diff --git a/spaces/1acneusushi/gradio-2dmoleculeeditor/data/1 nenokkadine movie download blu-ray rip moviesinstmank Everything you need to know about the plot cast and director of the film.md b/spaces/1acneusushi/gradio-2dmoleculeeditor/data/1 nenokkadine movie download blu-ray rip moviesinstmank Everything you need to know about the plot cast and director of the film.md
deleted file mode 100644
index c31f081cde8b8ef26e01f3f086f6dd2103548540..0000000000000000000000000000000000000000
--- a/spaces/1acneusushi/gradio-2dmoleculeeditor/data/1 nenokkadine movie download blu-ray rip moviesinstmank Everything you need to know about the plot cast and director of the film.md
+++ /dev/null
@@ -1,94 +0,0 @@
-
-
1 Nenokkadine Movie Download Blu-Ray Rip Moviesinstmank: A Guide for Telugu Movie Fans
-
If you are a fan of Telugu movies, you might have heard of 1 Nenokkadine, a psychological action thriller film starring Mahesh Babu and Kriti Sanon. The film was released in 2014 and received critical acclaim for its innovative plot, stunning visuals, and thrilling action sequences. The film also won several awards and became one of the highest-grossing Telugu films at the US box office.
-
But if you missed watching this film in theatres, or if you want to watch it again in high quality, you might be wondering how to download 1 Nenokkadine in Blu-Ray rip quality. In this article, we will guide you through the process of downloading 1 Nenokkadine in Blu-Ray rip quality, as well as explain why you should choose this format over others. We will also discuss some legal and ethical issues related to downloading movies online.
-
1 nenokkadine movie download blu-ray rip moviesinstmank
Before we get into the details of downloading 1 Nenokkadine, let us first give you a brief overview of what this film is about.
-
Plot summary
-
1 Nenokkadine revolves around the search by Gautham, a rock musician with schizophrenia, missing 25 percent of his brain's grey matter, for his parents with the help of Sameera, a journalist. Gautham was convinced that he was an orphan and assumes that his parents are dead; he does not remember their names or what they looked like. He hallucinates a mysterious person he believes is one of the three murderers of his parents. As he unravels the truth behind his past, he also faces danger from various enemies who want to stop him.
-
Cast and crew
-
The film was directed by Sukumar, who also co-wrote the story with Hari Prasad Jakka. The screenplay was written by Jeremy Zimmerman, Arjun Y.K., and Thota Srinivas. The film was produced by Ram Achanta, Gopichand Achanta, and Anil Sunkara on 14 Reels Entertainment banner and was distributed by Eros International.
-
The film stars Mahesh Babu as Gautham, Kriti Sanon as Sameera, Nassar as Chandrashekar's friend, Pradeep Rawat as Taxi Driver, Anuradha Hasan as Kalyani, Kelly Dorji as Antonio Rosarius, Sayaji Shinde as John Baasha, Krishna Murali Posani as Gulab Singh, Srinivasa Reddy as Police Constable, Ravi Varma as Michael, Anand as Chandra Sekhar (Gautham's Father), Supreeth Reddy as Sophiya Chaudhary in Item number, Gautham Ghattamaneni as Young Gautham.
-
Awards and accolades
-
The film received positive reviews from critics who praised its originality, cinematography, music, and performances. The film also won three awards from eight nominations at the 4th South Indian International Movie Awards, and two awards at the 11th CineMAA Awards. The film is considered one of the "25 Greatest Telugu Films Of The Decade" by Film Companion.
-
Why download 1 Nenokkadine in Blu-Ray quality?
-
Now that you know what 1 Nenokkadine is about, you might be wondering why you should download it in Blu-Ray quality. Here are some reasons why Blu-Ray is the best format for watching movies.
-
1 nenokkadine telugu full movie torrent yts
-1 nenokkadine 2014 hindi dubbed 720p zflix co
-1 nenokkadine 2014 bluray rip makemkv
-1 nenokkadine movie watch online amazon prime
-1 nenokkadine telugu action thriller mahesh babu
-1 nenokkadine 2014 dual audio hindi telugu
-1 nenokkadine movie review rating imdb
-1 nenokkadine movie songs download mp3
-1 nenokkadine movie subtitles download srt
-1 nenokkadine movie trailer youtube
-1 nenokkadine movie plot summary wikipedia
-1 nenokkadine movie box office collection
-1 nenokkadine movie director sukumar
-1 nenokkadine movie heroine kriti sanon
-1 nenokkadine movie psychological disorder amnesia
-1 nenokkadine movie climax twist spoiler
-1 nenokkadine movie best scenes video
-1 nenokkadine movie awards and nominations
-1 nenokkadine movie behind the scenes making
-1 nenokkadine movie fan art posters wallpapers
-1 nenokkadine movie quotes dialogues
-1 nenokkadine movie memes funny jokes
-1 nenokkadine movie trivia facts did you know
-1 nenokkadine movie comparison with ghajini memento
-1 nenokkadine movie analysis themes symbols motifs
-1 nenokkadine movie streaming platforms netflix hotstar
-1 nenokkadine movie download hd quality tamilrockers movierulz
-1 nenokkadine movie download free no sign up filmywap filmyzilla
-1 nenokkadine movie download google drive link telegram channel
-1 nenokkadine movie download in parts part 1 part 2 part 3 part 4 part 5 part 6 part7 part8 part9 part10 part11 part12 part13 part14 part15 part16 part17 part18 part19 part20
-
Benefits of Blu-Ray format
-
-
Blu-Ray discs can store up to 50 GB of data, which means they can contain more information and higher resolution than DVDs or CDs.
-
Blu-Ray discs can support up to 1080p HD video quality, which means they can deliver sharper images and more details than standard definition or even HD DVDs.
-
Blu-Ray discs can support up to 7.1 surround sound audio quality, which means they can provide richer sound effects and more immersive audio experience than stereo or Dolby Digital sound.
-
Blu-Ray discs can also offer interactive features such as menus, subtitles, bonus features, and online connectivity that enhance the viewing experience.
-
-
Comparison with other formats
-
-
-
Format
-
Data capacity
-
Video resolution
-
Audio quality
-
Interactive features
-
-
-
DVD
-
4.7 GB (single layer) or 8.5 GB (dual layer)
-
480p (SD) or 576p (PAL)
-
Stereo or Dolby Digital 5.1
-
Limited menus and subtitles
-
-
-
HD DVD
-
15 GB (single layer) or 30 GB (dual layer)
-
720p or 1080i (HD)
-
Dolby Digital Plus or Dolby TrueHD
-
Limited menus and subtitles
-
-
-
Blu-Ray
-
25 GB (single layer) or 50 GB (dual layer)
-
1080p (HD)
-
Dolby Digital Plus or Dolby TrueHD or DTS-HD Master Audio
-
Advanced menus and subtitles, bonus features, online connectivity
-
-
-
How to play Blu-Ray discs on your devices
-
To play Blu-Ray discs on your devices, you will need a Blu-Ray player or a Blu-Ray drive on your computer. You will also need a compatible TV or monitor that can support HD resolution and a sound system that can support surround sound. You will also need an HDMI cable to connect your Blu-Ray player or computer to your TV or monitor.
-
If you don't have a Blu-Ray player or drive on your computer, you can also use a software program that can play Blu-Ray discs on your computer. Some examples of such software are VLC Media Player , PowerDVD , WinDVD , etc. However, you will still need a compatible TV or monitor and a sound system to enjoy the full benefits of Blu-Ray quality.
-
How to download 1 Nenokkadine in Blu-Ray rip quality?
-
If you want to download 1 Nenokkadine in Blu-Ray rip quality instead of buying or renting a Blu-Ray disc , you will need to follow some steps and tips. However, before we get into that , we want to warn you about some legal and ethical issues related to downloading movies online.
-
Legal and ethical issues of downloading movies
-
Downloading movies online without paying for them is illegal in most countries. It is considered a form of piracy , which violates the intellectual property rights of the creators and distributors of the movies. Piracy can result in legal consequences such as fines , lawsuits , or even jail time . It can also harm the movie industry by reducing its revenue and discouraging its creativity . Therefore , we do not encourage or endorse downloading movies illegally . We only provide this information for educational purposes . If you decide to download movies online , you do so at your own risk . We are not responsible for any legal or ethical problems that may arise from your actions .
-
The best way to watch movies legally online is to use legitimate streaming services such as Netflix , Amazon Prime Video , Hulu , Disney+ , etc . These services offer a wide range of movies and
0a6ba089eb
-
-
\ No newline at end of file
diff --git a/spaces/1acneusushi/gradio-2dmoleculeeditor/data/Descargar Pelicula Completa Iq Formula Para Amar.epub no te pierdas esta pelcula llena de humor y emocin.md b/spaces/1acneusushi/gradio-2dmoleculeeditor/data/Descargar Pelicula Completa Iq Formula Para Amar.epub no te pierdas esta pelcula llena de humor y emocin.md
deleted file mode 100644
index f5b8b114688e3cfb6c6c2a28e998de971eb938ca..0000000000000000000000000000000000000000
--- a/spaces/1acneusushi/gradio-2dmoleculeeditor/data/Descargar Pelicula Completa Iq Formula Para Amar.epub no te pierdas esta pelcula llena de humor y emocin.md
+++ /dev/null
@@ -1,147 +0,0 @@
-
-
Descargar Pelicula Completa Iq Formula Para Amar.epub: Una comedia romántica con un toque de genialidad
-
Si te gustan las comedias románticas con un poco de ciencia y humor, te recomendamos que descargues la película completa Iq Formula Para Amar.epub. Esta película, estrenada en 1994, cuenta la historia de un mecánico que se enamora de la sobrina de Albert Einstein, y cómo el famoso científico y sus amigos intentan ayudarlo a conquistarla. Te contamos todo lo que necesitas saber sobre esta divertida y entrañable película, y cómo puedes descargarla en formato epub para disfrutarla en tu dispositivo favorito.
-
¿De qué trata la película?
-
La trama: un mecánico se enamora de la sobrina de Einstein
-
La película se ambienta en los años 50, en Princeton, Nueva Jersey. Ed Walters (Tim Robbins) es un mecánico de automóviles que se queda prendado de Catherine Boyd (Meg Ryan), una bella y brillante matemática que resulta ser la sobrina de Albert Einstein (Walter Matthau). Ed se las arregla para conocerla y entablar una amistad con ella, pero pronto se da cuenta de que tiene un problema: Catherine está comprometida con James Moreland (Stephen Fry), un profesor arrogante y pretencioso que no la valora ni la respeta.
-
Descargar Pelicula Completa Iq Formula Para Amar.epub
Ed no se rinde y decide luchar por el amor de Catherine, pero no está solo. Resulta que Einstein y sus colegas científicos (Lou Jacobi, Gene Saks y Joseph Maher) también están hartos de Moreland y quieren que Catherine sea feliz con alguien más. Así que deciden intervenir en el asunto y hacer pasar a Ed por un genio de la física, usando sus conocimientos y sus trucos para impresionar a Catherine y a su familia. Pero el plan no será tan fácil como parece, y habrá muchos obstáculos y enredos por el camino.
-
Los personajes: Tim Robbins, Meg Ryan y Walter Matthau
-
La película cuenta con un reparto de lujo, encabezado por Tim Robbins, Meg Ryan y Walter Matthau. Tim Robbins interpreta a Ed Walters, el simpático y honesto mecánico que se enamora perdidamente de Catherine. Robbins logra transmitir el carisma y la ternura de su personaje, así como su ingenio y su valentía para enfrentarse a los desafíos que le plantea el amor. Meg Ryan interpreta a Catherine Boyd, la inteligente y hermosa matemática que vive a la sombra de su tío Einstein. Ryan consigue darle a su personaje una mezcla de dulzura y determinación, así como una evolución desde la inseguridad hasta la confianza en sí misma. Walter Matthau interpreta a Albert Einstein, el genial y excéntrico científico que quiere lo mejor para su sobrina. Matthau hace una magnífica interpretación del famoso físico, mostrando su humor, su sabiduría y su humanidad.
-
El director: Fred Schepisi
-
La película está dirigida por Fred Schepisi, un cineasta australiano que ha trabajado en diversos géneros y países. Schepisi ha dirigido películas como Roxanne (1987), Seis grados de separación (1993) o La última orden (2011), entre otras. Schepisi demuestra su habilidad para combinar el romance, la comedia y el drama en esta película, creando una atmósfera cálida y divertida, pero también emotiva y profunda. Schepisi también cuida los aspectos técnicos de la película, como la fotografía, el vestuario o la música, recreando con fidelidad la época en la que se ambienta.
-
¿Por qué descargar la película en formato epub?
-
Las ventajas del formato epub
-
Si quieres ver esta película en tu dispositivo móvil o tu ordenador, te recomendamos que la descargues en formato epub. El formato epub es un formato estándar para libros electrónicos que tiene muchas ventajas sobre otros formatos como el pdf o el mp4. Algunas de estas ventajas son:
-
-
El formato epub es compatible con casi todos los dispositivos y aplicaciones de lectura electrónica.
-
El formato epub permite ajustar el tamaño y el tipo de letra, el brillo, el contraste o el color de fondo según tus preferencias.
-
El formato epub se adapta al tamaño y la orientación de tu pantalla, lo que facilita la lectura y evita tener que hacer zoom o desplazarte.
-
El formato epub tiene una menor ocupación de espacio que otros formatos, lo que te permite almacenar más archivos en tu memoria.
-
El formato epub conserva mejor la calidad de imagen y sonido que otros formatos comprimidos.
-
-
Los sitios web donde se puede descargar la película
-
Ahora que ya sabes las ventajas del formato epub, te preguntarás dónde puedes descargar la película completa Iq Formula Para Amar.epub. Hay muchos sitios web donde puedes encontrar esta película en este formato, pero no todos son seguros ni legales. Por eso te recomendamos que uses solo sitios web confiables y autorizados para descargar esta película. Algunos de estos sitios web son:
- Es uno de los sitios web más populares y fiables para comprar libros electrónicos. - Ofrece una gran variedad de títulos y géneros. - Tiene precios competitivos y ofertas frecuentes. - Permite leer los libros electrónicos en cualquier dispositivo con la aplicación Kindle.
-
- Hay que pagar por cada libro electrónico que se quiera descargar. - No todos los libros electrónicos están disponibles en todos los países. - No todos los libros electrónicos tienen una buena calidad o una buena traducción.
- Es uno de los sitios web más conocidos y usados para descargar películas gratis. - Ofrece una gran cantidad de películas en diferentes formatos e idiomas. - Tiene una interfaz sencilla e intuitiva. - Permite ver las películas online o descargarlas al ordenador.
-
- No es un sitio web legal ni seguro. - Puede contener virus o malware. - Puede violar los derechos de autor o las normas de propiedad intelectual. - Puede tener problemas con las descargas o las reproducciones.
-
-
-
Los requisitos para ver la película en epub
-
Para poder ver la película completa Iq Formula Para Amar.epub en tu dispositivo, necesitas cumplir algunos requisitos. Estos son:
-
-
Tener un dispositivo compatible con el formato epub, como un ordenador, una tablet, un smartphone o un lector de libros electrónicos.
-
Tener una aplicación o un programa que pueda reproducir archivos epub, como Calibre, Adobe Digital Editions, iBooks o Kindle.
-
Tener una conexión a internet para poder descargar la película desde el sitio web que elijas.
-
Tener espacio suficiente en tu memoria para almacenar el archivo epub, que suele tener un tamaño de unos pocos megabytes.
-
-
Si cumples estos requisitos, podrás disfrutar de la película en formato epub sin problemas.
-
Descargar I.Q. El Genio del Amor en Latino
-Ver IQ Fórmula para amar Online HD
-El genio del amor 1994 Película Online Gratis HD
-Formula Para Amar 1994 Comedia Romántica
-I.Q. 1994 Película Completa en Castellano
-Descargar El genio del amor Torrent
-Ver Película I.Q. Fórmula para amar Gratis
-El genio del amor película de Meg Ryan y Tim Robbins
-I.Q. El Genio del Amor Fred Schepisi
-Descargar Película I.Q. en Español Latino
-Ver IQ Fórmula para amar Subtitulada
-El genio del amor con Walter Matthau como Einstein
-I.Q. 1994 Película de Amor y Ciencia
-Descargar El genio del amor Mega
-Ver Película I.Q. Online Sin Cortes
-El genio del amor 1994 DVDrip Latino
-I.Q. Fórmula para amar Película Completa
-El genio del amor Descarga Directa
-Ver Película I.Q. Gratis en HD
-El genio del amor 1994 Audio Latino
-I.Q. El Genio del Amor Película de Comedia
-Descargar El genio del amor por Mediafire
-Ver Película I.Q. Fórmula para amar en Netflix
-El genio del amor 1994 Reparto y Sinopsis
-I.Q. El Genio del Amor Crítica y Opiniones
-Descargar El genio del amor 1080p Latino
-Ver Película I.Q. Fórmula para amar en Youtube
-El genio del amor 1994 Trailer Oficial
-I.Q. El Genio del Amor Banda Sonora Original
-Descargar El genio del amor 720p Latino
-Ver Película I.Q. Fórmula para amar en Amazon Prime Video
-El genio del amor 1994 Curiosidades y Anécdotas
-I.Q. El Genio del Amor Premios y Nominaciones
-Descargar El genio del amor DVDRip Latino
-Ver Película I.Q. Fórmula para amar en Hulu
-El genio del amor 1994 Escenas Eliminadas
-I.Q. El Genio del Amor Frases y Diálogos
-Descargar El genio del amor BluRay Rip Latino
-Ver Película I.Q. Fórmula para amar en Disney Plus
-El genio del amor 1994 Imágenes y Fotos
-I.Q. El Genio del Amor Guion Original
-Descargar El genio del amor HD Latino
-Ver Película I.Q. Fórmula para amar en HBO Max
-El genio del amor 1994 Cartel y Póster
-I.Q. El Genio del Amor Edición Especial
-
¿Qué opinan los críticos y el público de la película?
-
Las reseñas positivas de la película
-
La película Iq Formula Para Amar ha recibido muchas reseñas positivas de los críticos y el público que la han visto. Algunas de estas reseñas son:
-
-
"Una comedia romántica inteligente y encantadora, con un reparto estelar y una historia original y divertida. Tim Robbins y Meg Ryan tienen una química irresistible, y Walter Matthau está genial como Einstein. Una película que te hará reír y soñar".
-Roger Ebert, Chicago Sun-Times
-
-
-
"Una película deliciosa y entrañable, que combina el humor, el amor y la ciencia de una forma magistral. La película tiene un ritmo ágil y una dirección impecable, y los actores están fantásticos en sus papeles. Una película que te hará pasar un buen rato".
-Janet Maslin, The New York Times
-
-
-
"Una película que te sorprenderá por su originalidad y su ternura. La película tiene un guion ingenioso y unos diálogos chispeantes, y los actores hacen un trabajo maravilloso. Walter Matthau está sublime como Einstein, y Tim Robbins y Meg Ryan forman una pareja adorable. Una película que te enamorará".
-Leonard Maltin, Entertainment Tonight
-
-
Las reseñas negativas de la película
-
La película Iq Formula Para Amar también ha recibido algunas reseñas negativas de los críticos y el público que la han visto. Algunas de estas reseñas son:
-
-
"Una comedia romántica tonta y aburrida, con un argumento absurdo y unos personajes planos. Tim Robbins y Meg Ryan no tienen ninguna gracia ni carisma, y Walter Matthau está desaprovechado como Einstein. Una película que te hará bostezar y mirar el reloj".
-Peter Travers, Rolling Stone
-
-
-
"Una película decepcionante y predecible, que desperdicia una idea interesante y un reparto de lujo. La película tiene un tono cursi e infantil, y los actores están sobreactuados y poco creíbles. Walter Matthau hace una caricatura de Einstein, y Tim Robbins y Meg Ryan no tienen química ni encanto. Una película que te hará perder el tiempo".
-Rita Kempley, The Washington Post
-
-
-
"Una película mediocre y olvidable, que no aprovecha ni el humor ni el romance ni la ciencia. La película tiene un guion flojo y unos diálogos insulsos, y los actores están desganados y sin gracia. Walter Matthau está irreconocible como Einstein, y Tim Robbins y Meg Ryan no tienen ni chispa ni emoción. Una película que te hará arrepentirte de haberla visto".
-Owen Gleiberman, Entertainment Weekly
-
-
El impacto cultural de la película
-
A pesar de las reseñas negativas, la película Iq Formula Para Amar ha tenido un impacto cultural considerable desde su estreno. La película ha sido vista por millones de personas en todo el mundo, y ha sido nominada a varios premios, como el Globo de Oro al mejor actor de comedia o musical para Walter Matthau. La película también ha sido referenciada o parodiada en otros medios, como series de televisión, cómics o videojuegos. Algunos ejemplos son:
-
-
En la serie Los Simpson, en el episodio "La guerra secreta de Lisa Simpson", Bart ve la película en clase de ciencias y se burla de ella.
-
En la serie Futurama, en el episodio "El gran golpe de Bender", Bender se disfraza de Einstein para engañar a Fry.
-
En el cómic Calvin y Hobbes, en la tira del 15 de febrero de 1995, Calvin le pregunta a Hobbes si cree que Einstein se enamoró alguna vez.
-
En el videojuego Half-Life 2, en el capítulo "Una trampa rota", hay un científico llamado Isaac Kleiner que tiene un aspecto similar al de Einstein.
-
-
Conclusión
-
Iq Formula Para Amar es una comedia romántica con un toque de genialidad que te hará pasar un rato divertido y emocionante. La película cuenta la historia de un mecánico que se enamora de la sobrina de Einstein, y cómo el científico y sus amigos intentan ayudarlo a conquistarla. La película tiene un reparto excelente encabezado por Tim Robbins, Meg Ryan y Walter Matthau, y una dirección impecable por parte de Fred Schepisi. La película ha recibido tanto reseñas positivas como negativas por parte de los críticos y el público, pero ha tenido un impacto cultural notable desde su estreno.
-
Si quieres ver esta película en tu dispositivo móvil o tu ordenador, te recomendamos que la descargues en formato epub desde alguno de los sitios web confiables y autorizados que te hemos mencionado. El formato epub tiene muchas ventajas sobre otros formatos como el pdf o el mp4, ya que te permite ajustar la lectura a tus preferencias y conserva mejor la calidad de imagen y sonido.
-
No esperes más y descarga ya la película completa Iq Formula Para Amar.epub para disfrutarla cuando quieras.
-
Preguntas frecuentes
-
-
¿Qué significa el título Iq Formula Para Amar? El título hace referencia al coeficiente intelectual (IQ) que se supone que mide la inteligencia de las personas. En la película se juega con la idea de que Ed tiene un IQ muy alto gracias a las ayudas de Einstein y sus amigos.
-
¿Qué relación tiene Einstein con Catherine? Einstein es el tío materno de Catherine. Su madre era hermana del científico.
-
¿Qué es un magnet link? Un magnet link es un tipo especial de enlace que permite descargar archivos torrent sin necesidad de tener un archivo físico. Los magnet links contienen información sobre el nombre del archivo, su tamaño o su código hash.
-
¿Qué es Calibre? Calibre es una aplicación gratuita y multiplataforma que permite gestionar libros electrónicos en diferentes formatos. Con Calibre se puede organizar una biblioteca digital, convertir libros a otros formatos o sincronizarlos con dispositivos externos.
-
¿Qué otros libros o películas hay sobre Einstein? Einstein ha sido protagonista o personaje secundario de muchos libros o películas a lo largo de la historia. Algunos ejemplos son: El universo en una cáscara de nuez (Stephen Hawking), Einstein: su vida y su universo (Walter Isaacson), Genius (serie biográfica), El joven Einstein (película cómica) o IQ (película romántica).
-
- 0a6ba089eb
-
-
\ No newline at end of file
diff --git a/spaces/1gistliPinn/ChatGPT4/Examples/Aescripts StageTool 1.3.md b/spaces/1gistliPinn/ChatGPT4/Examples/Aescripts StageTool 1.3.md
deleted file mode 100644
index 2e09112299242a7246f1d13940cdd972c553bf1f..0000000000000000000000000000000000000000
--- a/spaces/1gistliPinn/ChatGPT4/Examples/Aescripts StageTool 1.3.md
+++ /dev/null
@@ -1,39 +0,0 @@
-
-`
Aescripts StageTool 1.3: A Powerful Tool for Creating Pixel Mappings in After Effects
`
-
-`
If you are a VJ who wants to create stunning pixel mappings for your performances, you might be interested in Aescripts StageTool 1.3. This is a plugin for Adobe After Effects that lets you easily create and position LED slices to match your stage, and export them to Resolume Arena 5, 6 and 7.
Create multiple output compositions with different resolutions and aspect ratios.
`
-`
Specify the properties of each slice, such as width, height, gap, rotation, color and logo.
`
-`
Use negative tiles to remove unwanted parts from your slice.
`
-`
Get live feedback of your slice with checkerboard, outlines, cross indicator and more.
`
-`
Screenshot your mask, input and output compositions for easy reference.
`
-`
Export XML files to Resolume Arena with rectangle masks included.
`
-`
`
-
-`
Aescripts StageTool 1.3 is compatible with After Effects CC 2018 and above. It requires a license that costs $56.00. You can also try it for free with some limitations, such as a maximum of 3 slices and no export function.
`
-
-`
To learn more about Aescripts StageTool 1.3 and see some examples of pixel mappings created with it, you can visit the official website[^1^] or watch the tutorial video[^2^].
`
-
-`
Pixel mapping is a technique that allows you to control individual pixels or groups of pixels on a LED screen or panel. By using pixel mapping, you can create dynamic and colorful patterns, animations and effects that enhance your VJ performances and visuals.
`
-
-`
Pixel mapping can be done in 2D or 3D, depending on the shape and layout of your LED screen or panel. You can use pixel mapping to create flat or curved surfaces, as well as volumetric shapes and structures. Pixel mapping can also be combined with video mapping, projection mapping and lighting effects to create immersive and interactive environments.
`
-
-`
To use pixel mapping, you need a software that can generate and send pixel data to your LED screen or panel. Aescripts StageTool 1.3 is one of the best options for pixel mapping in After Effects. It allows you to create pixel mappings with ease and flexibility, and export them to Resolume Arena, a popular VJ software that can play back your pixel mappings in real time.
`
-
-`
Some examples of pixel mapping applications are:
-`
-
-`
`
-`
Creating 1:1 pixel mappings for LCD monitors and plasma displays, which avoids loss of sharpness and incorrect aspect ratio due to scaling and stretching[^1^].
`
-`
Creating DMX pixel mappings for LED screens and panels, which enables users to control individual pixels or groups of pixels with different colors and effects[^2^].
`
-`
Creating bitmap pixel mappings for digital images, which allows users to store and manipulate pixels with different bits and channels[^3^].
`
-`
`
-
-`
Pixel mapping is a versatile and creative technique that can be used for various purposes and projects. Whether you want to create simple or complex pixel mappings, Aescripts StageTool 1.3 can help you achieve your goals with ease and efficiency.
` d5da3c52bf
-
-
\ No newline at end of file
diff --git a/spaces/1pelhydcardo/ChatGPT-prompt-generator/assets/Aprende a conseguir gemas infinitas en Clash Royale con Apkteca. com.md b/spaces/1pelhydcardo/ChatGPT-prompt-generator/assets/Aprende a conseguir gemas infinitas en Clash Royale con Apkteca. com.md
deleted file mode 100644
index 7d2441d9c76cb87ea23fbd9f279940d3fd399ceb..0000000000000000000000000000000000000000
--- a/spaces/1pelhydcardo/ChatGPT-prompt-generator/assets/Aprende a conseguir gemas infinitas en Clash Royale con Apkteca. com.md
+++ /dev/null
@@ -1,158 +0,0 @@
-
-
Clash Royale: A Guide to the Ultimate Strategy Game
-
If you are looking for a fun, addictive, and competitive multiplayer game that combines strategy, cards, and real-time battles, then you should definitely try Clash Royale. This game is developed by Supercell, the same company that created the popular Clash of Clans. In this guide, we will tell you everything you need to know about Clash Royale, from the basics of the game to the advanced tips and tricks that will help you win more battles and trophies. We will also show you how to use Clash Royale mod apk to get unlimited gems and coins, as well as the benefits and risks of doing so. Let's get started!
Clash Royale is a real-time strategy game that features your favorite characters from Clash of Clans, as well as many new ones. The game is set in a fantasy world where you can collect and upgrade dozens of cards that represent different troops, spells, buildings, and heroes. You can use these cards to build your own battle deck and challenge other players from around the world in fast-paced matches that last for three minutes. The goal is to destroy your opponent's three crown towers, or at least more than they destroy yours, before the time runs out.
-
The basics of the game
-
The game is divided into several arenas, each with a different theme and difficulty level. You start in the Training Camp, where you can learn the basic mechanics of the game and unlock some common cards. As you win battles and trophies, you can progress to higher arenas and unlock more cards, as well as chests, gold, gems, and other rewards. You can also lose trophies if you lose battles, so be careful not to drop too low.
-
The game uses a resource called elixir, which is generated automatically over time. You need elixir to play cards from your hand, which have different costs depending on their rarity and power. You can have up to four cards in your hand at a time, and you can draw a new card from your deck whenever you play one. You can also cycle your cards by playing them in the back of your arena, but be careful not to waste elixir or leave yourself vulnerable.
-
The main features of the game
-
Clash Royale has many features that make it an exciting and diverse game. Some of them are:
-
apkteca. com clash royale hack gemas ilimitadas
-apkteca. com clash royale mod apk gemas gratis
-apkteca. com clash royale descargar con gemas infinitas
-apkteca. com clash royale trucos para conseguir gemas
-apkteca. com clash royale como tener gemas infinitas
-apkteca. com clash royale actualizado con gemas infinitas
-apkteca. com clash royale servidor privado gemas infinitas
-apkteca. com clash royale apk modificado con gemas infinitas
-apkteca. com clash royale generador de gemas online
-apkteca. com clash royale hackear el juego con gemas infinitas
-apkteca. com clash royale descargar ultima version con gemas infinitas
-apkteca. com clash royale obtener gemas gratis sin verificacion
-apkteca. com clash royale como hackear el juego con gemas infinitas
-apkteca. com clash royale descargar gratis para android con gemas infinitas
-apkteca. com clash royale como conseguir gemas rapido y facil
-apkteca. com clash royale descargar mod apk con gemas ilimitadas
-apkteca. com clash royale como instalar el juego con gemas infinitas
-apkteca. com clash royale como jugar con gemas infinitas sin root
-apkteca. com clash royale descargar hack apk con gemas ilimitadas
-apkteca. com clash royale como ganar gemas gratis en el juego
-apkteca. com clash royale descargar para pc con gemas infinitas
-apkteca. com clash royale como descargar el juego con gemas infinitas
-apkteca. com clash royale como obtener gemas infinitas sin hack
-apkteca. com clash royale descargar para ios con gemas infinitas
-apkteca. com clash royale como tener gemas gratis sin descargar nada
-apkteca. com clash royale descargar apk hackeado con gemas ilimitadas
-apkteca. com clash royale como conseguir gemas gratis sin encuestas
-apkteca. com clash royale descargar para tablet con gemas infinitas
-apkteca. com clash royale como tener gemas infinitas en el juego
-apkteca. com clash royale descargar apk mod con gemas ilimitadas
-apkteca. com clash royale como conseguir gemas gratis sin root
-apkteca. com clash royale descargar para celular con gemas infinitas
-apkteca. com clash royale como tener gemas gratis en el juego
-apkteca. com clash royale descargar apk original con gemas infinitas
-apkteca. com clash royale como conseguir gemas gratis sin hackear
-apkteca. com clash royale descargar para laptop con gemas infinitas
-apkteca. com clash royale como tener gemas gratis y rapido
-apkteca. com clash royale descargar apk actualizado con gemas ilimitadas
-apkteca. com clash royale como conseguir gemas gratis sin descargar nada
-apkteca. com clash royale descargar para windows con gemas infinitas
-apkteca. com clash royale como tener gemas gratis y facilmente
-
-
Real-time multiplayer battles: You can battle against players from all over the world in real-time matches that are fast, fun, and unpredictable. You can also play friendly matches with your clanmates or practice against bots.
-
Card collection and upgrade: You can collect over 100 cards that feature different types of units, such as melee, ranged, flying, building-targeting, splash-damaging, etc. You can also upgrade your cards to make them stronger and unlock new abilities.
-
Deck building and customization: You can build your own battle deck with eight cards of your choice, depending on your preferred playstyle and strategy. You can also create different decks for different game modes and situations.
-
Game modes and challenges: You can play different game modes and challenges that offer variety and rewards. Some of them are:
-
-that contain cards, gold, gems, and other items. The higher your arena, the better the chests.
-
2v2: This is a mode where you can team up with another player or a friend and battle against another pair of players. You can share elixir and cards with your teammate and coordinate your moves.
-
Special events: These are temporary modes that have different rules and objectives, such as draft, triple elixir, sudden death, etc. You can earn special rewards and tokens by participating in these events.
-
Tournaments: These are custom matches that you can create or join with other players. You can set the rules, prizes, and duration of the tournaments.
-
Clan wars: These are clan-based competitions that last for two days. On the first day, you can play different game modes to earn clan cards and war trophies. On the second day, you can use the clan cards to build your war deck and battle against other clans.
-
-
-
How to play Clash Royale?
-
Now that you know what Clash Royale is and what it offers, let's see how you can play it and become a better player. Here are some of the most important aspects of the game that you need to master:
-
How to build your deck
-
Your deck is your main tool for winning battles. It consists of eight cards that you can choose from your card collection. You can have up to five different decks at a time, and you can switch between them before each battle. Building a good deck is not easy, but it is not impossible either. Here are some tips to help you:
-
-
Balance your elixir cost: Your elixir cost is the average amount of elixir that you need to play your cards. You want to have a balanced elixir cost that allows you to play your cards efficiently and not run out of elixir or have too much elixir. A good range for your elixir cost is between 3.0 and 4.5.
-
Balance your card types: Your card types are the categories that your cards belong to, such as troops, spells, buildings, etc. You want to have a balanced mix of card types that can deal with different situations and threats. A good rule of thumb is to have at least two spells, one building, and five troops in your deck.
-
Balance your roles and synergies: Your roles are the functions that your cards perform in your deck, such as win condition, support, defense, etc. You want to have a clear win condition that can deal damage to your opponent's towers, as well as support and defense cards that can protect and enhance your win condition. You also want to have synergies between your cards, which are combinations that work well together and create positive elixir trades or tower damage.
-
-
The best cards for each arena
-
The game has 13 arenas, each with its own theme and card pool. As you progress through the arenas, you will unlock new cards that can improve your deck and gameplay. However, not all cards are equally good in every arena. Some cards are more effective in lower arenas, while others are more useful in higher arenas. Here are some of the best cards for each arena:
- | Arena | Best Cards | | --- | --- | | Training Camp | Giant, Musketeer, Fireball | | Goblin Stadium | Goblin Barrel, Valkyrie, Skeleton Army | | Bone Pit | Baby Dragon, Tombstone, Balloon | | Barbarian Bowl | Hog Rider, Barbarians, Cannon | | P.E.K.K.A's Playhouse | P.E.K.K.A., Wizard, Zap | | Spell Valley | Fire Spirits, Furnace, Poison | | Builder's Workshop | Miner, Mortar, Tesla | | Royal Arena | Elite Barbarians, Royal Giant, Mega Minion | | Frozen Peak | Ice Spirit, Ice Golem, Bowler | Executioner | | Hog Mountain | Tornado, Royal Hogs, Inferno Dragon | | Electro Valley | Electro Wizard, Sparky, Zappies | | Spooky Town | Skeleton Barrel, Witch, Graveyard | | Legendary Arena | Lava Hound, Night Witch, The Log |
The best decks for different game modes
-
The game has different game modes that require different strategies and decks. Some of the most popular game modes are:
-
-
Classic and Grand Challenges: These are modes where you can pay gems to enter and win up to 12 matches before losing three. You can win huge rewards and cards by completing these challenges. The best decks for these modes are usually meta decks that are proven to be effective and consistent in the current game state. You can find these decks on websites like RoyaleAPI or Deck Shop.
-
Draft: This is a mode where you and your opponent take turns to choose from four cards each. You have to build your deck on the spot with the cards that you pick. The best decks for this mode are usually balanced decks that have a good mix of card types, roles, and synergies. You also have to pay attention to what cards your opponent picks and try to counter them.
-
Triple Elixir: This is a mode where you and your opponent generate elixir three times faster than normal. You have to play your cards quickly and aggressively to overwhelm your opponent. The best decks for this mode are usually heavy decks that have high-cost and high-impact cards, such as Golem, P.E.K.K.A., or Three Musketeers.
-
-
How to win battles
-
Winning battles in Clash Royale is not only about having a good deck, but also about knowing how to play it well. You have to be smart, fast, and adaptable to the changing situations of the game. Here are some tips to help you win more battles:
-
-
Know your win condition: Your win condition is the card or combination of cards that can deal the most damage to your opponent's towers. You have to know what your win condition is and how to use it effectively. You also have to know what your opponent's win condition is and how to stop it or prevent it.
-
Manage your elixir: Your elixir is your most valuable resource in the game. You have to use it wisely and efficiently. You have to avoid wasting elixir or overcommitting elixir on unnecessary or risky moves. You also have to try to gain an elixir advantage over your opponent by making positive elixir trades or applying pressure.
-
Control the tempo: The tempo is the pace and rhythm of the game. You have to control the tempo by dictating when and where the action happens. You have to adapt your tempo according to your deck and your opponent's deck. You can play fast or slow, aggressive or defensive, depending on what suits you best.
-
Use the right placement and timing: The placement and timing of your cards can make a huge difference in the outcome of the game. You have to use the right placement and timing for your cards to maximize their potential and minimize their weaknesses. You also have to use the right placement and timing for your spells to hit as many targets as possible or avoid hitting your own units.
-
-
The best tips and tricks for attacking and defending
-
Attacking and defending are the two main aspects of the game that you have to master. Here are some of the best tips and tricks for attacking and defending:
-
-
Attacking tips:
-
-
Use split pushes: A split push is when you attack both lanes at the same time with different units. This can confuse your opponent and force them to split their defense or choose one lane to defend.
-
Use counter pushes: A counter push is when you use the surviving units from your defense to launch an attack on the opposite lane. This can catch your opponent off guard and create a strong push with minimal elixir.
-
Use prediction spells: A prediction spell is when you cast a spell on a spot where you expect your opponent to play a card before they actually do. This can surprise your opponent and give you an edge in the battle.
-
-
Defending tips:
-
-Use kiting and pulling: Kiting and pulling are techniques that involve using a low-cost or fast-moving unit to lure your opponent's units away from your tower or into the range of your other units or spells. This can help you deal with high-damage or tanky units more easily.
-
Use distractions and diversions: Distractions and diversions are techniques that involve using a cheap or expendable unit to distract your opponent's units from attacking your tower or your main defense. This can help you buy time or reduce damage from your opponent's push.
-
Use the king tower activation: The king tower activation is when you use a card or a spell to make your opponent's unit hit your king tower, which is normally inactive until it is damaged. This can help you activate your king tower, which will provide extra damage and defense for the rest of the game.
-
-
-
The best strategies for different archetypes and matchups
-
The game has different archetypes, which are categories of decks that have similar characteristics and playstyles. Some of the most common archetypes are:
-
-
Beatdown: These are decks that rely on building a big push with a tanky unit, such as Golem or Giant, and supporting it with other units and spells. They are good at breaking through defenses and taking down towers, but they are weak against fast and cheap decks that can outcycle and pressure them.
-
Cycle: These are decks that rely on cycling through their cards quickly and playing them at the right moment. They are good at applying constant pressure and chip damage, but they are weak against heavy and splash decks that can counter their units easily.
-
Control: These are decks that rely on controlling the tempo and elixir of the game and playing defensively until they can launch a counterattack. They are good at defending and making positive elixir trades, but they are weak against siege and spell decks that can bypass their defense.
-
Siege: These are decks that rely on using long-range units, such as X-Bow or Mortar, to attack the opponent's towers from their side of the arena. They are good at keeping a distance and punishing mistakes, but they are weak against beatdown and swarm decks that can overwhelm them.
-
-
The game also has different matchups, which are combinations of archetypes that face each other in a battle. Some matchups are favorable, meaning that one archetype has an advantage over another, while others are unfavorable, meaning that one archetype has a disadvantage over another. Here are some examples of favorable and unfavorable matchups:
- | Favorable Matchups | Unfavorable Matchups | | --- | --- | | Beatdown vs Control | Beatdown vs Cycle | | Cycle vs Siege | Cycle vs Control | | Control vs Siege | Control vs Beatdown | | Siege vs Beatdown | Siege vs Cycle |
How to get more out of Clash Royale?
-
Clash Royale is a game that offers a lot of content and features for its players. However, there are some ways to get even more out of the game, such as joining a clan, unlocking seasonal rewards, and using magic items. There is also a way to get unlimited gems and coins by using Clash Royale mod apk, which is a modified version of the game that allows you to access premium features for free. Let's see how you can do these things:
-
How to join a clan and participate in clan wars
-
A clan is a group of players that can chat, donate cards, request cards, and play together in clan wars. Joining a clan is one of the best ways to improve your skills, make friends, and get more rewards. You can join a clan by searching for one in the game or by accepting an invitation from another player. You can also create your own clan if you have enough gems.
-
A clan war is a clan-based competition that lasts for two days. On the first day, you can play different game modes to earn clan cards and war trophies. On the second day, you can use the clan cards to build your war deck and battle against other clans. You can earn war chests, gold, gems, and other rewards by winning clan wars.
-
How to unlock and use seasonal rewards and magic items
-royale, which is a premium feature that gives you access to exclusive rewards and perks, such as unlimited entries, queue chests, and strike chests.
-
A magic item is a special item that can help you upgrade your cards faster and easier. There are different types of magic items, such as books, chests, tokens, and wild cards. You can get magic items by opening chests, completing quests, participating in events, or buying them with gems.
-
How to use Clash Royale mod apk for unlimited gems and coins
-
Gems and coins are the two main currencies in the game. Gems can be used to buy chests, cards, gold, passes, emotes, and other items. Coins can be used to upgrade your cards and buy cards from the shop. You can earn gems and coins by playing the game, opening chests, completing quests, or buying them with real money.
-
However, if you want to get unlimited gems and coins for free, you can use Clash Royale mod apk, which is a modified version of the game that gives you access to unlimited resources and features. Here are some of the benefits and risks of using mod apk:
-
The benefits and risks of using mod apk
-
Using mod apk can have some benefits, such as:
-
-
You can get unlimited gems and coins: This can help you unlock and upgrade all the cards in the game, as well as buy anything you want from the shop or the pass royale.
-
You can get unlimited resources and features: This can help you enjoy the game without any limitations or restrictions. You can play any game mode or challenge as many times as you want, as well as use any card or deck that you like.
-
You can have more fun and excitement: This can help you explore the game in a new way and experience new things that you might not be able to do in the original version of the game.
-
-
However, using mod apk can also have some risks, such as:
-
-
You can get banned from the game: This is the most serious risk of using mod apk. Supercell, the developer of the game, has a strict policy against cheating and hacking. If they detect that you are using mod apk or any other unauthorized software, they can ban your account permanently and prevent you from playing the game ever again.
-
You can get viruses or malware on your device: This is another serious risk of using mod apk. Some of the sources that provide mod apk may not be safe or reliable. They may contain viruses or malware that can harm your device or steal your personal information.
-
You can lose the fun and challenge of the game: This is a personal risk of using mod apk. Some of the players may feel that using mod apk takes away the fun and challenge of the game. They may feel that it is not fair or rewarding to play with unlimited resources and features that give them an unfair advantage over other players.
-
-
The best sources and steps for downloading and installing mod apk
-
If you still want to use mod apk despite the risks, you have to be careful and smart about it. You have to find a trustworthy source that provides a safe and working mod apk file. You also have to follow the right steps for downloading and installing mod apk on your device. Here are some of the best sources and steps for doing so:
- | Source | Steps | | --- | --- | | Apkteca.com | 1. Go to apkteca.com on your browser. 2. Search for Clash Royale mod apk. 3. Choose the latest version of the mod apk file. 4. Click on the download button and wait for it to finish. 5. Go to your device settings and enable unknown sources. 6. Go to your file manager and locate the downloaded mod apk file. 7. Tap on it and follow the instructions to install it. 8. Enjoy the game with unlimited gems and coins. | the latest version of the mod apk file. 4. Click on the download button and wait for it to finish. 5. Go to your device settings and enable unknown sources. 6. Go to your file manager and locate the downloaded mod apk file. 7. Tap on it and follow the instructions to install it. 8. Enjoy the game with unlimited gems and coins. | | Apkmodhub.com | 1. Go to apkmodhub.com on your browser. 2. Search for Clash Royale mod apk. 3. Choose the latest version of the mod apk file. 4. Click on the download button and wait for it to finish. 5. Go to your device settings and enable unknown sources. 6. Go to your file manager and locate the downloaded mod apk file. 7. Tap on it and follow the instructions to install it. 8. Enjoy the game with unlimited gems and coins. |
Conclusion
-
Clash Royale is a game that can provide you with hours of fun and entertainment, as well as challenge and satisfaction. It is a game that requires skill, strategy, and creativity, as well as luck and patience. It is a game that can be enjoyed by anyone, regardless of age, gender, or background.
-
In this guide, we have covered everything you need to know about Clash Royale, from the basics of the game to the advanced tips and tricks that will help you win more battles and trophies. We have also shown you how to use Clash Royale mod apk to get unlimited gems and coins, as well as the benefits and risks of doing so.
-
We hope that this guide has been helpful and informative for you, and that you have learned something new and useful from it. We also hope that you have enjoyed reading it as much as we have enjoyed writing it.
-
Now that you have read this guide, you are ready to play Clash Royale like a pro and have a blast with it. So what are you waiting for? Download Clash Royale now and join the millions of players who are already hooked on this amazing game!
-
Frequently Asked Questions
-
Here are some of the most frequently asked questions about Clash Royale:
-
-
Q: Is Clash Royale free to play? A: Yes, Clash Royale is free to play and download on both Android and iOS devices. However, it also offers in-app purchases that can enhance your gameplay and experience.
-
Q: Is Clash Royale a pay-to-win game? A: No, Clash Royale is not a pay-to-win game. Although paying money can help you progress faster and unlock more cards and features, it does not guarantee you victory or success. You still need skill, strategy, and practice to win battles and trophies.
-
Q: Is Clash Royale a fair game? A: Yes, Clash Royale is a fair game. The game uses a matchmaking system that pairs you with players who have similar levels of trophies and cards as you. The game also uses a random card generator that ensures that both you and your opponent have equal chances of getting good or bad cards.
-
Q: Is Clash Royale an online game? A: Yes, Clash Royale is an online game that requires an internet connection to play. You cannot play Clash Royale offline or without wifi.
-
Q: Is Clash Royale a safe game? A: Yes, Clash Royale is a safe game that does not contain any harmful or inappropriate content or ads. However, you should be careful when using mod apk or other third-party software that may compromise your device or account security.
-
197e85843d
-
-
\ No newline at end of file
diff --git a/spaces/1pelhydcardo/ChatGPT-prompt-generator/assets/Download Garena Blockman GO and Join Millions of Players in Various Minigames.md b/spaces/1pelhydcardo/ChatGPT-prompt-generator/assets/Download Garena Blockman GO and Join Millions of Players in Various Minigames.md
deleted file mode 100644
index 0822a2ba94d9915b87b97f43f1936d4635b64c01..0000000000000000000000000000000000000000
--- a/spaces/1pelhydcardo/ChatGPT-prompt-generator/assets/Download Garena Blockman GO and Join Millions of Players in Various Minigames.md
+++ /dev/null
@@ -1,116 +0,0 @@
-
-
How to Download Garena Blockman GO on Your Device
-
If you are looking for a fun and creative sandbox game that lets you play, craft, and share your experiences with your friends, then you should check out Garena Blockman GO. This is a free-to-play game that offers a variety of minigames from different genres, as well as a powerful editor mode that allows you to create and publish your own minigames. In this article, we will show you how to download Garena Blockman GO on your device, as well as some tips and tricks to play it like a pro.
-
What is Garena Blockman GO?
-
Garena Blockman GO is the first sandbox game from Garena, a leading global online games developer and publisher. It was released in July 2021 and has already attracted millions of players from all over the world. Garena Blockman GO is inspired by popular games like Minecraft and Roblox, but it has its own unique features and style.
In Garena Blockman GO, you can explore a wonderland of minigames that are created by other players or by yourself using the editor mode. You can join the adventures and venture into the countless minigames from all the different genres, such as action, adventure, role-playing, strategy, simulation, and more. You can also customize your avatar with creative selections of fashionable accessories, and show off your unique style to the world.
-
Garena Blockman GO is not only a game, but also a platform where you can make friends and chat with other players. You can join a party or a clan to team up with other players, or create your own clan and invite your friends. You can also upgrade your VIP membership to enjoy more benefits and rewards, such as exclusive items, skins, badges, and more.
-
Features of Garena Blockman GO
-
Some of the features that make Garena Blockman GO stand out from other sandbox games are:
-
-
Wonderland of minigames: In Garena Blockman GO, there is always something new and exciting for you to discover every day. You can join the adventures and venture into the countless minigames from all the different genres. Some of the popular minigames are:
-
Party Street: Collect graffitis from all over the city and spray it to your heart's content. You can experience this super cool street style in the Party Street and hop into a random party with all the other cool guys.
-
The Exorcists: A game of survival and betrayal. As one of the 4 exorcists, you must perform an exorcism in an abandoned school. But wait! There is an imposter hidden among you… Look for clues to find the imposter and complete the exorcism ritual through various missions. Meanwhile, the imposter must hide their real identity, mislead the other exorcists with the wrong clues and summon the devil to kill all the exorcists.
-
Frontline: A 30 vs 30 multiplayer battlefield shooting game. You'll take on a soldier's duty and participate in a simulated battle. To win the game, you can shoot, drive tanks and armored vehicles, direct your comrades to occupy the core areas, and cooperate with other players to secure the final victory for your team.
-
Bed Wars: A popular team-based PVP game that has drawn a large number of players from all over the world. You and your teammates need to protect your bed at all costs, while trying to destroy the beds of other teams. You can collect resources, buy items, upgrade weapons, and build bridges to attack other teams. The last team standing wins the game.
-
-
-
Editor mode: In Garena Blockman GO, you can unleash your creativity and imagination by creating your own minigames using the editor mode. You can design the map, the rules, the characters, the items, and everything else you want. You can also share your minigames with other players and let them play and rate your creations.
-
Avatar customization: In Garena Blockman GO, you can customize your avatar with creative selections of fashionable accessories, such as hats, glasses, masks, clothes, shoes, and more. You can also change your avatar's appearance, such as hair color, eye color, skin tone, and more. You can show off your unique style to the world and express yourself in different ways.
-
Party and clan system: In Garena Blockman GO, you can make friends and chat with other players from all over the world. You can join a party or a clan to team up with other players and enjoy the minigames together. You can also create your own clan and invite your friends to join. You can chat with your clan members, send gifts, participate in clan events, and compete with other clans for glory and rewards.
-
VIP membership: In Garena Blockman GO, you can upgrade your VIP membership to enjoy more benefits and rewards. As a VIP member, you can get exclusive items, skins, badges, and more. You can also get more golds, diamonds, coupons, and other resources every day. You can also enjoy faster loading speed, priority access to minigames, and more privileges in the game.
-
-
How to Download Garena Blockman GO on Your Device
-
Garena Blockman GO is available for Android, iOS, and PC devices. You can download it for free from the official website or from the app stores. Here are the steps to download Garena Blockman GO on your device:
-
How to Download Garena Blockman GO on Android
-
If you have an Android device, you can download Garena Blockman GO from the Google Play Store or from the official website. Here are the steps to download Garena Blockman GO on Android:
-
How to download garena blockman go on android
-Garena blockman go apk download latest version
-Garena blockman go free download for pc
-Garena blockman go mod apk unlimited money download
-Download garena blockman go and play with friends
-Garena blockman go online game download
-Garena blockman go hack download no root
-Garena blockman go sandbox game download
-Download garena blockman go from google play store
-Garena blockman go minigames download
-Garena blockman go bed wars download
-Garena blockman go egg wars download
-Garena blockman go sky block download
-Garena blockman go party street download
-Garena blockman go the exorcists download
-Garena blockman go frontline download
-Garena blockman go free city rp download
-Download garena blockman go for ios
-Download garena blockman go for windows 10
-Download garena blockman go for mac
-Download garena blockman go for laptop
-Download garena blockman go for chromebook
-Download garena blockman go for fire tablet
-Download garena blockman go for kindle fire
-Download garena blockman go for xbox one
-Download garena blockman go for ps4
-Download garena blockman go for nintendo switch
-Download garena blockman go offline mode
-Download garena blockman go without wifi
-Download garena blockman go without ads
-Download garena blockman go without vip subscription
-Download garena blockman go with unlimited coins and gems
-Download garena blockman go with all skins unlocked
-Download garena blockman go with custom maps and mods
-Download garena blockman go with voice chat and emojis
-Download garena blockman go with new updates and features
-Download garena blockman go with best graphics and performance
-Download garena blockman go with low storage and battery usage
-Download garena blockman go with easy controls and settings
-Download garena blockman go with fun and creative gameplay
-Garena blockman go app download review and rating
-Garena blockman go game download guide and tips
-Garena blockman go download error and solution
-Garena blockman go download link and qr code
-Garena blockman go official website and social media download
-Garena blockman go alternative games to download
-
-
Open the Google Play Store app on your Android device.
-
Search for "Garena Blockman GO" in the search bar.
-
Select the app from the search results and tap on "Install".
-
Wait for the app to download and install on your device.
-
Once the app is installed, tap on "Open" to launch it.
-
Enjoy playing Garena Blockman GO on your Android device.
-
-
Alternatively, you can download Garena Blockman GO from the official website by following these steps:
Tap on the "Download" button at the top right corner of the website.
-
Select "Android" from the options and tap on "Download APK".
-
Wait for the APK file to download on your device.
-
Once the APK file is downloaded, tap on it to install it.
-
You may need to enable "Unknown sources" in your device settings to allow the installation of apps from outside sources.
-
Once the app is installed, tap on it to launch it.
-
Enjoy playing Garena Blockman GO on your Android device.
-
-
How to Download Garena Blockman GO on iOS
-
If you have an iOS device, you can download Garena Blockman GO from the App Store or from the official website. Here are the steps to download Garena Blockman GO on iOS:
-
-
Open the App Store app on your iOS device.
-
Search for "Garena Blockman GO" in the search bar.
-
Select the app from the search results and tap on "Get".
-
Wait for the app to download and install on your device.
-
Once the app is installed, tap on it to launch it.
-
Enjoy playing Garena Blockman GO on your iOS device.
-
-
Alternatively, you can download Garena Blockman GO from the official website by following Garena Blockman GO from the official website for Android, iOS, or PC devices, you can update it by opening the app and tapping on the "Update" button at the bottom of the screen.
-
If you have downloaded Garena Blockman GO from an emulator for PC devices, you can update it by opening the Google Play Store app in the emulator and tapping on the "Update" button next to the app name.
-
-
Q: How do I delete Garena Blockman GO?
-
A: If you want to delete Garena Blockman GO from your device, you can follow these steps:
-
-
If you have downloaded Garena Blockman GO from the app stores for Android or iOS devices, you can delete it by holding down the app icon on your device screen and tapping on the "Uninstall" or "Delete" option.
-
If you have downloaded Garena Blockman GO from the official website for Android, iOS, or PC devices, you can delete it by opening the app and tapping on the "Settings" icon at the top right corner of the screen. Then, tap on the "Uninstall" option and confirm your choice.
-
If you have downloaded Garena Blockman GO from an emulator for PC devices, you can delete it by opening the emulator and holding down the app icon on the emulator screen. Then, tap on the "Uninstall" or "Delete" option and confirm your choice.
-
-
-
I hope this article has helped you learn more about Garena Blockman GO and how to download it on your device. If you have any feedback or suggestions, please feel free to leave a comment below. Thank you for reading and have a great day!
197e85843d
-
-
\ No newline at end of file
diff --git a/spaces/1phancelerku/anime-remove-background/Download Raid Shadow Legends Mod APK from BlackMod and Dominate the Game.md b/spaces/1phancelerku/anime-remove-background/Download Raid Shadow Legends Mod APK from BlackMod and Dominate the Game.md
deleted file mode 100644
index c9e4cce6e11ef988578e0a34846181507217a613..0000000000000000000000000000000000000000
--- a/spaces/1phancelerku/anime-remove-background/Download Raid Shadow Legends Mod APK from BlackMod and Dominate the Game.md
+++ /dev/null
@@ -1,96 +0,0 @@
-
-
Raid Shadow Legends Mod APK Blackmod: How to Download and Play
-
If you are a fan of fantasy RPG games, you might have heard of Raid Shadow Legends. It is a popular game that lets you collect and customize hundreds of champions, fight epic battles, and explore a vast world. But what if you want to enhance your gaming experience with some extra features? That's where Raid Shadow Legends Mod APK Blackmod comes in. In this article, we will tell you what this mod is, how to download and install it, and how to play it. Let's get started!
Raid Shadow Legends is a free-to-play mobile game developed by Plarium Global Ltd. It was released in 2018 and has since gained millions of players worldwide. The game is set in the fantasy realm of Teleria, where you can recruit and train over 500 champions from 16 factions, each with their own skills and abilities. You can use your champions to fight in various modes, such as campaign, dungeons, arena, clan boss, faction wars, and more. You can also join clans, chat with other players, and participate in events and tournaments.
-
The game features stunning 3D graphics, realistic animations, and immersive sound effects. It also has a rich story and lore that unfolds as you progress through the game. You can play the game on your Android or iOS device, or on your PC using an emulator.
-
What is Blackmod?
-
Blackmod is a website that provides modded APKs for various games. A modded APK is a modified version of the original game file that has some changes or additions to the game features. For example, some modded APKs may offer unlimited money, gems, coins, or resources. Others may unlock all levels, characters, items, or skills. Some may even give you access to cheats, hacks, or bots.
-
Blackmod is one of the most trusted and reliable sources of modded APKs on the internet. It has a large collection of games from different genres and categories. You can browse through the website and find the game you want to download. You can also read the description, features, screenshots, and reviews of each modded APK before downloading it.
-
What is Raid Shadow Legends Mod APK Blackmod?
-
Raid Shadow Legends Mod APK Blackmod is a modded version of Raid Shadow Legends that offers two main features: menu mod and increased battle speed. Menu mod is a feature that allows you to access a menu in the game that lets you toggle on or off various options, such as auto win, god mode, weak enemies, etc. Increased battle speed is a feature that allows you to speed up the battles by 1-10 times, which can be useful for farming, grinding, or saving time.
-
raid shadow legends mod apk blackmod free download
-raid shadow legends mod apk blackmod unlimited gems
-raid shadow legends mod apk blackmod latest version
-raid shadow legends mod apk blackmod no root
-raid shadow legends mod apk blackmod menu mod
-raid shadow legends mod apk blackmod increases battle speed
-raid shadow legends mod apk blackmod reddit
-raid shadow legends mod apk blackmod hack
-raid shadow legends mod apk blackmod online
-raid shadow legends mod apk blackmod android
-raid shadow legends mod apk blackmod ios
-raid shadow legends mod apk blackmod 2023
-raid shadow legends mod apk blackmod update
-raid shadow legends mod apk blackmod installation guide
-raid shadow legends mod apk blackmod gameplay
-raid shadow legends mod apk blackmod review
-raid shadow legends mod apk blackmod cheats
-raid shadow legends mod apk blackmod tips and tricks
-raid shadow legends mod apk blackmod best champions
-raid shadow legends mod apk blackmod tier list
-raid shadow legends mod apk blackmod clan boss
-raid shadow legends mod apk blackmod dungeons
-raid shadow legends mod apk blackmod arena
-raid shadow legends mod apk blackmod faction wars
-raid shadow legends mod apk blackmod doom tower
-raid shadow legends mod apk blackmod events and tournaments
-raid shadow legends mod apk blackmod fusion guide
-raid shadow legends mod apk blackmod masteries guide
-raid shadow legends mod apk blackmod artifacts guide
-raid shadow legends mod apk blackmod affinity guide
-raid shadow legends mod apk blackmod roles guide
-raid shadow legends mod apk blackmod skills guide
-raid shadow legends mod apk blackmod stats guide
-raid shadow legends mod apk blackmod gear guide
-raid shadow legends mod apk blackmod sets guide
-raid shadow legends mod apk blackmod farming guide
-raid shadow legends mod apk blackmod leveling guide
-raid shadow legends mod apk blackmod beginners guide
-raid shadow legends mod apk blackmod advanced guide
-raid shadow legends mod apk blackmod strategy guide
-raid shadow legends mod apk blackmod secrets and hidden features
-raid shadow legends mod apk blackmod support and feedback
-raid shadow legends mod apk blackmod bugs and issues
-raid shadow legends mod apk blackmod news and updates
-raid shadow legends mod apk blackmod community and forums
-raid shadow legends mod apk blackmod discord server
-raid shadow legends mod apk blackmod youtube channel
-raid shadow legends mod apk blackmod facebook page
-
With these features, you can enjoy the game more easily and conveniently. You can breeze through the campaign mode, defeat the bosses faster, dominate the arena, and collect more rewards. You can also experiment with different champions and strategies without worrying about losing or wasting resources.
-
How to download and install Raid Shadow Legends Mod APK Blackmod?
-
To download and install Raid Shadow Legends Mod APK Blackmod, you need to follow these steps:
Wait for the download to finish and then open the file.
-
If you have the original version of Raid Shadow Legends installed on your device, you need to uninstall it first. You can do this by going to your settings, apps, and selecting Raid Shadow Legends. Then, tap on uninstall and confirm.
-
After uninstalling the original version, you need to enable the installation of unknown sources on your device. You can do this by going to your settings, security, and toggling on the unknown sources option.
-
Now, you can install the modded APK by tapping on it and following the instructions.
-
Once the installation is done, you can open the game and enjoy the mod features.
-
-
Note: You may need to grant some permissions to the game, such as storage, phone, etc. You may also need to verify your device by completing a captcha or a short survey. This is to prevent bots and spam from abusing the mod.
-
How to play Raid Shadow Legends Mod APK Blackmod?
-
To play Raid Shadow Legends Mod APK Blackmod, you need to follow these tips and tricks:
-
-
When you start the game, you will see a menu icon on the top left corner of the screen. Tap on it to open the menu mod. Here, you can enable or disable the options you want, such as auto win, god mode, weak enemies, etc. You can also adjust the battle speed by sliding the bar from 1x to 10x.
-
Be careful when using the menu mod options, as some of them may cause the game to crash or freeze. For example, if you use auto win in arena mode, you may get stuck in a loop of winning and losing. To avoid this, you can turn off auto win before entering the arena mode.
-
Also, be aware that using the menu mod may get you banned from the game if you are detected by the developers or reported by other players. To avoid this, you can use the menu mod sparingly and only for personal use. Do not abuse the mod features or brag about them in public chat or forums.
-
Enjoy the game with the mod features and have fun collecting and upgrading your champions, fighting epic battles, and exploring Teleria.
-
-
Conclusion
-
Raid Shadow Legends Mod APK Blackmod is a great way to enhance your gaming experience with some extra features. You can download and install it easily from the link provided in this article. You can also play it with some tips and tricks that we shared with you. However, you should also be careful when using the mod features, as they may cause some issues or get you banned from the game. Therefore, use the mod at your own risk and discretion.
-
If you liked this article, please share it with your friends and fellow gamers. Also, let us know what you think about Raid Shadow Legends Mod APK Blackmod in the comments below. Have fun!
-
FAQs
-
Q: Is Raid Shadow Legends Mod APK Blackmod safe to use?
-
A: Raid Shadow Legends Mod APK Blackmod is safe to use as long as you download it from a trusted source and scan it with an antivirus before installing it. However, using any modded APK may pose some risks to your device or account, so use it at your own risk and discretion.
-
Q: Is Raid Shadow Legends Mod APK Blackmod compatible with my device?
-
A: Raid Shadow Legends Mod APK Blackmod is compatible with most Android devices that have Android 5.0 or higher. However, some devices may not support the mod features or run smoothly with them. Therefore, you may need to try different devices or settings to find the best performance.
-
Q: Can I update Raid Shadow Legends Mod APK Blackmod?
-
A: No, you cannot update Raid Shadow Legends Mod APK Blackmod from the Google Play Store or any other source. If you do so, you will lose the mod features and revert back to the original version of the game. To update the modded APK, you need to wait for a new version of it to be released by Blackmod or another modder.
-
Q: Can I play Raid Shadow Legends Mod APK Blackmod online with other players?
-
A: Yes, you can play Raid Shadow Legends Mod APK Blackmod online with other players who have the same version of the modded APK as you. However, you may not be able to play with players who have the original version of the game or a different version of the modded APK. Also, be careful when playing online with other players, as they may report you for using the mod features or cheat detection systems may catch you.
-
Q: Can I request more features for Raid Shadow Legends Mod APK Blackmod?
-
A A: You can request more features for Raid Shadow Legends Mod APK Blackmod by contacting the modder or the website that provides the modded APK. However, there is no guarantee that your request will be fulfilled or that the modder will update the modded APK regularly. Therefore, you may need to be patient and appreciate the features that are already available.
401be4b1e0
-
-
\ No newline at end of file
diff --git a/spaces/1phancelerku/anime-remove-background/Enjoy Retro Gaming on Your Smartphone with These Arcade Emulators for Android.md b/spaces/1phancelerku/anime-remove-background/Enjoy Retro Gaming on Your Smartphone with These Arcade Emulators for Android.md
deleted file mode 100644
index a5bf5fed825c4ea920a59198c63436b4881f4711..0000000000000000000000000000000000000000
--- a/spaces/1phancelerku/anime-remove-background/Enjoy Retro Gaming on Your Smartphone with These Arcade Emulators for Android.md
+++ /dev/null
@@ -1,231 +0,0 @@
-
-
How to Play Arcade Games on Your Android Device with Emulators
-
If you are a fan of retro gaming or just want to relive some of your childhood memories, you might be interested in playing arcade games on your Android device. Arcade games are video games that were originally played in coin-operated machines installed in public places such as restaurants, bars, amusement parks, etc. Some of the most popular arcade games are Pac-Man, Street Fighter II, Mortal Kombat, Donkey Kong, Space Invaders, Galaga, Metal Slug, Double Dragon, and many more. These games are known for their simple yet addictive gameplay, colorful graphics, catchy sound effects, and high scores. They are also part of the history and culture of video gaming and have influenced many modern games and genres.
-
But how can you play these arcade games on your Android device? The answer is emulators. Emulators are software programs that can mimic the hardware and software of different systems, such as arcade machines, consoles, computers, etc. By using emulators, you can run games that were designed for other platforms on your Android device. In this article, we will show you how to use emulators to play arcade games on your Android device, what are the benefits of using emulators, what are some of the best arcade emulators for Android, how to install and use them, and what are some of the best arcade games to play on your Android device. Let's get started!
Emulators are software programs that can simulate the hardware and software of different systems, such as arcade machines, consoles, computers, etc. Emulators can run on various devices, such as PCs, smartphones, tablets, etc. Emulators can allow you to play games that were designed for other platforms on your device. For example, you can use an emulator to play a Nintendo game on your PC or an arcade game on your Android device.
