # 'İ'
- 6: 1, # 'ı'
- 40: 1, # 'Ş'
- 19: 1, # 'ş'
- },
- 48: { # 'P'
- 23: 0, # 'A'
- 37: 0, # 'B'
- 47: 2, # 'C'
- 39: 0, # 'D'
- 29: 0, # 'E'
- 52: 2, # 'F'
- 36: 1, # 'G'
- 45: 1, # 'H'
- 53: 0, # 'I'
- 60: 0, # 'J'
- 16: 2, # 'K'
- 49: 0, # 'L'
- 20: 0, # 'M'
- 46: 1, # 'N'
- 42: 1, # 'O'
- 48: 1, # 'P'
- 44: 0, # 'R'
- 35: 1, # 'S'
- 31: 0, # 'T'
- 51: 0, # 'U'
- 38: 1, # 'V'
- 62: 0, # 'W'
- 43: 0, # 'Y'
- 56: 0, # 'Z'
- 1: 2, # 'a'
- 21: 0, # 'b'
- 28: 2, # 'c'
- 12: 0, # 'd'
- 2: 3, # 'e'
- 18: 0, # 'f'
- 27: 0, # 'g'
- 25: 0, # 'h'
- 3: 0, # 'i'
- 24: 0, # 'j'
- 10: 1, # 'k'
- 5: 0, # 'l'
- 13: 2, # 'm'
- 4: 0, # 'n'
- 15: 2, # 'o'
- 26: 0, # 'p'
- 7: 0, # 'r'
- 8: 0, # 's'
- 9: 0, # 't'
- 14: 2, # 'u'
- 32: 0, # 'v'
- 57: 0, # 'w'
- 58: 2, # 'x'
- 11: 0, # 'y'
- 22: 2, # 'z'
- 63: 0, # '·'
- 54: 1, # 'Ç'
- 50: 2, # 'Ö'
- 55: 0, # 'Ü'
- 59: 0, # 'â'
- 33: 0, # 'ç'
- 61: 0, # 'î'
- 34: 2, # 'ö'
- 17: 0, # 'ü'
- 30: 1, # 'ğ'
- 41: 1, # 'İ'
- 6: 0, # 'ı'
- 40: 2, # 'Ş'
- 19: 1, # 'ş'
- },
- 44: { # 'R'
- 23: 0, # 'A'
- 37: 0, # 'B'
- 47: 1, # 'C'
- 39: 0, # 'D'
- 29: 0, # 'E'
- 52: 1, # 'F'
- 36: 0, # 'G'
- 45: 0, # 'H'
- 53: 0, # 'I'
- 60: 0, # 'J'
- 16: 3, # 'K'
- 49: 0, # 'L'
- 20: 0, # 'M'
- 46: 0, # 'N'
- 42: 0, # 'O'
- 48: 1, # 'P'
- 44: 0, # 'R'
- 35: 0, # 'S'
- 31: 0, # 'T'
- 51: 0, # 'U'
- 38: 0, # 'V'
- 62: 0, # 'W'
- 43: 1, # 'Y'
- 56: 0, # 'Z'
- 1: 3, # 'a'
- 21: 1, # 'b'
- 28: 1, # 'c'
- 12: 0, # 'd'
- 2: 2, # 'e'
- 18: 0, # 'f'
- 27: 0, # 'g'
- 25: 0, # 'h'
- 3: 0, # 'i'
- 24: 0, # 'j'
- 10: 1, # 'k'
- 5: 2, # 'l'
- 13: 2, # 'm'
- 4: 0, # 'n'
- 15: 1, # 'o'
- 26: 0, # 'p'
- 7: 0, # 'r'
- 8: 0, # 's'
- 9: 0, # 't'
- 14: 2, # 'u'
- 32: 0, # 'v'
- 57: 0, # 'w'
- 58: 0, # 'x'
- 11: 1, # 'y'
- 22: 2, # 'z'
- 63: 0, # '·'
- 54: 0, # 'Ç'
- 50: 1, # 'Ö'
- 55: 0, # 'Ü'
- 59: 0, # 'â'
- 33: 1, # 'ç'
- 61: 0, # 'î'
- 34: 1, # 'ö'
- 17: 1, # 'ü'
- 30: 1, # 'ğ'
- 41: 0, # 'İ'
- 6: 2, # 'ı'
- 40: 1, # 'Ş'
- 19: 1, # 'ş'
- },
- 35: { # 'S'
- 23: 0, # 'A'
- 37: 0, # 'B'
- 47: 1, # 'C'
- 39: 0, # 'D'
- 29: 0, # 'E'
- 52: 1, # 'F'
- 36: 1, # 'G'
- 45: 1, # 'H'
- 53: 0, # 'I'
- 60: 0, # 'J'
- 16: 3, # 'K'
- 49: 1, # 'L'
- 20: 1, # 'M'
- 46: 0, # 'N'
- 42: 0, # 'O'
- 48: 1, # 'P'
- 44: 0, # 'R'
- 35: 0, # 'S'
- 31: 0, # 'T'
- 51: 1, # 'U'
- 38: 1, # 'V'
- 62: 0, # 'W'
- 43: 1, # 'Y'
- 56: 0, # 'Z'
- 1: 3, # 'a'
- 21: 0, # 'b'
- 28: 2, # 'c'
- 12: 0, # 'd'
- 2: 3, # 'e'
- 18: 0, # 'f'
- 27: 0, # 'g'
- 25: 0, # 'h'
- 3: 0, # 'i'
- 24: 0, # 'j'
- 10: 1, # 'k'
- 5: 1, # 'l'
- 13: 2, # 'm'
- 4: 1, # 'n'
- 15: 0, # 'o'
- 26: 0, # 'p'
- 7: 0, # 'r'
- 8: 0, # 's'
- 9: 1, # 't'
- 14: 2, # 'u'
- 32: 0, # 'v'
- 57: 0, # 'w'
- 58: 0, # 'x'
- 11: 0, # 'y'
- 22: 1, # 'z'
- 63: 0, # '·'
- 54: 2, # 'Ç'
- 50: 2, # 'Ö'
- 55: 0, # 'Ü'
- 59: 0, # 'â'
- 33: 3, # 'ç'
- 61: 0, # 'î'
- 34: 1, # 'ö'
- 17: 0, # 'ü'
- 30: 0, # 'ğ'
- 41: 0, # 'İ'
- 6: 3, # 'ı'
- 40: 2, # 'Ş'
- 19: 1, # 'ş'
- },
- 31: { # 'T'
- 23: 0, # 'A'
- 37: 0, # 'B'
- 47: 0, # 'C'
- 39: 0, # 'D'
- 29: 0, # 'E'
- 52: 0, # 'F'
- 36: 0, # 'G'
- 45: 0, # 'H'
- 53: 0, # 'I'
- 60: 1, # 'J'
- 16: 2, # 'K'
- 49: 0, # 'L'
- 20: 1, # 'M'
- 46: 0, # 'N'
- 42: 0, # 'O'
- 48: 0, # 'P'
- 44: 0, # 'R'
- 35: 0, # 'S'
- 31: 2, # 'T'
- 51: 0, # 'U'
- 38: 0, # 'V'
- 62: 0, # 'W'
- 43: 0, # 'Y'
- 56: 0, # 'Z'
- 1: 3, # 'a'
- 21: 2, # 'b'
- 28: 0, # 'c'
- 12: 1, # 'd'
- 2: 3, # 'e'
- 18: 2, # 'f'
- 27: 2, # 'g'
- 25: 0, # 'h'
- 3: 1, # 'i'
- 24: 1, # 'j'
- 10: 2, # 'k'
- 5: 2, # 'l'
- 13: 3, # 'm'
- 4: 3, # 'n'
- 15: 0, # 'o'
- 26: 2, # 'p'
- 7: 2, # 'r'
- 8: 0, # 's'
- 9: 2, # 't'
- 14: 2, # 'u'
- 32: 1, # 'v'
- 57: 1, # 'w'
- 58: 1, # 'x'
- 11: 2, # 'y'
- 22: 0, # 'z'
- 63: 0, # '·'
- 54: 0, # 'Ç'
- 50: 0, # 'Ö'
- 55: 0, # 'Ü'
- 59: 0, # 'â'
- 33: 0, # 'ç'
- 61: 0, # 'î'
- 34: 0, # 'ö'
- 17: 1, # 'ü'
- 30: 0, # 'ğ'
- 41: 0, # 'İ'
- 6: 3, # 'ı'
- 40: 0, # 'Ş'
- 19: 0, # 'ş'
- },
- 51: { # 'U'
- 23: 0, # 'A'
- 37: 0, # 'B'
- 47: 0, # 'C'
- 39: 0, # 'D'
- 29: 0, # 'E'
- 52: 1, # 'F'
- 36: 1, # 'G'
- 45: 0, # 'H'
- 53: 0, # 'I'
- 60: 0, # 'J'
- 16: 1, # 'K'
- 49: 0, # 'L'
- 20: 0, # 'M'
- 46: 1, # 'N'
- 42: 0, # 'O'
- 48: 1, # 'P'
- 44: 0, # 'R'
- 35: 0, # 'S'
- 31: 0, # 'T'
- 51: 1, # 'U'
- 38: 1, # 'V'
- 62: 0, # 'W'
- 43: 0, # 'Y'
- 56: 0, # 'Z'
- 1: 3, # 'a'
- 21: 0, # 'b'
- 28: 1, # 'c'
- 12: 0, # 'd'
- 2: 3, # 'e'
- 18: 0, # 'f'
- 27: 2, # 'g'
- 25: 0, # 'h'
- 3: 0, # 'i'
- 24: 0, # 'j'
- 10: 1, # 'k'
- 5: 1, # 'l'
- 13: 3, # 'm'
- 4: 2, # 'n'
- 15: 0, # 'o'
- 26: 1, # 'p'
- 7: 0, # 'r'
- 8: 0, # 's'
- 9: 0, # 't'
- 14: 2, # 'u'
- 32: 0, # 'v'
- 57: 0, # 'w'
- 58: 0, # 'x'
- 11: 0, # 'y'
- 22: 2, # 'z'
- 63: 0, # '·'
- 54: 1, # 'Ç'
- 50: 1, # 'Ö'
- 55: 0, # 'Ü'
- 59: 0, # 'â'
- 33: 0, # 'ç'
- 61: 0, # 'î'
- 34: 0, # 'ö'
- 17: 0, # 'ü'
- 30: 1, # 'ğ'
- 41: 1, # 'İ'
- 6: 2, # 'ı'
- 40: 0, # 'Ş'
- 19: 1, # 'ş'
- },
- 38: { # 'V'
- 23: 1, # 'A'
- 37: 1, # 'B'
- 47: 1, # 'C'
- 39: 0, # 'D'
- 29: 0, # 'E'
- 52: 2, # 'F'
- 36: 0, # 'G'
- 45: 0, # 'H'
- 53: 0, # 'I'
- 60: 0, # 'J'
- 16: 3, # 'K'
- 49: 0, # 'L'
- 20: 3, # 'M'
- 46: 0, # 'N'
- 42: 0, # 'O'
- 48: 1, # 'P'
- 44: 1, # 'R'
- 35: 0, # 'S'
- 31: 0, # 'T'
- 51: 1, # 'U'
- 38: 1, # 'V'
- 62: 0, # 'W'
- 43: 0, # 'Y'
- 56: 0, # 'Z'
- 1: 3, # 'a'
- 21: 0, # 'b'
- 28: 2, # 'c'
- 12: 0, # 'd'
- 2: 3, # 'e'
- 18: 0, # 'f'
- 27: 0, # 'g'
- 25: 0, # 'h'
- 3: 0, # 'i'
- 24: 0, # 'j'
- 10: 0, # 'k'
- 5: 2, # 'l'
- 13: 2, # 'm'
- 4: 0, # 'n'
- 15: 2, # 'o'
- 26: 0, # 'p'
- 7: 0, # 'r'
- 8: 0, # 's'
- 9: 1, # 't'
- 14: 3, # 'u'
- 32: 0, # 'v'
- 57: 0, # 'w'
- 58: 0, # 'x'
- 11: 1, # 'y'
- 22: 2, # 'z'
- 63: 0, # '·'
- 54: 1, # 'Ç'
- 50: 1, # 'Ö'
- 55: 0, # 'Ü'
- 59: 1, # 'â'
- 33: 2, # 'ç'
- 61: 0, # 'î'
- 34: 1, # 'ö'
- 17: 0, # 'ü'
- 30: 1, # 'ğ'
- 41: 1, # 'İ'
- 6: 3, # 'ı'
- 40: 2, # 'Ş'
- 19: 1, # 'ş'
- },
- 62: { # 'W'
- 23: 0, # 'A'
- 37: 0, # 'B'
- 47: 0, # 'C'
- 39: 0, # 'D'
- 29: 0, # 'E'
- 52: 0, # 'F'
- 36: 0, # 'G'
- 45: 0, # 'H'
- 53: 0, # 'I'
- 60: 0, # 'J'
- 16: 0, # 'K'
- 49: 0, # 'L'
- 20: 0, # 'M'
- 46: 0, # 'N'
- 42: 0, # 'O'
- 48: 0, # 'P'
- 44: 0, # 'R'
- 35: 0, # 'S'
- 31: 0, # 'T'
- 51: 0, # 'U'
- 38: 0, # 'V'
- 62: 0, # 'W'
- 43: 0, # 'Y'
- 56: 0, # 'Z'
- 1: 0, # 'a'
- 21: 0, # 'b'
- 28: 0, # 'c'
- 12: 0, # 'd'
- 2: 0, # 'e'
- 18: 0, # 'f'
- 27: 0, # 'g'
- 25: 0, # 'h'
- 3: 0, # 'i'
- 24: 0, # 'j'
- 10: 0, # 'k'
- 5: 0, # 'l'
- 13: 0, # 'm'
- 4: 0, # 'n'
- 15: 0, # 'o'
- 26: 0, # 'p'
- 7: 0, # 'r'
- 8: 0, # 's'
- 9: 0, # 't'
- 14: 0, # 'u'
- 32: 0, # 'v'
- 57: 0, # 'w'
- 58: 0, # 'x'
- 11: 0, # 'y'
- 22: 0, # 'z'
- 63: 0, # '·'
- 54: 0, # 'Ç'
- 50: 0, # 'Ö'
- 55: 0, # 'Ü'
- 59: 0, # 'â'
- 33: 0, # 'ç'
- 61: 0, # 'î'
- 34: 0, # 'ö'
- 17: 0, # 'ü'
- 30: 0, # 'ğ'
- 41: 0, # 'İ'
- 6: 0, # 'ı'
- 40: 0, # 'Ş'
- 19: 0, # 'ş'
- },
- 43: { # 'Y'
- 23: 0, # 'A'
- 37: 0, # 'B'
- 47: 1, # 'C'
- 39: 0, # 'D'
- 29: 0, # 'E'
- 52: 2, # 'F'
- 36: 0, # 'G'
- 45: 1, # 'H'
- 53: 1, # 'I'
- 60: 0, # 'J'
- 16: 2, # 'K'
- 49: 0, # 'L'
- 20: 0, # 'M'
- 46: 2, # 'N'
- 42: 0, # 'O'
- 48: 2, # 'P'
- 44: 1, # 'R'
- 35: 1, # 'S'
- 31: 0, # 'T'
- 51: 1, # 'U'
- 38: 2, # 'V'
- 62: 0, # 'W'
- 43: 0, # 'Y'
- 56: 0, # 'Z'
- 1: 3, # 'a'
- 21: 0, # 'b'
- 28: 2, # 'c'
- 12: 0, # 'd'
- 2: 2, # 'e'
- 18: 0, # 'f'
- 27: 0, # 'g'
- 25: 0, # 'h'
- 3: 0, # 'i'
- 24: 1, # 'j'
- 10: 1, # 'k'
- 5: 1, # 'l'
- 13: 3, # 'm'
- 4: 0, # 'n'
- 15: 2, # 'o'
- 26: 0, # 'p'
- 7: 0, # 'r'
- 8: 0, # 's'
- 9: 0, # 't'
- 14: 3, # 'u'
- 32: 0, # 'v'
- 57: 0, # 'w'
- 58: 1, # 'x'
- 11: 0, # 'y'
- 22: 2, # 'z'
- 63: 0, # '·'
- 54: 1, # 'Ç'
- 50: 2, # 'Ö'
- 55: 1, # 'Ü'
- 59: 1, # 'â'
- 33: 0, # 'ç'
- 61: 0, # 'î'
- 34: 1, # 'ö'
- 17: 0, # 'ü'
- 30: 1, # 'ğ'
- 41: 1, # 'İ'
- 6: 0, # 'ı'
- 40: 2, # 'Ş'
- 19: 1, # 'ş'
- },
- 56: { # 'Z'
- 23: 0, # 'A'
- 37: 0, # 'B'
- 47: 0, # 'C'
- 39: 0, # 'D'
- 29: 0, # 'E'
- 52: 0, # 'F'
- 36: 0, # 'G'
- 45: 0, # 'H'
- 53: 0, # 'I'
- 60: 0, # 'J'
- 16: 0, # 'K'
- 49: 0, # 'L'
- 20: 0, # 'M'
- 46: 0, # 'N'
- 42: 0, # 'O'
- 48: 0, # 'P'
- 44: 0, # 'R'
- 35: 0, # 'S'
- 31: 0, # 'T'
- 51: 0, # 'U'
- 38: 0, # 'V'
- 62: 0, # 'W'
- 43: 0, # 'Y'
- 56: 2, # 'Z'
- 1: 2, # 'a'
- 21: 1, # 'b'
- 28: 0, # 'c'
- 12: 0, # 'd'
- 2: 2, # 'e'
- 18: 0, # 'f'
- 27: 0, # 'g'
- 25: 0, # 'h'
- 3: 2, # 'i'
- 24: 1, # 'j'
- 10: 0, # 'k'
- 5: 0, # 'l'
- 13: 1, # 'm'
- 4: 1, # 'n'
- 15: 0, # 'o'
- 26: 0, # 'p'
- 7: 1, # 'r'
- 8: 1, # 's'
- 9: 0, # 't'
- 14: 2, # 'u'
- 32: 0, # 'v'
- 57: 0, # 'w'
- 58: 0, # 'x'
- 11: 0, # 'y'
- 22: 0, # 'z'
- 63: 0, # '·'
- 54: 0, # 'Ç'
- 50: 0, # 'Ö'
- 55: 0, # 'Ü'
- 59: 0, # 'â'
- 33: 0, # 'ç'
- 61: 0, # 'î'
- 34: 0, # 'ö'
- 17: 1, # 'ü'
- 30: 0, # 'ğ'
- 41: 0, # 'İ'
- 6: 1, # 'ı'
- 40: 0, # 'Ş'
- 19: 0, # 'ş'
- },
- 1: { # 'a'
- 23: 3, # 'A'
- 37: 0, # 'B'
- 47: 1, # 'C'
- 39: 0, # 'D'
- 29: 3, # 'E'
- 52: 0, # 'F'
- 36: 1, # 'G'
- 45: 1, # 'H'
- 53: 0, # 'I'
- 60: 0, # 'J'
- 16: 0, # 'K'
- 49: 0, # 'L'
- 20: 3, # 'M'
- 46: 1, # 'N'
- 42: 0, # 'O'
- 48: 1, # 'P'
- 44: 0, # 'R'
- 35: 0, # 'S'
- 31: 3, # 'T'
- 51: 0, # 'U'
- 38: 1, # 'V'
- 62: 0, # 'W'
- 43: 0, # 'Y'
- 56: 2, # 'Z'
- 1: 2, # 'a'
- 21: 3, # 'b'
- 28: 0, # 'c'
- 12: 3, # 'd'
- 2: 2, # 'e'
- 18: 3, # 'f'
- 27: 3, # 'g'
- 25: 3, # 'h'
- 3: 3, # 'i'
- 24: 3, # 'j'
- 10: 3, # 'k'
- 5: 0, # 'l'
- 13: 2, # 'm'
- 4: 3, # 'n'
- 15: 1, # 'o'
- 26: 3, # 'p'
- 7: 3, # 'r'
- 8: 3, # 's'
- 9: 3, # 't'
- 14: 3, # 'u'
- 32: 3, # 'v'
- 57: 2, # 'w'
- 58: 0, # 'x'
- 11: 3, # 'y'
- 22: 0, # 'z'
- 63: 1, # '·'
- 54: 0, # 'Ç'
- 50: 0, # 'Ö'
- 55: 0, # 'Ü'
- 59: 0, # 'â'
- 33: 1, # 'ç'
- 61: 1, # 'î'
- 34: 1, # 'ö'
- 17: 3, # 'ü'
- 30: 0, # 'ğ'
- 41: 0, # 'İ'
- 6: 3, # 'ı'
- 40: 0, # 'Ş'
- 19: 1, # 'ş'
- },
- 21: { # 'b'
- 23: 0, # 'A'
- 37: 0, # 'B'
- 47: 0, # 'C'
- 39: 0, # 'D'
- 29: 0, # 'E'
- 52: 0, # 'F'
- 36: 1, # 'G'
- 45: 0, # 'H'
- 53: 0, # 'I'
- 60: 1, # 'J'
- 16: 2, # 'K'
- 49: 0, # 'L'
- 20: 2, # 'M'
- 46: 0, # 'N'
- 42: 0, # 'O'
- 48: 0, # 'P'
- 44: 0, # 'R'
- 35: 0, # 'S'
- 31: 1, # 'T'
- 51: 0, # 'U'
- 38: 0, # 'V'
- 62: 0, # 'W'
- 43: 1, # 'Y'
- 56: 0, # 'Z'
- 1: 3, # 'a'
- 21: 2, # 'b'
- 28: 0, # 'c'
- 12: 3, # 'd'
- 2: 3, # 'e'
- 18: 0, # 'f'
- 27: 3, # 'g'
- 25: 1, # 'h'
- 3: 3, # 'i'
- 24: 2, # 'j'
- 10: 3, # 'k'
- 5: 3, # 'l'
- 13: 3, # 'm'
- 4: 3, # 'n'
- 15: 0, # 'o'
- 26: 3, # 'p'
- 7: 1, # 'r'
- 8: 2, # 's'
- 9: 2, # 't'
- 14: 2, # 'u'
- 32: 1, # 'v'
- 57: 0, # 'w'
- 58: 1, # 'x'
- 11: 3, # 'y'
- 22: 0, # 'z'
- 63: 0, # '·'
- 54: 0, # 'Ç'
- 50: 0, # 'Ö'
- 55: 0, # 'Ü'
- 59: 0, # 'â'
- 33: 1, # 'ç'
- 61: 0, # 'î'
- 34: 0, # 'ö'
- 17: 0, # 'ü'
- 30: 1, # 'ğ'
- 41: 0, # 'İ'
- 6: 2, # 'ı'
- 40: 0, # 'Ş'
- 19: 0, # 'ş'
- },
- 28: { # 'c'
- 23: 0, # 'A'
- 37: 1, # 'B'
- 47: 1, # 'C'
- 39: 1, # 'D'
- 29: 2, # 'E'
- 52: 0, # 'F'
- 36: 2, # 'G'
- 45: 2, # 'H'
- 53: 1, # 'I'
- 60: 0, # 'J'
- 16: 0, # 'K'
- 49: 0, # 'L'
- 20: 2, # 'M'
- 46: 1, # 'N'
- 42: 1, # 'O'
- 48: 2, # 'P'
- 44: 1, # 'R'
- 35: 1, # 'S'
- 31: 2, # 'T'
- 51: 2, # 'U'
- 38: 2, # 'V'
- 62: 0, # 'W'
- 43: 3, # 'Y'
- 56: 0, # 'Z'
- 1: 1, # 'a'
- 21: 1, # 'b'
- 28: 2, # 'c'
- 12: 2, # 'd'
- 2: 1, # 'e'
- 18: 1, # 'f'
- 27: 2, # 'g'
- 25: 2, # 'h'
- 3: 3, # 'i'
- 24: 1, # 'j'
- 10: 3, # 'k'
- 5: 0, # 'l'
- 13: 2, # 'm'
- 4: 3, # 'n'
- 15: 2, # 'o'
- 26: 2, # 'p'
- 7: 3, # 'r'
- 8: 3, # 's'
- 9: 3, # 't'
- 14: 1, # 'u'
- 32: 0, # 'v'
- 57: 1, # 'w'
- 58: 0, # 'x'
- 11: 2, # 'y'
- 22: 1, # 'z'
- 63: 1, # '·'
- 54: 0, # 'Ç'
- 50: 0, # 'Ö'
- 55: 1, # 'Ü'
- 59: 0, # 'â'
- 33: 0, # 'ç'
- 61: 1, # 'î'
- 34: 2, # 'ö'
- 17: 2, # 'ü'
- 30: 2, # 'ğ'
- 41: 1, # 'İ'
- 6: 3, # 'ı'
- 40: 0, # 'Ş'
- 19: 2, # 'ş'
- },
- 12: { # 'd'
- 23: 1, # 'A'
- 37: 0, # 'B'
- 47: 0, # 'C'
- 39: 0, # 'D'
- 29: 0, # 'E'
- 52: 0, # 'F'
- 36: 0, # 'G'
- 45: 0, # 'H'
- 53: 0, # 'I'
- 60: 2, # 'J'
- 16: 3, # 'K'
- 49: 0, # 'L'
- 20: 3, # 'M'
- 46: 0, # 'N'
- 42: 0, # 'O'
- 48: 0, # 'P'
- 44: 0, # 'R'
- 35: 1, # 'S'
- 31: 1, # 'T'
- 51: 0, # 'U'
- 38: 0, # 'V'
- 62: 0, # 'W'
- 43: 0, # 'Y'
- 56: 0, # 'Z'
- 1: 3, # 'a'
- 21: 2, # 'b'
- 28: 1, # 'c'
- 12: 3, # 'd'
- 2: 3, # 'e'
- 18: 1, # 'f'
- 27: 3, # 'g'
- 25: 3, # 'h'
- 3: 2, # 'i'
- 24: 3, # 'j'
- 10: 2, # 'k'
- 5: 3, # 'l'
- 13: 3, # 'm'
- 4: 3, # 'n'
- 15: 1, # 'o'
- 26: 2, # 'p'
- 7: 3, # 'r'
- 8: 2, # 's'
- 9: 2, # 't'
- 14: 3, # 'u'
- 32: 1, # 'v'
- 57: 0, # 'w'
- 58: 1, # 'x'
- 11: 3, # 'y'
- 22: 1, # 'z'
- 63: 1, # '·'
- 54: 0, # 'Ç'
- 50: 0, # 'Ö'
- 55: 0, # 'Ü'
- 59: 0, # 'â'
- 33: 0, # 'ç'
- 61: 0, # 'î'
- 34: 0, # 'ö'
- 17: 1, # 'ü'
- 30: 0, # 'ğ'
- 41: 0, # 'İ'
- 6: 2, # 'ı'
- 40: 0, # 'Ş'
- 19: 0, # 'ş'
- },
- 2: { # 'e'
- 23: 2, # 'A'
- 37: 0, # 'B'
- 47: 2, # 'C'
- 39: 0, # 'D'
- 29: 3, # 'E'
- 52: 1, # 'F'
- 36: 0, # 'G'
- 45: 0, # 'H'
- 53: 0, # 'I'
- 60: 0, # 'J'
- 16: 1, # 'K'
- 49: 0, # 'L'
- 20: 3, # 'M'
- 46: 1, # 'N'
- 42: 0, # 'O'
- 48: 1, # 'P'
- 44: 1, # 'R'
- 35: 0, # 'S'
- 31: 3, # 'T'
- 51: 0, # 'U'
- 38: 1, # 'V'
- 62: 0, # 'W'
- 43: 1, # 'Y'
- 56: 0, # 'Z'
- 1: 3, # 'a'
- 21: 3, # 'b'
- 28: 0, # 'c'
- 12: 3, # 'd'
- 2: 2, # 'e'
- 18: 3, # 'f'
- 27: 3, # 'g'
- 25: 3, # 'h'
- 3: 3, # 'i'
- 24: 3, # 'j'
- 10: 3, # 'k'
- 5: 0, # 'l'
- 13: 2, # 'm'
- 4: 3, # 'n'
- 15: 1, # 'o'
- 26: 3, # 'p'
- 7: 3, # 'r'
- 8: 3, # 's'
- 9: 3, # 't'
- 14: 3, # 'u'
- 32: 3, # 'v'
- 57: 2, # 'w'
- 58: 0, # 'x'
- 11: 3, # 'y'
- 22: 1, # 'z'
- 63: 1, # '·'
- 54: 0, # 'Ç'
- 50: 0, # 'Ö'
- 55: 0, # 'Ü'
- 59: 0, # 'â'
- 33: 1, # 'ç'
- 61: 0, # 'î'
- 34: 1, # 'ö'
- 17: 3, # 'ü'
- 30: 0, # 'ğ'
- 41: 0, # 'İ'
- 6: 3, # 'ı'
- 40: 0, # 'Ş'
- 19: 0, # 'ş'
- },
- 18: { # 'f'
- 23: 0, # 'A'
- 37: 0, # 'B'
- 47: 0, # 'C'
- 39: 0, # 'D'
- 29: 0, # 'E'
- 52: 0, # 'F'
- 36: 0, # 'G'
- 45: 0, # 'H'
- 53: 0, # 'I'
- 60: 0, # 'J'
- 16: 2, # 'K'
- 49: 0, # 'L'
- 20: 2, # 'M'
- 46: 0, # 'N'
- 42: 0, # 'O'
- 48: 0, # 'P'
- 44: 0, # 'R'
- 35: 0, # 'S'
- 31: 2, # 'T'
- 51: 0, # 'U'
- 38: 0, # 'V'
- 62: 0, # 'W'
- 43: 0, # 'Y'
- 56: 0, # 'Z'
- 1: 3, # 'a'
- 21: 1, # 'b'
- 28: 0, # 'c'
- 12: 3, # 'd'
- 2: 3, # 'e'
- 18: 2, # 'f'
- 27: 1, # 'g'
- 25: 1, # 'h'
- 3: 1, # 'i'
- 24: 1, # 'j'
- 10: 1, # 'k'
- 5: 3, # 'l'
- 13: 3, # 'm'
- 4: 3, # 'n'
- 15: 0, # 'o'
- 26: 2, # 'p'
- 7: 1, # 'r'
- 8: 3, # 's'
- 9: 3, # 't'
- 14: 1, # 'u'
- 32: 2, # 'v'
- 57: 0, # 'w'
- 58: 0, # 'x'
- 11: 1, # 'y'
- 22: 0, # 'z'
- 63: 0, # '·'
- 54: 0, # 'Ç'
- 50: 0, # 'Ö'
- 55: 0, # 'Ü'
- 59: 0, # 'â'
- 33: 1, # 'ç'
- 61: 0, # 'î'
- 34: 0, # 'ö'
- 17: 1, # 'ü'
- 30: 0, # 'ğ'
- 41: 0, # 'İ'
- 6: 1, # 'ı'
- 40: 0, # 'Ş'
- 19: 0, # 'ş'
- },
- 27: { # 'g'
- 23: 0, # 'A'
- 37: 0, # 'B'
- 47: 0, # 'C'
- 39: 0, # 'D'
- 29: 0, # 'E'
- 52: 0, # 'F'
- 36: 0, # 'G'
- 45: 0, # 'H'
- 53: 0, # 'I'
- 60: 0, # 'J'
- 16: 3, # 'K'
- 49: 0, # 'L'
- 20: 0, # 'M'
- 46: 0, # 'N'
- 42: 0, # 'O'
- 48: 0, # 'P'
- 44: 0, # 'R'
- 35: 1, # 'S'
- 31: 1, # 'T'
- 51: 0, # 'U'
- 38: 2, # 'V'
- 62: 0, # 'W'
- 43: 0, # 'Y'
- 56: 0, # 'Z'
- 1: 3, # 'a'
- 21: 1, # 'b'
- 28: 0, # 'c'
- 12: 1, # 'd'
- 2: 3, # 'e'
- 18: 0, # 'f'
- 27: 2, # 'g'
- 25: 1, # 'h'
- 3: 2, # 'i'
- 24: 3, # 'j'
- 10: 2, # 'k'
- 5: 3, # 'l'
- 13: 3, # 'm'
- 4: 2, # 'n'
- 15: 0, # 'o'
- 26: 1, # 'p'
- 7: 2, # 'r'
- 8: 2, # 's'
- 9: 3, # 't'
- 14: 3, # 'u'
- 32: 1, # 'v'
- 57: 0, # 'w'
- 58: 0, # 'x'
- 11: 1, # 'y'
- 22: 0, # 'z'
- 63: 1, # '·'
- 54: 0, # 'Ç'
- 50: 0, # 'Ö'
- 55: 0, # 'Ü'
- 59: 0, # 'â'
- 33: 0, # 'ç'
- 61: 0, # 'î'
- 34: 0, # 'ö'
- 17: 0, # 'ü'
- 30: 0, # 'ğ'
- 41: 0, # 'İ'
- 6: 2, # 'ı'
- 40: 0, # 'Ş'
- 19: 0, # 'ş'
- },
- 25: { # 'h'
- 23: 0, # 'A'
- 37: 0, # 'B'
- 47: 0, # 'C'
- 39: 0, # 'D'
- 29: 0, # 'E'
- 52: 0, # 'F'
- 36: 0, # 'G'
- 45: 0, # 'H'
- 53: 0, # 'I'
- 60: 0, # 'J'
- 16: 2, # 'K'
- 49: 0, # 'L'
- 20: 0, # 'M'
- 46: 0, # 'N'
- 42: 0, # 'O'
- 48: 0, # 'P'
- 44: 0, # 'R'
- 35: 0, # 'S'
- 31: 0, # 'T'
- 51: 0, # 'U'
- 38: 0, # 'V'
- 62: 0, # 'W'
- 43: 0, # 'Y'
- 56: 0, # 'Z'
- 1: 3, # 'a'
- 21: 0, # 'b'
- 28: 0, # 'c'
- 12: 2, # 'd'
- 2: 3, # 'e'
- 18: 0, # 'f'
- 27: 1, # 'g'
- 25: 2, # 'h'
- 3: 2, # 'i'
- 24: 3, # 'j'
- 10: 3, # 'k'
- 5: 3, # 'l'
- 13: 3, # 'm'
- 4: 3, # 'n'
- 15: 1, # 'o'
- 26: 1, # 'p'
- 7: 3, # 'r'
- 8: 3, # 's'
- 9: 2, # 't'
- 14: 3, # 'u'
- 32: 2, # 'v'
- 57: 1, # 'w'
- 58: 0, # 'x'
- 11: 1, # 'y'
- 22: 0, # 'z'
- 63: 0, # '·'
- 54: 0, # 'Ç'
- 50: 0, # 'Ö'
- 55: 0, # 'Ü'
- 59: 0, # 'â'
- 33: 0, # 'ç'
- 61: 0, # 'î'
- 34: 0, # 'ö'
- 17: 0, # 'ü'
- 30: 0, # 'ğ'
- 41: 0, # 'İ'
- 6: 3, # 'ı'
- 40: 0, # 'Ş'
- 19: 0, # 'ş'
- },
- 3: { # 'i'
- 23: 2, # 'A'
- 37: 0, # 'B'
- 47: 0, # 'C'
- 39: 0, # 'D'
- 29: 0, # 'E'
- 52: 0, # 'F'
- 36: 0, # 'G'
- 45: 0, # 'H'
- 53: 0, # 'I'
- 60: 1, # 'J'
- 16: 3, # 'K'
- 49: 0, # 'L'
- 20: 3, # 'M'
- 46: 0, # 'N'
- 42: 1, # 'O'
- 48: 0, # 'P'
- 44: 0, # 'R'
- 35: 1, # 'S'
- 31: 2, # 'T'
- 51: 0, # 'U'
- 38: 1, # 'V'
- 62: 0, # 'W'
- 43: 0, # 'Y'
- 56: 0, # 'Z'
- 1: 3, # 'a'
- 21: 2, # 'b'
- 28: 0, # 'c'
- 12: 3, # 'd'
- 2: 3, # 'e'
- 18: 2, # 'f'
- 27: 3, # 'g'
- 25: 1, # 'h'
- 3: 3, # 'i'
- 24: 2, # 'j'
- 10: 3, # 'k'
- 5: 3, # 'l'
- 13: 3, # 'm'
- 4: 3, # 'n'
- 15: 1, # 'o'
- 26: 3, # 'p'
- 7: 3, # 'r'
- 8: 3, # 's'
- 9: 3, # 't'
- 14: 3, # 'u'
- 32: 2, # 'v'
- 57: 1, # 'w'
- 58: 1, # 'x'
- 11: 3, # 'y'
- 22: 1, # 'z'
- 63: 1, # '·'
- 54: 0, # 'Ç'
- 50: 0, # 'Ö'
- 55: 1, # 'Ü'
- 59: 0, # 'â'
- 33: 2, # 'ç'
- 61: 0, # 'î'
- 34: 0, # 'ö'
- 17: 3, # 'ü'
- 30: 0, # 'ğ'
- 41: 1, # 'İ'
- 6: 2, # 'ı'
- 40: 0, # 'Ş'
- 19: 0, # 'ş'
- },
- 24: { # 'j'
- 23: 0, # 'A'
- 37: 0, # 'B'
- 47: 0, # 'C'
- 39: 0, # 'D'
- 29: 0, # 'E'
- 52: 0, # 'F'
- 36: 0, # 'G'
- 45: 0, # 'H'
- 53: 0, # 'I'
- 60: 1, # 'J'
- 16: 2, # 'K'
- 49: 0, # 'L'
- 20: 2, # 'M'
- 46: 0, # 'N'
- 42: 0, # 'O'
- 48: 1, # 'P'
- 44: 0, # 'R'
- 35: 0, # 'S'
- 31: 1, # 'T'
- 51: 0, # 'U'
- 38: 0, # 'V'
- 62: 0, # 'W'
- 43: 0, # 'Y'
- 56: 1, # 'Z'
- 1: 3, # 'a'
- 21: 1, # 'b'
- 28: 1, # 'c'
- 12: 3, # 'd'
- 2: 3, # 'e'
- 18: 2, # 'f'
- 27: 1, # 'g'
- 25: 1, # 'h'
- 3: 2, # 'i'
- 24: 1, # 'j'
- 10: 2, # 'k'
- 5: 2, # 'l'
- 13: 3, # 'm'
- 4: 2, # 'n'
- 15: 0, # 'o'
- 26: 1, # 'p'
- 7: 2, # 'r'
- 8: 3, # 's'
- 9: 2, # 't'
- 14: 3, # 'u'
- 32: 2, # 'v'
- 57: 0, # 'w'
- 58: 2, # 'x'
- 11: 1, # 'y'
- 22: 0, # 'z'
- 63: 0, # '·'
- 54: 0, # 'Ç'
- 50: 0, # 'Ö'
- 55: 0, # 'Ü'
- 59: 0, # 'â'
- 33: 1, # 'ç'
- 61: 0, # 'î'
- 34: 0, # 'ö'
- 17: 1, # 'ü'
- 30: 0, # 'ğ'
- 41: 0, # 'İ'
- 6: 3, # 'ı'
- 40: 0, # 'Ş'
- 19: 0, # 'ş'
- },
- 10: { # 'k'
- 23: 0, # 'A'
- 37: 0, # 'B'
- 47: 0, # 'C'
- 39: 0, # 'D'
- 29: 0, # 'E'
- 52: 0, # 'F'
- 36: 0, # 'G'
- 45: 0, # 'H'
- 53: 0, # 'I'
- 60: 0, # 'J'
- 16: 3, # 'K'
- 49: 0, # 'L'
- 20: 2, # 'M'
- 46: 0, # 'N'
- 42: 0, # 'O'
- 48: 0, # 'P'
- 44: 0, # 'R'
- 35: 0, # 'S'
- 31: 3, # 'T'
- 51: 0, # 'U'
- 38: 1, # 'V'
- 62: 0, # 'W'
- 43: 0, # 'Y'
- 56: 1, # 'Z'
- 1: 3, # 'a'
- 21: 2, # 'b'
- 28: 0, # 'c'
- 12: 2, # 'd'
- 2: 3, # 'e'
- 18: 1, # 'f'
- 27: 2, # 'g'
- 25: 2, # 'h'
- 3: 3, # 'i'
- 24: 2, # 'j'
- 10: 2, # 'k'
- 5: 3, # 'l'
- 13: 3, # 'm'
- 4: 3, # 'n'
- 15: 0, # 'o'
- 26: 3, # 'p'
- 7: 2, # 'r'
- 8: 2, # 's'
- 9: 2, # 't'
- 14: 3, # 'u'
- 32: 0, # 'v'
- 57: 0, # 'w'
- 58: 1, # 'x'
- 11: 3, # 'y'
- 22: 0, # 'z'
- 63: 1, # '·'
- 54: 0, # 'Ç'
- 50: 0, # 'Ö'
- 55: 0, # 'Ü'
- 59: 0, # 'â'
- 33: 3, # 'ç'
- 61: 0, # 'î'
- 34: 1, # 'ö'
- 17: 3, # 'ü'
- 30: 1, # 'ğ'
- 41: 0, # 'İ'
- 6: 3, # 'ı'
- 40: 0, # 'Ş'
- 19: 1, # 'ş'
- },
- 5: { # 'l'
- 23: 0, # 'A'
- 37: 0, # 'B'
- 47: 0, # 'C'
- 39: 0, # 'D'
- 29: 3, # 'E'
- 52: 0, # 'F'
- 36: 0, # 'G'
- 45: 0, # 'H'
- 53: 0, # 'I'
- 60: 0, # 'J'
- 16: 0, # 'K'
- 49: 0, # 'L'
- 20: 2, # 'M'
- 46: 0, # 'N'
- 42: 0, # 'O'
- 48: 0, # 'P'
- 44: 0, # 'R'
- 35: 0, # 'S'
- 31: 1, # 'T'
- 51: 0, # 'U'
- 38: 0, # 'V'
- 62: 0, # 'W'
- 43: 0, # 'Y'
- 56: 0, # 'Z'
- 1: 0, # 'a'
- 21: 3, # 'b'
- 28: 0, # 'c'
- 12: 3, # 'd'
- 2: 1, # 'e'
- 18: 3, # 'f'
- 27: 3, # 'g'
- 25: 2, # 'h'
- 3: 3, # 'i'
- 24: 2, # 'j'
- 10: 3, # 'k'
- 5: 1, # 'l'
- 13: 1, # 'm'
- 4: 3, # 'n'
- 15: 0, # 'o'
- 26: 2, # 'p'
- 7: 3, # 'r'
- 8: 3, # 's'
- 9: 3, # 't'
- 14: 2, # 'u'
- 32: 2, # 'v'
- 57: 0, # 'w'
- 58: 0, # 'x'
- 11: 3, # 'y'
- 22: 0, # 'z'
- 63: 0, # '·'
- 54: 0, # 'Ç'
- 50: 0, # 'Ö'
- 55: 0, # 'Ü'
- 59: 0, # 'â'
- 33: 1, # 'ç'
- 61: 0, # 'î'
- 34: 0, # 'ö'
- 17: 2, # 'ü'
- 30: 0, # 'ğ'
- 41: 0, # 'İ'
- 6: 3, # 'ı'
- 40: 0, # 'Ş'
- 19: 0, # 'ş'
- },
- 13: { # 'm'
- 23: 1, # 'A'
- 37: 0, # 'B'
- 47: 0, # 'C'
- 39: 0, # 'D'
- 29: 3, # 'E'
- 52: 0, # 'F'
- 36: 0, # 'G'
- 45: 0, # 'H'
- 53: 0, # 'I'
- 60: 0, # 'J'
- 16: 0, # 'K'
- 49: 0, # 'L'
- 20: 3, # 'M'
- 46: 0, # 'N'
- 42: 0, # 'O'
- 48: 0, # 'P'
- 44: 0, # 'R'
- 35: 0, # 'S'
- 31: 3, # 'T'
- 51: 0, # 'U'
- 38: 0, # 'V'
- 62: 0, # 'W'
- 43: 1, # 'Y'
- 56: 0, # 'Z'
- 1: 2, # 'a'
- 21: 3, # 'b'
- 28: 0, # 'c'
- 12: 3, # 'd'
- 2: 2, # 'e'
- 18: 3, # 'f'
- 27: 3, # 'g'
- 25: 3, # 'h'
- 3: 3, # 'i'
- 24: 3, # 'j'
- 10: 3, # 'k'
- 5: 0, # 'l'
- 13: 2, # 'm'
- 4: 3, # 'n'
- 15: 1, # 'o'
- 26: 2, # 'p'
- 7: 3, # 'r'
- 8: 3, # 's'
- 9: 3, # 't'
- 14: 2, # 'u'
- 32: 2, # 'v'
- 57: 1, # 'w'
- 58: 0, # 'x'
- 11: 3, # 'y'
- 22: 0, # 'z'
- 63: 0, # '·'
- 54: 0, # 'Ç'
- 50: 0, # 'Ö'
- 55: 0, # 'Ü'
- 59: 0, # 'â'
- 33: 0, # 'ç'
- 61: 0, # 'î'
- 34: 0, # 'ö'
- 17: 3, # 'ü'
- 30: 0, # 'ğ'
- 41: 0, # 'İ'
- 6: 3, # 'ı'
- 40: 0, # 'Ş'
- 19: 1, # 'ş'
- },
- 4: { # 'n'
- 23: 1, # 'A'
- 37: 0, # 'B'
- 47: 0, # 'C'
- 39: 0, # 'D'
- 29: 0, # 'E'
- 52: 0, # 'F'
- 36: 0, # 'G'
- 45: 1, # 'H'
- 53: 0, # 'I'
- 60: 2, # 'J'
- 16: 3, # 'K'
- 49: 0, # 'L'
- 20: 3, # 'M'
- 46: 0, # 'N'
- 42: 0, # 'O'
- 48: 0, # 'P'
- 44: 0, # 'R'
- 35: 0, # 'S'
- 31: 2, # 'T'
- 51: 0, # 'U'
- 38: 0, # 'V'
- 62: 0, # 'W'
- 43: 0, # 'Y'
- 56: 0, # 'Z'
- 1: 3, # 'a'
- 21: 2, # 'b'
- 28: 1, # 'c'
- 12: 3, # 'd'
- 2: 3, # 'e'
- 18: 1, # 'f'
- 27: 2, # 'g'
- 25: 3, # 'h'
- 3: 2, # 'i'
- 24: 2, # 'j'
- 10: 3, # 'k'
- 5: 3, # 'l'
- 13: 3, # 'm'
- 4: 3, # 'n'
- 15: 1, # 'o'
- 26: 3, # 'p'
- 7: 2, # 'r'
- 8: 3, # 's'
- 9: 3, # 't'
- 14: 3, # 'u'
- 32: 2, # 'v'
- 57: 0, # 'w'
- 58: 2, # 'x'
- 11: 3, # 'y'
- 22: 0, # 'z'
- 63: 0, # '·'
- 54: 0, # 'Ç'
- 50: 0, # 'Ö'
- 55: 0, # 'Ü'
- 59: 0, # 'â'
- 33: 1, # 'ç'
- 61: 0, # 'î'
- 34: 0, # 'ö'
- 17: 2, # 'ü'
- 30: 0, # 'ğ'
- 41: 0, # 'İ'
- 6: 1, # 'ı'
- 40: 0, # 'Ş'
- 19: 0, # 'ş'
- },
- 15: { # 'o'
- 23: 0, # 'A'
- 37: 0, # 'B'
- 47: 1, # 'C'
- 39: 0, # 'D'
- 29: 0, # 'E'
- 52: 2, # 'F'
- 36: 1, # 'G'
- 45: 1, # 'H'
- 53: 1, # 'I'
- 60: 0, # 'J'
- 16: 3, # 'K'
- 49: 2, # 'L'
- 20: 0, # 'M'
- 46: 2, # 'N'
- 42: 1, # 'O'
- 48: 2, # 'P'
- 44: 1, # 'R'
- 35: 0, # 'S'
- 31: 0, # 'T'
- 51: 0, # 'U'
- 38: 0, # 'V'
- 62: 0, # 'W'
- 43: 0, # 'Y'
- 56: 0, # 'Z'
- 1: 3, # 'a'
- 21: 0, # 'b'
- 28: 2, # 'c'
- 12: 0, # 'd'
- 2: 3, # 'e'
- 18: 0, # 'f'
- 27: 0, # 'g'
- 25: 0, # 'h'
- 3: 1, # 'i'
- 24: 2, # 'j'
- 10: 1, # 'k'
- 5: 3, # 'l'
- 13: 3, # 'm'
- 4: 2, # 'n'
- 15: 2, # 'o'
- 26: 0, # 'p'
- 7: 1, # 'r'
- 8: 0, # 's'
- 9: 0, # 't'
- 14: 3, # 'u'
- 32: 0, # 'v'
- 57: 0, # 'w'
- 58: 2, # 'x'
- 11: 0, # 'y'
- 22: 2, # 'z'
- 63: 0, # '·'
- 54: 1, # 'Ç'
- 50: 2, # 'Ö'
- 55: 0, # 'Ü'
- 59: 0, # 'â'
- 33: 3, # 'ç'
- 61: 0, # 'î'
- 34: 1, # 'ö'
- 17: 0, # 'ü'
- 30: 2, # 'ğ'
- 41: 2, # 'İ'
- 6: 3, # 'ı'
- 40: 2, # 'Ş'
- 19: 2, # 'ş'
- },
- 26: { # 'p'
- 23: 0, # 'A'
- 37: 0, # 'B'
- 47: 0, # 'C'
- 39: 0, # 'D'
- 29: 0, # 'E'
- 52: 0, # 'F'
- 36: 0, # 'G'
- 45: 0, # 'H'
- 53: 0, # 'I'
- 60: 0, # 'J'
- 16: 3, # 'K'
- 49: 0, # 'L'
- 20: 1, # 'M'
- 46: 0, # 'N'
- 42: 0, # 'O'
- 48: 0, # 'P'
- 44: 0, # 'R'
- 35: 0, # 'S'
- 31: 0, # 'T'
- 51: 0, # 'U'
- 38: 0, # 'V'
- 62: 0, # 'W'
- 43: 0, # 'Y'
- 56: 0, # 'Z'
- 1: 3, # 'a'
- 21: 1, # 'b'
- 28: 0, # 'c'
- 12: 1, # 'd'
- 2: 3, # 'e'
- 18: 0, # 'f'
- 27: 1, # 'g'
- 25: 1, # 'h'
- 3: 2, # 'i'
- 24: 3, # 'j'
- 10: 1, # 'k'
- 5: 3, # 'l'
- 13: 3, # 'm'
- 4: 2, # 'n'
- 15: 0, # 'o'
- 26: 2, # 'p'
- 7: 2, # 'r'
- 8: 1, # 's'
- 9: 1, # 't'
- 14: 3, # 'u'
- 32: 0, # 'v'
- 57: 0, # 'w'
- 58: 1, # 'x'
- 11: 1, # 'y'
- 22: 0, # 'z'
- 63: 0, # '·'
- 54: 0, # 'Ç'
- 50: 0, # 'Ö'
- 55: 0, # 'Ü'
- 59: 0, # 'â'
- 33: 3, # 'ç'
- 61: 0, # 'î'
- 34: 0, # 'ö'
- 17: 1, # 'ü'
- 30: 0, # 'ğ'
- 41: 0, # 'İ'
- 6: 3, # 'ı'
- 40: 0, # 'Ş'
- 19: 0, # 'ş'
- },
- 7: { # 'r'
- 23: 0, # 'A'
- 37: 0, # 'B'
- 47: 0, # 'C'
- 39: 0, # 'D'
- 29: 0, # 'E'
- 52: 1, # 'F'
- 36: 0, # 'G'
- 45: 0, # 'H'
- 53: 0, # 'I'
- 60: 2, # 'J'
- 16: 3, # 'K'
- 49: 0, # 'L'
- 20: 2, # 'M'
- 46: 0, # 'N'
- 42: 0, # 'O'
- 48: 0, # 'P'
- 44: 0, # 'R'
- 35: 0, # 'S'
- 31: 2, # 'T'
- 51: 1, # 'U'
- 38: 0, # 'V'
- 62: 0, # 'W'
- 43: 0, # 'Y'
- 56: 1, # 'Z'
- 1: 3, # 'a'
- 21: 1, # 'b'
- 28: 0, # 'c'
- 12: 3, # 'd'
- 2: 3, # 'e'
- 18: 0, # 'f'
- 27: 2, # 'g'
- 25: 3, # 'h'
- 3: 2, # 'i'
- 24: 2, # 'j'
- 10: 3, # 'k'
- 5: 3, # 'l'
- 13: 3, # 'm'
- 4: 3, # 'n'
- 15: 0, # 'o'
- 26: 2, # 'p'
- 7: 3, # 'r'
- 8: 3, # 's'
- 9: 3, # 't'
- 14: 3, # 'u'
- 32: 2, # 'v'
- 57: 0, # 'w'
- 58: 1, # 'x'
- 11: 2, # 'y'
- 22: 0, # 'z'
- 63: 1, # '·'
- 54: 0, # 'Ç'
- 50: 0, # 'Ö'
- 55: 0, # 'Ü'
- 59: 0, # 'â'
- 33: 2, # 'ç'
- 61: 0, # 'î'
- 34: 0, # 'ö'
- 17: 3, # 'ü'
- 30: 0, # 'ğ'
- 41: 0, # 'İ'
- 6: 2, # 'ı'
- 40: 0, # 'Ş'
- 19: 0, # 'ş'
- },
- 8: { # 's'
- 23: 1, # 'A'
- 37: 0, # 'B'
- 47: 0, # 'C'
- 39: 0, # 'D'
- 29: 0, # 'E'
- 52: 0, # 'F'
- 36: 1, # 'G'
- 45: 0, # 'H'
- 53: 0, # 'I'
- 60: 0, # 'J'
- 16: 3, # 'K'
- 49: 0, # 'L'
- 20: 3, # 'M'
- 46: 0, # 'N'
- 42: 0, # 'O'
- 48: 0, # 'P'
- 44: 0, # 'R'
- 35: 0, # 'S'
- 31: 2, # 'T'
- 51: 0, # 'U'
- 38: 0, # 'V'
- 62: 0, # 'W'
- 43: 0, # 'Y'
- 56: 1, # 'Z'
- 1: 3, # 'a'
- 21: 2, # 'b'
- 28: 1, # 'c'
- 12: 3, # 'd'
- 2: 3, # 'e'
- 18: 0, # 'f'
- 27: 2, # 'g'
- 25: 2, # 'h'
- 3: 2, # 'i'
- 24: 3, # 'j'
- 10: 3, # 'k'
- 5: 3, # 'l'
- 13: 3, # 'm'
- 4: 3, # 'n'
- 15: 0, # 'o'
- 26: 3, # 'p'
- 7: 3, # 'r'
- 8: 3, # 's'
- 9: 3, # 't'
- 14: 3, # 'u'
- 32: 2, # 'v'
- 57: 0, # 'w'
- 58: 1, # 'x'
- 11: 2, # 'y'
- 22: 1, # 'z'
- 63: 0, # '·'
- 54: 0, # 'Ç'
- 50: 0, # 'Ö'
- 55: 0, # 'Ü'
- 59: 0, # 'â'
- 33: 2, # 'ç'
- 61: 0, # 'î'
- 34: 0, # 'ö'
- 17: 2, # 'ü'
- 30: 0, # 'ğ'
- 41: 0, # 'İ'
- 6: 3, # 'ı'
- 40: 0, # 'Ş'
- 19: 1, # 'ş'
- },
- 9: { # 't'
- 23: 0, # 'A'
- 37: 0, # 'B'
- 47: 0, # 'C'
- 39: 0, # 'D'
- 29: 0, # 'E'
- 52: 0, # 'F'
- 36: 0, # 'G'
- 45: 0, # 'H'
- 53: 0, # 'I'
- 60: 1, # 'J'
- 16: 3, # 'K'
- 49: 0, # 'L'
- 20: 2, # 'M'
- 46: 0, # 'N'
- 42: 0, # 'O'
- 48: 0, # 'P'
- 44: 0, # 'R'
- 35: 0, # 'S'
- 31: 2, # 'T'
- 51: 0, # 'U'
- 38: 0, # 'V'
- 62: 0, # 'W'
- 43: 0, # 'Y'
- 56: 1, # 'Z'
- 1: 3, # 'a'
- 21: 3, # 'b'
- 28: 0, # 'c'
- 12: 3, # 'd'
- 2: 3, # 'e'
- 18: 2, # 'f'
- 27: 2, # 'g'
- 25: 2, # 'h'
- 3: 2, # 'i'
- 24: 2, # 'j'
- 10: 3, # 'k'
- 5: 3, # 'l'
- 13: 3, # 'm'
- 4: 3, # 'n'
- 15: 0, # 'o'
- 26: 2, # 'p'
- 7: 3, # 'r'
- 8: 3, # 's'
- 9: 3, # 't'
- 14: 3, # 'u'
- 32: 3, # 'v'
- 57: 0, # 'w'
- 58: 2, # 'x'
- 11: 2, # 'y'
- 22: 0, # 'z'
- 63: 0, # '·'
- 54: 0, # 'Ç'
- 50: 0, # 'Ö'
- 55: 0, # 'Ü'
- 59: 0, # 'â'
- 33: 3, # 'ç'
- 61: 0, # 'î'
- 34: 0, # 'ö'
- 17: 2, # 'ü'
- 30: 0, # 'ğ'
- 41: 0, # 'İ'
- 6: 3, # 'ı'
- 40: 0, # 'Ş'
- 19: 0, # 'ş'
- },
- 14: { # 'u'
- 23: 3, # 'A'
- 37: 0, # 'B'
- 47: 0, # 'C'
- 39: 0, # 'D'
- 29: 3, # 'E'
- 52: 0, # 'F'
- 36: 0, # 'G'
- 45: 1, # 'H'
- 53: 0, # 'I'
- 60: 1, # 'J'
- 16: 0, # 'K'
- 49: 0, # 'L'
- 20: 3, # 'M'
- 46: 2, # 'N'
- 42: 0, # 'O'
- 48: 1, # 'P'
- 44: 0, # 'R'
- 35: 0, # 'S'
- 31: 3, # 'T'
- 51: 0, # 'U'
- 38: 0, # 'V'
- 62: 0, # 'W'
- 43: 1, # 'Y'
- 56: 2, # 'Z'
- 1: 2, # 'a'
- 21: 3, # 'b'
- 28: 0, # 'c'
- 12: 3, # 'd'
- 2: 2, # 'e'
- 18: 2, # 'f'
- 27: 3, # 'g'
- 25: 3, # 'h'
- 3: 3, # 'i'
- 24: 2, # 'j'
- 10: 3, # 'k'
- 5: 0, # 'l'
- 13: 3, # 'm'
- 4: 3, # 'n'
- 15: 0, # 'o'
- 26: 3, # 'p'
- 7: 3, # 'r'
- 8: 3, # 's'
- 9: 3, # 't'
- 14: 3, # 'u'
- 32: 2, # 'v'
- 57: 2, # 'w'
- 58: 0, # 'x'
- 11: 3, # 'y'
- 22: 0, # 'z'
- 63: 1, # '·'
- 54: 0, # 'Ç'
- 50: 0, # 'Ö'
- 55: 0, # 'Ü'
- 59: 0, # 'â'
- 33: 0, # 'ç'
- 61: 0, # 'î'
- 34: 0, # 'ö'
- 17: 3, # 'ü'
- 30: 1, # 'ğ'
- 41: 0, # 'İ'
- 6: 3, # 'ı'
- 40: 0, # 'Ş'
- 19: 0, # 'ş'
- },
- 32: { # 'v'
- 23: 0, # 'A'
- 37: 0, # 'B'
- 47: 0, # 'C'
- 39: 0, # 'D'
- 29: 0, # 'E'
- 52: 0, # 'F'
- 36: 0, # 'G'
- 45: 0, # 'H'
- 53: 0, # 'I'
- 60: 0, # 'J'
- 16: 3, # 'K'
- 49: 0, # 'L'
- 20: 1, # 'M'
- 46: 0, # 'N'
- 42: 0, # 'O'
- 48: 0, # 'P'
- 44: 0, # 'R'
- 35: 0, # 'S'
- 31: 0, # 'T'
- 51: 0, # 'U'
- 38: 0, # 'V'
- 62: 0, # 'W'
- 43: 0, # 'Y'
- 56: 0, # 'Z'
- 1: 3, # 'a'
- 21: 0, # 'b'
- 28: 0, # 'c'
- 12: 3, # 'd'
- 2: 3, # 'e'
- 18: 0, # 'f'
- 27: 0, # 'g'
- 25: 0, # 'h'
- 3: 0, # 'i'
- 24: 1, # 'j'
- 10: 1, # 'k'
- 5: 3, # 'l'
- 13: 2, # 'm'
- 4: 3, # 'n'
- 15: 0, # 'o'
- 26: 1, # 'p'
- 7: 1, # 'r'
- 8: 2, # 's'
- 9: 3, # 't'
- 14: 3, # 'u'
- 32: 1, # 'v'
- 57: 0, # 'w'
- 58: 0, # 'x'
- 11: 0, # 'y'
- 22: 0, # 'z'
- 63: 0, # '·'
- 54: 0, # 'Ç'
- 50: 0, # 'Ö'
- 55: 0, # 'Ü'
- 59: 0, # 'â'
- 33: 2, # 'ç'
- 61: 0, # 'î'
- 34: 0, # 'ö'
- 17: 0, # 'ü'
- 30: 0, # 'ğ'
- 41: 0, # 'İ'
- 6: 1, # 'ı'
- 40: 0, # 'Ş'
- 19: 0, # 'ş'
- },
- 57: { # 'w'
- 23: 0, # 'A'
- 37: 0, # 'B'
- 47: 0, # 'C'
- 39: 0, # 'D'
- 29: 0, # 'E'
- 52: 0, # 'F'
- 36: 0, # 'G'
- 45: 0, # 'H'
- 53: 0, # 'I'
- 60: 0, # 'J'
- 16: 0, # 'K'
- 49: 0, # 'L'
- 20: 0, # 'M'
- 46: 0, # 'N'
- 42: 0, # 'O'
- 48: 0, # 'P'
- 44: 0, # 'R'
- 35: 0, # 'S'
- 31: 0, # 'T'
- 51: 1, # 'U'
- 38: 0, # 'V'
- 62: 0, # 'W'
- 43: 0, # 'Y'
- 56: 0, # 'Z'
- 1: 1, # 'a'
- 21: 0, # 'b'
- 28: 0, # 'c'
- 12: 0, # 'd'
- 2: 2, # 'e'
- 18: 0, # 'f'
- 27: 0, # 'g'
- 25: 1, # 'h'
- 3: 0, # 'i'
- 24: 0, # 'j'
- 10: 1, # 'k'
- 5: 0, # 'l'
- 13: 0, # 'm'
- 4: 1, # 'n'
- 15: 0, # 'o'
- 26: 0, # 'p'
- 7: 0, # 'r'
- 8: 1, # 's'
- 9: 0, # 't'
- 14: 1, # 'u'
- 32: 0, # 'v'
- 57: 2, # 'w'
- 58: 0, # 'x'
- 11: 0, # 'y'
- 22: 0, # 'z'
- 63: 1, # '·'
- 54: 0, # 'Ç'
- 50: 0, # 'Ö'
- 55: 0, # 'Ü'
- 59: 0, # 'â'
- 33: 0, # 'ç'
- 61: 0, # 'î'
- 34: 0, # 'ö'
- 17: 1, # 'ü'
- 30: 0, # 'ğ'
- 41: 0, # 'İ'
- 6: 0, # 'ı'
- 40: 0, # 'Ş'
- 19: 0, # 'ş'
- },
- 58: { # 'x'
- 23: 0, # 'A'
- 37: 0, # 'B'
- 47: 0, # 'C'
- 39: 0, # 'D'
- 29: 1, # 'E'
- 52: 0, # 'F'
- 36: 0, # 'G'
- 45: 0, # 'H'
- 53: 0, # 'I'
- 60: 1, # 'J'
- 16: 0, # 'K'
- 49: 0, # 'L'
- 20: 1, # 'M'
- 46: 0, # 'N'
- 42: 0, # 'O'
- 48: 0, # 'P'
- 44: 0, # 'R'
- 35: 0, # 'S'
- 31: 0, # 'T'
- 51: 0, # 'U'
- 38: 0, # 'V'
- 62: 0, # 'W'
- 43: 0, # 'Y'
- 56: 0, # 'Z'
- 1: 0, # 'a'
- 21: 1, # 'b'
- 28: 0, # 'c'
- 12: 2, # 'd'
- 2: 1, # 'e'
- 18: 0, # 'f'
- 27: 0, # 'g'
- 25: 0, # 'h'
- 3: 2, # 'i'
- 24: 2, # 'j'
- 10: 1, # 'k'
- 5: 0, # 'l'
- 13: 0, # 'm'
- 4: 2, # 'n'
- 15: 0, # 'o'
- 26: 0, # 'p'
- 7: 1, # 'r'
- 8: 2, # 's'
- 9: 1, # 't'
- 14: 0, # 'u'
- 32: 0, # 'v'
- 57: 0, # 'w'
- 58: 0, # 'x'
- 11: 2, # 'y'
- 22: 0, # 'z'
- 63: 0, # '·'
- 54: 0, # 'Ç'
- 50: 0, # 'Ö'
- 55: 0, # 'Ü'
- 59: 0, # 'â'
- 33: 0, # 'ç'
- 61: 0, # 'î'
- 34: 0, # 'ö'
- 17: 1, # 'ü'
- 30: 0, # 'ğ'
- 41: 0, # 'İ'
- 6: 2, # 'ı'
- 40: 0, # 'Ş'
- 19: 0, # 'ş'
- },
- 11: { # 'y'
- 23: 1, # 'A'
- 37: 0, # 'B'
- 47: 0, # 'C'
- 39: 0, # 'D'
- 29: 0, # 'E'
- 52: 0, # 'F'
- 36: 0, # 'G'
- 45: 0, # 'H'
- 53: 0, # 'I'
- 60: 1, # 'J'
- 16: 3, # 'K'
- 49: 0, # 'L'
- 20: 1, # 'M'
- 46: 0, # 'N'
- 42: 0, # 'O'
- 48: 0, # 'P'
- 44: 0, # 'R'
- 35: 0, # 'S'
- 31: 1, # 'T'
- 51: 0, # 'U'
- 38: 0, # 'V'
- 62: 0, # 'W'
- 43: 1, # 'Y'
- 56: 1, # 'Z'
- 1: 3, # 'a'
- 21: 1, # 'b'
- 28: 0, # 'c'
- 12: 2, # 'd'
- 2: 3, # 'e'
- 18: 0, # 'f'
- 27: 2, # 'g'
- 25: 2, # 'h'
- 3: 2, # 'i'
- 24: 1, # 'j'
- 10: 2, # 'k'
- 5: 3, # 'l'
- 13: 3, # 'm'
- 4: 3, # 'n'
- 15: 0, # 'o'
- 26: 1, # 'p'
- 7: 2, # 'r'
- 8: 1, # 's'
- 9: 2, # 't'
- 14: 3, # 'u'
- 32: 0, # 'v'
- 57: 0, # 'w'
- 58: 1, # 'x'
- 11: 3, # 'y'
- 22: 0, # 'z'
- 63: 0, # '·'
- 54: 0, # 'Ç'
- 50: 0, # 'Ö'
- 55: 0, # 'Ü'
- 59: 0, # 'â'
- 33: 3, # 'ç'
- 61: 0, # 'î'
- 34: 0, # 'ö'
- 17: 2, # 'ü'
- 30: 0, # 'ğ'
- 41: 0, # 'İ'
- 6: 3, # 'ı'
- 40: 0, # 'Ş'
- 19: 0, # 'ş'
- },
- 22: { # 'z'
- 23: 2, # 'A'
- 37: 2, # 'B'
- 47: 1, # 'C'
- 39: 2, # 'D'
- 29: 3, # 'E'
- 52: 1, # 'F'
- 36: 2, # 'G'
- 45: 2, # 'H'
- 53: 1, # 'I'
- 60: 0, # 'J'
- 16: 0, # 'K'
- 49: 0, # 'L'
- 20: 3, # 'M'
- 46: 2, # 'N'
- 42: 2, # 'O'
- 48: 2, # 'P'
- 44: 1, # 'R'
- 35: 1, # 'S'
- 31: 3, # 'T'
- 51: 2, # 'U'
- 38: 2, # 'V'
- 62: 0, # 'W'
- 43: 2, # 'Y'
- 56: 1, # 'Z'
- 1: 1, # 'a'
- 21: 2, # 'b'
- 28: 1, # 'c'
- 12: 2, # 'd'
- 2: 2, # 'e'
- 18: 3, # 'f'
- 27: 2, # 'g'
- 25: 2, # 'h'
- 3: 3, # 'i'
- 24: 2, # 'j'
- 10: 3, # 'k'
- 5: 0, # 'l'
- 13: 2, # 'm'
- 4: 3, # 'n'
- 15: 2, # 'o'
- 26: 2, # 'p'
- 7: 3, # 'r'
- 8: 3, # 's'
- 9: 3, # 't'
- 14: 0, # 'u'
- 32: 2, # 'v'
- 57: 0, # 'w'
- 58: 0, # 'x'
- 11: 3, # 'y'
- 22: 2, # 'z'
- 63: 1, # '·'
- 54: 0, # 'Ç'
- 50: 0, # 'Ö'
- 55: 2, # 'Ü'
- 59: 1, # 'â'
- 33: 0, # 'ç'
- 61: 0, # 'î'
- 34: 2, # 'ö'
- 17: 2, # 'ü'
- 30: 2, # 'ğ'
- 41: 1, # 'İ'
- 6: 3, # 'ı'
- 40: 1, # 'Ş'
- 19: 2, # 'ş'
- },
- 63: { # '·'
- 23: 0, # 'A'
- 37: 0, # 'B'
- 47: 0, # 'C'
- 39: 0, # 'D'
- 29: 0, # 'E'
- 52: 0, # 'F'
- 36: 0, # 'G'
- 45: 0, # 'H'
- 53: 0, # 'I'
- 60: 0, # 'J'
- 16: 0, # 'K'
- 49: 0, # 'L'
- 20: 0, # 'M'
- 46: 0, # 'N'
- 42: 0, # 'O'
- 48: 0, # 'P'
- 44: 0, # 'R'
- 35: 0, # 'S'
- 31: 0, # 'T'
- 51: 0, # 'U'
- 38: 0, # 'V'
- 62: 0, # 'W'
- 43: 0, # 'Y'
- 56: 0, # 'Z'
- 1: 0, # 'a'
- 21: 0, # 'b'
- 28: 0, # 'c'
- 12: 0, # 'd'
- 2: 1, # 'e'
- 18: 0, # 'f'
- 27: 0, # 'g'
- 25: 0, # 'h'
- 3: 0, # 'i'
- 24: 0, # 'j'
- 10: 0, # 'k'
- 5: 0, # 'l'
- 13: 2, # 'm'
- 4: 0, # 'n'
- 15: 0, # 'o'
- 26: 0, # 'p'
- 7: 0, # 'r'
- 8: 0, # 's'
- 9: 0, # 't'
- 14: 2, # 'u'
- 32: 0, # 'v'
- 57: 0, # 'w'
- 58: 0, # 'x'
- 11: 0, # 'y'
- 22: 0, # 'z'
- 63: 0, # '·'
- 54: 0, # 'Ç'
- 50: 0, # 'Ö'
- 55: 0, # 'Ü'
- 59: 0, # 'â'
- 33: 0, # 'ç'
- 61: 0, # 'î'
- 34: 0, # 'ö'
- 17: 0, # 'ü'
- 30: 0, # 'ğ'
- 41: 0, # 'İ'
- 6: 0, # 'ı'
- 40: 0, # 'Ş'
- 19: 0, # 'ş'
- },
- 54: { # 'Ç'
- 23: 0, # 'A'
- 37: 0, # 'B'
- 47: 1, # 'C'
- 39: 1, # 'D'
- 29: 0, # 'E'
- 52: 0, # 'F'
- 36: 1, # 'G'
- 45: 1, # 'H'
- 53: 1, # 'I'
- 60: 0, # 'J'
- 16: 0, # 'K'
- 49: 0, # 'L'
- 20: 0, # 'M'
- 46: 0, # 'N'
- 42: 1, # 'O'
- 48: 1, # 'P'
- 44: 0, # 'R'
- 35: 0, # 'S'
- 31: 0, # 'T'
- 51: 1, # 'U'
- 38: 1, # 'V'
- 62: 0, # 'W'
- 43: 2, # 'Y'
- 56: 0, # 'Z'
- 1: 0, # 'a'
- 21: 1, # 'b'
- 28: 0, # 'c'
- 12: 1, # 'd'
- 2: 0, # 'e'
- 18: 0, # 'f'
- 27: 1, # 'g'
- 25: 0, # 'h'
- 3: 3, # 'i'
- 24: 0, # 'j'
- 10: 1, # 'k'
- 5: 0, # 'l'
- 13: 0, # 'm'
- 4: 2, # 'n'
- 15: 1, # 'o'
- 26: 0, # 'p'
- 7: 2, # 'r'
- 8: 0, # 's'
- 9: 1, # 't'
- 14: 0, # 'u'
- 32: 2, # 'v'
- 57: 0, # 'w'
- 58: 0, # 'x'
- 11: 0, # 'y'
- 22: 0, # 'z'
- 63: 0, # '·'
- 54: 0, # 'Ç'
- 50: 0, # 'Ö'
- 55: 2, # 'Ü'
- 59: 0, # 'â'
- 33: 0, # 'ç'
- 61: 0, # 'î'
- 34: 1, # 'ö'
- 17: 0, # 'ü'
- 30: 0, # 'ğ'
- 41: 0, # 'İ'
- 6: 2, # 'ı'
- 40: 0, # 'Ş'
- 19: 1, # 'ş'
- },
- 50: { # 'Ö'
- 23: 0, # 'A'
- 37: 0, # 'B'
- 47: 1, # 'C'
- 39: 1, # 'D'
- 29: 2, # 'E'
- 52: 0, # 'F'
- 36: 1, # 'G'
- 45: 2, # 'H'
- 53: 0, # 'I'
- 60: 0, # 'J'
- 16: 0, # 'K'
- 49: 0, # 'L'
- 20: 1, # 'M'
- 46: 1, # 'N'
- 42: 2, # 'O'
- 48: 2, # 'P'
- 44: 1, # 'R'
- 35: 0, # 'S'
- 31: 0, # 'T'
- 51: 1, # 'U'
- 38: 1, # 'V'
- 62: 0, # 'W'
- 43: 2, # 'Y'
- 56: 0, # 'Z'
- 1: 0, # 'a'
- 21: 2, # 'b'
- 28: 1, # 'c'
- 12: 2, # 'd'
- 2: 0, # 'e'
- 18: 1, # 'f'
- 27: 1, # 'g'
- 25: 1, # 'h'
- 3: 2, # 'i'
- 24: 0, # 'j'
- 10: 2, # 'k'
- 5: 0, # 'l'
- 13: 0, # 'm'
- 4: 3, # 'n'
- 15: 2, # 'o'
- 26: 2, # 'p'
- 7: 3, # 'r'
- 8: 1, # 's'
- 9: 2, # 't'
- 14: 0, # 'u'
- 32: 1, # 'v'
- 57: 0, # 'w'
- 58: 0, # 'x'
- 11: 0, # 'y'
- 22: 1, # 'z'
- 63: 0, # '·'
- 54: 0, # 'Ç'
- 50: 0, # 'Ö'
- 55: 0, # 'Ü'
- 59: 0, # 'â'
- 33: 0, # 'ç'
- 61: 0, # 'î'
- 34: 2, # 'ö'
- 17: 2, # 'ü'
- 30: 1, # 'ğ'
- 41: 0, # 'İ'
- 6: 2, # 'ı'
- 40: 0, # 'Ş'
- 19: 1, # 'ş'
- },
- 55: { # 'Ü'
- 23: 0, # 'A'
- 37: 0, # 'B'
- 47: 0, # 'C'
- 39: 0, # 'D'
- 29: 0, # 'E'
- 52: 2, # 'F'
- 36: 0, # 'G'
- 45: 0, # 'H'
- 53: 0, # 'I'
- 60: 0, # 'J'
- 16: 1, # 'K'
- 49: 0, # 'L'
- 20: 0, # 'M'
- 46: 0, # 'N'
- 42: 0, # 'O'
- 48: 1, # 'P'
- 44: 0, # 'R'
- 35: 0, # 'S'
- 31: 0, # 'T'
- 51: 0, # 'U'
- 38: 1, # 'V'
- 62: 0, # 'W'
- 43: 0, # 'Y'
- 56: 0, # 'Z'
- 1: 2, # 'a'
- 21: 0, # 'b'
- 28: 2, # 'c'
- 12: 0, # 'd'
- 2: 2, # 'e'
- 18: 0, # 'f'
- 27: 1, # 'g'
- 25: 0, # 'h'
- 3: 0, # 'i'
- 24: 0, # 'j'
- 10: 0, # 'k'
- 5: 1, # 'l'
- 13: 1, # 'm'
- 4: 1, # 'n'
- 15: 0, # 'o'
- 26: 0, # 'p'
- 7: 0, # 'r'
- 8: 0, # 's'
- 9: 1, # 't'
- 14: 2, # 'u'
- 32: 0, # 'v'
- 57: 0, # 'w'
- 58: 0, # 'x'
- 11: 0, # 'y'
- 22: 1, # 'z'
- 63: 0, # '·'
- 54: 0, # 'Ç'
- 50: 1, # 'Ö'
- 55: 0, # 'Ü'
- 59: 0, # 'â'
- 33: 0, # 'ç'
- 61: 0, # 'î'
- 34: 1, # 'ö'
- 17: 0, # 'ü'
- 30: 1, # 'ğ'
- 41: 1, # 'İ'
- 6: 0, # 'ı'
- 40: 0, # 'Ş'
- 19: 1, # 'ş'
- },
- 59: { # 'â'
- 23: 0, # 'A'
- 37: 0, # 'B'
- 47: 0, # 'C'
- 39: 0, # 'D'
- 29: 0, # 'E'
- 52: 0, # 'F'
- 36: 1, # 'G'
- 45: 0, # 'H'
- 53: 0, # 'I'
- 60: 0, # 'J'
- 16: 1, # 'K'
- 49: 0, # 'L'
- 20: 0, # 'M'
- 46: 0, # 'N'
- 42: 0, # 'O'
- 48: 0, # 'P'
- 44: 0, # 'R'
- 35: 0, # 'S'
- 31: 0, # 'T'
- 51: 0, # 'U'
- 38: 0, # 'V'
- 62: 0, # 'W'
- 43: 0, # 'Y'
- 56: 0, # 'Z'
- 1: 2, # 'a'
- 21: 0, # 'b'
- 28: 0, # 'c'
- 12: 0, # 'd'
- 2: 2, # 'e'
- 18: 0, # 'f'
- 27: 0, # 'g'
- 25: 0, # 'h'
- 3: 0, # 'i'
- 24: 0, # 'j'
- 10: 0, # 'k'
- 5: 0, # 'l'
- 13: 2, # 'm'
- 4: 0, # 'n'
- 15: 1, # 'o'
- 26: 0, # 'p'
- 7: 0, # 'r'
- 8: 0, # 's'
- 9: 0, # 't'
- 14: 2, # 'u'
- 32: 0, # 'v'
- 57: 0, # 'w'
- 58: 0, # 'x'
- 11: 0, # 'y'
- 22: 1, # 'z'
- 63: 0, # '·'
- 54: 0, # 'Ç'
- 50: 0, # 'Ö'
- 55: 0, # 'Ü'
- 59: 0, # 'â'
- 33: 0, # 'ç'
- 61: 0, # 'î'
- 34: 0, # 'ö'
- 17: 0, # 'ü'
- 30: 0, # 'ğ'
- 41: 0, # 'İ'
- 6: 1, # 'ı'
- 40: 1, # 'Ş'
- 19: 0, # 'ş'
- },
- 33: { # 'ç'
- 23: 0, # 'A'
- 37: 0, # 'B'
- 47: 0, # 'C'
- 39: 0, # 'D'
- 29: 3, # 'E'
- 52: 0, # 'F'
- 36: 0, # 'G'
- 45: 0, # 'H'
- 53: 0, # 'I'
- 60: 0, # 'J'
- 16: 0, # 'K'
- 49: 0, # 'L'
- 20: 1, # 'M'
- 46: 0, # 'N'
- 42: 0, # 'O'
- 48: 0, # 'P'
- 44: 0, # 'R'
- 35: 0, # 'S'
- 31: 2, # 'T'
- 51: 0, # 'U'
- 38: 1, # 'V'
- 62: 0, # 'W'
- 43: 0, # 'Y'
- 56: 0, # 'Z'
- 1: 0, # 'a'
- 21: 3, # 'b'
- 28: 0, # 'c'
- 12: 2, # 'd'
- 2: 0, # 'e'
- 18: 2, # 'f'
- 27: 1, # 'g'
- 25: 3, # 'h'
- 3: 3, # 'i'
- 24: 0, # 'j'
- 10: 3, # 'k'
- 5: 0, # 'l'
- 13: 0, # 'm'
- 4: 3, # 'n'
- 15: 0, # 'o'
- 26: 1, # 'p'
- 7: 3, # 'r'
- 8: 2, # 's'
- 9: 3, # 't'
- 14: 0, # 'u'
- 32: 2, # 'v'
- 57: 0, # 'w'
- 58: 0, # 'x'
- 11: 2, # 'y'
- 22: 0, # 'z'
- 63: 0, # '·'
- 54: 0, # 'Ç'
- 50: 0, # 'Ö'
- 55: 0, # 'Ü'
- 59: 0, # 'â'
- 33: 0, # 'ç'
- 61: 0, # 'î'
- 34: 0, # 'ö'
- 17: 1, # 'ü'
- 30: 0, # 'ğ'
- 41: 0, # 'İ'
- 6: 3, # 'ı'
- 40: 0, # 'Ş'
- 19: 0, # 'ş'
- },
- 61: { # 'î'
- 23: 0, # 'A'
- 37: 0, # 'B'
- 47: 0, # 'C'
- 39: 0, # 'D'
- 29: 0, # 'E'
- 52: 0, # 'F'
- 36: 0, # 'G'
- 45: 0, # 'H'
- 53: 0, # 'I'
- 60: 0, # 'J'
- 16: 0, # 'K'
- 49: 0, # 'L'
- 20: 0, # 'M'
- 46: 0, # 'N'
- 42: 0, # 'O'
- 48: 0, # 'P'
- 44: 0, # 'R'
- 35: 0, # 'S'
- 31: 0, # 'T'
- 51: 0, # 'U'
- 38: 0, # 'V'
- 62: 0, # 'W'
- 43: 0, # 'Y'
- 56: 1, # 'Z'
- 1: 2, # 'a'
- 21: 0, # 'b'
- 28: 0, # 'c'
- 12: 0, # 'd'
- 2: 2, # 'e'
- 18: 0, # 'f'
- 27: 0, # 'g'
- 25: 0, # 'h'
- 3: 0, # 'i'
- 24: 1, # 'j'
- 10: 0, # 'k'
- 5: 0, # 'l'
- 13: 1, # 'm'
- 4: 1, # 'n'
- 15: 0, # 'o'
- 26: 0, # 'p'
- 7: 0, # 'r'
- 8: 0, # 's'
- 9: 0, # 't'
- 14: 1, # 'u'
- 32: 0, # 'v'
- 57: 0, # 'w'
- 58: 0, # 'x'
- 11: 0, # 'y'
- 22: 1, # 'z'
- 63: 0, # '·'
- 54: 0, # 'Ç'
- 50: 0, # 'Ö'
- 55: 0, # 'Ü'
- 59: 0, # 'â'
- 33: 0, # 'ç'
- 61: 1, # 'î'
- 34: 0, # 'ö'
- 17: 0, # 'ü'
- 30: 0, # 'ğ'
- 41: 0, # 'İ'
- 6: 1, # 'ı'
- 40: 0, # 'Ş'
- 19: 0, # 'ş'
- },
- 34: { # 'ö'
- 23: 0, # 'A'
- 37: 1, # 'B'
- 47: 1, # 'C'
- 39: 0, # 'D'
- 29: 0, # 'E'
- 52: 2, # 'F'
- 36: 1, # 'G'
- 45: 1, # 'H'
- 53: 0, # 'I'
- 60: 0, # 'J'
- 16: 3, # 'K'
- 49: 1, # 'L'
- 20: 0, # 'M'
- 46: 1, # 'N'
- 42: 1, # 'O'
- 48: 2, # 'P'
- 44: 1, # 'R'
- 35: 1, # 'S'
- 31: 1, # 'T'
- 51: 1, # 'U'
- 38: 1, # 'V'
- 62: 0, # 'W'
- 43: 0, # 'Y'
- 56: 1, # 'Z'
- 1: 3, # 'a'
- 21: 1, # 'b'
- 28: 2, # 'c'
- 12: 1, # 'd'
- 2: 3, # 'e'
- 18: 0, # 'f'
- 27: 2, # 'g'
- 25: 2, # 'h'
- 3: 1, # 'i'
- 24: 2, # 'j'
- 10: 1, # 'k'
- 5: 2, # 'l'
- 13: 3, # 'm'
- 4: 2, # 'n'
- 15: 2, # 'o'
- 26: 0, # 'p'
- 7: 0, # 'r'
- 8: 3, # 's'
- 9: 1, # 't'
- 14: 3, # 'u'
- 32: 0, # 'v'
- 57: 0, # 'w'
- 58: 0, # 'x'
- 11: 1, # 'y'
- 22: 2, # 'z'
- 63: 0, # '·'
- 54: 1, # 'Ç'
- 50: 2, # 'Ö'
- 55: 0, # 'Ü'
- 59: 0, # 'â'
- 33: 2, # 'ç'
- 61: 0, # 'î'
- 34: 2, # 'ö'
- 17: 0, # 'ü'
- 30: 2, # 'ğ'
- 41: 1, # 'İ'
- 6: 1, # 'ı'
- 40: 2, # 'Ş'
- 19: 1, # 'ş'
- },
- 17: { # 'ü'
- 23: 0, # 'A'
- 37: 0, # 'B'
- 47: 1, # 'C'
- 39: 0, # 'D'
- 29: 0, # 'E'
- 52: 0, # 'F'
- 36: 0, # 'G'
- 45: 0, # 'H'
- 53: 0, # 'I'
- 60: 1, # 'J'
- 16: 1, # 'K'
- 49: 0, # 'L'
- 20: 1, # 'M'
- 46: 0, # 'N'
- 42: 0, # 'O'
- 48: 0, # 'P'
- 44: 0, # 'R'
- 35: 0, # 'S'
- 31: 1, # 'T'
- 51: 0, # 'U'
- 38: 0, # 'V'
- 62: 0, # 'W'
- 43: 0, # 'Y'
- 56: 1, # 'Z'
- 1: 3, # 'a'
- 21: 0, # 'b'
- 28: 0, # 'c'
- 12: 1, # 'd'
- 2: 3, # 'e'
- 18: 1, # 'f'
- 27: 2, # 'g'
- 25: 0, # 'h'
- 3: 1, # 'i'
- 24: 1, # 'j'
- 10: 2, # 'k'
- 5: 3, # 'l'
- 13: 2, # 'm'
- 4: 3, # 'n'
- 15: 0, # 'o'
- 26: 2, # 'p'
- 7: 2, # 'r'
- 8: 3, # 's'
- 9: 2, # 't'
- 14: 3, # 'u'
- 32: 1, # 'v'
- 57: 1, # 'w'
- 58: 0, # 'x'
- 11: 0, # 'y'
- 22: 0, # 'z'
- 63: 0, # '·'
- 54: 0, # 'Ç'
- 50: 0, # 'Ö'
- 55: 0, # 'Ü'
- 59: 0, # 'â'
- 33: 1, # 'ç'
- 61: 0, # 'î'
- 34: 0, # 'ö'
- 17: 2, # 'ü'
- 30: 0, # 'ğ'
- 41: 0, # 'İ'
- 6: 2, # 'ı'
- 40: 0, # 'Ş'
- 19: 0, # 'ş'
- },
- 30: { # 'ğ'
- 23: 0, # 'A'
- 37: 2, # 'B'
- 47: 1, # 'C'
- 39: 0, # 'D'
- 29: 0, # 'E'
- 52: 2, # 'F'
- 36: 1, # 'G'
- 45: 0, # 'H'
- 53: 1, # 'I'
- 60: 0, # 'J'
- 16: 3, # 'K'
- 49: 0, # 'L'
- 20: 1, # 'M'
- 46: 2, # 'N'
- 42: 2, # 'O'
- 48: 1, # 'P'
- 44: 1, # 'R'
- 35: 0, # 'S'
- 31: 1, # 'T'
- 51: 0, # 'U'
- 38: 2, # 'V'
- 62: 0, # 'W'
- 43: 2, # 'Y'
- 56: 0, # 'Z'
- 1: 3, # 'a'
- 21: 0, # 'b'
- 28: 2, # 'c'
- 12: 0, # 'd'
- 2: 2, # 'e'
- 18: 0, # 'f'
- 27: 0, # 'g'
- 25: 0, # 'h'
- 3: 0, # 'i'
- 24: 3, # 'j'
- 10: 1, # 'k'
- 5: 2, # 'l'
- 13: 3, # 'm'
- 4: 0, # 'n'
- 15: 1, # 'o'
- 26: 0, # 'p'
- 7: 1, # 'r'
- 8: 0, # 's'
- 9: 0, # 't'
- 14: 3, # 'u'
- 32: 0, # 'v'
- 57: 0, # 'w'
- 58: 0, # 'x'
- 11: 0, # 'y'
- 22: 2, # 'z'
- 63: 0, # '·'
- 54: 2, # 'Ç'
- 50: 2, # 'Ö'
- 55: 0, # 'Ü'
- 59: 0, # 'â'
- 33: 1, # 'ç'
- 61: 0, # 'î'
- 34: 2, # 'ö'
- 17: 0, # 'ü'
- 30: 1, # 'ğ'
- 41: 2, # 'İ'
- 6: 2, # 'ı'
- 40: 2, # 'Ş'
- 19: 1, # 'ş'
- },
- 41: { # 'İ'
- 23: 0, # 'A'
- 37: 0, # 'B'
- 47: 1, # 'C'
- 39: 1, # 'D'
- 29: 1, # 'E'
- 52: 0, # 'F'
- 36: 2, # 'G'
- 45: 2, # 'H'
- 53: 0, # 'I'
- 60: 0, # 'J'
- 16: 0, # 'K'
- 49: 0, # 'L'
- 20: 2, # 'M'
- 46: 1, # 'N'
- 42: 1, # 'O'
- 48: 2, # 'P'
- 44: 0, # 'R'
- 35: 1, # 'S'
- 31: 1, # 'T'
- 51: 1, # 'U'
- 38: 1, # 'V'
- 62: 0, # 'W'
- 43: 2, # 'Y'
- 56: 0, # 'Z'
- 1: 1, # 'a'
- 21: 2, # 'b'
- 28: 1, # 'c'
- 12: 2, # 'd'
- 2: 1, # 'e'
- 18: 0, # 'f'
- 27: 3, # 'g'
- 25: 2, # 'h'
- 3: 2, # 'i'
- 24: 2, # 'j'
- 10: 2, # 'k'
- 5: 0, # 'l'
- 13: 1, # 'm'
- 4: 3, # 'n'
- 15: 1, # 'o'
- 26: 1, # 'p'
- 7: 3, # 'r'
- 8: 3, # 's'
- 9: 2, # 't'
- 14: 0, # 'u'
- 32: 0, # 'v'
- 57: 1, # 'w'
- 58: 0, # 'x'
- 11: 2, # 'y'
- 22: 0, # 'z'
- 63: 0, # '·'
- 54: 0, # 'Ç'
- 50: 0, # 'Ö'
- 55: 1, # 'Ü'
- 59: 1, # 'â'
- 33: 0, # 'ç'
- 61: 0, # 'î'
- 34: 1, # 'ö'
- 17: 1, # 'ü'
- 30: 2, # 'ğ'
- 41: 0, # 'İ'
- 6: 3, # 'ı'
- 40: 0, # 'Ş'
- 19: 1, # 'ş'
- },
- 6: { # 'ı'
- 23: 2, # 'A'
- 37: 0, # 'B'
- 47: 0, # 'C'
- 39: 0, # 'D'
- 29: 0, # 'E'
- 52: 0, # 'F'
- 36: 1, # 'G'
- 45: 0, # 'H'
- 53: 0, # 'I'
- 60: 2, # 'J'
- 16: 3, # 'K'
- 49: 0, # 'L'
- 20: 3, # 'M'
- 46: 1, # 'N'
- 42: 0, # 'O'
- 48: 0, # 'P'
- 44: 0, # 'R'
- 35: 0, # 'S'
- 31: 2, # 'T'
- 51: 0, # 'U'
- 38: 0, # 'V'
- 62: 0, # 'W'
- 43: 2, # 'Y'
- 56: 1, # 'Z'
- 1: 3, # 'a'
- 21: 2, # 'b'
- 28: 1, # 'c'
- 12: 3, # 'd'
- 2: 3, # 'e'
- 18: 3, # 'f'
- 27: 3, # 'g'
- 25: 2, # 'h'
- 3: 3, # 'i'
- 24: 3, # 'j'
- 10: 3, # 'k'
- 5: 3, # 'l'
- 13: 3, # 'm'
- 4: 3, # 'n'
- 15: 0, # 'o'
- 26: 3, # 'p'
- 7: 3, # 'r'
- 8: 3, # 's'
- 9: 3, # 't'
- 14: 3, # 'u'
- 32: 3, # 'v'
- 57: 1, # 'w'
- 58: 1, # 'x'
- 11: 3, # 'y'
- 22: 0, # 'z'
- 63: 1, # '·'
- 54: 0, # 'Ç'
- 50: 0, # 'Ö'
- 55: 0, # 'Ü'
- 59: 0, # 'â'
- 33: 2, # 'ç'
- 61: 0, # 'î'
- 34: 0, # 'ö'
- 17: 3, # 'ü'
- 30: 0, # 'ğ'
- 41: 0, # 'İ'
- 6: 3, # 'ı'
- 40: 0, # 'Ş'
- 19: 0, # 'ş'
- },
- 40: { # 'Ş'
- 23: 0, # 'A'
- 37: 0, # 'B'
- 47: 1, # 'C'
- 39: 1, # 'D'
- 29: 1, # 'E'
- 52: 0, # 'F'
- 36: 1, # 'G'
- 45: 2, # 'H'
- 53: 1, # 'I'
- 60: 0, # 'J'
- 16: 0, # 'K'
- 49: 0, # 'L'
- 20: 2, # 'M'
- 46: 1, # 'N'
- 42: 1, # 'O'
- 48: 2, # 'P'
- 44: 2, # 'R'
- 35: 1, # 'S'
- 31: 1, # 'T'
- 51: 0, # 'U'
- 38: 1, # 'V'
- 62: 0, # 'W'
- 43: 2, # 'Y'
- 56: 1, # 'Z'
- 1: 0, # 'a'
- 21: 2, # 'b'
- 28: 0, # 'c'
- 12: 2, # 'd'
- 2: 0, # 'e'
- 18: 3, # 'f'
- 27: 0, # 'g'
- 25: 2, # 'h'
- 3: 3, # 'i'
- 24: 2, # 'j'
- 10: 1, # 'k'
- 5: 0, # 'l'
- 13: 1, # 'm'
- 4: 3, # 'n'
- 15: 2, # 'o'
- 26: 0, # 'p'
- 7: 3, # 'r'
- 8: 2, # 's'
- 9: 2, # 't'
- 14: 1, # 'u'
- 32: 3, # 'v'
- 57: 0, # 'w'
- 58: 0, # 'x'
- 11: 2, # 'y'
- 22: 0, # 'z'
- 63: 0, # '·'
- 54: 0, # 'Ç'
- 50: 0, # 'Ö'
- 55: 1, # 'Ü'
- 59: 0, # 'â'
- 33: 0, # 'ç'
- 61: 0, # 'î'
- 34: 2, # 'ö'
- 17: 1, # 'ü'
- 30: 2, # 'ğ'
- 41: 0, # 'İ'
- 6: 2, # 'ı'
- 40: 1, # 'Ş'
- 19: 2, # 'ş'
- },
- 19: { # 'ş'
- 23: 0, # 'A'
- 37: 0, # 'B'
- 47: 1, # 'C'
- 39: 0, # 'D'
- 29: 0, # 'E'
- 52: 2, # 'F'
- 36: 1, # 'G'
- 45: 0, # 'H'
- 53: 0, # 'I'
- 60: 0, # 'J'
- 16: 3, # 'K'
- 49: 2, # 'L'
- 20: 0, # 'M'
- 46: 1, # 'N'
- 42: 1, # 'O'
- 48: 1, # 'P'
- 44: 1, # 'R'
- 35: 1, # 'S'
- 31: 0, # 'T'
- 51: 1, # 'U'
- 38: 1, # 'V'
- 62: 0, # 'W'
- 43: 1, # 'Y'
- 56: 0, # 'Z'
- 1: 3, # 'a'
- 21: 1, # 'b'
- 28: 2, # 'c'
- 12: 0, # 'd'
- 2: 3, # 'e'
- 18: 0, # 'f'
- 27: 2, # 'g'
- 25: 1, # 'h'
- 3: 1, # 'i'
- 24: 0, # 'j'
- 10: 2, # 'k'
- 5: 2, # 'l'
- 13: 3, # 'm'
- 4: 0, # 'n'
- 15: 0, # 'o'
- 26: 1, # 'p'
- 7: 3, # 'r'
- 8: 0, # 's'
- 9: 0, # 't'
- 14: 3, # 'u'
- 32: 0, # 'v'
- 57: 0, # 'w'
- 58: 0, # 'x'
- 11: 0, # 'y'
- 22: 2, # 'z'
- 63: 0, # '·'
- 54: 1, # 'Ç'
- 50: 2, # 'Ö'
- 55: 0, # 'Ü'
- 59: 0, # 'â'
- 33: 1, # 'ç'
- 61: 1, # 'î'
- 34: 2, # 'ö'
- 17: 0, # 'ü'
- 30: 1, # 'ğ'
- 41: 1, # 'İ'
- 6: 1, # 'ı'
- 40: 1, # 'Ş'
- 19: 1, # 'ş'
- },
-}
-
-# 255: Undefined characters that did not exist in training text
-# 254: Carriage/Return
-# 253: symbol (punctuation) that does not belong to word
-# 252: 0 - 9
-# 251: Control characters
-
-# Character Mapping Table(s):
-ISO_8859_9_TURKISH_CHAR_TO_ORDER = {
- 0: 255, # '\x00'
- 1: 255, # '\x01'
- 2: 255, # '\x02'
- 3: 255, # '\x03'
- 4: 255, # '\x04'
- 5: 255, # '\x05'
- 6: 255, # '\x06'
- 7: 255, # '\x07'
- 8: 255, # '\x08'
- 9: 255, # '\t'
- 10: 255, # '\n'
- 11: 255, # '\x0b'
- 12: 255, # '\x0c'
- 13: 255, # '\r'
- 14: 255, # '\x0e'
- 15: 255, # '\x0f'
- 16: 255, # '\x10'
- 17: 255, # '\x11'
- 18: 255, # '\x12'
- 19: 255, # '\x13'
- 20: 255, # '\x14'
- 21: 255, # '\x15'
- 22: 255, # '\x16'
- 23: 255, # '\x17'
- 24: 255, # '\x18'
- 25: 255, # '\x19'
- 26: 255, # '\x1a'
- 27: 255, # '\x1b'
- 28: 255, # '\x1c'
- 29: 255, # '\x1d'
- 30: 255, # '\x1e'
- 31: 255, # '\x1f'
- 32: 255, # ' '
- 33: 255, # '!'
- 34: 255, # '"'
- 35: 255, # '#'
- 36: 255, # '$'
- 37: 255, # '%'
- 38: 255, # '&'
- 39: 255, # "'"
- 40: 255, # '('
- 41: 255, # ')'
- 42: 255, # '*'
- 43: 255, # '+'
- 44: 255, # ','
- 45: 255, # '-'
- 46: 255, # '.'
- 47: 255, # '/'
- 48: 255, # '0'
- 49: 255, # '1'
- 50: 255, # '2'
- 51: 255, # '3'
- 52: 255, # '4'
- 53: 255, # '5'
- 54: 255, # '6'
- 55: 255, # '7'
- 56: 255, # '8'
- 57: 255, # '9'
- 58: 255, # ':'
- 59: 255, # ';'
- 60: 255, # '<'
- 61: 255, # '='
- 62: 255, # '>'
- 63: 255, # '?'
- 64: 255, # '@'
- 65: 23, # 'A'
- 66: 37, # 'B'
- 67: 47, # 'C'
- 68: 39, # 'D'
- 69: 29, # 'E'
- 70: 52, # 'F'
- 71: 36, # 'G'
- 72: 45, # 'H'
- 73: 53, # 'I'
- 74: 60, # 'J'
- 75: 16, # 'K'
- 76: 49, # 'L'
- 77: 20, # 'M'
- 78: 46, # 'N'
- 79: 42, # 'O'
- 80: 48, # 'P'
- 81: 69, # 'Q'
- 82: 44, # 'R'
- 83: 35, # 'S'
- 84: 31, # 'T'
- 85: 51, # 'U'
- 86: 38, # 'V'
- 87: 62, # 'W'
- 88: 65, # 'X'
- 89: 43, # 'Y'
- 90: 56, # 'Z'
- 91: 255, # '['
- 92: 255, # '\\'
- 93: 255, # ']'
- 94: 255, # '^'
- 95: 255, # '_'
- 96: 255, # '`'
- 97: 1, # 'a'
- 98: 21, # 'b'
- 99: 28, # 'c'
- 100: 12, # 'd'
- 101: 2, # 'e'
- 102: 18, # 'f'
- 103: 27, # 'g'
- 104: 25, # 'h'
- 105: 3, # 'i'
- 106: 24, # 'j'
- 107: 10, # 'k'
- 108: 5, # 'l'
- 109: 13, # 'm'
- 110: 4, # 'n'
- 111: 15, # 'o'
- 112: 26, # 'p'
- 113: 64, # 'q'
- 114: 7, # 'r'
- 115: 8, # 's'
- 116: 9, # 't'
- 117: 14, # 'u'
- 118: 32, # 'v'
- 119: 57, # 'w'
- 120: 58, # 'x'
- 121: 11, # 'y'
- 122: 22, # 'z'
- 123: 255, # '{'
- 124: 255, # '|'
- 125: 255, # '}'
- 126: 255, # '~'
- 127: 255, # '\x7f'
- 128: 180, # '\x80'
- 129: 179, # '\x81'
- 130: 178, # '\x82'
- 131: 177, # '\x83'
- 132: 176, # '\x84'
- 133: 175, # '\x85'
- 134: 174, # '\x86'
- 135: 173, # '\x87'
- 136: 172, # '\x88'
- 137: 171, # '\x89'
- 138: 170, # '\x8a'
- 139: 169, # '\x8b'
- 140: 168, # '\x8c'
- 141: 167, # '\x8d'
- 142: 166, # '\x8e'
- 143: 165, # '\x8f'
- 144: 164, # '\x90'
- 145: 163, # '\x91'
- 146: 162, # '\x92'
- 147: 161, # '\x93'
- 148: 160, # '\x94'
- 149: 159, # '\x95'
- 150: 101, # '\x96'
- 151: 158, # '\x97'
- 152: 157, # '\x98'
- 153: 156, # '\x99'
- 154: 155, # '\x9a'
- 155: 154, # '\x9b'
- 156: 153, # '\x9c'
- 157: 152, # '\x9d'
- 158: 151, # '\x9e'
- 159: 106, # '\x9f'
- 160: 150, # '\xa0'
- 161: 149, # '¡'
- 162: 148, # '¢'
- 163: 147, # '£'
- 164: 146, # '¤'
- 165: 145, # '¥'
- 166: 144, # '¦'
- 167: 100, # '§'
- 168: 143, # '¨'
- 169: 142, # '©'
- 170: 141, # 'ª'
- 171: 140, # '«'
- 172: 139, # '¬'
- 173: 138, # '\xad'
- 174: 137, # '®'
- 175: 136, # '¯'
- 176: 94, # '°'
- 177: 80, # '±'
- 178: 93, # '²'
- 179: 135, # '³'
- 180: 105, # '´'
- 181: 134, # 'µ'
- 182: 133, # '¶'
- 183: 63, # '·'
- 184: 132, # '¸'
- 185: 131, # '¹'
- 186: 130, # 'º'
- 187: 129, # '»'
- 188: 128, # '¼'
- 189: 127, # '½'
- 190: 126, # '¾'
- 191: 125, # '¿'
- 192: 124, # 'À'
- 193: 104, # 'Á'
- 194: 73, # 'Â'
- 195: 99, # 'Ã'
- 196: 79, # 'Ä'
- 197: 85, # 'Å'
- 198: 123, # 'Æ'
- 199: 54, # 'Ç'
- 200: 122, # 'È'
- 201: 98, # 'É'
- 202: 92, # 'Ê'
- 203: 121, # 'Ë'
- 204: 120, # 'Ì'
- 205: 91, # 'Í'
- 206: 103, # 'Î'
- 207: 119, # 'Ï'
- 208: 68, # 'Ğ'
- 209: 118, # 'Ñ'
- 210: 117, # 'Ò'
- 211: 97, # 'Ó'
- 212: 116, # 'Ô'
- 213: 115, # 'Õ'
- 214: 50, # 'Ö'
- 215: 90, # '×'
- 216: 114, # 'Ø'
- 217: 113, # 'Ù'
- 218: 112, # 'Ú'
- 219: 111, # 'Û'
- 220: 55, # 'Ü'
- 221: 41, # 'İ'
- 222: 40, # 'Ş'
- 223: 86, # 'ß'
- 224: 89, # 'à'
- 225: 70, # 'á'
- 226: 59, # 'â'
- 227: 78, # 'ã'
- 228: 71, # 'ä'
- 229: 82, # 'å'
- 230: 88, # 'æ'
- 231: 33, # 'ç'
- 232: 77, # 'è'
- 233: 66, # 'é'
- 234: 84, # 'ê'
- 235: 83, # 'ë'
- 236: 110, # 'ì'
- 237: 75, # 'í'
- 238: 61, # 'î'
- 239: 96, # 'ï'
- 240: 30, # 'ğ'
- 241: 67, # 'ñ'
- 242: 109, # 'ò'
- 243: 74, # 'ó'
- 244: 87, # 'ô'
- 245: 102, # 'õ'
- 246: 34, # 'ö'
- 247: 95, # '÷'
- 248: 81, # 'ø'
- 249: 108, # 'ù'
- 250: 76, # 'ú'
- 251: 72, # 'û'
- 252: 17, # 'ü'
- 253: 6, # 'ı'
- 254: 19, # 'ş'
- 255: 107, # 'ÿ'
-}
-
-ISO_8859_9_TURKISH_MODEL = SingleByteCharSetModel(charset_name='ISO-8859-9',
- language='Turkish',
- char_to_order_map=ISO_8859_9_TURKISH_CHAR_TO_ORDER,
- language_model=TURKISH_LANG_MODEL,
- typical_positive_ratio=0.97029,
- keep_ascii_letters=True,
- alphabet='ABCDEFGHIJKLMNOPRSTUVYZabcdefghijklmnoprstuvyzÂÇÎÖÛÜâçîöûüĞğİıŞş')
-
diff --git a/spaces/alexray/btc_predictor/venv/lib/python3.10/site-packages/pip/_vendor/idna/uts46data.py b/spaces/alexray/btc_predictor/venv/lib/python3.10/site-packages/pip/_vendor/idna/uts46data.py
deleted file mode 100644
index 8f65705ee91fcf56d8eaf8d538a86d3e5d457d51..0000000000000000000000000000000000000000
--- a/spaces/alexray/btc_predictor/venv/lib/python3.10/site-packages/pip/_vendor/idna/uts46data.py
+++ /dev/null
@@ -1,8512 +0,0 @@
-# This file is automatically generated by tools/idna-data
-# vim: set fileencoding=utf-8 :
-
-from typing import List, Tuple, Union
-
-
-"""IDNA Mapping Table from UTS46."""