-
But how do emulators work? Emulators work by translating the instructions and data of the original system into a format that your device can understand and execute. For example, an arcade emulator can translate the code and graphics of an arcade game into a format that your Android device can run. Emulators also have to emulate the input and output devices of the original system, such as joysticks, buttons, speakers, etc.
-
However, emulators alone are not enough to play games. You also need ROMs and BIOS files. ROMs are files that contain the data of the game itself, such as code, graphics, sound effects, etc. BIOS files are files that contain the data of the system itself, such as firmware, settings, etc. ROMs and BIOS files are usually extracted from the original cartridges or discs of the games or systems by using special devices or software. However, you should only download ROMs and BIOS files from legal sources or dump them from your own original cartridges or discs if you own them. Downloading ROMs and BIOS files from illegal sources or without owning the original games or systems is considered piracy and may violate intellectual property rights.
-
What Are the Benefits of Using Emulators for Android?
-
Using emulators for Android has many benefits for retro gamers who want to play arcade games on their devices. Here are some of them:
-
-
Convenience: You don't need to buy or maintain a physical arcade machine or console to play arcade games on your Android device. You just need to download an emulator app and some ROMs and BIOS files and you are good to go. You can also play arcade games anytime and anywhere with your Android device.
-
Cost-effectiveness: You don't need to spend money on coins or tokens to play arcade games on your Android device. You also don't need to buy expensive cartridges or discs of arcade games or systems. You just need to download some free or cheap emulator apps and some ROMs and BIOS files from legal sources or dump them from your own original cartridges or discs if you own them.
-
Nostalgia: You can relive some of your childhood memories or experience some of the classic arcade games that you missed out on by playing them on your Android device. You can also enjoy the retro graphics, sound effects, and gameplay of arcade games that may not be available on modern platforms.
-
Customization: You can customize your gaming experience by using different emulator apps and settings. You can adjust the screen size, resolution, orientation, aspect ratio, filters, frameskip, sound volume, etc. of your emulator app according to your preferences. You can also configure the controls and buttons of your emulator app according to your comfort and convenience.
-
Preservation: You can preserve some of the history and culture of video gaming by playing arcade games on your Android device. Arcade games are part of the heritage and legacy of video gaming and have influenced many modern games and genres. By using emulators for Android, you can help keep these arcade games alive and accessible for future generations.
-
-
What Are the Best Arcade Emulators for Android?
-
There are many arcade emulators for Android that you can choose from, but not all of them are equally good. Some of them may have better compatibility, performance, features, or user interface than others. To help you find the best arcade emulator for your Android device, we have selected some of the most popular and reliable ones and compared their features, pros, cons, and compatibility. Here are some of the best arcade emulators for Android:
-
MAME4droid
-
MAME4droid is one of the most popular and widely used arcade emulators for Android. MAME stands for Multiple Arcade Machine Emulator, and it is a project that aims to emulate as many arcade games as possible. MAME4droid is based on the MAME 0.139u1 version, which supports over 8,000 arcade games from various systems, such as Neo Geo, CPS1, CPS2, CPS3, Sega System 16, etc. MAME4droid also supports some console games that use similar hardware as arcade games, such as Sega Genesis, Sega Master System, etc.
-
Some of the features of MAME4droid are:
-
-
It supports native resolution and aspect ratio for each game.
-
It supports various input methods, such as touch screen, keyboard, gamepad, accelerometer, etc.
-
It supports cheat codes and save states.
-
It supports multiplayer mode via Bluetooth or Wi-Fi.
-
It has a user-friendly interface that allows you to browse and search games by category, name, year, manufacturer, etc.
-
-
Some of the pros of MAME4droid are:
-
arcade emulator android app
-arcade emulator android apk
-arcade emulator android download
-arcade emulator android games
-arcade emulator android reddit
-arcade emulator android roms
-arcade emulator android tv
-arcade emulator android 2023
-arcade emulator android best
-arcade emulator android free
-arcade emulator android mame
-arcade emulator android offline
-arcade emulator android online
-arcade emulator android ps2
-arcade emulator android snes
-best arcade emulators for android devices
-best arcade emulators for android pocket gamer
-best arcade emulators for android phones
-best arcade emulators for android tablets
-best arcade emulators for android tv box
-classicboy arcade emulator for android
-dolphin arcade emulator for android
-drastic ds arcade emulator for android
-epsxe for android arcade emulator
-gba.emu arcade emulator for android
-m64plus fz arcade emulator for android
-my boy! arcade emulator for android
-nostalgia.nes arcade emulator for android
-pizza boy gbc arcade emulator for android
-retroarch arcade emulator for android
-aethersx2 ps2 arcade emulator for android
-best game emulators for android digital trends
-best emulators for android 2023 android central
-best emulators for android to run old pc console and arcade classics
-how to play old school games on your phone with an arcade emulator app
-how to install and use an arcade emulator on your android device
-how to find and download roms for your favorite arcade games on android
-how to set up and configure a gamepad for your arcade emulator on android
-how to adjust per-game settings and optimize performance for your arcade emulator on android
-how to save and load states and cheats for your arcade games on android
-the best free and affordable arcade emulators for android in 2023
-the most popular and compatible arcade emulators for android in 2023
-the most accurate and authentic arcade emulators for android in 2023
-the most user-friendly and easy-to-use arcade emulators for android in 2023
-the most feature-rich and customizable arcade emulators for android in 2023
-
-
It has a high compatibility rate with many arcade games.
-
It has a good performance and speed with most games.
-
It has a lot of options and settings to customize your gaming experience.
-
It is free and open source.
-
-
Some of the cons of MAME4droid are:
-
-
It may not support some newer or more complex arcade games.
-
It may require a powerful device to run some games smoothly.
-
It may have some bugs or glitches with some games or features.
-
-
MAME4droid is compatible with Android devices running Android 2.3 or higher. You can download it from Google Play Store or other sources. You will also need to download ROMs and BIOS files from legal sources or dump them from your own original cartridges or discs if you own them. You can load ROMs into MAME4droid by placing them in the ROMs folder of your device's internal storage or external SD card.
-
RetroArch
-
RetroArch is another popular and versatile arcade emulator for Android. RetroArch is not just an emulator, but a platform that can run various emulators called cores. RetroArch can run emulators for many systems, such as arcade machines, consoles, computers, handhelds, etc. RetroArch can also run games that are not emulated but ported to the platform, such as Doom , Quake , Cave Story , etc.
-
Some of the features of RetroArch are:
-
-
It supports high-resolution graphics and shaders for each game.
-
It supports various input methods, such as touch screen, keyboard, gamepad, mouse, etc.
-
It supports cheat codes and save states.
-
It supports multiplayer mode via Bluetooth or Wi-Fi.
-
It has a user-friendly interface that allows you to browse and search games by category, name, system, core, etc.
-
-
Some of the pros of RetroArch are:
-
-
It has a high compatibility rate with many arcade games and other systems.
-
It has a good performance and speed with most games.
-
It has a lot of options and settings to customize your gaming experience.
-
It is free and open source.
-
-
Some of the cons of RetroArch are:
-
-
It may not support some newer or more complex arcade games or systems.
-
It may require a powerful device to run some games smoothly.
-
It may have some bugs or glitches with some games or features.
-
-
RetroArch is compatible with Android devices running Android 2.3 or higher. You can download it from Google Play Store or other sources. You will also need to download cores from within the app or from other sources. You will also need to download ROMs and BIOS files from legal sources or dump them from your own original cartridges or discs if you own them. sources. You will also need to download ROMs and BIOS files from legal sources or dump them from your own original cartridges or discs if you own them. You can load ROMs into Tiger Arcade by placing them in the ROMs folder of your device's internal storage or external SD card.
-
Arcade Games
-
Arcade Games is another arcade emulator for Android that offers a collection of classic arcade games that you can play online or offline. Arcade Games features over 200 arcade games from various systems, such as Atari, Nintendo, Sega, Capcom, etc. Some of the games included are Asteroids , Centipede , Frogger , Pac-Man , Space Invaders , Super Mario Bros , Tetris , etc.
-
Some of the features of Arcade Games are:
-
-
It supports native resolution and aspect ratio for each game.
-
It supports touch screen and gamepad input methods.
-
It supports cheat codes and save states.
-
It has a simple and intuitive interface that allows you to browse and search games by category, name, year, manufacturer, etc.
-
-
Some of the pros of Arcade Games are:
-
-
It has a high compatibility rate with many classic arcade games.
-
It has a good performance and speed with most games.
-
It has a minimalistic and user-friendly design.
-
It is free and ad-free.
-
-
Some of the cons of Arcade Games are:
-
-
It may not support some newer or more complex arcade games or systems.
-
It may require a stable internet connection to play some games online.
-
It may have some bugs or glitches with some games or features.
-
-
Arcade Games is compatible with Android devices running Android 4.1 or higher. You can download it from Google Play Store or other sources. You will also need to download ROMs and BIOS files from legal sources or dump them from your own original cartridges or discs if you own them. You can load ROMs into Arcade Games by placing them in the ROMs folder of your device's internal storage or external SD card.
-
Comparison Table of Arcade Emulators for Android
-
-
-
Name
-
Supported Systems
-
Number of Games
-
Online/Offline Mode
-
User Rating
-
-
-
MAME4droid
-
Arcade machines, consoles
-
Over 8,000
-
Offline
-
4.2/5
-
-
-
RetroArch
-
Arcade machines, consoles, computers, handhelds, etc.
-
Over 10,000
-
Offline
-
4.1/5
-
-
-
Nostalgia.Arcade
-
Arcade machines, consoles
-
Over 8,000
-
Offline
-
4.0/5
-
-
-
Tiger Arcade
-
Neo Geo games
-
Over 1,000
-
Offline
-
3.9/5
-
-
-
Arcade Games
-
Arcade machines, consoles
-
Over 200
-
Online/Offline
-
3.8/5
-
How to Install and Use Emulators for Android?
-
Now that you know some of the best arcade emulators for Android, you might be wondering how to install and use them. The process is not very complicated, but it may vary slightly depending on the emulator app and the source of the ROMs and BIOS files. Here are some general steps that you can follow to install and use emulators for Android:
-
-
Download an emulator app from Google Play Store or other sources. Make sure that the app is compatible with your device and the games that you want to play.
-
Download ROMs and BIOS files from legal sources or dump them from your own original cartridges or discs if you own them. Make sure that the ROMs and BIOS files are compatible with the emulator app and the games that you want to play.
-
Load ROMs into the emulator app by placing them in the ROMs folder of your device's internal storage or external SD card. Some emulator apps may have different folders for different systems or games, so make sure that you place the ROMs in the correct folder.
-
Load BIOS files into the emulator app by placing them in the BIOS folder of your device's internal storage or external SD card. Some emulator apps may require BIOS files for some systems or games, so make sure that you have the correct BIOS files.
-
Launch the emulator app and browse and select the game that you want to play. Some emulator apps may have a built-in game browser, while others may require you to manually locate and select the game file.
-
Configure settings and controls according to your preferences. You can adjust the screen size, resolution, orientation, aspect ratio, filters, frameskip, sound volume, etc. of your emulator app. You can also configure the controls and buttons of your emulator app according to your comfort and convenience. You can use touch screen, keyboard, gamepad, accelerometer, etc. as input methods.
-
Enjoy your favorite arcade games on your Android device!
-
-
What Are Some of the Best Arcade Games to Play on Your Android Device?
-
There are thousands of arcade games that you can play on your Android device with emulators, but not all of them are equally fun and enjoyable. Some of them may be more popular, more challenging, more appealing, or more nostalgic than others. To help you find some of the best arcade games to play on your Android device, we have selected some of them based on their popularity, genre, graphics, gameplay, and nostalgia factor. Here are some of the best arcade games to play on your Android device:
-
-
Pac-Man: Pac-Man is one of the most iconic and influential arcade games of all time. It was released by Namco in 1980 and became a worldwide phenomenon. The game involves controlling a yellow circle with a mouth that eats dots and fruits while avoiding four ghosts that chase it in a maze. The game is simple yet addictive and has spawned many sequels, spin-offs, remakes, and adaptations.
-
Street Fighter II: Street Fighter II is one of the most popular and influential fighting games of all time. It was released by Capcom in 1991 and revolutionized the genre with its fast-paced gameplay, diverse characters, special moves, combos, and competitive multiplayer mode. The game involves selecting one of eight fighters from different countries and fighting against other fighters in various stages until reaching the final boss.
-
Mortal Kombat: Mortal Kombat is another fighting game that became famous for its violent and bloody gameplay, realistic graphics, digitized actors, and controversial fatalities. It was released by Midway in 1992 and sparked a lot of controversy and censorship issues. The game involves selecting one of seven fighters from different realms and fighting against other fighters in various stages until reaching the final boss.
-
Donkey Kong: Donkey Kong is one of the first platform games that introduced Mario, one of the most famous video game characters of all time. It was released by Nintendo in 1981 and became a huge success. The game involves controlling Mario as he tries to rescue his girlfriend Pauline from a giant ape named Donkey Kong who throws barrels at him.
-
Space Invaders: Space Invaders is one of the first shooting games that started the space shooter genre. It was released by Taito in 1978 and became a cultural phenomenon. The game involves controlling a laser cannon that shoots at waves of alien invaders who descend from the top of the screen.
-
Galaga: Galaga is another shooting game that improved on Space Invaders with its colorful graphics, dynamic gameplay, bonus stages, and enemy formations. It was released by Namco in 1981 and became one of the most successful arcade games of all time. The game involves controlling a starship that shoots at waves of alien insects who fly in various patterns and formations.
-
Metal Slug: Metal Slug is a series of run and gun games that are known for their humorous and cartoonish graphics, explosive gameplay, and cooperative multiplayer mode. The first game was released by SNK in 1996 and became a cult classic. The game involves controlling one of four soldiers who fight against a rebel army and various enemies using various weapons, vehicles, and items.
-
Double Dragon: Double Dragon is one of the first beat 'em up games that popularized the genre with its cooperative multiplayer mode, branching paths, and weapon usage. It was released by Technos Japan in 1987 and became a hit. The game involves controlling one of two brothers who fight against a gang of thugs who kidnapped their girlfriend.
-
-
Conclusion
-
Arcade games are video games that were originally played in coin-operated machines installed in public places such as restaurants, bars, amusement parks, etc. They are popular among retro gamers who want to relive some of their childhood memories or experience some of the classic games that they missed out on. By using emulators, you can play arcade games on your Android device. Emulators are software programs that can mimic the hardware and software of different systems, such as arcade machines, consoles, computers, etc. By using emulators, you can run games that were designed for other platforms on your Android device.
-
Using emulators for Android has many benefits, such as convenience, cost-effectiveness, nostalgia, customization, and preservation. You can play arcade games anytime and anywhere with your Android device without spending money on coins or tokens or buying or maintaining a physical arcade machine or console. You can also enjoy the retro graphics, sound effects, and gameplay of arcade games that may not be available on modern platforms. You can also customize your gaming experience by using different emulator apps and settings. You can also preserve some of the history and culture of video gaming by playing arcade games on your Android device.
-
There are many arcade emulators for Android that you can choose from, but some of the best ones are MAME4droid, RetroArch, Nostalgia.Arcade, Tiger Arcade, and Arcade Games. These emulator apps have high compatibility rates with many arcade games from various systems, good performance and speed with most games, user-friendly interfaces and designs, and various features and options to enhance your gaming experience. You can download these emulator apps from Google Play Store or other sources. You will also need to download ROMs and BIOS files from legal sources or dump them from your own original cartridges or discs if you own them. You can load ROMs into these emulator apps by placing them in the ROMs folder of your device's internal storage or external SD card.
-
There are thousands of arcade games that you can play on your Android device with emulators, but some of the best ones are Pac-Man , Street Fighter II , Mortal Kombat , Donkey Kong , Space Invaders , Galaga , Metal Slug , Double Dragon , etc. These games are known for their simple yet addictive gameplay, colorful graphics, catchy sound effects, and high scores. They are also part of the history and culture of video gaming and have influenced many modern games and genres.
-
If you are a fan of retro gaming or just want to have some fun with arcade games on your Android device, you should definitely try out some of the best arcade emulators for Android. You will not regret it!
-
FAQs
-
Here are some frequently asked questions that you might have about arcade emulators for Android:
-
-
Are arcade emulators for Android legal?
-
Arcade emulators for Android are legal as long as you use them for personal and non-commercial purposes. However, downloading ROMs and BIOS files from illegal sources or without owning the original games or systems is considered piracy and may violate intellectual property rights. You should only download ROMs and BIOS files from legal sources or dump them from your own original cartridges or discs if you own them.
-
Are arcade emulators for Android safe?
-
Arcade emulators for Android are safe as long as you download them from reputable sources such as Google Play Store or official websites. However, downloading ROMs and BIOS files from unknown or untrusted sources may contain viruses or malware that may harm your device or compromise your privacy. You should only download ROMs and BIOS files from legal sources or dump them from your own original cartridges or discs if you own them.
-
Are arcade emulators for Android free?
-
Arcade emulators for Android are free as long as you download them from legal sources or dump them from your own original cartridges or discs if you own them. However, some emulator apps may have in-app purchases or ads that may require you to pay money or watch ads to access some features or games. You can choose to pay or not depending on your preference.
-
Which arcade emulator for Android is the best?
-
There is no definitive answer to this question, as different arcade emulators for Android may have different advantages and disadvantages depending on your device, the games that you want to play, and your personal preference. However, some of the most popular and reliable arcade emulators for Android are MAME4droid, RetroArch, Nostalgia.Arcade, Tiger Arcade, and Arcade Games. You can try them out and see which one suits you best.
-
How can I improve the performance and speed of arcade emulators for Android?
-
There are some factors that may affect the performance and speed of arcade emulators for Android, such as your device's specifications, the emulator app's settings, the game's complexity, etc. Here are some tips that may help you improve the performance and speed of arcade emulators for Android:
-
-
Use a powerful device that has a high CPU, RAM, GPU, and storage capacity.
-
Use a stable and fast internet connection if you play online games.
-
Use a compatible and updated emulator app that supports the games that you want to play.
-
Use compatible and legal ROMs and BIOS files that match the emulator app and the games that you want to play.
-
Adjust the emulator app's settings according to your device's specifications and your gaming preferences. You can lower the screen resolution, aspect ratio, filters, frameskip, sound volume, etc. to improve the performance and speed of the emulator app.
-
Close other apps and processes that may consume your device's resources or interfere with the emulator app.
-
401be4b1e0
-
-
\ No newline at end of file
diff --git a/spaces/1phancelerku/anime-remove-background/Farm Town Village Build Story Enjoy the Rural Life and Grow Your Own Crops.md b/spaces/1phancelerku/anime-remove-background/Farm Town Village Build Story Enjoy the Rural Life and Grow Your Own Crops.md
deleted file mode 100644
index 676badbf821fd85b914d156af7ff669655e3d295..0000000000000000000000000000000000000000
--- a/spaces/1phancelerku/anime-remove-background/Farm Town Village Build Story Enjoy the Rural Life and Grow Your Own Crops.md
+++ /dev/null
@@ -1,108 +0,0 @@
-
-
Farm Town Village Build Story: A Fun and Relaxing Farming Game
-
Do you love farming games? Do you want to experience the quiet charm of rural life? Do you want to build your own dream village with a view to a river? If you answered yes to any of these questions, then you should definitely check out Farm Town Village Build Story, a unique blend of farming and city-building simulation game. In this article, we will tell you everything you need to know about this game, including its features, tips and tricks, and how to download it for free.
Farm Town Village Build Story is a game developed by Foranj Games, a leading developer of casual games for mobile devices. The game was released in 2019 and has since gained over 10 million downloads and 4.5 stars rating on Google Play Store. The game is available for both Android and iOS devices, and you can play it offline or online.
-
What is Farm Town Village Build Story?
-
Farm Town Village Build Story is a game that combines the elements of farming and city-building. You start with a small farm near a township, where you can grow hay, corn, vegetables, fruits, berries, and flowers. You can also craft dozens of different treats from your harvest, such as lollipop, cookie, birthday cake, and more. You can then sell your goods to the townsfolk and friendly neighbors, who will give you cash and XP in return.
-
But farming is not the only thing you can do in this game. You can also build your own village with various buildings, such as houses, factories, community buildings, and a seaside trading port. You can also manage the distribution center of your village, where you can receive orders from different customers. You can also expand your village by repairing a circus and inviting tourists to visit your farm. You can also build a zoo and adopt lovely pets, such as pony, sheep, cow, pig, chicken, duck, dog, cat, and more.
-
farm town village build story game
-farm town village build story apk
-farm town village build story download
-farm town village build story mod apk
-farm town village build story cheats
-farm town village build story hack
-farm town village build story for pc
-farm town village build story online
-farm town village build story app
-farm town village build story free
-farm town village build story review
-farm town village build story tips
-farm town village build story guide
-farm town village build story walkthrough
-farm town village build story update
-farm town village build story play store
-farm town village build story facebook
-farm town village build story forum
-farm town village build story wiki
-farm town village build story reddit
-farm town village build story gameplay
-farm town village build story trailer
-farm town village build story video
-farm town village build story android
-farm town village build story ios
-farm town village build story iphone
-farm town village build story ipad
-farm town village build story windows
-farm town village build story mac
-farm town village build story laptop
-farm town village build story chromebook
-farm town village build story simulator
-farm town village build story casual game
-farm town village build story farming game
-farm town village build story management game
-farm town village build story simulation game
-farm town village build story relaxing game
-farm town village build story fun game
-farm town village build story cute game
-farm town village build story cartoon game
-farm town village build story 3d game
-farm town village build story offline game
-farm town village build story single player game
-farm town village build story family game
-farm town village build story kids game
-how to play farm town village build story
-how to install farm town village build story
-how to hack farm town village build story
-how to update farm town village build story
-
Why should you play Farm Town Village Build Story?
-
There are many reasons why you should play Farm Town Village Build Story. Here are some of them:
-
-
It is fun and relaxing. You can enjoy the simple pleasures of farming and village life, such as planting seeds, watering crops, harvesting fruits, feeding animals, baking cakes, making friends, and more.
-
It is colorful and cartoonish. The game has bright graphics and cute animations that will make you smile. The game also has cheerful music and sound effects that will enhance your mood.
-
It is challenging and rewarding. You can complete various tasks and quests that will test your skills and creativity. You can also earn coins and gems that you can use to upgrade your buildings and buy new items.
-
It is social and interactive. You can connect with other players from around the world through Facebook or Google Play Games. You can visit their farms, help them out, chat with them, send them gifts, or compete with them in leaderboards.
-
It is free and accessible. You can download the game for free from Google Play Store or App Store. You can also play the game offline or online, depending on your preference.
-
-
Features of Farm Town Village Build Story
-
Farm Town Village Build Story has many features that make it an enjoyable game to play. Here are some of them:
Build a zoo and invite lovely pets to your farm
-
Another feature of Farm Town Village Build Story is the zoo aspect. You can build a zoo on your farm and invite lovely pets to live there. You can adopt animals such as pony, sheep, cow, pig, chicken, duck, dog, cat, and more. You can also unlock exotic animals such as panda, tiger, lion, elephant, giraffe, and more. You can feed your animals, play with them, and watch them grow. You can also breed your animals and get baby animals that are even cuter.
-
But building a zoo is not enough. You can also decorate your zoo with various items, such as fences, paths, benches, lamps, statues, and more. You can also create habitats for your animals, such as grassland, forest, savanna, desert, and more. You can also attract visitors to your zoo and earn money from them. You can also complete zoo quests and get rewards.
-
Repair a circus and attract tourists to your village
-
Another feature of Farm Town Village Build Story is the circus aspect. You can repair a circus on your village and attract tourists to visit it. You can restore the circus tent, the stage, the seats, the lights, and the decorations. You can also hire performers for your circus, such as clowns, acrobats, magicians, and more. You can also train your animals to perform tricks for the audience.
-
But repairing a circus is not enough. You can also upgrade your circus with new equipment, such as trampoline, cannon, ball pool, and more. You can also create shows for your circus and earn applause from the spectators. You can also complete circus quests and get rewards.
Enlist the support of your friends and neighbors
-
Another feature of Farm Town Village Build Story is the social aspect. You can enlist the support of your friends and neighbors in your farming and village life. You can connect with other players through Facebook or Google Play Games. You can visit their farms and villages, help them out, chat with them, send them gifts, or compete with them in leaderboards. You can also trade goods with them through the market or the port. You can also join a group on Facebook to share your stories and get updates.
-
But enlisting the support of your friends and neighbors is not enough. You can also make new friends and neighbors in the game. You can meet different characters, such as Tom, Alice, Bob, Lisa, and more. You can also interact with them, learn their stories, complete their quests, and earn their trust. You can also unlock new areas and buildings by befriending them.
-
Decorate your farm with beautiful flowers and decorations
-
Another feature of Farm Town Village Build Story is the decoration aspect. You can decorate your farm with beautiful flowers and decorations. You can grow flowers, such as rose, tulip, sunflower, daisy, and more. You can also buy decorations, such as fences, paths, benches, lamps, statues, and more. You can also customize your farm with different themes, such as fairy tale, Halloween, Christmas, and more.
-
But decorating your farm is not enough. You can also decorate your village with various items. You can build houses, factories, community buildings, and a seaside trading port. You can also upgrade your buildings and make them more attractive. You can also create a unique landscape for your village with different terrains, such as grassland, forest, savanna, desert, and more.
-
Tips and tricks for playing Farm Town Village Build Story
-
Farm Town Village Build Story is a game that requires strategy and planning. Here are some tips and tricks that will help you play the game better:
Upgrade your storage and factories regularly
-
One of the tips for playing Farm Town Village Build Story is to upgrade your storage and factories regularly. You will need a lot of space to store your crops, fruits, flowers, and products. You will also need efficient factories to craft your goods faster and better. You can upgrade your storage and factories by using coins, gems, or materials. You can get coins by selling your goods, completing orders and quests, or visiting other players' farms. You can get gems by watching ads, leveling up, or buying them with real money. You can get materials by harvesting crops, opening chests, or requesting them from your friends.
-
Complete orders and quests to earn cash and XP
-
Another tip for playing Farm Town Village Build Story is to complete orders and quests to earn cash and XP. You can receive orders from different customers, such as townsfolk, neighbors, circus visitors, or port traders. You can also receive quests from different characters, such as Tom, Alice, Bob, Lisa, and more. You can complete orders and quests by delivering the required goods or performing the required actions. You can earn cash and XP by completing orders and quests. You can use cash to buy new items or upgrade your buildings. You can use XP to level up and unlock new features.
-
Use the market to buy and sell goods
-
Another tip for playing Farm Town Village Build Story is to use the market to buy and sell goods. You can access the market by tapping on the market stall on your farm. You can buy goods from other players or sell your own goods to them. You can set the price and quantity of your goods as you wish. You can also use the market to trade goods with your friends or neighbors. You can use the market to get the goods you need or make some extra cash.
Visit other players' farms and help them out
-
Another tip for playing Farm Town Village Build Story is to visit other players' farms and help them out. You can visit other players' farms by tapping on the map icon on the bottom right corner of the screen. You can see the farms of your friends, neighbors, or random players. You can help them out by watering their crops, feeding their animals, or harvesting their goods. You can also chat with them, send them gifts, or rate their farms. You can earn coins, XP, and materials by visiting other players' farms.
-
Join a group on Facebook to share your stories and get updates
-
Another tip for playing Farm Town Village Build Story is to join a group on Facebook to share your stories and get updates. You can join the official group of Farm Town Village Build Story by tapping on the Facebook icon on the top left corner of the screen. You can also search for other groups related to the game on Facebook. You can share your stories, screenshots, tips, and tricks with other players in the group. You can also get updates, news, events, and giveaways from the developers of the game.
-
Conclusion
-
Farm Town Village Build Story is a fun and relaxing farming game that you can play on your mobile device. You can grow a variety of crops and craft delicious treats. You can build a zoo and invite lovely pets to your farm. You can repair a circus and attract tourists to your village. You can enlist the support of your friends and neighbors. You can decorate your farm with beautiful flowers and decorations. You can also complete orders and quests to earn cash and XP. You can also use the market to buy and sell goods. You can also visit other players' farms and help them out. You can also join a group on Facebook to share your stories and get updates.
-
If you are looking for a game that combines the elements of farming and city-building, then you should definitely try Farm Town Village Build Story. It is free to download and play, and you can enjoy it offline or online. It is colorful and cartoonish, challenging and rewarding, social and interactive. It is a game that will make you smile and relax.
-
FAQs
-
Here are some frequently asked questions about Farm Town Village Build Story:
-
-
How do I download Farm Town Village Build Story?
-
You can download Farm Town Village Build Story from Google Play Store or App Store for free. Just search for the game name and tap on the install button.
-
How do I play Farm Town Village Build Story offline?
-
You can play Farm Town Village Build Story offline by turning off your internet connection before launching the game. However, some features may not be available offline, such as visiting other players' farms or connecting with Facebook.
-
How do I get more gems in Farm Town Village Build Story?
-
You can get more gems in Farm Town Village Build Story by watching ads, leveling up, or buying them with real money.
-
How do I unlock new areas and buildings in Farm Town Village Build Story?
-
You can unlock new areas and buildings in Farm Town Village Build Story by leveling up, befriending characters, completing quests, or using gems.
-
How do I contact the developers of Farm Town Village Build Story?
-
You can contact the developers of Farm Town Village Build Story by sending them an email at support@foranj.com or by joining their Facebook group.
-
197e85843d
-
-
\ No newline at end of file
diff --git a/spaces/1toTree/lora_test/ppdiffusers/pipelines/stable_diffusion/pipeline_stable_diffusion_inpaint.py b/spaces/1toTree/lora_test/ppdiffusers/pipelines/stable_diffusion/pipeline_stable_diffusion_inpaint.py
deleted file mode 100644
index 6a9d5e8322d0ae060ec71314b3f7ed5476e83500..0000000000000000000000000000000000000000
--- a/spaces/1toTree/lora_test/ppdiffusers/pipelines/stable_diffusion/pipeline_stable_diffusion_inpaint.py
+++ /dev/null
@@ -1,694 +0,0 @@
-# Copyright (c) 2022 PaddlePaddle Authors. All Rights Reserved.
-# Copyright 2022 The HuggingFace Team. All rights reserved.
-#
-# Licensed under the Apache License, Version 2.0 (the "License");
-# you may not use this file except in compliance with the License.
-# You may obtain a copy of the License at
-#
-# http://www.apache.org/licenses/LICENSE-2.0
-#
-# Unless required by applicable law or agreed to in writing, software
-# distributed under the License is distributed on an "AS IS" BASIS,
-# WITHOUT WARRANTIES OR CONDITIONS OF ANY KIND, either express or implied.
-# See the License for the specific language governing permissions and
-# limitations under the License.
-
-import inspect
-from typing import Callable, List, Optional, Union
-
-import numpy as np
-import paddle
-import paddle.nn.functional as F
-import PIL
-from packaging import version
-
-from paddlenlp.transformers import CLIPFeatureExtractor, CLIPTextModel, CLIPTokenizer
-
-from ...configuration_utils import FrozenDict
-from ...models import AutoencoderKL, UNet2DConditionModel
-from ...pipeline_utils import DiffusionPipeline
-from ...schedulers import (
- DDIMScheduler,
- DPMSolverMultistepScheduler,
- EulerAncestralDiscreteScheduler,
- EulerDiscreteScheduler,
- LMSDiscreteScheduler,
- PNDMScheduler,
-)
-from ...utils import deprecate, logging
-from . import StableDiffusionPipelineOutput
-from .safety_checker import StableDiffusionSafetyChecker
-
-logger = logging.get_logger(__name__) # pylint: disable=invalid-name
-
-
-def prepare_mask_and_masked_image(image, mask):
- """
- Prepares a pair (image, mask) to be consumed by the Stable Diffusion pipeline. This means that those inputs will be
- converted to ``paddle.Tensor`` with shapes ``batch x channels x height x width`` where ``channels`` is ``3`` for the
- ``image`` and ``1`` for the ``mask``.
- The ``image`` will be converted to ``paddle.float32`` and normalized to be in ``[-1, 1]``. The ``mask`` will be
- binarized (``mask > 0.5``) and cast to ``paddle.float32`` too.
- Args:
- image (Union[np.array, PIL.Image, paddle.Tensor]): The image to inpaint.
- It can be a ``PIL.Image``, or a ``height x width x 3`` ``np.array`` or a ``channels x height x width``
- ``paddle.Tensor`` or a ``batch x channels x height x width`` ``paddle.Tensor``.
- mask (_type_): The mask to apply to the image, i.e. regions to inpaint.
- It can be a ``PIL.Image``, or a ``height x width`` ``np.array`` or a ``1 x height x width``
- ``paddle.Tensor`` or a ``batch x 1 x height x width`` ``paddle.Tensor``.
- Raises:
- ValueError: ``paddle.Tensor`` images should be in the ``[-1, 1]`` range. ValueError: ``paddle.Tensor`` mask
- should be in the ``[0, 1]`` range. ValueError: ``mask`` and ``image`` should have the same spatial dimensions.
- TypeError: ``mask`` is a ``paddle.Tensor`` but ``image`` is not
- (ot the other way around).
- Returns:
- tuple[paddle.Tensor]: The pair (mask, masked_image) as ``paddle.Tensor`` with 4
- dimensions: ``batch x channels x height x width``.
- """
- if isinstance(image, paddle.Tensor):
- if not isinstance(mask, paddle.Tensor):
- raise TypeError(f"`image` is a paddle.Tensor but `mask` (type: {type(mask)} is not")
-
- # Batch single image
- if image.ndim == 3:
- assert image.shape[0] == 3, "Image outside a batch should be of shape (3, H, W)"
- image = image.unsqueeze(0)
-
- # Batch and add channel dim for single mask
- if mask.ndim == 2:
- mask = mask.unsqueeze(0).unsqueeze(0)
-
- # Batch single mask or add channel dim
- if mask.ndim == 3:
- # Single batched mask, no channel dim or single mask not batched but channel dim
- if mask.shape[0] == 1:
- mask = mask.unsqueeze(0)
-
- # Batched masks no channel dim
- else:
- mask = mask.unsqueeze(1)
-
- assert image.ndim == 4 and mask.ndim == 4, "Image and Mask must have 4 dimensions"
- assert image.shape[-2:] == mask.shape[-2:], "Image and Mask must have the same spatial dimensions"
- assert image.shape[0] == mask.shape[0], "Image and Mask must have the same batch size"
-
- # Check image is in [-1, 1]
- if image.min() < -1 or image.max() > 1:
- raise ValueError("Image should be in [-1, 1] range")
-
- # Check mask is in [0, 1]
- if mask.min() < 0 or mask.max() > 1:
- raise ValueError("Mask should be in [0, 1] range")
-
- # Binarize mask
- mask[mask < 0.5] = 0
- mask[mask >= 0.5] = 1
-
- # Image as float32
- image = image.cast(paddle.float32)
- elif isinstance(mask, paddle.Tensor):
- raise TypeError(f"`mask` is a paddle.Tensor but `image` (type: {type(image)} is not")
- else:
- # preprocess image
- if isinstance(image, (PIL.Image.Image, np.ndarray)):
- image = [image]
-
- if isinstance(image, list) and isinstance(image[0], PIL.Image.Image):
- image = [np.array(i.convert("RGB"))[None, :] for i in image]
- image = np.concatenate(image, axis=0)
- elif isinstance(image, list) and isinstance(image[0], np.ndarray):
- image = np.concatenate([i[None, :] for i in image], axis=0)
-
- image = image.transpose(0, 3, 1, 2)
- image = paddle.to_tensor(image).cast(paddle.float32) / 127.5 - 1.0
-
- # preprocess mask
- if isinstance(mask, (PIL.Image.Image, np.ndarray)):
- mask = [mask]
-
- if isinstance(mask, list) and isinstance(mask[0], PIL.Image.Image):
- mask = np.concatenate([np.array(m.convert("L"))[None, None, :] for m in mask], axis=0)
- mask = mask.astype(np.float32) / 255.0
- elif isinstance(mask, list) and isinstance(mask[0], np.ndarray):
- mask = np.concatenate([m[None, None, :] for m in mask], axis=0)
-
- mask[mask < 0.5] = 0
- mask[mask >= 0.5] = 1
- mask = paddle.to_tensor(mask)
-
- masked_image = image * (mask < 0.5)
-
- return mask, masked_image
-
-
-class StableDiffusionInpaintPipeline(DiffusionPipeline):
- r"""
- Pipeline for text-guided image inpainting using Stable Diffusion. *This is an experimental feature*.
-
- This model inherits from [`DiffusionPipeline`]. Check the superclass documentation for the generic methods the
- library implements for all the pipelines (such as downloading or saving, running on a particular xxxx, etc.)
-
- Args:
- vae ([`AutoencoderKL`]):
- Variational Auto-Encoder (VAE) Model to encode and decode images to and from latent representations.
- text_encoder ([`CLIPTextModel`]):
- Frozen text-encoder. Stable Diffusion uses the text portion of
- [CLIP](https://huggingface.co/docs/transformers/model_doc/clip#transformers.CLIPTextModel), specifically
- the [clip-vit-large-patch14](https://huggingface.co/openai/clip-vit-large-patch14) variant.
- tokenizer (`CLIPTokenizer`):
- Tokenizer of class
- [CLIPTokenizer](https://huggingface.co/docs/transformers/v4.21.0/en/model_doc/clip#transformers.CLIPTokenizer).
- unet ([`UNet2DConditionModel`]): Conditional U-Net architecture to denoise the encoded image latents.
- scheduler ([`SchedulerMixin`]):
- A scheduler to be used in combination with `unet` to denoise the encoded image latents. Can be one of
- [`DDIMScheduler`], [`LMSDiscreteScheduler`], [`PNDMScheduler`], [`EulerDiscreteScheduler`], [`EulerAncestralDiscreteScheduler`]
- or [`DPMSolverMultistepScheduler`].
- safety_checker ([`StableDiffusionSafetyChecker`]):
- Classification module that estimates whether generated images could be considered offensive or harmful.
- Please, refer to the [model card](https://huggingface.co/runwayml/stable-diffusion-v1-5) for details.
- feature_extractor ([`CLIPFeatureExtractor`]):
- Model that extracts features from generated images to be used as inputs for the `safety_checker`.
- """
- _optional_components = ["safety_checker", "feature_extractor"]
-
- def __init__(
- self,
- vae: AutoencoderKL,
- text_encoder: CLIPTextModel,
- tokenizer: CLIPTokenizer,
- unet: UNet2DConditionModel,
- scheduler: Union[
- DDIMScheduler,
- PNDMScheduler,
- LMSDiscreteScheduler,
- EulerDiscreteScheduler,
- EulerAncestralDiscreteScheduler,
- DPMSolverMultistepScheduler,
- ],
- safety_checker: StableDiffusionSafetyChecker,
- feature_extractor: CLIPFeatureExtractor,
- requires_safety_checker: bool = True,
- ):
- super().__init__()
-
- if hasattr(scheduler.config, "steps_offset") and scheduler.config.steps_offset != 1:
- deprecation_message = (
- f"The configuration file of this scheduler: {scheduler} is outdated. `steps_offset`"
- f" should be set to 1 instead of {scheduler.config.steps_offset}. Please make sure "
- "to update the config accordingly as leaving `steps_offset` might led to incorrect results"
- " in future versions. If you have downloaded this checkpoint from the Hugging Face Hub,"
- " it would be very nice if you could open a Pull request for the `scheduler/scheduler_config.json`"
- " file"
- )
- deprecate("steps_offset!=1", "1.0.0", deprecation_message, standard_warn=False)
- new_config = dict(scheduler.config)
- new_config["steps_offset"] = 1
- scheduler._internal_dict = FrozenDict(new_config)
-
- if hasattr(scheduler.config, "skip_prk_steps") and scheduler.config.skip_prk_steps is False:
- deprecation_message = (
- f"The configuration file of this scheduler: {scheduler} has not set the configuration"
- " `skip_prk_steps`. `skip_prk_steps` should be set to True in the configuration file. Please make"
- " sure to update the config accordingly as not setting `skip_prk_steps` in the config might lead to"
- " incorrect results in future versions. If you have downloaded this checkpoint from the Hugging Face"
- " Hub, it would be very nice if you could open a Pull request for the"
- " `scheduler/scheduler_config.json` file"
- )
- deprecate("skip_prk_steps not set", "1.0.0", deprecation_message, standard_warn=False)
- new_config = dict(scheduler.config)
- new_config["skip_prk_steps"] = True
- scheduler._internal_dict = FrozenDict(new_config)
-
- if safety_checker is None and requires_safety_checker:
- logger.warning(
- f"You have disabled the safety checker for {self.__class__} by passing `safety_checker=None`. Ensure"
- " that you abide to the conditions of the Stable Diffusion license and do not expose unfiltered"
- " results in services or applications open to the public. PaddleNLP team, diffusers team and Hugging Face"
- " strongly recommend to keep the safety filter enabled in all public facing circumstances, disabling"
- " it only for use-cases that involve analyzing network behavior or auditing its results. For more"
- " information, please have a look at https://github.com/huggingface/diffusers/pull/254 ."
- )
- if safety_checker is not None and feature_extractor is None:
- raise ValueError(
- "Make sure to define a feature extractor when loading {self.__class__} if you want to use the safety"
- " checker. If you do not want to use the safety checker, you can pass `'safety_checker=None'` instead."
- )
- is_unet_version_less_0_9_0 = hasattr(unet.config, "_ppdiffusers_version") and version.parse(
- version.parse(unet.config._ppdiffusers_version).base_version
- ) < version.parse("0.9.0.dev0")
- is_unet_sample_size_less_64 = hasattr(unet.config, "sample_size") and unet.config.sample_size < 64
- if is_unet_version_less_0_9_0 and is_unet_sample_size_less_64:
- deprecation_message = (
- "The configuration file of the unet has set the default `sample_size` to smaller than"
- " 64 which seems highly unlikely. If your checkpoint is a fine-tuned version of any of the"
- " following: \n- CompVis/stable-diffusion-v1-4 \n- CompVis/stable-diffusion-v1-3 \n-"
- " CompVis/stable-diffusion-v1-2 \n- CompVis/stable-diffusion-v1-1 \n- runwayml/stable-diffusion-v1-5"
- " \n- runwayml/stable-diffusion-inpainting \n you should change 'sample_size' to 64 in the"
- " configuration file. Please make sure to update the config accordingly as leaving `sample_size=32`"
- " in the config might lead to incorrect results in future versions. If you have downloaded this"
- " checkpoint from the Hugging Face Hub, it would be very nice if you could open a Pull request for"
- " the `unet/config.json` file"
- )
- deprecate("sample_size<64", "1.0.0", deprecation_message, standard_warn=False)
- new_config = dict(unet.config)
- new_config["sample_size"] = 64
- unet._internal_dict = FrozenDict(new_config)
- self.register_modules(
- vae=vae,
- text_encoder=text_encoder,
- tokenizer=tokenizer,
- unet=unet,
- scheduler=scheduler,
- safety_checker=safety_checker,
- feature_extractor=feature_extractor,
- )
- self.vae_scale_factor = 2 ** (len(self.vae.config.block_out_channels) - 1)
- self.register_to_config(requires_safety_checker=requires_safety_checker)
-
- # Copied from diffusers.pipelines.stable_diffusion.pipeline_stable_diffusion.StableDiffusionPipeline._encode_prompt
- def _encode_prompt(self, prompt, num_images_per_prompt, do_classifier_free_guidance, negative_prompt):
- r"""
- Encodes the prompt into text encoder hidden states.
-
- Args:
- prompt (`str` or `list(int)`):
- prompt to be encoded
- num_images_per_prompt (`int`):
- number of images that should be generated per prompt
- do_classifier_free_guidance (`bool`):
- whether to use classifier free guidance or not
- negative_prompt (`str` or `List[str]`):
- The prompt or prompts not to guide the image generation. Ignored when not using guidance (i.e., ignored
- if `guidance_scale` is less than `1`).
- """
- batch_size = len(prompt) if isinstance(prompt, list) else 1
-
- text_inputs = self.tokenizer(
- prompt,
- padding="max_length",
- max_length=self.tokenizer.model_max_length,
- truncation=True,
- return_tensors="pd",
- )
- text_input_ids = text_inputs.input_ids
- untruncated_ids = self.tokenizer(prompt, padding="longest", return_tensors="pd").input_ids
-
- if untruncated_ids.shape[-1] >= text_input_ids.shape[-1] and not paddle.equal_all(
- text_input_ids, untruncated_ids
- ):
- removed_text = self.tokenizer.batch_decode(untruncated_ids[:, self.tokenizer.model_max_length - 1 : -1])
- logger.warning(
- "The following part of your input was truncated because CLIP can only handle sequences up to"
- f" {self.tokenizer.model_max_length} tokens: {removed_text}"
- )
-
- config = (
- self.text_encoder.config
- if isinstance(self.text_encoder.config, dict)
- else self.text_encoder.config.to_dict()
- )
- if config.get("use_attention_mask", None) is not None and config["use_attention_mask"]:
- attention_mask = text_inputs.attention_mask
- else:
- attention_mask = None
-
- text_embeddings = self.text_encoder(
- text_input_ids,
- attention_mask=attention_mask,
- )
- text_embeddings = text_embeddings[0]
-
- # duplicate text embeddings for each generation per prompt, using mps friendly method
- bs_embed, seq_len, _ = text_embeddings.shape
- text_embeddings = text_embeddings.tile([1, num_images_per_prompt, 1])
- text_embeddings = text_embeddings.reshape([bs_embed * num_images_per_prompt, seq_len, -1])
-
- # get unconditional embeddings for classifier free guidance
- if do_classifier_free_guidance:
- uncond_tokens: List[str]
- if negative_prompt is None:
- uncond_tokens = [""] * batch_size
- elif type(prompt) is not type(negative_prompt):
- raise TypeError(
- f"`negative_prompt` should be the same type to `prompt`, but got {type(negative_prompt)} !="
- f" {type(prompt)}."
- )
- elif isinstance(negative_prompt, str):
- uncond_tokens = [negative_prompt]
- elif batch_size != len(negative_prompt):
- raise ValueError(
- f"`negative_prompt`: {negative_prompt} has batch size {len(negative_prompt)}, but `prompt`:"
- f" {prompt} has batch size {batch_size}. Please make sure that passed `negative_prompt` matches"
- " the batch size of `prompt`."
- )
- else:
- uncond_tokens = negative_prompt
-
- max_length = text_input_ids.shape[-1]
- uncond_input = self.tokenizer(
- uncond_tokens,
- padding="max_length",
- max_length=max_length,
- truncation=True,
- return_tensors="pd",
- )
-
- if config.get("use_attention_mask", None) is not None and config["use_attention_mask"]:
- attention_mask = uncond_input.attention_mask
- else:
- attention_mask = None
-
- uncond_embeddings = self.text_encoder(
- uncond_input.input_ids,
- attention_mask=attention_mask,
- )
- uncond_embeddings = uncond_embeddings[0]
-
- # duplicate unconditional embeddings for each generation per prompt, using mps friendly method
- seq_len = uncond_embeddings.shape[1]
- uncond_embeddings = uncond_embeddings.tile([1, num_images_per_prompt, 1])
- uncond_embeddings = uncond_embeddings.reshape([batch_size * num_images_per_prompt, seq_len, -1])
-
- # For classifier free guidance, we need to do two forward passes.
- # Here we concatenate the unconditional and text embeddings into a single batch
- # to avoid doing two forward passes
- text_embeddings = paddle.concat([uncond_embeddings, text_embeddings])
-
- return text_embeddings
-
- # Copied from diffusers.pipelines.stable_diffusion.pipeline_stable_diffusion.StableDiffusionPipeline.run_safety_checker
- def run_safety_checker(self, image, dtype):
- if self.safety_checker is not None:
- safety_checker_input = self.feature_extractor(self.numpy_to_pil(image), return_tensors="pd")
- image, has_nsfw_concept = self.safety_checker(
- images=image, clip_input=safety_checker_input.pixel_values.cast(dtype)
- )
- else:
- has_nsfw_concept = None
- return image, has_nsfw_concept
-
- # Copied from diffusers.pipelines.stable_diffusion.pipeline_stable_diffusion.StableDiffusionPipeline.prepare_extra_step_kwargs
- def prepare_extra_step_kwargs(self, generator, eta):
- # prepare extra kwargs for the scheduler step, since not all schedulers have the same signature
- # eta (η) is only used with the DDIMScheduler, it will be ignored for other schedulers.
- # eta corresponds to η in DDIM paper: https://arxiv.org/abs/2010.02502
- # and should be between [0, 1]
-
- accepts_eta = "eta" in set(inspect.signature(self.scheduler.step).parameters.keys())
- extra_step_kwargs = {}
- if accepts_eta:
- extra_step_kwargs["eta"] = eta
-
- # check if the scheduler accepts generator
- accepts_generator = "generator" in set(inspect.signature(self.scheduler.step).parameters.keys())
- if accepts_generator:
- extra_step_kwargs["generator"] = generator
- return extra_step_kwargs
-
- # Copied from diffusers.pipelines.stable_diffusion.pipeline_stable_diffusion.StableDiffusionPipeline.decode_latents
- def decode_latents(self, latents):
- latents = 1 / 0.18215 * latents
- image = self.vae.decode(latents).sample
- image = (image / 2 + 0.5).clip(0, 1)
- # we always cast to float32 as this does not cause significant overhead and is compatible with bfloa16
- image = image.transpose([0, 2, 3, 1]).cast("float32").numpy()
- return image
-
- # Copied from diffusers.pipelines.stable_diffusion.pipeline_stable_diffusion.StableDiffusionPipeline.check_inputs
- def check_inputs(self, prompt, height, width, callback_steps):
- if not isinstance(prompt, str) and not isinstance(prompt, list):
- raise ValueError(f"`prompt` has to be of type `str` or `list` but is {type(prompt)}")
-
- if height % 8 != 0 or width % 8 != 0:
- raise ValueError(f"`height` and `width` have to be divisible by 8 but are {height} and {width}.")
-
- if (callback_steps is None) or (
- callback_steps is not None and (not isinstance(callback_steps, int) or callback_steps <= 0)
- ):
- raise ValueError(
- f"`callback_steps` has to be a positive integer but is {callback_steps} of type"
- f" {type(callback_steps)}."
- )
-
- # Copied from diffusers.pipelines.stable_diffusion.pipeline_stable_diffusion.StableDiffusionPipeline.prepare_latents
- def prepare_latents(self, batch_size, num_channels_latents, height, width, dtype, generator, latents=None):
- shape = [batch_size, num_channels_latents, height // self.vae_scale_factor, width // self.vae_scale_factor]
- if isinstance(generator, list) and len(generator) != batch_size:
- raise ValueError(
- f"You have passed a list of generators of length {len(generator)}, but requested an effective batch"
- f" size of {batch_size}. Make sure the batch size matches the length of the generators."
- )
-
- if latents is None:
- if isinstance(generator, list):
- shape = [
- 1,
- ] + shape[1:]
- latents = [paddle.randn(shape, generator=generator[i], dtype=dtype) for i in range(batch_size)]
- latents = paddle.concat(latents, axis=0)
- else:
- latents = paddle.randn(shape, generator=generator, dtype=dtype)
- else:
- if latents.shape != shape:
- raise ValueError(f"Unexpected latents shape, got {latents.shape}, expected {shape}")
-
- # scale the initial noise by the standard deviation required by the scheduler
- latents = latents * self.scheduler.init_noise_sigma
- return latents
-
- def prepare_mask_latents(
- self, mask, masked_image, batch_size, height, width, dtype, generator, do_classifier_free_guidance
- ):
- # resize the mask to latents shape as we concatenate the mask to the latents
- # we do that before converting to dtype to avoid breaking in case we're using cpu_offload
- # and half precision
- mask = F.interpolate(mask, size=(height // self.vae_scale_factor, width // self.vae_scale_factor))
- mask = mask.cast(dtype=dtype)
-
- masked_image = masked_image.cast(dtype)
-
- # encode the mask image into latents space so we can concatenate it to the latents
- if isinstance(generator, list):
- masked_image_latents = [
- self.vae.encode(masked_image[i : i + 1]).latent_dist.sample(generator=generator[i])
- for i in range(batch_size)
- ]
- masked_image_latents = paddle.concat(masked_image_latents, axis=0)
- else:
- masked_image_latents = self.vae.encode(masked_image).latent_dist.sample(generator=generator)
- masked_image_latents = 0.18215 * masked_image_latents
-
- # duplicate mask and masked_image_latents for each generation per prompt, using mps friendly method
- if mask.shape[0] < batch_size:
- if not batch_size % mask.shape[0] == 0:
- raise ValueError(
- "The passed mask and the required batch size don't match. Masks are supposed to be duplicated to"
- f" a total batch size of {batch_size}, but {mask.shape[0]} masks were passed. Make sure the number"
- " of masks that you pass is divisible by the total requested batch size."
- )
- mask = mask.tile([batch_size // mask.shape[0], 1, 1, 1])
- if masked_image_latents.shape[0] < batch_size:
- if not batch_size % masked_image_latents.shape[0] == 0:
- raise ValueError(
- "The passed images and the required batch size don't match. Images are supposed to be duplicated"
- f" to a total batch size of {batch_size}, but {masked_image_latents.shape[0]} images were passed."
- " Make sure the number of images that you pass is divisible by the total requested batch size."
- )
- masked_image_latents = masked_image_latents.tile([batch_size // masked_image_latents.shape[0], 1, 1, 1])
-
- mask = paddle.concat([mask] * 2) if do_classifier_free_guidance else mask
- masked_image_latents = (
- paddle.concat([masked_image_latents] * 2) if do_classifier_free_guidance else masked_image_latents
- )
-
- masked_image_latents = masked_image_latents.cast(dtype)
- return mask, masked_image_latents
-
- @paddle.no_grad()
- def __call__(
- self,
- prompt: Union[str, List[str]],
- image: Union[paddle.Tensor, PIL.Image.Image],
- mask_image: Union[paddle.Tensor, PIL.Image.Image],
- height: Optional[int] = None,
- width: Optional[int] = None,
- num_inference_steps: int = 50,
- guidance_scale: float = 7.5,
- negative_prompt: Optional[Union[str, List[str]]] = None,
- num_images_per_prompt: Optional[int] = 1,
- eta: float = 0.0,
- generator: Optional[Union[paddle.Generator, List[paddle.Generator]]] = None,
- latents: Optional[paddle.Tensor] = None,
- output_type: Optional[str] = "pil",
- return_dict: bool = True,
- callback: Optional[Callable[[int, int, paddle.Tensor], None]] = None,
- callback_steps: Optional[int] = 1,
- ):
- r"""
- Function invoked when calling the pipeline for generation.
-
- Args:
- prompt (`str` or `List[str]`):
- The prompt or prompts to guide the image generation.
- image (`PIL.Image.Image`):
- `Image`, or tensor representing an image batch which will be inpainted, *i.e.* parts of the image will
- be masked out with `mask_image` and repainted according to `prompt`.
- mask_image (`PIL.Image.Image`):
- `Image`, or tensor representing an image batch, to mask `image`. White pixels in the mask will be
- repainted, while black pixels will be preserved. If `mask_image` is a PIL image, it will be converted
- to a single channel (luminance) before use. If it's a tensor, it should contain one color channel (L)
- instead of 3, so the expected shape would be `(B, H, W, 1)`.
- height (`int`, *optional*, defaults to self.unet.config.sample_size * self.vae_scale_factor):
- The height in pixels of the generated image.
- width (`int`, *optional*, defaults to self.unet.config.sample_size * self.vae_scale_factor):
- The width in pixels of the generated image.
- num_inference_steps (`int`, *optional*, defaults to 50):
- The number of denoising steps. More denoising steps usually lead to a higher quality image at the
- expense of slower inference.
- guidance_scale (`float`, *optional*, defaults to 7.5):
- Guidance scale as defined in [Classifier-Free Diffusion Guidance](https://arxiv.org/abs/2207.12598).
- `guidance_scale` is defined as `w` of equation 2. of [Imagen
- Paper](https://arxiv.org/pdf/2205.11487.pdf). Guidance scale is enabled by setting `guidance_scale >
- 1`. Higher guidance scale encourages to generate images that are closely linked to the text `prompt`,
- usually at the expense of lower image quality.
- negative_prompt (`str` or `List[str]`, *optional*):
- The prompt or prompts not to guide the image generation. Ignored when not using guidance (i.e., ignored
- if `guidance_scale` is less than `1`).
- num_images_per_prompt (`int`, *optional*, defaults to 1):
- The number of images to generate per prompt.
- eta (`float`, *optional*, defaults to 0.0):
- Corresponds to parameter eta (η) in the DDIM paper: https://arxiv.org/abs/2010.02502. Only applies to
- [`schedulers.DDIMScheduler`], will be ignored for others.
- generator (`paddle.Generator`, *optional*):
- One or a list of paddle generator(s) to make generation deterministic.
- latents (`paddle.Tensor`, *optional*):
- Pre-generated noisy latents, sampled from a Gaussian distribution, to be used as inputs for image
- generation. Can be used to tweak the same generation with different prompts. If not provided, a latents
- tensor will ge generated by sampling using the supplied random `generator`.
- output_type (`str`, *optional*, defaults to `"pil"`):
- The output format of the generate image. Choose between
- [PIL](https://pillow.readthedocs.io/en/stable/): `PIL.Image.Image` or `np.array`.
- return_dict (`bool`, *optional*, defaults to `True`):
- Whether or not to return a [`~pipelines.stable_diffusion.StableDiffusionPipelineOutput`] instead of a
- plain tuple.
- callback (`Callable`, *optional*):
- A function that will be called every `callback_steps` steps during inference. The function will be
- called with the following arguments: `callback(step: int, timestep: int, latents: paddle.Tensor)`.
- callback_steps (`int`, *optional*, defaults to 1):
- The frequency at which the `callback` function will be called. If not specified, the callback will be
- called at every step.
-
- Returns:
- [`~pipelines.stable_diffusion.StableDiffusionPipelineOutput`] or `tuple`:
- [`~pipelines.stable_diffusion.StableDiffusionPipelineOutput`] if `return_dict` is True, otherwise a `tuple.
- When returning a tuple, the first element is a list with the generated images, and the second element is a
- list of `bool`s denoting whether the corresponding generated image likely represents "not-safe-for-work"
- (nsfw) content, according to the `safety_checker`.
- """
- # 0. Default height and width to unet
- height = height or self.unet.config.sample_size * self.vae_scale_factor
- width = width or self.unet.config.sample_size * self.vae_scale_factor
-
- # 1. Check inputs
- self.check_inputs(prompt, height, width, callback_steps)
-
- # 2. Define call parameters
- batch_size = 1 if isinstance(prompt, str) else len(prompt)
- # here `guidance_scale` is defined analog to the guidance weight `w` of equation (2)
- # of the Imagen paper: https://arxiv.org/pdf/2205.11487.pdf . `guidance_scale = 1`
- # corresponds to doing no classifier free guidance.
- do_classifier_free_guidance = guidance_scale > 1.0
-
- # 3. Encode input prompt
- text_embeddings = self._encode_prompt(
- prompt, num_images_per_prompt, do_classifier_free_guidance, negative_prompt
- )
-
- # 4. Preprocess mask and image
- mask, masked_image = prepare_mask_and_masked_image(image, mask_image)
-
- # 5. set timesteps
- self.scheduler.set_timesteps(num_inference_steps)
- timesteps = self.scheduler.timesteps
-
- # 6. Prepare latent variables
- num_channels_latents = self.vae.config.latent_channels
- latents = self.prepare_latents(
- batch_size * num_images_per_prompt,
- num_channels_latents,
- height,
- width,
- text_embeddings.dtype,
- generator,
- latents,
- )
-
- # 7. Prepare mask latent variables
- mask, masked_image_latents = self.prepare_mask_latents(
- mask,
- masked_image,
- batch_size * num_images_per_prompt,
- height,
- width,
- text_embeddings.dtype,
- generator,
- do_classifier_free_guidance,
- )
-
- # 8. Check that sizes of mask, masked image and latents match
- num_channels_mask = mask.shape[1]
- num_channels_masked_image = masked_image_latents.shape[1]
- if num_channels_latents + num_channels_mask + num_channels_masked_image != self.unet.config.in_channels:
- raise ValueError(
- f"Incorrect configuration settings! The config of `pipeline.unet`: {self.unet.config} expects"
- f" {self.unet.config.in_channels} but received `num_channels_latents`: {num_channels_latents} +"
- f" `num_channels_mask`: {num_channels_mask} + `num_channels_masked_image`: {num_channels_masked_image}"
- f" = {num_channels_latents+num_channels_masked_image+num_channels_mask}. Please verify the config of"
- " `pipeline.unet` or your `mask_image` or `image` input."
- )
-
- # 9. Prepare extra step kwargs. TODO: Logic should ideally just be moved out of the pipeline
- extra_step_kwargs = self.prepare_extra_step_kwargs(generator, eta)
-
- # 10. Denoising loop
- num_warmup_steps = len(timesteps) - num_inference_steps * self.scheduler.order
- with self.progress_bar(total=num_inference_steps) as progress_bar:
- for i, t in enumerate(timesteps):
- # expand the latents if we are doing classifier free guidance
- latent_model_input = paddle.concat([latents] * 2) if do_classifier_free_guidance else latents
-
- # concat latents, mask, masked_image_latents in the channel dimension
- latent_model_input = self.scheduler.scale_model_input(latent_model_input, t)
- latent_model_input = paddle.concat([latent_model_input, mask, masked_image_latents], axis=1)
-
- # predict the noise residual
- noise_pred = self.unet(latent_model_input, t, encoder_hidden_states=text_embeddings).sample
-
- # perform guidance
- if do_classifier_free_guidance:
- noise_pred_uncond, noise_pred_text = noise_pred.chunk(2)
- noise_pred = noise_pred_uncond + guidance_scale * (noise_pred_text - noise_pred_uncond)
-
- # compute the previous noisy sample x_t -> x_t-1
- latents = self.scheduler.step(noise_pred, t, latents, **extra_step_kwargs).prev_sample
-
- # call the callback, if provided
- if i == len(timesteps) - 1 or ((i + 1) > num_warmup_steps and (i + 1) % self.scheduler.order == 0):
- progress_bar.update()
- if callback is not None and i % callback_steps == 0:
- callback(i, t, latents)
-
- # 11. Post-processing
- image = self.decode_latents(latents)
-
- # 12. Run safety checker
- image, has_nsfw_concept = self.run_safety_checker(image, text_embeddings.dtype)
-
- # 13. Convert to PIL
- if output_type == "pil":
- image = self.numpy_to_pil(image)
-
- if not return_dict:
- return (image, has_nsfw_concept)
-
- return StableDiffusionPipelineOutput(images=image, nsfw_content_detected=has_nsfw_concept)
diff --git a/spaces/2023Liu2023/bingo/src/components/ui/alert-dialog.tsx b/spaces/2023Liu2023/bingo/src/components/ui/alert-dialog.tsx
deleted file mode 100644
index 17fec4d16510328deacc1416569173c97761ef72..0000000000000000000000000000000000000000
--- a/spaces/2023Liu2023/bingo/src/components/ui/alert-dialog.tsx
+++ /dev/null
@@ -1,150 +0,0 @@
-'use client'
-
-import * as React from 'react'
-import * as AlertDialogPrimitive from '@radix-ui/react-alert-dialog'
-
-import { cn } from '@/lib/utils'
-import { buttonVariants } from '@/components/ui/button'
-
-const AlertDialog = AlertDialogPrimitive.Root
-
-const AlertDialogTrigger = AlertDialogPrimitive.Trigger
-
-const AlertDialogPortal = ({
- className,
- children,
- ...props
-}: AlertDialogPrimitive.AlertDialogPortalProps) => (
-
-
- {children}
-
-
-)
-AlertDialogPortal.displayName = AlertDialogPrimitive.Portal.displayName
-
-const AlertDialogOverlay = React.forwardRef<
- React.ElementRef,
- React.ComponentPropsWithoutRef
->(({ className, children, ...props }, ref) => (
-
-))
-AlertDialogOverlay.displayName = AlertDialogPrimitive.Overlay.displayName
-
-const AlertDialogContent = React.forwardRef<
- React.ElementRef,
- React.ComponentPropsWithoutRef
->(({ className, ...props }, ref) => (
-
-
-
-
-))
-AlertDialogContent.displayName = AlertDialogPrimitive.Content.displayName
-
-const AlertDialogHeader = ({
- className,
- ...props
-}: React.HTMLAttributes) => (
-
-)
-AlertDialogHeader.displayName = 'AlertDialogHeader'
-
-const AlertDialogFooter = ({
- className,
- ...props
-}: React.HTMLAttributes) => (
-
-)
-AlertDialogFooter.displayName = 'AlertDialogFooter'
-
-const AlertDialogTitle = React.forwardRef<
- React.ElementRef,
- React.ComponentPropsWithoutRef
->(({ className, ...props }, ref) => (
-
-))
-AlertDialogTitle.displayName = AlertDialogPrimitive.Title.displayName
-
-const AlertDialogDescription = React.forwardRef<
- React.ElementRef,
- React.ComponentPropsWithoutRef
->(({ className, ...props }, ref) => (
-
-))
-AlertDialogDescription.displayName =
- AlertDialogPrimitive.Description.displayName
-
-const AlertDialogAction = React.forwardRef<
- React.ElementRef,
- React.ComponentPropsWithoutRef
->(({ className, ...props }, ref) => (
-
-))
-AlertDialogAction.displayName = AlertDialogPrimitive.Action.displayName
-
-const AlertDialogCancel = React.forwardRef<
- React.ElementRef,
- React.ComponentPropsWithoutRef
->(({ className, ...props }, ref) => (
-
-))
-AlertDialogCancel.displayName = AlertDialogPrimitive.Cancel.displayName
-
-export {
- AlertDialog,
- AlertDialogTrigger,
- AlertDialogContent,
- AlertDialogHeader,
- AlertDialogFooter,
- AlertDialogTitle,
- AlertDialogDescription,
- AlertDialogAction,
- AlertDialogCancel
-}
diff --git a/spaces/AIFILMS/generate_human_motion/VQ-Trans/dataset/dataset_TM_train.py b/spaces/AIFILMS/generate_human_motion/VQ-Trans/dataset/dataset_TM_train.py
deleted file mode 100644
index 0b0223effb01c1cf57fa6b2b6fb8d9d01b83f84a..0000000000000000000000000000000000000000
--- a/spaces/AIFILMS/generate_human_motion/VQ-Trans/dataset/dataset_TM_train.py
+++ /dev/null
@@ -1,161 +0,0 @@
-import torch
-from torch.utils import data
-import numpy as np
-from os.path import join as pjoin
-import random
-import codecs as cs
-from tqdm import tqdm
-import utils.paramUtil as paramUtil
-from torch.utils.data._utils.collate import default_collate
-
-
-def collate_fn(batch):
- batch.sort(key=lambda x: x[3], reverse=True)
- return default_collate(batch)
-
-
-'''For use of training text-2-motion generative model'''
-class Text2MotionDataset(data.Dataset):
- def __init__(self, dataset_name, feat_bias = 5, unit_length = 4, codebook_size = 1024, tokenizer_name=None):
-
- self.max_length = 64
- self.pointer = 0
- self.dataset_name = dataset_name
-
- self.unit_length = unit_length
- # self.mot_start_idx = codebook_size
- self.mot_end_idx = codebook_size
- self.mot_pad_idx = codebook_size + 1
- if dataset_name == 't2m':
- self.data_root = './dataset/HumanML3D'
- self.motion_dir = pjoin(self.data_root, 'new_joint_vecs')
- self.text_dir = pjoin(self.data_root, 'texts')
- self.joints_num = 22
- radius = 4
- fps = 20
- self.max_motion_length = 26 if unit_length == 8 else 51
- dim_pose = 263
- kinematic_chain = paramUtil.t2m_kinematic_chain
- elif dataset_name == 'kit':
- self.data_root = './dataset/KIT-ML'
- self.motion_dir = pjoin(self.data_root, 'new_joint_vecs')
- self.text_dir = pjoin(self.data_root, 'texts')
- self.joints_num = 21
- radius = 240 * 8
- fps = 12.5
- dim_pose = 251
- self.max_motion_length = 26 if unit_length == 8 else 51
- kinematic_chain = paramUtil.kit_kinematic_chain
-
- split_file = pjoin(self.data_root, 'train.txt')
-
-
- id_list = []
- with cs.open(split_file, 'r') as f:
- for line in f.readlines():
- id_list.append(line.strip())
-
- new_name_list = []
- data_dict = {}
- for name in tqdm(id_list):
- try:
- m_token_list = np.load(pjoin(self.data_root, tokenizer_name, '%s.npy'%name))
-
- # Read text
- with cs.open(pjoin(self.text_dir, name + '.txt')) as f:
- text_data = []
- flag = False
- lines = f.readlines()
-
- for line in lines:
- try:
- text_dict = {}
- line_split = line.strip().split('#')
- caption = line_split[0]
- t_tokens = line_split[1].split(' ')
- f_tag = float(line_split[2])
- to_tag = float(line_split[3])
- f_tag = 0.0 if np.isnan(f_tag) else f_tag
- to_tag = 0.0 if np.isnan(to_tag) else to_tag
-
- text_dict['caption'] = caption
- text_dict['tokens'] = t_tokens
- if f_tag == 0.0 and to_tag == 0.0:
- flag = True
- text_data.append(text_dict)
- else:
- m_token_list_new = [tokens[int(f_tag*fps/unit_length) : int(to_tag*fps/unit_length)] for tokens in m_token_list if int(f_tag*fps/unit_length) < int(to_tag*fps/unit_length)]
-
- if len(m_token_list_new) == 0:
- continue
- new_name = '%s_%f_%f'%(name, f_tag, to_tag)
-
- data_dict[new_name] = {'m_token_list': m_token_list_new,
- 'text':[text_dict]}
- new_name_list.append(new_name)
- except:
- pass
-
- if flag:
- data_dict[name] = {'m_token_list': m_token_list,
- 'text':text_data}
- new_name_list.append(name)
- except:
- pass
- self.data_dict = data_dict
- self.name_list = new_name_list
-
- def __len__(self):
- return len(self.data_dict)
-
- def __getitem__(self, item):
- data = self.data_dict[self.name_list[item]]
- m_token_list, text_list = data['m_token_list'], data['text']
- m_tokens = random.choice(m_token_list)
-
- text_data = random.choice(text_list)
- caption= text_data['caption']
-
-
- coin = np.random.choice([False, False, True])
- # print(len(m_tokens))
- if coin:
- # drop one token at the head or tail
- coin2 = np.random.choice([True, False])
- if coin2:
- m_tokens = m_tokens[:-1]
- else:
- m_tokens = m_tokens[1:]
- m_tokens_len = m_tokens.shape[0]
-
- if m_tokens_len+1 < self.max_motion_length:
- m_tokens = np.concatenate([m_tokens, np.ones((1), dtype=int) * self.mot_end_idx, np.ones((self.max_motion_length-1-m_tokens_len), dtype=int) * self.mot_pad_idx], axis=0)
- else:
- m_tokens = np.concatenate([m_tokens, np.ones((1), dtype=int) * self.mot_end_idx], axis=0)
-
- return caption, m_tokens.reshape(-1), m_tokens_len
-
-
-
-
-def DATALoader(dataset_name,
- batch_size, codebook_size, tokenizer_name, unit_length=4,
- num_workers = 8) :
-
- train_loader = torch.utils.data.DataLoader(Text2MotionDataset(dataset_name, codebook_size = codebook_size, tokenizer_name = tokenizer_name, unit_length=unit_length),
- batch_size,
- shuffle=True,
- num_workers=num_workers,
- #collate_fn=collate_fn,
- drop_last = True)
-
-
- return train_loader
-
-
-def cycle(iterable):
- while True:
- for x in iterable:
- yield x
-
-
diff --git a/spaces/AIFILMS/generate_human_motion/VQ-Trans/options/get_eval_option.py b/spaces/AIFILMS/generate_human_motion/VQ-Trans/options/get_eval_option.py
deleted file mode 100644
index d0989ba1a8116068753ada2cb1806744e4512447..0000000000000000000000000000000000000000
--- a/spaces/AIFILMS/generate_human_motion/VQ-Trans/options/get_eval_option.py
+++ /dev/null
@@ -1,83 +0,0 @@
-from argparse import Namespace
-import re
-from os.path import join as pjoin
-
-
-def is_float(numStr):
- flag = False
- numStr = str(numStr).strip().lstrip('-').lstrip('+')
- try:
- reg = re.compile(r'^[-+]?[0-9]+\.[0-9]+$')
- res = reg.match(str(numStr))
- if res:
- flag = True
- except Exception as ex:
- print("is_float() - error: " + str(ex))
- return flag
-
-
-def is_number(numStr):
- flag = False
- numStr = str(numStr).strip().lstrip('-').lstrip('+')
- if str(numStr).isdigit():
- flag = True
- return flag
-
-
-def get_opt(opt_path, device):
- opt = Namespace()
- opt_dict = vars(opt)
-
- skip = ('-------------- End ----------------',
- '------------ Options -------------',
- '\n')
- print('Reading', opt_path)
- with open(opt_path) as f:
- for line in f:
- if line.strip() not in skip:
- # print(line.strip())
- key, value = line.strip().split(': ')
- if value in ('True', 'False'):
- opt_dict[key] = (value == 'True')
- # print(key, value)
- elif is_float(value):
- opt_dict[key] = float(value)
- elif is_number(value):
- opt_dict[key] = int(value)
- else:
- opt_dict[key] = str(value)
-
- # print(opt)
- opt_dict['which_epoch'] = 'finest'
- opt.save_root = pjoin(opt.checkpoints_dir, opt.dataset_name, opt.name)
- opt.model_dir = pjoin(opt.save_root, 'model')
- opt.meta_dir = pjoin(opt.save_root, 'meta')
-
- if opt.dataset_name == 't2m':
- opt.data_root = './dataset/HumanML3D/'
- opt.motion_dir = pjoin(opt.data_root, 'new_joint_vecs')
- opt.text_dir = pjoin(opt.data_root, 'texts')
- opt.joints_num = 22
- opt.dim_pose = 263
- opt.max_motion_length = 196
- opt.max_motion_frame = 196
- opt.max_motion_token = 55
- elif opt.dataset_name == 'kit':
- opt.data_root = './dataset/KIT-ML/'
- opt.motion_dir = pjoin(opt.data_root, 'new_joint_vecs')
- opt.text_dir = pjoin(opt.data_root, 'texts')
- opt.joints_num = 21
- opt.dim_pose = 251
- opt.max_motion_length = 196
- opt.max_motion_frame = 196
- opt.max_motion_token = 55
- else:
- raise KeyError('Dataset not recognized')
-
- opt.dim_word = 300
- opt.num_classes = 200 // opt.unit_length
- opt.is_train = False
- opt.is_continue = False
- opt.device = device
-
- return opt
\ No newline at end of file
diff --git a/spaces/AIGC-Audio/AudioGPT/text_to_audio/Make_An_Audio/ldm/models/diffusion/sampling_util.py b/spaces/AIGC-Audio/AudioGPT/text_to_audio/Make_An_Audio/ldm/models/diffusion/sampling_util.py
deleted file mode 100644
index 7eff02be6d7c54d43ee6680636ac0698dd3b3f33..0000000000000000000000000000000000000000
--- a/spaces/AIGC-Audio/AudioGPT/text_to_audio/Make_An_Audio/ldm/models/diffusion/sampling_util.py
+++ /dev/null
@@ -1,22 +0,0 @@
-import torch
-import numpy as np
-
-
-def append_dims(x, target_dims):
- """Appends dimensions to the end of a tensor until it has target_dims dimensions.
- From https://github.com/crowsonkb/k-diffusion/blob/master/k_diffusion/utils.py"""
- dims_to_append = target_dims - x.ndim
- if dims_to_append < 0:
- raise ValueError(f'input has {x.ndim} dims but target_dims is {target_dims}, which is less')
- return x[(...,) + (None,) * dims_to_append]
-
-
-def norm_thresholding(x0, value):
- s = append_dims(x0.pow(2).flatten(1).mean(1).sqrt().clamp(min=value), x0.ndim)
- return x0 * (value / s)
-
-
-def spatial_norm_thresholding(x0, value):
- # b c h w
- s = x0.pow(2).mean(1, keepdim=True).sqrt().clamp(min=value)
- return x0 * (value / s)
\ No newline at end of file
diff --git a/spaces/AIWaves/SOP_Generation-single/utils.py b/spaces/AIWaves/SOP_Generation-single/utils.py
deleted file mode 100644
index 275e6280a80187f9656987ee702e5719a0944e08..0000000000000000000000000000000000000000
--- a/spaces/AIWaves/SOP_Generation-single/utils.py
+++ /dev/null
@@ -1,482 +0,0 @@
-# coding=utf-8
-# Copyright 2023 The AIWaves Inc. team.
-
-#
-# Licensed under the Apache License, Version 2.0 (the "License");
-# you may not use this file except in compliance with the License.
-# You may obtain a copy of the License at
-#
-# http://www.apache.org/licenses/LICENSE-2.0
-#
-# Unless required by applicable law or agreed to in writing, software
-# distributed under the License is distributed on an "AS IS" BASIS,
-# WITHOUT WARRANTIES OR CONDITIONS OF ANY KIND, either express or implied.
-# See the License for the specific language governing permissions and
-# limitations under the License.
-"""helper functions for an LLM autonoumous agent"""
-import csv
-import random
-import json
-import pandas
-import numpy as np
-import requests
-import torch
-from tqdm import tqdm
-import re
-import datetime
-import string
-import random
-import os
-import openai
-from text2vec import semantic_search
-import re
-import datetime
-from langchain.document_loaders import UnstructuredFileLoader
-from langchain.text_splitter import CharacterTextSplitter
-from sentence_transformers import SentenceTransformer
-
-embed_model_name = os.environ["Embed_Model"] if "Embed_Model" in os.environ else "text-embedding-ada-002"
-if embed_model_name in ["text-embedding-ada-002"]:
- pass
-else:
- embedding_model = SentenceTransformer(
- embed_model_name, device=torch.device("cpu")
- )
-
-def get_embedding(sentence):
- if embed_model_name in ["text-embedding-ada-002"]:
- openai.api_key = os.environ["API_KEY"]
- if "PROXY" in os.environ:
- assert "http:" in os.environ["PROXY"] or "socks" in os.environ["PROXY"],"PROXY error,PROXY must be http or socks"
- openai.proxy = os.environ["PROXY"]
- if "API_BASE" in os.environ:
- openai.api_base = os.environ["API_BASE"]
- embedding_model = openai.Embedding
- embed = embedding_model.create(
- model=embed_model_name,
- input=sentence
- )
- embed = embed["data"][0]["embedding"]
- embed = torch.tensor(embed,dtype=torch.float32)
- else:
- embed = embedding_model.encode(sentence,convert_to_tensor=True)
- if len(embed.shape)==1:
- embed = embed.unsqueeze(0)
- return embed
-
-
-def get_code():
- return "".join(random.sample(string.ascii_letters + string.digits, 8))
-
-
-def get_content_between_a_b(start_tag, end_tag, text):
- """
-
- Args:
- start_tag (str): start_tag
- end_tag (str): end_tag
- text (str): complete sentence
-
- Returns:
- str: the content between start_tag and end_tag
- """
- extracted_text = ""
- start_index = text.find(start_tag)
- while start_index != -1:
- end_index = text.find(end_tag, start_index + len(start_tag))
- if end_index != -1:
- extracted_text += text[start_index +
- len(start_tag):end_index] + " "
- start_index = text.find(start_tag, end_index + len(end_tag))
- else:
- break
-
- return extracted_text.strip()
-
-
-def extract(text, type):
- """extract the content between
-
- Args:
- text (str): complete sentence
- type (str): tag
-
- Returns:
- str: content between
- """
- target_str = get_content_between_a_b(f"<{type}>", f"{type}>", text)
- return target_str
-
-def count_files_in_directory(directory):
- # 获取指定目录下的文件数目
- file_count = len([f for f in os.listdir(directory) if os.path.isfile(os.path.join(directory, f))])
- return file_count
-
-def delete_oldest_files(directory, num_to_keep):
- # 获取目录下文件列表,并按修改时间排序
- files = [(f, os.path.getmtime(os.path.join(directory, f))) for f in os.listdir(directory) if os.path.isfile(os.path.join(directory, f))]
-
- # 删除最开始的 num_to_keep 个文件
- for i in range(min(num_to_keep, len(files))):
- file_to_delete = os.path.join(directory, files[i][0])
- os.remove(file_to_delete)
-
-def delete_files_if_exceed_threshold(directory, threshold, num_to_keep):
- # 获取文件数目并进行处理
- file_count = count_files_in_directory(directory)
- if file_count > threshold:
- delete_count = file_count - num_to_keep
- delete_oldest_files(directory, delete_count)
-
-def save_logs(log_path, messages, response):
- if not os.path.exists(log_path):
- os.mkdir(log_path)
- delete_files_if_exceed_threshold(log_path, 20, 10)
- log_path = log_path if log_path else "logs"
- log = {}
- log["input"] = messages
- log["output"] = response
- os.makedirs(log_path, exist_ok=True)
- log_file = os.path.join(
- log_path,
- datetime.datetime.now().strftime("%Y-%m-%d-%H-%M-%S") + ".json")
- with open(log_file, "w", encoding="utf-8") as f:
- json.dump(log, f, ensure_ascii=False, indent=2)
-
-
-
-def semantic_search_word2vec(query_embedding, kb_embeddings, top_k):
- return semantic_search(query_embedding, kb_embeddings, top_k=top_k)
-
-
-def cut_sent(para):
- para = re.sub("([。!?\?])([^”’])", r"\1\n\2", para)
- para = re.sub("(\.{6})([^”’])", r"\1\n\2", para)
- para = re.sub("(\…{2})([^”’])", r"\1\n\2", para)
- para = re.sub("([。!?\?][”’])([^,。!?\?])", r"\1\n\2", para)
- para = para.rstrip()
- pieces = [i for i in para.split("\n") if i]
- batch_size = 3
- chucks = [
- " ".join(pieces[i:i + batch_size])
- for i in range(0, len(pieces), batch_size)
- ]
- return chucks
-
-
-def process_document(file_path):
- """
- Save QA_csv to json.
- Args:
- model: LLM to generate embeddings
- qa_dict: A dict contains Q&A
- save_path: where to save the json file.
- Json format:
- Dict[num,Dict[q:str,a:str,chunk:str,emb:List[float]]
- """
- final_dict = {}
- count = 0
- if file_path.endswith(".csv"):
- dataset = pandas.read_csv(file_path)
- questions = dataset["question"]
- answers = dataset["answer"]
- # embedding q+chunk
- for q, a in zip(questions, answers):
- for text in cut_sent(a):
- temp_dict = {}
- temp_dict["q"] = q
- temp_dict["a"] = a
- temp_dict["chunk"] = text
- temp_dict["emb"] = get_embedding(q + text).tolist()
- final_dict[count] = temp_dict
- count += 1
- # embedding chunk
- for q, a in zip(questions, answers):
- for text in cut_sent(a):
- temp_dict = {}
- temp_dict["q"] = q
- temp_dict["a"] = a
- temp_dict["chunk"] = text
- temp_dict["emb"] = get_embedding(text).tolist()
- final_dict[count] = temp_dict
- count += 1
- # embedding q
- for q, a in zip(questions, answers):
- temp_dict = {}
- temp_dict["q"] = q
- temp_dict["a"] = a
- temp_dict["chunk"] = a
- temp_dict["emb"] = get_embedding(q).tolist()
- final_dict[count] = temp_dict
- count += 1
- # embedding q+a
- for q, a in zip(questions, answers):
- temp_dict = {}
- temp_dict["q"] = q
- temp_dict["a"] = a
- temp_dict["chunk"] = a
- temp_dict["emb"] = get_embedding(q + a).tolist()
- final_dict[count] = temp_dict
- count += 1
- # embedding a
- for q, a in zip(questions, answers):
- temp_dict = {}
- temp_dict["q"] = q
- temp_dict["a"] = a
- temp_dict["chunk"] = a
- temp_dict["emb"] = get_embedding(a).tolist()
- final_dict[count] = temp_dict
- count += 1
- print(f"finish updating {len(final_dict)} data!")
- os.makedirs("temp_database", exist_ok=True)
- save_path = os.path.join(
- "temp_database/",
- file_path.split("/")[-1].replace("." + file_path.split(".")[1],
- ".json"),
- )
- print(save_path)
- with open(save_path, "w") as f:
- json.dump(final_dict, f, ensure_ascii=False, indent=2)
- return {"knowledge_base": save_path, "type": "QA"}
- else:
- loader = UnstructuredFileLoader(file_path)
- docs = loader.load()
- text_spiltter = CharacterTextSplitter(chunk_size=200,
- chunk_overlap=100)
- docs = text_spiltter.split_text(docs[0].page_content)
- os.makedirs("temp_database", exist_ok=True)
- save_path = os.path.join(
- "temp_database/",
- file_path.replace("." + file_path.split(".")[1], ".json"))
- final_dict = {}
- count = 0
- for c in tqdm(docs):
- temp_dict = {}
- temp_dict["chunk"] = c
- temp_dict["emb"] = get_embedding(c).tolist()
- final_dict[count] = temp_dict
- count += 1
- print(f"finish updating {len(final_dict)} data!")
- with open(save_path, "w") as f:
- json.dump(final_dict, f, ensure_ascii=False, indent=2)
- return {"knowledge_base": save_path, "type": "UnstructuredFile"}
-
-def load_knowledge_base_qa(path):
- """
- Load json format knowledge base.
- """
- print("path", path)
- with open(path, "r") as f:
- data = json.load(f)
- embeddings = []
- questions = []
- answers = []
- chunks = []
- for idx in range(len(data.keys())):
- embeddings.append(data[str(idx)]["emb"])
- questions.append(data[str(idx)]["q"])
- answers.append(data[str(idx)]["a"])
- chunks.append(data[str(idx)]["chunk"])
- embeddings = np.array(embeddings, dtype=np.float32)
- embeddings = torch.from_numpy(embeddings).squeeze()
- return embeddings, questions, answers, chunks
-
-
-def load_knowledge_base_UnstructuredFile(path):
- """
- Load json format knowledge base.
- """
- with open(path, "r") as f:
- data = json.load(f)
- embeddings = []
- chunks = []
- for idx in range(len(data.keys())):
- embeddings.append(data[str(idx)]["emb"])
- chunks.append(data[str(idx)]["chunk"])
- embeddings = np.array(embeddings, dtype=np.float32)
- embeddings = torch.from_numpy(embeddings).squeeze()
- return embeddings, chunks
-
-
-def cos_sim(a: torch.Tensor, b: torch.Tensor):
- """
- Computes the cosine similarity cos_sim(a[i], b[j]) for all i and j.
- :return: Matrix with res[i][j] = cos_sim(a[i], b[j])
- """
- if not isinstance(a, torch.Tensor):
- a = torch.tensor(a)
-
- if not isinstance(b, torch.Tensor):
- b = torch.tensor(b)
-
- if len(a.shape) == 1:
- a = a.unsqueeze(0)
-
- if len(b.shape) == 1:
- b = b.unsqueeze(0)
-
- a_norm = torch.nn.functional.normalize(a, p=2, dim=1)
- b_norm = torch.nn.functional.normalize(b, p=2, dim=1)
- return torch.mm(a_norm, b_norm.transpose(0, 1))
-
-
-def matching_a_b(a, b, requirements=None):
- a_embedder = get_embedding(a)
- # 获取embedder
- b_embeder = get_embedding(b)
- sim_scores = cos_sim(a_embedder, b_embeder)[0]
- return sim_scores
-
-
-def matching_category(inputtext,
- forest_name,
- requirements=None,
- cat_embedder=None,
- top_k=3):
- """
- Args:
- inputtext: the category name to be matched
- forest: search tree
- top_k: the default three highest scoring results
- Return:
- topk matching_result. List[List] [[top1_name,top2_name,top3_name],[top1_score,top2_score,top3_score]]
- """
-
- sim_scores = torch.zeros([100])
- if inputtext:
- input_embeder = get_embedding(inputtext)
- sim_scores = cos_sim(input_embeder, cat_embedder)[0]
-
- if requirements:
- requirements = requirements.split(" ")
- requirements_embedder = get_embedding(requirements)
- req_scores = cos_sim(requirements_embedder, cat_embedder)
- req_scores = torch.mean(req_scores, dim=0)
- total_scores = req_scores
- else:
- total_scores = sim_scores
-
- top_k_cat = torch.topk(total_scores, k=top_k)
- top_k_score, top_k_idx = top_k_cat[0], top_k_cat[1]
- top_k_name = [forest_name[top_k_idx[i]] for i in range(0, top_k)]
-
- return [top_k_name, top_k_score.tolist(), top_k_idx]
-
-
-def sample_with_order_preserved(lst, num):
- """Randomly sample from the list while maintaining the original order."""
- indices = list(range(len(lst)))
- sampled_indices = random.sample(indices, num)
- sampled_indices.sort() # 保持原顺序
- return [lst[i] for i in sampled_indices]
-
-
-def limit_values(data, max_values):
- """Reduce each key-value list in the dictionary to the specified size, keeping the order of the original list unchanged."""
- for key, values in data.items():
- if len(values) > max_values:
- data[key] = sample_with_order_preserved(values, max_values)
- return data
-
-
-def limit_keys(data, max_keys):
- """Reduce the dictionary to the specified number of keys."""
- keys = list(data.keys())
- if len(keys) > max_keys:
- keys = sample_with_order_preserved(keys, max_keys)
- data = {key: data[key] for key in keys}
- return data
-
-
-def flatten_dict(nested_dict):
- """
- flatten the dictionary
- """
- flattened_dict = {}
- for key, value in nested_dict.items():
- if isinstance(value, dict):
- flattened_subdict = flatten_dict(value)
- flattened_dict.update(flattened_subdict)
- else:
- flattened_dict[key] = value
- return flattened_dict
-
-
-def merge_list(list1, list2):
- for l in list2:
- if l not in list1:
- list1.append(l)
- return list1
-
-
-def Search_Engines(req):
- FETSIZE = eval(os.environ["FETSIZE"]) if "FETSIZE" in os.environ else 5
-
- new_dict = {"keyword": req, "catLeafName": "", "fetchSize": FETSIZE}
- url = os.environ["SHOPPING_SEARCH"]
- res = requests.post(
- url= url,
- json=new_dict,
- )
- user_dict = json.loads(res.text)
- if "data" in user_dict.keys():
- request_items = user_dict["data"]["items"] # 查询到的商品信息JSON
- top_category = user_dict["data"]["topCategories"]
- return request_items, top_category
- else:
- return []
-
-
-def search_with_api(requirements, categery):
-
- FETSIZE = eval(os.environ["FETSIZE"]) if "FETSIZE" in os.environ else 5
-
- request_items = []
- all_req_list = requirements.split(" ")
- count = 0
-
- while len(request_items) < FETSIZE and len(all_req_list) > 0:
- if count:
- all_req_list.pop(0)
- all_req = (" ").join(all_req_list)
- if categery not in all_req_list:
- all_req = all_req + " " + categery
- now_request_items, top_category = Search_Engines(all_req)
- request_items = merge_list(request_items, now_request_items)
- count += 1
- new_top = []
- for category in top_category:
- if "其它" in category or "其它" in category:
- continue
- else:
- new_top.append(category)
- if len(request_items) > FETSIZE:
- request_items = request_items[:FETSIZE]
- return request_items, new_top
-
-
-
-def get_relevant_history(query,history,embeddings):
- """
- Retrieve a list of key history entries based on a query using semantic search.
-
- Args:
- query (str): The input query for which key history is to be retrieved.
- history (list): A list of historical key entries.
- embeddings (numpy.ndarray): An array of embedding vectors for historical entries.
-
- Returns:
- list: A list of key history entries most similar to the query.
- """
- TOP_K = eval(os.environ["TOP_K"]) if "TOP_K" in os.environ else 2
- relevant_history = []
- query_embedding = get_embedding(query)
- hits = semantic_search(query_embedding, embeddings, top_k=min(TOP_K,embeddings.shape[0]))
- hits = hits[0]
- for hit in hits:
- matching_idx = hit["corpus_id"]
- try:
- relevant_history.append(history[matching_idx])
- except:
- return []
- return relevant_history
diff --git a/spaces/AMR-KELEG/ALDi/constants.py b/spaces/AMR-KELEG/ALDi/constants.py
deleted file mode 100644
index cbd591771f4d7d64b7e5b3fa6547aec41eb2183f..0000000000000000000000000000000000000000
--- a/spaces/AMR-KELEG/ALDi/constants.py
+++ /dev/null
@@ -1,4 +0,0 @@
-CHOICE_TEXT = "Input Text"
-CHOICE_FILE = "Upload File"
-TITLE = "ALDi: Arabic Level of Dialectness"
-MODEL_NAME = "AMR-KELEG/Sentence-ALDi"
diff --git a/spaces/Abhilashvj/planogram-compliance/utils/segment/loss.py b/spaces/Abhilashvj/planogram-compliance/utils/segment/loss.py
deleted file mode 100644
index effaadebd0d6f1b10846ec82cd93356cb3129624..0000000000000000000000000000000000000000
--- a/spaces/Abhilashvj/planogram-compliance/utils/segment/loss.py
+++ /dev/null
@@ -1,275 +0,0 @@
-import torch
-import torch.nn as nn
-import torch.nn.functional as F
-
-from ..general import xywh2xyxy
-from ..loss import FocalLoss, smooth_BCE
-from ..metrics import bbox_iou
-from ..torch_utils import de_parallel
-from .general import crop_mask
-
-
-class ComputeLoss:
- # Compute losses
- def __init__(self, model, autobalance=False, overlap=False):
- self.sort_obj_iou = False
- self.overlap = overlap
- device = next(model.parameters()).device # get model device
- h = model.hyp # hyperparameters
- self.device = device
-
- # Define criteria
- BCEcls = nn.BCEWithLogitsLoss(
- pos_weight=torch.tensor([h["cls_pw"]], device=device)
- )
- BCEobj = nn.BCEWithLogitsLoss(
- pos_weight=torch.tensor([h["obj_pw"]], device=device)
- )
-
- # Class label smoothing https://arxiv.org/pdf/1902.04103.pdf eqn 3
- self.cp, self.cn = smooth_BCE(
- eps=h.get("label_smoothing", 0.0)
- ) # positive, negative BCE targets
-
- # Focal loss
- g = h["fl_gamma"] # focal loss gamma
- if g > 0:
- BCEcls, BCEobj = FocalLoss(BCEcls, g), FocalLoss(BCEobj, g)
-
- m = de_parallel(model).model[-1] # Detect() module
- self.balance = {3: [4.0, 1.0, 0.4]}.get(
- m.nl, [4.0, 1.0, 0.25, 0.06, 0.02]
- ) # P3-P7
- self.ssi = (
- list(m.stride).index(16) if autobalance else 0
- ) # stride 16 index
- self.BCEcls, self.BCEobj, self.gr, self.hyp, self.autobalance = (
- BCEcls,
- BCEobj,
- 1.0,
- h,
- autobalance,
- )
- self.na = m.na # number of anchors
- self.nc = m.nc # number of classes
- self.nl = m.nl # number of layers
- self.nm = m.nm # number of masks
- self.anchors = m.anchors
- self.device = device
-
- def __call__(self, preds, targets, masks): # predictions, targets, model
- p, proto = preds
- (
- bs,
- nm,
- mask_h,
- mask_w,
- ) = proto.shape # batch size, number of masks, mask height, mask width
- lcls = torch.zeros(1, device=self.device)
- lbox = torch.zeros(1, device=self.device)
- lobj = torch.zeros(1, device=self.device)
- lseg = torch.zeros(1, device=self.device)
- tcls, tbox, indices, anchors, tidxs, xywhn = self.build_targets(
- p, targets
- ) # targets
-
- # Losses
- for i, pi in enumerate(p): # layer index, layer predictions
- b, a, gj, gi = indices[i] # image, anchor, gridy, gridx
- tobj = torch.zeros(
- pi.shape[:4], dtype=pi.dtype, device=self.device
- ) # target obj
-
- n = b.shape[0] # number of targets
- if n:
- pxy, pwh, _, pcls, pmask = pi[b, a, gj, gi].split(
- (2, 2, 1, self.nc, nm), 1
- ) # subset of predictions
-
- # Box regression
- pxy = pxy.sigmoid() * 2 - 0.5
- pwh = (pwh.sigmoid() * 2) ** 2 * anchors[i]
- pbox = torch.cat((pxy, pwh), 1) # predicted box
- iou = bbox_iou(
- pbox, tbox[i], CIoU=True
- ).squeeze() # iou(prediction, target)
- lbox += (1.0 - iou).mean() # iou loss
-
- # Objectness
- iou = iou.detach().clamp(0).type(tobj.dtype)
- if self.sort_obj_iou:
- j = iou.argsort()
- b, a, gj, gi, iou = b[j], a[j], gj[j], gi[j], iou[j]
- if self.gr < 1:
- iou = (1.0 - self.gr) + self.gr * iou
- tobj[b, a, gj, gi] = iou # iou ratio
-
- # Classification
- if self.nc > 1: # cls loss (only if multiple classes)
- t = torch.full_like(
- pcls, self.cn, device=self.device
- ) # targets
- t[range(n), tcls[i]] = self.cp
- lcls += self.BCEcls(pcls, t) # BCE
-
- # Mask regression
- if tuple(masks.shape[-2:]) != (mask_h, mask_w): # downsample
- masks = F.interpolate(
- masks[None], (mask_h, mask_w), mode="nearest"
- )[0]
- marea = xywhn[i][:, 2:].prod(
- 1
- ) # mask width, height normalized
- mxyxy = xywh2xyxy(
- xywhn[i]
- * torch.tensor(
- [mask_w, mask_h, mask_w, mask_h], device=self.device
- )
- )
- for bi in b.unique():
- j = b == bi # matching index
- if self.overlap:
- mask_gti = torch.where(
- masks[bi][None] == tidxs[i][j].view(-1, 1, 1),
- 1.0,
- 0.0,
- )
- else:
- mask_gti = masks[tidxs[i]][j]
- lseg += self.single_mask_loss(
- mask_gti, pmask[j], proto[bi], mxyxy[j], marea[j]
- )
-
- obji = self.BCEobj(pi[..., 4], tobj)
- lobj += obji * self.balance[i] # obj loss
- if self.autobalance:
- self.balance[i] = (
- self.balance[i] * 0.9999 + 0.0001 / obji.detach().item()
- )
-
- if self.autobalance:
- self.balance = [x / self.balance[self.ssi] for x in self.balance]
- lbox *= self.hyp["box"]
- lobj *= self.hyp["obj"]
- lcls *= self.hyp["cls"]
- lseg *= self.hyp["box"] / bs
-
- loss = lbox + lobj + lcls + lseg
- return loss * bs, torch.cat((lbox, lseg, lobj, lcls)).detach()
-
- def single_mask_loss(self, gt_mask, pred, proto, xyxy, area):
- # Mask loss for one image
- pred_mask = (pred @ proto.view(self.nm, -1)).view(
- -1, *proto.shape[1:]
- ) # (n,32) @ (32,80,80) -> (n,80,80)
- loss = F.binary_cross_entropy_with_logits(
- pred_mask, gt_mask, reduction="none"
- )
- return (crop_mask(loss, xyxy).mean(dim=(1, 2)) / area).mean()
-
- def build_targets(self, p, targets):
- # Build targets for compute_loss(), input targets(image,class,x,y,w,h)
- na, nt = self.na, targets.shape[0] # number of anchors, targets
- tcls, tbox, indices, anch, tidxs, xywhn = [], [], [], [], [], []
- gain = torch.ones(
- 8, device=self.device
- ) # normalized to gridspace gain
- ai = (
- torch.arange(na, device=self.device)
- .float()
- .view(na, 1)
- .repeat(1, nt)
- ) # same as .repeat_interleave(nt)
- if self.overlap:
- batch = p[0].shape[0]
- ti = []
- for i in range(batch):
- num = (
- targets[:, 0] == i
- ).sum() # find number of targets of each image
- ti.append(
- torch.arange(num, device=self.device)
- .float()
- .view(1, num)
- .repeat(na, 1)
- + 1
- ) # (na, num)
- ti = torch.cat(ti, 1) # (na, nt)
- else:
- ti = (
- torch.arange(nt, device=self.device)
- .float()
- .view(1, nt)
- .repeat(na, 1)
- )
- targets = torch.cat(
- (targets.repeat(na, 1, 1), ai[..., None], ti[..., None]), 2
- ) # append anchor indices
-
- g = 0.5 # bias
- off = (
- torch.tensor(
- [
- [0, 0],
- [1, 0],
- [0, 1],
- [-1, 0],
- [0, -1], # j,k,l,m
- # [1, 1], [1, -1], [-1, 1], [-1, -1], # jk,jm,lk,lm
- ],
- device=self.device,
- ).float()
- * g
- ) # offsets
-
- for i in range(self.nl):
- anchors, shape = self.anchors[i], p[i].shape
- gain[2:6] = torch.tensor(shape)[[3, 2, 3, 2]] # xyxy gain
-
- # Match targets to anchors
- t = targets * gain # shape(3,n,7)
- if nt:
- # Matches
- r = t[..., 4:6] / anchors[:, None] # wh ratio
- j = (
- torch.max(r, 1 / r).max(2)[0] < self.hyp["anchor_t"]
- ) # compare
- # j = wh_iou(anchors, t[:, 4:6]) > model.hyp['iou_t'] # iou(3,n)=wh_iou(anchors(3,2), gwh(n,2))
- t = t[j] # filter
-
- # Offsets
- gxy = t[:, 2:4] # grid xy
- gxi = gain[[2, 3]] - gxy # inverse
- j, k = ((gxy % 1 < g) & (gxy > 1)).T
- l, m = ((gxi % 1 < g) & (gxi > 1)).T
- j = torch.stack((torch.ones_like(j), j, k, l, m))
- t = t.repeat((5, 1, 1))[j]
- offsets = (torch.zeros_like(gxy)[None] + off[:, None])[j]
- else:
- t = targets[0]
- offsets = 0
-
- # Define
- bc, gxy, gwh, at = t.chunk(
- 4, 1
- ) # (image, class), grid xy, grid wh, anchors
- (a, tidx), (b, c) = (
- at.long().T,
- bc.long().T,
- ) # anchors, image, class
- gij = (gxy - offsets).long()
- gi, gj = gij.T # grid indices
-
- # Append
- indices.append(
- (b, a, gj.clamp_(0, shape[2] - 1), gi.clamp_(0, shape[3] - 1))
- ) # image, anchor, grid
- tbox.append(torch.cat((gxy - gij, gwh), 1)) # box
- anch.append(anchors[a]) # anchors
- tcls.append(c) # class
- tidxs.append(tidx)
- xywhn.append(
- torch.cat((gxy, gwh), 1) / gain[2:6]
- ) # xywh normalized
-
- return tcls, tbox, indices, anch, tidxs, xywhn
diff --git a/spaces/AchyuthGamer/OpenGPT/g4f/Provider/GetGpt.py b/spaces/AchyuthGamer/OpenGPT/g4f/Provider/GetGpt.py
deleted file mode 100644
index b96efaac78d8c2443d53e584b8bc9fae50de3114..0000000000000000000000000000000000000000
--- a/spaces/AchyuthGamer/OpenGPT/g4f/Provider/GetGpt.py
+++ /dev/null
@@ -1,88 +0,0 @@
-from __future__ import annotations
-
-import json
-import os
-import uuid
-
-import requests
-from Crypto.Cipher import AES
-
-from ..typing import Any, CreateResult
-from .base_provider import BaseProvider
-
-
-class GetGpt(BaseProvider):
- url = 'https://chat.getgpt.world/'
- supports_stream = True
- working = False
- supports_gpt_35_turbo = True
-
- @staticmethod
- def create_completion(
- model: str,
- messages: list[dict[str, str]],
- stream: bool, **kwargs: Any) -> CreateResult:
-
- headers = {
- 'Content-Type' : 'application/json',
- 'Referer' : 'https://chat.getgpt.world/',
- 'user-agent' : 'Mozilla/5.0 (Windows NT 10.0; Win64; x64) AppleWebKit/537.36 (KHTML, like Gecko) Chrome/114.0.0.0 Safari/537.36',
- }
-
- data = json.dumps(
- {
- 'messages' : messages,
- 'frequency_penalty' : kwargs.get('frequency_penalty', 0),
- 'max_tokens' : kwargs.get('max_tokens', 4000),
- 'model' : 'gpt-3.5-turbo',
- 'presence_penalty' : kwargs.get('presence_penalty', 0),
- 'temperature' : kwargs.get('temperature', 1),
- 'top_p' : kwargs.get('top_p', 1),
- 'stream' : True,
- 'uuid' : str(uuid.uuid4())
- }
- )
-
- res = requests.post('https://chat.getgpt.world/api/chat/stream',
- headers=headers, json={'signature': _encrypt(data)}, stream=True)
-
- res.raise_for_status()
- for line in res.iter_lines():
- if b'content' in line:
- line_json = json.loads(line.decode('utf-8').split('data: ')[1])
- yield (line_json['choices'][0]['delta']['content'])
-
- @classmethod
- @property
- def params(cls):
- params = [
- ('model', 'str'),
- ('messages', 'list[dict[str, str]]'),
- ('stream', 'bool'),
- ('temperature', 'float'),
- ('presence_penalty', 'int'),
- ('frequency_penalty', 'int'),
- ('top_p', 'int'),
- ('max_tokens', 'int'),
- ]
- param = ', '.join([': '.join(p) for p in params])
- return f'g4f.provider.{cls.__name__} supports: ({param})'
-
-
-def _encrypt(e: str):
- t = os.urandom(8).hex().encode('utf-8')
- n = os.urandom(8).hex().encode('utf-8')
- r = e.encode('utf-8')
-
- cipher = AES.new(t, AES.MODE_CBC, n)
- ciphertext = cipher.encrypt(_pad_data(r))
-
- return ciphertext.hex() + t.decode('utf-8') + n.decode('utf-8')
-
-
-def _pad_data(data: bytes) -> bytes:
- block_size = AES.block_size
- padding_size = block_size - len(data) % block_size
- padding = bytes([padding_size] * padding_size)
-
- return data + padding
diff --git a/spaces/AgentVerse/agentVerse/ui/src/phaser3-rex-plugins/plugins/imagebox-plugin.js b/spaces/AgentVerse/agentVerse/ui/src/phaser3-rex-plugins/plugins/imagebox-plugin.js
deleted file mode 100644
index b13dd39dad69a765abecc3f310e66cc7c3df0e24..0000000000000000000000000000000000000000
--- a/spaces/AgentVerse/agentVerse/ui/src/phaser3-rex-plugins/plugins/imagebox-plugin.js
+++ /dev/null
@@ -1,23 +0,0 @@
-import Factory from './gameobjects/container/imagebox/Factory.js';
-import Creator from './gameobjects/container/imagebox/Creator.js';
-import ImageBox from './gameobjects/container/imagebox/ImageBox.js';
-import SetValue from './utils/object/SetValue.js';
-
-class ImageBoxPlugin extends Phaser.Plugins.BasePlugin {
-
- constructor(pluginManager) {
- super(pluginManager);
-
- // Register our new Game Object type
- pluginManager.registerGameObject('rexImageBox', Factory, Creator);
- }
-
- start() {
- var eventEmitter = this.game.events;
- eventEmitter.on('destroy', this.destroy, this);
- }
-}
-
-SetValue(window, 'RexPlugins.GameObjects.ImageBox', ImageBox);
-
-export default ImageBoxPlugin;
\ No newline at end of file
diff --git a/spaces/AgentVerse/agentVerse/ui/src/phaser3-rex-plugins/templates/ui/canvasinput/CanvasInput.js b/spaces/AgentVerse/agentVerse/ui/src/phaser3-rex-plugins/templates/ui/canvasinput/CanvasInput.js
deleted file mode 100644
index 318c7182fb69ed5562fe4e8017c0dc3584fa6e24..0000000000000000000000000000000000000000
--- a/spaces/AgentVerse/agentVerse/ui/src/phaser3-rex-plugins/templates/ui/canvasinput/CanvasInput.js
+++ /dev/null
@@ -1,2 +0,0 @@
-import CanvasInput from '../../../plugins/canvasinput.js';
-export default CanvasInput;
\ No newline at end of file
diff --git a/spaces/AiMimicry/sovits-models/onnxexport/model_onnx.py b/spaces/AiMimicry/sovits-models/onnxexport/model_onnx.py
deleted file mode 100644
index e28bae95ec1e53aa05d06fc784ff86d55f228d60..0000000000000000000000000000000000000000
--- a/spaces/AiMimicry/sovits-models/onnxexport/model_onnx.py
+++ /dev/null
@@ -1,335 +0,0 @@
-import torch
-from torch import nn
-from torch.nn import functional as F
-
-import modules.attentions as attentions
-import modules.commons as commons
-import modules.modules as modules
-
-from torch.nn import Conv1d, ConvTranspose1d, AvgPool1d, Conv2d
-from torch.nn.utils import weight_norm, remove_weight_norm, spectral_norm
-
-import utils
-from modules.commons import init_weights, get_padding
-from vdecoder.hifigan.models import Generator
-from utils import f0_to_coarse
-
-
-class ResidualCouplingBlock(nn.Module):
- def __init__(self,
- channels,
- hidden_channels,
- kernel_size,
- dilation_rate,
- n_layers,
- n_flows=4,
- gin_channels=0):
- super().__init__()
- self.channels = channels
- self.hidden_channels = hidden_channels
- self.kernel_size = kernel_size
- self.dilation_rate = dilation_rate
- self.n_layers = n_layers
- self.n_flows = n_flows
- self.gin_channels = gin_channels
-
- self.flows = nn.ModuleList()
- for i in range(n_flows):
- self.flows.append(
- modules.ResidualCouplingLayer(channels, hidden_channels, kernel_size, dilation_rate, n_layers,
- gin_channels=gin_channels, mean_only=True))
- self.flows.append(modules.Flip())
-
- def forward(self, x, x_mask, g=None, reverse=False):
- if not reverse:
- for flow in self.flows:
- x, _ = flow(x, x_mask, g=g, reverse=reverse)
- else:
- for flow in reversed(self.flows):
- x = flow(x, x_mask, g=g, reverse=reverse)
- return x
-
-
-class Encoder(nn.Module):
- def __init__(self,
- in_channels,
- out_channels,
- hidden_channels,
- kernel_size,
- dilation_rate,
- n_layers,
- gin_channels=0):
- super().__init__()
- self.in_channels = in_channels
- self.out_channels = out_channels
- self.hidden_channels = hidden_channels
- self.kernel_size = kernel_size
- self.dilation_rate = dilation_rate
- self.n_layers = n_layers
- self.gin_channels = gin_channels
-
- self.pre = nn.Conv1d(in_channels, hidden_channels, 1)
- self.enc = modules.WN(hidden_channels, kernel_size, dilation_rate, n_layers, gin_channels=gin_channels)
- self.proj = nn.Conv1d(hidden_channels, out_channels * 2, 1)
-
- def forward(self, x, x_lengths, g=None):
- # print(x.shape,x_lengths.shape)
- x_mask = torch.unsqueeze(commons.sequence_mask(x_lengths, x.size(2)), 1).to(x.dtype)
- x = self.pre(x) * x_mask
- x = self.enc(x, x_mask, g=g)
- stats = self.proj(x) * x_mask
- m, logs = torch.split(stats, self.out_channels, dim=1)
- z = (m + torch.randn_like(m) * torch.exp(logs)) * x_mask
- return z, m, logs, x_mask
-
-
-class TextEncoder(nn.Module):
- def __init__(self,
- out_channels,
- hidden_channels,
- kernel_size,
- n_layers,
- gin_channels=0,
- filter_channels=None,
- n_heads=None,
- p_dropout=None):
- super().__init__()
- self.out_channels = out_channels
- self.hidden_channels = hidden_channels
- self.kernel_size = kernel_size
- self.n_layers = n_layers
- self.gin_channels = gin_channels
- self.proj = nn.Conv1d(hidden_channels, out_channels * 2, 1)
- self.f0_emb = nn.Embedding(256, hidden_channels)
-
- self.enc_ = attentions.Encoder(
- hidden_channels,
- filter_channels,
- n_heads,
- n_layers,
- kernel_size,
- p_dropout)
-
- def forward(self, x, x_mask, f0=None, z=None):
- x = x + self.f0_emb(f0).transpose(1, 2)
- x = self.enc_(x * x_mask, x_mask)
- stats = self.proj(x) * x_mask
- m, logs = torch.split(stats, self.out_channels, dim=1)
- z = (m + z * torch.exp(logs)) * x_mask
- return z, m, logs, x_mask
-
-
-class DiscriminatorP(torch.nn.Module):
- def __init__(self, period, kernel_size=5, stride=3, use_spectral_norm=False):
- super(DiscriminatorP, self).__init__()
- self.period = period
- self.use_spectral_norm = use_spectral_norm
- norm_f = weight_norm if use_spectral_norm == False else spectral_norm
- self.convs = nn.ModuleList([
- norm_f(Conv2d(1, 32, (kernel_size, 1), (stride, 1), padding=(get_padding(kernel_size, 1), 0))),
- norm_f(Conv2d(32, 128, (kernel_size, 1), (stride, 1), padding=(get_padding(kernel_size, 1), 0))),
- norm_f(Conv2d(128, 512, (kernel_size, 1), (stride, 1), padding=(get_padding(kernel_size, 1), 0))),
- norm_f(Conv2d(512, 1024, (kernel_size, 1), (stride, 1), padding=(get_padding(kernel_size, 1), 0))),
- norm_f(Conv2d(1024, 1024, (kernel_size, 1), 1, padding=(get_padding(kernel_size, 1), 0))),
- ])
- self.conv_post = norm_f(Conv2d(1024, 1, (3, 1), 1, padding=(1, 0)))
-
- def forward(self, x):
- fmap = []
-
- # 1d to 2d
- b, c, t = x.shape
- if t % self.period != 0: # pad first
- n_pad = self.period - (t % self.period)
- x = F.pad(x, (0, n_pad), "reflect")
- t = t + n_pad
- x = x.view(b, c, t // self.period, self.period)
-
- for l in self.convs:
- x = l(x)
- x = F.leaky_relu(x, modules.LRELU_SLOPE)
- fmap.append(x)
- x = self.conv_post(x)
- fmap.append(x)
- x = torch.flatten(x, 1, -1)
-
- return x, fmap
-
-
-class DiscriminatorS(torch.nn.Module):
- def __init__(self, use_spectral_norm=False):
- super(DiscriminatorS, self).__init__()
- norm_f = weight_norm if use_spectral_norm == False else spectral_norm
- self.convs = nn.ModuleList([
- norm_f(Conv1d(1, 16, 15, 1, padding=7)),
- norm_f(Conv1d(16, 64, 41, 4, groups=4, padding=20)),
- norm_f(Conv1d(64, 256, 41, 4, groups=16, padding=20)),
- norm_f(Conv1d(256, 1024, 41, 4, groups=64, padding=20)),
- norm_f(Conv1d(1024, 1024, 41, 4, groups=256, padding=20)),
- norm_f(Conv1d(1024, 1024, 5, 1, padding=2)),
- ])
- self.conv_post = norm_f(Conv1d(1024, 1, 3, 1, padding=1))
-
- def forward(self, x):
- fmap = []
-
- for l in self.convs:
- x = l(x)
- x = F.leaky_relu(x, modules.LRELU_SLOPE)
- fmap.append(x)
- x = self.conv_post(x)
- fmap.append(x)
- x = torch.flatten(x, 1, -1)
-
- return x, fmap
-
-
-class F0Decoder(nn.Module):
- def __init__(self,
- out_channels,
- hidden_channels,
- filter_channels,
- n_heads,
- n_layers,
- kernel_size,
- p_dropout,
- spk_channels=0):
- super().__init__()
- self.out_channels = out_channels
- self.hidden_channels = hidden_channels
- self.filter_channels = filter_channels
- self.n_heads = n_heads
- self.n_layers = n_layers
- self.kernel_size = kernel_size
- self.p_dropout = p_dropout
- self.spk_channels = spk_channels
-
- self.prenet = nn.Conv1d(hidden_channels, hidden_channels, 3, padding=1)
- self.decoder = attentions.FFT(
- hidden_channels,
- filter_channels,
- n_heads,
- n_layers,
- kernel_size,
- p_dropout)
- self.proj = nn.Conv1d(hidden_channels, out_channels, 1)
- self.f0_prenet = nn.Conv1d(1, hidden_channels, 3, padding=1)
- self.cond = nn.Conv1d(spk_channels, hidden_channels, 1)
-
- def forward(self, x, norm_f0, x_mask, spk_emb=None):
- x = torch.detach(x)
- if spk_emb is not None:
- x = x + self.cond(spk_emb)
- x += self.f0_prenet(norm_f0)
- x = self.prenet(x) * x_mask
- x = self.decoder(x * x_mask, x_mask)
- x = self.proj(x) * x_mask
- return x
-
-
-class SynthesizerTrn(nn.Module):
- """
- Synthesizer for Training
- """
-
- def __init__(self,
- spec_channels,
- segment_size,
- inter_channels,
- hidden_channels,
- filter_channels,
- n_heads,
- n_layers,
- kernel_size,
- p_dropout,
- resblock,
- resblock_kernel_sizes,
- resblock_dilation_sizes,
- upsample_rates,
- upsample_initial_channel,
- upsample_kernel_sizes,
- gin_channels,
- ssl_dim,
- n_speakers,
- sampling_rate=44100,
- **kwargs):
- super().__init__()
- self.spec_channels = spec_channels
- self.inter_channels = inter_channels
- self.hidden_channels = hidden_channels
- self.filter_channels = filter_channels
- self.n_heads = n_heads
- self.n_layers = n_layers
- self.kernel_size = kernel_size
- self.p_dropout = p_dropout
- self.resblock = resblock
- self.resblock_kernel_sizes = resblock_kernel_sizes
- self.resblock_dilation_sizes = resblock_dilation_sizes
- self.upsample_rates = upsample_rates
- self.upsample_initial_channel = upsample_initial_channel
- self.upsample_kernel_sizes = upsample_kernel_sizes
- self.segment_size = segment_size
- self.gin_channels = gin_channels
- self.ssl_dim = ssl_dim
- self.emb_g = nn.Embedding(n_speakers, gin_channels)
-
- self.pre = nn.Conv1d(ssl_dim, hidden_channels, kernel_size=5, padding=2)
-
- self.enc_p = TextEncoder(
- inter_channels,
- hidden_channels,
- filter_channels=filter_channels,
- n_heads=n_heads,
- n_layers=n_layers,
- kernel_size=kernel_size,
- p_dropout=p_dropout
- )
- hps = {
- "sampling_rate": sampling_rate,
- "inter_channels": inter_channels,
- "resblock": resblock,
- "resblock_kernel_sizes": resblock_kernel_sizes,
- "resblock_dilation_sizes": resblock_dilation_sizes,
- "upsample_rates": upsample_rates,
- "upsample_initial_channel": upsample_initial_channel,
- "upsample_kernel_sizes": upsample_kernel_sizes,
- "gin_channels": gin_channels,
- }
- self.dec = Generator(h=hps)
- self.enc_q = Encoder(spec_channels, inter_channels, hidden_channels, 5, 1, 16, gin_channels=gin_channels)
- self.flow = ResidualCouplingBlock(inter_channels, hidden_channels, 5, 1, 4, gin_channels=gin_channels)
- self.f0_decoder = F0Decoder(
- 1,
- hidden_channels,
- filter_channels,
- n_heads,
- n_layers,
- kernel_size,
- p_dropout,
- spk_channels=gin_channels
- )
- self.emb_uv = nn.Embedding(2, hidden_channels)
- self.predict_f0 = False
-
- def forward(self, c, f0, mel2ph, uv, noise=None, g=None):
-
- decoder_inp = F.pad(c, [0, 0, 1, 0])
- mel2ph_ = mel2ph.unsqueeze(2).repeat([1, 1, c.shape[-1]])
- c = torch.gather(decoder_inp, 1, mel2ph_).transpose(1, 2) # [B, T, H]
-
- c_lengths = (torch.ones(c.size(0)) * c.size(-1)).to(c.device)
- g = g.unsqueeze(0)
- g = self.emb_g(g).transpose(1, 2)
- x_mask = torch.unsqueeze(commons.sequence_mask(c_lengths, c.size(2)), 1).to(c.dtype)
- x = self.pre(c) * x_mask + self.emb_uv(uv.long()).transpose(1, 2)
-
- if self.predict_f0:
- lf0 = 2595. * torch.log10(1. + f0.unsqueeze(1) / 700.) / 500
- norm_lf0 = utils.normalize_f0(lf0, x_mask, uv, random_scale=False)
- pred_lf0 = self.f0_decoder(x, norm_lf0, x_mask, spk_emb=g)
- f0 = (700 * (torch.pow(10, pred_lf0 * 500 / 2595) - 1)).squeeze(1)
-
- z_p, m_p, logs_p, c_mask = self.enc_p(x, x_mask, f0=f0_to_coarse(f0), z=noise)
- z = self.flow(z_p, c_mask, g=g, reverse=True)
- o = self.dec(z * c_mask, g=g, f0=f0)
- return o
diff --git a/spaces/AlekseyKorshuk/rugpt3/app.py b/spaces/AlekseyKorshuk/rugpt3/app.py
deleted file mode 100644
index 2fc87527a16fe9d99d182452eb93005c461beca9..0000000000000000000000000000000000000000
--- a/spaces/AlekseyKorshuk/rugpt3/app.py
+++ /dev/null
@@ -1,30 +0,0 @@
-import gradio as gr
-from gradio import mix
-
-title = "RUGPT3"
-description = "Gradio Demo for RUGPT3. To use it, simply add your text, or click one of the examples to load them. Read more at the links below."
-
-
-examples = [
- ['Меня зовут Томас и мой основной']
-]
-
-io = gr.Interface.load("models/sberbank-ai/rugpt3large_based_on_gpt2")
-
-
-
-def inference(text):
-
- return io(text)
-
-
-
-gr.Interface(
- inference,
- [gr.inputs.Textbox(label="Input")],
- gr.outputs.Textbox(label="Output"),
- examples=examples,
- # article=article,
- title=title,
- description=description).launch(enable_queue=True, cache_examples=True)
-
\ No newline at end of file
diff --git a/spaces/Alpaca233/SadTalker/webui.bat b/spaces/Alpaca233/SadTalker/webui.bat
deleted file mode 100644
index 6ff83231242ac2260c38a2a4a7ba030aa707b1a3..0000000000000000000000000000000000000000
--- a/spaces/Alpaca233/SadTalker/webui.bat
+++ /dev/null
@@ -1,17 +0,0 @@
-@echo off
-
-IF NOT EXIST venv (
-python -m venv venv
-) ELSE (
-echo venv folder already exists, skipping creation...
-)
-call .\venv\Scripts\activate.bat
-
-set PYTHON="venv\Scripts\Python.exe"
-echo venv %PYTHON%
-
-%PYTHON% Launcher.py
-
-echo.
-echo Launch unsuccessful. Exiting.
-pause
\ No newline at end of file
diff --git a/spaces/Amon1/ChatGPTForAcadamic/crazy_functions/__init__.py b/spaces/Amon1/ChatGPTForAcadamic/crazy_functions/__init__.py
deleted file mode 100644
index e69de29bb2d1d6434b8b29ae775ad8c2e48c5391..0000000000000000000000000000000000000000
diff --git a/spaces/Androidonnxfork/CivitAi-to-Diffusers/diffusers/docs/source/en/api/pipelines/text_to_video_zero.md b/spaces/Androidonnxfork/CivitAi-to-Diffusers/diffusers/docs/source/en/api/pipelines/text_to_video_zero.md
deleted file mode 100644
index b64d72db0187a4619751ec777d3b7c40f938ec6f..0000000000000000000000000000000000000000
--- a/spaces/Androidonnxfork/CivitAi-to-Diffusers/diffusers/docs/source/en/api/pipelines/text_to_video_zero.md
+++ /dev/null
@@ -1,260 +0,0 @@
-
-
-# Text2Video-Zero
-
-[Text2Video-Zero: Text-to-Image Diffusion Models are Zero-Shot Video Generators](https://huggingface.co/papers/2303.13439) is by
-Levon Khachatryan,
-Andranik Movsisyan,
-Vahram Tadevosyan,
-Roberto Henschel,
-[Zhangyang Wang](https://www.ece.utexas.edu/people/faculty/atlas-wang), Shant Navasardyan, [Humphrey Shi](https://www.humphreyshi.com).
-
-Text2Video-Zero enables zero-shot video generation using either:
-1. A textual prompt
-2. A prompt combined with guidance from poses or edges
-3. Video Instruct-Pix2Pix (instruction-guided video editing)
-
-Results are temporally consistent and closely follow the guidance and textual prompts.
-
-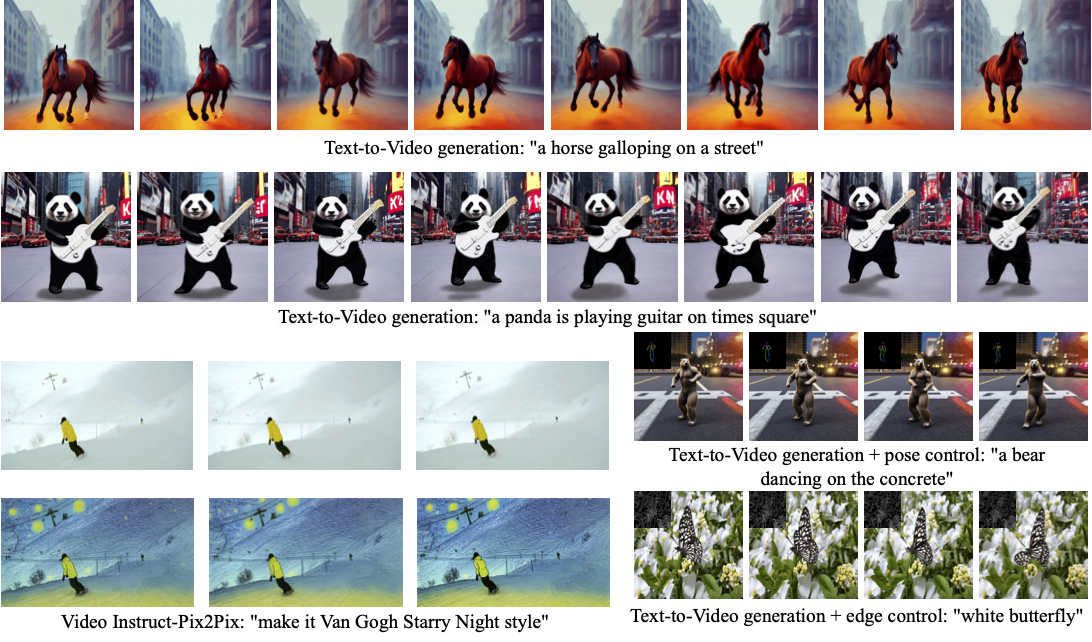
-
-The abstract from the paper is:
-
-*Recent text-to-video generation approaches rely on computationally heavy training and require large-scale video datasets. In this paper, we introduce a new task of zero-shot text-to-video generation and propose a low-cost approach (without any training or optimization) by leveraging the power of existing text-to-image synthesis methods (e.g., Stable Diffusion), making them suitable for the video domain.
-Our key modifications include (i) enriching the latent codes of the generated frames with motion dynamics to keep the global scene and the background time consistent; and (ii) reprogramming frame-level self-attention using a new cross-frame attention of each frame on the first frame, to preserve the context, appearance, and identity of the foreground object.
-Experiments show that this leads to low overhead, yet high-quality and remarkably consistent video generation. Moreover, our approach is not limited to text-to-video synthesis but is also applicable to other tasks such as conditional and content-specialized video generation, and Video Instruct-Pix2Pix, i.e., instruction-guided video editing.
-As experiments show, our method performs comparably or sometimes better than recent approaches, despite not being trained on additional video data.*
-
-You can find additional information about Text-to-Video Zero on the [project page](https://text2video-zero.github.io/), [paper](https://arxiv.org/abs/2303.13439), and [original codebase](https://github.com/Picsart-AI-Research/Text2Video-Zero).
-
-## Usage example
-
-### Text-To-Video
-
-To generate a video from prompt, run the following python command
-```python
-import torch
-import imageio
-from diffusers import TextToVideoZeroPipeline
-
-model_id = "runwayml/stable-diffusion-v1-5"
-pipe = TextToVideoZeroPipeline.from_pretrained(model_id, torch_dtype=torch.float16).to("cuda")
-
-prompt = "A panda is playing guitar on times square"
-result = pipe(prompt=prompt).images
-result = [(r * 255).astype("uint8") for r in result]
-imageio.mimsave("video.mp4", result, fps=4)
-```
-You can change these parameters in the pipeline call:
-* Motion field strength (see the [paper](https://arxiv.org/abs/2303.13439), Sect. 3.3.1):
- * `motion_field_strength_x` and `motion_field_strength_y`. Default: `motion_field_strength_x=12`, `motion_field_strength_y=12`
-* `T` and `T'` (see the [paper](https://arxiv.org/abs/2303.13439), Sect. 3.3.1)
- * `t0` and `t1` in the range `{0, ..., num_inference_steps}`. Default: `t0=45`, `t1=48`
-* Video length:
- * `video_length`, the number of frames video_length to be generated. Default: `video_length=8`
-
-We an also generate longer videos by doing the processing in a chunk-by-chunk manner:
-```python
-import torch
-import imageio
-from diffusers import TextToVideoZeroPipeline
-import numpy as np
-
-model_id = "runwayml/stable-diffusion-v1-5"
-pipe = TextToVideoZeroPipeline.from_pretrained(model_id, torch_dtype=torch.float16).to("cuda")
-seed = 0
-video_length = 8
-chunk_size = 4
-prompt = "A panda is playing guitar on times square"
-
-# Generate the video chunk-by-chunk
-result = []
-chunk_ids = np.arange(0, video_length, chunk_size - 1)
-generator = torch.Generator(device="cuda")
-for i in range(len(chunk_ids)):
- print(f"Processing chunk {i + 1} / {len(chunk_ids)}")
- ch_start = chunk_ids[i]
- ch_end = video_length if i == len(chunk_ids) - 1 else chunk_ids[i + 1]
- # Attach the first frame for Cross Frame Attention
- frame_ids = [0] + list(range(ch_start, ch_end))
- # Fix the seed for the temporal consistency
- generator.manual_seed(seed)
- output = pipe(prompt=prompt, video_length=len(frame_ids), generator=generator, frame_ids=frame_ids)
- result.append(output.images[1:])
-
-# Concatenate chunks and save
-result = np.concatenate(result)
-result = [(r * 255).astype("uint8") for r in result]
-imageio.mimsave("video.mp4", result, fps=4)
-```
-
-
-### Text-To-Video with Pose Control
-To generate a video from prompt with additional pose control
-
-1. Download a demo video
-
- ```python
- from huggingface_hub import hf_hub_download
-
- filename = "__assets__/poses_skeleton_gifs/dance1_corr.mp4"
- repo_id = "PAIR/Text2Video-Zero"
- video_path = hf_hub_download(repo_type="space", repo_id=repo_id, filename=filename)
- ```
-
-
-2. Read video containing extracted pose images
- ```python
- from PIL import Image
- import imageio
-
- reader = imageio.get_reader(video_path, "ffmpeg")
- frame_count = 8
- pose_images = [Image.fromarray(reader.get_data(i)) for i in range(frame_count)]
- ```
- To extract pose from actual video, read [ControlNet documentation](./stable_diffusion/controlnet).
-
-3. Run `StableDiffusionControlNetPipeline` with our custom attention processor
-
- ```python
- import torch
- from diffusers import StableDiffusionControlNetPipeline, ControlNetModel
- from diffusers.pipelines.text_to_video_synthesis.pipeline_text_to_video_zero import CrossFrameAttnProcessor
-
- model_id = "runwayml/stable-diffusion-v1-5"
- controlnet = ControlNetModel.from_pretrained("lllyasviel/sd-controlnet-openpose", torch_dtype=torch.float16)
- pipe = StableDiffusionControlNetPipeline.from_pretrained(
- model_id, controlnet=controlnet, torch_dtype=torch.float16
- ).to("cuda")
-
- # Set the attention processor
- pipe.unet.set_attn_processor(CrossFrameAttnProcessor(batch_size=2))
- pipe.controlnet.set_attn_processor(CrossFrameAttnProcessor(batch_size=2))
-
- # fix latents for all frames
- latents = torch.randn((1, 4, 64, 64), device="cuda", dtype=torch.float16).repeat(len(pose_images), 1, 1, 1)
-
- prompt = "Darth Vader dancing in a desert"
- result = pipe(prompt=[prompt] * len(pose_images), image=pose_images, latents=latents).images
- imageio.mimsave("video.mp4", result, fps=4)
- ```
-
-
-### Text-To-Video with Edge Control
-
-To generate a video from prompt with additional pose control,
-follow the steps described above for pose-guided generation using [Canny edge ControlNet model](https://huggingface.co/lllyasviel/sd-controlnet-canny).