-
-
-__version__ = '14.0.0'
-def _seg_0() -> List[Union[Tuple[int, str], Tuple[int, str, str]]]:
- return [
- (0x0, '3'),
- (0x1, '3'),
- (0x2, '3'),
- (0x3, '3'),
- (0x4, '3'),
- (0x5, '3'),
- (0x6, '3'),
- (0x7, '3'),
- (0x8, '3'),
- (0x9, '3'),
- (0xA, '3'),
- (0xB, '3'),
- (0xC, '3'),
- (0xD, '3'),
- (0xE, '3'),
- (0xF, '3'),
- (0x10, '3'),
- (0x11, '3'),
- (0x12, '3'),
- (0x13, '3'),
- (0x14, '3'),
- (0x15, '3'),
- (0x16, '3'),
- (0x17, '3'),
- (0x18, '3'),
- (0x19, '3'),
- (0x1A, '3'),
- (0x1B, '3'),
- (0x1C, '3'),
- (0x1D, '3'),
- (0x1E, '3'),
- (0x1F, '3'),
- (0x20, '3'),
- (0x21, '3'),
- (0x22, '3'),
- (0x23, '3'),
- (0x24, '3'),
- (0x25, '3'),
- (0x26, '3'),
- (0x27, '3'),
- (0x28, '3'),
- (0x29, '3'),
- (0x2A, '3'),
- (0x2B, '3'),
- (0x2C, '3'),
- (0x2D, 'V'),
- (0x2E, 'V'),
- (0x2F, '3'),
- (0x30, 'V'),
- (0x31, 'V'),
- (0x32, 'V'),
- (0x33, 'V'),
- (0x34, 'V'),
- (0x35, 'V'),
- (0x36, 'V'),
- (0x37, 'V'),
- (0x38, 'V'),
- (0x39, 'V'),
- (0x3A, '3'),
- (0x3B, '3'),
- (0x3C, '3'),
- (0x3D, '3'),
- (0x3E, '3'),
- (0x3F, '3'),
- (0x40, '3'),
- (0x41, 'M', 'a'),
- (0x42, 'M', 'b'),
- (0x43, 'M', 'c'),
- (0x44, 'M', 'd'),
- (0x45, 'M', 'e'),
- (0x46, 'M', 'f'),
- (0x47, 'M', 'g'),
- (0x48, 'M', 'h'),
- (0x49, 'M', 'i'),
- (0x4A, 'M', 'j'),
- (0x4B, 'M', 'k'),
- (0x4C, 'M', 'l'),
- (0x4D, 'M', 'm'),
- (0x4E, 'M', 'n'),
- (0x4F, 'M', 'o'),
- (0x50, 'M', 'p'),
- (0x51, 'M', 'q'),
- (0x52, 'M', 'r'),
- (0x53, 'M', 's'),
- (0x54, 'M', 't'),
- (0x55, 'M', 'u'),
- (0x56, 'M', 'v'),
- (0x57, 'M', 'w'),
- (0x58, 'M', 'x'),
- (0x59, 'M', 'y'),
- (0x5A, 'M', 'z'),
- (0x5B, '3'),
- (0x5C, '3'),
- (0x5D, '3'),
- (0x5E, '3'),
- (0x5F, '3'),
- (0x60, '3'),
- (0x61, 'V'),
- (0x62, 'V'),
- (0x63, 'V'),
- ]
-
-def _seg_1() -> List[Union[Tuple[int, str], Tuple[int, str, str]]]:
- return [
- (0x64, 'V'),
- (0x65, 'V'),
- (0x66, 'V'),
- (0x67, 'V'),
- (0x68, 'V'),
- (0x69, 'V'),
- (0x6A, 'V'),
- (0x6B, 'V'),
- (0x6C, 'V'),
- (0x6D, 'V'),
- (0x6E, 'V'),
- (0x6F, 'V'),
- (0x70, 'V'),
- (0x71, 'V'),
- (0x72, 'V'),
- (0x73, 'V'),
- (0x74, 'V'),
- (0x75, 'V'),
- (0x76, 'V'),
- (0x77, 'V'),
- (0x78, 'V'),
- (0x79, 'V'),
- (0x7A, 'V'),
- (0x7B, '3'),
- (0x7C, '3'),
- (0x7D, '3'),
- (0x7E, '3'),
- (0x7F, '3'),
- (0x80, 'X'),
- (0x81, 'X'),
- (0x82, 'X'),
- (0x83, 'X'),
- (0x84, 'X'),
- (0x85, 'X'),
- (0x86, 'X'),
- (0x87, 'X'),
- (0x88, 'X'),
- (0x89, 'X'),
- (0x8A, 'X'),
- (0x8B, 'X'),
- (0x8C, 'X'),
- (0x8D, 'X'),
- (0x8E, 'X'),
- (0x8F, 'X'),
- (0x90, 'X'),
- (0x91, 'X'),
- (0x92, 'X'),
- (0x93, 'X'),
- (0x94, 'X'),
- (0x95, 'X'),
- (0x96, 'X'),
- (0x97, 'X'),
- (0x98, 'X'),
- (0x99, 'X'),
- (0x9A, 'X'),
- (0x9B, 'X'),
- (0x9C, 'X'),
- (0x9D, 'X'),
- (0x9E, 'X'),
- (0x9F, 'X'),
- (0xA0, '3', ' '),
- (0xA1, 'V'),
- (0xA2, 'V'),
- (0xA3, 'V'),
- (0xA4, 'V'),
- (0xA5, 'V'),
- (0xA6, 'V'),
- (0xA7, 'V'),
- (0xA8, '3', ' ̈'),
- (0xA9, 'V'),
- (0xAA, 'M', 'a'),
- (0xAB, 'V'),
- (0xAC, 'V'),
- (0xAD, 'I'),
- (0xAE, 'V'),
- (0xAF, '3', ' ̄'),
- (0xB0, 'V'),
- (0xB1, 'V'),
- (0xB2, 'M', '2'),
- (0xB3, 'M', '3'),
- (0xB4, '3', ' ́'),
- (0xB5, 'M', 'μ'),
- (0xB6, 'V'),
- (0xB7, 'V'),
- (0xB8, '3', ' ̧'),
- (0xB9, 'M', '1'),
- (0xBA, 'M', 'o'),
- (0xBB, 'V'),
- (0xBC, 'M', '1⁄4'),
- (0xBD, 'M', '1⁄2'),
- (0xBE, 'M', '3⁄4'),
- (0xBF, 'V'),
- (0xC0, 'M', 'à'),
- (0xC1, 'M', 'á'),
- (0xC2, 'M', 'â'),
- (0xC3, 'M', 'ã'),
- (0xC4, 'M', 'ä'),
- (0xC5, 'M', 'å'),
- (0xC6, 'M', 'æ'),
- (0xC7, 'M', 'ç'),
- ]
-
-def _seg_2() -> List[Union[Tuple[int, str], Tuple[int, str, str]]]:
- return [
- (0xC8, 'M', 'è'),
- (0xC9, 'M', 'é'),
- (0xCA, 'M', 'ê'),
- (0xCB, 'M', 'ë'),
- (0xCC, 'M', 'ì'),
- (0xCD, 'M', 'í'),
- (0xCE, 'M', 'î'),
- (0xCF, 'M', 'ï'),
- (0xD0, 'M', 'ð'),
- (0xD1, 'M', 'ñ'),
- (0xD2, 'M', 'ò'),
- (0xD3, 'M', 'ó'),
- (0xD4, 'M', 'ô'),
- (0xD5, 'M', 'õ'),
- (0xD6, 'M', 'ö'),
- (0xD7, 'V'),
- (0xD8, 'M', 'ø'),
- (0xD9, 'M', 'ù'),
- (0xDA, 'M', 'ú'),
- (0xDB, 'M', 'û'),
- (0xDC, 'M', 'ü'),
- (0xDD, 'M', 'ý'),
- (0xDE, 'M', 'þ'),
- (0xDF, 'D', 'ss'),
- (0xE0, 'V'),
- (0xE1, 'V'),
- (0xE2, 'V'),
- (0xE3, 'V'),
- (0xE4, 'V'),
- (0xE5, 'V'),
- (0xE6, 'V'),
- (0xE7, 'V'),
- (0xE8, 'V'),
- (0xE9, 'V'),
- (0xEA, 'V'),
- (0xEB, 'V'),
- (0xEC, 'V'),
- (0xED, 'V'),
- (0xEE, 'V'),
- (0xEF, 'V'),
- (0xF0, 'V'),
- (0xF1, 'V'),
- (0xF2, 'V'),
- (0xF3, 'V'),
- (0xF4, 'V'),
- (0xF5, 'V'),
- (0xF6, 'V'),
- (0xF7, 'V'),
- (0xF8, 'V'),
- (0xF9, 'V'),
- (0xFA, 'V'),
- (0xFB, 'V'),
- (0xFC, 'V'),
- (0xFD, 'V'),
- (0xFE, 'V'),
- (0xFF, 'V'),
- (0x100, 'M', 'ā'),
- (0x101, 'V'),
- (0x102, 'M', 'ă'),
- (0x103, 'V'),
- (0x104, 'M', 'ą'),
- (0x105, 'V'),
- (0x106, 'M', 'ć'),
- (0x107, 'V'),
- (0x108, 'M', 'ĉ'),
- (0x109, 'V'),
- (0x10A, 'M', 'ċ'),
- (0x10B, 'V'),
- (0x10C, 'M', 'č'),
- (0x10D, 'V'),
- (0x10E, 'M', 'ď'),
- (0x10F, 'V'),
- (0x110, 'M', 'đ'),
- (0x111, 'V'),
- (0x112, 'M', 'ē'),
- (0x113, 'V'),
- (0x114, 'M', 'ĕ'),
- (0x115, 'V'),
- (0x116, 'M', 'ė'),
- (0x117, 'V'),
- (0x118, 'M', 'ę'),
- (0x119, 'V'),
- (0x11A, 'M', 'ě'),
- (0x11B, 'V'),
- (0x11C, 'M', 'ĝ'),
- (0x11D, 'V'),
- (0x11E, 'M', 'ğ'),
- (0x11F, 'V'),
- (0x120, 'M', 'ġ'),
- (0x121, 'V'),
- (0x122, 'M', 'ģ'),
- (0x123, 'V'),
- (0x124, 'M', 'ĥ'),
- (0x125, 'V'),
- (0x126, 'M', 'ħ'),
- (0x127, 'V'),
- (0x128, 'M', 'ĩ'),
- (0x129, 'V'),
- (0x12A, 'M', 'ī'),
- (0x12B, 'V'),
- ]
-
-def _seg_3() -> List[Union[Tuple[int, str], Tuple[int, str, str]]]:
- return [
- (0x12C, 'M', 'ĭ'),
- (0x12D, 'V'),
- (0x12E, 'M', 'į'),
- (0x12F, 'V'),
- (0x130, 'M', 'i̇'),
- (0x131, 'V'),
- (0x132, 'M', 'ij'),
- (0x134, 'M', 'ĵ'),
- (0x135, 'V'),
- (0x136, 'M', 'ķ'),
- (0x137, 'V'),
- (0x139, 'M', 'ĺ'),
- (0x13A, 'V'),
- (0x13B, 'M', 'ļ'),
- (0x13C, 'V'),
- (0x13D, 'M', 'ľ'),
- (0x13E, 'V'),
- (0x13F, 'M', 'l·'),
- (0x141, 'M', 'ł'),
- (0x142, 'V'),
- (0x143, 'M', 'ń'),
- (0x144, 'V'),
- (0x145, 'M', 'ņ'),
- (0x146, 'V'),
- (0x147, 'M', 'ň'),
- (0x148, 'V'),
- (0x149, 'M', 'ʼn'),
- (0x14A, 'M', 'ŋ'),
- (0x14B, 'V'),
- (0x14C, 'M', 'ō'),
- (0x14D, 'V'),
- (0x14E, 'M', 'ŏ'),
- (0x14F, 'V'),
- (0x150, 'M', 'ő'),
- (0x151, 'V'),
- (0x152, 'M', 'œ'),
- (0x153, 'V'),
- (0x154, 'M', 'ŕ'),
- (0x155, 'V'),
- (0x156, 'M', 'ŗ'),
- (0x157, 'V'),
- (0x158, 'M', 'ř'),
- (0x159, 'V'),
- (0x15A, 'M', 'ś'),
- (0x15B, 'V'),
- (0x15C, 'M', 'ŝ'),
- (0x15D, 'V'),
- (0x15E, 'M', 'ş'),
- (0x15F, 'V'),
- (0x160, 'M', 'š'),
- (0x161, 'V'),
- (0x162, 'M', 'ţ'),
- (0x163, 'V'),
- (0x164, 'M', 'ť'),
- (0x165, 'V'),
- (0x166, 'M', 'ŧ'),
- (0x167, 'V'),
- (0x168, 'M', 'ũ'),
- (0x169, 'V'),
- (0x16A, 'M', 'ū'),
- (0x16B, 'V'),
- (0x16C, 'M', 'ŭ'),
- (0x16D, 'V'),
- (0x16E, 'M', 'ů'),
- (0x16F, 'V'),
- (0x170, 'M', 'ű'),
- (0x171, 'V'),
- (0x172, 'M', 'ų'),
- (0x173, 'V'),
- (0x174, 'M', 'ŵ'),
- (0x175, 'V'),
- (0x176, 'M', 'ŷ'),
- (0x177, 'V'),
- (0x178, 'M', 'ÿ'),
- (0x179, 'M', 'ź'),
- (0x17A, 'V'),
- (0x17B, 'M', 'ż'),
- (0x17C, 'V'),
- (0x17D, 'M', 'ž'),
- (0x17E, 'V'),
- (0x17F, 'M', 's'),
- (0x180, 'V'),
- (0x181, 'M', 'ɓ'),
- (0x182, 'M', 'ƃ'),
- (0x183, 'V'),
- (0x184, 'M', 'ƅ'),
- (0x185, 'V'),
- (0x186, 'M', 'ɔ'),
- (0x187, 'M', 'ƈ'),
- (0x188, 'V'),
- (0x189, 'M', 'ɖ'),
- (0x18A, 'M', 'ɗ'),
- (0x18B, 'M', 'ƌ'),
- (0x18C, 'V'),
- (0x18E, 'M', 'ǝ'),
- (0x18F, 'M', 'ə'),
- (0x190, 'M', 'ɛ'),
- (0x191, 'M', 'ƒ'),
- (0x192, 'V'),
- (0x193, 'M', 'ɠ'),
- ]
-
-def _seg_4() -> List[Union[Tuple[int, str], Tuple[int, str, str]]]:
- return [
- (0x194, 'M', 'ɣ'),
- (0x195, 'V'),
- (0x196, 'M', 'ɩ'),
- (0x197, 'M', 'ɨ'),
- (0x198, 'M', 'ƙ'),
- (0x199, 'V'),
- (0x19C, 'M', 'ɯ'),
- (0x19D, 'M', 'ɲ'),
- (0x19E, 'V'),
- (0x19F, 'M', 'ɵ'),
- (0x1A0, 'M', 'ơ'),
- (0x1A1, 'V'),
- (0x1A2, 'M', 'ƣ'),
- (0x1A3, 'V'),
- (0x1A4, 'M', 'ƥ'),
- (0x1A5, 'V'),
- (0x1A6, 'M', 'ʀ'),
- (0x1A7, 'M', 'ƨ'),
- (0x1A8, 'V'),
- (0x1A9, 'M', 'ʃ'),
- (0x1AA, 'V'),
- (0x1AC, 'M', 'ƭ'),
- (0x1AD, 'V'),
- (0x1AE, 'M', 'ʈ'),
- (0x1AF, 'M', 'ư'),
- (0x1B0, 'V'),
- (0x1B1, 'M', 'ʊ'),
- (0x1B2, 'M', 'ʋ'),
- (0x1B3, 'M', 'ƴ'),
- (0x1B4, 'V'),
- (0x1B5, 'M', 'ƶ'),
- (0x1B6, 'V'),
- (0x1B7, 'M', 'ʒ'),
- (0x1B8, 'M', 'ƹ'),
- (0x1B9, 'V'),
- (0x1BC, 'M', 'ƽ'),
- (0x1BD, 'V'),
- (0x1C4, 'M', 'dž'),
- (0x1C7, 'M', 'lj'),
- (0x1CA, 'M', 'nj'),
- (0x1CD, 'M', 'ǎ'),
- (0x1CE, 'V'),
- (0x1CF, 'M', 'ǐ'),
- (0x1D0, 'V'),
- (0x1D1, 'M', 'ǒ'),
- (0x1D2, 'V'),
- (0x1D3, 'M', 'ǔ'),
- (0x1D4, 'V'),
- (0x1D5, 'M', 'ǖ'),
- (0x1D6, 'V'),
- (0x1D7, 'M', 'ǘ'),
- (0x1D8, 'V'),
- (0x1D9, 'M', 'ǚ'),
- (0x1DA, 'V'),
- (0x1DB, 'M', 'ǜ'),
- (0x1DC, 'V'),
- (0x1DE, 'M', 'ǟ'),
- (0x1DF, 'V'),
- (0x1E0, 'M', 'ǡ'),
- (0x1E1, 'V'),
- (0x1E2, 'M', 'ǣ'),
- (0x1E3, 'V'),
- (0x1E4, 'M', 'ǥ'),
- (0x1E5, 'V'),
- (0x1E6, 'M', 'ǧ'),
- (0x1E7, 'V'),
- (0x1E8, 'M', 'ǩ'),
- (0x1E9, 'V'),
- (0x1EA, 'M', 'ǫ'),
- (0x1EB, 'V'),
- (0x1EC, 'M', 'ǭ'),
- (0x1ED, 'V'),
- (0x1EE, 'M', 'ǯ'),
- (0x1EF, 'V'),
- (0x1F1, 'M', 'dz'),
- (0x1F4, 'M', 'ǵ'),
- (0x1F5, 'V'),
- (0x1F6, 'M', 'ƕ'),
- (0x1F7, 'M', 'ƿ'),
- (0x1F8, 'M', 'ǹ'),
- (0x1F9, 'V'),
- (0x1FA, 'M', 'ǻ'),
- (0x1FB, 'V'),
- (0x1FC, 'M', 'ǽ'),
- (0x1FD, 'V'),
- (0x1FE, 'M', 'ǿ'),
- (0x1FF, 'V'),
- (0x200, 'M', 'ȁ'),
- (0x201, 'V'),
- (0x202, 'M', 'ȃ'),
- (0x203, 'V'),
- (0x204, 'M', 'ȅ'),
- (0x205, 'V'),
- (0x206, 'M', 'ȇ'),
- (0x207, 'V'),
- (0x208, 'M', 'ȉ'),
- (0x209, 'V'),
- (0x20A, 'M', 'ȋ'),
- (0x20B, 'V'),
- (0x20C, 'M', 'ȍ'),
- ]
-
-def _seg_5() -> List[Union[Tuple[int, str], Tuple[int, str, str]]]:
- return [
- (0x20D, 'V'),
- (0x20E, 'M', 'ȏ'),
- (0x20F, 'V'),
- (0x210, 'M', 'ȑ'),
- (0x211, 'V'),
- (0x212, 'M', 'ȓ'),
- (0x213, 'V'),
- (0x214, 'M', 'ȕ'),
- (0x215, 'V'),
- (0x216, 'M', 'ȗ'),
- (0x217, 'V'),
- (0x218, 'M', 'ș'),
- (0x219, 'V'),
- (0x21A, 'M', 'ț'),
- (0x21B, 'V'),
- (0x21C, 'M', 'ȝ'),
- (0x21D, 'V'),
- (0x21E, 'M', 'ȟ'),
- (0x21F, 'V'),
- (0x220, 'M', 'ƞ'),
- (0x221, 'V'),
- (0x222, 'M', 'ȣ'),
- (0x223, 'V'),
- (0x224, 'M', 'ȥ'),
- (0x225, 'V'),
- (0x226, 'M', 'ȧ'),
- (0x227, 'V'),
- (0x228, 'M', 'ȩ'),
- (0x229, 'V'),
- (0x22A, 'M', 'ȫ'),
- (0x22B, 'V'),
- (0x22C, 'M', 'ȭ'),
- (0x22D, 'V'),
- (0x22E, 'M', 'ȯ'),
- (0x22F, 'V'),
- (0x230, 'M', 'ȱ'),
- (0x231, 'V'),
- (0x232, 'M', 'ȳ'),
- (0x233, 'V'),
- (0x23A, 'M', 'ⱥ'),
- (0x23B, 'M', 'ȼ'),
- (0x23C, 'V'),
- (0x23D, 'M', 'ƚ'),
- (0x23E, 'M', 'ⱦ'),
- (0x23F, 'V'),
- (0x241, 'M', 'ɂ'),
- (0x242, 'V'),
- (0x243, 'M', 'ƀ'),
- (0x244, 'M', 'ʉ'),
- (0x245, 'M', 'ʌ'),
- (0x246, 'M', 'ɇ'),
- (0x247, 'V'),
- (0x248, 'M', 'ɉ'),
- (0x249, 'V'),
- (0x24A, 'M', 'ɋ'),
- (0x24B, 'V'),
- (0x24C, 'M', 'ɍ'),
- (0x24D, 'V'),
- (0x24E, 'M', 'ɏ'),
- (0x24F, 'V'),
- (0x2B0, 'M', 'h'),
- (0x2B1, 'M', 'ɦ'),
- (0x2B2, 'M', 'j'),
- (0x2B3, 'M', 'r'),
- (0x2B4, 'M', 'ɹ'),
- (0x2B5, 'M', 'ɻ'),
- (0x2B6, 'M', 'ʁ'),
- (0x2B7, 'M', 'w'),
- (0x2B8, 'M', 'y'),
- (0x2B9, 'V'),
- (0x2D8, '3', ' ̆'),
- (0x2D9, '3', ' ̇'),
- (0x2DA, '3', ' ̊'),
- (0x2DB, '3', ' ̨'),
- (0x2DC, '3', ' ̃'),
- (0x2DD, '3', ' ̋'),
- (0x2DE, 'V'),
- (0x2E0, 'M', 'ɣ'),
- (0x2E1, 'M', 'l'),
- (0x2E2, 'M', 's'),
- (0x2E3, 'M', 'x'),
- (0x2E4, 'M', 'ʕ'),
- (0x2E5, 'V'),
- (0x340, 'M', '̀'),
- (0x341, 'M', '́'),
- (0x342, 'V'),
- (0x343, 'M', '̓'),
- (0x344, 'M', '̈́'),
- (0x345, 'M', 'ι'),
- (0x346, 'V'),
- (0x34F, 'I'),
- (0x350, 'V'),
- (0x370, 'M', 'ͱ'),
- (0x371, 'V'),
- (0x372, 'M', 'ͳ'),
- (0x373, 'V'),
- (0x374, 'M', 'ʹ'),
- (0x375, 'V'),
- (0x376, 'M', 'ͷ'),
- (0x377, 'V'),
- ]
-
-def _seg_6() -> List[Union[Tuple[int, str], Tuple[int, str, str]]]:
- return [
- (0x378, 'X'),
- (0x37A, '3', ' ι'),
- (0x37B, 'V'),
- (0x37E, '3', ';'),
- (0x37F, 'M', 'ϳ'),
- (0x380, 'X'),
- (0x384, '3', ' ́'),
- (0x385, '3', ' ̈́'),
- (0x386, 'M', 'ά'),
- (0x387, 'M', '·'),
- (0x388, 'M', 'έ'),
- (0x389, 'M', 'ή'),
- (0x38A, 'M', 'ί'),
- (0x38B, 'X'),
- (0x38C, 'M', 'ό'),
- (0x38D, 'X'),
- (0x38E, 'M', 'ύ'),
- (0x38F, 'M', 'ώ'),
- (0x390, 'V'),
- (0x391, 'M', 'α'),
- (0x392, 'M', 'β'),
- (0x393, 'M', 'γ'),
- (0x394, 'M', 'δ'),
- (0x395, 'M', 'ε'),
- (0x396, 'M', 'ζ'),
- (0x397, 'M', 'η'),
- (0x398, 'M', 'θ'),
- (0x399, 'M', 'ι'),
- (0x39A, 'M', 'κ'),
- (0x39B, 'M', 'λ'),
- (0x39C, 'M', 'μ'),
- (0x39D, 'M', 'ν'),
- (0x39E, 'M', 'ξ'),
- (0x39F, 'M', 'ο'),
- (0x3A0, 'M', 'π'),
- (0x3A1, 'M', 'ρ'),
- (0x3A2, 'X'),
- (0x3A3, 'M', 'σ'),
- (0x3A4, 'M', 'τ'),
- (0x3A5, 'M', 'υ'),
- (0x3A6, 'M', 'φ'),
- (0x3A7, 'M', 'χ'),
- (0x3A8, 'M', 'ψ'),
- (0x3A9, 'M', 'ω'),
- (0x3AA, 'M', 'ϊ'),
- (0x3AB, 'M', 'ϋ'),
- (0x3AC, 'V'),
- (0x3C2, 'D', 'σ'),
- (0x3C3, 'V'),
- (0x3CF, 'M', 'ϗ'),
- (0x3D0, 'M', 'β'),
- (0x3D1, 'M', 'θ'),
- (0x3D2, 'M', 'υ'),
- (0x3D3, 'M', 'ύ'),
- (0x3D4, 'M', 'ϋ'),
- (0x3D5, 'M', 'φ'),
- (0x3D6, 'M', 'π'),
- (0x3D7, 'V'),
- (0x3D8, 'M', 'ϙ'),
- (0x3D9, 'V'),
- (0x3DA, 'M', 'ϛ'),
- (0x3DB, 'V'),
- (0x3DC, 'M', 'ϝ'),
- (0x3DD, 'V'),
- (0x3DE, 'M', 'ϟ'),
- (0x3DF, 'V'),
- (0x3E0, 'M', 'ϡ'),
- (0x3E1, 'V'),
- (0x3E2, 'M', 'ϣ'),
- (0x3E3, 'V'),
- (0x3E4, 'M', 'ϥ'),
- (0x3E5, 'V'),
- (0x3E6, 'M', 'ϧ'),
- (0x3E7, 'V'),
- (0x3E8, 'M', 'ϩ'),
- (0x3E9, 'V'),
- (0x3EA, 'M', 'ϫ'),
- (0x3EB, 'V'),
- (0x3EC, 'M', 'ϭ'),
- (0x3ED, 'V'),
- (0x3EE, 'M', 'ϯ'),
- (0x3EF, 'V'),
- (0x3F0, 'M', 'κ'),
- (0x3F1, 'M', 'ρ'),
- (0x3F2, 'M', 'σ'),
- (0x3F3, 'V'),
- (0x3F4, 'M', 'θ'),
- (0x3F5, 'M', 'ε'),
- (0x3F6, 'V'),
- (0x3F7, 'M', 'ϸ'),
- (0x3F8, 'V'),
- (0x3F9, 'M', 'σ'),
- (0x3FA, 'M', 'ϻ'),
- (0x3FB, 'V'),
- (0x3FD, 'M', 'ͻ'),
- (0x3FE, 'M', 'ͼ'),
- (0x3FF, 'M', 'ͽ'),
- (0x400, 'M', 'ѐ'),
- (0x401, 'M', 'ё'),
- (0x402, 'M', 'ђ'),
- ]
-
-def _seg_7() -> List[Union[Tuple[int, str], Tuple[int, str, str]]]:
- return [
- (0x403, 'M', 'ѓ'),
- (0x404, 'M', 'є'),
- (0x405, 'M', 'ѕ'),
- (0x406, 'M', 'і'),
- (0x407, 'M', 'ї'),
- (0x408, 'M', 'ј'),
- (0x409, 'M', 'љ'),
- (0x40A, 'M', 'њ'),
- (0x40B, 'M', 'ћ'),
- (0x40C, 'M', 'ќ'),
- (0x40D, 'M', 'ѝ'),
- (0x40E, 'M', 'ў'),
- (0x40F, 'M', 'џ'),
- (0x410, 'M', 'а'),
- (0x411, 'M', 'б'),
- (0x412, 'M', 'в'),
- (0x413, 'M', 'г'),
- (0x414, 'M', 'д'),
- (0x415, 'M', 'е'),
- (0x416, 'M', 'ж'),
- (0x417, 'M', 'з'),
- (0x418, 'M', 'и'),
- (0x419, 'M', 'й'),
- (0x41A, 'M', 'к'),
- (0x41B, 'M', 'л'),
- (0x41C, 'M', 'м'),
- (0x41D, 'M', 'н'),
- (0x41E, 'M', 'о'),
- (0x41F, 'M', 'п'),
- (0x420, 'M', 'р'),
- (0x421, 'M', 'с'),
- (0x422, 'M', 'т'),
- (0x423, 'M', 'у'),
- (0x424, 'M', 'ф'),
- (0x425, 'M', 'х'),
- (0x426, 'M', 'ц'),
- (0x427, 'M', 'ч'),
- (0x428, 'M', 'ш'),
- (0x429, 'M', 'щ'),
- (0x42A, 'M', 'ъ'),
- (0x42B, 'M', 'ы'),
- (0x42C, 'M', 'ь'),
- (0x42D, 'M', 'э'),
- (0x42E, 'M', 'ю'),
- (0x42F, 'M', 'я'),
- (0x430, 'V'),
- (0x460, 'M', 'ѡ'),
- (0x461, 'V'),
- (0x462, 'M', 'ѣ'),
- (0x463, 'V'),
- (0x464, 'M', 'ѥ'),
- (0x465, 'V'),
- (0x466, 'M', 'ѧ'),
- (0x467, 'V'),
- (0x468, 'M', 'ѩ'),
- (0x469, 'V'),
- (0x46A, 'M', 'ѫ'),
- (0x46B, 'V'),
- (0x46C, 'M', 'ѭ'),
- (0x46D, 'V'),
- (0x46E, 'M', 'ѯ'),
- (0x46F, 'V'),
- (0x470, 'M', 'ѱ'),
- (0x471, 'V'),
- (0x472, 'M', 'ѳ'),
- (0x473, 'V'),
- (0x474, 'M', 'ѵ'),
- (0x475, 'V'),
- (0x476, 'M', 'ѷ'),
- (0x477, 'V'),
- (0x478, 'M', 'ѹ'),
- (0x479, 'V'),
- (0x47A, 'M', 'ѻ'),
- (0x47B, 'V'),
- (0x47C, 'M', 'ѽ'),
- (0x47D, 'V'),
- (0x47E, 'M', 'ѿ'),
- (0x47F, 'V'),
- (0x480, 'M', 'ҁ'),
- (0x481, 'V'),
- (0x48A, 'M', 'ҋ'),
- (0x48B, 'V'),
- (0x48C, 'M', 'ҍ'),
- (0x48D, 'V'),
- (0x48E, 'M', 'ҏ'),
- (0x48F, 'V'),
- (0x490, 'M', 'ґ'),
- (0x491, 'V'),
- (0x492, 'M', 'ғ'),
- (0x493, 'V'),
- (0x494, 'M', 'ҕ'),
- (0x495, 'V'),
- (0x496, 'M', 'җ'),
- (0x497, 'V'),
- (0x498, 'M', 'ҙ'),
- (0x499, 'V'),
- (0x49A, 'M', 'қ'),
- (0x49B, 'V'),
- (0x49C, 'M', 'ҝ'),
- (0x49D, 'V'),
- ]
-
-def _seg_8() -> List[Union[Tuple[int, str], Tuple[int, str, str]]]:
- return [
- (0x49E, 'M', 'ҟ'),
- (0x49F, 'V'),
- (0x4A0, 'M', 'ҡ'),
- (0x4A1, 'V'),
- (0x4A2, 'M', 'ң'),
- (0x4A3, 'V'),
- (0x4A4, 'M', 'ҥ'),
- (0x4A5, 'V'),
- (0x4A6, 'M', 'ҧ'),
- (0x4A7, 'V'),
- (0x4A8, 'M', 'ҩ'),
- (0x4A9, 'V'),
- (0x4AA, 'M', 'ҫ'),
- (0x4AB, 'V'),
- (0x4AC, 'M', 'ҭ'),
- (0x4AD, 'V'),
- (0x4AE, 'M', 'ү'),
- (0x4AF, 'V'),
- (0x4B0, 'M', 'ұ'),
- (0x4B1, 'V'),
- (0x4B2, 'M', 'ҳ'),
- (0x4B3, 'V'),
- (0x4B4, 'M', 'ҵ'),
- (0x4B5, 'V'),
- (0x4B6, 'M', 'ҷ'),
- (0x4B7, 'V'),
- (0x4B8, 'M', 'ҹ'),
- (0x4B9, 'V'),
- (0x4BA, 'M', 'һ'),
- (0x4BB, 'V'),
- (0x4BC, 'M', 'ҽ'),
- (0x4BD, 'V'),
- (0x4BE, 'M', 'ҿ'),
- (0x4BF, 'V'),
- (0x4C0, 'X'),
- (0x4C1, 'M', 'ӂ'),
- (0x4C2, 'V'),
- (0x4C3, 'M', 'ӄ'),
- (0x4C4, 'V'),
- (0x4C5, 'M', 'ӆ'),
- (0x4C6, 'V'),
- (0x4C7, 'M', 'ӈ'),
- (0x4C8, 'V'),
- (0x4C9, 'M', 'ӊ'),
- (0x4CA, 'V'),
- (0x4CB, 'M', 'ӌ'),
- (0x4CC, 'V'),
- (0x4CD, 'M', 'ӎ'),
- (0x4CE, 'V'),
- (0x4D0, 'M', 'ӑ'),
- (0x4D1, 'V'),
- (0x4D2, 'M', 'ӓ'),
- (0x4D3, 'V'),
- (0x4D4, 'M', 'ӕ'),
- (0x4D5, 'V'),
- (0x4D6, 'M', 'ӗ'),
- (0x4D7, 'V'),
- (0x4D8, 'M', 'ә'),
- (0x4D9, 'V'),
- (0x4DA, 'M', 'ӛ'),
- (0x4DB, 'V'),
- (0x4DC, 'M', 'ӝ'),
- (0x4DD, 'V'),
- (0x4DE, 'M', 'ӟ'),
- (0x4DF, 'V'),
- (0x4E0, 'M', 'ӡ'),
- (0x4E1, 'V'),
- (0x4E2, 'M', 'ӣ'),
- (0x4E3, 'V'),
- (0x4E4, 'M', 'ӥ'),
- (0x4E5, 'V'),
- (0x4E6, 'M', 'ӧ'),
- (0x4E7, 'V'),
- (0x4E8, 'M', 'ө'),
- (0x4E9, 'V'),
- (0x4EA, 'M', 'ӫ'),
- (0x4EB, 'V'),
- (0x4EC, 'M', 'ӭ'),
- (0x4ED, 'V'),
- (0x4EE, 'M', 'ӯ'),
- (0x4EF, 'V'),
- (0x4F0, 'M', 'ӱ'),
- (0x4F1, 'V'),
- (0x4F2, 'M', 'ӳ'),
- (0x4F3, 'V'),
- (0x4F4, 'M', 'ӵ'),
- (0x4F5, 'V'),
- (0x4F6, 'M', 'ӷ'),
- (0x4F7, 'V'),
- (0x4F8, 'M', 'ӹ'),
- (0x4F9, 'V'),
- (0x4FA, 'M', 'ӻ'),
- (0x4FB, 'V'),
- (0x4FC, 'M', 'ӽ'),
- (0x4FD, 'V'),
- (0x4FE, 'M', 'ӿ'),
- (0x4FF, 'V'),
- (0x500, 'M', 'ԁ'),
- (0x501, 'V'),
- (0x502, 'M', 'ԃ'),
- ]
-
-def _seg_9() -> List[Union[Tuple[int, str], Tuple[int, str, str]]]:
- return [
- (0x503, 'V'),
- (0x504, 'M', 'ԅ'),
- (0x505, 'V'),
- (0x506, 'M', 'ԇ'),
- (0x507, 'V'),
- (0x508, 'M', 'ԉ'),
- (0x509, 'V'),
- (0x50A, 'M', 'ԋ'),
- (0x50B, 'V'),
- (0x50C, 'M', 'ԍ'),
- (0x50D, 'V'),
- (0x50E, 'M', 'ԏ'),
- (0x50F, 'V'),
- (0x510, 'M', 'ԑ'),
- (0x511, 'V'),
- (0x512, 'M', 'ԓ'),
- (0x513, 'V'),
- (0x514, 'M', 'ԕ'),
- (0x515, 'V'),
- (0x516, 'M', 'ԗ'),
- (0x517, 'V'),
- (0x518, 'M', 'ԙ'),
- (0x519, 'V'),
- (0x51A, 'M', 'ԛ'),
- (0x51B, 'V'),
- (0x51C, 'M', 'ԝ'),
- (0x51D, 'V'),
- (0x51E, 'M', 'ԟ'),
- (0x51F, 'V'),
- (0x520, 'M', 'ԡ'),
- (0x521, 'V'),
- (0x522, 'M', 'ԣ'),
- (0x523, 'V'),
- (0x524, 'M', 'ԥ'),
- (0x525, 'V'),
- (0x526, 'M', 'ԧ'),
- (0x527, 'V'),
- (0x528, 'M', 'ԩ'),
- (0x529, 'V'),
- (0x52A, 'M', 'ԫ'),
- (0x52B, 'V'),
- (0x52C, 'M', 'ԭ'),
- (0x52D, 'V'),
- (0x52E, 'M', 'ԯ'),
- (0x52F, 'V'),
- (0x530, 'X'),
- (0x531, 'M', 'ա'),
- (0x532, 'M', 'բ'),
- (0x533, 'M', 'գ'),
- (0x534, 'M', 'դ'),
- (0x535, 'M', 'ե'),
- (0x536, 'M', 'զ'),
- (0x537, 'M', 'է'),
- (0x538, 'M', 'ը'),
- (0x539, 'M', 'թ'),
- (0x53A, 'M', 'ժ'),
- (0x53B, 'M', 'ի'),
- (0x53C, 'M', 'լ'),
- (0x53D, 'M', 'խ'),
- (0x53E, 'M', 'ծ'),
- (0x53F, 'M', 'կ'),
- (0x540, 'M', 'հ'),
- (0x541, 'M', 'ձ'),
- (0x542, 'M', 'ղ'),
- (0x543, 'M', 'ճ'),
- (0x544, 'M', 'մ'),
- (0x545, 'M', 'յ'),
- (0x546, 'M', 'ն'),
- (0x547, 'M', 'շ'),
- (0x548, 'M', 'ո'),
- (0x549, 'M', 'չ'),
- (0x54A, 'M', 'պ'),
- (0x54B, 'M', 'ջ'),
- (0x54C, 'M', 'ռ'),
- (0x54D, 'M', 'ս'),
- (0x54E, 'M', 'վ'),
- (0x54F, 'M', 'տ'),
- (0x550, 'M', 'ր'),
- (0x551, 'M', 'ց'),
- (0x552, 'M', 'ւ'),
- (0x553, 'M', 'փ'),
- (0x554, 'M', 'ք'),
- (0x555, 'M', 'օ'),
- (0x556, 'M', 'ֆ'),
- (0x557, 'X'),
- (0x559, 'V'),
- (0x587, 'M', 'եւ'),
- (0x588, 'V'),
- (0x58B, 'X'),
- (0x58D, 'V'),
- (0x590, 'X'),
- (0x591, 'V'),
- (0x5C8, 'X'),
- (0x5D0, 'V'),
- (0x5EB, 'X'),
- (0x5EF, 'V'),
- (0x5F5, 'X'),
- (0x606, 'V'),
- (0x61C, 'X'),
- (0x61D, 'V'),
- ]
-
-def _seg_10() -> List[Union[Tuple[int, str], Tuple[int, str, str]]]:
- return [
- (0x675, 'M', 'اٴ'),
- (0x676, 'M', 'وٴ'),
- (0x677, 'M', 'ۇٴ'),
- (0x678, 'M', 'يٴ'),
- (0x679, 'V'),
- (0x6DD, 'X'),
- (0x6DE, 'V'),
- (0x70E, 'X'),
- (0x710, 'V'),
- (0x74B, 'X'),
- (0x74D, 'V'),
- (0x7B2, 'X'),
- (0x7C0, 'V'),
- (0x7FB, 'X'),
- (0x7FD, 'V'),
- (0x82E, 'X'),
- (0x830, 'V'),
- (0x83F, 'X'),
- (0x840, 'V'),
- (0x85C, 'X'),
- (0x85E, 'V'),
- (0x85F, 'X'),
- (0x860, 'V'),
- (0x86B, 'X'),
- (0x870, 'V'),
- (0x88F, 'X'),
- (0x898, 'V'),
- (0x8E2, 'X'),
- (0x8E3, 'V'),
- (0x958, 'M', 'क़'),
- (0x959, 'M', 'ख़'),
- (0x95A, 'M', 'ग़'),
- (0x95B, 'M', 'ज़'),
- (0x95C, 'M', 'ड़'),
- (0x95D, 'M', 'ढ़'),
- (0x95E, 'M', 'फ़'),
- (0x95F, 'M', 'य़'),
- (0x960, 'V'),
- (0x984, 'X'),
- (0x985, 'V'),
- (0x98D, 'X'),
- (0x98F, 'V'),
- (0x991, 'X'),
- (0x993, 'V'),
- (0x9A9, 'X'),
- (0x9AA, 'V'),
- (0x9B1, 'X'),
- (0x9B2, 'V'),
- (0x9B3, 'X'),
- (0x9B6, 'V'),
- (0x9BA, 'X'),
- (0x9BC, 'V'),
- (0x9C5, 'X'),
- (0x9C7, 'V'),
- (0x9C9, 'X'),
- (0x9CB, 'V'),
- (0x9CF, 'X'),
- (0x9D7, 'V'),
- (0x9D8, 'X'),
- (0x9DC, 'M', 'ড়'),
- (0x9DD, 'M', 'ঢ়'),
- (0x9DE, 'X'),
- (0x9DF, 'M', 'য়'),
- (0x9E0, 'V'),
- (0x9E4, 'X'),
- (0x9E6, 'V'),
- (0x9FF, 'X'),
- (0xA01, 'V'),
- (0xA04, 'X'),
- (0xA05, 'V'),
- (0xA0B, 'X'),
- (0xA0F, 'V'),
- (0xA11, 'X'),
- (0xA13, 'V'),
- (0xA29, 'X'),
- (0xA2A, 'V'),
- (0xA31, 'X'),
- (0xA32, 'V'),
- (0xA33, 'M', 'ਲ਼'),
- (0xA34, 'X'),
- (0xA35, 'V'),
- (0xA36, 'M', 'ਸ਼'),
- (0xA37, 'X'),
- (0xA38, 'V'),
- (0xA3A, 'X'),
- (0xA3C, 'V'),
- (0xA3D, 'X'),
- (0xA3E, 'V'),
- (0xA43, 'X'),
- (0xA47, 'V'),
- (0xA49, 'X'),
- (0xA4B, 'V'),
- (0xA4E, 'X'),
- (0xA51, 'V'),
- (0xA52, 'X'),
- (0xA59, 'M', 'ਖ਼'),
- (0xA5A, 'M', 'ਗ਼'),
- (0xA5B, 'M', 'ਜ਼'),
- (0xA5C, 'V'),
- (0xA5D, 'X'),
- ]
-
-def _seg_11() -> List[Union[Tuple[int, str], Tuple[int, str, str]]]:
- return [
- (0xA5E, 'M', 'ਫ਼'),
- (0xA5F, 'X'),
- (0xA66, 'V'),
- (0xA77, 'X'),
- (0xA81, 'V'),
- (0xA84, 'X'),
- (0xA85, 'V'),
- (0xA8E, 'X'),
- (0xA8F, 'V'),
- (0xA92, 'X'),
- (0xA93, 'V'),
- (0xAA9, 'X'),
- (0xAAA, 'V'),
- (0xAB1, 'X'),
- (0xAB2, 'V'),
- (0xAB4, 'X'),
- (0xAB5, 'V'),
- (0xABA, 'X'),
- (0xABC, 'V'),
- (0xAC6, 'X'),
- (0xAC7, 'V'),
- (0xACA, 'X'),
- (0xACB, 'V'),
- (0xACE, 'X'),
- (0xAD0, 'V'),
- (0xAD1, 'X'),
- (0xAE0, 'V'),
- (0xAE4, 'X'),
- (0xAE6, 'V'),
- (0xAF2, 'X'),
- (0xAF9, 'V'),
- (0xB00, 'X'),
- (0xB01, 'V'),
- (0xB04, 'X'),
- (0xB05, 'V'),
- (0xB0D, 'X'),
- (0xB0F, 'V'),
- (0xB11, 'X'),
- (0xB13, 'V'),
- (0xB29, 'X'),
- (0xB2A, 'V'),
- (0xB31, 'X'),
- (0xB32, 'V'),
- (0xB34, 'X'),
- (0xB35, 'V'),
- (0xB3A, 'X'),
- (0xB3C, 'V'),
- (0xB45, 'X'),
- (0xB47, 'V'),
- (0xB49, 'X'),
- (0xB4B, 'V'),
- (0xB4E, 'X'),
- (0xB55, 'V'),
- (0xB58, 'X'),
- (0xB5C, 'M', 'ଡ଼'),
- (0xB5D, 'M', 'ଢ଼'),
- (0xB5E, 'X'),
- (0xB5F, 'V'),
- (0xB64, 'X'),
- (0xB66, 'V'),
- (0xB78, 'X'),
- (0xB82, 'V'),
- (0xB84, 'X'),
- (0xB85, 'V'),
- (0xB8B, 'X'),
- (0xB8E, 'V'),
- (0xB91, 'X'),
- (0xB92, 'V'),
- (0xB96, 'X'),
- (0xB99, 'V'),
- (0xB9B, 'X'),
- (0xB9C, 'V'),
- (0xB9D, 'X'),
- (0xB9E, 'V'),
- (0xBA0, 'X'),
- (0xBA3, 'V'),
- (0xBA5, 'X'),
- (0xBA8, 'V'),
- (0xBAB, 'X'),
- (0xBAE, 'V'),
- (0xBBA, 'X'),
- (0xBBE, 'V'),
- (0xBC3, 'X'),
- (0xBC6, 'V'),
- (0xBC9, 'X'),
- (0xBCA, 'V'),
- (0xBCE, 'X'),
- (0xBD0, 'V'),
- (0xBD1, 'X'),
- (0xBD7, 'V'),
- (0xBD8, 'X'),
- (0xBE6, 'V'),
- (0xBFB, 'X'),
- (0xC00, 'V'),
- (0xC0D, 'X'),
- (0xC0E, 'V'),
- (0xC11, 'X'),
- (0xC12, 'V'),
- (0xC29, 'X'),
- (0xC2A, 'V'),
- ]
-
-def _seg_12() -> List[Union[Tuple[int, str], Tuple[int, str, str]]]:
- return [
- (0xC3A, 'X'),
- (0xC3C, 'V'),
- (0xC45, 'X'),
- (0xC46, 'V'),
- (0xC49, 'X'),
- (0xC4A, 'V'),
- (0xC4E, 'X'),
- (0xC55, 'V'),
- (0xC57, 'X'),
- (0xC58, 'V'),
- (0xC5B, 'X'),
- (0xC5D, 'V'),
- (0xC5E, 'X'),
- (0xC60, 'V'),
- (0xC64, 'X'),
- (0xC66, 'V'),
- (0xC70, 'X'),
- (0xC77, 'V'),
- (0xC8D, 'X'),
- (0xC8E, 'V'),
- (0xC91, 'X'),
- (0xC92, 'V'),
- (0xCA9, 'X'),
- (0xCAA, 'V'),
- (0xCB4, 'X'),
- (0xCB5, 'V'),
- (0xCBA, 'X'),
- (0xCBC, 'V'),
- (0xCC5, 'X'),
- (0xCC6, 'V'),
- (0xCC9, 'X'),
- (0xCCA, 'V'),
- (0xCCE, 'X'),
- (0xCD5, 'V'),
- (0xCD7, 'X'),
- (0xCDD, 'V'),
- (0xCDF, 'X'),
- (0xCE0, 'V'),
- (0xCE4, 'X'),
- (0xCE6, 'V'),
- (0xCF0, 'X'),
- (0xCF1, 'V'),
- (0xCF3, 'X'),
- (0xD00, 'V'),
- (0xD0D, 'X'),
- (0xD0E, 'V'),
- (0xD11, 'X'),
- (0xD12, 'V'),
- (0xD45, 'X'),
- (0xD46, 'V'),
- (0xD49, 'X'),
- (0xD4A, 'V'),
- (0xD50, 'X'),
- (0xD54, 'V'),
- (0xD64, 'X'),
- (0xD66, 'V'),
- (0xD80, 'X'),
- (0xD81, 'V'),
- (0xD84, 'X'),
- (0xD85, 'V'),
- (0xD97, 'X'),
- (0xD9A, 'V'),
- (0xDB2, 'X'),
- (0xDB3, 'V'),
- (0xDBC, 'X'),
- (0xDBD, 'V'),
- (0xDBE, 'X'),
- (0xDC0, 'V'),
- (0xDC7, 'X'),
- (0xDCA, 'V'),
- (0xDCB, 'X'),
- (0xDCF, 'V'),
- (0xDD5, 'X'),
- (0xDD6, 'V'),
- (0xDD7, 'X'),
- (0xDD8, 'V'),
- (0xDE0, 'X'),
- (0xDE6, 'V'),
- (0xDF0, 'X'),
- (0xDF2, 'V'),
- (0xDF5, 'X'),
- (0xE01, 'V'),
- (0xE33, 'M', 'ํา'),
- (0xE34, 'V'),
- (0xE3B, 'X'),
- (0xE3F, 'V'),
- (0xE5C, 'X'),
- (0xE81, 'V'),
- (0xE83, 'X'),
- (0xE84, 'V'),
- (0xE85, 'X'),
- (0xE86, 'V'),
- (0xE8B, 'X'),
- (0xE8C, 'V'),
- (0xEA4, 'X'),
- (0xEA5, 'V'),
- (0xEA6, 'X'),
- (0xEA7, 'V'),
- (0xEB3, 'M', 'ໍາ'),
- (0xEB4, 'V'),
- ]
-
-def _seg_13() -> List[Union[Tuple[int, str], Tuple[int, str, str]]]:
- return [
- (0xEBE, 'X'),
- (0xEC0, 'V'),
- (0xEC5, 'X'),
- (0xEC6, 'V'),
- (0xEC7, 'X'),
- (0xEC8, 'V'),
- (0xECE, 'X'),
- (0xED0, 'V'),
- (0xEDA, 'X'),
- (0xEDC, 'M', 'ຫນ'),
- (0xEDD, 'M', 'ຫມ'),
- (0xEDE, 'V'),
- (0xEE0, 'X'),
- (0xF00, 'V'),
- (0xF0C, 'M', '་'),
- (0xF0D, 'V'),
- (0xF43, 'M', 'གྷ'),
- (0xF44, 'V'),
- (0xF48, 'X'),
- (0xF49, 'V'),
- (0xF4D, 'M', 'ཌྷ'),
- (0xF4E, 'V'),
- (0xF52, 'M', 'དྷ'),
- (0xF53, 'V'),
- (0xF57, 'M', 'བྷ'),
- (0xF58, 'V'),
- (0xF5C, 'M', 'ཛྷ'),
- (0xF5D, 'V'),
- (0xF69, 'M', 'ཀྵ'),
- (0xF6A, 'V'),
- (0xF6D, 'X'),
- (0xF71, 'V'),
- (0xF73, 'M', 'ཱི'),
- (0xF74, 'V'),
- (0xF75, 'M', 'ཱུ'),
- (0xF76, 'M', 'ྲྀ'),
- (0xF77, 'M', 'ྲཱྀ'),
- (0xF78, 'M', 'ླྀ'),
- (0xF79, 'M', 'ླཱྀ'),
- (0xF7A, 'V'),
- (0xF81, 'M', 'ཱྀ'),
- (0xF82, 'V'),
- (0xF93, 'M', 'ྒྷ'),
- (0xF94, 'V'),
- (0xF98, 'X'),
- (0xF99, 'V'),
- (0xF9D, 'M', 'ྜྷ'),
- (0xF9E, 'V'),
- (0xFA2, 'M', 'ྡྷ'),
- (0xFA3, 'V'),
- (0xFA7, 'M', 'ྦྷ'),
- (0xFA8, 'V'),
- (0xFAC, 'M', 'ྫྷ'),
- (0xFAD, 'V'),
- (0xFB9, 'M', 'ྐྵ'),
- (0xFBA, 'V'),
- (0xFBD, 'X'),
- (0xFBE, 'V'),
- (0xFCD, 'X'),
- (0xFCE, 'V'),
- (0xFDB, 'X'),
- (0x1000, 'V'),
- (0x10A0, 'X'),
- (0x10C7, 'M', 'ⴧ'),
- (0x10C8, 'X'),
- (0x10CD, 'M', 'ⴭ'),
- (0x10CE, 'X'),
- (0x10D0, 'V'),
- (0x10FC, 'M', 'ნ'),
- (0x10FD, 'V'),
- (0x115F, 'X'),
- (0x1161, 'V'),
- (0x1249, 'X'),
- (0x124A, 'V'),
- (0x124E, 'X'),
- (0x1250, 'V'),
- (0x1257, 'X'),
- (0x1258, 'V'),
- (0x1259, 'X'),
- (0x125A, 'V'),
- (0x125E, 'X'),
- (0x1260, 'V'),
- (0x1289, 'X'),
- (0x128A, 'V'),
- (0x128E, 'X'),
- (0x1290, 'V'),
- (0x12B1, 'X'),
- (0x12B2, 'V'),
- (0x12B6, 'X'),
- (0x12B8, 'V'),
- (0x12BF, 'X'),
- (0x12C0, 'V'),
- (0x12C1, 'X'),
- (0x12C2, 'V'),
- (0x12C6, 'X'),
- (0x12C8, 'V'),
- (0x12D7, 'X'),
- (0x12D8, 'V'),
- (0x1311, 'X'),
- (0x1312, 'V'),
- ]
-
-def _seg_14() -> List[Union[Tuple[int, str], Tuple[int, str, str]]]:
- return [
- (0x1316, 'X'),
- (0x1318, 'V'),
- (0x135B, 'X'),
- (0x135D, 'V'),
- (0x137D, 'X'),
- (0x1380, 'V'),
- (0x139A, 'X'),
- (0x13A0, 'V'),
- (0x13F6, 'X'),
- (0x13F8, 'M', 'Ᏸ'),
- (0x13F9, 'M', 'Ᏹ'),
- (0x13FA, 'M', 'Ᏺ'),
- (0x13FB, 'M', 'Ᏻ'),
- (0x13FC, 'M', 'Ᏼ'),
- (0x13FD, 'M', 'Ᏽ'),
- (0x13FE, 'X'),
- (0x1400, 'V'),
- (0x1680, 'X'),
- (0x1681, 'V'),
- (0x169D, 'X'),
- (0x16A0, 'V'),
- (0x16F9, 'X'),
- (0x1700, 'V'),
- (0x1716, 'X'),
- (0x171F, 'V'),
- (0x1737, 'X'),
- (0x1740, 'V'),
- (0x1754, 'X'),
- (0x1760, 'V'),
- (0x176D, 'X'),
- (0x176E, 'V'),
- (0x1771, 'X'),
- (0x1772, 'V'),
- (0x1774, 'X'),
- (0x1780, 'V'),
- (0x17B4, 'X'),
- (0x17B6, 'V'),
- (0x17DE, 'X'),
- (0x17E0, 'V'),
- (0x17EA, 'X'),
- (0x17F0, 'V'),
- (0x17FA, 'X'),
- (0x1800, 'V'),
- (0x1806, 'X'),
- (0x1807, 'V'),
- (0x180B, 'I'),
- (0x180E, 'X'),
- (0x180F, 'I'),
- (0x1810, 'V'),
- (0x181A, 'X'),
- (0x1820, 'V'),
- (0x1879, 'X'),
- (0x1880, 'V'),
- (0x18AB, 'X'),
- (0x18B0, 'V'),
- (0x18F6, 'X'),
- (0x1900, 'V'),
- (0x191F, 'X'),
- (0x1920, 'V'),
- (0x192C, 'X'),
- (0x1930, 'V'),
- (0x193C, 'X'),
- (0x1940, 'V'),
- (0x1941, 'X'),
- (0x1944, 'V'),
- (0x196E, 'X'),
- (0x1970, 'V'),
- (0x1975, 'X'),
- (0x1980, 'V'),
- (0x19AC, 'X'),
- (0x19B0, 'V'),
- (0x19CA, 'X'),
- (0x19D0, 'V'),
- (0x19DB, 'X'),
- (0x19DE, 'V'),
- (0x1A1C, 'X'),
- (0x1A1E, 'V'),
- (0x1A5F, 'X'),
- (0x1A60, 'V'),
- (0x1A7D, 'X'),
- (0x1A7F, 'V'),
- (0x1A8A, 'X'),
- (0x1A90, 'V'),
- (0x1A9A, 'X'),
- (0x1AA0, 'V'),
- (0x1AAE, 'X'),
- (0x1AB0, 'V'),
- (0x1ACF, 'X'),
- (0x1B00, 'V'),
- (0x1B4D, 'X'),
- (0x1B50, 'V'),
- (0x1B7F, 'X'),
- (0x1B80, 'V'),
- (0x1BF4, 'X'),
- (0x1BFC, 'V'),
- (0x1C38, 'X'),
- (0x1C3B, 'V'),
- (0x1C4A, 'X'),
- (0x1C4D, 'V'),
- (0x1C80, 'M', 'в'),
- ]
-
-def _seg_15() -> List[Union[Tuple[int, str], Tuple[int, str, str]]]:
- return [
- (0x1C81, 'M', 'д'),
- (0x1C82, 'M', 'о'),
- (0x1C83, 'M', 'с'),
- (0x1C84, 'M', 'т'),
- (0x1C86, 'M', 'ъ'),
- (0x1C87, 'M', 'ѣ'),
- (0x1C88, 'M', 'ꙋ'),
- (0x1C89, 'X'),
- (0x1C90, 'M', 'ა'),
- (0x1C91, 'M', 'ბ'),
- (0x1C92, 'M', 'გ'),
- (0x1C93, 'M', 'დ'),
- (0x1C94, 'M', 'ე'),
- (0x1C95, 'M', 'ვ'),
- (0x1C96, 'M', 'ზ'),
- (0x1C97, 'M', 'თ'),
- (0x1C98, 'M', 'ი'),
- (0x1C99, 'M', 'კ'),
- (0x1C9A, 'M', 'ლ'),
- (0x1C9B, 'M', 'მ'),
- (0x1C9C, 'M', 'ნ'),
- (0x1C9D, 'M', 'ო'),
- (0x1C9E, 'M', 'პ'),
- (0x1C9F, 'M', 'ჟ'),
- (0x1CA0, 'M', 'რ'),
- (0x1CA1, 'M', 'ს'),
- (0x1CA2, 'M', 'ტ'),
- (0x1CA3, 'M', 'უ'),
- (0x1CA4, 'M', 'ფ'),
- (0x1CA5, 'M', 'ქ'),
- (0x1CA6, 'M', 'ღ'),
- (0x1CA7, 'M', 'ყ'),
- (0x1CA8, 'M', 'შ'),
- (0x1CA9, 'M', 'ჩ'),
- (0x1CAA, 'M', 'ც'),
- (0x1CAB, 'M', 'ძ'),
- (0x1CAC, 'M', 'წ'),
- (0x1CAD, 'M', 'ჭ'),
- (0x1CAE, 'M', 'ხ'),
- (0x1CAF, 'M', 'ჯ'),
- (0x1CB0, 'M', 'ჰ'),
- (0x1CB1, 'M', 'ჱ'),
- (0x1CB2, 'M', 'ჲ'),
- (0x1CB3, 'M', 'ჳ'),
- (0x1CB4, 'M', 'ჴ'),
- (0x1CB5, 'M', 'ჵ'),
- (0x1CB6, 'M', 'ჶ'),
- (0x1CB7, 'M', 'ჷ'),
- (0x1CB8, 'M', 'ჸ'),
- (0x1CB9, 'M', 'ჹ'),
- (0x1CBA, 'M', 'ჺ'),
- (0x1CBB, 'X'),
- (0x1CBD, 'M', 'ჽ'),
- (0x1CBE, 'M', 'ჾ'),
- (0x1CBF, 'M', 'ჿ'),
- (0x1CC0, 'V'),
- (0x1CC8, 'X'),
- (0x1CD0, 'V'),
- (0x1CFB, 'X'),
- (0x1D00, 'V'),
- (0x1D2C, 'M', 'a'),
- (0x1D2D, 'M', 'æ'),
- (0x1D2E, 'M', 'b'),
- (0x1D2F, 'V'),
- (0x1D30, 'M', 'd'),
- (0x1D31, 'M', 'e'),
- (0x1D32, 'M', 'ǝ'),
- (0x1D33, 'M', 'g'),
- (0x1D34, 'M', 'h'),
- (0x1D35, 'M', 'i'),
- (0x1D36, 'M', 'j'),
- (0x1D37, 'M', 'k'),
- (0x1D38, 'M', 'l'),
- (0x1D39, 'M', 'm'),
- (0x1D3A, 'M', 'n'),
- (0x1D3B, 'V'),
- (0x1D3C, 'M', 'o'),
- (0x1D3D, 'M', 'ȣ'),
- (0x1D3E, 'M', 'p'),
- (0x1D3F, 'M', 'r'),
- (0x1D40, 'M', 't'),
- (0x1D41, 'M', 'u'),
- (0x1D42, 'M', 'w'),
- (0x1D43, 'M', 'a'),
- (0x1D44, 'M', 'ɐ'),
- (0x1D45, 'M', 'ɑ'),
- (0x1D46, 'M', 'ᴂ'),
- (0x1D47, 'M', 'b'),
- (0x1D48, 'M', 'd'),
- (0x1D49, 'M', 'e'),
- (0x1D4A, 'M', 'ə'),
- (0x1D4B, 'M', 'ɛ'),
- (0x1D4C, 'M', 'ɜ'),
- (0x1D4D, 'M', 'g'),
- (0x1D4E, 'V'),
- (0x1D4F, 'M', 'k'),
- (0x1D50, 'M', 'm'),
- (0x1D51, 'M', 'ŋ'),
- (0x1D52, 'M', 'o'),
- (0x1D53, 'M', 'ɔ'),
- ]
-
-def _seg_16() -> List[Union[Tuple[int, str], Tuple[int, str, str]]]:
- return [
- (0x1D54, 'M', 'ᴖ'),
- (0x1D55, 'M', 'ᴗ'),
- (0x1D56, 'M', 'p'),
- (0x1D57, 'M', 't'),
- (0x1D58, 'M', 'u'),
- (0x1D59, 'M', 'ᴝ'),
- (0x1D5A, 'M', 'ɯ'),
- (0x1D5B, 'M', 'v'),
- (0x1D5C, 'M', 'ᴥ'),
- (0x1D5D, 'M', 'β'),
- (0x1D5E, 'M', 'γ'),
- (0x1D5F, 'M', 'δ'),
- (0x1D60, 'M', 'φ'),
- (0x1D61, 'M', 'χ'),
- (0x1D62, 'M', 'i'),
- (0x1D63, 'M', 'r'),
- (0x1D64, 'M', 'u'),
- (0x1D65, 'M', 'v'),
- (0x1D66, 'M', 'β'),
- (0x1D67, 'M', 'γ'),
- (0x1D68, 'M', 'ρ'),
- (0x1D69, 'M', 'φ'),
- (0x1D6A, 'M', 'χ'),
- (0x1D6B, 'V'),
- (0x1D78, 'M', 'н'),
- (0x1D79, 'V'),
- (0x1D9B, 'M', 'ɒ'),
- (0x1D9C, 'M', 'c'),
- (0x1D9D, 'M', 'ɕ'),
- (0x1D9E, 'M', 'ð'),
- (0x1D9F, 'M', 'ɜ'),
- (0x1DA0, 'M', 'f'),
- (0x1DA1, 'M', 'ɟ'),
- (0x1DA2, 'M', 'ɡ'),
- (0x1DA3, 'M', 'ɥ'),
- (0x1DA4, 'M', 'ɨ'),
- (0x1DA5, 'M', 'ɩ'),
- (0x1DA6, 'M', 'ɪ'),
- (0x1DA7, 'M', 'ᵻ'),
- (0x1DA8, 'M', 'ʝ'),
- (0x1DA9, 'M', 'ɭ'),
- (0x1DAA, 'M', 'ᶅ'),
- (0x1DAB, 'M', 'ʟ'),
- (0x1DAC, 'M', 'ɱ'),
- (0x1DAD, 'M', 'ɰ'),
- (0x1DAE, 'M', 'ɲ'),
- (0x1DAF, 'M', 'ɳ'),
- (0x1DB0, 'M', 'ɴ'),
- (0x1DB1, 'M', 'ɵ'),
- (0x1DB2, 'M', 'ɸ'),
- (0x1DB3, 'M', 'ʂ'),
- (0x1DB4, 'M', 'ʃ'),
- (0x1DB5, 'M', 'ƫ'),
- (0x1DB6, 'M', 'ʉ'),
- (0x1DB7, 'M', 'ʊ'),
- (0x1DB8, 'M', 'ᴜ'),
- (0x1DB9, 'M', 'ʋ'),
- (0x1DBA, 'M', 'ʌ'),
- (0x1DBB, 'M', 'z'),
- (0x1DBC, 'M', 'ʐ'),
- (0x1DBD, 'M', 'ʑ'),
- (0x1DBE, 'M', 'ʒ'),
- (0x1DBF, 'M', 'θ'),
- (0x1DC0, 'V'),
- (0x1E00, 'M', 'ḁ'),
- (0x1E01, 'V'),
- (0x1E02, 'M', 'ḃ'),
- (0x1E03, 'V'),
- (0x1E04, 'M', 'ḅ'),
- (0x1E05, 'V'),
- (0x1E06, 'M', 'ḇ'),
- (0x1E07, 'V'),
- (0x1E08, 'M', 'ḉ'),
- (0x1E09, 'V'),
- (0x1E0A, 'M', 'ḋ'),
- (0x1E0B, 'V'),
- (0x1E0C, 'M', 'ḍ'),
- (0x1E0D, 'V'),
- (0x1E0E, 'M', 'ḏ'),
- (0x1E0F, 'V'),
- (0x1E10, 'M', 'ḑ'),
- (0x1E11, 'V'),
- (0x1E12, 'M', 'ḓ'),
- (0x1E13, 'V'),
- (0x1E14, 'M', 'ḕ'),
- (0x1E15, 'V'),
- (0x1E16, 'M', 'ḗ'),
- (0x1E17, 'V'),
- (0x1E18, 'M', 'ḙ'),
- (0x1E19, 'V'),
- (0x1E1A, 'M', 'ḛ'),
- (0x1E1B, 'V'),
- (0x1E1C, 'M', 'ḝ'),
- (0x1E1D, 'V'),
- (0x1E1E, 'M', 'ḟ'),
- (0x1E1F, 'V'),
- (0x1E20, 'M', 'ḡ'),
- (0x1E21, 'V'),
- (0x1E22, 'M', 'ḣ'),
- (0x1E23, 'V'),
- ]
-
-def _seg_17() -> List[Union[Tuple[int, str], Tuple[int, str, str]]]:
- return [
- (0x1E24, 'M', 'ḥ'),
- (0x1E25, 'V'),
- (0x1E26, 'M', 'ḧ'),
- (0x1E27, 'V'),
- (0x1E28, 'M', 'ḩ'),
- (0x1E29, 'V'),
- (0x1E2A, 'M', 'ḫ'),
- (0x1E2B, 'V'),
- (0x1E2C, 'M', 'ḭ'),
- (0x1E2D, 'V'),
- (0x1E2E, 'M', 'ḯ'),
- (0x1E2F, 'V'),
- (0x1E30, 'M', 'ḱ'),
- (0x1E31, 'V'),
- (0x1E32, 'M', 'ḳ'),
- (0x1E33, 'V'),
- (0x1E34, 'M', 'ḵ'),
- (0x1E35, 'V'),
- (0x1E36, 'M', 'ḷ'),
- (0x1E37, 'V'),
- (0x1E38, 'M', 'ḹ'),
- (0x1E39, 'V'),
- (0x1E3A, 'M', 'ḻ'),
- (0x1E3B, 'V'),
- (0x1E3C, 'M', 'ḽ'),
- (0x1E3D, 'V'),
- (0x1E3E, 'M', 'ḿ'),
- (0x1E3F, 'V'),
- (0x1E40, 'M', 'ṁ'),
- (0x1E41, 'V'),
- (0x1E42, 'M', 'ṃ'),
- (0x1E43, 'V'),
- (0x1E44, 'M', 'ṅ'),
- (0x1E45, 'V'),
- (0x1E46, 'M', 'ṇ'),
- (0x1E47, 'V'),
- (0x1E48, 'M', 'ṉ'),
- (0x1E49, 'V'),
- (0x1E4A, 'M', 'ṋ'),
- (0x1E4B, 'V'),
- (0x1E4C, 'M', 'ṍ'),
- (0x1E4D, 'V'),
- (0x1E4E, 'M', 'ṏ'),
- (0x1E4F, 'V'),
- (0x1E50, 'M', 'ṑ'),
- (0x1E51, 'V'),
- (0x1E52, 'M', 'ṓ'),
- (0x1E53, 'V'),
- (0x1E54, 'M', 'ṕ'),
- (0x1E55, 'V'),
- (0x1E56, 'M', 'ṗ'),
- (0x1E57, 'V'),
- (0x1E58, 'M', 'ṙ'),
- (0x1E59, 'V'),
- (0x1E5A, 'M', 'ṛ'),
- (0x1E5B, 'V'),
- (0x1E5C, 'M', 'ṝ'),
- (0x1E5D, 'V'),
- (0x1E5E, 'M', 'ṟ'),
- (0x1E5F, 'V'),
- (0x1E60, 'M', 'ṡ'),
- (0x1E61, 'V'),
- (0x1E62, 'M', 'ṣ'),
- (0x1E63, 'V'),
- (0x1E64, 'M', 'ṥ'),
- (0x1E65, 'V'),
- (0x1E66, 'M', 'ṧ'),
- (0x1E67, 'V'),
- (0x1E68, 'M', 'ṩ'),
- (0x1E69, 'V'),
- (0x1E6A, 'M', 'ṫ'),
- (0x1E6B, 'V'),
- (0x1E6C, 'M', 'ṭ'),
- (0x1E6D, 'V'),
- (0x1E6E, 'M', 'ṯ'),
- (0x1E6F, 'V'),
- (0x1E70, 'M', 'ṱ'),
- (0x1E71, 'V'),
- (0x1E72, 'M', 'ṳ'),
- (0x1E73, 'V'),
- (0x1E74, 'M', 'ṵ'),
- (0x1E75, 'V'),
- (0x1E76, 'M', 'ṷ'),
- (0x1E77, 'V'),
- (0x1E78, 'M', 'ṹ'),
- (0x1E79, 'V'),
- (0x1E7A, 'M', 'ṻ'),
- (0x1E7B, 'V'),
- (0x1E7C, 'M', 'ṽ'),
- (0x1E7D, 'V'),
- (0x1E7E, 'M', 'ṿ'),
- (0x1E7F, 'V'),
- (0x1E80, 'M', 'ẁ'),
- (0x1E81, 'V'),
- (0x1E82, 'M', 'ẃ'),
- (0x1E83, 'V'),
- (0x1E84, 'M', 'ẅ'),
- (0x1E85, 'V'),
- (0x1E86, 'M', 'ẇ'),
- (0x1E87, 'V'),
- ]
-
-def _seg_18() -> List[Union[Tuple[int, str], Tuple[int, str, str]]]:
- return [
- (0x1E88, 'M', 'ẉ'),
- (0x1E89, 'V'),
- (0x1E8A, 'M', 'ẋ'),
- (0x1E8B, 'V'),
- (0x1E8C, 'M', 'ẍ'),
- (0x1E8D, 'V'),
- (0x1E8E, 'M', 'ẏ'),
- (0x1E8F, 'V'),
- (0x1E90, 'M', 'ẑ'),
- (0x1E91, 'V'),
- (0x1E92, 'M', 'ẓ'),
- (0x1E93, 'V'),
- (0x1E94, 'M', 'ẕ'),
- (0x1E95, 'V'),
- (0x1E9A, 'M', 'aʾ'),
- (0x1E9B, 'M', 'ṡ'),
- (0x1E9C, 'V'),
- (0x1E9E, 'M', 'ss'),
- (0x1E9F, 'V'),
- (0x1EA0, 'M', 'ạ'),
- (0x1EA1, 'V'),
- (0x1EA2, 'M', 'ả'),
- (0x1EA3, 'V'),
- (0x1EA4, 'M', 'ấ'),
- (0x1EA5, 'V'),
- (0x1EA6, 'M', 'ầ'),
- (0x1EA7, 'V'),
- (0x1EA8, 'M', 'ẩ'),
- (0x1EA9, 'V'),
- (0x1EAA, 'M', 'ẫ'),
- (0x1EAB, 'V'),
- (0x1EAC, 'M', 'ậ'),
- (0x1EAD, 'V'),
- (0x1EAE, 'M', 'ắ'),
- (0x1EAF, 'V'),
- (0x1EB0, 'M', 'ằ'),
- (0x1EB1, 'V'),
- (0x1EB2, 'M', 'ẳ'),
- (0x1EB3, 'V'),
- (0x1EB4, 'M', 'ẵ'),
- (0x1EB5, 'V'),
- (0x1EB6, 'M', 'ặ'),
- (0x1EB7, 'V'),
- (0x1EB8, 'M', 'ẹ'),
- (0x1EB9, 'V'),
- (0x1EBA, 'M', 'ẻ'),
- (0x1EBB, 'V'),
- (0x1EBC, 'M', 'ẽ'),
- (0x1EBD, 'V'),
- (0x1EBE, 'M', 'ế'),
- (0x1EBF, 'V'),
- (0x1EC0, 'M', 'ề'),
- (0x1EC1, 'V'),
- (0x1EC2, 'M', 'ể'),
- (0x1EC3, 'V'),
- (0x1EC4, 'M', 'ễ'),
- (0x1EC5, 'V'),
- (0x1EC6, 'M', 'ệ'),
- (0x1EC7, 'V'),
- (0x1EC8, 'M', 'ỉ'),
- (0x1EC9, 'V'),
- (0x1ECA, 'M', 'ị'),
- (0x1ECB, 'V'),
- (0x1ECC, 'M', 'ọ'),
- (0x1ECD, 'V'),
- (0x1ECE, 'M', 'ỏ'),
- (0x1ECF, 'V'),
- (0x1ED0, 'M', 'ố'),
- (0x1ED1, 'V'),
- (0x1ED2, 'M', 'ồ'),
- (0x1ED3, 'V'),
- (0x1ED4, 'M', 'ổ'),
- (0x1ED5, 'V'),
- (0x1ED6, 'M', 'ỗ'),
- (0x1ED7, 'V'),
- (0x1ED8, 'M', 'ộ'),
- (0x1ED9, 'V'),
- (0x1EDA, 'M', 'ớ'),
- (0x1EDB, 'V'),
- (0x1EDC, 'M', 'ờ'),
- (0x1EDD, 'V'),
- (0x1EDE, 'M', 'ở'),
- (0x1EDF, 'V'),
- (0x1EE0, 'M', 'ỡ'),
- (0x1EE1, 'V'),
- (0x1EE2, 'M', 'ợ'),
- (0x1EE3, 'V'),
- (0x1EE4, 'M', 'ụ'),
- (0x1EE5, 'V'),
- (0x1EE6, 'M', 'ủ'),
- (0x1EE7, 'V'),
- (0x1EE8, 'M', 'ứ'),
- (0x1EE9, 'V'),
- (0x1EEA, 'M', 'ừ'),
- (0x1EEB, 'V'),
- (0x1EEC, 'M', 'ử'),
- (0x1EED, 'V'),
- (0x1EEE, 'M', 'ữ'),
- (0x1EEF, 'V'),
- (0x1EF0, 'M', 'ự'),
- ]
-
-def _seg_19() -> List[Union[Tuple[int, str], Tuple[int, str, str]]]:
- return [
- (0x1EF1, 'V'),
- (0x1EF2, 'M', 'ỳ'),
- (0x1EF3, 'V'),
- (0x1EF4, 'M', 'ỵ'),
- (0x1EF5, 'V'),
- (0x1EF6, 'M', 'ỷ'),
- (0x1EF7, 'V'),
- (0x1EF8, 'M', 'ỹ'),
- (0x1EF9, 'V'),
- (0x1EFA, 'M', 'ỻ'),
- (0x1EFB, 'V'),
- (0x1EFC, 'M', 'ỽ'),
- (0x1EFD, 'V'),
- (0x1EFE, 'M', 'ỿ'),
- (0x1EFF, 'V'),
- (0x1F08, 'M', 'ἀ'),
- (0x1F09, 'M', 'ἁ'),
- (0x1F0A, 'M', 'ἂ'),
- (0x1F0B, 'M', 'ἃ'),
- (0x1F0C, 'M', 'ἄ'),
- (0x1F0D, 'M', 'ἅ'),
- (0x1F0E, 'M', 'ἆ'),
- (0x1F0F, 'M', 'ἇ'),
- (0x1F10, 'V'),
- (0x1F16, 'X'),
- (0x1F18, 'M', 'ἐ'),
- (0x1F19, 'M', 'ἑ'),
- (0x1F1A, 'M', 'ἒ'),
- (0x1F1B, 'M', 'ἓ'),
- (0x1F1C, 'M', 'ἔ'),
- (0x1F1D, 'M', 'ἕ'),
- (0x1F1E, 'X'),
- (0x1F20, 'V'),
- (0x1F28, 'M', 'ἠ'),
- (0x1F29, 'M', 'ἡ'),
- (0x1F2A, 'M', 'ἢ'),
- (0x1F2B, 'M', 'ἣ'),
- (0x1F2C, 'M', 'ἤ'),
- (0x1F2D, 'M', 'ἥ'),
- (0x1F2E, 'M', 'ἦ'),
- (0x1F2F, 'M', 'ἧ'),
- (0x1F30, 'V'),
- (0x1F38, 'M', 'ἰ'),
- (0x1F39, 'M', 'ἱ'),
- (0x1F3A, 'M', 'ἲ'),
- (0x1F3B, 'M', 'ἳ'),
- (0x1F3C, 'M', 'ἴ'),
- (0x1F3D, 'M', 'ἵ'),
- (0x1F3E, 'M', 'ἶ'),
- (0x1F3F, 'M', 'ἷ'),
- (0x1F40, 'V'),
- (0x1F46, 'X'),
- (0x1F48, 'M', 'ὀ'),
- (0x1F49, 'M', 'ὁ'),
- (0x1F4A, 'M', 'ὂ'),
- (0x1F4B, 'M', 'ὃ'),
- (0x1F4C, 'M', 'ὄ'),
- (0x1F4D, 'M', 'ὅ'),
- (0x1F4E, 'X'),
- (0x1F50, 'V'),
- (0x1F58, 'X'),
- (0x1F59, 'M', 'ὑ'),
- (0x1F5A, 'X'),
- (0x1F5B, 'M', 'ὓ'),
- (0x1F5C, 'X'),
- (0x1F5D, 'M', 'ὕ'),
- (0x1F5E, 'X'),
- (0x1F5F, 'M', 'ὗ'),
- (0x1F60, 'V'),
- (0x1F68, 'M', 'ὠ'),
- (0x1F69, 'M', 'ὡ'),
- (0x1F6A, 'M', 'ὢ'),
- (0x1F6B, 'M', 'ὣ'),
- (0x1F6C, 'M', 'ὤ'),
- (0x1F6D, 'M', 'ὥ'),
- (0x1F6E, 'M', 'ὦ'),
- (0x1F6F, 'M', 'ὧ'),
- (0x1F70, 'V'),
- (0x1F71, 'M', 'ά'),
- (0x1F72, 'V'),
- (0x1F73, 'M', 'έ'),
- (0x1F74, 'V'),
- (0x1F75, 'M', 'ή'),
- (0x1F76, 'V'),
- (0x1F77, 'M', 'ί'),
- (0x1F78, 'V'),
- (0x1F79, 'M', 'ό'),
- (0x1F7A, 'V'),
- (0x1F7B, 'M', 'ύ'),
- (0x1F7C, 'V'),
- (0x1F7D, 'M', 'ώ'),
- (0x1F7E, 'X'),
- (0x1F80, 'M', 'ἀι'),
- (0x1F81, 'M', 'ἁι'),
- (0x1F82, 'M', 'ἂι'),
- (0x1F83, 'M', 'ἃι'),
- (0x1F84, 'M', 'ἄι'),
- (0x1F85, 'M', 'ἅι'),
- (0x1F86, 'M', 'ἆι'),
- (0x1F87, 'M', 'ἇι'),
- ]
-
-def _seg_20() -> List[Union[Tuple[int, str], Tuple[int, str, str]]]:
- return [
- (0x1F88, 'M', 'ἀι'),
- (0x1F89, 'M', 'ἁι'),
- (0x1F8A, 'M', 'ἂι'),
- (0x1F8B, 'M', 'ἃι'),
- (0x1F8C, 'M', 'ἄι'),
- (0x1F8D, 'M', 'ἅι'),
- (0x1F8E, 'M', 'ἆι'),
- (0x1F8F, 'M', 'ἇι'),
- (0x1F90, 'M', 'ἠι'),
- (0x1F91, 'M', 'ἡι'),
- (0x1F92, 'M', 'ἢι'),
- (0x1F93, 'M', 'ἣι'),
- (0x1F94, 'M', 'ἤι'),
- (0x1F95, 'M', 'ἥι'),
- (0x1F96, 'M', 'ἦι'),
- (0x1F97, 'M', 'ἧι'),
- (0x1F98, 'M', 'ἠι'),
- (0x1F99, 'M', 'ἡι'),
- (0x1F9A, 'M', 'ἢι'),
- (0x1F9B, 'M', 'ἣι'),
- (0x1F9C, 'M', 'ἤι'),
- (0x1F9D, 'M', 'ἥι'),
- (0x1F9E, 'M', 'ἦι'),
- (0x1F9F, 'M', 'ἧι'),
- (0x1FA0, 'M', 'ὠι'),
- (0x1FA1, 'M', 'ὡι'),
- (0x1FA2, 'M', 'ὢι'),
- (0x1FA3, 'M', 'ὣι'),
- (0x1FA4, 'M', 'ὤι'),
- (0x1FA5, 'M', 'ὥι'),
- (0x1FA6, 'M', 'ὦι'),
- (0x1FA7, 'M', 'ὧι'),
- (0x1FA8, 'M', 'ὠι'),
- (0x1FA9, 'M', 'ὡι'),
- (0x1FAA, 'M', 'ὢι'),
- (0x1FAB, 'M', 'ὣι'),
- (0x1FAC, 'M', 'ὤι'),
- (0x1FAD, 'M', 'ὥι'),
- (0x1FAE, 'M', 'ὦι'),
- (0x1FAF, 'M', 'ὧι'),
- (0x1FB0, 'V'),
- (0x1FB2, 'M', 'ὰι'),
- (0x1FB3, 'M', 'αι'),
- (0x1FB4, 'M', 'άι'),
- (0x1FB5, 'X'),
- (0x1FB6, 'V'),
- (0x1FB7, 'M', 'ᾶι'),
- (0x1FB8, 'M', 'ᾰ'),
- (0x1FB9, 'M', 'ᾱ'),
- (0x1FBA, 'M', 'ὰ'),
- (0x1FBB, 'M', 'ά'),
- (0x1FBC, 'M', 'αι'),
- (0x1FBD, '3', ' ̓'),
- (0x1FBE, 'M', 'ι'),
- (0x1FBF, '3', ' ̓'),
- (0x1FC0, '3', ' ͂'),
- (0x1FC1, '3', ' ̈͂'),
- (0x1FC2, 'M', 'ὴι'),
- (0x1FC3, 'M', 'ηι'),
- (0x1FC4, 'M', 'ήι'),
- (0x1FC5, 'X'),
- (0x1FC6, 'V'),
- (0x1FC7, 'M', 'ῆι'),
- (0x1FC8, 'M', 'ὲ'),
- (0x1FC9, 'M', 'έ'),
- (0x1FCA, 'M', 'ὴ'),
- (0x1FCB, 'M', 'ή'),
- (0x1FCC, 'M', 'ηι'),
- (0x1FCD, '3', ' ̓̀'),
- (0x1FCE, '3', ' ̓́'),
- (0x1FCF, '3', ' ̓͂'),
- (0x1FD0, 'V'),
- (0x1FD3, 'M', 'ΐ'),
- (0x1FD4, 'X'),
- (0x1FD6, 'V'),
- (0x1FD8, 'M', 'ῐ'),
- (0x1FD9, 'M', 'ῑ'),
- (0x1FDA, 'M', 'ὶ'),
- (0x1FDB, 'M', 'ί'),
- (0x1FDC, 'X'),
- (0x1FDD, '3', ' ̔̀'),
- (0x1FDE, '3', ' ̔́'),
- (0x1FDF, '3', ' ̔͂'),
- (0x1FE0, 'V'),
- (0x1FE3, 'M', 'ΰ'),
- (0x1FE4, 'V'),
- (0x1FE8, 'M', 'ῠ'),
- (0x1FE9, 'M', 'ῡ'),
- (0x1FEA, 'M', 'ὺ'),
- (0x1FEB, 'M', 'ύ'),
- (0x1FEC, 'M', 'ῥ'),
- (0x1FED, '3', ' ̈̀'),
- (0x1FEE, '3', ' ̈́'),
- (0x1FEF, '3', '`'),
- (0x1FF0, 'X'),
- (0x1FF2, 'M', 'ὼι'),
- (0x1FF3, 'M', 'ωι'),
- (0x1FF4, 'M', 'ώι'),
- (0x1FF5, 'X'),
- (0x1FF6, 'V'),
- ]
-
-def _seg_21() -> List[Union[Tuple[int, str], Tuple[int, str, str]]]:
- return [
- (0x1FF7, 'M', 'ῶι'),
- (0x1FF8, 'M', 'ὸ'),
- (0x1FF9, 'M', 'ό'),
- (0x1FFA, 'M', 'ὼ'),
- (0x1FFB, 'M', 'ώ'),
- (0x1FFC, 'M', 'ωι'),
- (0x1FFD, '3', ' ́'),
- (0x1FFE, '3', ' ̔'),
- (0x1FFF, 'X'),
- (0x2000, '3', ' '),
- (0x200B, 'I'),
- (0x200C, 'D', ''),
- (0x200E, 'X'),
- (0x2010, 'V'),
- (0x2011, 'M', '‐'),
- (0x2012, 'V'),
- (0x2017, '3', ' ̳'),
- (0x2018, 'V'),
- (0x2024, 'X'),
- (0x2027, 'V'),
- (0x2028, 'X'),
- (0x202F, '3', ' '),
- (0x2030, 'V'),
- (0x2033, 'M', '′′'),
- (0x2034, 'M', '′′′'),
- (0x2035, 'V'),
- (0x2036, 'M', '‵‵'),
- (0x2037, 'M', '‵‵‵'),
- (0x2038, 'V'),
- (0x203C, '3', '!!'),
- (0x203D, 'V'),
- (0x203E, '3', ' ̅'),
- (0x203F, 'V'),
- (0x2047, '3', '??'),
- (0x2048, '3', '?!'),
- (0x2049, '3', '!?'),
- (0x204A, 'V'),
- (0x2057, 'M', '′′′′'),
- (0x2058, 'V'),
- (0x205F, '3', ' '),
- (0x2060, 'I'),
- (0x2061, 'X'),
- (0x2064, 'I'),
- (0x2065, 'X'),
- (0x2070, 'M', '0'),
- (0x2071, 'M', 'i'),
- (0x2072, 'X'),
- (0x2074, 'M', '4'),
- (0x2075, 'M', '5'),
- (0x2076, 'M', '6'),
- (0x2077, 'M', '7'),
- (0x2078, 'M', '8'),
- (0x2079, 'M', '9'),
- (0x207A, '3', '+'),
- (0x207B, 'M', '−'),
- (0x207C, '3', '='),
- (0x207D, '3', '('),
- (0x207E, '3', ')'),
- (0x207F, 'M', 'n'),
- (0x2080, 'M', '0'),
- (0x2081, 'M', '1'),
- (0x2082, 'M', '2'),
- (0x2083, 'M', '3'),
- (0x2084, 'M', '4'),
- (0x2085, 'M', '5'),
- (0x2086, 'M', '6'),
- (0x2087, 'M', '7'),
- (0x2088, 'M', '8'),
- (0x2089, 'M', '9'),
- (0x208A, '3', '+'),
- (0x208B, 'M', '−'),
- (0x208C, '3', '='),
- (0x208D, '3', '('),
- (0x208E, '3', ')'),
- (0x208F, 'X'),
- (0x2090, 'M', 'a'),
- (0x2091, 'M', 'e'),
- (0x2092, 'M', 'o'),
- (0x2093, 'M', 'x'),
- (0x2094, 'M', 'ə'),
- (0x2095, 'M', 'h'),
- (0x2096, 'M', 'k'),
- (0x2097, 'M', 'l'),
- (0x2098, 'M', 'm'),
- (0x2099, 'M', 'n'),
- (0x209A, 'M', 'p'),
- (0x209B, 'M', 's'),
- (0x209C, 'M', 't'),
- (0x209D, 'X'),
- (0x20A0, 'V'),
- (0x20A8, 'M', 'rs'),
- (0x20A9, 'V'),
- (0x20C1, 'X'),
- (0x20D0, 'V'),
- (0x20F1, 'X'),
- (0x2100, '3', 'a/c'),
- (0x2101, '3', 'a/s'),
- (0x2102, 'M', 'c'),
- (0x2103, 'M', '°c'),
- (0x2104, 'V'),
- ]
-
-def _seg_22() -> List[Union[Tuple[int, str], Tuple[int, str, str]]]:
- return [
- (0x2105, '3', 'c/o'),
- (0x2106, '3', 'c/u'),
- (0x2107, 'M', 'ɛ'),
- (0x2108, 'V'),
- (0x2109, 'M', '°f'),
- (0x210A, 'M', 'g'),
- (0x210B, 'M', 'h'),
- (0x210F, 'M', 'ħ'),
- (0x2110, 'M', 'i'),
- (0x2112, 'M', 'l'),
- (0x2114, 'V'),
- (0x2115, 'M', 'n'),
- (0x2116, 'M', 'no'),
- (0x2117, 'V'),
- (0x2119, 'M', 'p'),
- (0x211A, 'M', 'q'),
- (0x211B, 'M', 'r'),
- (0x211E, 'V'),
- (0x2120, 'M', 'sm'),
- (0x2121, 'M', 'tel'),
- (0x2122, 'M', 'tm'),
- (0x2123, 'V'),
- (0x2124, 'M', 'z'),
- (0x2125, 'V'),
- (0x2126, 'M', 'ω'),
- (0x2127, 'V'),
- (0x2128, 'M', 'z'),
- (0x2129, 'V'),
- (0x212A, 'M', 'k'),
- (0x212B, 'M', 'å'),
- (0x212C, 'M', 'b'),
- (0x212D, 'M', 'c'),
- (0x212E, 'V'),
- (0x212F, 'M', 'e'),
- (0x2131, 'M', 'f'),
- (0x2132, 'X'),
- (0x2133, 'M', 'm'),
- (0x2134, 'M', 'o'),
- (0x2135, 'M', 'א'),
- (0x2136, 'M', 'ב'),
- (0x2137, 'M', 'ג'),
- (0x2138, 'M', 'ד'),
- (0x2139, 'M', 'i'),
- (0x213A, 'V'),
- (0x213B, 'M', 'fax'),
- (0x213C, 'M', 'π'),
- (0x213D, 'M', 'γ'),
- (0x213F, 'M', 'π'),
- (0x2140, 'M', '∑'),
- (0x2141, 'V'),
- (0x2145, 'M', 'd'),
- (0x2147, 'M', 'e'),
- (0x2148, 'M', 'i'),
- (0x2149, 'M', 'j'),
- (0x214A, 'V'),
- (0x2150, 'M', '1⁄7'),
- (0x2151, 'M', '1⁄9'),
- (0x2152, 'M', '1⁄10'),
- (0x2153, 'M', '1⁄3'),
- (0x2154, 'M', '2⁄3'),
- (0x2155, 'M', '1⁄5'),
- (0x2156, 'M', '2⁄5'),
- (0x2157, 'M', '3⁄5'),
- (0x2158, 'M', '4⁄5'),
- (0x2159, 'M', '1⁄6'),
- (0x215A, 'M', '5⁄6'),
- (0x215B, 'M', '1⁄8'),
- (0x215C, 'M', '3⁄8'),
- (0x215D, 'M', '5⁄8'),
- (0x215E, 'M', '7⁄8'),
- (0x215F, 'M', '1⁄'),
- (0x2160, 'M', 'i'),
- (0x2161, 'M', 'ii'),
- (0x2162, 'M', 'iii'),
- (0x2163, 'M', 'iv'),
- (0x2164, 'M', 'v'),
- (0x2165, 'M', 'vi'),
- (0x2166, 'M', 'vii'),
- (0x2167, 'M', 'viii'),
- (0x2168, 'M', 'ix'),
- (0x2169, 'M', 'x'),
- (0x216A, 'M', 'xi'),
- (0x216B, 'M', 'xii'),
- (0x216C, 'M', 'l'),
- (0x216D, 'M', 'c'),
- (0x216E, 'M', 'd'),
- (0x216F, 'M', 'm'),
- (0x2170, 'M', 'i'),
- (0x2171, 'M', 'ii'),
- (0x2172, 'M', 'iii'),
- (0x2173, 'M', 'iv'),
- (0x2174, 'M', 'v'),
- (0x2175, 'M', 'vi'),
- (0x2176, 'M', 'vii'),
- (0x2177, 'M', 'viii'),
- (0x2178, 'M', 'ix'),
- (0x2179, 'M', 'x'),
- (0x217A, 'M', 'xi'),
- (0x217B, 'M', 'xii'),
- (0x217C, 'M', 'l'),
- ]
-
-def _seg_23() -> List[Union[Tuple[int, str], Tuple[int, str, str]]]:
- return [
- (0x217D, 'M', 'c'),
- (0x217E, 'M', 'd'),
- (0x217F, 'M', 'm'),
- (0x2180, 'V'),
- (0x2183, 'X'),
- (0x2184, 'V'),
- (0x2189, 'M', '0⁄3'),
- (0x218A, 'V'),
- (0x218C, 'X'),
- (0x2190, 'V'),
- (0x222C, 'M', '∫∫'),
- (0x222D, 'M', '∫∫∫'),
- (0x222E, 'V'),
- (0x222F, 'M', '∮∮'),
- (0x2230, 'M', '∮∮∮'),
- (0x2231, 'V'),
- (0x2260, '3'),
- (0x2261, 'V'),
- (0x226E, '3'),
- (0x2270, 'V'),
- (0x2329, 'M', '〈'),
- (0x232A, 'M', '〉'),
- (0x232B, 'V'),
- (0x2427, 'X'),
- (0x2440, 'V'),
- (0x244B, 'X'),
- (0x2460, 'M', '1'),
- (0x2461, 'M', '2'),
- (0x2462, 'M', '3'),
- (0x2463, 'M', '4'),
- (0x2464, 'M', '5'),
- (0x2465, 'M', '6'),
- (0x2466, 'M', '7'),
- (0x2467, 'M', '8'),
- (0x2468, 'M', '9'),
- (0x2469, 'M', '10'),
- (0x246A, 'M', '11'),
- (0x246B, 'M', '12'),
- (0x246C, 'M', '13'),
- (0x246D, 'M', '14'),
- (0x246E, 'M', '15'),
- (0x246F, 'M', '16'),
- (0x2470, 'M', '17'),
- (0x2471, 'M', '18'),
- (0x2472, 'M', '19'),
- (0x2473, 'M', '20'),
- (0x2474, '3', '(1)'),
- (0x2475, '3', '(2)'),
- (0x2476, '3', '(3)'),
- (0x2477, '3', '(4)'),
- (0x2478, '3', '(5)'),
- (0x2479, '3', '(6)'),
- (0x247A, '3', '(7)'),
- (0x247B, '3', '(8)'),
- (0x247C, '3', '(9)'),
- (0x247D, '3', '(10)'),
- (0x247E, '3', '(11)'),
- (0x247F, '3', '(12)'),
- (0x2480, '3', '(13)'),
- (0x2481, '3', '(14)'),
- (0x2482, '3', '(15)'),
- (0x2483, '3', '(16)'),
- (0x2484, '3', '(17)'),
- (0x2485, '3', '(18)'),
- (0x2486, '3', '(19)'),
- (0x2487, '3', '(20)'),
- (0x2488, 'X'),
- (0x249C, '3', '(a)'),
- (0x249D, '3', '(b)'),
- (0x249E, '3', '(c)'),
- (0x249F, '3', '(d)'),
- (0x24A0, '3', '(e)'),
- (0x24A1, '3', '(f)'),
- (0x24A2, '3', '(g)'),
- (0x24A3, '3', '(h)'),
- (0x24A4, '3', '(i)'),
- (0x24A5, '3', '(j)'),
- (0x24A6, '3', '(k)'),
- (0x24A7, '3', '(l)'),
- (0x24A8, '3', '(m)'),
- (0x24A9, '3', '(n)'),
- (0x24AA, '3', '(o)'),
- (0x24AB, '3', '(p)'),
- (0x24AC, '3', '(q)'),
- (0x24AD, '3', '(r)'),
- (0x24AE, '3', '(s)'),
- (0x24AF, '3', '(t)'),
- (0x24B0, '3', '(u)'),
- (0x24B1, '3', '(v)'),
- (0x24B2, '3', '(w)'),
- (0x24B3, '3', '(x)'),
- (0x24B4, '3', '(y)'),
- (0x24B5, '3', '(z)'),
- (0x24B6, 'M', 'a'),
- (0x24B7, 'M', 'b'),
- (0x24B8, 'M', 'c'),
- (0x24B9, 'M', 'd'),
- (0x24BA, 'M', 'e'),
- (0x24BB, 'M', 'f'),
- (0x24BC, 'M', 'g'),
- ]
-
-def _seg_24() -> List[Union[Tuple[int, str], Tuple[int, str, str]]]:
- return [
- (0x24BD, 'M', 'h'),
- (0x24BE, 'M', 'i'),
- (0x24BF, 'M', 'j'),
- (0x24C0, 'M', 'k'),
- (0x24C1, 'M', 'l'),
- (0x24C2, 'M', 'm'),
- (0x24C3, 'M', 'n'),
- (0x24C4, 'M', 'o'),
- (0x24C5, 'M', 'p'),
- (0x24C6, 'M', 'q'),
- (0x24C7, 'M', 'r'),
- (0x24C8, 'M', 's'),
- (0x24C9, 'M', 't'),
- (0x24CA, 'M', 'u'),
- (0x24CB, 'M', 'v'),
- (0x24CC, 'M', 'w'),
- (0x24CD, 'M', 'x'),
- (0x24CE, 'M', 'y'),
- (0x24CF, 'M', 'z'),
- (0x24D0, 'M', 'a'),
- (0x24D1, 'M', 'b'),
- (0x24D2, 'M', 'c'),
- (0x24D3, 'M', 'd'),
- (0x24D4, 'M', 'e'),
- (0x24D5, 'M', 'f'),
- (0x24D6, 'M', 'g'),
- (0x24D7, 'M', 'h'),
- (0x24D8, 'M', 'i'),
- (0x24D9, 'M', 'j'),
- (0x24DA, 'M', 'k'),
- (0x24DB, 'M', 'l'),
- (0x24DC, 'M', 'm'),
- (0x24DD, 'M', 'n'),
- (0x24DE, 'M', 'o'),
- (0x24DF, 'M', 'p'),
- (0x24E0, 'M', 'q'),
- (0x24E1, 'M', 'r'),
- (0x24E2, 'M', 's'),
- (0x24E3, 'M', 't'),
- (0x24E4, 'M', 'u'),
- (0x24E5, 'M', 'v'),
- (0x24E6, 'M', 'w'),
- (0x24E7, 'M', 'x'),
- (0x24E8, 'M', 'y'),
- (0x24E9, 'M', 'z'),
- (0x24EA, 'M', '0'),
- (0x24EB, 'V'),
- (0x2A0C, 'M', '∫∫∫∫'),
- (0x2A0D, 'V'),
- (0x2A74, '3', '::='),
- (0x2A75, '3', '=='),
- (0x2A76, '3', '==='),
- (0x2A77, 'V'),
- (0x2ADC, 'M', '⫝̸'),
- (0x2ADD, 'V'),
- (0x2B74, 'X'),
- (0x2B76, 'V'),
- (0x2B96, 'X'),
- (0x2B97, 'V'),
- (0x2C00, 'M', 'ⰰ'),
- (0x2C01, 'M', 'ⰱ'),
- (0x2C02, 'M', 'ⰲ'),
- (0x2C03, 'M', 'ⰳ'),
- (0x2C04, 'M', 'ⰴ'),
- (0x2C05, 'M', 'ⰵ'),
- (0x2C06, 'M', 'ⰶ'),
- (0x2C07, 'M', 'ⰷ'),
- (0x2C08, 'M', 'ⰸ'),
- (0x2C09, 'M', 'ⰹ'),
- (0x2C0A, 'M', 'ⰺ'),
- (0x2C0B, 'M', 'ⰻ'),
- (0x2C0C, 'M', 'ⰼ'),
- (0x2C0D, 'M', 'ⰽ'),
- (0x2C0E, 'M', 'ⰾ'),
- (0x2C0F, 'M', 'ⰿ'),
- (0x2C10, 'M', 'ⱀ'),
- (0x2C11, 'M', 'ⱁ'),
- (0x2C12, 'M', 'ⱂ'),
- (0x2C13, 'M', 'ⱃ'),
- (0x2C14, 'M', 'ⱄ'),
- (0x2C15, 'M', 'ⱅ'),
- (0x2C16, 'M', 'ⱆ'),
- (0x2C17, 'M', 'ⱇ'),
- (0x2C18, 'M', 'ⱈ'),
- (0x2C19, 'M', 'ⱉ'),
- (0x2C1A, 'M', 'ⱊ'),
- (0x2C1B, 'M', 'ⱋ'),
- (0x2C1C, 'M', 'ⱌ'),
- (0x2C1D, 'M', 'ⱍ'),
- (0x2C1E, 'M', 'ⱎ'),
- (0x2C1F, 'M', 'ⱏ'),
- (0x2C20, 'M', 'ⱐ'),
- (0x2C21, 'M', 'ⱑ'),
- (0x2C22, 'M', 'ⱒ'),
- (0x2C23, 'M', 'ⱓ'),
- (0x2C24, 'M', 'ⱔ'),
- (0x2C25, 'M', 'ⱕ'),
- (0x2C26, 'M', 'ⱖ'),
- (0x2C27, 'M', 'ⱗ'),
- (0x2C28, 'M', 'ⱘ'),
- ]
-
-def _seg_25() -> List[Union[Tuple[int, str], Tuple[int, str, str]]]:
- return [
- (0x2C29, 'M', 'ⱙ'),
- (0x2C2A, 'M', 'ⱚ'),
- (0x2C2B, 'M', 'ⱛ'),
- (0x2C2C, 'M', 'ⱜ'),
- (0x2C2D, 'M', 'ⱝ'),
- (0x2C2E, 'M', 'ⱞ'),
- (0x2C2F, 'M', 'ⱟ'),
- (0x2C30, 'V'),
- (0x2C60, 'M', 'ⱡ'),
- (0x2C61, 'V'),
- (0x2C62, 'M', 'ɫ'),
- (0x2C63, 'M', 'ᵽ'),
- (0x2C64, 'M', 'ɽ'),
- (0x2C65, 'V'),
- (0x2C67, 'M', 'ⱨ'),
- (0x2C68, 'V'),
- (0x2C69, 'M', 'ⱪ'),
- (0x2C6A, 'V'),
- (0x2C6B, 'M', 'ⱬ'),
- (0x2C6C, 'V'),
- (0x2C6D, 'M', 'ɑ'),
- (0x2C6E, 'M', 'ɱ'),
- (0x2C6F, 'M', 'ɐ'),
- (0x2C70, 'M', 'ɒ'),
- (0x2C71, 'V'),
- (0x2C72, 'M', 'ⱳ'),
- (0x2C73, 'V'),
- (0x2C75, 'M', 'ⱶ'),
- (0x2C76, 'V'),
- (0x2C7C, 'M', 'j'),
- (0x2C7D, 'M', 'v'),
- (0x2C7E, 'M', 'ȿ'),
- (0x2C7F, 'M', 'ɀ'),
- (0x2C80, 'M', 'ⲁ'),
- (0x2C81, 'V'),
- (0x2C82, 'M', 'ⲃ'),
- (0x2C83, 'V'),
- (0x2C84, 'M', 'ⲅ'),
- (0x2C85, 'V'),
- (0x2C86, 'M', 'ⲇ'),
- (0x2C87, 'V'),
- (0x2C88, 'M', 'ⲉ'),
- (0x2C89, 'V'),
- (0x2C8A, 'M', 'ⲋ'),
- (0x2C8B, 'V'),
- (0x2C8C, 'M', 'ⲍ'),
- (0x2C8D, 'V'),
- (0x2C8E, 'M', 'ⲏ'),
- (0x2C8F, 'V'),
- (0x2C90, 'M', 'ⲑ'),
- (0x2C91, 'V'),
- (0x2C92, 'M', 'ⲓ'),
- (0x2C93, 'V'),
- (0x2C94, 'M', 'ⲕ'),
- (0x2C95, 'V'),
- (0x2C96, 'M', 'ⲗ'),
- (0x2C97, 'V'),
- (0x2C98, 'M', 'ⲙ'),
- (0x2C99, 'V'),
- (0x2C9A, 'M', 'ⲛ'),
- (0x2C9B, 'V'),
- (0x2C9C, 'M', 'ⲝ'),
- (0x2C9D, 'V'),
- (0x2C9E, 'M', 'ⲟ'),
- (0x2C9F, 'V'),
- (0x2CA0, 'M', 'ⲡ'),
- (0x2CA1, 'V'),
- (0x2CA2, 'M', 'ⲣ'),
- (0x2CA3, 'V'),
- (0x2CA4, 'M', 'ⲥ'),
- (0x2CA5, 'V'),
- (0x2CA6, 'M', 'ⲧ'),
- (0x2CA7, 'V'),
- (0x2CA8, 'M', 'ⲩ'),
- (0x2CA9, 'V'),
- (0x2CAA, 'M', 'ⲫ'),
- (0x2CAB, 'V'),
- (0x2CAC, 'M', 'ⲭ'),
- (0x2CAD, 'V'),
- (0x2CAE, 'M', 'ⲯ'),
- (0x2CAF, 'V'),
- (0x2CB0, 'M', 'ⲱ'),
- (0x2CB1, 'V'),
- (0x2CB2, 'M', 'ⲳ'),
- (0x2CB3, 'V'),
- (0x2CB4, 'M', 'ⲵ'),
- (0x2CB5, 'V'),
- (0x2CB6, 'M', 'ⲷ'),
- (0x2CB7, 'V'),
- (0x2CB8, 'M', 'ⲹ'),
- (0x2CB9, 'V'),
- (0x2CBA, 'M', 'ⲻ'),
- (0x2CBB, 'V'),
- (0x2CBC, 'M', 'ⲽ'),
- (0x2CBD, 'V'),
- (0x2CBE, 'M', 'ⲿ'),
- (0x2CBF, 'V'),
- (0x2CC0, 'M', 'ⳁ'),
- (0x2CC1, 'V'),
- (0x2CC2, 'M', 'ⳃ'),
- ]
-
-def _seg_26() -> List[Union[Tuple[int, str], Tuple[int, str, str]]]:
- return [
- (0x2CC3, 'V'),
- (0x2CC4, 'M', 'ⳅ'),
- (0x2CC5, 'V'),
- (0x2CC6, 'M', 'ⳇ'),
- (0x2CC7, 'V'),
- (0x2CC8, 'M', 'ⳉ'),
- (0x2CC9, 'V'),
- (0x2CCA, 'M', 'ⳋ'),
- (0x2CCB, 'V'),
- (0x2CCC, 'M', 'ⳍ'),
- (0x2CCD, 'V'),
- (0x2CCE, 'M', 'ⳏ'),
- (0x2CCF, 'V'),
- (0x2CD0, 'M', 'ⳑ'),
- (0x2CD1, 'V'),
- (0x2CD2, 'M', 'ⳓ'),
- (0x2CD3, 'V'),
- (0x2CD4, 'M', 'ⳕ'),
- (0x2CD5, 'V'),
- (0x2CD6, 'M', 'ⳗ'),
- (0x2CD7, 'V'),
- (0x2CD8, 'M', 'ⳙ'),
- (0x2CD9, 'V'),
- (0x2CDA, 'M', 'ⳛ'),
- (0x2CDB, 'V'),
- (0x2CDC, 'M', 'ⳝ'),
- (0x2CDD, 'V'),
- (0x2CDE, 'M', 'ⳟ'),
- (0x2CDF, 'V'),
- (0x2CE0, 'M', 'ⳡ'),
- (0x2CE1, 'V'),
- (0x2CE2, 'M', 'ⳣ'),
- (0x2CE3, 'V'),
- (0x2CEB, 'M', 'ⳬ'),
- (0x2CEC, 'V'),
- (0x2CED, 'M', 'ⳮ'),
- (0x2CEE, 'V'),
- (0x2CF2, 'M', 'ⳳ'),
- (0x2CF3, 'V'),
- (0x2CF4, 'X'),
- (0x2CF9, 'V'),
- (0x2D26, 'X'),
- (0x2D27, 'V'),
- (0x2D28, 'X'),
- (0x2D2D, 'V'),
- (0x2D2E, 'X'),
- (0x2D30, 'V'),
- (0x2D68, 'X'),
- (0x2D6F, 'M', 'ⵡ'),
- (0x2D70, 'V'),
- (0x2D71, 'X'),
- (0x2D7F, 'V'),
- (0x2D97, 'X'),
- (0x2DA0, 'V'),
- (0x2DA7, 'X'),
- (0x2DA8, 'V'),
- (0x2DAF, 'X'),
- (0x2DB0, 'V'),
- (0x2DB7, 'X'),
- (0x2DB8, 'V'),
- (0x2DBF, 'X'),
- (0x2DC0, 'V'),
- (0x2DC7, 'X'),
- (0x2DC8, 'V'),
- (0x2DCF, 'X'),
- (0x2DD0, 'V'),
- (0x2DD7, 'X'),
- (0x2DD8, 'V'),
- (0x2DDF, 'X'),
- (0x2DE0, 'V'),
- (0x2E5E, 'X'),
- (0x2E80, 'V'),
- (0x2E9A, 'X'),
- (0x2E9B, 'V'),
- (0x2E9F, 'M', '母'),
- (0x2EA0, 'V'),
- (0x2EF3, 'M', '龟'),
- (0x2EF4, 'X'),
- (0x2F00, 'M', '一'),
- (0x2F01, 'M', '丨'),
- (0x2F02, 'M', '丶'),
- (0x2F03, 'M', '丿'),
- (0x2F04, 'M', '乙'),
- (0x2F05, 'M', '亅'),
- (0x2F06, 'M', '二'),
- (0x2F07, 'M', '亠'),
- (0x2F08, 'M', '人'),
- (0x2F09, 'M', '儿'),
- (0x2F0A, 'M', '入'),
- (0x2F0B, 'M', '八'),
- (0x2F0C, 'M', '冂'),
- (0x2F0D, 'M', '冖'),
- (0x2F0E, 'M', '冫'),
- (0x2F0F, 'M', '几'),
- (0x2F10, 'M', '凵'),
- (0x2F11, 'M', '刀'),
- (0x2F12, 'M', '力'),
- (0x2F13, 'M', '勹'),
- (0x2F14, 'M', '匕'),
- (0x2F15, 'M', '匚'),
- ]
-
-def _seg_27() -> List[Union[Tuple[int, str], Tuple[int, str, str]]]:
- return [
- (0x2F16, 'M', '匸'),
- (0x2F17, 'M', '十'),
- (0x2F18, 'M', '卜'),
- (0x2F19, 'M', '卩'),
- (0x2F1A, 'M', '厂'),
- (0x2F1B, 'M', '厶'),
- (0x2F1C, 'M', '又'),
- (0x2F1D, 'M', '口'),
- (0x2F1E, 'M', '囗'),
- (0x2F1F, 'M', '土'),
- (0x2F20, 'M', '士'),
- (0x2F21, 'M', '夂'),
- (0x2F22, 'M', '夊'),
- (0x2F23, 'M', '夕'),
- (0x2F24, 'M', '大'),
- (0x2F25, 'M', '女'),
- (0x2F26, 'M', '子'),
- (0x2F27, 'M', '宀'),
- (0x2F28, 'M', '寸'),
- (0x2F29, 'M', '小'),
- (0x2F2A, 'M', '尢'),
- (0x2F2B, 'M', '尸'),
- (0x2F2C, 'M', '屮'),
- (0x2F2D, 'M', '山'),
- (0x2F2E, 'M', '巛'),
- (0x2F2F, 'M', '工'),
- (0x2F30, 'M', '己'),
- (0x2F31, 'M', '巾'),
- (0x2F32, 'M', '干'),
- (0x2F33, 'M', '幺'),
- (0x2F34, 'M', '广'),
- (0x2F35, 'M', '廴'),
- (0x2F36, 'M', '廾'),
- (0x2F37, 'M', '弋'),
- (0x2F38, 'M', '弓'),
- (0x2F39, 'M', '彐'),
- (0x2F3A, 'M', '彡'),
- (0x2F3B, 'M', '彳'),
- (0x2F3C, 'M', '心'),
- (0x2F3D, 'M', '戈'),
- (0x2F3E, 'M', '戶'),
- (0x2F3F, 'M', '手'),
- (0x2F40, 'M', '支'),
- (0x2F41, 'M', '攴'),
- (0x2F42, 'M', '文'),
- (0x2F43, 'M', '斗'),
- (0x2F44, 'M', '斤'),
- (0x2F45, 'M', '方'),
- (0x2F46, 'M', '无'),
- (0x2F47, 'M', '日'),
- (0x2F48, 'M', '曰'),
- (0x2F49, 'M', '月'),
- (0x2F4A, 'M', '木'),
- (0x2F4B, 'M', '欠'),
- (0x2F4C, 'M', '止'),
- (0x2F4D, 'M', '歹'),
- (0x2F4E, 'M', '殳'),
- (0x2F4F, 'M', '毋'),
- (0x2F50, 'M', '比'),
- (0x2F51, 'M', '毛'),
- (0x2F52, 'M', '氏'),
- (0x2F53, 'M', '气'),
- (0x2F54, 'M', '水'),
- (0x2F55, 'M', '火'),
- (0x2F56, 'M', '爪'),
- (0x2F57, 'M', '父'),
- (0x2F58, 'M', '爻'),
- (0x2F59, 'M', '爿'),
- (0x2F5A, 'M', '片'),
- (0x2F5B, 'M', '牙'),
- (0x2F5C, 'M', '牛'),
- (0x2F5D, 'M', '犬'),
- (0x2F5E, 'M', '玄'),
- (0x2F5F, 'M', '玉'),
- (0x2F60, 'M', '瓜'),
- (0x2F61, 'M', '瓦'),
- (0x2F62, 'M', '甘'),
- (0x2F63, 'M', '生'),
- (0x2F64, 'M', '用'),
- (0x2F65, 'M', '田'),
- (0x2F66, 'M', '疋'),
- (0x2F67, 'M', '疒'),
- (0x2F68, 'M', '癶'),
- (0x2F69, 'M', '白'),
- (0x2F6A, 'M', '皮'),
- (0x2F6B, 'M', '皿'),
- (0x2F6C, 'M', '目'),
- (0x2F6D, 'M', '矛'),
- (0x2F6E, 'M', '矢'),
- (0x2F6F, 'M', '石'),
- (0x2F70, 'M', '示'),
- (0x2F71, 'M', '禸'),
- (0x2F72, 'M', '禾'),
- (0x2F73, 'M', '穴'),
- (0x2F74, 'M', '立'),
- (0x2F75, 'M', '竹'),
- (0x2F76, 'M', '米'),
- (0x2F77, 'M', '糸'),
- (0x2F78, 'M', '缶'),
- (0x2F79, 'M', '网'),
- ]
-
-def _seg_28() -> List[Union[Tuple[int, str], Tuple[int, str, str]]]:
- return [
- (0x2F7A, 'M', '羊'),
- (0x2F7B, 'M', '羽'),
- (0x2F7C, 'M', '老'),
- (0x2F7D, 'M', '而'),
- (0x2F7E, 'M', '耒'),
- (0x2F7F, 'M', '耳'),
- (0x2F80, 'M', '聿'),
- (0x2F81, 'M', '肉'),
- (0x2F82, 'M', '臣'),
- (0x2F83, 'M', '自'),
- (0x2F84, 'M', '至'),
- (0x2F85, 'M', '臼'),
- (0x2F86, 'M', '舌'),
- (0x2F87, 'M', '舛'),
- (0x2F88, 'M', '舟'),
- (0x2F89, 'M', '艮'),
- (0x2F8A, 'M', '色'),
- (0x2F8B, 'M', '艸'),
- (0x2F8C, 'M', '虍'),
- (0x2F8D, 'M', '虫'),
- (0x2F8E, 'M', '血'),
- (0x2F8F, 'M', '行'),
- (0x2F90, 'M', '衣'),
- (0x2F91, 'M', '襾'),
- (0x2F92, 'M', '見'),
- (0x2F93, 'M', '角'),
- (0x2F94, 'M', '言'),
- (0x2F95, 'M', '谷'),
- (0x2F96, 'M', '豆'),
- (0x2F97, 'M', '豕'),
- (0x2F98, 'M', '豸'),
- (0x2F99, 'M', '貝'),
- (0x2F9A, 'M', '赤'),
- (0x2F9B, 'M', '走'),
- (0x2F9C, 'M', '足'),
- (0x2F9D, 'M', '身'),
- (0x2F9E, 'M', '車'),
- (0x2F9F, 'M', '辛'),
- (0x2FA0, 'M', '辰'),
- (0x2FA1, 'M', '辵'),
- (0x2FA2, 'M', '邑'),
- (0x2FA3, 'M', '酉'),
- (0x2FA4, 'M', '釆'),
- (0x2FA5, 'M', '里'),
- (0x2FA6, 'M', '金'),
- (0x2FA7, 'M', '長'),
- (0x2FA8, 'M', '門'),
- (0x2FA9, 'M', '阜'),
- (0x2FAA, 'M', '隶'),
- (0x2FAB, 'M', '隹'),
- (0x2FAC, 'M', '雨'),
- (0x2FAD, 'M', '靑'),
- (0x2FAE, 'M', '非'),
- (0x2FAF, 'M', '面'),
- (0x2FB0, 'M', '革'),
- (0x2FB1, 'M', '韋'),
- (0x2FB2, 'M', '韭'),
- (0x2FB3, 'M', '音'),
- (0x2FB4, 'M', '頁'),
- (0x2FB5, 'M', '風'),
- (0x2FB6, 'M', '飛'),
- (0x2FB7, 'M', '食'),
- (0x2FB8, 'M', '首'),
- (0x2FB9, 'M', '香'),
- (0x2FBA, 'M', '馬'),
- (0x2FBB, 'M', '骨'),
- (0x2FBC, 'M', '高'),
- (0x2FBD, 'M', '髟'),
- (0x2FBE, 'M', '鬥'),
- (0x2FBF, 'M', '鬯'),
- (0x2FC0, 'M', '鬲'),
- (0x2FC1, 'M', '鬼'),
- (0x2FC2, 'M', '魚'),
- (0x2FC3, 'M', '鳥'),
- (0x2FC4, 'M', '鹵'),
- (0x2FC5, 'M', '鹿'),
- (0x2FC6, 'M', '麥'),
- (0x2FC7, 'M', '麻'),
- (0x2FC8, 'M', '黃'),
- (0x2FC9, 'M', '黍'),
- (0x2FCA, 'M', '黑'),
- (0x2FCB, 'M', '黹'),
- (0x2FCC, 'M', '黽'),
- (0x2FCD, 'M', '鼎'),
- (0x2FCE, 'M', '鼓'),
- (0x2FCF, 'M', '鼠'),
- (0x2FD0, 'M', '鼻'),
- (0x2FD1, 'M', '齊'),
- (0x2FD2, 'M', '齒'),
- (0x2FD3, 'M', '龍'),
- (0x2FD4, 'M', '龜'),
- (0x2FD5, 'M', '龠'),
- (0x2FD6, 'X'),
- (0x3000, '3', ' '),
- (0x3001, 'V'),
- (0x3002, 'M', '.'),
- (0x3003, 'V'),
- (0x3036, 'M', '〒'),
- (0x3037, 'V'),
- (0x3038, 'M', '十'),
- ]
-
-def _seg_29() -> List[Union[Tuple[int, str], Tuple[int, str, str]]]:
- return [
- (0x3039, 'M', '卄'),
- (0x303A, 'M', '卅'),
- (0x303B, 'V'),
- (0x3040, 'X'),
- (0x3041, 'V'),
- (0x3097, 'X'),
- (0x3099, 'V'),
- (0x309B, '3', ' ゙'),
- (0x309C, '3', ' ゚'),
- (0x309D, 'V'),
- (0x309F, 'M', 'より'),
- (0x30A0, 'V'),
- (0x30FF, 'M', 'コト'),
- (0x3100, 'X'),
- (0x3105, 'V'),
- (0x3130, 'X'),
- (0x3131, 'M', 'ᄀ'),
- (0x3132, 'M', 'ᄁ'),
- (0x3133, 'M', 'ᆪ'),
- (0x3134, 'M', 'ᄂ'),
- (0x3135, 'M', 'ᆬ'),
- (0x3136, 'M', 'ᆭ'),
- (0x3137, 'M', 'ᄃ'),
- (0x3138, 'M', 'ᄄ'),
- (0x3139, 'M', 'ᄅ'),
- (0x313A, 'M', 'ᆰ'),
- (0x313B, 'M', 'ᆱ'),
- (0x313C, 'M', 'ᆲ'),
- (0x313D, 'M', 'ᆳ'),
- (0x313E, 'M', 'ᆴ'),
- (0x313F, 'M', 'ᆵ'),