-
-
-### Video Instruct-Pix2Pix
-
-To perform text-guided video editing (with [InstructPix2Pix](./stable_diffusion/pix2pix)):
-
-1. Download a demo video
-
- ```python
- from huggingface_hub import hf_hub_download
-
- filename = "__assets__/pix2pix video/camel.mp4"
- repo_id = "PAIR/Text2Video-Zero"
- video_path = hf_hub_download(repo_type="space", repo_id=repo_id, filename=filename)
- ```
-
-2. Read video from path
- ```python
- from PIL import Image
- import imageio
-
- reader = imageio.get_reader(video_path, "ffmpeg")
- frame_count = 8
- video = [Image.fromarray(reader.get_data(i)) for i in range(frame_count)]
- ```
-
-3. Run `StableDiffusionInstructPix2PixPipeline` with our custom attention processor
- ```python
- import torch
- from diffusers import StableDiffusionInstructPix2PixPipeline
- from diffusers.pipelines.text_to_video_synthesis.pipeline_text_to_video_zero import CrossFrameAttnProcessor
-
- model_id = "timbrooks/instruct-pix2pix"
- pipe = StableDiffusionInstructPix2PixPipeline.from_pretrained(model_id, torch_dtype=torch.float16).to("cuda")
- pipe.unet.set_attn_processor(CrossFrameAttnProcessor(batch_size=3))
-
- prompt = "make it Van Gogh Starry Night style"
- result = pipe(prompt=[prompt] * len(video), image=video).images
- imageio.mimsave("edited_video.mp4", result, fps=4)
- ```
-
-
-### DreamBooth specialization
-
-Methods **Text-To-Video**, **Text-To-Video with Pose Control** and **Text-To-Video with Edge Control**
-can run with custom [DreamBooth](../training/dreambooth) models, as shown below for
-[Canny edge ControlNet model](https://huggingface.co/lllyasviel/sd-controlnet-canny) and
-[Avatar style DreamBooth](https://huggingface.co/PAIR/text2video-zero-controlnet-canny-avatar) model
-
-1. Download a demo video
-
- ```python
- from huggingface_hub import hf_hub_download
-
- filename = "__assets__/canny_videos_mp4/girl_turning.mp4"
- repo_id = "PAIR/Text2Video-Zero"
- video_path = hf_hub_download(repo_type="space", repo_id=repo_id, filename=filename)
- ```
-
-2. Read video from path
- ```python
- from PIL import Image
- import imageio
-
- reader = imageio.get_reader(video_path, "ffmpeg")
- frame_count = 8
- canny_edges = [Image.fromarray(reader.get_data(i)) for i in range(frame_count)]
- ```
-
-3. Run `StableDiffusionControlNetPipeline` with custom trained DreamBooth model
- ```python
- import torch
- from diffusers import StableDiffusionControlNetPipeline, ControlNetModel
- from diffusers.pipelines.text_to_video_synthesis.pipeline_text_to_video_zero import CrossFrameAttnProcessor
-
- # set model id to custom model
- model_id = "PAIR/text2video-zero-controlnet-canny-avatar"
- controlnet = ControlNetModel.from_pretrained("lllyasviel/sd-controlnet-canny", torch_dtype=torch.float16)
- pipe = StableDiffusionControlNetPipeline.from_pretrained(
- model_id, controlnet=controlnet, torch_dtype=torch.float16
- ).to("cuda")
-
- # Set the attention processor
- pipe.unet.set_attn_processor(CrossFrameAttnProcessor(batch_size=2))
- pipe.controlnet.set_attn_processor(CrossFrameAttnProcessor(batch_size=2))
-
- # fix latents for all frames
- latents = torch.randn((1, 4, 64, 64), device="cuda", dtype=torch.float16).repeat(len(canny_edges), 1, 1, 1)
-
- prompt = "oil painting of a beautiful girl avatar style"
- result = pipe(prompt=[prompt] * len(canny_edges), image=canny_edges, latents=latents).images
- imageio.mimsave("video.mp4", result, fps=4)
- ```
-
-You can filter out some available DreamBooth-trained models with [this link](https://huggingface.co/models?search=dreambooth).
-
-
-## TextToVideoZeroPipeline
-[[autodoc]] TextToVideoZeroPipeline
- - all
- - __call__
-
-## TextToVideoPipelineOutput
-[[autodoc]] pipelines.text_to_video_synthesis.pipeline_text_to_video_zero.TextToVideoPipelineOutput
\ No newline at end of file
diff --git a/spaces/Androidonnxfork/CivitAi-to-Diffusers/diffusers/src/diffusers/pipelines/semantic_stable_diffusion/__init__.py b/spaces/Androidonnxfork/CivitAi-to-Diffusers/diffusers/src/diffusers/pipelines/semantic_stable_diffusion/__init__.py
deleted file mode 100644
index 95d3604bcf090366bef5083dc916b499346cd3aa..0000000000000000000000000000000000000000
--- a/spaces/Androidonnxfork/CivitAi-to-Diffusers/diffusers/src/diffusers/pipelines/semantic_stable_diffusion/__init__.py
+++ /dev/null
@@ -1,36 +0,0 @@
-from dataclasses import dataclass
-from enum import Enum
-from typing import List, Optional, Union
-
-import numpy as np
-import PIL
-from PIL import Image
-
-from ...utils import BaseOutput, OptionalDependencyNotAvailable, is_torch_available, is_transformers_available
-
-
-@dataclass
-class SemanticStableDiffusionPipelineOutput(BaseOutput):
- """
- Output class for Stable Diffusion pipelines.
-
- Args:
- images (`List[PIL.Image.Image]` or `np.ndarray`)
- List of denoised PIL images of length `batch_size` or NumPy array of shape `(batch_size, height, width,
- num_channels)`.
- nsfw_content_detected (`List[bool]`)
- List indicating whether the corresponding generated image contains “not-safe-for-work” (nsfw) content or
- `None` if safety checking could not be performed.
- """
-
- images: Union[List[PIL.Image.Image], np.ndarray]
- nsfw_content_detected: Optional[List[bool]]
-
-
-try:
- if not (is_transformers_available() and is_torch_available()):
- raise OptionalDependencyNotAvailable()
-except OptionalDependencyNotAvailable:
- from ...utils.dummy_torch_and_transformers_objects import * # noqa F403
-else:
- from .pipeline_semantic_stable_diffusion import SemanticStableDiffusionPipeline
diff --git a/spaces/Androidonnxfork/CivitAi-to-Diffusers/diffusers/src/diffusers/schedulers/scheduling_dpmsolver_singlestep.py b/spaces/Androidonnxfork/CivitAi-to-Diffusers/diffusers/src/diffusers/schedulers/scheduling_dpmsolver_singlestep.py
deleted file mode 100644
index 93975a27fc6e3899c009b5576ed74753ea62abbb..0000000000000000000000000000000000000000
--- a/spaces/Androidonnxfork/CivitAi-to-Diffusers/diffusers/src/diffusers/schedulers/scheduling_dpmsolver_singlestep.py
+++ /dev/null
@@ -1,737 +0,0 @@
-# Copyright 2023 TSAIL Team and The HuggingFace Team. All rights reserved.
-#
-# Licensed under the Apache License, Version 2.0 (the "License");
-# you may not use this file except in compliance with the License.
-# You may obtain a copy of the License at
-#
-# http://www.apache.org/licenses/LICENSE-2.0
-#
-# Unless required by applicable law or agreed to in writing, software
-# distributed under the License is distributed on an "AS IS" BASIS,
-# WITHOUT WARRANTIES OR CONDITIONS OF ANY KIND, either express or implied.
-# See the License for the specific language governing permissions and
-# limitations under the License.
-
-# DISCLAIMER: This file is strongly influenced by https://github.com/LuChengTHU/dpm-solver
-
-import math
-from typing import List, Optional, Tuple, Union
-
-import numpy as np
-import torch
-
-from ..configuration_utils import ConfigMixin, register_to_config
-from ..utils import logging
-from .scheduling_utils import KarrasDiffusionSchedulers, SchedulerMixin, SchedulerOutput
-
-
-logger = logging.get_logger(__name__) # pylint: disable=invalid-name
-
-
-# Copied from diffusers.schedulers.scheduling_ddpm.betas_for_alpha_bar
-def betas_for_alpha_bar(
- num_diffusion_timesteps,
- max_beta=0.999,
- alpha_transform_type="cosine",
-):
- """
- Create a beta schedule that discretizes the given alpha_t_bar function, which defines the cumulative product of
- (1-beta) over time from t = [0,1].
-
- Contains a function alpha_bar that takes an argument t and transforms it to the cumulative product of (1-beta) up
- to that part of the diffusion process.
-
-
- Args:
- num_diffusion_timesteps (`int`): the number of betas to produce.
- max_beta (`float`): the maximum beta to use; use values lower than 1 to
- prevent singularities.
- alpha_transform_type (`str`, *optional*, default to `cosine`): the type of noise schedule for alpha_bar.
- Choose from `cosine` or `exp`
-
- Returns:
- betas (`np.ndarray`): the betas used by the scheduler to step the model outputs
- """
- if alpha_transform_type == "cosine":
-
- def alpha_bar_fn(t):
- return math.cos((t + 0.008) / 1.008 * math.pi / 2) ** 2
-
- elif alpha_transform_type == "exp":
-
- def alpha_bar_fn(t):
- return math.exp(t * -12.0)
-
- else:
- raise ValueError(f"Unsupported alpha_tranform_type: {alpha_transform_type}")
-
- betas = []
- for i in range(num_diffusion_timesteps):
- t1 = i / num_diffusion_timesteps
- t2 = (i + 1) / num_diffusion_timesteps
- betas.append(min(1 - alpha_bar_fn(t2) / alpha_bar_fn(t1), max_beta))
- return torch.tensor(betas, dtype=torch.float32)
-
-
-class DPMSolverSinglestepScheduler(SchedulerMixin, ConfigMixin):
- """
- DPM-Solver (and the improved version DPM-Solver++) is a fast dedicated high-order solver for diffusion ODEs with
- the convergence order guarantee. Empirically, sampling by DPM-Solver with only 20 steps can generate high-quality
- samples, and it can generate quite good samples even in only 10 steps.
-
- For more details, see the original paper: https://arxiv.org/abs/2206.00927 and https://arxiv.org/abs/2211.01095
-
- Currently, we support the singlestep DPM-Solver for both noise prediction models and data prediction models. We
- recommend to use `solver_order=2` for guided sampling, and `solver_order=3` for unconditional sampling.
-
- We also support the "dynamic thresholding" method in Imagen (https://arxiv.org/abs/2205.11487). For pixel-space
- diffusion models, you can set both `algorithm_type="dpmsolver++"` and `thresholding=True` to use the dynamic
- thresholding. Note that the thresholding method is unsuitable for latent-space diffusion models (such as
- stable-diffusion).
-
- [`~ConfigMixin`] takes care of storing all config attributes that are passed in the scheduler's `__init__`
- function, such as `num_train_timesteps`. They can be accessed via `scheduler.config.num_train_timesteps`.
- [`SchedulerMixin`] provides general loading and saving functionality via the [`SchedulerMixin.save_pretrained`] and
- [`~SchedulerMixin.from_pretrained`] functions.
-
- Args:
- num_train_timesteps (`int`): number of diffusion steps used to train the model.
- beta_start (`float`): the starting `beta` value of inference.
- beta_end (`float`): the final `beta` value.
- beta_schedule (`str`):
- the beta schedule, a mapping from a beta range to a sequence of betas for stepping the model. Choose from
- `linear`, `scaled_linear`, or `squaredcos_cap_v2`.
- trained_betas (`np.ndarray`, optional):
- option to pass an array of betas directly to the constructor to bypass `beta_start`, `beta_end` etc.
- solver_order (`int`, default `2`):
- the order of DPM-Solver; can be `1` or `2` or `3`. We recommend to use `solver_order=2` for guided
- sampling, and `solver_order=3` for unconditional sampling.
- prediction_type (`str`, default `epsilon`):
- indicates whether the model predicts the noise (epsilon), or the data / `x0`. One of `epsilon`, `sample`,
- or `v-prediction`.
- thresholding (`bool`, default `False`):
- whether to use the "dynamic thresholding" method (introduced by Imagen, https://arxiv.org/abs/2205.11487).
- For pixel-space diffusion models, you can set both `algorithm_type=dpmsolver++` and `thresholding=True` to
- use the dynamic thresholding. Note that the thresholding method is unsuitable for latent-space diffusion
- models (such as stable-diffusion).
- dynamic_thresholding_ratio (`float`, default `0.995`):
- the ratio for the dynamic thresholding method. Default is `0.995`, the same as Imagen
- (https://arxiv.org/abs/2205.11487).
- sample_max_value (`float`, default `1.0`):
- the threshold value for dynamic thresholding. Valid only when `thresholding=True` and
- `algorithm_type="dpmsolver++`.
- algorithm_type (`str`, default `dpmsolver++`):
- the algorithm type for the solver. Either `dpmsolver` or `dpmsolver++`. The `dpmsolver` type implements the
- algorithms in https://arxiv.org/abs/2206.00927, and the `dpmsolver++` type implements the algorithms in
- https://arxiv.org/abs/2211.01095. We recommend to use `dpmsolver++` with `solver_order=2` for guided
- sampling (e.g. stable-diffusion).
- solver_type (`str`, default `midpoint`):
- the solver type for the second-order solver. Either `midpoint` or `heun`. The solver type slightly affects
- the sample quality, especially for small number of steps. We empirically find that `midpoint` solvers are
- slightly better, so we recommend to use the `midpoint` type.
- lower_order_final (`bool`, default `True`):
- whether to use lower-order solvers in the final steps. For singlestep schedulers, we recommend to enable
- this to use up all the function evaluations.
- use_karras_sigmas (`bool`, *optional*, defaults to `False`):
- This parameter controls whether to use Karras sigmas (Karras et al. (2022) scheme) for step sizes in the
- noise schedule during the sampling process. If True, the sigmas will be determined according to a sequence
- of noise levels {σi} as defined in Equation (5) of the paper https://arxiv.org/pdf/2206.00364.pdf.
- lambda_min_clipped (`float`, default `-inf`):
- the clipping threshold for the minimum value of lambda(t) for numerical stability. This is critical for
- cosine (squaredcos_cap_v2) noise schedule.
- variance_type (`str`, *optional*):
- Set to "learned" or "learned_range" for diffusion models that predict variance. For example, OpenAI's
- guided-diffusion (https://github.com/openai/guided-diffusion) predicts both mean and variance of the
- Gaussian distribution in the model's output. DPM-Solver only needs the "mean" output because it is based on
- diffusion ODEs. whether the model's output contains the predicted Gaussian variance. For example, OpenAI's
- guided-diffusion (https://github.com/openai/guided-diffusion) predicts both mean and variance of the
- Gaussian distribution in the model's output. DPM-Solver only needs the "mean" output because it is based on
- diffusion ODEs.
-
- """
-
- _compatibles = [e.name for e in KarrasDiffusionSchedulers]
- order = 1
-
- @register_to_config
- def __init__(
- self,
- num_train_timesteps: int = 1000,
- beta_start: float = 0.0001,
- beta_end: float = 0.02,
- beta_schedule: str = "linear",
- trained_betas: Optional[np.ndarray] = None,
- solver_order: int = 2,
- prediction_type: str = "epsilon",
- thresholding: bool = False,
- dynamic_thresholding_ratio: float = 0.995,
- sample_max_value: float = 1.0,
- algorithm_type: str = "dpmsolver++",
- solver_type: str = "midpoint",
- lower_order_final: bool = True,
- use_karras_sigmas: Optional[bool] = False,
- lambda_min_clipped: float = -float("inf"),
- variance_type: Optional[str] = None,
- ):
- if trained_betas is not None:
- self.betas = torch.tensor(trained_betas, dtype=torch.float32)
- elif beta_schedule == "linear":
- self.betas = torch.linspace(beta_start, beta_end, num_train_timesteps, dtype=torch.float32)
- elif beta_schedule == "scaled_linear":
- # this schedule is very specific to the latent diffusion model.
- self.betas = (
- torch.linspace(beta_start**0.5, beta_end**0.5, num_train_timesteps, dtype=torch.float32) ** 2
- )
- elif beta_schedule == "squaredcos_cap_v2":
- # Glide cosine schedule
- self.betas = betas_for_alpha_bar(num_train_timesteps)
- else:
- raise NotImplementedError(f"{beta_schedule} does is not implemented for {self.__class__}")
-
- self.alphas = 1.0 - self.betas
- self.alphas_cumprod = torch.cumprod(self.alphas, dim=0)
- # Currently we only support VP-type noise schedule
- self.alpha_t = torch.sqrt(self.alphas_cumprod)
- self.sigma_t = torch.sqrt(1 - self.alphas_cumprod)
- self.lambda_t = torch.log(self.alpha_t) - torch.log(self.sigma_t)
-
- # standard deviation of the initial noise distribution
- self.init_noise_sigma = 1.0
-
- # settings for DPM-Solver
- if algorithm_type not in ["dpmsolver", "dpmsolver++"]:
- if algorithm_type == "deis":
- self.register_to_config(algorithm_type="dpmsolver++")
- else:
- raise NotImplementedError(f"{algorithm_type} does is not implemented for {self.__class__}")
- if solver_type not in ["midpoint", "heun"]:
- if solver_type in ["logrho", "bh1", "bh2"]:
- self.register_to_config(solver_type="midpoint")
- else:
- raise NotImplementedError(f"{solver_type} does is not implemented for {self.__class__}")
-
- # setable values
- self.num_inference_steps = None
- timesteps = np.linspace(0, num_train_timesteps - 1, num_train_timesteps, dtype=np.float32)[::-1].copy()
- self.timesteps = torch.from_numpy(timesteps)
- self.model_outputs = [None] * solver_order
- self.sample = None
- self.order_list = self.get_order_list(num_train_timesteps)
-
- def get_order_list(self, num_inference_steps: int) -> List[int]:
- """
- Computes the solver order at each time step.
-
- Args:
- num_inference_steps (`int`):
- the number of diffusion steps used when generating samples with a pre-trained model.
- """
- steps = num_inference_steps
- order = self.config.solver_order
- if self.config.lower_order_final:
- if order == 3:
- if steps % 3 == 0:
- orders = [1, 2, 3] * (steps // 3 - 1) + [1, 2] + [1]
- elif steps % 3 == 1:
- orders = [1, 2, 3] * (steps // 3) + [1]
- else:
- orders = [1, 2, 3] * (steps // 3) + [1, 2]
- elif order == 2:
- if steps % 2 == 0:
- orders = [1, 2] * (steps // 2)
- else:
- orders = [1, 2] * (steps // 2) + [1]
- elif order == 1:
- orders = [1] * steps
- else:
- if order == 3:
- orders = [1, 2, 3] * (steps // 3)
- elif order == 2:
- orders = [1, 2] * (steps // 2)
- elif order == 1:
- orders = [1] * steps
- return orders
-
- def set_timesteps(self, num_inference_steps: int, device: Union[str, torch.device] = None):
- """
- Sets the timesteps used for the diffusion chain. Supporting function to be run before inference.
-
- Args:
- num_inference_steps (`int`):
- the number of diffusion steps used when generating samples with a pre-trained model.
- device (`str` or `torch.device`, optional):
- the device to which the timesteps should be moved to. If `None`, the timesteps are not moved.
- """
- self.num_inference_steps = num_inference_steps
- # Clipping the minimum of all lambda(t) for numerical stability.
- # This is critical for cosine (squaredcos_cap_v2) noise schedule.
- clipped_idx = torch.searchsorted(torch.flip(self.lambda_t, [0]), self.config.lambda_min_clipped)
- timesteps = (
- np.linspace(0, self.config.num_train_timesteps - 1 - clipped_idx, num_inference_steps + 1)
- .round()[::-1][:-1]
- .copy()
- .astype(np.int64)
- )
-
- sigmas = np.array(((1 - self.alphas_cumprod) / self.alphas_cumprod) ** 0.5)
- if self.config.use_karras_sigmas:
- log_sigmas = np.log(sigmas)
- sigmas = self._convert_to_karras(in_sigmas=sigmas, num_inference_steps=num_inference_steps)
- timesteps = np.array([self._sigma_to_t(sigma, log_sigmas) for sigma in sigmas]).round()
- timesteps = np.flip(timesteps).copy().astype(np.int64)
-
- self.sigmas = torch.from_numpy(sigmas)
-
- self.timesteps = torch.from_numpy(timesteps).to(device)
- self.model_outputs = [None] * self.config.solver_order
- self.sample = None
-
- if not self.config.lower_order_final and num_inference_steps % self.config.solver_order != 0:
- logger.warn(
- "Changing scheduler {self.config} to have `lower_order_final` set to True to handle uneven amount of inference steps. Please make sure to always use an even number of `num_inference steps when using `lower_order_final=True`."
- )
- self.register_to_config(lower_order_final=True)
-
- self.order_list = self.get_order_list(num_inference_steps)
-
- # Copied from diffusers.schedulers.scheduling_ddpm.DDPMScheduler._threshold_sample
- def _threshold_sample(self, sample: torch.FloatTensor) -> torch.FloatTensor:
- """
- "Dynamic thresholding: At each sampling step we set s to a certain percentile absolute pixel value in xt0 (the
- prediction of x_0 at timestep t), and if s > 1, then we threshold xt0 to the range [-s, s] and then divide by
- s. Dynamic thresholding pushes saturated pixels (those near -1 and 1) inwards, thereby actively preventing
- pixels from saturation at each step. We find that dynamic thresholding results in significantly better
- photorealism as well as better image-text alignment, especially when using very large guidance weights."
-
- https://arxiv.org/abs/2205.11487
- """
- dtype = sample.dtype
- batch_size, channels, height, width = sample.shape
-
- if dtype not in (torch.float32, torch.float64):
- sample = sample.float() # upcast for quantile calculation, and clamp not implemented for cpu half
-
- # Flatten sample for doing quantile calculation along each image
- sample = sample.reshape(batch_size, channels * height * width)
-
- abs_sample = sample.abs() # "a certain percentile absolute pixel value"
-
- s = torch.quantile(abs_sample, self.config.dynamic_thresholding_ratio, dim=1)
- s = torch.clamp(
- s, min=1, max=self.config.sample_max_value
- ) # When clamped to min=1, equivalent to standard clipping to [-1, 1]
-
- s = s.unsqueeze(1) # (batch_size, 1) because clamp will broadcast along dim=0
- sample = torch.clamp(sample, -s, s) / s # "we threshold xt0 to the range [-s, s] and then divide by s"
-
- sample = sample.reshape(batch_size, channels, height, width)
- sample = sample.to(dtype)
-
- return sample
-
- # Copied from diffusers.schedulers.scheduling_euler_discrete.EulerDiscreteScheduler._sigma_to_t
- def _sigma_to_t(self, sigma, log_sigmas):
- # get log sigma
- log_sigma = np.log(sigma)
-
- # get distribution
- dists = log_sigma - log_sigmas[:, np.newaxis]
-
- # get sigmas range
- low_idx = np.cumsum((dists >= 0), axis=0).argmax(axis=0).clip(max=log_sigmas.shape[0] - 2)
- high_idx = low_idx + 1
-
- low = log_sigmas[low_idx]
- high = log_sigmas[high_idx]
-
- # interpolate sigmas
- w = (low - log_sigma) / (low - high)
- w = np.clip(w, 0, 1)
-
- # transform interpolation to time range
- t = (1 - w) * low_idx + w * high_idx
- t = t.reshape(sigma.shape)
- return t
-
- # Copied from diffusers.schedulers.scheduling_euler_discrete.EulerDiscreteScheduler._convert_to_karras
- def _convert_to_karras(self, in_sigmas: torch.FloatTensor, num_inference_steps) -> torch.FloatTensor:
- """Constructs the noise schedule of Karras et al. (2022)."""
-
- sigma_min: float = in_sigmas[-1].item()
- sigma_max: float = in_sigmas[0].item()
-
- rho = 7.0 # 7.0 is the value used in the paper
- ramp = np.linspace(0, 1, num_inference_steps)
- min_inv_rho = sigma_min ** (1 / rho)
- max_inv_rho = sigma_max ** (1 / rho)
- sigmas = (max_inv_rho + ramp * (min_inv_rho - max_inv_rho)) ** rho
- return sigmas
-
- def convert_model_output(
- self, model_output: torch.FloatTensor, timestep: int, sample: torch.FloatTensor
- ) -> torch.FloatTensor:
- """
- Convert the model output to the corresponding type that the algorithm (DPM-Solver / DPM-Solver++) needs.
-
- DPM-Solver is designed to discretize an integral of the noise prediction model, and DPM-Solver++ is designed to
- discretize an integral of the data prediction model. So we need to first convert the model output to the
- corresponding type to match the algorithm.
-
- Note that the algorithm type and the model type is decoupled. That is to say, we can use either DPM-Solver or
- DPM-Solver++ for both noise prediction model and data prediction model.
-
- Args:
- model_output (`torch.FloatTensor`): direct output from learned diffusion model.
- timestep (`int`): current discrete timestep in the diffusion chain.
- sample (`torch.FloatTensor`):
- current instance of sample being created by diffusion process.
-
- Returns:
- `torch.FloatTensor`: the converted model output.
- """
- # DPM-Solver++ needs to solve an integral of the data prediction model.
- if self.config.algorithm_type == "dpmsolver++":
- if self.config.prediction_type == "epsilon":
- # DPM-Solver and DPM-Solver++ only need the "mean" output.
- if self.config.variance_type in ["learned_range"]:
- model_output = model_output[:, :3]
- alpha_t, sigma_t = self.alpha_t[timestep], self.sigma_t[timestep]
- x0_pred = (sample - sigma_t * model_output) / alpha_t
- elif self.config.prediction_type == "sample":
- x0_pred = model_output
- elif self.config.prediction_type == "v_prediction":
- alpha_t, sigma_t = self.alpha_t[timestep], self.sigma_t[timestep]
- x0_pred = alpha_t * sample - sigma_t * model_output
- else:
- raise ValueError(
- f"prediction_type given as {self.config.prediction_type} must be one of `epsilon`, `sample`, or"
- " `v_prediction` for the DPMSolverSinglestepScheduler."
- )
-
- if self.config.thresholding:
- x0_pred = self._threshold_sample(x0_pred)
-
- return x0_pred
- # DPM-Solver needs to solve an integral of the noise prediction model.
- elif self.config.algorithm_type == "dpmsolver":
- if self.config.prediction_type == "epsilon":
- # DPM-Solver and DPM-Solver++ only need the "mean" output.
- if self.config.variance_type in ["learned_range"]:
- model_output = model_output[:, :3]
- return model_output
- elif self.config.prediction_type == "sample":
- alpha_t, sigma_t = self.alpha_t[timestep], self.sigma_t[timestep]
- epsilon = (sample - alpha_t * model_output) / sigma_t
- return epsilon
- elif self.config.prediction_type == "v_prediction":
- alpha_t, sigma_t = self.alpha_t[timestep], self.sigma_t[timestep]
- epsilon = alpha_t * model_output + sigma_t * sample
- return epsilon
- else:
- raise ValueError(
- f"prediction_type given as {self.config.prediction_type} must be one of `epsilon`, `sample`, or"
- " `v_prediction` for the DPMSolverSinglestepScheduler."
- )
-
- def dpm_solver_first_order_update(
- self,
- model_output: torch.FloatTensor,
- timestep: int,
- prev_timestep: int,
- sample: torch.FloatTensor,
- ) -> torch.FloatTensor:
- """
- One step for the first-order DPM-Solver (equivalent to DDIM).
-
- See https://arxiv.org/abs/2206.00927 for the detailed derivation.
-
- Args:
- model_output (`torch.FloatTensor`): direct output from learned diffusion model.
- timestep (`int`): current discrete timestep in the diffusion chain.
- prev_timestep (`int`): previous discrete timestep in the diffusion chain.
- sample (`torch.FloatTensor`):
- current instance of sample being created by diffusion process.
-
- Returns:
- `torch.FloatTensor`: the sample tensor at the previous timestep.
- """
- lambda_t, lambda_s = self.lambda_t[prev_timestep], self.lambda_t[timestep]
- alpha_t, alpha_s = self.alpha_t[prev_timestep], self.alpha_t[timestep]
- sigma_t, sigma_s = self.sigma_t[prev_timestep], self.sigma_t[timestep]
- h = lambda_t - lambda_s
- if self.config.algorithm_type == "dpmsolver++":
- x_t = (sigma_t / sigma_s) * sample - (alpha_t * (torch.exp(-h) - 1.0)) * model_output
- elif self.config.algorithm_type == "dpmsolver":
- x_t = (alpha_t / alpha_s) * sample - (sigma_t * (torch.exp(h) - 1.0)) * model_output
- return x_t
-
- def singlestep_dpm_solver_second_order_update(
- self,
- model_output_list: List[torch.FloatTensor],
- timestep_list: List[int],
- prev_timestep: int,
- sample: torch.FloatTensor,
- ) -> torch.FloatTensor:
- """
- One step for the second-order singlestep DPM-Solver.
-
- It computes the solution at time `prev_timestep` from the time `timestep_list[-2]`.
-
- Args:
- model_output_list (`List[torch.FloatTensor]`):
- direct outputs from learned diffusion model at current and latter timesteps.
- timestep (`int`): current and latter discrete timestep in the diffusion chain.
- prev_timestep (`int`): previous discrete timestep in the diffusion chain.
- sample (`torch.FloatTensor`):
- current instance of sample being created by diffusion process.
-
- Returns:
- `torch.FloatTensor`: the sample tensor at the previous timestep.
- """
- t, s0, s1 = prev_timestep, timestep_list[-1], timestep_list[-2]
- m0, m1 = model_output_list[-1], model_output_list[-2]
- lambda_t, lambda_s0, lambda_s1 = self.lambda_t[t], self.lambda_t[s0], self.lambda_t[s1]
- alpha_t, alpha_s1 = self.alpha_t[t], self.alpha_t[s1]
- sigma_t, sigma_s1 = self.sigma_t[t], self.sigma_t[s1]
- h, h_0 = lambda_t - lambda_s1, lambda_s0 - lambda_s1
- r0 = h_0 / h
- D0, D1 = m1, (1.0 / r0) * (m0 - m1)
- if self.config.algorithm_type == "dpmsolver++":
- # See https://arxiv.org/abs/2211.01095 for detailed derivations
- if self.config.solver_type == "midpoint":
- x_t = (
- (sigma_t / sigma_s1) * sample
- - (alpha_t * (torch.exp(-h) - 1.0)) * D0
- - 0.5 * (alpha_t * (torch.exp(-h) - 1.0)) * D1
- )
- elif self.config.solver_type == "heun":
- x_t = (
- (sigma_t / sigma_s1) * sample
- - (alpha_t * (torch.exp(-h) - 1.0)) * D0
- + (alpha_t * ((torch.exp(-h) - 1.0) / h + 1.0)) * D1
- )
- elif self.config.algorithm_type == "dpmsolver":
- # See https://arxiv.org/abs/2206.00927 for detailed derivations
- if self.config.solver_type == "midpoint":
- x_t = (
- (alpha_t / alpha_s1) * sample
- - (sigma_t * (torch.exp(h) - 1.0)) * D0
- - 0.5 * (sigma_t * (torch.exp(h) - 1.0)) * D1
- )
- elif self.config.solver_type == "heun":
- x_t = (
- (alpha_t / alpha_s1) * sample
- - (sigma_t * (torch.exp(h) - 1.0)) * D0
- - (sigma_t * ((torch.exp(h) - 1.0) / h - 1.0)) * D1
- )
- return x_t
-
- def singlestep_dpm_solver_third_order_update(
- self,
- model_output_list: List[torch.FloatTensor],
- timestep_list: List[int],
- prev_timestep: int,
- sample: torch.FloatTensor,
- ) -> torch.FloatTensor:
- """
- One step for the third-order singlestep DPM-Solver.
-
- It computes the solution at time `prev_timestep` from the time `timestep_list[-3]`.
-
- Args:
- model_output_list (`List[torch.FloatTensor]`):
- direct outputs from learned diffusion model at current and latter timesteps.
- timestep (`int`): current and latter discrete timestep in the diffusion chain.
- prev_timestep (`int`): previous discrete timestep in the diffusion chain.
- sample (`torch.FloatTensor`):
- current instance of sample being created by diffusion process.
-
- Returns:
- `torch.FloatTensor`: the sample tensor at the previous timestep.
- """
- t, s0, s1, s2 = prev_timestep, timestep_list[-1], timestep_list[-2], timestep_list[-3]
- m0, m1, m2 = model_output_list[-1], model_output_list[-2], model_output_list[-3]
- lambda_t, lambda_s0, lambda_s1, lambda_s2 = (
- self.lambda_t[t],
- self.lambda_t[s0],
- self.lambda_t[s1],
- self.lambda_t[s2],
- )
- alpha_t, alpha_s2 = self.alpha_t[t], self.alpha_t[s2]
- sigma_t, sigma_s2 = self.sigma_t[t], self.sigma_t[s2]
- h, h_0, h_1 = lambda_t - lambda_s2, lambda_s0 - lambda_s2, lambda_s1 - lambda_s2
- r0, r1 = h_0 / h, h_1 / h
- D0 = m2
- D1_0, D1_1 = (1.0 / r1) * (m1 - m2), (1.0 / r0) * (m0 - m2)
- D1 = (r0 * D1_0 - r1 * D1_1) / (r0 - r1)
- D2 = 2.0 * (D1_1 - D1_0) / (r0 - r1)
- if self.config.algorithm_type == "dpmsolver++":
- # See https://arxiv.org/abs/2206.00927 for detailed derivations
- if self.config.solver_type == "midpoint":
- x_t = (
- (sigma_t / sigma_s2) * sample
- - (alpha_t * (torch.exp(-h) - 1.0)) * D0
- + (alpha_t * ((torch.exp(-h) - 1.0) / h + 1.0)) * D1_1
- )
- elif self.config.solver_type == "heun":
- x_t = (
- (sigma_t / sigma_s2) * sample
- - (alpha_t * (torch.exp(-h) - 1.0)) * D0
- + (alpha_t * ((torch.exp(-h) - 1.0) / h + 1.0)) * D1
- - (alpha_t * ((torch.exp(-h) - 1.0 + h) / h**2 - 0.5)) * D2
- )
- elif self.config.algorithm_type == "dpmsolver":
- # See https://arxiv.org/abs/2206.00927 for detailed derivations
- if self.config.solver_type == "midpoint":
- x_t = (
- (alpha_t / alpha_s2) * sample
- - (sigma_t * (torch.exp(h) - 1.0)) * D0
- - (sigma_t * ((torch.exp(h) - 1.0) / h - 1.0)) * D1_1
- )
- elif self.config.solver_type == "heun":
- x_t = (
- (alpha_t / alpha_s2) * sample
- - (sigma_t * (torch.exp(h) - 1.0)) * D0
- - (sigma_t * ((torch.exp(h) - 1.0) / h - 1.0)) * D1
- - (sigma_t * ((torch.exp(h) - 1.0 - h) / h**2 - 0.5)) * D2
- )
- return x_t
-
- def singlestep_dpm_solver_update(
- self,
- model_output_list: List[torch.FloatTensor],
- timestep_list: List[int],
- prev_timestep: int,
- sample: torch.FloatTensor,
- order: int,
- ) -> torch.FloatTensor:
- """
- One step for the singlestep DPM-Solver.
-
- Args:
- model_output_list (`List[torch.FloatTensor]`):
- direct outputs from learned diffusion model at current and latter timesteps.
- timestep (`int`): current and latter discrete timestep in the diffusion chain.
- prev_timestep (`int`): previous discrete timestep in the diffusion chain.
- sample (`torch.FloatTensor`):
- current instance of sample being created by diffusion process.
- order (`int`):
- the solver order at this step.
-
- Returns:
- `torch.FloatTensor`: the sample tensor at the previous timestep.
- """
- if order == 1:
- return self.dpm_solver_first_order_update(model_output_list[-1], timestep_list[-1], prev_timestep, sample)
- elif order == 2:
- return self.singlestep_dpm_solver_second_order_update(
- model_output_list, timestep_list, prev_timestep, sample
- )
- elif order == 3:
- return self.singlestep_dpm_solver_third_order_update(
- model_output_list, timestep_list, prev_timestep, sample
- )
- else:
- raise ValueError(f"Order must be 1, 2, 3, got {order}")
-
- def step(
- self,
- model_output: torch.FloatTensor,
- timestep: int,
- sample: torch.FloatTensor,
- return_dict: bool = True,
- ) -> Union[SchedulerOutput, Tuple]:
- """
- Step function propagating the sample with the singlestep DPM-Solver.
-
- Args:
- model_output (`torch.FloatTensor`): direct output from learned diffusion model.
- timestep (`int`): current discrete timestep in the diffusion chain.
- sample (`torch.FloatTensor`):
- current instance of sample being created by diffusion process.
- return_dict (`bool`): option for returning tuple rather than SchedulerOutput class
-
- Returns:
- [`~scheduling_utils.SchedulerOutput`] or `tuple`: [`~scheduling_utils.SchedulerOutput`] if `return_dict` is
- True, otherwise a `tuple`. When returning a tuple, the first element is the sample tensor.
-
- """
- if self.num_inference_steps is None:
- raise ValueError(
- "Number of inference steps is 'None', you need to run 'set_timesteps' after creating the scheduler"
- )
-
- if isinstance(timestep, torch.Tensor):
- timestep = timestep.to(self.timesteps.device)
- step_index = (self.timesteps == timestep).nonzero()
- if len(step_index) == 0:
- step_index = len(self.timesteps) - 1
- else:
- step_index = step_index.item()
- prev_timestep = 0 if step_index == len(self.timesteps) - 1 else self.timesteps[step_index + 1]
-
- model_output = self.convert_model_output(model_output, timestep, sample)
- for i in range(self.config.solver_order - 1):
- self.model_outputs[i] = self.model_outputs[i + 1]
- self.model_outputs[-1] = model_output
-
- order = self.order_list[step_index]
-
- # For img2img denoising might start with order>1 which is not possible
- # In this case make sure that the first two steps are both order=1
- while self.model_outputs[-order] is None:
- order -= 1
-
- # For single-step solvers, we use the initial value at each time with order = 1.
- if order == 1:
- self.sample = sample
-
- timestep_list = [self.timesteps[step_index - i] for i in range(order - 1, 0, -1)] + [timestep]
- prev_sample = self.singlestep_dpm_solver_update(
- self.model_outputs, timestep_list, prev_timestep, self.sample, order
- )
-
- if not return_dict:
- return (prev_sample,)
-
- return SchedulerOutput(prev_sample=prev_sample)
-
- def scale_model_input(self, sample: torch.FloatTensor, *args, **kwargs) -> torch.FloatTensor:
- """
- Ensures interchangeability with schedulers that need to scale the denoising model input depending on the
- current timestep.
-
- Args:
- sample (`torch.FloatTensor`): input sample
-
- Returns:
- `torch.FloatTensor`: scaled input sample
- """
- return sample
-
- # Copied from diffusers.schedulers.scheduling_ddpm.DDPMScheduler.add_noise
- def add_noise(
- self,
- original_samples: torch.FloatTensor,
- noise: torch.FloatTensor,
- timesteps: torch.IntTensor,
- ) -> torch.FloatTensor:
- # Make sure alphas_cumprod and timestep have same device and dtype as original_samples
- alphas_cumprod = self.alphas_cumprod.to(device=original_samples.device, dtype=original_samples.dtype)
- timesteps = timesteps.to(original_samples.device)
-
- sqrt_alpha_prod = alphas_cumprod[timesteps] ** 0.5
- sqrt_alpha_prod = sqrt_alpha_prod.flatten()
- while len(sqrt_alpha_prod.shape) < len(original_samples.shape):
- sqrt_alpha_prod = sqrt_alpha_prod.unsqueeze(-1)
-
- sqrt_one_minus_alpha_prod = (1 - alphas_cumprod[timesteps]) ** 0.5
- sqrt_one_minus_alpha_prod = sqrt_one_minus_alpha_prod.flatten()
- while len(sqrt_one_minus_alpha_prod.shape) < len(original_samples.shape):
- sqrt_one_minus_alpha_prod = sqrt_one_minus_alpha_prod.unsqueeze(-1)
-
- noisy_samples = sqrt_alpha_prod * original_samples + sqrt_one_minus_alpha_prod * noise
- return noisy_samples
-
- def __len__(self):
- return self.config.num_train_timesteps
diff --git a/spaces/Andy1621/uniformer_image_detection/configs/_base_/datasets/coco_instance_semantic.py b/spaces/Andy1621/uniformer_image_detection/configs/_base_/datasets/coco_instance_semantic.py
deleted file mode 100644
index f7c072ec92731af85952840128f6527bc799913a..0000000000000000000000000000000000000000
--- a/spaces/Andy1621/uniformer_image_detection/configs/_base_/datasets/coco_instance_semantic.py
+++ /dev/null
@@ -1,53 +0,0 @@
-dataset_type = 'CocoDataset'
-data_root = 'data/coco/'
-img_norm_cfg = dict(
- mean=[123.675, 116.28, 103.53], std=[58.395, 57.12, 57.375], to_rgb=True)
-train_pipeline = [
- dict(type='LoadImageFromFile'),
- dict(
- type='LoadAnnotations', with_bbox=True, with_mask=True, with_seg=True),
- dict(type='Resize', img_scale=(1333, 800), keep_ratio=True),
- dict(type='RandomFlip', flip_ratio=0.5),
- dict(type='Normalize', **img_norm_cfg),
- dict(type='Pad', size_divisor=32),
- dict(type='SegRescale', scale_factor=1 / 8),
- dict(type='DefaultFormatBundle'),
- dict(
- type='Collect',
- keys=['img', 'gt_bboxes', 'gt_labels', 'gt_masks', 'gt_semantic_seg']),
-]
-test_pipeline = [
- dict(type='LoadImageFromFile'),
- dict(
- type='MultiScaleFlipAug',
- img_scale=(1333, 800),
- flip=False,
- transforms=[
- dict(type='Resize', keep_ratio=True),
- dict(type='RandomFlip', flip_ratio=0.5),
- dict(type='Normalize', **img_norm_cfg),
- dict(type='Pad', size_divisor=32),
- dict(type='ImageToTensor', keys=['img']),
- dict(type='Collect', keys=['img']),
- ])
-]
-data = dict(
- samples_per_gpu=2,
- workers_per_gpu=2,
- train=dict(
- type=dataset_type,
- ann_file=data_root + 'annotations/instances_train2017.json',
- img_prefix=data_root + 'train2017/',
- seg_prefix=data_root + 'stuffthingmaps/train2017/',
- pipeline=train_pipeline),
- val=dict(
- type=dataset_type,
- ann_file=data_root + 'annotations/instances_val2017.json',
- img_prefix=data_root + 'val2017/',
- pipeline=test_pipeline),
- test=dict(
- type=dataset_type,
- ann_file=data_root + 'annotations/instances_val2017.json',
- img_prefix=data_root + 'val2017/',
- pipeline=test_pipeline))
-evaluation = dict(metric=['bbox', 'segm'])
diff --git a/spaces/Andy1621/uniformer_image_detection/configs/atss/atss_r101_fpn_1x_coco.py b/spaces/Andy1621/uniformer_image_detection/configs/atss/atss_r101_fpn_1x_coco.py
deleted file mode 100644
index 695779ab31b5f848f8c85c13cc4ca637c8590ba7..0000000000000000000000000000000000000000
--- a/spaces/Andy1621/uniformer_image_detection/configs/atss/atss_r101_fpn_1x_coco.py
+++ /dev/null
@@ -1,5 +0,0 @@
-_base_ = './atss_r50_fpn_1x_coco.py'
-model = dict(
- pretrained='torchvision://resnet101',
- backbone=dict(depth=101),
-)
diff --git a/spaces/Andy1621/uniformer_image_segmentation/configs/gcnet/gcnet_r50-d8_512x512_80k_ade20k.py b/spaces/Andy1621/uniformer_image_segmentation/configs/gcnet/gcnet_r50-d8_512x512_80k_ade20k.py
deleted file mode 100644
index 89d5e1ae0f3ef44626f3b5534c504cbce7389a32..0000000000000000000000000000000000000000
--- a/spaces/Andy1621/uniformer_image_segmentation/configs/gcnet/gcnet_r50-d8_512x512_80k_ade20k.py
+++ /dev/null
@@ -1,6 +0,0 @@
-_base_ = [
- '../_base_/models/gcnet_r50-d8.py', '../_base_/datasets/ade20k.py',
- '../_base_/default_runtime.py', '../_base_/schedules/schedule_80k.py'
-]
-model = dict(
- decode_head=dict(num_classes=150), auxiliary_head=dict(num_classes=150))
diff --git a/spaces/Anonymous-sub/Rerender/ControlNet/annotator/uniformer/mmcv/video/io.py b/spaces/Anonymous-sub/Rerender/ControlNet/annotator/uniformer/mmcv/video/io.py
deleted file mode 100644
index 9879154227f640c262853b92c219461c6f67ee8e..0000000000000000000000000000000000000000
--- a/spaces/Anonymous-sub/Rerender/ControlNet/annotator/uniformer/mmcv/video/io.py
+++ /dev/null
@@ -1,318 +0,0 @@
-# Copyright (c) OpenMMLab. All rights reserved.
-import os.path as osp
-from collections import OrderedDict
-
-import cv2
-from cv2 import (CAP_PROP_FOURCC, CAP_PROP_FPS, CAP_PROP_FRAME_COUNT,
- CAP_PROP_FRAME_HEIGHT, CAP_PROP_FRAME_WIDTH,
- CAP_PROP_POS_FRAMES, VideoWriter_fourcc)
-
-from annotator.uniformer.mmcv.utils import (check_file_exist, mkdir_or_exist, scandir,
- track_progress)
-
-
-class Cache:
-
- def __init__(self, capacity):
- self._cache = OrderedDict()
- self._capacity = int(capacity)
- if capacity <= 0:
- raise ValueError('capacity must be a positive integer')
-
- @property
- def capacity(self):
- return self._capacity
-
- @property
- def size(self):
- return len(self._cache)
-
- def put(self, key, val):
- if key in self._cache:
- return
- if len(self._cache) >= self.capacity:
- self._cache.popitem(last=False)
- self._cache[key] = val
-
- def get(self, key, default=None):
- val = self._cache[key] if key in self._cache else default
- return val
-
-
-class VideoReader:
- """Video class with similar usage to a list object.
-
- This video warpper class provides convenient apis to access frames.
- There exists an issue of OpenCV's VideoCapture class that jumping to a
- certain frame may be inaccurate. It is fixed in this class by checking
- the position after jumping each time.
- Cache is used when decoding videos. So if the same frame is visited for
- the second time, there is no need to decode again if it is stored in the
- cache.
-
- :Example:
-
- >>> import annotator.uniformer.mmcv as mmcv
- >>> v = mmcv.VideoReader('sample.mp4')
- >>> len(v) # get the total frame number with `len()`
- 120
- >>> for img in v: # v is iterable
- >>> mmcv.imshow(img)
- >>> v[5] # get the 6th frame
- """
-
- def __init__(self, filename, cache_capacity=10):
- # Check whether the video path is a url
- if not filename.startswith(('https://', 'http://')):
- check_file_exist(filename, 'Video file not found: ' + filename)
- self._vcap = cv2.VideoCapture(filename)
- assert cache_capacity > 0
- self._cache = Cache(cache_capacity)
- self._position = 0
- # get basic info
- self._width = int(self._vcap.get(CAP_PROP_FRAME_WIDTH))
- self._height = int(self._vcap.get(CAP_PROP_FRAME_HEIGHT))
- self._fps = self._vcap.get(CAP_PROP_FPS)
- self._frame_cnt = int(self._vcap.get(CAP_PROP_FRAME_COUNT))
- self._fourcc = self._vcap.get(CAP_PROP_FOURCC)
-
- @property
- def vcap(self):
- """:obj:`cv2.VideoCapture`: The raw VideoCapture object."""
- return self._vcap
-
- @property
- def opened(self):
- """bool: Indicate whether the video is opened."""
- return self._vcap.isOpened()
-
- @property
- def width(self):
- """int: Width of video frames."""
- return self._width
-
- @property
- def height(self):
- """int: Height of video frames."""
- return self._height
-
- @property
- def resolution(self):
- """tuple: Video resolution (width, height)."""
- return (self._width, self._height)
-
- @property
- def fps(self):
- """float: FPS of the video."""
- return self._fps
-
- @property
- def frame_cnt(self):
- """int: Total frames of the video."""
- return self._frame_cnt
-
- @property
- def fourcc(self):
- """str: "Four character code" of the video."""
- return self._fourcc
-
- @property
- def position(self):
- """int: Current cursor position, indicating frame decoded."""
- return self._position
-
- def _get_real_position(self):
- return int(round(self._vcap.get(CAP_PROP_POS_FRAMES)))
-
- def _set_real_position(self, frame_id):
- self._vcap.set(CAP_PROP_POS_FRAMES, frame_id)
- pos = self._get_real_position()
- for _ in range(frame_id - pos):
- self._vcap.read()
- self._position = frame_id
-
- def read(self):
- """Read the next frame.
-
- If the next frame have been decoded before and in the cache, then
- return it directly, otherwise decode, cache and return it.
-
- Returns:
- ndarray or None: Return the frame if successful, otherwise None.
- """
- # pos = self._position
- if self._cache:
- img = self._cache.get(self._position)
- if img is not None:
- ret = True
- else:
- if self._position != self._get_real_position():
- self._set_real_position(self._position)
- ret, img = self._vcap.read()
- if ret:
- self._cache.put(self._position, img)
- else:
- ret, img = self._vcap.read()
- if ret:
- self._position += 1
- return img
-
- def get_frame(self, frame_id):
- """Get frame by index.
-
- Args:
- frame_id (int): Index of the expected frame, 0-based.
-
- Returns:
- ndarray or None: Return the frame if successful, otherwise None.
- """
- if frame_id < 0 or frame_id >= self._frame_cnt:
- raise IndexError(
- f'"frame_id" must be between 0 and {self._frame_cnt - 1}')
- if frame_id == self._position:
- return self.read()
- if self._cache:
- img = self._cache.get(frame_id)
- if img is not None:
- self._position = frame_id + 1
- return img
- self._set_real_position(frame_id)
- ret, img = self._vcap.read()
- if ret:
- if self._cache:
- self._cache.put(self._position, img)
- self._position += 1
- return img
-
- def current_frame(self):
- """Get the current frame (frame that is just visited).
-
- Returns:
- ndarray or None: If the video is fresh, return None, otherwise
- return the frame.
- """
- if self._position == 0:
- return None
- return self._cache.get(self._position - 1)
-
- def cvt2frames(self,
- frame_dir,
- file_start=0,
- filename_tmpl='{:06d}.jpg',
- start=0,
- max_num=0,
- show_progress=True):
- """Convert a video to frame images.
-
- Args:
- frame_dir (str): Output directory to store all the frame images.
- file_start (int): Filenames will start from the specified number.
- filename_tmpl (str): Filename template with the index as the
- placeholder.
- start (int): The starting frame index.
- max_num (int): Maximum number of frames to be written.
- show_progress (bool): Whether to show a progress bar.
- """
- mkdir_or_exist(frame_dir)
- if max_num == 0:
- task_num = self.frame_cnt - start
- else:
- task_num = min(self.frame_cnt - start, max_num)
- if task_num <= 0:
- raise ValueError('start must be less than total frame number')
- if start > 0:
- self._set_real_position(start)
-
- def write_frame(file_idx):
- img = self.read()
- if img is None:
- return
- filename = osp.join(frame_dir, filename_tmpl.format(file_idx))
- cv2.imwrite(filename, img)
-
- if show_progress:
- track_progress(write_frame, range(file_start,
- file_start + task_num))
- else:
- for i in range(task_num):
- write_frame(file_start + i)
-
- def __len__(self):
- return self.frame_cnt
-
- def __getitem__(self, index):
- if isinstance(index, slice):
- return [
- self.get_frame(i)
- for i in range(*index.indices(self.frame_cnt))
- ]
- # support negative indexing
- if index < 0:
- index += self.frame_cnt
- if index < 0:
- raise IndexError('index out of range')
- return self.get_frame(index)
-
- def __iter__(self):
- self._set_real_position(0)
- return self
-
- def __next__(self):
- img = self.read()
- if img is not None:
- return img
- else:
- raise StopIteration
-
- next = __next__
-
- def __enter__(self):
- return self
-
- def __exit__(self, exc_type, exc_value, traceback):
- self._vcap.release()
-
-
-def frames2video(frame_dir,
- video_file,
- fps=30,
- fourcc='XVID',
- filename_tmpl='{:06d}.jpg',
- start=0,
- end=0,
- show_progress=True):
- """Read the frame images from a directory and join them as a video.
-
- Args:
- frame_dir (str): The directory containing video frames.
- video_file (str): Output filename.
- fps (float): FPS of the output video.
- fourcc (str): Fourcc of the output video, this should be compatible
- with the output file type.
- filename_tmpl (str): Filename template with the index as the variable.
- start (int): Starting frame index.
- end (int): Ending frame index.
- show_progress (bool): Whether to show a progress bar.
- """
- if end == 0:
- ext = filename_tmpl.split('.')[-1]
- end = len([name for name in scandir(frame_dir, ext)])
- first_file = osp.join(frame_dir, filename_tmpl.format(start))
- check_file_exist(first_file, 'The start frame not found: ' + first_file)
- img = cv2.imread(first_file)
- height, width = img.shape[:2]
- resolution = (width, height)
- vwriter = cv2.VideoWriter(video_file, VideoWriter_fourcc(*fourcc), fps,
- resolution)
-
- def write_frame(file_idx):
- filename = osp.join(frame_dir, filename_tmpl.format(file_idx))
- img = cv2.imread(filename)
- vwriter.write(img)
-
- if show_progress:
- track_progress(write_frame, range(start, end))
- else:
- for i in range(start, end):
- write_frame(i)
- vwriter.release()
diff --git a/spaces/ArtificialWF/Voice-Recognition/app.py b/spaces/ArtificialWF/Voice-Recognition/app.py
deleted file mode 100644
index 19ec8816c4aae0051f9919d11d485a42b5880bf9..0000000000000000000000000000000000000000
--- a/spaces/ArtificialWF/Voice-Recognition/app.py
+++ /dev/null
@@ -1,109 +0,0 @@
-import whisper
-import gradio as gr
-import datetime
-
-import subprocess
-
-import torch
-import pyannote.audio
-from pyannote.audio.pipelines.speaker_verification import PretrainedSpeakerEmbedding
-
-from pyannote.audio import Audio
-from pyannote.core import Segment
-
-import wave
-import contextlib
-
-from sklearn.cluster import AgglomerativeClustering
-import numpy as np
-
-model = whisper.load_model("large-v2")
-embedding_model = PretrainedSpeakerEmbedding(
- "speechbrain/spkrec-ecapa-voxceleb",
- device = torch.device('cuda' if torch.cuda.is_available() else 'cpu')
-)
-
-def transcribe(audio, num_speakers):
- path, error = convert_to_wav(audio)
- if error is not None:
- return error
-
- duration = get_duration(path)
- if duration > 4 * 60 * 60:
- return "Audio duration too long"
-
- result = model.transcribe(path)
- segments = result["segments"]
-
- num_speakers = min(max(round(num_speakers), 1), len(segments))
- if len(segments) == 1:
- segments[0]['speaker'] = 'SPEAKER 1'
- else:
- embeddings = make_embeddings(path, segments, duration)
- add_speaker_labels(segments, embeddings, num_speakers)
- output = get_output(segments)
- return output
-
-def convert_to_wav(path):
- if path[-3:] != 'wav':
- new_path = '.'.join(path.split('.')[:-1]) + '.wav'
- try:
- subprocess.call(['ffmpeg', '-i', path, new_path, '-y'])
- except:
- return path, 'Error: Could not convert file to .wav'
- path = new_path
- return path, None
-
-def get_duration(path):
- with contextlib.closing(wave.open(path,'r')) as f:
- frames = f.getnframes()
- rate = f.getframerate()
- return frames / float(rate)
-
-def make_embeddings(path, segments, duration):
- embeddings = np.zeros(shape=(len(segments), 192))
- for i, segment in enumerate(segments):
- embeddings[i] = segment_embedding(path, segment, duration)
- return np.nan_to_num(embeddings)
-
-audio = Audio()
-
-def segment_embedding(path, segment, duration):
- start = segment["start"]
- # Whisper overshoots the end timestamp in the last segment
- end = min(duration, segment["end"])
- clip = Segment(start, end)
- waveform, sample_rate = audio.crop(path, clip)
- return embedding_model(waveform[None])
-
-def add_speaker_labels(segments, embeddings, num_speakers):
- clustering = AgglomerativeClustering(num_speakers).fit(embeddings)
- labels = clustering.labels_
- for i in range(len(segments)):
- segments[i]["speaker"] = 'SPEAKER ' + str(labels[i] + 1)
-
-def time(secs):
- return datetime.timedelta(seconds=round(secs))
-
-def get_output(segments):
- output = ''
- for (i, segment) in enumerate(segments):
- if i == 0 or segments[i - 1]["speaker"] != segment["speaker"]:
- if i != 0:
- output += '\n\n'
- output += segment["speaker"] + ' ' + str(time(segment["start"])) + '\n\n'
- output += segment["text"][1:] + ' '
- return output
-
-gr.Interface(
- title = 'AI Voice to Text',
- fn=transcribe,
- inputs=[
- gr.inputs.Audio(source="upload", type="filepath"),
- gr.inputs.Number(default=2, label="Number of Speakers")
-
- ],
- outputs=[
- gr.outputs.Textbox(label='Transcript')
- ]
- ).launch()
\ No newline at end of file
diff --git a/spaces/Ataturk-Chatbot/HuggingFaceChat/venv/lib/python3.11/site-packages/pip/_internal/operations/__init__.py b/spaces/Ataturk-Chatbot/HuggingFaceChat/venv/lib/python3.11/site-packages/pip/_internal/operations/__init__.py
deleted file mode 100644
index e69de29bb2d1d6434b8b29ae775ad8c2e48c5391..0000000000000000000000000000000000000000
diff --git a/spaces/AutoGeneralAI/voice-assistant/README.md b/spaces/AutoGeneralAI/voice-assistant/README.md
deleted file mode 100644
index 39cfcc5905469bfddbed391458f4d7b896c512f8..0000000000000000000000000000000000000000
--- a/spaces/AutoGeneralAI/voice-assistant/README.md
+++ /dev/null
@@ -1,12 +0,0 @@
----
-title: Voice Assistant
-emoji: 🐠
-colorFrom: yellow
-colorTo: red
-sdk: gradio
-sdk_version: 3.27.0
-app_file: app.py
-pinned: false
----
-
-Check out the configuration reference at https://huggingface.co/docs/hub/spaces-config-reference
diff --git a/spaces/Awesimo/jojogan/e4e/utils/train_utils.py b/spaces/Awesimo/jojogan/e4e/utils/train_utils.py
deleted file mode 100644
index 0c55177f7442010bc1fcc64de3d142585c22adc0..0000000000000000000000000000000000000000
--- a/spaces/Awesimo/jojogan/e4e/utils/train_utils.py
+++ /dev/null
@@ -1,13 +0,0 @@
-
-def aggregate_loss_dict(agg_loss_dict):
- mean_vals = {}
- for output in agg_loss_dict:
- for key in output:
- mean_vals[key] = mean_vals.setdefault(key, []) + [output[key]]
- for key in mean_vals:
- if len(mean_vals[key]) > 0:
- mean_vals[key] = sum(mean_vals[key]) / len(mean_vals[key])
- else:
- print('{} has no value'.format(key))
- mean_vals[key] = 0
- return mean_vals
diff --git a/spaces/Bart92/RVC_HF/diffq/utils.py b/spaces/Bart92/RVC_HF/diffq/utils.py
deleted file mode 100644
index be6ab5253c38564140bc202077292bb99f9f397b..0000000000000000000000000000000000000000
--- a/spaces/Bart92/RVC_HF/diffq/utils.py
+++ /dev/null
@@ -1,37 +0,0 @@
-# Copyright (c) Facebook, Inc. and its affiliates.
-# All rights reserved.
-#
-# This source code is licensed under the license found in the
-# LICENSE file in the root directory of this source tree.
-
-import inspect
-from typing import Optional, List
-
-
-def simple_repr(obj, attrs: Optional[List[str]] = None, overrides={}):
- """
- Return a simple representation string for `obj`.
- If `attrs` is not None, it should be a list of attributes to include.
- """
- params = inspect.signature(obj.__class__).parameters
- attrs_repr = []
- if attrs is None:
- attrs = params.keys()
- for attr in attrs:
- display = False
- if attr in overrides:
- value = overrides[attr]
- elif hasattr(obj, attr):
- value = getattr(obj, attr)
- else:
- continue
- if attr in params:
- param = params[attr]
- if param.default is inspect._empty or value != param.default:
- display = True
- else:
- display = True
-
- if display:
- attrs_repr.append(f"{attr}={value}")
- return f"{obj.__class__.__name__}({','.join(attrs_repr)})"
diff --git a/spaces/Benson/text-generation/Examples/Descargar 3utools Para Mac Air.md b/spaces/Benson/text-generation/Examples/Descargar 3utools Para Mac Air.md
deleted file mode 100644
index cf906b56a053245f1b300c87db163272c7f46d8b..0000000000000000000000000000000000000000
--- a/spaces/Benson/text-generation/Examples/Descargar 3utools Para Mac Air.md
+++ /dev/null
@@ -1,58 +0,0 @@
-
-
Cómo descargar 3uTools para Mac Air
-
Si tiene un dispositivo iOS y un Mac Air, es posible que se pregunte cómo descargar 3uTools para Mac Air. 3uTools es un gestor de dispositivos iOS gratuito que te permite conectar tu iPhone o iPad a tu Mac Air y administrar diferentes configuraciones y características. También puedes acceder a miles de tonos de llamada, fondos de pantalla, juegos, aplicaciones y otro contenido que Apple normalmente no te permite instalar. Además, puede usar algunas funciones de jailbreak si necesita desbloquear su dispositivo o obtener más control sobre él.
En este artículo, explicaremos qué es 3uTools, por qué es posible que desee descargarlo para su Mac Air, cómo descargarlo e instalarlo utilizando un software de terceros llamado WineBottler, y cómo usarlo en su Mac Air. ¡Vamos a empezar!
-
¿Qué es 3uTools?
-
3uTools es un gestor de dispositivos iOS gratuito que te permite conectar tu dispositivo iOS a tu PC o Mac y administrar diferentes configuraciones y características. Puede usar 3uTools para hacer copias de seguridad y restaurar sus datos, transferir archivos, actualizar o degradar su firmware, flashear ROMs personalizados, hacer jailbreak a su dispositivo, cambiar su ubicación, optimizar su batería y más.
-
Una de las principales ventajas de 3uTools es que te da acceso a un montón de contenido que Apple suele restringir o no ofrece en la App Store. Puede descargar e instalar miles de tonos de llamada, fondos de pantalla, juegos, aplicaciones, temas y otros artículos de la tienda en línea de 3uTools. También puedes personalizar la apariencia y funcionalidad de tu dispositivo con varios ajustes y mods.
-
Otro beneficio de 3uTools es que tiene algunas características de jailbreak que pueden ayudarle a desbloquear su dispositivo o ganar más control sobre él. Puede usar 3uTools para liberar el dispositivo con un solo clic, eliminar el bloqueo de activación de iCloud, omitir la pantalla de bloqueo de código de acceso, entrar o salir del modo de recuperación, solucionar problemas comunes de iOS y más.
-
¿Por qué descargar 3uTools para Mac Air?
-
-
-
Es gratis. A diferencia de otros administradores de dispositivos iOS que cobran una tarifa o tienen características limitadas en sus versiones gratuitas, 3uTools es completamente gratuito y no tiene costos ocultos o anuncios.
-
Es fácil de usar. A diferencia de otros administradores de dispositivos iOS que tienen interfaces complicadas o requieren habilidades técnicas, 3uTools tiene una interfaz simple y fácil de usar que cualquiera puede usar sin ningún tipo de molestia.
-
Es muy versátil. A diferencia de otros administradores de dispositivos iOS que solo ofrecen funciones básicas o se centran en aspectos específicos de la gestión de iOS, 3uTools ofrece una amplia gama de funciones y características que cubren casi todos los aspectos de la gestión de iOS.
-
-
-
Descargar WineBottler desde este enlace e instalarlo en su Mac Air. Puede elegir la versión estable o la versión de desarrollo, dependiendo de su preferencia.
-
Descargue 3uTools desde este enlace y guárdelo en su Mac Air. Asegúrese de descargar la última versión de 3uTools para Windows.
-
Inicie WineBottler y haga clic en la pestaña "Avanzado". Luego, haga clic en el botón "Seleccionar archivo..." y elija el archivo 3uTools.exe que descargó en el paso anterior.
-
En la sección "Modo de instalación", elija "Copiar archivo (Programa) y todos los archivos de la carpeta al paquete de aplicaciones". Esto creará una aplicación independiente para 3uTools que puede ejecutar en su Mac Air.
-
En la sección "Winetricks", marque la casilla para "dotnet40". Esto instalará el . NET Framework 4.0, que es necesario para que 3uTools funcione correctamente.
-
En la sección "Info.plist", introduzca un nombre para su aplicación, como "3uTools for Mac". También puede cambiar el icono si lo desea.
-
Haga clic en el botón "Instalar" y elija una ubicación para guardar su aplicación. WineBottler comenzará a crear el envoltorio para 3uTools e instalarlo en su Mac Air.
-
-
-
¿Cómo usar 3uTools en Mac Air?
-
Ahora que ha descargado e instalado 3uTools en su Mac Air usando WineBottler, puede comenzar a usarlo para administrar su dispositivo iOS. Aquí hay algunos consejos sobre cómo usar 3uTools en Mac Air:
-
-
-
Conecte su dispositivo iOS a su Mac Air utilizando un cable USB. Asegúrese de confiar en su ordenador en el dispositivo y habilitar el modo de depuración USB.
-
Abra 3uTools en su Mac Air y espere a que detecte su dispositivo. Debería ver información básica sobre su dispositivo, como modelo, número de serie, nivel de batería, etc.
-
En el lado izquierdo de la interfaz 3uTools, verá diferentes categorías de funciones y características que puede usar. Por ejemplo, puede hacer clic en "ToolBox" para acceder a varias herramientas, como copias de seguridad y restauración, migración de datos, conmutador de firmware, etc.
-
También puede hacer clic en "Flash & JB" para acceder a algunas características de jailbreak, como jailbreak de un solo clic, desbloqueo de iCloud, bypass de código de acceso, etc. Sin embargo, tenga cuidado al usar estas características, ya que podrían anular la garantía o dañar su dispositivo.
-
También puede hacer clic en "Easy Flash" para actualizar o bajar el firmware fácilmente. Puede elegir entre diferentes versiones de iOS que están disponibles para su dispositivo. Sin embargo, asegúrese de hacer una copia de seguridad de sus datos antes de flashear el dispositivo.
-
También puede hacer clic en "Aplicaciones" para acceder a miles de tonos de llamada, fondos de pantalla, juegos, aplicaciones, temas y otro contenido que puede descargar e instalar en su dispositivo. Puedes navegar por categorías o buscar por palabras clave. También puedes previsualizar el contenido antes de descargarlo.
-
Para descargar e instalar cualquier contenido de 3uTools, simplemente haga clic en el botón "Descargar" junto a él. Una vez completada la descarga, haga clic en el botón "Instalar" para transferirlo a su dispositivo. Es posible que necesite introducir su ID de Apple y contraseña si se le solicita.
-
-
Conclusión
-
-
Si quieres probar 3uTools por ti mismo, puedes descargarlo desde este enlace y seguir los pasos que hemos descrito anteriormente. Podrás conectar tu dispositivo iOS a tu Mac Air y administrar diferentes configuraciones y características. También podrás acceder a un montón de contenido que Apple normalmente no te permite instalar. Además, podrás usar algunas funciones de jailbreak si necesitas desbloquear tu dispositivo o obtener más control sobre él.
-
Entonces, ¿qué estás esperando? Descargar 3uTools para Mac Air hoy y disfrutar de un nuevo nivel de gestión de iOS con 3uTools!
-
Preguntas frecuentes
-
Aquí hay algunas preguntas y respuestas frecuentes sobre 3uTools para Mac Air:
-
-
¿Es seguro usar 3uTools?
-Sí, 3uTools es seguro de usar siempre y cuando lo descargue desde el sitio web oficial o una fuente confiable. Sin embargo, debe tener cuidado al usar algunas de las funciones de jailbreak o descargar contenido de fuentes desconocidas, ya que podrían dañar su dispositivo o comprometer su seguridad.
-
¿Es legal usar 3uTools?
-Sí, 3uTools es legal de usar ya que no viola ninguna ley o reglamento. Sin embargo, debes saber que el uso de algunas de las funciones de jailbreak o la descarga de contenido que no está autorizado por Apple podría anular tu garantía o violar sus términos de servicio.