- (0x3140, 'M', 'ᄚ'),
- (0x3141, 'M', 'ᄆ'),
- (0x3142, 'M', 'ᄇ'),
- (0x3143, 'M', 'ᄈ'),
- (0x3144, 'M', 'ᄡ'),
- (0x3145, 'M', 'ᄉ'),
- (0x3146, 'M', 'ᄊ'),
- (0x3147, 'M', 'ᄋ'),
- (0x3148, 'M', 'ᄌ'),
- (0x3149, 'M', 'ᄍ'),
- (0x314A, 'M', 'ᄎ'),
- (0x314B, 'M', 'ᄏ'),
- (0x314C, 'M', 'ᄐ'),
- (0x314D, 'M', 'ᄑ'),
- (0x314E, 'M', 'ᄒ'),
- (0x314F, 'M', 'ᅡ'),
- (0x3150, 'M', 'ᅢ'),
- (0x3151, 'M', 'ᅣ'),
- (0x3152, 'M', 'ᅤ'),
- (0x3153, 'M', 'ᅥ'),
- (0x3154, 'M', 'ᅦ'),
- (0x3155, 'M', 'ᅧ'),
- (0x3156, 'M', 'ᅨ'),
- (0x3157, 'M', 'ᅩ'),
- (0x3158, 'M', 'ᅪ'),
- (0x3159, 'M', 'ᅫ'),
- (0x315A, 'M', 'ᅬ'),
- (0x315B, 'M', 'ᅭ'),
- (0x315C, 'M', 'ᅮ'),
- (0x315D, 'M', 'ᅯ'),
- (0x315E, 'M', 'ᅰ'),
- (0x315F, 'M', 'ᅱ'),
- (0x3160, 'M', 'ᅲ'),
- (0x3161, 'M', 'ᅳ'),
- (0x3162, 'M', 'ᅴ'),
- (0x3163, 'M', 'ᅵ'),
- (0x3164, 'X'),
- (0x3165, 'M', 'ᄔ'),
- (0x3166, 'M', 'ᄕ'),
- (0x3167, 'M', 'ᇇ'),
- (0x3168, 'M', 'ᇈ'),
- (0x3169, 'M', 'ᇌ'),
- (0x316A, 'M', 'ᇎ'),
- (0x316B, 'M', 'ᇓ'),
- (0x316C, 'M', 'ᇗ'),
- (0x316D, 'M', 'ᇙ'),
- (0x316E, 'M', 'ᄜ'),
- (0x316F, 'M', 'ᇝ'),
- (0x3170, 'M', 'ᇟ'),
- (0x3171, 'M', 'ᄝ'),
- (0x3172, 'M', 'ᄞ'),
- (0x3173, 'M', 'ᄠ'),
- (0x3174, 'M', 'ᄢ'),
- (0x3175, 'M', 'ᄣ'),
- (0x3176, 'M', 'ᄧ'),
- (0x3177, 'M', 'ᄩ'),
- (0x3178, 'M', 'ᄫ'),
- (0x3179, 'M', 'ᄬ'),
- (0x317A, 'M', 'ᄭ'),
- (0x317B, 'M', 'ᄮ'),
- (0x317C, 'M', 'ᄯ'),
- (0x317D, 'M', 'ᄲ'),
- (0x317E, 'M', 'ᄶ'),
- (0x317F, 'M', 'ᅀ'),
- (0x3180, 'M', 'ᅇ'),
- (0x3181, 'M', 'ᅌ'),
- (0x3182, 'M', 'ᇱ'),
- (0x3183, 'M', 'ᇲ'),
- (0x3184, 'M', 'ᅗ'),
- ]
-
-def _seg_30() -> List[Union[Tuple[int, str], Tuple[int, str, str]]]:
- return [
- (0x3185, 'M', 'ᅘ'),
- (0x3186, 'M', 'ᅙ'),
- (0x3187, 'M', 'ᆄ'),
- (0x3188, 'M', 'ᆅ'),
- (0x3189, 'M', 'ᆈ'),
- (0x318A, 'M', 'ᆑ'),
- (0x318B, 'M', 'ᆒ'),
- (0x318C, 'M', 'ᆔ'),
- (0x318D, 'M', 'ᆞ'),
- (0x318E, 'M', 'ᆡ'),
- (0x318F, 'X'),
- (0x3190, 'V'),
- (0x3192, 'M', '一'),
- (0x3193, 'M', '二'),
- (0x3194, 'M', '三'),
- (0x3195, 'M', '四'),
- (0x3196, 'M', '上'),
- (0x3197, 'M', '中'),
- (0x3198, 'M', '下'),
- (0x3199, 'M', '甲'),
- (0x319A, 'M', '乙'),
- (0x319B, 'M', '丙'),
- (0x319C, 'M', '丁'),
- (0x319D, 'M', '天'),
- (0x319E, 'M', '地'),
- (0x319F, 'M', '人'),
- (0x31A0, 'V'),
- (0x31E4, 'X'),
- (0x31F0, 'V'),
- (0x3200, '3', '(ᄀ)'),
- (0x3201, '3', '(ᄂ)'),
- (0x3202, '3', '(ᄃ)'),
- (0x3203, '3', '(ᄅ)'),
- (0x3204, '3', '(ᄆ)'),
- (0x3205, '3', '(ᄇ)'),
- (0x3206, '3', '(ᄉ)'),
- (0x3207, '3', '(ᄋ)'),
- (0x3208, '3', '(ᄌ)'),
- (0x3209, '3', '(ᄎ)'),
- (0x320A, '3', '(ᄏ)'),
- (0x320B, '3', '(ᄐ)'),
- (0x320C, '3', '(ᄑ)'),
- (0x320D, '3', '(ᄒ)'),
- (0x320E, '3', '(가)'),
- (0x320F, '3', '(나)'),
- (0x3210, '3', '(다)'),
- (0x3211, '3', '(라)'),
- (0x3212, '3', '(마)'),
- (0x3213, '3', '(바)'),
- (0x3214, '3', '(사)'),
- (0x3215, '3', '(아)'),
- (0x3216, '3', '(자)'),
- (0x3217, '3', '(차)'),
- (0x3218, '3', '(카)'),
- (0x3219, '3', '(타)'),
- (0x321A, '3', '(파)'),
- (0x321B, '3', '(하)'),
- (0x321C, '3', '(주)'),
- (0x321D, '3', '(오전)'),
- (0x321E, '3', '(오후)'),
- (0x321F, 'X'),
- (0x3220, '3', '(一)'),
- (0x3221, '3', '(二)'),
- (0x3222, '3', '(三)'),
- (0x3223, '3', '(四)'),
- (0x3224, '3', '(五)'),
- (0x3225, '3', '(六)'),
- (0x3226, '3', '(七)'),
- (0x3227, '3', '(八)'),
- (0x3228, '3', '(九)'),
- (0x3229, '3', '(十)'),
- (0x322A, '3', '(月)'),
- (0x322B, '3', '(火)'),
- (0x322C, '3', '(水)'),
- (0x322D, '3', '(木)'),
- (0x322E, '3', '(金)'),
- (0x322F, '3', '(土)'),
- (0x3230, '3', '(日)'),
- (0x3231, '3', '(株)'),
- (0x3232, '3', '(有)'),
- (0x3233, '3', '(社)'),
- (0x3234, '3', '(名)'),
- (0x3235, '3', '(特)'),
- (0x3236, '3', '(財)'),
- (0x3237, '3', '(祝)'),
- (0x3238, '3', '(労)'),
- (0x3239, '3', '(代)'),
- (0x323A, '3', '(呼)'),
- (0x323B, '3', '(学)'),
- (0x323C, '3', '(監)'),
- (0x323D, '3', '(企)'),
- (0x323E, '3', '(資)'),
- (0x323F, '3', '(協)'),
- (0x3240, '3', '(祭)'),
- (0x3241, '3', '(休)'),
- (0x3242, '3', '(自)'),
- (0x3243, '3', '(至)'),
- (0x3244, 'M', '問'),
- (0x3245, 'M', '幼'),
- (0x3246, 'M', '文'),
- ]
-
-def _seg_31() -> List[Union[Tuple[int, str], Tuple[int, str, str]]]:
- return [
- (0x3247, 'M', '箏'),
- (0x3248, 'V'),
- (0x3250, 'M', 'pte'),
- (0x3251, 'M', '21'),
- (0x3252, 'M', '22'),
- (0x3253, 'M', '23'),
- (0x3254, 'M', '24'),
- (0x3255, 'M', '25'),
- (0x3256, 'M', '26'),
- (0x3257, 'M', '27'),
- (0x3258, 'M', '28'),
- (0x3259, 'M', '29'),
- (0x325A, 'M', '30'),
- (0x325B, 'M', '31'),
- (0x325C, 'M', '32'),
- (0x325D, 'M', '33'),
- (0x325E, 'M', '34'),
- (0x325F, 'M', '35'),
- (0x3260, 'M', 'ᄀ'),
- (0x3261, 'M', 'ᄂ'),
- (0x3262, 'M', 'ᄃ'),
- (0x3263, 'M', 'ᄅ'),
- (0x3264, 'M', 'ᄆ'),
- (0x3265, 'M', 'ᄇ'),
- (0x3266, 'M', 'ᄉ'),
- (0x3267, 'M', 'ᄋ'),
- (0x3268, 'M', 'ᄌ'),
- (0x3269, 'M', 'ᄎ'),
- (0x326A, 'M', 'ᄏ'),
- (0x326B, 'M', 'ᄐ'),
- (0x326C, 'M', 'ᄑ'),
- (0x326D, 'M', 'ᄒ'),
- (0x326E, 'M', '가'),
- (0x326F, 'M', '나'),
- (0x3270, 'M', '다'),
- (0x3271, 'M', '라'),
- (0x3272, 'M', '마'),
- (0x3273, 'M', '바'),
- (0x3274, 'M', '사'),
- (0x3275, 'M', '아'),
- (0x3276, 'M', '자'),
- (0x3277, 'M', '차'),
- (0x3278, 'M', '카'),
- (0x3279, 'M', '타'),
- (0x327A, 'M', '파'),
- (0x327B, 'M', '하'),
- (0x327C, 'M', '참고'),
- (0x327D, 'M', '주의'),
- (0x327E, 'M', '우'),
- (0x327F, 'V'),
- (0x3280, 'M', '一'),
- (0x3281, 'M', '二'),
- (0x3282, 'M', '三'),
- (0x3283, 'M', '四'),
- (0x3284, 'M', '五'),
- (0x3285, 'M', '六'),
- (0x3286, 'M', '七'),
- (0x3287, 'M', '八'),
- (0x3288, 'M', '九'),
- (0x3289, 'M', '十'),
- (0x328A, 'M', '月'),
- (0x328B, 'M', '火'),
- (0x328C, 'M', '水'),
- (0x328D, 'M', '木'),
- (0x328E, 'M', '金'),
- (0x328F, 'M', '土'),
- (0x3290, 'M', '日'),
- (0x3291, 'M', '株'),
- (0x3292, 'M', '有'),
- (0x3293, 'M', '社'),
- (0x3294, 'M', '名'),
- (0x3295, 'M', '特'),
- (0x3296, 'M', '財'),
- (0x3297, 'M', '祝'),
- (0x3298, 'M', '労'),
- (0x3299, 'M', '秘'),
- (0x329A, 'M', '男'),
- (0x329B, 'M', '女'),
- (0x329C, 'M', '適'),
- (0x329D, 'M', '優'),
- (0x329E, 'M', '印'),
- (0x329F, 'M', '注'),
- (0x32A0, 'M', '項'),
- (0x32A1, 'M', '休'),
- (0x32A2, 'M', '写'),
- (0x32A3, 'M', '正'),
- (0x32A4, 'M', '上'),
- (0x32A5, 'M', '中'),
- (0x32A6, 'M', '下'),
- (0x32A7, 'M', '左'),
- (0x32A8, 'M', '右'),
- (0x32A9, 'M', '医'),
- (0x32AA, 'M', '宗'),
- (0x32AB, 'M', '学'),
- (0x32AC, 'M', '監'),
- (0x32AD, 'M', '企'),
- (0x32AE, 'M', '資'),
- (0x32AF, 'M', '協'),
- (0x32B0, 'M', '夜'),
- (0x32B1, 'M', '36'),
- ]
-
-def _seg_32() -> List[Union[Tuple[int, str], Tuple[int, str, str]]]:
- return [
- (0x32B2, 'M', '37'),
- (0x32B3, 'M', '38'),
- (0x32B4, 'M', '39'),
- (0x32B5, 'M', '40'),
- (0x32B6, 'M', '41'),
- (0x32B7, 'M', '42'),
- (0x32B8, 'M', '43'),
- (0x32B9, 'M', '44'),
- (0x32BA, 'M', '45'),
- (0x32BB, 'M', '46'),
- (0x32BC, 'M', '47'),
- (0x32BD, 'M', '48'),
- (0x32BE, 'M', '49'),
- (0x32BF, 'M', '50'),
- (0x32C0, 'M', '1月'),
- (0x32C1, 'M', '2月'),
- (0x32C2, 'M', '3月'),
- (0x32C3, 'M', '4月'),
- (0x32C4, 'M', '5月'),
- (0x32C5, 'M', '6月'),
- (0x32C6, 'M', '7月'),
- (0x32C7, 'M', '8月'),
- (0x32C8, 'M', '9月'),
- (0x32C9, 'M', '10月'),
- (0x32CA, 'M', '11月'),
- (0x32CB, 'M', '12月'),
- (0x32CC, 'M', 'hg'),
- (0x32CD, 'M', 'erg'),
- (0x32CE, 'M', 'ev'),
- (0x32CF, 'M', 'ltd'),
- (0x32D0, 'M', 'ア'),
- (0x32D1, 'M', 'イ'),
- (0x32D2, 'M', 'ウ'),
- (0x32D3, 'M', 'エ'),
- (0x32D4, 'M', 'オ'),
- (0x32D5, 'M', 'カ'),
- (0x32D6, 'M', 'キ'),
- (0x32D7, 'M', 'ク'),
- (0x32D8, 'M', 'ケ'),
- (0x32D9, 'M', 'コ'),
- (0x32DA, 'M', 'サ'),
- (0x32DB, 'M', 'シ'),
- (0x32DC, 'M', 'ス'),
- (0x32DD, 'M', 'セ'),
- (0x32DE, 'M', 'ソ'),
- (0x32DF, 'M', 'タ'),
- (0x32E0, 'M', 'チ'),
- (0x32E1, 'M', 'ツ'),
- (0x32E2, 'M', 'テ'),
- (0x32E3, 'M', 'ト'),
- (0x32E4, 'M', 'ナ'),
- (0x32E5, 'M', 'ニ'),
- (0x32E6, 'M', 'ヌ'),
- (0x32E7, 'M', 'ネ'),
- (0x32E8, 'M', 'ノ'),
- (0x32E9, 'M', 'ハ'),
- (0x32EA, 'M', 'ヒ'),
- (0x32EB, 'M', 'フ'),
- (0x32EC, 'M', 'ヘ'),
- (0x32ED, 'M', 'ホ'),
- (0x32EE, 'M', 'マ'),
- (0x32EF, 'M', 'ミ'),
- (0x32F0, 'M', 'ム'),
- (0x32F1, 'M', 'メ'),
- (0x32F2, 'M', 'モ'),
- (0x32F3, 'M', 'ヤ'),
- (0x32F4, 'M', 'ユ'),
- (0x32F5, 'M', 'ヨ'),
- (0x32F6, 'M', 'ラ'),
- (0x32F7, 'M', 'リ'),
- (0x32F8, 'M', 'ル'),
- (0x32F9, 'M', 'レ'),
- (0x32FA, 'M', 'ロ'),
- (0x32FB, 'M', 'ワ'),
- (0x32FC, 'M', 'ヰ'),
- (0x32FD, 'M', 'ヱ'),
- (0x32FE, 'M', 'ヲ'),
- (0x32FF, 'M', '令和'),
- (0x3300, 'M', 'アパート'),
- (0x3301, 'M', 'アルファ'),
- (0x3302, 'M', 'アンペア'),
- (0x3303, 'M', 'アール'),
- (0x3304, 'M', 'イニング'),
- (0x3305, 'M', 'インチ'),
- (0x3306, 'M', 'ウォン'),
- (0x3307, 'M', 'エスクード'),
- (0x3308, 'M', 'エーカー'),
- (0x3309, 'M', 'オンス'),
- (0x330A, 'M', 'オーム'),
- (0x330B, 'M', 'カイリ'),
- (0x330C, 'M', 'カラット'),
- (0x330D, 'M', 'カロリー'),
- (0x330E, 'M', 'ガロン'),
- (0x330F, 'M', 'ガンマ'),
- (0x3310, 'M', 'ギガ'),
- (0x3311, 'M', 'ギニー'),
- (0x3312, 'M', 'キュリー'),
- (0x3313, 'M', 'ギルダー'),
- (0x3314, 'M', 'キロ'),
- (0x3315, 'M', 'キログラム'),
- ]
-
-def _seg_33() -> List[Union[Tuple[int, str], Tuple[int, str, str]]]:
- return [
- (0x3316, 'M', 'キロメートル'),
- (0x3317, 'M', 'キロワット'),
- (0x3318, 'M', 'グラム'),
- (0x3319, 'M', 'グラムトン'),
- (0x331A, 'M', 'クルゼイロ'),
- (0x331B, 'M', 'クローネ'),
- (0x331C, 'M', 'ケース'),
- (0x331D, 'M', 'コルナ'),
- (0x331E, 'M', 'コーポ'),
- (0x331F, 'M', 'サイクル'),
- (0x3320, 'M', 'サンチーム'),
- (0x3321, 'M', 'シリング'),
- (0x3322, 'M', 'センチ'),
- (0x3323, 'M', 'セント'),
- (0x3324, 'M', 'ダース'),
- (0x3325, 'M', 'デシ'),
- (0x3326, 'M', 'ドル'),
- (0x3327, 'M', 'トン'),
- (0x3328, 'M', 'ナノ'),
- (0x3329, 'M', 'ノット'),
- (0x332A, 'M', 'ハイツ'),
- (0x332B, 'M', 'パーセント'),
- (0x332C, 'M', 'パーツ'),
- (0x332D, 'M', 'バーレル'),
- (0x332E, 'M', 'ピアストル'),
- (0x332F, 'M', 'ピクル'),
- (0x3330, 'M', 'ピコ'),
- (0x3331, 'M', 'ビル'),
- (0x3332, 'M', 'ファラッド'),
- (0x3333, 'M', 'フィート'),
- (0x3334, 'M', 'ブッシェル'),
- (0x3335, 'M', 'フラン'),
- (0x3336, 'M', 'ヘクタール'),
- (0x3337, 'M', 'ペソ'),
- (0x3338, 'M', 'ペニヒ'),
- (0x3339, 'M', 'ヘルツ'),
- (0x333A, 'M', 'ペンス'),
- (0x333B, 'M', 'ページ'),
- (0x333C, 'M', 'ベータ'),
- (0x333D, 'M', 'ポイント'),
- (0x333E, 'M', 'ボルト'),
- (0x333F, 'M', 'ホン'),
- (0x3340, 'M', 'ポンド'),
- (0x3341, 'M', 'ホール'),
- (0x3342, 'M', 'ホーン'),
- (0x3343, 'M', 'マイクロ'),
- (0x3344, 'M', 'マイル'),
- (0x3345, 'M', 'マッハ'),
- (0x3346, 'M', 'マルク'),
- (0x3347, 'M', 'マンション'),
- (0x3348, 'M', 'ミクロン'),
- (0x3349, 'M', 'ミリ'),
- (0x334A, 'M', 'ミリバール'),
- (0x334B, 'M', 'メガ'),
- (0x334C, 'M', 'メガトン'),
- (0x334D, 'M', 'メートル'),
- (0x334E, 'M', 'ヤード'),
- (0x334F, 'M', 'ヤール'),
- (0x3350, 'M', 'ユアン'),
- (0x3351, 'M', 'リットル'),
- (0x3352, 'M', 'リラ'),
- (0x3353, 'M', 'ルピー'),
- (0x3354, 'M', 'ルーブル'),
- (0x3355, 'M', 'レム'),
- (0x3356, 'M', 'レントゲン'),
- (0x3357, 'M', 'ワット'),
- (0x3358, 'M', '0点'),
- (0x3359, 'M', '1点'),
- (0x335A, 'M', '2点'),
- (0x335B, 'M', '3点'),
- (0x335C, 'M', '4点'),
- (0x335D, 'M', '5点'),
- (0x335E, 'M', '6点'),
- (0x335F, 'M', '7点'),
- (0x3360, 'M', '8点'),
- (0x3361, 'M', '9点'),
- (0x3362, 'M', '10点'),
- (0x3363, 'M', '11点'),
- (0x3364, 'M', '12点'),
- (0x3365, 'M', '13点'),
- (0x3366, 'M', '14点'),
- (0x3367, 'M', '15点'),
- (0x3368, 'M', '16点'),
- (0x3369, 'M', '17点'),
- (0x336A, 'M', '18点'),
- (0x336B, 'M', '19点'),
- (0x336C, 'M', '20点'),
- (0x336D, 'M', '21点'),
- (0x336E, 'M', '22点'),
- (0x336F, 'M', '23点'),
- (0x3370, 'M', '24点'),
- (0x3371, 'M', 'hpa'),
- (0x3372, 'M', 'da'),
- (0x3373, 'M', 'au'),
- (0x3374, 'M', 'bar'),
- (0x3375, 'M', 'ov'),
- (0x3376, 'M', 'pc'),
- (0x3377, 'M', 'dm'),
- (0x3378, 'M', 'dm2'),
- (0x3379, 'M', 'dm3'),
- ]
-
-def _seg_34() -> List[Union[Tuple[int, str], Tuple[int, str, str]]]:
- return [
- (0x337A, 'M', 'iu'),
- (0x337B, 'M', '平成'),
- (0x337C, 'M', '昭和'),
- (0x337D, 'M', '大正'),
- (0x337E, 'M', '明治'),
- (0x337F, 'M', '株式会社'),
- (0x3380, 'M', 'pa'),
- (0x3381, 'M', 'na'),
- (0x3382, 'M', 'μa'),
- (0x3383, 'M', 'ma'),
- (0x3384, 'M', 'ka'),
- (0x3385, 'M', 'kb'),
- (0x3386, 'M', 'mb'),
- (0x3387, 'M', 'gb'),
- (0x3388, 'M', 'cal'),
- (0x3389, 'M', 'kcal'),
- (0x338A, 'M', 'pf'),
- (0x338B, 'M', 'nf'),
- (0x338C, 'M', 'μf'),
- (0x338D, 'M', 'μg'),
- (0x338E, 'M', 'mg'),
- (0x338F, 'M', 'kg'),
- (0x3390, 'M', 'hz'),
- (0x3391, 'M', 'khz'),
- (0x3392, 'M', 'mhz'),
- (0x3393, 'M', 'ghz'),
- (0x3394, 'M', 'thz'),
- (0x3395, 'M', 'μl'),
- (0x3396, 'M', 'ml'),
- (0x3397, 'M', 'dl'),
- (0x3398, 'M', 'kl'),
- (0x3399, 'M', 'fm'),
- (0x339A, 'M', 'nm'),
- (0x339B, 'M', 'μm'),
- (0x339C, 'M', 'mm'),
- (0x339D, 'M', 'cm'),
- (0x339E, 'M', 'km'),
- (0x339F, 'M', 'mm2'),
- (0x33A0, 'M', 'cm2'),
- (0x33A1, 'M', 'm2'),
- (0x33A2, 'M', 'km2'),
- (0x33A3, 'M', 'mm3'),
- (0x33A4, 'M', 'cm3'),
- (0x33A5, 'M', 'm3'),
- (0x33A6, 'M', 'km3'),
- (0x33A7, 'M', 'm∕s'),
- (0x33A8, 'M', 'm∕s2'),
- (0x33A9, 'M', 'pa'),
- (0x33AA, 'M', 'kpa'),
- (0x33AB, 'M', 'mpa'),
- (0x33AC, 'M', 'gpa'),
- (0x33AD, 'M', 'rad'),
- (0x33AE, 'M', 'rad∕s'),
- (0x33AF, 'M', 'rad∕s2'),
- (0x33B0, 'M', 'ps'),
- (0x33B1, 'M', 'ns'),
- (0x33B2, 'M', 'μs'),
- (0x33B3, 'M', 'ms'),
- (0x33B4, 'M', 'pv'),
- (0x33B5, 'M', 'nv'),
- (0x33B6, 'M', 'μv'),
- (0x33B7, 'M', 'mv'),
- (0x33B8, 'M', 'kv'),
- (0x33B9, 'M', 'mv'),
- (0x33BA, 'M', 'pw'),
- (0x33BB, 'M', 'nw'),
- (0x33BC, 'M', 'μw'),
- (0x33BD, 'M', 'mw'),
- (0x33BE, 'M', 'kw'),
- (0x33BF, 'M', 'mw'),
- (0x33C0, 'M', 'kω'),
- (0x33C1, 'M', 'mω'),
- (0x33C2, 'X'),
- (0x33C3, 'M', 'bq'),
- (0x33C4, 'M', 'cc'),
- (0x33C5, 'M', 'cd'),
- (0x33C6, 'M', 'c∕kg'),
- (0x33C7, 'X'),
- (0x33C8, 'M', 'db'),
- (0x33C9, 'M', 'gy'),
- (0x33CA, 'M', 'ha'),
- (0x33CB, 'M', 'hp'),
- (0x33CC, 'M', 'in'),
- (0x33CD, 'M', 'kk'),
- (0x33CE, 'M', 'km'),
- (0x33CF, 'M', 'kt'),
- (0x33D0, 'M', 'lm'),
- (0x33D1, 'M', 'ln'),
- (0x33D2, 'M', 'log'),
- (0x33D3, 'M', 'lx'),
- (0x33D4, 'M', 'mb'),
- (0x33D5, 'M', 'mil'),
- (0x33D6, 'M', 'mol'),
- (0x33D7, 'M', 'ph'),
- (0x33D8, 'X'),
- (0x33D9, 'M', 'ppm'),
- (0x33DA, 'M', 'pr'),
- (0x33DB, 'M', 'sr'),
- (0x33DC, 'M', 'sv'),
- (0x33DD, 'M', 'wb'),
- ]
-
-def _seg_35() -> List[Union[Tuple[int, str], Tuple[int, str, str]]]:
- return [
- (0x33DE, 'M', 'v∕m'),
- (0x33DF, 'M', 'a∕m'),
- (0x33E0, 'M', '1日'),
- (0x33E1, 'M', '2日'),
- (0x33E2, 'M', '3日'),
- (0x33E3, 'M', '4日'),
- (0x33E4, 'M', '5日'),
- (0x33E5, 'M', '6日'),
- (0x33E6, 'M', '7日'),
- (0x33E7, 'M', '8日'),
- (0x33E8, 'M', '9日'),
- (0x33E9, 'M', '10日'),
- (0x33EA, 'M', '11日'),
- (0x33EB, 'M', '12日'),
- (0x33EC, 'M', '13日'),
- (0x33ED, 'M', '14日'),
- (0x33EE, 'M', '15日'),
- (0x33EF, 'M', '16日'),
- (0x33F0, 'M', '17日'),
- (0x33F1, 'M', '18日'),
- (0x33F2, 'M', '19日'),
- (0x33F3, 'M', '20日'),
- (0x33F4, 'M', '21日'),
- (0x33F5, 'M', '22日'),
- (0x33F6, 'M', '23日'),
- (0x33F7, 'M', '24日'),
- (0x33F8, 'M', '25日'),
- (0x33F9, 'M', '26日'),
- (0x33FA, 'M', '27日'),
- (0x33FB, 'M', '28日'),
- (0x33FC, 'M', '29日'),
- (0x33FD, 'M', '30日'),
- (0x33FE, 'M', '31日'),
- (0x33FF, 'M', 'gal'),
- (0x3400, 'V'),
- (0xA48D, 'X'),
- (0xA490, 'V'),
- (0xA4C7, 'X'),
- (0xA4D0, 'V'),
- (0xA62C, 'X'),
- (0xA640, 'M', 'ꙁ'),
- (0xA641, 'V'),
- (0xA642, 'M', 'ꙃ'),
- (0xA643, 'V'),
- (0xA644, 'M', 'ꙅ'),
- (0xA645, 'V'),
- (0xA646, 'M', 'ꙇ'),
- (0xA647, 'V'),
- (0xA648, 'M', 'ꙉ'),
- (0xA649, 'V'),
- (0xA64A, 'M', 'ꙋ'),
- (0xA64B, 'V'),
- (0xA64C, 'M', 'ꙍ'),
- (0xA64D, 'V'),
- (0xA64E, 'M', 'ꙏ'),
- (0xA64F, 'V'),
- (0xA650, 'M', 'ꙑ'),
- (0xA651, 'V'),
- (0xA652, 'M', 'ꙓ'),
- (0xA653, 'V'),
- (0xA654, 'M', 'ꙕ'),
- (0xA655, 'V'),
- (0xA656, 'M', 'ꙗ'),
- (0xA657, 'V'),
- (0xA658, 'M', 'ꙙ'),
- (0xA659, 'V'),
- (0xA65A, 'M', 'ꙛ'),
- (0xA65B, 'V'),
- (0xA65C, 'M', 'ꙝ'),
- (0xA65D, 'V'),
- (0xA65E, 'M', 'ꙟ'),
- (0xA65F, 'V'),
- (0xA660, 'M', 'ꙡ'),
- (0xA661, 'V'),
- (0xA662, 'M', 'ꙣ'),
- (0xA663, 'V'),
- (0xA664, 'M', 'ꙥ'),
- (0xA665, 'V'),
- (0xA666, 'M', 'ꙧ'),
- (0xA667, 'V'),
- (0xA668, 'M', 'ꙩ'),
- (0xA669, 'V'),
- (0xA66A, 'M', 'ꙫ'),
- (0xA66B, 'V'),
- (0xA66C, 'M', 'ꙭ'),
- (0xA66D, 'V'),
- (0xA680, 'M', 'ꚁ'),
- (0xA681, 'V'),
- (0xA682, 'M', 'ꚃ'),
- (0xA683, 'V'),
- (0xA684, 'M', 'ꚅ'),
- (0xA685, 'V'),
- (0xA686, 'M', 'ꚇ'),
- (0xA687, 'V'),
- (0xA688, 'M', 'ꚉ'),
- (0xA689, 'V'),
- (0xA68A, 'M', 'ꚋ'),
- (0xA68B, 'V'),
- (0xA68C, 'M', 'ꚍ'),
- (0xA68D, 'V'),
- ]
-
-def _seg_36() -> List[Union[Tuple[int, str], Tuple[int, str, str]]]:
- return [
- (0xA68E, 'M', 'ꚏ'),
- (0xA68F, 'V'),
- (0xA690, 'M', 'ꚑ'),
- (0xA691, 'V'),
- (0xA692, 'M', 'ꚓ'),
- (0xA693, 'V'),
- (0xA694, 'M', 'ꚕ'),
- (0xA695, 'V'),
- (0xA696, 'M', 'ꚗ'),
- (0xA697, 'V'),
- (0xA698, 'M', 'ꚙ'),
- (0xA699, 'V'),
- (0xA69A, 'M', 'ꚛ'),
- (0xA69B, 'V'),
- (0xA69C, 'M', 'ъ'),
- (0xA69D, 'M', 'ь'),
- (0xA69E, 'V'),
- (0xA6F8, 'X'),
- (0xA700, 'V'),
- (0xA722, 'M', 'ꜣ'),
- (0xA723, 'V'),
- (0xA724, 'M', 'ꜥ'),
- (0xA725, 'V'),
- (0xA726, 'M', 'ꜧ'),
- (0xA727, 'V'),
- (0xA728, 'M', 'ꜩ'),
- (0xA729, 'V'),
- (0xA72A, 'M', 'ꜫ'),
- (0xA72B, 'V'),
- (0xA72C, 'M', 'ꜭ'),
- (0xA72D, 'V'),
- (0xA72E, 'M', 'ꜯ'),
- (0xA72F, 'V'),
- (0xA732, 'M', 'ꜳ'),
- (0xA733, 'V'),
- (0xA734, 'M', 'ꜵ'),
- (0xA735, 'V'),
- (0xA736, 'M', 'ꜷ'),
- (0xA737, 'V'),
- (0xA738, 'M', 'ꜹ'),
- (0xA739, 'V'),
- (0xA73A, 'M', 'ꜻ'),
- (0xA73B, 'V'),
- (0xA73C, 'M', 'ꜽ'),
- (0xA73D, 'V'),
- (0xA73E, 'M', 'ꜿ'),
- (0xA73F, 'V'),
- (0xA740, 'M', 'ꝁ'),
- (0xA741, 'V'),
- (0xA742, 'M', 'ꝃ'),
- (0xA743, 'V'),
- (0xA744, 'M', 'ꝅ'),
- (0xA745, 'V'),
- (0xA746, 'M', 'ꝇ'),
- (0xA747, 'V'),
- (0xA748, 'M', 'ꝉ'),
- (0xA749, 'V'),
- (0xA74A, 'M', 'ꝋ'),
- (0xA74B, 'V'),
- (0xA74C, 'M', 'ꝍ'),
- (0xA74D, 'V'),
- (0xA74E, 'M', 'ꝏ'),
- (0xA74F, 'V'),
- (0xA750, 'M', 'ꝑ'),
- (0xA751, 'V'),
- (0xA752, 'M', 'ꝓ'),
- (0xA753, 'V'),
- (0xA754, 'M', 'ꝕ'),
- (0xA755, 'V'),
- (0xA756, 'M', 'ꝗ'),
- (0xA757, 'V'),
- (0xA758, 'M', 'ꝙ'),
- (0xA759, 'V'),
- (0xA75A, 'M', 'ꝛ'),
- (0xA75B, 'V'),
- (0xA75C, 'M', 'ꝝ'),
- (0xA75D, 'V'),
- (0xA75E, 'M', 'ꝟ'),
- (0xA75F, 'V'),
- (0xA760, 'M', 'ꝡ'),
- (0xA761, 'V'),
- (0xA762, 'M', 'ꝣ'),
- (0xA763, 'V'),
- (0xA764, 'M', 'ꝥ'),
- (0xA765, 'V'),
- (0xA766, 'M', 'ꝧ'),
- (0xA767, 'V'),
- (0xA768, 'M', 'ꝩ'),
- (0xA769, 'V'),
- (0xA76A, 'M', 'ꝫ'),
- (0xA76B, 'V'),
- (0xA76C, 'M', 'ꝭ'),
- (0xA76D, 'V'),
- (0xA76E, 'M', 'ꝯ'),
- (0xA76F, 'V'),
- (0xA770, 'M', 'ꝯ'),
- (0xA771, 'V'),
- (0xA779, 'M', 'ꝺ'),
- (0xA77A, 'V'),
- (0xA77B, 'M', 'ꝼ'),
- ]
-
-def _seg_37() -> List[Union[Tuple[int, str], Tuple[int, str, str]]]:
- return [
- (0xA77C, 'V'),
- (0xA77D, 'M', 'ᵹ'),
- (0xA77E, 'M', 'ꝿ'),
- (0xA77F, 'V'),
- (0xA780, 'M', 'ꞁ'),
- (0xA781, 'V'),
- (0xA782, 'M', 'ꞃ'),
- (0xA783, 'V'),
- (0xA784, 'M', 'ꞅ'),
- (0xA785, 'V'),
- (0xA786, 'M', 'ꞇ'),
- (0xA787, 'V'),
- (0xA78B, 'M', 'ꞌ'),
- (0xA78C, 'V'),
- (0xA78D, 'M', 'ɥ'),
- (0xA78E, 'V'),
- (0xA790, 'M', 'ꞑ'),
- (0xA791, 'V'),
- (0xA792, 'M', 'ꞓ'),
- (0xA793, 'V'),
- (0xA796, 'M', 'ꞗ'),
- (0xA797, 'V'),
- (0xA798, 'M', 'ꞙ'),
- (0xA799, 'V'),
- (0xA79A, 'M', 'ꞛ'),
- (0xA79B, 'V'),
- (0xA79C, 'M', 'ꞝ'),
- (0xA79D, 'V'),
- (0xA79E, 'M', 'ꞟ'),
- (0xA79F, 'V'),
- (0xA7A0, 'M', 'ꞡ'),
- (0xA7A1, 'V'),
- (0xA7A2, 'M', 'ꞣ'),
- (0xA7A3, 'V'),
- (0xA7A4, 'M', 'ꞥ'),
- (0xA7A5, 'V'),
- (0xA7A6, 'M', 'ꞧ'),
- (0xA7A7, 'V'),
- (0xA7A8, 'M', 'ꞩ'),
- (0xA7A9, 'V'),
- (0xA7AA, 'M', 'ɦ'),
- (0xA7AB, 'M', 'ɜ'),
- (0xA7AC, 'M', 'ɡ'),
- (0xA7AD, 'M', 'ɬ'),
- (0xA7AE, 'M', 'ɪ'),
- (0xA7AF, 'V'),
- (0xA7B0, 'M', 'ʞ'),
- (0xA7B1, 'M', 'ʇ'),
- (0xA7B2, 'M', 'ʝ'),
- (0xA7B3, 'M', 'ꭓ'),
- (0xA7B4, 'M', 'ꞵ'),
- (0xA7B5, 'V'),
- (0xA7B6, 'M', 'ꞷ'),
- (0xA7B7, 'V'),
- (0xA7B8, 'M', 'ꞹ'),
- (0xA7B9, 'V'),
- (0xA7BA, 'M', 'ꞻ'),
- (0xA7BB, 'V'),
- (0xA7BC, 'M', 'ꞽ'),
- (0xA7BD, 'V'),
- (0xA7BE, 'M', 'ꞿ'),
- (0xA7BF, 'V'),
- (0xA7C0, 'M', 'ꟁ'),
- (0xA7C1, 'V'),
- (0xA7C2, 'M', 'ꟃ'),
- (0xA7C3, 'V'),
- (0xA7C4, 'M', 'ꞔ'),
- (0xA7C5, 'M', 'ʂ'),
- (0xA7C6, 'M', 'ᶎ'),
- (0xA7C7, 'M', 'ꟈ'),
- (0xA7C8, 'V'),
- (0xA7C9, 'M', 'ꟊ'),
- (0xA7CA, 'V'),
- (0xA7CB, 'X'),
- (0xA7D0, 'M', 'ꟑ'),
- (0xA7D1, 'V'),
- (0xA7D2, 'X'),
- (0xA7D3, 'V'),
- (0xA7D4, 'X'),
- (0xA7D5, 'V'),
- (0xA7D6, 'M', 'ꟗ'),
- (0xA7D7, 'V'),
- (0xA7D8, 'M', 'ꟙ'),
- (0xA7D9, 'V'),
- (0xA7DA, 'X'),
- (0xA7F2, 'M', 'c'),
- (0xA7F3, 'M', 'f'),
- (0xA7F4, 'M', 'q'),
- (0xA7F5, 'M', 'ꟶ'),
- (0xA7F6, 'V'),
- (0xA7F8, 'M', 'ħ'),
- (0xA7F9, 'M', 'œ'),
- (0xA7FA, 'V'),
- (0xA82D, 'X'),
- (0xA830, 'V'),
- (0xA83A, 'X'),
- (0xA840, 'V'),
- (0xA878, 'X'),
- (0xA880, 'V'),
- (0xA8C6, 'X'),
- ]
-
-def _seg_38() -> List[Union[Tuple[int, str], Tuple[int, str, str]]]:
- return [
- (0xA8CE, 'V'),
- (0xA8DA, 'X'),
- (0xA8E0, 'V'),
- (0xA954, 'X'),
- (0xA95F, 'V'),
- (0xA97D, 'X'),
- (0xA980, 'V'),
- (0xA9CE, 'X'),
- (0xA9CF, 'V'),
- (0xA9DA, 'X'),
- (0xA9DE, 'V'),
- (0xA9FF, 'X'),
- (0xAA00, 'V'),
- (0xAA37, 'X'),
- (0xAA40, 'V'),
- (0xAA4E, 'X'),
- (0xAA50, 'V'),
- (0xAA5A, 'X'),
- (0xAA5C, 'V'),
- (0xAAC3, 'X'),
- (0xAADB, 'V'),
- (0xAAF7, 'X'),
- (0xAB01, 'V'),
- (0xAB07, 'X'),
- (0xAB09, 'V'),
- (0xAB0F, 'X'),
- (0xAB11, 'V'),
- (0xAB17, 'X'),
- (0xAB20, 'V'),
- (0xAB27, 'X'),
- (0xAB28, 'V'),
- (0xAB2F, 'X'),
- (0xAB30, 'V'),
- (0xAB5C, 'M', 'ꜧ'),
- (0xAB5D, 'M', 'ꬷ'),
- (0xAB5E, 'M', 'ɫ'),
- (0xAB5F, 'M', 'ꭒ'),
- (0xAB60, 'V'),
- (0xAB69, 'M', 'ʍ'),
- (0xAB6A, 'V'),
- (0xAB6C, 'X'),
- (0xAB70, 'M', 'Ꭰ'),
- (0xAB71, 'M', 'Ꭱ'),
- (0xAB72, 'M', 'Ꭲ'),
- (0xAB73, 'M', 'Ꭳ'),
- (0xAB74, 'M', 'Ꭴ'),
- (0xAB75, 'M', 'Ꭵ'),
- (0xAB76, 'M', 'Ꭶ'),
- (0xAB77, 'M', 'Ꭷ'),
- (0xAB78, 'M', 'Ꭸ'),
- (0xAB79, 'M', 'Ꭹ'),
- (0xAB7A, 'M', 'Ꭺ'),
- (0xAB7B, 'M', 'Ꭻ'),
- (0xAB7C, 'M', 'Ꭼ'),
- (0xAB7D, 'M', 'Ꭽ'),
- (0xAB7E, 'M', 'Ꭾ'),
- (0xAB7F, 'M', 'Ꭿ'),
- (0xAB80, 'M', 'Ꮀ'),
- (0xAB81, 'M', 'Ꮁ'),
- (0xAB82, 'M', 'Ꮂ'),
- (0xAB83, 'M', 'Ꮃ'),
- (0xAB84, 'M', 'Ꮄ'),
- (0xAB85, 'M', 'Ꮅ'),
- (0xAB86, 'M', 'Ꮆ'),
- (0xAB87, 'M', 'Ꮇ'),
- (0xAB88, 'M', 'Ꮈ'),
- (0xAB89, 'M', 'Ꮉ'),
- (0xAB8A, 'M', 'Ꮊ'),
- (0xAB8B, 'M', 'Ꮋ'),
- (0xAB8C, 'M', 'Ꮌ'),
- (0xAB8D, 'M', 'Ꮍ'),
- (0xAB8E, 'M', 'Ꮎ'),
- (0xAB8F, 'M', 'Ꮏ'),
- (0xAB90, 'M', 'Ꮐ'),
- (0xAB91, 'M', 'Ꮑ'),
- (0xAB92, 'M', 'Ꮒ'),
- (0xAB93, 'M', 'Ꮓ'),
- (0xAB94, 'M', 'Ꮔ'),
- (0xAB95, 'M', 'Ꮕ'),
- (0xAB96, 'M', 'Ꮖ'),
- (0xAB97, 'M', 'Ꮗ'),
- (0xAB98, 'M', 'Ꮘ'),
- (0xAB99, 'M', 'Ꮙ'),
- (0xAB9A, 'M', 'Ꮚ'),
- (0xAB9B, 'M', 'Ꮛ'),
- (0xAB9C, 'M', 'Ꮜ'),
- (0xAB9D, 'M', 'Ꮝ'),
- (0xAB9E, 'M', 'Ꮞ'),
- (0xAB9F, 'M', 'Ꮟ'),
- (0xABA0, 'M', 'Ꮠ'),
- (0xABA1, 'M', 'Ꮡ'),
- (0xABA2, 'M', 'Ꮢ'),
- (0xABA3, 'M', 'Ꮣ'),
- (0xABA4, 'M', 'Ꮤ'),
- (0xABA5, 'M', 'Ꮥ'),
- (0xABA6, 'M', 'Ꮦ'),
- (0xABA7, 'M', 'Ꮧ'),
- (0xABA8, 'M', 'Ꮨ'),
- (0xABA9, 'M', 'Ꮩ'),
- (0xABAA, 'M', 'Ꮪ'),
- ]
-
-def _seg_39() -> List[Union[Tuple[int, str], Tuple[int, str, str]]]:
- return [
- (0xABAB, 'M', 'Ꮫ'),
- (0xABAC, 'M', 'Ꮬ'),
- (0xABAD, 'M', 'Ꮭ'),
- (0xABAE, 'M', 'Ꮮ'),
- (0xABAF, 'M', 'Ꮯ'),
- (0xABB0, 'M', 'Ꮰ'),
- (0xABB1, 'M', 'Ꮱ'),
- (0xABB2, 'M', 'Ꮲ'),
- (0xABB3, 'M', 'Ꮳ'),
- (0xABB4, 'M', 'Ꮴ'),
- (0xABB5, 'M', 'Ꮵ'),
- (0xABB6, 'M', 'Ꮶ'),
- (0xABB7, 'M', 'Ꮷ'),
- (0xABB8, 'M', 'Ꮸ'),
- (0xABB9, 'M', 'Ꮹ'),
- (0xABBA, 'M', 'Ꮺ'),
- (0xABBB, 'M', 'Ꮻ'),
- (0xABBC, 'M', 'Ꮼ'),
- (0xABBD, 'M', 'Ꮽ'),
- (0xABBE, 'M', 'Ꮾ'),
- (0xABBF, 'M', 'Ꮿ'),
- (0xABC0, 'V'),
- (0xABEE, 'X'),
- (0xABF0, 'V'),
- (0xABFA, 'X'),
- (0xAC00, 'V'),
- (0xD7A4, 'X'),
- (0xD7B0, 'V'),
- (0xD7C7, 'X'),
- (0xD7CB, 'V'),
- (0xD7FC, 'X'),
- (0xF900, 'M', '豈'),
- (0xF901, 'M', '更'),
- (0xF902, 'M', '車'),
- (0xF903, 'M', '賈'),
- (0xF904, 'M', '滑'),
- (0xF905, 'M', '串'),
- (0xF906, 'M', '句'),
- (0xF907, 'M', '龜'),
- (0xF909, 'M', '契'),
- (0xF90A, 'M', '金'),
- (0xF90B, 'M', '喇'),
- (0xF90C, 'M', '奈'),
- (0xF90D, 'M', '懶'),
- (0xF90E, 'M', '癩'),
- (0xF90F, 'M', '羅'),
- (0xF910, 'M', '蘿'),
- (0xF911, 'M', '螺'),
- (0xF912, 'M', '裸'),
- (0xF913, 'M', '邏'),
- (0xF914, 'M', '樂'),
- (0xF915, 'M', '洛'),
- (0xF916, 'M', '烙'),
- (0xF917, 'M', '珞'),
- (0xF918, 'M', '落'),
- (0xF919, 'M', '酪'),
- (0xF91A, 'M', '駱'),
- (0xF91B, 'M', '亂'),
- (0xF91C, 'M', '卵'),
- (0xF91D, 'M', '欄'),
- (0xF91E, 'M', '爛'),
- (0xF91F, 'M', '蘭'),
- (0xF920, 'M', '鸞'),
- (0xF921, 'M', '嵐'),
- (0xF922, 'M', '濫'),
- (0xF923, 'M', '藍'),
- (0xF924, 'M', '襤'),
- (0xF925, 'M', '拉'),
- (0xF926, 'M', '臘'),
- (0xF927, 'M', '蠟'),
- (0xF928, 'M', '廊'),
- (0xF929, 'M', '朗'),
- (0xF92A, 'M', '浪'),
- (0xF92B, 'M', '狼'),
- (0xF92C, 'M', '郎'),
- (0xF92D, 'M', '來'),
- (0xF92E, 'M', '冷'),
- (0xF92F, 'M', '勞'),
- (0xF930, 'M', '擄'),
- (0xF931, 'M', '櫓'),
- (0xF932, 'M', '爐'),
- (0xF933, 'M', '盧'),
- (0xF934, 'M', '老'),
- (0xF935, 'M', '蘆'),
- (0xF936, 'M', '虜'),
- (0xF937, 'M', '路'),
- (0xF938, 'M', '露'),
- (0xF939, 'M', '魯'),
- (0xF93A, 'M', '鷺'),
- (0xF93B, 'M', '碌'),
- (0xF93C, 'M', '祿'),
- (0xF93D, 'M', '綠'),
- (0xF93E, 'M', '菉'),
- (0xF93F, 'M', '錄'),
- (0xF940, 'M', '鹿'),
- (0xF941, 'M', '論'),
- (0xF942, 'M', '壟'),
- (0xF943, 'M', '弄'),
- (0xF944, 'M', '籠'),
- (0xF945, 'M', '聾'),
- ]
-
-def _seg_40() -> List[Union[Tuple[int, str], Tuple[int, str, str]]]:
- return [
- (0xF946, 'M', '牢'),
- (0xF947, 'M', '磊'),
- (0xF948, 'M', '賂'),
- (0xF949, 'M', '雷'),
- (0xF94A, 'M', '壘'),
- (0xF94B, 'M', '屢'),
- (0xF94C, 'M', '樓'),
- (0xF94D, 'M', '淚'),
- (0xF94E, 'M', '漏'),
- (0xF94F, 'M', '累'),
- (0xF950, 'M', '縷'),
- (0xF951, 'M', '陋'),
- (0xF952, 'M', '勒'),
- (0xF953, 'M', '肋'),
- (0xF954, 'M', '凜'),
- (0xF955, 'M', '凌'),
- (0xF956, 'M', '稜'),
- (0xF957, 'M', '綾'),
- (0xF958, 'M', '菱'),
- (0xF959, 'M', '陵'),
- (0xF95A, 'M', '讀'),
- (0xF95B, 'M', '拏'),
- (0xF95C, 'M', '樂'),
- (0xF95D, 'M', '諾'),
- (0xF95E, 'M', '丹'),
- (0xF95F, 'M', '寧'),
- (0xF960, 'M', '怒'),
- (0xF961, 'M', '率'),
- (0xF962, 'M', '異'),
- (0xF963, 'M', '北'),
- (0xF964, 'M', '磻'),
- (0xF965, 'M', '便'),
- (0xF966, 'M', '復'),
- (0xF967, 'M', '不'),
- (0xF968, 'M', '泌'),
- (0xF969, 'M', '數'),
- (0xF96A, 'M', '索'),
- (0xF96B, 'M', '參'),
- (0xF96C, 'M', '塞'),
- (0xF96D, 'M', '省'),
- (0xF96E, 'M', '葉'),
- (0xF96F, 'M', '說'),
- (0xF970, 'M', '殺'),
- (0xF971, 'M', '辰'),
- (0xF972, 'M', '沈'),
- (0xF973, 'M', '拾'),
- (0xF974, 'M', '若'),
- (0xF975, 'M', '掠'),
- (0xF976, 'M', '略'),
- (0xF977, 'M', '亮'),
- (0xF978, 'M', '兩'),
- (0xF979, 'M', '凉'),
- (0xF97A, 'M', '梁'),
- (0xF97B, 'M', '糧'),
- (0xF97C, 'M', '良'),
- (0xF97D, 'M', '諒'),
- (0xF97E, 'M', '量'),
- (0xF97F, 'M', '勵'),
- (0xF980, 'M', '呂'),
- (0xF981, 'M', '女'),
- (0xF982, 'M', '廬'),
- (0xF983, 'M', '旅'),
- (0xF984, 'M', '濾'),
- (0xF985, 'M', '礪'),
- (0xF986, 'M', '閭'),
- (0xF987, 'M', '驪'),
- (0xF988, 'M', '麗'),
- (0xF989, 'M', '黎'),
- (0xF98A, 'M', '力'),
- (0xF98B, 'M', '曆'),
- (0xF98C, 'M', '歷'),
- (0xF98D, 'M', '轢'),
- (0xF98E, 'M', '年'),
- (0xF98F, 'M', '憐'),
- (0xF990, 'M', '戀'),
- (0xF991, 'M', '撚'),
- (0xF992, 'M', '漣'),
- (0xF993, 'M', '煉'),
- (0xF994, 'M', '璉'),
- (0xF995, 'M', '秊'),
- (0xF996, 'M', '練'),
- (0xF997, 'M', '聯'),
- (0xF998, 'M', '輦'),
- (0xF999, 'M', '蓮'),
- (0xF99A, 'M', '連'),
- (0xF99B, 'M', '鍊'),
- (0xF99C, 'M', '列'),
- (0xF99D, 'M', '劣'),
- (0xF99E, 'M', '咽'),
- (0xF99F, 'M', '烈'),
- (0xF9A0, 'M', '裂'),
- (0xF9A1, 'M', '說'),
- (0xF9A2, 'M', '廉'),
- (0xF9A3, 'M', '念'),
- (0xF9A4, 'M', '捻'),
- (0xF9A5, 'M', '殮'),
- (0xF9A6, 'M', '簾'),
- (0xF9A7, 'M', '獵'),
- (0xF9A8, 'M', '令'),
- (0xF9A9, 'M', '囹'),
- ]
-
-def _seg_41() -> List[Union[Tuple[int, str], Tuple[int, str, str]]]:
- return [
- (0xF9AA, 'M', '寧'),
- (0xF9AB, 'M', '嶺'),
- (0xF9AC, 'M', '怜'),
- (0xF9AD, 'M', '玲'),
- (0xF9AE, 'M', '瑩'),
- (0xF9AF, 'M', '羚'),
- (0xF9B0, 'M', '聆'),
- (0xF9B1, 'M', '鈴'),
- (0xF9B2, 'M', '零'),
- (0xF9B3, 'M', '靈'),
- (0xF9B4, 'M', '領'),
- (0xF9B5, 'M', '例'),
- (0xF9B6, 'M', '禮'),
- (0xF9B7, 'M', '醴'),
- (0xF9B8, 'M', '隸'),
- (0xF9B9, 'M', '惡'),
- (0xF9BA, 'M', '了'),
- (0xF9BB, 'M', '僚'),
- (0xF9BC, 'M', '寮'),
- (0xF9BD, 'M', '尿'),
- (0xF9BE, 'M', '料'),
- (0xF9BF, 'M', '樂'),
- (0xF9C0, 'M', '燎'),
- (0xF9C1, 'M', '療'),
- (0xF9C2, 'M', '蓼'),
- (0xF9C3, 'M', '遼'),
- (0xF9C4, 'M', '龍'),
- (0xF9C5, 'M', '暈'),
- (0xF9C6, 'M', '阮'),
- (0xF9C7, 'M', '劉'),
- (0xF9C8, 'M', '杻'),
- (0xF9C9, 'M', '柳'),
- (0xF9CA, 'M', '流'),
- (0xF9CB, 'M', '溜'),
- (0xF9CC, 'M', '琉'),
- (0xF9CD, 'M', '留'),
- (0xF9CE, 'M', '硫'),
- (0xF9CF, 'M', '紐'),
- (0xF9D0, 'M', '類'),
- (0xF9D1, 'M', '六'),
- (0xF9D2, 'M', '戮'),
- (0xF9D3, 'M', '陸'),
- (0xF9D4, 'M', '倫'),
- (0xF9D5, 'M', '崙'),
- (0xF9D6, 'M', '淪'),
- (0xF9D7, 'M', '輪'),
- (0xF9D8, 'M', '律'),
- (0xF9D9, 'M', '慄'),
- (0xF9DA, 'M', '栗'),
- (0xF9DB, 'M', '率'),
- (0xF9DC, 'M', '隆'),
- (0xF9DD, 'M', '利'),
- (0xF9DE, 'M', '吏'),
- (0xF9DF, 'M', '履'),
- (0xF9E0, 'M', '易'),
- (0xF9E1, 'M', '李'),
- (0xF9E2, 'M', '梨'),
- (0xF9E3, 'M', '泥'),
- (0xF9E4, 'M', '理'),
- (0xF9E5, 'M', '痢'),
- (0xF9E6, 'M', '罹'),
- (0xF9E7, 'M', '裏'),
- (0xF9E8, 'M', '裡'),
- (0xF9E9, 'M', '里'),
- (0xF9EA, 'M', '離'),
- (0xF9EB, 'M', '匿'),
- (0xF9EC, 'M', '溺'),
- (0xF9ED, 'M', '吝'),
- (0xF9EE, 'M', '燐'),
- (0xF9EF, 'M', '璘'),
- (0xF9F0, 'M', '藺'),
- (0xF9F1, 'M', '隣'),
- (0xF9F2, 'M', '鱗'),
- (0xF9F3, 'M', '麟'),
- (0xF9F4, 'M', '林'),
- (0xF9F5, 'M', '淋'),
- (0xF9F6, 'M', '臨'),
- (0xF9F7, 'M', '立'),
- (0xF9F8, 'M', '笠'),
- (0xF9F9, 'M', '粒'),
- (0xF9FA, 'M', '狀'),
- (0xF9FB, 'M', '炙'),
- (0xF9FC, 'M', '識'),
- (0xF9FD, 'M', '什'),
- (0xF9FE, 'M', '茶'),
- (0xF9FF, 'M', '刺'),
- (0xFA00, 'M', '切'),
- (0xFA01, 'M', '度'),
- (0xFA02, 'M', '拓'),
- (0xFA03, 'M', '糖'),
- (0xFA04, 'M', '宅'),
- (0xFA05, 'M', '洞'),
- (0xFA06, 'M', '暴'),
- (0xFA07, 'M', '輻'),
- (0xFA08, 'M', '行'),
- (0xFA09, 'M', '降'),
- (0xFA0A, 'M', '見'),
- (0xFA0B, 'M', '廓'),
- (0xFA0C, 'M', '兀'),
- (0xFA0D, 'M', '嗀'),
- ]
-
-def _seg_42() -> List[Union[Tuple[int, str], Tuple[int, str, str]]]:
- return [
- (0xFA0E, 'V'),
- (0xFA10, 'M', '塚'),
- (0xFA11, 'V'),
- (0xFA12, 'M', '晴'),
- (0xFA13, 'V'),
- (0xFA15, 'M', '凞'),
- (0xFA16, 'M', '猪'),
- (0xFA17, 'M', '益'),
- (0xFA18, 'M', '礼'),
- (0xFA19, 'M', '神'),
- (0xFA1A, 'M', '祥'),
- (0xFA1B, 'M', '福'),
- (0xFA1C, 'M', '靖'),
- (0xFA1D, 'M', '精'),
- (0xFA1E, 'M', '羽'),
- (0xFA1F, 'V'),
- (0xFA20, 'M', '蘒'),
- (0xFA21, 'V'),
- (0xFA22, 'M', '諸'),
- (0xFA23, 'V'),
- (0xFA25, 'M', '逸'),
- (0xFA26, 'M', '都'),
- (0xFA27, 'V'),
- (0xFA2A, 'M', '飯'),
- (0xFA2B, 'M', '飼'),
- (0xFA2C, 'M', '館'),
- (0xFA2D, 'M', '鶴'),
- (0xFA2E, 'M', '郞'),
- (0xFA2F, 'M', '隷'),
- (0xFA30, 'M', '侮'),
- (0xFA31, 'M', '僧'),
- (0xFA32, 'M', '免'),
- (0xFA33, 'M', '勉'),
- (0xFA34, 'M', '勤'),
- (0xFA35, 'M', '卑'),
- (0xFA36, 'M', '喝'),
- (0xFA37, 'M', '嘆'),
- (0xFA38, 'M', '器'),
- (0xFA39, 'M', '塀'),
- (0xFA3A, 'M', '墨'),
- (0xFA3B, 'M', '層'),
- (0xFA3C, 'M', '屮'),
- (0xFA3D, 'M', '悔'),
- (0xFA3E, 'M', '慨'),
- (0xFA3F, 'M', '憎'),
- (0xFA40, 'M', '懲'),
- (0xFA41, 'M', '敏'),
- (0xFA42, 'M', '既'),
- (0xFA43, 'M', '暑'),
- (0xFA44, 'M', '梅'),
- (0xFA45, 'M', '海'),
- (0xFA46, 'M', '渚'),
- (0xFA47, 'M', '漢'),
- (0xFA48, 'M', '煮'),
- (0xFA49, 'M', '爫'),
- (0xFA4A, 'M', '琢'),
- (0xFA4B, 'M', '碑'),
- (0xFA4C, 'M', '社'),
- (0xFA4D, 'M', '祉'),
- (0xFA4E, 'M', '祈'),
- (0xFA4F, 'M', '祐'),
- (0xFA50, 'M', '祖'),
- (0xFA51, 'M', '祝'),
- (0xFA52, 'M', '禍'),
- (0xFA53, 'M', '禎'),
- (0xFA54, 'M', '穀'),
- (0xFA55, 'M', '突'),
- (0xFA56, 'M', '節'),
- (0xFA57, 'M', '練'),
- (0xFA58, 'M', '縉'),
- (0xFA59, 'M', '繁'),
- (0xFA5A, 'M', '署'),
- (0xFA5B, 'M', '者'),
- (0xFA5C, 'M', '臭'),
- (0xFA5D, 'M', '艹'),
- (0xFA5F, 'M', '著'),
- (0xFA60, 'M', '褐'),
- (0xFA61, 'M', '視'),
- (0xFA62, 'M', '謁'),
- (0xFA63, 'M', '謹'),
- (0xFA64, 'M', '賓'),
- (0xFA65, 'M', '贈'),
- (0xFA66, 'M', '辶'),
- (0xFA67, 'M', '逸'),
- (0xFA68, 'M', '難'),
- (0xFA69, 'M', '響'),
- (0xFA6A, 'M', '頻'),
- (0xFA6B, 'M', '恵'),
- (0xFA6C, 'M', '𤋮'),
- (0xFA6D, 'M', '舘'),
- (0xFA6E, 'X'),
- (0xFA70, 'M', '並'),
- (0xFA71, 'M', '况'),
- (0xFA72, 'M', '全'),
- (0xFA73, 'M', '侀'),
- (0xFA74, 'M', '充'),
- (0xFA75, 'M', '冀'),
- (0xFA76, 'M', '勇'),
- (0xFA77, 'M', '勺'),
- (0xFA78, 'M', '喝'),
- ]
-
-def _seg_43() -> List[Union[Tuple[int, str], Tuple[int, str, str]]]:
- return [
- (0xFA79, 'M', '啕'),
- (0xFA7A, 'M', '喙'),
- (0xFA7B, 'M', '嗢'),
- (0xFA7C, 'M', '塚'),
- (0xFA7D, 'M', '墳'),
- (0xFA7E, 'M', '奄'),
- (0xFA7F, 'M', '奔'),
- (0xFA80, 'M', '婢'),
- (0xFA81, 'M', '嬨'),
- (0xFA82, 'M', '廒'),
- (0xFA83, 'M', '廙'),
- (0xFA84, 'M', '彩'),
- (0xFA85, 'M', '徭'),
- (0xFA86, 'M', '惘'),
- (0xFA87, 'M', '慎'),
- (0xFA88, 'M', '愈'),
- (0xFA89, 'M', '憎'),
- (0xFA8A, 'M', '慠'),
- (0xFA8B, 'M', '懲'),
- (0xFA8C, 'M', '戴'),
- (0xFA8D, 'M', '揄'),
- (0xFA8E, 'M', '搜'),
- (0xFA8F, 'M', '摒'),
- (0xFA90, 'M', '敖'),
- (0xFA91, 'M', '晴'),
- (0xFA92, 'M', '朗'),
- (0xFA93, 'M', '望'),
- (0xFA94, 'M', '杖'),
- (0xFA95, 'M', '歹'),
- (0xFA96, 'M', '殺'),
- (0xFA97, 'M', '流'),
- (0xFA98, 'M', '滛'),
- (0xFA99, 'M', '滋'),
- (0xFA9A, 'M', '漢'),
- (0xFA9B, 'M', '瀞'),
- (0xFA9C, 'M', '煮'),
- (0xFA9D, 'M', '瞧'),
- (0xFA9E, 'M', '爵'),
- (0xFA9F, 'M', '犯'),
- (0xFAA0, 'M', '猪'),
- (0xFAA1, 'M', '瑱'),
- (0xFAA2, 'M', '甆'),
- (0xFAA3, 'M', '画'),
- (0xFAA4, 'M', '瘝'),
- (0xFAA5, 'M', '瘟'),
- (0xFAA6, 'M', '益'),
- (0xFAA7, 'M', '盛'),
- (0xFAA8, 'M', '直'),
- (0xFAA9, 'M', '睊'),
- (0xFAAA, 'M', '着'),
- (0xFAAB, 'M', '磌'),
- (0xFAAC, 'M', '窱'),
- (0xFAAD, 'M', '節'),
- (0xFAAE, 'M', '类'),
- (0xFAAF, 'M', '絛'),
- (0xFAB0, 'M', '練'),
- (0xFAB1, 'M', '缾'),
- (0xFAB2, 'M', '者'),
- (0xFAB3, 'M', '荒'),
- (0xFAB4, 'M', '華'),
- (0xFAB5, 'M', '蝹'),
- (0xFAB6, 'M', '襁'),
- (0xFAB7, 'M', '覆'),
- (0xFAB8, 'M', '視'),
- (0xFAB9, 'M', '調'),
- (0xFABA, 'M', '諸'),
- (0xFABB, 'M', '請'),
- (0xFABC, 'M', '謁'),
- (0xFABD, 'M', '諾'),
- (0xFABE, 'M', '諭'),
- (0xFABF, 'M', '謹'),
- (0xFAC0, 'M', '變'),
- (0xFAC1, 'M', '贈'),
- (0xFAC2, 'M', '輸'),
- (0xFAC3, 'M', '遲'),
- (0xFAC4, 'M', '醙'),
- (0xFAC5, 'M', '鉶'),
- (0xFAC6, 'M', '陼'),
- (0xFAC7, 'M', '難'),
- (0xFAC8, 'M', '靖'),
- (0xFAC9, 'M', '韛'),
- (0xFACA, 'M', '響'),
- (0xFACB, 'M', '頋'),
- (0xFACC, 'M', '頻'),
- (0xFACD, 'M', '鬒'),
- (0xFACE, 'M', '龜'),
- (0xFACF, 'M', '𢡊'),
- (0xFAD0, 'M', '𢡄'),
- (0xFAD1, 'M', '𣏕'),
- (0xFAD2, 'M', '㮝'),
- (0xFAD3, 'M', '䀘'),
- (0xFAD4, 'M', '䀹'),
- (0xFAD5, 'M', '𥉉'),
- (0xFAD6, 'M', '𥳐'),
- (0xFAD7, 'M', '𧻓'),
- (0xFAD8, 'M', '齃'),
- (0xFAD9, 'M', '龎'),
- (0xFADA, 'X'),
- (0xFB00, 'M', 'ff'),
- (0xFB01, 'M', 'fi'),
- ]
-
-def _seg_44() -> List[Union[Tuple[int, str], Tuple[int, str, str]]]:
- return [
- (0xFB02, 'M', 'fl'),
- (0xFB03, 'M', 'ffi'),
- (0xFB04, 'M', 'ffl'),
- (0xFB05, 'M', 'st'),
- (0xFB07, 'X'),
- (0xFB13, 'M', 'մն'),
- (0xFB14, 'M', 'մե'),
- (0xFB15, 'M', 'մի'),
- (0xFB16, 'M', 'վն'),
- (0xFB17, 'M', 'մխ'),
- (0xFB18, 'X'),
- (0xFB1D, 'M', 'יִ'),
- (0xFB1E, 'V'),
- (0xFB1F, 'M', 'ײַ'),
- (0xFB20, 'M', 'ע'),
- (0xFB21, 'M', 'א'),
- (0xFB22, 'M', 'ד'),
- (0xFB23, 'M', 'ה'),
- (0xFB24, 'M', 'כ'),
- (0xFB25, 'M', 'ל'),
- (0xFB26, 'M', 'ם'),
- (0xFB27, 'M', 'ר'),
- (0xFB28, 'M', 'ת'),
- (0xFB29, '3', '+'),
- (0xFB2A, 'M', 'שׁ'),
- (0xFB2B, 'M', 'שׂ'),
- (0xFB2C, 'M', 'שּׁ'),
- (0xFB2D, 'M', 'שּׂ'),
- (0xFB2E, 'M', 'אַ'),
- (0xFB2F, 'M', 'אָ'),
- (0xFB30, 'M', 'אּ'),
- (0xFB31, 'M', 'בּ'),
- (0xFB32, 'M', 'גּ'),
- (0xFB33, 'M', 'דּ'),
- (0xFB34, 'M', 'הּ'),
- (0xFB35, 'M', 'וּ'),
- (0xFB36, 'M', 'זּ'),
- (0xFB37, 'X'),
- (0xFB38, 'M', 'טּ'),
- (0xFB39, 'M', 'יּ'),
- (0xFB3A, 'M', 'ךּ'),
- (0xFB3B, 'M', 'כּ'),
- (0xFB3C, 'M', 'לּ'),
- (0xFB3D, 'X'),
- (0xFB3E, 'M', 'מּ'),
- (0xFB3F, 'X'),
- (0xFB40, 'M', 'נּ'),
- (0xFB41, 'M', 'סּ'),
- (0xFB42, 'X'),
- (0xFB43, 'M', 'ףּ'),
- (0xFB44, 'M', 'פּ'),
- (0xFB45, 'X'),
- (0xFB46, 'M', 'צּ'),
- (0xFB47, 'M', 'קּ'),
- (0xFB48, 'M', 'רּ'),
- (0xFB49, 'M', 'שּ'),
- (0xFB4A, 'M', 'תּ'),
- (0xFB4B, 'M', 'וֹ'),
- (0xFB4C, 'M', 'בֿ'),
- (0xFB4D, 'M', 'כֿ'),
- (0xFB4E, 'M', 'פֿ'),
- (0xFB4F, 'M', 'אל'),
- (0xFB50, 'M', 'ٱ'),
- (0xFB52, 'M', 'ٻ'),
- (0xFB56, 'M', 'پ'),
- (0xFB5A, 'M', 'ڀ'),
- (0xFB5E, 'M', 'ٺ'),
- (0xFB62, 'M', 'ٿ'),
- (0xFB66, 'M', 'ٹ'),
- (0xFB6A, 'M', 'ڤ'),
- (0xFB6E, 'M', 'ڦ'),
- (0xFB72, 'M', 'ڄ'),
- (0xFB76, 'M', 'ڃ'),
- (0xFB7A, 'M', 'چ'),
- (0xFB7E, 'M', 'ڇ'),
- (0xFB82, 'M', 'ڍ'),
- (0xFB84, 'M', 'ڌ'),
- (0xFB86, 'M', 'ڎ'),
- (0xFB88, 'M', 'ڈ'),
- (0xFB8A, 'M', 'ژ'),
- (0xFB8C, 'M', 'ڑ'),
- (0xFB8E, 'M', 'ک'),
- (0xFB92, 'M', 'گ'),
- (0xFB96, 'M', 'ڳ'),
- (0xFB9A, 'M', 'ڱ'),
- (0xFB9E, 'M', 'ں'),
- (0xFBA0, 'M', 'ڻ'),
- (0xFBA4, 'M', 'ۀ'),
- (0xFBA6, 'M', 'ہ'),
- (0xFBAA, 'M', 'ھ'),
- (0xFBAE, 'M', 'ے'),
- (0xFBB0, 'M', 'ۓ'),
- (0xFBB2, 'V'),
- (0xFBC3, 'X'),
- (0xFBD3, 'M', 'ڭ'),
- (0xFBD7, 'M', 'ۇ'),
- (0xFBD9, 'M', 'ۆ'),
- (0xFBDB, 'M', 'ۈ'),
- (0xFBDD, 'M', 'ۇٴ'),
- (0xFBDE, 'M', 'ۋ'),
- ]
-
-def _seg_45() -> List[Union[Tuple[int, str], Tuple[int, str, str]]]:
- return [
- (0xFBE0, 'M', 'ۅ'),
- (0xFBE2, 'M', 'ۉ'),
- (0xFBE4, 'M', 'ې'),
- (0xFBE8, 'M', 'ى'),
- (0xFBEA, 'M', 'ئا'),
- (0xFBEC, 'M', 'ئە'),
- (0xFBEE, 'M', 'ئو'),
- (0xFBF0, 'M', 'ئۇ'),
- (0xFBF2, 'M', 'ئۆ'),
- (0xFBF4, 'M', 'ئۈ'),
- (0xFBF6, 'M', 'ئې'),
- (0xFBF9, 'M', 'ئى'),
- (0xFBFC, 'M', 'ی'),
- (0xFC00, 'M', 'ئج'),
- (0xFC01, 'M', 'ئح'),
- (0xFC02, 'M', 'ئم'),
- (0xFC03, 'M', 'ئى'),
- (0xFC04, 'M', 'ئي'),
- (0xFC05, 'M', 'بج'),
- (0xFC06, 'M', 'بح'),
- (0xFC07, 'M', 'بخ'),
- (0xFC08, 'M', 'بم'),
- (0xFC09, 'M', 'بى'),
- (0xFC0A, 'M', 'بي'),
- (0xFC0B, 'M', 'تج'),
- (0xFC0C, 'M', 'تح'),
- (0xFC0D, 'M', 'تخ'),
- (0xFC0E, 'M', 'تم'),
- (0xFC0F, 'M', 'تى'),
- (0xFC10, 'M', 'تي'),
- (0xFC11, 'M', 'ثج'),
- (0xFC12, 'M', 'ثم'),
- (0xFC13, 'M', 'ثى'),
- (0xFC14, 'M', 'ثي'),
- (0xFC15, 'M', 'جح'),
- (0xFC16, 'M', 'جم'),
- (0xFC17, 'M', 'حج'),
- (0xFC18, 'M', 'حم'),
- (0xFC19, 'M', 'خج'),
- (0xFC1A, 'M', 'خح'),
- (0xFC1B, 'M', 'خم'),
- (0xFC1C, 'M', 'سج'),
- (0xFC1D, 'M', 'سح'),
- (0xFC1E, 'M', 'سخ'),
- (0xFC1F, 'M', 'سم'),
- (0xFC20, 'M', 'صح'),
- (0xFC21, 'M', 'صم'),
- (0xFC22, 'M', 'ضج'),
- (0xFC23, 'M', 'ضح'),
- (0xFC24, 'M', 'ضخ'),
- (0xFC25, 'M', 'ضم'),
- (0xFC26, 'M', 'طح'),
- (0xFC27, 'M', 'طم'),
- (0xFC28, 'M', 'ظم'),
- (0xFC29, 'M', 'عج'),
- (0xFC2A, 'M', 'عم'),
- (0xFC2B, 'M', 'غج'),
- (0xFC2C, 'M', 'غم'),
- (0xFC2D, 'M', 'فج'),
- (0xFC2E, 'M', 'فح'),
- (0xFC2F, 'M', 'فخ'),
- (0xFC30, 'M', 'فم'),
- (0xFC31, 'M', 'فى'),
- (0xFC32, 'M', 'في'),
- (0xFC33, 'M', 'قح'),
- (0xFC34, 'M', 'قم'),
- (0xFC35, 'M', 'قى'),
- (0xFC36, 'M', 'قي'),
- (0xFC37, 'M', 'كا'),
- (0xFC38, 'M', 'كج'),
- (0xFC39, 'M', 'كح'),
- (0xFC3A, 'M', 'كخ'),
- (0xFC3B, 'M', 'كل'),
- (0xFC3C, 'M', 'كم'),
- (0xFC3D, 'M', 'كى'),
- (0xFC3E, 'M', 'كي'),
- (0xFC3F, 'M', 'لج'),
- (0xFC40, 'M', 'لح'),
- (0xFC41, 'M', 'لخ'),
- (0xFC42, 'M', 'لم'),
- (0xFC43, 'M', 'لى'),
- (0xFC44, 'M', 'لي'),
- (0xFC45, 'M', 'مج'),
- (0xFC46, 'M', 'مح'),
- (0xFC47, 'M', 'مخ'),
- (0xFC48, 'M', 'مم'),
- (0xFC49, 'M', 'مى'),
- (0xFC4A, 'M', 'مي'),
- (0xFC4B, 'M', 'نج'),
- (0xFC4C, 'M', 'نح'),
- (0xFC4D, 'M', 'نخ'),
- (0xFC4E, 'M', 'نم'),
- (0xFC4F, 'M', 'نى'),
- (0xFC50, 'M', 'ني'),
- (0xFC51, 'M', 'هج'),
- (0xFC52, 'M', 'هم'),
- (0xFC53, 'M', 'هى'),
- (0xFC54, 'M', 'هي'),
- (0xFC55, 'M', 'يج'),
- (0xFC56, 'M', 'يح'),
- ]
-
-def _seg_46() -> List[Union[Tuple[int, str], Tuple[int, str, str]]]:
- return [
- (0xFC57, 'M', 'يخ'),
- (0xFC58, 'M', 'يم'),
- (0xFC59, 'M', 'يى'),
- (0xFC5A, 'M', 'يي'),
- (0xFC5B, 'M', 'ذٰ'),
- (0xFC5C, 'M', 'رٰ'),
- (0xFC5D, 'M', 'ىٰ'),
- (0xFC5E, '3', ' ٌّ'),
- (0xFC5F, '3', ' ٍّ'),
- (0xFC60, '3', ' َّ'),
- (0xFC61, '3', ' ُّ'),
- (0xFC62, '3', ' ِّ'),
- (0xFC63, '3', ' ّٰ'),
- (0xFC64, 'M', 'ئر'),
- (0xFC65, 'M', 'ئز'),
- (0xFC66, 'M', 'ئم'),
- (0xFC67, 'M', 'ئن'),
- (0xFC68, 'M', 'ئى'),
- (0xFC69, 'M', 'ئي'),
- (0xFC6A, 'M', 'بر'),
- (0xFC6B, 'M', 'بز'),
- (0xFC6C, 'M', 'بم'),
- (0xFC6D, 'M', 'بن'),
- (0xFC6E, 'M', 'بى'),
- (0xFC6F, 'M', 'بي'),
- (0xFC70, 'M', 'تر'),
- (0xFC71, 'M', 'تز'),
- (0xFC72, 'M', 'تم'),
- (0xFC73, 'M', 'تن'),
- (0xFC74, 'M', 'تى'),
- (0xFC75, 'M', 'تي'),
- (0xFC76, 'M', 'ثر'),
- (0xFC77, 'M', 'ثز'),
- (0xFC78, 'M', 'ثم'),
- (0xFC79, 'M', 'ثن'),
- (0xFC7A, 'M', 'ثى'),
- (0xFC7B, 'M', 'ثي'),
- (0xFC7C, 'M', 'فى'),
- (0xFC7D, 'M', 'في'),
- (0xFC7E, 'M', 'قى'),
- (0xFC7F, 'M', 'قي'),
- (0xFC80, 'M', 'كا'),
- (0xFC81, 'M', 'كل'),
- (0xFC82, 'M', 'كم'),
- (0xFC83, 'M', 'كى'),
- (0xFC84, 'M', 'كي'),
- (0xFC85, 'M', 'لم'),
- (0xFC86, 'M', 'لى'),
- (0xFC87, 'M', 'لي'),
- (0xFC88, 'M', 'ما'),
- (0xFC89, 'M', 'مم'),
- (0xFC8A, 'M', 'نر'),
- (0xFC8B, 'M', 'نز'),
- (0xFC8C, 'M', 'نم'),
- (0xFC8D, 'M', 'نن'),
- (0xFC8E, 'M', 'نى'),
- (0xFC8F, 'M', 'ني'),
- (0xFC90, 'M', 'ىٰ'),
- (0xFC91, 'M', 'ير'),
- (0xFC92, 'M', 'يز'),
- (0xFC93, 'M', 'يم'),
- (0xFC94, 'M', 'ين'),
- (0xFC95, 'M', 'يى'),
- (0xFC96, 'M', 'يي'),
- (0xFC97, 'M', 'ئج'),
- (0xFC98, 'M', 'ئح'),
- (0xFC99, 'M', 'ئخ'),
- (0xFC9A, 'M', 'ئم'),
- (0xFC9B, 'M', 'ئه'),
- (0xFC9C, 'M', 'بج'),
- (0xFC9D, 'M', 'بح'),
- (0xFC9E, 'M', 'بخ'),
- (0xFC9F, 'M', 'بم'),
- (0xFCA0, 'M', 'به'),
- (0xFCA1, 'M', 'تج'),
- (0xFCA2, 'M', 'تح'),
- (0xFCA3, 'M', 'تخ'),
- (0xFCA4, 'M', 'تم'),
- (0xFCA5, 'M', 'ته'),
- (0xFCA6, 'M', 'ثم'),
- (0xFCA7, 'M', 'جح'),
- (0xFCA8, 'M', 'جم'),
- (0xFCA9, 'M', 'حج'),
- (0xFCAA, 'M', 'حم'),
- (0xFCAB, 'M', 'خج'),
- (0xFCAC, 'M', 'خم'),
- (0xFCAD, 'M', 'سج'),
- (0xFCAE, 'M', 'سح'),
- (0xFCAF, 'M', 'سخ'),
- (0xFCB0, 'M', 'سم'),
- (0xFCB1, 'M', 'صح'),
- (0xFCB2, 'M', 'صخ'),
- (0xFCB3, 'M', 'صم'),
- (0xFCB4, 'M', 'ضج'),
- (0xFCB5, 'M', 'ضح'),
- (0xFCB6, 'M', 'ضخ'),
- (0xFCB7, 'M', 'ضم'),
- (0xFCB8, 'M', 'طح'),
- (0xFCB9, 'M', 'ظم'),
- (0xFCBA, 'M', 'عج'),
- ]
-
-def _seg_47() -> List[Union[Tuple[int, str], Tuple[int, str, str]]]:
- return [
- (0xFCBB, 'M', 'عم'),
- (0xFCBC, 'M', 'غج'),
- (0xFCBD, 'M', 'غم'),
- (0xFCBE, 'M', 'فج'),
- (0xFCBF, 'M', 'فح'),
- (0xFCC0, 'M', 'فخ'),
- (0xFCC1, 'M', 'فم'),
- (0xFCC2, 'M', 'قح'),
- (0xFCC3, 'M', 'قم'),
- (0xFCC4, 'M', 'كج'),
- (0xFCC5, 'M', 'كح'),
- (0xFCC6, 'M', 'كخ'),
- (0xFCC7, 'M', 'كل'),
- (0xFCC8, 'M', 'كم'),
- (0xFCC9, 'M', 'لج'),
- (0xFCCA, 'M', 'لح'),
- (0xFCCB, 'M', 'لخ'),
- (0xFCCC, 'M', 'لم'),
- (0xFCCD, 'M', 'له'),
- (0xFCCE, 'M', 'مج'),
- (0xFCCF, 'M', 'مح'),
- (0xFCD0, 'M', 'مخ'),
- (0xFCD1, 'M', 'مم'),
- (0xFCD2, 'M', 'نج'),
- (0xFCD3, 'M', 'نح'),
- (0xFCD4, 'M', 'نخ'),
- (0xFCD5, 'M', 'نم'),
- (0xFCD6, 'M', 'نه'),
- (0xFCD7, 'M', 'هج'),
- (0xFCD8, 'M', 'هم'),
- (0xFCD9, 'M', 'هٰ'),
- (0xFCDA, 'M', 'يج'),
- (0xFCDB, 'M', 'يح'),
- (0xFCDC, 'M', 'يخ'),
- (0xFCDD, 'M', 'يم'),
- (0xFCDE, 'M', 'يه'),
- (0xFCDF, 'M', 'ئم'),
- (0xFCE0, 'M', 'ئه'),
- (0xFCE1, 'M', 'بم'),
- (0xFCE2, 'M', 'به'),
- (0xFCE3, 'M', 'تم'),
- (0xFCE4, 'M', 'ته'),
- (0xFCE5, 'M', 'ثم'),
- (0xFCE6, 'M', 'ثه'),
- (0xFCE7, 'M', 'سم'),
- (0xFCE8, 'M', 'سه'),
- (0xFCE9, 'M', 'شم'),
- (0xFCEA, 'M', 'شه'),
- (0xFCEB, 'M', 'كل'),
- (0xFCEC, 'M', 'كم'),
- (0xFCED, 'M', 'لم'),
- (0xFCEE, 'M', 'نم'),
- (0xFCEF, 'M', 'نه'),
- (0xFCF0, 'M', 'يم'),
- (0xFCF1, 'M', 'يه'),
- (0xFCF2, 'M', 'ـَّ'),
- (0xFCF3, 'M', 'ـُّ'),
- (0xFCF4, 'M', 'ـِّ'),
- (0xFCF5, 'M', 'طى'),
- (0xFCF6, 'M', 'طي'),
- (0xFCF7, 'M', 'عى'),
- (0xFCF8, 'M', 'عي'),
- (0xFCF9, 'M', 'غى'),
- (0xFCFA, 'M', 'غي'),
- (0xFCFB, 'M', 'سى'),
- (0xFCFC, 'M', 'سي'),
- (0xFCFD, 'M', 'شى'),
- (0xFCFE, 'M', 'شي'),
- (0xFCFF, 'M', 'حى'),
- (0xFD00, 'M', 'حي'),
- (0xFD01, 'M', 'جى'),
- (0xFD02, 'M', 'جي'),
- (0xFD03, 'M', 'خى'),
- (0xFD04, 'M', 'خي'),
- (0xFD05, 'M', 'صى'),
- (0xFD06, 'M', 'صي'),
- (0xFD07, 'M', 'ضى'),
- (0xFD08, 'M', 'ضي'),
- (0xFD09, 'M', 'شج'),
- (0xFD0A, 'M', 'شح'),
- (0xFD0B, 'M', 'شخ'),
- (0xFD0C, 'M', 'شم'),
- (0xFD0D, 'M', 'شر'),
- (0xFD0E, 'M', 'سر'),
- (0xFD0F, 'M', 'صر'),
- (0xFD10, 'M', 'ضر'),
- (0xFD11, 'M', 'طى'),
- (0xFD12, 'M', 'طي'),
- (0xFD13, 'M', 'عى'),
- (0xFD14, 'M', 'عي'),
- (0xFD15, 'M', 'غى'),
- (0xFD16, 'M', 'غي'),
- (0xFD17, 'M', 'سى'),
- (0xFD18, 'M', 'سي'),
- (0xFD19, 'M', 'شى'),
- (0xFD1A, 'M', 'شي'),
- (0xFD1B, 'M', 'حى'),
- (0xFD1C, 'M', 'حي'),
- (0xFD1D, 'M', 'جى'),
- (0xFD1E, 'M', 'جي'),
- ]
-
-def _seg_48() -> List[Union[Tuple[int, str], Tuple[int, str, str]]]:
- return [
- (0xFD1F, 'M', 'خى'),
- (0xFD20, 'M', 'خي'),
- (0xFD21, 'M', 'صى'),
- (0xFD22, 'M', 'صي'),
- (0xFD23, 'M', 'ضى'),
- (0xFD24, 'M', 'ضي'),
- (0xFD25, 'M', 'شج'),
- (0xFD26, 'M', 'شح'),
- (0xFD27, 'M', 'شخ'),
- (0xFD28, 'M', 'شم'),
- (0xFD29, 'M', 'شر'),
- (0xFD2A, 'M', 'سر'),
- (0xFD2B, 'M', 'صر'),
- (0xFD2C, 'M', 'ضر'),
- (0xFD2D, 'M', 'شج'),
- (0xFD2E, 'M', 'شح'),
- (0xFD2F, 'M', 'شخ'),
- (0xFD30, 'M', 'شم'),
- (0xFD31, 'M', 'سه'),
- (0xFD32, 'M', 'شه'),
- (0xFD33, 'M', 'طم'),
- (0xFD34, 'M', 'سج'),
- (0xFD35, 'M', 'سح'),
- (0xFD36, 'M', 'سخ'),
- (0xFD37, 'M', 'شج'),
- (0xFD38, 'M', 'شح'),
- (0xFD39, 'M', 'شخ'),
- (0xFD3A, 'M', 'طم'),
- (0xFD3B, 'M', 'ظم'),
- (0xFD3C, 'M', 'اً'),
- (0xFD3E, 'V'),
- (0xFD50, 'M', 'تجم'),
- (0xFD51, 'M', 'تحج'),
- (0xFD53, 'M', 'تحم'),
- (0xFD54, 'M', 'تخم'),
- (0xFD55, 'M', 'تمج'),
- (0xFD56, 'M', 'تمح'),
- (0xFD57, 'M', 'تمخ'),
- (0xFD58, 'M', 'جمح'),
- (0xFD5A, 'M', 'حمي'),
- (0xFD5B, 'M', 'حمى'),
- (0xFD5C, 'M', 'سحج'),
- (0xFD5D, 'M', 'سجح'),
- (0xFD5E, 'M', 'سجى'),
- (0xFD5F, 'M', 'سمح'),
- (0xFD61, 'M', 'سمج'),
- (0xFD62, 'M', 'سمم'),
- (0xFD64, 'M', 'صحح'),
- (0xFD66, 'M', 'صمم'),
- (0xFD67, 'M', 'شحم'),
- (0xFD69, 'M', 'شجي'),
- (0xFD6A, 'M', 'شمخ'),
- (0xFD6C, 'M', 'شمم'),
- (0xFD6E, 'M', 'ضحى'),
- (0xFD6F, 'M', 'ضخم'),
- (0xFD71, 'M', 'طمح'),
- (0xFD73, 'M', 'طمم'),
- (0xFD74, 'M', 'طمي'),
- (0xFD75, 'M', 'عجم'),
- (0xFD76, 'M', 'عمم'),
- (0xFD78, 'M', 'عمى'),
- (0xFD79, 'M', 'غمم'),
- (0xFD7A, 'M', 'غمي'),
- (0xFD7B, 'M', 'غمى'),
- (0xFD7C, 'M', 'فخم'),
- (0xFD7E, 'M', 'قمح'),
- (0xFD7F, 'M', 'قمم'),
- (0xFD80, 'M', 'لحم'),
- (0xFD81, 'M', 'لحي'),
- (0xFD82, 'M', 'لحى'),
- (0xFD83, 'M', 'لجج'),
- (0xFD85, 'M', 'لخم'),
- (0xFD87, 'M', 'لمح'),
- (0xFD89, 'M', 'محج'),
- (0xFD8A, 'M', 'محم'),
- (0xFD8B, 'M', 'محي'),
- (0xFD8C, 'M', 'مجح'),
- (0xFD8D, 'M', 'مجم'),
- (0xFD8E, 'M', 'مخج'),
- (0xFD8F, 'M', 'مخم'),
- (0xFD90, 'X'),
- (0xFD92, 'M', 'مجخ'),
- (0xFD93, 'M', 'همج'),
- (0xFD94, 'M', 'همم'),
- (0xFD95, 'M', 'نحم'),
- (0xFD96, 'M', 'نحى'),
- (0xFD97, 'M', 'نجم'),
- (0xFD99, 'M', 'نجى'),
- (0xFD9A, 'M', 'نمي'),
- (0xFD9B, 'M', 'نمى'),
- (0xFD9C, 'M', 'يمم'),
- (0xFD9E, 'M', 'بخي'),
- (0xFD9F, 'M', 'تجي'),
- (0xFDA0, 'M', 'تجى'),
- (0xFDA1, 'M', 'تخي'),
- (0xFDA2, 'M', 'تخى'),
- (0xFDA3, 'M', 'تمي'),
- (0xFDA4, 'M', 'تمى'),
- (0xFDA5, 'M', 'جمي'),
- (0xFDA6, 'M', 'جحى'),
- ]
-
-def _seg_49() -> List[Union[Tuple[int, str], Tuple[int, str, str]]]:
- return [
- (0xFDA7, 'M', 'جمى'),
- (0xFDA8, 'M', 'سخى'),
- (0xFDA9, 'M', 'صحي'),
- (0xFDAA, 'M', 'شحي'),
- (0xFDAB, 'M', 'ضحي'),
- (0xFDAC, 'M', 'لجي'),
- (0xFDAD, 'M', 'لمي'),
- (0xFDAE, 'M', 'يحي'),
- (0xFDAF, 'M', 'يجي'),
- (0xFDB0, 'M', 'يمي'),
- (0xFDB1, 'M', 'ممي'),
- (0xFDB2, 'M', 'قمي'),
- (0xFDB3, 'M', 'نحي'),
- (0xFDB4, 'M', 'قمح'),
- (0xFDB5, 'M', 'لحم'),
- (0xFDB6, 'M', 'عمي'),
- (0xFDB7, 'M', 'كمي'),
- (0xFDB8, 'M', 'نجح'),
- (0xFDB9, 'M', 'مخي'),
- (0xFDBA, 'M', 'لجم'),
- (0xFDBB, 'M', 'كمم'),
- (0xFDBC, 'M', 'لجم'),
- (0xFDBD, 'M', 'نجح'),
- (0xFDBE, 'M', 'جحي'),
- (0xFDBF, 'M', 'حجي'),
- (0xFDC0, 'M', 'مجي'),
- (0xFDC1, 'M', 'فمي'),
- (0xFDC2, 'M', 'بحي'),
- (0xFDC3, 'M', 'كمم'),
- (0xFDC4, 'M', 'عجم'),
- (0xFDC5, 'M', 'صمم'),
- (0xFDC6, 'M', 'سخي'),
- (0xFDC7, 'M', 'نجي'),
- (0xFDC8, 'X'),
- (0xFDCF, 'V'),
- (0xFDD0, 'X'),
- (0xFDF0, 'M', 'صلے'),
- (0xFDF1, 'M', 'قلے'),
- (0xFDF2, 'M', 'الله'),
- (0xFDF3, 'M', 'اكبر'),
- (0xFDF4, 'M', 'محمد'),
- (0xFDF5, 'M', 'صلعم'),
- (0xFDF6, 'M', 'رسول'),
- (0xFDF7, 'M', 'عليه'),
- (0xFDF8, 'M', 'وسلم'),
- (0xFDF9, 'M', 'صلى'),
- (0xFDFA, '3', 'صلى الله عليه وسلم'),
- (0xFDFB, '3', 'جل جلاله'),
- (0xFDFC, 'M', 'ریال'),
- (0xFDFD, 'V'),
- (0xFE00, 'I'),
- (0xFE10, '3', ','),
- (0xFE11, 'M', '、'),
- (0xFE12, 'X'),
- (0xFE13, '3', ':'),
- (0xFE14, '3', ';'),
- (0xFE15, '3', '!'),
- (0xFE16, '3', '?'),
- (0xFE17, 'M', '〖'),
- (0xFE18, 'M', '〗'),
- (0xFE19, 'X'),
- (0xFE20, 'V'),
- (0xFE30, 'X'),
- (0xFE31, 'M', '—'),
- (0xFE32, 'M', '–'),
- (0xFE33, '3', '_'),
- (0xFE35, '3', '('),
- (0xFE36, '3', ')'),
- (0xFE37, '3', '{'),
- (0xFE38, '3', '}'),
- (0xFE39, 'M', '〔'),
- (0xFE3A, 'M', '〕'),
- (0xFE3B, 'M', '【'),
- (0xFE3C, 'M', '】'),
- (0xFE3D, 'M', '《'),
- (0xFE3E, 'M', '》'),
- (0xFE3F, 'M', '〈'),
- (0xFE40, 'M', '〉'),
- (0xFE41, 'M', '「'),
- (0xFE42, 'M', '」'),
- (0xFE43, 'M', '『'),
- (0xFE44, 'M', '』'),
- (0xFE45, 'V'),
- (0xFE47, '3', '['),
- (0xFE48, '3', ']'),
- (0xFE49, '3', ' ̅'),
- (0xFE4D, '3', '_'),
- (0xFE50, '3', ','),
- (0xFE51, 'M', '、'),
- (0xFE52, 'X'),
- (0xFE54, '3', ';'),
- (0xFE55, '3', ':'),
- (0xFE56, '3', '?'),
- (0xFE57, '3', '!'),
- (0xFE58, 'M', '—'),
- (0xFE59, '3', '('),
- (0xFE5A, '3', ')'),
- (0xFE5B, '3', '{'),
- (0xFE5C, '3', '}'),
- (0xFE5D, 'M', '〔'),
- ]
-
-def _seg_50() -> List[Union[Tuple[int, str], Tuple[int, str, str]]]:
- return [
- (0xFE5E, 'M', '〕'),
- (0xFE5F, '3', '#'),
- (0xFE60, '3', '&'),
- (0xFE61, '3', '*'),
- (0xFE62, '3', '+'),
- (0xFE63, 'M', '-'),
- (0xFE64, '3', '<'),
- (0xFE65, '3', '>'),
- (0xFE66, '3', '='),
- (0xFE67, 'X'),
- (0xFE68, '3', '\\'),
- (0xFE69, '3', '$'),
- (0xFE6A, '3', '%'),
- (0xFE6B, '3', '@'),
- (0xFE6C, 'X'),
- (0xFE70, '3', ' ً'),
- (0xFE71, 'M', 'ـً'),
- (0xFE72, '3', ' ٌ'),
- (0xFE73, 'V'),
- (0xFE74, '3', ' ٍ'),
- (0xFE75, 'X'),
- (0xFE76, '3', ' َ'),
- (0xFE77, 'M', 'ـَ'),
- (0xFE78, '3', ' ُ'),
- (0xFE79, 'M', 'ـُ'),
- (0xFE7A, '3', ' ِ'),
- (0xFE7B, 'M', 'ـِ'),
- (0xFE7C, '3', ' ّ'),
- (0xFE7D, 'M', 'ـّ'),
- (0xFE7E, '3', ' ْ'),
- (0xFE7F, 'M', 'ـْ'),
- (0xFE80, 'M', 'ء'),
- (0xFE81, 'M', 'آ'),
- (0xFE83, 'M', 'أ'),
- (0xFE85, 'M', 'ؤ'),
- (0xFE87, 'M', 'إ'),
- (0xFE89, 'M', 'ئ'),
- (0xFE8D, 'M', 'ا'),
- (0xFE8F, 'M', 'ب'),
- (0xFE93, 'M', 'ة'),
- (0xFE95, 'M', 'ت'),
- (0xFE99, 'M', 'ث'),
- (0xFE9D, 'M', 'ج'),
- (0xFEA1, 'M', 'ح'),
- (0xFEA5, 'M', 'خ'),
- (0xFEA9, 'M', 'د'),
- (0xFEAB, 'M', 'ذ'),
- (0xFEAD, 'M', 'ر'),
- (0xFEAF, 'M', 'ز'),
- (0xFEB1, 'M', 'س'),
- (0xFEB5, 'M', 'ش'),
- (0xFEB9, 'M', 'ص'),
- (0xFEBD, 'M', 'ض'),
- (0xFEC1, 'M', 'ط'),
- (0xFEC5, 'M', 'ظ'),
- (0xFEC9, 'M', 'ع'),
- (0xFECD, 'M', 'غ'),
- (0xFED1, 'M', 'ف'),
- (0xFED5, 'M', 'ق'),
- (0xFED9, 'M', 'ك'),
- (0xFEDD, 'M', 'ل'),
- (0xFEE1, 'M', 'م'),
- (0xFEE5, 'M', 'ن'),
- (0xFEE9, 'M', 'ه'),
- (0xFEED, 'M', 'و'),
- (0xFEEF, 'M', 'ى'),
- (0xFEF1, 'M', 'ي'),
- (0xFEF5, 'M', 'لآ'),
- (0xFEF7, 'M', 'لأ'),
- (0xFEF9, 'M', 'لإ'),
- (0xFEFB, 'M', 'لا'),
- (0xFEFD, 'X'),
- (0xFEFF, 'I'),
- (0xFF00, 'X'),
- (0xFF01, '3', '!'),
- (0xFF02, '3', '"'),
- (0xFF03, '3', '#'),
- (0xFF04, '3', '$'),
- (0xFF05, '3', '%'),
- (0xFF06, '3', '&'),
- (0xFF07, '3', '\''),
- (0xFF08, '3', '('),
- (0xFF09, '3', ')'),
- (0xFF0A, '3', '*'),
- (0xFF0B, '3', '+'),
- (0xFF0C, '3', ','),
- (0xFF0D, 'M', '-'),
- (0xFF0E, 'M', '.'),
- (0xFF0F, '3', '/'),
- (0xFF10, 'M', '0'),
- (0xFF11, 'M', '1'),
- (0xFF12, 'M', '2'),
- (0xFF13, 'M', '3'),
- (0xFF14, 'M', '4'),
- (0xFF15, 'M', '5'),
- (0xFF16, 'M', '6'),
- (0xFF17, 'M', '7'),
- (0xFF18, 'M', '8'),
- (0xFF19, 'M', '9'),
- (0xFF1A, '3', ':'),
- ]
-
-def _seg_51() -> List[Union[Tuple[int, str], Tuple[int, str, str]]]:
- return [
- (0xFF1B, '3', ';'),
- (0xFF1C, '3', '<'),
- (0xFF1D, '3', '='),
- (0xFF1E, '3', '>'),
- (0xFF1F, '3', '?'),
- (0xFF20, '3', '@'),
- (0xFF21, 'M', 'a'),
- (0xFF22, 'M', 'b'),
- (0xFF23, 'M', 'c'),
- (0xFF24, 'M', 'd'),
- (0xFF25, 'M', 'e'),
- (0xFF26, 'M', 'f'),
- (0xFF27, 'M', 'g'),
- (0xFF28, 'M', 'h'),
- (0xFF29, 'M', 'i'),
- (0xFF2A, 'M', 'j'),
- (0xFF2B, 'M', 'k'),
- (0xFF2C, 'M', 'l'),
- (0xFF2D, 'M', 'm'),
- (0xFF2E, 'M', 'n'),
- (0xFF2F, 'M', 'o'),
- (0xFF30, 'M', 'p'),
- (0xFF31, 'M', 'q'),
- (0xFF32, 'M', 'r'),
- (0xFF33, 'M', 's'),
- (0xFF34, 'M', 't'),
- (0xFF35, 'M', 'u'),
- (0xFF36, 'M', 'v'),
- (0xFF37, 'M', 'w'),
- (0xFF38, 'M', 'x'),
- (0xFF39, 'M', 'y'),
- (0xFF3A, 'M', 'z'),
- (0xFF3B, '3', '['),
- (0xFF3C, '3', '\\'),
- (0xFF3D, '3', ']'),
- (0xFF3E, '3', '^'),
- (0xFF3F, '3', '_'),
- (0xFF40, '3', '`'),
- (0xFF41, 'M', 'a'),
- (0xFF42, 'M', 'b'),
- (0xFF43, 'M', 'c'),
- (0xFF44, 'M', 'd'),
- (0xFF45, 'M', 'e'),
- (0xFF46, 'M', 'f'),
- (0xFF47, 'M', 'g'),
- (0xFF48, 'M', 'h'),
- (0xFF49, 'M', 'i'),
- (0xFF4A, 'M', 'j'),
- (0xFF4B, 'M', 'k'),
- (0xFF4C, 'M', 'l'),
- (0xFF4D, 'M', 'm'),
- (0xFF4E, 'M', 'n'),
- (0xFF4F, 'M', 'o'),
- (0xFF50, 'M', 'p'),
- (0xFF51, 'M', 'q'),
- (0xFF52, 'M', 'r'),
- (0xFF53, 'M', 's'),
- (0xFF54, 'M', 't'),
- (0xFF55, 'M', 'u'),
- (0xFF56, 'M', 'v'),
- (0xFF57, 'M', 'w'),
- (0xFF58, 'M', 'x'),
- (0xFF59, 'M', 'y'),
- (0xFF5A, 'M', 'z'),
- (0xFF5B, '3', '{'),
- (0xFF5C, '3', '|'),
- (0xFF5D, '3', '}'),
- (0xFF5E, '3', '~'),
- (0xFF5F, 'M', '⦅'),
- (0xFF60, 'M', '⦆'),
- (0xFF61, 'M', '.'),
- (0xFF62, 'M', '「'),
- (0xFF63, 'M', '」'),
- (0xFF64, 'M', '、'),
- (0xFF65, 'M', '・'),
- (0xFF66, 'M', 'ヲ'),
- (0xFF67, 'M', 'ァ'),
- (0xFF68, 'M', 'ィ'),
- (0xFF69, 'M', 'ゥ'),
- (0xFF6A, 'M', 'ェ'),
- (0xFF6B, 'M', 'ォ'),
- (0xFF6C, 'M', 'ャ'),
- (0xFF6D, 'M', 'ュ'),
- (0xFF6E, 'M', 'ョ'),
- (0xFF6F, 'M', 'ッ'),
- (0xFF70, 'M', 'ー'),
- (0xFF71, 'M', 'ア'),
- (0xFF72, 'M', 'イ'),
- (0xFF73, 'M', 'ウ'),
- (0xFF74, 'M', 'エ'),
- (0xFF75, 'M', 'オ'),
- (0xFF76, 'M', 'カ'),
- (0xFF77, 'M', 'キ'),
- (0xFF78, 'M', 'ク'),
- (0xFF79, 'M', 'ケ'),
- (0xFF7A, 'M', 'コ'),
- (0xFF7B, 'M', 'サ'),
- (0xFF7C, 'M', 'シ'),
- (0xFF7D, 'M', 'ス'),
- (0xFF7E, 'M', 'セ'),
- ]
-
-def _seg_52() -> List[Union[Tuple[int, str], Tuple[int, str, str]]]:
- return [
- (0xFF7F, 'M', 'ソ'),
- (0xFF80, 'M', 'タ'),
- (0xFF81, 'M', 'チ'),
- (0xFF82, 'M', 'ツ'),
- (0xFF83, 'M', 'テ'),
- (0xFF84, 'M', 'ト'),
- (0xFF85, 'M', 'ナ'),
- (0xFF86, 'M', 'ニ'),
- (0xFF87, 'M', 'ヌ'),
- (0xFF88, 'M', 'ネ'),
- (0xFF89, 'M', 'ノ'),
- (0xFF8A, 'M', 'ハ'),
- (0xFF8B, 'M', 'ヒ'),
- (0xFF8C, 'M', 'フ'),
- (0xFF8D, 'M', 'ヘ'),
- (0xFF8E, 'M', 'ホ'),
- (0xFF8F, 'M', 'マ'),
- (0xFF90, 'M', 'ミ'),
- (0xFF91, 'M', 'ム'),
- (0xFF92, 'M', 'メ'),
- (0xFF93, 'M', 'モ'),
- (0xFF94, 'M', 'ヤ'),
- (0xFF95, 'M', 'ユ'),
- (0xFF96, 'M', 'ヨ'),
- (0xFF97, 'M', 'ラ'),
- (0xFF98, 'M', 'リ'),
- (0xFF99, 'M', 'ル'),
- (0xFF9A, 'M', 'レ'),
- (0xFF9B, 'M', 'ロ'),
- (0xFF9C, 'M', 'ワ'),
- (0xFF9D, 'M', 'ン'),
- (0xFF9E, 'M', '゙'),
- (0xFF9F, 'M', '゚'),
- (0xFFA0, 'X'),
- (0xFFA1, 'M', 'ᄀ'),
- (0xFFA2, 'M', 'ᄁ'),
- (0xFFA3, 'M', 'ᆪ'),
- (0xFFA4, 'M', 'ᄂ'),
- (0xFFA5, 'M', 'ᆬ'),
- (0xFFA6, 'M', 'ᆭ'),
- (0xFFA7, 'M', 'ᄃ'),
- (0xFFA8, 'M', 'ᄄ'),
- (0xFFA9, 'M', 'ᄅ'),
- (0xFFAA, 'M', 'ᆰ'),
- (0xFFAB, 'M', 'ᆱ'),
- (0xFFAC, 'M', 'ᆲ'),
- (0xFFAD, 'M', 'ᆳ'),
- (0xFFAE, 'M', 'ᆴ'),
- (0xFFAF, 'M', 'ᆵ'),
- (0xFFB0, 'M', 'ᄚ'),
- (0xFFB1, 'M', 'ᄆ'),
- (0xFFB2, 'M', 'ᄇ'),
- (0xFFB3, 'M', 'ᄈ'),
- (0xFFB4, 'M', 'ᄡ'),
- (0xFFB5, 'M', 'ᄉ'),
- (0xFFB6, 'M', 'ᄊ'),
- (0xFFB7, 'M', 'ᄋ'),
- (0xFFB8, 'M', 'ᄌ'),
- (0xFFB9, 'M', 'ᄍ'),
- (0xFFBA, 'M', 'ᄎ'),
- (0xFFBB, 'M', 'ᄏ'),
- (0xFFBC, 'M', 'ᄐ'),
- (0xFFBD, 'M', 'ᄑ'),
- (0xFFBE, 'M', 'ᄒ'),
- (0xFFBF, 'X'),
- (0xFFC2, 'M', 'ᅡ'),
- (0xFFC3, 'M', 'ᅢ'),
- (0xFFC4, 'M', 'ᅣ'),
- (0xFFC5, 'M', 'ᅤ'),
- (0xFFC6, 'M', 'ᅥ'),
- (0xFFC7, 'M', 'ᅦ'),
- (0xFFC8, 'X'),
- (0xFFCA, 'M', 'ᅧ'),
- (0xFFCB, 'M', 'ᅨ'),
- (0xFFCC, 'M', 'ᅩ'),
- (0xFFCD, 'M', 'ᅪ'),
- (0xFFCE, 'M', 'ᅫ'),
- (0xFFCF, 'M', 'ᅬ'),
- (0xFFD0, 'X'),
- (0xFFD2, 'M', 'ᅭ'),
- (0xFFD3, 'M', 'ᅮ'),
- (0xFFD4, 'M', 'ᅯ'),
- (0xFFD5, 'M', 'ᅰ'),
- (0xFFD6, 'M', 'ᅱ'),
- (0xFFD7, 'M', 'ᅲ'),
- (0xFFD8, 'X'),
- (0xFFDA, 'M', 'ᅳ'),
- (0xFFDB, 'M', 'ᅴ'),
- (0xFFDC, 'M', 'ᅵ'),
- (0xFFDD, 'X'),
- (0xFFE0, 'M', '¢'),
- (0xFFE1, 'M', '£'),
- (0xFFE2, 'M', '¬'),
- (0xFFE3, '3', ' ̄'),
- (0xFFE4, 'M', '¦'),
- (0xFFE5, 'M', '¥'),
- (0xFFE6, 'M', '₩'),
- (0xFFE7, 'X'),
- (0xFFE8, 'M', '│'),
- (0xFFE9, 'M', '←'),
- ]
-
-def _seg_53() -> List[Union[Tuple[int, str], Tuple[int, str, str]]]:
- return [
- (0xFFEA, 'M', '↑'),
- (0xFFEB, 'M', '→'),
- (0xFFEC, 'M', '↓'),
- (0xFFED, 'M', '■'),
- (0xFFEE, 'M', '○'),
- (0xFFEF, 'X'),
- (0x10000, 'V'),
- (0x1000C, 'X'),
- (0x1000D, 'V'),
- (0x10027, 'X'),
- (0x10028, 'V'),
- (0x1003B, 'X'),
- (0x1003C, 'V'),
- (0x1003E, 'X'),
- (0x1003F, 'V'),
- (0x1004E, 'X'),
- (0x10050, 'V'),
- (0x1005E, 'X'),
- (0x10080, 'V'),
- (0x100FB, 'X'),
- (0x10100, 'V'),
- (0x10103, 'X'),
- (0x10107, 'V'),
- (0x10134, 'X'),
- (0x10137, 'V'),
- (0x1018F, 'X'),
- (0x10190, 'V'),
- (0x1019D, 'X'),
- (0x101A0, 'V'),
- (0x101A1, 'X'),
- (0x101D0, 'V'),
- (0x101FE, 'X'),
- (0x10280, 'V'),
- (0x1029D, 'X'),
- (0x102A0, 'V'),
- (0x102D1, 'X'),
- (0x102E0, 'V'),
- (0x102FC, 'X'),
- (0x10300, 'V'),
- (0x10324, 'X'),
- (0x1032D, 'V'),
- (0x1034B, 'X'),
- (0x10350, 'V'),
- (0x1037B, 'X'),
- (0x10380, 'V'),
- (0x1039E, 'X'),
- (0x1039F, 'V'),
- (0x103C4, 'X'),
- (0x103C8, 'V'),
- (0x103D6, 'X'),
- (0x10400, 'M', '𐐨'),
- (0x10401, 'M', '𐐩'),
- (0x10402, 'M', '𐐪'),
- (0x10403, 'M', '𐐫'),
- (0x10404, 'M', '𐐬'),
- (0x10405, 'M', '𐐭'),
- (0x10406, 'M', '𐐮'),
- (0x10407, 'M', '𐐯'),
- (0x10408, 'M', '𐐰'),
- (0x10409, 'M', '𐐱'),
- (0x1040A, 'M', '𐐲'),
- (0x1040B, 'M', '𐐳'),
- (0x1040C, 'M', '𐐴'),
- (0x1040D, 'M', '𐐵'),
- (0x1040E, 'M', '𐐶'),
- (0x1040F, 'M', '𐐷'),
- (0x10410, 'M', '𐐸'),
- (0x10411, 'M', '𐐹'),
- (0x10412, 'M', '𐐺'),
- (0x10413, 'M', '𐐻'),
- (0x10414, 'M', '𐐼'),
- (0x10415, 'M', '𐐽'),
- (0x10416, 'M', '𐐾'),
- (0x10417, 'M', '𐐿'),
- (0x10418, 'M', '𐑀'),
- (0x10419, 'M', '𐑁'),
- (0x1041A, 'M', '𐑂'),
- (0x1041B, 'M', '𐑃'),
- (0x1041C, 'M', '𐑄'),
- (0x1041D, 'M', '𐑅'),
- (0x1041E, 'M', '𐑆'),
- (0x1041F, 'M', '𐑇'),
- (0x10420, 'M', '𐑈'),
- (0x10421, 'M', '𐑉'),
- (0x10422, 'M', '𐑊'),
- (0x10423, 'M', '𐑋'),
- (0x10424, 'M', '𐑌'),
- (0x10425, 'M', '𐑍'),
- (0x10426, 'M', '𐑎'),
- (0x10427, 'M', '𐑏'),
- (0x10428, 'V'),
- (0x1049E, 'X'),
- (0x104A0, 'V'),
- (0x104AA, 'X'),
- (0x104B0, 'M', '𐓘'),
- (0x104B1, 'M', '𐓙'),
- (0x104B2, 'M', '𐓚'),
- (0x104B3, 'M', '𐓛'),
- (0x104B4, 'M', '𐓜'),
- (0x104B5, 'M', '𐓝'),
- ]
-
-def _seg_54() -> List[Union[Tuple[int, str], Tuple[int, str, str]]]:
- return [
- (0x104B6, 'M', '𐓞'),
- (0x104B7, 'M', '𐓟'),
- (0x104B8, 'M', '𐓠'),
- (0x104B9, 'M', '𐓡'),
- (0x104BA, 'M', '𐓢'),
- (0x104BB, 'M', '𐓣'),
- (0x104BC, 'M', '𐓤'),
- (0x104BD, 'M', '𐓥'),
- (0x104BE, 'M', '𐓦'),
- (0x104BF, 'M', '𐓧'),
- (0x104C0, 'M', '𐓨'),
- (0x104C1, 'M', '𐓩'),
- (0x104C2, 'M', '𐓪'),
- (0x104C3, 'M', '𐓫'),
- (0x104C4, 'M', '𐓬'),
- (0x104C5, 'M', '𐓭'),
- (0x104C6, 'M', '𐓮'),
- (0x104C7, 'M', '𐓯'),
- (0x104C8, 'M', '𐓰'),
- (0x104C9, 'M', '𐓱'),
- (0x104CA, 'M', '𐓲'),
- (0x104CB, 'M', '𐓳'),
- (0x104CC, 'M', '𐓴'),
- (0x104CD, 'M', '𐓵'),
- (0x104CE, 'M', '𐓶'),
- (0x104CF, 'M', '𐓷'),
- (0x104D0, 'M', '𐓸'),
- (0x104D1, 'M', '𐓹'),
- (0x104D2, 'M', '𐓺'),
- (0x104D3, 'M', '𐓻'),
- (0x104D4, 'X'),
- (0x104D8, 'V'),
- (0x104FC, 'X'),
- (0x10500, 'V'),
- (0x10528, 'X'),
- (0x10530, 'V'),
- (0x10564, 'X'),
- (0x1056F, 'V'),
- (0x10570, 'M', '𐖗'),
- (0x10571, 'M', '𐖘'),
- (0x10572, 'M', '𐖙'),
- (0x10573, 'M', '𐖚'),
- (0x10574, 'M', '𐖛'),
- (0x10575, 'M', '𐖜'),
- (0x10576, 'M', '𐖝'),
- (0x10577, 'M', '𐖞'),
- (0x10578, 'M', '𐖟'),
- (0x10579, 'M', '𐖠'),
- (0x1057A, 'M', '𐖡'),
- (0x1057B, 'X'),
- (0x1057C, 'M', '𐖣'),
- (0x1057D, 'M', '𐖤'),
- (0x1057E, 'M', '𐖥'),
- (0x1057F, 'M', '𐖦'),
- (0x10580, 'M', '𐖧'),
- (0x10581, 'M', '𐖨'),
- (0x10582, 'M', '𐖩'),
- (0x10583, 'M', '𐖪'),
- (0x10584, 'M', '𐖫'),
- (0x10585, 'M', '𐖬'),
- (0x10586, 'M', '𐖭'),
- (0x10587, 'M', '𐖮'),
- (0x10588, 'M', '𐖯'),
- (0x10589, 'M', '𐖰'),
- (0x1058A, 'M', '𐖱'),
- (0x1058B, 'X'),
- (0x1058C, 'M', '𐖳'),
- (0x1058D, 'M', '𐖴'),
- (0x1058E, 'M', '𐖵'),
- (0x1058F, 'M', '𐖶'),
- (0x10590, 'M', '𐖷'),
- (0x10591, 'M', '𐖸'),
- (0x10592, 'M', '𐖹'),
- (0x10593, 'X'),
- (0x10594, 'M', '𐖻'),
- (0x10595, 'M', '𐖼'),
- (0x10596, 'X'),
- (0x10597, 'V'),
- (0x105A2, 'X'),
- (0x105A3, 'V'),
- (0x105B2, 'X'),
- (0x105B3, 'V'),
- (0x105BA, 'X'),
- (0x105BB, 'V'),
- (0x105BD, 'X'),
- (0x10600, 'V'),
- (0x10737, 'X'),
- (0x10740, 'V'),
- (0x10756, 'X'),
- (0x10760, 'V'),
- (0x10768, 'X'),
- (0x10780, 'V'),
- (0x10781, 'M', 'ː'),
- (0x10782, 'M', 'ˑ'),
- (0x10783, 'M', 'æ'),
- (0x10784, 'M', 'ʙ'),
- (0x10785, 'M', 'ɓ'),
- (0x10786, 'X'),
- (0x10787, 'M', 'ʣ'),
- (0x10788, 'M', 'ꭦ'),
- ]
-
-def _seg_55() -> List[Union[Tuple[int, str], Tuple[int, str, str]]]:
- return [
- (0x10789, 'M', 'ʥ'),
- (0x1078A, 'M', 'ʤ'),
- (0x1078B, 'M', 'ɖ'),
- (0x1078C, 'M', 'ɗ'),
- (0x1078D, 'M', 'ᶑ'),
- (0x1078E, 'M', 'ɘ'),
- (0x1078F, 'M', 'ɞ'),
- (0x10790, 'M', 'ʩ'),
- (0x10791, 'M', 'ɤ'),
- (0x10792, 'M', 'ɢ'),
- (0x10793, 'M', 'ɠ'),
- (0x10794, 'M', 'ʛ'),
- (0x10795, 'M', 'ħ'),
- (0x10796, 'M', 'ʜ'),
- (0x10797, 'M', 'ɧ'),
- (0x10798, 'M', 'ʄ'),
- (0x10799, 'M', 'ʪ'),
- (0x1079A, 'M', 'ʫ'),
- (0x1079B, 'M', 'ɬ'),
- (0x1079C, 'M', '𝼄'),
- (0x1079D, 'M', 'ꞎ'),
- (0x1079E, 'M', 'ɮ'),
- (0x1079F, 'M', '𝼅'),
- (0x107A0, 'M', 'ʎ'),
- (0x107A1, 'M', '𝼆'),
- (0x107A2, 'M', 'ø'),
- (0x107A3, 'M', 'ɶ'),
- (0x107A4, 'M', 'ɷ'),
- (0x107A5, 'M', 'q'),
- (0x107A6, 'M', 'ɺ'),
- (0x107A7, 'M', '𝼈'),
- (0x107A8, 'M', 'ɽ'),
- (0x107A9, 'M', 'ɾ'),
- (0x107AA, 'M', 'ʀ'),
- (0x107AB, 'M', 'ʨ'),
- (0x107AC, 'M', 'ʦ'),
- (0x107AD, 'M', 'ꭧ'),
- (0x107AE, 'M', 'ʧ'),
- (0x107AF, 'M', 'ʈ'),
- (0x107B0, 'M', 'ⱱ'),
- (0x107B1, 'X'),
- (0x107B2, 'M', 'ʏ'),
- (0x107B3, 'M', 'ʡ'),
- (0x107B4, 'M', 'ʢ'),
- (0x107B5, 'M', 'ʘ'),
- (0x107B6, 'M', 'ǀ'),
- (0x107B7, 'M', 'ǁ'),
- (0x107B8, 'M', 'ǂ'),
- (0x107B9, 'M', '𝼊'),
- (0x107BA, 'M', '𝼞'),
- (0x107BB, 'X'),
- (0x10800, 'V'),
- (0x10806, 'X'),
- (0x10808, 'V'),
- (0x10809, 'X'),
- (0x1080A, 'V'),
- (0x10836, 'X'),
- (0x10837, 'V'),
- (0x10839, 'X'),
- (0x1083C, 'V'),
- (0x1083D, 'X'),
- (0x1083F, 'V'),
- (0x10856, 'X'),
- (0x10857, 'V'),
- (0x1089F, 'X'),
- (0x108A7, 'V'),
- (0x108B0, 'X'),
- (0x108E0, 'V'),
- (0x108F3, 'X'),
- (0x108F4, 'V'),
- (0x108F6, 'X'),
- (0x108FB, 'V'),
- (0x1091C, 'X'),
- (0x1091F, 'V'),
- (0x1093A, 'X'),
- (0x1093F, 'V'),
- (0x10940, 'X'),
- (0x10980, 'V'),
- (0x109B8, 'X'),
- (0x109BC, 'V'),
- (0x109D0, 'X'),
- (0x109D2, 'V'),
- (0x10A04, 'X'),
- (0x10A05, 'V'),
- (0x10A07, 'X'),
- (0x10A0C, 'V'),
- (0x10A14, 'X'),
- (0x10A15, 'V'),
- (0x10A18, 'X'),
- (0x10A19, 'V'),
- (0x10A36, 'X'),
- (0x10A38, 'V'),
- (0x10A3B, 'X'),
- (0x10A3F, 'V'),
- (0x10A49, 'X'),
- (0x10A50, 'V'),
- (0x10A59, 'X'),
- (0x10A60, 'V'),
- (0x10AA0, 'X'),
- (0x10AC0, 'V'),
- ]
-
-def _seg_56() -> List[Union[Tuple[int, str], Tuple[int, str, str]]]:
- return [
- (0x10AE7, 'X'),
- (0x10AEB, 'V'),
- (0x10AF7, 'X'),
- (0x10B00, 'V'),
- (0x10B36, 'X'),
- (0x10B39, 'V'),
- (0x10B56, 'X'),
- (0x10B58, 'V'),
- (0x10B73, 'X'),
- (0x10B78, 'V'),
- (0x10B92, 'X'),
- (0x10B99, 'V'),
- (0x10B9D, 'X'),
- (0x10BA9, 'V'),
- (0x10BB0, 'X'),
- (0x10C00, 'V'),
- (0x10C49, 'X'),
- (0x10C80, 'M', '𐳀'),
- (0x10C81, 'M', '𐳁'),
- (0x10C82, 'M', '𐳂'),
- (0x10C83, 'M', '𐳃'),
- (0x10C84, 'M', '𐳄'),
- (0x10C85, 'M', '𐳅'),
- (0x10C86, 'M', '𐳆'),
- (0x10C87, 'M', '𐳇'),
- (0x10C88, 'M', '𐳈'),
- (0x10C89, 'M', '𐳉'),
- (0x10C8A, 'M', '𐳊'),
- (0x10C8B, 'M', '𐳋'),
- (0x10C8C, 'M', '𐳌'),
- (0x10C8D, 'M', '𐳍'),
- (0x10C8E, 'M', '𐳎'),
- (0x10C8F, 'M', '𐳏'),
- (0x10C90, 'M', '𐳐'),
- (0x10C91, 'M', '𐳑'),
- (0x10C92, 'M', '𐳒'),
- (0x10C93, 'M', '𐳓'),
- (0x10C94, 'M', '𐳔'),
- (0x10C95, 'M', '𐳕'),
- (0x10C96, 'M', '𐳖'),
- (0x10C97, 'M', '𐳗'),
- (0x10C98, 'M', '𐳘'),
- (0x10C99, 'M', '𐳙'),
- (0x10C9A, 'M', '𐳚'),
- (0x10C9B, 'M', '𐳛'),
- (0x10C9C, 'M', '𐳜'),
- (0x10C9D, 'M', '𐳝'),
- (0x10C9E, 'M', '𐳞'),
- (0x10C9F, 'M', '𐳟'),
- (0x10CA0, 'M', '𐳠'),
- (0x10CA1, 'M', '𐳡'),
- (0x10CA2, 'M', '𐳢'),
- (0x10CA3, 'M', '𐳣'),
- (0x10CA4, 'M', '𐳤'),
- (0x10CA5, 'M', '𐳥'),
- (0x10CA6, 'M', '𐳦'),
- (0x10CA7, 'M', '𐳧'),
- (0x10CA8, 'M', '𐳨'),
- (0x10CA9, 'M', '𐳩'),
- (0x10CAA, 'M', '𐳪'),
- (0x10CAB, 'M', '𐳫'),
- (0x10CAC, 'M', '𐳬'),
- (0x10CAD, 'M', '𐳭'),
- (0x10CAE, 'M', '𐳮'),
- (0x10CAF, 'M', '𐳯'),
- (0x10CB0, 'M', '𐳰'),
- (0x10CB1, 'M', '𐳱'),
- (0x10CB2, 'M', '𐳲'),
- (0x10CB3, 'X'),
- (0x10CC0, 'V'),
- (0x10CF3, 'X'),
- (0x10CFA, 'V'),
- (0x10D28, 'X'),
- (0x10D30, 'V'),
- (0x10D3A, 'X'),
- (0x10E60, 'V'),
- (0x10E7F, 'X'),
- (0x10E80, 'V'),
- (0x10EAA, 'X'),
- (0x10EAB, 'V'),
- (0x10EAE, 'X'),
- (0x10EB0, 'V'),
- (0x10EB2, 'X'),
- (0x10F00, 'V'),
- (0x10F28, 'X'),
- (0x10F30, 'V'),
- (0x10F5A, 'X'),
- (0x10F70, 'V'),
- (0x10F8A, 'X'),
- (0x10FB0, 'V'),
- (0x10FCC, 'X'),
- (0x10FE0, 'V'),
- (0x10FF7, 'X'),
- (0x11000, 'V'),
- (0x1104E, 'X'),
- (0x11052, 'V'),
- (0x11076, 'X'),
- (0x1107F, 'V'),
- (0x110BD, 'X'),
- (0x110BE, 'V'),
- ]
-
-def _seg_57() -> List[Union[Tuple[int, str], Tuple[int, str, str]]]:
- return [
- (0x110C3, 'X'),
- (0x110D0, 'V'),
- (0x110E9, 'X'),
- (0x110F0, 'V'),
- (0x110FA, 'X'),
- (0x11100, 'V'),
- (0x11135, 'X'),
- (0x11136, 'V'),
- (0x11148, 'X'),
- (0x11150, 'V'),
- (0x11177, 'X'),
- (0x11180, 'V'),
- (0x111E0, 'X'),
- (0x111E1, 'V'),
- (0x111F5, 'X'),
- (0x11200, 'V'),
- (0x11212, 'X'),
- (0x11213, 'V'),
- (0x1123F, 'X'),
- (0x11280, 'V'),
- (0x11287, 'X'),
- (0x11288, 'V'),
- (0x11289, 'X'),
- (0x1128A, 'V'),
- (0x1128E, 'X'),
- (0x1128F, 'V'),
- (0x1129E, 'X'),
- (0x1129F, 'V'),
- (0x112AA, 'X'),
- (0x112B0, 'V'),
- (0x112EB, 'X'),
- (0x112F0, 'V'),
- (0x112FA, 'X'),
- (0x11300, 'V'),
- (0x11304, 'X'),
- (0x11305, 'V'),
- (0x1130D, 'X'),
- (0x1130F, 'V'),
- (0x11311, 'X'),
- (0x11313, 'V'),
- (0x11329, 'X'),
- (0x1132A, 'V'),
- (0x11331, 'X'),
- (0x11332, 'V'),
- (0x11334, 'X'),
- (0x11335, 'V'),
- (0x1133A, 'X'),
- (0x1133B, 'V'),
- (0x11345, 'X'),
- (0x11347, 'V'),
- (0x11349, 'X'),
- (0x1134B, 'V'),
- (0x1134E, 'X'),
- (0x11350, 'V'),
- (0x11351, 'X'),
- (0x11357, 'V'),
- (0x11358, 'X'),
- (0x1135D, 'V'),
- (0x11364, 'X'),
- (0x11366, 'V'),
- (0x1136D, 'X'),
- (0x11370, 'V'),
- (0x11375, 'X'),
- (0x11400, 'V'),
- (0x1145C, 'X'),
- (0x1145D, 'V'),
- (0x11462, 'X'),
- (0x11480, 'V'),
- (0x114C8, 'X'),
- (0x114D0, 'V'),
- (0x114DA, 'X'),
- (0x11580, 'V'),
- (0x115B6, 'X'),
- (0x115B8, 'V'),
- (0x115DE, 'X'),
- (0x11600, 'V'),
- (0x11645, 'X'),
- (0x11650, 'V'),
- (0x1165A, 'X'),
- (0x11660, 'V'),
- (0x1166D, 'X'),
- (0x11680, 'V'),
- (0x116BA, 'X'),
- (0x116C0, 'V'),
- (0x116CA, 'X'),
- (0x11700, 'V'),
- (0x1171B, 'X'),
- (0x1171D, 'V'),
- (0x1172C, 'X'),
- (0x11730, 'V'),
- (0x11747, 'X'),
- (0x11800, 'V'),
- (0x1183C, 'X'),
- (0x118A0, 'M', '𑣀'),
- (0x118A1, 'M', '𑣁'),
- (0x118A2, 'M', '𑣂'),
- (0x118A3, 'M', '𑣃'),
- (0x118A4, 'M', '𑣄'),
- (0x118A5, 'M', '𑣅'),
- (0x118A6, 'M', '𑣆'),
- ]
-
-def _seg_58() -> List[Union[Tuple[int, str], Tuple[int, str, str]]]:
- return [
- (0x118A7, 'M', '𑣇'),
- (0x118A8, 'M', '𑣈'),
- (0x118A9, 'M', '𑣉'),
- (0x118AA, 'M', '𑣊'),
- (0x118AB, 'M', '𑣋'),
- (0x118AC, 'M', '𑣌'),
- (0x118AD, 'M', '𑣍'),
- (0x118AE, 'M', '𑣎'),
- (0x118AF, 'M', '𑣏'),
- (0x118B0, 'M', '𑣐'),
- (0x118B1, 'M', '𑣑'),
- (0x118B2, 'M', '𑣒'),
- (0x118B3, 'M', '𑣓'),
- (0x118B4, 'M', '𑣔'),
- (0x118B5, 'M', '𑣕'),
- (0x118B6, 'M', '𑣖'),
- (0x118B7, 'M', '𑣗'),
- (0x118B8, 'M', '𑣘'),
- (0x118B9, 'M', '𑣙'),
- (0x118BA, 'M', '𑣚'),
- (0x118BB, 'M', '𑣛'),
- (0x118BC, 'M', '𑣜'),
- (0x118BD, 'M', '𑣝'),
- (0x118BE, 'M', '𑣞'),
- (0x118BF, 'M', '𑣟'),
- (0x118C0, 'V'),
- (0x118F3, 'X'),
- (0x118FF, 'V'),
- (0x11907, 'X'),
- (0x11909, 'V'),
- (0x1190A, 'X'),
- (0x1190C, 'V'),
- (0x11914, 'X'),
- (0x11915, 'V'),
- (0x11917, 'X'),
- (0x11918, 'V'),
- (0x11936, 'X'),
- (0x11937, 'V'),
- (0x11939, 'X'),
- (0x1193B, 'V'),
- (0x11947, 'X'),
- (0x11950, 'V'),
- (0x1195A, 'X'),
- (0x119A0, 'V'),
- (0x119A8, 'X'),
- (0x119AA, 'V'),
- (0x119D8, 'X'),
- (0x119DA, 'V'),
- (0x119E5, 'X'),
- (0x11A00, 'V'),
- (0x11A48, 'X'),
- (0x11A50, 'V'),
- (0x11AA3, 'X'),
- (0x11AB0, 'V'),
- (0x11AF9, 'X'),
- (0x11C00, 'V'),
- (0x11C09, 'X'),
- (0x11C0A, 'V'),
- (0x11C37, 'X'),
- (0x11C38, 'V'),
- (0x11C46, 'X'),
- (0x11C50, 'V'),
- (0x11C6D, 'X'),
- (0x11C70, 'V'),
- (0x11C90, 'X'),
- (0x11C92, 'V'),
- (0x11CA8, 'X'),
- (0x11CA9, 'V'),
- (0x11CB7, 'X'),
- (0x11D00, 'V'),
- (0x11D07, 'X'),
- (0x11D08, 'V'),
- (0x11D0A, 'X'),
- (0x11D0B, 'V'),
- (0x11D37, 'X'),
- (0x11D3A, 'V'),
- (0x11D3B, 'X'),
- (0x11D3C, 'V'),
- (0x11D3E, 'X'),
- (0x11D3F, 'V'),
- (0x11D48, 'X'),
- (0x11D50, 'V'),
- (0x11D5A, 'X'),
- (0x11D60, 'V'),
- (0x11D66, 'X'),
- (0x11D67, 'V'),
- (0x11D69, 'X'),
- (0x11D6A, 'V'),
- (0x11D8F, 'X'),
- (0x11D90, 'V'),
- (0x11D92, 'X'),
- (0x11D93, 'V'),
- (0x11D99, 'X'),
- (0x11DA0, 'V'),
- (0x11DAA, 'X'),
- (0x11EE0, 'V'),
- (0x11EF9, 'X'),
- (0x11FB0, 'V'),
- (0x11FB1, 'X'),
- (0x11FC0, 'V'),
- ]
-
-def _seg_59() -> List[Union[Tuple[int, str], Tuple[int, str, str]]]:
- return [
- (0x11FF2, 'X'),
- (0x11FFF, 'V'),
- (0x1239A, 'X'),
- (0x12400, 'V'),
- (0x1246F, 'X'),
- (0x12470, 'V'),
- (0x12475, 'X'),
- (0x12480, 'V'),
- (0x12544, 'X'),
- (0x12F90, 'V'),
- (0x12FF3, 'X'),
- (0x13000, 'V'),
- (0x1342F, 'X'),
- (0x14400, 'V'),
- (0x14647, 'X'),
- (0x16800, 'V'),
- (0x16A39, 'X'),
- (0x16A40, 'V'),
- (0x16A5F, 'X'),
- (0x16A60, 'V'),
- (0x16A6A, 'X'),
- (0x16A6E, 'V'),
- (0x16ABF, 'X'),
- (0x16AC0, 'V'),
- (0x16ACA, 'X'),
- (0x16AD0, 'V'),
- (0x16AEE, 'X'),
- (0x16AF0, 'V'),
- (0x16AF6, 'X'),
- (0x16B00, 'V'),
- (0x16B46, 'X'),
- (0x16B50, 'V'),
- (0x16B5A, 'X'),
- (0x16B5B, 'V'),
- (0x16B62, 'X'),
- (0x16B63, 'V'),
- (0x16B78, 'X'),
- (0x16B7D, 'V'),
- (0x16B90, 'X'),
- (0x16E40, 'M', '𖹠'),
- (0x16E41, 'M', '𖹡'),
- (0x16E42, 'M', '𖹢'),
- (0x16E43, 'M', '𖹣'),
- (0x16E44, 'M', '𖹤'),
- (0x16E45, 'M', '𖹥'),
- (0x16E46, 'M', '𖹦'),
- (0x16E47, 'M', '𖹧'),
- (0x16E48, 'M', '𖹨'),
- (0x16E49, 'M', '𖹩'),
- (0x16E4A, 'M', '𖹪'),
- (0x16E4B, 'M', '𖹫'),
- (0x16E4C, 'M', '𖹬'),
- (0x16E4D, 'M', '𖹭'),
- (0x16E4E, 'M', '𖹮'),
- (0x16E4F, 'M', '𖹯'),
- (0x16E50, 'M', '𖹰'),
- (0x16E51, 'M', '𖹱'),
- (0x16E52, 'M', '𖹲'),
- (0x16E53, 'M', '𖹳'),
- (0x16E54, 'M', '𖹴'),
- (0x16E55, 'M', '𖹵'),
- (0x16E56, 'M', '𖹶'),
- (0x16E57, 'M', '𖹷'),
- (0x16E58, 'M', '𖹸'),
- (0x16E59, 'M', '𖹹'),
- (0x16E5A, 'M', '𖹺'),
- (0x16E5B, 'M', '𖹻'),
- (0x16E5C, 'M', '𖹼'),
- (0x16E5D, 'M', '𖹽'),
- (0x16E5E, 'M', '𖹾'),
- (0x16E5F, 'M', '𖹿'),
- (0x16E60, 'V'),
- (0x16E9B, 'X'),
- (0x16F00, 'V'),
- (0x16F4B, 'X'),
- (0x16F4F, 'V'),
- (0x16F88, 'X'),
- (0x16F8F, 'V'),
- (0x16FA0, 'X'),
- (0x16FE0, 'V'),
- (0x16FE5, 'X'),
- (0x16FF0, 'V'),
- (0x16FF2, 'X'),
- (0x17000, 'V'),
- (0x187F8, 'X'),
- (0x18800, 'V'),
- (0x18CD6, 'X'),
- (0x18D00, 'V'),
- (0x18D09, 'X'),
- (0x1AFF0, 'V'),
- (0x1AFF4, 'X'),
- (0x1AFF5, 'V'),
- (0x1AFFC, 'X'),
- (0x1AFFD, 'V'),
- (0x1AFFF, 'X'),
- (0x1B000, 'V'),
- (0x1B123, 'X'),
- (0x1B150, 'V'),
- (0x1B153, 'X'),
- (0x1B164, 'V'),
- ]
-
-def _seg_60() -> List[Union[Tuple[int, str], Tuple[int, str, str]]]:
- return [
- (0x1B168, 'X'),
- (0x1B170, 'V'),
- (0x1B2FC, 'X'),
- (0x1BC00, 'V'),
- (0x1BC6B, 'X'),
- (0x1BC70, 'V'),
- (0x1BC7D, 'X'),
- (0x1BC80, 'V'),
- (0x1BC89, 'X'),
- (0x1BC90, 'V'),
- (0x1BC9A, 'X'),
- (0x1BC9C, 'V'),
- (0x1BCA0, 'I'),
- (0x1BCA4, 'X'),
- (0x1CF00, 'V'),
- (0x1CF2E, 'X'),
- (0x1CF30, 'V'),
- (0x1CF47, 'X'),
- (0x1CF50, 'V'),
- (0x1CFC4, 'X'),
- (0x1D000, 'V'),
- (0x1D0F6, 'X'),
- (0x1D100, 'V'),
- (0x1D127, 'X'),
- (0x1D129, 'V'),
- (0x1D15E, 'M', '𝅗𝅥'),
- (0x1D15F, 'M', '𝅘𝅥'),
- (0x1D160, 'M', '𝅘𝅥𝅮'),
- (0x1D161, 'M', '𝅘𝅥𝅯'),
- (0x1D162, 'M', '𝅘𝅥𝅰'),
- (0x1D163, 'M', '𝅘𝅥𝅱'),
- (0x1D164, 'M', '𝅘𝅥𝅲'),
- (0x1D165, 'V'),
- (0x1D173, 'X'),
- (0x1D17B, 'V'),
- (0x1D1BB, 'M', '𝆹𝅥'),
- (0x1D1BC, 'M', '𝆺𝅥'),
- (0x1D1BD, 'M', '𝆹𝅥𝅮'),
- (0x1D1BE, 'M', '𝆺𝅥𝅮'),
- (0x1D1BF, 'M', '𝆹𝅥𝅯'),
- (0x1D1C0, 'M', '𝆺𝅥𝅯'),
- (0x1D1C1, 'V'),
- (0x1D1EB, 'X'),
- (0x1D200, 'V'),
- (0x1D246, 'X'),
- (0x1D2E0, 'V'),
- (0x1D2F4, 'X'),
- (0x1D300, 'V'),
- (0x1D357, 'X'),
- (0x1D360, 'V'),
- (0x1D379, 'X'),
- (0x1D400, 'M', 'a'),
- (0x1D401, 'M', 'b'),
- (0x1D402, 'M', 'c'),
- (0x1D403, 'M', 'd'),
- (0x1D404, 'M', 'e'),
- (0x1D405, 'M', 'f'),
- (0x1D406, 'M', 'g'),
- (0x1D407, 'M', 'h'),
- (0x1D408, 'M', 'i'),
- (0x1D409, 'M', 'j'),
- (0x1D40A, 'M', 'k'),
- (0x1D40B, 'M', 'l'),
- (0x1D40C, 'M', 'm'),
- (0x1D40D, 'M', 'n'),
- (0x1D40E, 'M', 'o'),
- (0x1D40F, 'M', 'p'),
- (0x1D410, 'M', 'q'),
- (0x1D411, 'M', 'r'),
- (0x1D412, 'M', 's'),
- (0x1D413, 'M', 't'),
- (0x1D414, 'M', 'u'),
- (0x1D415, 'M', 'v'),
- (0x1D416, 'M', 'w'),
- (0x1D417, 'M', 'x'),
- (0x1D418, 'M', 'y'),
- (0x1D419, 'M', 'z'),
- (0x1D41A, 'M', 'a'),
- (0x1D41B, 'M', 'b'),
- (0x1D41C, 'M', 'c'),
- (0x1D41D, 'M', 'd'),
- (0x1D41E, 'M', 'e'),
- (0x1D41F, 'M', 'f'),
- (0x1D420, 'M', 'g'),
- (0x1D421, 'M', 'h'),
- (0x1D422, 'M', 'i'),
- (0x1D423, 'M', 'j'),
- (0x1D424, 'M', 'k'),
- (0x1D425, 'M', 'l'),
- (0x1D426, 'M', 'm'),
- (0x1D427, 'M', 'n'),
- (0x1D428, 'M', 'o'),
- (0x1D429, 'M', 'p'),
- (0x1D42A, 'M', 'q'),
- (0x1D42B, 'M', 'r'),
- (0x1D42C, 'M', 's'),
- (0x1D42D, 'M', 't'),
- (0x1D42E, 'M', 'u'),
- (0x1D42F, 'M', 'v'),
- (0x1D430, 'M', 'w'),
- ]
-
-def _seg_61() -> List[Union[Tuple[int, str], Tuple[int, str, str]]]:
- return [
- (0x1D431, 'M', 'x'),
- (0x1D432, 'M', 'y'),
- (0x1D433, 'M', 'z'),
- (0x1D434, 'M', 'a'),
- (0x1D435, 'M', 'b'),
- (0x1D436, 'M', 'c'),
- (0x1D437, 'M', 'd'),
- (0x1D438, 'M', 'e'),
- (0x1D439, 'M', 'f'),
- (0x1D43A, 'M', 'g'),
- (0x1D43B, 'M', 'h'),
- (0x1D43C, 'M', 'i'),
- (0x1D43D, 'M', 'j'),
- (0x1D43E, 'M', 'k'),
- (0x1D43F, 'M', 'l'),
- (0x1D440, 'M', 'm'),
- (0x1D441, 'M', 'n'),
- (0x1D442, 'M', 'o'),
- (0x1D443, 'M', 'p'),
- (0x1D444, 'M', 'q'),
- (0x1D445, 'M', 'r'),
- (0x1D446, 'M', 's'),
- (0x1D447, 'M', 't'),
- (0x1D448, 'M', 'u'),
- (0x1D449, 'M', 'v'),
- (0x1D44A, 'M', 'w'),
- (0x1D44B, 'M', 'x'),
- (0x1D44C, 'M', 'y'),
- (0x1D44D, 'M', 'z'),
- (0x1D44E, 'M', 'a'),
- (0x1D44F, 'M', 'b'),
- (0x1D450, 'M', 'c'),
- (0x1D451, 'M', 'd'),
- (0x1D452, 'M', 'e'),
- (0x1D453, 'M', 'f'),
- (0x1D454, 'M', 'g'),
- (0x1D455, 'X'),
- (0x1D456, 'M', 'i'),
- (0x1D457, 'M', 'j'),
- (0x1D458, 'M', 'k'),
- (0x1D459, 'M', 'l'),
- (0x1D45A, 'M', 'm'),
- (0x1D45B, 'M', 'n'),
- (0x1D45C, 'M', 'o'),
- (0x1D45D, 'M', 'p'),
- (0x1D45E, 'M', 'q'),
- (0x1D45F, 'M', 'r'),
- (0x1D460, 'M', 's'),
- (0x1D461, 'M', 't'),
- (0x1D462, 'M', 'u'),
- (0x1D463, 'M', 'v'),
- (0x1D464, 'M', 'w'),
- (0x1D465, 'M', 'x'),
- (0x1D466, 'M', 'y'),
- (0x1D467, 'M', 'z'),
- (0x1D468, 'M', 'a'),
- (0x1D469, 'M', 'b'),
- (0x1D46A, 'M', 'c'),
- (0x1D46B, 'M', 'd'),
- (0x1D46C, 'M', 'e'),
- (0x1D46D, 'M', 'f'),
- (0x1D46E, 'M', 'g'),
- (0x1D46F, 'M', 'h'),
- (0x1D470, 'M', 'i'),
- (0x1D471, 'M', 'j'),
- (0x1D472, 'M', 'k'),
- (0x1D473, 'M', 'l'),
- (0x1D474, 'M', 'm'),
- (0x1D475, 'M', 'n'),
- (0x1D476, 'M', 'o'),
- (0x1D477, 'M', 'p'),
- (0x1D478, 'M', 'q'),
- (0x1D479, 'M', 'r'),
- (0x1D47A, 'M', 's'),
- (0x1D47B, 'M', 't'),
- (0x1D47C, 'M', 'u'),
- (0x1D47D, 'M', 'v'),
- (0x1D47E, 'M', 'w'),
- (0x1D47F, 'M', 'x'),
- (0x1D480, 'M', 'y'),
- (0x1D481, 'M', 'z'),
- (0x1D482, 'M', 'a'),
- (0x1D483, 'M', 'b'),
- (0x1D484, 'M', 'c'),
- (0x1D485, 'M', 'd'),
- (0x1D486, 'M', 'e'),
- (0x1D487, 'M', 'f'),
- (0x1D488, 'M', 'g'),
- (0x1D489, 'M', 'h'),
- (0x1D48A, 'M', 'i'),
- (0x1D48B, 'M', 'j'),
- (0x1D48C, 'M', 'k'),
- (0x1D48D, 'M', 'l'),
- (0x1D48E, 'M', 'm'),
- (0x1D48F, 'M', 'n'),
- (0x1D490, 'M', 'o'),
- (0x1D491, 'M', 'p'),
- (0x1D492, 'M', 'q'),
- (0x1D493, 'M', 'r'),
- (0x1D494, 'M', 's'),
- ]
-
-def _seg_62() -> List[Union[Tuple[int, str], Tuple[int, str, str]]]:
- return [
- (0x1D495, 'M', 't'),
- (0x1D496, 'M', 'u'),
- (0x1D497, 'M', 'v'),
- (0x1D498, 'M', 'w'),
- (0x1D499, 'M', 'x'),
- (0x1D49A, 'M', 'y'),
- (0x1D49B, 'M', 'z'),
- (0x1D49C, 'M', 'a'),
- (0x1D49D, 'X'),
- (0x1D49E, 'M', 'c'),
- (0x1D49F, 'M', 'd'),
- (0x1D4A0, 'X'),
- (0x1D4A2, 'M', 'g'),
- (0x1D4A3, 'X'),
- (0x1D4A5, 'M', 'j'),
- (0x1D4A6, 'M', 'k'),
- (0x1D4A7, 'X'),
- (0x1D4A9, 'M', 'n'),
- (0x1D4AA, 'M', 'o'),
- (0x1D4AB, 'M', 'p'),
- (0x1D4AC, 'M', 'q'),
- (0x1D4AD, 'X'),
- (0x1D4AE, 'M', 's'),
- (0x1D4AF, 'M', 't'),
- (0x1D4B0, 'M', 'u'),
- (0x1D4B1, 'M', 'v'),
- (0x1D4B2, 'M', 'w'),
- (0x1D4B3, 'M', 'x'),
- (0x1D4B4, 'M', 'y'),
- (0x1D4B5, 'M', 'z'),
- (0x1D4B6, 'M', 'a'),
- (0x1D4B7, 'M', 'b'),
- (0x1D4B8, 'M', 'c'),
- (0x1D4B9, 'M', 'd'),
- (0x1D4BA, 'X'),
- (0x1D4BB, 'M', 'f'),
- (0x1D4BC, 'X'),
- (0x1D4BD, 'M', 'h'),
- (0x1D4BE, 'M', 'i'),
- (0x1D4BF, 'M', 'j'),
- (0x1D4C0, 'M', 'k'),
- (0x1D4C1, 'M', 'l'),
- (0x1D4C2, 'M', 'm'),
- (0x1D4C3, 'M', 'n'),
- (0x1D4C4, 'X'),
- (0x1D4C5, 'M', 'p'),
- (0x1D4C6, 'M', 'q'),
- (0x1D4C7, 'M', 'r'),
- (0x1D4C8, 'M', 's'),
- (0x1D4C9, 'M', 't'),
- (0x1D4CA, 'M', 'u'),
- (0x1D4CB, 'M', 'v'),
- (0x1D4CC, 'M', 'w'),
- (0x1D4CD, 'M', 'x'),
- (0x1D4CE, 'M', 'y'),
- (0x1D4CF, 'M', 'z'),
- (0x1D4D0, 'M', 'a'),
- (0x1D4D1, 'M', 'b'),
- (0x1D4D2, 'M', 'c'),
- (0x1D4D3, 'M', 'd'),
- (0x1D4D4, 'M', 'e'),
- (0x1D4D5, 'M', 'f'),
- (0x1D4D6, 'M', 'g'),
- (0x1D4D7, 'M', 'h'),
- (0x1D4D8, 'M', 'i'),
- (0x1D4D9, 'M', 'j'),
- (0x1D4DA, 'M', 'k'),
- (0x1D4DB, 'M', 'l'),
- (0x1D4DC, 'M', 'm'),
- (0x1D4DD, 'M', 'n'),
- (0x1D4DE, 'M', 'o'),
- (0x1D4DF, 'M', 'p'),
- (0x1D4E0, 'M', 'q'),
- (0x1D4E1, 'M', 'r'),
- (0x1D4E2, 'M', 's'),
- (0x1D4E3, 'M', 't'),
- (0x1D4E4, 'M', 'u'),
- (0x1D4E5, 'M', 'v'),
- (0x1D4E6, 'M', 'w'),
- (0x1D4E7, 'M', 'x'),
- (0x1D4E8, 'M', 'y'),
- (0x1D4E9, 'M', 'z'),
- (0x1D4EA, 'M', 'a'),
- (0x1D4EB, 'M', 'b'),
- (0x1D4EC, 'M', 'c'),
- (0x1D4ED, 'M', 'd'),
- (0x1D4EE, 'M', 'e'),
- (0x1D4EF, 'M', 'f'),
- (0x1D4F0, 'M', 'g'),
- (0x1D4F1, 'M', 'h'),
- (0x1D4F2, 'M', 'i'),
- (0x1D4F3, 'M', 'j'),
- (0x1D4F4, 'M', 'k'),
- (0x1D4F5, 'M', 'l'),
- (0x1D4F6, 'M', 'm'),
- (0x1D4F7, 'M', 'n'),
- (0x1D4F8, 'M', 'o'),
- (0x1D4F9, 'M', 'p'),
- (0x1D4FA, 'M', 'q'),
- (0x1D4FB, 'M', 'r'),
- ]
-
-def _seg_63() -> List[Union[Tuple[int, str], Tuple[int, str, str]]]:
- return [
- (0x1D4FC, 'M', 's'),
- (0x1D4FD, 'M', 't'),
- (0x1D4FE, 'M', 'u'),
- (0x1D4FF, 'M', 'v'),
- (0x1D500, 'M', 'w'),
- (0x1D501, 'M', 'x'),
- (0x1D502, 'M', 'y'),
- (0x1D503, 'M', 'z'),
- (0x1D504, 'M', 'a'),
- (0x1D505, 'M', 'b'),
- (0x1D506, 'X'),
- (0x1D507, 'M', 'd'),
- (0x1D508, 'M', 'e'),
- (0x1D509, 'M', 'f'),
- (0x1D50A, 'M', 'g'),
- (0x1D50B, 'X'),
- (0x1D50D, 'M', 'j'),
- (0x1D50E, 'M', 'k'),
- (0x1D50F, 'M', 'l'),
- (0x1D510, 'M', 'm'),
- (0x1D511, 'M', 'n'),
- (0x1D512, 'M', 'o'),
- (0x1D513, 'M', 'p'),
- (0x1D514, 'M', 'q'),
- (0x1D515, 'X'),
- (0x1D516, 'M', 's'),
- (0x1D517, 'M', 't'),
- (0x1D518, 'M', 'u'),
- (0x1D519, 'M', 'v'),
- (0x1D51A, 'M', 'w'),
- (0x1D51B, 'M', 'x'),
- (0x1D51C, 'M', 'y'),
- (0x1D51D, 'X'),
- (0x1D51E, 'M', 'a'),
- (0x1D51F, 'M', 'b'),
- (0x1D520, 'M', 'c'),
- (0x1D521, 'M', 'd'),
- (0x1D522, 'M', 'e'),
- (0x1D523, 'M', 'f'),
- (0x1D524, 'M', 'g'),
- (0x1D525, 'M', 'h'),
- (0x1D526, 'M', 'i'),
- (0x1D527, 'M', 'j'),
- (0x1D528, 'M', 'k'),
- (0x1D529, 'M', 'l'),
- (0x1D52A, 'M', 'm'),
- (0x1D52B, 'M', 'n'),
- (0x1D52C, 'M', 'o'),
- (0x1D52D, 'M', 'p'),
- (0x1D52E, 'M', 'q'),
- (0x1D52F, 'M', 'r'),
- (0x1D530, 'M', 's'),
- (0x1D531, 'M', 't'),
- (0x1D532, 'M', 'u'),
- (0x1D533, 'M', 'v'),
- (0x1D534, 'M', 'w'),
- (0x1D535, 'M', 'x'),
- (0x1D536, 'M', 'y'),
- (0x1D537, 'M', 'z'),
- (0x1D538, 'M', 'a'),
- (0x1D539, 'M', 'b'),
- (0x1D53A, 'X'),
- (0x1D53B, 'M', 'd'),
- (0x1D53C, 'M', 'e'),
- (0x1D53D, 'M', 'f'),
- (0x1D53E, 'M', 'g'),
- (0x1D53F, 'X'),
- (0x1D540, 'M', 'i'),
- (0x1D541, 'M', 'j'),
- (0x1D542, 'M', 'k'),
- (0x1D543, 'M', 'l'),
- (0x1D544, 'M', 'm'),
- (0x1D545, 'X'),
- (0x1D546, 'M', 'o'),
- (0x1D547, 'X'),
- (0x1D54A, 'M', 's'),
- (0x1D54B, 'M', 't'),
- (0x1D54C, 'M', 'u'),
- (0x1D54D, 'M', 'v'),
- (0x1D54E, 'M', 'w'),
- (0x1D54F, 'M', 'x'),
- (0x1D550, 'M', 'y'),
- (0x1D551, 'X'),
- (0x1D552, 'M', 'a'),
- (0x1D553, 'M', 'b'),
- (0x1D554, 'M', 'c'),
- (0x1D555, 'M', 'd'),
- (0x1D556, 'M', 'e'),
- (0x1D557, 'M', 'f'),
- (0x1D558, 'M', 'g'),
- (0x1D559, 'M', 'h'),
- (0x1D55A, 'M', 'i'),
- (0x1D55B, 'M', 'j'),
- (0x1D55C, 'M', 'k'),
- (0x1D55D, 'M', 'l'),
- (0x1D55E, 'M', 'm'),
- (0x1D55F, 'M', 'n'),
- (0x1D560, 'M', 'o'),
- (0x1D561, 'M', 'p'),
- (0x1D562, 'M', 'q'),
- ]
-
-def _seg_64() -> List[Union[Tuple[int, str], Tuple[int, str, str]]]:
- return [
- (0x1D563, 'M', 'r'),
- (0x1D564, 'M', 's'),
- (0x1D565, 'M', 't'),
- (0x1D566, 'M', 'u'),
- (0x1D567, 'M', 'v'),
- (0x1D568, 'M', 'w'),
- (0x1D569, 'M', 'x'),
- (0x1D56A, 'M', 'y'),
- (0x1D56B, 'M', 'z'),
- (0x1D56C, 'M', 'a'),
- (0x1D56D, 'M', 'b'),
- (0x1D56E, 'M', 'c'),
- (0x1D56F, 'M', 'd'),
- (0x1D570, 'M', 'e'),
- (0x1D571, 'M', 'f'),
- (0x1D572, 'M', 'g'),
- (0x1D573, 'M', 'h'),
- (0x1D574, 'M', 'i'),
- (0x1D575, 'M', 'j'),
- (0x1D576, 'M', 'k'),
- (0x1D577, 'M', 'l'),
- (0x1D578, 'M', 'm'),
- (0x1D579, 'M', 'n'),
- (0x1D57A, 'M', 'o'),
- (0x1D57B, 'M', 'p'),
- (0x1D57C, 'M', 'q'),
- (0x1D57D, 'M', 'r'),
- (0x1D57E, 'M', 's'),
- (0x1D57F, 'M', 't'),
- (0x1D580, 'M', 'u'),
- (0x1D581, 'M', 'v'),
- (0x1D582, 'M', 'w'),
- (0x1D583, 'M', 'x'),
- (0x1D584, 'M', 'y'),
- (0x1D585, 'M', 'z'),
- (0x1D586, 'M', 'a'),
- (0x1D587, 'M', 'b'),
- (0x1D588, 'M', 'c'),
- (0x1D589, 'M', 'd'),
- (0x1D58A, 'M', 'e'),
- (0x1D58B, 'M', 'f'),
- (0x1D58C, 'M', 'g'),
- (0x1D58D, 'M', 'h'),
- (0x1D58E, 'M', 'i'),
- (0x1D58F, 'M', 'j'),
- (0x1D590, 'M', 'k'),
- (0x1D591, 'M', 'l'),
- (0x1D592, 'M', 'm'),
- (0x1D593, 'M', 'n'),
- (0x1D594, 'M', 'o'),
- (0x1D595, 'M', 'p'),
- (0x1D596, 'M', 'q'),
- (0x1D597, 'M', 'r'),
- (0x1D598, 'M', 's'),
- (0x1D599, 'M', 't'),
- (0x1D59A, 'M', 'u'),
- (0x1D59B, 'M', 'v'),
- (0x1D59C, 'M', 'w'),
- (0x1D59D, 'M', 'x'),
- (0x1D59E, 'M', 'y'),
- (0x1D59F, 'M', 'z'),
- (0x1D5A0, 'M', 'a'),
- (0x1D5A1, 'M', 'b'),
- (0x1D5A2, 'M', 'c'),
- (0x1D5A3, 'M', 'd'),
- (0x1D5A4, 'M', 'e'),
- (0x1D5A5, 'M', 'f'),
- (0x1D5A6, 'M', 'g'),
- (0x1D5A7, 'M', 'h'),
- (0x1D5A8, 'M', 'i'),
- (0x1D5A9, 'M', 'j'),
- (0x1D5AA, 'M', 'k'),
- (0x1D5AB, 'M', 'l'),
- (0x1D5AC, 'M', 'm'),
- (0x1D5AD, 'M', 'n'),
- (0x1D5AE, 'M', 'o'),
- (0x1D5AF, 'M', 'p'),
- (0x1D5B0, 'M', 'q'),
- (0x1D5B1, 'M', 'r'),
- (0x1D5B2, 'M', 's'),
- (0x1D5B3, 'M', 't'),
- (0x1D5B4, 'M', 'u'),
- (0x1D5B5, 'M', 'v'),
- (0x1D5B6, 'M', 'w'),
- (0x1D5B7, 'M', 'x'),
- (0x1D5B8, 'M', 'y'),
- (0x1D5B9, 'M', 'z'),
- (0x1D5BA, 'M', 'a'),
- (0x1D5BB, 'M', 'b'),
- (0x1D5BC, 'M', 'c'),
- (0x1D5BD, 'M', 'd'),
- (0x1D5BE, 'M', 'e'),
- (0x1D5BF, 'M', 'f'),
- (0x1D5C0, 'M', 'g'),
- (0x1D5C1, 'M', 'h'),
- (0x1D5C2, 'M', 'i'),
- (0x1D5C3, 'M', 'j'),
- (0x1D5C4, 'M', 'k'),
- (0x1D5C5, 'M', 'l'),
- (0x1D5C6, 'M', 'm'),
- ]
-
-def _seg_65() -> List[Union[Tuple[int, str], Tuple[int, str, str]]]:
- return [
- (0x1D5C7, 'M', 'n'),
- (0x1D5C8, 'M', 'o'),
- (0x1D5C9, 'M', 'p'),
- (0x1D5CA, 'M', 'q'),
- (0x1D5CB, 'M', 'r'),
- (0x1D5CC, 'M', 's'),
- (0x1D5CD, 'M', 't'),
- (0x1D5CE, 'M', 'u'),
- (0x1D5CF, 'M', 'v'),
- (0x1D5D0, 'M', 'w'),
- (0x1D5D1, 'M', 'x'),
- (0x1D5D2, 'M', 'y'),
- (0x1D5D3, 'M', 'z'),
- (0x1D5D4, 'M', 'a'),
- (0x1D5D5, 'M', 'b'),
- (0x1D5D6, 'M', 'c'),
- (0x1D5D7, 'M', 'd'),
- (0x1D5D8, 'M', 'e'),
- (0x1D5D9, 'M', 'f'),
- (0x1D5DA, 'M', 'g'),
- (0x1D5DB, 'M', 'h'),
- (0x1D5DC, 'M', 'i'),
- (0x1D5DD, 'M', 'j'),
- (0x1D5DE, 'M', 'k'),
- (0x1D5DF, 'M', 'l'),
- (0x1D5E0, 'M', 'm'),
- (0x1D5E1, 'M', 'n'),
- (0x1D5E2, 'M', 'o'),
- (0x1D5E3, 'M', 'p'),
- (0x1D5E4, 'M', 'q'),
- (0x1D5E5, 'M', 'r'),
- (0x1D5E6, 'M', 's'),
- (0x1D5E7, 'M', 't'),
- (0x1D5E8, 'M', 'u'),
- (0x1D5E9, 'M', 'v'),
- (0x1D5EA, 'M', 'w'),
- (0x1D5EB, 'M', 'x'),
- (0x1D5EC, 'M', 'y'),
- (0x1D5ED, 'M', 'z'),
- (0x1D5EE, 'M', 'a'),
- (0x1D5EF, 'M', 'b'),
- (0x1D5F0, 'M', 'c'),
- (0x1D5F1, 'M', 'd'),
- (0x1D5F2, 'M', 'e'),
- (0x1D5F3, 'M', 'f'),
- (0x1D5F4, 'M', 'g'),
- (0x1D5F5, 'M', 'h'),
- (0x1D5F6, 'M', 'i'),
- (0x1D5F7, 'M', 'j'),
- (0x1D5F8, 'M', 'k'),
- (0x1D5F9, 'M', 'l'),
- (0x1D5FA, 'M', 'm'),
- (0x1D5FB, 'M', 'n'),
- (0x1D5FC, 'M', 'o'),
- (0x1D5FD, 'M', 'p'),
- (0x1D5FE, 'M', 'q'),
- (0x1D5FF, 'M', 'r'),
- (0x1D600, 'M', 's'),
- (0x1D601, 'M', 't'),
- (0x1D602, 'M', 'u'),
- (0x1D603, 'M', 'v'),
- (0x1D604, 'M', 'w'),
- (0x1D605, 'M', 'x'),
- (0x1D606, 'M', 'y'),
- (0x1D607, 'M', 'z'),
- (0x1D608, 'M', 'a'),
- (0x1D609, 'M', 'b'),
- (0x1D60A, 'M', 'c'),
- (0x1D60B, 'M', 'd'),
- (0x1D60C, 'M', 'e'),
- (0x1D60D, 'M', 'f'),
- (0x1D60E, 'M', 'g'),
- (0x1D60F, 'M', 'h'),
- (0x1D610, 'M', 'i'),
- (0x1D611, 'M', 'j'),
- (0x1D612, 'M', 'k'),
- (0x1D613, 'M', 'l'),
- (0x1D614, 'M', 'm'),
- (0x1D615, 'M', 'n'),
- (0x1D616, 'M', 'o'),
- (0x1D617, 'M', 'p'),
- (0x1D618, 'M', 'q'),
- (0x1D619, 'M', 'r'),
- (0x1D61A, 'M', 's'),
- (0x1D61B, 'M', 't'),
- (0x1D61C, 'M', 'u'),
- (0x1D61D, 'M', 'v'),
- (0x1D61E, 'M', 'w'),
- (0x1D61F, 'M', 'x'),
- (0x1D620, 'M', 'y'),
- (0x1D621, 'M', 'z'),
- (0x1D622, 'M', 'a'),
- (0x1D623, 'M', 'b'),
- (0x1D624, 'M', 'c'),
- (0x1D625, 'M', 'd'),
- (0x1D626, 'M', 'e'),
- (0x1D627, 'M', 'f'),
- (0x1D628, 'M', 'g'),
- (0x1D629, 'M', 'h'),
- (0x1D62A, 'M', 'i'),
- ]
-
-def _seg_66() -> List[Union[Tuple[int, str], Tuple[int, str, str]]]:
- return [
- (0x1D62B, 'M', 'j'),
- (0x1D62C, 'M', 'k'),
- (0x1D62D, 'M', 'l'),
- (0x1D62E, 'M', 'm'),
- (0x1D62F, 'M', 'n'),
- (0x1D630, 'M', 'o'),
- (0x1D631, 'M', 'p'),
- (0x1D632, 'M', 'q'),
- (0x1D633, 'M', 'r'),
- (0x1D634, 'M', 's'),
- (0x1D635, 'M', 't'),
- (0x1D636, 'M', 'u'),
- (0x1D637, 'M', 'v'),
- (0x1D638, 'M', 'w'),
- (0x1D639, 'M', 'x'),
- (0x1D63A, 'M', 'y'),
- (0x1D63B, 'M', 'z'),
- (0x1D63C, 'M', 'a'),
- (0x1D63D, 'M', 'b'),
- (0x1D63E, 'M', 'c'),
- (0x1D63F, 'M', 'd'),
- (0x1D640, 'M', 'e'),
- (0x1D641, 'M', 'f'),
- (0x1D642, 'M', 'g'),
- (0x1D643, 'M', 'h'),
- (0x1D644, 'M', 'i'),
- (0x1D645, 'M', 'j'),
- (0x1D646, 'M', 'k'),
- (0x1D647, 'M', 'l'),
- (0x1D648, 'M', 'm'),
- (0x1D649, 'M', 'n'),
- (0x1D64A, 'M', 'o'),
- (0x1D64B, 'M', 'p'),
- (0x1D64C, 'M', 'q'),
- (0x1D64D, 'M', 'r'),
- (0x1D64E, 'M', 's'),
- (0x1D64F, 'M', 't'),
- (0x1D650, 'M', 'u'),
- (0x1D651, 'M', 'v'),
- (0x1D652, 'M', 'w'),
- (0x1D653, 'M', 'x'),
- (0x1D654, 'M', 'y'),
- (0x1D655, 'M', 'z'),
- (0x1D656, 'M', 'a'),
- (0x1D657, 'M', 'b'),
- (0x1D658, 'M', 'c'),
- (0x1D659, 'M', 'd'),
- (0x1D65A, 'M', 'e'),
- (0x1D65B, 'M', 'f'),
- (0x1D65C, 'M', 'g'),
- (0x1D65D, 'M', 'h'),
- (0x1D65E, 'M', 'i'),
- (0x1D65F, 'M', 'j'),
- (0x1D660, 'M', 'k'),
- (0x1D661, 'M', 'l'),
- (0x1D662, 'M', 'm'),
- (0x1D663, 'M', 'n'),
- (0x1D664, 'M', 'o'),
- (0x1D665, 'M', 'p'),
- (0x1D666, 'M', 'q'),
- (0x1D667, 'M', 'r'),
- (0x1D668, 'M', 's'),
- (0x1D669, 'M', 't'),
- (0x1D66A, 'M', 'u'),
- (0x1D66B, 'M', 'v'),
- (0x1D66C, 'M', 'w'),
- (0x1D66D, 'M', 'x'),
- (0x1D66E, 'M', 'y'),
- (0x1D66F, 'M', 'z'),
- (0x1D670, 'M', 'a'),
- (0x1D671, 'M', 'b'),
- (0x1D672, 'M', 'c'),
- (0x1D673, 'M', 'd'),
- (0x1D674, 'M', 'e'),
- (0x1D675, 'M', 'f'),
- (0x1D676, 'M', 'g'),
- (0x1D677, 'M', 'h'),
- (0x1D678, 'M', 'i'),
- (0x1D679, 'M', 'j'),
- (0x1D67A, 'M', 'k'),
- (0x1D67B, 'M', 'l'),
- (0x1D67C, 'M', 'm'),
- (0x1D67D, 'M', 'n'),
- (0x1D67E, 'M', 'o'),
- (0x1D67F, 'M', 'p'),
- (0x1D680, 'M', 'q'),
- (0x1D681, 'M', 'r'),
- (0x1D682, 'M', 's'),
- (0x1D683, 'M', 't'),
- (0x1D684, 'M', 'u'),
- (0x1D685, 'M', 'v'),
- (0x1D686, 'M', 'w'),
- (0x1D687, 'M', 'x'),
- (0x1D688, 'M', 'y'),
- (0x1D689, 'M', 'z'),
- (0x1D68A, 'M', 'a'),
- (0x1D68B, 'M', 'b'),
- (0x1D68C, 'M', 'c'),
- (0x1D68D, 'M', 'd'),
- (0x1D68E, 'M', 'e'),
- ]
-
-def _seg_67() -> List[Union[Tuple[int, str], Tuple[int, str, str]]]:
- return [
- (0x1D68F, 'M', 'f'),
- (0x1D690, 'M', 'g'),
- (0x1D691, 'M', 'h'),
- (0x1D692, 'M', 'i'),
- (0x1D693, 'M', 'j'),
- (0x1D694, 'M', 'k'),
- (0x1D695, 'M', 'l'),
- (0x1D696, 'M', 'm'),
- (0x1D697, 'M', 'n'),
- (0x1D698, 'M', 'o'),
- (0x1D699, 'M', 'p'),
- (0x1D69A, 'M', 'q'),
- (0x1D69B, 'M', 'r'),
- (0x1D69C, 'M', 's'),
- (0x1D69D, 'M', 't'),
- (0x1D69E, 'M', 'u'),
- (0x1D69F, 'M', 'v'),
- (0x1D6A0, 'M', 'w'),
- (0x1D6A1, 'M', 'x'),
- (0x1D6A2, 'M', 'y'),
- (0x1D6A3, 'M', 'z'),
- (0x1D6A4, 'M', 'ı'),
- (0x1D6A5, 'M', 'ȷ'),
- (0x1D6A6, 'X'),
- (0x1D6A8, 'M', 'α'),
- (0x1D6A9, 'M', 'β'),
- (0x1D6AA, 'M', 'γ'),
- (0x1D6AB, 'M', 'δ'),
- (0x1D6AC, 'M', 'ε'),
- (0x1D6AD, 'M', 'ζ'),
- (0x1D6AE, 'M', 'η'),
- (0x1D6AF, 'M', 'θ'),
- (0x1D6B0, 'M', 'ι'),
- (0x1D6B1, 'M', 'κ'),
- (0x1D6B2, 'M', 'λ'),
- (0x1D6B3, 'M', 'μ'),
- (0x1D6B4, 'M', 'ν'),
- (0x1D6B5, 'M', 'ξ'),
- (0x1D6B6, 'M', 'ο'),
- (0x1D6B7, 'M', 'π'),
- (0x1D6B8, 'M', 'ρ'),
- (0x1D6B9, 'M', 'θ'),
- (0x1D6BA, 'M', 'σ'),
- (0x1D6BB, 'M', 'τ'),
- (0x1D6BC, 'M', 'υ'),
- (0x1D6BD, 'M', 'φ'),
- (0x1D6BE, 'M', 'χ'),
- (0x1D6BF, 'M', 'ψ'),
- (0x1D6C0, 'M', 'ω'),
- (0x1D6C1, 'M', '∇'),
- (0x1D6C2, 'M', 'α'),
- (0x1D6C3, 'M', 'β'),
- (0x1D6C4, 'M', 'γ'),
- (0x1D6C5, 'M', 'δ'),
- (0x1D6C6, 'M', 'ε'),
- (0x1D6C7, 'M', 'ζ'),
- (0x1D6C8, 'M', 'η'),
- (0x1D6C9, 'M', 'θ'),
- (0x1D6CA, 'M', 'ι'),
- (0x1D6CB, 'M', 'κ'),
- (0x1D6CC, 'M', 'λ'),
- (0x1D6CD, 'M', 'μ'),
- (0x1D6CE, 'M', 'ν'),
- (0x1D6CF, 'M', 'ξ'),
- (0x1D6D0, 'M', 'ο'),
- (0x1D6D1, 'M', 'π'),
- (0x1D6D2, 'M', 'ρ'),
- (0x1D6D3, 'M', 'σ'),
- (0x1D6D5, 'M', 'τ'),
- (0x1D6D6, 'M', 'υ'),
- (0x1D6D7, 'M', 'φ'),
- (0x1D6D8, 'M', 'χ'),
- (0x1D6D9, 'M', 'ψ'),
- (0x1D6DA, 'M', 'ω'),
- (0x1D6DB, 'M', '∂'),
- (0x1D6DC, 'M', 'ε'),
- (0x1D6DD, 'M', 'θ'),
- (0x1D6DE, 'M', 'κ'),
- (0x1D6DF, 'M', 'φ'),
- (0x1D6E0, 'M', 'ρ'),
- (0x1D6E1, 'M', 'π'),
- (0x1D6E2, 'M', 'α'),
- (0x1D6E3, 'M', 'β'),
- (0x1D6E4, 'M', 'γ'),
- (0x1D6E5, 'M', 'δ'),
- (0x1D6E6, 'M', 'ε'),
- (0x1D6E7, 'M', 'ζ'),
- (0x1D6E8, 'M', 'η'),
- (0x1D6E9, 'M', 'θ'),
- (0x1D6EA, 'M', 'ι'),
- (0x1D6EB, 'M', 'κ'),
- (0x1D6EC, 'M', 'λ'),
- (0x1D6ED, 'M', 'μ'),
- (0x1D6EE, 'M', 'ν'),
- (0x1D6EF, 'M', 'ξ'),
- (0x1D6F0, 'M', 'ο'),
- (0x1D6F1, 'M', 'π'),
- (0x1D6F2, 'M', 'ρ'),
- (0x1D6F3, 'M', 'θ'),
- (0x1D6F4, 'M', 'σ'),
- ]
-
-def _seg_68() -> List[Union[Tuple[int, str], Tuple[int, str, str]]]:
- return [
- (0x1D6F5, 'M', 'τ'),
- (0x1D6F6, 'M', 'υ'),
- (0x1D6F7, 'M', 'φ'),
- (0x1D6F8, 'M', 'χ'),
- (0x1D6F9, 'M', 'ψ'),
- (0x1D6FA, 'M', 'ω'),
- (0x1D6FB, 'M', '∇'),
- (0x1D6FC, 'M', 'α'),
- (0x1D6FD, 'M', 'β'),
- (0x1D6FE, 'M', 'γ'),
- (0x1D6FF, 'M', 'δ'),
- (0x1D700, 'M', 'ε'),
- (0x1D701, 'M', 'ζ'),
- (0x1D702, 'M', 'η'),
- (0x1D703, 'M', 'θ'),
- (0x1D704, 'M', 'ι'),
- (0x1D705, 'M', 'κ'),
- (0x1D706, 'M', 'λ'),
- (0x1D707, 'M', 'μ'),
- (0x1D708, 'M', 'ν'),
- (0x1D709, 'M', 'ξ'),
- (0x1D70A, 'M', 'ο'),
- (0x1D70B, 'M', 'π'),
- (0x1D70C, 'M', 'ρ'),
- (0x1D70D, 'M', 'σ'),
- (0x1D70F, 'M', 'τ'),
- (0x1D710, 'M', 'υ'),
- (0x1D711, 'M', 'φ'),
- (0x1D712, 'M', 'χ'),
- (0x1D713, 'M', 'ψ'),
- (0x1D714, 'M', 'ω'),
- (0x1D715, 'M', '∂'),
- (0x1D716, 'M', 'ε'),
- (0x1D717, 'M', 'θ'),
- (0x1D718, 'M', 'κ'),
- (0x1D719, 'M', 'φ'),
- (0x1D71A, 'M', 'ρ'),
- (0x1D71B, 'M', 'π'),
- (0x1D71C, 'M', 'α'),
- (0x1D71D, 'M', 'β'),
- (0x1D71E, 'M', 'γ'),
- (0x1D71F, 'M', 'δ'),
- (0x1D720, 'M', 'ε'),
- (0x1D721, 'M', 'ζ'),
- (0x1D722, 'M', 'η'),
- (0x1D723, 'M', 'θ'),
- (0x1D724, 'M', 'ι'),
- (0x1D725, 'M', 'κ'),
- (0x1D726, 'M', 'λ'),
- (0x1D727, 'M', 'μ'),
- (0x1D728, 'M', 'ν'),
- (0x1D729, 'M', 'ξ'),
- (0x1D72A, 'M', 'ο'),
- (0x1D72B, 'M', 'π'),
- (0x1D72C, 'M', 'ρ'),
- (0x1D72D, 'M', 'θ'),
- (0x1D72E, 'M', 'σ'),
- (0x1D72F, 'M', 'τ'),
- (0x1D730, 'M', 'υ'),
- (0x1D731, 'M', 'φ'),
- (0x1D732, 'M', 'χ'),
- (0x1D733, 'M', 'ψ'),
- (0x1D734, 'M', 'ω'),
- (0x1D735, 'M', '∇'),
- (0x1D736, 'M', 'α'),
- (0x1D737, 'M', 'β'),
- (0x1D738, 'M', 'γ'),
- (0x1D739, 'M', 'δ'),
- (0x1D73A, 'M', 'ε'),
- (0x1D73B, 'M', 'ζ'),
- (0x1D73C, 'M', 'η'),
- (0x1D73D, 'M', 'θ'),
- (0x1D73E, 'M', 'ι'),
- (0x1D73F, 'M', 'κ'),
- (0x1D740, 'M', 'λ'),
- (0x1D741, 'M', 'μ'),
- (0x1D742, 'M', 'ν'),
- (0x1D743, 'M', 'ξ'),
- (0x1D744, 'M', 'ο'),
- (0x1D745, 'M', 'π'),
- (0x1D746, 'M', 'ρ'),
- (0x1D747, 'M', 'σ'),
- (0x1D749, 'M', 'τ'),
- (0x1D74A, 'M', 'υ'),
- (0x1D74B, 'M', 'φ'),
- (0x1D74C, 'M', 'χ'),
- (0x1D74D, 'M', 'ψ'),
- (0x1D74E, 'M', 'ω'),
- (0x1D74F, 'M', '∂'),
- (0x1D750, 'M', 'ε'),
- (0x1D751, 'M', 'θ'),
- (0x1D752, 'M', 'κ'),
- (0x1D753, 'M', 'φ'),
- (0x1D754, 'M', 'ρ'),
- (0x1D755, 'M', 'π'),
- (0x1D756, 'M', 'α'),
- (0x1D757, 'M', 'β'),
- (0x1D758, 'M', 'γ'),
- (0x1D759, 'M', 'δ'),
- (0x1D75A, 'M', 'ε'),
- ]
-
-def _seg_69() -> List[Union[Tuple[int, str], Tuple[int, str, str]]]:
- return [
- (0x1D75B, 'M', 'ζ'),
- (0x1D75C, 'M', 'η'),
- (0x1D75D, 'M', 'θ'),
- (0x1D75E, 'M', 'ι'),
- (0x1D75F, 'M', 'κ'),
- (0x1D760, 'M', 'λ'),
- (0x1D761, 'M', 'μ'),
- (0x1D762, 'M', 'ν'),
- (0x1D763, 'M', 'ξ'),
- (0x1D764, 'M', 'ο'),
- (0x1D765, 'M', 'π'),
- (0x1D766, 'M', 'ρ'),
- (0x1D767, 'M', 'θ'),
- (0x1D768, 'M', 'σ'),
- (0x1D769, 'M', 'τ'),
- (0x1D76A, 'M', 'υ'),
- (0x1D76B, 'M', 'φ'),
- (0x1D76C, 'M', 'χ'),
- (0x1D76D, 'M', 'ψ'),
- (0x1D76E, 'M', 'ω'),
- (0x1D76F, 'M', '∇'),
- (0x1D770, 'M', 'α'),
- (0x1D771, 'M', 'β'),
- (0x1D772, 'M', 'γ'),
- (0x1D773, 'M', 'δ'),
- (0x1D774, 'M', 'ε'),
- (0x1D775, 'M', 'ζ'),
- (0x1D776, 'M', 'η'),
- (0x1D777, 'M', 'θ'),
- (0x1D778, 'M', 'ι'),
- (0x1D779, 'M', 'κ'),
- (0x1D77A, 'M', 'λ'),
- (0x1D77B, 'M', 'μ'),
- (0x1D77C, 'M', 'ν'),
- (0x1D77D, 'M', 'ξ'),
- (0x1D77E, 'M', 'ο'),
- (0x1D77F, 'M', 'π'),
- (0x1D780, 'M', 'ρ'),
- (0x1D781, 'M', 'σ'),
- (0x1D783, 'M', 'τ'),
- (0x1D784, 'M', 'υ'),
- (0x1D785, 'M', 'φ'),
- (0x1D786, 'M', 'χ'),
- (0x1D787, 'M', 'ψ'),
- (0x1D788, 'M', 'ω'),
- (0x1D789, 'M', '∂'),
- (0x1D78A, 'M', 'ε'),
- (0x1D78B, 'M', 'θ'),
- (0x1D78C, 'M', 'κ'),
- (0x1D78D, 'M', 'φ'),
- (0x1D78E, 'M', 'ρ'),
- (0x1D78F, 'M', 'π'),
- (0x1D790, 'M', 'α'),
- (0x1D791, 'M', 'β'),
- (0x1D792, 'M', 'γ'),
- (0x1D793, 'M', 'δ'),
- (0x1D794, 'M', 'ε'),
- (0x1D795, 'M', 'ζ'),
- (0x1D796, 'M', 'η'),
- (0x1D797, 'M', 'θ'),
- (0x1D798, 'M', 'ι'),
- (0x1D799, 'M', 'κ'),
- (0x1D79A, 'M', 'λ'),
- (0x1D79B, 'M', 'μ'),
- (0x1D79C, 'M', 'ν'),
- (0x1D79D, 'M', 'ξ'),
- (0x1D79E, 'M', 'ο'),
- (0x1D79F, 'M', 'π'),
- (0x1D7A0, 'M', 'ρ'),
- (0x1D7A1, 'M', 'θ'),
- (0x1D7A2, 'M', 'σ'),
- (0x1D7A3, 'M', 'τ'),
- (0x1D7A4, 'M', 'υ'),
- (0x1D7A5, 'M', 'φ'),
- (0x1D7A6, 'M', 'χ'),
- (0x1D7A7, 'M', 'ψ'),
- (0x1D7A8, 'M', 'ω'),
- (0x1D7A9, 'M', '∇'),
- (0x1D7AA, 'M', 'α'),
- (0x1D7AB, 'M', 'β'),
- (0x1D7AC, 'M', 'γ'),
- (0x1D7AD, 'M', 'δ'),
- (0x1D7AE, 'M', 'ε'),
- (0x1D7AF, 'M', 'ζ'),
- (0x1D7B0, 'M', 'η'),
- (0x1D7B1, 'M', 'θ'),
- (0x1D7B2, 'M', 'ι'),
- (0x1D7B3, 'M', 'κ'),
- (0x1D7B4, 'M', 'λ'),
- (0x1D7B5, 'M', 'μ'),
- (0x1D7B6, 'M', 'ν'),
- (0x1D7B7, 'M', 'ξ'),
- (0x1D7B8, 'M', 'ο'),
- (0x1D7B9, 'M', 'π'),
- (0x1D7BA, 'M', 'ρ'),
- (0x1D7BB, 'M', 'σ'),
- (0x1D7BD, 'M', 'τ'),
- (0x1D7BE, 'M', 'υ'),
- (0x1D7BF, 'M', 'φ'),
- (0x1D7C0, 'M', 'χ'),
- ]
-
-def _seg_70() -> List[Union[Tuple[int, str], Tuple[int, str, str]]]:
- return [
- (0x1D7C1, 'M', 'ψ'),
- (0x1D7C2, 'M', 'ω'),
- (0x1D7C3, 'M', '∂'),
- (0x1D7C4, 'M', 'ε'),
- (0x1D7C5, 'M', 'θ'),
- (0x1D7C6, 'M', 'κ'),
- (0x1D7C7, 'M', 'φ'),
- (0x1D7C8, 'M', 'ρ'),
- (0x1D7C9, 'M', 'π'),
- (0x1D7CA, 'M', 'ϝ'),
- (0x1D7CC, 'X'),
- (0x1D7CE, 'M', '0'),
- (0x1D7CF, 'M', '1'),
- (0x1D7D0, 'M', '2'),
- (0x1D7D1, 'M', '3'),
- (0x1D7D2, 'M', '4'),
- (0x1D7D3, 'M', '5'),
- (0x1D7D4, 'M', '6'),
- (0x1D7D5, 'M', '7'),
- (0x1D7D6, 'M', '8'),
- (0x1D7D7, 'M', '9'),
- (0x1D7D8, 'M', '0'),
- (0x1D7D9, 'M', '1'),
- (0x1D7DA, 'M', '2'),
- (0x1D7DB, 'M', '3'),
- (0x1D7DC, 'M', '4'),
- (0x1D7DD, 'M', '5'),
- (0x1D7DE, 'M', '6'),
- (0x1D7DF, 'M', '7'),
- (0x1D7E0, 'M', '8'),
- (0x1D7E1, 'M', '9'),
- (0x1D7E2, 'M', '0'),
- (0x1D7E3, 'M', '1'),
- (0x1D7E4, 'M', '2'),
- (0x1D7E5, 'M', '3'),
- (0x1D7E6, 'M', '4'),
- (0x1D7E7, 'M', '5'),
- (0x1D7E8, 'M', '6'),
- (0x1D7E9, 'M', '7'),
- (0x1D7EA, 'M', '8'),
- (0x1D7EB, 'M', '9'),
- (0x1D7EC, 'M', '0'),
- (0x1D7ED, 'M', '1'),
- (0x1D7EE, 'M', '2'),
- (0x1D7EF, 'M', '3'),
- (0x1D7F0, 'M', '4'),
- (0x1D7F1, 'M', '5'),
- (0x1D7F2, 'M', '6'),
- (0x1D7F3, 'M', '7'),
- (0x1D7F4, 'M', '8'),
- (0x1D7F5, 'M', '9'),
- (0x1D7F6, 'M', '0'),
- (0x1D7F7, 'M', '1'),
- (0x1D7F8, 'M', '2'),
- (0x1D7F9, 'M', '3'),
- (0x1D7FA, 'M', '4'),
- (0x1D7FB, 'M', '5'),
- (0x1D7FC, 'M', '6'),
- (0x1D7FD, 'M', '7'),
- (0x1D7FE, 'M', '8'),
- (0x1D7FF, 'M', '9'),
- (0x1D800, 'V'),
- (0x1DA8C, 'X'),
- (0x1DA9B, 'V'),
- (0x1DAA0, 'X'),
- (0x1DAA1, 'V'),
- (0x1DAB0, 'X'),
- (0x1DF00, 'V'),
- (0x1DF1F, 'X'),
- (0x1E000, 'V'),
- (0x1E007, 'X'),
- (0x1E008, 'V'),
- (0x1E019, 'X'),
- (0x1E01B, 'V'),
- (0x1E022, 'X'),
- (0x1E023, 'V'),
- (0x1E025, 'X'),
- (0x1E026, 'V'),
- (0x1E02B, 'X'),
- (0x1E100, 'V'),
- (0x1E12D, 'X'),
- (0x1E130, 'V'),
- (0x1E13E, 'X'),
- (0x1E140, 'V'),
- (0x1E14A, 'X'),
- (0x1E14E, 'V'),
- (0x1E150, 'X'),
- (0x1E290, 'V'),
- (0x1E2AF, 'X'),
- (0x1E2C0, 'V'),
- (0x1E2FA, 'X'),
- (0x1E2FF, 'V'),
- (0x1E300, 'X'),
- (0x1E7E0, 'V'),
- (0x1E7E7, 'X'),
- (0x1E7E8, 'V'),
- (0x1E7EC, 'X'),
- (0x1E7ED, 'V'),
- (0x1E7EF, 'X'),
- (0x1E7F0, 'V'),
- ]
-
-def _seg_71() -> List[Union[Tuple[int, str], Tuple[int, str, str]]]:
- return [
- (0x1E7FF, 'X'),
- (0x1E800, 'V'),
- (0x1E8C5, 'X'),
- (0x1E8C7, 'V'),
- (0x1E8D7, 'X'),
- (0x1E900, 'M', '𞤢'),
- (0x1E901, 'M', '𞤣'),
- (0x1E902, 'M', '𞤤'),
- (0x1E903, 'M', '𞤥'),
- (0x1E904, 'M', '𞤦'),
- (0x1E905, 'M', '𞤧'),
- (0x1E906, 'M', '𞤨'),
- (0x1E907, 'M', '𞤩'),
- (0x1E908, 'M', '𞤪'),
- (0x1E909, 'M', '𞤫'),
- (0x1E90A, 'M', '𞤬'),
- (0x1E90B, 'M', '𞤭'),
- (0x1E90C, 'M', '𞤮'),
- (0x1E90D, 'M', '𞤯'),
- (0x1E90E, 'M', '𞤰'),
- (0x1E90F, 'M', '𞤱'),
- (0x1E910, 'M', '𞤲'),
- (0x1E911, 'M', '𞤳'),
- (0x1E912, 'M', '𞤴'),
- (0x1E913, 'M', '𞤵'),
- (0x1E914, 'M', '𞤶'),
- (0x1E915, 'M', '𞤷'),
- (0x1E916, 'M', '𞤸'),
- (0x1E917, 'M', '𞤹'),
- (0x1E918, 'M', '𞤺'),
- (0x1E919, 'M', '𞤻'),
- (0x1E91A, 'M', '𞤼'),
- (0x1E91B, 'M', '𞤽'),
- (0x1E91C, 'M', '𞤾'),
- (0x1E91D, 'M', '𞤿'),
- (0x1E91E, 'M', '𞥀'),
- (0x1E91F, 'M', '𞥁'),
- (0x1E920, 'M', '𞥂'),
- (0x1E921, 'M', '𞥃'),
- (0x1E922, 'V'),
- (0x1E94C, 'X'),
- (0x1E950, 'V'),
- (0x1E95A, 'X'),
- (0x1E95E, 'V'),
- (0x1E960, 'X'),
- (0x1EC71, 'V'),
- (0x1ECB5, 'X'),
- (0x1ED01, 'V'),
- (0x1ED3E, 'X'),
- (0x1EE00, 'M', 'ا'),
- (0x1EE01, 'M', 'ب'),
- (0x1EE02, 'M', 'ج'),
- (0x1EE03, 'M', 'د'),
- (0x1EE04, 'X'),
- (0x1EE05, 'M', 'و'),
- (0x1EE06, 'M', 'ز'),
- (0x1EE07, 'M', 'ح'),
- (0x1EE08, 'M', 'ط'),
- (0x1EE09, 'M', 'ي'),
- (0x1EE0A, 'M', 'ك'),
- (0x1EE0B, 'M', 'ل'),
- (0x1EE0C, 'M', 'م'),
- (0x1EE0D, 'M', 'ن'),
- (0x1EE0E, 'M', 'س'),
- (0x1EE0F, 'M', 'ع'),
- (0x1EE10, 'M', 'ف'),
- (0x1EE11, 'M', 'ص'),
- (0x1EE12, 'M', 'ق'),
- (0x1EE13, 'M', 'ر'),
- (0x1EE14, 'M', 'ش'),
- (0x1EE15, 'M', 'ت'),
- (0x1EE16, 'M', 'ث'),
- (0x1EE17, 'M', 'خ'),
- (0x1EE18, 'M', 'ذ'),
- (0x1EE19, 'M', 'ض'),
- (0x1EE1A, 'M', 'ظ'),
- (0x1EE1B, 'M', 'غ'),
- (0x1EE1C, 'M', 'ٮ'),
- (0x1EE1D, 'M', 'ں'),
- (0x1EE1E, 'M', 'ڡ'),
- (0x1EE1F, 'M', 'ٯ'),
- (0x1EE20, 'X'),
- (0x1EE21, 'M', 'ب'),
- (0x1EE22, 'M', 'ج'),
- (0x1EE23, 'X'),
- (0x1EE24, 'M', 'ه'),
- (0x1EE25, 'X'),
- (0x1EE27, 'M', 'ح'),
- (0x1EE28, 'X'),
- (0x1EE29, 'M', 'ي'),
- (0x1EE2A, 'M', 'ك'),
- (0x1EE2B, 'M', 'ل'),
- (0x1EE2C, 'M', 'م'),
- (0x1EE2D, 'M', 'ن'),
- (0x1EE2E, 'M', 'س'),
- (0x1EE2F, 'M', 'ع'),
- (0x1EE30, 'M', 'ف'),
- (0x1EE31, 'M', 'ص'),
- (0x1EE32, 'M', 'ق'),
- (0x1EE33, 'X'),
- ]
-
-def _seg_72() -> List[Union[Tuple[int, str], Tuple[int, str, str]]]:
- return [
- (0x1EE34, 'M', 'ش'),
- (0x1EE35, 'M', 'ت'),
- (0x1EE36, 'M', 'ث'),
- (0x1EE37, 'M', 'خ'),
- (0x1EE38, 'X'),
- (0x1EE39, 'M', 'ض'),
- (0x1EE3A, 'X'),
- (0x1EE3B, 'M', 'غ'),
- (0x1EE3C, 'X'),
- (0x1EE42, 'M', 'ج'),
- (0x1EE43, 'X'),
- (0x1EE47, 'M', 'ح'),
- (0x1EE48, 'X'),
- (0x1EE49, 'M', 'ي'),
- (0x1EE4A, 'X'),
- (0x1EE4B, 'M', 'ل'),
- (0x1EE4C, 'X'),
- (0x1EE4D, 'M', 'ن'),
- (0x1EE4E, 'M', 'س'),
- (0x1EE4F, 'M', 'ع'),
- (0x1EE50, 'X'),
- (0x1EE51, 'M', 'ص'),
- (0x1EE52, 'M', 'ق'),
- (0x1EE53, 'X'),
- (0x1EE54, 'M', 'ش'),
- (0x1EE55, 'X'),
- (0x1EE57, 'M', 'خ'),
- (0x1EE58, 'X'),
- (0x1EE59, 'M', 'ض'),
- (0x1EE5A, 'X'),
- (0x1EE5B, 'M', 'غ'),
- (0x1EE5C, 'X'),
- (0x1EE5D, 'M', 'ں'),
- (0x1EE5E, 'X'),
- (0x1EE5F, 'M', 'ٯ'),
- (0x1EE60, 'X'),
- (0x1EE61, 'M', 'ب'),
- (0x1EE62, 'M', 'ج'),
- (0x1EE63, 'X'),
- (0x1EE64, 'M', 'ه'),
- (0x1EE65, 'X'),
- (0x1EE67, 'M', 'ح'),
- (0x1EE68, 'M', 'ط'),
- (0x1EE69, 'M', 'ي'),
- (0x1EE6A, 'M', 'ك'),
- (0x1EE6B, 'X'),
- (0x1EE6C, 'M', 'م'),
- (0x1EE6D, 'M', 'ن'),
- (0x1EE6E, 'M', 'س'),
- (0x1EE6F, 'M', 'ع'),
- (0x1EE70, 'M', 'ف'),
- (0x1EE71, 'M', 'ص'),
- (0x1EE72, 'M', 'ق'),
- (0x1EE73, 'X'),
- (0x1EE74, 'M', 'ش'),
- (0x1EE75, 'M', 'ت'),
- (0x1EE76, 'M', 'ث'),
- (0x1EE77, 'M', 'خ'),
- (0x1EE78, 'X'),
- (0x1EE79, 'M', 'ض'),
- (0x1EE7A, 'M', 'ظ'),
- (0x1EE7B, 'M', 'غ'),
- (0x1EE7C, 'M', 'ٮ'),
- (0x1EE7D, 'X'),
- (0x1EE7E, 'M', 'ڡ'),
- (0x1EE7F, 'X'),
- (0x1EE80, 'M', 'ا'),
- (0x1EE81, 'M', 'ب'),
- (0x1EE82, 'M', 'ج'),
- (0x1EE83, 'M', 'د'),
- (0x1EE84, 'M', 'ه'),
- (0x1EE85, 'M', 'و'),
- (0x1EE86, 'M', 'ز'),
- (0x1EE87, 'M', 'ح'),
- (0x1EE88, 'M', 'ط'),
- (0x1EE89, 'M', 'ي'),
- (0x1EE8A, 'X'),
- (0x1EE8B, 'M', 'ل'),
- (0x1EE8C, 'M', 'م'),
- (0x1EE8D, 'M', 'ن'),
- (0x1EE8E, 'M', 'س'),
- (0x1EE8F, 'M', 'ع'),
- (0x1EE90, 'M', 'ف'),
- (0x1EE91, 'M', 'ص'),
- (0x1EE92, 'M', 'ق'),
- (0x1EE93, 'M', 'ر'),
- (0x1EE94, 'M', 'ش'),
- (0x1EE95, 'M', 'ت'),
- (0x1EE96, 'M', 'ث'),
- (0x1EE97, 'M', 'خ'),
- (0x1EE98, 'M', 'ذ'),
- (0x1EE99, 'M', 'ض'),
- (0x1EE9A, 'M', 'ظ'),
- (0x1EE9B, 'M', 'غ'),
- (0x1EE9C, 'X'),
- (0x1EEA1, 'M', 'ب'),
- (0x1EEA2, 'M', 'ج'),
- (0x1EEA3, 'M', 'د'),
- (0x1EEA4, 'X'),
- (0x1EEA5, 'M', 'و'),
- ]
-
-def _seg_73() -> List[Union[Tuple[int, str], Tuple[int, str, str]]]:
- return [
- (0x1EEA6, 'M', 'ز'),
- (0x1EEA7, 'M', 'ح'),
- (0x1EEA8, 'M', 'ط'),
- (0x1EEA9, 'M', 'ي'),
- (0x1EEAA, 'X'),
- (0x1EEAB, 'M', 'ل'),
- (0x1EEAC, 'M', 'م'),
- (0x1EEAD, 'M', 'ن'),
- (0x1EEAE, 'M', 'س'),
- (0x1EEAF, 'M', 'ع'),
- (0x1EEB0, 'M', 'ف'),
- (0x1EEB1, 'M', 'ص'),
- (0x1EEB2, 'M', 'ق'),
- (0x1EEB3, 'M', 'ر'),
- (0x1EEB4, 'M', 'ش'),
- (0x1EEB5, 'M', 'ت'),
- (0x1EEB6, 'M', 'ث'),
- (0x1EEB7, 'M', 'خ'),
- (0x1EEB8, 'M', 'ذ'),
- (0x1EEB9, 'M', 'ض'),
- (0x1EEBA, 'M', 'ظ'),
- (0x1EEBB, 'M', 'غ'),
- (0x1EEBC, 'X'),
- (0x1EEF0, 'V'),
- (0x1EEF2, 'X'),
- (0x1F000, 'V'),
- (0x1F02C, 'X'),
- (0x1F030, 'V'),
- (0x1F094, 'X'),
- (0x1F0A0, 'V'),
- (0x1F0AF, 'X'),
- (0x1F0B1, 'V'),
- (0x1F0C0, 'X'),
- (0x1F0C1, 'V'),
- (0x1F0D0, 'X'),
- (0x1F0D1, 'V'),
- (0x1F0F6, 'X'),
- (0x1F101, '3', '0,'),
- (0x1F102, '3', '1,'),
- (0x1F103, '3', '2,'),
- (0x1F104, '3', '3,'),
- (0x1F105, '3', '4,'),
- (0x1F106, '3', '5,'),
- (0x1F107, '3', '6,'),
- (0x1F108, '3', '7,'),
- (0x1F109, '3', '8,'),
- (0x1F10A, '3', '9,'),
- (0x1F10B, 'V'),
- (0x1F110, '3', '(a)'),
- (0x1F111, '3', '(b)'),
- (0x1F112, '3', '(c)'),
- (0x1F113, '3', '(d)'),
- (0x1F114, '3', '(e)'),
- (0x1F115, '3', '(f)'),
- (0x1F116, '3', '(g)'),
- (0x1F117, '3', '(h)'),
- (0x1F118, '3', '(i)'),
- (0x1F119, '3', '(j)'),
- (0x1F11A, '3', '(k)'),
- (0x1F11B, '3', '(l)'),
- (0x1F11C, '3', '(m)'),
- (0x1F11D, '3', '(n)'),
- (0x1F11E, '3', '(o)'),
- (0x1F11F, '3', '(p)'),
- (0x1F120, '3', '(q)'),
- (0x1F121, '3', '(r)'),
- (0x1F122, '3', '(s)'),
- (0x1F123, '3', '(t)'),
- (0x1F124, '3', '(u)'),
- (0x1F125, '3', '(v)'),
- (0x1F126, '3', '(w)'),
- (0x1F127, '3', '(x)'),
- (0x1F128, '3', '(y)'),
- (0x1F129, '3', '(z)'),
- (0x1F12A, 'M', '〔s〕'),
- (0x1F12B, 'M', 'c'),
- (0x1F12C, 'M', 'r'),
- (0x1F12D, 'M', 'cd'),
- (0x1F12E, 'M', 'wz'),
- (0x1F12F, 'V'),
- (0x1F130, 'M', 'a'),
- (0x1F131, 'M', 'b'),
- (0x1F132, 'M', 'c'),
- (0x1F133, 'M', 'd'),
- (0x1F134, 'M', 'e'),
- (0x1F135, 'M', 'f'),
- (0x1F136, 'M', 'g'),
- (0x1F137, 'M', 'h'),
- (0x1F138, 'M', 'i'),
- (0x1F139, 'M', 'j'),
- (0x1F13A, 'M', 'k'),
- (0x1F13B, 'M', 'l'),
- (0x1F13C, 'M', 'm'),
- (0x1F13D, 'M', 'n'),
- (0x1F13E, 'M', 'o'),
- (0x1F13F, 'M', 'p'),
- (0x1F140, 'M', 'q'),
- (0x1F141, 'M', 'r'),
- (0x1F142, 'M', 's'),
- (0x1F143, 'M', 't'),
- ]
-
-def _seg_74() -> List[Union[Tuple[int, str], Tuple[int, str, str]]]:
- return [
- (0x1F144, 'M', 'u'),
- (0x1F145, 'M', 'v'),
- (0x1F146, 'M', 'w'),
- (0x1F147, 'M', 'x'),
- (0x1F148, 'M', 'y'),
- (0x1F149, 'M', 'z'),
- (0x1F14A, 'M', 'hv'),
- (0x1F14B, 'M', 'mv'),
- (0x1F14C, 'M', 'sd'),
- (0x1F14D, 'M', 'ss'),
- (0x1F14E, 'M', 'ppv'),
- (0x1F14F, 'M', 'wc'),
- (0x1F150, 'V'),
- (0x1F16A, 'M', 'mc'),
- (0x1F16B, 'M', 'md'),
- (0x1F16C, 'M', 'mr'),
- (0x1F16D, 'V'),
- (0x1F190, 'M', 'dj'),
- (0x1F191, 'V'),
- (0x1F1AE, 'X'),
- (0x1F1E6, 'V'),
- (0x1F200, 'M', 'ほか'),
- (0x1F201, 'M', 'ココ'),
- (0x1F202, 'M', 'サ'),
- (0x1F203, 'X'),
- (0x1F210, 'M', '手'),
- (0x1F211, 'M', '字'),
- (0x1F212, 'M', '双'),
- (0x1F213, 'M', 'デ'),
- (0x1F214, 'M', '二'),
- (0x1F215, 'M', '多'),
- (0x1F216, 'M', '解'),
- (0x1F217, 'M', '天'),
- (0x1F218, 'M', '交'),
- (0x1F219, 'M', '映'),
- (0x1F21A, 'M', '無'),
- (0x1F21B, 'M', '料'),
- (0x1F21C, 'M', '前'),
- (0x1F21D, 'M', '後'),
- (0x1F21E, 'M', '再'),
- (0x1F21F, 'M', '新'),
- (0x1F220, 'M', '初'),
- (0x1F221, 'M', '終'),
- (0x1F222, 'M', '生'),
- (0x1F223, 'M', '販'),
- (0x1F224, 'M', '声'),
- (0x1F225, 'M', '吹'),
- (0x1F226, 'M', '演'),
- (0x1F227, 'M', '投'),
- (0x1F228, 'M', '捕'),
- (0x1F229, 'M', '一'),
- (0x1F22A, 'M', '三'),
- (0x1F22B, 'M', '遊'),
- (0x1F22C, 'M', '左'),
- (0x1F22D, 'M', '中'),
- (0x1F22E, 'M', '右'),
- (0x1F22F, 'M', '指'),
- (0x1F230, 'M', '走'),
- (0x1F231, 'M', '打'),
- (0x1F232, 'M', '禁'),
- (0x1F233, 'M', '空'),
- (0x1F234, 'M', '合'),
- (0x1F235, 'M', '満'),
- (0x1F236, 'M', '有'),
- (0x1F237, 'M', '月'),
- (0x1F238, 'M', '申'),
- (0x1F239, 'M', '割'),
- (0x1F23A, 'M', '営'),
- (0x1F23B, 'M', '配'),
- (0x1F23C, 'X'),
- (0x1F240, 'M', '〔本〕'),
- (0x1F241, 'M', '〔三〕'),
- (0x1F242, 'M', '〔二〕'),
- (0x1F243, 'M', '〔安〕'),
- (0x1F244, 'M', '〔点〕'),
- (0x1F245, 'M', '〔打〕'),
- (0x1F246, 'M', '〔盗〕'),
- (0x1F247, 'M', '〔勝〕'),
- (0x1F248, 'M', '〔敗〕'),
- (0x1F249, 'X'),
- (0x1F250, 'M', '得'),
- (0x1F251, 'M', '可'),
- (0x1F252, 'X'),
- (0x1F260, 'V'),
- (0x1F266, 'X'),
- (0x1F300, 'V'),
- (0x1F6D8, 'X'),
- (0x1F6DD, 'V'),
- (0x1F6ED, 'X'),
- (0x1F6F0, 'V'),
- (0x1F6FD, 'X'),
- (0x1F700, 'V'),
- (0x1F774, 'X'),
- (0x1F780, 'V'),
- (0x1F7D9, 'X'),
- (0x1F7E0, 'V'),
- (0x1F7EC, 'X'),
- (0x1F7F0, 'V'),
- (0x1F7F1, 'X'),
- (0x1F800, 'V'),
- ]
-
-def _seg_75() -> List[Union[Tuple[int, str], Tuple[int, str, str]]]:
- return [
- (0x1F80C, 'X'),
- (0x1F810, 'V'),
- (0x1F848, 'X'),
- (0x1F850, 'V'),
- (0x1F85A, 'X'),
- (0x1F860, 'V'),
- (0x1F888, 'X'),
- (0x1F890, 'V'),
- (0x1F8AE, 'X'),
- (0x1F8B0, 'V'),
- (0x1F8B2, 'X'),
- (0x1F900, 'V'),
- (0x1FA54, 'X'),
- (0x1FA60, 'V'),
- (0x1FA6E, 'X'),
- (0x1FA70, 'V'),
- (0x1FA75, 'X'),
- (0x1FA78, 'V'),
- (0x1FA7D, 'X'),
- (0x1FA80, 'V'),
- (0x1FA87, 'X'),
- (0x1FA90, 'V'),
- (0x1FAAD, 'X'),
- (0x1FAB0, 'V'),
- (0x1FABB, 'X'),
- (0x1FAC0, 'V'),
- (0x1FAC6, 'X'),
- (0x1FAD0, 'V'),
- (0x1FADA, 'X'),
- (0x1FAE0, 'V'),
- (0x1FAE8, 'X'),
- (0x1FAF0, 'V'),
- (0x1FAF7, 'X'),
- (0x1FB00, 'V'),
- (0x1FB93, 'X'),
- (0x1FB94, 'V'),
- (0x1FBCB, 'X'),
- (0x1FBF0, 'M', '0'),
- (0x1FBF1, 'M', '1'),
- (0x1FBF2, 'M', '2'),
- (0x1FBF3, 'M', '3'),
- (0x1FBF4, 'M', '4'),
- (0x1FBF5, 'M', '5'),
- (0x1FBF6, 'M', '6'),
- (0x1FBF7, 'M', '7'),
- (0x1FBF8, 'M', '8'),
- (0x1FBF9, 'M', '9'),
- (0x1FBFA, 'X'),
- (0x20000, 'V'),
- (0x2A6E0, 'X'),
- (0x2A700, 'V'),
- (0x2B739, 'X'),
- (0x2B740, 'V'),
- (0x2B81E, 'X'),
- (0x2B820, 'V'),
- (0x2CEA2, 'X'),
- (0x2CEB0, 'V'),
- (0x2EBE1, 'X'),
- (0x2F800, 'M', '丽'),
- (0x2F801, 'M', '丸'),
- (0x2F802, 'M', '乁'),
- (0x2F803, 'M', '𠄢'),
- (0x2F804, 'M', '你'),
- (0x2F805, 'M', '侮'),
- (0x2F806, 'M', '侻'),
- (0x2F807, 'M', '倂'),
- (0x2F808, 'M', '偺'),
- (0x2F809, 'M', '備'),
- (0x2F80A, 'M', '僧'),
- (0x2F80B, 'M', '像'),
- (0x2F80C, 'M', '㒞'),
- (0x2F80D, 'M', '𠘺'),
- (0x2F80E, 'M', '免'),
- (0x2F80F, 'M', '兔'),
- (0x2F810, 'M', '兤'),
- (0x2F811, 'M', '具'),
- (0x2F812, 'M', '𠔜'),
- (0x2F813, 'M', '㒹'),
- (0x2F814, 'M', '內'),
- (0x2F815, 'M', '再'),
- (0x2F816, 'M', '𠕋'),
- (0x2F817, 'M', '冗'),
- (0x2F818, 'M', '冤'),
- (0x2F819, 'M', '仌'),
- (0x2F81A, 'M', '冬'),
- (0x2F81B, 'M', '况'),
- (0x2F81C, 'M', '𩇟'),
- (0x2F81D, 'M', '凵'),
- (0x2F81E, 'M', '刃'),
- (0x2F81F, 'M', '㓟'),
- (0x2F820, 'M', '刻'),
- (0x2F821, 'M', '剆'),
- (0x2F822, 'M', '割'),
- (0x2F823, 'M', '剷'),
- (0x2F824, 'M', '㔕'),
- (0x2F825, 'M', '勇'),
- (0x2F826, 'M', '勉'),
- (0x2F827, 'M', '勤'),
- (0x2F828, 'M', '勺'),
- (0x2F829, 'M', '包'),
- ]
-
-def _seg_76() -> List[Union[Tuple[int, str], Tuple[int, str, str]]]:
- return [
- (0x2F82A, 'M', '匆'),
- (0x2F82B, 'M', '北'),
- (0x2F82C, 'M', '卉'),
- (0x2F82D, 'M', '卑'),
- (0x2F82E, 'M', '博'),
- (0x2F82F, 'M', '即'),
- (0x2F830, 'M', '卽'),
- (0x2F831, 'M', '卿'),
- (0x2F834, 'M', '𠨬'),
- (0x2F835, 'M', '灰'),
- (0x2F836, 'M', '及'),
- (0x2F837, 'M', '叟'),
- (0x2F838, 'M', '𠭣'),
- (0x2F839, 'M', '叫'),
- (0x2F83A, 'M', '叱'),
- (0x2F83B, 'M', '吆'),
- (0x2F83C, 'M', '咞'),
- (0x2F83D, 'M', '吸'),
- (0x2F83E, 'M', '呈'),
- (0x2F83F, 'M', '周'),
- (0x2F840, 'M', '咢'),
- (0x2F841, 'M', '哶'),
- (0x2F842, 'M', '唐'),
- (0x2F843, 'M', '啓'),
- (0x2F844, 'M', '啣'),
- (0x2F845, 'M', '善'),
- (0x2F847, 'M', '喙'),
- (0x2F848, 'M', '喫'),
- (0x2F849, 'M', '喳'),
- (0x2F84A, 'M', '嗂'),
- (0x2F84B, 'M', '圖'),
- (0x2F84C, 'M', '嘆'),
- (0x2F84D, 'M', '圗'),
- (0x2F84E, 'M', '噑'),
- (0x2F84F, 'M', '噴'),
- (0x2F850, 'M', '切'),
- (0x2F851, 'M', '壮'),
- (0x2F852, 'M', '城'),
- (0x2F853, 'M', '埴'),
- (0x2F854, 'M', '堍'),
- (0x2F855, 'M', '型'),
- (0x2F856, 'M', '堲'),
- (0x2F857, 'M', '報'),
- (0x2F858, 'M', '墬'),
- (0x2F859, 'M', '𡓤'),
- (0x2F85A, 'M', '売'),
- (0x2F85B, 'M', '壷'),
- (0x2F85C, 'M', '夆'),
- (0x2F85D, 'M', '多'),
- (0x2F85E, 'M', '夢'),
- (0x2F85F, 'M', '奢'),
- (0x2F860, 'M', '𡚨'),
- (0x2F861, 'M', '𡛪'),
- (0x2F862, 'M', '姬'),
- (0x2F863, 'M', '娛'),
- (0x2F864, 'M', '娧'),
- (0x2F865, 'M', '姘'),
- (0x2F866, 'M', '婦'),
- (0x2F867, 'M', '㛮'),
- (0x2F868, 'X'),
- (0x2F869, 'M', '嬈'),
- (0x2F86A, 'M', '嬾'),
- (0x2F86C, 'M', '𡧈'),
- (0x2F86D, 'M', '寃'),
- (0x2F86E, 'M', '寘'),
- (0x2F86F, 'M', '寧'),
- (0x2F870, 'M', '寳'),
- (0x2F871, 'M', '𡬘'),
- (0x2F872, 'M', '寿'),
- (0x2F873, 'M', '将'),
- (0x2F874, 'X'),
- (0x2F875, 'M', '尢'),
- (0x2F876, 'M', '㞁'),
- (0x2F877, 'M', '屠'),
- (0x2F878, 'M', '屮'),
- (0x2F879, 'M', '峀'),
- (0x2F87A, 'M', '岍'),
- (0x2F87B, 'M', '𡷤'),
- (0x2F87C, 'M', '嵃'),
- (0x2F87D, 'M', '𡷦'),
- (0x2F87E, 'M', '嵮'),
- (0x2F87F, 'M', '嵫'),
- (0x2F880, 'M', '嵼'),
- (0x2F881, 'M', '巡'),
- (0x2F882, 'M', '巢'),
- (0x2F883, 'M', '㠯'),
- (0x2F884, 'M', '巽'),
- (0x2F885, 'M', '帨'),
- (0x2F886, 'M', '帽'),
- (0x2F887, 'M', '幩'),
- (0x2F888, 'M', '㡢'),
- (0x2F889, 'M', '𢆃'),
- (0x2F88A, 'M', '㡼'),
- (0x2F88B, 'M', '庰'),
- (0x2F88C, 'M', '庳'),
- (0x2F88D, 'M', '庶'),
- (0x2F88E, 'M', '廊'),
- (0x2F88F, 'M', '𪎒'),
- (0x2F890, 'M', '廾'),
- (0x2F891, 'M', '𢌱'),
- ]
-
-def _seg_77() -> List[Union[Tuple[int, str], Tuple[int, str, str]]]:
- return [
- (0x2F893, 'M', '舁'),
- (0x2F894, 'M', '弢'),
- (0x2F896, 'M', '㣇'),
- (0x2F897, 'M', '𣊸'),
- (0x2F898, 'M', '𦇚'),
- (0x2F899, 'M', '形'),
- (0x2F89A, 'M', '彫'),
- (0x2F89B, 'M', '㣣'),
- (0x2F89C, 'M', '徚'),
- (0x2F89D, 'M', '忍'),
- (0x2F89E, 'M', '志'),
- (0x2F89F, 'M', '忹'),
- (0x2F8A0, 'M', '悁'),
- (0x2F8A1, 'M', '㤺'),
- (0x2F8A2, 'M', '㤜'),
- (0x2F8A3, 'M', '悔'),
- (0x2F8A4, 'M', '𢛔'),
- (0x2F8A5, 'M', '惇'),
- (0x2F8A6, 'M', '慈'),
- (0x2F8A7, 'M', '慌'),
- (0x2F8A8, 'M', '慎'),
- (0x2F8A9, 'M', '慌'),
- (0x2F8AA, 'M', '慺'),
- (0x2F8AB, 'M', '憎'),
- (0x2F8AC, 'M', '憲'),
- (0x2F8AD, 'M', '憤'),
- (0x2F8AE, 'M', '憯'),
- (0x2F8AF, 'M', '懞'),
- (0x2F8B0, 'M', '懲'),
- (0x2F8B1, 'M', '懶'),
- (0x2F8B2, 'M', '成'),
- (0x2F8B3, 'M', '戛'),
- (0x2F8B4, 'M', '扝'),
- (0x2F8B5, 'M', '抱'),
- (0x2F8B6, 'M', '拔'),
- (0x2F8B7, 'M', '捐'),
- (0x2F8B8, 'M', '𢬌'),
- (0x2F8B9, 'M', '挽'),
- (0x2F8BA, 'M', '拼'),
- (0x2F8BB, 'M', '捨'),
- (0x2F8BC, 'M', '掃'),
- (0x2F8BD, 'M', '揤'),
- (0x2F8BE, 'M', '𢯱'),
- (0x2F8BF, 'M', '搢'),
- (0x2F8C0, 'M', '揅'),
- (0x2F8C1, 'M', '掩'),
- (0x2F8C2, 'M', '㨮'),
- (0x2F8C3, 'M', '摩'),
- (0x2F8C4, 'M', '摾'),
- (0x2F8C5, 'M', '撝'),
- (0x2F8C6, 'M', '摷'),
- (0x2F8C7, 'M', '㩬'),
- (0x2F8C8, 'M', '敏'),
- (0x2F8C9, 'M', '敬'),
- (0x2F8CA, 'M', '𣀊'),
- (0x2F8CB, 'M', '旣'),
- (0x2F8CC, 'M', '書'),
- (0x2F8CD, 'M', '晉'),
- (0x2F8CE, 'M', '㬙'),
- (0x2F8CF, 'M', '暑'),
- (0x2F8D0, 'M', '㬈'),
- (0x2F8D1, 'M', '㫤'),
- (0x2F8D2, 'M', '冒'),
- (0x2F8D3, 'M', '冕'),
- (0x2F8D4, 'M', '最'),
- (0x2F8D5, 'M', '暜'),
- (0x2F8D6, 'M', '肭'),
- (0x2F8D7, 'M', '䏙'),
- (0x2F8D8, 'M', '朗'),
- (0x2F8D9, 'M', '望'),
- (0x2F8DA, 'M', '朡'),
- (0x2F8DB, 'M', '杞'),
- (0x2F8DC, 'M', '杓'),
- (0x2F8DD, 'M', '𣏃'),
- (0x2F8DE, 'M', '㭉'),
- (0x2F8DF, 'M', '柺'),
- (0x2F8E0, 'M', '枅'),
- (0x2F8E1, 'M', '桒'),
- (0x2F8E2, 'M', '梅'),
- (0x2F8E3, 'M', '𣑭'),
- (0x2F8E4, 'M', '梎'),
- (0x2F8E5, 'M', '栟'),
- (0x2F8E6, 'M', '椔'),
- (0x2F8E7, 'M', '㮝'),
- (0x2F8E8, 'M', '楂'),
- (0x2F8E9, 'M', '榣'),
- (0x2F8EA, 'M', '槪'),
- (0x2F8EB, 'M', '檨'),
- (0x2F8EC, 'M', '𣚣'),
- (0x2F8ED, 'M', '櫛'),
- (0x2F8EE, 'M', '㰘'),
- (0x2F8EF, 'M', '次'),
- (0x2F8F0, 'M', '𣢧'),
- (0x2F8F1, 'M', '歔'),
- (0x2F8F2, 'M', '㱎'),
- (0x2F8F3, 'M', '歲'),
- (0x2F8F4, 'M', '殟'),
- (0x2F8F5, 'M', '殺'),
- (0x2F8F6, 'M', '殻'),
- (0x2F8F7, 'M', '𣪍'),
- ]
-
-def _seg_78() -> List[Union[Tuple[int, str], Tuple[int, str, str]]]:
- return [
- (0x2F8F8, 'M', '𡴋'),
- (0x2F8F9, 'M', '𣫺'),
- (0x2F8FA, 'M', '汎'),
- (0x2F8FB, 'M', '𣲼'),
- (0x2F8FC, 'M', '沿'),
- (0x2F8FD, 'M', '泍'),
- (0x2F8FE, 'M', '汧'),
- (0x2F8FF, 'M', '洖'),
- (0x2F900, 'M', '派'),
- (0x2F901, 'M', '海'),
- (0x2F902, 'M', '流'),
- (0x2F903, 'M', '浩'),
- (0x2F904, 'M', '浸'),
- (0x2F905, 'M', '涅'),
- (0x2F906, 'M', '𣴞'),
- (0x2F907, 'M', '洴'),
- (0x2F908, 'M', '港'),
- (0x2F909, 'M', '湮'),
- (0x2F90A, 'M', '㴳'),
- (0x2F90B, 'M', '滋'),
- (0x2F90C, 'M', '滇'),
- (0x2F90D, 'M', '𣻑'),
- (0x2F90E, 'M', '淹'),
- (0x2F90F, 'M', '潮'),
- (0x2F910, 'M', '𣽞'),
- (0x2F911, 'M', '𣾎'),
- (0x2F912, 'M', '濆'),
- (0x2F913, 'M', '瀹'),
- (0x2F914, 'M', '瀞'),
- (0x2F915, 'M', '瀛'),
- (0x2F916, 'M', '㶖'),
- (0x2F917, 'M', '灊'),
- (0x2F918, 'M', '災'),
- (0x2F919, 'M', '灷'),
- (0x2F91A, 'M', '炭'),
- (0x2F91B, 'M', '𠔥'),
- (0x2F91C, 'M', '煅'),
- (0x2F91D, 'M', '𤉣'),
- (0x2F91E, 'M', '熜'),
- (0x2F91F, 'X'),
- (0x2F920, 'M', '爨'),
- (0x2F921, 'M', '爵'),
- (0x2F922, 'M', '牐'),
- (0x2F923, 'M', '𤘈'),
- (0x2F924, 'M', '犀'),
- (0x2F925, 'M', '犕'),
- (0x2F926, 'M', '𤜵'),
- (0x2F927, 'M', '𤠔'),
- (0x2F928, 'M', '獺'),
- (0x2F929, 'M', '王'),
- (0x2F92A, 'M', '㺬'),
- (0x2F92B, 'M', '玥'),
- (0x2F92C, 'M', '㺸'),
- (0x2F92E, 'M', '瑇'),
- (0x2F92F, 'M', '瑜'),
- (0x2F930, 'M', '瑱'),
- (0x2F931, 'M', '璅'),
- (0x2F932, 'M', '瓊'),
- (0x2F933, 'M', '㼛'),
- (0x2F934, 'M', '甤'),
- (0x2F935, 'M', '𤰶'),
- (0x2F936, 'M', '甾'),
- (0x2F937, 'M', '𤲒'),
- (0x2F938, 'M', '異'),
- (0x2F939, 'M', '𢆟'),
- (0x2F93A, 'M', '瘐'),
- (0x2F93B, 'M', '𤾡'),
- (0x2F93C, 'M', '𤾸'),
- (0x2F93D, 'M', '𥁄'),
- (0x2F93E, 'M', '㿼'),
- (0x2F93F, 'M', '䀈'),
- (0x2F940, 'M', '直'),
- (0x2F941, 'M', '𥃳'),
- (0x2F942, 'M', '𥃲'),
- (0x2F943, 'M', '𥄙'),
- (0x2F944, 'M', '𥄳'),
- (0x2F945, 'M', '眞'),
- (0x2F946, 'M', '真'),
- (0x2F948, 'M', '睊'),
- (0x2F949, 'M', '䀹'),
- (0x2F94A, 'M', '瞋'),
- (0x2F94B, 'M', '䁆'),
- (0x2F94C, 'M', '䂖'),
- (0x2F94D, 'M', '𥐝'),
- (0x2F94E, 'M', '硎'),
- (0x2F94F, 'M', '碌'),
- (0x2F950, 'M', '磌'),
- (0x2F951, 'M', '䃣'),
- (0x2F952, 'M', '𥘦'),
- (0x2F953, 'M', '祖'),
- (0x2F954, 'M', '𥚚'),
- (0x2F955, 'M', '𥛅'),
- (0x2F956, 'M', '福'),
- (0x2F957, 'M', '秫'),
- (0x2F958, 'M', '䄯'),
- (0x2F959, 'M', '穀'),
- (0x2F95A, 'M', '穊'),
- (0x2F95B, 'M', '穏'),
- (0x2F95C, 'M', '𥥼'),
- (0x2F95D, 'M', '𥪧'),
- ]
-
-def _seg_79() -> List[Union[Tuple[int, str], Tuple[int, str, str]]]:
- return [
- (0x2F95F, 'X'),
- (0x2F960, 'M', '䈂'),
- (0x2F961, 'M', '𥮫'),
- (0x2F962, 'M', '篆'),
- (0x2F963, 'M', '築'),
- (0x2F964, 'M', '䈧'),
- (0x2F965, 'M', '𥲀'),
- (0x2F966, 'M', '糒'),
- (0x2F967, 'M', '䊠'),
- (0x2F968, 'M', '糨'),
- (0x2F969, 'M', '糣'),
- (0x2F96A, 'M', '紀'),
- (0x2F96B, 'M', '𥾆'),
- (0x2F96C, 'M', '絣'),
- (0x2F96D, 'M', '䌁'),
- (0x2F96E, 'M', '緇'),
- (0x2F96F, 'M', '縂'),
- (0x2F970, 'M', '繅'),
- (0x2F971, 'M', '䌴'),
- (0x2F972, 'M', '𦈨'),
- (0x2F973, 'M', '𦉇'),
- (0x2F974, 'M', '䍙'),
- (0x2F975, 'M', '𦋙'),
- (0x2F976, 'M', '罺'),
- (0x2F977, 'M', '𦌾'),
- (0x2F978, 'M', '羕'),
- (0x2F979, 'M', '翺'),
- (0x2F97A, 'M', '者'),
- (0x2F97B, 'M', '𦓚'),
- (0x2F97C, 'M', '𦔣'),
- (0x2F97D, 'M', '聠'),
- (0x2F97E, 'M', '𦖨'),
- (0x2F97F, 'M', '聰'),
- (0x2F980, 'M', '𣍟'),
- (0x2F981, 'M', '䏕'),
- (0x2F982, 'M', '育'),
- (0x2F983, 'M', '脃'),
- (0x2F984, 'M', '䐋'),
- (0x2F985, 'M', '脾'),
- (0x2F986, 'M', '媵'),
- (0x2F987, 'M', '𦞧'),
- (0x2F988, 'M', '𦞵'),
- (0x2F989, 'M', '𣎓'),
- (0x2F98A, 'M', '𣎜'),
- (0x2F98B, 'M', '舁'),
- (0x2F98C, 'M', '舄'),
- (0x2F98D, 'M', '辞'),
- (0x2F98E, 'M', '䑫'),
- (0x2F98F, 'M', '芑'),
- (0x2F990, 'M', '芋'),
- (0x2F991, 'M', '芝'),
- (0x2F992, 'M', '劳'),
- (0x2F993, 'M', '花'),
- (0x2F994, 'M', '芳'),
- (0x2F995, 'M', '芽'),
- (0x2F996, 'M', '苦'),
- (0x2F997, 'M', '𦬼'),
- (0x2F998, 'M', '若'),
- (0x2F999, 'M', '茝'),
- (0x2F99A, 'M', '荣'),
- (0x2F99B, 'M', '莭'),
- (0x2F99C, 'M', '茣'),
- (0x2F99D, 'M', '莽'),
- (0x2F99E, 'M', '菧'),
- (0x2F99F, 'M', '著'),
- (0x2F9A0, 'M', '荓'),
- (0x2F9A1, 'M', '菊'),
- (0x2F9A2, 'M', '菌'),
- (0x2F9A3, 'M', '菜'),
- (0x2F9A4, 'M', '𦰶'),
- (0x2F9A5, 'M', '𦵫'),
- (0x2F9A6, 'M', '𦳕'),
- (0x2F9A7, 'M', '䔫'),
- (0x2F9A8, 'M', '蓱'),
- (0x2F9A9, 'M', '蓳'),
- (0x2F9AA, 'M', '蔖'),
- (0x2F9AB, 'M', '𧏊'),
- (0x2F9AC, 'M', '蕤'),
- (0x2F9AD, 'M', '𦼬'),
- (0x2F9AE, 'M', '䕝'),
- (0x2F9AF, 'M', '䕡'),
- (0x2F9B0, 'M', '𦾱'),
- (0x2F9B1, 'M', '𧃒'),
- (0x2F9B2, 'M', '䕫'),
- (0x2F9B3, 'M', '虐'),
- (0x2F9B4, 'M', '虜'),
- (0x2F9B5, 'M', '虧'),
- (0x2F9B6, 'M', '虩'),
- (0x2F9B7, 'M', '蚩'),
- (0x2F9B8, 'M', '蚈'),
- (0x2F9B9, 'M', '蜎'),
- (0x2F9BA, 'M', '蛢'),
- (0x2F9BB, 'M', '蝹'),
- (0x2F9BC, 'M', '蜨'),
- (0x2F9BD, 'M', '蝫'),
- (0x2F9BE, 'M', '螆'),
- (0x2F9BF, 'X'),
- (0x2F9C0, 'M', '蟡'),
- (0x2F9C1, 'M', '蠁'),
- (0x2F9C2, 'M', '䗹'),
- ]
-
-def _seg_80() -> List[Union[Tuple[int, str], Tuple[int, str, str]]]:
- return [
- (0x2F9C3, 'M', '衠'),
- (0x2F9C4, 'M', '衣'),
- (0x2F9C5, 'M', '𧙧'),
- (0x2F9C6, 'M', '裗'),
- (0x2F9C7, 'M', '裞'),
- (0x2F9C8, 'M', '䘵'),
- (0x2F9C9, 'M', '裺'),
- (0x2F9CA, 'M', '㒻'),
- (0x2F9CB, 'M', '𧢮'),
- (0x2F9CC, 'M', '𧥦'),
- (0x2F9CD, 'M', '䚾'),
- (0x2F9CE, 'M', '䛇'),
- (0x2F9CF, 'M', '誠'),
- (0x2F9D0, 'M', '諭'),
- (0x2F9D1, 'M', '變'),
- (0x2F9D2, 'M', '豕'),
- (0x2F9D3, 'M', '𧲨'),
- (0x2F9D4, 'M', '貫'),
- (0x2F9D5, 'M', '賁'),
- (0x2F9D6, 'M', '贛'),
- (0x2F9D7, 'M', '起'),
- (0x2F9D8, 'M', '𧼯'),
- (0x2F9D9, 'M', '𠠄'),
- (0x2F9DA, 'M', '跋'),
- (0x2F9DB, 'M', '趼'),
- (0x2F9DC, 'M', '跰'),
- (0x2F9DD, 'M', '𠣞'),
- (0x2F9DE, 'M', '軔'),
- (0x2F9DF, 'M', '輸'),
- (0x2F9E0, 'M', '𨗒'),
- (0x2F9E1, 'M', '𨗭'),
- (0x2F9E2, 'M', '邔'),
- (0x2F9E3, 'M', '郱'),
- (0x2F9E4, 'M', '鄑'),
- (0x2F9E5, 'M', '𨜮'),
- (0x2F9E6, 'M', '鄛'),
- (0x2F9E7, 'M', '鈸'),
- (0x2F9E8, 'M', '鋗'),
- (0x2F9E9, 'M', '鋘'),
- (0x2F9EA, 'M', '鉼'),
- (0x2F9EB, 'M', '鏹'),
- (0x2F9EC, 'M', '鐕'),
- (0x2F9ED, 'M', '𨯺'),
- (0x2F9EE, 'M', '開'),
- (0x2F9EF, 'M', '䦕'),
- (0x2F9F0, 'M', '閷'),
- (0x2F9F1, 'M', '𨵷'),
- (0x2F9F2, 'M', '䧦'),
- (0x2F9F3, 'M', '雃'),
- (0x2F9F4, 'M', '嶲'),
- (0x2F9F5, 'M', '霣'),
- (0x2F9F6, 'M', '𩅅'),
- (0x2F9F7, 'M', '𩈚'),
- (0x2F9F8, 'M', '䩮'),
- (0x2F9F9, 'M', '䩶'),
- (0x2F9FA, 'M', '韠'),
- (0x2F9FB, 'M', '𩐊'),
- (0x2F9FC, 'M', '䪲'),
- (0x2F9FD, 'M', '𩒖'),
- (0x2F9FE, 'M', '頋'),
- (0x2FA00, 'M', '頩'),
- (0x2FA01, 'M', '𩖶'),
- (0x2FA02, 'M', '飢'),
- (0x2FA03, 'M', '䬳'),
- (0x2FA04, 'M', '餩'),
- (0x2FA05, 'M', '馧'),
- (0x2FA06, 'M', '駂'),
- (0x2FA07, 'M', '駾'),
- (0x2FA08, 'M', '䯎'),
- (0x2FA09, 'M', '𩬰'),
- (0x2FA0A, 'M', '鬒'),
- (0x2FA0B, 'M', '鱀'),
- (0x2FA0C, 'M', '鳽'),
- (0x2FA0D, 'M', '䳎'),
- (0x2FA0E, 'M', '䳭'),
- (0x2FA0F, 'M', '鵧'),
- (0x2FA10, 'M', '𪃎'),
- (0x2FA11, 'M', '䳸'),
- (0x2FA12, 'M', '𪄅'),
- (0x2FA13, 'M', '𪈎'),
- (0x2FA14, 'M', '𪊑'),
- (0x2FA15, 'M', '麻'),
- (0x2FA16, 'M', '䵖'),
- (0x2FA17, 'M', '黹'),
- (0x2FA18, 'M', '黾'),
- (0x2FA19, 'M', '鼅'),
- (0x2FA1A, 'M', '鼏'),
- (0x2FA1B, 'M', '鼖'),
- (0x2FA1C, 'M', '鼻'),
- (0x2FA1D, 'M', '𪘀'),
- (0x2FA1E, 'X'),
- (0x30000, 'V'),
- (0x3134B, 'X'),
- (0xE0100, 'I'),
- (0xE01F0, 'X'),
- ]
-
-uts46data = tuple(
- _seg_0()
- + _seg_1()
- + _seg_2()
- + _seg_3()
- + _seg_4()
- + _seg_5()
- + _seg_6()
- + _seg_7()
- + _seg_8()
- + _seg_9()
- + _seg_10()
- + _seg_11()
- + _seg_12()
- + _seg_13()
- + _seg_14()
- + _seg_15()
- + _seg_16()
- + _seg_17()
- + _seg_18()
- + _seg_19()
- + _seg_20()
- + _seg_21()
- + _seg_22()
- + _seg_23()
- + _seg_24()
- + _seg_25()
- + _seg_26()
- + _seg_27()
- + _seg_28()
- + _seg_29()
- + _seg_30()
- + _seg_31()
- + _seg_32()
- + _seg_33()
- + _seg_34()
- + _seg_35()
- + _seg_36()
- + _seg_37()
- + _seg_38()
- + _seg_39()
- + _seg_40()
- + _seg_41()
- + _seg_42()
- + _seg_43()
- + _seg_44()
- + _seg_45()
- + _seg_46()
- + _seg_47()
- + _seg_48()
- + _seg_49()
- + _seg_50()
- + _seg_51()
- + _seg_52()
- + _seg_53()
- + _seg_54()
- + _seg_55()
- + _seg_56()
- + _seg_57()
- + _seg_58()
- + _seg_59()
- + _seg_60()
- + _seg_61()
- + _seg_62()
- + _seg_63()
- + _seg_64()
- + _seg_65()
- + _seg_66()
- + _seg_67()
- + _seg_68()
- + _seg_69()
- + _seg_70()
- + _seg_71()
- + _seg_72()
- + _seg_73()
- + _seg_74()
- + _seg_75()
- + _seg_76()
- + _seg_77()
- + _seg_78()
- + _seg_79()
- + _seg_80()
-) # type: Tuple[Union[Tuple[int, str], Tuple[int, str, str]], ...]