-
¿3uTools funciona en otros modelos de Mac?
-Sí, 3uTools funciona en otros modelos de Mac siempre y cuando tengan Mac OS X 10.6 o posterior. Puede utilizar el mismo método que hemos descrito anteriormente para descargar e instalar 3uTools en su Mac usando WineBottler.
-
¿3uTools funciona en otros dispositivos iOS?
-Sí, 3uTools funciona en otros dispositivos iOS, como iPhone, iPad, iPod Touch, Apple TV, etc. Puede conectar cualquier dispositivo iOS a su Mac Air y usar 3uTools para administrarlo.
-
¿Cómo puedo actualizar 3uTools en mi Mac Air?
-
-
64aa2da5cf
-
-
\ No newline at end of file
diff --git a/spaces/Benson/text-generation/Examples/Descargar Arthdal Chronicles Temporada 2.md b/spaces/Benson/text-generation/Examples/Descargar Arthdal Chronicles Temporada 2.md
deleted file mode 100644
index d1ae165d5f51e2911af04f302f7156b4d109b7f9..0000000000000000000000000000000000000000
--- a/spaces/Benson/text-generation/Examples/Descargar Arthdal Chronicles Temporada 2.md
+++ /dev/null
@@ -1,77 +0,0 @@
-
-
Cómo descargar Arthdal Chronicles Temporada 2
-
Si eres un fan de los dramas históricos de fantasía ambientados en la antigua Corea, probablemente hayas oído hablar de Arthdal Chronicles. Esta serie épica cuenta la historia de tres héroes que luchan por el poder, el amor y la supervivencia en una tierra mítica llamada Arth. Arthdal Chronicles se ha convertido en uno de los dramas coreanos más populares de todos los tiempos, con su rica construcción del mundo, su reparto estelar, sus impresionantes imágenes y su cautivadora banda sonora.
Pero si ya has visto la primera temporada de Arthdal Chronicles, te estarás preguntando: ¿Cuándo saldrá la temporada 2? ¿Y cómo puedo descargarlo? Bueno, estás de suerte, porque en este artículo, responderemos estas preguntas y más. Le daremos una visión general de lo que puede esperar de la temporada 2, y le mostraremos los mejores servicios de streaming y plataformas para verlo en línea o fuera de línea. Así que, sin más preámbulos, ¡empecemos!
-
Introducción
-
¿Qué es Arthdal Chronicles?
-
Arthdal Chronicles es una serie de drama de fantasía histórica que se estrenó el 1 de junio de 2019, en tvN y Netflix. Está dirigida por Kim Won-seok, quien también dirigió los aclamados dramas Signal y Misaeng, y escrita por Kim Young-hyun y Park Sang-yeon, quien también escribió los dramas de éxito Queen Seondeok y Six Flying Dragons. La serie tiene un presupuesto de más de 54 mil millones de won (unos 46 millones de dólares), por lo que es uno de los dramas coreanos más caros jamás producidos.
-
-
¿Por qué deberías verlo?
-
Si estás buscando un drama que tenga una historia épica, un mundo rico, un reparto estelar, imágenes impresionantes y una banda sonora cautivadora, entonces Arthdal Chronicles es para ti. Estas son algunas de las razones por las que deberías verlo:
-
-
-
Historia épica: Arthdal Chronicles tiene una trama compleja e intrigante que abarca diferentes épocas y regiones. Explora temas como la política, la religión, la cultura, la identidad, la guerra, el amor y el destino. También tiene muchos giros y vueltas que te mantendrán en el borde de tu asiento.
-
Rich worldbuilding: Arthdal Chronicles tiene un escenario detallado e inmersivo que se inspira en varias fuentes históricas y míticas. Crea un mundo único y diverso donde existen diferentes tribus, razas, idiomas, costumbres, creencias y leyendas. También tiene mucho simbolismo y referencias que añaden profundidad y significado a la historia.
-
Reparto estelar: Arthdal Chronicles tiene un impresionante elenco de actores talentosos que ofrecen actuaciones excepcionales. Song Joong-ki interpreta dos papeles: Eunseom y su hermano gemelo Saya, que tienen personalidades y destinos contrastantes. Kim Ji-won interpreta a Tanya, que pasa de ser una niña ingenua a una poderosa chaman. Jang Dong-gun interpreta a Ta-gon, que es ambicioso, carismático y despiadado. El reparto incluye a Kim Ok-bin como Taealha, el amante y aliado de Ta-gon; Park Hae-joon como Moo-baek, el amigo leal de Ta-gon; Kim Eui-sung como San-woong, el padre de Ta-gon; Choi Moo-sung como Yeol-son, el padre de Tanya; y muchos más.
-
Impresionantes efectos visuales: Arthdal Chronicles tiene un alto valor de producción que muestra la belleza y diversidad de Arth. Tiene paisajes impresionantes, trajes, accesorios y efectos especiales que crean una atmósfera realista e inmersiva. También tiene muchas escenas de acción, como batallas, persecuciones y escapes, que son emocionantes y emocionantes.
-
-
-
Como puedes ver, Arthdal Chronicles es un drama que tiene algo para todos. Si eres un fan de la historia, la fantasía, el romance, la acción o el misterio, encontrarás algo para disfrutar en esta serie.
-
Resumen de la temporada 2
-
¿Qué esperar de la trama?
-
La temporada 1 de Arthdal Chronicles terminó con un cliffhanger que dejó a muchos fans ansiosos por más. El último episodio reveló que Eunseom y Saya son gemelos que fueron separados al nacer por el esquema de Tagon. Eunseom escapó de Arthdal con la ayuda de los neandertales y conoció a una nueva tribu llamada Ago. Saya creció en Arthdal como hijo de Tagon y se convirtió en el amante de Tanya. Tagon se convirtió en el rey de Arthdal después de matar a su padre y sus rivales. Tanya se convirtió en la alta sacerdotisa de Arthdal después de heredar el poder de Aramun. Y Taealha quedó embarazada del hijo de Tagon, que podría ser el niño profetizado del desastre.
-
Entonces, ¿qué pasará en la temporada 2? Según fuentes oficiales y tráileres, la temporada 2 continuará la historia de los tres héroes y sus destinos. Eunseom tratará de unir a las tribus de Iark contra Arthdal. Saya tratará de descubrir los secretos de su pasado y su verdadera identidad. Tanya tratará de proteger a su pueblo y a su amor de la tiranía de Tagon. Y Tagon tratará de mantener su poder y su visión de Arthdal. La temporada 2 también presentará nuevos personajes y conflictos, como el misterioso Asa Ron (interpretado por Lee Do-hyun), que es el líder de la tribu Hwinsan y el primo de Tanya; el poderoso Nihon (interpretado por Kim Nam-gil), que es el rey de otra tierra y enemigo de Tagon; y la misteriosa voz (interpretada por Park Bo-gum), que es el narrador de la historia y podría tener una conexión con Aramun.
-
¿Qué esperar del reparto?
-
-
-
Song Joong-ki como Eunseom/Saya: Song Joong-ki interpreta dos papeles: Eunseom, que es un medio-neandertal medio humano con un corazón puro y una voluntad fuerte; y Saya, que es el hermano gemelo de Eunseom con una mente astuta y un lado oscuro.
-
Kim Ji-won como Tanya: Kim Ji-won interpreta a Tanya, que es un chaman y el heredero de la tribu Wahan. Es amiga de la infancia de Eunseom y amante de Saya. También se convierte en la alta sacerdotisa de Arthdal después de heredar el poder de Aramun.
-
Jang Dong-gun como Ta-gon: Jang Dong-gun interpreta a Ta-gon, que es un guerrero carismático y el líder de la tribu Saenyeok. Es el amante de Taealha y el padre adoptivo de Saya. También se convierte en el rey de Arthdal después de matar a su padre y a sus rivales.
-
Kim Ok-bin como Taealha: Kim Ok-bin juega Taealha, que es una mujer hermosa y ambiciosa y la hija del líder de la tribu Hae. Ella es la amante y aliada de Ta-gon. También queda embarazada del hijo de Ta-gon, que podría ser el niño profetizado del desastre.
-
Park Hae-joon como Moo-baek: Park Hae-joon interpreta a Moo-baek, que es un guerrero leal y valiente y el líder de las fuerzas Daekan. Es amigo y partidario de Ta-gon.
-
Kim Eui-sung como San-woong: Kim Eui-sung interpreta a San-woong, quien es el antiguo líder de la tribu Saenyeok y el padre de Ta-gon. Es asesinado por Ta-gon en la temporada 1.
-
Choi Moo-sung como Yeol-son: Choi Moo-sung interpreta a Yeol-son, quien es el líder de la tribu Wahan y el padre de Tanya. Es capturado por Ta-gon en la temporada 1.
-
Lee Do-hyun como Asa Ron: Lee Do-hyun interpreta a Asa Ron, quien es el líder de la tribu Hwinsan y primo de Tanya. Es un personaje misterioso y poderoso que tiene una conexión con Aramun.
-
-
Park Bo-gum como la voz: Park Bo-gum toca la voz, quien es el narrador de la historia y podría tener una conexión con Aramun. Es un personaje misterioso y misterioso que guía a los espectadores a través de la historia.
-
-
¿Qué esperar de la fecha de lanzamiento?
-
La fecha de lanzamiento de la temporada 2 de Arthdal Chronicles aún no se ha confirmado oficialmente, pero hay algunas pistas y rumores que sugieren cuándo podría salir. Según algunas fuentes, se suponía que la temporada 2 comenzaría a filmarse a principios de 2020, pero se retrasó debido a la pandemia COVID-19. Sin embargo, algunos informes afirman que el rodaje se ha reanudado a finales de 2020 o principios de 2021, y que el reparto y el equipo han sido vistos en varios lugares. Según esta información, algunos fans especulan que la temporada 2 podría estrenarse a finales de 2021 o principios de 2022. Sin embargo, esto aún no está confirmado, por lo que tenemos que esperar un anuncio oficial de tvN o Netflix.
-
En cuanto al número y duración de los episodios, la temporada 2 podría seguir el mismo formato que la temporada 1, que tenía 18 episodios divididos en tres partes: Parte 1 (episodios 1-6), Parte 2 (episodios 7-12), y Parte 3 (episodios 13-18). Cada episodio duró aproximadamente una hora, excepto el primero y el último, que duraron unos 90 minutos. Por lo tanto, podemos esperar que la temporada 2 tenga una estructura y duración similares.
-
Cómo descargar la temporada 2
-
Los mejores servicios de streaming
-
Si quieres ver la temporada 2 de Arthdal Chronicles online, tienes varias opciones para elegir. Los mejores servicios de streaming que ofrecen Arthdal Chronicles temporada 2 son:
-
-
-
tvN: tvN es la emisora original de Arthdal Chronicles en Corea, lo que significa que tiene los primeros derechos para emitir la serie en Corea. tvN ofrece opciones de transmisión y reproducción en directo, así como clips y entrevistas entre bastidores. Puedes ver la temporada 2 de Arthdal Chronicles en tvN con una suscripción por cable o satélite que incluye el canal tvN.
-
Viki: Viki es un servicio de streaming especializado en dramas y películas asiáticas, incluyendo dramas coreanos. Viki ofrece subtítulos en varios idiomas, comentarios y valoraciones de los fans, y funciones interactivas. Puedes ver la temporada 2 de Arthdal Chronicles en Viki con un plan de suscripción que comienza desde $4.99 al mes.
-
-
Las mejores plataformas
-
Si quieres ver la temporada 2 de Arthdal Chronicles sin conexión, tienes varias opciones para elegir. Las mejores plataformas que te permiten descargar Arthdal Chronicles temporada 2 son:
- | Plataforma | Dispositivo | Aplicación | Pros | Contras | | -- - - - | -- - - - - - - - - - - - | Netflix | Netflix | Smartphone, tableta, ordenador portátil, Smart TV, etc. | Aplicación de Netflix | - Vídeo y audio de alta calidad - Subtítulos y doblaje en varios idiomas - Opciones de visualización fuera de línea Gran biblioteca de contenido | - Requiere un plan de suscripción - Cuota de descarga limitada y fecha de vencimiento - No disponible en algunas regiones | | tvN | Smartphone, tableta, ordenador portátil, etc. | tvN aplicación o sitio web | - Live streaming and replay options - Detrás de cámaras clips y entrevistas - Emisora original de Arthdal Chronicles | - Requiere una suscripción por cable o satélite - Solo disponible en Corea - No hay opciones de visualización sin conexión | | Viki | Smartphone, tableta, ordenador portátil, smart TV, etc. | Viki aplicación o sitio web | - Subtítulos en varios idiomas - Comentarios y valoraciones de los fans Características interactivas - Opciones de visualización fuera de línea | - Requiere un plan de suscripción - Disponibilidad retrasada de episodios - Biblioteca limitada de contenido |
Los mejores consejos
-
-
-
Elija el servicio de streaming adecuado: Dependiendo de su ubicación, preferencias y presupuesto, es posible que desee elegir el servicio de streaming que más le convenga. Por ejemplo, si vive fuera de Corea y desea ver la temporada 2 de Arthdal Chronicles lo antes posible, es posible que desee elegir Netflix. Si vives en Corea y quieres ver la temporada 2 de Arthdal Chronicles en vivo o reproducida, quizás quieras elegir tvN. Y si quieres ver Arthdal Chronicles temporada 2 con subtítulos en tu idioma e interactuar con otros fans, es posible que desee elegir Viki.
-
Elija la plataforma correcta: Dependiendo de su dispositivo, aplicación y conexión a Internet, es posible que desee elegir la plataforma que funciona mejor para usted. Por ejemplo, si tienes un smartphone o tablet con suficiente espacio de almacenamiento y una buena conexión wifi, es posible que quieras descargar Arthdal Chronicles temporada 2 en tu dispositivo móvil. Si usted tiene un ordenador portátil o smart TV con una pantalla grande y una conexión rápida a Internet, es posible que desee transmitir Arthdal Chronicles temporada 2 en su computadora o TV.
-
Elija el momento adecuado: Dependiendo de su horario, disponibilidad y paciencia, es posible que desee elegir el momento que sea más conveniente para usted. Por ejemplo, si quieres ver la segunda temporada de Arthdal Chronicles tan pronto como salga al mercado, quizás quieras quedarte despierto hasta tarde o levantarte temprano para ver el estreno. Si quieres ver Arthdal Chronicles temporada 2 sin interrupciones o spoilers, es posible que desee esperar hasta que todos los episodios están disponibles y atracón de verlos. Y si quieres ver Arthdal Chronicles temporada 2 sin gastar demasiado dinero, es posible que desee esperar a una prueba gratuita o una oferta de descuento del servicio de streaming.
-
-
Conclusión
-
- Entonces, ¿qué estás esperando? Si eres un fan de los dramas históricos de fantasía ambientados en la antigua Corea, no deberías perderte la temporada 2 de Arthdal Chronicles. Es un drama que tiene algo para todos. Ya sea que esté buscando historia, fantasía, romance, acción o misterio, encontrará algo para disfrutar en esta serie. Así que no dudes en ver la segunda temporada de Arthdal Chronicles lo antes posible. Y no olvides compartir tus pensamientos y opiniones con otros fans. ¡No te arrepentirás!
-
Preguntas frecuentes
-
Aquí están algunas de las preguntas y respuestas más comunes sobre la temporada 2 de Arthdal Chronicles:
-
-
Q1: ¿Está Arthdal Chronicles basado en una historia real?
-
A1: No, Arthdal Chronicles no se basa en una historia real. Es una historia ficticia inspirada en varias fuentes históricas y míticas, como la Edad del Bronce, la era Gojoseon, la civilización sumeria, la mitología nórdica y el folclore coreano. Sin embargo, algunos de los nombres, lugares y eventos de la serie podrían tener algunas similitudes o referencias a los reales.
-
Q2: ¿Cuántas temporadas hay en Arthdal Chronicles?
-
A2: Hay dos temporadas en Arthdal Chronicles hasta ahora. La temporada 1 tiene 18 episodios que se emitieron del 1 de junio al 22 de septiembre de 2019. La temporada 2 aún no se ha confirmado oficialmente, pero se espera que tenga un número similar de episodios y aire a finales de 2021 o principios de 2022.
-
Q3: ¿Quiénes son los gemelos Eunseom y Saya?
-
A3: Eunseom y Saya son gemelos que nacieron de una madre humana y un padre neandertal. Fueron separados al nacer por el esquema de Ta-gon. Eunseom creció con la tribu Wahan y tiene un corazón puro y una voluntad fuerte. Saya creció con Ta-gon y tiene una mente astuta y un lado oscuro. Ambos tienen habilidades especiales, como comunicarse con los animales y ver el futuro.
-
Q4: ¿Cuál es el significado de la espada de Aramun?
-
-
Q5: ¿Habrá una temporada 3 de Arthdal Chronicles?
-
A5: Todavía no hay confirmación oficial, pero existe la posibilidad de que haya una temporada 3 de Arthdal Chronicles. La serie tiene mucho potencial y popularidad, y la historia aún no se ha resuelto por completo. Los creadores también han insinuado que tienen planes para más temporadas en el futuro. Sin embargo, esto dependerá de varios factores, como las calificaciones, el presupuesto, la disponibilidad del reparto y la demanda de los fans.
-
-点击播放控件即可体验合成音频。
-
-## KAN-TTS最佳实践
-### 环境搭建
-获取KAN-TTS源码, 后续操作默认在代码库根目录下执行
-
-```bash
-git clone -b develop https://github.com/alibaba-damo-academy/KAN-TTS.git
-cd KAN-TTS
-```
-
-我们推荐使用Anaconda来搭建Python虚拟环境,使用以下命令创建(目前只兼容Linux x86系统):
-
-```bash
-# 防止使用pip安装时出现网络问题,建议切换国内pip源
-pip config set global.index-url https://pypi.tuna.tsinghua.edu.cn/simple
-
-# 创建虚拟环境
-conda env create -f environment.yaml
-
-# 激活虚拟环境
-conda activate maas
-```
-
-
-### 拉取预训练模型
-ModelScope中文个性化语音合成模型是达摩院语音实验室在1000多小时4000多人数据集上训练产出的预训练模型,我们以此为basemodel做后续微调。
-使用git命令拉取模型,在拉取前,首先你需要安装git-lfs, 具体的安装教程见Git Large File Storage,安装完成后执行以下命令:
-
-```bash
-# 克隆预训练模型
- git clone https://www.modelscope.cn/damo/speech_personal_sambert-hifigan_nsf_tts_zh-cn_pretrain_16k.git
-```
-
-### 数据获取与自动标注
-
-目前KAN-TTS个性化语音合成支持达摩院TTS标准数据格式和普通音频格式。
-其中达摩院TTS标准数据格式,wav文件夹下存放了音频文件,prosody文件夹下的.txt文件对应的是音频文件的文本标注,interval文件夹下存放的是音素级别的时间戳标注,如下:
-```
-.
-├── interval
-│ ├── 500001.interval
-│ ├── 500002.interval
-│ ├── 500003.interval
-│ ├── ...
-│ └── 500020.interval
-├── prosody
-│ └── prosody.txt
-└── wav
- ├── 500001.wav
- ├── 500002.wav
- ├── ...
- └── 500020.wav
-```
-
-普通音频数据,不携带时间戳标注和prosody标注,如下:
-```
-.
-└── wav
- ├── 1.wav
- ├── 2.wav
- ├── ...
- └── 20.wav
-```
-
-**快速开始**:你可以从ModelScope下载经过阿里标准格式处理的[AISHELL-3开源语音合成数据集](https://modelscope.cn/datasets/speech_tts/AISHELL-3/summary),用来进行后续操作。如果你只有普通音频格式的数据,那么可以采用PTTS Autolabel自动化标注工具进行格式转换,下面介绍使用Notebook 进行PTTS Autolable自动化标注的方式。
-
-#### PTTS Autolable自动化标注
-
-打开右上角的Notebook,上传[sample_test_male](https://isv-data.oss-cn-hangzhou.aliyuncs.com/ics/MaaS/TTS/download_files/test_male.zip)的20条音频文件到目录如/mnt/workspace/Data/ptts_spk0_wav下面
-```
-ptts_spk0_wav
-├── 01_000001.wav
-├── 01_000002.wav
-├── 01_000003.wav
-├── 01_000004.wav
-├── 01_000005.wav
-├── 01_000006.wav
-├── 01_000007.wav
-├── 01_000008.wav
-├── 01_000009.wav
-├── 01_000010.wav
-├── 01_000011.wav
-├── 01_000012.wav
-├── 01_000013.wav
-├── 01_000014.wav
-├── 01_000015.wav
-├── 01_000016.wav
-├── 01_000017.wav
-├── 01_000018.wav
-├── 01_000019.wav
-└── 01_000020.wav
-```
-在Notebook的Jupyter中安装tts-autolabel
-
-``` Python
-# 运行此代码块安装tts-autolabel
-import sys
-!{sys.executable} -m pip install tts-autolabel -f https://modelscope.oss-cn-beijing.aliyuncs.com/releases/repo.html
-```
-``` Python
-# 导入run_auto_label工具, 初次运行会下载相关库文件
-from modelscope.tools import run_auto_label
-```
-
-``` Python
-# 运行 autolabel进行自动标注,20句音频的自动标注约4分钟
-import os
-
-input_wav = '/mnt/workspace/Data/ptts_spk0_wav' # wav audio path
-work_dir = '/mnt/workspace/Data/ptts_spk0_autolabel' # output path
-os.makedirs(work_dir, exist_ok=True)
-
-ret, report = run_auto_label(input_wav = input_wav,
- work_dir = work_dir,
- resource_revision='v1.0.4')
-print(report)
-```
-
-完成数据标注后,下载对应文件夹`/mnt/workspace/Data/ptts_spk0_autolabel`, 使用`KAN-TTS`的数据前处理脚本做训练前数据准备。
-
-我们选择个性化语音合成配置文件进行特征提取操作,这里我们以提供的16k采样率为例kantts/configs/audio_config_se_16k.yaml
-运行以下命令来进行特征提取,其中--speaker代表该数据集对应发音人的名称,用户可以随意命名。
-```bash
-# 特征提取
-python kantts/preprocess/data_process.py --voice_input_dir ptts_spk0_autolabel --voice_output_dir training_stage/test_male_ptts_feats --audio_config kantts/configs/audio_config_se_16k.yaml --speaker F7 --se_model speech_personal_sambert-hifigan_nsf_tts_zh-cn_pretrain_16k/basemodel_16k/speaker_embedding/se.*
-
-# 扩充epoch
-stage0=training_stage
-voice=test_male_ptts_feats
-
-cat $stage0/$voice/am_valid.lst >> $stage0/$voice/am_train.lst
-lines=0
-while [ $lines -lt 400 ]
-do
- shuf $stage0/$voice/am_train.lst >> $stage0/$voice/am_train.lst.tmp
- lines=$(wc -l < "$stage0/$voice/am_train.lst.tmp")
-done
-mv $stage0/$voice/am_train.lst.tmp $stage0/$voice/am_train.lst
-```
-
-个性化语音合成只需要20句目标说话人的语音,特征提取只需要运行一小段时间,提取完毕后你会在training_stage/test_male_ptts_feats目录下得到如下结构的文件:
-```
-# 基于阿里标准格式数据所提取出的特征目录
-├── am_train.lst
-├── am_valid.lst
-├── audio_config.yaml
-├── badlist.txt
-├── data_process_stdout.log
-├── duration
-├── energy
-├── f0
-├── frame_energy
-├── frame_f0
-├── frame_uv
-├── mel
-├── raw_duration
-├── raw_metafile.txt
-├── Script.xml
-├── se
-├── train.lst
-├── valid.lst
-└── wav
-```
-至此数据准备工作就算完成了。
-
-微调声学模型
-KAN-TTS的训练脚本是配置驱动的,我们使用预训练模型中的speech_personal_sambert-hifigan_nsf_tts_zh-cn_pretrain_16k/basemodel_16k/sambert/config.yaml作为训练配置文件。
-我们提供默认的PTTS 默认使用的finetune参数值,希望在basemodel的基础上继续微调一定步数并保存,即train_max_steps配置项。
-
-```bash
-# 将train_max_steps改为 2400301
-...
-train_max_steps: 2400301
-...
-```
-完成上述必要的配置项修改后,我们就可以使用以下命令训练声学模型了:
-```bash
-# 训练声学模型
-CUDA_VISIBLE_DEVICES=0 python kantts/bin/train_sambert.py --model_config speech_personal_sambert-hifigan_nsf_tts_zh-cn_pretrain_16k/basemodel_16k/sambert/config.yaml --root_dir training_stage/test_male_ptts_feats --stage_dir training_stage/test_male_ptts_sambert_ckpt --resume_path speech_personal_sambert-hifigan_nsf_tts_zh-cn_pretrain_16k/basemodel_16k/sambert/ckpt/checkpoint_*.pth
-```
-
-体验微调模型效果
-在声学模型微调完毕后,我们就可以使用产出的模型文件和预训练的声码器来合成语音了,在此之前需要做一些准备工作。
-
-将我们想要合成的文本写入一个文件test.txt,每句话按行分隔,如下所示
-```
-徐玠诡谲多智,善揣摩,知道徐知询不可辅佐,掌握着他的短处以归附徐知诰。
-许乐夫生于山东省临朐县杨善镇大辛庄,毕业于抗大一分校。
-宣统元年(1909年),顺德绅士冯国材在香山大黄圃成立安洲农务分会,管辖东海十六沙,冯国材任总理。
-学生们大多住在校区宿舍,通过参加不同的体育文化俱乐部及社交活动,形成一个友谊长存的社会圈。
-学校的“三节一会”(艺术节、社团节、科技节、运动会)是显示青春才华的盛大活动。
-雪是先天自闭症患者,不懂与人沟通,却拥有灵敏听觉,而且对复杂动作过目不忘。
-勋章通过一柱状螺孔和螺钉附着在衣物上。
-雅恩雷根斯堡足球俱乐部()是一家位于德国雷根斯堡的足球俱乐部,处于德国足球丙级联赛。
-亚历山大·格罗滕迪克于1957年证明了一个深远的推广,现在叫做格罗滕迪克–黎曼–罗赫定理。
-```
-
-运行以下命令进行合成,其中se_file为特征提取环节抽取的speaker embedding,voc_ckpt为basemodel_16k中的预训练模型:
-
-``` bash
-# 运行合成语音
-CUDA_VISIBLE_DEVICES=0 python kantts/bin/text_to_wav.py --txt test.txt --output_dir res/test_male_ptts_syn --res_zip speech_sambert-hifigan_tts_zh-cn_multisp_pretrain_16k/resource.zip --am_ckpt training_stage/test_male_ptts_sambert_ckpt/ckpt/checkpoint_2400300.pth --voc_ckpt speech_sambert-hifigan_tts_zh-cn_multisp_pretrain_16k/hifigan/ckpt/checkpoint_2400000.pth --se_file training_stage/test_male_ptts_feats/se/se.npy
-```
-
-完成后在res/test_male_ptts_syn/res_wavs文件夹下就可以获得合成结果
-```
-.
-├── 0.wav
-├── 1.wav
-├── 2.wav
-├── 3.wav
-├── 4.wav
-├── 5.wav
-├── 6.wav
-├── 7.wav
-└── 8.wav
-```
-
-合成样音
-
-
-
-
-
-原始录音
-
-
-
-
-
-## 模型局限性以及可能的偏差
-* 该发音人支持中文及英文混合,TN规则为中文
-
-## 训练数据介绍
-使用约4000个不同发音人,共计约1000小时数据训练, 主要为中文语料, 包含少量英文语料。
-
-## 模型训练流程
-模型所需训练数据格式为:音频(.wav), 文本标注(.txt), 音素时长标注(.interval), 个性化语音合成模型微调训练时间需要2~5分钟。
-
-### 预处理
-模型训练需对音频文件提取声学特征(梅尔频谱);音素时长根据配置项中的帧长将时间单位转换成帧数;文本标注,根据配置项中的音素集、音调分类、边界分类转换成对应的one-hot编号;
-
-
-## 引用
-如果你觉得这个该模型对有所帮助,请考虑引用下面的相关的论文:
-
-```BibTeX
-@inproceedings{li2020robutrans,
- title={Robutrans: A robust transformer-based text-to-speech model},
- author={Li, Naihan and Liu, Yanqing and Wu, Yu and Liu, Shujie and Zhao, Sheng and Liu, Ming},
- booktitle={Proceedings of the AAAI Conference on Artificial Intelligence},
- volume={34},
- number={05},
- pages={8228--8235},
- year={2020}
-}
-```
-
-```BibTeX
-@article{devlin2018bert,
- title={Bert: Pre-training of deep bidirectional transformers for language understanding},
- author={Devlin, Jacob and Chang, Ming-Wei and Lee, Kenton and Toutanova, Kristina},
- journal={arXiv preprint arXiv:1810.04805},
- year={2018}
-}
-```
-```BibTeX
-@article{kong2020hifi,
- title={Hifi-gan: Generative adversarial networks for efficient and high fidelity speech synthesis},
- author={Kong, Jungil and Kim, Jaehyeon and Bae, Jaekyoung},
- journal={Advances in Neural Information Processing Systems},
- volume={33},
- pages={17022--17033},
- year={2020}
-}
-```
-
-本模型参考了以下实现
-- [1] [ming024's FastSpeech2 Implementation](https://github.com/ming024/FastSpeech2)
-- [2] [jik876/hifi-gan](https://github.com/jik876/hifi-gan)
-- [3] [kan-bayashi/ParallelWaveGAN](https://github.com/kan-bayashi/ParallelWaveGAN)
-- [4] [mozilla/TTS](https://github.com/mozilla/TTS)
-- [5] [espnet/espnet](https://github.com/espnet/espnet)
-
-
-
diff --git a/spaces/Laurie/IDEA-CCNL-Ziya-LLaMA-13B-v1/app.py b/spaces/Laurie/IDEA-CCNL-Ziya-LLaMA-13B-v1/app.py
deleted file mode 100644
index 591daf871d3b52023add1ca487c501a2036493c3..0000000000000000000000000000000000000000
--- a/spaces/Laurie/IDEA-CCNL-Ziya-LLaMA-13B-v1/app.py
+++ /dev/null
@@ -1,3 +0,0 @@
-import gradio as gr
-
-gr.Interface.load("models/IDEA-CCNL/Ziya-LLaMA-13B-v1").launch()
\ No newline at end of file
diff --git a/spaces/LightChen2333/OpenSLU/static/css/style.css b/spaces/LightChen2333/OpenSLU/static/css/style.css
deleted file mode 100644
index 4298d946ab63c672517d16cde93535f0553f520b..0000000000000000000000000000000000000000
--- a/spaces/LightChen2333/OpenSLU/static/css/style.css
+++ /dev/null
@@ -1,98 +0,0 @@
-.card {
- --phoenix-card-spacer-y: 1.5rem;
- --phoenix-card-spacer-x: 1.5rem;
- --phoenix-card-title-spacer-y: 1rem;
- --phoenix-card-border-width: 1px;
- --phoenix-card-border-color: var(--phoenix-gray-200);
- --phoenix-card-border-radius: 0.5rem;
- --phoenix-card-box-shadow: ;
- --phoenix-card-inner-border-radius: calc(0.5rem - 1px);
- --phoenix-card-cap-padding-y: 1.5rem;
- --phoenix-card-cap-padding-x: 1.5rem;
- --phoenix-card-cap-bg: var(--phoenix-card-cap-bg);
- --phoenix-card-cap-color: ;
- --phoenix-card-height: ;
- --phoenix-card-color: ;
- --phoenix-card-bg: #fff;
- --phoenix-card-img-overlay-padding: 1rem;
- --phoenix-card-group-margin: 1rem;
- position: relative;
- display: -webkit-box;
- display: -ms-flexbox;
- display: flex;
- -webkit-box-orient: vertical;
- -webkit-box-direction: normal;
- -ms-flex-direction: column;
- flex-direction: column;
- min-width: 0;
- height: var(--phoenix-card-height);
- word-wrap: break-word;
- background-color: var(--phoenix-card-bg);
- background-clip: border-box;
- border: var(--phoenix-card-border-width) solid var(--phoenix-card-border-color);
- border-radius: var(--phoenix-card-border-radius);
- -webkit-box-shadow: var(--phoenix-card-box-shadow);
- box-shadow: var(--phoenix-card-box-shadow);
-}
-.h-100 {
- height: 100% !important;
-}
-.card-body {
- -webkit-box-flex: 1;
- -ms-flex: 1 1 auto;
- flex: 1 1 auto;
- padding: var(--phoenix-card-spacer-y) var(--phoenix-card-spacer-x);
- color: var(--phoenix-card-color);
-}
-
-.justify-content-between {
- -webkit-box-pack: justify !important;
- -ms-flex-pack: justify !important;
- justify-content: space-between !important;
-}
-.d-flex {
- display: -webkit-box !important;
- display: -ms-flexbox !important;
- display: flex !important;
-}
-.pt-3 {
- padding-top: 1rem !important;
-}
-.mb-2 {
- margin-bottom: 0.5rem !important;
-}
-.align-items-center {
- -webkit-box-align: center !important;
- -ms-flex-align: center !important;
- align-items: center !important;
-}
-.bullet-item {
- height: 0.5rem;
- width: 1rem;
- border-radius: 2px;
-}
-.bg-primary {
- --phoenix-bg-opacity: 1;
- background-color: rgba(var(--phoenix-primary-rgb), var(--phoenix-bg-opacity)) !important;
-}
-.me-2 {
- margin-right: 0.5rem !important;
-}
-.flex-1 {
- -webkit-box-flex: 1;
- -ms-flex: 1;
- flex: 1;
-}
-.text-900 {
- --phoenix-text-opacity: 1;
- color: rgba(var(--phoenix-900-rgb), var(--phoenix-text-opacity)) !important;
-}
-.fw-semi-bold {
- font-weight: 600 !important;
-}
-.mb-0 {
- margin-bottom: 0 !important;
-}
-h6, .h6 {
- font-size: 0.8rem;
-}
\ No newline at end of file
diff --git a/spaces/LuxOAI/HUXTT/app.py b/spaces/LuxOAI/HUXTT/app.py
deleted file mode 100644
index c732bd25882a6f850f97373435eb9fbfaa698bd1..0000000000000000000000000000000000000000
--- a/spaces/LuxOAI/HUXTT/app.py
+++ /dev/null
@@ -1,52 +0,0 @@
-import openai
-import gradio as gr
-import json
-
-openai.api_key = "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"
-
-def save_conversation():
- with open('conversation.json', 'w') as f:
- json.dump(messages, f)
-
-def load_conversation():
- try:
- with open('conversation.json', 'r') as f:
- return json.load(f)
- except FileNotFoundError:
- return []
-
-messages = load_conversation()
-
-if not messages:
- messages.append({"role": "system", "content": "You are a knowledgeable assistant specialized in recruiting and hiring, and familiar with ADP Workforce Now Recruitment and various hiring and CRM tools."})
-
-def CustomChatGPT(user_input):
- messages.append({"role": "user", "content": user_input})
-
- # Ensure the conversation fits within the model's maximum token limit
- conversation = messages[-4096:]
-
- try:
- response = openai.ChatCompletion.create(
- model="gpt-3.5-turbo",
- messages=conversation,
- max_tokens=1000,
- temperature=0.7)
- except openai.api_resources.request_error.RequestError as e:
- print(f"Received error from OpenAI: {e}")
- return "I'm sorry, but I'm unable to generate a response at this time."
-
- ChatGPT_reply = response["choices"][0]["message"]["content"]
- messages.append({"role": "assistant", "content": ChatGPT_reply})
-
- save_conversation()
-
- return ChatGPT_reply
-
-interface = gr.Interface(fn=CustomChatGPT,
- inputs="textbox",
- outputs="textbox",
- title="HR HELPER",
- description="Chat with a specialized assistant that can answer questions about recruiting, hiring, and various HR and CRM tools. Developed by A. Leschik.")
-
-interface.launch()
diff --git a/spaces/LuxOAI/ResumeBud/README.md b/spaces/LuxOAI/ResumeBud/README.md
deleted file mode 100644
index 8d29af5a837ec491622bed243b65c4269dca3c48..0000000000000000000000000000000000000000
--- a/spaces/LuxOAI/ResumeBud/README.md
+++ /dev/null
@@ -1,13 +0,0 @@
----
-title: ResumeBud
-emoji: 🐠
-colorFrom: red
-colorTo: green
-sdk: gradio
-sdk_version: 3.32.0
-app_file: app.py
-pinned: false
-license: openrail
----
-
-Check out the configuration reference at https://huggingface.co/docs/hub/spaces-config-reference
diff --git a/spaces/Lycorisdeve/DeepDanbooru_string/README.md b/spaces/Lycorisdeve/DeepDanbooru_string/README.md
deleted file mode 100644
index 4330b6f969246dc764a34ea254d2e807159f1c55..0000000000000000000000000000000000000000
--- a/spaces/Lycorisdeve/DeepDanbooru_string/README.md
+++ /dev/null
@@ -1,39 +0,0 @@
----
-title: DeepDanbooru String
-emoji: 💬
-colorFrom: blue
-colorTo: red
-sdk: gradio
-sdk_version: 3.6
-app_file: app.py
-pinned: false
-duplicated_from: NoCrypt/DeepDanbooru_string
----
-
-# Configuration
-
-`title`: _string_
-Display title for the Space
-
-`emoji`: _string_
-Space emoji (emoji-only character allowed)
-
-`colorFrom`: _string_
-Color for Thumbnail gradient (red, yellow, green, blue, indigo, purple, pink, gray)
-
-`colorTo`: _string_
-Color for Thumbnail gradient (red, yellow, green, blue, indigo, purple, pink, gray)
-
-`sdk`: _string_
-Can be either `gradio`, `streamlit`, or `static`
-
-`sdk_version` : _string_
-Only applicable for `streamlit` SDK.
-See [doc](https://hf.co/docs/hub/spaces) for more info on supported versions.
-
-`app_file`: _string_
-Path to your main application file (which contains either `gradio` or `streamlit` Python code, or `static` html code).
-Path is relative to the root of the repository.
-
-`pinned`: _boolean_
-Whether the Space stays on top of your list.
diff --git a/spaces/Make-A-Protagonist/Make-A-Protagonist-inference/Make-A-Protagonist/experts/XMem/inference/interact/s2m/__init__.py b/spaces/Make-A-Protagonist/Make-A-Protagonist-inference/Make-A-Protagonist/experts/XMem/inference/interact/s2m/__init__.py
deleted file mode 100644
index e69de29bb2d1d6434b8b29ae775ad8c2e48c5391..0000000000000000000000000000000000000000
diff --git a/spaces/Makiing/coolb-in-gtest/tests/kblob.ts b/spaces/Makiing/coolb-in-gtest/tests/kblob.ts
deleted file mode 100644
index 9e15b41c1c94a690beb61b23cdb42fc78767ccd2..0000000000000000000000000000000000000000
--- a/spaces/Makiing/coolb-in-gtest/tests/kblob.ts
+++ /dev/null
@@ -1,27 +0,0 @@
-import FormData from 'form-data'
-
-import { fetch } from '@/lib/isomorphic'
-
-const formData = new FormData()
-
-const knowledgeRequest = {"imageInfo":{"url":"https://www.baidu.com/img/PCfb_5bf082d29588c07f842ccde3f97243ea.png"},"knowledgeRequest":{"invokedSkills":["ImageById"],"subscriptionId":"Bing.Chat.Multimodal","invokedSkillsRequestData":{"enableFaceBlur":true},"convoData":{"convoid":"51D|BingProdUnAuthenticatedUsers|E3DCA904FF236C67C3450163BCEC64CFF3F618CC8A4AFD75FD518F5ED0ADA080","convotone":"Creative"}}}
-
-formData.append('knowledgeRequest', JSON.stringify(knowledgeRequest))
-
-
-fetch('https://bing.vcanbb.top/images/kblob',
- {
- method: 'POST',
- body: formData.getBuffer(),
- headers: {
- "sec-ch-ua": "\"Not/A)Brand\";v=\"99\", \"Google Chrome\";v=\"115\", \"Chromium\";v=\"115\"",
- "sec-ch-ua-mobile": "?0",
- "sec-ch-ua-platform": "\"Windows\"",
- "Referer": "https://bing.vcanbb.top/web/index.html",
- "Referrer-Policy": "origin-when-cross-origin",
- ...formData.getHeaders()
- }
-
- }
-).then(res => res.text())
-.then(res => console.log('res', res))
diff --git a/spaces/Malmika/Osana-WEB-GPT/app_update02.py b/spaces/Malmika/Osana-WEB-GPT/app_update02.py
deleted file mode 100644
index 0be90bba24313876717017d4294e67d6746b1419..0000000000000000000000000000000000000000
--- a/spaces/Malmika/Osana-WEB-GPT/app_update02.py
+++ /dev/null
@@ -1,134 +0,0 @@
-import json
-import requests
-import wikipedia
-import gradio as gr
-import transformers
-import spacy
-from bs4 import BeautifulSoup
-from transformers import AutoTokenizer, AutoModelForSeq2SeqLM
-
-
-# Set up Google SERP API credentials
-serp_api_key = '5924c6cfe5fec240e39838ff06439c8d36d294a0' # Replace with your actual Google SERP API key
-
- # Load the Pegasus model
-tokenizer = AutoTokenizer.from_pretrained("facebook/bart-large-cnn")
-model = AutoModelForSeq2SeqLM.from_pretrained("facebook/bart-large-cnn")
-
-# Function to send a message and receive a response from the chatbot
-def chat(message):
- try:
- # You can add your chatbot implementation here
- return "."
- except Exception as e:
- print("An error occurred:", e)
- return ""
-
-# Function to get the latest answers from Google SERP API
-def get_latest_answers(query):
- url = "https://google.serper.dev/search"
-
- payload = json.dumps({
- "q": query
- })
- headers = {
- 'X-API-KEY': serp_api_key,
- 'Content-Type': 'application/json'
- }
-
- response = requests.request("POST", url, headers=headers, data=payload)
-
- try:
- # Parse the response JSON
- data = json.loads(response.text)
-
- # Extract details from the response
- output = ""
-
- if 'knowledgeGraph' in data:
- knowledge_graph = data['knowledgeGraph']
- output += "Website: {}\n".format(knowledge_graph.get('website'))
- output += "Description: {}\n".format(knowledge_graph.get('description'))
-
- if 'organic' in data:
- organic_results = data['organic']
- for result in organic_results:
- output += "Snippet: {}\n".format(result.get('snippet'))
-
- if 'peopleAlsoAsk' in data:
- people_also_ask = data['peopleAlsoAsk']
- for question in people_also_ask:
- output += "Snippet: {}\n".format(question.get('snippet'))
-
- return output
-
- except json.JSONDecodeError:
- print(".")
- return ""
-
- except Exception as e:
- print(".")
- return ""
-
-# Function to search Wikipedia for an answer and summarize it
-def search_wikipedia(query):
- try:
- search_results = wikipedia.search(query)
-
- # Get the page summary of the first search result
- if search_results:
- page_title = search_results[0]
- page_summary = wikipedia.summary(page_title)
- return page_summary
- else:
- print(".")
- return None
- except wikipedia.exceptions.DisambiguationError as e:
- # Handle disambiguation error
- print(".")
- return None
- except wikipedia.exceptions.PageError as e:
- # Handle page not found error
- print(".")
- return None
- except Exception as e:
- # Handle other exceptions
- print(".")
- return None
-
-# Function to generate summarized paragraph using Google Pegasus summarization
-def generate_summary(user_input):
- output = get_latest_answers(user_input)
- page_summary = search_wikipedia(user_input)
- chat_answer = chat(user_input)
-
- # Combine the input text from various sources
- input_text = f"Google:\n{output}\nWikipedia :\n{page_summary}\n"
-
-
- # Tokenize and generate a summary
- input_ids = tokenizer.encode(input_text, return_tensors="pt", max_length=1024, truncation=True)
- summary_ids = model.generate(input_ids, max_length=200, min_length=50, length_penalty=2.0, num_beams=4, early_stopping=True)
-
- # Decode the summary
- summarized_paragraph = tokenizer.decode(summary_ids[0], skip_special_tokens=True)
-
- return summarized_paragraph
-
-# Define the Gradio interface
-def summarizer_interface(user_input):
- summarized_text = generate_summary(user_input)
- return summarized_text
-
-iface = gr.Interface(
- fn=summarizer_interface,
- inputs="text",
- outputs="text",
- title="Osana Web-GPT",
- description="Enter your query and get the latest and better answer.",
- theme="black",
- layout="horizontal",
-)
-
-# Launch the interface
-iface.launch()
diff --git a/spaces/Marshalls/testmtd/inference/generate.py b/spaces/Marshalls/testmtd/inference/generate.py
deleted file mode 100644
index a62f636319308743649390d8415e2b2de4a788f4..0000000000000000000000000000000000000000
--- a/spaces/Marshalls/testmtd/inference/generate.py
+++ /dev/null
@@ -1,172 +0,0 @@
-import sys
-import os
-THIS_DIR = os.path.dirname(os.path.abspath(__file__))
-ROOT_DIR = os.path.abspath(os.path.join(THIS_DIR, os.pardir))#os.pardir--get parent directory
-sys.path.append(ROOT_DIR)
-import numpy as np; import scipy.linalg
-# LUL
-w_shape = [219,219]
-w_init = np.linalg.qr(np.random.randn(*w_shape))[0].astype(np.float32)
-#np.random.randn(size)--Generate the matrix according to size
-#np.linalg.qr--caculate matrix QR decomposition
-np_p, np_l, np_u = scipy.linalg.lu(w_init)
-
-from training.datasets import create_dataset, create_dataloader
-
-from models import create_model
-from training.options.train_options import TrainOptions
-import torch
-import pytorch_lightning as pl
-import numpy as np
-import pickle, json, yaml
-import sklearn
-import argparse
-import os, glob
-from pathlib import Path
-
-from analysis.visualization.generate_video_from_mats import generate_video_from_mats
-from analysis.visualization.generate_video_from_expmaps import generate_video_from_expmaps
-from analysis.visualization.generate_video_from_moglow_pos import generate_video_from_moglow_loc
-
-from training.utils import get_latest_checkpoint
-
-if __name__ == '__main__':
- print("Hi")
- parser = argparse.ArgumentParser(description='Generate with model')
- parser.add_argument('--data_dir', type=str)
- parser.add_argument('--seeds', type=str, help='in the format: mod,seq_id;mod,seq_id')
- parser.add_argument('--seeds_file', type=str, help='file from which to choose a random seed')
- parser.add_argument('--output_folder', type=str)
- parser.add_argument('--audio_format', type=str, default="wav")
- parser.add_argument('--experiment_name', type=str)
- parser.add_argument('--seq_id', type=str)
- parser.add_argument('--max_length', type=int, default=-1)
- parser.add_argument('--no-use_scalers', dest='use_scalers', action='store_false')#action='store_false'--set the Bool Value 0
- parser.add_argument('--generate_video', action='store_true')#action='store_true'--set the Bool Value 1--choose to generate video
- parser.add_argument('--generate_bvh', action='store_true')
- parser.add_argument('--generate_ground_truth', action='store_true')
- parser.add_argument('--fps', type=int, default=20)
- parser.add_argument('--seed',type=str,help='nothing')
- args = parser.parse_args()
- data_dir = args.data_dir
- audio_format = args.audio_format
- fps = args.fps
- output_folder = args.output_folder
- print("##############################################################################")
- print(output_folder)
- print("##############################################################################")
- seq_id = args.seq_id
- seed=args.seed
- if args.seeds is not None:
- seeds = {mod:seq for mod,seq in [tuple(x.split(",")) for x in args.seeds.split(";")]}
- else:
- seeds = {}
-
- if seq_id is None:
- temp_base_filenames = [x[:-1] for x in open(data_dir + "/base_filenames_test.txt", "r").readlines()]#find the name of the song in the txt created before
- seq_id = np.random.choice(temp_base_filenames)
- if args.seeds_file is not None:
- print("choosing random seed from "+args.seeds_file)
- temp_base_filenames = [x[:-1] for x in open(args.seeds_file, "r").readlines()]
- seq_id = np.random.choice(temp_base_filenames)
-
-
- print('seq_id: ',seq_id)
-
- #load hparams file
- default_save_path = "training/experiments/"+args.experiment_name
- logs_path = default_save_path
- latest_checkpoint = get_latest_checkpoint(logs_path)
- print(latest_checkpoint)
- checkpoint_dir = Path(latest_checkpoint).parent.parent.absolute()
- # exp_opt = json.loads(open("training/experiments/"+args.experiment_name+"/opt.json","r").read())
- exp_opt = yaml.safe_load(open(str(checkpoint_dir)+"/hparams.yaml","r").read())#yaml is a file saving the parameters(type=dictionery)
- opt = vars(TrainOptions().parse(parse_args=["--model", exp_opt["model"]]))
- #print(opt)
- opt.update(exp_opt)
- # opt["cond_concat_dims"] = True
- # opt["bn_momentum"] = 0.0
- opt["batch_size"] = 1
- opt["phase"] = "inference"
- opt["tpu_cores"] = 0
- class Struct:
- def __init__(self, **entries):
- self.__dict__.update(entries)
- #print(opt)
- opt = Struct(**opt)
-
- input_mods = opt.input_modalities.split(",")
- output_mods = opt.output_modalities.split(",")
- output_time_offsets = [int(x) for x in str(opt.output_time_offsets).split(",")]
- if args.use_scalers:
- scalers = [x+"_scaler.pkl" for x in output_mods]
- else:
- scalers = []
-
- # Load latest trained checkpoint from experiment
- model = create_model(opt)
- model = model.load_from_checkpoint(latest_checkpoint, opt=opt)
-
- # Load input features (sequences must have been processed previously into features)
- features = {}
- for i,mod in enumerate(input_mods):
- if mod in seeds:
- feature = np.load(data_dir+"/"+seeds[mod]+"."+mod+".npy")
- elif (seed is not None and i==0):
- #feature = np.load(data_dir+"/"+seq_id+"."+mod+".npy")
- feature=np.load(seed+'.npy')
- else:
- feature=np.load(data_dir+'/'+seq_id+'.'+mod+'.npy')
- if args.max_length != -1:
- feature = feature[:args.max_length]
- if model.input_fix_length_types[i] == "single":
- features["in_"+mod] = np.expand_dims(np.expand_dims(feature,1),1)
- else:
- features["in_"+mod] = np.expand_dims(feature,1)
-
- # Generate prediction
- if torch.cuda.is_available():
- model.cuda()
- #import pdb;pdb.set_trace()
- #import time
- #start_time = time.time()
- predicted_mods = model.generate(features, ground_truth=args.generate_ground_truth)
- #print("--- %s seconds ---" % (time.time() - start_time))
- if len(predicted_mods) == 0:
- print("Sequence too short!")
- else:
- # import pdb;pdb.set_trace()
- for i, mod in enumerate(output_mods):
- predicted_mod = predicted_mods[i].cpu().numpy()
- if len(scalers)>0:
- transform = pickle.load(open(data_dir+"/"+scalers[i], "rb"))
- predicted_mod = transform.inverse_transform(predicted_mod)
- #print(predicted_mod)
- predicted_features_file = output_folder+"/"+args.experiment_name+"/predicted_mods/"+seq_id+"."+mod+".generated"
- np.save(predicted_features_file,predicted_mod)
- predicted_features_file += ".npy"
- if args.generate_video:
- trim_audio = output_time_offsets[i] / fps #converting trim_audio from being in frames (which is more convenient as thats how we specify the output_shift in the models), to seconds
- #print("trim_audio: ",trim_audio)
-
- audio_file = data_dir + "/" + seq_id + "."+audio_format
-
- output_folder = output_folder+"/"+args.experiment_name+"/videos/"
- if mod == "joint_angles_scaled":
- generate_video_from_mats(predicted_features_file,output_folder,audio_file,trim_audio,fps,plot_mats)
- elif mod == "expmap_scaled" or mod == "expmap_scaled_20" or mod == "expmap_cr_scaled_20":
- pipeline_file = f'{data_dir}/motion_{mod}_data_pipe.sav'
- generate_video_from_expmaps(predicted_features_file,pipeline_file,output_folder,audio_file,trim_audio,args.generate_bvh)
- elif mod == "position_scaled":
- control_file = f'{data_dir}/{seq_id}.moglow_control_scaled.npy'
- data = np.load(predicted_features_file)[:,0,:]
- control = np.load(control_file)
- if args.use_scalers:
- transform = pickle.load(open(data_dir+"/moglow_control_scaled_scaler.pkl", "rb"))
- control = transform.inverse_transform(control)
- control = control[int(opt.output_time_offsets.split(",")[0]):]
- generate_video_from_moglow_loc(data,control,output_folder,seq_id,audio_file,fps,trim_audio)
- else:
- print("Warning: mod "+mod+" not supported")
- # raise NotImplementedError(f'Feature type {feature_type} not implemented')
- pass
diff --git a/spaces/Mellow-ai/PhotoAI_Mellow/annotator/uniformer/configs/_base_/models/deeplabv3plus_r50-d8.py b/spaces/Mellow-ai/PhotoAI_Mellow/annotator/uniformer/configs/_base_/models/deeplabv3plus_r50-d8.py
deleted file mode 100644
index 050e39e091d816df9028d23aa3ecf9db74e441e1..0000000000000000000000000000000000000000
--- a/spaces/Mellow-ai/PhotoAI_Mellow/annotator/uniformer/configs/_base_/models/deeplabv3plus_r50-d8.py
+++ /dev/null
@@ -1,46 +0,0 @@
-# model settings
-norm_cfg = dict(type='SyncBN', requires_grad=True)
-model = dict(
- type='EncoderDecoder',
- pretrained='open-mmlab://resnet50_v1c',
- backbone=dict(
- type='ResNetV1c',
- depth=50,
- num_stages=4,
- out_indices=(0, 1, 2, 3),
- dilations=(1, 1, 2, 4),
- strides=(1, 2, 1, 1),
- norm_cfg=norm_cfg,
- norm_eval=False,
- style='pytorch',
- contract_dilation=True),
- decode_head=dict(
- type='DepthwiseSeparableASPPHead',
- in_channels=2048,
- in_index=3,
- channels=512,
- dilations=(1, 12, 24, 36),
- c1_in_channels=256,
- c1_channels=48,
- dropout_ratio=0.1,
- num_classes=19,
- norm_cfg=norm_cfg,
- align_corners=False,
- loss_decode=dict(
- type='CrossEntropyLoss', use_sigmoid=False, loss_weight=1.0)),
- auxiliary_head=dict(
- type='FCNHead',
- in_channels=1024,
- in_index=2,
- channels=256,
- num_convs=1,
- concat_input=False,
- dropout_ratio=0.1,
- num_classes=19,
- norm_cfg=norm_cfg,
- align_corners=False,
- loss_decode=dict(
- type='CrossEntropyLoss', use_sigmoid=False, loss_weight=0.4)),
- # model training and testing settings
- train_cfg=dict(),
- test_cfg=dict(mode='whole'))
diff --git a/spaces/MisterZee/PIFu-Clothed-Human-Digitization/PIFu/lib/model/ConvPIFuNet.py b/spaces/MisterZee/PIFu-Clothed-Human-Digitization/PIFu/lib/model/ConvPIFuNet.py
deleted file mode 100644
index 1d43d262aa237d03db0cf329b4d199061ee6a006..0000000000000000000000000000000000000000
--- a/spaces/MisterZee/PIFu-Clothed-Human-Digitization/PIFu/lib/model/ConvPIFuNet.py
+++ /dev/null
@@ -1,99 +0,0 @@
-import torch
-import torch.nn as nn
-import torch.nn.functional as F
-from .BasePIFuNet import BasePIFuNet
-from .SurfaceClassifier import SurfaceClassifier
-from .DepthNormalizer import DepthNormalizer
-from .ConvFilters import *
-from ..net_util import init_net
-
-class ConvPIFuNet(BasePIFuNet):
- '''
- Conv Piximp network is the standard 3-phase network that we will use.
- The image filter is a pure multi-layer convolutional network,
- while during feature extraction phase all features in the pyramid at the projected location
- will be aggregated.
- It does the following:
- 1. Compute image feature pyramids and store it in self.im_feat_list
- 2. Calculate calibration and indexing on each of the feat, and append them together
- 3. Classification.
- '''
-
- def __init__(self,
- opt,
- projection_mode='orthogonal',
- error_term=nn.MSELoss(),
- ):
- super(ConvPIFuNet, self).__init__(
- projection_mode=projection_mode,
- error_term=error_term)
-
- self.name = 'convpifu'
-
- self.opt = opt
- self.num_views = self.opt.num_views
-
- self.image_filter = self.define_imagefilter(opt)
-
- self.surface_classifier = SurfaceClassifier(
- filter_channels=self.opt.mlp_dim,
- num_views=self.opt.num_views,
- no_residual=self.opt.no_residual,
- last_op=nn.Sigmoid())
-
- self.normalizer = DepthNormalizer(opt)
-
- # This is a list of [B x Feat_i x H x W] features
- self.im_feat_list = []
-
- init_net(self)
-
- def define_imagefilter(self, opt):
- net = None
- if opt.netIMF == 'multiconv':
- net = MultiConv(opt.enc_dim)
- elif 'resnet' in opt.netIMF:
- net = ResNet(model=opt.netIMF)
- elif opt.netIMF == 'vgg16':
- net = Vgg16()
- else:
- raise NotImplementedError('model name [%s] is not recognized' % opt.imf_type)
-
- return net
-
- def filter(self, images):
- '''
- Filter the input images
- store all intermediate features.
- :param images: [B, C, H, W] input images
- '''
- self.im_feat_list = self.image_filter(images)
-
- def query(self, points, calibs, transforms=None, labels=None):
- '''
- Given 3D points, query the network predictions for each point.
- Image features should be pre-computed before this call.
- store all intermediate features.
- query() function may behave differently during training/testing.
- :param points: [B, 3, N] world space coordinates of points
- :param calibs: [B, 3, 4] calibration matrices for each image
- :param transforms: Optional [B, 2, 3] image space coordinate transforms
- :param labels: Optional [B, Res, N] gt labeling
- :return: [B, Res, N] predictions for each point
- '''
- if labels is not None:
- self.labels = labels
-
- xyz = self.projection(points, calibs, transforms)
- xy = xyz[:, :2, :]
- z = xyz[:, 2:3, :]
-
- z_feat = self.normalizer(z)
-
- # This is a list of [B, Feat_i, N] features
- point_local_feat_list = [self.index(im_feat, xy) for im_feat in self.im_feat_list]
- point_local_feat_list.append(z_feat)
- # [B, Feat_all, N]
- point_local_feat = torch.cat(point_local_feat_list, 1)
-
- self.preds = self.surface_classifier(point_local_feat)
diff --git a/spaces/MrBodean/VoiceClone/demo_toolbox.py b/spaces/MrBodean/VoiceClone/demo_toolbox.py
deleted file mode 100644
index ea30a29275965c7e2b815cd703e891a5ca53e97b..0000000000000000000000000000000000000000
--- a/spaces/MrBodean/VoiceClone/demo_toolbox.py
+++ /dev/null
@@ -1,43 +0,0 @@
-from pathlib import Path
-from toolbox import Toolbox
-from utils.argutils import print_args
-from utils.modelutils import check_model_paths
-import argparse
-import os
-
-
-if __name__ == '__main__':
- parser = argparse.ArgumentParser(
- description="Runs the toolbox",
- formatter_class=argparse.ArgumentDefaultsHelpFormatter
- )
-
- parser.add_argument("-d", "--datasets_root", type=Path, help= \
- "Path to the directory containing your datasets. See toolbox/__init__.py for a list of "
- "supported datasets.", default=None)
- parser.add_argument("-e", "--enc_models_dir", type=Path, default="encoder/saved_models",
- help="Directory containing saved encoder models")
- parser.add_argument("-s", "--syn_models_dir", type=Path, default="synthesizer/saved_models",
- help="Directory containing saved synthesizer models")
- parser.add_argument("-v", "--voc_models_dir", type=Path, default="vocoder/saved_models",
- help="Directory containing saved vocoder models")
- parser.add_argument("--cpu", action="store_true", help=\
- "If True, processing is done on CPU, even when a GPU is available.")
- parser.add_argument("--seed", type=int, default=None, help=\
- "Optional random number seed value to make toolbox deterministic.")
- parser.add_argument("--no_mp3_support", action="store_true", help=\
- "If True, no mp3 files are allowed.")
- args = parser.parse_args()
- print_args(args, parser)
-
- if args.cpu:
- # Hide GPUs from Pytorch to force CPU processing
- os.environ["CUDA_VISIBLE_DEVICES"] = "-1"
- del args.cpu
-
- ## Remind the user to download pretrained models if needed
- check_model_paths(encoder_path=args.enc_models_dir, synthesizer_path=args.syn_models_dir,
- vocoder_path=args.voc_models_dir)
-
- # Launch the toolbox
- Toolbox(**vars(args))
diff --git a/spaces/MrBodean/VoiceClone/encoder/config.py b/spaces/MrBodean/VoiceClone/encoder/config.py
deleted file mode 100644
index 1c21312f3de971bfa008254c6035cebc09f05e4c..0000000000000000000000000000000000000000
--- a/spaces/MrBodean/VoiceClone/encoder/config.py
+++ /dev/null
@@ -1,45 +0,0 @@
-librispeech_datasets = {
- "train": {
- "clean": ["LibriSpeech/train-clean-100", "LibriSpeech/train-clean-360"],
- "other": ["LibriSpeech/train-other-500"]
- },
- "test": {
- "clean": ["LibriSpeech/test-clean"],
- "other": ["LibriSpeech/test-other"]
- },
- "dev": {
- "clean": ["LibriSpeech/dev-clean"],
- "other": ["LibriSpeech/dev-other"]
- },
-}
-libritts_datasets = {
- "train": {
- "clean": ["LibriTTS/train-clean-100", "LibriTTS/train-clean-360"],
- "other": ["LibriTTS/train-other-500"]
- },
- "test": {
- "clean": ["LibriTTS/test-clean"],
- "other": ["LibriTTS/test-other"]
- },
- "dev": {
- "clean": ["LibriTTS/dev-clean"],
- "other": ["LibriTTS/dev-other"]
- },
-}
-voxceleb_datasets = {
- "voxceleb1" : {
- "train": ["VoxCeleb1/wav"],
- "test": ["VoxCeleb1/test_wav"]
- },
- "voxceleb2" : {
- "train": ["VoxCeleb2/dev/aac"],
- "test": ["VoxCeleb2/test_wav"]
- }
-}
-
-other_datasets = [
- "LJSpeech-1.1",
- "VCTK-Corpus/wav48",
-]
-
-anglophone_nationalites = ["australia", "canada", "ireland", "uk", "usa"]
diff --git a/spaces/NAACL2022/CLIP-Caption-Reward/scripts_FineCapEval/clipscore_prepro_feats.py b/spaces/NAACL2022/CLIP-Caption-Reward/scripts_FineCapEval/clipscore_prepro_feats.py
deleted file mode 100644
index 5e085078ecd67e4e390bc50b543c14d4934cb260..0000000000000000000000000000000000000000
--- a/spaces/NAACL2022/CLIP-Caption-Reward/scripts_FineCapEval/clipscore_prepro_feats.py
+++ /dev/null
@@ -1,154 +0,0 @@
-"""
-Preprocess a raw json dataset into features files for use in data_loader.py
-
-Input: json file that has the form
-[{ file_path: 'path/img.jpg', captions: ['a caption', ...] }, ...]
-example element in this list would look like
-{'captions': [u'A man with a red helmet on a small moped on a dirt road. ', u'Man riding a motor bike on a dirt road on the countryside.', u'A man riding on the back of a motorcycle.', u'A dirt path with a young person on a motor bike rests to the foreground of a verdant area with a bridge and a background of cloud-wreathed mountains. ', u'A man in a red shirt and a red hat is on a motorcycle on a hill side.'], 'file_path': u'val2014/COCO_val2014_000000391895.jpg', 'id': 391895}
-
-This script reads this json, does some basic preprocessing on the captions
-(e.g. lowercase, etc.), creates a special UNK token, and encodes everything to arrays
-
-Output: two folders of features
-"""
-
-from __future__ import absolute_import
-from __future__ import division
-from __future__ import print_function
-
-import os
-import json
-import argparse
-from random import shuffle, seed
-import string
-# non-standard dependencies:
-import h5py
-from six.moves import cPickle
-import numpy as np
-import torch
-import torchvision.models as models
-import skimage.io
-
-from torchvision.transforms import Compose, Resize, CenterCrop, ToTensor, Normalize
-from PIL import Image
-from torch import nn
-
-# preprocess = Compose([
-# Resize((448, 448), interpolation=Image.BICUBIC),
-# CenterCrop((448, 448)),
-# ToTensor()
-# ])
-
-
-# from clip.clip import load
-# from timm.models.vision_transformer import resize_pos_embed
-# import timm
-
-# from captioning.utils.resnet_utils import myResnet
-# import captioning.utils.resnet as resnet
-
-from captioning.utils.clipscore import CLIPScore
-
-from tqdm import tqdm
-
-
-def main(params):
-
- clipscore_model = CLIPScore()
- clipscore_model.to('cuda')
-
- imgs = json.load(open(params['input_json'], 'r'))
- imgs = imgs['images']
- N = len(imgs)
-
- seed(123) # make reproducible
-
- # dir_fc = params['output_dir']+'_clip_'+save_model_type+'_fc'
- # dir_att = params['output_dir']+'_clip_'+save_model_type+'_att'
-
- vis_dir_fc = params['output_dir']+'_clipscore_vis'
- if not os.path.isdir(vis_dir_fc):
- os.mkdir(vis_dir_fc)
-
- # text_dir_fc = params['output_dir']+'_clipscore_text'
- # if not os.path.isdir(text_dir_fc):
- # os.mkdir(text_dir_fc)
-
- # if not os.path.isdir(dir_att):
- # os.mkdir(dir_att)
-
- for i,img in enumerate(tqdm(imgs)):
- # load the image
-
- # img_path = os.path.join(params['images_root'], img['filepath'], img['filename'])
- # img_path = os.path.join(params['images_root'], img['file_name'])
- img_path = os.path.join(params['images_root'], img['file_path'])
-
- img_feat = clipscore_model.image_extract(img_path)
- img_feat = img_feat.view(512)
-
- # for d in img['sentences']:
- # text = d['raw'].strip()
- # text_feat = clipscore_model.text_extract(text)
-
-
- # with torch.no_grad():
-
- # image = preprocess(Image.open(os.path.join(params['images_root'], img['filepath'], img['filename']) ).convert("RGB"))
- # image = torch.tensor(np.stack([image])).cuda()
- # image -= mean
- # image /= std
- # if "RN" in params["model_type"]:
- # tmp_att, tmp_fc = model.encode_image(image)
- # tmp_att = tmp_att[0].permute(1, 2, 0)
- # tmp_fc = tmp_fc[0]
- # elif params["model_type"] == 'vit_base_patch32_224_in21k':
- # x = model(image)
- # tmp_fc = x[0, 0, :]
- # tmp_att = x[0, 1:, :].reshape( 14, 14, 768 )
- # else:
- # x = model.visual.conv1(image.half()) # shape = [*, width, grid, grid]
- # x = x.reshape(x.shape[0], x.shape[1], -1) # shape = [*, width, grid ** 2]
- # x = x.permute(0, 2, 1) # shape = [*, grid ** 2, width]
- # x = torch.cat([model.visual.class_embedding.to(x.dtype) + torch.zeros(x.shape[0], 1, x.shape[-1], dtype=x.dtype, device=x.device), x], dim=1) # shape = [*, grid ** 2 + 1, width]
- # x = x + model.visual.positional_embedding.to(x.dtype)[:x.shape[1], :]
- # x = model.visual.ln_pre(x)
-
- # x = x.permute(1, 0, 2) # NLD -> LND
-
- # for layer_idx, layer in enumerate(model.visual.transformer.resblocks):
- # x = layer(x)
-
- # x = x.permute(1, 0, 2)
- # tmp_fc = x[0, 0, :]
- # tmp_att = x[0, 1:, :].reshape( 14, 14, 768 )
-
- np.save(os.path.join(vis_dir_fc, str(img['id'])), img_feat.data.cpu().float().numpy())
- # np.save(os.path.join(text_dir_fc, str(img['cocoid'])), tmp_fc.data.cpu().float().numpy())
-
-
- # np.savez_compressed(os.path.join(dir_att, str(img['cocoid'])), feat=tmp_att.data.cpu().float().numpy())
-
- # if i % 1000 == 0:
- # print('processing %d/%d (%.2f%% done)' % (i, N, i*100.0/N))
- print('wrote ', vis_dir_fc)
-
-if __name__ == "__main__":
-
- parser = argparse.ArgumentParser()
-
- # input json
- # dataset_coco.json
- parser.add_argument('--input_json', required=True, help='input json file to process into hdf5')
- parser.add_argument('--output_dir', default='data', help='output h5 file')
-
- # options
- parser.add_argument('--images_root', default='', help='root location in which images are stored, to be prepended to file_path in input json')
- # parser.add_argument('--att_size', default=14, type=int, help='14x14 or 7x7')
- # parser.add_argument('--model_type', default='RN50', type=str, help='RN50, RN101, RN50x4, ViT-B/32, vit_base_patch32_224_in21k')
-
- args = parser.parse_args()
- params = vars(args) # convert to ordinary dict
- print('parsed input parameters:')
- print(json.dumps(params, indent = 2))
- main(params)
diff --git a/spaces/NCTCMumbai/NCTC/models/official/nlp/bert/tokenization.py b/spaces/NCTCMumbai/NCTC/models/official/nlp/bert/tokenization.py
deleted file mode 100644
index b0f7e27e320c727c4eee511fc63ebb63929250c7..0000000000000000000000000000000000000000
--- a/spaces/NCTCMumbai/NCTC/models/official/nlp/bert/tokenization.py
+++ /dev/null
@@ -1,545 +0,0 @@
-# coding=utf-8
-# Copyright 2019 The TensorFlow Authors. All Rights Reserved.
-#
-# Licensed under the Apache License, Version 2.0 (the "License");
-# you may not use this file except in compliance with the License.
-# You may obtain a copy of the License at
-#
-# http://www.apache.org/licenses/LICENSE-2.0
-#
-# Unless required by applicable law or agreed to in writing, software
-# distributed under the License is distributed on an "AS IS" BASIS,
-# WITHOUT WARRANTIES OR CONDITIONS OF ANY KIND, either express or implied.
-# See the License for the specific language governing permissions and
-# limitations under the License.
-# ==============================================================================
-"""Tokenization classes implementation.
-
-The file is forked from:
-https://github.com/google-research/bert/blob/master/tokenization.py.
-"""
-
-from __future__ import absolute_import
-from __future__ import division
-from __future__ import print_function
-
-import collections
-import re
-import unicodedata
-
-import six
-import tensorflow as tf
-
-import sentencepiece as spm
-
-SPIECE_UNDERLINE = "▁"
-
-
-def validate_case_matches_checkpoint(do_lower_case, init_checkpoint):
- """Checks whether the casing config is consistent with the checkpoint name."""
-
- # The casing has to be passed in by the user and there is no explicit check
- # as to whether it matches the checkpoint. The casing information probably
- # should have been stored in the bert_config.json file, but it's not, so
- # we have to heuristically detect it to validate.
-
- if not init_checkpoint:
- return
-
- m = re.match("^.*?([A-Za-z0-9_-]+)/bert_model.ckpt", init_checkpoint)
- if m is None:
- return
-
- model_name = m.group(1)
-
- lower_models = [
- "uncased_L-24_H-1024_A-16", "uncased_L-12_H-768_A-12",
- "multilingual_L-12_H-768_A-12", "chinese_L-12_H-768_A-12"
- ]
-
- cased_models = [
- "cased_L-12_H-768_A-12", "cased_L-24_H-1024_A-16",
- "multi_cased_L-12_H-768_A-12"
- ]
-
- is_bad_config = False
- if model_name in lower_models and not do_lower_case:
- is_bad_config = True
- actual_flag = "False"
- case_name = "lowercased"
- opposite_flag = "True"
-
- if model_name in cased_models and do_lower_case:
- is_bad_config = True
- actual_flag = "True"
- case_name = "cased"
- opposite_flag = "False"
-
- if is_bad_config:
- raise ValueError(
- "You passed in `--do_lower_case=%s` with `--init_checkpoint=%s`. "
- "However, `%s` seems to be a %s model, so you "
- "should pass in `--do_lower_case=%s` so that the fine-tuning matches "
- "how the model was pre-training. If this error is wrong, please "
- "just comment out this check." %
- (actual_flag, init_checkpoint, model_name, case_name, opposite_flag))
-
-
-def convert_to_unicode(text):
- """Converts `text` to Unicode (if it's not already), assuming utf-8 input."""
- if six.PY3:
- if isinstance(text, str):
- return text
- elif isinstance(text, bytes):
- return text.decode("utf-8", "ignore")
- else:
- raise ValueError("Unsupported string type: %s" % (type(text)))
- elif six.PY2:
- if isinstance(text, str):
- return text.decode("utf-8", "ignore")
- elif isinstance(text, unicode):
- return text
- else:
- raise ValueError("Unsupported string type: %s" % (type(text)))
- else:
- raise ValueError("Not running on Python2 or Python 3?")
-
-
-def printable_text(text):
- """Returns text encoded in a way suitable for print or `tf.logging`."""
-
- # These functions want `str` for both Python2 and Python3, but in one case
- # it's a Unicode string and in the other it's a byte string.
- if six.PY3:
- if isinstance(text, str):
- return text
- elif isinstance(text, bytes):
- return text.decode("utf-8", "ignore")
- else:
- raise ValueError("Unsupported string type: %s" % (type(text)))
- elif six.PY2:
- if isinstance(text, str):
- return text
- elif isinstance(text, unicode):
- return text.encode("utf-8")
- else:
- raise ValueError("Unsupported string type: %s" % (type(text)))
- else:
- raise ValueError("Not running on Python2 or Python 3?")
-
-
-def load_vocab(vocab_file):
- """Loads a vocabulary file into a dictionary."""
- vocab = collections.OrderedDict()
- index = 0
- with tf.io.gfile.GFile(vocab_file, "r") as reader:
- while True:
- token = convert_to_unicode(reader.readline())
- if not token:
- break
- token = token.strip()
- vocab[token] = index
- index += 1
- return vocab
-
-
-def convert_by_vocab(vocab, items):
- """Converts a sequence of [tokens|ids] using the vocab."""
- output = []
- for item in items:
- output.append(vocab[item])
- return output
-
-
-def convert_tokens_to_ids(vocab, tokens):
- return convert_by_vocab(vocab, tokens)
-
-
-def convert_ids_to_tokens(inv_vocab, ids):
- return convert_by_vocab(inv_vocab, ids)
-
-
-def whitespace_tokenize(text):
- """Runs basic whitespace cleaning and splitting on a piece of text."""
- text = text.strip()
- if not text:
- return []
- tokens = text.split()
- return tokens
-
-
-class FullTokenizer(object):
- """Runs end-to-end tokenziation."""
-
- def __init__(self, vocab_file, do_lower_case=True, split_on_punc=True):
- self.vocab = load_vocab(vocab_file)
- self.inv_vocab = {v: k for k, v in self.vocab.items()}
- self.basic_tokenizer = BasicTokenizer(
- do_lower_case=do_lower_case, split_on_punc=split_on_punc)
- self.wordpiece_tokenizer = WordpieceTokenizer(vocab=self.vocab)
-
- def tokenize(self, text):
- split_tokens = []
- for token in self.basic_tokenizer.tokenize(text):
- for sub_token in self.wordpiece_tokenizer.tokenize(token):
- split_tokens.append(sub_token)
-
- return split_tokens
-
- def convert_tokens_to_ids(self, tokens):
- return convert_by_vocab(self.vocab, tokens)
-
- def convert_ids_to_tokens(self, ids):
- return convert_by_vocab(self.inv_vocab, ids)
-
-
-class BasicTokenizer(object):
- """Runs basic tokenization (punctuation splitting, lower casing, etc.)."""
-
- def __init__(self, do_lower_case=True, split_on_punc=True):
- """Constructs a BasicTokenizer.
-
- Args:
- do_lower_case: Whether to lower case the input.
- split_on_punc: Whether to apply split on punctuations. By default BERT
- starts a new token for punctuations. This makes detokenization difficult
- for tasks like seq2seq decoding.
- """
- self.do_lower_case = do_lower_case
- self.split_on_punc = split_on_punc
-
- def tokenize(self, text):
- """Tokenizes a piece of text."""
- text = convert_to_unicode(text)
- text = self._clean_text(text)
-
- # This was added on November 1st, 2018 for the multilingual and Chinese
- # models. This is also applied to the English models now, but it doesn't
- # matter since the English models were not trained on any Chinese data
- # and generally don't have any Chinese data in them (there are Chinese
- # characters in the vocabulary because Wikipedia does have some Chinese
- # words in the English Wikipedia.).
- text = self._tokenize_chinese_chars(text)
-
- orig_tokens = whitespace_tokenize(text)
- split_tokens = []
- for token in orig_tokens:
- if self.do_lower_case:
- token = token.lower()
- token = self._run_strip_accents(token)
- if self.split_on_punc:
- split_tokens.extend(self._run_split_on_punc(token))
- else:
- split_tokens.append(token)
-
- output_tokens = whitespace_tokenize(" ".join(split_tokens))
- return output_tokens
-
- def _run_strip_accents(self, text):
- """Strips accents from a piece of text."""
- text = unicodedata.normalize("NFD", text)
- output = []
- for char in text:
- cat = unicodedata.category(char)
- if cat == "Mn":
- continue
- output.append(char)
- return "".join(output)
-
- def _run_split_on_punc(self, text):
- """Splits punctuation on a piece of text."""
- chars = list(text)
- i = 0
- start_new_word = True
- output = []
- while i < len(chars):
- char = chars[i]
- if _is_punctuation(char):
- output.append([char])
- start_new_word = True
- else:
- if start_new_word:
- output.append([])
- start_new_word = False
- output[-1].append(char)
- i += 1
-
- return ["".join(x) for x in output]
-
- def _tokenize_chinese_chars(self, text):
- """Adds whitespace around any CJK character."""
- output = []
- for char in text:
- cp = ord(char)
- if self._is_chinese_char(cp):
- output.append(" ")
- output.append(char)
- output.append(" ")
- else:
- output.append(char)
- return "".join(output)
-
- def _is_chinese_char(self, cp):
- """Checks whether CP is the codepoint of a CJK character."""
- # This defines a "chinese character" as anything in the CJK Unicode block:
- # https://en.wikipedia.org/wiki/CJK_Unified_Ideographs_(Unicode_block)
- #
- # Note that the CJK Unicode block is NOT all Japanese and Korean characters,
- # despite its name. The modern Korean Hangul alphabet is a different block,
- # as is Japanese Hiragana and Katakana. Those alphabets are used to write
- # space-separated words, so they are not treated specially and handled
- # like the all of the other languages.
- if ((cp >= 0x4E00 and cp <= 0x9FFF) or #
- (cp >= 0x3400 and cp <= 0x4DBF) or #
- (cp >= 0x20000 and cp <= 0x2A6DF) or #
- (cp >= 0x2A700 and cp <= 0x2B73F) or #
- (cp >= 0x2B740 and cp <= 0x2B81F) or #
- (cp >= 0x2B820 and cp <= 0x2CEAF) or
- (cp >= 0xF900 and cp <= 0xFAFF) or #
- (cp >= 0x2F800 and cp <= 0x2FA1F)): #
- return True
-
- return False
-
- def _clean_text(self, text):
- """Performs invalid character removal and whitespace cleanup on text."""
- output = []
- for char in text:
- cp = ord(char)
- if cp == 0 or cp == 0xfffd or _is_control(char):
- continue
- if _is_whitespace(char):
- output.append(" ")
- else:
- output.append(char)
- return "".join(output)
-
-
-class WordpieceTokenizer(object):
- """Runs WordPiece tokenziation."""
-
- def __init__(self, vocab, unk_token="[UNK]", max_input_chars_per_word=400):
- self.vocab = vocab
- self.unk_token = unk_token
- self.max_input_chars_per_word = max_input_chars_per_word
-
- def tokenize(self, text):
- """Tokenizes a piece of text into its word pieces.
-
- This uses a greedy longest-match-first algorithm to perform tokenization
- using the given vocabulary.
-
- For example:
- input = "unaffable"
- output = ["un", "##aff", "##able"]
-
- Args:
- text: A single token or whitespace separated tokens. This should have
- already been passed through `BasicTokenizer.
-
- Returns:
- A list of wordpiece tokens.
- """
-
- text = convert_to_unicode(text)
-
- output_tokens = []
- for token in whitespace_tokenize(text):
- chars = list(token)
- if len(chars) > self.max_input_chars_per_word:
- output_tokens.append(self.unk_token)
- continue
-
- is_bad = False
- start = 0
- sub_tokens = []
- while start < len(chars):
- end = len(chars)
- cur_substr = None
- while start < end:
- substr = "".join(chars[start:end])
- if start > 0:
- substr = "##" + substr
- if substr in self.vocab:
- cur_substr = substr
- break
- end -= 1
- if cur_substr is None:
- is_bad = True
- break
- sub_tokens.append(cur_substr)
- start = end
-
- if is_bad:
- output_tokens.append(self.unk_token)
- else:
- output_tokens.extend(sub_tokens)
- return output_tokens
-
-
-def _is_whitespace(char):
- """Checks whether `chars` is a whitespace character."""
- # \t, \n, and \r are technically control characters but we treat them
- # as whitespace since they are generally considered as such.
- if char == " " or char == "\t" or char == "\n" or char == "\r":
- return True
- cat = unicodedata.category(char)
- if cat == "Zs":
- return True
- return False
-
-
-def _is_control(char):
- """Checks whether `chars` is a control character."""
- # These are technically control characters but we count them as whitespace
- # characters.
- if char == "\t" or char == "\n" or char == "\r":
- return False
- cat = unicodedata.category(char)
- if cat in ("Cc", "Cf"):
- return True
- return False
-
-
-def _is_punctuation(char):
- """Checks whether `chars` is a punctuation character."""
- cp = ord(char)
- # We treat all non-letter/number ASCII as punctuation.
- # Characters such as "^", "$", and "`" are not in the Unicode
- # Punctuation class but we treat them as punctuation anyways, for
- # consistency.
- if ((cp >= 33 and cp <= 47) or (cp >= 58 and cp <= 64) or
- (cp >= 91 and cp <= 96) or (cp >= 123 and cp <= 126)):
- return True
- cat = unicodedata.category(char)
- if cat.startswith("P"):
- return True
- return False
-
-
-def preprocess_text(inputs, remove_space=True, lower=False):
- """Preprocesses data by removing extra space and normalize data.
-
- This method is used together with sentence piece tokenizer and is forked from:
- https://github.com/google-research/google-research/blob/master/albert/tokenization.py
-
- Args:
- inputs: The input text.
- remove_space: Whether to remove the extra space.
- lower: Whether to lowercase the text.
-
- Returns:
- The preprocessed text.
-
- """
- outputs = inputs
- if remove_space:
- outputs = " ".join(inputs.strip().split())
-
- if six.PY2 and isinstance(outputs, str):
- try:
- outputs = six.ensure_text(outputs, "utf-8")
- except UnicodeDecodeError:
- outputs = six.ensure_text(outputs, "latin-1")
-
- outputs = unicodedata.normalize("NFKD", outputs)
- outputs = "".join([c for c in outputs if not unicodedata.combining(c)])
- if lower:
- outputs = outputs.lower()
-
- return outputs
-
-
-def encode_pieces(sp_model, text, sample=False):
- """Segements text into pieces.
-
- This method is used together with sentence piece tokenizer and is forked from:
- https://github.com/google-research/google-research/blob/master/albert/tokenization.py
-
-
- Args:
- sp_model: A spm.SentencePieceProcessor object.
- text: The input text to be segemented.
- sample: Whether to randomly sample a segmentation output or return a
- deterministic one.
-
- Returns:
- A list of token pieces.
- """
- if six.PY2 and isinstance(text, six.text_type):
- text = six.ensure_binary(text, "utf-8")
-
- if not sample:
- pieces = sp_model.EncodeAsPieces(text)
- else:
- pieces = sp_model.SampleEncodeAsPieces(text, 64, 0.1)
- new_pieces = []
- for piece in pieces:
- piece = printable_text(piece)
- if len(piece) > 1 and piece[-1] == "," and piece[-2].isdigit():
- cur_pieces = sp_model.EncodeAsPieces(piece[:-1].replace(
- SPIECE_UNDERLINE, ""))
- if piece[0] != SPIECE_UNDERLINE and cur_pieces[0][0] == SPIECE_UNDERLINE:
- if len(cur_pieces[0]) == 1:
- cur_pieces = cur_pieces[1:]
- else:
- cur_pieces[0] = cur_pieces[0][1:]
- cur_pieces.append(piece[-1])
- new_pieces.extend(cur_pieces)
- else:
- new_pieces.append(piece)
-
- return new_pieces
-
-
-def encode_ids(sp_model, text, sample=False):
- """Segments text and return token ids.
-
- This method is used together with sentence piece tokenizer and is forked from:
- https://github.com/google-research/google-research/blob/master/albert/tokenization.py
-
- Args:
- sp_model: A spm.SentencePieceProcessor object.
- text: The input text to be segemented.
- sample: Whether to randomly sample a segmentation output or return a
- deterministic one.
-
- Returns:
- A list of token ids.
- """
- pieces = encode_pieces(sp_model, text, sample=sample)
- ids = [sp_model.PieceToId(piece) for piece in pieces]
- return ids
-
-
-class FullSentencePieceTokenizer(object):
- """Runs end-to-end sentence piece tokenization.
-
- The interface of this class is intended to keep the same as above
- `FullTokenizer` class for easier usage.
- """
-
- def __init__(self, sp_model_file):
- """Inits FullSentencePieceTokenizer.
-
- Args:
- sp_model_file: The path to the sentence piece model file.
- """
- self.sp_model = spm.SentencePieceProcessor()
- self.sp_model.Load(sp_model_file)
- self.vocab = {
- self.sp_model.IdToPiece(i): i
- for i in six.moves.range(self.sp_model.GetPieceSize())
- }
-
- def tokenize(self, text):
- """Tokenizes text into pieces."""
- return encode_pieces(self.sp_model, text)
-
- def convert_tokens_to_ids(self, tokens):
- """Converts a list of tokens to a list of ids."""
- return [self.sp_model.PieceToId(printable_text(token)) for token in tokens]
-
- def convert_ids_to_tokens(self, ids):
- """Converts a list of ids ot a list of tokens."""
- return [self.sp_model.IdToPiece(id_) for id_ in ids]
diff --git a/spaces/NingKanae/anime-voice-generator/monotonic_align/__init__.py b/spaces/NingKanae/anime-voice-generator/monotonic_align/__init__.py
deleted file mode 100644
index e97eecc595dd3bd97d0104ec62799e2e5efea57c..0000000000000000000000000000000000000000
--- a/spaces/NingKanae/anime-voice-generator/monotonic_align/__init__.py
+++ /dev/null
@@ -1,20 +0,0 @@
-from numpy import zeros, int32, float32
-from torch import from_numpy
-
-from .core import maximum_path_jit
-
-
-def maximum_path(neg_cent, mask):
- """ numba optimized version.
- neg_cent: [b, t_t, t_s]
- mask: [b, t_t, t_s]
- """
- device = neg_cent.device
- dtype = neg_cent.dtype
- neg_cent = neg_cent.data.cpu().numpy().astype(float32)
- path = zeros(neg_cent.shape, dtype=int32)
-
- t_t_max = mask.sum(1)[:, 0].data.cpu().numpy().astype(int32)
- t_s_max = mask.sum(2)[:, 0].data.cpu().numpy().astype(int32)
- maximum_path_jit(path, neg_cent, t_t_max, t_s_max)
- return from_numpy(path).to(device=device, dtype=dtype)
diff --git a/spaces/Noobian/How-To-Generator/README.md b/spaces/Noobian/How-To-Generator/README.md
deleted file mode 100644
index 336d34d9039e7fe1326aa30aa2faaf5074ba3045..0000000000000000000000000000000000000000
--- a/spaces/Noobian/How-To-Generator/README.md
+++ /dev/null
@@ -1,12 +0,0 @@
----
-title: How To Generatot
-emoji: 🌍
-colorFrom: yellow
-colorTo: green
-sdk: gradio
-sdk_version: 3.18.0
-app_file: app.py
-pinned: false
----
-
-Check out the configuration reference at https://huggingface.co/docs/hub/spaces-config-reference
diff --git a/spaces/OFA-Sys/OFA-Generic_Interface/fairseq/examples/fast_noisy_channel/noisy_channel_beam_search.py b/spaces/OFA-Sys/OFA-Generic_Interface/fairseq/examples/fast_noisy_channel/noisy_channel_beam_search.py
deleted file mode 100644
index 23869ebcd0c438f36e310c8ccddd3b5c07a71182..0000000000000000000000000000000000000000
--- a/spaces/OFA-Sys/OFA-Generic_Interface/fairseq/examples/fast_noisy_channel/noisy_channel_beam_search.py
+++ /dev/null
@@ -1,71 +0,0 @@
-# Copyright (c) Facebook, Inc. and its affiliates.
-#
-# This source code is licensed under the MIT license found in the
-# LICENSE file in the root directory of this source tree.
-
-import torch
-from fairseq.search import Search
-
-
-class NoisyChannelBeamSearch(Search):
-
- def __init__(self, tgt_dict):
- super().__init__(tgt_dict)
- self.fw_scores_buf = None
- self.lm_scores_buf = None
-
- def _init_buffers(self, t):
- # super()._init_buffers(t)
- if self.fw_scores_buf is None:
- self.scores_buf = t.new()
- self.indices_buf = torch.LongTensor().to(device=t.device)
- self.beams_buf = torch.LongTensor().to(device=t.device)
- self.fw_scores_buf = t.new()
- self.lm_scores_buf = t.new()
-
- def combine_fw_bw(self, combine_method, fw_cum, bw, step):
- if combine_method == "noisy_channel":
- fw_norm = fw_cum.div(step + 1)
- lprobs = bw + fw_norm
- elif combine_method == "lm_only":
- lprobs = bw + fw_cum
-
- return lprobs
-
- def step(self, step, fw_lprobs, scores, bw_lprobs, lm_lprobs, combine_method):
- self._init_buffers(fw_lprobs)
- bsz, beam_size, vocab_size = fw_lprobs.size()
-
- if step == 0:
- # at the first step all hypotheses are equally likely, so use
- # only the first beam
- fw_lprobs = fw_lprobs[:, ::beam_size, :].contiguous()
- bw_lprobs = bw_lprobs[:, ::beam_size, :].contiguous()
- # nothing to add since we are at the first step
- fw_lprobs_cum = fw_lprobs
-
- else:
- # make probs contain cumulative scores for each hypothesis
- raw_scores = (scores[:, :, step - 1].unsqueeze(-1))
- fw_lprobs_cum = (fw_lprobs.add(raw_scores))
-
- combined_lprobs = self.combine_fw_bw(combine_method, fw_lprobs_cum, bw_lprobs, step)
-
- # choose the top k according to the combined noisy channel model score
- torch.topk(
- combined_lprobs.view(bsz, -1),
- k=min(
- # Take the best 2 x beam_size predictions. We'll choose the first
- # beam_size of these which don't predict eos to continue with.
- beam_size * 2,
- combined_lprobs.view(bsz, -1).size(1) - 1, # -1 so we never select pad
- ),
- out=(self.scores_buf, self.indices_buf),
- )
- # save corresponding fw and lm scores
- self.fw_scores_buf = torch.gather(fw_lprobs_cum.view(bsz, -1), 1, self.indices_buf)
- self.lm_scores_buf = torch.gather(lm_lprobs.view(bsz, -1), 1, self.indices_buf)
- # Project back into relative indices and beams
- self.beams_buf = self.indices_buf // vocab_size
- self.indices_buf.fmod_(vocab_size)
- return self.scores_buf, self.fw_scores_buf, self.lm_scores_buf, self.indices_buf, self.beams_buf
diff --git a/spaces/OFA-Sys/OFA-Generic_Interface/fairseq/fairseq/clib/libbleu/module.cpp b/spaces/OFA-Sys/OFA-Generic_Interface/fairseq/fairseq/clib/libbleu/module.cpp
deleted file mode 100644
index 35288b3177185670135f7bdc1f1589c5bb992304..0000000000000000000000000000000000000000
--- a/spaces/OFA-Sys/OFA-Generic_Interface/fairseq/fairseq/clib/libbleu/module.cpp
+++ /dev/null
@@ -1,33 +0,0 @@
-/**
- * Copyright 2017-present, Facebook, Inc.
- * All rights reserved.
- *
- * This source code is licensed under the license found in the
- * LICENSE file in the root directory of this source tree.
- */
-
-#include
-
-static PyMethodDef method_def[] = {{NULL, NULL, 0, NULL}}; // NOLINT
-
-static struct PyModuleDef module_def = {
- PyModuleDef_HEAD_INIT,
- "libbleu", /* name of module */
- // NOLINTNEXTLINE
- NULL, /* module documentation, may be NULL */
- -1, /* size of per-interpreter state of the module,
- or -1 if the module keeps state in global variables. */
- method_def}; // NOLINT
-
-#if PY_MAJOR_VERSION == 2
-PyMODINIT_FUNC init_libbleu()
-#else
-PyMODINIT_FUNC PyInit_libbleu()
-#endif
-{
- PyObject* m = PyModule_Create(&module_def);
- if (!m) {
- return NULL;
- }
- return m;
-}
diff --git a/spaces/OFA-Sys/OFA-Image_Caption/fairseq/examples/speech_recognition/new/__init__.py b/spaces/OFA-Sys/OFA-Image_Caption/fairseq/examples/speech_recognition/new/__init__.py
deleted file mode 100644
index e69de29bb2d1d6434b8b29ae775ad8c2e48c5391..0000000000000000000000000000000000000000
diff --git a/spaces/OFA-Sys/OFA-Image_Caption/fairseq/examples/wav2vec/unsupervised/tasks/__init__.py b/spaces/OFA-Sys/OFA-Image_Caption/fairseq/examples/wav2vec/unsupervised/tasks/__init__.py
deleted file mode 100644
index 6d7dd625e09451be671908578f93148f371f53cd..0000000000000000000000000000000000000000
--- a/spaces/OFA-Sys/OFA-Image_Caption/fairseq/examples/wav2vec/unsupervised/tasks/__init__.py
+++ /dev/null
@@ -1,11 +0,0 @@
-# Copyright (c) Facebook, Inc. and its affiliates.
-#
-# This source code is licensed under the MIT license found in the
-# LICENSE file in the root directory of this source tree.
-
-from .unpaired_audio_text import UnpairedAudioText
-
-
-__all__ = [
- "UnpairedAudioText",
-]
diff --git a/spaces/OFA-Sys/OFA-Image_Caption/fairseq/fairseq/models/roberta/alignment_utils.py b/spaces/OFA-Sys/OFA-Image_Caption/fairseq/fairseq/models/roberta/alignment_utils.py
deleted file mode 100644
index ccc7f74cb94d5b8baa2d4e9dfd44f653d47ee43e..0000000000000000000000000000000000000000
--- a/spaces/OFA-Sys/OFA-Image_Caption/fairseq/fairseq/models/roberta/alignment_utils.py
+++ /dev/null
@@ -1,118 +0,0 @@
-# Copyright (c) Facebook, Inc. and its affiliates.
-#
-# This source code is licensed under the MIT license found in the
-# LICENSE file in the root directory of this source tree.
-
-from collections import Counter
-from typing import List
-
-import torch
-
-
-def align_bpe_to_words(roberta, bpe_tokens: torch.LongTensor, other_tokens: List[str]):
- """
- Helper to align GPT-2 BPE to other tokenization formats (e.g., spaCy).
-
- Args:
- roberta (RobertaHubInterface): RoBERTa instance
- bpe_tokens (torch.LongTensor): GPT-2 BPE tokens of shape `(T_bpe)`
- other_tokens (List[str]): other tokens of shape `(T_words)`
-
- Returns:
- List[str]: mapping from *other_tokens* to corresponding *bpe_tokens*.
- """
- assert bpe_tokens.dim() == 1
- assert bpe_tokens[0] == 0
-
- def clean(text):
- return text.strip()
-
- # remove whitespaces to simplify alignment
- bpe_tokens = [roberta.task.source_dictionary.string([x]) for x in bpe_tokens]
- bpe_tokens = [
- clean(roberta.bpe.decode(x) if x not in {"", ""} else x) for x in bpe_tokens
- ]
- other_tokens = [clean(str(o)) for o in other_tokens]
-
- # strip leading
- bpe_tokens = bpe_tokens[1:]
- assert "".join(bpe_tokens) == "".join(other_tokens)
-
- # create alignment from every word to a list of BPE tokens
- alignment = []
- bpe_toks = filter(lambda item: item[1] != "", enumerate(bpe_tokens, start=1))
- j, bpe_tok = next(bpe_toks)
- for other_tok in other_tokens:
- bpe_indices = []
- while True:
- if other_tok.startswith(bpe_tok):
- bpe_indices.append(j)
- other_tok = other_tok[len(bpe_tok) :]
- try:
- j, bpe_tok = next(bpe_toks)
- except StopIteration:
- j, bpe_tok = None, None
- elif bpe_tok.startswith(other_tok):
- # other_tok spans multiple BPE tokens
- bpe_indices.append(j)
- bpe_tok = bpe_tok[len(other_tok) :]
- other_tok = ""
- else:
- raise Exception('Cannot align "{}" and "{}"'.format(other_tok, bpe_tok))
- if other_tok == "":
- break
- assert len(bpe_indices) > 0
- alignment.append(bpe_indices)
- assert len(alignment) == len(other_tokens)
-
- return alignment
-
-
-def align_features_to_words(roberta, features, alignment):
- """
- Align given features to words.
-
- Args:
- roberta (RobertaHubInterface): RoBERTa instance
- features (torch.Tensor): features to align of shape `(T_bpe x C)`
- alignment: alignment between BPE tokens and words returned by
- func:`align_bpe_to_words`.
- """
- assert features.dim() == 2
-
- bpe_counts = Counter(j for bpe_indices in alignment for j in bpe_indices)
- assert bpe_counts[0] == 0 # shouldn't be aligned
- denom = features.new([bpe_counts.get(j, 1) for j in range(len(features))])
- weighted_features = features / denom.unsqueeze(-1)
-
- output = [weighted_features[0]]
- largest_j = -1
- for bpe_indices in alignment:
- output.append(weighted_features[bpe_indices].sum(dim=0))
- largest_j = max(largest_j, *bpe_indices)
- for j in range(largest_j + 1, len(features)):
- output.append(weighted_features[j])
- output = torch.stack(output)
- assert torch.all(torch.abs(output.sum(dim=0) - features.sum(dim=0)) < 1e-4)
- return output
-
-
-def spacy_nlp():
- if getattr(spacy_nlp, "_nlp", None) is None:
- try:
- from spacy.lang.en import English
-
- spacy_nlp._nlp = English()
- except ImportError:
- raise ImportError("Please install spacy with: pip install spacy")
- return spacy_nlp._nlp
-
-
-def spacy_tokenizer():
- if getattr(spacy_tokenizer, "_tokenizer", None) is None:
- try:
- nlp = spacy_nlp()
- spacy_tokenizer._tokenizer = nlp.Defaults.create_tokenizer(nlp)
- except ImportError:
- raise ImportError("Please install spacy with: pip install spacy")
- return spacy_tokenizer._tokenizer
diff --git a/spaces/OFA-Sys/OFA-vqa/fairseq/examples/speech_synthesis/data_utils.py b/spaces/OFA-Sys/OFA-vqa/fairseq/examples/speech_synthesis/data_utils.py
deleted file mode 100644
index f43a4a90046fb9ee4944dc06ba377c1faade141d..0000000000000000000000000000000000000000
--- a/spaces/OFA-Sys/OFA-vqa/fairseq/examples/speech_synthesis/data_utils.py
+++ /dev/null
@@ -1,320 +0,0 @@
-# Copyright (c) Facebook, Inc. and its affiliates.
-#
-# This source code is licensed under the MIT license found in the
-# LICENSE file in the root directory of this source tree.
-
-import os
-from pathlib import Path
-from typing import Optional, List, Dict
-import zipfile
-import tempfile
-from dataclasses import dataclass
-from itertools import groupby
-
-import torch
-import torch.nn.functional as F
-import numpy as np
-from tqdm import tqdm
-
-from examples.speech_to_text.data_utils import load_tsv_to_dicts
-from fairseq.data.audio.audio_utils import TTSSpectrogram, TTSMelScale
-
-
-def trim_or_pad_to_target_length(
- data_1d_or_2d: np.ndarray, target_length: int
-) -> np.ndarray:
- assert len(data_1d_or_2d.shape) in {1, 2}
- delta = data_1d_or_2d.shape[0] - target_length
- if delta >= 0: # trim if being longer
- data_1d_or_2d = data_1d_or_2d[: target_length]
- else: # pad if being shorter
- if len(data_1d_or_2d.shape) == 1:
- data_1d_or_2d = np.concatenate(
- [data_1d_or_2d, np.zeros(-delta)], axis=0
- )
- else:
- data_1d_or_2d = np.concatenate(
- [data_1d_or_2d, np.zeros((-delta, data_1d_or_2d.shape[1]))],
- axis=0
- )
- return data_1d_or_2d
-
-
-def extract_logmel_spectrogram(
- waveform: torch.Tensor, sample_rate: int,
- output_path: Optional[Path] = None, win_length: int = 1024,
- hop_length: int = 256, n_fft: int = 1024,
- win_fn: callable = torch.hann_window, n_mels: int = 80,
- f_min: float = 0., f_max: float = 8000, eps: float = 1e-5,
- overwrite: bool = False, target_length: Optional[int] = None
-):
- if output_path is not None and output_path.is_file() and not overwrite:
- return
-
- spectrogram_transform = TTSSpectrogram(
- n_fft=n_fft, win_length=win_length, hop_length=hop_length,
- window_fn=win_fn
- )
- mel_scale_transform = TTSMelScale(
- n_mels=n_mels, sample_rate=sample_rate, f_min=f_min, f_max=f_max,
- n_stft=n_fft // 2 + 1
- )
- spectrogram = spectrogram_transform(waveform)
- mel_spec = mel_scale_transform(spectrogram)
- logmel_spec = torch.clamp(mel_spec, min=eps).log()
- assert len(logmel_spec.shape) == 3 and logmel_spec.shape[0] == 1
- logmel_spec = logmel_spec.squeeze().t() # D x T -> T x D
- if target_length is not None:
- trim_or_pad_to_target_length(logmel_spec, target_length)
-
- if output_path is not None:
- np.save(output_path.as_posix(), logmel_spec)
- else:
- return logmel_spec
-
-
-def extract_pitch(
- waveform: torch.Tensor, sample_rate: int,
- output_path: Optional[Path] = None, hop_length: int = 256,
- log_scale: bool = True, phoneme_durations: Optional[List[int]] = None
-):
- if output_path is not None and output_path.is_file():
- return
-
- try:
- import pyworld
- except ImportError:
- raise ImportError("Please install PyWORLD: pip install pyworld")
-
- _waveform = waveform.squeeze(0).double().numpy()
- pitch, t = pyworld.dio(
- _waveform, sample_rate, frame_period=hop_length / sample_rate * 1000
- )
- pitch = pyworld.stonemask(_waveform, pitch, t, sample_rate)
-
- if phoneme_durations is not None:
- pitch = trim_or_pad_to_target_length(pitch, sum(phoneme_durations))
- try:
- from scipy.interpolate import interp1d
- except ImportError:
- raise ImportError("Please install SciPy: pip install scipy")
- nonzero_ids = np.where(pitch != 0)[0]
- interp_fn = interp1d(
- nonzero_ids,
- pitch[nonzero_ids],
- fill_value=(pitch[nonzero_ids[0]], pitch[nonzero_ids[-1]]),
- bounds_error=False,
- )
- pitch = interp_fn(np.arange(0, len(pitch)))
- d_cumsum = np.cumsum(np.concatenate([np.array([0]), phoneme_durations]))
- pitch = np.array(
- [
- np.mean(pitch[d_cumsum[i-1]: d_cumsum[i]])
- for i in range(1, len(d_cumsum))
- ]
- )
- assert len(pitch) == len(phoneme_durations)
-
- if log_scale:
- pitch = np.log(pitch + 1)
-
- if output_path is not None:
- np.save(output_path.as_posix(), pitch)
- else:
- return pitch
-
-
-def extract_energy(
- waveform: torch.Tensor, output_path: Optional[Path] = None,
- hop_length: int = 256, n_fft: int = 1024, log_scale: bool = True,
- phoneme_durations: Optional[List[int]] = None
-):
- if output_path is not None and output_path.is_file():
- return
-
- assert len(waveform.shape) == 2 and waveform.shape[0] == 1
- waveform = waveform.view(1, 1, waveform.shape[1])
- waveform = F.pad(
- waveform.unsqueeze(1), [n_fft // 2, n_fft // 2, 0, 0],
- mode="reflect"
- )
- waveform = waveform.squeeze(1)
-
- fourier_basis = np.fft.fft(np.eye(n_fft))
- cutoff = int((n_fft / 2 + 1))
- fourier_basis = np.vstack(
- [np.real(fourier_basis[:cutoff, :]),
- np.imag(fourier_basis[:cutoff, :])]
- )
-
- forward_basis = torch.FloatTensor(fourier_basis[:, None, :])
- forward_transform = F.conv1d(
- waveform, forward_basis, stride=hop_length, padding=0
- )
-
- real_part = forward_transform[:, :cutoff, :]
- imag_part = forward_transform[:, cutoff:, :]
- magnitude = torch.sqrt(real_part ** 2 + imag_part ** 2)
- energy = torch.norm(magnitude, dim=1).squeeze(0).numpy()
-
- if phoneme_durations is not None:
- energy = trim_or_pad_to_target_length(energy, sum(phoneme_durations))
- d_cumsum = np.cumsum(np.concatenate([np.array([0]), phoneme_durations]))
- energy = np.array(
- [
- np.mean(energy[d_cumsum[i - 1]: d_cumsum[i]])
- for i in range(1, len(d_cumsum))
- ]
- )
- assert len(energy) == len(phoneme_durations)
-
- if log_scale:
- energy = np.log(energy + 1)
-
- if output_path is not None:
- np.save(output_path.as_posix(), energy)
- else:
- return energy
-
-
-def get_global_cmvn(feature_root: Path, output_path: Optional[Path] = None):
- mean_x, mean_x2, n_frames = None, None, 0
- feature_paths = feature_root.glob("*.npy")
- for p in tqdm(feature_paths):
- with open(p, 'rb') as f:
- frames = np.load(f).squeeze()
-
- n_frames += frames.shape[0]
-
- cur_mean_x = frames.sum(axis=0)
- if mean_x is None:
- mean_x = cur_mean_x
- else:
- mean_x += cur_mean_x
-
- cur_mean_x2 = (frames ** 2).sum(axis=0)
- if mean_x2 is None:
- mean_x2 = cur_mean_x2
- else:
- mean_x2 += cur_mean_x2
-
- mean_x /= n_frames
- mean_x2 /= n_frames
- var_x = mean_x2 - mean_x ** 2
- std_x = np.sqrt(np.maximum(var_x, 1e-10))
-
- if output_path is not None:
- with open(output_path, 'wb') as f:
- np.savez(f, mean=mean_x, std=std_x)
- else:
- return {"mean": mean_x, "std": std_x}
-
-
-def ipa_phonemize(text, lang="en-us", use_g2p=False):
- if use_g2p:
- assert lang == "en-us", "g2pE phonemizer only works for en-us"
- try:
- from g2p_en import G2p
- g2p = G2p()
- return " ".join("|" if p == " " else p for p in g2p(text))
- except ImportError:
- raise ImportError(
- "Please install phonemizer: pip install g2p_en"
- )
- else:
- try:
- from phonemizer import phonemize
- from phonemizer.separator import Separator
- return phonemize(
- text, backend='espeak', language=lang,
- separator=Separator(word="| ", phone=" ")
- )
- except ImportError:
- raise ImportError(
- "Please install phonemizer: pip install phonemizer"
- )
-
-
-@dataclass
-class ForceAlignmentInfo(object):
- tokens: List[str]
- frame_durations: List[int]
- start_sec: Optional[float]
- end_sec: Optional[float]
-
-
-def get_mfa_alignment_by_sample_id(
- textgrid_zip_path: str, sample_id: str, sample_rate: int,
- hop_length: int, silence_phones: List[str] = ("sil", "sp", "spn")
-) -> ForceAlignmentInfo:
- try:
- import tgt
- except ImportError:
- raise ImportError("Please install TextGridTools: pip install tgt")
-
- filename = f"{sample_id}.TextGrid"
- out_root = Path(tempfile.gettempdir())
- tgt_path = out_root / filename
- with zipfile.ZipFile(textgrid_zip_path) as f_zip:
- f_zip.extract(filename, path=out_root)
- textgrid = tgt.io.read_textgrid(tgt_path.as_posix())
- os.remove(tgt_path)
-
- phones, frame_durations = [], []
- start_sec, end_sec, end_idx = 0, 0, 0
- for t in textgrid.get_tier_by_name("phones")._objects:
- s, e, p = t.start_time, t.end_time, t.text
- # Trim leading silences
- if len(phones) == 0:
- if p in silence_phones:
- continue
- else:
- start_sec = s
- phones.append(p)
- if p not in silence_phones:
- end_sec = e
- end_idx = len(phones)
- r = sample_rate / hop_length
- frame_durations.append(int(np.round(e * r) - np.round(s * r)))
- # Trim tailing silences
- phones = phones[:end_idx]
- frame_durations = frame_durations[:end_idx]
-
- return ForceAlignmentInfo(
- tokens=phones, frame_durations=frame_durations, start_sec=start_sec,
- end_sec=end_sec
- )
-
-
-def get_mfa_alignment(
- textgrid_zip_path: str, sample_ids: List[str], sample_rate: int,
- hop_length: int
-) -> Dict[str, ForceAlignmentInfo]:
- return {
- i: get_mfa_alignment_by_sample_id(
- textgrid_zip_path, i, sample_rate, hop_length
- ) for i in tqdm(sample_ids)
- }
-
-
-def get_unit_alignment(
- id_to_unit_tsv_path: str, sample_ids: List[str]
-) -> Dict[str, ForceAlignmentInfo]:
- id_to_units = {
- e["id"]: e["units"] for e in load_tsv_to_dicts(id_to_unit_tsv_path)
- }
- id_to_units = {i: id_to_units[i].split() for i in sample_ids}
- id_to_units_collapsed = {
- i: [uu for uu, _ in groupby(u)] for i, u in id_to_units.items()
- }
- id_to_durations = {
- i: [len(list(g)) for _, g in groupby(u)] for i, u in id_to_units.items()
- }
-
- return {
- i: ForceAlignmentInfo(
- tokens=id_to_units_collapsed[i], frame_durations=id_to_durations[i],
- start_sec=None, end_sec=None
- )
- for i in sample_ids
- }
diff --git a/spaces/OFA-Sys/OFA-vqa/fairseq/fairseq/benchmark/dummy_masked_lm.py b/spaces/OFA-Sys/OFA-vqa/fairseq/fairseq/benchmark/dummy_masked_lm.py
deleted file mode 100644
index 12b9c5d0f55993bf8750564882a351fc3f8055f0..0000000000000000000000000000000000000000
--- a/spaces/OFA-Sys/OFA-vqa/fairseq/fairseq/benchmark/dummy_masked_lm.py
+++ /dev/null
@@ -1,94 +0,0 @@
-# Copyright (c) Facebook, Inc. and its affiliates.
-#
-# This source code is licensed under the MIT license found in the
-# LICENSE file in the root directory of this source tree.
-
-import logging
-from dataclasses import dataclass, field
-from typing import Optional
-
-import torch
-from omegaconf import II
-
-from .dummy_dataset import DummyDataset
-from fairseq.data import Dictionary
-from fairseq.dataclass import FairseqDataclass
-from fairseq.tasks import FairseqTask, register_task
-
-logger = logging.getLogger(__name__)
-
-
-@dataclass
-class DummyMaskedLMConfig(FairseqDataclass):
- dict_size: int = 49996
- dataset_size: int = 100000
- tokens_per_sample: int = field(
- default=512,
- metadata={
- "help": "max number of total tokens over all"
- " segments per sample for BERT dataset"
- },
- )
- batch_size: Optional[int] = II("dataset.batch_size")
- max_tokens: Optional[int] = II("dataset.max_tokens")
- max_target_positions: int = II("task.tokens_per_sample")
-
-
-@register_task("dummy_masked_lm", dataclass=DummyMaskedLMConfig)
-class DummyMaskedLMTask(FairseqTask):
- def __init__(self, cfg: DummyMaskedLMConfig):
- super().__init__(cfg)
-
- self.dictionary = Dictionary()
- for i in range(cfg.dict_size):
- self.dictionary.add_symbol("word{}".format(i))
- logger.info("dictionary: {} types".format(len(self.dictionary)))
- # add mask token
- self.mask_idx = self.dictionary.add_symbol("")
- self.dictionary.pad_to_multiple_(8) # often faster if divisible by 8
-
- mask_idx = 0
- pad_idx = 1
- seq = torch.arange(cfg.tokens_per_sample) + pad_idx + 1
- mask = torch.arange(2, cfg.tokens_per_sample, 7) # ~15%
- src = seq.clone()
- src[mask] = mask_idx
- tgt = torch.full_like(seq, pad_idx)
- tgt[mask] = seq[mask]
-
- self.dummy_src = src
- self.dummy_tgt = tgt
-
- def load_dataset(self, split, epoch=1, combine=False, **kwargs):
- """Load a given dataset split.
- Args:
- split (str): name of the split (e.g., train, valid, test)
- """
- if self.cfg.batch_size is not None:
- bsz = self.cfg.batch_size
- else:
- bsz = max(1, self.cfg.max_tokens // self.cfg.tokens_per_sample)
- self.datasets[split] = DummyDataset(
- {
- "id": 1,
- "net_input": {
- "src_tokens": torch.stack([self.dummy_src for _ in range(bsz)]),
- "src_lengths": torch.full(
- (bsz,), self.cfg.tokens_per_sample, dtype=torch.long
- ),
- },
- "target": torch.stack([self.dummy_tgt for _ in range(bsz)]),
- "nsentences": bsz,
- "ntokens": bsz * self.cfg.tokens_per_sample,
- },
- num_items=self.cfg.dataset_size,
- item_size=self.cfg.tokens_per_sample,
- )
-
- @property
- def source_dictionary(self):
- return self.dictionary
-
- @property
- def target_dictionary(self):
- return self.dictionary
diff --git a/spaces/ORI-Muchim/ONFIRETTS/utils.py b/spaces/ORI-Muchim/ONFIRETTS/utils.py
deleted file mode 100644
index 4cb5b43d0ca2bae496e7871b2094f2ffb26ab642..0000000000000000000000000000000000000000
--- a/spaces/ORI-Muchim/ONFIRETTS/utils.py
+++ /dev/null
@@ -1,226 +0,0 @@
-import os
-import glob
-import sys
-import argparse
-import logging
-import json
-import subprocess
-import numpy as np
-from scipy.io.wavfile import read
-import torch
-
-MATPLOTLIB_FLAG = False
-
-logging.basicConfig(stream=sys.stdout, level=logging.ERROR)
-logger = logging
-
-
-def load_checkpoint(checkpoint_path, model, optimizer=None):
- assert os.path.isfile(checkpoint_path)
- checkpoint_dict = torch.load(checkpoint_path, map_location='cpu')
- iteration = checkpoint_dict['iteration']
- learning_rate = checkpoint_dict['learning_rate']
- if optimizer is not None:
- optimizer.load_state_dict(checkpoint_dict['optimizer'])
- saved_state_dict = checkpoint_dict['model']
- if hasattr(model, 'module'):
- state_dict = model.module.state_dict()
- else:
- state_dict = model.state_dict()
- new_state_dict = {}
- for k, v in state_dict.items():
- try:
- new_state_dict[k] = saved_state_dict[k]
- except:
- logger.info("%s is not in the checkpoint" % k)
- new_state_dict[k] = v
- if hasattr(model, 'module'):
- model.module.load_state_dict(new_state_dict)
- else:
- model.load_state_dict(new_state_dict)
- logger.info("Loaded checkpoint '{}' (iteration {})".format(
- checkpoint_path, iteration))
- return model, optimizer, learning_rate, iteration
-
-
-def plot_spectrogram_to_numpy(spectrogram):
- global MATPLOTLIB_FLAG
- if not MATPLOTLIB_FLAG:
- import matplotlib
- matplotlib.use("Agg")
- MATPLOTLIB_FLAG = True
- mpl_logger = logging.getLogger('matplotlib')
- mpl_logger.setLevel(logging.WARNING)
- import matplotlib.pylab as plt
- import numpy as np
-
- fig, ax = plt.subplots(figsize=(10, 2))
- im = ax.imshow(spectrogram, aspect="auto", origin="lower",
- interpolation='none')
- plt.colorbar(im, ax=ax)
- plt.xlabel("Frames")
- plt.ylabel("Channels")
- plt.tight_layout()
-
- fig.canvas.draw()
- data = np.fromstring(fig.canvas.tostring_rgb(), dtype=np.uint8, sep='')
- data = data.reshape(fig.canvas.get_width_height()[::-1] + (3,))
- plt.close()
- return data
-
-
-def plot_alignment_to_numpy(alignment, info=None):
- global MATPLOTLIB_FLAG
- if not MATPLOTLIB_FLAG:
- import matplotlib
- matplotlib.use("Agg")
- MATPLOTLIB_FLAG = True
- mpl_logger = logging.getLogger('matplotlib')
- mpl_logger.setLevel(logging.WARNING)
- import matplotlib.pylab as plt
- import numpy as np
-
- fig, ax = plt.subplots(figsize=(6, 4))
- im = ax.imshow(alignment.transpose(), aspect='auto', origin='lower',
- interpolation='none')
- fig.colorbar(im, ax=ax)
- xlabel = 'Decoder timestep'
- if info is not None:
- xlabel += '\n\n' + info
- plt.xlabel(xlabel)
- plt.ylabel('Encoder timestep')
- plt.tight_layout()
-
- fig.canvas.draw()
- data = np.fromstring(fig.canvas.tostring_rgb(), dtype=np.uint8, sep='')
- data = data.reshape(fig.canvas.get_width_height()[::-1] + (3,))
- plt.close()
- return data
-
-
-def load_wav_to_torch(full_path):
- sampling_rate, data = read(full_path)
- return torch.FloatTensor(data.astype(np.float32)), sampling_rate
-
-
-def load_filepaths_and_text(filename, split="|"):
- with open(filename, encoding='utf-8') as f:
- filepaths_and_text = [line.strip().split(split) for line in f]
- return filepaths_and_text
-
-
-def get_hparams(init=True):
- parser = argparse.ArgumentParser()
- parser.add_argument('-c', '--config', type=str, default="./configs/base.json",
- help='JSON file for configuration')
- parser.add_argument('-m', '--model', type=str, required=True,
- help='Model name')
-
- args = parser.parse_args()
- model_dir = os.path.join("./logs", args.model)
-
- if not os.path.exists(model_dir):
- os.makedirs(model_dir)
-
- config_path = args.config
- config_save_path = os.path.join(model_dir, "config.json")
- if init:
- with open(config_path, "r") as f:
- data = f.read()
- with open(config_save_path, "w") as f:
- f.write(data)
- else:
- with open(config_save_path, "r") as f:
- data = f.read()
- config = json.loads(data)
-
- hparams = HParams(**config)
- hparams.model_dir = model_dir
- return hparams
-
-
-def get_hparams_from_dir(model_dir):
- config_save_path = os.path.join(model_dir, "config.json")
- with open(config_save_path, "r") as f:
- data = f.read()
- config = json.loads(data)
-
- hparams = HParams(**config)
- hparams.model_dir = model_dir
- return hparams
-
-
-def get_hparams_from_file(config_path):
- with open(config_path, "r", encoding="utf-8") as f:
- data = f.read()
- config = json.loads(data)
-
- hparams = HParams(**config)
- return hparams
-
-
-def check_git_hash(model_dir):
- source_dir = os.path.dirname(os.path.realpath(__file__))
- if not os.path.exists(os.path.join(source_dir, ".git")):
- logger.warn("{} is not a git repository, therefore hash value comparison will be ignored.".format(
- source_dir
- ))
- return
-
- cur_hash = subprocess.getoutput("git rev-parse HEAD")
-
- path = os.path.join(model_dir, "githash")
- if os.path.exists(path):
- saved_hash = open(path).read()
- if saved_hash != cur_hash:
- logger.warn("git hash values are different. {}(saved) != {}(current)".format(
- saved_hash[:8], cur_hash[:8]))
- else:
- open(path, "w").write(cur_hash)
-
-
-def get_logger(model_dir, filename="train.log"):
- global logger
- logger = logging.getLogger(os.path.basename(model_dir))
- logger.setLevel(logging.DEBUG)
-
- formatter = logging.Formatter("%(asctime)s\t%(name)s\t%(levelname)s\t%(message)s")
- if not os.path.exists(model_dir):
- os.makedirs(model_dir)
- h = logging.FileHandler(os.path.join(model_dir, filename))
- h.setLevel(logging.DEBUG)
- h.setFormatter(formatter)
- logger.addHandler(h)
- return logger
-
-
-class HParams():
- def __init__(self, **kwargs):
- for k, v in kwargs.items():
- if type(v) == dict:
- v = HParams(**v)
- self[k] = v
-
- def keys(self):
- return self.__dict__.keys()
-
- def items(self):
- return self.__dict__.items()
-
- def values(self):
- return self.__dict__.values()
-
- def __len__(self):
- return len(self.__dict__)
-
- def __getitem__(self, key):
- return getattr(self, key)
-
- def __setitem__(self, key, value):
- return setattr(self, key, value)
-
- def __contains__(self, key):
- return key in self.__dict__
-
- def __repr__(self):
- return self.__dict__.__repr__()
diff --git a/spaces/Omnibus/Video-Diffusion-WebUI/video_diffusion/tuneavideo/models/unet.py b/spaces/Omnibus/Video-Diffusion-WebUI/video_diffusion/tuneavideo/models/unet.py
deleted file mode 100644
index d0cf2d5d1bd6605ea8cb0a86fd08d6466d5aba6f..0000000000000000000000000000000000000000
--- a/spaces/Omnibus/Video-Diffusion-WebUI/video_diffusion/tuneavideo/models/unet.py
+++ /dev/null
@@ -1,437 +0,0 @@
-# Adapted from https://github.com/huggingface/diffusers/blob/main/src/diffusers/models/unet_2d_condition.py
-
-import json
-import os
-from dataclasses import dataclass
-from typing import List, Optional, Tuple, Union
-
-import torch
-import torch.nn as nn
-import torch.utils.checkpoint
-from diffusers.configuration_utils import ConfigMixin, register_to_config
-from diffusers.models.embeddings import TimestepEmbedding, Timesteps
-from diffusers.models.modeling_utils import ModelMixin
-from diffusers.utils import BaseOutput, logging
-
-from .resnet import InflatedConv3d
-from .unet_blocks import (
- CrossAttnDownBlock3D,
- CrossAttnUpBlock3D,
- DownBlock3D,
- UNetMidBlock3DCrossAttn,
- UpBlock3D,
- get_down_block,
- get_up_block,
-)
-
-logger = logging.get_logger(__name__) # pylint: disable=invalid-name
-
-
-@dataclass
-class UNet3DConditionOutput(BaseOutput):
- sample: torch.FloatTensor
-
-
-class UNet3DConditionModel(ModelMixin, ConfigMixin):
- _supports_gradient_checkpointing = True
-
- @register_to_config
- def __init__(
- self,
- sample_size: Optional[int] = None,
- in_channels: int = 4,
- out_channels: int = 4,
- center_input_sample: bool = False,
- flip_sin_to_cos: bool = True,
- freq_shift: int = 0,
- down_block_types: Tuple[str] = (
- "CrossAttnDownBlock3D",
- "CrossAttnDownBlock3D",
- "CrossAttnDownBlock3D",
- "DownBlock3D",
- ),
- mid_block_type: str = "UNetMidBlock3DCrossAttn",
- up_block_types: Tuple[str] = ("UpBlock3D", "CrossAttnUpBlock3D", "CrossAttnUpBlock3D", "CrossAttnUpBlock3D"),
- only_cross_attention: Union[bool, Tuple[bool]] = False,
- block_out_channels: Tuple[int] = (320, 640, 1280, 1280),
- layers_per_block: int = 2,
- downsample_padding: int = 1,
- mid_block_scale_factor: float = 1,
- act_fn: str = "silu",
- norm_num_groups: int = 32,
- norm_eps: float = 1e-5,
- cross_attention_dim: int = 1280,
- attention_head_dim: Union[int, Tuple[int]] = 8,
- dual_cross_attention: bool = False,
- use_linear_projection: bool = False,
- class_embed_type: Optional[str] = None,
- num_class_embeds: Optional[int] = None,
- upcast_attention: bool = False,
- resnet_time_scale_shift: str = "default",
- ):
- super().__init__()
-
- self.sample_size = sample_size
- time_embed_dim = block_out_channels[0] * 4
-
- # input
- self.conv_in = InflatedConv3d(in_channels, block_out_channels[0], kernel_size=3, padding=(1, 1))
-
- # time
- self.time_proj = Timesteps(block_out_channels[0], flip_sin_to_cos, freq_shift)
- timestep_input_dim = block_out_channels[0]
-
- self.time_embedding = TimestepEmbedding(timestep_input_dim, time_embed_dim)
-
- # class embedding
- if class_embed_type is None and num_class_embeds is not None:
- self.class_embedding = nn.Embedding(num_class_embeds, time_embed_dim)
- elif class_embed_type == "timestep":
- self.class_embedding = TimestepEmbedding(timestep_input_dim, time_embed_dim)
- elif class_embed_type == "identity":
- self.class_embedding = nn.Identity(time_embed_dim, time_embed_dim)
- else:
- self.class_embedding = None
-
- self.down_blocks = nn.ModuleList([])
- self.mid_block = None
- self.up_blocks = nn.ModuleList([])
-
- if isinstance(only_cross_attention, bool):
- only_cross_attention = [only_cross_attention] * len(down_block_types)
-
- if isinstance(attention_head_dim, int):
- attention_head_dim = (attention_head_dim,) * len(down_block_types)
-
- # down
- output_channel = block_out_channels[0]
- for i, down_block_type in enumerate(down_block_types):
- input_channel = output_channel
- output_channel = block_out_channels[i]
- is_final_block = i == len(block_out_channels) - 1
-
- down_block = get_down_block(
- down_block_type,
- num_layers=layers_per_block,
- in_channels=input_channel,
- out_channels=output_channel,
- temb_channels=time_embed_dim,
- add_downsample=not is_final_block,
- resnet_eps=norm_eps,
- resnet_act_fn=act_fn,
- resnet_groups=norm_num_groups,
- cross_attention_dim=cross_attention_dim,
- attn_num_head_channels=attention_head_dim[i],
- downsample_padding=downsample_padding,
- dual_cross_attention=dual_cross_attention,
- use_linear_projection=use_linear_projection,
- only_cross_attention=only_cross_attention[i],
- upcast_attention=upcast_attention,
- resnet_time_scale_shift=resnet_time_scale_shift,
- )
- self.down_blocks.append(down_block)
-
- # mid
- if mid_block_type == "UNetMidBlock3DCrossAttn":
- self.mid_block = UNetMidBlock3DCrossAttn(
- in_channels=block_out_channels[-1],
- temb_channels=time_embed_dim,
- resnet_eps=norm_eps,
- resnet_act_fn=act_fn,
- output_scale_factor=mid_block_scale_factor,
- resnet_time_scale_shift=resnet_time_scale_shift,
- cross_attention_dim=cross_attention_dim,
- attn_num_head_channels=attention_head_dim[-1],
- resnet_groups=norm_num_groups,
- dual_cross_attention=dual_cross_attention,
- use_linear_projection=use_linear_projection,
- upcast_attention=upcast_attention,
- )
- else:
- raise ValueError(f"unknown mid_block_type : {mid_block_type}")
-
- # count how many layers upsample the videos
- self.num_upsamplers = 0
-
- # up
- reversed_block_out_channels = list(reversed(block_out_channels))
- reversed_attention_head_dim = list(reversed(attention_head_dim))
- only_cross_attention = list(reversed(only_cross_attention))
- output_channel = reversed_block_out_channels[0]
- for i, up_block_type in enumerate(up_block_types):
- is_final_block = i == len(block_out_channels) - 1
-
- prev_output_channel = output_channel
- output_channel = reversed_block_out_channels[i]
- input_channel = reversed_block_out_channels[min(i + 1, len(block_out_channels) - 1)]
-
- # add upsample block for all BUT final layer
- if not is_final_block:
- add_upsample = True
- self.num_upsamplers += 1
- else:
- add_upsample = False
-
- up_block = get_up_block(
- up_block_type,
- num_layers=layers_per_block + 1,
- in_channels=input_channel,
- out_channels=output_channel,
- prev_output_channel=prev_output_channel,
- temb_channels=time_embed_dim,
- add_upsample=add_upsample,
- resnet_eps=norm_eps,
- resnet_act_fn=act_fn,
- resnet_groups=norm_num_groups,
- cross_attention_dim=cross_attention_dim,
- attn_num_head_channels=reversed_attention_head_dim[i],
- dual_cross_attention=dual_cross_attention,
- use_linear_projection=use_linear_projection,
- only_cross_attention=only_cross_attention[i],
- upcast_attention=upcast_attention,
- resnet_time_scale_shift=resnet_time_scale_shift,
- )
- self.up_blocks.append(up_block)
- prev_output_channel = output_channel
-
- # out
- self.conv_norm_out = nn.GroupNorm(num_channels=block_out_channels[0], num_groups=norm_num_groups, eps=norm_eps)
- self.conv_act = nn.SiLU()
- self.conv_out = InflatedConv3d(block_out_channels[0], out_channels, kernel_size=3, padding=1)
-
- def set_attention_slice(self, slice_size):
- r"""
- Enable sliced attention computation.
-
- When this option is enabled, the attention module will split the input tensor in slices, to compute attention
- in several steps. This is useful to save some memory in exchange for a small speed decrease.
-
- Args:
- slice_size (`str` or `int` or `list(int)`, *optional*, defaults to `"auto"`):
- When `"auto"`, halves the input to the attention heads, so attention will be computed in two steps. If
- `"max"`, maxium amount of memory will be saved by running only one slice at a time. If a number is
- provided, uses as many slices as `attention_head_dim // slice_size`. In this case, `attention_head_dim`
- must be a multiple of `slice_size`.
- """
- sliceable_head_dims = []
-
- def fn_recursive_retrieve_slicable_dims(module: torch.nn.Module):
- if hasattr(module, "set_attention_slice"):
- sliceable_head_dims.append(module.sliceable_head_dim)
-
- for child in module.children():
- fn_recursive_retrieve_slicable_dims(child)
-
- # retrieve number of attention layers
- for module in self.children():
- fn_recursive_retrieve_slicable_dims(module)
-
- num_slicable_layers = len(sliceable_head_dims)
-
- if slice_size == "auto":
- # half the attention head size is usually a good trade-off between
- # speed and memory
- slice_size = [dim // 2 for dim in sliceable_head_dims]
- elif slice_size == "max":
- # make smallest slice possible
- slice_size = num_slicable_layers * [1]
-
- slice_size = num_slicable_layers * [slice_size] if not isinstance(slice_size, list) else slice_size
-
- if len(slice_size) != len(sliceable_head_dims):
- raise ValueError(
- f"You have provided {len(slice_size)}, but {self.config} has {len(sliceable_head_dims)} different"
- f" attention layers. Make sure to match `len(slice_size)` to be {len(sliceable_head_dims)}."
- )
-
- for i in range(len(slice_size)):
- size = slice_size[i]
- dim = sliceable_head_dims[i]
- if size is not None and size > dim:
- raise ValueError(f"size {size} has to be smaller or equal to {dim}.")
-
- # Recursively walk through all the children.
- # Any children which exposes the set_attention_slice method
- # gets the message
- def fn_recursive_set_attention_slice(module: torch.nn.Module, slice_size: List[int]):
- if hasattr(module, "set_attention_slice"):
- module.set_attention_slice(slice_size.pop())
-
- for child in module.children():
- fn_recursive_set_attention_slice(child, slice_size)
-
- reversed_slice_size = list(reversed(slice_size))
- for module in self.children():
- fn_recursive_set_attention_slice(module, reversed_slice_size)
-
- def _set_gradient_checkpointing(self, module, value=False):
- if isinstance(module, (CrossAttnDownBlock3D, DownBlock3D, CrossAttnUpBlock3D, UpBlock3D)):
- module.gradient_checkpointing = value
-
- def forward(
- self,
- sample: torch.FloatTensor,
- timestep: Union[torch.Tensor, float, int],
- encoder_hidden_states: torch.Tensor,
- class_labels: Optional[torch.Tensor] = None,
- attention_mask: Optional[torch.Tensor] = None,
- return_dict: bool = True,
- ) -> Union[UNet3DConditionOutput, Tuple]:
- r"""
- Args:
- sample (`torch.FloatTensor`): (batch, channel, height, width) noisy inputs tensor
- timestep (`torch.FloatTensor` or `float` or `int`): (batch) timesteps
- encoder_hidden_states (`torch.FloatTensor`): (batch, sequence_length, feature_dim) encoder hidden states
- return_dict (`bool`, *optional*, defaults to `True`):
- Whether or not to return a [`models.unet_2d_condition.UNet2DConditionOutput`] instead of a plain tuple.
-
- Returns:
- [`~models.unet_2d_condition.UNet2DConditionOutput`] or `tuple`:
- [`~models.unet_2d_condition.UNet2DConditionOutput`] if `return_dict` is True, otherwise a `tuple`. When
- returning a tuple, the first element is the sample tensor.
- """
- # By default samples have to be AT least a multiple of the overall upsampling factor.
- # The overall upsampling factor is equal to 2 ** (# num of upsampling layears).
- # However, the upsampling interpolation output size can be forced to fit any upsampling size
- # on the fly if necessary.
- default_overall_up_factor = 2**self.num_upsamplers
-
- # upsample size should be forwarded when sample is not a multiple of `default_overall_up_factor`
- forward_upsample_size = False
- upsample_size = None
-
- if any(s % default_overall_up_factor != 0 for s in sample.shape[-2:]):
- logger.info("Forward upsample size to force interpolation output size.")