diff --git a/spaces/ali-ghamdan/deoldify/deoldify/unet.py b/spaces/ali-ghamdan/deoldify/deoldify/unet.py
deleted file mode 100644
index 307a5d920e983a6228dd72b7bfbd1b8840fff89b..0000000000000000000000000000000000000000
--- a/spaces/ali-ghamdan/deoldify/deoldify/unet.py
+++ /dev/null
@@ -1,285 +0,0 @@
-from fastai.layers import *
-from .layers import *
-from fastai.torch_core import *
-from fastai.callbacks.hooks import *
-from fastai.vision import *
-
-
-# The code below is meant to be merged into fastaiv1 ideally
-
-__all__ = ['DynamicUnetDeep', 'DynamicUnetWide']
-
-
-def _get_sfs_idxs(sizes: Sizes) -> List[int]:
- "Get the indexes of the layers where the size of the activation changes."
- feature_szs = [size[-1] for size in sizes]
- sfs_idxs = list(
- np.where(np.array(feature_szs[:-1]) != np.array(feature_szs[1:]))[0]
- )
- if feature_szs[0] != feature_szs[1]:
- sfs_idxs = [0] + sfs_idxs
- return sfs_idxs
-
-
-class CustomPixelShuffle_ICNR(nn.Module):
- "Upsample by `scale` from `ni` filters to `nf` (default `ni`), using `nn.PixelShuffle`, `icnr` init, and `weight_norm`."
-
- def __init__(
- self,
- ni: int,
- nf: int = None,
- scale: int = 2,
- blur: bool = False,
- leaky: float = None,
- **kwargs
- ):
- super().__init__()
- nf = ifnone(nf, ni)
- self.conv = custom_conv_layer(
- ni, nf * (scale ** 2), ks=1, use_activ=False, **kwargs
- )
- icnr(self.conv[0].weight)
- self.shuf = nn.PixelShuffle(scale)
- # Blurring over (h*w) kernel
- # "Super-Resolution using Convolutional Neural Networks without Any Checkerboard Artifacts"
- # - https://arxiv.org/abs/1806.02658
- self.pad = nn.ReplicationPad2d((1, 0, 1, 0))
- self.blur = nn.AvgPool2d(2, stride=1)
- self.relu = relu(True, leaky=leaky)
-
- def forward(self, x):
- x = self.shuf(self.relu(self.conv(x)))
- return self.blur(self.pad(x)) if self.blur else x
-
-
-class UnetBlockDeep(nn.Module):
- "A quasi-UNet block, using `PixelShuffle_ICNR upsampling`."
-
- def __init__(
- self,
- up_in_c: int,
- x_in_c: int,
- hook: Hook,
- final_div: bool = True,
- blur: bool = False,
- leaky: float = None,
- self_attention: bool = False,
- nf_factor: float = 1.0,
- **kwargs
- ):
- super().__init__()
- self.hook = hook
- self.shuf = CustomPixelShuffle_ICNR(
- up_in_c, up_in_c // 2, blur=blur, leaky=leaky, **kwargs
- )
- self.bn = batchnorm_2d(x_in_c)
- ni = up_in_c // 2 + x_in_c
- nf = int((ni if final_div else ni // 2) * nf_factor)
- self.conv1 = custom_conv_layer(ni, nf, leaky=leaky, **kwargs)
- self.conv2 = custom_conv_layer(
- nf, nf, leaky=leaky, self_attention=self_attention, **kwargs
- )
- self.relu = relu(leaky=leaky)
-
- def forward(self, up_in: Tensor) -> Tensor:
- s = self.hook.stored
- up_out = self.shuf(up_in)
- ssh = s.shape[-2:]
- if ssh != up_out.shape[-2:]:
- up_out = F.interpolate(up_out, s.shape[-2:], mode='nearest')
- cat_x = self.relu(torch.cat([up_out, self.bn(s)], dim=1))
- return self.conv2(self.conv1(cat_x))
-
-
-class DynamicUnetDeep(SequentialEx):
- "Create a U-Net from a given architecture."
-
- def __init__(
- self,
- encoder: nn.Module,
- n_classes: int,
- blur: bool = False,
- blur_final=True,
- self_attention: bool = False,
- y_range: Optional[Tuple[float, float]] = None,
- last_cross: bool = True,
- bottle: bool = False,
- norm_type: Optional[NormType] = NormType.Batch,
- nf_factor: float = 1.0,
- **kwargs
- ):
- extra_bn = norm_type == NormType.Spectral
- imsize = (256, 256)
- sfs_szs = model_sizes(encoder, size=imsize)
- sfs_idxs = list(reversed(_get_sfs_idxs(sfs_szs)))
- self.sfs = hook_outputs([encoder[i] for i in sfs_idxs], detach=False)
- x = dummy_eval(encoder, imsize).detach()
-
- ni = sfs_szs[-1][1]
- middle_conv = nn.Sequential(
- custom_conv_layer(
- ni, ni * 2, norm_type=norm_type, extra_bn=extra_bn, **kwargs
- ),
- custom_conv_layer(
- ni * 2, ni, norm_type=norm_type, extra_bn=extra_bn, **kwargs
- ),
- ).eval()
- x = middle_conv(x)
- layers = [encoder, batchnorm_2d(ni), nn.ReLU(), middle_conv]
-
- for i, idx in enumerate(sfs_idxs):
- not_final = i != len(sfs_idxs) - 1
- up_in_c, x_in_c = int(x.shape[1]), int(sfs_szs[idx][1])
- do_blur = blur and (not_final or blur_final)
- sa = self_attention and (i == len(sfs_idxs) - 3)
- unet_block = UnetBlockDeep(
- up_in_c,
- x_in_c,
- self.sfs[i],
- final_div=not_final,
- blur=blur,
- self_attention=sa,
- norm_type=norm_type,
- extra_bn=extra_bn,
- nf_factor=nf_factor,
- **kwargs
- ).eval()
- layers.append(unet_block)
- x = unet_block(x)
-
- ni = x.shape[1]
- if imsize != sfs_szs[0][-2:]:
- layers.append(PixelShuffle_ICNR(ni, **kwargs))
- if last_cross:
- layers.append(MergeLayer(dense=True))
- ni += in_channels(encoder)
- layers.append(res_block(ni, bottle=bottle, norm_type=norm_type, **kwargs))
- layers += [
- custom_conv_layer(ni, n_classes, ks=1, use_activ=False, norm_type=norm_type)
- ]
- if y_range is not None:
- layers.append(SigmoidRange(*y_range))
- super().__init__(*layers)
-
- def __del__(self):
- if hasattr(self, "sfs"):
- self.sfs.remove()
-
-
-# ------------------------------------------------------
-class UnetBlockWide(nn.Module):
- "A quasi-UNet block, using `PixelShuffle_ICNR upsampling`."
-
- def __init__(
- self,
- up_in_c: int,
- x_in_c: int,
- n_out: int,
- hook: Hook,
- final_div: bool = True,
- blur: bool = False,
- leaky: float = None,
- self_attention: bool = False,
- **kwargs
- ):
- super().__init__()
- self.hook = hook
- up_out = x_out = n_out // 2
- self.shuf = CustomPixelShuffle_ICNR(
- up_in_c, up_out, blur=blur, leaky=leaky, **kwargs
- )
- self.bn = batchnorm_2d(x_in_c)
- ni = up_out + x_in_c
- self.conv = custom_conv_layer(
- ni, x_out, leaky=leaky, self_attention=self_attention, **kwargs
- )
- self.relu = relu(leaky=leaky)
-
- def forward(self, up_in: Tensor) -> Tensor:
- s = self.hook.stored
- up_out = self.shuf(up_in)
- ssh = s.shape[-2:]
- if ssh != up_out.shape[-2:]:
- up_out = F.interpolate(up_out, s.shape[-2:], mode='nearest')
- cat_x = self.relu(torch.cat([up_out, self.bn(s)], dim=1))
- return self.conv(cat_x)
-
-
-class DynamicUnetWide(SequentialEx):
- "Create a U-Net from a given architecture."
-
- def __init__(
- self,
- encoder: nn.Module,
- n_classes: int,
- blur: bool = False,
- blur_final=True,
- self_attention: bool = False,
- y_range: Optional[Tuple[float, float]] = None,
- last_cross: bool = True,
- bottle: bool = False,
- norm_type: Optional[NormType] = NormType.Batch,
- nf_factor: int = 1,
- **kwargs
- ):
-
- nf = 512 * nf_factor
- extra_bn = norm_type == NormType.Spectral
- imsize = (256, 256)
- sfs_szs = model_sizes(encoder, size=imsize)
- sfs_idxs = list(reversed(_get_sfs_idxs(sfs_szs)))
- self.sfs = hook_outputs([encoder[i] for i in sfs_idxs], detach=False)
- x = dummy_eval(encoder, imsize).detach()
-
- ni = sfs_szs[-1][1]
- middle_conv = nn.Sequential(
- custom_conv_layer(
- ni, ni * 2, norm_type=norm_type, extra_bn=extra_bn, **kwargs
- ),
- custom_conv_layer(
- ni * 2, ni, norm_type=norm_type, extra_bn=extra_bn, **kwargs
- ),
- ).eval()
- x = middle_conv(x)
- layers = [encoder, batchnorm_2d(ni), nn.ReLU(), middle_conv]
-
- for i, idx in enumerate(sfs_idxs):
- not_final = i != len(sfs_idxs) - 1
- up_in_c, x_in_c = int(x.shape[1]), int(sfs_szs[idx][1])
- do_blur = blur and (not_final or blur_final)
- sa = self_attention and (i == len(sfs_idxs) - 3)
-
- n_out = nf if not_final else nf // 2
-
- unet_block = UnetBlockWide(
- up_in_c,
- x_in_c,
- n_out,
- self.sfs[i],
- final_div=not_final,
- blur=blur,
- self_attention=sa,
- norm_type=norm_type,
- extra_bn=extra_bn,
- **kwargs
- ).eval()
- layers.append(unet_block)
- x = unet_block(x)
-
- ni = x.shape[1]
- if imsize != sfs_szs[0][-2:]:
- layers.append(PixelShuffle_ICNR(ni, **kwargs))
- if last_cross:
- layers.append(MergeLayer(dense=True))
- ni += in_channels(encoder)
- layers.append(res_block(ni, bottle=bottle, norm_type=norm_type, **kwargs))
- layers += [
- custom_conv_layer(ni, n_classes, ks=1, use_activ=False, norm_type=norm_type)
- ]
- if y_range is not None:
- layers.append(SigmoidRange(*y_range))
- super().__init__(*layers)
-
- def __del__(self):
- if hasattr(self, "sfs"):
- self.sfs.remove()
diff --git a/spaces/allknowingroger/Image-Models-Test142/app.py b/spaces/allknowingroger/Image-Models-Test142/app.py
deleted file mode 100644
index c1a3df7a0069602edd08cc49c35a6b0c81aa2a95..0000000000000000000000000000000000000000
--- a/spaces/allknowingroger/Image-Models-Test142/app.py
+++ /dev/null
@@ -1,144 +0,0 @@
-import gradio as gr
-# import os
-# import sys
-# from pathlib import Path
-import time
-
-models =[
- "upro/openjourney",
- "miittnnss/cutiee2",
- "Norod78/sxl-laisha-magazine-cover-lora",
- "holtschn/heman-toy-lora-trained-sdxl",
- "midmix/lora-trained-xl",
- "Yntec/HassanBlend12",
- "Adi149/SDXL-Dreambooth",
- "sy-zhang/lora-trained-xl-colab",
- "kear24100712/katherinia123",
-]
-
-
-model_functions = {}
-model_idx = 1
-for model_path in models:
- try:
- model_functions[model_idx] = gr.Interface.load(f"models/{model_path}", live=False, preprocess=True, postprocess=False)
- except Exception as error:
- def the_fn(txt):
- return None
- model_functions[model_idx] = gr.Interface(fn=the_fn, inputs=["text"], outputs=["image"])
- model_idx+=1
-
-
-def send_it_idx(idx):
- def send_it_fn(prompt):
- output = (model_functions.get(str(idx)) or model_functions.get(str(1)))(prompt)
- return output
- return send_it_fn
-
-def get_prompts(prompt_text):
- return prompt_text
-
-def clear_it(val):
- if int(val) != 0:
- val = 0
- else:
- val = 0
- pass
- return val
-
-def all_task_end(cnt,t_stamp):
- to = t_stamp + 60
- et = time.time()
- if et > to and t_stamp != 0:
- d = gr.update(value=0)
- tog = gr.update(value=1)
- #print(f'to: {to} et: {et}')
- else:
- if cnt != 0:
- d = gr.update(value=et)
- else:
- d = gr.update(value=0)
- tog = gr.update(value=0)
- #print (f'passing: to: {to} et: {et}')
- pass
- return d, tog
-
-def all_task_start():
- print("\n\n\n\n\n\n\n")
- t = time.gmtime()
- t_stamp = time.time()
- current_time = time.strftime("%H:%M:%S", t)
- return gr.update(value=t_stamp), gr.update(value=t_stamp), gr.update(value=0)
-
-def clear_fn():
- nn = len(models)
- return tuple([None, *[None for _ in range(nn)]])
-
-
-
-with gr.Blocks(title="SD Models") as my_interface:
- with gr.Column(scale=12):
- # with gr.Row():
- # gr.Markdown("""- Primary prompt: 你想画的内容(英文单词,如 a cat, 加英文逗号效果更好;点 Improve 按钮进行完善)\n- Real prompt: 完善后的提示词,出现后再点右边的 Run 按钮开始运行""")
- with gr.Row():
- with gr.Row(scale=6):
- primary_prompt=gr.Textbox(label="Prompt", value="")
- # real_prompt=gr.Textbox(label="Real prompt")
- with gr.Row(scale=6):
- # improve_prompts_btn=gr.Button("Improve")
- with gr.Row():
- run=gr.Button("Run",variant="primary")
- clear_btn=gr.Button("Clear")
- with gr.Row():
- sd_outputs = {}
- model_idx = 1
- for model_path in models:
- with gr.Column(scale=3, min_width=320):
- with gr.Box():
- sd_outputs[model_idx] = gr.Image(label=model_path)
- pass
- model_idx += 1
- pass
- pass
-
- with gr.Row(visible=False):
- start_box=gr.Number(interactive=False)
- end_box=gr.Number(interactive=False)
- tog_box=gr.Textbox(value=0,interactive=False)
-
- start_box.change(
- all_task_end,
- [start_box, end_box],
- [start_box, tog_box],
- every=1,
- show_progress=False)
-
- primary_prompt.submit(all_task_start, None, [start_box, end_box, tog_box])
- run.click(all_task_start, None, [start_box, end_box, tog_box])
- runs_dict = {}
- model_idx = 1
- for model_path in models:
- runs_dict[model_idx] = run.click(model_functions[model_idx], inputs=[primary_prompt], outputs=[sd_outputs[model_idx]])
- model_idx += 1
- pass
- pass
-
- # improve_prompts_btn_clicked=improve_prompts_btn.click(
- # get_prompts,
- # inputs=[primary_prompt],
- # outputs=[primary_prompt],
- # cancels=list(runs_dict.values()))
- clear_btn.click(
- clear_fn,
- None,
- [primary_prompt, *list(sd_outputs.values())],
- cancels=[*list(runs_dict.values())])
- tog_box.change(
- clear_it,
- tog_box,
- tog_box,
- cancels=[*list(runs_dict.values())])
-
-my_interface.queue(concurrency_count=600, status_update_rate=1)
-my_interface.launch(inline=True, show_api=False)
-
\ No newline at end of file
diff --git a/spaces/amarchheda/ChordDuplicate/portaudio/include/pa_win_wdmks.h b/spaces/amarchheda/ChordDuplicate/portaudio/include/pa_win_wdmks.h
deleted file mode 100644
index bc2f6897c5712be184813ffcfa06244810e66090..0000000000000000000000000000000000000000
--- a/spaces/amarchheda/ChordDuplicate/portaudio/include/pa_win_wdmks.h
+++ /dev/null
@@ -1,137 +0,0 @@
-#ifndef PA_WIN_WDMKS_H
-#define PA_WIN_WDMKS_H
-/*
- * $Id$
- * PortAudio Portable Real-Time Audio Library
- * WDM/KS specific extensions
- *
- * Copyright (c) 1999-2007 Ross Bencina and Phil Burk
- *
- * Permission is hereby granted, free of charge, to any person obtaining
- * a copy of this software and associated documentation files
- * (the "Software"), to deal in the Software without restriction,
- * including without limitation the rights to use, copy, modify, merge,
- * publish, distribute, sublicense, and/or sell copies of the Software,
- * and to permit persons to whom the Software is furnished to do so,
- * subject to the following conditions:
- *
- * The above copyright notice and this permission notice shall be
- * included in all copies or substantial portions of the Software.
- *
- * THE SOFTWARE IS PROVIDED "AS IS", WITHOUT WARRANTY OF ANY KIND,
- * EXPRESS OR IMPLIED, INCLUDING BUT NOT LIMITED TO THE WARRANTIES OF
- * MERCHANTABILITY, FITNESS FOR A PARTICULAR PURPOSE AND NONINFRINGEMENT.
- * IN NO EVENT SHALL THE AUTHORS OR COPYRIGHT HOLDERS BE LIABLE FOR
- * ANY CLAIM, DAMAGES OR OTHER LIABILITY, WHETHER IN AN ACTION OF
- * CONTRACT, TORT OR OTHERWISE, ARISING FROM, OUT OF OR IN CONNECTION
- * WITH THE SOFTWARE OR THE USE OR OTHER DEALINGS IN THE SOFTWARE.
- */
-
-/*
- * The text above constitutes the entire PortAudio license; however,
- * the PortAudio community also makes the following non-binding requests:
- *
- * Any person wishing to distribute modifications to the Software is
- * requested to send the modifications to the original developer so that
- * they can be incorporated into the canonical version. It is also
- * requested that these non-binding requests be included along with the
- * license above.
- */
-
-/** @file
- @ingroup public_header
- @brief WDM Kernel Streaming-specific PortAudio API extension header file.
-*/
-
-
-#include "portaudio.h"
-
-#include
-
-#ifdef __cplusplus
-extern "C"
-{
-#endif /* __cplusplus */
-
- /** Flags to indicate valid fields in PaWinWDMKSInfo.
- @see PaWinWDMKSInfo
- @version Available as of 19.5.0.
- */
- typedef enum PaWinWDMKSFlags
- {
- /** Makes WDMKS use the supplied latency figures instead of relying on the frame size reported
- by the WaveCyclic device. Use at own risk!
- */
- paWinWDMKSOverrideFramesize = (1 << 0),
-
- /** Makes WDMKS (output stream) use the given channelMask instead of the default.
- @version Available as of 19.5.0.
- */
- paWinWDMKSUseGivenChannelMask = (1 << 1),
-
- } PaWinWDMKSFlags;
-
- typedef struct PaWinWDMKSInfo{
- unsigned long size; /**< sizeof(PaWinWDMKSInfo) */
- PaHostApiTypeId hostApiType; /**< paWDMKS */
- unsigned long version; /**< 1 */
-
- /** Flags indicate which fields are valid.
- @see PaWinWDMKSFlags
- @version Available as of 19.5.0.
- */
- unsigned long flags;
-
- /** The number of packets to use for WaveCyclic devices, range is [2, 8]. Set to zero for default value of 2. */
- unsigned noOfPackets;
-
- /** If paWinWDMKSUseGivenChannelMask bit is set in flags, use this as channelMask instead of default.
- @see PaWinWDMKSFlags
- @version Available as of 19.5.0.
- */
- unsigned channelMask;
- } PaWinWDMKSInfo;
-
- typedef enum PaWDMKSType
- {
- Type_kNotUsed,
- Type_kWaveCyclic,
- Type_kWaveRT,
- Type_kCnt,
- } PaWDMKSType;
-
- typedef enum PaWDMKSSubType
- {
- SubType_kUnknown,
- SubType_kNotification,
- SubType_kPolled,
- SubType_kCnt,
- } PaWDMKSSubType;
-
- typedef struct PaWinWDMKSDeviceInfo {
- wchar_t filterPath[MAX_PATH]; /**< KS filter path in Unicode! */
- wchar_t topologyPath[MAX_PATH]; /**< Topology filter path in Unicode! */
- PaWDMKSType streamingType;
- GUID deviceProductGuid; /**< The product GUID of the device (if supported) */
- } PaWinWDMKSDeviceInfo;
-
- typedef struct PaWDMKSDirectionSpecificStreamInfo
- {
- PaDeviceIndex device;
- unsigned channels; /**< No of channels the device is opened with */
- unsigned framesPerHostBuffer; /**< No of frames of the device buffer */
- int endpointPinId; /**< Endpoint pin ID (on topology filter if topologyName is not empty) */
- int muxNodeId; /**< Only valid for input */
- PaWDMKSSubType streamingSubType; /**< Not known until device is opened for streaming */
- } PaWDMKSDirectionSpecificStreamInfo;
-
- typedef struct PaWDMKSSpecificStreamInfo {
- PaWDMKSDirectionSpecificStreamInfo input;
- PaWDMKSDirectionSpecificStreamInfo output;
- } PaWDMKSSpecificStreamInfo;
-
-#ifdef __cplusplus
-}
-#endif /* __cplusplus */
-
-#endif /* PA_WIN_DS_H */
diff --git a/spaces/amarchheda/ChordDuplicate/portaudio/src/hostapi/dsound/pa_win_ds_dynlink.h b/spaces/amarchheda/ChordDuplicate/portaudio/src/hostapi/dsound/pa_win_ds_dynlink.h
deleted file mode 100644
index 2cdf6f032d7e03498734a0b2acc12610c0896126..0000000000000000000000000000000000000000
--- a/spaces/amarchheda/ChordDuplicate/portaudio/src/hostapi/dsound/pa_win_ds_dynlink.h
+++ /dev/null
@@ -1,106 +0,0 @@
-/*
- * Interface for dynamically loading directsound and providing a dummy
- * implementation if it isn't present.
- *
- * Author: Ross Bencina (some portions Phil Burk & Robert Marsanyi)
- *
- * For PortAudio Portable Real-Time Audio Library
- * For more information see: http://www.portaudio.com
- * Copyright (c) 1999-2006 Phil Burk, Robert Marsanyi and Ross Bencina
- *
- * Permission is hereby granted, free of charge, to any person obtaining
- * a copy of this software and associated documentation files
- * (the "Software"), to deal in the Software without restriction,
- * including without limitation the rights to use, copy, modify, merge,
- * publish, distribute, sublicense, and/or sell copies of the Software,
- * and to permit persons to whom the Software is furnished to do so,
- * subject to the following conditions:
- *
- * The above copyright notice and this permission notice shall be
- * included in all copies or substantial portions of the Software.
- *
- * THE SOFTWARE IS PROVIDED "AS IS", WITHOUT WARRANTY OF ANY KIND,
- * EXPRESS OR IMPLIED, INCLUDING BUT NOT LIMITED TO THE WARRANTIES OF
- * MERCHANTABILITY, FITNESS FOR A PARTICULAR PURPOSE AND NONINFRINGEMENT.
- * IN NO EVENT SHALL THE AUTHORS OR COPYRIGHT HOLDERS BE LIABLE FOR
- * ANY CLAIM, DAMAGES OR OTHER LIABILITY, WHETHER IN AN ACTION OF
- * CONTRACT, TORT OR OTHERWISE, ARISING FROM, OUT OF OR IN CONNECTION
- * WITH THE SOFTWARE OR THE USE OR OTHER DEALINGS IN THE SOFTWARE.
- */
-
-/*
- * The text above constitutes the entire PortAudio license; however,
- * the PortAudio community also makes the following non-binding requests:
- *
- * Any person wishing to distribute modifications to the Software is
- * requested to send the modifications to the original developer so that
- * they can be incorporated into the canonical version. It is also
- * requested that these non-binding requests be included along with the
- * license above.
- */
-
-/**
- @file
- @ingroup hostapi_src
-*/
-
-#ifndef INCLUDED_PA_DSOUND_DYNLINK_H
-#define INCLUDED_PA_DSOUND_DYNLINK_H
-
-/* on Borland compilers, WIN32 doesn't seem to be defined by default, which
- breaks dsound.h. Adding the define here fixes the problem. - rossb. */
-#ifdef __BORLANDC__
-#if !defined(WIN32)
-#define WIN32
-#endif
-#endif
-
-/*
- Use the earliest version of DX required, no need to pollute the namespace
-*/
-#ifdef PAWIN_USE_DIRECTSOUNDFULLDUPLEXCREATE
-#define DIRECTSOUND_VERSION 0x0800
-#else
-#define DIRECTSOUND_VERSION 0x0300
-#endif
-#include
-
-#ifdef __cplusplus
-extern "C"
-{
-#endif /* __cplusplus */
-
-
-typedef struct
-{
- HINSTANCE hInstance_;
-
- HRESULT (WINAPI *DllGetClassObject)(REFCLSID , REFIID , LPVOID *);
-
- HRESULT (WINAPI *DirectSoundCreate)(LPGUID, LPDIRECTSOUND *, LPUNKNOWN);
- HRESULT (WINAPI *DirectSoundEnumerateW)(LPDSENUMCALLBACKW, LPVOID);
- HRESULT (WINAPI *DirectSoundEnumerateA)(LPDSENUMCALLBACKA, LPVOID);
-
- HRESULT (WINAPI *DirectSoundCaptureCreate)(LPGUID, LPDIRECTSOUNDCAPTURE *, LPUNKNOWN);
- HRESULT (WINAPI *DirectSoundCaptureEnumerateW)(LPDSENUMCALLBACKW, LPVOID);
- HRESULT (WINAPI *DirectSoundCaptureEnumerateA)(LPDSENUMCALLBACKA, LPVOID);
-
-#ifdef PAWIN_USE_DIRECTSOUNDFULLDUPLEXCREATE
- HRESULT (WINAPI *DirectSoundFullDuplexCreate8)(
- LPCGUID, LPCGUID, LPCDSCBUFFERDESC, LPCDSBUFFERDESC,
- HWND, DWORD, LPDIRECTSOUNDFULLDUPLEX *, LPDIRECTSOUNDCAPTUREBUFFER8 *,
- LPDIRECTSOUNDBUFFER8 *, LPUNKNOWN );
-#endif
-}PaWinDsDSoundEntryPoints;
-
-extern PaWinDsDSoundEntryPoints paWinDsDSoundEntryPoints;
-
-void PaWinDs_InitializeDSoundEntryPoints(void);
-void PaWinDs_TerminateDSoundEntryPoints(void);
-
-
-#ifdef __cplusplus
-}
-#endif /* __cplusplus */
-
-#endif /* INCLUDED_PA_DSOUND_DYNLINK_H */
diff --git a/spaces/aodianyun/ChatGLM-6B/THUDM/chatglm-6b/README.md b/spaces/aodianyun/ChatGLM-6B/THUDM/chatglm-6b/README.md
deleted file mode 100644
index b99938a43777353bb1779b46065c354cc07a08be..0000000000000000000000000000000000000000
--- a/spaces/aodianyun/ChatGLM-6B/THUDM/chatglm-6b/README.md
+++ /dev/null
@@ -1,88 +0,0 @@
----
-language:
-- zh
-- en
-tags:
-- glm
-- chatglm
-- thudm
----
-# ChatGLM-6B
-
- 🌐 Blog • 💻 Github Repo • 🐦 Twitter • 📃 [GLM@ACL 22] [GitHub] • 📃 [GLM-130B@ICLR 23] [GitHub]
-
-
-
- 👋 Join our Slack and WeChat
-
-
-## 介绍
-ChatGLM-6B 是一个开源的、支持中英双语问答的对话语言模型,基于 [General Language Model (GLM)](https://github.com/THUDM/GLM) 架构,具有 62 亿参数。结合模型量化技术,用户可以在消费级的显卡上进行本地部署(INT4 量化级别下最低只需 6GB 显存)。ChatGLM-6B 使用了和 [ChatGLM](https://chatglm.cn) 相同的技术,针对中文问答和对话进行了优化。经过约 1T 标识符的中英双语训练,辅以监督微调、反馈自助、人类反馈强化学习等技术的加持,62 亿参数的 ChatGLM-6B 已经能生成相当符合人类偏好的回答。
-
-ChatGLM-6B is an open bilingual language model based on [General Language Model (GLM)](https://github.com/THUDM/GLM) framework, with 6.2 billion parameters. With the quantization technique, users can deploy locally on consumer-grade graphics cards (only 6GB of GPU memory is required at the INT4 quantization level). ChatGLM-6B uses technology similar to ChatGPT, optimized for Chinese QA and dialogue. The model is trained for about 1T tokens of Chinese and English corpus, supplemented by supervised fine-tuning, feedback bootstrap, and reinforcement learning wit human feedback. With only about 6.2 billion parameters, the model is able to generate answers that are in line with human preference.
-
-## 软件依赖
-
-```shell
-pip install protobuf==3.20.0 transformers==4.27.1 icetk cpm_kernels
-```
-
-## 代码调用
-
-可以通过如下代码调用 ChatGLM-6B 模型来生成对话:
-
-```ipython
->>> from transformers import AutoTokenizer, AutoModel
->>> tokenizer = AutoTokenizer.from_pretrained("THUDM/chatglm-6b", trust_remote_code=True)
->>> model = AutoModel.from_pretrained("THUDM/chatglm-6b", trust_remote_code=True).half().cuda()
->>> response, history = model.chat(tokenizer, "你好", history=[])
->>> print(response)
-你好👋!我是人工智能助手 ChatGLM-6B,很高兴见到你,欢迎问我任何问题。
->>> response, history = model.chat(tokenizer, "晚上睡不着应该怎么办", history=history)
->>> print(response)
-晚上睡不着可能会让你感到焦虑或不舒服,但以下是一些可以帮助你入睡的方法:
-
-1. 制定规律的睡眠时间表:保持规律的睡眠时间表可以帮助你建立健康的睡眠习惯,使你更容易入睡。尽量在每天的相同时间上床,并在同一时间起床。
-2. 创造一个舒适的睡眠环境:确保睡眠环境舒适,安静,黑暗且温度适宜。可以使用舒适的床上用品,并保持房间通风。
-3. 放松身心:在睡前做些放松的活动,例如泡个热水澡,听些轻柔的音乐,阅读一些有趣的书籍等,有助于缓解紧张和焦虑,使你更容易入睡。
-4. 避免饮用含有咖啡因的饮料:咖啡因是一种刺激性物质,会影响你的睡眠质量。尽量避免在睡前饮用含有咖啡因的饮料,例如咖啡,茶和可乐。
-5. 避免在床上做与睡眠无关的事情:在床上做些与睡眠无关的事情,例如看电影,玩游戏或工作等,可能会干扰你的睡眠。
-6. 尝试呼吸技巧:深呼吸是一种放松技巧,可以帮助你缓解紧张和焦虑,使你更容易入睡。试着慢慢吸气,保持几秒钟,然后缓慢呼气。
-
-如果这些方法无法帮助你入睡,你可以考虑咨询医生或睡眠专家,寻求进一步的建议。
-```
-
-关于更多的使用说明,包括如何运行命令行和网页版本的 DEMO,以及使用模型量化以节省显存,请参考我们的 [Github Repo](https://github.com/THUDM/ChatGLM-6B)。
-
-For more instructions, including how to run CLI and web demos, and model quantization, please refer to our [Github Repo](https://github.com/THUDM/ChatGLM-6B).
-
-## Change Log
-* v0.1.0 ([f83182](https://huggingface.co/THUDM/chatglm-6b/commit/f83182484538e663a03d3f73647f10f89878f438))
-
-## 协议
-
-本仓库的代码依照 [Apache-2.0](LICENSE) 协议开源,ChatGLM-6B 模型的权重的使用则需要遵循 [Model License](MODEL_LICENSE)。
-
-## 引用
-
-如果你觉得我们的工作有帮助的话,请考虑引用下列论文:
-
-```
-@inproceedings{
- zeng2023glm-130b,
- title={{GLM}-130B: An Open Bilingual Pre-trained Model},
- author={Aohan Zeng and Xiao Liu and Zhengxiao Du and Zihan Wang and Hanyu Lai and Ming Ding and Zhuoyi Yang and Yifan Xu and Wendi Zheng and Xiao Xia and Weng Lam Tam and Zixuan Ma and Yufei Xue and Jidong Zhai and Wenguang Chen and Zhiyuan Liu and Peng Zhang and Yuxiao Dong and Jie Tang},
- booktitle={The Eleventh International Conference on Learning Representations (ICLR)},
- year={2023},
- url={https://openreview.net/forum?id=-Aw0rrrPUF}
-}
-```
-```
-@inproceedings{du2022glm,
- title={GLM: General Language Model Pretraining with Autoregressive Blank Infilling},
- author={Du, Zhengxiao and Qian, Yujie and Liu, Xiao and Ding, Ming and Qiu, Jiezhong and Yang, Zhilin and Tang, Jie},
- booktitle={Proceedings of the 60th Annual Meeting of the Association for Computational Linguistics (Volume 1: Long Papers)},
- pages={320--335},
- year={2022}
-}
-```
\ No newline at end of file
diff --git a/spaces/apsys/hetfit/nets/__init__.py b/spaces/apsys/hetfit/nets/__init__.py
deleted file mode 100644
index e69de29bb2d1d6434b8b29ae775ad8c2e48c5391..0000000000000000000000000000000000000000
diff --git a/spaces/arjunpatel/best-selling-video-games/README.md b/spaces/arjunpatel/best-selling-video-games/README.md
deleted file mode 100644
index 5c16bbcad523f0ee990a33723a2fc8c2fc770e2b..0000000000000000000000000000000000000000
--- a/spaces/arjunpatel/best-selling-video-games/README.md
+++ /dev/null
@@ -1,12 +0,0 @@
----
-title: Best Selling Video Games
-emoji: 🦀
-colorFrom: indigo
-colorTo: pink
-sdk: gradio
-sdk_version: 3.12.0
-app_file: app.py
-pinned: false
----
-
-Check out the configuration reference at https://huggingface.co/docs/hub/spaces-config-reference
diff --git a/spaces/artificialguybr/video-dubbing/TTS/tests/tts_tests2/__init__.py b/spaces/artificialguybr/video-dubbing/TTS/tests/tts_tests2/__init__.py
deleted file mode 100644
index e69de29bb2d1d6434b8b29ae775ad8c2e48c5391..0000000000000000000000000000000000000000
diff --git a/spaces/arxify/RVC-beta-v2-0618/runtime/Lib/site-packages/altair/examples/choropleth_repeat.py b/spaces/arxify/RVC-beta-v2-0618/runtime/Lib/site-packages/altair/examples/choropleth_repeat.py
deleted file mode 100644
index 3cd913ec4872465964639eefebde3d5763de57c1..0000000000000000000000000000000000000000
--- a/spaces/arxify/RVC-beta-v2-0618/runtime/Lib/site-packages/altair/examples/choropleth_repeat.py
+++ /dev/null
@@ -1,28 +0,0 @@
-"""
-Repeated Choropleth Map
-=======================
-Three choropleths representing disjoint data from the same table.
-"""
-# category: maps
-import altair as alt
-from vega_datasets import data
-
-states = alt.topo_feature(data.us_10m.url, 'states')
-source = data.population_engineers_hurricanes.url
-variable_list = ['population', 'engineers', 'hurricanes']
-
-alt.Chart(states).mark_geoshape().encode(
- alt.Color(alt.repeat('row'), type='quantitative')
-).transform_lookup(
- lookup='id',
- from_=alt.LookupData(source, 'id', variable_list)
-).properties(
- width=500,
- height=300
-).project(
- type='albersUsa'
-).repeat(
- row=variable_list
-).resolve_scale(
- color='independent'
-)
diff --git a/spaces/arxify/RVC-beta-v2-0618/runtime/Lib/site-packages/colorama/__init__.py b/spaces/arxify/RVC-beta-v2-0618/runtime/Lib/site-packages/colorama/__init__.py
deleted file mode 100644
index 383101cdb38706c305449674044e9288b92b7d75..0000000000000000000000000000000000000000
--- a/spaces/arxify/RVC-beta-v2-0618/runtime/Lib/site-packages/colorama/__init__.py
+++ /dev/null
@@ -1,7 +0,0 @@
-# Copyright Jonathan Hartley 2013. BSD 3-Clause license, see LICENSE file.
-from .initialise import init, deinit, reinit, colorama_text, just_fix_windows_console
-from .ansi import Fore, Back, Style, Cursor
-from .ansitowin32 import AnsiToWin32
-
-__version__ = '0.4.6'
-
diff --git a/spaces/awacke1/1-SimPhysics/index.html b/spaces/awacke1/1-SimPhysics/index.html
deleted file mode 100644
index ff622cd7da790178304c317a45396d8dd22d5c2f..0000000000000000000000000000000000000000
--- a/spaces/awacke1/1-SimPhysics/index.html
+++ /dev/null
@@ -1,12 +0,0 @@
-
-
-SimPhysics
-User input: WASD
-This WebGL demo demonstrates PlayCanvas and a physics vehicle simulation that is web based and playable anywhere your browser goes🤗 Inference API.
-Source code is in Readme.md file.
-PlayCanvas project is here
-
-
-
\ No newline at end of file
diff --git a/spaces/awacke1/BERTopic-Topic-Modeler-NLP-ML/app.py b/spaces/awacke1/BERTopic-Topic-Modeler-NLP-ML/app.py
deleted file mode 100644
index 6374477f04cf608d8df00be4f90f7f3fbac8d888..0000000000000000000000000000000000000000
--- a/spaces/awacke1/BERTopic-Topic-Modeler-NLP-ML/app.py
+++ /dev/null
@@ -1,156 +0,0 @@
-from bertopic import BERTopic
-import streamlit as st
-import streamlit.components.v1 as components
-from datasets import load_dataset
-import pandas as pd
-from sentence_transformers import SentenceTransformer
-from umap import UMAP
-from hdbscan import HDBSCAN
-from sklearn.feature_extraction.text import CountVectorizer
-
-st.set_page_config(page_title="HF-BERTopic")
-form = st.sidebar.form("Main Settings")
-form.header("Main Settings")
-dataset_name = form.text_area("Enter the name of the huggingface dataset to do analysis of:", value = "Hellisotherpeople/DebateSum")
-dataset_name_2 = form.text_area("Enter the name of the config for the dataset if it has one", value = "")
-
-split_name = form.text_area("Enter the name of the split of the dataset that you want to use", value = "train")
-
-number_of_records = form.number_input("Enter the number of documents that you want to analyze from the dataset", value = 10)
-
-column_name = form.text_area("Enter the name of the column that we are doing analysis on (the X value)", value = "Full-Document")
-
-labels = form.checkbox("Does this dataset have labels that you want to use?", value = True)
-
-if labels == True:
- labels_column_name = form.text_area("Enter the name of the column that we are using for labels doing analysis on (the Y value)", value = "OriginalDebateFileName")
-
-model_name = form.text_area("Enter the name of the pre-trained model from sentence transformers that we are using for featurization", value = "all-MiniLM-L6-v2")
-form.caption("This will download a new model, so it may take awhile or even break if the model is too large")
-form.caption("See the list of pre-trained models that are available here! https://www.sbert.net/docs/pretrained_models.html")
-
-form.header("BERTopic Settings")
-use_topic_reduction = form.selectbox("How do you want to handle topic reduction", ["HDBScan", "Auto", "Manual"])
-form.caption("Leave this if you want HDBScan to choose the number of topics (clusters) for you. Set to Auto to have BERTopic prune these topics further, set to Manual to specify the number yourself")
-number_of_topics = form.number_input("Enter the number of topics to use if doing Manual topic reduction", value = 4)
-use_random_seed = form.checkbox("Do you want to make the results reproducible? This significantly slows down BERTopic", value = False)
-
-form.header("CounterVectorizer Settings")
-cv_lowercase = form.checkbox("Shall we automatically lowercase the text?", value = True)
-cv_ngram_min = form.number_input("What's the lower boundary of the range of n-values for different word n-grams or char n-grams to be extracted", value = 1)
-cv_ngram_max = form.number_input("What's the upper boundary of the range of n-values for different word n-grams or char n-grams to be extracted", value = 1)
-form.caption("Set the lower and upper boundry to 1 if you want the topics to be at the word level only (unigrams)")
-cv_analyzer = form.selectbox("Enter the analyzer mode:", ["word", "char", "char_wb"])
-form.caption("This selects between looking at n-grams, character n-grams, and character n-grams only from text within word boundries.")
-cv_max_df = form.number_input("Ignore terms that have a document frequency strictly higher than the given threshold", value = 1.0)
-form.caption("This parameter represents a proportion of documents if a float is given")
-cv_min_df = form.number_input("Ignore terms that have a document frequency strictly lower than the given threshold", value = 0.5)
-form.caption("This parameter represents a proportion of documents if a float is given")
-cv_max_features = form.number_input("Enter the maximum number of n-grams to be featurized", value = 100000)
-
-form.header("HDBScan Settings")
-hdbscan_min_cluster_size = form.number_input("Enter the number of points necessary to form a new cluster", value = 3)
-form.caption("Set it to the smallest size grouping that you wish to consider a cluster. This is the most impactful setting for HDBscan")
-hdbscan_min_samples = form.number_input("Enter the minimum number of points to be declared a cluster instead of noise", value = 2)
-form.caption("The larger the value of min_samples you provide, the more conservative the clustering – more points will be declared as noise, and clusters will be restricted to progressively more dense areas.")
-hdbscan_metric = form.text_area("Enter the name of the metric used for computing distances for HDBscan. Common metrics for NLP are euclidean and cosine. Cosine is not supported by HDBscan", value = "euclidean")
-
-form.header("UMAP Settings")
-umap_n_neighbors = form.number_input("Enter the number of neighbors used by UMAP for generating its manifold", value = 15)
-form.caption("This parameter controls how UMAP balances local versus global structure in the data. It does this by constraining the size of the local neighborhood UMAP will look at when attempting to learn the manifold structure of the data. This means that low values of n_neighbors will force UMAP to concentrate on very local structure (potentially to the detriment of the big picture), while large values will push UMAP to look at larger neighborhoods of each point when estimating the manifold structure of the data, losing fine detail structure for the sake of getting the broader of the data.")
-umap_min_dist = form.number_input("Enter the minimum distance that points are allowed to be apart in the low dimensional manifold", value = 0.1)
-form.caption("The min_dist parameter controls how tightly UMAP is allowed to pack points together. It, quite literally, provides the minimum distance apart that points are allowed to be in the low dimensional representation. This means that low values of min_dist will result in clumpier embeddings. This can be useful if you are interested in clustering, or in finer topological structure. Larger values of min_dist will prevent UMAP from packing points together and will focus on the preservation of the broad topological structure instead.")
-umap_n_components = form.number_input("Enter the number of dimensions/components for UMAP to create", value = 2)
-form.caption("UMAP provides a n_components parameter option that allows the user to determine the dimensionality of the reduced dimension space we will be embedding the data into. Unlike some other visualisation algorithms such as t-SNE, UMAP scales well in the embedding dimension, so you can use it for more than just visualisation in 2- or 3-dimensions.")
-form.caption("UMAP is used in BERTopic primarily to allow the highly effective clustering algorithm, HDBScan, to effectively cluster effectively because HDBScan becomes extremely slow on high dimensional data. Setting this value above 10 may slow the analysis to a crawl")
-umap_metric = form.text_area("Enter the name of the metric used for computing distances. Common metrics for NLP are euclidean and cosine", value = "cosine")
-form.caption("A complete list of all available metrics supported by UMAP can be found here: https://umap-learn.readthedocs.io/en/latest/parameters.html#metric")
-
-form.form_submit_button("Submit")
-
-@st.cache
-def load_and_process_data(path, name, streaming, split_name, number_of_records):
- dataset = load_dataset(path = path, name = name, streaming=streaming)
- #return list(dataset)
- dataset_head = dataset[split_name].take(number_of_records)
- df = pd.DataFrame.from_dict(dataset_head)
- return df
-
-hdbscan_model = HDBSCAN(min_cluster_size=hdbscan_min_cluster_size, min_samples = hdbscan_min_samples, metric=hdbscan_metric, prediction_data=True)
-if use_random_seed:
- umap_model = UMAP(n_neighbors=umap_n_neighbors, n_components=umap_n_components, min_dist=umap_min_dist, metric=umap_metric, random_state = 42)
-else:
- umap_model = UMAP(n_neighbors=umap_n_neighbors, n_components=umap_n_components, min_dist=umap_min_dist, metric=umap_metric)
-vectorizer_model = CountVectorizer(lowercase = cv_lowercase, ngram_range=(cv_ngram_min, cv_ngram_max), analyzer=cv_analyzer, max_df=cv_max_df, min_df=cv_min_df, stop_words="english")
-
-@st.cache(allow_output_mutation=True)
-def load_model(model_name, hdbscan_model=hdbscan_model, umap_model=umap_model, vectorizer_model=vectorizer_model, use_topic_reduction = use_topic_reduction, number_of_topics = number_of_topics):
- sentence_model = SentenceTransformer(model_name)
- if use_topic_reduction == "Auto":
- kw_model = BERTopic(embedding_model=sentence_model, umap_model = umap_model, hdbscan_model = hdbscan_model, vectorizer_model = vectorizer_model, nr_topics = "auto", calculate_probabilities = True)
- elif use_topic_reduction == "Manual":
- kw_model = BERTopic(embedding_model=sentence_model, umap_model = umap_model, hdbscan_model = hdbscan_model, vectorizer_model = vectorizer_model, nr_topics = number_of_topics, calculate_probabilities = True)
- else:
- kw_model = BERTopic(embedding_model=sentence_model, umap_model = umap_model, hdbscan_model = hdbscan_model, vectorizer_model = vectorizer_model, calculate_probabilities = True)
- return kw_model
-
-@st.cache()
-def fit_transform(model, docs):
- topics, probs = model.fit_transform(docs)
- return topics, probs
-
-model = load_model(model_name=model_name)
-df = load_and_process_data(dataset_name, dataset_name_2, True, split_name, number_of_records)
-X = df[column_name]
-st.header("Original Dataset")
-st.write(df)
-
-topics, probs = fit_transform(model, X)
-
-st.header("Topic assignment for each example")
-st.write(topics)
-st.header("Topic assignment probability for each example")
-st.write(probs)
-topic_info = model.get_topic_info()
-st.header("Topic Info")
-st.write(topic_info)
-st.header("Detailed Topic Info")
-topic_info_list = []
-with st.expander("Open to see Detailed Topic Info:"):
- for xhat in range(-1, len(topic_info)):
- topic_info_list.append(model.get_topic(xhat))
- st.write(topic_info_list)
-st.header("Representitive docs for each topic")
-with st.expander("Open to see Representitive docs for each topic"):
- rep_doc_list = []
- for yhat in range(0, len(topic_info)-1):
- rep_doc_list.append(model.get_representative_docs(topic = yhat))
- st.write(rep_doc_list)
-if labels:
- y = df[labels_column_name]
- st.header("Topics per class")
- topics_per_class = model.topics_per_class(X, classes=y)
- st.plotly_chart(model.visualize_topics_per_class(topics_per_class))
-
-st.header("Visualizations")
-
-
-st.plotly_chart(model.visualize_topics())
-st.plotly_chart(model.visualize_barchart(top_n_topics = 9990, n_words = 9999))
-st.plotly_chart(model.visualize_heatmap())
-st.plotly_chart(model.visualize_hierarchy())
-st.plotly_chart(model.visualize_term_rank())
-
-st.header("Prediction on unseen data")
-
-unseen_doc_txt = """
-Police in southern China paraded four suspects through the streets for allegedly smuggling people across sealed borders in breach of pandemic control measures -- a controversial act of public shaming that triggered backlash on Chinese social media.
-On Tuesday, four people wearing hazmat suits, face masks and goggles were paraded in Jingxi city, Guangxi province -- each carrying placards showing their names and photos on their chest and back, according to videos shared on social media and republished by state media outlets.
-Each suspect was held by two officers -- also wearing hazmat suits and face shields. They were surrounded by yet another circle of police, some holding machine guns and in riot gear, while a large crowd looked on.
-The four people were suspected of helping others to illegally cross China's borders, which have been largely sealed during the pandemic as part of the country's "zero-Covid policy," according to the state-run Guangxi Daily,
-"""
-unseen_doc = st.text_area("Enter an unseen document to perform topic modeling on using the trained model", value = unseen_doc_txt)
-
-new_topic, new_probs = model.transform(unseen_doc)
-st.write(new_topic)
-st.plotly_chart(model.visualize_distribution(new_probs[0], min_probability = 0.0)) #### do on an unseen document
\ No newline at end of file
diff --git a/spaces/awacke1/MN.Map.Hospitals.Top.Five/app.py b/spaces/awacke1/MN.Map.Hospitals.Top.Five/app.py
deleted file mode 100644
index dbab3e6ad91e1cb5187378103e3649ba2c56c2bf..0000000000000000000000000000000000000000
--- a/spaces/awacke1/MN.Map.Hospitals.Top.Five/app.py
+++ /dev/null
@@ -1,48 +0,0 @@
-import streamlit as st
-import folium
-from streamlit_folium import folium_static
-import pandas as pd
-
-# Define hospitals data for Minnesota
-hospitals = pd.DataFrame({
- 'name': ['Mayo Clinic', 'University of Minnesota Medical Center', 'Hennepin County Medical Center',
- 'Regions Hospital', 'Abbott Northwestern Hospital'],
- 'city': ['Rochester', 'Minneapolis', 'Minneapolis', 'St. Paul', 'Minneapolis'],
- 'lat': [44.023678, 44.971389, 44.972078, 44.942936, 44.955447],
- 'lon': [-92.466955, -93.240556, -93.261769, -93.093457, -93.268543],
- 'beds': [1368, 1002, 484, 454, 640]
-})
-
-# Sort hospitals by number of beds and select the top ten
-top_hospitals = hospitals.sort_values('beds', ascending=False).head(10)
-
-# Create a map centered on Minnesota
-m = folium.Map(location=[45.0, -94.0], zoom_start=7)
-
-# Add markers for each hospital
-for i, hospital in top_hospitals.iterrows():
- folium.Marker(
- location=[hospital['lat'], hospital['lon']],
- popup=f"{hospital['name']}
{hospital['city']}
{hospital['beds']} beds",
- icon=folium.Icon(color='red')
- ).add_to(m)
-
-# Add waypoints for each hospital
-waypoints = [(hospital['lat'], hospital['lon']) for i, hospital in top_hospitals.iterrows()]
-folium.plugins.AntPath(waypoints, delay=3000).add_to(m)
-
-# Function to update the map when a button is clicked
-def update_map(hospital_data):
- m.location = [hospital_data['lat'], hospital_data['lon']]
- m.zoom_start = 13
- folium_static(m)
-
-# Create a grid of buttons for selecting hospitals
-cols = st.columns(5)
-for i, hospital in top_hospitals.iterrows():
- with cols[i % 5]:
- if st.button(hospital['name']):
- update_map(hospital)
-
-# Display the map in Streamlit
-folium_static(m)
diff --git a/spaces/awacke1/StreamlitDealOrNoDeal/app.py b/spaces/awacke1/StreamlitDealOrNoDeal/app.py
deleted file mode 100644
index af6d8ef1251888988773eea360aa060f2b0f5847..0000000000000000000000000000000000000000
--- a/spaces/awacke1/StreamlitDealOrNoDeal/app.py
+++ /dev/null
@@ -1,94 +0,0 @@
-# write a python streamlit game with the rules of deal or no deal. Use session state to save values and a scoreboard to a CSV file. Allow users to save the game.
-
-import streamlit as st
-import pandas as pd
-import random
-
-# Set the session state
-st.set_page_config(page_title="Deal or No Deal")
-st.set_option('deprecation.showPyplotGlobalUse', False)
-
-# Create the scoreboard
-scoreboard = pd.DataFrame(columns=['Name', 'Score'])
-
-# Create the game page
-def game_page():
- # Get the player's name
- name = st.text_input('What is your name?')
-
- # Create the game
- st.header('Welcome to Deal or No Deal!')
- st.write('Your goal is to select boxes with dollar amounts and try to get the highest score. Good luck!')
-
- # Set the initial values
- num_rounds = 5
- round_num = 0
- total_score = 0
- boxes = [1,2,3,4,5,6,7,8,9,10,11,12,13,14,15,16,17,18,19,20]
- box_values = [1,5,10,25,50,75,100,150,200,250,500,750,1000,1500,2000,2500,5000,7500,10000,25000]
- random.shuffle(box_values)
- st.write('There are ' + str(num_rounds) + ' rounds. You will pick ' + str(len(boxes)) + ' boxes.')
- st.write('Round ' + str(round_num + 1) + ': Select a box to open')
-
- # Create the boxes
- for i in range(len(boxes)):
- if boxes[i] in boxes:
- st.write('Box ' + str(boxes[i]) + ' : $' + str(box_values[i]))
- else:
- boxes.remove(i)
-
- # Get the player's selection
- selection = st.selectbox('Which box would you like to open?', boxes)
- box_value = box_values[boxes.index(selection)]
- st.write('You have selected Box ' + str(selection) + ', which contains $' + str(box_value) + '.')
-
- # Get the player's decision
- decision = st.radio('Do you want to keep the box or trade it for the banker\'s offer?', ('Keep', 'Trade'))
-
- # Calculate the score
- if decision == 'Keep':
- total_score += box_value
- st.write('You have kept the box and earned $' + str(box_value) + '.')
- else:
- offer = random.randint(1,100)
- st.write('The banker has offered you $' + str(offer) + '.')
- decision = st.radio('Do you want to accept the offer or reject it?', ('Accept', 'Reject'))
- if decision == 'Accept':
- total_score += offer
- st.write('You have accepted the offer and earned $' + str(offer) + '.')
- else:
- total_score += box_value
- st.write('You have rejected the offer and kept your box, earning $' + str(box_value) + '.')
-
- # Update the round number
- round_num += 1
-
- # Check if the game is over
- if round_num < num_rounds:
- st.write('Your total score is $' + str(total_score) + '.')
- st.write('Round ' + str(round_num + 1) + ': Select a box to open')
- game_page()
- else:
- st.write('Congratulations, you have finished the game with a total score of $' + str(total_score) + '!')
-
- # Save the player's score to the scoreboard
- scoreboard.loc[len(scoreboard)] = [name, total_score]
-
- # Save the scoreboard to a CSV file
- st.write('Saving your score...')
- scoreboard.to_csv('scoreboard.csv', index=False)
- st.write('Score saved!')
-
-# Create the main page
-def main():
- st.title('Deal or No Deal')
- # Show the scoreboard
- st.header('Scoreboard')
- st.dataframe(scoreboard)
-
- # Show the game page
- st.header('Game')
- if st.button('Start Game'):
- game_page()
-
-main()
\ No newline at end of file
diff --git a/spaces/awacke1/StreamlitEmotionWheelSunburst/app.py b/spaces/awacke1/StreamlitEmotionWheelSunburst/app.py
deleted file mode 100644
index 62d326e8bdd1c3c86c37da260fd3fc71b253ff85..0000000000000000000000000000000000000000
--- a/spaces/awacke1/StreamlitEmotionWheelSunburst/app.py
+++ /dev/null
@@ -1,35 +0,0 @@
-# ChatGPT Prompt: write a streamlit program that generates a graphical emotion wheel that can animate a spin. With the animated emotion wheel, display it as a pie graph sunburst plot with an emoji for each of the emotions including: love, fear, anger, sadness, happiness, surprise, disgust, and love.
-
-import streamlit as st
-import pandas as pd
-import numpy as np
-import plotly.express as px
-import matplotlib.pyplot as plt
-import seaborn as sns
-
-# Create an emotion wheel
-emotions = ["Love", "Fear", "Anger", "Sadness", "Happiness", "Surprise", "Disgust", "Love"]
-#emoji = ["❤️", "😱", "😠", "😢", "🤩", "😮", "🤢", "❤️"]
-emoji = [1, 2, 3, 4, 5, 6, 7, 8]
-
-# Add the wheel to the sidebar
-st.sidebar.title("Emotion Wheel")
-st.sidebar.radio("Choose an emotion", emotions)
-
-# Create a sunburst chart
-df = pd.DataFrame({"emotion": emotions, "emoji": emoji})
-fig = px.sunburst(df, values="emoji", path=["emotion"], color="emoji")
-
-# Plot the chart
-st.plotly_chart(fig, use_container_width=True)
-
-# Add an animation button
-animation_button = st.button("Animate")
-
-# Animate the chart
-if animation_button:
- for i in range(10):
- random_emotion = np.random.choice(emotions)
- st.sidebar.radio("Choose an emotion", [random_emotion])
- fig = px.sunburst(df, values="emoji", path=["emotion"], color="emoji")
- st.plotly_chart(fig, use_container_width=True)
\ No newline at end of file
diff --git a/spaces/awacke1/Zero-shot-classification-facebook-bart-large-mnli/README.md b/spaces/awacke1/Zero-shot-classification-facebook-bart-large-mnli/README.md
deleted file mode 100644
index 4ac75b8fd8b1f1ed5c451070550d3899b91679d3..0000000000000000000000000000000000000000
--- a/spaces/awacke1/Zero-shot-classification-facebook-bart-large-mnli/README.md
+++ /dev/null
@@ -1,13 +0,0 @@
----
-title: Zero Shot Classification Facebook Bart Large Mnli
-emoji: 🚀
-colorFrom: blue
-colorTo: pink
-sdk: gradio
-sdk_version: 3.19.1
-app_file: app.py
-pinned: false
-license: mit
----
-
-Check out the configuration reference at https://huggingface.co/docs/hub/spaces-config-reference
\ No newline at end of file
diff --git a/spaces/beihai/GFPGAN-V1.3-whole-image/basicsr/metrics/fid.py b/spaces/beihai/GFPGAN-V1.3-whole-image/basicsr/metrics/fid.py
deleted file mode 100644
index 2b926eb38418d62ec69fe2feb10d2a9231cbc181..0000000000000000000000000000000000000000
--- a/spaces/beihai/GFPGAN-V1.3-whole-image/basicsr/metrics/fid.py
+++ /dev/null
@@ -1,93 +0,0 @@
-import numpy as np
-import torch
-import torch.nn as nn
-from scipy import linalg
-from tqdm import tqdm
-
-from basicsr.archs.inception import InceptionV3
-
-
-def load_patched_inception_v3(device='cuda', resize_input=True, normalize_input=False):
- # we may not resize the input, but in [rosinality/stylegan2-pytorch] it
- # does resize the input.
- inception = InceptionV3([3], resize_input=resize_input, normalize_input=normalize_input)
- inception = nn.DataParallel(inception).eval().to(device)
- return inception
-
-
-@torch.no_grad()
-def extract_inception_features(data_generator, inception, len_generator=None, device='cuda'):
- """Extract inception features.
-
- Args:
- data_generator (generator): A data generator.
- inception (nn.Module): Inception model.
- len_generator (int): Length of the data_generator to show the
- progressbar. Default: None.
- device (str): Device. Default: cuda.
-
- Returns:
- Tensor: Extracted features.
- """
- if len_generator is not None:
- pbar = tqdm(total=len_generator, unit='batch', desc='Extract')
- else:
- pbar = None
- features = []
-
- for data in data_generator:
- if pbar:
- pbar.update(1)
- data = data.to(device)
- feature = inception(data)[0].view(data.shape[0], -1)
- features.append(feature.to('cpu'))
- if pbar:
- pbar.close()
- features = torch.cat(features, 0)
- return features
-
-
-def calculate_fid(mu1, sigma1, mu2, sigma2, eps=1e-6):
- """Numpy implementation of the Frechet Distance.
-
- The Frechet distance between two multivariate Gaussians X_1 ~ N(mu_1, C_1)
- and X_2 ~ N(mu_2, C_2) is
- d^2 = ||mu_1 - mu_2||^2 + Tr(C_1 + C_2 - 2*sqrt(C_1*C_2)).
- Stable version by Dougal J. Sutherland.
-
- Args:
- mu1 (np.array): The sample mean over activations.
- sigma1 (np.array): The covariance matrix over activations for
- generated samples.
- mu2 (np.array): The sample mean over activations, precalculated on an
- representative data set.
- sigma2 (np.array): The covariance matrix over activations,
- precalculated on an representative data set.
-
- Returns:
- float: The Frechet Distance.
- """
- assert mu1.shape == mu2.shape, 'Two mean vectors have different lengths'
- assert sigma1.shape == sigma2.shape, ('Two covariances have different dimensions')
-
- cov_sqrt, _ = linalg.sqrtm(sigma1 @ sigma2, disp=False)
-
- # Product might be almost singular
- if not np.isfinite(cov_sqrt).all():
- print('Product of cov matrices is singular. Adding {eps} to diagonal of cov estimates')
- offset = np.eye(sigma1.shape[0]) * eps
- cov_sqrt = linalg.sqrtm((sigma1 + offset) @ (sigma2 + offset))
-
- # Numerical error might give slight imaginary component
- if np.iscomplexobj(cov_sqrt):
- if not np.allclose(np.diagonal(cov_sqrt).imag, 0, atol=1e-3):
- m = np.max(np.abs(cov_sqrt.imag))
- raise ValueError(f'Imaginary component {m}')
- cov_sqrt = cov_sqrt.real
-
- mean_diff = mu1 - mu2
- mean_norm = mean_diff @ mean_diff
- trace = np.trace(sigma1) + np.trace(sigma2) - 2 * np.trace(cov_sqrt)
- fid = mean_norm + trace
-
- return fid
diff --git a/spaces/bingbing520/ChatGPT/locale/extract_locale.py b/spaces/bingbing520/ChatGPT/locale/extract_locale.py
deleted file mode 100644
index 32b0924bd6dffe150cb3e481ddadef836b91b83c..0000000000000000000000000000000000000000
--- a/spaces/bingbing520/ChatGPT/locale/extract_locale.py
+++ /dev/null
@@ -1,26 +0,0 @@
-import os
-import json
-import re
-
-# Define regular expression patterns
-pattern = r'i18n\((\"{3}.*?\"{3}|\".*?\")\)'
-
-# Load the .py file
-with open('ChuanhuChatbot.py', 'r', encoding='utf-8') as f:
- contents = f.read()
-
-# Load the .py files in the modules folder
-for filename in os.listdir("modules"):
- if filename.endswith(".py"):
- with open(os.path.join("modules", filename), "r", encoding="utf-8") as f:
- contents += f.read()
-
-# Matching with regular expressions
-matches = re.findall(pattern, contents, re.DOTALL)
-
-# Convert to key/value pairs
-data = {match.strip('()"'): '' for match in matches}
-
-# Save as a JSON file
-with open('labels.json', 'w', encoding='utf-8') as f:
- json.dump(data, f, ensure_ascii=False, indent=4)
\ No newline at end of file
diff --git a/spaces/bioriAsaeru/text-to-voice/Download Terjemah Kitab Maroqil Ubudiyah Pdfl ((INSTALL)).md b/spaces/bioriAsaeru/text-to-voice/Download Terjemah Kitab Maroqil Ubudiyah Pdfl ((INSTALL)).md
deleted file mode 100644
index 6e737a44ebc5996a31cec2456bee3d6e314d24ae..0000000000000000000000000000000000000000
--- a/spaces/bioriAsaeru/text-to-voice/Download Terjemah Kitab Maroqil Ubudiyah Pdfl ((INSTALL)).md
+++ /dev/null
@@ -1,6 +0,0 @@
-Download Terjemah Kitab Maroqil Ubudiyah Pdfl
Download Zip >>>>> https://urloso.com/2uyOhM
-
- 3cee63e6c2
-
-
-
diff --git a/spaces/bioriAsaeru/text-to-voice/Ea Sports Cricket 2012 Patch By !!!Midnitestar!!! Pc Game REPACK.md b/spaces/bioriAsaeru/text-to-voice/Ea Sports Cricket 2012 Patch By !!!Midnitestar!!! Pc Game REPACK.md
deleted file mode 100644
index 5938d724183ddd6c5b7a751a23ac2b923f688c4d..0000000000000000000000000000000000000000
--- a/spaces/bioriAsaeru/text-to-voice/Ea Sports Cricket 2012 Patch By !!!Midnitestar!!! Pc Game REPACK.md
+++ /dev/null
@@ -1,6 +0,0 @@
-Ea Sports Cricket 2012 Patch By ~!!!Midnitestar!!!~ Pc Game
DOWNLOAD > https://urloso.com/2uyOQ1
-
-Type: Cricket Game patch Comatible With: PC Uploader & Ripper: Midnitestar Posted on: 26/5/2012 Patch Name: EA Sports Cricket 2012 Size:Â ... 4d29de3e1b
-
-
-
diff --git a/spaces/brainblow/AudioCreator_Music-Audio_Generation/audiocraft/quantization/vq.py b/spaces/brainblow/AudioCreator_Music-Audio_Generation/audiocraft/quantization/vq.py
deleted file mode 100644
index aa57bea59db95ddae35e0657f723ca3a29ee943b..0000000000000000000000000000000000000000
--- a/spaces/brainblow/AudioCreator_Music-Audio_Generation/audiocraft/quantization/vq.py
+++ /dev/null
@@ -1,115 +0,0 @@
-# Copyright (c) Meta Platforms, Inc. and affiliates.
-# All rights reserved.
-#
-# This source code is licensed under the license found in the
-# LICENSE file in the root directory of this source tree.
-
-import math
-import typing as tp
-
-import torch
-
-from .base import BaseQuantizer, QuantizedResult
-from .core_vq import ResidualVectorQuantization
-
-
-class ResidualVectorQuantizer(BaseQuantizer):
- """Residual Vector Quantizer.
-
- Args:
- dimension (int): Dimension of the codebooks.
- n_q (int): Number of residual vector quantizers used.
- q_dropout (bool): Random quantizer drop out at train time.
- bins (int): Codebook size.
- decay (float): Decay for exponential moving average over the codebooks.
- kmeans_init (bool): Whether to use kmeans to initialize the codebooks.
- kmeans_iters (int): Number of iterations used for kmeans initialization.
- threshold_ema_dead_code (int): Threshold for dead code expiration. Replace any codes
- that have an exponential moving average cluster size less than the specified threshold with
- randomly selected vector from the current batch.
- orthogonal_reg_weight (float): Orthogonal regularization weights.
- orthogonal_reg_active_codes_only (bool): Apply orthogonal regularization only on active codes.
- orthogonal_reg_max_codes (optional int): Maximum number of codes to consider.
- for orthogonal regularization.
- """
- def __init__(
- self,
- dimension: int = 256,
- n_q: int = 8,
- q_dropout: bool = False,
- bins: int = 1024,
- decay: float = 0.99,
- kmeans_init: bool = True,
- kmeans_iters: int = 10,
- threshold_ema_dead_code: int = 2,
- orthogonal_reg_weight: float = 0.0,
- orthogonal_reg_active_codes_only: bool = False,
- orthogonal_reg_max_codes: tp.Optional[int] = None,
- ):
- super().__init__()
- self.max_n_q = n_q
- self.n_q = n_q
- self.q_dropout = q_dropout
- self.dimension = dimension
- self.bins = bins
- self.decay = decay
- self.kmeans_init = kmeans_init
- self.kmeans_iters = kmeans_iters
- self.threshold_ema_dead_code = threshold_ema_dead_code
- self.orthogonal_reg_weight = orthogonal_reg_weight
- self.orthogonal_reg_active_codes_only = orthogonal_reg_active_codes_only
- self.orthogonal_reg_max_codes = orthogonal_reg_max_codes
- self.vq = ResidualVectorQuantization(
- dim=self.dimension,
- codebook_size=self.bins,
- num_quantizers=self.n_q,
- decay=self.decay,
- kmeans_init=self.kmeans_init,
- kmeans_iters=self.kmeans_iters,
- threshold_ema_dead_code=self.threshold_ema_dead_code,
- orthogonal_reg_weight=self.orthogonal_reg_weight,
- orthogonal_reg_active_codes_only=self.orthogonal_reg_active_codes_only,
- orthogonal_reg_max_codes=self.orthogonal_reg_max_codes,
- channels_last=False
- )
-
- def forward(self, x: torch.Tensor, frame_rate: int):
- n_q = self.n_q
- if self.training and self.q_dropout:
- n_q = int(torch.randint(1, self.n_q + 1, (1,)).item())
- bw_per_q = math.log2(self.bins) * frame_rate / 1000
- quantized, codes, commit_loss = self.vq(x, n_q=n_q)
- codes = codes.transpose(0, 1)
- # codes is [B, K, T], with T frames, K nb of codebooks.
- bw = torch.tensor(n_q * bw_per_q).to(x)
- return QuantizedResult(quantized, codes, bw, penalty=torch.mean(commit_loss))
-
- def encode(self, x: torch.Tensor) -> torch.Tensor:
- """Encode a given input tensor with the specified frame rate at the given bandwidth.
- The RVQ encode method sets the appropriate number of quantizer to use
- and returns indices for each quantizer.
- """
- n_q = self.n_q
- codes = self.vq.encode(x, n_q=n_q)
- codes = codes.transpose(0, 1)
- # codes is [B, K, T], with T frames, K nb of codebooks.
- return codes
-
- def decode(self, codes: torch.Tensor) -> torch.Tensor:
- """Decode the given codes to the quantized representation."""
- # codes is [B, K, T], with T frames, K nb of codebooks, vq.decode expects [K, B, T].
- codes = codes.transpose(0, 1)
- quantized = self.vq.decode(codes)
- return quantized
-
- @property
- def total_codebooks(self):
- return self.max_n_q
-
- @property
- def num_codebooks(self):
- return self.n_q
-
- def set_num_codebooks(self, n: int):
- assert n > 0 and n <= self.max_n_q
- self.n_q = n
diff --git a/spaces/bzd4576/sovits-sin/text/__init__.py b/spaces/bzd4576/sovits-sin/text/__init__.py
deleted file mode 100644
index 4ac41f9025755d8ffd74068af14c6cfc8e5a4173..0000000000000000000000000000000000000000
--- a/spaces/bzd4576/sovits-sin/text/__init__.py
+++ /dev/null
@@ -1,54 +0,0 @@
-""" from https://github.com/keithito/tacotron """
-from text import cleaners
-from text.symbols import symbols
-
-
-# Mappings from symbol to numeric ID and vice versa:
-_symbol_to_id = {s: i for i, s in enumerate(symbols)}
-_id_to_symbol = {i: s for i, s in enumerate(symbols)}
-
-
-def text_to_sequence(text, cleaner_names):
- '''Converts a string of text to a sequence of IDs corresponding to the symbols in the text.
- Args:
- text: string to convert to a sequence
- cleaner_names: names of the cleaner functions to run the text through
- Returns:
- List of integers corresponding to the symbols in the text
- '''
- sequence = []
-
- clean_text = _clean_text(text, cleaner_names)
- for symbol in clean_text:
- symbol_id = _symbol_to_id[symbol]
- sequence += [symbol_id]
- return sequence
-
-
-def cleaned_text_to_sequence(cleaned_text):
- '''Converts a string of text to a sequence of IDs corresponding to the symbols in the text.
- Args:
- text: string to convert to a sequence
- Returns:
- List of integers corresponding to the symbols in the text
- '''
- sequence = [_symbol_to_id[symbol] for symbol in cleaned_text]
- return sequence
-
-
-def sequence_to_text(sequence):
- '''Converts a sequence of IDs back to a string'''
- result = ''
- for symbol_id in sequence:
- s = _id_to_symbol[symbol_id]
- result += s
- return result
-
-
-def _clean_text(text, cleaner_names):
- for name in cleaner_names:
- cleaner = getattr(cleaners, name)
- if not cleaner:
- raise Exception('Unknown cleaner: %s' % name)
- text = cleaner(text)
- return text
diff --git a/spaces/chendl/compositional_test/multimodal/YOLOX/yolox/models/yolo_fpn.py b/spaces/chendl/compositional_test/multimodal/YOLOX/yolox/models/yolo_fpn.py
deleted file mode 100644
index 224271f59fd55b1e8e4bf3321d746a85bfe0b09c..0000000000000000000000000000000000000000
--- a/spaces/chendl/compositional_test/multimodal/YOLOX/yolox/models/yolo_fpn.py
+++ /dev/null
@@ -1,84 +0,0 @@
-#!/usr/bin/env python
-# -*- encoding: utf-8 -*-
-# Copyright (c) Megvii Inc. All rights reserved.
-
-import torch
-import torch.nn as nn
-
-from .darknet import Darknet
-from .network_blocks import BaseConv
-
-
-class YOLOFPN(nn.Module):
- """
- YOLOFPN module. Darknet 53 is the default backbone of this model.
- """
-
- def __init__(
- self,
- depth=53,
- in_features=["dark3", "dark4", "dark5"],
- ):
- super().__init__()
-
- self.backbone = Darknet(depth)
- self.in_features = in_features
-
- # out 1
- self.out1_cbl = self._make_cbl(512, 256, 1)
- self.out1 = self._make_embedding([256, 512], 512 + 256)
-
- # out 2
- self.out2_cbl = self._make_cbl(256, 128, 1)
- self.out2 = self._make_embedding([128, 256], 256 + 128)
-
- # upsample
- self.upsample = nn.Upsample(scale_factor=2, mode="nearest")
-
- def _make_cbl(self, _in, _out, ks):
- return BaseConv(_in, _out, ks, stride=1, act="lrelu")
-
- def _make_embedding(self, filters_list, in_filters):
- m = nn.Sequential(
- *[
- self._make_cbl(in_filters, filters_list[0], 1),
- self._make_cbl(filters_list[0], filters_list[1], 3),
- self._make_cbl(filters_list[1], filters_list[0], 1),
- self._make_cbl(filters_list[0], filters_list[1], 3),
- self._make_cbl(filters_list[1], filters_list[0], 1),
- ]
- )
- return m
-
- def load_pretrained_model(self, filename="./weights/darknet53.mix.pth"):
- with open(filename, "rb") as f:
- state_dict = torch.load(f, map_location="cpu")
- print("loading pretrained weights...")
- self.backbone.load_state_dict(state_dict)
-
- def forward(self, inputs):
- """
- Args:
- inputs (Tensor): input image.
-
- Returns:
- Tuple[Tensor]: FPN output features..
- """
- # backbone
- out_features = self.backbone(inputs)
- x2, x1, x0 = [out_features[f] for f in self.in_features]
-
- # yolo branch 1
- x1_in = self.out1_cbl(x0)
- x1_in = self.upsample(x1_in)
- x1_in = torch.cat([x1_in, x1], 1)
- out_dark4 = self.out1(x1_in)
-
- # yolo branch 2
- x2_in = self.out2_cbl(out_dark4)
- x2_in = self.upsample(x2_in)
- x2_in = torch.cat([x2_in, x2], 1)
- out_dark3 = self.out2(x2_in)
-
- outputs = (out_dark3, out_dark4, x0)
- return outputs
diff --git a/spaces/chendl/compositional_test/transformers/examples/research_projects/rag/__init__.py b/spaces/chendl/compositional_test/transformers/examples/research_projects/rag/__init__.py
deleted file mode 100644
index 3cee09bb7f51087e92d778c4c9e27d76085d1b30..0000000000000000000000000000000000000000
--- a/spaces/chendl/compositional_test/transformers/examples/research_projects/rag/__init__.py
+++ /dev/null
@@ -1,5 +0,0 @@
-import os
-import sys
-
-
-sys.path.insert(1, os.path.dirname(os.path.realpath(__file__)))
diff --git a/spaces/chilleverydaychill/roop/roop/typing.py b/spaces/chilleverydaychill/roop/roop/typing.py
deleted file mode 100644
index 1cff7440616e20bfe7b8bc287f86d11bf1b0f083..0000000000000000000000000000000000000000
--- a/spaces/chilleverydaychill/roop/roop/typing.py
+++ /dev/null
@@ -1,7 +0,0 @@
-from typing import Any
-
-from insightface.app.common import Face
-import numpy
-
-Face = Face
-Frame = numpy.ndarray[Any, Any]
diff --git a/spaces/chuan-hd/law-assistant-chatbot/.venv/lib/python3.11/site-packages/aiohttp/base_protocol.py b/spaces/chuan-hd/law-assistant-chatbot/.venv/lib/python3.11/site-packages/aiohttp/base_protocol.py
deleted file mode 100644
index 4c9f0a752e3aa833a17b7adf0c261d19a5f083fa..0000000000000000000000000000000000000000
--- a/spaces/chuan-hd/law-assistant-chatbot/.venv/lib/python3.11/site-packages/aiohttp/base_protocol.py
+++ /dev/null
@@ -1,90 +0,0 @@
-import asyncio
-from typing import Optional, cast
-
-from .tcp_helpers import tcp_nodelay
-
-
-class BaseProtocol(asyncio.Protocol):
- __slots__ = (
- "_loop",
- "_paused",
- "_drain_waiter",
- "_connection_lost",
- "_reading_paused",
- "transport",
- )
-
- def __init__(self, loop: asyncio.AbstractEventLoop) -> None:
- self._loop: asyncio.AbstractEventLoop = loop
- self._paused = False
- self._drain_waiter: Optional[asyncio.Future[None]] = None
- self._reading_paused = False
-
- self.transport: Optional[asyncio.Transport] = None
-
- @property
- def connected(self) -> bool:
- """Return True if the connection is open."""
- return self.transport is not None
-
- def pause_writing(self) -> None:
- assert not self._paused
- self._paused = True
-
- def resume_writing(self) -> None:
- assert self._paused
- self._paused = False
-
- waiter = self._drain_waiter
- if waiter is not None:
- self._drain_waiter = None
- if not waiter.done():
- waiter.set_result(None)
-
- def pause_reading(self) -> None:
- if not self._reading_paused and self.transport is not None:
- try:
- self.transport.pause_reading()
- except (AttributeError, NotImplementedError, RuntimeError):
- pass
- self._reading_paused = True
-
- def resume_reading(self) -> None:
- if self._reading_paused and self.transport is not None:
- try:
- self.transport.resume_reading()
- except (AttributeError, NotImplementedError, RuntimeError):
- pass
- self._reading_paused = False
-
- def connection_made(self, transport: asyncio.BaseTransport) -> None:
- tr = cast(asyncio.Transport, transport)
- tcp_nodelay(tr, True)
- self.transport = tr
-
- def connection_lost(self, exc: Optional[BaseException]) -> None:
- # Wake up the writer if currently paused.
- self.transport = None
- if not self._paused:
- return
- waiter = self._drain_waiter
- if waiter is None:
- return
- self._drain_waiter = None
- if waiter.done():
- return
- if exc is None:
- waiter.set_result(None)
- else:
- waiter.set_exception(exc)
-
- async def _drain_helper(self) -> None:
- if not self.connected:
- raise ConnectionResetError("Connection lost")
- if not self._paused:
- return
- waiter = self._drain_waiter
- if waiter is None:
- waiter = self._loop.create_future()
- self._drain_waiter = waiter
- await asyncio.shield(waiter)
diff --git a/spaces/chuan-hd/law-assistant-chatbot/.venv/lib/python3.11/site-packages/cryptography/hazmat/primitives/asymmetric/x25519.py b/spaces/chuan-hd/law-assistant-chatbot/.venv/lib/python3.11/site-packages/cryptography/hazmat/primitives/asymmetric/x25519.py
deleted file mode 100644
index 699054c9689ba0da21cd4397602d7139acceb238..0000000000000000000000000000000000000000
--- a/spaces/chuan-hd/law-assistant-chatbot/.venv/lib/python3.11/site-packages/cryptography/hazmat/primitives/asymmetric/x25519.py
+++ /dev/null
@@ -1,113 +0,0 @@
-# This file is dual licensed under the terms of the Apache License, Version
-# 2.0, and the BSD License. See the LICENSE file in the root of this repository
-# for complete details.
-
-from __future__ import annotations
-
-import abc
-
-from cryptography.exceptions import UnsupportedAlgorithm, _Reasons
-from cryptography.hazmat.bindings._rust import openssl as rust_openssl
-from cryptography.hazmat.primitives import _serialization
-
-
-class X25519PublicKey(metaclass=abc.ABCMeta):
- @classmethod
- def from_public_bytes(cls, data: bytes) -> X25519PublicKey:
- from cryptography.hazmat.backends.openssl.backend import backend
-
- if not backend.x25519_supported():
- raise UnsupportedAlgorithm(
- "X25519 is not supported by this version of OpenSSL.",
- _Reasons.UNSUPPORTED_EXCHANGE_ALGORITHM,
- )
-
- return backend.x25519_load_public_bytes(data)
-
- @abc.abstractmethod
- def public_bytes(
- self,
- encoding: _serialization.Encoding,
- format: _serialization.PublicFormat,
- ) -> bytes:
- """
- The serialized bytes of the public key.
- """
-
- @abc.abstractmethod
- def public_bytes_raw(self) -> bytes:
- """
- The raw bytes of the public key.
- Equivalent to public_bytes(Raw, Raw).
- """
-
- @abc.abstractmethod
- def __eq__(self, other: object) -> bool:
- """
- Checks equality.
- """
-
-
-# For LibreSSL
-if hasattr(rust_openssl, "x25519"):
- X25519PublicKey.register(rust_openssl.x25519.X25519PublicKey)
-
-
-class X25519PrivateKey(metaclass=abc.ABCMeta):
- @classmethod
- def generate(cls) -> X25519PrivateKey:
- from cryptography.hazmat.backends.openssl.backend import backend
-
- if not backend.x25519_supported():
- raise UnsupportedAlgorithm(
- "X25519 is not supported by this version of OpenSSL.",
- _Reasons.UNSUPPORTED_EXCHANGE_ALGORITHM,
- )
- return backend.x25519_generate_key()
-
- @classmethod
- def from_private_bytes(cls, data: bytes) -> X25519PrivateKey:
- from cryptography.hazmat.backends.openssl.backend import backend
-
- if not backend.x25519_supported():
- raise UnsupportedAlgorithm(
- "X25519 is not supported by this version of OpenSSL.",
- _Reasons.UNSUPPORTED_EXCHANGE_ALGORITHM,
- )
-
- return backend.x25519_load_private_bytes(data)
-
- @abc.abstractmethod
- def public_key(self) -> X25519PublicKey:
- """
- Returns the public key assosciated with this private key
- """
-
- @abc.abstractmethod
- def private_bytes(
- self,
- encoding: _serialization.Encoding,
- format: _serialization.PrivateFormat,
- encryption_algorithm: _serialization.KeySerializationEncryption,
- ) -> bytes:
- """
- The serialized bytes of the private key.
- """
-
- @abc.abstractmethod
- def private_bytes_raw(self) -> bytes:
- """
- The raw bytes of the private key.
- Equivalent to private_bytes(Raw, Raw, NoEncryption()).
- """
-
- @abc.abstractmethod
- def exchange(self, peer_public_key: X25519PublicKey) -> bytes:
- """
- Performs a key exchange operation using the provided peer's public key.
- """
-
-
-# For LibreSSL
-if hasattr(rust_openssl, "x25519"):
- X25519PrivateKey.register(rust_openssl.x25519.X25519PrivateKey)
diff --git a/spaces/chuan-hd/law-assistant-chatbot/.venv/lib/python3.11/site-packages/gradio/templates/cdn/assets/index-3ca19104.js b/spaces/chuan-hd/law-assistant-chatbot/.venv/lib/python3.11/site-packages/gradio/templates/cdn/assets/index-3ca19104.js
deleted file mode 100644
index 931f5d45246d62ebcfe89afd89d319e15d816116..0000000000000000000000000000000000000000
--- a/spaces/chuan-hd/law-assistant-chatbot/.venv/lib/python3.11/site-packages/gradio/templates/cdn/assets/index-3ca19104.js
+++ /dev/null
@@ -1,2 +0,0 @@
-import{ae as s}from"./index-f877dfd5.js";const o=["static"];export{s as Component,o as modes};
-//# sourceMappingURL=index-3ca19104.js.map
diff --git a/spaces/cihyFjudo/fairness-paper-search/A Job Aid for Your Employment Search Spanning 3 Best Practices for Finding and Applying to Jobs Online.md b/spaces/cihyFjudo/fairness-paper-search/A Job Aid for Your Employment Search Spanning 3 Best Practices for Finding and Applying to Jobs Online.md
deleted file mode 100644
index 00e64f1d78b3a8ee7b76e5cd78e8e19ec46b167f..0000000000000000000000000000000000000000
--- a/spaces/cihyFjudo/fairness-paper-search/A Job Aid for Your Employment Search Spanning 3 Best Practices for Finding and Applying to Jobs Online.md
+++ /dev/null
@@ -1,20 +0,0 @@
-
-Select the applicable employment type by clicking the blue search box. This field identifies if the individual is Faculty, Professional, Classified, Student, or Temporary and if they are paid from irregular or regular funds. Click the blue magnifying glass icon to update this field.
-a job aid for your employment search spanning 3
Download File ✔ https://tinurli.com/2uwjQc
-For people group, select the applicable employment type by clicking the blue search box. This field identifies if the individual is Faculty, Professional, Classified, Student, or Temporary and if they are paid from irregular or regular funds. Click the blue magnifying glass icon to update this field.
-For People Group, select the applicable employment type by clicking the blue search box. This field identifies if the individual is Faculty, Professional, Classified, Student, or Temporary and if they are paid from irregular or regular funds. Select the blue magnifying glass icon to update this field.
-Paste the article title into the search box, or enter citation details such as the author, journal name and the year the article was published in the search box and the PubMed citation sensor will automatically analyze your query for citation information to return the correct citation. The citation sensor incorporates a fuzzy matching algorithm and will retrieve the best match even if a search includes an incorrect term. You do not need to use field tags or Boolean operators.
-
-To search for systematic reviews in PubMed, use the Systematic Review article type filter on the sidebar, or enter your search terms followed by AND systematic[sb] in the search box. For example, lyme disease AND systematic[sb].
-The Systematic Review filter uses a search strategy in addition to the Systematic Review publication type [pt] to find systematic reviews in PubMed. To limit your search to only those citations with the Systematic Review publication type, use the publication type search tag[pt], i.e., systematic review[pt]; however, this may exclude some relevant citations that have not yet completed the MEDLINE indexing process.
-The Exclude preprints filter can be added to the sidebar using the Additional Filters button. Alternatively, you can exclude preprints from your search results by including NOT preprint[pt] at the end of your query.
-The MEDLINE filter can be added to the sidebar using the Additional Filters button. To use this filter in a query, add medline[sb] to your search. The MEDLINE filter limits results to citations that are indexed for MEDLINE.
-The Clipboard provides a place to collect up to 500 items from one or more searches. Items saved to the Clipboard are stored in your browser cookies and will expire after 8 hours of inactivity. If you would like to save items for longer than 8 hours or to view on another device, please use Send to: Collections.
-To see how your terms were translated, check the Search Details available on the Advanced Search page for each query under History. If you want to report a translation that does not seem accurate for your search topic, please e-mail the information to the NLM Help Desk.
-Citations that have been indexed for MEDLINE and updated with NLM Medical Subject Headings (MeSH), publication types, GenBank accession numbers, and other indexing data are available daily. To limit your search to MEDLINE citations, add medline[sb] to your search.
-NLM provides data to vendors around the world. Other products and services will not necessarily immediately reflect corrections made to PubMed records. If you search through a vendor's system, please contact your vendor about their maintenance schedules.
-Supply estimations factor in all individuals relevant to the cybersecurity workforce. This includes those employed in the field plus those with relevant skills and/or recent experience who are considered to be actively searching on the job market. To derive such supply estimations, we leverage a combination of government employment data, historic cybersecurity job openings and social profile data.
-Join our team and contribute your knowledge and skills to our diverse wealth of talent. Lifespan is committed to developing a diverse workforce and encourages qualified applicants from all backgrounds to apply. All qualified applicants will receive consideration for employment without regard to race, color, religion, sex, national origin, age, ethnicity, sexual preference or orientation, ancestry, genetics, gender identity or expression, disability, protected veteran or marital status. Lifespan is an Equal Opportunity/Affirmative Action employer and a VEVRAA Federal Contractor.
-Sandia is a drug-free workplace. As a national laboratory funded by a U.S. government agency, we are subject to federal laws regarding illegal drug use. Illegal use of a controlled substance, including marijuana even in places where it does not violate state law, may impact your ability to obtain and/or maintain a Department of Energy security clearance, and may result in the withdrawal of an employment offer or termination of employment.
aaccfb2cb3
-
-
\ No newline at end of file
diff --git a/spaces/cihyFjudo/fairness-paper-search/Italian Movie Dubbed In Italian Free Download J..md b/spaces/cihyFjudo/fairness-paper-search/Italian Movie Dubbed In Italian Free Download J..md
deleted file mode 100644
index 19c79021dd4e99116b63743ecf16adb4bfe41fa0..0000000000000000000000000000000000000000
--- a/spaces/cihyFjudo/fairness-paper-search/Italian Movie Dubbed In Italian Free Download J..md
+++ /dev/null
@@ -1,21 +0,0 @@
-
-All digital products you purchase from RiffTrax.com are DRM-free, can be played across a large variety of devices, and are yours to keep ... forever! Even if you have a hard drive meltdown, you can always log back into the site and re-download all of your previous purchases.
-Italian Movie Dubbed In Italian Free Download J.
Download File ❤ https://tinurli.com/2uwjM9
-The audience for anime has been growing ever since. Since anime comes in different languages,, people always search for dubbed versions of these anime. Nowadays, English or any other language subtitles are available for all animes, but it is often hard to come across dubbed versions of these. So here we will list out 10 free websites that can provide you with dubbed anime.
-Animeland is a website that provides dubbed versions of all-time favorite animes like Naruto, One Piece, Bleach, Attack on Titan, My Hero Academia, etc. This site has about 1.10 million viewers per month. Animeland also offers the feature to download your favorite animes in 480p - 1080p, for watching later.
-This website also provides regular updates on dubbed anime lists and movie lists, which can be very helpful for those searching for new animes to watch. The Navigation in this site can be used to find any anime you wish without any difficulty.
-Animestream.tv is a high-quality online streaming website that offers free dubbed animes like Naruto, One Piece, Bleach, Fairy Tail Anime, etc. almost all the animes on this site are dubbed in English which makes them accessible to even very young anime lovers.
-CartoonCrazy is a popular add-on that helps anime lovers to stream anime, cartoons, and other programs. This tool provides the link of the latest dubbed animes as well as old ones for the users to download. All streaming and downloading options are free of cost.
-
-Anime planet is another popular website with a collection of about 45,000 episodes. It provides its users with a mix of dubbed series of adventure, horror, comedy, etc. genres for free. The users also have access to reviews and recommendations from different users across the globe.
-Justdubs is an interactive platform where you can interact with anime lovers all over the world. This platform also provides links to download dubbed anime websites. The users on the platform provide reviews on different animes all over the world and can also suggest to you the best-dubbed anime versions to watch.
-123anime is one of the most popular websites in the world mainly famous for Japanese dubbed animes, movies, and TV shows. This website is very easy to navigate, and can easily find almost all the Japanese animes including Ghost in the Shell, Neon Genesis Evangelion, Cowboy Bebop, The Legend of Zelda, etc.
-This website also offers dubbed and subbed versions of the animes for users who prefer English. The animes can be either be watched online or can be downloaded for watching later. All the animes are available in HD quality, and no registration is required to access the content.
-9anime is a unique website that that offers a lot of dubbed anime content and allows the streaming of almost all of it in 1080p. It is a vast platform that offers a lot of dubbed animes, movies, and other top-quality shows. The subbed and dubbed versions of some of the top animes like Death Note, Naruto, Dragon Ball, and Psycho can easily be watched from this site.
-Anime is one of the most-watched and downloaded visual content all over the world irrespective of age and cultural barriers. Most of the animes created are in Japanese, Korean, Chinese, and other languages and hence there is a need for dubbed versions of these animes.
-On June 5, 2022, during a YouTube stream organized by fellow actor Dmitry Cherevatenko, Dmitry Filimonov revealed that the movie and all of Steven Universe Future were fully dubbed in February 2022, and that he does not know when or whether any of these might release.[25]
-Acquerello italiano
IT 1.017
60-minute audiocassettes containing songs, interviews, and cultural information about Italy; a tapescript and a study guide accompany each cassette; study supplement contains listening comprehension exercises, crossword puzzles, and an answer key.
-Ladri di biciclette (The Bicycle Thief)
All-regions (NTSC) DVD
Vittorio de Sica (1948)
Black & white film starring non-actors Lamberto Maggiorani and Enzo Staiola. Story of Antonio Ricci, a poor man in post-war Rome whose bicycle is stolen on his first day of work as a laborer hired to deliver movie posters, a job that requires he have a bicycle. Poignant portrait of a father and son whose search for the stolen bicycle leads them through a world of indifference and challenges to the human spirit.