- forward_upsample_size = True
-
- # prepare attention_mask
- if attention_mask is not None:
- attention_mask = (1 - attention_mask.to(sample.dtype)) * -10000.0
- attention_mask = attention_mask.unsqueeze(1)
-
- # center input if necessary
- if self.config.center_input_sample:
- sample = 2 * sample - 1.0
-
- # time
- timesteps = timestep
- if not torch.is_tensor(timesteps):
- # This would be a good case for the `match` statement (Python 3.10+)
- is_mps = sample.device.type == "mps"
- if isinstance(timestep, float):
- dtype = torch.float32 if is_mps else torch.float64
- else:
- dtype = torch.int32 if is_mps else torch.int64
- timesteps = torch.tensor([timesteps], dtype=dtype, device=sample.device)
- elif len(timesteps.shape) == 0:
- timesteps = timesteps[None].to(sample.device)
-
- # broadcast to batch dimension in a way that's compatible with ONNX/Core ML
- timesteps = timesteps.expand(sample.shape[0])
-
- t_emb = self.time_proj(timesteps)
-
- # timesteps does not contain any weights and will always return f32 tensors
- # but time_embedding might actually be running in fp16. so we need to cast here.
- # there might be better ways to encapsulate this.
- t_emb = t_emb.to(dtype=self.dtype)
- emb = self.time_embedding(t_emb)
-
- if self.class_embedding is not None:
- if class_labels is None:
- raise ValueError("class_labels should be provided when num_class_embeds > 0")
-
- if self.config.class_embed_type == "timestep":
- class_labels = self.time_proj(class_labels)
-
- class_emb = self.class_embedding(class_labels).to(dtype=self.dtype)
- emb = emb + class_emb
-
- # pre-process
- sample = self.conv_in(sample)
-
- # down
- down_block_res_samples = (sample,)
- for downsample_block in self.down_blocks:
- if hasattr(downsample_block, "has_cross_attention") and downsample_block.has_cross_attention:
- sample, res_samples = downsample_block(
- hidden_states=sample,
- temb=emb,
- encoder_hidden_states=encoder_hidden_states,
- attention_mask=attention_mask,
- )
- else:
- sample, res_samples = downsample_block(hidden_states=sample, temb=emb)
-
- down_block_res_samples += res_samples
-
- # mid
- sample = self.mid_block(sample, emb, encoder_hidden_states=encoder_hidden_states, attention_mask=attention_mask)
-
- # up
- for i, upsample_block in enumerate(self.up_blocks):
- is_final_block = i == len(self.up_blocks) - 1
-
- res_samples = down_block_res_samples[-len(upsample_block.resnets) :]
- down_block_res_samples = down_block_res_samples[: -len(upsample_block.resnets)]
-
- # if we have not reached the final block and need to forward the
- # upsample size, we do it here
- if not is_final_block and forward_upsample_size:
- upsample_size = down_block_res_samples[-1].shape[2:]
-
- if hasattr(upsample_block, "has_cross_attention") and upsample_block.has_cross_attention:
- sample = upsample_block(
- hidden_states=sample,
- temb=emb,
- res_hidden_states_tuple=res_samples,
- encoder_hidden_states=encoder_hidden_states,
- upsample_size=upsample_size,
- attention_mask=attention_mask,
- )
- else:
- sample = upsample_block(
- hidden_states=sample, temb=emb, res_hidden_states_tuple=res_samples, upsample_size=upsample_size
- )
- # post-process
- sample = self.conv_norm_out(sample)
- sample = self.conv_act(sample)
- sample = self.conv_out(sample)
-
- if not return_dict:
- return (sample,)
-
- return UNet3DConditionOutput(sample=sample)
-
- @classmethod
- def from_pretrained_2d(cls, pretrained_model_path, subfolder=None):
- if subfolder is not None:
- pretrained_model_path = os.path.join(pretrained_model_path, subfolder)
-
- config_file = os.path.join(pretrained_model_path, "config.json")
- if not os.path.isfile(config_file):
- raise RuntimeError(f"{config_file} does not exist")
- with open(config_file, "r") as f:
- config = json.load(f)
- config["_class_name"] = cls.__name__
- config["down_block_types"] = [
- "CrossAttnDownBlock3D",
- "CrossAttnDownBlock3D",
- "CrossAttnDownBlock3D",
- "DownBlock3D",
- ]
- config["up_block_types"] = ["UpBlock3D", "CrossAttnUpBlock3D", "CrossAttnUpBlock3D", "CrossAttnUpBlock3D"]
-
- from diffusers.utils import WEIGHTS_NAME
-
- model = cls.from_config(config)
- model_file = os.path.join(pretrained_model_path, WEIGHTS_NAME)
- if not os.path.isfile(model_file):
- raise RuntimeError(f"{model_file} does not exist")
- state_dict = torch.load(model_file, map_location="cpu")
- for k, v in model.state_dict().items():
- if "_temp." in k:
- state_dict.update({k: v})
- model.load_state_dict(state_dict)
-
- return model
diff --git a/spaces/OpenGVLab/InternGPT/iGPT/models/grit_src/grit/modeling/text/text_decoder.py b/spaces/OpenGVLab/InternGPT/iGPT/models/grit_src/grit/modeling/text/text_decoder.py
deleted file mode 100644
index 071baa7a52d21d7132cc492f070cba066d17aa43..0000000000000000000000000000000000000000
--- a/spaces/OpenGVLab/InternGPT/iGPT/models/grit_src/grit/modeling/text/text_decoder.py
+++ /dev/null
@@ -1,672 +0,0 @@
-# Modified by Jialian Wu from
-# https://github.com/microsoft/GenerativeImage2Text/blob/main/generativeimage2text/layers/decoder.py
-# and https://github.com/kdexd/virtex
-from torch import nn
-import torch
-import functools
-from torch.nn import functional as F
-import warnings
-
-
-class TextualHead(nn.Module):
- def __init__(self,
- visual_feature_size: int, vocab_size: int, hidden_size: int):
- super().__init__()
- self.visual_feature_size = visual_feature_size
- self.vocab_size = vocab_size
- self.hidden_size = hidden_size
-
- @property
- def textual_feature_size(self):
- return self.hidden_size
-
-
-class WordAndPositionalEmbedding(nn.Module):
- def __init__(
- self,
- vocab_size: int,
- hidden_size: int,
- dropout: float = 0.0,
- max_caption_length: int = 30,
- padding_idx: int = 0,
- ):
- super().__init__()
- self.vocab_size = vocab_size
- self.padding_idx = padding_idx
-
- #self.words = nn.Embedding(vocab_size, hidden_size, padding_idx=padding_idx)
- self.words = nn.Embedding(vocab_size, hidden_size)
-
- # We provide no "padding index" for positional embeddings. We zero out
- # the positional embeddings of padded positions as a post-processing.
- self.positions = nn.Embedding(max_caption_length, hidden_size)
- self.layer_norm = nn.LayerNorm(
- hidden_size, eps=1e-8, elementwise_affine=True
- )
- self.dropout = nn.Dropout(p=dropout)
-
- def forward(self, tokens: torch.Tensor):
- position_indices = self._create_position_indices(tokens)
-
- # shape: (batch_size, max_caption_length, hidden_size)
- word_embeddings = self.words(tokens)
- position_embeddings = self.positions(position_indices)
-
- # shape: (batch_size, max_caption_length, hidden_size)
- embeddings = self.layer_norm(word_embeddings + position_embeddings)
- embeddings = self.dropout(embeddings)
-
- return embeddings
-
- @functools.lru_cache(maxsize=128)
- def _create_position_indices(self, tokens: torch.Tensor):
-
- # Create position indices of the same size as token indices.
- batch_size, max_caption_length = tokens.size()
- positions = torch.arange(
- max_caption_length, dtype=tokens.dtype, device=tokens.device
- )
- # shape: (batch_size, max_caption_length)
- positions = positions.unsqueeze(0).expand(batch_size, max_caption_length)
- return positions
-
-
-class BertEncoderAsDecoder(nn.Module):
- def __init__(self, encoder):
- super().__init__()
- self.encoder = encoder
-
- def forward(self, tgt, memory,
- tgt_mask=None,
- tgt_key_padding_mask=None,
- memory_key_padding_mask=None,
- tgt_bi_valid_mask=None,
- encoder_history_states=None,
- ):
- assert tgt_key_padding_mask is None, 'not supported'
- assert tgt_mask.dim() == 2
- assert tgt_mask.shape[0] == tgt_mask.shape[1]
- # tgt_mask should always be 0/negative infinity
- tgt = tgt.transpose(0, 1)
- memory = memory.transpose(0, 1)
-
- hidden_states = torch.cat((memory, tgt), dim=1)
- num_tgt = tgt.shape[1]
- num_memory = memory.shape[1]
- device = tgt.device
- dtype = tgt.dtype
- top_left = torch.zeros((num_memory, num_memory), device=device, dtype=dtype)
- top_right = torch.full((num_memory, num_tgt), float('-inf'), device=tgt.device, dtype=dtype,)
- bottom_left = torch.zeros((num_tgt, num_memory), dtype=dtype, device=tgt_mask.device,)
- left = torch.cat((top_left, bottom_left), dim=0)
- right = torch.cat((top_right, tgt_mask.to(dtype)), dim=0)
-
- full_attention_mask = torch.cat((left, right), dim=1)[None, :]
-
- if memory_key_padding_mask is None:
- memory_key_padding_mask = torch.full((memory.shape[0], memory.shape[1]), fill_value=False, device=device)
- # if it is False, it means valid. That is, it is not a padding
- assert memory_key_padding_mask.dtype == torch.bool
- zero_negative_infinity = torch.zeros_like(memory_key_padding_mask, dtype=tgt.dtype)
- zero_negative_infinity[memory_key_padding_mask] = float('-inf')
- full_attention_mask = full_attention_mask.expand((memory_key_padding_mask.shape[0], num_memory + num_tgt, num_memory + num_tgt))
- full_attention_mask = full_attention_mask.clone()
- origin_left = full_attention_mask[:, :, :num_memory]
- update = zero_negative_infinity[:, None, :]
- full_attention_mask[:, :, :num_memory] = origin_left + update
-
- if tgt_bi_valid_mask is not None:
- # verify the correctness
- bs = full_attention_mask.shape[0]
- # during inference, tgt_bi_valid_mask's length is not changed, but
- # num_tgt can be increased
- max_valid_target = tgt_bi_valid_mask.shape[1]
- mask = tgt_bi_valid_mask[:, None, :].expand((bs, num_memory+num_tgt, max_valid_target))
- full_attention_mask[:, :, num_memory:(num_memory+max_valid_target)][mask] = 0
-
- # add axis for multi-head
- full_attention_mask = full_attention_mask[:, None, :, :]
-
- if encoder_history_states is None:
- result = self.encoder(
- hidden_states=hidden_states,
- attention_mask=full_attention_mask,
- encoder_history_states=encoder_history_states,
- )
- result = list(result)
- result[0] = result[0][:, num_memory:].transpose(0, 1)
- if self.encoder.output_hidden_states:
- return result[0], result[1]
- else:
- # make it back-compatible
- return result[0]
- else:
- encoder_out = self.encoder(
- hidden_states=hidden_states[:, -1:],
- attention_mask=full_attention_mask[:, :, -1:],
- encoder_history_states=encoder_history_states,
- )
- result = encoder_out[0].transpose(0, 1)
- if self.encoder.output_hidden_states:
- return result, encoder_out[1]
- else:
- return result
-
-
-def create_transformer(decoder_type, norm_type,
- textual_feature_size,
- attention_heads,
- feedforward_size,
- dropout,
- num_layers,
- output_hidden_states=False,
- use_mlp_wrapper=None,
- use_act_checkpoint=True,
- ):
- assert norm_type in ['post', 'pre']
- if decoder_type is None:
- LayerClass = (
- nn.TransformerDecoderLayer
- if norm_type == "post"
- else PreNormTransformerDecoderLayer
- )
- _layer = LayerClass(
- textual_feature_size,
- attention_heads,
- dim_feedforward=feedforward_size,
- dropout=dropout,
- activation="gelu",
- )
- return nn.TransformerDecoder(_layer, num_layers)
- elif decoder_type == 'bert_en':
- from .modeling_bert import BertConfig, BertEncoder
- config = BertConfig(
- vocab_size_or_config_json_file=30522,
- hidden_size=textual_feature_size,
- num_hidden_layers=num_layers,
- num_attention_heads=attention_heads,
- intermediate_size=feedforward_size,
- hidden_act="gelu",
- hidden_dropout_prob=0.1,
- attention_probs_dropout_prob=0.1,
- layer_norm_eps=1e-12,
- )
- config.pre_norm = (norm_type == 'pre')
- config.use_mlp_wrapper = use_mlp_wrapper
- config.output_hidden_states = output_hidden_states
- encoder = BertEncoder(config, use_act_checkpoint=use_act_checkpoint)
- return BertEncoderAsDecoder(encoder)
-
-
-class PreNormTransformerDecoderLayer(nn.TransformerDecoderLayer):
- def forward(self, tgt, memory, tgt_mask=None, memory_mask=None,
- tgt_key_padding_mask=None, memory_key_padding_mask=None):
- # fmt: off
- # We use the members (modules) from super-class, just the order of
- # operations is changed here. First layernorm, then attention.
- tgt2 = self.norm1(tgt)
- tgt2, _ = self.self_attn(
- tgt2, tgt2, tgt2, attn_mask=tgt_mask,
- key_padding_mask=tgt_key_padding_mask
- )
- tgt = tgt + self.dropout1(tgt2)
-
- # Layernorm first, then decoder attention.
- tgt2 = self.norm2(tgt)
- tgt2, _ = self.multihead_attn(
- tgt2, memory, memory, attn_mask=memory_mask,
- key_padding_mask=memory_key_padding_mask
- )
- tgt = tgt + self.dropout2(tgt2)
-
- # Layernorm first, then transformation through feedforward network.
- tgt2 = self.norm3(tgt)
- tgt2 = self.linear2(self.dropout(self.activation(self.linear1(tgt2))))
- tgt = tgt + self.dropout3(tgt2)
- return tgt
-
-
-class TransformerDecoderTextualHead(TextualHead):
- def __init__(
- self,
- object_feature_size: int,
- vocab_size: int,
- hidden_size: int,
- num_layers: int,
- attention_heads: int,
- feedforward_size: int,
- dropout: float = 0.1,
- norm_type: str = "post",
- mask_future_positions: bool = True,
- max_caption_length: int = 1024,
- padding_idx: int = 0,
- decoder_type=None,
- not_tie_weight=None,
- output_hidden_states=None,
- use_mlp_wrapper=None,
- use_act_checkpoint=True,
- ):
- super().__init__(object_feature_size, vocab_size, hidden_size)
- self.num_layers = num_layers
- self.attention_heads = attention_heads
- self.feedforward_size = feedforward_size
- self.dropout = dropout
- assert mask_future_positions
- self.padding_idx = padding_idx
-
- self.object_feature_projection = nn.Sequential(
- nn.Linear(object_feature_size, self.textual_feature_size),
- nn.LayerNorm(self.textual_feature_size))
-
- self.embedding = WordAndPositionalEmbedding(
- self.vocab_size,
- self.textual_feature_size,
- dropout=dropout,
- max_caption_length=max_caption_length,
- padding_idx=padding_idx,
- )
- self.transformer = create_transformer(
- decoder_type=decoder_type,
- norm_type=norm_type,
- textual_feature_size=self.textual_feature_size,
- attention_heads=self.attention_heads,
- feedforward_size=self.feedforward_size,
- dropout=dropout,
- num_layers=self.num_layers,
- output_hidden_states=output_hidden_states,
- use_mlp_wrapper=use_mlp_wrapper,
- use_act_checkpoint=use_act_checkpoint,
- )
- self.apply(self._init_weights)
-
- # Create an output linear layer and tie the input and output word
- # embeddings to reduce parametejs.
- self.output = nn.Linear(self.textual_feature_size, vocab_size)
- if not not_tie_weight:
- self.output.weight = self.embedding.words.weight
-
- @staticmethod
- def _init_weights(module):
- """Initialize weights like BERT - N(0.0, 0.02), bias = 0."""
-
- if isinstance(module, nn.Linear):
- module.weight.data.normal_(mean=0.0, std=0.02)
- elif isinstance(module, nn.MultiheadAttention):
- module.in_proj_weight.data.normal_(mean=0.0, std=0.02)
- module.out_proj.weight.data.normal_(mean=0.0, std=0.02)
- elif isinstance(module, nn.Embedding):
- module.weight.data.normal_(mean=0.0, std=0.02)
- if module.padding_idx is not None:
- module.weight.data[module.padding_idx].zero_()
-
- def forward(
- self,
- hidden_states,
- text_tokens,
- ):
- projected_object_features = self.object_feature_projection(hidden_states) if hidden_states is not None else None
- batch_size, max_text_length = text_tokens.size()
- text_embeddings = self.embedding(text_tokens)
-
- # An additive mask for masking the future (one direction).
- uni_mask_zero_neg = self._generate_future_mask(
- max_text_length, text_embeddings.dtype, text_embeddings.device
- )
-
- # We transpose the first two dimensions of tokens embeddings and visual
- # features, as required by decoder.
- text_embeddings = text_embeddings.transpose(0, 1)
-
- projected_object_features = projected_object_features.transpose(0, 1)
-
- # if transformer here is the pytorch/decoder, there is no chance, the
- # output is always tensor
- trans_out = self.transformer(
- text_embeddings,
- projected_object_features,
- tgt_mask=uni_mask_zero_neg,
- )
- if isinstance(trans_out, tuple):
- textual_features = trans_out[0]
- else:
- assert isinstance(trans_out, torch.Tensor)
- textual_features = trans_out
- # Undo the transpose and bring batch to dim 0.
- # shape: (batch_size, max_caption_length, hidden_size)
- textual_features = textual_features.transpose(0, 1)
-
- # shape: (batch_size, max_caption_length, vocab_size)
- output_logits = self.output(textual_features)
- if isinstance(trans_out, tuple):
- return output_logits, trans_out[1]
- else:
- return output_logits
-
- def _generate_future_mask(
- self, size: int, dtype: torch.dtype, device: torch.device
- ):
- # Default mask is for forward direction. Flip for backward direction.
- mask = torch.triu(
- torch.ones(size, size, device=device, dtype=dtype), diagonal=1
- )
- mask = mask.masked_fill(mask == 1, float("-inf"))
- return mask
-
-
-class AutoRegressiveBeamSearch(object):
- def __init__(
- self,
- end_token_id: int,
- max_steps: int = 50,
- beam_size: int = 5,
- objectdet=True,
- per_node_beam_size: int = 2,
- ):
- self._eos_index = end_token_id
- self.max_steps = max_steps
- self.beam_size = beam_size
- self.objectdet = objectdet
- self.per_node_beam_size = per_node_beam_size or beam_size
-
- def search(self, begin_tokens, step):
- if self.beam_size > 1 and self.objectdet:
- only_return_best = False
- else:
- only_return_best = True
-
- batch_size = begin_tokens.size()[0]
-
- predictions = begin_tokens.unsqueeze(1).expand((batch_size, self.beam_size, begin_tokens.shape[-1]))
- # Calculate the first timestep. This is done outside the main loop
- # because we are going from a single decoder input (the output from the
- # encoder) to the top `beam_size` decoder outputs. On the other hand,
- # within the main loop we are going from the `beam_size` elements of the
- # beam to `beam_size`^2 candidates from which we will select the top
- # `beam_size` elements for the next iteration.
- # shape: (batch_size, num_classes)
- start_class_logits = step(begin_tokens)
-
- # Convert logits to logprobs.
- # shape: (batch_size * beam_size, vocab_size)
- start_class_logprobs = F.log_softmax(start_class_logits, dim=1)
-
- num_classes = start_class_logprobs.size()[1]
-
- # shape: (batch_size, beam_size), (batch_size, beam_size)
- start_top_logprobs, start_predicted_classes = start_class_logprobs.topk(
- self.beam_size
- )
-
- if (
- self.beam_size == 1
- and (start_predicted_classes == self._eos_index).all()
- ):
- warnings.warn(
- "Empty object description predicted. You may want to increase beam"
- "size or ensure your step function is working properly.",
- RuntimeWarning,
- )
- if only_return_best:
- return start_predicted_classes, start_top_logprobs
- else:
- return start_predicted_classes.unsqueeze(-1), start_top_logprobs
-
- # The log probs for the last time step.
- # shape: (batch_size, beam_size)
- last_logprobs = start_top_logprobs
-
- # shape: (batch_size, beam_size, sequence_length)
- predictions = torch.cat([predictions, start_predicted_classes.unsqueeze(-1)], dim=-1)
-
- # Log probability tensor that mandates that the end token is selected.
- # shape: (batch_size * beam_size, num_classes)
- logprobs_after_end = start_class_logprobs.new_full(
- (batch_size * self.beam_size, num_classes), float("-inf")
- )
- logprobs_after_end[:, self._eos_index] = 0.0
-
- logits_after_end = start_class_logprobs.new_full(
- (batch_size * self.beam_size, num_classes), float("-inf")
- )
- logits_after_end[:, self._eos_index] = 0
-
- while predictions.shape[-1] < self.max_steps:
- # shape: (batch_size * beam_size,)
- last_predictions = predictions[:, :, -1].reshape(batch_size * self.beam_size)
-
- # If every predicted token from the last step is `self._eos_index`,
- # then we can stop early.
- if (last_predictions == self._eos_index).all():
- break
-
- predictions_so_far = predictions.view(
- batch_size * self.beam_size, -1
- )
- # shape: (batch_size * beam_size, num_classes)
- class_logits = step(predictions_so_far)
-
- # Set logprobs of last predicted tokens as high negative value to avoid
- # repetition in description.
- class_logits = class_logits.scatter(1, predictions_so_far[:, -1].view((-1, 1)), -10000)
-
- # shape: (batch_size * beam_size, num_classes)
- last_predictions_expanded = last_predictions.unsqueeze(-1).expand(
- batch_size * self.beam_size, num_classes
- )
-
- # Here we are finding any beams where we predicted the end token in
- # the previous timestep and replacing the distribution with a
- # one-hot distribution, forcing the beam to predict the end token
- # this timestep as well.
- class_logits = torch.where(
- last_predictions_expanded == self._eos_index,
- logits_after_end,
- class_logits,
- )
-
- # Convert logits to logprobs.
- # shape: (batch_size * beam_size, vocab_size)
- class_logprobs = F.log_softmax(class_logits, dim=1)
-
- # shape (both): (batch_size * beam_size, per_node_beam_size)
- top_logprobs, predicted_classes = class_logprobs.topk(
- self.per_node_beam_size
- )
-
- # Here we expand the last log probs to `(batch_size * beam_size,
- # per_node_beam_size)` so that we can add them to the current log
- # probs for this timestep. This lets us maintain the log
- # probability of each element on the beam.
- # shape: (batch_size * beam_size, per_node_beam_size)
- expanded_last_logprobs = (
- last_logprobs.unsqueeze(2)
- .expand(batch_size, self.beam_size, self.per_node_beam_size)
- .reshape(batch_size * self.beam_size, self.per_node_beam_size)
- )
- # shape: (batch_size * beam_size, per_node_beam_size)
- summed_top_logprobs = top_logprobs + expanded_last_logprobs
-
- # shape: (batch_size, beam_size * per_node_beam_size)
- reshaped_summed = summed_top_logprobs.reshape(
- batch_size, self.beam_size * self.per_node_beam_size
- )
- # shape: (batch_size, beam_size * per_node_beam_size)
- reshaped_predicted_classes = predicted_classes.reshape(
- batch_size, self.beam_size * self.per_node_beam_size
- )
- # Append the predictions to the current beam.
- reshaped_beam = (
- predictions.view(batch_size * self.beam_size, 1, -1)
- .repeat(1, self.per_node_beam_size, 1)
- .reshape(batch_size, self.beam_size * self.per_node_beam_size, -1)
- )
- # batch_size, (beam_size * per_node_beach_size), #token
- reshaped_beam = torch.cat([reshaped_beam, reshaped_predicted_classes.unsqueeze(-1)], dim=-1)
-
- # Keep only the top `beam_size` beam indices.
- # shape: (batch_size, beam_size), (batch_size, beam_size)
- restricted_beam_logprobs, restricted_beam_indices = reshaped_summed.topk(
- self.beam_size
- )
- predictions = reshaped_beam.gather(
- 1, restricted_beam_indices.unsqueeze(-1).repeat(1,1,reshaped_beam.shape[-1])
- )
-
- # shape: (batch_size, beam_size)
- last_logprobs = restricted_beam_logprobs
-
- if not torch.isfinite(last_logprobs).all():
- warnings.warn(
- "Infinite log probs encountered. Some final descriptions may not "
- "make sense. This can happen when the beam size is larger than"
- " the number of valid (non-zero probability) transitions that "
- "the step function produces.",
- RuntimeWarning,
- )
-
- # Optionally select best beam and its logprobs.
- if only_return_best:
- # shape: (batch_size, sequence_length)
- predictions = predictions[:, 0, :]
- last_logprobs = last_logprobs[:, 0]
- num_valid = (predictions != self._eos_index).sum(dim=-1)
- num_valid += (predictions == self._eos_index).sum(dim=-1) > 0
- num_valid = num_valid - begin_tokens.shape[1]
- num_valid = num_valid.clip(min=1)
-
- last_logprobs = last_logprobs / num_valid
-
- return predictions, last_logprobs
-
-
-class GRiTTextDecoder(nn.Module):
- def __init__(
- self,
- transformer,
- begin_token_id=101,
- beamsearch_decode=None,
- loss_type=None,
- tokenizer=None,
- ):
- super().__init__()
- self.textual = transformer
- self.padding_idx = self.textual.padding_idx
-
- self.begin_token_id = begin_token_id
- self.beamsearch_decode = beamsearch_decode
- self.tokenizer = tokenizer
-
- if loss_type is None:
- self.loss = nn.CrossEntropyLoss(ignore_index=self.padding_idx)
- elif loss_type == 'smooth':
- self.loss = SmoothLabelCrossEntropyLoss(ignore_index=self.padding_idx)
- else:
- raise NotImplementedError(loss_type)
-
- def forward(self, batch):
- object_features = batch['object_features']
-
- if self.training:
- caption_token_input = batch["text_tokens"]
-
- output_logits = self.textual(
- object_features,
- caption_token_input,
- )
-
- if 'need_predict' in batch:
- # in place should also be good, but we do not choose that for
- # safety as we may use it in prediction results in future
- target = batch["text_tokens"].clone()
- target[batch['need_predict'] == 0] = self.padding_idx
- else:
- target = batch["text_tokens"]
-
- feat = output_logits[:, :-1].contiguous()
- target = target[:, 1:].contiguous()
- feat = feat.view(-1, self.textual.vocab_size)
- target = target.view(-1)
-
- valid_mask = target != self.padding_idx
- target = target[valid_mask]
- feat = feat[valid_mask]
- loss = self.loss(feat, target)
-
- return loss
- else:
- output_dict = self.infer(object_features)
- return output_dict
-
- def infer(self, object_features):
- batch_size = object_features.size(0)
- begin_tokens = object_features.new_full(
- (batch_size, 1), self.begin_token_id
- ).long()
-
- decoding_step = functools.partial(
- self.decoding_step, object_features
- )
-
- object_description_tokens, logprobs = self.beamsearch_decode.search(
- begin_tokens, decoding_step
- )
-
- output_dict = {
- 'predictions': object_description_tokens,
- 'logprobs': logprobs,
- }
-
- return output_dict
-
- def decoding_step(self, object_features, partial_text):
- batch_size = object_features.shape[0]
- beam_size = int(partial_text.size(0) / batch_size)
- if beam_size > 1:
- batch_size, num_token, channels = object_features.size()
- object_features = object_features.unsqueeze(1).repeat(1, beam_size, 1, 1)
- object_features = object_features.view(
- batch_size * beam_size, num_token, channels
- )
-
- text_lengths = torch.ones_like(partial_text)
- if len(text_lengths.size()) != 2:
- partial_text = partial_text.unsqueeze(1)
-
- # shape: (batch_size * beam_size, partial_caption_length, vocab_size)
- logits = self.textual(
- object_features,
- partial_text,
- )
-
- return logits[:, -1, :].float()
-
-
-class SmoothLabelCrossEntropyLoss(nn.Module):
- def __init__(self, eps=0.1, log_prefix='', ignore_index=None):
- super().__init__()
- self.eps = eps
- self.log_soft = nn.LogSoftmax(dim=1)
- self.kl = nn.KLDivLoss(reduction='none')
-
- self.iter = 0
- self.max_loss = 0
- self.min_loss = 0
- self.log_prefix = log_prefix
- self.ignore_index = ignore_index
-
- def forward(self, feature, target):
- feature = feature.float()
- if self.ignore_index is not None:
- valid_mask = target != self.ignore_index
- target = target[valid_mask]
- feature = feature[valid_mask]
- assert target.numel() > 0
- self.iter += 1
- eps = self.eps
- n_class = feature.size(1)
- one_hot = torch.zeros_like(feature).scatter(1, target.view(-1, 1), 1)
- one_hot = one_hot * (1 - eps) + (1 - one_hot) * eps / (n_class - 1)
- log_prb = self.log_soft(feature)
- loss = self.kl(log_prb, one_hot)
- return loss.sum(dim=1).mean()
-
diff --git a/spaces/OpenGVLab/InternGPT/iGPT/models/grit_src/third_party/CenterNet2/tests/test_engine.py b/spaces/OpenGVLab/InternGPT/iGPT/models/grit_src/third_party/CenterNet2/tests/test_engine.py
deleted file mode 100644
index 6f6a0997d2a670e40e26286b258773ae56536a87..0000000000000000000000000000000000000000
--- a/spaces/OpenGVLab/InternGPT/iGPT/models/grit_src/third_party/CenterNet2/tests/test_engine.py
+++ /dev/null
@@ -1,186 +0,0 @@
-# Copyright (c) Facebook, Inc. and its affiliates.
-
-import json
-import math
-import os
-import tempfile
-import time
-import unittest
-from unittest import mock
-import torch
-from fvcore.common.checkpoint import Checkpointer
-from torch import nn
-
-from detectron2 import model_zoo
-from detectron2.config import configurable, get_cfg
-from detectron2.engine import DefaultTrainer, SimpleTrainer, default_setup, hooks
-from detectron2.modeling.meta_arch import META_ARCH_REGISTRY
-from detectron2.utils.events import CommonMetricPrinter, JSONWriter
-
-
-@META_ARCH_REGISTRY.register()
-class _SimpleModel(nn.Module):
- @configurable
- def __init__(self, sleep_sec=0):
- super().__init__()
- self.mod = nn.Linear(10, 20)
- self.sleep_sec = sleep_sec
-
- @classmethod
- def from_config(cls, cfg):
- return {}
-
- def forward(self, x):
- if self.sleep_sec > 0:
- time.sleep(self.sleep_sec)
- return {"loss": x.sum() + sum([x.mean() for x in self.parameters()])}
-
-
-class TestTrainer(unittest.TestCase):
- def _data_loader(self, device):
- device = torch.device(device)
- while True:
- yield torch.rand(3, 3).to(device)
-
- def test_simple_trainer(self, device="cpu"):
- model = _SimpleModel().to(device=device)
- trainer = SimpleTrainer(
- model, self._data_loader(device), torch.optim.SGD(model.parameters(), 0.1)
- )
- trainer.train(0, 10)
-
- @unittest.skipIf(not torch.cuda.is_available(), "CUDA not available")
- def test_simple_trainer_cuda(self):
- self.test_simple_trainer(device="cuda")
-
- def test_writer_hooks(self):
- model = _SimpleModel(sleep_sec=0.1)
- trainer = SimpleTrainer(
- model, self._data_loader("cpu"), torch.optim.SGD(model.parameters(), 0.1)
- )
-
- max_iter = 50
-
- with tempfile.TemporaryDirectory(prefix="detectron2_test") as d:
- json_file = os.path.join(d, "metrics.json")
- writers = [CommonMetricPrinter(max_iter), JSONWriter(json_file)]
-
- trainer.register_hooks(
- [hooks.EvalHook(0, lambda: {"metric": 100}), hooks.PeriodicWriter(writers)]
- )
- with self.assertLogs(writers[0].logger) as logs:
- trainer.train(0, max_iter)
-
- with open(json_file, "r") as f:
- data = [json.loads(line.strip()) for line in f]
- self.assertEqual([x["iteration"] for x in data], [19, 39, 49, 50])
- # the eval metric is in the last line with iter 50
- self.assertIn("metric", data[-1], "Eval metric must be in last line of JSON!")
-
- # test logged messages from CommonMetricPrinter
- self.assertEqual(len(logs.output), 3)
- for log, iter in zip(logs.output, [19, 39, 49]):
- self.assertIn(f"iter: {iter}", log)
-
- self.assertIn("eta: 0:00:00", logs.output[-1], "Last ETA must be 0!")
-
- def test_default_trainer(self):
- # TODO: this test requires manifold access, so changed device to CPU. see: T88318502
- cfg = get_cfg()
- cfg.MODEL.DEVICE = "cpu"
- cfg.MODEL.META_ARCHITECTURE = "_SimpleModel"
- cfg.DATASETS.TRAIN = ("coco_2017_val_100",)
- with tempfile.TemporaryDirectory(prefix="detectron2_test") as d:
- cfg.OUTPUT_DIR = d
- trainer = DefaultTrainer(cfg)
-
- # test property
- self.assertIs(trainer.model, trainer._trainer.model)
- trainer.model = _SimpleModel()
- self.assertIs(trainer.model, trainer._trainer.model)
-
- def test_checkpoint_resume(self):
- model = _SimpleModel()
- dataloader = self._data_loader("cpu")
- opt = torch.optim.SGD(model.parameters(), 0.1)
- scheduler = torch.optim.lr_scheduler.StepLR(opt, 3)
-
- with tempfile.TemporaryDirectory(prefix="detectron2_test") as d:
- trainer = SimpleTrainer(model, dataloader, opt)
- checkpointer = Checkpointer(model, d, opt=opt, trainer=trainer)
-
- trainer.register_hooks(
- [
- hooks.LRScheduler(scheduler=scheduler),
- # checkpoint after scheduler to properly save the state of scheduler
- hooks.PeriodicCheckpointer(checkpointer, 10),
- ]
- )
-
- trainer.train(0, 12)
- self.assertAlmostEqual(opt.param_groups[0]["lr"], 1e-5)
- self.assertEqual(scheduler.last_epoch, 12)
- del trainer
-
- opt = torch.optim.SGD(model.parameters(), 999) # lr will be loaded
- trainer = SimpleTrainer(model, dataloader, opt)
- scheduler = torch.optim.lr_scheduler.StepLR(opt, 3)
- trainer.register_hooks(
- [
- hooks.LRScheduler(scheduler=scheduler),
- ]
- )
- checkpointer = Checkpointer(model, d, opt=opt, trainer=trainer)
- checkpointer.resume_or_load("non_exist.pth")
- self.assertEqual(trainer.iter, 11) # last finished iter number (0-based in Trainer)
- # number of times `scheduler.step()` was called (1-based)
- self.assertEqual(scheduler.last_epoch, 12)
- self.assertAlmostEqual(opt.param_groups[0]["lr"], 1e-5)
-
- def test_eval_hook(self):
- model = _SimpleModel()
- dataloader = self._data_loader("cpu")
- opt = torch.optim.SGD(model.parameters(), 0.1)
-
- for total_iter, period, eval_count in [(30, 15, 2), (31, 15, 3), (20, 0, 1)]:
- test_func = mock.Mock(return_value={"metric": 3.0})
- trainer = SimpleTrainer(model, dataloader, opt)
- trainer.register_hooks([hooks.EvalHook(period, test_func)])
- trainer.train(0, total_iter)
- self.assertEqual(test_func.call_count, eval_count)
-
- def test_best_checkpointer(self):
- model = _SimpleModel()
- dataloader = self._data_loader("cpu")
- opt = torch.optim.SGD(model.parameters(), 0.1)
- metric_name = "metric"
- total_iter = 40
- test_period = 10
- test_cases = [
- ("max", iter([0.3, 0.4, 0.35, 0.5]), 3),
- ("min", iter([1.0, 0.8, 0.9, 0.9]), 2),
- ("min", iter([math.nan, 0.8, 0.9, 0.9]), 1),
- ]
- for mode, metrics, call_count in test_cases:
- trainer = SimpleTrainer(model, dataloader, opt)
- with tempfile.TemporaryDirectory(prefix="detectron2_test") as d:
- checkpointer = Checkpointer(model, d, opt=opt, trainer=trainer)
- trainer.register_hooks(
- [
- hooks.EvalHook(test_period, lambda: {metric_name: next(metrics)}),
- hooks.BestCheckpointer(test_period, checkpointer, metric_name, mode=mode),
- ]
- )
- with mock.patch.object(checkpointer, "save") as mock_save_method:
- trainer.train(0, total_iter)
- self.assertEqual(mock_save_method.call_count, call_count)
-
- def test_setup_config(self):
- with tempfile.TemporaryDirectory(prefix="detectron2_test") as d:
- cfg = get_cfg()
- cfg.OUTPUT_DIR = os.path.join(d, "yacs")
- default_setup(cfg, {})
-
- cfg = model_zoo.get_config("COCO-InstanceSegmentation/mask_rcnn_R_50_FPN_1x.py")
- cfg.train.output_dir = os.path.join(d, "omegaconf")
- default_setup(cfg, {})
diff --git a/spaces/OpenMotionLab/MotionGPT/pyrender/tests/unit/test_lights.py b/spaces/OpenMotionLab/MotionGPT/pyrender/tests/unit/test_lights.py
deleted file mode 100644
index ffde856b21e8cce9532f0308fcd1c7eb2d1eba90..0000000000000000000000000000000000000000
--- a/spaces/OpenMotionLab/MotionGPT/pyrender/tests/unit/test_lights.py
+++ /dev/null
@@ -1,104 +0,0 @@
-import numpy as np
-import pytest
-
-from pyrender import (DirectionalLight, SpotLight, PointLight, Texture,
- PerspectiveCamera, OrthographicCamera)
-from pyrender.constants import SHADOW_TEX_SZ
-
-
-def test_directional_light():
-
- d = DirectionalLight()
- assert d.name is None
- assert np.all(d.color == 1.0)
- assert d.intensity == 1.0
-
- d.name = 'direc'
- with pytest.raises(ValueError):
- d.color = None
- with pytest.raises(TypeError):
- d.intensity = None
-
- d = DirectionalLight(color=[0.0, 0.0, 0.0])
- assert np.all(d.color == 0.0)
-
- d._generate_shadow_texture()
- st = d.shadow_texture
- assert isinstance(st, Texture)
- assert st.width == st.height == SHADOW_TEX_SZ
-
- sc = d._get_shadow_camera(scene_scale=5.0)
- assert isinstance(sc, OrthographicCamera)
- assert sc.xmag == sc.ymag == 5.0
- assert sc.znear == 0.01 * 5.0
- assert sc.zfar == 10 * 5.0
-
-
-def test_spot_light():
-
- s = SpotLight()
- assert s.name is None
- assert np.all(s.color == 1.0)
- assert s.intensity == 1.0
- assert s.innerConeAngle == 0.0
- assert s.outerConeAngle == np.pi / 4.0
- assert s.range is None
-
- with pytest.raises(ValueError):
- s.range = -1.0
-
- with pytest.raises(ValueError):
- s.range = 0.0
-
- with pytest.raises(ValueError):
- s.innerConeAngle = -1.0
-
- with pytest.raises(ValueError):
- s.innerConeAngle = np.pi / 3.0
-
- with pytest.raises(ValueError):
- s.outerConeAngle = -1.0
-
- with pytest.raises(ValueError):
- s.outerConeAngle = np.pi
-
- s.range = 5.0
- s.outerConeAngle = np.pi / 2 - 0.05
- s.innerConeAngle = np.pi / 3
- s.innerConeAngle = 0.0
- s.outerConeAngle = np.pi / 4.0
-
- s._generate_shadow_texture()
- st = s.shadow_texture
- assert isinstance(st, Texture)
- assert st.width == st.height == SHADOW_TEX_SZ
-
- sc = s._get_shadow_camera(scene_scale=5.0)
- assert isinstance(sc, PerspectiveCamera)
- assert sc.znear == 0.01 * 5.0
- assert sc.zfar == 10 * 5.0
- assert sc.aspectRatio == 1.0
- assert np.allclose(sc.yfov, np.pi / 16.0 * 9.0) # Plus pi / 16
-
-
-def test_point_light():
-
- s = PointLight()
- assert s.name is None
- assert np.all(s.color == 1.0)
- assert s.intensity == 1.0
- assert s.range is None
-
- with pytest.raises(ValueError):
- s.range = -1.0
-
- with pytest.raises(ValueError):
- s.range = 0.0
-
- s.range = 5.0
-
- with pytest.raises(NotImplementedError):
- s._generate_shadow_texture()
-
- with pytest.raises(NotImplementedError):
- s._get_shadow_camera(scene_scale=5.0)
diff --git a/spaces/PKUWilliamYang/StyleGANEX/scripts/calc_id_loss_parallel.py b/spaces/PKUWilliamYang/StyleGANEX/scripts/calc_id_loss_parallel.py
deleted file mode 100644
index efc82bf851b252e92c45be3c87be877616f44ead..0000000000000000000000000000000000000000
--- a/spaces/PKUWilliamYang/StyleGANEX/scripts/calc_id_loss_parallel.py
+++ /dev/null
@@ -1,119 +0,0 @@
-from argparse import ArgumentParser
-import time
-import numpy as np
-import os
-import json
-import sys
-from PIL import Image
-import multiprocessing as mp
-import math
-import torch
-import torchvision.transforms as trans
-
-sys.path.append(".")
-sys.path.append("..")
-
-from models.mtcnn.mtcnn import MTCNN
-from models.encoders.model_irse import IR_101
-from configs.paths_config import model_paths
-CIRCULAR_FACE_PATH = model_paths['circular_face']
-
-
-def chunks(lst, n):
- """Yield successive n-sized chunks from lst."""
- for i in range(0, len(lst), n):
- yield lst[i:i + n]
-
-
-def extract_on_paths(file_paths):
- facenet = IR_101(input_size=112)
- facenet.load_state_dict(torch.load(CIRCULAR_FACE_PATH))
- facenet.cuda()
- facenet.eval()
- mtcnn = MTCNN()
- id_transform = trans.Compose([
- trans.ToTensor(),
- trans.Normalize([0.5, 0.5, 0.5], [0.5, 0.5, 0.5])
- ])
-
- pid = mp.current_process().name
- print('\t{} is starting to extract on {} images'.format(pid, len(file_paths)))
- tot_count = len(file_paths)
- count = 0
-
- scores_dict = {}
- for res_path, gt_path in file_paths:
- count += 1
- if count % 100 == 0:
- print('{} done with {}/{}'.format(pid, count, tot_count))
- if True:
- input_im = Image.open(res_path)
- input_im, _ = mtcnn.align(input_im)
- if input_im is None:
- print('{} skipping {}'.format(pid, res_path))
- continue
-
- input_id = facenet(id_transform(input_im).unsqueeze(0).cuda())[0]
-
- result_im = Image.open(gt_path)
- result_im, _ = mtcnn.align(result_im)
- if result_im is None:
- print('{} skipping {}'.format(pid, gt_path))
- continue
-
- result_id = facenet(id_transform(result_im).unsqueeze(0).cuda())[0]
- score = float(input_id.dot(result_id))
- scores_dict[os.path.basename(gt_path)] = score
-
- return scores_dict
-
-
-def parse_args():
- parser = ArgumentParser(add_help=False)
- parser.add_argument('--num_threads', type=int, default=4)
- parser.add_argument('--data_path', type=str, default='results')
- parser.add_argument('--gt_path', type=str, default='gt_images')
- args = parser.parse_args()
- return args
-
-
-def run(args):
- file_paths = []
- for f in os.listdir(args.data_path):
- image_path = os.path.join(args.data_path, f)
- gt_path = os.path.join(args.gt_path, f)
- if f.endswith(".jpg") or f.endswith('.png'):
- file_paths.append([image_path, gt_path.replace('.png','.jpg')])
-
- file_chunks = list(chunks(file_paths, int(math.ceil(len(file_paths) / args.num_threads))))
- pool = mp.Pool(args.num_threads)
- print('Running on {} paths\nHere we goooo'.format(len(file_paths)))
-
- tic = time.time()
- results = pool.map(extract_on_paths, file_chunks)
- scores_dict = {}
- for d in results:
- scores_dict.update(d)
-
- all_scores = list(scores_dict.values())
- mean = np.mean(all_scores)
- std = np.std(all_scores)
- result_str = 'New Average score is {:.2f}+-{:.2f}'.format(mean, std)
- print(result_str)
-
- out_path = os.path.join(os.path.dirname(args.data_path), 'inference_metrics')
- if not os.path.exists(out_path):
- os.makedirs(out_path)
-
- with open(os.path.join(out_path, 'stat_id.txt'), 'w') as f:
- f.write(result_str)
- with open(os.path.join(out_path, 'scores_id.json'), 'w') as f:
- json.dump(scores_dict, f)
-
- toc = time.time()
- print('Mischief managed in {}s'.format(toc - tic))
-
-
-if __name__ == '__main__':
- args = parse_args()
- run(args)
diff --git a/spaces/PSLD/PSLD/diffusion-posterior-sampling/util/logger.py b/spaces/PSLD/PSLD/diffusion-posterior-sampling/util/logger.py
deleted file mode 100644
index 7992959680b35ab36fd9649774ad872f06847158..0000000000000000000000000000000000000000
--- a/spaces/PSLD/PSLD/diffusion-posterior-sampling/util/logger.py
+++ /dev/null
@@ -1,12 +0,0 @@
-import logging
-
-def get_logger():
- logger = logging.getLogger(name='DPS')
- logger.setLevel(logging.INFO)
-
- formatter = logging.Formatter("%(asctime)s [%(name)s] >> %(message)s")
- stream_handler = logging.StreamHandler()
- stream_handler.setFormatter(formatter)
- logger.addHandler(stream_handler)
-
- return logger
\ No newline at end of file
diff --git a/spaces/PaddlePaddle/resnet_v2_34_imagenet/app.py b/spaces/PaddlePaddle/resnet_v2_34_imagenet/app.py
deleted file mode 100644
index 6f9fc9f75f2c7d8bc5d202efe413ebcfc6e2ab80..0000000000000000000000000000000000000000
--- a/spaces/PaddlePaddle/resnet_v2_34_imagenet/app.py
+++ /dev/null
@@ -1,18 +0,0 @@
-import gradio as gr
-import paddlehub as hub
-
-classifier = hub.Module(name="resnet_v2_34_imagenet")
-
-def inference(img):
- test_img_path = img
- input_dict = {"image": [test_img_path]}
- result = classifier.classification(data=input_dict)
- print(result)
- return result[0][0]
-
-
-title="resnet_v2_34_imagenet"
-description="The ResNet series model is one of the important models in the field of image classification. The residual unit proposed in the model effectively solves the difficult problem of deep network training, and improves the accuracy of the model by increasing the depth of the model. The PaddleHub Module has a ResNet34 structure and is trained on the ImageNet-2012 dataset. The input image size is 224 x 224 x 3, and it supports prediction directly through the command line or Python interface."
-
-examples=[['cat2.jpg']]
-gr.Interface(inference,gr.inputs.Image(type="filepath"),"label",title=title,description=description,examples=examples).launch(enable_queue=True,cache_examples=True)
\ No newline at end of file
diff --git a/spaces/PatrickTyBrown/LoanDocumentClassifier/README.md b/spaces/PatrickTyBrown/LoanDocumentClassifier/README.md
deleted file mode 100644
index 9ef3746bea64d426f950ddfc56f7d0b43d6164ab..0000000000000000000000000000000000000000
--- a/spaces/PatrickTyBrown/LoanDocumentClassifier/README.md
+++ /dev/null
@@ -1,12 +0,0 @@
----
-title: LoanDocumentClassifier
-emoji: 👁
-colorFrom: gray
-colorTo: pink
-sdk: gradio
-app_file: app.py
-pinned: false
-license: apache-2.0
----
-
-Check out the configuration reference at https://huggingface.co/docs/hub/spaces-config-reference
diff --git a/spaces/Pattr/DrumClassification/lilypond-2.24.2/lib/guile/2.2/ccache/ice-9/and-let-star.go b/spaces/Pattr/DrumClassification/lilypond-2.24.2/lib/guile/2.2/ccache/ice-9/and-let-star.go
deleted file mode 100644
index 9ec2e48045f8fde5ab14abf2d295e66634994d79..0000000000000000000000000000000000000000
Binary files a/spaces/Pattr/DrumClassification/lilypond-2.24.2/lib/guile/2.2/ccache/ice-9/and-let-star.go and /dev/null differ
diff --git a/spaces/Pattr/DrumClassification/lilypond-2.24.2/lib/guile/2.2/ccache/scripts/disassemble.go b/spaces/Pattr/DrumClassification/lilypond-2.24.2/lib/guile/2.2/ccache/scripts/disassemble.go
deleted file mode 100644
index 19f5842dcf2ee20d7288f3d8aee6a70d86d09af0..0000000000000000000000000000000000000000
Binary files a/spaces/Pattr/DrumClassification/lilypond-2.24.2/lib/guile/2.2/ccache/scripts/disassemble.go and /dev/null differ
diff --git a/spaces/Raspberry-ai/main/.env/lib/python3.11/site-packages/pkg_resources/_vendor/more_itertools/recipes.py b/spaces/Raspberry-ai/main/.env/lib/python3.11/site-packages/pkg_resources/_vendor/more_itertools/recipes.py
deleted file mode 100644
index a2596423a4c3dbd15a357241477a0af0a531f9ec..0000000000000000000000000000000000000000
--- a/spaces/Raspberry-ai/main/.env/lib/python3.11/site-packages/pkg_resources/_vendor/more_itertools/recipes.py
+++ /dev/null
@@ -1,698 +0,0 @@
-"""Imported from the recipes section of the itertools documentation.
-
-All functions taken from the recipes section of the itertools library docs
-[1]_.
-Some backward-compatible usability improvements have been made.
-
-.. [1] http://docs.python.org/library/itertools.html#recipes
-
-"""
-import warnings
-from collections import deque
-from itertools import (
- chain,
- combinations,
- count,
- cycle,
- groupby,
- islice,
- repeat,
- starmap,
- tee,
- zip_longest,
-)
-import operator
-from random import randrange, sample, choice
-
-__all__ = [
- 'all_equal',
- 'before_and_after',
- 'consume',
- 'convolve',
- 'dotproduct',
- 'first_true',
- 'flatten',
- 'grouper',
- 'iter_except',
- 'ncycles',
- 'nth',
- 'nth_combination',
- 'padnone',
- 'pad_none',
- 'pairwise',
- 'partition',
- 'powerset',
- 'prepend',
- 'quantify',
- 'random_combination_with_replacement',
- 'random_combination',
- 'random_permutation',
- 'random_product',
- 'repeatfunc',
- 'roundrobin',
- 'sliding_window',
- 'tabulate',
- 'tail',
- 'take',
- 'triplewise',
- 'unique_everseen',
- 'unique_justseen',
-]
-
-
-def take(n, iterable):
- """Return first *n* items of the iterable as a list.
-
- >>> take(3, range(10))
- [0, 1, 2]
-
- If there are fewer than *n* items in the iterable, all of them are
- returned.
-
- >>> take(10, range(3))
- [0, 1, 2]
-
- """
- return list(islice(iterable, n))
-
-
-def tabulate(function, start=0):
- """Return an iterator over the results of ``func(start)``,
- ``func(start + 1)``, ``func(start + 2)``...
-
- *func* should be a function that accepts one integer argument.
-
- If *start* is not specified it defaults to 0. It will be incremented each
- time the iterator is advanced.
-
- >>> square = lambda x: x ** 2
- >>> iterator = tabulate(square, -3)
- >>> take(4, iterator)
- [9, 4, 1, 0]
-
- """
- return map(function, count(start))
-
-
-def tail(n, iterable):
- """Return an iterator over the last *n* items of *iterable*.
-
- >>> t = tail(3, 'ABCDEFG')
- >>> list(t)
- ['E', 'F', 'G']
-
- """
- return iter(deque(iterable, maxlen=n))
-
-
-def consume(iterator, n=None):
- """Advance *iterable* by *n* steps. If *n* is ``None``, consume it
- entirely.
-
- Efficiently exhausts an iterator without returning values. Defaults to
- consuming the whole iterator, but an optional second argument may be
- provided to limit consumption.
-
- >>> i = (x for x in range(10))
- >>> next(i)
- 0
- >>> consume(i, 3)
- >>> next(i)
- 4
- >>> consume(i)
- >>> next(i)
- Traceback (most recent call last):
- File "", line 1, in
- StopIteration
-
- If the iterator has fewer items remaining than the provided limit, the
- whole iterator will be consumed.
-
- >>> i = (x for x in range(3))
- >>> consume(i, 5)
- >>> next(i)
- Traceback (most recent call last):
- File "", line 1, in
- StopIteration
-
- """
- # Use functions that consume iterators at C speed.
- if n is None:
- # feed the entire iterator into a zero-length deque
- deque(iterator, maxlen=0)
- else:
- # advance to the empty slice starting at position n
- next(islice(iterator, n, n), None)
-
-
-def nth(iterable, n, default=None):
- """Returns the nth item or a default value.
-
- >>> l = range(10)
- >>> nth(l, 3)
- 3
- >>> nth(l, 20, "zebra")
- 'zebra'
-
- """
- return next(islice(iterable, n, None), default)
-
-
-def all_equal(iterable):
- """
- Returns ``True`` if all the elements are equal to each other.
-
- >>> all_equal('aaaa')
- True
- >>> all_equal('aaab')
- False
-
- """
- g = groupby(iterable)
- return next(g, True) and not next(g, False)
-
-
-def quantify(iterable, pred=bool):
- """Return the how many times the predicate is true.
-
- >>> quantify([True, False, True])
- 2
-
- """
- return sum(map(pred, iterable))
-
-
-def pad_none(iterable):
- """Returns the sequence of elements and then returns ``None`` indefinitely.
-
- >>> take(5, pad_none(range(3)))
- [0, 1, 2, None, None]
-
- Useful for emulating the behavior of the built-in :func:`map` function.
-
- See also :func:`padded`.
-
- """
- return chain(iterable, repeat(None))
-
-
-padnone = pad_none
-
-
-def ncycles(iterable, n):
- """Returns the sequence elements *n* times
-
- >>> list(ncycles(["a", "b"], 3))
- ['a', 'b', 'a', 'b', 'a', 'b']
-
- """
- return chain.from_iterable(repeat(tuple(iterable), n))
-
-
-def dotproduct(vec1, vec2):
- """Returns the dot product of the two iterables.
-
- >>> dotproduct([10, 10], [20, 20])
- 400
-
- """
- return sum(map(operator.mul, vec1, vec2))
-
-
-def flatten(listOfLists):
- """Return an iterator flattening one level of nesting in a list of lists.
-
- >>> list(flatten([[0, 1], [2, 3]]))
- [0, 1, 2, 3]
-
- See also :func:`collapse`, which can flatten multiple levels of nesting.
-
- """
- return chain.from_iterable(listOfLists)
-
-
-def repeatfunc(func, times=None, *args):
- """Call *func* with *args* repeatedly, returning an iterable over the
- results.
-
- If *times* is specified, the iterable will terminate after that many
- repetitions:
-
- >>> from operator import add
- >>> times = 4
- >>> args = 3, 5
- >>> list(repeatfunc(add, times, *args))
- [8, 8, 8, 8]
-
- If *times* is ``None`` the iterable will not terminate:
-
- >>> from random import randrange
- >>> times = None
- >>> args = 1, 11
- >>> take(6, repeatfunc(randrange, times, *args)) # doctest:+SKIP
- [2, 4, 8, 1, 8, 4]
-
- """
- if times is None:
- return starmap(func, repeat(args))
- return starmap(func, repeat(args, times))
-
-
-def _pairwise(iterable):
- """Returns an iterator of paired items, overlapping, from the original
-
- >>> take(4, pairwise(count()))
- [(0, 1), (1, 2), (2, 3), (3, 4)]
-
- On Python 3.10 and above, this is an alias for :func:`itertools.pairwise`.
-
- """
- a, b = tee(iterable)
- next(b, None)
- yield from zip(a, b)
-
-
-try:
- from itertools import pairwise as itertools_pairwise
-except ImportError:
- pairwise = _pairwise
-else:
-
- def pairwise(iterable):
- yield from itertools_pairwise(iterable)
-
- pairwise.__doc__ = _pairwise.__doc__
-
-
-def grouper(iterable, n, fillvalue=None):
- """Collect data into fixed-length chunks or blocks.
-
- >>> list(grouper('ABCDEFG', 3, 'x'))
- [('A', 'B', 'C'), ('D', 'E', 'F'), ('G', 'x', 'x')]
-
- """
- if isinstance(iterable, int):
- warnings.warn(
- "grouper expects iterable as first parameter", DeprecationWarning
- )
- n, iterable = iterable, n
- args = [iter(iterable)] * n
- return zip_longest(fillvalue=fillvalue, *args)
-
-
-def roundrobin(*iterables):
- """Yields an item from each iterable, alternating between them.
-
- >>> list(roundrobin('ABC', 'D', 'EF'))
- ['A', 'D', 'E', 'B', 'F', 'C']
-
- This function produces the same output as :func:`interleave_longest`, but
- may perform better for some inputs (in particular when the number of
- iterables is small).
-
- """
- # Recipe credited to George Sakkis
- pending = len(iterables)
- nexts = cycle(iter(it).__next__ for it in iterables)
- while pending:
- try:
- for next in nexts:
- yield next()
- except StopIteration:
- pending -= 1
- nexts = cycle(islice(nexts, pending))
-
-
-def partition(pred, iterable):
- """
- Returns a 2-tuple of iterables derived from the input iterable.
- The first yields the items that have ``pred(item) == False``.
- The second yields the items that have ``pred(item) == True``.
-
- >>> is_odd = lambda x: x % 2 != 0
- >>> iterable = range(10)
- >>> even_items, odd_items = partition(is_odd, iterable)
- >>> list(even_items), list(odd_items)
- ([0, 2, 4, 6, 8], [1, 3, 5, 7, 9])
-
- If *pred* is None, :func:`bool` is used.
-
- >>> iterable = [0, 1, False, True, '', ' ']
- >>> false_items, true_items = partition(None, iterable)
- >>> list(false_items), list(true_items)
- ([0, False, ''], [1, True, ' '])
-
- """
- if pred is None:
- pred = bool
-
- evaluations = ((pred(x), x) for x in iterable)
- t1, t2 = tee(evaluations)
- return (
- (x for (cond, x) in t1 if not cond),
- (x for (cond, x) in t2 if cond),
- )
-
-
-def powerset(iterable):
- """Yields all possible subsets of the iterable.
-
- >>> list(powerset([1, 2, 3]))
- [(), (1,), (2,), (3,), (1, 2), (1, 3), (2, 3), (1, 2, 3)]
-
- :func:`powerset` will operate on iterables that aren't :class:`set`
- instances, so repeated elements in the input will produce repeated elements
- in the output. Use :func:`unique_everseen` on the input to avoid generating
- duplicates:
-
- >>> seq = [1, 1, 0]
- >>> list(powerset(seq))
- [(), (1,), (1,), (0,), (1, 1), (1, 0), (1, 0), (1, 1, 0)]
- >>> from more_itertools import unique_everseen
- >>> list(powerset(unique_everseen(seq)))
- [(), (1,), (0,), (1, 0)]
-
- """
- s = list(iterable)
- return chain.from_iterable(combinations(s, r) for r in range(len(s) + 1))
-
-
-def unique_everseen(iterable, key=None):
- """
- Yield unique elements, preserving order.
-
- >>> list(unique_everseen('AAAABBBCCDAABBB'))
- ['A', 'B', 'C', 'D']
- >>> list(unique_everseen('ABBCcAD', str.lower))
- ['A', 'B', 'C', 'D']
-
- Sequences with a mix of hashable and unhashable items can be used.
- The function will be slower (i.e., `O(n^2)`) for unhashable items.
-
- Remember that ``list`` objects are unhashable - you can use the *key*
- parameter to transform the list to a tuple (which is hashable) to
- avoid a slowdown.
-
- >>> iterable = ([1, 2], [2, 3], [1, 2])
- >>> list(unique_everseen(iterable)) # Slow
- [[1, 2], [2, 3]]
- >>> list(unique_everseen(iterable, key=tuple)) # Faster
- [[1, 2], [2, 3]]
-
- Similary, you may want to convert unhashable ``set`` objects with
- ``key=frozenset``. For ``dict`` objects,
- ``key=lambda x: frozenset(x.items())`` can be used.
-
- """
- seenset = set()
- seenset_add = seenset.add
- seenlist = []
- seenlist_add = seenlist.append
- use_key = key is not None
-
- for element in iterable:
- k = key(element) if use_key else element
- try:
- if k not in seenset:
- seenset_add(k)
- yield element
- except TypeError:
- if k not in seenlist:
- seenlist_add(k)
- yield element
-
-
-def unique_justseen(iterable, key=None):
- """Yields elements in order, ignoring serial duplicates
-
- >>> list(unique_justseen('AAAABBBCCDAABBB'))
- ['A', 'B', 'C', 'D', 'A', 'B']
- >>> list(unique_justseen('ABBCcAD', str.lower))
- ['A', 'B', 'C', 'A', 'D']
-
- """
- return map(next, map(operator.itemgetter(1), groupby(iterable, key)))
-
-
-def iter_except(func, exception, first=None):
- """Yields results from a function repeatedly until an exception is raised.
-
- Converts a call-until-exception interface to an iterator interface.
- Like ``iter(func, sentinel)``, but uses an exception instead of a sentinel
- to end the loop.
-
- >>> l = [0, 1, 2]
- >>> list(iter_except(l.pop, IndexError))
- [2, 1, 0]
-
- Multiple exceptions can be specified as a stopping condition:
-
- >>> l = [1, 2, 3, '...', 4, 5, 6]
- >>> list(iter_except(lambda: 1 + l.pop(), (IndexError, TypeError)))
- [7, 6, 5]
- >>> list(iter_except(lambda: 1 + l.pop(), (IndexError, TypeError)))
- [4, 3, 2]
- >>> list(iter_except(lambda: 1 + l.pop(), (IndexError, TypeError)))
- []
-
- """
- try:
- if first is not None:
- yield first()
- while 1:
- yield func()
- except exception:
- pass
-
-
-def first_true(iterable, default=None, pred=None):
- """
- Returns the first true value in the iterable.
-
- If no true value is found, returns *default*
-
- If *pred* is not None, returns the first item for which
- ``pred(item) == True`` .
-
- >>> first_true(range(10))
- 1
- >>> first_true(range(10), pred=lambda x: x > 5)
- 6
- >>> first_true(range(10), default='missing', pred=lambda x: x > 9)
- 'missing'
-
- """
- return next(filter(pred, iterable), default)
-
-
-def random_product(*args, repeat=1):
- """Draw an item at random from each of the input iterables.
-
- >>> random_product('abc', range(4), 'XYZ') # doctest:+SKIP
- ('c', 3, 'Z')
-
- If *repeat* is provided as a keyword argument, that many items will be
- drawn from each iterable.
-
- >>> random_product('abcd', range(4), repeat=2) # doctest:+SKIP
- ('a', 2, 'd', 3)
-
- This equivalent to taking a random selection from
- ``itertools.product(*args, **kwarg)``.
-
- """
- pools = [tuple(pool) for pool in args] * repeat
- return tuple(choice(pool) for pool in pools)
-
-
-def random_permutation(iterable, r=None):
- """Return a random *r* length permutation of the elements in *iterable*.
-
- If *r* is not specified or is ``None``, then *r* defaults to the length of
- *iterable*.
-
- >>> random_permutation(range(5)) # doctest:+SKIP
- (3, 4, 0, 1, 2)
-
- This equivalent to taking a random selection from
- ``itertools.permutations(iterable, r)``.
-
- """
- pool = tuple(iterable)
- r = len(pool) if r is None else r
- return tuple(sample(pool, r))
-
-
-def random_combination(iterable, r):
- """Return a random *r* length subsequence of the elements in *iterable*.
-
- >>> random_combination(range(5), 3) # doctest:+SKIP
- (2, 3, 4)
-
- This equivalent to taking a random selection from
- ``itertools.combinations(iterable, r)``.
-
- """
- pool = tuple(iterable)
- n = len(pool)
- indices = sorted(sample(range(n), r))
- return tuple(pool[i] for i in indices)
-
-
-def random_combination_with_replacement(iterable, r):
- """Return a random *r* length subsequence of elements in *iterable*,
- allowing individual elements to be repeated.
-
- >>> random_combination_with_replacement(range(3), 5) # doctest:+SKIP
- (0, 0, 1, 2, 2)
-
- This equivalent to taking a random selection from
- ``itertools.combinations_with_replacement(iterable, r)``.
-
- """
- pool = tuple(iterable)
- n = len(pool)
- indices = sorted(randrange(n) for i in range(r))
- return tuple(pool[i] for i in indices)
-
-
-def nth_combination(iterable, r, index):
- """Equivalent to ``list(combinations(iterable, r))[index]``.
-
- The subsequences of *iterable* that are of length *r* can be ordered
- lexicographically. :func:`nth_combination` computes the subsequence at
- sort position *index* directly, without computing the previous
- subsequences.
-
- >>> nth_combination(range(5), 3, 5)
- (0, 3, 4)
-
- ``ValueError`` will be raised If *r* is negative or greater than the length
- of *iterable*.
- ``IndexError`` will be raised if the given *index* is invalid.
- """
- pool = tuple(iterable)
- n = len(pool)
- if (r < 0) or (r > n):
- raise ValueError
-
- c = 1
- k = min(r, n - r)
- for i in range(1, k + 1):
- c = c * (n - k + i) // i
-
- if index < 0:
- index += c
-
- if (index < 0) or (index >= c):
- raise IndexError
-
- result = []
- while r:
- c, n, r = c * r // n, n - 1, r - 1
- while index >= c:
- index -= c
- c, n = c * (n - r) // n, n - 1
- result.append(pool[-1 - n])
-
- return tuple(result)
-
-
-def prepend(value, iterator):
- """Yield *value*, followed by the elements in *iterator*.
-
- >>> value = '0'
- >>> iterator = ['1', '2', '3']
- >>> list(prepend(value, iterator))
- ['0', '1', '2', '3']
-
- To prepend multiple values, see :func:`itertools.chain`
- or :func:`value_chain`.
-
- """
- return chain([value], iterator)
-
-
-def convolve(signal, kernel):
- """Convolve the iterable *signal* with the iterable *kernel*.
-
- >>> signal = (1, 2, 3, 4, 5)
- >>> kernel = [3, 2, 1]
- >>> list(convolve(signal, kernel))
- [3, 8, 14, 20, 26, 14, 5]
-
- Note: the input arguments are not interchangeable, as the *kernel*
- is immediately consumed and stored.
-
- """
- kernel = tuple(kernel)[::-1]
- n = len(kernel)
- window = deque([0], maxlen=n) * n
- for x in chain(signal, repeat(0, n - 1)):
- window.append(x)
- yield sum(map(operator.mul, kernel, window))
-
-
-def before_and_after(predicate, it):
- """A variant of :func:`takewhile` that allows complete access to the
- remainder of the iterator.
-
- >>> it = iter('ABCdEfGhI')
- >>> all_upper, remainder = before_and_after(str.isupper, it)
- >>> ''.join(all_upper)
- 'ABC'
- >>> ''.join(remainder) # takewhile() would lose the 'd'
- 'dEfGhI'
-
- Note that the first iterator must be fully consumed before the second
- iterator can generate valid results.