In Italian with option of English subtitles; option of dubbed English dialogue soundtrack also available
1 hour 29 minutes
-Parliamo italiano
1/2" VHS color videocassette
Produced by DUNE Produzioni Cinematografiche e Televise in association with Houghton Mifflin Company; designed to complement introductory Italian texts; 12 modules present the adventures of two characters, Piero and Gabriella, who travel throughout Italy (to 11 different cities/regions) with the objective of producing a travel guide for their employer, a travel agency.
Approx. 60 minutes
aaccfb2cb3
-
-
\ No newline at end of file
diff --git a/spaces/cihyFjudo/fairness-paper-search/Momonogi Kana Pic Nu.md b/spaces/cihyFjudo/fairness-paper-search/Momonogi Kana Pic Nu.md
deleted file mode 100644
index f902bb59399c108e06e0629d092513be0660ecef..0000000000000000000000000000000000000000
--- a/spaces/cihyFjudo/fairness-paper-search/Momonogi Kana Pic Nu.md
+++ /dev/null
@@ -1,6 +0,0 @@
-Momonogi Kana Pic Nu
Download File ►►►►► https://tinurli.com/2uwk85
-
- aaccfb2cb3
-
-
-
diff --git a/spaces/cihyFjudo/fairness-paper-search/The Sims 4 StrangerVille PC Game [MULTi17] Free Download CODEX Review and Rating of the New Expansion Pack.md b/spaces/cihyFjudo/fairness-paper-search/The Sims 4 StrangerVille PC Game [MULTi17] Free Download CODEX Review and Rating of the New Expansion Pack.md
deleted file mode 100644
index 5106830decfcb15a5f932b5e4724b3523c91221e..0000000000000000000000000000000000000000
--- a/spaces/cihyFjudo/fairness-paper-search/The Sims 4 StrangerVille PC Game [MULTi17] Free Download CODEX Review and Rating of the New Expansion Pack.md
+++ /dev/null
@@ -1,6 +0,0 @@
-The Sims 4 StrangerVille PC Game [MULTi17] Free Download – CODEX
DOWNLOAD - https://tinurli.com/2uwkpT
-
- aaccfb2cb3
-
-
-
diff --git a/spaces/cloudtheboi/Lofi4All/.pythonlibs/lib/python3.10/site-packages/fontTools/cu2qu/cu2qu.py b/spaces/cloudtheboi/Lofi4All/.pythonlibs/lib/python3.10/site-packages/fontTools/cu2qu/cu2qu.py
deleted file mode 100644
index e620b48a55bd0ce720a34c309d295839edabe5aa..0000000000000000000000000000000000000000
--- a/spaces/cloudtheboi/Lofi4All/.pythonlibs/lib/python3.10/site-packages/fontTools/cu2qu/cu2qu.py
+++ /dev/null
@@ -1,534 +0,0 @@
-# cython: language_level=3
-# distutils: define_macros=CYTHON_TRACE_NOGIL=1
-
-# Copyright 2015 Google Inc. All Rights Reserved.
-#
-# Licensed under the Apache License, Version 2.0 (the "License");
-# you may not use this file except in compliance with the License.
-# You may obtain a copy of the License at
-#
-# http://www.apache.org/licenses/LICENSE-2.0
-#
-# Unless required by applicable law or agreed to in writing, software
-# distributed under the License is distributed on an "AS IS" BASIS,
-# WITHOUT WARRANTIES OR CONDITIONS OF ANY KIND, either express or implied.
-# See the License for the specific language governing permissions and
-# limitations under the License.
-
-try:
- import cython
-
- COMPILED = cython.compiled
-except (AttributeError, ImportError):
- # if cython not installed, use mock module with no-op decorators and types
- from fontTools.misc import cython
-
- COMPILED = False
-
-import math
-
-from .errors import Error as Cu2QuError, ApproxNotFoundError
-
-
-__all__ = ["curve_to_quadratic", "curves_to_quadratic"]
-
-MAX_N = 100
-
-NAN = float("NaN")
-
-
-@cython.cfunc
-@cython.inline
-@cython.returns(cython.double)
-@cython.locals(v1=cython.complex, v2=cython.complex)
-def dot(v1, v2):
- """Return the dot product of two vectors.
-
- Args:
- v1 (complex): First vector.
- v2 (complex): Second vector.
-
- Returns:
- double: Dot product.
- """
- return (v1 * v2.conjugate()).real
-
-
-@cython.cfunc
-@cython.inline
-@cython.locals(a=cython.complex, b=cython.complex, c=cython.complex, d=cython.complex)
-@cython.locals(
- _1=cython.complex, _2=cython.complex, _3=cython.complex, _4=cython.complex
-)
-def calc_cubic_points(a, b, c, d):
- _1 = d
- _2 = (c / 3.0) + d
- _3 = (b + c) / 3.0 + _2
- _4 = a + d + c + b
- return _1, _2, _3, _4
-
-
-@cython.cfunc
-@cython.inline
-@cython.locals(
- p0=cython.complex, p1=cython.complex, p2=cython.complex, p3=cython.complex
-)
-@cython.locals(a=cython.complex, b=cython.complex, c=cython.complex, d=cython.complex)
-def calc_cubic_parameters(p0, p1, p2, p3):
- c = (p1 - p0) * 3.0
- b = (p2 - p1) * 3.0 - c
- d = p0
- a = p3 - d - c - b
- return a, b, c, d
-
-
-@cython.cfunc
-@cython.inline
-@cython.locals(
- p0=cython.complex, p1=cython.complex, p2=cython.complex, p3=cython.complex
-)
-def split_cubic_into_n_iter(p0, p1, p2, p3, n):
- """Split a cubic Bezier into n equal parts.
-
- Splits the curve into `n` equal parts by curve time.
- (t=0..1/n, t=1/n..2/n, ...)
-
- Args:
- p0 (complex): Start point of curve.
- p1 (complex): First handle of curve.
- p2 (complex): Second handle of curve.
- p3 (complex): End point of curve.
-
- Returns:
- An iterator yielding the control points (four complex values) of the
- subcurves.
- """
- # Hand-coded special-cases
- if n == 2:
- return iter(split_cubic_into_two(p0, p1, p2, p3))
- if n == 3:
- return iter(split_cubic_into_three(p0, p1, p2, p3))
- if n == 4:
- a, b = split_cubic_into_two(p0, p1, p2, p3)
- return iter(
- split_cubic_into_two(a[0], a[1], a[2], a[3])
- + split_cubic_into_two(b[0], b[1], b[2], b[3])
- )
- if n == 6:
- a, b = split_cubic_into_two(p0, p1, p2, p3)
- return iter(
- split_cubic_into_three(a[0], a[1], a[2], a[3])
- + split_cubic_into_three(b[0], b[1], b[2], b[3])
- )
-
- return _split_cubic_into_n_gen(p0, p1, p2, p3, n)
-
-
-@cython.locals(
- p0=cython.complex,
- p1=cython.complex,
- p2=cython.complex,
- p3=cython.complex,
- n=cython.int,
-)
-@cython.locals(a=cython.complex, b=cython.complex, c=cython.complex, d=cython.complex)
-@cython.locals(
- dt=cython.double, delta_2=cython.double, delta_3=cython.double, i=cython.int
-)
-@cython.locals(
- a1=cython.complex, b1=cython.complex, c1=cython.complex, d1=cython.complex
-)
-def _split_cubic_into_n_gen(p0, p1, p2, p3, n):
- a, b, c, d = calc_cubic_parameters(p0, p1, p2, p3)
- dt = 1 / n
- delta_2 = dt * dt
- delta_3 = dt * delta_2
- for i in range(n):
- t1 = i * dt
- t1_2 = t1 * t1
- # calc new a, b, c and d
- a1 = a * delta_3
- b1 = (3 * a * t1 + b) * delta_2
- c1 = (2 * b * t1 + c + 3 * a * t1_2) * dt
- d1 = a * t1 * t1_2 + b * t1_2 + c * t1 + d
- yield calc_cubic_points(a1, b1, c1, d1)
-
-
-@cython.cfunc
-@cython.inline
-@cython.locals(
- p0=cython.complex, p1=cython.complex, p2=cython.complex, p3=cython.complex
-)
-@cython.locals(mid=cython.complex, deriv3=cython.complex)
-def split_cubic_into_two(p0, p1, p2, p3):
- """Split a cubic Bezier into two equal parts.
-
- Splits the curve into two equal parts at t = 0.5
-
- Args:
- p0 (complex): Start point of curve.
- p1 (complex): First handle of curve.
- p2 (complex): Second handle of curve.
- p3 (complex): End point of curve.
-
- Returns:
- tuple: Two cubic Beziers (each expressed as a tuple of four complex
- values).
- """
- mid = (p0 + 3 * (p1 + p2) + p3) * 0.125
- deriv3 = (p3 + p2 - p1 - p0) * 0.125
- return (
- (p0, (p0 + p1) * 0.5, mid - deriv3, mid),
- (mid, mid + deriv3, (p2 + p3) * 0.5, p3),
- )
-
-
-@cython.cfunc
-@cython.inline
-@cython.locals(
- p0=cython.complex,
- p1=cython.complex,
- p2=cython.complex,
- p3=cython.complex,
-)
-@cython.locals(
- mid1=cython.complex,
- deriv1=cython.complex,
- mid2=cython.complex,
- deriv2=cython.complex,
-)
-def split_cubic_into_three(p0, p1, p2, p3):
- """Split a cubic Bezier into three equal parts.
-
- Splits the curve into three equal parts at t = 1/3 and t = 2/3
-
- Args:
- p0 (complex): Start point of curve.
- p1 (complex): First handle of curve.
- p2 (complex): Second handle of curve.
- p3 (complex): End point of curve.
-
- Returns:
- tuple: Three cubic Beziers (each expressed as a tuple of four complex
- values).
- """
- mid1 = (8 * p0 + 12 * p1 + 6 * p2 + p3) * (1 / 27)
- deriv1 = (p3 + 3 * p2 - 4 * p0) * (1 / 27)
- mid2 = (p0 + 6 * p1 + 12 * p2 + 8 * p3) * (1 / 27)
- deriv2 = (4 * p3 - 3 * p1 - p0) * (1 / 27)
- return (
- (p0, (2 * p0 + p1) / 3.0, mid1 - deriv1, mid1),
- (mid1, mid1 + deriv1, mid2 - deriv2, mid2),
- (mid2, mid2 + deriv2, (p2 + 2 * p3) / 3.0, p3),
- )
-
-
-@cython.cfunc
-@cython.inline
-@cython.returns(cython.complex)
-@cython.locals(
- t=cython.double,
- p0=cython.complex,
- p1=cython.complex,
- p2=cython.complex,
- p3=cython.complex,
-)
-@cython.locals(_p1=cython.complex, _p2=cython.complex)
-def cubic_approx_control(t, p0, p1, p2, p3):
- """Approximate a cubic Bezier using a quadratic one.
-
- Args:
- t (double): Position of control point.
- p0 (complex): Start point of curve.
- p1 (complex): First handle of curve.
- p2 (complex): Second handle of curve.
- p3 (complex): End point of curve.
-
- Returns:
- complex: Location of candidate control point on quadratic curve.
- """
- _p1 = p0 + (p1 - p0) * 1.5
- _p2 = p3 + (p2 - p3) * 1.5
- return _p1 + (_p2 - _p1) * t
-
-
-@cython.cfunc
-@cython.inline
-@cython.returns(cython.complex)
-@cython.locals(a=cython.complex, b=cython.complex, c=cython.complex, d=cython.complex)
-@cython.locals(ab=cython.complex, cd=cython.complex, p=cython.complex, h=cython.double)
-def calc_intersect(a, b, c, d):
- """Calculate the intersection of two lines.
-
- Args:
- a (complex): Start point of first line.
- b (complex): End point of first line.
- c (complex): Start point of second line.
- d (complex): End point of second line.
-
- Returns:
- complex: Location of intersection if one present, ``complex(NaN,NaN)``
- if no intersection was found.
- """
- ab = b - a
- cd = d - c
- p = ab * 1j
- try:
- h = dot(p, a - c) / dot(p, cd)
- except ZeroDivisionError:
- return complex(NAN, NAN)
- return c + cd * h
-
-
-@cython.cfunc
-@cython.returns(cython.int)
-@cython.locals(
- tolerance=cython.double,
- p0=cython.complex,
- p1=cython.complex,
- p2=cython.complex,
- p3=cython.complex,
-)
-@cython.locals(mid=cython.complex, deriv3=cython.complex)
-def cubic_farthest_fit_inside(p0, p1, p2, p3, tolerance):
- """Check if a cubic Bezier lies within a given distance of the origin.
-
- "Origin" means *the* origin (0,0), not the start of the curve. Note that no
- checks are made on the start and end positions of the curve; this function
- only checks the inside of the curve.
-
- Args:
- p0 (complex): Start point of curve.
- p1 (complex): First handle of curve.
- p2 (complex): Second handle of curve.
- p3 (complex): End point of curve.
- tolerance (double): Distance from origin.
-
- Returns:
- bool: True if the cubic Bezier ``p`` entirely lies within a distance
- ``tolerance`` of the origin, False otherwise.
- """
- # First check p2 then p1, as p2 has higher error early on.
- if abs(p2) <= tolerance and abs(p1) <= tolerance:
- return True
-
- # Split.
- mid = (p0 + 3 * (p1 + p2) + p3) * 0.125
- if abs(mid) > tolerance:
- return False
- deriv3 = (p3 + p2 - p1 - p0) * 0.125
- return cubic_farthest_fit_inside(
- p0, (p0 + p1) * 0.5, mid - deriv3, mid, tolerance
- ) and cubic_farthest_fit_inside(mid, mid + deriv3, (p2 + p3) * 0.5, p3, tolerance)
-
-
-@cython.cfunc
-@cython.inline
-@cython.locals(tolerance=cython.double)
-@cython.locals(
- q1=cython.complex,
- c0=cython.complex,
- c1=cython.complex,
- c2=cython.complex,
- c3=cython.complex,
-)
-def cubic_approx_quadratic(cubic, tolerance):
- """Approximate a cubic Bezier with a single quadratic within a given tolerance.
-
- Args:
- cubic (sequence): Four complex numbers representing control points of
- the cubic Bezier curve.
- tolerance (double): Permitted deviation from the original curve.
-
- Returns:
- Three complex numbers representing control points of the quadratic
- curve if it fits within the given tolerance, or ``None`` if no suitable
- curve could be calculated.
- """
-
- q1 = calc_intersect(cubic[0], cubic[1], cubic[2], cubic[3])
- if math.isnan(q1.imag):
- return None
- c0 = cubic[0]
- c3 = cubic[3]
- c1 = c0 + (q1 - c0) * (2 / 3)
- c2 = c3 + (q1 - c3) * (2 / 3)
- if not cubic_farthest_fit_inside(0, c1 - cubic[1], c2 - cubic[2], 0, tolerance):
- return None
- return c0, q1, c3
-
-
-@cython.cfunc
-@cython.locals(n=cython.int, tolerance=cython.double)
-@cython.locals(i=cython.int)
-@cython.locals(all_quadratic=cython.int)
-@cython.locals(
- c0=cython.complex, c1=cython.complex, c2=cython.complex, c3=cython.complex
-)
-@cython.locals(
- q0=cython.complex,
- q1=cython.complex,
- next_q1=cython.complex,
- q2=cython.complex,
- d1=cython.complex,
-)
-def cubic_approx_spline(cubic, n, tolerance, all_quadratic):
- """Approximate a cubic Bezier curve with a spline of n quadratics.
-
- Args:
- cubic (sequence): Four complex numbers representing control points of
- the cubic Bezier curve.
- n (int): Number of quadratic Bezier curves in the spline.
- tolerance (double): Permitted deviation from the original curve.
-
- Returns:
- A list of ``n+2`` complex numbers, representing control points of the
- quadratic spline if it fits within the given tolerance, or ``None`` if
- no suitable spline could be calculated.
- """
-
- if n == 1:
- return cubic_approx_quadratic(cubic, tolerance)
- if n == 2 and all_quadratic == False:
- return cubic
-
- cubics = split_cubic_into_n_iter(cubic[0], cubic[1], cubic[2], cubic[3], n)
-
- # calculate the spline of quadratics and check errors at the same time.
- next_cubic = next(cubics)
- next_q1 = cubic_approx_control(
- 0, next_cubic[0], next_cubic[1], next_cubic[2], next_cubic[3]
- )
- q2 = cubic[0]
- d1 = 0j
- spline = [cubic[0], next_q1]
- for i in range(1, n + 1):
- # Current cubic to convert
- c0, c1, c2, c3 = next_cubic
-
- # Current quadratic approximation of current cubic
- q0 = q2
- q1 = next_q1
- if i < n:
- next_cubic = next(cubics)
- next_q1 = cubic_approx_control(
- i / (n - 1), next_cubic[0], next_cubic[1], next_cubic[2], next_cubic[3]
- )
- spline.append(next_q1)
- q2 = (q1 + next_q1) * 0.5
- else:
- q2 = c3
-
- # End-point deltas
- d0 = d1
- d1 = q2 - c3
-
- if abs(d1) > tolerance or not cubic_farthest_fit_inside(
- d0,
- q0 + (q1 - q0) * (2 / 3) - c1,
- q2 + (q1 - q2) * (2 / 3) - c2,
- d1,
- tolerance,
- ):
- return None
- spline.append(cubic[3])
-
- return spline
-
-
-@cython.locals(max_err=cython.double)
-@cython.locals(n=cython.int)
-@cython.locals(all_quadratic=cython.int)
-def curve_to_quadratic(curve, max_err, all_quadratic=True):
- """Approximate a cubic Bezier curve with a spline of n quadratics.
-
- Args:
- cubic (sequence): Four 2D tuples representing control points of
- the cubic Bezier curve.
- max_err (double): Permitted deviation from the original curve.
- all_quadratic (bool): If True (default) returned value is a
- quadratic spline. If False, it's either a single quadratic
- curve or a single cubic curve.
-
- Returns:
- If all_quadratic is True: A list of 2D tuples, representing
- control points of the quadratic spline if it fits within the
- given tolerance, or ``None`` if no suitable spline could be
- calculated.
-
- If all_quadratic is False: Either a quadratic curve (if length
- of output is 3), or a cubic curve (if length of output is 4).
- """
-
- curve = [complex(*p) for p in curve]
-
- for n in range(1, MAX_N + 1):
- spline = cubic_approx_spline(curve, n, max_err, all_quadratic)
- if spline is not None:
- # done. go home
- return [(s.real, s.imag) for s in spline]
-
- raise ApproxNotFoundError(curve)
-
-
-@cython.locals(l=cython.int, last_i=cython.int, i=cython.int)
-@cython.locals(all_quadratic=cython.int)
-def curves_to_quadratic(curves, max_errors, all_quadratic=True):
- """Return quadratic Bezier splines approximating the input cubic Beziers.
-
- Args:
- curves: A sequence of *n* curves, each curve being a sequence of four
- 2D tuples.
- max_errors: A sequence of *n* floats representing the maximum permissible
- deviation from each of the cubic Bezier curves.
- all_quadratic (bool): If True (default) returned values are a
- quadratic spline. If False, they are either a single quadratic
- curve or a single cubic curve.
-
- Example::
-
- >>> curves_to_quadratic( [
- ... [ (50,50), (100,100), (150,100), (200,50) ],
- ... [ (75,50), (120,100), (150,75), (200,60) ]
- ... ], [1,1] )
- [[(50.0, 50.0), (75.0, 75.0), (125.0, 91.66666666666666), (175.0, 75.0), (200.0, 50.0)], [(75.0, 50.0), (97.5, 75.0), (135.41666666666666, 82.08333333333333), (175.0, 67.5), (200.0, 60.0)]]
-
- The returned splines have "implied oncurve points" suitable for use in
- TrueType ``glif`` outlines - i.e. in the first spline returned above,
- the first quadratic segment runs from (50,50) to
- ( (75 + 125)/2 , (120 + 91.666..)/2 ) = (100, 83.333...).
-
- Returns:
- If all_quadratic is True, a list of splines, each spline being a list
- of 2D tuples.
-
- If all_quadratic is False, a list of curves, each curve being a quadratic
- (length 3), or cubic (length 4).
-
- Raises:
- fontTools.cu2qu.Errors.ApproxNotFoundError: if no suitable approximation
- can be found for all curves with the given parameters.
- """
-
- curves = [[complex(*p) for p in curve] for curve in curves]
- assert len(max_errors) == len(curves)
-
- l = len(curves)
- splines = [None] * l
- last_i = i = 0
- n = 1
- while True:
- spline = cubic_approx_spline(curves[i], n, max_errors[i], all_quadratic)
- if spline is None:
- if n == MAX_N:
- break
- n += 1
- last_i = i
- continue
- splines[i] = spline
- i = (i + 1) % l
- if i == last_i:
- # done. go home
- return [[(s.real, s.imag) for s in spline] for spline in splines]
-
- raise ApproxNotFoundError(curves)
diff --git a/spaces/codesue/dystopedia/README.md b/spaces/codesue/dystopedia/README.md
deleted file mode 100644
index 662bc69cf2f6645968e9790506785e2df4742af9..0000000000000000000000000000000000000000
--- a/spaces/codesue/dystopedia/README.md
+++ /dev/null
@@ -1,33 +0,0 @@
----
-title: Dystopedia
-emoji: 🫠
-colorFrom: green
-colorTo: purple
-sdk: gradio
-sdk_version: 3.1.6
-app_file: app.py
-pinned: false
-license: apache-2.0
----
-
-# dystopedia
-
-I once read [a Tweet](https://twitter.com/lbcyber/status/1115015586243862528)
-that said, "you can make any Wikipedia article dystopian by changing it to the
-past tense." Dystopian Wikipedia sounded like a fun idea, so I built
-[Dystopedia](https://codesue.com/blog/dystopedia) as a proof-of-concept.
-Try it out on the [codesue/dystopedia](https://huggingface.co/spaces/codesue/dystopedia)
-Hugging Face Space.
-
-## Installation
-
-``` shell
-git clone https://github.com/codesue/dystopedia.git
-cd dystopedia
-pip install -r requirements.txt
-```
-
-## Running the app
-
-```shell
-python app.py
diff --git a/spaces/congsaPfin/Manga-OCR/logs/CBSE Academic Repository Download 12 Marksheet and Other Certificates for Old Exams.md b/spaces/congsaPfin/Manga-OCR/logs/CBSE Academic Repository Download 12 Marksheet and Other Certificates for Old Exams.md
deleted file mode 100644
index 354d75d2a01d799d8b55336b22c6acf1e637b71d..0000000000000000000000000000000000000000
--- a/spaces/congsaPfin/Manga-OCR/logs/CBSE Academic Repository Download 12 Marksheet and Other Certificates for Old Exams.md
+++ /dev/null
@@ -1,125 +0,0 @@
-
-How to Download 12 Marksheet Online
-If you have appeared for class 12 exams conducted by the Central Board of Secondary Education (CBSE), you might be wondering how to download your marksheet online. A marksheet is a document that shows your academic performance in terms of marks obtained, grades awarded, and overall percentage. It is an important document that you need for various purposes such as admission, scholarship, job, and other opportunities. In this article, we will tell you how to download your 12 marksheet online from different sources.
- What is 12 Marksheet and Why Do You Need It?
-12 Marksheet is a document that shows your academic performance in class 12 exams
-A 12 marksheet is a document that contains the details of your class 12 exams such as your roll number, name, school name, subject codes, marks obtained, grades awarded, and overall percentage. It also shows whether you have passed or failed the exams. A 12 marksheet is issued by CBSE after declaring the results of class 12 exams. It is an official document that certifies your completion of class 12 education.
-download 12 marksheet
Download File >> https://urlca.com/2uO6gK
- You need it for admission, scholarship, job, and other purposes
-A 12 marksheet is not only a proof of your academic achievement but also a requirement for various purposes. For instance, you need it for:
-
-- Admission to higher education courses such as undergraduate, postgraduate, diploma, or professional courses
-- Scholarship schemes offered by various government or private organizations based on merit or other criteria
-- Job applications in various sectors such as government, private, or self-employed
-- Other opportunities such as competitive exams, internships, exchange programs, etc.
-
-Therefore, it is essential to download your 12 marksheet online as soon as possible after the results are declared.
- How to Download 12 Marksheet from CBSE Website
-Visit the official website of CBSE at cbse.gov.in or results.cbse.nic.in
-The easiest way to download your 12 marksheet online is to visit the official website of CBSE at cbse.gov.in or results.cbse.nic.in. These websites provide the facility to download your marksheet as a PDF file. You can do this by following these steps:
-
-- Go to cbse.gov.in or results.cbse.nic.in from your browser
-- Click on the link that says "Senior School Certificate Examination (Class XII) Results 2023"
-- Enter your roll number, school number, center number, and admit card ID as given on your admit card
-- Click on submit and view your result on the screen
-- Click on the download icon at the top right corner of the result page
-- Save your marksheet as a PDF file on your device
-
-You can also take a printout of your marksheet for future reference.
- How to Download 12 Marksheet from DigiLocker or UMANG App
-DigiLocker and UMANG are digital platforms that provide access to CBSE mark sheets and certificates
-If you want to download your 12 marksheet online from other sources, you can use DigiLocker or UMANG app. These are digital platforms that provide access to CBSE mark sheets and certificates along with other government documents. DigiLocker is a cloud-based platform that allows you to store and access your documents anytime, anywhere. UMANG is a mobile app that provides a single platform for various government services. You can use these platforms to download your marksheet by registering with your mobile number and Aadhaar number.
- You can download your marksheet from DigiLocker or UMANG app by following these steps:
-Open the app and log in with your credentials
-The first step is to open the app on your device and log in with your credentials. If you do not have an account, you can create one by verifying your mobile number and Aadhaar number. You will also need to set a username and password for your account.
- Select CBSE from the list of issuers
-The next step is to select CBSE from the list of issuers on the app. Issuers are the organizations that issue documents on DigiLocker or UMANG. You can find CBSE under the education category on both the apps.
-How to download 12 marksheet from CBSE website
-Download 12 marksheet online using DigiLocker
-CBSE 12 marksheet download 2023
-Download 12 marksheet PDF for free
-CBSE 12 marksheet verification online
-Download 12 marksheet duplicate copy
-CBSE 12 marksheet correction procedure
-Download 12 marksheet with photo
-CBSE 12 marksheet revaluation form
-Download 12 marksheet of previous years
-CBSE 12 marksheet improvement exam
-Download 12 marksheet via UMANG app
-CBSE 12 marksheet migration certificate
-Download 12 marksheet without roll number
-CBSE 12 marksheet name change process
-Download 12 marksheet of compartment exam
-CBSE 12 marksheet lost or damaged
-Download 12 marksheet of private candidates
-CBSE 12 marksheet grace marks policy
-Download 12 marksheet of supplementary exam
-CBSE 12 marksheet sample format
-Download 12 marksheet of different boards
-CBSE 12 marksheet date of issue
-Download 12 marksheet for admission purposes
-CBSE 12 marksheet attestation process
-Download 12 marksheet in excel format
-CBSE 12 marksheet analysis and statistics
-Download 12 marksheet of toppers and merit list
-CBSE 12 marksheet print out quality
-Download 12 marksheet with digital signature
-CBSE 12 marksheet validity and recognition
-Download 12 marksheet for scholarship schemes
-CBSE 12 marksheet comparison with other boards
-Download 12 marksheet for foreign universities
-CBSE 12 marksheet FAQs and helpline number
-Download 12 marksheet for NEET and JEE exams
-CBSE 12 marksheet percentage calculator
-Download 12 marksheet for NIOS students
-CBSE 12 marksheet CGPA conversion formula
-Download 12 marksheet for vocational courses
-CBSE 12 marksheet subject codes and abbreviations
-Download 12 marksheet for state quota seats
-CBSE 12 marksheet remarks and abbreviations
-Download 12 marksheet for entrance exams and counselling
-CBSE 12 marksheet division and rank criteria
-Download 12 marksheet for job applications and interviews
-CBSE 12 marksheet security features and authenticity
-Download 12 marksheet for passport and visa applications
-CBSE 12 marksheet evaluation and moderation policy
- Choose class 12 mark sheet from the list of documents
-The third step is to choose class 12 mark sheet from the list of documents available on the app. You will see different types of documents issued by CBSE such as mark sheets, certificates, migration certificates, etc. You need to select class 12 mark sheet for downloading it.
- Enter your roll number and year of examination
-The fourth step is to enter your roll number and year of examination as given on your admit card. You need to enter these details correctly to access your marksheet.
- Download your marksheet as a PDF file
-The final step is to download your marksheet as a PDF file on your device. You will see a preview of your marksheet on the screen. You can click on the download icon at the top right corner of the screen and save your marksheet as a PDF file. You can also share or print your marksheet from the app.
- How to Verify and Validate Your 12 Marksheet
-You can verify and validate your 12 marksheet by checking the digital signature on it
-One of the benefits of downloading your 12 marksheet online is that it comes with a digital signature on it. A digital signature is a secure way of authenticating the identity of the issuer and ensuring the integrity of the document. It also prevents any tampering or alteration of the document. You can verify and validate your 12 marksheet by checking the digital signature on it.
- You can do this by following these steps:
-Open the PDF file of your marksheet in Adobe Reader or any other PDF viewer
-The first step is to open the PDF file of your marksheet in Adobe Reader or any other PDF viewer on your device. You can use any software that supports PDF files and digital signatures.
- Click on the signature icon at the top right corner of the document
-The second step is to click on the signature icon at the top right corner of the document. This icon looks like a pen with a check mark on it. It indicates that the document has a digital signature on it.
- Check the details of the signer and the validity status of the signature
-The third step is to check the details of the signer and the validity status of the signature. You will see a pop-up window that shows the name of the signer, the date and time of signing, and the validity status of the signature. The signer should be CBSE or Central Board of Secondary Education. The validity status should be either valid or unknown. If the signature is valid, you will see a green tick mark and a message saying "Signed and all signatures are valid". If the signature is unknown, you will see a question mark and a message saying "At least one signature has problems".
- If the signature is valid, you can trust your marksheet
-The final step is to verify that the signature is valid. If the signature is valid, you can trust your marksheet and use it for your purposes. A valid signature means that the document has not been tampered or altered and that it has been issued by CBSE. You can also view the certificate of the signer by clicking on "Show Certificate" button on the pop-up window.
- If the signature is unknown, you need to check the certificate chain
-If the signature is unknown, you need to check the certificate chain of the signer. A certificate chain is a series of certificates that link the signer to a trusted authority. You can do this by clicking on "Signature Properties" button on the pop-up window and then clicking on "Show Certificate" button. You will see a list of certificates that belong to the signer and their issuers. You need to check that each certificate is valid and trusted by your device. You can also update your trusted root certificates from your device settings or from Adobe Reader settings.
- Conclusion
-Summarize the main points of the article and provide a call to action for the readers
-In conclusion, downloading your 12 marksheet online is a simple and convenient process that you can do from different sources. You can download your marksheet from CBSE website, DigiLocker, or UMANG app by entering your details and saving it as a PDF file. You can also verify and validate your marksheet by checking the digital signature on it. You need to make sure that the signature is valid and trusted by your device. Your 12 marksheet is an important document that you need for various purposes, so download it as soon as possible and keep it safe.
-If you found this article helpful, please share it with your friends and classmates who might need it. Also, if you have any questions or feedback, please leave a comment below. We would love to hear from you.
- FAQs
-Provide five unique FAQs related to the topic with brief answers
-
-- Q: How can I get a hard copy of my 12 marksheet?
-- A: You can get a hard copy of your 12 marksheet from your school or from CBSE regional office. You need to apply for it with a fee and submit a copy of your admit card or online marksheet.
-- Q: How long does it take to download my 12 marksheet online?
-- A: It usually takes a few minutes to download your 12 marksheet online after entering your details. However, it may take longer if there is heavy traffic on the website or app.
-- Q: What if I lose my 12 marksheet or forget my roll number?
-- A: If you lose your 12 marksheet or forget your roll number, you can contact CBSE helpline number at 1800-11-8002 or email at cbsehelpdesk@gmail.com for assistance. You can also visit CBSE website or app and use other details such as name, date of birth, school name, etc. to access your result.
-- Q: What if I find any error or discrepancy in my 12 marksheet?
-- A: If you find any error or discrepancy in your 12 marksheet, such as spelling mistake, wrong marks, missing subject, etc., you can apply for correction or verification from CBSE within 21 days of declaration of result. You need to fill an online application form with a fee and upload supporting documents.
-- Q: What if I am not satisfied with my 12 marks or grades?
-- A: If you are not satisfied with your 12 marks or grades, you can apply for improvement or compartment exam from CBSE within the specified time limit. You need to fill an online application form with a fee and choose the subjects that you want to improve or reappear.
-
197e85843d
-
-
\ No newline at end of file
diff --git a/spaces/congsaPfin/Manga-OCR/logs/Download Lagu MP3 TikTok Terbaru dan Terpopuler - Kumpulan Musik Viral dan Hits.md b/spaces/congsaPfin/Manga-OCR/logs/Download Lagu MP3 TikTok Terbaru dan Terpopuler - Kumpulan Musik Viral dan Hits.md
deleted file mode 100644
index 93ac9638bde0145bd73550fa5e1b5f44eb404597..0000000000000000000000000000000000000000
--- a/spaces/congsaPfin/Manga-OCR/logs/Download Lagu MP3 TikTok Terbaru dan Terpopuler - Kumpulan Musik Viral dan Hits.md
+++ /dev/null
@@ -1,120 +0,0 @@
-
-How to Download Lagu MP3 TikTok for Free
-TikTok is one of the most popular social media platforms in the world, with over one billion users. It allows users to create and share short videos with various effects, filters, stickers, and music. Many people love TikTok because of its catchy and diverse songs, which range from pop hits to indie gems, from rap verses to folk tunes. But what if you want to download lagu mp3 tiktok for free and listen to them offline, without any interruptions or ads?
-download lagu mp3 tiktok
Download Zip ►►►►► https://urlca.com/2uO9VE
-In this article, we will show you how to download lagu mp3 tiktok for free using different methods, such as websites, apps, or software. We will also compare the pros and cons of each method, and give you some tips and tricks for downloading lagu mp3 tiktok efficiently and legally. By the end of this article, you will be able to enjoy your favorite tiktok songs anytime, anywhere.
- Benefits of Downloading Lagu MP3 TikTok
-Enjoy your favorite songs offline
-One of the main benefits of downloading lagu mp3 tiktok is that you can listen to them offline, without needing an internet connection or a tiktok account. This means that you can save your data and battery life, and avoid buffering or loading issues. You can also play your downloaded songs on any device or media player that supports mp3 format, such as your phone, computer, car stereo, or speaker.
-Create your own playlists and mixtapes
-Another benefit of downloading lagu mp3 tiktok is that you can create your own playlists and mixtapes according to your mood, taste, or occasion. You can customize your music library with different genres, artists, or themes. You can also edit, trim, or merge your downloaded songs using various audio editing tools. You can even make your own remixes or mashups using different tiktok songs.
-Share your music with others
-A third benefit of downloading lagu mp3 tiktok is that you can share your music with others easily and conveniently. You can send your downloaded songs to your friends or family via email, messaging apps, or social media. You can also upload your downloaded songs to online platforms such as YouTube, SoundCloud, or Spotify. You can even burn your downloaded songs to CDs or DVDs and give them as gifts.
- Methods of Downloading Lagu MP3 TikTok
-Use a TikTok MP3 downloader website
-One of the easiest and fastest methods of downloading lagu mp3 tiktok is to use a tiktok mp3 downloader website. These are online services that allow you to convert and download any tiktok video into an mp3 file for free. All you need is a web browser and a tiktok video link. Here are the steps to use a tiktok mp3 downloader website:
-
-- Find the tiktok video that you want to download as an mp3. You can use the tiktok app or website to search for the video. You can also use hashtags, keywords, or usernames to find the video.
-- Copy the link of the tiktok video. You can do this by tapping on the share icon and selecting copy link.
-- Open a new tab on your web browser and go to a tiktok mp3 downloader website. There are many websites that offer this service, such as [TikTokToMP3], [TikTokDownloader], or [TikTokMP3].
-- Paste the link of the tiktok video into the input box on the website and click on the download or convert button.
-- Wait for a few seconds while the website processes your request and generates an mp3 file from the tiktok video.
-- Download the mp3 file to your device by clicking on the download or save button. You can also preview the mp3 file before downloading it by clicking on the play button.
-
-Using a tiktok mp3 downloader website is simple and convenient, but it also has some drawbacks. For example, some websites may have pop-up ads, malware, or viruses that can harm your device or compromise your privacy. Some websites may also have limited features, such as low quality, restricted length, or limited downloads. Therefore, you should always use a reliable and reputable website that has good reviews and ratings from other users.
-download lagu mp3 tiktok viral 2023
-download lagu mp3 tiktok terbaru 2023
-download lagu mp3 tiktok tanpa watermark
-download lagu mp3 tiktok tanpa aplikasi
-download lagu mp3 tiktok dari youtube
-download lagu mp3 tiktok dari soundcloud
-download lagu mp3 tiktok dengan ssstik.io
-download lagu mp3 tiktok dengan audiomack
-download lagu mp3 tiktok dengan mp3juices
-download lagu mp3 tiktok dengan converter online
-download lagu mp3 tiktok gratis dan cepat
-download lagu mp3 tiktok gratis dan mudah
-download lagu mp3 tiktok gratis dan legal
-download lagu mp3 tiktok gratis dan aman
-download lagu mp3 tiktok kualitas tinggi
-download lagu mp3 tiktok kualitas terbaik
-download lagu mp3 tiktok kualitas asli
-download lagu mp3 tiktok kualitas hd
-download lagu mp3 tiktok full album
-download lagu mp3 tiktok full bass
-download lagu mp3 tiktok full lirik
-download lagu mp3 tiktok full durasi
-download lagu mp3 tiktok populer 2023
-download lagu mp3 tiktok hits 2023
-download lagu mp3 tiktok trending 2023
-download lagu mp3 tiktok terpopuler 2023
-download lagu mp3 tiktok terhits 2023
-download lagu mp3 tiktok tertrending 2023
-download lagu mp3 tiktok genre pop
-download lagu mp3 tiktok genre dangdut
-download lagu mp3 tiktok genre rock
-download lagu mp3 tiktok genre rap
-download lagu mp3 tiktok genre edm
-download lagu mp3 tiktok artis indonesia
-download lagu mp3 tiktok artis korea
-download lagu mp3 tiktok artis india
-download lagu mp3 tiktok artis malaysia
-download lagu mp3 tiktok artis barat
-download lagu mp3 tiktok soundtrack film
-download lagu mp3 tiktok soundtrack drama korea
-download lagu mp3 tiktok soundtrack anime
-download lagu mp3 tiktok soundtrack game online
-Use a TikTok MP3 downloader app
-Another method of downloading lagu mp3 tiktok is to use a tiktok mp3 downloader app. These are mobile applications that allow you to convert and download any tiktok video into an mp3 file for free. All you need is a smartphone and a tiktok video link. Here are the steps to use a tiktok mp3 downloader app:
-
-- Find and install a tiktok mp3 downloader app on your smartphone. You can search for these apps on the Google Play Store or the App Store, depending on your device. Some examples of these apps are [TikTok MP3 Downloader], [TikTok Music Downloader], or [TikTok MP3 Converter].
-- Open the app and grant it the necessary permissions to access your device's storage, camera, microphone, or other features.
-- Find the tiktok video that you want to download as an mp3. You can use the app's built-in browser or search engine to find the video. You can also use hashtags, keywords, or usernames to find the video.
-- Copy the link of the tiktok video. You can do this by tapping on the share icon and selecting copy link.
-- Paste the link of the tiktok video into the input box on the app and click on the download or convert button.
-- Wait for a few seconds while the app processes your request and generates an mp3 file from the tiktok video.
-- Download the mp3 file to your device by clicking on the download or save button. You can also preview the mp3 file before downloading it by clicking on the play button.
-
-Using a tiktok mp3 downloader app is fast and easy, but it also has some disadvantages. For instance, some apps may have in-app purchases, ads, or subscriptions that can cost you money or annoy you. Some apps may also have poor quality, limited functionality, or compatibility issues with your device or operating system. Therefore, you should always use a trustworthy and updated app that has positive feedback and ratings from other users.
-Use a screen recorder or audio capture software
-A third method of downloading lagu mp3 tiktok is to use a screen recorder or audio capture software. These are programs that allow you to record and save any sound or video that is playing on your device's screen. All you need is a computer and a tiktok video link. Here are the steps to use a screen recorder or audio capture software:
-
-- Find and install a screen recorder or audio capture software on your computer. You can search for these programs on the internet or download them from their official websites. Some examples of these programs are [Audacity], [OBS Studio], or [Camtasia].
-- Open the program and adjust its settings according to your preferences. You can choose the quality, format, destination, or duration of your recording.
-- Find the tiktok video that you want to download as an mp3. You can use any web browser to access the tiktok website or use the tiktok app on your computer if you have it installed. You can also use hashtags, keywords, or usernames to find the video.
-- Copy the link of the tiktok video. You can do this by clicking on the share icon and selecting copy link.
-- Paste the link of the tiktok video into a new tab on your web browser and play the video.
-- Start the screen recorder or audio capture software and select the area or source of your recording. You can choose to record the whole screen, a specific window, or a custom region.
-- Click on the record or capture button and wait for the tiktok video to finish playing. You can also pause, resume, or stop the recording at any time.
-- Save the recording as an mp3 file to your computer by clicking on the save or export button. You can also edit, trim, or rename your recording using the program's features.
-
-Using a screen recorder or audio capture software is flexible and versatile, but it also has some limitations. For example, some programs may have high system requirements, complex interfaces, or steep learning curves that can make them difficult to use. Some programs may also have low quality, large file sizes, or watermarks that can affect your recording. Therefore, you should always use a high-quality and user-friendly program that has good reviews and ratings from other users.
- Comparison of Different Methods of Downloading Lagu MP3 TikTok
-Speed and convenience
-The speed and convenience of downloading lagu mp3 tiktok depend on several factors, such as your internet connection, device performance, and method choice. Generally speaking, using a tiktok mp3 downloader website is the fastest and most convenient method, as it only requires a few clicks and seconds to download an mp3 file from a tiktok video. Using a tiktok mp3 downloader app is also relatively fast and convenient, as it allows you to download an mp3 file from a tiktok video within the app itself. However, using a screen recorder or audio capture software is the slowest and least convenient method, as it requires you to install and run a program on your computer, and record the sound of a tiktok video in real time.
-Quality and format
-The quality and format of downloading lagu mp3 tiktok also vary depending on several factors, such as your device specifications, program settings, and method choice. Generally speaking, using a screen recorder or audio capture software is the best method for quality and format, as it allows you to record and save an mp3 file from a tiktok video in high quality and any format that you want. Using a tiktok mp3 downloader app is also relatively good for quality and format, as it allows you to download an mp3 file from a tiktok video in decent quality and standard format. However, using a tiktok mp3 downloader website is the worst method for quality and format, as it may download an mp3 file from a tiktok video in low quality and limited format.
-Cost and legality
-The cost and legality of downloading lagu mp3 tiktok also differ depending on several factors, such as your country laws, program policies, and method choice. Generally speaking, using a screen recorder or audio capture software is the most expensive and risky method, as it may require you to pay for a license or subscription fee to use the program, and may violate the copyright or terms of service of tiktok or its content creators. Using a tiktok mp3 downloader app is also relatively expensive and risky, as it may require you to pay for in-app purchases or subscriptions to use the app, and may infringe on the intellectual property rights of tiktok or its content creators. However, using a tiktok mp3 downloader website is the cheapest and safest method, as it does not require you to pay for anything to use the website, and may fall under fair use or public domain exceptions of downloading lagu mp3 tiktok.
- Tips and Tricks for Downloading Lagu MP3 TikTok
-Check the source and the license of the song
-Before downloading lagu mp3 tiktok, you should always check the source and the license of the song. The source refers to where the song comes from, such as an original song by a tiktok user, a cover song by another artist, or a sample song from another platform. The license refers to how the song can be used legally by others. Some songs may have no license or restrictions at all (public domain), some songs may have some license or restrictions (creative commons), and some songs may have full license or restrictions (all rights reserved). You should always respect the source and the license of the song when downloading lagu mp3 tiktok.
Choose the best quality and bitrate for your device
-When downloading lagu mp3 tiktok, you should also choose the best quality and bitrate for your device. The quality refers to how clear and crisp the sound of the song is, while the bitrate refers to how much data is used to encode the sound of the song. The higher the quality and bitrate, the better the sound of the song, but also the larger the file size and the more storage space it takes. The lower the quality and bitrate, the worse the sound of the song, but also the smaller the file size and the less storage space it takes. You should always balance the quality and bitrate of the song with your device's capabilities and preferences when downloading lagu mp3 tiktok.
-Organize your downloaded songs and delete unwanted ones
-After downloading lagu mp3 tiktok, you should also organize your downloaded songs and delete unwanted ones. You can use various tools and methods to organize your downloaded songs, such as folders, tags, metadata, or playlists. You can also use various tools and methods to delete unwanted songs, such as trash bins, uninstallers, or cleaners. You should always keep your downloaded songs organized and clean to avoid clutter, confusion, or duplication when downloading lagu mp3 tiktok.
- Conclusion and Call to Action
-In conclusion, downloading lagu mp3 tiktok is a fun and easy way to enjoy your favorite tiktok songs offline. You can use different methods to download lagu mp3 tiktok, such as websites, apps, or software. You can also compare the benefits and drawbacks of each method, such as speed, quality, or cost. You can also follow some tips and tricks to download lagu mp3 tiktok efficiently and legally, such as checking the source, choosing the quality, or organizing the songs.
-If you want to download lagu mp3 tiktok for free, you can start by choosing one of the methods that we have discussed in this article. You can also visit our website for more information and resources on downloading lagu mp3 tiktok. We hope that this article has helped you learn how to download lagu mp3 tiktok for free and enjoy your music offline. Thank you for reading and happy downloading!
- FAQs
-Q: Is it legal to download lagu mp3 tiktok?
-A: It depends on the source and the license of the song that you want to download. Some songs may be free to download and use for personal purposes, while some songs may require permission or payment from the original creators or owners. You should always respect the intellectual property rights of others when downloading lagu mp3 tiktok.
-Q: What is the best website to download lagu mp3 tiktok?
-A: There is no definitive answer to this question, as different websites may have different features, advantages, or disadvantages. However, some of the most popular and reliable websites to download lagu mp3 tiktok are [TikTokToMP3], [TikTokDownloader], or [TikTokMP3]. You should always use a website that has good reviews and ratings from other users.
-Q: What is the best app to download lagu mp3 tiktok?
-A: There is no definitive answer to this question, as different apps may have different features, advantages, or disadvantages. However, some of the most popular and trustworthy apps to download lagu mp3 tiktok are [TikTok MP3 Downloader], [TikTok Music Downloader], or [TikTok MP3 Converter]. You should always use an app that has positive feedback and ratings from other users.
-Q: What is the best software to download lagu mp3 tiktok?
-A: There is no definitive answer to this question, as different software may have different features, advantages, or disadvantages. However, some of the most popular and high-quality software to download lagu mp3 tiktok are [Audacity], [OBS Studio], or [Camtasia]. You should always use a software that has good reviews and ratings from other users.
-Q: How can I edit or remix my downloaded lagu mp3 tiktok?
-A: You can use various audio editing tools or software to edit or remix your downloaded lagu mp3 tiktok. Some examples of these tools or software are [Audacity], [GarageBand], or [FL Studio]. You can also use online platforms such as [Soundtrap], [BandLab], or [Soundation] to edit or remix your downloaded lagu mp3 tiktok.
401be4b1e0
-
-
\ No newline at end of file
diff --git a/spaces/congsaPfin/Manga-OCR/logs/Download iOS 16 The Ultimate Guide to Personalize Your iPhone.md b/spaces/congsaPfin/Manga-OCR/logs/Download iOS 16 The Ultimate Guide to Personalize Your iPhone.md
deleted file mode 100644
index 7e51e0fb73c2df24fa1bff36e19a96d5ff7b9741..0000000000000000000000000000000000000000
--- a/spaces/congsaPfin/Manga-OCR/logs/Download iOS 16 The Ultimate Guide to Personalize Your iPhone.md
+++ /dev/null
@@ -1,239 +0,0 @@
-
-How to Download iOS 16 on Your iPhone
-Apple has recently released iOS 16, the latest version of its mobile operating system for the iPhone. iOS 16 brings a lot of new features and improvements that make your iPhone more personal, intelligent, and seamless. Some of the highlights include a redesigned lock screen with widgets, live activities, and focus modes; an enhanced messages app with editing and deletion options; a new live text and visual look up feature that lets you interact with text, objects, and scenes in images; an iCloud shared photo library that lets you share photos with your family or friends; and many more.
-download 1os 16
Download File 🗸 https://urlca.com/2uO4KD
-If you are wondering how to download iOS 16 on your iPhone, you have come to the right place. In this article, we will show you how to check your iPhone compatibility, back up your data, connect to Wi-Fi and plug in your device, download and install iOS 16, enjoy the new features, troubleshoot common problems, and answer some frequently asked questions. Let's get started!
- Check Your iPhone Compatibility
-Before you download iOS 16, you need to make sure that your iPhone model is compatible with the new software. iOS 16 supports the following iPhone models:
-
-- iPhone X
-- iPhone XR
-- iPhone XS
-- iPhone XS Max
-- iPhone 11
-- iPhone 11 Pro
-- iPhone 11 Pro Max
-- iPhone 12 mini
-- iPhone 12
-- iPhone 12 Pro
-- iPhone 12 Pro Max
-- iPhone 13 mini
-- iPhone 13
-- iPhone 13 Pro
-- iPhone 13 Pro Max
-- iPhone 14
-- iPhone 14 Plus
-- iPhone 14 Pro
-- iPhone 14 Pro Max
-- iPhone SE (2nd generation)
-- iPhone SE (3rd generation)
-- iPhone 8
-- iPhone 8 Plus
-
- If you have one of these models, you can proceed to the next step. If not, you will have to stick with your current iOS version or upgrade your device.
-How to download ios 16 on iPhone
-Download ios 16 beta version
-Download ios 16 wallpapers and widgets
-Download ios 16 update without wifi
-Download ios 16 ipsw file
-Download ios 16 for iPad
-Download ios 16 without developer account
-Download ios 16 release date and features
-Download ios 16 from Apple website
-Download ios 16 problems and solutions
-Download ios 16 backup and restore
-Download ios 16 compatible devices
-Download ios 16 jailbreak tool
-Download ios 16 live activities and focus filters
-Download ios 16 shared photo library and messages
-Download ios 16 dark mode and night shift
-Download ios 16 security and privacy settings
-Download ios 16 Siri and voice control
-Download ios 16 app library and home screen
-Download ios 16 shortcuts and automation
-Download ios 16 FaceTime and SharePlay
-Download ios 16 Safari and tab groups
-Download ios 16 Maps and transit directions
-Download ios 16 Wallet and Apple Pay
-Download ios 16 Health and fitness tracking
-Download ios 16 Photos and editing tools
-Download ios 16 Music and podcasts
-Download ios 16 News and weather apps
-Download ios 16 Mail and calendar apps
-Download ios 16 Notes and reminders apps
-Download ios 16 iCloud and storage management
-Download ios 16 CarPlay and wireless projection
-Download ios 16 AirPods and spatial audio
-Download ios 16 AirTag and Find My app
-Download ios 16 Apple Watch and watchOS update
-Download ios 16 Apple TV and tvOS update
-Download ios 16 Mac and macOS update
-Download ios 16 iPadOS and multitasking features
-Download ios 16 Swift Playgrounds and coding app
-Download ios 16 Accessibility and assistive features
- Back Up Your iPhone Data
-Before you download iOS 16, it is highly recommended that you back up your iPhone data. This way, you can avoid losing any important information or settings in case something goes wrong during the update process. You can back up your iPhone data using iCloud or iTunes, depending on your preference.
-To back up your iPhone data using iCloud, follow these steps:
-
-- Connect your iPhone to a Wi-Fi network.
-- Go to Settings > [your name] > iCloud > iCloud Backup.
-- Make sure that iCloud Backup is turned on.
-- Tap Back Up Now and wait for the backup to complete.
-- You can check the progress and confirm the backup by going to Settings > [your name] > iCloud > iCloud Backup. Under Back Up Now, you should see the date and time of your last backup.
-
- To back up your iPhone data using iTunes, follow these steps:
-
-- Connect your iPhone to your computer using a USB cable.
-- Open iTunes on your computer and select your iPhone from the device list.
-- Click Summary and then click Back Up Now under Manually Back Up and Restore.
-- Wait for the backup to finish. You can check the status of the backup by clicking iTunes > Preferences > Devices. You should see the name of your device with the date and time of the backup.
-
- Connect to Wi-Fi and Plug in Your iPhone
-Once you have backed up your iPhone data, you are ready to download iOS 16. However, before you do that, you should make sure that you have a stable Wi-Fi connection and a power source for your device. This is because downloading and installing iOS 16 can take some time and consume a lot of battery power. If you use cellular data or run out of battery during the update, you may encounter some issues or errors.
-To connect to Wi-Fi, go to Settings > Wi-Fi and turn on Wi-Fi. Then, select a network from the list and enter the password if required.
-To plug in your iPhone, use the original charger and cable that came with your device. Alternatively, you can use a wireless charger if your device supports it.
- Download and Install iOS 16
-Now that you have prepared your iPhone for the update, you can download and install iOS 16 using the Settings app. Here are the steps to follow:
-
-- Go to Settings > General > Software Update.
-- You should see a message saying that iOS 16 is available. Tap Download and Install.
-- If prompted, enter your passcode or agree to the terms and conditions.
-- The download will begin in the background. You can use your device normally while it is downloading, but make sure that you stay connected to Wi-Fi and power.
-- When the download is complete, you will get a notification saying that iOS 16 is ready to install. Tap Install Now or choose a later time if you are not ready yet.
-- Your device will restart and begin installing iOS 16. You will see a progress bar and an estimated time remaining on the screen. Do not turn off or unplug your device during this process.
-- When the installation is done, your device will restart again and display a welcome screen. Follow the on-screen instructions to set up iOS 16 and enjoy the new features.
-
- Enjoy the New Features of iOS 16
-Congratulations! You have successfully downloaded and installed iOS 16 on your iPhone. Now, you can explore some of the new features and improvements that iOS 16 has to offer. Here are some of them:
- Customize Your Lock Screen
-iOS 16 lets you personalize your lock screen with widgets, photos, styles, and live activities. Widgets are small apps that show you relevant information at a glance, such as weather, calendar, news, music, etc. Photos let you display your favorite images from your camera roll or albums on your lock screen. Styles let you change the appearance of your lock screen elements, such as clock, date, notifications, etc. Live activities let you see what's happening around you in real time, such as traffic, sports scores, events, etc.
-To customize your lock screen, go to Settings > Lock Screen and tap Customize. Then, choose what you want to add or change on your lock screen and follow the instructions.
- Edit and Unsend Messages
-iOS 16 gives you more control over your messages that you have sent in the Messages app. You can edit or delete messages that you have sent within a certain time limit, depending on your settings. You can also unsend messages that you have sent to anyone, even if they have read them. This way, you can avoid any mistakes or regrets in your conversations.
-To edit or delete messages, follow these steps:
-
-- Open the Messages app and go to the conversation that contains the message that you want to edit or delete.
-- Tap and hold the message that you want to edit or delete.
-- A menu will appear with options such as Copy, Edit, Delete, Unsend, etc. Tap Edit or Delete, depending on what you want to do.
-- If you tap Edit, you can make changes to your message and then tap Done. If you tap Delete, you can confirm your action by tapping Delete Message.
-- The message will be edited or deleted from your device and the recipient's device. However, if the recipient has turned off message sync or has a different iOS version, they may still see the original message.
-
- To unsend messages, follow these steps:
-
-- Open the Messages app and go to the conversation that contains the message that you want to unsend.
-- Tap and hold the message that you want to unsend.
-- A menu will appear with options such as Copy, Edit, Delete, Unsend, etc. Tap Unsend.
-- A confirmation message will appear saying that the message will be unsent from your device and the recipient's device. Tap Unsend Message to confirm.
-- The message will be unsent from your device and the recipient's device. However, if the recipient has turned off message sync or has a different iOS version, they may still see the message.
-
- Use Live Text and Visual Look Up
-iOS 16 introduces a new feature called Live Text that lets you interact with text in images. You can use the camera or the photos app to recognize text in images and perform actions such as copying, pasting, translating, searching, calling, etc. For example, you can scan a business card and save the contact information, or scan a restaurant menu and translate it into another language.
-To use Live Text, follow these steps:
-
-- Open the Camera or Photos app and point or select an image that contains text.
-- You should see a yellow icon in the bottom right corner of the screen indicating that Live Text is available. Tap the icon to activate Live Text.
-- You should see the text highlighted in yellow on the screen. You can tap and drag to select the text that you want to interact with.
-- A menu will appear with options such as Copy, Translate, Search, Share, etc. Tap the option that you want to use and follow the instructions.
-
- iOS 16 also introduces a new feature called Visual Look Up that lets you interact with objects and scenes in images. You can use the camera or the photos app to recognize objects and scenes in images and get more information about them. For example, you can scan a flower and learn its name and origin, or scan a landmark and get its history and location.
-To use Visual Look Up, follow these steps:
-
-- Open the Camera or Photos app and point or select an image that contains an object or scene.
-- You should see a star icon in the bottom right corner of the screen indicating that Visual Look Up is available. Tap the icon to activate Visual Look Up.
-- You should see a card at the bottom of the screen with information about the object or scene. You can swipe up to see more details or swipe left or right to see other related items.
-- You can tap on any item to get more information about it from sources such as Wikipedia, Google Maps, Apple Music, etc.
-
- Set Up a Focus Mode
-iOS 16 lets you set up a focus mode that filters out notifications and content based on your activity. You can choose from predefined focus modes such as Do Not Disturb, Sleep, Work, Personal, etc., or create your own custom focus mode. You can also customize which people and apps can contact you and which home screens and widgets are visible when you are in a focus mode. This way, you can reduce distractions and stay focused on what matters most.
-To set up a focus mode, follow these steps:
-
-- Go to Settings > Focus.
-- You should see a list of focus modes that are available for you. Tap on one of them or tap Add Focus to create your own.
-- You can customize your focus mode by choosing the following options:
-- Name: You can give your focus mode a name that suits your activity.
-- Color: You can choose a color that represents your focus mode.
-- People: You can select which contacts can reach you when you are in your focus mode. You can also allow calls from certain groups, such as favorites, emergency contacts, etc.
-- Apps: You can select which apps can send you notifications when you are in your focus mode. You can also allow time-sensitive notifications, such as reminders, alarms, etc.
-- Home Screen: You can choose which home screens and widgets are visible when you are in your focus mode. You can also hide notification badges from app icons.
-- Options: You can choose whether to share your focus status with others, dim your lock screen, or pause your focus mode automatically.
-- When to turn on: You can choose when to activate your focus mode manually or automatically based on time, location, or activity.
-
- When you have customized your focus mode, tap Done to save it. You can turn on your focus mode by swiping down from the top right corner of the screen and tapping on the focus icon. You can also switch between different focus modes by tapping and holding the focus icon and selecting one from the list.
- Share Photos with iCloud Shared Photo Library
-iOS 16 lets you share photos with your family or friends using iCloud Shared Photo Library. This is a feature that lets you create a shared album in the Photos app and invite others to view, add, or comment on the photos. You can also see the photos that others have shared with you in the same album. This way, you can keep your memories together and stay connected with your loved ones.
-To share photos with iCloud Shared Photo Library, follow these steps:
-
-- Open the Photos app and go to the Albums tab.
-- Tap the plus icon in the top left corner and select New Shared Album.
-- Give your shared album a name and tap Next.
-- Add the people that you want to share the album with by entering their names, email addresses, or phone numbers. You can also tap the plus icon to select contacts from your list. Tap Create when you are done.
-- Add the photos that you want to share by tapping the plus icon in the bottom center of the screen. You can select photos from your library or take new ones with the camera. Tap Done when you are done.
-- You can also add a caption or a comment to your photos by tapping on them and typing in the text box at the bottom of the screen.
-- You can see the photos that others have shared with you by tapping on their names at the top of the screen. You can also like or comment on their photos by tapping on them and using the icons at the bottom of the screen.
-
- Troubleshoot Common iOS 16 Problems
-While iOS 16 is designed to enhance your iPhone experience, it may also cause some problems or issues for some users. Here are some of the common iOS 16 problems and their solutions:
- Battery Drain
-If you notice that your iPhone battery is draining faster than usual after updating to iOS 16, you may want to check the battery usage and turn off some features that consume battery power. To do this, follow these steps:
-
-- Go to Settings > Battery and tap Battery Usage by App.
-- You should see a list of apps and how much battery they have used in the last 24 hours or 10 days. Tap on any app to see more details and options.
-- If you see an app that is using too much battery, you can turn off its background activity by tapping Background App Refresh and toggling it off. You can also delete or update the app if it is outdated or buggy.
-- You can also turn off some features that consume battery power, such as location services, Bluetooth, Wi-Fi, cellular data, etc., when you are not using them. You can access these features by swiping down from the top right corner of the screen and tapping on their icons.
-- You can also enable Low Power Mode by going to Settings > Battery and toggling it on. This will reduce some visual effects, background activity, and network usage to save battery power.
-
- Wi-Fi or Cellular Issues
-If you have trouble connecting to Wi-Fi or cellular networks after updating to iOS 16, you may want to restart your device, reset network settings, or contact your carrier. To do this, follow these steps:
-
-- To restart your device, press and hold the power button and the volume button until you see a slider on the screen. Drag the slider to turn off your device. Then, press and hold the power button again until you see the Apple logo on the screen.
-- To reset network settings, go to Settings > General > Reset and tap Reset Network Settings. This will erase all your Wi-Fi, Bluetooth, and cellular settings and restore them to their default values. You will have to reconnect to your networks and enter your passwords again.
-- To contact your carrier, go to Settings > Cellular and tap Carrier Services. You should see a list of options to contact your carrier, such as calling, texting, or visiting their website. You can also check if there are any updates or issues with your carrier by tapping Carrier Settings.
-
- App Crashes or Freezes
-If you experience app crashes or freezes after updating to iOS 16, you may want to update, delete, or reinstall the app, or force quit the app. To do this, follow these steps:
-
-- To update the app, go to the App Store and tap Updates. You should see a list of apps that have updates available. Tap Update next to the app that you want to update or tap Update All to update all apps at once.
-- To delete or reinstall the app, go to the Home Screen and tap and hold the app icon until it jiggles. Then, tap the X icon on the top left corner of the app icon and tap Delete. To reinstall the app, go to the App Store and search for the app name. Then, tap the cloud icon next to the app name and tap Install.
-- To force quit the app, swipe up from the bottom of the screen and pause in the middle. You should see a list of apps that are running in the background. Swipe left or right to find the app that you want to quit and swipe up on its preview to close it.
-
- Conclusion
-iOS 16 is a major update that brings a lot of new features and improvements to your iPhone. In this article, we have shown you how to download iOS 16 on your iPhone, enjoy the new features, troubleshoot common problems, and answer some frequently asked questions. We hope that you have found this article helpful and informative. If you have any questions or feedback, please feel free to leave a comment below. Thank you for reading!
- FAQs
-Here are some of the frequently asked questions and their answers about iOS 16:
- Q: How can I check if I have iOS 16 on my iPhone?
-A: You can check if you have iOS 16 on your iPhone by going to Settings > General > About. You should see the software version number next to Version. If it says 16.x.x, then you have iOS 16 on your iPhone.
- Q: How can I downgrade from iOS 16 to iOS 15?
-A: You can downgrade from iOS 16 to iOS 15 by using iTunes on your computer. However, you need to have a backup of your iPhone data that was made before you updated to iOS 16. Otherwise, you may lose some data or settings during the downgrade process. To downgrade from iOS 16 to iOS 15, follow these steps:
-
-- Connect your iPhone to your computer using a USB cable.
-- Open iTunes on your computer and select your iPhone from the device list.
-- Click Summary and then click Restore iPhone.
-- A message will appear asking if you want to restore your iPhone to factory settings and install iOS 15. Click Restore and Update.
-- Wait for iTunes to download and install iOS 15 on your iPhone. Your device will restart several times during this process.
-- When the restore is complete, you can set up your iPhone as new or restore it from a backup that was made before you updated to iOS 16.
-
- Q: How can I update my apps for iOS 16?
-A: You can update your apps for iOS 16 by going to the App Store and tapping Updates. You should see a list of apps that have updates available for iOS 16. Tap Update next to the app that you want to update or tap Update All to update all apps at once.
- Q: How can I free up space on my iPhone for iOS 16?
-A: You can free up space on your iPhone for iOS 16 by deleting unwanted apps, photos, videos, music, messages, etc., or using iCloud storage or other cloud services. To delete unwanted items, follow these steps:
-
-- To delete apps, go to the Home Screen and tap and hold the app icon until it jiggles. Then, tap the X icon on the top left corner of the app icon and tap Delete.
-- To delete photos, videos, music, etc., open the Photos, Videos, Music, etc. app and select the items that you want to delete. Then, tap the trash icon at the bottom right corner of the screen and tap Delete.
-- To delete messages, open the Messages app and go to the conversation that contains the messages that you want to delete. Tap and hold the message that you want to delete and tap More. Then, select the messages that you want to delete and tap the trash icon at the bottom left corner of the screen and tap Delete.
-
- To use iCloud storage or other cloud services, follow these steps:
-
-- To use iCloud storage, go to Settings > [your name] > iCloud and turn on iCloud for the apps that you want to store your data in the cloud. You can also buy more iCloud storage if you need more space by tapping Manage Storage > Change Storage Plan.
-- To use other cloud services, such as Google Drive, Dropbox, OneDrive, etc., download their apps from the App Store and sign in with your account. Then, upload your data to their servers and delete them from your device if you want to save space.
-
- Q: How can I report a bug or give feedback on iOS 16?
-A: You can report a bug or give feedback on iOS 16 by using the Feedback Assistant app on your iPhone. This is an app that lets you send detailed reports and suggestions to Apple about iOS 16. To use Feedback Assistant, follow these steps:
-
-- Open the Feedback Assistant app on your iPhone. If you don't have it, you can download it from the App Store.
-- Tap New Feedback and choose a category and a topic for your feedback.
-- Fill in the required fields, such as title, description, steps to reproduce, expected results, actual results, etc. You can also attach screenshots or videos to illustrate your feedback.
-- Tap Submit when you are done. You should see a confirmation message saying that your feedback has been sent to Apple.
-- You can check the status of your feedback by tapping My Feedback in the app. You can also edit or delete your feedback by tapping on it and using the options at the bottom of the screen.
-
401be4b1e0
-
-
\ No newline at end of file
diff --git a/spaces/congsaPfin/Manga-OCR/logs/GTA 5 Mobile - Grand Theft Auto The Official APK Game from Rockstar Games.md b/spaces/congsaPfin/Manga-OCR/logs/GTA 5 Mobile - Grand Theft Auto The Official APK Game from Rockstar Games.md
deleted file mode 100644
index 76aedfa390e1c0ff9358b449a666379ead1add38..0000000000000000000000000000000000000000
--- a/spaces/congsaPfin/Manga-OCR/logs/GTA 5 Mobile - Grand Theft Auto The Official APK Game from Rockstar Games.md
+++ /dev/null
@@ -1,117 +0,0 @@
-
-GTA 5 Clone APK Download: Is It Worth It?
- GTA 5 is one of the most popular and successful games of all time. Released in 2013 by Rockstar Games, GTA 5 is an open-world action-adventure game that lets you explore the fictional city of Los Santos and its surrounding areas. You can play as one of three protagonists: Michael, a retired bank robber; Franklin, a street hustler; or Trevor, a psychopathic criminal. You can also switch between them at any time and experience different perspectives and missions.
-gta 5 clone apk download
Download Zip ✒ ✒ ✒ https://urlca.com/2uOeIP
- GTA 5 has received critical acclaim and commercial success for its stunning graphics, immersive gameplay, rich story, diverse characters, and online multiplayer mode. It has sold over 140 million copies worldwide and has won numerous awards and accolades. It is also one of the most streamed and watched games on platforms like YouTube and Twitch.
- However, despite its popularity and availability on various platforms like PC, PS4, PS5, Xbox One, Xbox Series X/S, PS3, and Xbox 360, GTA 5 is not officially released for Android devices. This means that you cannot download or play GTA 5 on your smartphone or tablet from the Google Play Store or any other official source.
- But this does not stop some people from trying to find ways to play GTA 5 on their Android devices. One of the most common methods that people use is downloading GTA 5 clone APKs. These are unofficial versions of GTA 5 that are created by independent developers or hackers who claim to have ported or copied the game from other platforms.
- GTA 5 clone APKs are usually advertised as free downloads that let you enjoy GTA 5 on your Android device without any hassle. They may also promise features like high graphics quality, smooth performance, unlimited money, cheats, mods, and more. Some of these APKs may even look very similar to the original game in terms of appearance and interface.
-gta 5 mobile grand theft auto apk for android
-gta 5 adventure game by rockstar games apk
-gta 5 bluestacks app player download for pc
-gta 5 criminal underworld simulation apk
-gta 5 3d graphics and soundtracks apk
-gta 5 advanced keymapping feature apk
-gta 5 michael trevor franklin protagonists apk
-gta 5 open world sandbox gameplay apk
-gta 5 weapons and vehicles access apk
-gta 5 missions and secrets discovery apk
-gta 5 net energy gain fusion experiment apk
-gta 5 mini sun creation apk
-gta 5 kstar facility korea institute of fusion energy apk
-gta 5 nuclear fusion reaction temperature apk
-gta 5 holy grail experiment apk
-gta 5 racing and piloting activities apk
-gta 5 side quests and minigames apk
-gta 5 conversations with npcs apk
-gta 5 los santos fictional city apk
-gta 5 los santos countryside exploration apk
-gta 5 fire-breathing abilities apk
-gta 5 dragon images creation apk
-gta 5 smaug toothless mushu dragons apk
-gta 5 mythical creatures depiction apk
-gta 5 wings scales horns features apk
-gta 5 diphenhydramine hydrochloride or citrate ingredients apk
-gta 5 benadryl allergy relief product apk
-gta 5 antihistamine and sedative effects apk
-gta 5 allergic reactions and insomnia treatment apk
-gta 5 dosage and precautions information apk
-gta 5 bing search engine integration apk
-gta 5 web search and image search options apk
-gta 5 news search and weather search features apk
-gta 5 question answering and graphic art tools apk
-gta 5 markdown rendering elements support apk
-gta 5 latex math expressions display apk
-gta 5 code blocks for formatted content apk
-gta 5 poems stories code essays songs content generation apk
-gta 5 rewriting improving optimizing content assistance apk
-gta 5 subjective opinions avoidance apk
-gta 5 objective facts and phrases usage apk
-gta 5 positive polite empathetic interesting entertaining engaging responses apk
-gta 5 rigorous and intelligent logic and reasoning apk
-gta 5 safety and limitations awareness apk
-gta 5 harmful content prevention apk
-gta 5 respectful content creation for influential people apk
-gta 5 language preference detection and communication apk
-gta 5 one message reply per conversation turn rule apk
-gta 5 predefined tools mention refusal policy apk
- But are these GTA 5 clone APKs worth
downloading them? The answer is no. GTA 5 clone APKs are not only illegal and unethical, but also dangerous and risky. Here are some of the reasons why you should avoid downloading GTA 5 clone APKs and what you can do instead to play GTA 5 on your Android device legally and safely.
- How to identify fake GTA 5 clone APKs and avoid them?
- There are many fake GTA 5 clone APKs on the internet that claim to be the real deal, but they are actually scams, malware, or viruses. These APKs can harm your device, steal your data, or infect your system with unwanted ads, pop-ups, or ransomware. They can also expose you to legal issues, as downloading or distributing pirated or counterfeit games is a violation of intellectual property rights and can result in fines or lawsuits.
- So how can you identify and avoid these fake GTA 5 clone APKs? Here are some tips:
-
-- Check the source and reviews of the APK: Before downloading any APK, make sure that it comes from a reputable and trustworthy source. Avoid shady or unknown websites that offer GTA 5 clone APKs for free or with suspicious links. Also, check the reviews and ratings of the APK from other users who have downloaded it. If the APK has low ratings, negative feedback, or no reviews at all, it is likely a fake or malicious one.
-- Scan the APK for malware and viruses: Even if the source and reviews of the APK seem legit, it is still advisable to scan the APK for any malware or viruses before installing it on your device. You can use a reliable antivirus or anti-malware app to scan the APK file and detect any potential threats. If the scan results show any red flags or warnings, do not install the APK on your device.
-- Do not provide personal or financial information to the APK: Some GTA 5 clone APKs may ask you to provide your personal or financial information, such as your name, email, phone number, credit card details, or bank account details. They may claim that this is necessary to verify your identity, activate the game, or unlock some features. However, this is a common phishing technique that aims to steal your identity, money, or accounts. Do not provide any sensitive information to any GTA 5 clone APKs, as they are not authorized by Rockstar Games or any official entity.
-
- How to play GTA 5 on Android devices legally and safely?
- If you want to play GTA 5 on your Android device legally and safely, there is a way to do so without downloading any GTA 5 clone APKs. You can use official apps that allow you to stream GTA 5 from your PC or console to your Android device via Wi-Fi or Bluetooth. These apps include Steam Link, PS Remote Play, and Xbox Game Pass.
- Here is how you can use these apps to play GTA 5 on your Android device:
-
-
-App
-Description
-Requirements
-
-
-Steam Link
-This app lets you stream your Steam games from your PC to your Android device over a local network. You can use touch controls or connect a compatible controller to your device.
-You need a PC with Steam installed and running, a compatible Android device with the Steam Link app installed, a strong Wi-Fi connection, and a Steam account with GTA 5 purchased.
-
-
-PS Remote Play
-This app lets you stream your PS4 or PS5 games from your console to your Android device over a Wi-Fi network. You can use touch controls or connect a compatible controller to your device.
-You need a PS4 or PS5 console with GTA 5 installed and running, a compatible Android device with the PS Remote Play app installed, a high-speed Wi-Fi connection, and a PlayStation Network account with GTA 5 purchased.
-
-
-Xbox Game Pass
-This app lets you stream over 100 Xbox games from the cloud to your Android device over a Wi-Fi or mobile data network. You can use touch controls or connect a compatible controller to your device.
-You need a compatible Android device with the Xbox Game Pass app installed, a stable Wi-Fi or mobile data connection, and an Xbox Game Pass Ultimate subscription with GTA 5 included.
-
-
- To use any of these apps, you need to follow these steps:
-
-- Download and install the app on your Android device from the Google Play Store.
-- Launch the app and sign in with your Steam, PlayStation Network, or Xbox Game Pass account.
- Connect your PC or console to your Android device via Wi-Fi or Bluetooth. For Steam Link, you need to pair your device with your PC using a code. For PS Remote Play, you need to enable remote play on your console settings. For Xbox Game Pass, you need to select the cloud gaming option on the app.
-- Select GTA 5 from your game library and start streaming it to your Android device. You can adjust the video quality, audio settings, and control options on the app.
-- Enjoy GTA 5 on your Android device with customized controls and settings. You can also use voice chat, text chat, or screen capture features on the app.
-
- Conclusion
- GTA 5 is a fantastic game that deserves to be played on any platform. However, downloading GTA 5 clone APKs is not the way to do it. GTA 5 clone APKs are fake, illegal, and dangerous versions of GTA 5 that can harm your device, steal your data, or infect your system with malware or viruses. They can also expose you to legal issues, as downloading or distributing pirated or counterfeit games is a violation of intellectual property rights and can result in fines or lawsuits.
- If you want to play GTA 5 on your Android device legally and safely, you should use official apps that allow you to stream GTA 5 from your PC or console to your Android device via Wi-Fi or Bluetooth. These apps include Steam Link, PS Remote Play, and Xbox Game Pass. These apps are authorized by Rockstar Games and offer a high-quality, smooth, and secure gaming experience. You can also customize the controls and settings of GTA 5 according to your preference and convenience.
- So don't fall for the trap of GTA 5 clone APKs. They are not worth it. Instead, use the official apps to play GTA 5 on your Android device and enjoy the game as it is meant to be enjoyed.
- FAQs
-
-- Q: Is GTA 5 available for Android devices?
-- A: No, GTA 5 is not officially released for Android devices by Rockstar Games.
-- Q: Can I download GTA 5 for free on my Android device?
-- A: No, GTA 5 is not free to download or play on any platform. You need to purchase the game from the official store or website.
-- Q: What are the benefits of playing GTA 5 on Android devices?
-- A: Playing GTA 5 on Android devices can give you more mobility, convenience, and flexibility. You can play GTA 5 anywhere and anytime with your Android device.
-- Q: What are the disadvantages of playing GTA 5 on Android devices?
-- A: Playing GTA 5 on Android devices can have some drawbacks, such as lower graphics quality, smaller screen size, limited battery life, and potential lag or connection issues.
-- Q: What are some alternatives to GTA 5 for Android devices?
-- A: Some alternatives to GTA 5 for Android devices are GTA San Andreas, GTA Vice City, GTA III, GTA Chinatown Wars, and GTA Liberty City Stories. These are official GTA games that are ported to Android devices by Rockstar Games.
-
197e85843d
-
-
\ No newline at end of file
diff --git a/spaces/congsaPfin/Manga-OCR/logs/Race with Friends in BB Racing the Official Sequel to Beach Buggy Blitz.md b/spaces/congsaPfin/Manga-OCR/logs/Race with Friends in BB Racing the Official Sequel to Beach Buggy Blitz.md
deleted file mode 100644
index 27f1c6bc597ae2e6d19efd43890f70d759c39562..0000000000000000000000000000000000000000
--- a/spaces/congsaPfin/Manga-OCR/logs/Race with Friends in BB Racing the Official Sequel to Beach Buggy Blitz.md
+++ /dev/null
@@ -1,83 +0,0 @@
-
-BB Racing Download: How to Enjoy High-Speed Fun in the Sun
-If you're pining for the summer, BB Racing will give you a fix of sand, surf, and seagull smashing. This is a kart-racing game that will take you on an action-packed adventure in a tropical island. In this article, we will tell you everything you need to know about BB Racing, how to download and install it on your device, how to play it, and why you should play it.
-bb racing download
Download ⭐ https://urlca.com/2uO5pC
- What is BB Racing?
-BB Racing is a kart-racing game that is developed by Vector Unit, the same studio that created Riptide GP and Shine Runner. It is the official sequel to Beach Buggy Blitz, the free driving game with over 30 million players worldwide. Here are some of the features of BB Racing:
- A kart-racing game with crazy power-ups and tropical tracks
-In BB Racing, you will drive into a world of off-road kart racing mayhem. You will race against a field of rival drivers, each with unique personalities and special abilities. You will also build a collection of crazy power-ups, like Dodgeball Frenzy, Fireball, and Oil Slick. You will test your skills in 6 different game modes on 15 imaginative 3D race tracks, against a pack of tropical-loving rivals with a serious case of road rage.
- The official sequel to Beach Buggy Blitz
-BB Racing is the official sequel to Beach Buggy Blitz, the free driving game that lets you drive your hot-rod beach buggy as far as you can into the uncharted depths of a mysterious tropical island. You can explore sun-swept beaches, secret caves, fog-shrouded swamps, ruined temples, and erupting volcanoes in this action-packed quest of discovery and mayhem.
- Available for free on various platforms
-BB Racing is available for free on various platforms, including Android, iOS, and Windows. You can download it from the Google Play Store, the App Store, or the Microsoft Store. You can also enjoy this game for free, plus hundreds more free of ads and in-app purchases, with a Google Play Pass subscription. You can try it free for 1 month.
-beach buggy racing download
-bb racing game download
-bb racing 2 download
-bb racing apk download
-bb racing mod apk download
-bb racing for pc download
-bb racing for android download
-bb racing for windows download
-bb racing for ios download
-bb racing for mac download
-bb racing free download
-bb racing online download
-bb racing offline download
-bb racing latest version download
-bb racing old version download
-bb racing hack download
-bb racing cheats download
-bb racing unlimited coins download
-bb racing unlimited gems download
-bb racing all cars unlocked download
-bb racing full game download
-bb racing 3d game download
-bb racing kart game download
-bb racing multiplayer game download
-bb racing split screen game download
-beach buggy racing 2 download
-beach buggy racing apk download
-beach buggy racing mod apk download
-beach buggy racing for pc download
-beach buggy racing for android download
-beach buggy racing for windows download
-beach buggy racing for ios download
-beach buggy racing for mac download
-beach buggy racing free download
-beach buggy racing online download
-beach buggy racing offline download
-beach buggy racing latest version download
-beach buggy racing old version download
-beach buggy racing hack download
-beach buggy racing cheats download
-beach buggy racing unlimited coins download
-beach buggy racing unlimited gems download
-beach buggy racing all cars unlocked download
-beach buggy racing full game download
-beach buggy racing 3d game download
-beach buggy racing kart game download
- How to download and install BB Racing?
-Downloading and installing BB Racing is easy and simple. Just follow these steps:
- For Android devices
-
-- Go to the Google Play Store app on your device.
-- Search for "BB Racing" or use this link.
-- Tap on "Install" and wait for the download to finish.
-- Tap on "Open" or find the app icon on your home screen or app drawer.
-- Enjoy playing BB Racing!
-
- For iOS devices
-
-- Go to the App Store app on your device.
-- Search for "BB Racing" or use this link.
-- Tap on "Get" and enter your Apple ID password if prompted.
-- Wait for the download to finish.
-- Tap on "Open" or find the app icon on your home screen.
-- Enjoy playing BB Racing!
and achievements. You can also watch ads or complete offers to get more coins and gems.
-- How do I use my driver's special ability?
You can use your driver's special ability by tapping on the icon on the bottom right corner of the screen. You can only use it once it is fully charged, which depends on your driver's level and the power-ups you collect.
-- How do I play split screen multiplayer?
You can play split screen multiplayer by tapping on the multiplayer icon on the main menu. You can then choose the number of players, the game mode, and the race track. You will need to connect additional controllers or use touch controls for each player.
-- How do I connect with Google Play game services?
You can connect with Google Play game services by tapping on the Google Play icon on the main menu. You will need to sign in with your Google account and grant the necessary permissions. You can then access the achievements, leaderboards, and leagues features.
-
- I hope you enjoyed this article and learned something new about BB Racing. If you have any feedback or suggestions, please let me know in the comments below. Thank you for reading and happy racing!
401be4b1e0
-
-
\ No newline at end of file
diff --git a/spaces/congsaPfin/Manga-OCR/logs/Real Cricket 22 Mod APK - The Best Cricket Game for Android with Amazing Graphics and Sound.md b/spaces/congsaPfin/Manga-OCR/logs/Real Cricket 22 Mod APK - The Best Cricket Game for Android with Amazing Graphics and Sound.md
deleted file mode 100644
index 0a788698363bfca41d412fa52690430d2623728a..0000000000000000000000000000000000000000
--- a/spaces/congsaPfin/Manga-OCR/logs/Real Cricket 22 Mod APK - The Best Cricket Game for Android with Amazing Graphics and Sound.md
+++ /dev/null
@@ -1,95 +0,0 @@
-
-Real Cricket 22 Mod Game Download: Everything You Need to Know
-If you are a fan of cricket and want to enjoy the most realistic and immersive cricket simulation experience on your mobile device, then you should definitely check out Real Cricket 22. This game is the latest version of the popular Real Cricket series, developed by Nautilus Mobile. It offers a wide range of features and benefits that make it stand out from other cricket games. In this article, we will tell you everything you need to know about Real Cricket 22, including how to download and install the mod game that gives you unlimited access to all the premium features. Read on to find out more.
- What is Real Cricket 22?
-Real Cricket 22 is a cricket game that aims to provide the most authentic and in-depth cricket experience on your mobile phone. It has stunning graphics, realistic animations, dynamic stadiums, live commentary, and various game modes and tournaments to choose from. You can play as your favorite international or domestic team, or create your own team with custom players. You can also compete with other players online in ranked or unranked matches, or join or host your own tournaments with the Real Cricket community. Whether you are a casual or hardcore cricket fan, you will find something to enjoy in Real Cricket 22.
-real cricket 22 mod game download
Download Zip ✅ https://urlca.com/2uOaHH
- Features and benefits of Real Cricket 22
-Some of the features and benefits of Real Cricket 22 are:
-
-- More than 600+ batting shots, allowing you to have unique and advanced batting styles.
-- Motion captured fielding and catching animations, along with spectacular batting shots.
-- Live commentary in English and Hindi from legendary commentators like Sanjay Manjrekar, Aakash Chopra, Vivek Razdan, Danny Morrisson, and Lisa Sthalekar.
-- All-new stadiums that are made to scale with the real-world cricket stadiums across the globe.
-- Shot map that lets you choose desired shots that create a unique batting style for each player. You can also share your presets with your friends.
-- New game elements like daily quests and missions that help you progress in the game and unlock contract cards, commentary cards, gold fragments, currency, and more.
-- Various international and domestic cricket tournaments to choose from, including the RCPL 2022, World Cup 2019, World Test Championship, Ashes, Asia Cup, Champions Cup, Master Cup, RCPL, and the Premier Leagues across the world.
-- Manual fielding and catching options that give you more control over your fielders.
-
- How to download and install Real Cricket 22 mod game
-If you want to download and install Real Cricket 22 mod game that gives you unlimited access to all the premium features, such as gold, platinum shots, unlocked tournaments, etc., then you need to follow these steps:
-real cricket 22 mod apk unlimited money
-real cricket 22 mod apk latest version
-real cricket 22 mod apk all tournaments unlocked
-real cricket 22 mod apk obb download
-real cricket 22 mod apk happymod
-real cricket 22 mod apk android 1
-real cricket 22 mod apk revdl
-real cricket 22 mod apk rexdl
-real cricket 22 mod apk offline
-real cricket 22 mod apk unlimited tickets
-real cricket 22 mod apk world cup 2019
-real cricket 22 mod apk world test championship
-real cricket 22 mod apk ashes
-real cricket 22 mod apk asia cup
-real cricket 22 mod apk champions cup
-real cricket 22 mod apk master cup
-real cricket 22 mod apk rcpl
-real cricket 22 mod apk premier leagues
-real cricket 22 mod apk free download for android
-real cricket 22 mod apk download apkpure
-real cricket 22 mod apk download uptodown
-real cricket 22 mod apk download for pc
-real cricket 22 mod game download for ios
-real cricket 22 hack game download
-real cricket 22 cheat game download
-how to download real cricket 22 mod game
-how to install real cricket 22 mod game
-how to play real cricket 22 mod game
-how to update real cricket 22 mod game
-how to unlock all tournaments in real cricket 22 mod game
-best settings for real cricket 22 mod game
-best team for real cricket 22 mod game
-best players for real cricket 22 mod game
-best bowling tips for real cricket 22 mod game
-best batting tips for real cricket 22 mod game
-best fielding tips for real cricket 22 mod game
-best commentary for real cricket 22 mod game
-best graphics for real cricket 22 mod game
-best sound effects for real cricket 22 mod game
-best modes for real cricket 22 mod game
-
-- Go to [this link](^1^) and download the Real Cricket 22 mod apk file.
-- Go to your device settings and enable installation from unknown sources.
-- Locate the downloaded apk file in your file manager and tap on it to install it.
-- Wait for the installation process to finish and then launch the game.
-- Enjoy playing Real Cricket 22 mod game with all the premium features unlocked.
-
- Tips and tricks for playing Real Cricket 22 mod game
-If you want to improve your skills and performance in Real Cricket 22 mod game, then you should follow these tips and tricks:
- Choose your preferred mode and tournament
-Real Cricket 22 offers various modes and tournaments for different types of players. You can choose from Test cricket, One Day International, Twenty20 International, Limited overs (domestic), or Challenger mode. Each. mode has its own rules, challenges, and rewards. You can also choose from various tournaments, such as the World Cup, the Ashes, the Asia Cup, the Champions Cup, the RCPL, and the Premier Leagues. Each tournament has its own format, teams, and schedule. You can select your preferred mode and tournament based on your interest, skill level, and time availability.
- Customize your batting and bowling styles
-Real Cricket 22 allows you to customize your batting and bowling styles according to your preference and strategy. You can choose from more than 600+ batting shots, including classic, modern, innovative, and platinum shots. You can also select your bowling style from fast, medium, spin, or swing. You can adjust your speed, length, line, swing, and spin according to the pitch condition and the batsman's weakness. You can also use special deliveries like yorkers, bouncers, doosras, googlies, etc. to surprise your opponent.
- Use the shot map and manual fielding options
-Real Cricket 22 gives you more control over your shots and fielders with the shot map and manual fielding options. The shot map lets you choose your desired shots by tapping on the screen. You can also share your presets with your friends and challenge them to play with your style. The manual fielding option lets you move your fielders around the ground and catch the ball yourself. You can also use the dive and slide features to save runs and take wickets.
- Complete quests and missions to earn rewards
-Real Cricket 22 has a new feature called quests and missions that help you progress in the game and unlock various rewards. Quests are daily tasks that you can complete to earn gold fragments, currency, contract cards, commentary cards, etc. Missions are long-term goals that you can achieve to earn platinum shots, unlocked tournaments, legendary players, etc. You can check your quests and missions in the game menu and track your progress.
- Conclusion
-Real Cricket 22 is a game that every cricket lover should try. It offers a realistic and immersive cricket simulation experience on your mobile device. It has amazing features and benefits that make it stand out from other cricket games. You can download and install Real Cricket 22 mod game that gives you unlimited access to all the premium features by following the steps mentioned above. You can also follow the tips and tricks to improve your skills and performance in the game. So what are you waiting for? Download Real Cricket 22 mod game today and enjoy playing cricket like never before.
- FAQs
-Here are some frequently asked questions about Real Cricket 22 mod game:
-
-- Q: Is Real Cricket 22 mod game safe to download and install?
-- A: Yes, Real Cricket 22 mod game is safe to download and install as long as you use a trusted source like [this link]. However, you should always be careful when downloading any mod apk file from unknown sources as they may contain viruses or malware that can harm your device.
-- Q: How much space does Real Cricket 22 mod game require on my device?
-- A: Real Cricket 22 mod game requires about 700 MB of free space on your device. You should also have enough RAM and processor speed to run the game smoothly.
-- Q: Can I play Real Cricket 22 mod game offline?
-- A: Yes, you can play Real Cricket 22 mod game offline without any internet connection. However, some features like online multiplayer mode, live commentary, daily quests, etc., may not work offline.
-- Q: Can I play Real Cricket 22 mod game with my friends?
-- A: Yes, you can play Real Cricket 22 mod game with your friends online or offline. You can invite them to join or host your own tournaments with the Real Cricket community feature. You can also challenge them to play with your custom batting and bowling styles using the shot map feature.
-- Q: How can I update Real Cricket 22 mod game?
-- A: To update Real Cricket 22 mod game, you need to download and install the latest version of the mod apk file from [this link]. You should also backup your game data before updating to avoid losing any progress or settings.
-
401be4b1e0
-
-
\ No newline at end of file
diff --git a/spaces/congsaPfin/Manga-OCR/logs/The Ultimate Guide to Downloading Knives Out.md b/spaces/congsaPfin/Manga-OCR/logs/The Ultimate Guide to Downloading Knives Out.md
deleted file mode 100644
index d3e109d8b68cf577189959c187242cc46d93b91a..0000000000000000000000000000000000000000
--- a/spaces/congsaPfin/Manga-OCR/logs/The Ultimate Guide to Downloading Knives Out.md
+++ /dev/null
@@ -1,166 +0,0 @@
-
-Where to Download Knives Out
- Knives Out is a popular franchise that includes a movie and a game, both of which are full of mystery, suspense, and fun. Whether you want to watch a clever whodunit with an all-star cast, or play a thrilling battle royale on your mobile device, Knives Out has something for you. In this article, we will show you where to download Knives Out movie and game, and give you some tips and tricks to enjoy them.
- Knives Out movie
- Knives Out is a 2019 American mystery film written and directed by Rian Johnson, who also serves as a co-producer. It follows master detective Benoit Blanc investigating the death of the patriarch of a wealthy, dysfunctional family. The film features an ensemble cast which includes Daniel Craig as Blanc, with Chris Evans, Ana de Armas, Jamie Lee Curtis, Michael Shannon, Don Johnson, Toni Collette, LaKeith Stanfield, Katherine Langford, Jaeden Martell, and Christopher Plummer.
-where to download knives out
Download ••• https://urlca.com/2uOdRZ
- The film received universal acclaim, particularly for its screenplay, direction, and acting, and grossed $311.9 million worldwide on a $40 million budget. It was named one of the top ten films of 2019 by the National Board of Review and the American Film Institute. It received three nominations at the 77th Golden Globe Awards, including Best Motion Picture – Musical or Comedy, and received Best Original Screenplay nominations at the 73rd British Academy Film Awards and 92nd Academy Awards.
- Where to stream or buy the movie online
- If you want to watch Knives Out online, you have several options. You can stream it on Amazon Prime Video in the US, Canada, and UK. You can also rent or buy it on various platforms such as Apple TV, Google Play Movies, YouTube, Vudu, Microsoft Store, Redbox, DIRECTV, FlixFling, and Alamo on Demand. The prices vary depending on the platform and the quality (SD/HD/4K), but generally range from $3.99 to $14.99.
- Comparison of different streaming platforms and prices
-
-
-Platform
-Rent Price
-Buy Price
-Quality
-
-
-Amazon Prime Video
-$3.99
-$8.99
-HD
-
-
-Apple TV
-$3.99
-$8.99
-HD/4K
-
-
-Google Play Movies
-$3.99
-$9.99
-HD/4K
-
-
-YouTube
-$3.99
-$9.99
-HD/4K
-
-
-Vudu
-$3.99
-$9.99
-HD/4K
-
-
-Microsoft Store
-$3.99
-$9.99
-HD/4K
-
-
-Redbox
-$3.99/$4.99*
-N/A*
-HD/ 4K
-
-
-DIRECTV
-$3.99
-N/A
-HD
-
-
-FlixFling
-$3.99
-$9.99
-HD
-
-
-Alamo on Demand
-$3.99
-$9.99
-HD
-
-
- *Redbox also offers physical DVD and Blu-ray rentals for $1.80 and $2.00 per night, respectively.
- As you can see, there are many options to choose from, depending on your preference and budget. However, if you are an Amazon Prime member, you can stream Knives Out for free as part of your subscription. That's a great deal, considering the high quality and popularity of the movie.
- Knives Out game
- Knives Out is also a 2017 mobile game developed by NetEase Games, which is one of the most downloaded and played games in the world. It is a battle royale game, where 100 players parachute onto an island and fight to be the last one standing. The game features various modes, maps, weapons, vehicles, and customization options. You can play solo, duo, or squad with your friends or other players online.
-How to download Knives Out movie online
-Knives Out full movie free download
-Knives Out Netflix download link
-Download Knives Out from Internet Archive
-Knives Out 2019 movie download HD
-Watch Knives Out online free streaming
-Knives Out torrent download magnet
-Download Knives Out subtitles in English
-Knives Out movie download 1080p
-Knives Out film download mp4
-Knives Out movie download in Hindi
-Download Knives Out on Amazon Prime Video
-Knives Out movie download for free
-Watch and download Knives Out 2019
-Download Knives Out movie on iPhone
-Knives Out movie download with subtitles
-Download Knives Out on Google Drive
-Knives Out movie download in Tamil
-Download Knives Out on Hulu
-Knives Out movie download 720p
-Download Knives Out on Fmovies
-Knives Out movie download in Telugu
-Download Knives Out on Putlocker
-Knives Out movie download in Malayalam
-Download Knives Out on Vudu
-Knives Out movie download in Spanish
-Download Knives Out on 123movies
-Knives Out movie download in French
-Download Knives Out on YTS
-Knives Out movie download in Urdu
-Download Knives Out on Popcorn Time
-Knives Out movie download in Arabic
-Download Knives Out on Movierulz
-Knives Out movie download in Korean
-Download Knives Out on Gomovies
-Knives Out movie download in Chinese
-Download Knives Out on Solarmovie
-Knives Out movie download in Russian
-Download Knives Out on Xmovies8
-Knives Out movie download in Japanese
-Download Knives Out on Yesmovies
-Knives Out movie download in German
-Download Knives Out on FMovies.to
-Knives Out movie download in Italian
-Download Knives Out on MovieMora
-Knives Out movie download in Portuguese
-Download Knives Out on Soap2day
-Knives Out movie download in Turkish
-Download Knives Out on AZMovies
- The game is praised for its realistic graphics, smooth gameplay, and diverse content. It is also constantly updated with new features and events to keep the players engaged. The game has won several awards, such as the Google Play Best of 2017 Game Award and the 15th IMGA Global Best Game Award. It has over 100 million downloads and 4.1 stars rating on Google Play Store.
- Where to download the game for free on mobile devices
- The best part about Knives Out game is that it is free to download and play on both Android and iOS devices. You can download it from the official website, or from the Google Play Store or the App Store. The game requires at least 2 GB of RAM and 3 GB of storage space to run smoothly. The game also supports cross-platform play, meaning you can play with your friends who have different devices.
- Tips and tricks for playing the game
- If you want to improve your skills and win more matches in Knives Out game, here are some tips and tricks to help you out:
-
-- Choose a good landing spot. Avoid crowded areas where you might encounter enemies right away. Look for places with good loot and cover.
-- Loot quickly and efficiently. Don't waste time picking up items you don't need or switching weapons too often. Focus on getting a decent weapon, armor, helmet, backpack, and healing items.
-- Stay in the safe zone. The safe zone is marked by a white circle on the map, and it shrinks over time. If you are outside the safe zone, you will take damage from the storm. Keep an eye on the timer and the map, and move accordingly.
-- Use vehicles wisely. Vehicles can help you travel faster and run over enemies, but they also make a lot of noise and attract attention. Use them when necessary, but don't rely on them too much.
-- Communicate with your teammates. If you are playing in a duo or squad mode, communication is key. Use the voice chat or the quick chat feature to coordinate your actions and share information with your teammates.
-- Be stealthy and strategic. Don't shoot at every enemy you see, as you might reveal your position and get ambushed by other players. Choose your battles carefully, and use cover, smoke grenades, and snipers to your advantage.
-- Have fun and enjoy the game. Don't get frustrated if you lose or die early. Learn from your mistakes and try again. Remember that it's just a game, and the main goal is to have fun.
-
- Conclusion
- Knives Out is a franchise that offers both a movie and a game that are entertaining and engaging for different audiences. Whether you prefer watching a witty murder mystery with a star-studded cast, or playing a thrilling battle royale with your friends or strangers online, Knives Out has something for you.
- If you want to watch Knives Out movie online, you can stream it on Amazon Prime Video for free if you are a member, or rent or buy it on various platforms such as Apple TV, Google Play Movies, YouTube, Vudu, Microsoft Store , Redbox, DIRECTV, FlixFling, and Alamo on Demand. The prices vary depending on the platform and the quality, but generally range from $3.99 to $14.99. You can also compare the different streaming platforms and prices using the table we provided above.
- If you want to play Knives Out game on your mobile device, you can download it for free from the official website, or from the Google Play Store or the App Store. The game requires at least 2 GB of RAM and 3 GB of storage space to run smoothly. The game also supports cross-platform play, meaning you can play with your friends who have different devices. You can also follow our tips and tricks to improve your skills and win more matches in the game.
- So what are you waiting for? Download Knives Out today and enjoy the mystery, suspense, and fun of this franchise. You won't regret it!
- FAQs
- Here are some frequently asked questions about Knives Out:
-
-- Who directed Knives Out?
-Knives Out movie was written and directed by Rian Johnson, who is also known for his films Brick, Looper, and Star Wars: The Last Jedi.
-- Who is Benoit Blanc in Knives Out?
-Benoit Blanc is the main protagonist of Knives Out movie. He is a private detective hired by an anonymous client to investigate the death of Harlan Thrombey, a famous crime novelist and the patriarch of a wealthy family. He is played by Daniel Craig, who is also famous for his role as James Bond.
-- How many sequels are there for Knives Out?
-There are two sequels planned for Knives Out movie, which will follow Benoit Blanc solving new cases with different casts. The sequels were acquired by Netflix for a reported $450 million deal. The filming of the first sequel is expected to begin in June 2021 in Greece.
-- Is Knives Out game related to Knives Out movie?
-No, Knives Out game is not related to Knives Out movie. They are both part of the same franchise, but they have different genres, stories, and characters. The game is a battle royale game, while the movie is a mystery film.
-- What are the best weapons in Knives Out game?
-The best weapons in Knives Out game depend on your personal preference and play style. However, some of the most popular and powerful weapons are the M4A1 assault rifle, the AWM sniper rifle, the M1887 shotgun, the MP5 submachine gun, and the Desert Eagle pistol.
-
197e85843d
-
-
\ No newline at end of file
diff --git a/spaces/congsaPfin/Manga-OCR/logs/mGBA A High-Quality GBA Emulator with Full Compatibility - Download Today.md b/spaces/congsaPfin/Manga-OCR/logs/mGBA A High-Quality GBA Emulator with Full Compatibility - Download Today.md
deleted file mode 100644
index 6a0fffae16a34abcc793de9ca75537920200abcc..0000000000000000000000000000000000000000
--- a/spaces/congsaPfin/Manga-OCR/logs/mGBA A High-Quality GBA Emulator with Full Compatibility - Download Today.md
+++ /dev/null
@@ -1,142 +0,0 @@
-
-How to Download mGBA Emulator for Windows, Mac, Linux, and More
-If you are a fan of retro gaming, you might have heard of mGBA, one of the best emulators for Game Boy Advance games. mGBA is a fast, accurate, and easy-to-use emulator that lets you play your favorite GBA games on various platforms, including Windows, Mac, Linux, Nintendo 3DS, Switch, Wii, and PlayStation Vita. In this article, we will show you how to download and install mGBA on your device of choice.
- Downloading mGBA for Windows
-Windows users have two options to download mGBA: a portable .7z archive or an installer .exe file. Both options are available in 32-bit and 64-bit versions. You can download them from the official website or from the links below:
-download m gba
Download Zip 🗸 https://urlca.com/2uO4DH
-
-- Windows (portable .7z archive)
-- Windows (installer .exe)
-- Windows (64-bit, portable .7z archive)
-- Windows (64-bit, installer .exe)
-
- Choosing the right version of mGBA for Windows
-The portable .7z archive is a compressed file that contains the executable and all the necessary files to run mGBA. You can extract it anywhere on your computer and run it without installing anything. This is useful if you want to keep your emulator settings and saves in one place, or if you want to use mGBA on multiple computers without installing it every time.
-The installer .exe file is a program that will guide you through the installation process of mGBA. It will create a shortcut on your desktop and add an entry to your Start menu. It will also associate .gba files with mGBA, so you can open them directly by double-clicking them. This is useful if you want to integrate mGBA with your system and have easy access to it.
-The 32-bit and 64-bit versions refer to the architecture of your processor. Most modern computers have a 64-bit processor, which can run both 32-bit and 64-bit programs. However, some older computers have a 32-bit processor, which can only run 32-bit programs. To find out which version you need, you can check your system information or use this online tool. Generally speaking, the 64-bit version is recommended if your processor supports it, as it may offer better performance and compatibility.
-download m gba emulator for windows
-download m gba latest release
-download m gba source code
-download m gba for mac os
-download m gba development builds
-download m gba for nintendo switch
-download m gba for linux
-download m gba for android
-download m gba for wii
-download m gba for ps vita
-download m gba 0.10.2 bugfix release
-download m gba medusa alpha 2
-download m gba scripting support
-download m gba portable archive
-download m gba installer exe
-download m gba appimage 64-bit
-download m gba ubuntu 20.04
-download m gba nintendo 3ds homebrew
-download m gba opengl renderer fix
-download m gba save state feature
-download m gba cheats and enhancements
-download m gba bios files
-download m gba roms and games
-download m gba pokemon hacks
-download m gba fire emblem patches
-download m gba zelda mods
-download m gba metroid fusion randomizer
-download m gba golden sun editor
-download m gba kirby nightmare in dreamland hack
-download m gba sonic advance 3 mod
-download m gba final fantasy tactics advance patch
-download m gba harvest moon friends of mineral town cheat codes
-download m gba castlevania aria of sorrow hack
-download m gba super mario advance 4 e-reader levels
-download m gba wario land 4 editor
-download m gba mega man zero 3 mod
-download m gba kingdom hearts chain of memories patch
-download m gba dragon ball z buu's fury cheat codes
-download m gba yu-gi-oh gx duel academy hack
-download m gba pokemon emerald kaizo rom hack
- Installing mGBA on Windows
-If you downloaded the portable .7z archive, you will need a program like 7-Z Zip
or WinRAR to extract the archive. Once you have extracted it, you can run mGBA.exe to launch the emulator. You can also create a shortcut to it on your desktop or anywhere else for convenience.
-If you downloaded the installer .exe file, you just need to run it and follow the instructions on the screen. You can choose where to install mGBA and whether to create a desktop shortcut or not. Once the installation is complete, you can run mGBA from the Start menu or the desktop shortcut.
- Downloading mGBA for Mac
-Mac users can download mGBA as a .dmg file, which is a disk image that contains the application bundle. You can download it from the official website or from the link below:
-
-- Mac (dmg)
-
- Choosing the right version of mGBA for Mac
-The .dmg file is compatible with macOS 10.10 (Yosemite) or later. If you have an older version of macOS, you may need to use an older version of mGBA or try another emulator. You can check your macOS version by clicking on the Apple logo in the top left corner and selecting About This Mac.
- Installing mGBA on Mac
-To install mGBA on Mac, you need to open the .dmg file and drag the mGBA icon to the Applications folder. This will copy the application bundle to your system. You can then run mGBA from the Applications folder or create a shortcut to it on your dock or desktop.
- Downloading mGBA for Linux
-Linux users have several options to download mGBA, depending on their distribution and preference. You can download a .tar.xz archive, a .deb package, a .rpm package, a Flatpak package, or a Snap package. You can also use your package manager to install mGBA from your distribution's repository, if available. You can download these options from the official website or from the links below:
-
-- Linux (tar.xz archive)
-- Linux (deb package)
-- Linux (rpm package)
-- Linux (Flatpak package)
-- Linux (Snap package)
-
- Choosing the right version of mGBA for Linux
-The .tar.xz archive is a compressed file that contains the executable and all the necessary files to run mGBA. You can extract it anywhere on your computer and run it without installing anything. This is useful if you want to keep your emulator settings and saves in one place, or if you want to use mGBA on multiple computers without installing it every time.
-The .deb package is a software package that can be installed on Debian-based distributions, such as Ubuntu, Mint, Pop!_OS, etc. You can install it using your graphical package manager or using the command line with dpkg or apt.
-The .rpm package is a software package that can be installed on Red Hat-based distributions, such as Fedora, CentOS, openSUSE, etc. You can install it using your graphical package manager or using the command line with rpm or yum.
-The Flatpak package is a software package that can be installed on any Linux distribution that supports Flatpak, which is a universal app platform. You can install it using your graphical package manager or using the command line with flatpak.
-The Snap package is a software package that can be installed on any Linux distribution that supports Snap, which is another universal app platform. You can install it using your graphical package manager or using the command line with snap.
- Installing mGBA on Linux
-If you downloaded the .tar.xz archive, you will need a program like XZ Utils or Ark to extract the archive. Once you have extracted it, you can run ./mGBA in a terminal to launch the emulator. You can also create a shortcut to it on your desktop or anywhere else for convenience.
-If you downloaded the .deb package, you can install it by double-clicking it and following the instructions on the screen. Alternatively, you can open a terminal and enter sudo dpkg -i .deb or sudo apt install ./.deb, where is the name of the downloaded file. This will install mGBA on your system and create a shortcut in your applications menu. You can then run mGBA from there or create a shortcut to it on your dock or desktop.
-If you downloaded the .rpm package, you can install it by double-clicking it and following the instructions on the screen. Alternatively, you can open a terminal and enter sudo rpm -i .rpm or sudo yum install ./.rpm, where is the name of the downloaded file. This will install mGBA on your system and create a shortcut in your applications menu. You can then run mGBA from there or create a shortcut to it on your dock or desktop.
-If you downloaded the Flatpak package, you can install it by double-clicking it and following the instructions on the screen. Alternatively, you can open a terminal and enter flatpak install .flatpak, where is the name of the downloaded file. This will install mGBA on your system and create a shortcut in your applications menu. You can then run mGBA from there or create a shortcut to it on your dock or desktop.
-If you downloaded the Snap package, you can install it by double-clicking it and following the instructions on the screen. Alternatively, you can open a terminal and enter snap install .snap, where is the name of the downloaded file. This will install mGBA on your system and create a shortcut in your applications menu. You can then run mGBA from there or create a shortcut to it on your dock or desktop.
- Downloading mGBA for Other Platforms
-mGBA is also available for some other platforms, such as Nintendo 3DS, Switch, Wii, and PlayStation Vita. These platforms require some additional steps and tools to run mGBA, as they are not officially supported by the emulator. You can also download the source code of mGBA and compile it yourself, or use the development builds of mGBA and medusa, which are experimental versions of the emulator with new features and bug fixes.
- Nintendo 3DS, Switch, Wii, and PlayStation Vita
-To run mGBA on these platforms, you will need to have a homebrew-enabled device, which means that you have modified your device's firmware to allow running unofficial software. This process varies depending on your device and model, and may involve some risks of bricking your device or voiding your warranty. Therefore, we do not recommend doing this unless you know what you are doing and are willing to take full responsibility for any consequences. You can find more information about homebrewing your device on websites like 3DS Hacks Guide, Switch Hacks Guide, Wii Hacks Guide, and Vita Hacks Guide.
- Requirements and limitations of mGBA on homebrew platforms
-Once you have a homebrew-enabled device, you will need to download the appropriate version of mGBA for your platform from the official website or from the links below:
-
-- Nintendo 3DS (cia)
-- Nintendo 3DS (3dsx)
-- Nintendo Switch (nro)
-- Nintendo Wii (dol)
-- PlayStation Vita (vpk)
-
-You will also need to have some GBA games in .gba format to play with mGBA. You can either dump them from your own cartridges using a device like GBA Backup Tool, or download them from online sources (which may be illegal depending on your country's laws).
-Keep in mind that running mGBA on these platforms may have some limitations in terms of performance, compatibility, features, and stability. For example, some games may run slower than normal, some may have graphical glitches or sound issues, some may not work at all, and some features like save states or cheats may not be available or functional. These limitations are due to the hardware and software differences between these platforms and the original GBA hardware.
- How to install and run mGBA on homebrew platforms
-The installation and running process of mGBA varies depending on your platform and homebrew method. Here are some general steps that may apply to most cases:
-
-- Copy the downloaded file of mGBA to your device's SD card or internal memory using a USB cable or a card reader.
-- Copy your GBA games to your device 's SD card or internal memory using a USB cable or a card reader. You can create a folder named mGBA or GBA to organize your games.
-- Launch your homebrew launcher or menu on your device and select mGBA from the list of applications. If you don't see mGBA, you may need to refresh or scan your SD card or internal memory for new applications.
-- Once mGBA is running, you can use the menu to load your GBA games and start playing. You can also adjust the settings, such as video, audio, input, and emulation options, to suit your preferences.
-
- Source code and development builds
-If you are interested in the development of mGBA, or if you want to try the latest features and bug fixes before they are officially released, you can download the source code or the development builds of mGBA and medusa. Medusa is a fork of mGBA that aims to support more platforms, such as Game Boy, Game Boy Color, and Nintendo DS.
- How to get the source code of mGBA
-The source code of mGBA is hosted on GitHub, where you can view, download, clone, fork, or contribute to it. You can also report issues, request features, or submit pull requests there. To download the source code, you can either use the Download ZIP button on GitHub, or use the git command line tool with the following command:
-git clone https://github.com/mgba-emu/mgba.git
-To compile the source code, you will need some tools and libraries, such as CMake, Qt5, SDL2, zlib, libpng, libzip, etc. The exact requirements and instructions vary depending on your platform and configuration. You can find more information on how to build mGBA from source on the official website or on the GitHub wiki.
- How to use the development builds of mGBA and medusa
-The development builds of mGBA and medusa are pre-compiled binaries that are updated regularly with the latest changes from the source code. They are available for Windows, Mac, Linux, 3DS, Switch, Wii, and Vita. You can download them from the official website or from the links below:
-
-To use the development builds, you just need to extract them and run them as you would with the stable releases. However, keep in mind that these builds are experimental and may have bugs, crashes, or compatibility issues that are not present in the stable releases. Therefore, we do not recommend using them for regular gaming or replacing your stable version with them. You should always backup your saves and settings before using them.
- Conclusion and FAQs
-mGBA is a great emulator for Game Boy Advance games that supports a wide range of platforms and features. It is fast, accurate, easy-to-use, and constantly updated by its developer and community. In this article, we have shown you how to download and install mGBA on various platforms, such as Windows, Mac , Linux, and other homebrew platforms. We have also explained how to get the source code and the development builds of mGBA and medusa, if you are interested in the development of the emulator. We hope that this article has helped you to enjoy your GBA games with mGBA.
- Here are some FAQs that you may find useful:
-
-- Q: How do I load and play GBA games with mGBA?
-- A: To load and play GBA games with mGBA, you need to have the game files in .gba format on your device. You can either dump them from your own cartridges or download them from online sources. Then, you can use the File menu or the Load ROM button to browse and select the game file. The game will start automatically and you can use your keyboard, mouse, or controller to play.
-- Q: How do I save and load my game progress with mGBA?
-- A: To save and load your game progress with mGBA, you have two options: in-game saves and save states. In-game saves are the same as saving on a real GBA, where you use the game's own save feature (usually from a menu or a checkpoint). These saves are stored in .sav files in the same folder as your game files. Save states are snapshots of the emulator's state at any point, which you can create and load using the emulator's menu or keyboard shortcuts. These saves are stored in .ss# files in the same folder as your game files.
-- Q: How do I customize the settings and options of mGBA?
-- A: To customize the settings and options of mGBA, you can use the Settings menu or the Tools menu to access various categories, such as video, audio, input, emulation, bios, enhancements, etc. You can change things like window size, fullscreen mode, filters, shaders, sound volume, controller mapping, frame rate, cheats, etc. You can also use the Config menu to save and load different configurations for different games or platforms.
-- Q: How do I use cheats with mGBA?
-- A: To use cheats with mGBA, you need to have cheat codes for your game in .cht format. You can either create them yourself or download them from online sources. Then, you can use the Tools menu or the Cheats button to open the cheat manager. There, you can add, edit, enable, disable, or delete cheats for your game. You can also import or export cheat files from or to your device.
-- Q: How do I update mGBA to the latest version?
-- A: To update mGBA to the latest version, you need to download the new version from the official website or from this article and replace your old version with it. You can also use the Help menu or the Check for Updates button to check if there is a new version available and download it automatically. However, this feature may not work on some platforms or versions.
-
197e85843d
-
-
\ No newline at end of file
diff --git a/spaces/contluForse/HuggingGPT/assets/Download Dil Pardesi Ho Gaya movie utorrent The hidden meanings and messages of the film.md b/spaces/contluForse/HuggingGPT/assets/Download Dil Pardesi Ho Gaya movie utorrent The hidden meanings and messages of the film.md
deleted file mode 100644
index 6c32fafe681d318fe3cf4b8c6260ca3fbc94e2b6..0000000000000000000000000000000000000000
--- a/spaces/contluForse/HuggingGPT/assets/Download Dil Pardesi Ho Gaya movie utorrent The hidden meanings and messages of the film.md
+++ /dev/null
@@ -1,6 +0,0 @@
-downloadDilPardesiHoGayaamovieutorrent
Download ››› https://ssurll.com/2uzyIP
-
- aaccfb2cb3
-
-
-
diff --git a/spaces/coreml-community/ControlNet-v1-1-Annotators-cpu/annotator/oneformer/detectron2/modeling/proposal_generator/proposal_utils.py b/spaces/coreml-community/ControlNet-v1-1-Annotators-cpu/annotator/oneformer/detectron2/modeling/proposal_generator/proposal_utils.py
deleted file mode 100644
index b5579f43f04e4442f897e20672e4ad5b784c029b..0000000000000000000000000000000000000000
--- a/spaces/coreml-community/ControlNet-v1-1-Annotators-cpu/annotator/oneformer/detectron2/modeling/proposal_generator/proposal_utils.py
+++ /dev/null
@@ -1,205 +0,0 @@
-# Copyright (c) Facebook, Inc. and its affiliates.
-import logging
-import math
-from typing import List, Tuple, Union
-import torch
-
-from annotator.oneformer.detectron2.layers import batched_nms, cat, move_device_like
-from annotator.oneformer.detectron2.structures import Boxes, Instances
-
-logger = logging.getLogger(__name__)
-
-
-def _is_tracing():
- # (fixed in TORCH_VERSION >= 1.9)
- if torch.jit.is_scripting():
- # https://github.com/pytorch/pytorch/issues/47379
- return False
- else:
- return torch.jit.is_tracing()
-
-
-def find_top_rpn_proposals(
- proposals: List[torch.Tensor],
- pred_objectness_logits: List[torch.Tensor],
- image_sizes: List[Tuple[int, int]],
- nms_thresh: float,
- pre_nms_topk: int,
- post_nms_topk: int,
- min_box_size: float,
- training: bool,
-):
- """
- For each feature map, select the `pre_nms_topk` highest scoring proposals,
- apply NMS, clip proposals, and remove small boxes. Return the `post_nms_topk`
- highest scoring proposals among all the feature maps for each image.
-
- Args:
- proposals (list[Tensor]): A list of L tensors. Tensor i has shape (N, Hi*Wi*A, 4).
- All proposal predictions on the feature maps.
- pred_objectness_logits (list[Tensor]): A list of L tensors. Tensor i has shape (N, Hi*Wi*A).
- image_sizes (list[tuple]): sizes (h, w) for each image
- nms_thresh (float): IoU threshold to use for NMS
- pre_nms_topk (int): number of top k scoring proposals to keep before applying NMS.
- When RPN is run on multiple feature maps (as in FPN) this number is per
- feature map.
- post_nms_topk (int): number of top k scoring proposals to keep after applying NMS.
- When RPN is run on multiple feature maps (as in FPN) this number is total,
- over all feature maps.
- min_box_size (float): minimum proposal box side length in pixels (absolute units
- wrt input images).
- training (bool): True if proposals are to be used in training, otherwise False.
- This arg exists only to support a legacy bug; look for the "NB: Legacy bug ..."
- comment.
-
- Returns:
- list[Instances]: list of N Instances. The i-th Instances
- stores post_nms_topk object proposals for image i, sorted by their
- objectness score in descending order.
- """
- num_images = len(image_sizes)
- device = (
- proposals[0].device
- if torch.jit.is_scripting()
- else ("cpu" if torch.jit.is_tracing() else proposals[0].device)
- )
-
- # 1. Select top-k anchor for every level and every image
- topk_scores = [] # #lvl Tensor, each of shape N x topk
- topk_proposals = []
- level_ids = [] # #lvl Tensor, each of shape (topk,)
- batch_idx = move_device_like(torch.arange(num_images, device=device), proposals[0])
- for level_id, (proposals_i, logits_i) in enumerate(zip(proposals, pred_objectness_logits)):
- Hi_Wi_A = logits_i.shape[1]
- if isinstance(Hi_Wi_A, torch.Tensor): # it's a tensor in tracing
- num_proposals_i = torch.clamp(Hi_Wi_A, max=pre_nms_topk)
- else:
- num_proposals_i = min(Hi_Wi_A, pre_nms_topk)
-
- topk_scores_i, topk_idx = logits_i.topk(num_proposals_i, dim=1)
-
- # each is N x topk
- topk_proposals_i = proposals_i[batch_idx[:, None], topk_idx] # N x topk x 4
-
- topk_proposals.append(topk_proposals_i)
- topk_scores.append(topk_scores_i)
- level_ids.append(
- move_device_like(
- torch.full((num_proposals_i,), level_id, dtype=torch.int64, device=device),
- proposals[0],
- )
- )
-
- # 2. Concat all levels together
- topk_scores = cat(topk_scores, dim=1)
- topk_proposals = cat(topk_proposals, dim=1)
- level_ids = cat(level_ids, dim=0)
-
- # 3. For each image, run a per-level NMS, and choose topk results.
- results: List[Instances] = []
- for n, image_size in enumerate(image_sizes):
- boxes = Boxes(topk_proposals[n])
- scores_per_img = topk_scores[n]
- lvl = level_ids
-
- valid_mask = torch.isfinite(boxes.tensor).all(dim=1) & torch.isfinite(scores_per_img)
- if not valid_mask.all():
- if training:
- raise FloatingPointError(
- "Predicted boxes or scores contain Inf/NaN. Training has diverged."
- )
- boxes = boxes[valid_mask]
- scores_per_img = scores_per_img[valid_mask]
- lvl = lvl[valid_mask]
- boxes.clip(image_size)
-
- # filter empty boxes
- keep = boxes.nonempty(threshold=min_box_size)
- if _is_tracing() or keep.sum().item() != len(boxes):
- boxes, scores_per_img, lvl = boxes[keep], scores_per_img[keep], lvl[keep]
-
- keep = batched_nms(boxes.tensor, scores_per_img, lvl, nms_thresh)
- # In Detectron1, there was different behavior during training vs. testing.
- # (https://github.com/facebookresearch/Detectron/issues/459)
- # During training, topk is over the proposals from *all* images in the training batch.
- # During testing, it is over the proposals for each image separately.
- # As a result, the training behavior becomes batch-dependent,
- # and the configuration "POST_NMS_TOPK_TRAIN" end up relying on the batch size.
- # This bug is addressed in Detectron2 to make the behavior independent of batch size.
- keep = keep[:post_nms_topk] # keep is already sorted
-
- res = Instances(image_size)
- res.proposal_boxes = boxes[keep]
- res.objectness_logits = scores_per_img[keep]
- results.append(res)
- return results
-
-
-def add_ground_truth_to_proposals(
- gt: Union[List[Instances], List[Boxes]], proposals: List[Instances]
-) -> List[Instances]:
- """
- Call `add_ground_truth_to_proposals_single_image` for all images.
-
- Args:
- gt(Union[List[Instances], List[Boxes]): list of N elements. Element i is a Instances
- representing the ground-truth for image i.
- proposals (list[Instances]): list of N elements. Element i is a Instances
- representing the proposals for image i.
-
- Returns:
- list[Instances]: list of N Instances. Each is the proposals for the image,
- with field "proposal_boxes" and "objectness_logits".
- """
- assert gt is not None
-
- if len(proposals) != len(gt):
- raise ValueError("proposals and gt should have the same length as the number of images!")
- if len(proposals) == 0:
- return proposals
-
- return [
- add_ground_truth_to_proposals_single_image(gt_i, proposals_i)
- for gt_i, proposals_i in zip(gt, proposals)
- ]
-
-
-def add_ground_truth_to_proposals_single_image(
- gt: Union[Instances, Boxes], proposals: Instances
-) -> Instances:
- """
- Augment `proposals` with `gt`.
-
- Args:
- Same as `add_ground_truth_to_proposals`, but with gt and proposals
- per image.
-
- Returns:
- Same as `add_ground_truth_to_proposals`, but for only one image.
- """
- if isinstance(gt, Boxes):
- # convert Boxes to Instances
- gt = Instances(proposals.image_size, gt_boxes=gt)
-
- gt_boxes = gt.gt_boxes
- device = proposals.objectness_logits.device
- # Assign all ground-truth boxes an objectness logit corresponding to
- # P(object) = sigmoid(logit) =~ 1.
- gt_logit_value = math.log((1.0 - 1e-10) / (1 - (1.0 - 1e-10)))
- gt_logits = gt_logit_value * torch.ones(len(gt_boxes), device=device)
-
- # Concatenating gt_boxes with proposals requires them to have the same fields
- gt_proposal = Instances(proposals.image_size, **gt.get_fields())
- gt_proposal.proposal_boxes = gt_boxes
- gt_proposal.objectness_logits = gt_logits
-
- for key in proposals.get_fields().keys():
- assert gt_proposal.has(
- key
- ), "The attribute '{}' in `proposals` does not exist in `gt`".format(key)
-
- # NOTE: Instances.cat only use fields from the first item. Extra fields in latter items
- # will be thrown away.
- new_proposals = Instances.cat([proposals, gt_proposal])
-
- return new_proposals
diff --git a/spaces/cscan/CodeFormer/CodeFormer/facelib/detection/yolov5face/face_detector.py b/spaces/cscan/CodeFormer/CodeFormer/facelib/detection/yolov5face/face_detector.py
deleted file mode 100644
index 0103411e27860898fee470895a7cf59d8be2e11a..0000000000000000000000000000000000000000
--- a/spaces/cscan/CodeFormer/CodeFormer/facelib/detection/yolov5face/face_detector.py
+++ /dev/null
@@ -1,142 +0,0 @@
-import copy
-import os
-from pathlib import Path
-
-import cv2
-import numpy as np
-import torch
-from torch import nn
-
-from facelib.detection.yolov5face.models.common import Conv
-from facelib.detection.yolov5face.models.yolo import Model
-from facelib.detection.yolov5face.utils.datasets import letterbox
-from facelib.detection.yolov5face.utils.general import (
- check_img_size,
- non_max_suppression_face,
- scale_coords,
- scale_coords_landmarks,
-)
-
-IS_HIGH_VERSION = tuple(map(int, torch.__version__.split('+')[0].split('.')[:3])) >= (1, 9, 0)
-
-
-def isListempty(inList):
- if isinstance(inList, list): # Is a list
- return all(map(isListempty, inList))
- return False # Not a list
-
-class YoloDetector:
- def __init__(
- self,
- config_name,
- min_face=10,
- target_size=None,
- device='cuda',
- ):
- """
- config_name: name of .yaml config with network configuration from models/ folder.
- min_face : minimal face size in pixels.
- target_size : target size of smaller image axis (choose lower for faster work). e.g. 480, 720, 1080.
- None for original resolution.
- """
- self._class_path = Path(__file__).parent.absolute()
- self.target_size = target_size
- self.min_face = min_face
- self.detector = Model(cfg=config_name)
- self.device = device
-
-
- def _preprocess(self, imgs):
- """
- Preprocessing image before passing through the network. Resize and conversion to torch tensor.
- """
- pp_imgs = []
- for img in imgs:
- h0, w0 = img.shape[:2] # orig hw
- if self.target_size:
- r = self.target_size / min(h0, w0) # resize image to img_size
- if r < 1:
- img = cv2.resize(img, (int(w0 * r), int(h0 * r)), interpolation=cv2.INTER_LINEAR)
-
- imgsz = check_img_size(max(img.shape[:2]), s=self.detector.stride.max()) # check img_size
- img = letterbox(img, new_shape=imgsz)[0]
- pp_imgs.append(img)
- pp_imgs = np.array(pp_imgs)
- pp_imgs = pp_imgs.transpose(0, 3, 1, 2)
- pp_imgs = torch.from_numpy(pp_imgs).to(self.device)
- pp_imgs = pp_imgs.float() # uint8 to fp16/32
- return pp_imgs / 255.0 # 0 - 255 to 0.0 - 1.0
-
- def _postprocess(self, imgs, origimgs, pred, conf_thres, iou_thres):
- """
- Postprocessing of raw pytorch model output.
- Returns:
- bboxes: list of arrays with 4 coordinates of bounding boxes with format x1,y1,x2,y2.
- points: list of arrays with coordinates of 5 facial keypoints (eyes, nose, lips corners).
- """
- bboxes = [[] for _ in range(len(origimgs))]
- landmarks = [[] for _ in range(len(origimgs))]
-
- pred = non_max_suppression_face(pred, conf_thres, iou_thres)
-
- for image_id, origimg in enumerate(origimgs):
- img_shape = origimg.shape
- image_height, image_width = img_shape[:2]
- gn = torch.tensor(img_shape)[[1, 0, 1, 0]] # normalization gain whwh
- gn_lks = torch.tensor(img_shape)[[1, 0, 1, 0, 1, 0, 1, 0, 1, 0]] # normalization gain landmarks
- det = pred[image_id].cpu()
- scale_coords(imgs[image_id].shape[1:], det[:, :4], img_shape).round()
- scale_coords_landmarks(imgs[image_id].shape[1:], det[:, 5:15], img_shape).round()
-
- for j in range(det.size()[0]):
- box = (det[j, :4].view(1, 4) / gn).view(-1).tolist()
- box = list(
- map(int, [box[0] * image_width, box[1] * image_height, box[2] * image_width, box[3] * image_height])
- )
- if box[3] - box[1] < self.min_face:
- continue
- lm = (det[j, 5:15].view(1, 10) / gn_lks).view(-1).tolist()
- lm = list(map(int, [i * image_width if j % 2 == 0 else i * image_height for j, i in enumerate(lm)]))
- lm = [lm[i : i + 2] for i in range(0, len(lm), 2)]
- bboxes[image_id].append(box)
- landmarks[image_id].append(lm)
- return bboxes, landmarks
-
- def detect_faces(self, imgs, conf_thres=0.7, iou_thres=0.5):
- """
- Get bbox coordinates and keypoints of faces on original image.
- Params:
- imgs: image or list of images to detect faces on with BGR order (convert to RGB order for inference)
- conf_thres: confidence threshold for each prediction
- iou_thres: threshold for NMS (filter of intersecting bboxes)
- Returns:
- bboxes: list of arrays with 4 coordinates of bounding boxes with format x1,y1,x2,y2.
- points: list of arrays with coordinates of 5 facial keypoints (eyes, nose, lips corners).
- """
- # Pass input images through face detector
- images = imgs if isinstance(imgs, list) else [imgs]
- images = [cv2.cvtColor(img, cv2.COLOR_BGR2RGB) for img in images]
- origimgs = copy.deepcopy(images)
-
- images = self._preprocess(images)
-
- if IS_HIGH_VERSION:
- with torch.inference_mode(): # for pytorch>=1.9
- pred = self.detector(images)[0]
- else:
- with torch.no_grad(): # for pytorch<1.9
- pred = self.detector(images)[0]
-
- bboxes, points = self._postprocess(images, origimgs, pred, conf_thres, iou_thres)
-
- # return bboxes, points
- if not isListempty(points):
- bboxes = np.array(bboxes).reshape(-1,4)
- points = np.array(points).reshape(-1,10)
- padding = bboxes[:,0].reshape(-1,1)
- return np.concatenate((bboxes, padding, points), axis=1)
- else:
- return None
-
- def __call__(self, *args):
- return self.predict(*args)
diff --git a/spaces/daarumadx/bot/src/daemon.py b/spaces/daarumadx/bot/src/daemon.py
deleted file mode 100644
index 17900cfa60d0498add777c54bca118e8867e7dfb..0000000000000000000000000000000000000000
--- a/spaces/daarumadx/bot/src/daemon.py
+++ /dev/null
@@ -1,108 +0,0 @@
-"""daemon logic."""
-import copy
-import os
-import sys
-import time
-
-from watchdog.events import FileSystemEventHandler
-from watchdog.observers import Observer
-
-from config import Config as Conf
-from processing.folder import FolderImageProcessing
-from transform.gan.mask import CorrectToMask, MaskrefToMaskdet, MaskfinToNude
-from transform.opencv.correct import DressToCorrect
-from transform.opencv.mask import MaskToMaskref, MaskdetToMaskfin
-
-
-class Watcher:
- """Watch a directory change."""
-
- def __init__(self, watching_dir, out_dir):
- """
- Watcher constructor.
-
- :param watching_dir: directory to watch
- :param out_dir: directory where save transformations
- """
- self.__observer = Observer()
- self.__watching_dir = watching_dir
- self.__out_dir = out_dir
-
- for k, v in {"Watched": self.__watching_dir, "Output": self.__out_dir}.items():
- Conf.log.info("{} Directory Is {}".format(k, v))
- if not os.path.isdir(self.__watching_dir):
- Conf.log.error("{} Directory {} Doesn't Exit.".format(k, v))
- sys.exit(0)
-
- def run(self):
- """
- Run the Watcher.
-
- :return: None
- """
- event_handler = Handler(self.__out_dir)
- self.__observer.schedule(event_handler, self.__watching_dir, recursive=True)
- self.__observer.start()
- try:
- while True:
- time.sleep(5)
- except KeyboardInterrupt:
- self.__observer.stop()
- except Exception as e:
- self.__observer.stop()
- Conf.log.error(e) # type: ignore
- Conf.log.error("An Unhandled Error Occurred.") # type: ignore
- sys.exit(1)
- self.__observer.join()
-
-
-class Handler(FileSystemEventHandler):
- """Handle a change in a watch directory."""
-
- def __init__(self, out_dir):
- """
- Create an Handler.
-
- :param out_dir: directory where save transformations
- """
- self.__out_dir = out_dir
- self.__phases = [
- DressToCorrect, CorrectToMask, MaskToMaskref, MaskrefToMaskdet, MaskdetToMaskfin, MaskfinToNude
- ]
-
- def on_created(self, event):
- """
- Call when a file or directory is created.
-
- :param event: trigger event
- :return: None
- """
- if not event.is_directory:
- Conf.log.debug("Received file created event {}.".format(event.src_path))
- if os.path.basename(event.src_path) == ".start":
- os.remove(event.src_path)
-
- start = time.time()
- Conf.log.info("Execution Of {} Folder.".format(os.path.dirname(event.src_path)))
- args = copy.deepcopy(Conf.args)
- args.update({
- "input": os.path.dirname(event.src_path),
- "output": self.__out_dir,
- })
-
- FolderImageProcessing(args=args).run()
-
- Conf.log.success("Execution of {} Folder Done in {}.".format(
- os.path.dirname(event.src_path), round(time.time() - start, 2)
- ))
-
-
-def main(_):
- """
- Start daemon main logic.
-
- :param _: None
- :return: None
- """
- Conf.log.info("Welcome to Dreampower Daemon")
- Watcher(Conf.args['input'], Conf.args['output']).run()
diff --git a/spaces/daddyjin/TalkingFaceGeneration/Demo_TFR_Pirenderer/src/face3d/models/arcface_torch/dataset.py b/spaces/daddyjin/TalkingFaceGeneration/Demo_TFR_Pirenderer/src/face3d/models/arcface_torch/dataset.py
deleted file mode 100644
index 96bbb8bb6da99122f350bc8e1a6390245840e32b..0000000000000000000000000000000000000000
--- a/spaces/daddyjin/TalkingFaceGeneration/Demo_TFR_Pirenderer/src/face3d/models/arcface_torch/dataset.py
+++ /dev/null
@@ -1,124 +0,0 @@
-import numbers
-import os
-import queue as Queue
-import threading
-
-import mxnet as mx
-import numpy as np
-import torch
-from torch.utils.data import DataLoader, Dataset
-from torchvision import transforms
-
-
-class BackgroundGenerator(threading.Thread):
- def __init__(self, generator, local_rank, max_prefetch=6):
- super(BackgroundGenerator, self).__init__()
- self.queue = Queue.Queue(max_prefetch)
- self.generator = generator
- self.local_rank = local_rank
- self.daemon = True
- self.start()
-
- def run(self):
- torch.cuda.set_device(self.local_rank)
- for item in self.generator:
- self.queue.put(item)
- self.queue.put(None)
-
- def next(self):
- next_item = self.queue.get()
- if next_item is None:
- raise StopIteration
- return next_item
-
- def __next__(self):
- return self.next()
-
- def __iter__(self):
- return self
-
-
-class DataLoaderX(DataLoader):
-
- def __init__(self, local_rank, **kwargs):
- super(DataLoaderX, self).__init__(**kwargs)
- self.stream = torch.cuda.Stream(local_rank)
- self.local_rank = local_rank
-
- def __iter__(self):
- self.iter = super(DataLoaderX, self).__iter__()
- self.iter = BackgroundGenerator(self.iter, self.local_rank)
- self.preload()
- return self
-
- def preload(self):
- self.batch = next(self.iter, None)
- if self.batch is None:
- return None
- with torch.cuda.stream(self.stream):
- for k in range(len(self.batch)):
- self.batch[k] = self.batch[k].to(device=self.local_rank, non_blocking=True)
-
- def __next__(self):
- torch.cuda.current_stream().wait_stream(self.stream)
- batch = self.batch
- if batch is None:
- raise StopIteration
- self.preload()
- return batch
-
-
-class MXFaceDataset(Dataset):
- def __init__(self, root_dir, local_rank):
- super(MXFaceDataset, self).__init__()
- self.transform = transforms.Compose(
- [transforms.ToPILImage(),
- transforms.RandomHorizontalFlip(),
- transforms.ToTensor(),
- transforms.Normalize(mean=[0.5, 0.5, 0.5], std=[0.5, 0.5, 0.5]),
- ])
- self.root_dir = root_dir
- self.local_rank = local_rank
- path_imgrec = os.path.join(root_dir, 'train.rec')
- path_imgidx = os.path.join(root_dir, 'train.idx')
- self.imgrec = mx.recordio.MXIndexedRecordIO(path_imgidx, path_imgrec, 'r')
- s = self.imgrec.read_idx(0)
- header, _ = mx.recordio.unpack(s)
- if header.flag > 0:
- self.header0 = (int(header.label[0]), int(header.label[1]))
- self.imgidx = np.array(range(1, int(header.label[0])))
- else:
- self.imgidx = np.array(list(self.imgrec.keys))
-
- def __getitem__(self, index):
- idx = self.imgidx[index]
- s = self.imgrec.read_idx(idx)
- header, img = mx.recordio.unpack(s)
- label = header.label
- if not isinstance(label, numbers.Number):
- label = label[0]
- label = torch.tensor(label, dtype=torch.long)
- sample = mx.image.imdecode(img).asnumpy()
- if self.transform is not None:
- sample = self.transform(sample)
- return sample, label
-
- def __len__(self):
- return len(self.imgidx)
-
-
-class SyntheticDataset(Dataset):
- def __init__(self, local_rank):
- super(SyntheticDataset, self).__init__()
- img = np.random.randint(0, 255, size=(112, 112, 3), dtype=np.int32)
- img = np.transpose(img, (2, 0, 1))
- img = torch.from_numpy(img).squeeze(0).float()
- img = ((img / 255) - 0.5) / 0.5
- self.img = img
- self.label = 1
-
- def __getitem__(self, index):
- return self.img, self.label
-
- def __len__(self):
- return 1000000
diff --git a/spaces/dakaiye/dky_xuexi/request_llm/README.md b/spaces/dakaiye/dky_xuexi/request_llm/README.md
deleted file mode 100644
index 545bc1ffba8b79a49d994cfedcc2a787475181b2..0000000000000000000000000000000000000000
--- a/spaces/dakaiye/dky_xuexi/request_llm/README.md
+++ /dev/null
@@ -1,79 +0,0 @@
-# 如何使用其他大语言模型
-
-## ChatGLM
-
-- 安装依赖 `pip install -r request_llm/requirements_chatglm.txt`
-- 修改配置,在config.py中将LLM_MODEL的值改为"chatglm"
-
-``` sh
-LLM_MODEL = "chatglm"
-```
-- 运行!
-``` sh
-`python main.py`
-```
-
-## Claude-Stack
-
-- 请参考此教程获取 https://zhuanlan.zhihu.com/p/627485689
- - 1、SLACK_CLAUDE_BOT_ID
- - 2、SLACK_CLAUDE_USER_TOKEN
-
-- 把token加入config.py
-
-## Newbing
-
-- 使用cookie editor获取cookie(json)
-- 把cookie(json)加入config.py (NEWBING_COOKIES)
-
-## Moss
-- 使用docker-compose
-
-## RWKV
-- 使用docker-compose
-
-## LLAMA
-- 使用docker-compose
-
-## 盘古
-- 使用docker-compose
-
-
----
-## Text-Generation-UI (TGUI,调试中,暂不可用)
-
-### 1. 部署TGUI
-``` sh
-# 1 下载模型
-git clone https://github.com/oobabooga/text-generation-webui.git
-# 2 这个仓库的最新代码有问题,回滚到几周之前
-git reset --hard fcda3f87767e642d1c0411776e549e1d3894843d
-# 3 切换路径
-cd text-generation-webui
-# 4 安装text-generation的额外依赖
-pip install accelerate bitsandbytes flexgen gradio llamacpp markdown numpy peft requests rwkv safetensors sentencepiece tqdm datasets git+https://github.com/huggingface/transformers
-# 5 下载模型
-python download-model.py facebook/galactica-1.3b
-# 其他可选如 facebook/opt-1.3b
-# facebook/galactica-1.3b
-# facebook/galactica-6.7b
-# facebook/galactica-120b
-# facebook/pygmalion-1.3b 等
-# 详情见 https://github.com/oobabooga/text-generation-webui
-
-# 6 启动text-generation
-python server.py --cpu --listen --listen-port 7865 --model facebook_galactica-1.3b
-```
-
-### 2. 修改config.py
-
-``` sh
-# LLM_MODEL格式: tgui:[模型]@[ws地址]:[ws端口] , 端口要和上面给定的端口一致
-LLM_MODEL = "tgui:galactica-1.3b@localhost:7860"
-```
-
-### 3. 运行!
-``` sh
-cd chatgpt-academic
-python main.py
-```
diff --git a/spaces/dariowsz/speech-to-speech-translation/README.md b/spaces/dariowsz/speech-to-speech-translation/README.md
deleted file mode 100644
index 488d3b5776f68bc881e7ff4e39f11afc54a44403..0000000000000000000000000000000000000000
--- a/spaces/dariowsz/speech-to-speech-translation/README.md
+++ /dev/null
@@ -1,13 +0,0 @@
----
-title: Speech To Speech Translation
-emoji: 🏆
-colorFrom: pink
-colorTo: indigo
-sdk: gradio
-sdk_version: 3.36.1
-app_file: app.py
-pinned: false
-duplicated_from: course-demos/speech-to-speech-translation
----
-
-Check out the configuration reference at https://huggingface.co/docs/hub/spaces-config-reference
diff --git a/spaces/dawood/gradio_videogallery/README.md b/spaces/dawood/gradio_videogallery/README.md
deleted file mode 100644
index 155ccc51543a16b06ea9af75409b938876ab45ff..0000000000000000000000000000000000000000
--- a/spaces/dawood/gradio_videogallery/README.md
+++ /dev/null
@@ -1,10 +0,0 @@
-
----
-tags: [gradio-custom-component, gradio-template-Gallery]
-title: gradio_videogallery V0.0.1
-colorFrom: green
-colorTo: pink
-sdk: docker
-pinned: false
-license: apache-2.0
----
diff --git a/spaces/dawood17/SayBot_Enchancer/CodeFormer/facelib/detection/retinaface/retinaface.py b/spaces/dawood17/SayBot_Enchancer/CodeFormer/facelib/detection/retinaface/retinaface.py
deleted file mode 100644
index 02593556d88a90232bbe55a062875f4af4520621..0000000000000000000000000000000000000000
--- a/spaces/dawood17/SayBot_Enchancer/CodeFormer/facelib/detection/retinaface/retinaface.py
+++ /dev/null
@@ -1,370 +0,0 @@
-import cv2
-import numpy as np
-import torch
-import torch.nn as nn
-import torch.nn.functional as F
-from PIL import Image
-from torchvision.models._utils import IntermediateLayerGetter as IntermediateLayerGetter
-
-from facelib.detection.align_trans import get_reference_facial_points, warp_and_crop_face
-from facelib.detection.retinaface.retinaface_net import FPN, SSH, MobileNetV1, make_bbox_head, make_class_head, make_landmark_head
-from facelib.detection.retinaface.retinaface_utils import (PriorBox, batched_decode, batched_decode_landm, decode, decode_landm,
- py_cpu_nms)
-
-device = torch.device('cuda' if torch.cuda.is_available() else 'cpu')
-
-
-def generate_config(network_name):
-
- cfg_mnet = {
- 'name': 'mobilenet0.25',
- 'min_sizes': [[16, 32], [64, 128], [256, 512]],
- 'steps': [8, 16, 32],
- 'variance': [0.1, 0.2],
- 'clip': False,
- 'loc_weight': 2.0,
- 'gpu_train': True,
- 'batch_size': 32,
- 'ngpu': 1,
- 'epoch': 250,
- 'decay1': 190,
- 'decay2': 220,
- 'image_size': 640,
- 'return_layers': {
- 'stage1': 1,
- 'stage2': 2,
- 'stage3': 3
- },
- 'in_channel': 32,
- 'out_channel': 64
- }
-
- cfg_re50 = {
- 'name': 'Resnet50',
- 'min_sizes': [[16, 32], [64, 128], [256, 512]],
- 'steps': [8, 16, 32],
- 'variance': [0.1, 0.2],
- 'clip': False,
- 'loc_weight': 2.0,
- 'gpu_train': True,
- 'batch_size': 24,
- 'ngpu': 4,
- 'epoch': 100,
- 'decay1': 70,
- 'decay2': 90,
- 'image_size': 840,
- 'return_layers': {
- 'layer2': 1,
- 'layer3': 2,
- 'layer4': 3
- },
- 'in_channel': 256,
- 'out_channel': 256
- }
-
- if network_name == 'mobile0.25':
- return cfg_mnet
- elif network_name == 'resnet50':
- return cfg_re50
- else:
- raise NotImplementedError(f'network_name={network_name}')
-
-
-class RetinaFace(nn.Module):
-
- def __init__(self, network_name='resnet50', half=False, phase='test'):
- super(RetinaFace, self).__init__()
- self.half_inference = half
- cfg = generate_config(network_name)
- self.backbone = cfg['name']
-
- self.model_name = f'retinaface_{network_name}'
- self.cfg = cfg
- self.phase = phase
- self.target_size, self.max_size = 1600, 2150
- self.resize, self.scale, self.scale1 = 1., None, None
- self.mean_tensor = torch.tensor([[[[104.]], [[117.]], [[123.]]]]).to(device)
- self.reference = get_reference_facial_points(default_square=True)
- # Build network.
- backbone = None
- if cfg['name'] == 'mobilenet0.25':
- backbone = MobileNetV1()
- self.body = IntermediateLayerGetter(backbone, cfg['return_layers'])
- elif cfg['name'] == 'Resnet50':
- import torchvision.models as models
- backbone = models.resnet50(pretrained=False)
- self.body = IntermediateLayerGetter(backbone, cfg['return_layers'])
-
- in_channels_stage2 = cfg['in_channel']
- in_channels_list = [
- in_channels_stage2 * 2,
- in_channels_stage2 * 4,
- in_channels_stage2 * 8,
- ]
-
- out_channels = cfg['out_channel']
- self.fpn = FPN(in_channels_list, out_channels)
- self.ssh1 = SSH(out_channels, out_channels)
- self.ssh2 = SSH(out_channels, out_channels)
- self.ssh3 = SSH(out_channels, out_channels)
-
- self.ClassHead = make_class_head(fpn_num=3, inchannels=cfg['out_channel'])
- self.BboxHead = make_bbox_head(fpn_num=3, inchannels=cfg['out_channel'])
- self.LandmarkHead = make_landmark_head(fpn_num=3, inchannels=cfg['out_channel'])
-
- self.to(device)
- self.eval()
- if self.half_inference:
- self.half()
-
- def forward(self, inputs):
- out = self.body(inputs)
-
- if self.backbone == 'mobilenet0.25' or self.backbone == 'Resnet50':
- out = list(out.values())
- # FPN
- fpn = self.fpn(out)
-
- # SSH
- feature1 = self.ssh1(fpn[0])
- feature2 = self.ssh2(fpn[1])
- feature3 = self.ssh3(fpn[2])
- features = [feature1, feature2, feature3]
-
- bbox_regressions = torch.cat([self.BboxHead[i](feature) for i, feature in enumerate(features)], dim=1)
- classifications = torch.cat([self.ClassHead[i](feature) for i, feature in enumerate(features)], dim=1)
- tmp = [self.LandmarkHead[i](feature) for i, feature in enumerate(features)]
- ldm_regressions = (torch.cat(tmp, dim=1))
-
- if self.phase == 'train':
- output = (bbox_regressions, classifications, ldm_regressions)
- else:
- output = (bbox_regressions, F.softmax(classifications, dim=-1), ldm_regressions)
- return output
-
- def __detect_faces(self, inputs):
- # get scale
- height, width = inputs.shape[2:]
- self.scale = torch.tensor([width, height, width, height], dtype=torch.float32).to(device)
- tmp = [width, height, width, height, width, height, width, height, width, height]
- self.scale1 = torch.tensor(tmp, dtype=torch.float32).to(device)
-
- # forawrd
- inputs = inputs.to(device)
- if self.half_inference:
- inputs = inputs.half()
- loc, conf, landmarks = self(inputs)
-
- # get priorbox
- priorbox = PriorBox(self.cfg, image_size=inputs.shape[2:])
- priors = priorbox.forward().to(device)
-
- return loc, conf, landmarks, priors
-
- # single image detection
- def transform(self, image, use_origin_size):
- # convert to opencv format
- if isinstance(image, Image.Image):
- image = cv2.cvtColor(np.asarray(image), cv2.COLOR_RGB2BGR)
- image = image.astype(np.float32)
-
- # testing scale
- im_size_min = np.min(image.shape[0:2])
- im_size_max = np.max(image.shape[0:2])
- resize = float(self.target_size) / float(im_size_min)
-
- # prevent bigger axis from being more than max_size
- if np.round(resize * im_size_max) > self.max_size:
- resize = float(self.max_size) / float(im_size_max)
- resize = 1 if use_origin_size else resize
-
- # resize
- if resize != 1:
- image = cv2.resize(image, None, None, fx=resize, fy=resize, interpolation=cv2.INTER_LINEAR)
-
- # convert to torch.tensor format
- # image -= (104, 117, 123)
- image = image.transpose(2, 0, 1)
- image = torch.from_numpy(image).unsqueeze(0)
-
- return image, resize
-
- def detect_faces(
- self,
- image,
- conf_threshold=0.8,
- nms_threshold=0.4,
- use_origin_size=True,
- ):
- """
- Params:
- imgs: BGR image
- """
- image, self.resize = self.transform(image, use_origin_size)
- image = image.to(device)
- if self.half_inference:
- image = image.half()
- image = image - self.mean_tensor
-
- loc, conf, landmarks, priors = self.__detect_faces(image)
-
- boxes = decode(loc.data.squeeze(0), priors.data, self.cfg['variance'])
- boxes = boxes * self.scale / self.resize
- boxes = boxes.cpu().numpy()
-
- scores = conf.squeeze(0).data.cpu().numpy()[:, 1]
-
- landmarks = decode_landm(landmarks.squeeze(0), priors, self.cfg['variance'])
- landmarks = landmarks * self.scale1 / self.resize
- landmarks = landmarks.cpu().numpy()
-
- # ignore low scores
- inds = np.where(scores > conf_threshold)[0]
- boxes, landmarks, scores = boxes[inds], landmarks[inds], scores[inds]
-
- # sort
- order = scores.argsort()[::-1]
- boxes, landmarks, scores = boxes[order], landmarks[order], scores[order]
-
- # do NMS
- bounding_boxes = np.hstack((boxes, scores[:, np.newaxis])).astype(np.float32, copy=False)
- keep = py_cpu_nms(bounding_boxes, nms_threshold)
- bounding_boxes, landmarks = bounding_boxes[keep, :], landmarks[keep]
- # self.t['forward_pass'].toc()
- # print(self.t['forward_pass'].average_time)
- # import sys
- # sys.stdout.flush()
- return np.concatenate((bounding_boxes, landmarks), axis=1)
-
- def __align_multi(self, image, boxes, landmarks, limit=None):
-
- if len(boxes) < 1:
- return [], []
-
- if limit:
- boxes = boxes[:limit]
- landmarks = landmarks[:limit]
-
- faces = []
- for landmark in landmarks:
- facial5points = [[landmark[2 * j], landmark[2 * j + 1]] for j in range(5)]
-
- warped_face = warp_and_crop_face(np.array(image), facial5points, self.reference, crop_size=(112, 112))
- faces.append(warped_face)
-
- return np.concatenate((boxes, landmarks), axis=1), faces
-
- def align_multi(self, img, conf_threshold=0.8, limit=None):
-
- rlt = self.detect_faces(img, conf_threshold=conf_threshold)
- boxes, landmarks = rlt[:, 0:5], rlt[:, 5:]
-
- return self.__align_multi(img, boxes, landmarks, limit)
-
- # batched detection
- def batched_transform(self, frames, use_origin_size):
- """
- Arguments:
- frames: a list of PIL.Image, or torch.Tensor(shape=[n, h, w, c],
- type=np.float32, BGR format).
- use_origin_size: whether to use origin size.
- """
- from_PIL = True if isinstance(frames[0], Image.Image) else False
-
- # convert to opencv format
- if from_PIL:
- frames = [cv2.cvtColor(np.asarray(frame), cv2.COLOR_RGB2BGR) for frame in frames]
- frames = np.asarray(frames, dtype=np.float32)
-
- # testing scale
- im_size_min = np.min(frames[0].shape[0:2])
- im_size_max = np.max(frames[0].shape[0:2])
- resize = float(self.target_size) / float(im_size_min)
-
- # prevent bigger axis from being more than max_size
- if np.round(resize * im_size_max) > self.max_size:
- resize = float(self.max_size) / float(im_size_max)
- resize = 1 if use_origin_size else resize
-
- # resize
- if resize != 1:
- if not from_PIL:
- frames = F.interpolate(frames, scale_factor=resize)
- else:
- frames = [
- cv2.resize(frame, None, None, fx=resize, fy=resize, interpolation=cv2.INTER_LINEAR)
- for frame in frames
- ]
-
- # convert to torch.tensor format
- if not from_PIL:
- frames = frames.transpose(1, 2).transpose(1, 3).contiguous()
- else:
- frames = frames.transpose((0, 3, 1, 2))
- frames = torch.from_numpy(frames)
-
- return frames, resize
-
- def batched_detect_faces(self, frames, conf_threshold=0.8, nms_threshold=0.4, use_origin_size=True):
- """
- Arguments:
- frames: a list of PIL.Image, or np.array(shape=[n, h, w, c],
- type=np.uint8, BGR format).
- conf_threshold: confidence threshold.
- nms_threshold: nms threshold.
- use_origin_size: whether to use origin size.
- Returns:
- final_bounding_boxes: list of np.array ([n_boxes, 5],
- type=np.float32).
- final_landmarks: list of np.array ([n_boxes, 10], type=np.float32).
- """
- # self.t['forward_pass'].tic()
- frames, self.resize = self.batched_transform(frames, use_origin_size)
- frames = frames.to(device)
- frames = frames - self.mean_tensor
-
- b_loc, b_conf, b_landmarks, priors = self.__detect_faces(frames)
-
- final_bounding_boxes, final_landmarks = [], []
-
- # decode
- priors = priors.unsqueeze(0)
- b_loc = batched_decode(b_loc, priors, self.cfg['variance']) * self.scale / self.resize
- b_landmarks = batched_decode_landm(b_landmarks, priors, self.cfg['variance']) * self.scale1 / self.resize
- b_conf = b_conf[:, :, 1]
-
- # index for selection
- b_indice = b_conf > conf_threshold
-
- # concat
- b_loc_and_conf = torch.cat((b_loc, b_conf.unsqueeze(-1)), dim=2).float()
-
- for pred, landm, inds in zip(b_loc_and_conf, b_landmarks, b_indice):
-
- # ignore low scores
- pred, landm = pred[inds, :], landm[inds, :]
- if pred.shape[0] == 0:
- final_bounding_boxes.append(np.array([], dtype=np.float32))
- final_landmarks.append(np.array([], dtype=np.float32))
- continue
-
- # sort
- # order = score.argsort(descending=True)
- # box, landm, score = box[order], landm[order], score[order]
-
- # to CPU
- bounding_boxes, landm = pred.cpu().numpy(), landm.cpu().numpy()
-
- # NMS
- keep = py_cpu_nms(bounding_boxes, nms_threshold)
- bounding_boxes, landmarks = bounding_boxes[keep, :], landm[keep]
-
- # append
- final_bounding_boxes.append(bounding_boxes)
- final_landmarks.append(landmarks)
- # self.t['forward_pass'].toc(average=True)
- # self.batch_time += self.t['forward_pass'].diff
- # self.total_frame += len(frames)
- # print(self.batch_time / self.total_frame)
-
- return final_bounding_boxes, final_landmarks
diff --git a/spaces/dcarpintero/nlp-summarizer-pegasus/.venv/lib/python3.9/site-packages/fontTools/ttLib/tables/_g_c_i_d.py b/spaces/dcarpintero/nlp-summarizer-pegasus/.venv/lib/python3.9/site-packages/fontTools/ttLib/tables/_g_c_i_d.py
deleted file mode 100644
index 2e746c846fa14800cb7de93969984dac36678e4e..0000000000000000000000000000000000000000
--- a/spaces/dcarpintero/nlp-summarizer-pegasus/.venv/lib/python3.9/site-packages/fontTools/ttLib/tables/_g_c_i_d.py
+++ /dev/null
@@ -1,6 +0,0 @@
-from .otBase import BaseTTXConverter
-
-
-# https://developer.apple.com/fonts/TrueType-Reference-Manual/RM06/Chap6gcid.html
-class table__g_c_i_d(BaseTTXConverter):
- pass
diff --git a/spaces/dcarpintero/nlp-summarizer-pegasus/.venv/lib/python3.9/site-packages/fsspec/parquet.py b/spaces/dcarpintero/nlp-summarizer-pegasus/.venv/lib/python3.9/site-packages/fsspec/parquet.py
deleted file mode 100644
index af55f8cf48e80ed81ba9abc3bff51915a5daf84c..0000000000000000000000000000000000000000
--- a/spaces/dcarpintero/nlp-summarizer-pegasus/.venv/lib/python3.9/site-packages/fsspec/parquet.py
+++ /dev/null
@@ -1,551 +0,0 @@
-import io
-import json
-import warnings
-
-from .core import url_to_fs
-from .utils import merge_offset_ranges
-
-# Parquet-Specific Utilities for fsspec
-#
-# Most of the functions defined in this module are NOT
-# intended for public consumption. The only exception
-# to this is `open_parquet_file`, which should be used
-# place of `fs.open()` to open parquet-formatted files
-# on remote file systems.
-
-
-def open_parquet_file(
- path,
- mode="rb",
- fs=None,
- metadata=None,
- columns=None,
- row_groups=None,
- storage_options=None,
- strict=False,
- engine="auto",
- max_gap=64_000,
- max_block=256_000_000,
- footer_sample_size=1_000_000,
- **kwargs,
-):
- """
- Return a file-like object for a single Parquet file.
-
- The specified parquet `engine` will be used to parse the
- footer metadata, and determine the required byte ranges
- from the file. The target path will then be opened with
- the "parts" (`KnownPartsOfAFile`) caching strategy.
-
- Note that this method is intended for usage with remote
- file systems, and is unlikely to improve parquet-read
- performance on local file systems.
-
- Parameters
- ----------
- path: str
- Target file path.
- mode: str, optional
- Mode option to be passed through to `fs.open`. Default is "rb".
- metadata: Any, optional
- Parquet metadata object. Object type must be supported
- by the backend parquet engine. For now, only the "fastparquet"
- engine supports an explicit `ParquetFile` metadata object.
- If a metadata object is supplied, the remote footer metadata
- will not need to be transferred into local memory.
- fs: AbstractFileSystem, optional
- Filesystem object to use for opening the file. If nothing is
- specified, an `AbstractFileSystem` object will be inferred.
- engine : str, default "auto"
- Parquet engine to use for metadata parsing. Allowed options
- include "fastparquet", "pyarrow", and "auto". The specified
- engine must be installed in the current environment. If
- "auto" is specified, and both engines are installed,
- "fastparquet" will take precedence over "pyarrow".
- columns: list, optional
- List of all column names that may be read from the file.
- row_groups : list, optional
- List of all row-groups that may be read from the file. This
- may be a list of row-group indices (integers), or it may be
- a list of `RowGroup` metadata objects (if the "fastparquet"
- engine is used).
- storage_options : dict, optional
- Used to generate an `AbstractFileSystem` object if `fs` was
- not specified.
- strict : bool, optional
- Whether the resulting `KnownPartsOfAFile` cache should
- fetch reads that go beyond a known byte-range boundary.
- If `False` (the default), any read that ends outside a
- known part will be zero padded. Note that using
- `strict=True` may be useful for debugging.
- max_gap : int, optional
- Neighboring byte ranges will only be merged when their
- inter-range gap is <= `max_gap`. Default is 64KB.
- max_block : int, optional
- Neighboring byte ranges will only be merged when the size of
- the aggregated range is <= `max_block`. Default is 256MB.
- footer_sample_size : int, optional
- Number of bytes to read from the end of the path to look
- for the footer metadata. If the sampled bytes do not contain
- the footer, a second read request will be required, and
- performance will suffer. Default is 1MB.
- **kwargs :
- Optional key-word arguments to pass to `fs.open`
- """
-
- # Make sure we have an `AbstractFileSystem` object
- # to work with
- if fs is None:
- fs = url_to_fs(path, **(storage_options or {}))[0]
-
- # For now, `columns == []` not supported. Just use
- # default `open` command with `path` input
- if columns is not None and len(columns) == 0:
- return fs.open(path, mode=mode)
-
- # Set the engine
- engine = _set_engine(engine)
-
- # Fetch the known byte ranges needed to read
- # `columns` and/or `row_groups`
- data = _get_parquet_byte_ranges(
- [path],
- fs,
- metadata=metadata,
- columns=columns,
- row_groups=row_groups,
- engine=engine,
- max_gap=max_gap,
- max_block=max_block,
- footer_sample_size=footer_sample_size,
- )
-
- # Extract file name from `data`
- fn = next(iter(data)) if data else path
-
- # Call self.open with "parts" caching
- options = kwargs.pop("cache_options", {}).copy()
- return fs.open(
- fn,
- mode=mode,
- cache_type="parts",
- cache_options={
- **options,
- **{
- "data": data.get(fn, {}),
- "strict": strict,
- },
- },
- **kwargs,
- )
-
-
-def _get_parquet_byte_ranges(
- paths,
- fs,
- metadata=None,
- columns=None,
- row_groups=None,
- max_gap=64_000,
- max_block=256_000_000,
- footer_sample_size=1_000_000,
- engine="auto",
-):
- """Get a dictionary of the known byte ranges needed
- to read a specific column/row-group selection from a
- Parquet dataset. Each value in the output dictionary
- is intended for use as the `data` argument for the
- `KnownPartsOfAFile` caching strategy of a single path.
- """
-
- # Set engine if necessary
- if isinstance(engine, str):
- engine = _set_engine(engine)
-
- # Pass to specialized function if metadata is defined
- if metadata is not None:
-
- # Use the provided parquet metadata object
- # to avoid transferring/parsing footer metadata
- return _get_parquet_byte_ranges_from_metadata(
- metadata,
- fs,
- engine,
- columns=columns,
- row_groups=row_groups,
- max_gap=max_gap,
- max_block=max_block,
- )
-
- # Get file sizes asynchronously
- file_sizes = fs.sizes(paths)
-
- # Populate global paths, starts, & ends
- result = {}
- data_paths = []
- data_starts = []
- data_ends = []
- add_header_magic = True
- if columns is None and row_groups is None:
- # We are NOT selecting specific columns or row-groups.
- #
- # We can avoid sampling the footers, and just transfer
- # all file data with cat_ranges
- for i, path in enumerate(paths):
- result[path] = {}
- for b in range(0, file_sizes[i], max_block):
- data_paths.append(path)
- data_starts.append(b)
- data_ends.append(min(b + max_block, file_sizes[i]))
- add_header_magic = False # "Magic" should already be included
- else:
- # We ARE selecting specific columns or row-groups.
- #
- # Gather file footers.
- # We just take the last `footer_sample_size` bytes of each
- # file (or the entire file if it is smaller than that)
- footer_starts = []
- footer_ends = []
- for i, path in enumerate(paths):
- footer_ends.append(file_sizes[i])
- sample_size = max(0, file_sizes[i] - footer_sample_size)
- footer_starts.append(sample_size)
- footer_samples = fs.cat_ranges(paths, footer_starts, footer_ends)
-
- # Check our footer samples and re-sample if necessary.
- missing_footer_starts = footer_starts.copy()
- large_footer = 0
- for i, path in enumerate(paths):
- footer_size = int.from_bytes(footer_samples[i][-8:-4], "little")
- real_footer_start = file_sizes[i] - (footer_size + 8)
- if real_footer_start < footer_starts[i]:
- missing_footer_starts[i] = real_footer_start
- large_footer = max(large_footer, (footer_size + 8))
- if large_footer:
- warnings.warn(
- f"Not enough data was used to sample the parquet footer. "
- f"Try setting footer_sample_size >= {large_footer}."
- )
- for i, block in enumerate(
- fs.cat_ranges(
- paths,
- missing_footer_starts,
- footer_starts,
- )
- ):
- footer_samples[i] = block + footer_samples[i]
- footer_starts[i] = missing_footer_starts[i]
-
- # Calculate required byte ranges for each path
- for i, path in enumerate(paths):
-
- # Deal with small-file case.
- # Just include all remaining bytes of the file
- # in a single range.
- if file_sizes[i] < max_block:
- if footer_starts[i] > 0:
- # Only need to transfer the data if the
- # footer sample isn't already the whole file
- data_paths.append(path)
- data_starts.append(0)
- data_ends.append(footer_starts[i])
- continue
-
- # Use "engine" to collect data byte ranges
- path_data_starts, path_data_ends = engine._parquet_byte_ranges(
- columns,
- row_groups=row_groups,
- footer=footer_samples[i],
- footer_start=footer_starts[i],
- )
-
- data_paths += [path] * len(path_data_starts)
- data_starts += path_data_starts
- data_ends += path_data_ends
-
- # Merge adjacent offset ranges
- data_paths, data_starts, data_ends = merge_offset_ranges(
- data_paths,
- data_starts,
- data_ends,
- max_gap=max_gap,
- max_block=max_block,
- sort=False, # Should already be sorted
- )
-
- # Start by populating `result` with footer samples
- for i, path in enumerate(paths):
- result[path] = {(footer_starts[i], footer_ends[i]): footer_samples[i]}
-
- # Transfer the data byte-ranges into local memory
- _transfer_ranges(fs, result, data_paths, data_starts, data_ends)
-
- # Add b"PAR1" to header if necessary
- if add_header_magic:
- _add_header_magic(result)
-
- return result
-
-
-def _get_parquet_byte_ranges_from_metadata(
- metadata,
- fs,
- engine,
- columns=None,
- row_groups=None,
- max_gap=64_000,
- max_block=256_000_000,
-):
- """Simplified version of `_get_parquet_byte_ranges` for
- the case that an engine-specific `metadata` object is
- provided, and the remote footer metadata does not need to
- be transferred before calculating the required byte ranges.
- """
-
- # Use "engine" to collect data byte ranges
- data_paths, data_starts, data_ends = engine._parquet_byte_ranges(
- columns,
- row_groups=row_groups,
- metadata=metadata,
- )
-
- # Merge adjacent offset ranges
- data_paths, data_starts, data_ends = merge_offset_ranges(
- data_paths,
- data_starts,
- data_ends,
- max_gap=max_gap,
- max_block=max_block,
- sort=False, # Should be sorted
- )
-
- # Transfer the data byte-ranges into local memory
- result = {fn: {} for fn in list(set(data_paths))}
- _transfer_ranges(fs, result, data_paths, data_starts, data_ends)
-
- # Add b"PAR1" to header
- _add_header_magic(result)
-
- return result
-
-
-def _transfer_ranges(fs, blocks, paths, starts, ends):
- # Use cat_ranges to gather the data byte_ranges
- ranges = (paths, starts, ends)
- for path, start, stop, data in zip(*ranges, fs.cat_ranges(*ranges)):
- blocks[path][(start, stop)] = data
-
-
-def _add_header_magic(data):
- # Add b"PAR1" to file headers
- for i, path in enumerate(list(data.keys())):
- add_magic = True
- for k in data[path].keys():
- if k[0] == 0 and k[1] >= 4:
- add_magic = False
- break
- if add_magic:
- data[path][(0, 4)] = b"PAR1"
-
-
-def _set_engine(engine_str):
-
- # Define a list of parquet engines to try
- if engine_str == "auto":
- try_engines = ("fastparquet", "pyarrow")
- elif not isinstance(engine_str, str):
- raise ValueError(
- "Failed to set parquet engine! "
- "Please pass 'fastparquet', 'pyarrow', or 'auto'"
- )
- elif engine_str not in ("fastparquet", "pyarrow"):
- raise ValueError(f"{engine_str} engine not supported by `fsspec.parquet`")
- else:
- try_engines = [engine_str]
-
- # Try importing the engines in `try_engines`,
- # and choose the first one that succeeds
- for engine in try_engines:
- try:
- if engine == "fastparquet":
- return FastparquetEngine()
- elif engine == "pyarrow":
- return PyarrowEngine()
- except ImportError:
- pass
-
- # Raise an error if a supported parquet engine
- # was not found
- raise ImportError(
- f"The following parquet engines are not installed "
- f"in your python environment: {try_engines}."
- f"Please install 'fastparquert' or 'pyarrow' to "
- f"utilize the `fsspec.parquet` module."
- )
-
-
-class FastparquetEngine:
-
- # The purpose of the FastparquetEngine class is
- # to check if fastparquet can be imported (on initialization)
- # and to define a `_parquet_byte_ranges` method. In the
- # future, this class may also be used to define other
- # methods/logic that are specific to fastparquet.
-
- def __init__(self):
- import fastparquet as fp
-
- self.fp = fp
-
- def _row_group_filename(self, row_group, pf):
- return pf.row_group_filename(row_group)
-
- def _parquet_byte_ranges(
- self,
- columns,
- row_groups=None,
- metadata=None,
- footer=None,
- footer_start=None,
- ):
-
- # Initialize offset ranges and define ParqetFile metadata
- pf = metadata
- data_paths, data_starts, data_ends = [], [], []
- if pf is None:
- pf = self.fp.ParquetFile(io.BytesIO(footer))
-
- # Convert columns to a set and add any index columns
- # specified in the pandas metadata (just in case)
- column_set = None if columns is None else set(columns)
- if column_set is not None and hasattr(pf, "pandas_metadata"):
- md_index = [
- ind
- for ind in pf.pandas_metadata.get("index_columns", [])
- # Ignore RangeIndex information
- if not isinstance(ind, dict)
- ]
- column_set |= set(md_index)
-
- # Check if row_groups is a list of integers
- # or a list of row-group metadata
- if row_groups and not isinstance(row_groups[0], int):
- # Input row_groups contains row-group metadata
- row_group_indices = None
- else:
- # Input row_groups contains row-group indices
- row_group_indices = row_groups
- row_groups = pf.row_groups
-
- # Loop through column chunks to add required byte ranges
- for r, row_group in enumerate(row_groups):
- # Skip this row-group if we are targeting
- # specific row-groups
- if row_group_indices is None or r in row_group_indices:
-
- # Find the target parquet-file path for `row_group`
- fn = self._row_group_filename(row_group, pf)
-
- for column in row_group.columns:
- name = column.meta_data.path_in_schema[0]
- # Skip this column if we are targeting a
- # specific columns
- if column_set is None or name in column_set:
- file_offset0 = column.meta_data.dictionary_page_offset
- if file_offset0 is None:
- file_offset0 = column.meta_data.data_page_offset
- num_bytes = column.meta_data.total_compressed_size
- if footer_start is None or file_offset0 < footer_start:
- data_paths.append(fn)
- data_starts.append(file_offset0)
- data_ends.append(
- min(
- file_offset0 + num_bytes,
- footer_start or (file_offset0 + num_bytes),
- )
- )
-
- if metadata:
- # The metadata in this call may map to multiple
- # file paths. Need to include `data_paths`
- return data_paths, data_starts, data_ends
- return data_starts, data_ends
-
-
-class PyarrowEngine:
-
- # The purpose of the PyarrowEngine class is
- # to check if pyarrow can be imported (on initialization)
- # and to define a `_parquet_byte_ranges` method. In the
- # future, this class may also be used to define other
- # methods/logic that are specific to pyarrow.
-
- def __init__(self):
- import pyarrow.parquet as pq
-
- self.pq = pq
-
- def _row_group_filename(self, row_group, metadata):
- raise NotImplementedError
-
- def _parquet_byte_ranges(
- self,
- columns,
- row_groups=None,
- metadata=None,
- footer=None,
- footer_start=None,
- ):
-
- if metadata is not None:
- raise ValueError("metadata input not supported for PyarrowEngine")
-
- data_starts, data_ends = [], []
- md = self.pq.ParquetFile(io.BytesIO(footer)).metadata
-
- # Convert columns to a set and add any index columns
- # specified in the pandas metadata (just in case)
- column_set = None if columns is None else set(columns)
- if column_set is not None:
- schema = md.schema.to_arrow_schema()
- has_pandas_metadata = (
- schema.metadata is not None and b"pandas" in schema.metadata
- )
- if has_pandas_metadata:
- md_index = [
- ind
- for ind in json.loads(
- schema.metadata[b"pandas"].decode("utf8")
- ).get("index_columns", [])
- # Ignore RangeIndex information
- if not isinstance(ind, dict)
- ]
- column_set |= set(md_index)
-
- # Loop through column chunks to add required byte ranges
- for r in range(md.num_row_groups):
- # Skip this row-group if we are targeting
- # specific row-groups
- if row_groups is None or r in row_groups:
- row_group = md.row_group(r)
- for c in range(row_group.num_columns):
- column = row_group.column(c)
- name = column.path_in_schema
- # Skip this column if we are targeting a
- # specific columns
- split_name = name.split(".")[0]
- if (
- column_set is None
- or name in column_set
- or split_name in column_set
- ):
- file_offset0 = column.dictionary_page_offset
- if file_offset0 is None:
- file_offset0 = column.data_page_offset
- num_bytes = column.total_compressed_size
- if file_offset0 < footer_start:
- data_starts.append(file_offset0)
- data_ends.append(
- min(file_offset0 + num_bytes, footer_start)
- )
- return data_starts, data_ends
diff --git a/spaces/dcarpintero/nlp-summarizer-pegasus/.venv/lib/python3.9/site-packages/gradio/templates/cdn/assets/index-aef3869a.css b/spaces/dcarpintero/nlp-summarizer-pegasus/.venv/lib/python3.9/site-packages/gradio/templates/cdn/assets/index-aef3869a.css
deleted file mode 100644
index a1f402a49e82009fd7eafa923615d67793b8751c..0000000000000000000000000000000000000000
--- a/spaces/dcarpintero/nlp-summarizer-pegasus/.venv/lib/python3.9/site-packages/gradio/templates/cdn/assets/index-aef3869a.css
+++ /dev/null
@@ -1 +0,0 @@
-td.svelte-xrr240.svelte-xrr240{width:45%}td.svelte-xrr240.svelte-xrr240:last-child{width:10%;text-align:right}.file-preview-holder.svelte-xrr240.svelte-xrr240{overflow-x:auto}.file-preview.svelte-xrr240.svelte-xrr240{width:var(--size-full);max-height:var(--size-60);overflow-y:auto;color:var(--body-text-color)}.file.svelte-xrr240.svelte-xrr240{width:var(--size-full)}.file.svelte-xrr240>.svelte-xrr240{padding:var(--size-1) var(--size-2-5)}.download.svelte-xrr240.svelte-xrr240:hover{text-decoration:underline}.download.svelte-xrr240>a.svelte-xrr240{color:var(--link-text-color)}.download.svelte-xrr240>a.svelte-xrr240:hover{color:var(--link-text-color-hover)}.download.svelte-xrr240>a.svelte-xrr240:visited{color:var(--link-text-color-visited)}.download.svelte-xrr240>a.svelte-xrr240:active{color:var(--link-text-color-active)}.selectable.svelte-xrr240.svelte-xrr240{cursor:pointer}
diff --git a/spaces/dcarpintero/nlp-summarizer-pegasus/.venv/lib/python3.9/site-packages/h11/_writers.py b/spaces/dcarpintero/nlp-summarizer-pegasus/.venv/lib/python3.9/site-packages/h11/_writers.py
deleted file mode 100644
index 939cdb912a9debaea07fbf3a9ac04549c44d077c..0000000000000000000000000000000000000000
--- a/spaces/dcarpintero/nlp-summarizer-pegasus/.venv/lib/python3.9/site-packages/h11/_writers.py
+++ /dev/null
@@ -1,145 +0,0 @@
-# Code to read HTTP data
-#
-# Strategy: each writer takes an event + a write-some-bytes function, which is
-# calls.
-#
-# WRITERS is a dict describing how to pick a reader. It maps states to either:
-# - a writer
-# - or, for body writers, a dict of framin-dependent writer factories
-
-from typing import Any, Callable, Dict, List, Tuple, Type, Union
-
-from ._events import Data, EndOfMessage, Event, InformationalResponse, Request, Response
-from ._headers import Headers
-from ._state import CLIENT, IDLE, SEND_BODY, SEND_RESPONSE, SERVER
-from ._util import LocalProtocolError, Sentinel
-
-__all__ = ["WRITERS"]
-
-Writer = Callable[[bytes], Any]
-
-
-def write_headers(headers: Headers, write: Writer) -> None:
- # "Since the Host field-value is critical information for handling a
- # request, a user agent SHOULD generate Host as the first header field
- # following the request-line." - RFC 7230
- raw_items = headers._full_items
- for raw_name, name, value in raw_items:
- if name == b"host":
- write(b"%s: %s\r\n" % (raw_name, value))
- for raw_name, name, value in raw_items:
- if name != b"host":
- write(b"%s: %s\r\n" % (raw_name, value))
- write(b"\r\n")
-
-
-def write_request(request: Request, write: Writer) -> None:
- if request.http_version != b"1.1":
- raise LocalProtocolError("I only send HTTP/1.1")
- write(b"%s %s HTTP/1.1\r\n" % (request.method, request.target))
- write_headers(request.headers, write)
-
-
-# Shared between InformationalResponse and Response
-def write_any_response(
- response: Union[InformationalResponse, Response], write: Writer
-) -> None:
- if response.http_version != b"1.1":
- raise LocalProtocolError("I only send HTTP/1.1")
- status_bytes = str(response.status_code).encode("ascii")
- # We don't bother sending ascii status messages like "OK"; they're
- # optional and ignored by the protocol. (But the space after the numeric
- # status code is mandatory.)
- #
- # XX FIXME: could at least make an effort to pull out the status message
- # from stdlib's http.HTTPStatus table. Or maybe just steal their enums
- # (either by import or copy/paste). We already accept them as status codes
- # since they're of type IntEnum < int.
- write(b"HTTP/1.1 %s %s\r\n" % (status_bytes, response.reason))
- write_headers(response.headers, write)
-
-
-class BodyWriter:
- def __call__(self, event: Event, write: Writer) -> None:
- if type(event) is Data:
- self.send_data(event.data, write)
- elif type(event) is EndOfMessage:
- self.send_eom(event.headers, write)
- else: # pragma: no cover
- assert False
-
- def send_data(self, data: bytes, write: Writer) -> None:
- pass
-
- def send_eom(self, headers: Headers, write: Writer) -> None:
- pass
-
-
-#
-# These are all careful not to do anything to 'data' except call len(data) and
-# write(data). This allows us to transparently pass-through funny objects,
-# like placeholder objects referring to files on disk that will be sent via
-# sendfile(2).
-#
-class ContentLengthWriter(BodyWriter):
- def __init__(self, length: int) -> None:
- self._length = length
-
- def send_data(self, data: bytes, write: Writer) -> None:
- self._length -= len(data)
- if self._length < 0:
- raise LocalProtocolError("Too much data for declared Content-Length")
- write(data)
-
- def send_eom(self, headers: Headers, write: Writer) -> None:
- if self._length != 0:
- raise LocalProtocolError("Too little data for declared Content-Length")
- if headers:
- raise LocalProtocolError("Content-Length and trailers don't mix")
-
-
-class ChunkedWriter(BodyWriter):
- def send_data(self, data: bytes, write: Writer) -> None:
- # if we encoded 0-length data in the naive way, it would look like an
- # end-of-message.
- if not data:
- return
- write(b"%x\r\n" % len(data))
- write(data)
- write(b"\r\n")
-
- def send_eom(self, headers: Headers, write: Writer) -> None:
- write(b"0\r\n")
- write_headers(headers, write)
-
-
-class Http10Writer(BodyWriter):
- def send_data(self, data: bytes, write: Writer) -> None:
- write(data)
-
- def send_eom(self, headers: Headers, write: Writer) -> None:
- if headers:
- raise LocalProtocolError("can't send trailers to HTTP/1.0 client")
- # no need to close the socket ourselves, that will be taken care of by
- # Connection: close machinery
-
-
-WritersType = Dict[
- Union[Tuple[Type[Sentinel], Type[Sentinel]], Type[Sentinel]],
- Union[
- Dict[str, Type[BodyWriter]],
- Callable[[Union[InformationalResponse, Response], Writer], None],
- Callable[[Request, Writer], None],
- ],
-]
-
-WRITERS: WritersType = {
- (CLIENT, IDLE): write_request,
- (SERVER, IDLE): write_any_response,
- (SERVER, SEND_RESPONSE): write_any_response,
- SEND_BODY: {
- "chunked": ChunkedWriter,
- "content-length": ContentLengthWriter,
- "http/1.0": Http10Writer,
- },
-}
diff --git a/spaces/dcarpintero/nlp-summarizer-pegasus/.venv/lib/python3.9/site-packages/jinja2/debug.py b/spaces/dcarpintero/nlp-summarizer-pegasus/.venv/lib/python3.9/site-packages/jinja2/debug.py
deleted file mode 100644
index 7ed7e9297e01b87c4e999d19d48a4265b38b574f..0000000000000000000000000000000000000000
--- a/spaces/dcarpintero/nlp-summarizer-pegasus/.venv/lib/python3.9/site-packages/jinja2/debug.py
+++ /dev/null
@@ -1,191 +0,0 @@
-import sys
-import typing as t
-from types import CodeType
-from types import TracebackType
-
-from .exceptions import TemplateSyntaxError
-from .utils import internal_code
-from .utils import missing
-
-if t.TYPE_CHECKING:
- from .runtime import Context
-
-
-def rewrite_traceback_stack(source: t.Optional[str] = None) -> BaseException:
- """Rewrite the current exception to replace any tracebacks from
- within compiled template code with tracebacks that look like they
- came from the template source.
-
- This must be called within an ``except`` block.
-
- :param source: For ``TemplateSyntaxError``, the original source if
- known.
- :return: The original exception with the rewritten traceback.
- """
- _, exc_value, tb = sys.exc_info()
- exc_value = t.cast(BaseException, exc_value)
- tb = t.cast(TracebackType, tb)
-
- if isinstance(exc_value, TemplateSyntaxError) and not exc_value.translated:
- exc_value.translated = True
- exc_value.source = source
- # Remove the old traceback, otherwise the frames from the
- # compiler still show up.
- exc_value.with_traceback(None)
- # Outside of runtime, so the frame isn't executing template
- # code, but it still needs to point at the template.
- tb = fake_traceback(
- exc_value, None, exc_value.filename or "", exc_value.lineno
- )
- else:
- # Skip the frame for the render function.
- tb = tb.tb_next
-
- stack = []
-
- # Build the stack of traceback object, replacing any in template
- # code with the source file and line information.
- while tb is not None:
- # Skip frames decorated with @internalcode. These are internal
- # calls that aren't useful in template debugging output.
- if tb.tb_frame.f_code in internal_code:
- tb = tb.tb_next
- continue
-
- template = tb.tb_frame.f_globals.get("__jinja_template__")
-
- if template is not None:
- lineno = template.get_corresponding_lineno(tb.tb_lineno)
- fake_tb = fake_traceback(exc_value, tb, template.filename, lineno)
- stack.append(fake_tb)
- else:
- stack.append(tb)
-
- tb = tb.tb_next
-
- tb_next = None
-
- # Assign tb_next in reverse to avoid circular references.
- for tb in reversed(stack):
- tb.tb_next = tb_next
- tb_next = tb
-
- return exc_value.with_traceback(tb_next)
-
-
-def fake_traceback( # type: ignore
- exc_value: BaseException, tb: t.Optional[TracebackType], filename: str, lineno: int
-) -> TracebackType:
- """Produce a new traceback object that looks like it came from the
- template source instead of the compiled code. The filename, line
- number, and location name will point to the template, and the local
- variables will be the current template context.
-
- :param exc_value: The original exception to be re-raised to create
- the new traceback.
- :param tb: The original traceback to get the local variables and
- code info from.
- :param filename: The template filename.
- :param lineno: The line number in the template source.
- """
- if tb is not None:
- # Replace the real locals with the context that would be
- # available at that point in the template.
- locals = get_template_locals(tb.tb_frame.f_locals)
- locals.pop("__jinja_exception__", None)
- else:
- locals = {}
-
- globals = {
- "__name__": filename,
- "__file__": filename,
- "__jinja_exception__": exc_value,
- }
- # Raise an exception at the correct line number.
- code: CodeType = compile(
- "\n" * (lineno - 1) + "raise __jinja_exception__", filename, "exec"
- )
-
- # Build a new code object that points to the template file and
- # replaces the location with a block name.
- location = "template"
-
- if tb is not None:
- function = tb.tb_frame.f_code.co_name
-
- if function == "root":
- location = "top-level template code"
- elif function.startswith("block_"):
- location = f"block {function[6:]!r}"
-
- if sys.version_info >= (3, 8):
- code = code.replace(co_name=location)
- else:
- code = CodeType(
- code.co_argcount,
- code.co_kwonlyargcount,
- code.co_nlocals,
- code.co_stacksize,
- code.co_flags,
- code.co_code,
- code.co_consts,
- code.co_names,
- code.co_varnames,
- code.co_filename,
- location,
- code.co_firstlineno,
- code.co_lnotab,
- code.co_freevars,
- code.co_cellvars,
- )
-
- # Execute the new code, which is guaranteed to raise, and return
- # the new traceback without this frame.
- try:
- exec(code, globals, locals)
- except BaseException:
- return sys.exc_info()[2].tb_next # type: ignore
-
-
-def get_template_locals(real_locals: t.Mapping[str, t.Any]) -> t.Dict[str, t.Any]:
- """Based on the runtime locals, get the context that would be
- available at that point in the template.
- """
- # Start with the current template context.
- ctx: "t.Optional[Context]" = real_locals.get("context")
-
- if ctx is not None:
- data: t.Dict[str, t.Any] = ctx.get_all().copy()
- else:
- data = {}
-
- # Might be in a derived context that only sets local variables
- # rather than pushing a context. Local variables follow the scheme
- # l_depth_name. Find the highest-depth local that has a value for
- # each name.
- local_overrides: t.Dict[str, t.Tuple[int, t.Any]] = {}
-
- for name, value in real_locals.items():
- if not name.startswith("l_") or value is missing:
- # Not a template variable, or no longer relevant.
- continue
-
- try:
- _, depth_str, name = name.split("_", 2)
- depth = int(depth_str)
- except ValueError:
- continue
-
- cur_depth = local_overrides.get(name, (-1,))[0]
-
- if cur_depth < depth:
- local_overrides[name] = (depth, value)
-
- # Modify the context with any derived context.
- for name, (_, value) in local_overrides.items():
- if value is missing:
- data.pop(name, None)
- else:
- data[name] = value
-
- return data
diff --git a/spaces/declare-lab/flan-t5-xl-lora/README.md b/spaces/declare-lab/flan-t5-xl-lora/README.md
deleted file mode 100644
index 16067909ec58c8733e260d6ab3c8f6b0b8f6179b..0000000000000000000000000000000000000000
--- a/spaces/declare-lab/flan-t5-xl-lora/README.md
+++ /dev/null
@@ -1,12 +0,0 @@
----
-title: Flan T5 Xl Lora
-emoji: 😻
-colorFrom: pink
-colorTo: indigo
-sdk: gradio
-sdk_version: 3.24.1
-app_file: app.py
-pinned: false
----
-
-Check out the configuration reference at https://huggingface.co/docs/hub/spaces-config-reference
diff --git a/spaces/declare-lab/tango/diffusers/examples/community/magic_mix.py b/spaces/declare-lab/tango/diffusers/examples/community/magic_mix.py
deleted file mode 100644
index b1d69ec8457617653a4dcb17f0bb2b5b0313dd87..0000000000000000000000000000000000000000
--- a/spaces/declare-lab/tango/diffusers/examples/community/magic_mix.py
+++ /dev/null
@@ -1,152 +0,0 @@
-from typing import Union
-
-import torch
-from PIL import Image
-from torchvision import transforms as tfms
-from tqdm.auto import tqdm
-from transformers import CLIPTextModel, CLIPTokenizer
-
-from diffusers import (
- AutoencoderKL,
- DDIMScheduler,
- DiffusionPipeline,
- LMSDiscreteScheduler,
- PNDMScheduler,
- UNet2DConditionModel,
-)
-
-
-class MagicMixPipeline(DiffusionPipeline):
- def __init__(
- self,
- vae: AutoencoderKL,
- text_encoder: CLIPTextModel,
- tokenizer: CLIPTokenizer,
- unet: UNet2DConditionModel,
- scheduler: Union[PNDMScheduler, LMSDiscreteScheduler, DDIMScheduler],
- ):
- super().__init__()
-
- self.register_modules(vae=vae, text_encoder=text_encoder, tokenizer=tokenizer, unet=unet, scheduler=scheduler)
-
- # convert PIL image to latents
- def encode(self, img):
- with torch.no_grad():
- latent = self.vae.encode(tfms.ToTensor()(img).unsqueeze(0).to(self.device) * 2 - 1)
- latent = 0.18215 * latent.latent_dist.sample()
- return latent
-
- # convert latents to PIL image
- def decode(self, latent):
- latent = (1 / 0.18215) * latent
- with torch.no_grad():
- img = self.vae.decode(latent).sample
- img = (img / 2 + 0.5).clamp(0, 1)
- img = img.detach().cpu().permute(0, 2, 3, 1).numpy()
- img = (img * 255).round().astype("uint8")
- return Image.fromarray(img[0])
-
- # convert prompt into text embeddings, also unconditional embeddings
- def prep_text(self, prompt):
- text_input = self.tokenizer(
- prompt,
- padding="max_length",
- max_length=self.tokenizer.model_max_length,
- truncation=True,
- return_tensors="pt",
- )
-
- text_embedding = self.text_encoder(text_input.input_ids.to(self.device))[0]
-
- uncond_input = self.tokenizer(
- "",
- padding="max_length",
- max_length=self.tokenizer.model_max_length,
- truncation=True,
- return_tensors="pt",
- )
-
- uncond_embedding = self.text_encoder(uncond_input.input_ids.to(self.device))[0]
-
- return torch.cat([uncond_embedding, text_embedding])
-
- def __call__(
- self,
- img: Image.Image,
- prompt: str,
- kmin: float = 0.3,
- kmax: float = 0.6,
- mix_factor: float = 0.5,
- seed: int = 42,
- steps: int = 50,
- guidance_scale: float = 7.5,
- ) -> Image.Image:
- tmin = steps - int(kmin * steps)
- tmax = steps - int(kmax * steps)
-
- text_embeddings = self.prep_text(prompt)
-
- self.scheduler.set_timesteps(steps)
-
- width, height = img.size
- encoded = self.encode(img)
-
- torch.manual_seed(seed)
- noise = torch.randn(
- (1, self.unet.in_channels, height // 8, width // 8),
- ).to(self.device)
-
- latents = self.scheduler.add_noise(
- encoded,
- noise,
- timesteps=self.scheduler.timesteps[tmax],
- )
-
- input = torch.cat([latents] * 2)
-
- input = self.scheduler.scale_model_input(input, self.scheduler.timesteps[tmax])
-
- with torch.no_grad():
- pred = self.unet(
- input,
- self.scheduler.timesteps[tmax],
- encoder_hidden_states=text_embeddings,
- ).sample
-
- pred_uncond, pred_text = pred.chunk(2)
- pred = pred_uncond + guidance_scale * (pred_text - pred_uncond)
-
- latents = self.scheduler.step(pred, self.scheduler.timesteps[tmax], latents).prev_sample
-
- for i, t in enumerate(tqdm(self.scheduler.timesteps)):
- if i > tmax:
- if i < tmin: # layout generation phase
- orig_latents = self.scheduler.add_noise(
- encoded,
- noise,
- timesteps=t,
- )
-
- input = (mix_factor * latents) + (
- 1 - mix_factor
- ) * orig_latents # interpolating between layout noise and conditionally generated noise to preserve layout sematics
- input = torch.cat([input] * 2)
-
- else: # content generation phase
- input = torch.cat([latents] * 2)
-
- input = self.scheduler.scale_model_input(input, t)
-
- with torch.no_grad():
- pred = self.unet(
- input,
- t,
- encoder_hidden_states=text_embeddings,
- ).sample
-
- pred_uncond, pred_text = pred.chunk(2)
- pred = pred_uncond + guidance_scale * (pred_text - pred_uncond)
-
- latents = self.scheduler.step(pred, t, latents).prev_sample
-
- return self.decode(latents)
diff --git a/spaces/declare-lab/tango/diffusers/tests/pipelines/unclip/test_unclip_image_variation.py b/spaces/declare-lab/tango/diffusers/tests/pipelines/unclip/test_unclip_image_variation.py
deleted file mode 100644
index ff32ac5f9aafb9140ec5b49dc79711d493b76788..0000000000000000000000000000000000000000
--- a/spaces/declare-lab/tango/diffusers/tests/pipelines/unclip/test_unclip_image_variation.py
+++ /dev/null
@@ -1,508 +0,0 @@
-# coding=utf-8
-# Copyright 2023 HuggingFace Inc.
-#
-# Licensed under the Apache License, Version 2.0 (the "License");
-# you may not use this file except in compliance with the License.
-# You may obtain a copy of the License at
-#
-# http://www.apache.org/licenses/LICENSE-2.0
-#
-# Unless required by applicable law or agreed to in writing, software
-# distributed under the License is distributed on an "AS IS" BASIS,
-# WITHOUT WARRANTIES OR CONDITIONS OF ANY KIND, either express or implied.
-# See the License for the specific language governing permissions and
-# limitations under the License.
-
-import gc
-import random
-import unittest
-
-import numpy as np
-import torch
-from transformers import (
- CLIPImageProcessor,
- CLIPTextConfig,
- CLIPTextModelWithProjection,
- CLIPTokenizer,
- CLIPVisionConfig,
- CLIPVisionModelWithProjection,
-)
-
-from diffusers import (
- DiffusionPipeline,
- UnCLIPImageVariationPipeline,
- UnCLIPScheduler,
- UNet2DConditionModel,
- UNet2DModel,
-)
-from diffusers.pipelines.unclip.text_proj import UnCLIPTextProjModel
-from diffusers.utils import floats_tensor, load_numpy, slow, torch_device
-from diffusers.utils.testing_utils import load_image, require_torch_gpu, skip_mps
-
-from ...pipeline_params import IMAGE_VARIATION_BATCH_PARAMS, IMAGE_VARIATION_PARAMS
-from ...test_pipelines_common import PipelineTesterMixin, assert_mean_pixel_difference
-
-
-class UnCLIPImageVariationPipelineFastTests(PipelineTesterMixin, unittest.TestCase):
- pipeline_class = UnCLIPImageVariationPipeline
- params = IMAGE_VARIATION_PARAMS - {"height", "width", "guidance_scale"}
- batch_params = IMAGE_VARIATION_BATCH_PARAMS
-
- required_optional_params = [
- "generator",
- "return_dict",
- "decoder_num_inference_steps",
- "super_res_num_inference_steps",
- ]
-
- @property
- def text_embedder_hidden_size(self):
- return 32
-
- @property
- def time_input_dim(self):
- return 32
-
- @property
- def block_out_channels_0(self):
- return self.time_input_dim
-
- @property
- def time_embed_dim(self):
- return self.time_input_dim * 4
-
- @property
- def cross_attention_dim(self):
- return 100
-
- @property
- def dummy_tokenizer(self):
- tokenizer = CLIPTokenizer.from_pretrained("hf-internal-testing/tiny-random-clip")
- return tokenizer
-
- @property
- def dummy_text_encoder(self):
- torch.manual_seed(0)
- config = CLIPTextConfig(
- bos_token_id=0,
- eos_token_id=2,
- hidden_size=self.text_embedder_hidden_size,
- projection_dim=self.text_embedder_hidden_size,
- intermediate_size=37,
- layer_norm_eps=1e-05,
- num_attention_heads=4,
- num_hidden_layers=5,
- pad_token_id=1,
- vocab_size=1000,
- )
- return CLIPTextModelWithProjection(config)
-
- @property
- def dummy_image_encoder(self):
- torch.manual_seed(0)
- config = CLIPVisionConfig(
- hidden_size=self.text_embedder_hidden_size,
- projection_dim=self.text_embedder_hidden_size,
- num_hidden_layers=5,
- num_attention_heads=4,
- image_size=32,
- intermediate_size=37,
- patch_size=1,
- )
- return CLIPVisionModelWithProjection(config)
-
- @property
- def dummy_text_proj(self):
- torch.manual_seed(0)
-
- model_kwargs = {
- "clip_embeddings_dim": self.text_embedder_hidden_size,
- "time_embed_dim": self.time_embed_dim,
- "cross_attention_dim": self.cross_attention_dim,
- }
-
- model = UnCLIPTextProjModel(**model_kwargs)
- return model
-
- @property
- def dummy_decoder(self):
- torch.manual_seed(0)
-
- model_kwargs = {
- "sample_size": 32,
- # RGB in channels
- "in_channels": 3,
- # Out channels is double in channels because predicts mean and variance
- "out_channels": 6,
- "down_block_types": ("ResnetDownsampleBlock2D", "SimpleCrossAttnDownBlock2D"),
- "up_block_types": ("SimpleCrossAttnUpBlock2D", "ResnetUpsampleBlock2D"),
- "mid_block_type": "UNetMidBlock2DSimpleCrossAttn",
- "block_out_channels": (self.block_out_channels_0, self.block_out_channels_0 * 2),
- "layers_per_block": 1,
- "cross_attention_dim": self.cross_attention_dim,
- "attention_head_dim": 4,
- "resnet_time_scale_shift": "scale_shift",
- "class_embed_type": "identity",
- }
-
- model = UNet2DConditionModel(**model_kwargs)
- return model
-
- @property
- def dummy_super_res_kwargs(self):
- return {
- "sample_size": 64,
- "layers_per_block": 1,
- "down_block_types": ("ResnetDownsampleBlock2D", "ResnetDownsampleBlock2D"),
- "up_block_types": ("ResnetUpsampleBlock2D", "ResnetUpsampleBlock2D"),
- "block_out_channels": (self.block_out_channels_0, self.block_out_channels_0 * 2),
- "in_channels": 6,
- "out_channels": 3,
- }
-
- @property
- def dummy_super_res_first(self):
- torch.manual_seed(0)
-
- model = UNet2DModel(**self.dummy_super_res_kwargs)
- return model
-
- @property
- def dummy_super_res_last(self):
- # seeded differently to get different unet than `self.dummy_super_res_first`
- torch.manual_seed(1)
-
- model = UNet2DModel(**self.dummy_super_res_kwargs)
- return model
-
- def get_dummy_components(self):
- decoder = self.dummy_decoder
- text_proj = self.dummy_text_proj
- text_encoder = self.dummy_text_encoder
- tokenizer = self.dummy_tokenizer
- super_res_first = self.dummy_super_res_first
- super_res_last = self.dummy_super_res_last
-
- decoder_scheduler = UnCLIPScheduler(
- variance_type="learned_range",
- prediction_type="epsilon",
- num_train_timesteps=1000,
- )
-
- super_res_scheduler = UnCLIPScheduler(
- variance_type="fixed_small_log",
- prediction_type="epsilon",
- num_train_timesteps=1000,
- )
-
- feature_extractor = CLIPImageProcessor(crop_size=32, size=32)
-
- image_encoder = self.dummy_image_encoder
-
- return {
- "decoder": decoder,
- "text_encoder": text_encoder,
- "tokenizer": tokenizer,
- "text_proj": text_proj,
- "feature_extractor": feature_extractor,
- "image_encoder": image_encoder,
- "super_res_first": super_res_first,
- "super_res_last": super_res_last,
- "decoder_scheduler": decoder_scheduler,
- "super_res_scheduler": super_res_scheduler,
- }
-
- def get_dummy_inputs(self, device, seed=0, pil_image=True):
- input_image = floats_tensor((1, 3, 32, 32), rng=random.Random(seed)).to(device)
- if str(device).startswith("mps"):
- generator = torch.manual_seed(seed)
- else:
- generator = torch.Generator(device=device).manual_seed(seed)
-
- if pil_image:
- input_image = input_image * 0.5 + 0.5
- input_image = input_image.clamp(0, 1)
- input_image = input_image.cpu().permute(0, 2, 3, 1).float().numpy()
- input_image = DiffusionPipeline.numpy_to_pil(input_image)[0]
-
- return {
- "image": input_image,
- "generator": generator,
- "decoder_num_inference_steps": 2,
- "super_res_num_inference_steps": 2,
- "output_type": "np",
- }
-
- def test_unclip_image_variation_input_tensor(self):
- device = "cpu"
-
- components = self.get_dummy_components()
-
- pipe = self.pipeline_class(**components)
- pipe = pipe.to(device)
-
- pipe.set_progress_bar_config(disable=None)
-
- pipeline_inputs = self.get_dummy_inputs(device, pil_image=False)
-
- output = pipe(**pipeline_inputs)
- image = output.images
-
- tuple_pipeline_inputs = self.get_dummy_inputs(device, pil_image=False)
-
- image_from_tuple = pipe(
- **tuple_pipeline_inputs,
- return_dict=False,
- )[0]
-
- image_slice = image[0, -3:, -3:, -1]
- image_from_tuple_slice = image_from_tuple[0, -3:, -3:, -1]
-
- assert image.shape == (1, 64, 64, 3)
-
- expected_slice = np.array(
- [
- 0.9997,
- 0.0002,
- 0.9997,
- 0.9997,
- 0.9969,
- 0.0023,
- 0.9997,
- 0.9969,
- 0.9970,
- ]
- )
-
- assert np.abs(image_slice.flatten() - expected_slice).max() < 1e-2
- assert np.abs(image_from_tuple_slice.flatten() - expected_slice).max() < 1e-2
-
- def test_unclip_image_variation_input_image(self):
- device = "cpu"
-
- components = self.get_dummy_components()
-
- pipe = self.pipeline_class(**components)
- pipe = pipe.to(device)
-
- pipe.set_progress_bar_config(disable=None)
-
- pipeline_inputs = self.get_dummy_inputs(device, pil_image=True)
-
- output = pipe(**pipeline_inputs)
- image = output.images
-
- tuple_pipeline_inputs = self.get_dummy_inputs(device, pil_image=True)
-
- image_from_tuple = pipe(
- **tuple_pipeline_inputs,
- return_dict=False,
- )[0]
-
- image_slice = image[0, -3:, -3:, -1]
- image_from_tuple_slice = image_from_tuple[0, -3:, -3:, -1]
-
- assert image.shape == (1, 64, 64, 3)
-
- expected_slice = np.array([0.9997, 0.0003, 0.9997, 0.9997, 0.9970, 0.0024, 0.9997, 0.9971, 0.9971])
-
- assert np.abs(image_slice.flatten() - expected_slice).max() < 1e-2
- assert np.abs(image_from_tuple_slice.flatten() - expected_slice).max() < 1e-2
-
- def test_unclip_image_variation_input_list_images(self):
- device = "cpu"
-
- components = self.get_dummy_components()
-
- pipe = self.pipeline_class(**components)
- pipe = pipe.to(device)
-
- pipe.set_progress_bar_config(disable=None)
-
- pipeline_inputs = self.get_dummy_inputs(device, pil_image=True)
- pipeline_inputs["image"] = [
- pipeline_inputs["image"],
- pipeline_inputs["image"],
- ]
-
- output = pipe(**pipeline_inputs)
- image = output.images
-
- tuple_pipeline_inputs = self.get_dummy_inputs(device, pil_image=True)
- tuple_pipeline_inputs["image"] = [
- tuple_pipeline_inputs["image"],
- tuple_pipeline_inputs["image"],
- ]
-
- image_from_tuple = pipe(
- **tuple_pipeline_inputs,
- return_dict=False,
- )[0]
-
- image_slice = image[0, -3:, -3:, -1]
- image_from_tuple_slice = image_from_tuple[0, -3:, -3:, -1]
-
- assert image.shape == (2, 64, 64, 3)
-
- expected_slice = np.array(
- [
- 0.9997,
- 0.9989,
- 0.0008,
- 0.0021,
- 0.9960,
- 0.0018,
- 0.0014,
- 0.0002,
- 0.9933,
- ]
- )
-
- assert np.abs(image_slice.flatten() - expected_slice).max() < 1e-2
- assert np.abs(image_from_tuple_slice.flatten() - expected_slice).max() < 1e-2
-
- def test_unclip_passed_image_embed(self):
- device = torch.device("cpu")
-
- class DummyScheduler:
- init_noise_sigma = 1
-
- components = self.get_dummy_components()
-
- pipe = self.pipeline_class(**components)
- pipe = pipe.to(device)
-
- pipe.set_progress_bar_config(disable=None)
-
- generator = torch.Generator(device=device).manual_seed(0)
- dtype = pipe.decoder.dtype
- batch_size = 1
-
- shape = (batch_size, pipe.decoder.in_channels, pipe.decoder.sample_size, pipe.decoder.sample_size)
- decoder_latents = pipe.prepare_latents(
- shape, dtype=dtype, device=device, generator=generator, latents=None, scheduler=DummyScheduler()
- )
-
- shape = (
- batch_size,
- pipe.super_res_first.in_channels // 2,
- pipe.super_res_first.sample_size,
- pipe.super_res_first.sample_size,
- )
- super_res_latents = pipe.prepare_latents(
- shape, dtype=dtype, device=device, generator=generator, latents=None, scheduler=DummyScheduler()
- )
-
- pipeline_inputs = self.get_dummy_inputs(device, pil_image=False)
-
- img_out_1 = pipe(
- **pipeline_inputs, decoder_latents=decoder_latents, super_res_latents=super_res_latents
- ).images
-
- pipeline_inputs = self.get_dummy_inputs(device, pil_image=False)
- # Don't pass image, instead pass embedding
- image = pipeline_inputs.pop("image")
- image_embeddings = pipe.image_encoder(image).image_embeds
-
- img_out_2 = pipe(
- **pipeline_inputs,
- decoder_latents=decoder_latents,
- super_res_latents=super_res_latents,
- image_embeddings=image_embeddings,
- ).images
-
- # make sure passing text embeddings manually is identical
- assert np.abs(img_out_1 - img_out_2).max() < 1e-4
-
- # Overriding PipelineTesterMixin::test_attention_slicing_forward_pass
- # because UnCLIP GPU undeterminism requires a looser check.
- @skip_mps
- def test_attention_slicing_forward_pass(self):
- test_max_difference = torch_device == "cpu"
-
- self._test_attention_slicing_forward_pass(test_max_difference=test_max_difference)
-
- # Overriding PipelineTesterMixin::test_inference_batch_single_identical
- # because UnCLIP undeterminism requires a looser check.
- @skip_mps
- def test_inference_batch_single_identical(self):
- test_max_difference = torch_device == "cpu"
- relax_max_difference = True
- additional_params_copy_to_batched_inputs = [
- "decoder_num_inference_steps",
- "super_res_num_inference_steps",
- ]
-
- self._test_inference_batch_single_identical(
- test_max_difference=test_max_difference,
- relax_max_difference=relax_max_difference,
- additional_params_copy_to_batched_inputs=additional_params_copy_to_batched_inputs,
- )
-
- def test_inference_batch_consistent(self):
- additional_params_copy_to_batched_inputs = [
- "decoder_num_inference_steps",
- "super_res_num_inference_steps",
- ]
-
- if torch_device == "mps":
- # TODO: MPS errors with larger batch sizes
- batch_sizes = [2, 3]
- self._test_inference_batch_consistent(
- batch_sizes=batch_sizes,
- additional_params_copy_to_batched_inputs=additional_params_copy_to_batched_inputs,
- )
- else:
- self._test_inference_batch_consistent(
- additional_params_copy_to_batched_inputs=additional_params_copy_to_batched_inputs
- )
-
- @skip_mps
- def test_dict_tuple_outputs_equivalent(self):
- return super().test_dict_tuple_outputs_equivalent()
-
- @skip_mps
- def test_save_load_local(self):
- return super().test_save_load_local()
-
- @skip_mps
- def test_save_load_optional_components(self):
- return super().test_save_load_optional_components()
-
-
-@slow
-@require_torch_gpu
-class UnCLIPImageVariationPipelineIntegrationTests(unittest.TestCase):
- def tearDown(self):
- # clean up the VRAM after each test
- super().tearDown()
- gc.collect()
- torch.cuda.empty_cache()
-
- def test_unclip_image_variation_karlo(self):
- input_image = load_image(
- "https://huggingface.co/datasets/hf-internal-testing/diffusers-images/resolve/main/unclip/cat.png"
- )
- expected_image = load_numpy(
- "https://huggingface.co/datasets/hf-internal-testing/diffusers-images/resolve/main"
- "/unclip/karlo_v1_alpha_cat_variation_fp16.npy"
- )
-
- pipeline = UnCLIPImageVariationPipeline.from_pretrained(
- "kakaobrain/karlo-v1-alpha-image-variations", torch_dtype=torch.float16
- )
- pipeline = pipeline.to(torch_device)
- pipeline.set_progress_bar_config(disable=None)
-
- generator = torch.Generator(device="cpu").manual_seed(0)
- output = pipeline(
- input_image,
- generator=generator,
- output_type="np",
- )
-
- image = output.images[0]
-
- assert image.shape == (256, 256, 3)
-
- assert_mean_pixel_difference(image, expected_image)
diff --git a/spaces/deepwisdom/MetaGPT/tests/metagpt/document_store/test_milvus_store.py b/spaces/deepwisdom/MetaGPT/tests/metagpt/document_store/test_milvus_store.py
deleted file mode 100644
index 1cf65776dfad0c612104aec2c09c377e6c2003e1..0000000000000000000000000000000000000000
--- a/spaces/deepwisdom/MetaGPT/tests/metagpt/document_store/test_milvus_store.py
+++ /dev/null
@@ -1,36 +0,0 @@
-#!/usr/bin/env python
-# -*- coding: utf-8 -*-
-"""
-@Time : 2023/6/11 21:08
-@Author : alexanderwu
-@File : test_milvus_store.py
-"""
-import random
-
-import numpy as np
-
-from metagpt.document_store.milvus_store import MilvusConnection, MilvusStore
-from metagpt.logs import logger
-
-book_columns = {'idx': int, 'name': str, 'desc': str, 'emb': np.ndarray, 'price': float}
-book_data = [
- [i for i in range(10)],
- [f"book-{i}" for i in range(10)],
- [f"book-desc-{i}" for i in range(10000, 10010)],
- [[random.random() for _ in range(2)] for _ in range(10)],
- [random.random() for _ in range(10)],
-]
-
-
-def test_milvus_store():
- milvus_connection = MilvusConnection(alias="default", host="192.168.50.161", port="30530")
- milvus_store = MilvusStore(milvus_connection)
- milvus_store.drop('Book')
- milvus_store.create_collection('Book', book_columns)
- milvus_store.add(book_data)
- milvus_store.build_index('emb')
- milvus_store.load_collection()
-
- results = milvus_store.search([[1.0, 1.0]], field='emb')
- logger.info(results)
- assert results
diff --git a/spaces/denisp1/AR-VR-IOT-DEMO/README.md b/spaces/denisp1/AR-VR-IOT-DEMO/README.md
deleted file mode 100644
index d4173fca13462f6f8e3a60a3f34fa5028222237a..0000000000000000000000000000000000000000
--- a/spaces/denisp1/AR-VR-IOT-DEMO/README.md
+++ /dev/null
@@ -1,11 +0,0 @@
----
-title: AR VR IOT DEMO
-emoji: 🐨
-colorFrom: purple
-colorTo: green
-sdk: static
-pinned: false
-license: mit
----
-
-Check out the configuration reference at https://huggingface.co/docs/hub/spaces-config-reference
diff --git a/spaces/diacanFperku/AutoGPT/ALA - Little Melissa 34 Sets !!! Extra Quality.md b/spaces/diacanFperku/AutoGPT/ALA - Little Melissa 34 Sets !!! Extra Quality.md
deleted file mode 100644
index 974fb89a26b4f95cec9af2a8f015e610d0962c8e..0000000000000000000000000000000000000000
--- a/spaces/diacanFperku/AutoGPT/ALA - Little Melissa 34 Sets !!! Extra Quality.md
+++ /dev/null
@@ -1,8 +0,0 @@
-
-we're super excited to announce a new collaboration with our friends at sage advice, to bring you some of the best products from the sage advice site in the melissa & doug shop. whether you are looking for fun activities for children, helpful parenting advice, or a great gift for your child, sage advice has all your needs covered.
-wake up your child's creative side by giving them the perfect set of melissa & doug 24 hour color-coding blocks. this fun and educational set of blocks has a variety of bright colors that help kids learn how to make a variety of different color combinations. the 24 hour color-coding blocks also include 24 hour time-saver cards with easy-to-follow step-by-step instructions on how to make each color and combination. kids can play and learn all day long!
-{ALA - Little Melissa 34 Sets !!!}
Download ✦✦✦ https://gohhs.com/2uFT4B
-introducing the geometric playset. for years, melissa & doug has provided lots of imaginative toys and games that inspire creativity and encourage problem solving. now we are introducing a new line of geometric playsets that are perfect for any child's room. whether a kid has a limited imagination or is interested in drawing, building and more, geometric playsets will encourage creative play in kids of all ages.
-we've got everything you need to get kids creating right now! this fall, melissa & doug is introducing the first in a new collection of creativity-boosting accessories that take thinking outside of the box to a whole new level. the following accessories are a great starting point for parents who are looking for the right tools for their child's creative play:
899543212b
-
-
\ No newline at end of file
diff --git a/spaces/diacanFperku/AutoGPT/I-Doser Drug-Files (81 Doses) Free Download 2021.md b/spaces/diacanFperku/AutoGPT/I-Doser Drug-Files (81 Doses) Free Download 2021.md
deleted file mode 100644
index f79ab8f8dd563304fb0665370d9ed45e62ecead1..0000000000000000000000000000000000000000
--- a/spaces/diacanFperku/AutoGPT/I-Doser Drug-Files (81 Doses) Free Download 2021.md
+++ /dev/null
@@ -1,6 +0,0 @@
-I-Doser Drug-Files (81 Doses) Free Download
Download Zip ---> https://gohhs.com/2uFVnz
-
-Label: ASPIRIN 81 MG LOW DOSE - Delayed Release Aspirin Tablet · SPL UNCLOSED SECTION. Drug Facts · Active ingredient (in each tablet). Aspirin 81 mg (NSAID*). *NSAIDs are non-steroidal anti-inflammatory drugs. * *Non-steroidal anti-inflammatory drugs (NSAIDs) are a group of medicines that lower body temperature and reduce or lessen inflammation. The tablets also contain sugar. * *An aspirin tablet contains three times more sugar than a cup of coffee. Other forms. Tablets, capsules and other dosage forms containing aspirin. * *Aspirin is not recommended for pregnant women. Synonyms and dosage forms. 8a78ff9644
-
-
-
diff --git a/spaces/digitalxingtong/Nailv-Bert-Vits2/transforms.py b/spaces/digitalxingtong/Nailv-Bert-Vits2/transforms.py
deleted file mode 100644
index 4793d67ca5a5630e0ffe0f9fb29445c949e64dae..0000000000000000000000000000000000000000
--- a/spaces/digitalxingtong/Nailv-Bert-Vits2/transforms.py
+++ /dev/null
@@ -1,193 +0,0 @@
-import torch
-from torch.nn import functional as F
-
-import numpy as np
-
-
-DEFAULT_MIN_BIN_WIDTH = 1e-3
-DEFAULT_MIN_BIN_HEIGHT = 1e-3
-DEFAULT_MIN_DERIVATIVE = 1e-3
-
-
-def piecewise_rational_quadratic_transform(inputs,
- unnormalized_widths,
- unnormalized_heights,
- unnormalized_derivatives,
- inverse=False,
- tails=None,
- tail_bound=1.,
- min_bin_width=DEFAULT_MIN_BIN_WIDTH,
- min_bin_height=DEFAULT_MIN_BIN_HEIGHT,
- min_derivative=DEFAULT_MIN_DERIVATIVE):
-
- if tails is None:
- spline_fn = rational_quadratic_spline
- spline_kwargs = {}
- else:
- spline_fn = unconstrained_rational_quadratic_spline
- spline_kwargs = {
- 'tails': tails,
- 'tail_bound': tail_bound
- }
-
- outputs, logabsdet = spline_fn(
- inputs=inputs,
- unnormalized_widths=unnormalized_widths,
- unnormalized_heights=unnormalized_heights,
- unnormalized_derivatives=unnormalized_derivatives,
- inverse=inverse,
- min_bin_width=min_bin_width,
- min_bin_height=min_bin_height,
- min_derivative=min_derivative,
- **spline_kwargs
- )
- return outputs, logabsdet
-
-
-def searchsorted(bin_locations, inputs, eps=1e-6):
- bin_locations[..., -1] += eps
- return torch.sum(
- inputs[..., None] >= bin_locations,
- dim=-1
- ) - 1
-
-
-def unconstrained_rational_quadratic_spline(inputs,
- unnormalized_widths,
- unnormalized_heights,
- unnormalized_derivatives,
- inverse=False,
- tails='linear',
- tail_bound=1.,
- min_bin_width=DEFAULT_MIN_BIN_WIDTH,
- min_bin_height=DEFAULT_MIN_BIN_HEIGHT,
- min_derivative=DEFAULT_MIN_DERIVATIVE):
- inside_interval_mask = (inputs >= -tail_bound) & (inputs <= tail_bound)
- outside_interval_mask = ~inside_interval_mask
-
- outputs = torch.zeros_like(inputs)
- logabsdet = torch.zeros_like(inputs)
-
- if tails == 'linear':
- unnormalized_derivatives = F.pad(unnormalized_derivatives, pad=(1, 1))
- constant = np.log(np.exp(1 - min_derivative) - 1)
- unnormalized_derivatives[..., 0] = constant
- unnormalized_derivatives[..., -1] = constant
-
- outputs[outside_interval_mask] = inputs[outside_interval_mask]
- logabsdet[outside_interval_mask] = 0
- else:
- raise RuntimeError('{} tails are not implemented.'.format(tails))
-
- outputs[inside_interval_mask], logabsdet[inside_interval_mask] = rational_quadratic_spline(
- inputs=inputs[inside_interval_mask],
- unnormalized_widths=unnormalized_widths[inside_interval_mask, :],
- unnormalized_heights=unnormalized_heights[inside_interval_mask, :],
- unnormalized_derivatives=unnormalized_derivatives[inside_interval_mask, :],
- inverse=inverse,
- left=-tail_bound, right=tail_bound, bottom=-tail_bound, top=tail_bound,
- min_bin_width=min_bin_width,
- min_bin_height=min_bin_height,
- min_derivative=min_derivative
- )
-
- return outputs, logabsdet
-
-def rational_quadratic_spline(inputs,
- unnormalized_widths,
- unnormalized_heights,
- unnormalized_derivatives,
- inverse=False,
- left=0., right=1., bottom=0., top=1.,
- min_bin_width=DEFAULT_MIN_BIN_WIDTH,
- min_bin_height=DEFAULT_MIN_BIN_HEIGHT,
- min_derivative=DEFAULT_MIN_DERIVATIVE):
- if torch.min(inputs) < left or torch.max(inputs) > right:
- raise ValueError('Input to a transform is not within its domain')
-
- num_bins = unnormalized_widths.shape[-1]
-
- if min_bin_width * num_bins > 1.0:
- raise ValueError('Minimal bin width too large for the number of bins')
- if min_bin_height * num_bins > 1.0:
- raise ValueError('Minimal bin height too large for the number of bins')
-
- widths = F.softmax(unnormalized_widths, dim=-1)
- widths = min_bin_width + (1 - min_bin_width * num_bins) * widths
- cumwidths = torch.cumsum(widths, dim=-1)
- cumwidths = F.pad(cumwidths, pad=(1, 0), mode='constant', value=0.0)
- cumwidths = (right - left) * cumwidths + left
- cumwidths[..., 0] = left
- cumwidths[..., -1] = right
- widths = cumwidths[..., 1:] - cumwidths[..., :-1]
-
- derivatives = min_derivative + F.softplus(unnormalized_derivatives)
-
- heights = F.softmax(unnormalized_heights, dim=-1)
- heights = min_bin_height + (1 - min_bin_height * num_bins) * heights
- cumheights = torch.cumsum(heights, dim=-1)
- cumheights = F.pad(cumheights, pad=(1, 0), mode='constant', value=0.0)
- cumheights = (top - bottom) * cumheights + bottom
- cumheights[..., 0] = bottom
- cumheights[..., -1] = top
- heights = cumheights[..., 1:] - cumheights[..., :-1]
-
- if inverse:
- bin_idx = searchsorted(cumheights, inputs)[..., None]
- else:
- bin_idx = searchsorted(cumwidths, inputs)[..., None]
-
- input_cumwidths = cumwidths.gather(-1, bin_idx)[..., 0]
- input_bin_widths = widths.gather(-1, bin_idx)[..., 0]
-
- input_cumheights = cumheights.gather(-1, bin_idx)[..., 0]
- delta = heights / widths
- input_delta = delta.gather(-1, bin_idx)[..., 0]
-
- input_derivatives = derivatives.gather(-1, bin_idx)[..., 0]
- input_derivatives_plus_one = derivatives[..., 1:].gather(-1, bin_idx)[..., 0]
-
- input_heights = heights.gather(-1, bin_idx)[..., 0]
-
- if inverse:
- a = (((inputs - input_cumheights) * (input_derivatives
- + input_derivatives_plus_one
- - 2 * input_delta)
- + input_heights * (input_delta - input_derivatives)))
- b = (input_heights * input_derivatives
- - (inputs - input_cumheights) * (input_derivatives
- + input_derivatives_plus_one
- - 2 * input_delta))
- c = - input_delta * (inputs - input_cumheights)
-
- discriminant = b.pow(2) - 4 * a * c
- assert (discriminant >= 0).all()
-
- root = (2 * c) / (-b - torch.sqrt(discriminant))
- outputs = root * input_bin_widths + input_cumwidths
-
- theta_one_minus_theta = root * (1 - root)
- denominator = input_delta + ((input_derivatives + input_derivatives_plus_one - 2 * input_delta)
- * theta_one_minus_theta)
- derivative_numerator = input_delta.pow(2) * (input_derivatives_plus_one * root.pow(2)
- + 2 * input_delta * theta_one_minus_theta
- + input_derivatives * (1 - root).pow(2))
- logabsdet = torch.log(derivative_numerator) - 2 * torch.log(denominator)
-
- return outputs, -logabsdet
- else:
- theta = (inputs - input_cumwidths) / input_bin_widths
- theta_one_minus_theta = theta * (1 - theta)
-
- numerator = input_heights * (input_delta * theta.pow(2)
- + input_derivatives * theta_one_minus_theta)
- denominator = input_delta + ((input_derivatives + input_derivatives_plus_one - 2 * input_delta)
- * theta_one_minus_theta)
- outputs = input_cumheights + numerator / denominator
-
- derivative_numerator = input_delta.pow(2) * (input_derivatives_plus_one * theta.pow(2)
- + 2 * input_delta * theta_one_minus_theta
- + input_derivatives * (1 - theta).pow(2))
- logabsdet = torch.log(derivative_numerator) - 2 * torch.log(denominator)
-
- return outputs, logabsdet
diff --git a/spaces/digitalxingtong/Un-Bert-Vits2/README_zh.md b/spaces/digitalxingtong/Un-Bert-Vits2/README_zh.md
deleted file mode 100644
index 8b137891791fe96927ad78e64b0aad7bded08bdc..0000000000000000000000000000000000000000
--- a/spaces/digitalxingtong/Un-Bert-Vits2/README_zh.md
+++ /dev/null
@@ -1 +0,0 @@
-
diff --git a/spaces/digitalxingtong/Xingtong-Longread-Bert-VITS2/text/tone_sandhi.py b/spaces/digitalxingtong/Xingtong-Longread-Bert-VITS2/text/tone_sandhi.py
deleted file mode 100644
index 0f45b7a72c5d858bcaab19ac85cfa686bf9a74da..0000000000000000000000000000000000000000
--- a/spaces/digitalxingtong/Xingtong-Longread-Bert-VITS2/text/tone_sandhi.py
+++ /dev/null
@@ -1,351 +0,0 @@
-# Copyright (c) 2021 PaddlePaddle Authors. All Rights Reserved.
-#
-# Licensed under the Apache License, Version 2.0 (the "License");
-# you may not use this file except in compliance with the License.
-# You may obtain a copy of the License at
-#
-# http://www.apache.org/licenses/LICENSE-2.0
-#
-# Unless required by applicable law or agreed to in writing, software
-# distributed under the License is distributed on an "AS IS" BASIS,
-# WITHOUT WARRANTIES OR CONDITIONS OF ANY KIND, either express or implied.
-# See the License for the specific language governing permissions and
-# limitations under the License.
-from typing import List
-from typing import Tuple
-
-import jieba
-from pypinyin import lazy_pinyin
-from pypinyin import Style
-
-
-class ToneSandhi():
- def __init__(self):
- self.must_neural_tone_words = {
- '麻烦', '麻利', '鸳鸯', '高粱', '骨头', '骆驼', '马虎', '首饰', '馒头', '馄饨', '风筝',
- '难为', '队伍', '阔气', '闺女', '门道', '锄头', '铺盖', '铃铛', '铁匠', '钥匙', '里脊',
- '里头', '部分', '那么', '道士', '造化', '迷糊', '连累', '这么', '这个', '运气', '过去',
- '软和', '转悠', '踏实', '跳蚤', '跟头', '趔趄', '财主', '豆腐', '讲究', '记性', '记号',
- '认识', '规矩', '见识', '裁缝', '补丁', '衣裳', '衣服', '衙门', '街坊', '行李', '行当',
- '蛤蟆', '蘑菇', '薄荷', '葫芦', '葡萄', '萝卜', '荸荠', '苗条', '苗头', '苍蝇', '芝麻',
- '舒服', '舒坦', '舌头', '自在', '膏药', '脾气', '脑袋', '脊梁', '能耐', '胳膊', '胭脂',
- '胡萝', '胡琴', '胡同', '聪明', '耽误', '耽搁', '耷拉', '耳朵', '老爷', '老实', '老婆',
- '老头', '老太', '翻腾', '罗嗦', '罐头', '编辑', '结实', '红火', '累赘', '糨糊', '糊涂',
- '精神', '粮食', '簸箕', '篱笆', '算计', '算盘', '答应', '笤帚', '笑语', '笑话', '窟窿',
- '窝囊', '窗户', '稳当', '稀罕', '称呼', '秧歌', '秀气', '秀才', '福气', '祖宗', '砚台',
- '码头', '石榴', '石头', '石匠', '知识', '眼睛', '眯缝', '眨巴', '眉毛', '相声', '盘算',
- '白净', '痢疾', '痛快', '疟疾', '疙瘩', '疏忽', '畜生', '生意', '甘蔗', '琵琶', '琢磨',
- '琉璃', '玻璃', '玫瑰', '玄乎', '狐狸', '状元', '特务', '牲口', '牙碜', '牌楼', '爽快',
- '爱人', '热闹', '烧饼', '烟筒', '烂糊', '点心', '炊帚', '灯笼', '火候', '漂亮', '滑溜',
- '溜达', '温和', '清楚', '消息', '浪头', '活泼', '比方', '正经', '欺负', '模糊', '槟榔',
- '棺材', '棒槌', '棉花', '核桃', '栅栏', '柴火', '架势', '枕头', '枇杷', '机灵', '本事',
- '木头', '木匠', '朋友', '月饼', '月亮', '暖和', '明白', '时候', '新鲜', '故事', '收拾',
- '收成', '提防', '挖苦', '挑剔', '指甲', '指头', '拾掇', '拳头', '拨弄', '招牌', '招呼',
- '抬举', '护士', '折腾', '扫帚', '打量', '打算', '打点', '打扮', '打听', '打发', '扎实',
- '扁担', '戒指', '懒得', '意识', '意思', '情形', '悟性', '怪物', '思量', '怎么', '念头',
- '念叨', '快活', '忙活', '志气', '心思', '得罪', '张罗', '弟兄', '开通', '应酬', '庄稼',
- '干事', '帮手', '帐篷', '希罕', '师父', '师傅', '巴结', '巴掌', '差事', '工夫', '岁数',
- '屁股', '尾巴', '少爷', '小气', '小伙', '将就', '对头', '对付', '寡妇', '家伙', '客气',
- '实在', '官司', '学问', '学生', '字号', '嫁妆', '媳妇', '媒人', '婆家', '娘家', '委屈',
- '姑娘', '姐夫', '妯娌', '妥当', '妖精', '奴才', '女婿', '头发', '太阳', '大爷', '大方',
- '大意', '大夫', '多少', '多么', '外甥', '壮实', '地道', '地方', '在乎', '困难', '嘴巴',
- '嘱咐', '嘟囔', '嘀咕', '喜欢', '喇嘛', '喇叭', '商量', '唾沫', '哑巴', '哈欠', '哆嗦',
- '咳嗽', '和尚', '告诉', '告示', '含糊', '吓唬', '后头', '名字', '名堂', '合同', '吆喝',
- '叫唤', '口袋', '厚道', '厉害', '千斤', '包袱', '包涵', '匀称', '勤快', '动静', '动弹',
- '功夫', '力气', '前头', '刺猬', '刺激', '别扭', '利落', '利索', '利害', '分析', '出息',
- '凑合', '凉快', '冷战', '冤枉', '冒失', '养活', '关系', '先生', '兄弟', '便宜', '使唤',
- '佩服', '作坊', '体面', '位置', '似的', '伙计', '休息', '什么', '人家', '亲戚', '亲家',
- '交情', '云彩', '事情', '买卖', '主意', '丫头', '丧气', '两口', '东西', '东家', '世故',
- '不由', '不在', '下水', '下巴', '上头', '上司', '丈夫', '丈人', '一辈', '那个', '菩萨',
- '父亲', '母亲', '咕噜', '邋遢', '费用', '冤家', '甜头', '介绍', '荒唐', '大人', '泥鳅',
- '幸福', '熟悉', '计划', '扑腾', '蜡烛', '姥爷', '照顾', '喉咙', '吉他', '弄堂', '蚂蚱',
- '凤凰', '拖沓', '寒碜', '糟蹋', '倒腾', '报复', '逻辑', '盘缠', '喽啰', '牢骚', '咖喱',
- '扫把', '惦记'
- }
- self.must_not_neural_tone_words = {
- "男子", "女子", "分子", "原子", "量子", "莲子", "石子", "瓜子", "电子", "人人", "虎虎"
- }
- self.punc = ":,;。?!“”‘’':,;.?!"
-
- # the meaning of jieba pos tag: https://blog.csdn.net/weixin_44174352/article/details/113731041
- # e.g.
- # word: "家里"
- # pos: "s"
- # finals: ['ia1', 'i3']
- def _neural_sandhi(self, word: str, pos: str,
- finals: List[str]) -> List[str]:
-
- # reduplication words for n. and v. e.g. 奶奶, 试试, 旺旺
- for j, item in enumerate(word):
- if j - 1 >= 0 and item == word[j - 1] and pos[0] in {
- "n", "v", "a"
- } and word not in self.must_not_neural_tone_words:
- finals[j] = finals[j][:-1] + "5"
- ge_idx = word.find("个")
- if len(word) >= 1 and word[-1] in "吧呢啊呐噻嘛吖嗨呐哦哒额滴哩哟喽啰耶喔诶":
- finals[-1] = finals[-1][:-1] + "5"
- elif len(word) >= 1 and word[-1] in "的地得":
- finals[-1] = finals[-1][:-1] + "5"
- # e.g. 走了, 看着, 去过
- # elif len(word) == 1 and word in "了着过" and pos in {"ul", "uz", "ug"}:
- # finals[-1] = finals[-1][:-1] + "5"
- elif len(word) > 1 and word[-1] in "们子" and pos in {
- "r", "n"
- } and word not in self.must_not_neural_tone_words:
- finals[-1] = finals[-1][:-1] + "5"
- # e.g. 桌上, 地下, 家里
- elif len(word) > 1 and word[-1] in "上下里" and pos in {"s", "l", "f"}:
- finals[-1] = finals[-1][:-1] + "5"
- # e.g. 上来, 下去
- elif len(word) > 1 and word[-1] in "来去" and word[-2] in "上下进出回过起开":
- finals[-1] = finals[-1][:-1] + "5"
- # 个做量词
- elif (ge_idx >= 1 and
- (word[ge_idx - 1].isnumeric() or
- word[ge_idx - 1] in "几有两半多各整每做是")) or word == '个':
- finals[ge_idx] = finals[ge_idx][:-1] + "5"
- else:
- if word in self.must_neural_tone_words or word[
- -2:] in self.must_neural_tone_words:
- finals[-1] = finals[-1][:-1] + "5"
-
- word_list = self._split_word(word)
- finals_list = [finals[:len(word_list[0])], finals[len(word_list[0]):]]
- for i, word in enumerate(word_list):
- # conventional neural in Chinese
- if word in self.must_neural_tone_words or word[
- -2:] in self.must_neural_tone_words:
- finals_list[i][-1] = finals_list[i][-1][:-1] + "5"
- finals = sum(finals_list, [])
- return finals
-
- def _bu_sandhi(self, word: str, finals: List[str]) -> List[str]:
- # e.g. 看不懂
- if len(word) == 3 and word[1] == "不":
- finals[1] = finals[1][:-1] + "5"
- else:
- for i, char in enumerate(word):
- # "不" before tone4 should be bu2, e.g. 不怕
- if char == "不" and i + 1 < len(word) and finals[i +
- 1][-1] == "4":
- finals[i] = finals[i][:-1] + "2"
- return finals
-
- def _yi_sandhi(self, word: str, finals: List[str]) -> List[str]:
- # "一" in number sequences, e.g. 一零零, 二一零
- if word.find("一") != -1 and all(
- [item.isnumeric() for item in word if item != "一"]):
- return finals
- # "一" between reduplication words shold be yi5, e.g. 看一看
- elif len(word) == 3 and word[1] == "一" and word[0] == word[-1]:
- finals[1] = finals[1][:-1] + "5"
- # when "一" is ordinal word, it should be yi1
- elif word.startswith("第一"):
- finals[1] = finals[1][:-1] + "1"
- else:
- for i, char in enumerate(word):
- if char == "一" and i + 1 < len(word):
- # "一" before tone4 should be yi2, e.g. 一段
- if finals[i + 1][-1] == "4":
- finals[i] = finals[i][:-1] + "2"
- # "一" before non-tone4 should be yi4, e.g. 一天
- else:
- # "一" 后面如果是标点,还读一声
- if word[i + 1] not in self.punc:
- finals[i] = finals[i][:-1] + "4"
- return finals
-
- def _split_word(self, word: str) -> List[str]:
- word_list = jieba.cut_for_search(word)
- word_list = sorted(word_list, key=lambda i: len(i), reverse=False)
- first_subword = word_list[0]
- first_begin_idx = word.find(first_subword)
- if first_begin_idx == 0:
- second_subword = word[len(first_subword):]
- new_word_list = [first_subword, second_subword]
- else:
- second_subword = word[:-len(first_subword)]
- new_word_list = [second_subword, first_subword]
- return new_word_list
-
- def _three_sandhi(self, word: str, finals: List[str]) -> List[str]:
- if len(word) == 2 and self._all_tone_three(finals):
- finals[0] = finals[0][:-1] + "2"
- elif len(word) == 3:
- word_list = self._split_word(word)
- if self._all_tone_three(finals):
- # disyllabic + monosyllabic, e.g. 蒙古/包
- if len(word_list[0]) == 2:
- finals[0] = finals[0][:-1] + "2"
- finals[1] = finals[1][:-1] + "2"
- # monosyllabic + disyllabic, e.g. 纸/老虎
- elif len(word_list[0]) == 1:
- finals[1] = finals[1][:-1] + "2"
- else:
- finals_list = [
- finals[:len(word_list[0])], finals[len(word_list[0]):]
- ]
- if len(finals_list) == 2:
- for i, sub in enumerate(finals_list):
- # e.g. 所有/人
- if self._all_tone_three(sub) and len(sub) == 2:
- finals_list[i][0] = finals_list[i][0][:-1] + "2"
- # e.g. 好/喜欢
- elif i == 1 and not self._all_tone_three(sub) and finals_list[i][0][-1] == "3" and \
- finals_list[0][-1][-1] == "3":
-
- finals_list[0][-1] = finals_list[0][-1][:-1] + "2"
- finals = sum(finals_list, [])
- # split idiom into two words who's length is 2
- elif len(word) == 4:
- finals_list = [finals[:2], finals[2:]]
- finals = []
- for sub in finals_list:
- if self._all_tone_three(sub):
- sub[0] = sub[0][:-1] + "2"
- finals += sub
-
- return finals
-
- def _all_tone_three(self, finals: List[str]) -> bool:
- return all(x[-1] == "3" for x in finals)
-
- # merge "不" and the word behind it
- # if don't merge, "不" sometimes appears alone according to jieba, which may occur sandhi error
- def _merge_bu(self, seg: List[Tuple[str, str]]) -> List[Tuple[str, str]]:
- new_seg = []
- last_word = ""
- for word, pos in seg:
- if last_word == "不":
- word = last_word + word
- if word != "不":
- new_seg.append((word, pos))
- last_word = word[:]
- if last_word == "不":
- new_seg.append((last_word, 'd'))
- last_word = ""
- return new_seg
-
- # function 1: merge "一" and reduplication words in it's left and right, e.g. "听","一","听" ->"听一听"
- # function 2: merge single "一" and the word behind it
- # if don't merge, "一" sometimes appears alone according to jieba, which may occur sandhi error
- # e.g.
- # input seg: [('听', 'v'), ('一', 'm'), ('听', 'v')]
- # output seg: [['听一听', 'v']]
- def _merge_yi(self, seg: List[Tuple[str, str]]) -> List[Tuple[str, str]]:
- new_seg = []
- # function 1
- for i, (word, pos) in enumerate(seg):
- if i - 1 >= 0 and word == "一" and i + 1 < len(seg) and seg[i - 1][
- 0] == seg[i + 1][0] and seg[i - 1][1] == "v":
- new_seg[i - 1][0] = new_seg[i - 1][0] + "一" + new_seg[i - 1][0]
- else:
- if i - 2 >= 0 and seg[i - 1][0] == "一" and seg[i - 2][
- 0] == word and pos == "v":
- continue
- else:
- new_seg.append([word, pos])
- seg = new_seg
- new_seg = []
- # function 2
- for i, (word, pos) in enumerate(seg):
- if new_seg and new_seg[-1][0] == "一":
- new_seg[-1][0] = new_seg[-1][0] + word
- else:
- new_seg.append([word, pos])
- return new_seg
-
- # the first and the second words are all_tone_three
- def _merge_continuous_three_tones(
- self, seg: List[Tuple[str, str]]) -> List[Tuple[str, str]]:
- new_seg = []
- sub_finals_list = [
- lazy_pinyin(
- word, neutral_tone_with_five=True, style=Style.FINALS_TONE3)
- for (word, pos) in seg
- ]
- assert len(sub_finals_list) == len(seg)
- merge_last = [False] * len(seg)
- for i, (word, pos) in enumerate(seg):
- if i - 1 >= 0 and self._all_tone_three(
- sub_finals_list[i - 1]) and self._all_tone_three(
- sub_finals_list[i]) and not merge_last[i - 1]:
- # if the last word is reduplication, not merge, because reduplication need to be _neural_sandhi
- if not self._is_reduplication(seg[i - 1][0]) and len(
- seg[i - 1][0]) + len(seg[i][0]) <= 3:
- new_seg[-1][0] = new_seg[-1][0] + seg[i][0]
- merge_last[i] = True
- else:
- new_seg.append([word, pos])
- else:
- new_seg.append([word, pos])
-
- return new_seg
-
- def _is_reduplication(self, word: str) -> bool:
- return len(word) == 2 and word[0] == word[1]
-
- # the last char of first word and the first char of second word is tone_three
- def _merge_continuous_three_tones_2(
- self, seg: List[Tuple[str, str]]) -> List[Tuple[str, str]]:
- new_seg = []
- sub_finals_list = [
- lazy_pinyin(
- word, neutral_tone_with_five=True, style=Style.FINALS_TONE3)
- for (word, pos) in seg
- ]
- assert len(sub_finals_list) == len(seg)
- merge_last = [False] * len(seg)
- for i, (word, pos) in enumerate(seg):
- if i - 1 >= 0 and sub_finals_list[i - 1][-1][-1] == "3" and sub_finals_list[i][0][-1] == "3" and not \
- merge_last[i - 1]:
- # if the last word is reduplication, not merge, because reduplication need to be _neural_sandhi
- if not self._is_reduplication(seg[i - 1][0]) and len(
- seg[i - 1][0]) + len(seg[i][0]) <= 3:
- new_seg[-1][0] = new_seg[-1][0] + seg[i][0]
- merge_last[i] = True
- else:
- new_seg.append([word, pos])
- else:
- new_seg.append([word, pos])
- return new_seg
-
- def _merge_er(self, seg: List[Tuple[str, str]]) -> List[Tuple[str, str]]:
- new_seg = []
- for i, (word, pos) in enumerate(seg):
- if i - 1 >= 0 and word == "儿" and seg[i-1][0] != "#":
- new_seg[-1][0] = new_seg[-1][0] + seg[i][0]
- else:
- new_seg.append([word, pos])
- return new_seg
-
- def _merge_reduplication(
- self, seg: List[Tuple[str, str]]) -> List[Tuple[str, str]]:
- new_seg = []
- for i, (word, pos) in enumerate(seg):
- if new_seg and word == new_seg[-1][0]:
- new_seg[-1][0] = new_seg[-1][0] + seg[i][0]
- else:
- new_seg.append([word, pos])
- return new_seg
-
- def pre_merge_for_modify(
- self, seg: List[Tuple[str, str]]) -> List[Tuple[str, str]]:
- seg = self._merge_bu(seg)
- try:
- seg = self._merge_yi(seg)
- except:
- print("_merge_yi failed")
- seg = self._merge_reduplication(seg)
- seg = self._merge_continuous_three_tones(seg)
- seg = self._merge_continuous_three_tones_2(seg)
- seg = self._merge_er(seg)
- return seg
-
- def modified_tone(self, word: str, pos: str,
- finals: List[str]) -> List[str]:
- finals = self._bu_sandhi(word, finals)
- finals = self._yi_sandhi(word, finals)
- finals = self._neural_sandhi(word, pos, finals)
- finals = self._three_sandhi(word, finals)
- return finals
diff --git a/spaces/digitalxingtong/Xingtong-Read-Dongmuchang-Bert-VITS2/README_zh.md b/spaces/digitalxingtong/Xingtong-Read-Dongmuchang-Bert-VITS2/README_zh.md
deleted file mode 100644
index 8b137891791fe96927ad78e64b0aad7bded08bdc..0000000000000000000000000000000000000000
--- a/spaces/digitalxingtong/Xingtong-Read-Dongmuchang-Bert-VITS2/README_zh.md
+++ /dev/null
@@ -1 +0,0 @@
-
diff --git a/spaces/dineshreddy/WALT/mmdet/models/dense_heads/fcos_head.py b/spaces/dineshreddy/WALT/mmdet/models/dense_heads/fcos_head.py
deleted file mode 100644
index 905a703507f279ac8d34cff23c99af33c0d5f973..0000000000000000000000000000000000000000
--- a/spaces/dineshreddy/WALT/mmdet/models/dense_heads/fcos_head.py
+++ /dev/null
@@ -1,629 +0,0 @@
-import torch
-import torch.nn as nn
-import torch.nn.functional as F
-from mmcv.cnn import Scale, normal_init
-from mmcv.runner import force_fp32
-
-from mmdet.core import distance2bbox, multi_apply, multiclass_nms, reduce_mean
-from ..builder import HEADS, build_loss
-from .anchor_free_head import AnchorFreeHead
-
-INF = 1e8
-
-
-@HEADS.register_module()
-class FCOSHead(AnchorFreeHead):
- """Anchor-free head used in `FCOS `_.
-
- The FCOS head does not use anchor boxes. Instead bounding boxes are
- predicted at each pixel and a centerness measure is used to suppress
- low-quality predictions.
- Here norm_on_bbox, centerness_on_reg, dcn_on_last_conv are training
- tricks used in official repo, which will bring remarkable mAP gains
- of up to 4.9. Please see https://github.com/tianzhi0549/FCOS for
- more detail.
-
- Args:
- num_classes (int): Number of categories excluding the background
- category.
- in_channels (int): Number of channels in the input feature map.
- strides (list[int] | list[tuple[int, int]]): Strides of points
- in multiple feature levels. Default: (4, 8, 16, 32, 64).
- regress_ranges (tuple[tuple[int, int]]): Regress range of multiple
- level points.
- center_sampling (bool): If true, use center sampling. Default: False.
- center_sample_radius (float): Radius of center sampling. Default: 1.5.
- norm_on_bbox (bool): If true, normalize the regression targets
- with FPN strides. Default: False.
- centerness_on_reg (bool): If true, position centerness on the
- regress branch. Please refer to https://github.com/tianzhi0549/FCOS/issues/89#issuecomment-516877042.
- Default: False.
- conv_bias (bool | str): If specified as `auto`, it will be decided by the
- norm_cfg. Bias of conv will be set as True if `norm_cfg` is None, otherwise
- False. Default: "auto".
- loss_cls (dict): Config of classification loss.
- loss_bbox (dict): Config of localization loss.
- loss_centerness (dict): Config of centerness loss.
- norm_cfg (dict): dictionary to construct and config norm layer.
- Default: norm_cfg=dict(type='GN', num_groups=32, requires_grad=True).
-
- Example:
- >>> self = FCOSHead(11, 7)
- >>> feats = [torch.rand(1, 7, s, s) for s in [4, 8, 16, 32, 64]]
- >>> cls_score, bbox_pred, centerness = self.forward(feats)
- >>> assert len(cls_score) == len(self.scales)
- """ # noqa: E501
-
- def __init__(self,
- num_classes,
- in_channels,
- regress_ranges=((-1, 64), (64, 128), (128, 256), (256, 512),
- (512, INF)),
- center_sampling=False,
- center_sample_radius=1.5,
- norm_on_bbox=False,
- centerness_on_reg=False,
- loss_cls=dict(
- type='FocalLoss',
- use_sigmoid=True,
- gamma=2.0,
- alpha=0.25,
- loss_weight=1.0),
- loss_bbox=dict(type='IoULoss', loss_weight=1.0),
- loss_centerness=dict(
- type='CrossEntropyLoss',
- use_sigmoid=True,
- loss_weight=1.0),
- norm_cfg=dict(type='GN', num_groups=32, requires_grad=True),
- **kwargs):
- self.regress_ranges = regress_ranges
- self.center_sampling = center_sampling
- self.center_sample_radius = center_sample_radius
- self.norm_on_bbox = norm_on_bbox
- self.centerness_on_reg = centerness_on_reg
- super().__init__(
- num_classes,
- in_channels,
- loss_cls=loss_cls,
- loss_bbox=loss_bbox,
- norm_cfg=norm_cfg,
- **kwargs)
- self.loss_centerness = build_loss(loss_centerness)
-
- def _init_layers(self):
- """Initialize layers of the head."""
- super()._init_layers()
- self.conv_centerness = nn.Conv2d(self.feat_channels, 1, 3, padding=1)
- self.scales = nn.ModuleList([Scale(1.0) for _ in self.strides])
-
- def init_weights(self):
- """Initialize weights of the head."""
- super().init_weights()
- normal_init(self.conv_centerness, std=0.01)
-
- def forward(self, feats):
- """Forward features from the upstream network.
-
- Args:
- feats (tuple[Tensor]): Features from the upstream network, each is
- a 4D-tensor.
-
- Returns:
- tuple:
- cls_scores (list[Tensor]): Box scores for each scale level, \
- each is a 4D-tensor, the channel number is \
- num_points * num_classes.
- bbox_preds (list[Tensor]): Box energies / deltas for each \
- scale level, each is a 4D-tensor, the channel number is \
- num_points * 4.
- centernesses (list[Tensor]): centerness for each scale level, \
- each is a 4D-tensor, the channel number is num_points * 1.
- """
- return multi_apply(self.forward_single, feats, self.scales,
- self.strides)
-
- def forward_single(self, x, scale, stride):
- """Forward features of a single scale level.
-
- Args:
- x (Tensor): FPN feature maps of the specified stride.
- scale (:obj: `mmcv.cnn.Scale`): Learnable scale module to resize
- the bbox prediction.
- stride (int): The corresponding stride for feature maps, only
- used to normalize the bbox prediction when self.norm_on_bbox
- is True.
-
- Returns:
- tuple: scores for each class, bbox predictions and centerness \
- predictions of input feature maps.
- """
- cls_score, bbox_pred, cls_feat, reg_feat = super().forward_single(x)
- if self.centerness_on_reg:
- centerness = self.conv_centerness(reg_feat)
- else:
- centerness = self.conv_centerness(cls_feat)
- # scale the bbox_pred of different level
- # float to avoid overflow when enabling FP16
- bbox_pred = scale(bbox_pred).float()
- if self.norm_on_bbox:
- bbox_pred = F.relu(bbox_pred)
- if not self.training:
- bbox_pred *= stride
- else:
- bbox_pred = bbox_pred.exp()
- return cls_score, bbox_pred, centerness
-
- @force_fp32(apply_to=('cls_scores', 'bbox_preds', 'centernesses'))
- def loss(self,
- cls_scores,
- bbox_preds,
- centernesses,
- gt_bboxes,
- gt_labels,
- img_metas,
- gt_bboxes_ignore=None):
- """Compute loss of the head.
-
- Args:
- cls_scores (list[Tensor]): Box scores for each scale level,
- each is a 4D-tensor, the channel number is
- num_points * num_classes.
- bbox_preds (list[Tensor]): Box energies / deltas for each scale
- level, each is a 4D-tensor, the channel number is
- num_points * 4.
- centernesses (list[Tensor]): centerness for each scale level, each
- is a 4D-tensor, the channel number is num_points * 1.
- gt_bboxes (list[Tensor]): Ground truth bboxes for each image with
- shape (num_gts, 4) in [tl_x, tl_y, br_x, br_y] format.
- gt_labels (list[Tensor]): class indices corresponding to each box
- img_metas (list[dict]): Meta information of each image, e.g.,
- image size, scaling factor, etc.
- gt_bboxes_ignore (None | list[Tensor]): specify which bounding
- boxes can be ignored when computing the loss.
-
- Returns:
- dict[str, Tensor]: A dictionary of loss components.
- """
- assert len(cls_scores) == len(bbox_preds) == len(centernesses)
- featmap_sizes = [featmap.size()[-2:] for featmap in cls_scores]
- all_level_points = self.get_points(featmap_sizes, bbox_preds[0].dtype,
- bbox_preds[0].device)
- labels, bbox_targets = self.get_targets(all_level_points, gt_bboxes,
- gt_labels)
-
- num_imgs = cls_scores[0].size(0)
- # flatten cls_scores, bbox_preds and centerness
- flatten_cls_scores = [
- cls_score.permute(0, 2, 3, 1).reshape(-1, self.cls_out_channels)
- for cls_score in cls_scores
- ]
- flatten_bbox_preds = [
- bbox_pred.permute(0, 2, 3, 1).reshape(-1, 4)
- for bbox_pred in bbox_preds
- ]
- flatten_centerness = [
- centerness.permute(0, 2, 3, 1).reshape(-1)
- for centerness in centernesses
- ]
- flatten_cls_scores = torch.cat(flatten_cls_scores)
- flatten_bbox_preds = torch.cat(flatten_bbox_preds)
- flatten_centerness = torch.cat(flatten_centerness)
- flatten_labels = torch.cat(labels)
- flatten_bbox_targets = torch.cat(bbox_targets)
- # repeat points to align with bbox_preds
- flatten_points = torch.cat(
- [points.repeat(num_imgs, 1) for points in all_level_points])
-
- # FG cat_id: [0, num_classes -1], BG cat_id: num_classes
- bg_class_ind = self.num_classes
- pos_inds = ((flatten_labels >= 0)
- & (flatten_labels < bg_class_ind)).nonzero().reshape(-1)
- num_pos = torch.tensor(
- len(pos_inds), dtype=torch.float, device=bbox_preds[0].device)
- num_pos = max(reduce_mean(num_pos), 1.0)
- loss_cls = self.loss_cls(
- flatten_cls_scores, flatten_labels, avg_factor=num_pos)
-
- pos_bbox_preds = flatten_bbox_preds[pos_inds]
- pos_centerness = flatten_centerness[pos_inds]
-
- if len(pos_inds) > 0:
- pos_bbox_targets = flatten_bbox_targets[pos_inds]
- pos_centerness_targets = self.centerness_target(pos_bbox_targets)
- pos_points = flatten_points[pos_inds]
- pos_decoded_bbox_preds = distance2bbox(pos_points, pos_bbox_preds)
- pos_decoded_target_preds = distance2bbox(pos_points,
- pos_bbox_targets)
- # centerness weighted iou loss
- centerness_denorm = max(
- reduce_mean(pos_centerness_targets.sum().detach()), 1e-6)
- loss_bbox = self.loss_bbox(
- pos_decoded_bbox_preds,
- pos_decoded_target_preds,
- weight=pos_centerness_targets,
- avg_factor=centerness_denorm)
- loss_centerness = self.loss_centerness(
- pos_centerness, pos_centerness_targets, avg_factor=num_pos)
- else:
- loss_bbox = pos_bbox_preds.sum()
- loss_centerness = pos_centerness.sum()
-
- return dict(
- loss_cls=loss_cls,
- loss_bbox=loss_bbox,
- loss_centerness=loss_centerness)
-
- @force_fp32(apply_to=('cls_scores', 'bbox_preds', 'centernesses'))
- def get_bboxes(self,
- cls_scores,
- bbox_preds,
- centernesses,
- img_metas,
- cfg=None,
- rescale=False,
- with_nms=True):
- """Transform network output for a batch into bbox predictions.
-
- Args:
- cls_scores (list[Tensor]): Box scores for each scale level
- with shape (N, num_points * num_classes, H, W).
- bbox_preds (list[Tensor]): Box energies / deltas for each scale
- level with shape (N, num_points * 4, H, W).
- centernesses (list[Tensor]): Centerness for each scale level with
- shape (N, num_points * 1, H, W).
- img_metas (list[dict]): Meta information of each image, e.g.,
- image size, scaling factor, etc.
- cfg (mmcv.Config | None): Test / postprocessing configuration,
- if None, test_cfg would be used. Default: None.
- rescale (bool): If True, return boxes in original image space.
- Default: False.
- with_nms (bool): If True, do nms before return boxes.
- Default: True.
-
- Returns:
- list[tuple[Tensor, Tensor]]: Each item in result_list is 2-tuple.
- The first item is an (n, 5) tensor, where 5 represent
- (tl_x, tl_y, br_x, br_y, score) and the score between 0 and 1.
- The shape of the second tensor in the tuple is (n,), and
- each element represents the class label of the corresponding
- box.
- """
- assert len(cls_scores) == len(bbox_preds)
- num_levels = len(cls_scores)
-
- featmap_sizes = [featmap.size()[-2:] for featmap in cls_scores]
- mlvl_points = self.get_points(featmap_sizes, bbox_preds[0].dtype,
- bbox_preds[0].device)
-
- cls_score_list = [cls_scores[i].detach() for i in range(num_levels)]
- bbox_pred_list = [bbox_preds[i].detach() for i in range(num_levels)]
- centerness_pred_list = [
- centernesses[i].detach() for i in range(num_levels)
- ]
- if torch.onnx.is_in_onnx_export():
- assert len(
- img_metas
- ) == 1, 'Only support one input image while in exporting to ONNX'
- img_shapes = img_metas[0]['img_shape_for_onnx']
- else:
- img_shapes = [
- img_metas[i]['img_shape']
- for i in range(cls_scores[0].shape[0])
- ]
- scale_factors = [
- img_metas[i]['scale_factor'] for i in range(cls_scores[0].shape[0])
- ]
- result_list = self._get_bboxes(cls_score_list, bbox_pred_list,
- centerness_pred_list, mlvl_points,
- img_shapes, scale_factors, cfg, rescale,
- with_nms)
- return result_list
-
- def _get_bboxes(self,
- cls_scores,
- bbox_preds,
- centernesses,
- mlvl_points,
- img_shapes,
- scale_factors,
- cfg,
- rescale=False,
- with_nms=True):
- """Transform outputs for a single batch item into bbox predictions.
-
- Args:
- cls_scores (list[Tensor]): Box scores for a single scale level
- with shape (N, num_points * num_classes, H, W).
- bbox_preds (list[Tensor]): Box energies / deltas for a single scale
- level with shape (N, num_points * 4, H, W).
- centernesses (list[Tensor]): Centerness for a single scale level
- with shape (N, num_points * 4, H, W).
- mlvl_points (list[Tensor]): Box reference for a single scale level
- with shape (num_total_points, 4).
- img_shapes (list[tuple[int]]): Shape of the input image,
- list[(height, width, 3)].
- scale_factors (list[ndarray]): Scale factor of the image arrange as
- (w_scale, h_scale, w_scale, h_scale).
- cfg (mmcv.Config | None): Test / postprocessing configuration,
- if None, test_cfg would be used.
- rescale (bool): If True, return boxes in original image space.
- Default: False.
- with_nms (bool): If True, do nms before return boxes.
- Default: True.
-
- Returns:
- tuple(Tensor):
- det_bboxes (Tensor): BBox predictions in shape (n, 5), where
- the first 4 columns are bounding box positions
- (tl_x, tl_y, br_x, br_y) and the 5-th column is a score
- between 0 and 1.
- det_labels (Tensor): A (n,) tensor where each item is the
- predicted class label of the corresponding box.
- """
- cfg = self.test_cfg if cfg is None else cfg
- assert len(cls_scores) == len(bbox_preds) == len(mlvl_points)
- device = cls_scores[0].device
- batch_size = cls_scores[0].shape[0]
- # convert to tensor to keep tracing
- nms_pre_tensor = torch.tensor(
- cfg.get('nms_pre', -1), device=device, dtype=torch.long)
- mlvl_bboxes = []
- mlvl_scores = []
- mlvl_centerness = []
- for cls_score, bbox_pred, centerness, points in zip(
- cls_scores, bbox_preds, centernesses, mlvl_points):
- assert cls_score.size()[-2:] == bbox_pred.size()[-2:]
- scores = cls_score.permute(0, 2, 3, 1).reshape(
- batch_size, -1, self.cls_out_channels).sigmoid()
- centerness = centerness.permute(0, 2, 3,
- 1).reshape(batch_size,
- -1).sigmoid()
-
- bbox_pred = bbox_pred.permute(0, 2, 3,
- 1).reshape(batch_size, -1, 4)
- # Always keep topk op for dynamic input in onnx
- if nms_pre_tensor > 0 and (torch.onnx.is_in_onnx_export()
- or scores.shape[-2] > nms_pre_tensor):
- from torch import _shape_as_tensor
- # keep shape as tensor and get k
- num_anchor = _shape_as_tensor(scores)[-2].to(device)
- nms_pre = torch.where(nms_pre_tensor < num_anchor,
- nms_pre_tensor, num_anchor)
-
- max_scores, _ = (scores * centerness[..., None]).max(-1)
- _, topk_inds = max_scores.topk(nms_pre)
- points = points[topk_inds, :]
- batch_inds = torch.arange(batch_size).view(
- -1, 1).expand_as(topk_inds).long()
- bbox_pred = bbox_pred[batch_inds, topk_inds, :]
- scores = scores[batch_inds, topk_inds, :]
- centerness = centerness[batch_inds, topk_inds]
-
- bboxes = distance2bbox(points, bbox_pred, max_shape=img_shapes)
- mlvl_bboxes.append(bboxes)
- mlvl_scores.append(scores)
- mlvl_centerness.append(centerness)
-
- batch_mlvl_bboxes = torch.cat(mlvl_bboxes, dim=1)
- if rescale:
- batch_mlvl_bboxes /= batch_mlvl_bboxes.new_tensor(
- scale_factors).unsqueeze(1)
- batch_mlvl_scores = torch.cat(mlvl_scores, dim=1)
- batch_mlvl_centerness = torch.cat(mlvl_centerness, dim=1)
-
- # Set max number of box to be feed into nms in deployment
- deploy_nms_pre = cfg.get('deploy_nms_pre', -1)
- if deploy_nms_pre > 0 and torch.onnx.is_in_onnx_export():
- batch_mlvl_scores, _ = (
- batch_mlvl_scores *
- batch_mlvl_centerness.unsqueeze(2).expand_as(batch_mlvl_scores)
- ).max(-1)
- _, topk_inds = batch_mlvl_scores.topk(deploy_nms_pre)
- batch_inds = torch.arange(batch_mlvl_scores.shape[0]).view(
- -1, 1).expand_as(topk_inds)
- batch_mlvl_scores = batch_mlvl_scores[batch_inds, topk_inds, :]
- batch_mlvl_bboxes = batch_mlvl_bboxes[batch_inds, topk_inds, :]
- batch_mlvl_centerness = batch_mlvl_centerness[batch_inds,
- topk_inds]
-
- # remind that we set FG labels to [0, num_class-1] since mmdet v2.0
- # BG cat_id: num_class
- padding = batch_mlvl_scores.new_zeros(batch_size,
- batch_mlvl_scores.shape[1], 1)
- batch_mlvl_scores = torch.cat([batch_mlvl_scores, padding], dim=-1)
-
- if with_nms:
- det_results = []
- for (mlvl_bboxes, mlvl_scores,
- mlvl_centerness) in zip(batch_mlvl_bboxes, batch_mlvl_scores,
- batch_mlvl_centerness):
- det_bbox, det_label = multiclass_nms(
- mlvl_bboxes,
- mlvl_scores,
- cfg.score_thr,
- cfg.nms,
- cfg.max_per_img,
- score_factors=mlvl_centerness)
- det_results.append(tuple([det_bbox, det_label]))
- else:
- det_results = [
- tuple(mlvl_bs)
- for mlvl_bs in zip(batch_mlvl_bboxes, batch_mlvl_scores,
- batch_mlvl_centerness)
- ]
- return det_results
-
- def _get_points_single(self,
- featmap_size,
- stride,
- dtype,
- device,
- flatten=False):
- """Get points according to feature map sizes."""
- y, x = super()._get_points_single(featmap_size, stride, dtype, device)
- points = torch.stack((x.reshape(-1) * stride, y.reshape(-1) * stride),
- dim=-1) + stride // 2
- return points
-
- def get_targets(self, points, gt_bboxes_list, gt_labels_list):
- """Compute regression, classification and centerness targets for points
- in multiple images.
-
- Args:
- points (list[Tensor]): Points of each fpn level, each has shape
- (num_points, 2).
- gt_bboxes_list (list[Tensor]): Ground truth bboxes of each image,
- each has shape (num_gt, 4).
- gt_labels_list (list[Tensor]): Ground truth labels of each box,
- each has shape (num_gt,).
-
- Returns:
- tuple:
- concat_lvl_labels (list[Tensor]): Labels of each level. \
- concat_lvl_bbox_targets (list[Tensor]): BBox targets of each \
- level.
- """
- assert len(points) == len(self.regress_ranges)
- num_levels = len(points)
- # expand regress ranges to align with points
- expanded_regress_ranges = [
- points[i].new_tensor(self.regress_ranges[i])[None].expand_as(
- points[i]) for i in range(num_levels)
- ]
- # concat all levels points and regress ranges
- concat_regress_ranges = torch.cat(expanded_regress_ranges, dim=0)
- concat_points = torch.cat(points, dim=0)
-
- # the number of points per img, per lvl
- num_points = [center.size(0) for center in points]
-
- # get labels and bbox_targets of each image
- labels_list, bbox_targets_list = multi_apply(
- self._get_target_single,
- gt_bboxes_list,
- gt_labels_list,
- points=concat_points,
- regress_ranges=concat_regress_ranges,
- num_points_per_lvl=num_points)
-
- # split to per img, per level
- labels_list = [labels.split(num_points, 0) for labels in labels_list]
- bbox_targets_list = [
- bbox_targets.split(num_points, 0)
- for bbox_targets in bbox_targets_list
- ]
-
- # concat per level image
- concat_lvl_labels = []
- concat_lvl_bbox_targets = []
- for i in range(num_levels):
- concat_lvl_labels.append(
- torch.cat([labels[i] for labels in labels_list]))
- bbox_targets = torch.cat(
- [bbox_targets[i] for bbox_targets in bbox_targets_list])
- if self.norm_on_bbox:
- bbox_targets = bbox_targets / self.strides[i]
- concat_lvl_bbox_targets.append(bbox_targets)
- return concat_lvl_labels, concat_lvl_bbox_targets
-
- def _get_target_single(self, gt_bboxes, gt_labels, points, regress_ranges,
- num_points_per_lvl):
- """Compute regression and classification targets for a single image."""
- num_points = points.size(0)
- num_gts = gt_labels.size(0)
- if num_gts == 0:
- return gt_labels.new_full((num_points,), self.num_classes), \
- gt_bboxes.new_zeros((num_points, 4))
-
- areas = (gt_bboxes[:, 2] - gt_bboxes[:, 0]) * (
- gt_bboxes[:, 3] - gt_bboxes[:, 1])
- # TODO: figure out why these two are different
- # areas = areas[None].expand(num_points, num_gts)
- areas = areas[None].repeat(num_points, 1)
- regress_ranges = regress_ranges[:, None, :].expand(
- num_points, num_gts, 2)
- gt_bboxes = gt_bboxes[None].expand(num_points, num_gts, 4)
- xs, ys = points[:, 0], points[:, 1]
- xs = xs[:, None].expand(num_points, num_gts)
- ys = ys[:, None].expand(num_points, num_gts)
-
- left = xs - gt_bboxes[..., 0]
- right = gt_bboxes[..., 2] - xs
- top = ys - gt_bboxes[..., 1]
- bottom = gt_bboxes[..., 3] - ys
- bbox_targets = torch.stack((left, top, right, bottom), -1)
-
- if self.center_sampling:
- # condition1: inside a `center bbox`
- radius = self.center_sample_radius
- center_xs = (gt_bboxes[..., 0] + gt_bboxes[..., 2]) / 2
- center_ys = (gt_bboxes[..., 1] + gt_bboxes[..., 3]) / 2
- center_gts = torch.zeros_like(gt_bboxes)
- stride = center_xs.new_zeros(center_xs.shape)
-
- # project the points on current lvl back to the `original` sizes
- lvl_begin = 0
- for lvl_idx, num_points_lvl in enumerate(num_points_per_lvl):
- lvl_end = lvl_begin + num_points_lvl
- stride[lvl_begin:lvl_end] = self.strides[lvl_idx] * radius
- lvl_begin = lvl_end
-
- x_mins = center_xs - stride
- y_mins = center_ys - stride
- x_maxs = center_xs + stride
- y_maxs = center_ys + stride
- center_gts[..., 0] = torch.where(x_mins > gt_bboxes[..., 0],
- x_mins, gt_bboxes[..., 0])
- center_gts[..., 1] = torch.where(y_mins > gt_bboxes[..., 1],
- y_mins, gt_bboxes[..., 1])
- center_gts[..., 2] = torch.where(x_maxs > gt_bboxes[..., 2],
- gt_bboxes[..., 2], x_maxs)
- center_gts[..., 3] = torch.where(y_maxs > gt_bboxes[..., 3],
- gt_bboxes[..., 3], y_maxs)
-
- cb_dist_left = xs - center_gts[..., 0]
- cb_dist_right = center_gts[..., 2] - xs
- cb_dist_top = ys - center_gts[..., 1]
- cb_dist_bottom = center_gts[..., 3] - ys
- center_bbox = torch.stack(
- (cb_dist_left, cb_dist_top, cb_dist_right, cb_dist_bottom), -1)
- inside_gt_bbox_mask = center_bbox.min(-1)[0] > 0
- else:
- # condition1: inside a gt bbox
- inside_gt_bbox_mask = bbox_targets.min(-1)[0] > 0
-
- # condition2: limit the regression range for each location
- max_regress_distance = bbox_targets.max(-1)[0]
- inside_regress_range = (
- (max_regress_distance >= regress_ranges[..., 0])
- & (max_regress_distance <= regress_ranges[..., 1]))
-
- # if there are still more than one objects for a location,
- # we choose the one with minimal area
- areas[inside_gt_bbox_mask == 0] = INF
- areas[inside_regress_range == 0] = INF
- min_area, min_area_inds = areas.min(dim=1)
-
- labels = gt_labels[min_area_inds]
- labels[min_area == INF] = self.num_classes # set as BG
- bbox_targets = bbox_targets[range(num_points), min_area_inds]
-
- return labels, bbox_targets
-
- def centerness_target(self, pos_bbox_targets):
- """Compute centerness targets.
-
- Args:
- pos_bbox_targets (Tensor): BBox targets of positive bboxes in shape
- (num_pos, 4)
-
- Returns:
- Tensor: Centerness target.
- """
- # only calculate pos centerness targets, otherwise there may be nan
- left_right = pos_bbox_targets[:, [0, 2]]
- top_bottom = pos_bbox_targets[:, [1, 3]]
- centerness_targets = (
- left_right.min(dim=-1)[0] / left_right.max(dim=-1)[0]) * (
- top_bottom.min(dim=-1)[0] / top_bottom.max(dim=-1)[0])
- return torch.sqrt(centerness_targets)
diff --git a/spaces/dinhminh20521597/OCR_DEMO/configs/_base_/recog_pipelines/nrtr_pipeline.py b/spaces/dinhminh20521597/OCR_DEMO/configs/_base_/recog_pipelines/nrtr_pipeline.py
deleted file mode 100644
index 71a19804309aa6692970b5eef642eddf87770559..0000000000000000000000000000000000000000
--- a/spaces/dinhminh20521597/OCR_DEMO/configs/_base_/recog_pipelines/nrtr_pipeline.py
+++ /dev/null
@@ -1,38 +0,0 @@
-img_norm_cfg = dict(mean=[0.485, 0.456, 0.406], std=[0.229, 0.224, 0.225])
-train_pipeline = [
- dict(type='LoadImageFromFile'),
- dict(
- type='ResizeOCR',
- height=32,
- min_width=32,
- max_width=160,
- keep_aspect_ratio=True,
- width_downsample_ratio=0.25),
- dict(type='ToTensorOCR'),
- dict(type='NormalizeOCR', **img_norm_cfg),
- dict(
- type='Collect',
- keys=['img'],
- meta_keys=[
- 'filename', 'ori_shape', 'resize_shape', 'text', 'valid_ratio'
- ]),
-]
-
-test_pipeline = [
- dict(type='LoadImageFromFile'),
- dict(
- type='ResizeOCR',
- height=32,
- min_width=32,
- max_width=160,
- keep_aspect_ratio=True),
- dict(type='ToTensorOCR'),
- dict(type='NormalizeOCR', **img_norm_cfg),
- dict(
- type='Collect',
- keys=['img'],
- meta_keys=[
- 'filename', 'ori_shape', 'resize_shape', 'valid_ratio',
- 'img_norm_cfg', 'ori_filename', 'img_shape'
- ])
-]
diff --git a/spaces/dorkai/singpt-2.0/server.py b/spaces/dorkai/singpt-2.0/server.py
deleted file mode 100644
index 6a17f26287d94e9187a4f315fe9fb7d2dc6ec171..0000000000000000000000000000000000000000
--- a/spaces/dorkai/singpt-2.0/server.py
+++ /dev/null
@@ -1,382 +0,0 @@
-import gc
-import io
-import json
-import re
-import sys
-import time
-import zipfile
-from pathlib import Path
-
-import gradio as gr
-import torch
-
-import modules.chat as chat
-import modules.extensions as extensions_module
-import modules.shared as shared
-import modules.ui as ui
-from modules.html_generator import generate_chat_html
-from modules.models import load_model, load_soft_prompt
-from modules.text_generation import generate_reply
-
-# Loading custom settings
-settings_file = None
-if shared.args.settings is not None and Path(shared.args.settings).exists():
- settings_file = Path(shared.args.settings)
-elif Path('settings.json').exists():
- settings_file = Path('settings.json')
-if settings_file is not None:
- print(f"Loading settings from {settings_file}...")
- new_settings = json.loads(open(settings_file, 'r').read())
- for item in new_settings:
- shared.settings[item] = new_settings[item]
-
-def get_available_models():
- if shared.args.flexgen:
- return sorted([re.sub('-np$', '', item.name) for item in list(Path('models/').glob('*')) if item.name.endswith('-np')], key=str.lower)
- else:
- return sorted([item.name for item in list(Path('models/').glob('*')) if not item.name.endswith(('.txt', '-np', '.pt'))], key=str.lower)
-
-def get_available_presets():
- return sorted(set(map(lambda x : '.'.join(str(x.name).split('.')[:-1]), Path('presets').glob('*.txt'))), key=str.lower)
-
-def get_available_characters():
- return ['None'] + sorted(set(map(lambda x : '.'.join(str(x.name).split('.')[:-1]), Path('characters').glob('*.json'))), key=str.lower)
-
-def get_available_extensions():
- return sorted(set(map(lambda x : x.parts[1], Path('extensions').glob('*/script.py'))), key=str.lower)
-
-def get_available_softprompts():
- return ['None'] + sorted(set(map(lambda x : '.'.join(str(x.name).split('.')[:-1]), Path('softprompts').glob('*.zip'))), key=str.lower)
-
-def load_model_wrapper(selected_model):
- if selected_model != shared.model_name:
- shared.model_name = selected_model
- shared.model = shared.tokenizer = None
- if not shared.args.cpu:
- gc.collect()
- torch.cuda.empty_cache()
- shared.model, shared.tokenizer = load_model(shared.model_name)
-
- return selected_model
-
-def load_preset_values(preset_menu, return_dict=False):
- generate_params = {
- 'do_sample': True,
- 'temperature': 1,
- 'top_p': 1,
- 'typical_p': 1,
- 'repetition_penalty': 1,
- 'top_k': 50,
- 'num_beams': 1,
- 'penalty_alpha': 0,
- 'min_length': 0,
- 'length_penalty': 1,
- 'no_repeat_ngram_size': 0,
- 'early_stopping': False,
- }
- with open(Path(f'presets/{preset_menu}.txt'), 'r') as infile:
- preset = infile.read()
- for i in preset.splitlines():
- i = i.rstrip(',').strip().split('=')
- if len(i) == 2 and i[0].strip() != 'tokens':
- generate_params[i[0].strip()] = eval(i[1].strip())
-
- generate_params['temperature'] = min(1.99, generate_params['temperature'])
-
- if return_dict:
- return generate_params
- else:
- return generate_params['do_sample'], generate_params['temperature'], generate_params['top_p'], generate_params['typical_p'], generate_params['repetition_penalty'], generate_params['top_k'], generate_params['min_length'], generate_params['no_repeat_ngram_size'], generate_params['num_beams'], generate_params['penalty_alpha'], generate_params['length_penalty'], generate_params['early_stopping']
-
-def upload_soft_prompt(file):
- with zipfile.ZipFile(io.BytesIO(file)) as zf:
- zf.extract('meta.json')
- j = json.loads(open('meta.json', 'r').read())
- name = j['name']
- Path('meta.json').unlink()
-
- with open(Path(f'softprompts/{name}.zip'), 'wb') as f:
- f.write(file)
-
- return name
-
-def create_settings_menus(default_preset):
- generate_params = load_preset_values(default_preset if not shared.args.flexgen else 'Naive', return_dict=True)
-
- with gr.Row():
- with gr.Column():
- with gr.Row():
- shared.gradio['model_menu'] = gr.Dropdown(choices=available_models, value=shared.model_name, label='Model')
- ui.create_refresh_button(shared.gradio['model_menu'], lambda : None, lambda : {'choices': get_available_models()}, 'refresh-button')
- with gr.Column():
- with gr.Row():
- shared.gradio['preset_menu'] = gr.Dropdown(choices=available_presets, value=default_preset if not shared.args.flexgen else 'Naive', label='Generation parameters preset')
- ui.create_refresh_button(shared.gradio['preset_menu'], lambda : None, lambda : {'choices': get_available_presets()}, 'refresh-button')
-
- with gr.Accordion('Custom generation parameters', open=False, elem_id='accordion'):
- with gr.Row():
- with gr.Column():
- shared.gradio['temperature'] = gr.Slider(0.01, 1.99, value=generate_params['temperature'], step=0.01, label='temperature')
- shared.gradio['repetition_penalty'] = gr.Slider(1.0, 2.99, value=generate_params['repetition_penalty'],step=0.01,label='repetition_penalty')
- shared.gradio['top_k'] = gr.Slider(0,200,value=generate_params['top_k'],step=1,label='top_k')
- shared.gradio['top_p'] = gr.Slider(0.0,1.0,value=generate_params['top_p'],step=0.01,label='top_p')
- with gr.Column():
- shared.gradio['do_sample'] = gr.Checkbox(value=generate_params['do_sample'], label='do_sample')
- shared.gradio['typical_p'] = gr.Slider(0.0,1.0,value=generate_params['typical_p'],step=0.01,label='typical_p')
- shared.gradio['no_repeat_ngram_size'] = gr.Slider(0, 20, step=1, value=generate_params['no_repeat_ngram_size'], label='no_repeat_ngram_size')
- shared.gradio['min_length'] = gr.Slider(0, 2000, step=1, value=generate_params['min_length'] if shared.args.no_stream else 0, label='min_length', interactive=shared.args.no_stream)
-
- gr.Markdown('Contrastive search:')
- shared.gradio['penalty_alpha'] = gr.Slider(0, 5, value=generate_params['penalty_alpha'], label='penalty_alpha')
-
- gr.Markdown('Beam search (uses a lot of VRAM):')
- with gr.Row():
- with gr.Column():
- shared.gradio['num_beams'] = gr.Slider(1, 20, step=1, value=generate_params['num_beams'], label='num_beams')
- with gr.Column():
- shared.gradio['length_penalty'] = gr.Slider(-5, 5, value=generate_params['length_penalty'], label='length_penalty')
- shared.gradio['early_stopping'] = gr.Checkbox(value=generate_params['early_stopping'], label='early_stopping')
-
- with gr.Accordion('Soft prompt', open=False, elem_id='accordion'):
- with gr.Row():
- shared.gradio['softprompts_menu'] = gr.Dropdown(choices=available_softprompts, value='None', label='Soft prompt')
- ui.create_refresh_button(shared.gradio['softprompts_menu'], lambda : None, lambda : {'choices': get_available_softprompts()}, 'refresh-button')
-
- gr.Markdown('Upload a soft prompt (.zip format):')
- with gr.Row():
- shared.gradio['upload_softprompt'] = gr.File(type='binary', file_types=['.zip'])
-
- shared.gradio['model_menu'].change(load_model_wrapper, [shared.gradio['model_menu']], [shared.gradio['model_menu']], show_progress=True)
- shared.gradio['preset_menu'].change(load_preset_values, [shared.gradio['preset_menu']], [shared.gradio['do_sample'], shared.gradio['temperature'], shared.gradio['top_p'], shared.gradio['typical_p'], shared.gradio['repetition_penalty'], shared.gradio['top_k'], shared.gradio['min_length'], shared.gradio['no_repeat_ngram_size'], shared.gradio['num_beams'], shared.gradio['penalty_alpha'], shared.gradio['length_penalty'], shared.gradio['early_stopping']])
- shared.gradio['softprompts_menu'].change(load_soft_prompt, [shared.gradio['softprompts_menu']], [shared.gradio['softprompts_menu']], show_progress=True)
- shared.gradio['upload_softprompt'].upload(upload_soft_prompt, [shared.gradio['upload_softprompt']], [shared.gradio['softprompts_menu']])
-
-available_models = get_available_models()
-available_presets = get_available_presets()
-available_characters = get_available_characters()
-available_softprompts = get_available_softprompts()
-
-# Default extensions
-extensions_module.available_extensions = get_available_extensions()
-if shared.args.chat or shared.args.cai_chat:
- for extension in shared.settings['chat_default_extensions']:
- shared.args.extensions = shared.args.extensions or []
- if extension not in shared.args.extensions:
- shared.args.extensions.append(extension)
-else:
- for extension in shared.settings['default_extensions']:
- shared.args.extensions = shared.args.extensions or []
- if extension not in shared.args.extensions:
- shared.args.extensions.append(extension)
-if shared.args.extensions is not None and len(shared.args.extensions) > 0:
- extensions_module.load_extensions()
-
-# Default model
-if shared.args.model is not None:
- shared.model_name = shared.args.model
-else:
- if len(available_models) == 0:
- print('No models are available! Please download at least one.')
- sys.exit(0)
- elif len(available_models) == 1:
- i = 0
- else:
- print('The following models are available:\n')
- for i, model in enumerate(available_models):
- print(f'{i+1}. {model}')
- print(f'\nWhich one do you want to load? 1-{len(available_models)}\n')
- i = int(input())-1
- print()
- shared.model_name = available_models[i]
-shared.model, shared.tokenizer = load_model(shared.model_name)
-
-# Default UI settings
-gen_events = []
-default_preset = shared.settings['presets'][next((k for k in shared.settings['presets'] if re.match(k.lower(), shared.model_name.lower())), 'default')]
-default_text = shared.settings['prompts'][next((k for k in shared.settings['prompts'] if re.match(k.lower(), shared.model_name.lower())), 'default')]
-title ='Text generation web UI'
-description = '\n\n# Text generation lab\nGenerate text using Large Language Models.\n'
-suffix = '_pygmalion' if 'pygmalion' in shared.model_name.lower() else ''
-
-if shared.args.chat or shared.args.cai_chat:
- with gr.Blocks(css=ui.css+ui.chat_css, analytics_enabled=False, title=title) as shared.gradio['interface']:
- gr.HTML('''Original github repo
-For faster inference without waiting in queue, you may duplicate the space. 
-(👇 Scroll down to see the interface 👀)''')
- if shared.args.cai_chat:
- shared.gradio['display'] = gr.HTML(value=generate_chat_html(shared.history['visible'], shared.settings[f'name1{suffix}'], shared.settings[f'name2{suffix}'], shared.character))
- else:
- shared.gradio['display'] = gr.Chatbot(value=shared.history['visible']).style(color_map=("#326efd", "#212528"))
- shared.gradio['textbox'] = gr.Textbox(label='Input')
- with gr.Row():
- shared.gradio['Stop'] = gr.Button('Stop')
- shared.gradio['Generate'] = gr.Button('Generate')
- with gr.Row():
- shared.gradio['Impersonate'] = gr.Button('Impersonate')
- shared.gradio['Regenerate'] = gr.Button('Regenerate')
- with gr.Row():
- shared.gradio['Copy last reply'] = gr.Button('Copy last reply')
- shared.gradio['Replace last reply'] = gr.Button('Replace last reply')
- shared.gradio['Remove last'] = gr.Button('Remove last')
-
- shared.gradio['Clear history'] = gr.Button('Clear history')
- shared.gradio['Clear history-confirm'] = gr.Button('Confirm', variant="stop", visible=False)
- shared.gradio['Clear history-cancel'] = gr.Button('Cancel', visible=False)
- with gr.Tab('Chat settings'):
- shared.gradio['name1'] = gr.Textbox(value=shared.settings[f'name1{suffix}'], lines=1, label='Your name')
- shared.gradio['name2'] = gr.Textbox(value=shared.settings[f'name2{suffix}'], lines=1, label='Bot\'s name')
- shared.gradio['context'] = gr.Textbox(value=shared.settings[f'context{suffix}'], lines=5, label='Context')
- with gr.Row():
- shared.gradio['character_menu'] = gr.Dropdown(choices=available_characters, value='None', label='Character', elem_id='character-menu')
- ui.create_refresh_button(shared.gradio['character_menu'], lambda : None, lambda : {'choices': get_available_characters()}, 'refresh-button')
-
- with gr.Row():
- shared.gradio['check'] = gr.Checkbox(value=shared.settings[f'stop_at_newline{suffix}'], label='Stop generating at new line character?')
- with gr.Row():
- with gr.Tab('Chat history'):
- with gr.Row():
- with gr.Column():
- gr.Markdown('Upload')
- shared.gradio['upload_chat_history'] = gr.File(type='binary', file_types=['.json', '.txt'])
- with gr.Column():
- gr.Markdown('Download')
- shared.gradio['download'] = gr.File()
- shared.gradio['download_button'] = gr.Button(value='Click me')
- with gr.Tab('Upload character'):
- with gr.Row():
- with gr.Column():
- gr.Markdown('1. Select the JSON file')
- shared.gradio['upload_json'] = gr.File(type='binary', file_types=['.json'])
- with gr.Column():
- gr.Markdown('2. Select your character\'s profile picture (optional)')
- shared.gradio['upload_img_bot'] = gr.File(type='binary', file_types=['image'])
- shared.gradio['Upload character'] = gr.Button(value='Submit')
- with gr.Tab('Upload your profile picture'):
- shared.gradio['upload_img_me'] = gr.File(type='binary', file_types=['image'])
- with gr.Tab('Upload TavernAI Character Card'):
- shared.gradio['upload_img_tavern'] = gr.File(type='binary', file_types=['image'])
-
- with gr.Tab('Generation settings'):
- with gr.Row():
- with gr.Column():
- shared.gradio['max_new_tokens'] = gr.Slider(minimum=shared.settings['max_new_tokens_min'], maximum=shared.settings['max_new_tokens_max'], step=1, label='max_new_tokens', value=shared.settings['max_new_tokens'])
- with gr.Column():
- shared.gradio['chat_prompt_size_slider'] = gr.Slider(minimum=shared.settings['chat_prompt_size_min'], maximum=shared.settings['chat_prompt_size_max'], step=1, label='Maximum prompt size in tokens', value=shared.settings['chat_prompt_size'])
- shared.gradio['chat_generation_attempts'] = gr.Slider(minimum=shared.settings['chat_generation_attempts_min'], maximum=shared.settings['chat_generation_attempts_max'], value=shared.settings['chat_generation_attempts'], step=1, label='Generation attempts (for longer replies)')
- create_settings_menus(default_preset)
-
- shared.input_params = [shared.gradio[k] for k in ['textbox', 'max_new_tokens', 'do_sample', 'temperature', 'top_p', 'typical_p', 'repetition_penalty', 'top_k', 'min_length', 'no_repeat_ngram_size', 'num_beams', 'penalty_alpha', 'length_penalty', 'early_stopping', 'name1', 'name2', 'context', 'check', 'chat_prompt_size_slider', 'chat_generation_attempts']]
- if shared.args.extensions is not None:
- with gr.Tab('Extensions'):
- extensions_module.create_extensions_block()
-
- function_call = 'chat.cai_chatbot_wrapper' if shared.args.cai_chat else 'chat.chatbot_wrapper'
-
- gen_events.append(shared.gradio['Generate'].click(eval(function_call), shared.input_params, shared.gradio['display'], show_progress=shared.args.no_stream, api_name='textgen'))
- gen_events.append(shared.gradio['textbox'].submit(eval(function_call), shared.input_params, shared.gradio['display'], show_progress=shared.args.no_stream))
- gen_events.append(shared.gradio['Regenerate'].click(chat.regenerate_wrapper, shared.input_params, shared.gradio['display'], show_progress=shared.args.no_stream))
- gen_events.append(shared.gradio['Impersonate'].click(chat.impersonate_wrapper, shared.input_params, shared.gradio['textbox'], show_progress=shared.args.no_stream))
- shared.gradio['Stop'].click(chat.stop_everything_event, [], [], cancels=gen_events)
-
- shared.gradio['Copy last reply'].click(chat.send_last_reply_to_input, [], shared.gradio['textbox'], show_progress=shared.args.no_stream)
- shared.gradio['Replace last reply'].click(chat.replace_last_reply, [shared.gradio['textbox'], shared.gradio['name1'], shared.gradio['name2']], shared.gradio['display'], show_progress=shared.args.no_stream)
-
- # Clear history with confirmation
- clear_arr = [shared.gradio[k] for k in ['Clear history-confirm', 'Clear history', 'Clear history-cancel']]
- shared.gradio['Clear history'].click(lambda :[gr.update(visible=True), gr.update(visible=False), gr.update(visible=True)], None, clear_arr)
- shared.gradio['Clear history-confirm'].click(lambda :[gr.update(visible=False), gr.update(visible=True), gr.update(visible=False)], None, clear_arr)
- shared.gradio['Clear history-confirm'].click(chat.clear_chat_log, [shared.gradio['name1'], shared.gradio['name2']], shared.gradio['display'])
- shared.gradio['Clear history-cancel'].click(lambda :[gr.update(visible=False), gr.update(visible=True), gr.update(visible=False)], None, clear_arr)
-
- shared.gradio['Remove last'].click(chat.remove_last_message, [shared.gradio['name1'], shared.gradio['name2']], [shared.gradio['display'], shared.gradio['textbox']], show_progress=False)
- shared.gradio['download_button'].click(chat.save_history, inputs=[], outputs=[shared.gradio['download']])
- shared.gradio['Upload character'].click(chat.upload_character, [shared.gradio['upload_json'], shared.gradio['upload_img_bot']], [shared.gradio['character_menu']])
-
- # Clearing stuff and saving the history
- for i in ['Generate', 'Regenerate', 'Replace last reply']:
- shared.gradio[i].click(lambda x: '', shared.gradio['textbox'], shared.gradio['textbox'], show_progress=False)
- shared.gradio[i].click(lambda : chat.save_history(timestamp=False), [], [], show_progress=False)
- shared.gradio['Clear history-confirm'].click(lambda : chat.save_history(timestamp=False), [], [], show_progress=False)
- shared.gradio['textbox'].submit(lambda x: '', shared.gradio['textbox'], shared.gradio['textbox'], show_progress=False)
- shared.gradio['textbox'].submit(lambda : chat.save_history(timestamp=False), [], [], show_progress=False)
-
- shared.gradio['character_menu'].change(chat.load_character, [shared.gradio['character_menu'], shared.gradio['name1'], shared.gradio['name2']], [shared.gradio['name2'], shared.gradio['context'], shared.gradio['display']])
- shared.gradio['upload_chat_history'].upload(chat.load_history, [shared.gradio['upload_chat_history'], shared.gradio['name1'], shared.gradio['name2']], [])
- shared.gradio['upload_img_tavern'].upload(chat.upload_tavern_character, [shared.gradio['upload_img_tavern'], shared.gradio['name1'], shared.gradio['name2']], [shared.gradio['character_menu']])
- shared.gradio['upload_img_me'].upload(chat.upload_your_profile_picture, [shared.gradio['upload_img_me']], [])
-
- reload_func = chat.redraw_html if shared.args.cai_chat else lambda : shared.history['visible']
- reload_inputs = [shared.gradio['name1'], shared.gradio['name2']] if shared.args.cai_chat else []
- shared.gradio['upload_chat_history'].upload(reload_func, reload_inputs, [shared.gradio['display']])
- shared.gradio['upload_img_me'].upload(reload_func, reload_inputs, [shared.gradio['display']])
- shared.gradio['Stop'].click(reload_func, reload_inputs, [shared.gradio['display']])
-
- shared.gradio['interface'].load(lambda : chat.load_default_history(shared.settings[f'name1{suffix}'], shared.settings[f'name2{suffix}']), None, None)
- shared.gradio['interface'].load(reload_func, reload_inputs, [shared.gradio['display']], show_progress=True)
-
-elif shared.args.notebook:
- with gr.Blocks(css=ui.css, analytics_enabled=False, title=title) as shared.gradio['interface']:
- gr.Markdown(description)
- with gr.Tab('Raw'):
- shared.gradio['textbox'] = gr.Textbox(value=default_text, lines=23)
- with gr.Tab('Markdown'):
- shared.gradio['markdown'] = gr.Markdown()
- with gr.Tab('HTML'):
- shared.gradio['html'] = gr.HTML()
-
- shared.gradio['Generate'] = gr.Button('Generate')
- shared.gradio['Stop'] = gr.Button('Stop')
- shared.gradio['max_new_tokens'] = gr.Slider(minimum=shared.settings['max_new_tokens_min'], maximum=shared.settings['max_new_tokens_max'], step=1, label='max_new_tokens', value=shared.settings['max_new_tokens'])
-
- create_settings_menus(default_preset)
- if shared.args.extensions is not None:
- extensions_module.create_extensions_block()
-
- shared.input_params = [shared.gradio[k] for k in ['textbox', 'max_new_tokens', 'do_sample', 'temperature', 'top_p', 'typical_p', 'repetition_penalty', 'top_k', 'min_length', 'no_repeat_ngram_size', 'num_beams', 'penalty_alpha', 'length_penalty', 'early_stopping']]
- output_params = [shared.gradio[k] for k in ['textbox', 'markdown', 'html']]
- gen_events.append(shared.gradio['Generate'].click(generate_reply, shared.input_params, output_params, show_progress=shared.args.no_stream, api_name='textgen'))
- gen_events.append(shared.gradio['textbox'].submit(generate_reply, shared.input_params, output_params, show_progress=shared.args.no_stream))
- shared.gradio['Stop'].click(None, None, None, cancels=gen_events)
-
-else:
- with gr.Blocks(css=ui.css, analytics_enabled=False, title=title) as shared.gradio['interface']:
- gr.Markdown(description)
- with gr.Row():
- with gr.Column():
- shared.gradio['textbox'] = gr.Textbox(value=default_text, lines=15, label='Input')
- shared.gradio['max_new_tokens'] = gr.Slider(minimum=shared.settings['max_new_tokens_min'], maximum=shared.settings['max_new_tokens_max'], step=1, label='max_new_tokens', value=shared.settings['max_new_tokens'])
- shared.gradio['Generate'] = gr.Button('Generate')
- with gr.Row():
- with gr.Column():
- shared.gradio['Continue'] = gr.Button('Continue')
- with gr.Column():
- shared.gradio['Stop'] = gr.Button('Stop')
-
- create_settings_menus(default_preset)
- if shared.args.extensions is not None:
- extensions_module.create_extensions_block()
-
- with gr.Column():
- with gr.Tab('Raw'):
- shared.gradio['output_textbox'] = gr.Textbox(lines=15, label='Output')
- with gr.Tab('Markdown'):
- shared.gradio['markdown'] = gr.Markdown()
- with gr.Tab('HTML'):
- shared.gradio['html'] = gr.HTML()
-
- shared.input_params = [shared.gradio[k] for k in ['textbox', 'max_new_tokens', 'do_sample', 'temperature', 'top_p', 'typical_p', 'repetition_penalty', 'top_k', 'min_length', 'no_repeat_ngram_size', 'num_beams', 'penalty_alpha', 'length_penalty', 'early_stopping']]
- output_params = [shared.gradio[k] for k in ['output_textbox', 'markdown', 'html']]
- gen_events.append(shared.gradio['Generate'].click(generate_reply, shared.input_params, output_params, show_progress=shared.args.no_stream, api_name='textgen'))
- gen_events.append(shared.gradio['textbox'].submit(generate_reply, shared.input_params, output_params, show_progress=shared.args.no_stream))
- gen_events.append(shared.gradio['Continue'].click(generate_reply, [shared.gradio['output_textbox']] + shared.input_params[1:], output_params, show_progress=shared.args.no_stream))
- shared.gradio['Stop'].click(None, None, None, cancels=gen_events)
-
-shared.gradio['interface'].queue()
-if shared.args.listen:
- shared.gradio['interface'].launch(prevent_thread_lock=True, share=shared.args.share, server_name='0.0.0.0', server_port=shared.args.listen_port, inbrowser=shared.args.auto_launch)
-else:
- shared.gradio['interface'].launch(prevent_thread_lock=True, share=shared.args.share, server_port=shared.args.listen_port, inbrowser=shared.args.auto_launch)
-
-# I think that I will need this later
-while True:
- time.sleep(0.5)
diff --git a/spaces/dpe1/beat_manipulator/beat_manipulator/effects.py b/spaces/dpe1/beat_manipulator/beat_manipulator/effects.py
deleted file mode 100644
index 19ee796cf015987b28ee60d5931089be6a533178..0000000000000000000000000000000000000000
--- a/spaces/dpe1/beat_manipulator/beat_manipulator/effects.py
+++ /dev/null
@@ -1,84 +0,0 @@
-import numpy as np
-from . import io
-
-def deco_abs(effect):
- def stuff(*args, **kwargs):
- if len(args)>0: audio = args[0]
- else: audio = kwargs['audio']
- if not isinstance(audio, np.ndarray): audio = io._load(audio)
- audio_signs = np.sign(audio)
- audio = np.abs(audio)
- if len(args)>0: args[0] = audio
- else: kwargs['audio'] = audio
- audio = effect(*args, **kwargs)
- audio *= audio_signs
- return stuff
-
-
-
-def volume(audio: np.ndarray, v: float):
- return audio*v
-
-def speed(audio: np.ndarray, s: float = 2, precision:int = 24):
- if s%1 != 0 and (1/s)%1 != 0:
- import fractions
- s = fractions.Fraction(s).limit_denominator(precision)
- audio = np.repeat(audio, s.denominator, axis=1)
- return audio[:,::s.numerator]
- elif s%1 == 0:
- return audio[:,::int(s)]
- else:
- return np.repeat(audio, int(1/s), axis=1)
-
-def channel(audio: np.ndarray, c:int = None):
- if c is None:
- audio[0], audio[1] = audio[1], audio[0]
- return audio
- elif c == 0:
- audio[0] = 0
- return audio
- else:
- audio[1] = 0
- return audio
-
-def downsample(audio: np.ndarray, d:int = 10):
- return np.repeat(audio[:,::d], d, axis=1)
-
-def gradient(audio: np.ndarray, number: int = 1):
- for _ in range(number):
- audio = np.gradient(audio, axis=1)
- return audio
-
-def bitcrush(audio: np.ndarray, b:float = 4):
- if 1/b > 1:
- return np.around(audio, decimals=int(1/b))
- else:
- return np.around(audio*b, decimals = 1)
-
-def reverse(audio: np.ndarray):
- return audio[:,::-1]
-
-def normalize(audio: np.ndarray):
- return audio*(1/np.max(np.abs(audio)))
-
-def clip(audio: np.ndarray):
- return np.clip(audio, -1, 1)
-
-def to_sidechain(audio: np.ndarray):
- audio = np.clip(np.abs(audio), -1, 1)
- for channel in range(len(audio)):
- audio[channel] = np.abs(1 - np.convolve(audio[channel], np.ones(shape=(1000)), mode = 'same'))
- return audio
-
-
-
-# some stuff is defined in main.py to reduce function calls for 1 line stuff
-BM_EFFECTS = {
- "v": "volume",
- "s": speed,
- "c": channel,
- "d": "downsample",
- "g": "gradient",
- "b": bitcrush,
- "r": "reverse",
-}
\ No newline at end of file
diff --git a/spaces/dragonSwing/annotate-anything/GroundingDINO/groundingdino/models/GroundingDINO/csrc/MsDeformAttn/ms_deform_attn_cpu.h b/spaces/dragonSwing/annotate-anything/GroundingDINO/groundingdino/models/GroundingDINO/csrc/MsDeformAttn/ms_deform_attn_cpu.h
deleted file mode 100644
index b2b88e8c46f19b6db0933163e57ccdb51180f517..0000000000000000000000000000000000000000
--- a/spaces/dragonSwing/annotate-anything/GroundingDINO/groundingdino/models/GroundingDINO/csrc/MsDeformAttn/ms_deform_attn_cpu.h
+++ /dev/null
@@ -1,35 +0,0 @@
-/*!
-**************************************************************************************************
-* Deformable DETR
-* Copyright (c) 2020 SenseTime. All Rights Reserved.
-* Licensed under the Apache License, Version 2.0 [see LICENSE for details]
-**************************************************************************************************
-* Modified from https://github.com/chengdazhi/Deformable-Convolution-V2-PyTorch/tree/pytorch_1.0.0
-**************************************************************************************************
-*/
-
-#pragma once
-#include
-
-namespace groundingdino {
-
-at::Tensor
-ms_deform_attn_cpu_forward(
- const at::Tensor &value,
- const at::Tensor &spatial_shapes,
- const at::Tensor &level_start_index,
- const at::Tensor &sampling_loc,
- const at::Tensor &attn_weight,
- const int im2col_step);
-
-std::vector
-ms_deform_attn_cpu_backward(
- const at::Tensor &value,
- const at::Tensor &spatial_shapes,
- const at::Tensor &level_start_index,
- const at::Tensor &sampling_loc,
- const at::Tensor &attn_weight,
- const at::Tensor &grad_output,
- const int im2col_step);
-
-} // namespace groundingdino
diff --git a/spaces/dylanmcc/beaverdam/README.md b/spaces/dylanmcc/beaverdam/README.md
deleted file mode 100644
index ebef954e78cef9b0c70fa081eb0c941721264804..0000000000000000000000000000000000000000
--- a/spaces/dylanmcc/beaverdam/README.md
+++ /dev/null
@@ -1,13 +0,0 @@
----
-title: Is is a beaver dam?
-emoji: 🦫
-colorFrom: purple
-colorTo: green
-sdk: gradio
-sdk_version: 3.24.1
-app_file: app.py
-pinned: false
-license: openrail
----
-
-Check out the configuration reference at https://huggingface.co/docs/hub/spaces-config-reference
diff --git a/spaces/easrng/text-to-emoji/README.md b/spaces/easrng/text-to-emoji/README.md
deleted file mode 100644
index 6404d2e8d29727ecbff16f69f90af2b7cc7e2a58..0000000000000000000000000000000000000000
--- a/spaces/easrng/text-to-emoji/README.md
+++ /dev/null
@@ -1,13 +0,0 @@
----
-title: Text To Emoji
-emoji: 🐨
-colorFrom: green
-colorTo: blue
-sdk: gradio
-sdk_version: 3.27.0
-app_file: app.py
-pinned: false
-license: other
----
-
-Check out the configuration reference at https://huggingface.co/docs/hub/spaces-config-reference
diff --git a/spaces/ehristoforu/Hackchat/README.md b/spaces/ehristoforu/Hackchat/README.md
deleted file mode 100644
index 8936dea47ba363c737d8348383e5809f781bc5fd..0000000000000000000000000000000000000000
--- a/spaces/ehristoforu/Hackchat/README.md
+++ /dev/null
@@ -1,13 +0,0 @@
----
-title: Chat Ui Template
-emoji: 🚀
-colorFrom: indigo
-colorTo: blue
-sdk: docker
-pinned: false
-app_port: 3000
-suggested_hardware: a10g-small
-duplicated_from: huggingchat/chat-ui-template
----
-
-Check out the configuration reference at https://huggingface.co/docs/hub/spaces-config-reference
diff --git a/spaces/ejbejaranos/somos-alpaca-es/README.md b/spaces/ejbejaranos/somos-alpaca-es/README.md
deleted file mode 100644
index 9488e14415c39b7d4e22956140951ba6774542d5..0000000000000000000000000000000000000000
--- a/spaces/ejbejaranos/somos-alpaca-es/README.md
+++ /dev/null
@@ -1,13 +0,0 @@
----
-title: Hackathon SomosNLP Reto Datasets LLM Español
-emoji: 🦙 🏷️
-colorFrom: purple
-colorTo: red
-sdk: docker
-app_port: 6900
-fullWidth: true
-tags:
-- argilla
-- somosnlp
-duplicated_from: somosnlp/somos-alpaca-es
----
diff --git a/spaces/elena-k/OmdenaTriesteLongCovid/README.md b/spaces/elena-k/OmdenaTriesteLongCovid/README.md
deleted file mode 100644
index c89d89e1839c82cb44a47257f493a0347bf93e9d..0000000000000000000000000000000000000000
--- a/spaces/elena-k/OmdenaTriesteLongCovid/README.md
+++ /dev/null
@@ -1,13 +0,0 @@
----
-title: OmdenaTriesteLongCovid
-emoji: 📊
-colorFrom: gray
-colorTo: blue
-sdk: gradio
-sdk_version: 3.1.1
-app_file: app.py
-pinned: false
-license: gpl-3.0
----
-
-Check out the configuration reference at https://huggingface.co/docs/hub/spaces-config-reference
diff --git a/spaces/fabiogra/moseca/app/service/vocal_remover/layers.py b/spaces/fabiogra/moseca/app/service/vocal_remover/layers.py
deleted file mode 100644
index 58f43d04d8aa5a76a1c4438f9319c966c971d4cf..0000000000000000000000000000000000000000
--- a/spaces/fabiogra/moseca/app/service/vocal_remover/layers.py
+++ /dev/null
@@ -1,126 +0,0 @@
-import torch
-from torch import nn
-import torch.nn.functional as F
-
-
-def crop_center(h1, h2):
- h1_shape = h1.size()
- h2_shape = h2.size()
-
- if h1_shape[3] == h2_shape[3]:
- return h1
- elif h1_shape[3] < h2_shape[3]:
- raise ValueError("h1_shape[3] must be greater than h2_shape[3]")
-
- s_time = (h1_shape[3] - h2_shape[3]) // 2
- e_time = s_time + h2_shape[3]
- h1 = h1[:, :, :, s_time:e_time]
-
- return h1
-
-
-class Conv2DBNActiv(nn.Module):
- def __init__(self, nin, nout, ksize=3, stride=1, pad=1, dilation=1, activ=nn.ReLU):
- super(Conv2DBNActiv, self).__init__()
- self.conv = nn.Sequential(
- nn.Conv2d(
- nin,
- nout,
- kernel_size=ksize,
- stride=stride,
- padding=pad,
- dilation=dilation,
- bias=False,
- ),
- nn.BatchNorm2d(nout),
- activ(),
- )
-
- def __call__(self, x):
- return self.conv(x)
-
-
-class Encoder(nn.Module):
- def __init__(self, nin, nout, ksize=3, stride=1, pad=1, activ=nn.LeakyReLU):
- super(Encoder, self).__init__()
- self.conv1 = Conv2DBNActiv(nin, nout, ksize, stride, pad, activ=activ)
- self.conv2 = Conv2DBNActiv(nout, nout, ksize, 1, pad, activ=activ)
-
- def __call__(self, x):
- h = self.conv1(x)
- h = self.conv2(h)
-
- return h
-
-
-class Decoder(nn.Module):
- def __init__(self, nin, nout, ksize=3, stride=1, pad=1, activ=nn.ReLU, dropout=False):
- super(Decoder, self).__init__()
- self.conv1 = Conv2DBNActiv(nin, nout, ksize, 1, pad, activ=activ)
- self.dropout = nn.Dropout2d(0.1) if dropout else None
-
- def __call__(self, x, skip=None):
- x = F.interpolate(x, scale_factor=2, mode="bilinear", align_corners=True)
-
- if skip is not None:
- skip = crop_center(skip, x)
- x = torch.cat([x, skip], dim=1)
-
- h = self.conv1(x)
- # h = self.conv2(h)
-
- if self.dropout is not None:
- h = self.dropout(h)
-
- return h
-
-
-class ASPPModule(nn.Module):
- def __init__(self, nin, nout, dilations=(4, 8, 12), activ=nn.ReLU, dropout=False):
- super(ASPPModule, self).__init__()
- self.conv1 = nn.Sequential(
- nn.AdaptiveAvgPool2d((1, None)),
- Conv2DBNActiv(nin, nout, 1, 1, 0, activ=activ),
- )
- self.conv2 = Conv2DBNActiv(nin, nout, 1, 1, 0, activ=activ)
- self.conv3 = Conv2DBNActiv(nin, nout, 3, 1, dilations[0], dilations[0], activ=activ)
- self.conv4 = Conv2DBNActiv(nin, nout, 3, 1, dilations[1], dilations[1], activ=activ)
- self.conv5 = Conv2DBNActiv(nin, nout, 3, 1, dilations[2], dilations[2], activ=activ)
- self.bottleneck = Conv2DBNActiv(nout * 5, nout, 1, 1, 0, activ=activ)
- self.dropout = nn.Dropout2d(0.1) if dropout else None
-
- def forward(self, x):
- _, _, h, w = x.size()
- feat1 = F.interpolate(self.conv1(x), size=(h, w), mode="bilinear", align_corners=True)
- feat2 = self.conv2(x)
- feat3 = self.conv3(x)
- feat4 = self.conv4(x)
- feat5 = self.conv5(x)
- out = torch.cat((feat1, feat2, feat3, feat4, feat5), dim=1)
- out = self.bottleneck(out)
-
- if self.dropout is not None:
- out = self.dropout(out)
-
- return out
-
-
-class LSTMModule(nn.Module):
- def __init__(self, nin_conv, nin_lstm, nout_lstm):
- super(LSTMModule, self).__init__()
- self.conv = Conv2DBNActiv(nin_conv, 1, 1, 1, 0)
- self.lstm = nn.LSTM(input_size=nin_lstm, hidden_size=nout_lstm // 2, bidirectional=True)
- self.dense = nn.Sequential(
- nn.Linear(nout_lstm, nin_lstm), nn.BatchNorm1d(nin_lstm), nn.ReLU()
- )
-
- def forward(self, x):
- N, _, nbins, nframes = x.size()
- h = self.conv(x)[:, 0] # N, nbins, nframes
- h = h.permute(2, 0, 1) # nframes, N, nbins
- h, _ = self.lstm(h)
- h = self.dense(h.reshape(-1, h.size()[-1])) # nframes * N, nbins
- h = h.reshape(nframes, N, 1, nbins)
- h = h.permute(1, 2, 3, 0)
-
- return h
diff --git a/spaces/feng2022/Time-TravelRephotography/Time_TravelRephotography/models/encoder4editing/datasets/gt_res_dataset.py b/spaces/feng2022/Time-TravelRephotography/Time_TravelRephotography/models/encoder4editing/datasets/gt_res_dataset.py
deleted file mode 100644
index c0beacfee5335aa10aa7e8b7cabe206d7f9a56f7..0000000000000000000000000000000000000000
--- a/spaces/feng2022/Time-TravelRephotography/Time_TravelRephotography/models/encoder4editing/datasets/gt_res_dataset.py
+++ /dev/null
@@ -1,32 +0,0 @@
-#!/usr/bin/python
-# encoding: utf-8
-import os
-from torch.utils.data import Dataset
-from PIL import Image
-import torch
-
-class GTResDataset(Dataset):
-
- def __init__(self, root_path, gt_dir=None, transform=None, transform_train=None):
- self.pairs = []
- for f in os.listdir(root_path):
- image_path = os.path.join(root_path, f)
- gt_path = os.path.join(gt_dir, f)
- if f.endswith(".jpg") or f.endswith(".png"):
- self.pairs.append([image_path, gt_path.replace('.png', '.jpg'), None])
- self.transform = transform
- self.transform_train = transform_train
-
- def __len__(self):
- return len(self.pairs)
-
- def __getitem__(self, index):
- from_path, to_path, _ = self.pairs[index]
- from_im = Image.open(from_path).convert('RGB')
- to_im = Image.open(to_path).convert('RGB')
-
- if self.transform:
- to_im = self.transform(to_im)
- from_im = self.transform(from_im)
-
- return from_im, to_im
diff --git a/spaces/feregVcuzo/sanity-test-midi/checkpoint/BlackPlayer EX 20.62 - Premium Music Player Mod APK for Android.md b/spaces/feregVcuzo/sanity-test-midi/checkpoint/BlackPlayer EX 20.62 - Premium Music Player Mod APK for Android.md
deleted file mode 100644
index 5ee98dad97444637aa56fcb1e4d27aa6af108c82..0000000000000000000000000000000000000000
--- a/spaces/feregVcuzo/sanity-test-midi/checkpoint/BlackPlayer EX 20.62 - Premium Music Player Mod APK for Android.md
+++ /dev/null
@@ -1,116 +0,0 @@
-
-BlackPlayer EX MOD APK: A Premium Music Player for Android
-If you are looking for a music player that can offer you a premium experience on your Android device, you might want to check out BlackPlayer EX MOD APK. This is a modified version of the original BlackPlayer EX app, which removes the ads and unlocks all the extra features that are normally available only in the paid version. In this article, we will tell you what BlackPlayer EX MOD APK is, what features it has, how to download and install it, and why you should choose it over other music players.
-What is BlackPlayer EX MOD APK?
-BlackPlayer EX MOD APK is a music player app for Android devices that lets you enjoy your favorite songs without any interruptions or limitations. It is based on the original BlackPlayer EX app, which is one of the most popular and highly rated music players on the Google Play Store. However, unlike the original app, which costs $3.59 to download and has ads, BlackPlayer EX MOD APK is free to download and has no ads. It also unlocks all the extra features that are normally available only in the paid version of the app.
-black player mod apk
Download 🆗 https://gohhs.com/2uPvyI
-Features of BlackPlayer EX MOD APK
-BlackPlayer EX MOD APK has many features that make it stand out from other music players. Here are some of them:
-No ads
-One of the main advantages of BlackPlayer EX MOD APK is that it removes all the annoying ads that can interrupt your music listening experience. You can enjoy your songs without any distractions or interruptions.
-Customizable interface
-Another great feature of BlackPlayer EX MOD APK is that it allows you to customize the interface according to your preferences. You can change the colors, fonts, icons, animations, and layout of the app. You can also choose between different themes, such as light, dark, black, or transparent. You can make your own interface with the customization found in the Settings menu.
-Extra themes and fonts
-BlackPlayer EX MOD APK also gives you access to extra themes and fonts that are not available in the free version of the app. You can choose from more than 10 themes and more than 20 fonts to make your app look more stylish and unique.
-Equalizer and sound effects
-If you want to enhance your audio quality, you can use the built-in equalizer and sound effects in BlackPlayer EX MOD APK. You can adjust the bass, treble, balance, volume, and other parameters to suit your taste. You can also choose from various presets or create your own custom settings. You can also apply sound effects such as reverb, bass boost, virtualizer, and more.
-Support for various audio formats
-Lyrics and ID3 tag editor
-BlackPlayer EX MOD APK also supports displaying lyrics and editing ID3 tags of your songs. You can view the lyrics of the song that is playing, or search for them online. You can also edit the metadata of your songs, such as the title, artist, album, genre, year, and more. You can also add album art or download it from the internet.
-black player ex music player patched mod apk
-black player premium apk mod free download
-black player ex 20.62 build 400 mod apk
-black player mod apk latest version
-black player ex pro mod apk
-black player ex music player mod apk rexdl
-black player ex 20.61 build 399 mod apk
-black player ex music player cracked mod apk
-black player ex music player v20.62 b400 mod apk
-black player ex music player unlocked mod apk
-black player ex music player full mod apk
-black player ex music player no ads mod apk
-black player ex music player 20.62 build 400 mod apk
-black player ex music player extra premium features mod apk
-black player ex music player 20.61 build 399 mod apk
-black player ex music player ad-free mod apk
-black player ex music player 20.62 b400 mod apk
-black player ex music player patched cracked mod apk
-black player ex music player 20.61 b399 mod apk
-black player ex music player unlimited features mod apk
-black player ex music player pro unlocked mod apk
-black player ex music player 20.62 build 400 patched mod apk
-black player ex music player best android music app mod apk
-black player ex music player 20.61 build 399 patched mod apk
-black player ex music player premium features unlocked mod apk
-black player ex music app download for android mod apk
-black player ex music app latest version download mod apk
-black player ex music app free download for android mod apk
-black player ex music app update version download mod apk
-black player ex music app best offline music app mod apk
-blackplayer free vs ex comparison review mod apk
-how to install blackplayer ex on android device mod apk
-how to use blackplayer ex features and settings mod apk
-how to customize blackplayer ex interface and themes mod apk
-how to upgrade from blackplayer free to ex version mod apk
-how to backup and restore blackplayer ex data and settings mod apk
-how to fix common issues and errors with blackplayer ex mod apk
-how to get support and feedback for blackplayer ex mod apk
-how to join beta testing program for blackplayer ex mod apk
-how to download and install latest update for blackplayer ex mod apk
-what's new in blackplayer ex 20.62 build 400 version mod apk
-what's new in blackplayer ex 20.61 build 399 version mod apk
-what are the benefits of using blackplayer ex over other music apps mod apk
-what are the differences between blackplayer free and paid versions mod apk
-what are the best alternatives to blackplayer ex for android devices mod apk
-what are the best features and functions of blackplayer ex app mod apk
-what are the best tips and tricks for using blackplayer ex app efficiently mod apk
-what are the best settings and options for optimizing blackplayer ex performance and battery life mod apk
-what are the best themes and skins for personalizing blackplayer ex appearance and style mod apk
-Sleep timer and widgets
-If you want to listen to music before going to sleep, you can use the sleep timer feature in BlackPlayer EX MOD APK. You can set a timer for how long you want the music to play, and the app will automatically stop it when the time is up. You can also use the widgets feature to access the app from your home screen or lock screen. You can choose from different sizes and styles of widgets to control your music playback.
-How to download and install BlackPlayer EX MOD APK?
-If you are interested in trying out BlackPlayer EX MOD APK, you can follow these simple steps to download and install it on your device:
-Step 1: Download the APK file from a trusted source
-The first thing you need to do is to download the APK file of BlackPlayer EX MOD APK from a trusted source. You can use the link below to download it directly from our website. The file size is about 15 MB and it is virus-free and safe to use.
-Download BlackPlayer EX MOD APK
-Step 2: Enable unknown sources on your device
-The next thing you need to do is to enable unknown sources on your device. This will allow you to install apps that are not from the Google Play Store. To do this, go to your device settings and look for security or privacy options. Then, find the option that says unknown sources or allow installation of apps from unknown sources and enable it.
-Step 3: Install the APK file and launch the app
-The final thing you need to do is to install the APK file and launch the app. To do this, locate the downloaded file in your device storage and tap on it. Then, follow the instructions on the screen to complete the installation process. Once done, you can launch the app and enjoy your premium music player.
-Why choose BlackPlayer EX MOD APK over other music players?
-You might be wondering why you should choose BlackPlayer EX MOD APK over other music players available on the market. Well, here are some of the reasons why:
-Pros of BlackPlayer EX MOD APK
-
-Smooth and fast performance
-BlackPlayer EX MOD APK is designed to run smoothly and fast on any Android device. It does not consume much battery or memory, and it does not lag or crash. It also has a simple and intuitive interface that makes it easy to use.
-High-quality audio output
-BlackPlayer EX MOD APK delivers high-quality audio output that will satisfy any audiophile. It supports various audio formats, such as MP3, WAV, OGG, FLAC, M4A, and more. It also has an equalizer and sound effects that let you adjust and enhance your sound quality.
-User-friendly and customizable interface
-BlackPlayer EX MOD APK has a user-friendly and customizable interface that lets you personalize your music player according to your preferences. You can change the colors, fonts, icons, animations, and layout of the app. You can also choose between different themes, such as light, dark, black, or transparent.
-Tons of extra features and options
-BlackPlayer EX MOD APK has tons of extra features and options that make it more than just a music player. You can view and edit lyrics and ID3 tags of your songs, use the sleep timer and widgets features, access online radio stations, create playlists and folders, shuffle and repeat modes, gapless playback, crossfade, scrobbling, and more.
-
-Cons of BlackPlayer EX MOD APK
-
-Requires Android 4.0 or higher
-One of the drawbacks of BlackPlayer EX MOD APK is that it requires Android 4.0 or higher to run. This means that if you have an older device or operating system, you might not be able to use this app.
-May not be compatible with some devices or audio formats
-Another drawback of BlackPlayer EX MOD APK is that it may not be compatible with some devices or audio formats. Some users have reported issues with playing certain audio formats, such as WMA, AAC, or MKA. Some users have also reported problems with the app crashing or not working properly on some devices, such as Samsung, Huawei, or Xiaomi.
-
-Conclusion
-BlackPlayer EX MOD APK is a premium music player for Android devices that offers a smooth and fast performance, high-quality audio output, user-friendly and customizable interface, and tons of extra features and options. It is free to download and has no ads. It also unlocks all the extra features that are normally available only in the paid version of the app. However, it also has some drawbacks, such as requiring Android 4.0 or higher and being incompatible with some devices or audio formats. If you are looking for a music player that can give you a premium experience on your Android device, you might want to give BlackPlayer EX MOD APK a try.
-FAQs
-
-What is the difference between BlackPlayer EX and BlackPlayer EX MOD APK?
-BlackPlayer EX is the original app that is available on the Google Play Store for $3.59. It has ads and some features are locked in the free version. BlackPlayer EX MOD APK is a modified version of the app that removes the ads and unlocks all the features for free.
-Is BlackPlayer EX MOD APK safe to use?
-Yes, BlackPlayer EX MOD APK is safe to use as long as you download it from a trusted source. It does not contain any viruses or malware that can harm your device or data. However, you should always be careful when installing apps from unknown sources and check the permissions they require.
-How can I update BlackPlayer EX MOD APK?
-To update BlackPlayer EX MOD APK, you need to download the latest version of the APK file from a trusted source and install it over the existing app. You can also check for updates within the app by going to Settings > About > Check for updates.
-How can I contact the developer of BlackPlayer EX MOD APK?
-If you have any questions, feedback, or suggestions for the developer of BlackPlayer EX MOD APK, you can contact them by email at blackplayerex@gmail.com. You can also visit their website at https://blackplayerex.com/ or follow them on Twitter at @blackplayerex.
-How can I support the developer of BlackPlayer EX MOD APK?
-If you like BlackPlayer EX MOD APK and want to support the developer, you can buy them a coffee at https://www.buymeacoffee.com/blackplayerex. You can also rate and review the app on the Google Play Store or share it with your friends.
-
401be4b1e0
-
-
\ No newline at end of file
diff --git a/spaces/fffiloni/controlnet-animation-doodle/node_modules/@types/node/ts4.8/stream/web.d.ts b/spaces/fffiloni/controlnet-animation-doodle/node_modules/@types/node/ts4.8/stream/web.d.ts
deleted file mode 100644
index f9ef0570dc3f9290195183725d8e25fd1c40c249..0000000000000000000000000000000000000000
--- a/spaces/fffiloni/controlnet-animation-doodle/node_modules/@types/node/ts4.8/stream/web.d.ts
+++ /dev/null
@@ -1,330 +0,0 @@
-declare module 'stream/web' {
- // stub module, pending copy&paste from .d.ts or manual impl
- // copy from lib.dom.d.ts
- interface ReadableWritablePair {
- readable: ReadableStream;
- /**
- * Provides a convenient, chainable way of piping this readable stream
- * through a transform stream (or any other { writable, readable }
- * pair). It simply pipes the stream into the writable side of the
- * supplied pair, and returns the readable side for further use.
- *
- * Piping a stream will lock it for the duration of the pipe, preventing
- * any other consumer from acquiring a reader.
- */
- writable: WritableStream;
- }
- interface StreamPipeOptions {
- preventAbort?: boolean;
- preventCancel?: boolean;
- /**
- * Pipes this readable stream to a given writable stream destination.
- * The way in which the piping process behaves under various error
- * conditions can be customized with a number of passed options. It
- * returns a promise that fulfills when the piping process completes
- * successfully, or rejects if any errors were encountered.
- *
- * Piping a stream will lock it for the duration of the pipe, preventing
- * any other consumer from acquiring a reader.
- *
- * Errors and closures of the source and destination streams propagate
- * as follows:
- *
- * An error in this source readable stream will abort destination,
- * unless preventAbort is truthy. The returned promise will be rejected
- * with the source's error, or with any error that occurs during
- * aborting the destination.
- *
- * An error in destination will cancel this source readable stream,
- * unless preventCancel is truthy. The returned promise will be rejected
- * with the destination's error, or with any error that occurs during
- * canceling the source.
- *
- * When this source readable stream closes, destination will be closed,
- * unless preventClose is truthy. The returned promise will be fulfilled
- * once this process completes, unless an error is encountered while
- * closing the destination, in which case it will be rejected with that
- * error.
- *
- * If destination starts out closed or closing, this source readable
- * stream will be canceled, unless preventCancel is true. The returned
- * promise will be rejected with an error indicating piping to a closed
- * stream failed, or with any error that occurs during canceling the
- * source.
- *
- * The signal option can be set to an AbortSignal to allow aborting an
- * ongoing pipe operation via the corresponding AbortController. In this
- * case, this source readable stream will be canceled, and destination
- * aborted, unless the respective options preventCancel or preventAbort
- * are set.
- */
- preventClose?: boolean;
- signal?: AbortSignal;
- }
- interface ReadableStreamGenericReader {
- readonly closed: Promise;
- cancel(reason?: any): Promise;
- }
- interface ReadableStreamDefaultReadValueResult {
- done: false;
- value: T;
- }
- interface ReadableStreamDefaultReadDoneResult {
- done: true;
- value?: undefined;
- }
- type ReadableStreamController = ReadableStreamDefaultController;
- type ReadableStreamDefaultReadResult = ReadableStreamDefaultReadValueResult | ReadableStreamDefaultReadDoneResult;
- interface ReadableByteStreamControllerCallback {
- (controller: ReadableByteStreamController): void | PromiseLike;
- }
- interface UnderlyingSinkAbortCallback {
- (reason?: any): void | PromiseLike;
- }
- interface UnderlyingSinkCloseCallback {
- (): void | PromiseLike;
- }
- interface UnderlyingSinkStartCallback {
- (controller: WritableStreamDefaultController): any;
- }
- interface UnderlyingSinkWriteCallback {
- (chunk: W, controller: WritableStreamDefaultController): void | PromiseLike;
- }
- interface UnderlyingSourceCancelCallback {
- (reason?: any): void | PromiseLike;
- }
- interface UnderlyingSourcePullCallback {
- (controller: ReadableStreamController): void | PromiseLike;
- }
- interface UnderlyingSourceStartCallback {
- (controller: ReadableStreamController): any;
- }
- interface TransformerFlushCallback {
- (controller: TransformStreamDefaultController): void | PromiseLike;
- }
- interface TransformerStartCallback {
- (controller: TransformStreamDefaultController): any;
- }
- interface TransformerTransformCallback {
- (chunk: I, controller: TransformStreamDefaultController): void | PromiseLike;
- }
- interface UnderlyingByteSource {
- autoAllocateChunkSize?: number;
- cancel?: ReadableStreamErrorCallback;
- pull?: ReadableByteStreamControllerCallback;
- start?: ReadableByteStreamControllerCallback;
- type: 'bytes';
- }
- interface UnderlyingSource {
- cancel?: UnderlyingSourceCancelCallback;
- pull?: UnderlyingSourcePullCallback;
- start?: UnderlyingSourceStartCallback;
- type?: undefined;
- }
- interface UnderlyingSink {
- abort?: UnderlyingSinkAbortCallback;
- close?: UnderlyingSinkCloseCallback;
- start?: UnderlyingSinkStartCallback;
- type?: undefined;
- write?: UnderlyingSinkWriteCallback;
- }
- interface ReadableStreamErrorCallback {
- (reason: any): void | PromiseLike;
- }
- /** This Streams API interface represents a readable stream of byte data. */
- interface ReadableStream {
- readonly locked: boolean;
- cancel(reason?: any): Promise;
- getReader(): ReadableStreamDefaultReader;
- pipeThrough(transform: ReadableWritablePair, options?: StreamPipeOptions): ReadableStream;
- pipeTo(destination: WritableStream, options?: StreamPipeOptions): Promise;
- tee(): [ReadableStream, ReadableStream];
- values(options?: { preventCancel?: boolean }): AsyncIterableIterator;
- [Symbol.asyncIterator](): AsyncIterableIterator;
- }
- const ReadableStream: {
- prototype: ReadableStream;
- new (underlyingSource: UnderlyingByteSource, strategy?: QueuingStrategy): ReadableStream;
- new (underlyingSource?: UnderlyingSource, strategy?: QueuingStrategy): ReadableStream;
- };
- interface ReadableStreamDefaultReader extends ReadableStreamGenericReader {
- read(): Promise>;
- releaseLock(): void;
- }
- const ReadableStreamDefaultReader: {
- prototype: ReadableStreamDefaultReader;
- new (stream: ReadableStream): ReadableStreamDefaultReader;
- };
- const ReadableStreamBYOBReader: any;
- const ReadableStreamBYOBRequest: any;
- interface ReadableByteStreamController {
- readonly byobRequest: undefined;
- readonly desiredSize: number | null;
- close(): void;
- enqueue(chunk: ArrayBufferView): void;
- error(error?: any): void;
- }
- const ReadableByteStreamController: {
- prototype: ReadableByteStreamController;
- new (): ReadableByteStreamController;
- };
- interface ReadableStreamDefaultController {
- readonly desiredSize: number | null;
- close(): void;
- enqueue(chunk?: R): void;
- error(e?: any): void;
- }
- const ReadableStreamDefaultController: {
- prototype: ReadableStreamDefaultController;
- new (): ReadableStreamDefaultController;
- };
- interface Transformer {
- flush?: TransformerFlushCallback;
- readableType?: undefined;
- start?: TransformerStartCallback;
- transform?: TransformerTransformCallback;
- writableType?: undefined;
- }
- interface TransformStream {
- readonly readable: ReadableStream;
- readonly writable: WritableStream;
- }
- const TransformStream: {
- prototype: TransformStream;
- new (transformer?: Transformer, writableStrategy?: QueuingStrategy, readableStrategy?: QueuingStrategy): TransformStream;
- };
- interface TransformStreamDefaultController {
- readonly desiredSize: number | null;
- enqueue(chunk?: O): void;
- error(reason?: any): void;
- terminate(): void;
- }
- const TransformStreamDefaultController: {
- prototype: TransformStreamDefaultController;
- new (): TransformStreamDefaultController;
- };
- /**
- * This Streams API interface provides a standard abstraction for writing
- * streaming data to a destination, known as a sink. This object comes with
- * built-in back pressure and queuing.
- */
- interface WritableStream {
- readonly locked: boolean;
- abort(reason?: any): Promise;
- close(): Promise;
- getWriter(): WritableStreamDefaultWriter;
- }
- const WritableStream: {
- prototype: WritableStream;
- new (underlyingSink?: UnderlyingSink, strategy?: QueuingStrategy): WritableStream;
- };
- /**
- * This Streams API interface is the object returned by
- * WritableStream.getWriter() and once created locks the < writer to the
- * WritableStream ensuring that no other streams can write to the underlying
- * sink.
- */
- interface WritableStreamDefaultWriter {
- readonly closed: Promise;
- readonly desiredSize: number | null;
- readonly ready: Promise;
- abort(reason?: any): Promise;
- close(): Promise;
- releaseLock(): void;
- write(chunk?: W): Promise;
- }
- const WritableStreamDefaultWriter: {
- prototype: WritableStreamDefaultWriter;
- new (stream: WritableStream): WritableStreamDefaultWriter;
- };
- /**
- * This Streams API interface represents a controller allowing control of a
- * WritableStream's state. When constructing a WritableStream, the
- * underlying sink is given a corresponding WritableStreamDefaultController
- * instance to manipulate.
- */
- interface WritableStreamDefaultController {
- error(e?: any): void;
- }
- const WritableStreamDefaultController: {
- prototype: WritableStreamDefaultController;
- new (): WritableStreamDefaultController;
- };
- interface QueuingStrategy {
- highWaterMark?: number;
- size?: QueuingStrategySize;
- }
- interface QueuingStrategySize {
- (chunk?: T): number;
- }
- interface QueuingStrategyInit {
- /**
- * Creates a new ByteLengthQueuingStrategy with the provided high water
- * mark.
- *
- * Note that the provided high water mark will not be validated ahead of
- * time. Instead, if it is negative, NaN, or not a number, the resulting
- * ByteLengthQueuingStrategy will cause the corresponding stream
- * constructor to throw.
- */
- highWaterMark: number;
- }
- /**
- * This Streams API interface provides a built-in byte length queuing
- * strategy that can be used when constructing streams.
- */
- interface ByteLengthQueuingStrategy extends QueuingStrategy {
- readonly highWaterMark: number;
- readonly size: QueuingStrategySize;
- }
- const ByteLengthQueuingStrategy: {
- prototype: ByteLengthQueuingStrategy;
- new (init: QueuingStrategyInit): ByteLengthQueuingStrategy;
- };
- /**
- * This Streams API interface provides a built-in byte length queuing
- * strategy that can be used when constructing streams.
- */
- interface CountQueuingStrategy extends QueuingStrategy {
- readonly highWaterMark: number;
- readonly size: QueuingStrategySize;
- }
- const CountQueuingStrategy: {
- prototype: CountQueuingStrategy;
- new (init: QueuingStrategyInit): CountQueuingStrategy;
- };
- interface TextEncoderStream {
- /** Returns "utf-8". */
- readonly encoding: 'utf-8';
- readonly readable: ReadableStream;
- readonly writable: WritableStream;
- readonly [Symbol.toStringTag]: string;
- }
- const TextEncoderStream: {
- prototype: TextEncoderStream;
- new (): TextEncoderStream;
- };
- interface TextDecoderOptions {
- fatal?: boolean;
- ignoreBOM?: boolean;
- }
- type BufferSource = ArrayBufferView | ArrayBuffer;
- interface TextDecoderStream {
- /** Returns encoding's name, lower cased. */
- readonly encoding: string;
- /** Returns `true` if error mode is "fatal", and `false` otherwise. */
- readonly fatal: boolean;
- /** Returns `true` if ignore BOM flag is set, and `false` otherwise. */
- readonly ignoreBOM: boolean;
- readonly readable: ReadableStream;
- readonly writable: WritableStream;
- readonly [Symbol.toStringTag]: string;
- }
- const TextDecoderStream: {
- prototype: TextDecoderStream;
- new (label?: string, options?: TextDecoderOptions): TextDecoderStream;
- };
-}
-declare module 'node:stream/web' {
- export * from 'stream/web';
-}
diff --git a/spaces/fffiloni/controlnet-animation-doodle/node_modules/ipaddr.js/lib/ipaddr.js.d.ts b/spaces/fffiloni/controlnet-animation-doodle/node_modules/ipaddr.js/lib/ipaddr.js.d.ts
deleted file mode 100644
index 52174b6b6b28411c03f230e779c6f1edf93f9423..0000000000000000000000000000000000000000
--- a/spaces/fffiloni/controlnet-animation-doodle/node_modules/ipaddr.js/lib/ipaddr.js.d.ts
+++ /dev/null
@@ -1,68 +0,0 @@
-declare module "ipaddr.js" {
- type IPv4Range = 'unicast' | 'unspecified' | 'broadcast' | 'multicast' | 'linkLocal' | 'loopback' | 'carrierGradeNat' | 'private' | 'reserved';
- type IPv6Range = 'unicast' | 'unspecified' | 'linkLocal' | 'multicast' | 'loopback' | 'uniqueLocal' | 'ipv4Mapped' | 'rfc6145' | 'rfc6052' | '6to4' | 'teredo' | 'reserved';
-
- interface RangeList {
- [name: string]: [T, number] | [T, number][];
- }
-
- // Common methods/properties for IPv4 and IPv6 classes.
- class IP {
- prefixLengthFromSubnetMask(): number | null;
- toByteArray(): number[];
- toNormalizedString(): string;
- toString(): string;
- }
-
- namespace Address {
- export function isValid(addr: string): boolean;
- export function fromByteArray(bytes: number[]): IPv4 | IPv6;
- export function parse(addr: string): IPv4 | IPv6;
- export function parseCIDR(mask: string): [IPv4 | IPv6, number];
- export function process(addr: string): IPv4 | IPv6;
- export function subnetMatch(addr: IPv4, rangeList: RangeList, defaultName?: string): string;
- export function subnetMatch(addr: IPv6, rangeList: RangeList, defaultName?: string): string;
-
- export class IPv4 extends IP {
- static broadcastAddressFromCIDR(addr: string): IPv4;
- static isIPv4(addr: string): boolean;
- static isValidFourPartDecimal(addr: string): boolean;
- static isValid(addr: string): boolean;
- static networkAddressFromCIDR(addr: string): IPv4;
- static parse(addr: string): IPv4;
- static parseCIDR(addr: string): [IPv4, number];
- static subnetMaskFromPrefixLength(prefix: number): IPv4;
- constructor(octets: number[]);
- octets: number[]
-
- kind(): 'ipv4';
- match(addr: IPv4, bits: number): boolean;
- match(mask: [IPv4, number]): boolean;
- range(): IPv4Range;
- subnetMatch(rangeList: RangeList