- """
- it = iter(it)
- transition = []
-
- def true_iterator():
- for elem in it:
- if predicate(elem):
- yield elem
- else:
- transition.append(elem)
- return
-
- def remainder_iterator():
- yield from transition
- yield from it
-
- return true_iterator(), remainder_iterator()
-
-
-def triplewise(iterable):
- """Return overlapping triplets from *iterable*.
-
- >>> list(triplewise('ABCDE'))
- [('A', 'B', 'C'), ('B', 'C', 'D'), ('C', 'D', 'E')]
-
- """
- for (a, _), (b, c) in pairwise(pairwise(iterable)):
- yield a, b, c
-
-
-def sliding_window(iterable, n):
- """Return a sliding window of width *n* over *iterable*.
-
- >>> list(sliding_window(range(6), 4))
- [(0, 1, 2, 3), (1, 2, 3, 4), (2, 3, 4, 5)]
-
- If *iterable* has fewer than *n* items, then nothing is yielded:
-
- >>> list(sliding_window(range(3), 4))
- []
-
- For a variant with more features, see :func:`windowed`.
- """
- it = iter(iterable)
- window = deque(islice(it, n), maxlen=n)
- if len(window) == n:
- yield tuple(window)
- for x in it:
- window.append(x)
- yield tuple(window)
diff --git a/spaces/Raspberry-ai/main/.env/lib/python3.11/site-packages/pkg_resources/_vendor/pyparsing/diagram/__init__.py b/spaces/Raspberry-ai/main/.env/lib/python3.11/site-packages/pkg_resources/_vendor/pyparsing/diagram/__init__.py
deleted file mode 100644
index 898644755cbbf9a8d4df562663114a7eb7e11fd1..0000000000000000000000000000000000000000
--- a/spaces/Raspberry-ai/main/.env/lib/python3.11/site-packages/pkg_resources/_vendor/pyparsing/diagram/__init__.py
+++ /dev/null
@@ -1,642 +0,0 @@
-import railroad
-import pyparsing
-import typing
-from typing import (
- List,
- NamedTuple,
- Generic,
- TypeVar,
- Dict,
- Callable,
- Set,
- Iterable,
-)
-from jinja2 import Template
-from io import StringIO
-import inspect
-
-
-jinja2_template_source = """\
-
-
-
- {% if not head %}
-
- {% else %}
- {{ head | safe }}
- {% endif %}
-
-
-{{ body | safe }}
-{% for diagram in diagrams %}
-
-
{{ diagram.title }}
-
{{ diagram.text }}
-
- {{ diagram.svg }}
-
-
-{% endfor %}
-
-
-"""
-
-template = Template(jinja2_template_source)
-
-# Note: ideally this would be a dataclass, but we're supporting Python 3.5+ so we can't do this yet
-NamedDiagram = NamedTuple(
- "NamedDiagram",
- [("name", str), ("diagram", typing.Optional[railroad.DiagramItem]), ("index", int)],
-)
-"""
-A simple structure for associating a name with a railroad diagram
-"""
-
-T = TypeVar("T")
-
-
-class EachItem(railroad.Group):
- """
- Custom railroad item to compose a:
- - Group containing a
- - OneOrMore containing a
- - Choice of the elements in the Each
- with the group label indicating that all must be matched
- """
-
- all_label = "[ALL]"
-
- def __init__(self, *items):
- choice_item = railroad.Choice(len(items) - 1, *items)
- one_or_more_item = railroad.OneOrMore(item=choice_item)
- super().__init__(one_or_more_item, label=self.all_label)
-
-
-class AnnotatedItem(railroad.Group):
- """
- Simple subclass of Group that creates an annotation label
- """
-
- def __init__(self, label: str, item):
- super().__init__(item=item, label="[{}]".format(label) if label else label)
-
-
-class EditablePartial(Generic[T]):
- """
- Acts like a functools.partial, but can be edited. In other words, it represents a type that hasn't yet been
- constructed.
- """
-
- # We need this here because the railroad constructors actually transform the data, so can't be called until the
- # entire tree is assembled
-
- def __init__(self, func: Callable[..., T], args: list, kwargs: dict):
- self.func = func
- self.args = args
- self.kwargs = kwargs
-
- @classmethod
- def from_call(cls, func: Callable[..., T], *args, **kwargs) -> "EditablePartial[T]":
- """
- If you call this function in the same way that you would call the constructor, it will store the arguments
- as you expect. For example EditablePartial.from_call(Fraction, 1, 3)() == Fraction(1, 3)
- """
- return EditablePartial(func=func, args=list(args), kwargs=kwargs)
-
- @property
- def name(self):
- return self.kwargs["name"]
-
- def __call__(self) -> T:
- """
- Evaluate the partial and return the result
- """
- args = self.args.copy()
- kwargs = self.kwargs.copy()
-
- # This is a helpful hack to allow you to specify varargs parameters (e.g. *args) as keyword args (e.g.
- # args=['list', 'of', 'things'])
- arg_spec = inspect.getfullargspec(self.func)
- if arg_spec.varargs in self.kwargs:
- args += kwargs.pop(arg_spec.varargs)
-
- return self.func(*args, **kwargs)
-
-
-def railroad_to_html(diagrams: List[NamedDiagram], **kwargs) -> str:
- """
- Given a list of NamedDiagram, produce a single HTML string that visualises those diagrams
- :params kwargs: kwargs to be passed in to the template
- """
- data = []
- for diagram in diagrams:
- if diagram.diagram is None:
- continue
- io = StringIO()
- diagram.diagram.writeSvg(io.write)
- title = diagram.name
- if diagram.index == 0:
- title += " (root)"
- data.append({"title": title, "text": "", "svg": io.getvalue()})
-
- return template.render(diagrams=data, **kwargs)
-
-
-def resolve_partial(partial: "EditablePartial[T]") -> T:
- """
- Recursively resolves a collection of Partials into whatever type they are
- """
- if isinstance(partial, EditablePartial):
- partial.args = resolve_partial(partial.args)
- partial.kwargs = resolve_partial(partial.kwargs)
- return partial()
- elif isinstance(partial, list):
- return [resolve_partial(x) for x in partial]
- elif isinstance(partial, dict):
- return {key: resolve_partial(x) for key, x in partial.items()}
- else:
- return partial
-
-
-def to_railroad(
- element: pyparsing.ParserElement,
- diagram_kwargs: typing.Optional[dict] = None,
- vertical: int = 3,
- show_results_names: bool = False,
- show_groups: bool = False,
-) -> List[NamedDiagram]:
- """
- Convert a pyparsing element tree into a list of diagrams. This is the recommended entrypoint to diagram
- creation if you want to access the Railroad tree before it is converted to HTML
- :param element: base element of the parser being diagrammed
- :param diagram_kwargs: kwargs to pass to the Diagram() constructor
- :param vertical: (optional) - int - limit at which number of alternatives should be
- shown vertically instead of horizontally
- :param show_results_names - bool to indicate whether results name annotations should be
- included in the diagram
- :param show_groups - bool to indicate whether groups should be highlighted with an unlabeled
- surrounding box
- """
- # Convert the whole tree underneath the root
- lookup = ConverterState(diagram_kwargs=diagram_kwargs or {})
- _to_diagram_element(
- element,
- lookup=lookup,
- parent=None,
- vertical=vertical,
- show_results_names=show_results_names,
- show_groups=show_groups,
- )
-
- root_id = id(element)
- # Convert the root if it hasn't been already
- if root_id in lookup:
- if not element.customName:
- lookup[root_id].name = ""
- lookup[root_id].mark_for_extraction(root_id, lookup, force=True)
-
- # Now that we're finished, we can convert from intermediate structures into Railroad elements
- diags = list(lookup.diagrams.values())
- if len(diags) > 1:
- # collapse out duplicate diags with the same name
- seen = set()
- deduped_diags = []
- for d in diags:
- # don't extract SkipTo elements, they are uninformative as subdiagrams
- if d.name == "...":
- continue
- if d.name is not None and d.name not in seen:
- seen.add(d.name)
- deduped_diags.append(d)
- resolved = [resolve_partial(partial) for partial in deduped_diags]
- else:
- # special case - if just one diagram, always display it, even if
- # it has no name
- resolved = [resolve_partial(partial) for partial in diags]
- return sorted(resolved, key=lambda diag: diag.index)
-
-
-def _should_vertical(
- specification: int, exprs: Iterable[pyparsing.ParserElement]
-) -> bool:
- """
- Returns true if we should return a vertical list of elements
- """
- if specification is None:
- return False
- else:
- return len(_visible_exprs(exprs)) >= specification
-
-
-class ElementState:
- """
- State recorded for an individual pyparsing Element
- """
-
- # Note: this should be a dataclass, but we have to support Python 3.5
- def __init__(
- self,
- element: pyparsing.ParserElement,
- converted: EditablePartial,
- parent: EditablePartial,
- number: int,
- name: str = None,
- parent_index: typing.Optional[int] = None,
- ):
- #: The pyparsing element that this represents
- self.element: pyparsing.ParserElement = element
- #: The name of the element
- self.name: typing.Optional[str] = name
- #: The output Railroad element in an unconverted state
- self.converted: EditablePartial = converted
- #: The parent Railroad element, which we store so that we can extract this if it's duplicated
- self.parent: EditablePartial = parent
- #: The order in which we found this element, used for sorting diagrams if this is extracted into a diagram
- self.number: int = number
- #: The index of this inside its parent
- self.parent_index: typing.Optional[int] = parent_index
- #: If true, we should extract this out into a subdiagram
- self.extract: bool = False
- #: If true, all of this element's children have been filled out
- self.complete: bool = False
-
- def mark_for_extraction(
- self, el_id: int, state: "ConverterState", name: str = None, force: bool = False
- ):
- """
- Called when this instance has been seen twice, and thus should eventually be extracted into a sub-diagram
- :param el_id: id of the element
- :param state: element/diagram state tracker
- :param name: name to use for this element's text
- :param force: If true, force extraction now, regardless of the state of this. Only useful for extracting the
- root element when we know we're finished
- """
- self.extract = True
-
- # Set the name
- if not self.name:
- if name:
- # Allow forcing a custom name
- self.name = name
- elif self.element.customName:
- self.name = self.element.customName
- else:
- self.name = ""
-
- # Just because this is marked for extraction doesn't mean we can do it yet. We may have to wait for children
- # to be added
- # Also, if this is just a string literal etc, don't bother extracting it
- if force or (self.complete and _worth_extracting(self.element)):
- state.extract_into_diagram(el_id)
-
-
-class ConverterState:
- """
- Stores some state that persists between recursions into the element tree
- """
-
- def __init__(self, diagram_kwargs: typing.Optional[dict] = None):
- #: A dictionary mapping ParserElements to state relating to them
- self._element_diagram_states: Dict[int, ElementState] = {}
- #: A dictionary mapping ParserElement IDs to subdiagrams generated from them
- self.diagrams: Dict[int, EditablePartial[NamedDiagram]] = {}
- #: The index of the next unnamed element
- self.unnamed_index: int = 1
- #: The index of the next element. This is used for sorting
- self.index: int = 0
- #: Shared kwargs that are used to customize the construction of diagrams
- self.diagram_kwargs: dict = diagram_kwargs or {}
- self.extracted_diagram_names: Set[str] = set()
-
- def __setitem__(self, key: int, value: ElementState):
- self._element_diagram_states[key] = value
-
- def __getitem__(self, key: int) -> ElementState:
- return self._element_diagram_states[key]
-
- def __delitem__(self, key: int):
- del self._element_diagram_states[key]
-
- def __contains__(self, key: int):
- return key in self._element_diagram_states
-
- def generate_unnamed(self) -> int:
- """
- Generate a number used in the name of an otherwise unnamed diagram
- """
- self.unnamed_index += 1
- return self.unnamed_index
-
- def generate_index(self) -> int:
- """
- Generate a number used to index a diagram
- """
- self.index += 1
- return self.index
-
- def extract_into_diagram(self, el_id: int):
- """
- Used when we encounter the same token twice in the same tree. When this
- happens, we replace all instances of that token with a terminal, and
- create a new subdiagram for the token
- """
- position = self[el_id]
-
- # Replace the original definition of this element with a regular block
- if position.parent:
- ret = EditablePartial.from_call(railroad.NonTerminal, text=position.name)
- if "item" in position.parent.kwargs:
- position.parent.kwargs["item"] = ret
- elif "items" in position.parent.kwargs:
- position.parent.kwargs["items"][position.parent_index] = ret
-
- # If the element we're extracting is a group, skip to its content but keep the title
- if position.converted.func == railroad.Group:
- content = position.converted.kwargs["item"]
- else:
- content = position.converted
-
- self.diagrams[el_id] = EditablePartial.from_call(
- NamedDiagram,
- name=position.name,
- diagram=EditablePartial.from_call(
- railroad.Diagram, content, **self.diagram_kwargs
- ),
- index=position.number,
- )
-
- del self[el_id]
-
-
-def _worth_extracting(element: pyparsing.ParserElement) -> bool:
- """
- Returns true if this element is worth having its own sub-diagram. Simply, if any of its children
- themselves have children, then its complex enough to extract
- """
- children = element.recurse()
- return any(child.recurse() for child in children)
-
-
-def _apply_diagram_item_enhancements(fn):
- """
- decorator to ensure enhancements to a diagram item (such as results name annotations)
- get applied on return from _to_diagram_element (we do this since there are several
- returns in _to_diagram_element)
- """
-
- def _inner(
- element: pyparsing.ParserElement,
- parent: typing.Optional[EditablePartial],
- lookup: ConverterState = None,
- vertical: int = None,
- index: int = 0,
- name_hint: str = None,
- show_results_names: bool = False,
- show_groups: bool = False,
- ) -> typing.Optional[EditablePartial]:
-
- ret = fn(
- element,
- parent,
- lookup,
- vertical,
- index,
- name_hint,
- show_results_names,
- show_groups,
- )
-
- # apply annotation for results name, if present
- if show_results_names and ret is not None:
- element_results_name = element.resultsName
- if element_results_name:
- # add "*" to indicate if this is a "list all results" name
- element_results_name += "" if element.modalResults else "*"
- ret = EditablePartial.from_call(
- railroad.Group, item=ret, label=element_results_name
- )
-
- return ret
-
- return _inner
-
-
-def _visible_exprs(exprs: Iterable[pyparsing.ParserElement]):
- non_diagramming_exprs = (
- pyparsing.ParseElementEnhance,
- pyparsing.PositionToken,
- pyparsing.And._ErrorStop,
- )
- return [
- e
- for e in exprs
- if not (e.customName or e.resultsName or isinstance(e, non_diagramming_exprs))
- ]
-
-
-@_apply_diagram_item_enhancements
-def _to_diagram_element(
- element: pyparsing.ParserElement,
- parent: typing.Optional[EditablePartial],
- lookup: ConverterState = None,
- vertical: int = None,
- index: int = 0,
- name_hint: str = None,
- show_results_names: bool = False,
- show_groups: bool = False,
-) -> typing.Optional[EditablePartial]:
- """
- Recursively converts a PyParsing Element to a railroad Element
- :param lookup: The shared converter state that keeps track of useful things
- :param index: The index of this element within the parent
- :param parent: The parent of this element in the output tree
- :param vertical: Controls at what point we make a list of elements vertical. If this is an integer (the default),
- it sets the threshold of the number of items before we go vertical. If True, always go vertical, if False, never
- do so
- :param name_hint: If provided, this will override the generated name
- :param show_results_names: bool flag indicating whether to add annotations for results names
- :returns: The converted version of the input element, but as a Partial that hasn't yet been constructed
- :param show_groups: bool flag indicating whether to show groups using bounding box
- """
- exprs = element.recurse()
- name = name_hint or element.customName or element.__class__.__name__
-
- # Python's id() is used to provide a unique identifier for elements
- el_id = id(element)
-
- element_results_name = element.resultsName
-
- # Here we basically bypass processing certain wrapper elements if they contribute nothing to the diagram
- if not element.customName:
- if isinstance(
- element,
- (
- # pyparsing.TokenConverter,
- # pyparsing.Forward,
- pyparsing.Located,
- ),
- ):
- # However, if this element has a useful custom name, and its child does not, we can pass it on to the child
- if exprs:
- if not exprs[0].customName:
- propagated_name = name
- else:
- propagated_name = None
-
- return _to_diagram_element(
- element.expr,
- parent=parent,
- lookup=lookup,
- vertical=vertical,
- index=index,
- name_hint=propagated_name,
- show_results_names=show_results_names,
- show_groups=show_groups,
- )
-
- # If the element isn't worth extracting, we always treat it as the first time we say it
- if _worth_extracting(element):
- if el_id in lookup:
- # If we've seen this element exactly once before, we are only just now finding out that it's a duplicate,
- # so we have to extract it into a new diagram.
- looked_up = lookup[el_id]
- looked_up.mark_for_extraction(el_id, lookup, name=name_hint)
- ret = EditablePartial.from_call(railroad.NonTerminal, text=looked_up.name)
- return ret
-
- elif el_id in lookup.diagrams:
- # If we have seen the element at least twice before, and have already extracted it into a subdiagram, we
- # just put in a marker element that refers to the sub-diagram
- ret = EditablePartial.from_call(
- railroad.NonTerminal, text=lookup.diagrams[el_id].kwargs["name"]
- )
- return ret
-
- # Recursively convert child elements
- # Here we find the most relevant Railroad element for matching pyparsing Element
- # We use ``items=[]`` here to hold the place for where the child elements will go once created
- if isinstance(element, pyparsing.And):
- # detect And's created with ``expr*N`` notation - for these use a OneOrMore with a repeat
- # (all will have the same name, and resultsName)
- if not exprs:
- return None
- if len(set((e.name, e.resultsName) for e in exprs)) == 1:
- ret = EditablePartial.from_call(
- railroad.OneOrMore, item="", repeat=str(len(exprs))
- )
- elif _should_vertical(vertical, exprs):
- ret = EditablePartial.from_call(railroad.Stack, items=[])
- else:
- ret = EditablePartial.from_call(railroad.Sequence, items=[])
- elif isinstance(element, (pyparsing.Or, pyparsing.MatchFirst)):
- if not exprs:
- return None
- if _should_vertical(vertical, exprs):
- ret = EditablePartial.from_call(railroad.Choice, 0, items=[])
- else:
- ret = EditablePartial.from_call(railroad.HorizontalChoice, items=[])
- elif isinstance(element, pyparsing.Each):
- if not exprs:
- return None
- ret = EditablePartial.from_call(EachItem, items=[])
- elif isinstance(element, pyparsing.NotAny):
- ret = EditablePartial.from_call(AnnotatedItem, label="NOT", item="")
- elif isinstance(element, pyparsing.FollowedBy):
- ret = EditablePartial.from_call(AnnotatedItem, label="LOOKAHEAD", item="")
- elif isinstance(element, pyparsing.PrecededBy):
- ret = EditablePartial.from_call(AnnotatedItem, label="LOOKBEHIND", item="")
- elif isinstance(element, pyparsing.Group):
- if show_groups:
- ret = EditablePartial.from_call(AnnotatedItem, label="", item="")
- else:
- ret = EditablePartial.from_call(railroad.Group, label="", item="")
- elif isinstance(element, pyparsing.TokenConverter):
- ret = EditablePartial.from_call(
- AnnotatedItem, label=type(element).__name__.lower(), item=""
- )
- elif isinstance(element, pyparsing.Opt):
- ret = EditablePartial.from_call(railroad.Optional, item="")
- elif isinstance(element, pyparsing.OneOrMore):
- ret = EditablePartial.from_call(railroad.OneOrMore, item="")
- elif isinstance(element, pyparsing.ZeroOrMore):
- ret = EditablePartial.from_call(railroad.ZeroOrMore, item="")
- elif isinstance(element, pyparsing.Group):
- ret = EditablePartial.from_call(
- railroad.Group, item=None, label=element_results_name
- )
- elif isinstance(element, pyparsing.Empty) and not element.customName:
- # Skip unnamed "Empty" elements
- ret = None
- elif len(exprs) > 1:
- ret = EditablePartial.from_call(railroad.Sequence, items=[])
- elif len(exprs) > 0 and not element_results_name:
- ret = EditablePartial.from_call(railroad.Group, item="", label=name)
- else:
- terminal = EditablePartial.from_call(railroad.Terminal, element.defaultName)
- ret = terminal
-
- if ret is None:
- return
-
- # Indicate this element's position in the tree so we can extract it if necessary
- lookup[el_id] = ElementState(
- element=element,
- converted=ret,
- parent=parent,
- parent_index=index,
- number=lookup.generate_index(),
- )
- if element.customName:
- lookup[el_id].mark_for_extraction(el_id, lookup, element.customName)
-
- i = 0
- for expr in exprs:
- # Add a placeholder index in case we have to extract the child before we even add it to the parent
- if "items" in ret.kwargs:
- ret.kwargs["items"].insert(i, None)
-
- item = _to_diagram_element(
- expr,
- parent=ret,
- lookup=lookup,
- vertical=vertical,
- index=i,
- show_results_names=show_results_names,
- show_groups=show_groups,
- )
-
- # Some elements don't need to be shown in the diagram
- if item is not None:
- if "item" in ret.kwargs:
- ret.kwargs["item"] = item
- elif "items" in ret.kwargs:
- # If we've already extracted the child, don't touch this index, since it's occupied by a nonterminal
- ret.kwargs["items"][i] = item
- i += 1
- elif "items" in ret.kwargs:
- # If we're supposed to skip this element, remove it from the parent
- del ret.kwargs["items"][i]
-
- # If all this items children are none, skip this item
- if ret and (
- ("items" in ret.kwargs and len(ret.kwargs["items"]) == 0)
- or ("item" in ret.kwargs and ret.kwargs["item"] is None)
- ):
- ret = EditablePartial.from_call(railroad.Terminal, name)
-
- # Mark this element as "complete", ie it has all of its children
- if el_id in lookup:
- lookup[el_id].complete = True
-
- if el_id in lookup and lookup[el_id].extract and lookup[el_id].complete:
- lookup.extract_into_diagram(el_id)
- if ret is not None:
- ret = EditablePartial.from_call(
- railroad.NonTerminal, text=lookup.diagrams[el_id].kwargs["name"]
- )
-
- return ret
diff --git a/spaces/Realcat/image-matching-webui/third_party/ASpanFormer/configs/data/megadepth_trainval_832.py b/spaces/Realcat/image-matching-webui/third_party/ASpanFormer/configs/data/megadepth_trainval_832.py
deleted file mode 100644
index 48b9bd095d64c681d0e64ee9416fb63fbd1f27b5..0000000000000000000000000000000000000000
--- a/spaces/Realcat/image-matching-webui/third_party/ASpanFormer/configs/data/megadepth_trainval_832.py
+++ /dev/null
@@ -1,26 +0,0 @@
-from configs.data.base import cfg
-
-
-TRAIN_BASE_PATH = "data/megadepth/index"
-cfg.DATASET.TRAINVAL_DATA_SOURCE = "MegaDepth"
-cfg.DATASET.TRAIN_DATA_ROOT = "data/megadepth/train"
-cfg.DATASET.TRAIN_NPZ_ROOT = f"{TRAIN_BASE_PATH}/scene_info_0.1_0.7"
-cfg.DATASET.TRAIN_LIST_PATH = f"{TRAIN_BASE_PATH}/trainvaltest_list/train_list.txt"
-cfg.DATASET.MIN_OVERLAP_SCORE_TRAIN = 0.0
-
-TEST_BASE_PATH = "data/megadepth/index"
-cfg.DATASET.TEST_DATA_SOURCE = "MegaDepth"
-cfg.DATASET.VAL_DATA_ROOT = cfg.DATASET.TEST_DATA_ROOT = "data/megadepth/test"
-cfg.DATASET.VAL_NPZ_ROOT = (
- cfg.DATASET.TEST_NPZ_ROOT
-) = f"{TEST_BASE_PATH}/scene_info_val_1500"
-cfg.DATASET.VAL_LIST_PATH = (
- cfg.DATASET.TEST_LIST_PATH
-) = f"{TEST_BASE_PATH}/trainvaltest_list/val_list.txt"
-cfg.DATASET.MIN_OVERLAP_SCORE_TEST = 0.0 # for both test and val
-
-# 368 scenes in total for MegaDepth
-# (with difficulty balanced (further split each scene to 3 sub-scenes))
-cfg.TRAINER.N_SAMPLES_PER_SUBSET = 100
-
-cfg.DATASET.MGDPT_IMG_RESIZE = 832 # for training on 32GB meme GPUs
diff --git a/spaces/Realcat/image-matching-webui/third_party/SGMNet/datadump/dumper/base_dumper.py b/spaces/Realcat/image-matching-webui/third_party/SGMNet/datadump/dumper/base_dumper.py
deleted file mode 100644
index 039c565d9afcb744d30594f3697d45e8d1f234f9..0000000000000000000000000000000000000000
--- a/spaces/Realcat/image-matching-webui/third_party/SGMNet/datadump/dumper/base_dumper.py
+++ /dev/null
@@ -1,128 +0,0 @@
-from abc import ABCMeta, abstractmethod
-import os
-import h5py
-import numpy as np
-from tqdm import trange
-from torch.multiprocessing import Pool, set_start_method
-
-set_start_method("spawn", force=True)
-
-import sys
-
-ROOT_DIR = os.path.abspath(os.path.join(os.path.dirname(__file__), "../../"))
-sys.path.insert(0, ROOT_DIR)
-from components import load_component
-
-
-class BaseDumper(metaclass=ABCMeta):
- def __init__(self, config):
- self.config = config
- self.img_seq = []
- self.dump_seq = [] # feature dump seq
-
- @abstractmethod
- def get_seqs(self):
- raise NotImplementedError
-
- @abstractmethod
- def format_dump_folder(self):
- raise NotImplementedError
-
- @abstractmethod
- def format_dump_data(self):
- raise NotImplementedError
-
- def initialize(self):
- self.extractor = load_component(
- "extractor", self.config["extractor"]["name"], self.config["extractor"]
- )
- self.get_seqs()
- self.format_dump_folder()
-
- def extract(self, index):
- img_path, dump_path = self.img_seq[index], self.dump_seq[index]
- if not self.config["extractor"]["overwrite"] and os.path.exists(dump_path):
- return
- kp, desc = self.extractor.run(img_path)
- self.write_feature(kp, desc, dump_path)
-
- def dump_feature(self):
- print("Extrating features...")
- self.num_img = len(self.dump_seq)
- pool = Pool(self.config["extractor"]["num_process"])
- iteration_num = self.num_img // self.config["extractor"]["num_process"]
- if self.num_img % self.config["extractor"]["num_process"] != 0:
- iteration_num += 1
- for index in trange(iteration_num):
- indicies_list = range(
- index * self.config["extractor"]["num_process"],
- min(
- (index + 1) * self.config["extractor"]["num_process"], self.num_img
- ),
- )
- pool.map(self.extract, indicies_list)
- pool.close()
- pool.join()
-
- def write_feature(self, pts, desc, filename):
- with h5py.File(filename, "w") as ifp:
- ifp.create_dataset("keypoints", pts.shape, dtype=np.float32)
- ifp.create_dataset("descriptors", desc.shape, dtype=np.float32)
- ifp["keypoints"][:] = pts
- ifp["descriptors"][:] = desc
-
- def form_standard_dataset(self):
- dataset_path = os.path.join(
- self.config["dataset_dump_dir"],
- self.config["data_name"]
- + "_"
- + self.config["extractor"]["name"]
- + "_"
- + str(self.config["extractor"]["num_kpt"])
- + ".hdf5",
- )
-
- pair_data_type = ["K1", "K2", "R", "T", "e", "f"]
- num_pairs = len(self.data["K1"])
- with h5py.File(dataset_path, "w") as f:
- print("collecting pair info...")
- for type in pair_data_type:
- dg = f.create_group(type)
- for idx in range(num_pairs):
- data_item = np.asarray(self.data[type][idx])
- dg.create_dataset(
- str(idx), data_item.shape, data_item.dtype, data=data_item
- )
-
- for type in ["img_path1", "img_path2"]:
- dg = f.create_group(type)
- for idx in range(num_pairs):
- dg.create_dataset(
- str(idx),
- [1],
- h5py.string_dtype(encoding="ascii"),
- data=self.data[type][idx].encode("ascii"),
- )
-
- # dump desc
- print("collecting desc and kpt...")
- desc1_g, desc2_g, kpt1_g, kpt2_g = (
- f.create_group("desc1"),
- f.create_group("desc2"),
- f.create_group("kpt1"),
- f.create_group("kpt2"),
- )
- for idx in trange(num_pairs):
- desc_file1, desc_file2 = h5py.File(
- self.data["fea_path1"][idx], "r"
- ), h5py.File(self.data["fea_path2"][idx], "r")
- desc1, desc2, kpt1, kpt2 = (
- desc_file1["descriptors"][()],
- desc_file2["descriptors"][()],
- desc_file1["keypoints"][()],
- desc_file2["keypoints"][()],
- )
- desc1_g.create_dataset(str(idx), desc1.shape, desc1.dtype, data=desc1)
- desc2_g.create_dataset(str(idx), desc2.shape, desc2.dtype, data=desc2)
- kpt1_g.create_dataset(str(idx), kpt1.shape, kpt1.dtype, data=kpt1)
- kpt2_g.create_dataset(str(idx), kpt2.shape, kpt2.dtype, data=kpt2)
diff --git a/spaces/Sarst/VITS-Umamusume-voice-synthesizer2/utils.py b/spaces/Sarst/VITS-Umamusume-voice-synthesizer2/utils.py
deleted file mode 100644
index 9794e0fc3463a5e8fad05c037cce64683059a6d3..0000000000000000000000000000000000000000
--- a/spaces/Sarst/VITS-Umamusume-voice-synthesizer2/utils.py
+++ /dev/null
@@ -1,226 +0,0 @@
-import os
-import glob
-import sys
-import argparse
-import logging
-import json
-import subprocess
-import numpy as np
-from scipy.io.wavfile import read
-import torch
-
-MATPLOTLIB_FLAG = False
-
-logging.basicConfig(stream=sys.stdout, level=logging.ERROR)
-logger = logging
-
-
-def load_checkpoint(checkpoint_path, model, optimizer=None):
- assert os.path.isfile(checkpoint_path)
- checkpoint_dict = torch.load(checkpoint_path, map_location='cpu')
- iteration = checkpoint_dict['iteration']
- learning_rate = checkpoint_dict['learning_rate']
- if optimizer is not None:
- optimizer.load_state_dict(checkpoint_dict['optimizer'])
- saved_state_dict = checkpoint_dict['model']
- if hasattr(model, 'module'):
- state_dict = model.module.state_dict()
- else:
- state_dict = model.state_dict()
- new_state_dict = {}
- for k, v in state_dict.items():
- try:
- new_state_dict[k] = saved_state_dict[k]
- except:
- logger.info("%s is not in the checkpoint" % k)
- new_state_dict[k] = v
- if hasattr(model, 'module'):
- model.module.load_state_dict(new_state_dict)
- else:
- model.load_state_dict(new_state_dict)
- logger.info("Loaded checkpoint '{}' (iteration {})".format(
- checkpoint_path, iteration))
- return model, optimizer, learning_rate, iteration
-
-
-def plot_spectrogram_to_numpy(spectrogram):
- global MATPLOTLIB_FLAG
- if not MATPLOTLIB_FLAG:
- import matplotlib
- matplotlib.use("Agg")
- MATPLOTLIB_FLAG = True
- mpl_logger = logging.getLogger('matplotlib')
- mpl_logger.setLevel(logging.WARNING)
- import matplotlib.pylab as plt
- import numpy as np
-
- fig, ax = plt.subplots(figsize=(10, 2))
- im = ax.imshow(spectrogram, aspect="auto", origin="lower",
- interpolation='none')
- plt.colorbar(im, ax=ax)
- plt.xlabel("Frames")
- plt.ylabel("Channels")
- plt.tight_layout()
-
- fig.canvas.draw()
- data = np.fromstring(fig.canvas.tostring_rgb(), dtype=np.uint8, sep='')
- data = data.reshape(fig.canvas.get_width_height()[::-1] + (3,))
- plt.close()
- return data
-
-
-def plot_alignment_to_numpy(alignment, info=None):
- global MATPLOTLIB_FLAG
- if not MATPLOTLIB_FLAG:
- import matplotlib
- matplotlib.use("Agg")
- MATPLOTLIB_FLAG = True
- mpl_logger = logging.getLogger('matplotlib')
- mpl_logger.setLevel(logging.WARNING)
- import matplotlib.pylab as plt
- import numpy as np
-
- fig, ax = plt.subplots(figsize=(6, 4))
- im = ax.imshow(alignment.transpose(), aspect='auto', origin='lower',
- interpolation='none')
- fig.colorbar(im, ax=ax)
- xlabel = 'Decoder timestep'
- if info is not None:
- xlabel += '\n\n' + info
- plt.xlabel(xlabel)
- plt.ylabel('Encoder timestep')
- plt.tight_layout()
-
- fig.canvas.draw()
- data = np.fromstring(fig.canvas.tostring_rgb(), dtype=np.uint8, sep='')
- data = data.reshape(fig.canvas.get_width_height()[::-1] + (3,))
- plt.close()
- return data
-
-
-def load_wav_to_torch(full_path):
- sampling_rate, data = read(full_path)
- return torch.FloatTensor(data.astype(np.float32)), sampling_rate
-
-
-def load_filepaths_and_text(filename, split="|"):
- with open(filename, encoding='utf-8') as f:
- filepaths_and_text = [line.strip().split(split) for line in f]
- return filepaths_and_text
-
-
-def get_hparams(init=True):
- parser = argparse.ArgumentParser()
- parser.add_argument('-c', '--config', type=str, default="./configs/base.json",
- help='JSON file for configuration')
- parser.add_argument('-m', '--model', type=str, required=True,
- help='Model name')
-
- args = parser.parse_args()
- model_dir = os.path.join("./logs", args.model)
-
- if not os.path.exists(model_dir):
- os.makedirs(model_dir)
-
- config_path = args.config
- config_save_path = os.path.join(model_dir, "config.json")
- if init:
- with open(config_path, "r") as f:
- data = f.read()
- with open(config_save_path, "w") as f:
- f.write(data)
- else:
- with open(config_save_path, "r") as f:
- data = f.read()
- config = json.loads(data)
-
- hparams = HParams(**config)
- hparams.model_dir = model_dir
- return hparams
-
-
-def get_hparams_from_dir(model_dir):
- config_save_path = os.path.join(model_dir, "config.json")
- with open(config_save_path, "r") as f:
- data = f.read()
- config = json.loads(data)
-
- hparams = HParams(**config)
- hparams.model_dir = model_dir
- return hparams
-
-
-def get_hparams_from_file(config_path):
- with open(config_path, "r", encoding="utf-8") as f:
- data = f.read()
- config = json.loads(data)
-
- hparams = HParams(**config)
- return hparams
-
-
-def check_git_hash(model_dir):
- source_dir = os.path.dirname(os.path.realpath(__file__))
- if not os.path.exists(os.path.join(source_dir, ".git")):
- logger.warn("{} is not a git repository, therefore hash value comparison will be ignored.".format(
- source_dir
- ))
- return
-
- cur_hash = subprocess.getoutput("git rev-parse HEAD")
-
- path = os.path.join(model_dir, "githash")
- if os.path.exists(path):
- saved_hash = open(path).read()
- if saved_hash != cur_hash:
- logger.warn("git hash values are different. {}(saved) != {}(current)".format(
- saved_hash[:8], cur_hash[:8]))
- else:
- open(path, "w").write(cur_hash)
-
-
-def get_logger(model_dir, filename="train.log"):
- global logger
- logger = logging.getLogger(os.path.basename(model_dir))
- logger.setLevel(logging.DEBUG)
-
- formatter = logging.Formatter("%(asctime)s\t%(name)s\t%(levelname)s\t%(message)s")
- if not os.path.exists(model_dir):
- os.makedirs(model_dir)
- h = logging.FileHandler(os.path.join(model_dir, filename))
- h.setLevel(logging.DEBUG)
- h.setFormatter(formatter)
- logger.addHandler(h)
- return logger
-
-
-class HParams():
- def __init__(self, **kwargs):
- for k, v in kwargs.items():
- if type(v) == dict:
- v = HParams(**v)
- self[k] = v
-
- def keys(self):
- return self.__dict__.keys()
-
- def items(self):
- return self.__dict__.items()
-
- def values(self):
- return self.__dict__.values()
-
- def __len__(self):
- return len(self.__dict__)
-
- def __getitem__(self, key):
- return getattr(self, key)
-
- def __setitem__(self, key, value):
- return setattr(self, key, value)
-
- def __contains__(self, key):
- return key in self.__dict__
-
- def __repr__(self):
- return self.__dict__.__repr__()
\ No newline at end of file
diff --git a/spaces/SeViLA/SeViLA/lavis/common/vqa_tools/vqa_eval.py b/spaces/SeViLA/SeViLA/lavis/common/vqa_tools/vqa_eval.py
deleted file mode 100644
index ee808b349bb6166c744338b02af2bc84a68650ff..0000000000000000000000000000000000000000
--- a/spaces/SeViLA/SeViLA/lavis/common/vqa_tools/vqa_eval.py
+++ /dev/null
@@ -1,324 +0,0 @@
-"""
- Copyright (c) 2022, salesforce.com, inc.
- All rights reserved.
- SPDX-License-Identifier: BSD-3-Clause
- For full license text, see the LICENSE file in the repo root or https://opensource.org/licenses/BSD-3-Clause
-"""
-
-# coding=utf-8
-
-__author__ = "aagrawal"
-
-# This code is based on the code written by Tsung-Yi Lin for MSCOCO Python API available at the following link:
-# (https://github.com/tylin/coco-caption/blob/master/pycocoevalcap/eval.py).
-import sys
-import re
-
-
-class VQAEval:
- def __init__(self, vqa=None, vqaRes=None, n=2):
- self.n = n
- self.accuracy = {}
- self.evalQA = {}
- self.evalQuesType = {}
- self.evalAnsType = {}
- self.vqa = vqa
- self.vqaRes = vqaRes
- if vqa is not None:
- self.params = {"question_id": vqa.getQuesIds()}
- self.contractions = {
- "aint": "ain't",
- "arent": "aren't",
- "cant": "can't",
- "couldve": "could've",
- "couldnt": "couldn't",
- "couldn'tve": "couldn't've",
- "couldnt've": "couldn't've",
- "didnt": "didn't",
- "doesnt": "doesn't",
- "dont": "don't",
- "hadnt": "hadn't",
- "hadnt've": "hadn't've",
- "hadn'tve": "hadn't've",
- "hasnt": "hasn't",
- "havent": "haven't",
- "hed": "he'd",
- "hed've": "he'd've",
- "he'dve": "he'd've",
- "hes": "he's",
- "howd": "how'd",
- "howll": "how'll",
- "hows": "how's",
- "Id've": "I'd've",
- "I'dve": "I'd've",
- "Im": "I'm",
- "Ive": "I've",
- "isnt": "isn't",
- "itd": "it'd",
- "itd've": "it'd've",
- "it'dve": "it'd've",
- "itll": "it'll",
- "let's": "let's",
- "maam": "ma'am",
- "mightnt": "mightn't",
- "mightnt've": "mightn't've",
- "mightn'tve": "mightn't've",
- "mightve": "might've",
- "mustnt": "mustn't",
- "mustve": "must've",
- "neednt": "needn't",
- "notve": "not've",
- "oclock": "o'clock",
- "oughtnt": "oughtn't",
- "ow's'at": "'ow's'at",
- "'ows'at": "'ow's'at",
- "'ow'sat": "'ow's'at",
- "shant": "shan't",
- "shed've": "she'd've",
- "she'dve": "she'd've",
- "she's": "she's",
- "shouldve": "should've",
- "shouldnt": "shouldn't",
- "shouldnt've": "shouldn't've",
- "shouldn'tve": "shouldn't've",
- "somebody'd": "somebodyd",
- "somebodyd've": "somebody'd've",
- "somebody'dve": "somebody'd've",
- "somebodyll": "somebody'll",
- "somebodys": "somebody's",
- "someoned": "someone'd",
- "someoned've": "someone'd've",
- "someone'dve": "someone'd've",
- "someonell": "someone'll",
- "someones": "someone's",
- "somethingd": "something'd",
- "somethingd've": "something'd've",
- "something'dve": "something'd've",
- "somethingll": "something'll",
- "thats": "that's",
- "thered": "there'd",
- "thered've": "there'd've",
- "there'dve": "there'd've",
- "therere": "there're",
- "theres": "there's",
- "theyd": "they'd",
- "theyd've": "they'd've",
- "they'dve": "they'd've",
- "theyll": "they'll",
- "theyre": "they're",
- "theyve": "they've",
- "twas": "'twas",
- "wasnt": "wasn't",
- "wed've": "we'd've",
- "we'dve": "we'd've",
- "weve": "we've",
- "werent": "weren't",
- "whatll": "what'll",
- "whatre": "what're",
- "whats": "what's",
- "whatve": "what've",
- "whens": "when's",
- "whered": "where'd",
- "wheres": "where's",
- "whereve": "where've",
- "whod": "who'd",
- "whod've": "who'd've",
- "who'dve": "who'd've",
- "wholl": "who'll",
- "whos": "who's",
- "whove": "who've",
- "whyll": "why'll",
- "whyre": "why're",
- "whys": "why's",
- "wont": "won't",
- "wouldve": "would've",
- "wouldnt": "wouldn't",
- "wouldnt've": "wouldn't've",
- "wouldn'tve": "wouldn't've",
- "yall": "y'all",
- "yall'll": "y'all'll",
- "y'allll": "y'all'll",
- "yall'd've": "y'all'd've",
- "y'alld've": "y'all'd've",
- "y'all'dve": "y'all'd've",
- "youd": "you'd",
- "youd've": "you'd've",
- "you'dve": "you'd've",
- "youll": "you'll",
- "youre": "you're",
- "youve": "you've",
- }
- self.manualMap = {
- "none": "0",
- "zero": "0",
- "one": "1",
- "two": "2",
- "three": "3",
- "four": "4",
- "five": "5",
- "six": "6",
- "seven": "7",
- "eight": "8",
- "nine": "9",
- "ten": "10",
- }
- self.articles = ["a", "an", "the"]
-
- self.periodStrip = re.compile("(?!<=\d)(\.)(?!\d)")
- self.commaStrip = re.compile("(\d)(,)(\d)")
- self.punct = [
- ";",
- r"/",
- "[",
- "]",
- '"',
- "{",
- "}",
- "(",
- ")",
- "=",
- "+",
- "\\",
- "_",
- "-",
- ">",
- "<",
- "@",
- "`",
- ",",
- "?",
- "!",
- ]
-
- def evaluate(self, quesIds=None):
- if quesIds == None:
- quesIds = [quesId for quesId in self.params["question_id"]]
- gts = {}
- res = {}
- for quesId in quesIds:
- gts[quesId] = self.vqa.qa[quesId]
- res[quesId] = self.vqaRes.qa[quesId]
-
- # =================================================
- # Compute accuracy
- # =================================================
- accQA = []
- accQuesType = {}
- accAnsType = {}
- print("computing accuracy")
- step = 0
- for quesId in quesIds:
- resAns = res[quesId]["answer"]
- resAns = resAns.replace("\n", " ")
- resAns = resAns.replace("\t", " ")
- resAns = resAns.strip()
- resAns = self.processPunctuation(resAns)
- resAns = self.processDigitArticle(resAns)
- gtAcc = []
- gtAnswers = [ans["answer"] for ans in gts[quesId]["answers"]]
- if len(set(gtAnswers)) > 1:
- for ansDic in gts[quesId]["answers"]:
- ansDic["answer"] = self.processPunctuation(ansDic["answer"])
- for gtAnsDatum in gts[quesId]["answers"]:
- otherGTAns = [
- item for item in gts[quesId]["answers"] if item != gtAnsDatum
- ]
- matchingAns = [item for item in otherGTAns if item["answer"] == resAns]
- acc = min(1, float(len(matchingAns)) / 3)
- gtAcc.append(acc)
- quesType = gts[quesId]["question_type"]
- ansType = gts[quesId]["answer_type"]
- avgGTAcc = float(sum(gtAcc)) / len(gtAcc)
- accQA.append(avgGTAcc)
- if quesType not in accQuesType:
- accQuesType[quesType] = []
- accQuesType[quesType].append(avgGTAcc)
- if ansType not in accAnsType:
- accAnsType[ansType] = []
- accAnsType[ansType].append(avgGTAcc)
- self.setEvalQA(quesId, avgGTAcc)
- self.setEvalQuesType(quesId, quesType, avgGTAcc)
- self.setEvalAnsType(quesId, ansType, avgGTAcc)
- if step % 100 == 0:
- self.updateProgress(step / float(len(quesIds)))
- step = step + 1
-
- self.setAccuracy(accQA, accQuesType, accAnsType)
- print("Done computing accuracy")
-
- def processPunctuation(self, inText):
- outText = inText
- for p in self.punct:
- if (p + " " in inText or " " + p in inText) or (
- re.search(self.commaStrip, inText) != None
- ):
- outText = outText.replace(p, "")
- else:
- outText = outText.replace(p, " ")
- outText = self.periodStrip.sub("", outText, re.UNICODE)
- return outText
-
- def processDigitArticle(self, inText):
- outText = []
- tempText = inText.lower().split()
- for word in tempText:
- word = self.manualMap.setdefault(word, word)
- if word not in self.articles:
- outText.append(word)
- else:
- pass
- for wordId, word in enumerate(outText):
- if word in self.contractions:
- outText[wordId] = self.contractions[word]
- outText = " ".join(outText)
- return outText
-
- def setAccuracy(self, accQA, accQuesType, accAnsType):
- self.accuracy["overall"] = round(100 * float(sum(accQA)) / len(accQA), self.n)
- self.accuracy["perQuestionType"] = {
- quesType: round(
- 100 * float(sum(accQuesType[quesType])) / len(accQuesType[quesType]),
- self.n,
- )
- for quesType in accQuesType
- }
- self.accuracy["perAnswerType"] = {
- ansType: round(
- 100 * float(sum(accAnsType[ansType])) / len(accAnsType[ansType]), self.n
- )
- for ansType in accAnsType
- }
-
- def setEvalQA(self, quesId, acc):
- self.evalQA[quesId] = round(100 * acc, self.n)
-
- def setEvalQuesType(self, quesId, quesType, acc):
- if quesType not in self.evalQuesType:
- self.evalQuesType[quesType] = {}
- self.evalQuesType[quesType][quesId] = round(100 * acc, self.n)
-
- def setEvalAnsType(self, quesId, ansType, acc):
- if ansType not in self.evalAnsType:
- self.evalAnsType[ansType] = {}
- self.evalAnsType[ansType][quesId] = round(100 * acc, self.n)
-
- def updateProgress(self, progress):
- barLength = 20
- status = ""
- if isinstance(progress, int):
- progress = float(progress)
- if not isinstance(progress, float):
- progress = 0
- status = "error: progress var must be float\r\n"
- if progress < 0:
- progress = 0
- status = "Halt...\r\n"
- if progress >= 1:
- progress = 1
- status = "Done...\r\n"
- block = int(round(barLength * progress))
- text = "\rFinshed Percent: [{0}] {1}% {2}".format(
- "#" * block + "-" * (barLength - block), int(progress * 100), status
- )
- sys.stdout.write(text)
- sys.stdout.flush()
diff --git a/spaces/SeViLA/SeViLA/lavis/datasets/download_scripts/DownloadConceptualCaptions/download_data_cc3m.py b/spaces/SeViLA/SeViLA/lavis/datasets/download_scripts/DownloadConceptualCaptions/download_data_cc3m.py
deleted file mode 100644
index 2edd7a224436f7fa2d923501caadd40db040f8a1..0000000000000000000000000000000000000000
--- a/spaces/SeViLA/SeViLA/lavis/datasets/download_scripts/DownloadConceptualCaptions/download_data_cc3m.py
+++ /dev/null
@@ -1,229 +0,0 @@
-"""
- Copyright (c) 2022, salesforce.com, inc.
- All rights reserved.
- SPDX-License-Identifier: BSD-3-Clause
- For full license text, see the LICENSE file in the repo root or https://opensource.org/licenses/BSD-3-Clause
-"""
-
-import glob
-from pathlib import Path
-import time
-from omegaconf import OmegaConf
-import pandas as pd
-import numpy as np
-import requests
-import zlib
-import os
-import io
-import shelve
-from lavis.common.utils import get_abs_path, get_cache_path
-import magic # pip install python-magic
-import json
-from multiprocessing import Pool
-from tqdm import tqdm
-from PIL import Image
-from torchvision.transforms import functional as TF
-
-headers = {
- #'User-Agent': 'Mozilla/5.0 (X11; Linux x86_64) AppleWebKit/537.36 (KHTML, like Gecko) Chrome/71.0.3578.98 Safari/537.36',
- "User-Agent": "Googlebot-Image/1.0", # Pretend to be googlebot
- "X-Forwarded-For": "64.18.15.200",
-}
-
-
-def _df_split_apply(tup_arg):
- split_ind, subset, func = tup_arg
- r = subset.apply(func, axis=1)
- return (split_ind, r)
-
-
-def df_multiprocess(df, processes, chunk_size, func, dataset_name):
- print("Generating parts...")
- with shelve.open(
- "%s_%s_%s_results.tmp" % (dataset_name, func.__name__, chunk_size)
- ) as results:
-
- pbar = tqdm(total=len(df), position=0)
- # Resume:
- finished_chunks = set([int(k) for k in results.keys()])
- pbar.desc = "Resuming"
- for k in results.keys():
- pbar.update(len(results[str(k)][1]))
-
- pool_data = (
- (index, df[i : i + chunk_size], func)
- for index, i in enumerate(range(0, len(df), chunk_size))
- if index not in finished_chunks
- )
- print(
- int(len(df) / chunk_size),
- "parts.",
- chunk_size,
- "per part.",
- "Using",
- processes,
- "processes",
- )
-
- pbar.desc = "Downloading"
- with Pool(processes) as pool:
- for i, result in enumerate(
- pool.imap_unordered(_df_split_apply, pool_data, 2)
- ):
- results[str(result[0])] = result
- pbar.update(len(result[1]))
- pbar.close()
-
- print("Finished Downloading.")
- return
-
-
-# Unique name based on url
-def _file_name(row):
- name = (
- "%s/%s_%s"
- % (
- # row["folder"],
- storage_dir,
- row.name,
- (zlib.crc32(row["url"].encode("utf-8")) & 0xFFFFFFFF),
- )
- + ".jpg"
- )
- return name
-
-
-# For checking mimetypes separately without download
-def check_mimetype(row):
- if os.path.isfile(str(row["file"])):
- row["mimetype"] = magic.from_file(row["file"], mime=True)
- row["size"] = os.stat(row["file"]).st_size
- return row
-
-
-# Don't download image, just check with a HEAD request, can't resume.
-# Can use this instead of download_image to get HTTP status codes.
-def check_download(row):
- fname = _file_name(row)
- try:
- # not all sites will support HEAD
- response = requests.head(
- row["url"], stream=False, timeout=5, allow_redirects=True, headers=headers
- )
- row["status"] = response.status_code
- row["headers"] = dict(response.headers)
- except:
- # log errors later, set error as 408 timeout
- row["status"] = 408
- return row
- if response.ok:
- row["file"] = fname
- return row
-
-
-def resize_img(req):
- image = Image.open(req).convert("RGB")
- image = TF.resize(
- # image, size=(resize_size, resize_size)
- image,
- size=resize_size,
- ) # , interpolation=Image.LANCZOS)
- return image
-
-
-def download_image(row):
- fname = _file_name(row)
- # Skip Already downloaded, retry others later
- if os.path.isfile(fname):
- row["status"] = 200
- row["file"] = fname
- row["mimetype"] = magic.from_file(row["file"], mime=True)
- row["size"] = os.stat(row["file"]).st_size
- return row
-
- try:
- # use smaller timeout to skip errors, but can result in failed downloads
- response = requests.get(
- row["url"], stream=False, timeout=5, allow_redirects=True, headers=headers
- )
- row["status"] = response.status_code
- # row['headers'] = dict(response.headers)
- except Exception as e:
- # log errors later, set error as 408 timeout
- row["status"] = 408
- return row
-
- if response.ok:
- try:
- # some sites respond with gzip transport encoding
- response.raw.decode_content = True
- img = resize_img(io.BytesIO(response.content))
- img.save(fname)
-
- row["mimetype"] = magic.from_file(fname, mime=True)
- row["size"] = os.stat(fname).st_size
-
- except Exception as e:
- # # This is if it times out during a download or decode
- row["status"] = 408
-
- row["file"] = fname
- return row
-
-
-def open_tsv(fname, folder):
- print("Opening %s Data File..." % fname)
- df = pd.read_csv(
- fname, sep="\t", names=["caption", "url"]
- ) # , usecols=range(1, 2))
- df["folder"] = folder
- print("Processing", len(df), " Images:")
- return df
-
-
-def df_from_shelve(chunk_size, func, dataset_name):
- print("Generating Dataframe from results...")
- with shelve.open(
- "%s_%s_%s_results.tmp" % (dataset_name, func.__name__, chunk_size)
- ) as results:
- keylist = sorted([int(k) for k in results.keys()])
- df = pd.concat([results[str(k)][1] for k in keylist], sort=True)
- return df
-
-
-resize_size = 384
-
-config_path = get_abs_path("configs/datasets/conceptual_caption/defaults_3m.yaml")
-
-storage_dir = OmegaConf.load(
- config_path
-).datasets.conceptual_caption_3m.build_info.images.storage
-storage_dir = Path(get_cache_path(storage_dir))
-
-os.makedirs(storage_dir, exist_ok=True)
-
-# number of processes in the pool can be larger than cores
-num_processes = 32
-# chunk_size is how many images per chunk per process - changing this resets progress when restarting.
-images_per_part = 100
-
-data_name = "cc3m"
-df = open_tsv("Train_GCC-training.tsv", data_name)
-df_multiprocess(
- df=df,
- processes=num_processes,
- chunk_size=images_per_part,
- func=download_image,
- dataset_name=data_name,
-)
-df = df_from_shelve(
- chunk_size=images_per_part, func=download_image, dataset_name=data_name
-)
-df.to_csv(
- "downloaded_%s_report.tsv.gz" % data_name,
- compression="gzip",
- sep="\t",
- header=False,
- index=False,
-)
-print("Saved.")
diff --git a/spaces/ServerX/PorcoDiaz/demucs/model.py b/spaces/ServerX/PorcoDiaz/demucs/model.py
deleted file mode 100644
index e9d932f4d014f7b95b394d2e24ed5edc379ded8d..0000000000000000000000000000000000000000
--- a/spaces/ServerX/PorcoDiaz/demucs/model.py
+++ /dev/null
@@ -1,202 +0,0 @@
-# Copyright (c) Facebook, Inc. and its affiliates.
-# All rights reserved.
-#
-# This source code is licensed under the license found in the
-# LICENSE file in the root directory of this source tree.
-
-import math
-
-import julius
-from torch import nn
-
-from .utils import capture_init, center_trim
-
-
-class BLSTM(nn.Module):
- def __init__(self, dim, layers=1):
- super().__init__()
- self.lstm = nn.LSTM(bidirectional=True, num_layers=layers, hidden_size=dim, input_size=dim)
- self.linear = nn.Linear(2 * dim, dim)
-
- def forward(self, x):
- x = x.permute(2, 0, 1)
- x = self.lstm(x)[0]
- x = self.linear(x)
- x = x.permute(1, 2, 0)
- return x
-
-
-def rescale_conv(conv, reference):
- std = conv.weight.std().detach()
- scale = (std / reference)**0.5
- conv.weight.data /= scale
- if conv.bias is not None:
- conv.bias.data /= scale
-
-
-def rescale_module(module, reference):
- for sub in module.modules():
- if isinstance(sub, (nn.Conv1d, nn.ConvTranspose1d)):
- rescale_conv(sub, reference)
-
-
-class Demucs(nn.Module):
- @capture_init
- def __init__(self,
- sources,
- audio_channels=2,
- channels=64,
- depth=6,
- rewrite=True,
- glu=True,
- rescale=0.1,
- resample=True,
- kernel_size=8,
- stride=4,
- growth=2.,
- lstm_layers=2,
- context=3,
- normalize=False,
- samplerate=44100,
- segment_length=4 * 10 * 44100):
- """
- Args:
- sources (list[str]): list of source names
- audio_channels (int): stereo or mono
- channels (int): first convolution channels
- depth (int): number of encoder/decoder layers
- rewrite (bool): add 1x1 convolution to each encoder layer
- and a convolution to each decoder layer.
- For the decoder layer, `context` gives the kernel size.
- glu (bool): use glu instead of ReLU
- resample_input (bool): upsample x2 the input and downsample /2 the output.
- rescale (int): rescale initial weights of convolutions
- to get their standard deviation closer to `rescale`
- kernel_size (int): kernel size for convolutions
- stride (int): stride for convolutions
- growth (float): multiply (resp divide) number of channels by that
- for each layer of the encoder (resp decoder)
- lstm_layers (int): number of lstm layers, 0 = no lstm
- context (int): kernel size of the convolution in the
- decoder before the transposed convolution. If > 1,
- will provide some context from neighboring time
- steps.
- samplerate (int): stored as meta information for easing
- future evaluations of the model.
- segment_length (int): stored as meta information for easing
- future evaluations of the model. Length of the segments on which
- the model was trained.
- """
-
- super().__init__()
- self.audio_channels = audio_channels
- self.sources = sources
- self.kernel_size = kernel_size
- self.context = context
- self.stride = stride
- self.depth = depth
- self.resample = resample
- self.channels = channels
- self.normalize = normalize
- self.samplerate = samplerate
- self.segment_length = segment_length
-
- self.encoder = nn.ModuleList()
- self.decoder = nn.ModuleList()
-
- if glu:
- activation = nn.GLU(dim=1)
- ch_scale = 2
- else:
- activation = nn.ReLU()
- ch_scale = 1
- in_channels = audio_channels
- for index in range(depth):
- encode = []
- encode += [nn.Conv1d(in_channels, channels, kernel_size, stride), nn.ReLU()]
- if rewrite:
- encode += [nn.Conv1d(channels, ch_scale * channels, 1), activation]
- self.encoder.append(nn.Sequential(*encode))
-
- decode = []
- if index > 0:
- out_channels = in_channels
- else:
- out_channels = len(self.sources) * audio_channels
- if rewrite:
- decode += [nn.Conv1d(channels, ch_scale * channels, context), activation]
- decode += [nn.ConvTranspose1d(channels, out_channels, kernel_size, stride)]
- if index > 0:
- decode.append(nn.ReLU())
- self.decoder.insert(0, nn.Sequential(*decode))
- in_channels = channels
- channels = int(growth * channels)
-
- channels = in_channels
-
- if lstm_layers:
- self.lstm = BLSTM(channels, lstm_layers)
- else:
- self.lstm = None
-
- if rescale:
- rescale_module(self, reference=rescale)
-
- def valid_length(self, length):
- """
- Return the nearest valid length to use with the model so that
- there is no time steps left over in a convolutions, e.g. for all
- layers, size of the input - kernel_size % stride = 0.
-
- If the mixture has a valid length, the estimated sources
- will have exactly the same length when context = 1. If context > 1,
- the two signals can be center trimmed to match.
-
- For training, extracts should have a valid length.For evaluation
- on full tracks we recommend passing `pad = True` to :method:`forward`.
- """
- if self.resample:
- length *= 2
- for _ in range(self.depth):
- length = math.ceil((length - self.kernel_size) / self.stride) + 1
- length = max(1, length)
- length += self.context - 1
- for _ in range(self.depth):
- length = (length - 1) * self.stride + self.kernel_size
-
- if self.resample:
- length = math.ceil(length / 2)
- return int(length)
-
- def forward(self, mix):
- x = mix
-
- if self.normalize:
- mono = mix.mean(dim=1, keepdim=True)
- mean = mono.mean(dim=-1, keepdim=True)
- std = mono.std(dim=-1, keepdim=True)
- else:
- mean = 0
- std = 1
-
- x = (x - mean) / (1e-5 + std)
-
- if self.resample:
- x = julius.resample_frac(x, 1, 2)
-
- saved = []
- for encode in self.encoder:
- x = encode(x)
- saved.append(x)
- if self.lstm:
- x = self.lstm(x)
- for decode in self.decoder:
- skip = center_trim(saved.pop(-1), x)
- x = x + skip
- x = decode(x)
-
- if self.resample:
- x = julius.resample_frac(x, 2, 1)
- x = x * std + mean
- x = x.view(x.size(0), len(self.sources), self.audio_channels, x.size(-1))
- return x
diff --git a/spaces/SimFG/LangChain-Zilliz-Cloud/app.py b/spaces/SimFG/LangChain-Zilliz-Cloud/app.py
deleted file mode 100644
index 27156dd6ee9a5b202376a48d80057c0ce2166b58..0000000000000000000000000000000000000000
--- a/spaces/SimFG/LangChain-Zilliz-Cloud/app.py
+++ /dev/null
@@ -1,123 +0,0 @@
-from typing import Callable, Optional
-
-import gradio as gr
-from langchain.embeddings.openai import OpenAIEmbeddings
-from langchain.vectorstores import Zilliz
-from langchain.document_loaders import WebBaseLoader
-from langchain.text_splitter import CharacterTextSplitter
-from langchain.chains import RetrievalQAWithSourcesChain
-from langchain.llms import OpenAI
-
-chain: Optional[Callable] = None
-
-
-def web_loader(url_list, openai_key, zilliz_uri, user, password):
- if not url_list:
- return "please enter url list"
- loader = WebBaseLoader(url_list.split())
- docs = loader.load()
-
- text_splitter = CharacterTextSplitter(chunk_size=1024, chunk_overlap=0)
- docs = text_splitter.split_documents(docs)
- embeddings = OpenAIEmbeddings(model="ada", openai_api_key=openai_key)
-
- docsearch = Zilliz.from_documents(
- docs,
- embedding=embeddings,
- connection_args={
- "uri": zilliz_uri,
- "user": user,
- "password": password,
- "secure": True,
- },
- )
-
- global chain
- chain = RetrievalQAWithSourcesChain.from_chain_type(
- OpenAI(temperature=0, openai_api_key=openai_key),
- chain_type="map_reduce",
- retriever=docsearch.as_retriever(),
- )
- return "success to load data"
-
-
-def query(question):
- global chain
- # "What is milvus?"
- if not chain:
- return "please load the data first"
- return chain(inputs={"question": question}, return_only_outputs=True).get(
- "answer", "fail to get answer"
- )
-
-
-if __name__ == "__main__":
- block = gr.Blocks()
- with block as demo:
- gr.Markdown(
- """
-
Langchain And Zilliz Cloud Example
- This is how to use Zilliz Cloud as vector store in LangChain.
- The purpose of this example is to allow you to input multiple URLs (separated by newlines) and then ask questions about the content of the corresponding web pages.
-
- ## 📋 Prerequisite:
-
- 1. 🔑 To obtain an OpenAI key, please visit https://platform.openai.com/account/api-keys.
- 2. 💻 Create a Zilliz Cloud account to get free credits for usage by visiting https://cloud.zilliz.com.
- 3. 🗄️ Create a database in Zilliz Cloud.
-
- ## 📝 Steps for usage:
-
- 1. 🖋️ Fill in the url list input box with multiple URLs.
- 2. 🔑 Fill in the OpenAI API key in the openai api key input box.
- 3. 🌩️ Fill in the Zilliz Cloud connection parameters, including the connection URL, corresponding username, and password.
- 4. 🚀 Click the Load Data button to load the data. When the load status text box prompts that the data has been successfully loaded, proceed to the next step.
- 5. ❓ In the question input box, enter the relevant question about the web page.
- 6. 🔍 Click the Generate button to search for the answer to the question. The final answer will be displayed in the question answer text box.
- """
- )
- url_list_text = gr.Textbox(
- label="url list",
- lines=3,
- placeholder="https://milvus.io/docs/overview.md",
- )
- openai_key_text = gr.Textbox(label="openai api key", type="password", placeholder="sk-******")
- with gr.Row():
- zilliz_uri_text = gr.Textbox(
- label="zilliz cloud uri",
- placeholder="https://..vectordb.zillizcloud.com:",
- )
- user_text = gr.Textbox(label="username", placeholder="db_admin")
- password_text = gr.Textbox(
- label="password", type="password", placeholder="******"
- )
- loader_output = gr.Textbox(label="load status")
- loader_btn = gr.Button("Load Data")
- loader_btn.click(
- fn=web_loader,
- inputs=[
- url_list_text,
- openai_key_text,
- zilliz_uri_text,
- user_text,
- password_text,
- ],
- outputs=loader_output,
- api_name="web_load",
- )
-
- question_text = gr.Textbox(
- label="question",
- lines=3,
- placeholder="What is milvus?",
- )
- query_output = gr.Textbox(label="question answer", lines=3)
- query_btn = gr.Button("Generate")
- query_btn.click(
- fn=query,
- inputs=[question_text],
- outputs=query_output,
- api_name="generate_answer",
- )
-
- demo.queue().launch(server_name="0.0.0.0", share=False)
diff --git a/spaces/SkalskiP/SAM_and_MetaCLIP/README.md b/spaces/SkalskiP/SAM_and_MetaCLIP/README.md
deleted file mode 100644
index 07a6b7e34de038a83a0eb830631ba8ebb4e90f1c..0000000000000000000000000000000000000000
--- a/spaces/SkalskiP/SAM_and_MetaCLIP/README.md
+++ /dev/null
@@ -1,12 +0,0 @@
----
-title: SAM And MetaCLIP
-emoji: 👁
-colorFrom: pink
-colorTo: yellow
-sdk: gradio
-sdk_version: 3.50.2
-app_file: app.py
-pinned: false
----
-
-Check out the configuration reference at https://huggingface.co/docs/hub/spaces-config-reference
diff --git a/spaces/Slava917/pronunciation-trainer/README.md b/spaces/Slava917/pronunciation-trainer/README.md
deleted file mode 100644
index a33bec35da8b807a3407feb7b1a6789e6e402a3c..0000000000000000000000000000000000000000
--- a/spaces/Slava917/pronunciation-trainer/README.md
+++ /dev/null
@@ -1,12 +0,0 @@
----
-title: Pronunciation Trainer
-emoji: 📉
-colorFrom: pink
-colorTo: yellow
-sdk: gradio
-sdk_version: 2.7.5.2
-app_file: app.py
-pinned: false
----
-
-Check out the configuration reference at https://huggingface.co/docs/hub/spaces#reference
diff --git a/spaces/Snowling/White-box-Cartoonization/app.py b/spaces/Snowling/White-box-Cartoonization/app.py
deleted file mode 100644
index c55ced56bd87a85f59d1c8ef84b7eca87422720f..0000000000000000000000000000000000000000
--- a/spaces/Snowling/White-box-Cartoonization/app.py
+++ /dev/null
@@ -1,108 +0,0 @@
-#!/usr/bin/env python
-
-from __future__ import annotations
-import argparse
-import functools
-import os
-import pathlib
-import sys
-from typing import Callable
-import uuid
-
-import gradio as gr
-import huggingface_hub
-import numpy as np
-import PIL.Image
-
-from io import BytesIO
-from wbc.cartoonize import Cartoonize
-
-ORIGINAL_REPO_URL = 'https://github.com/SystemErrorWang/White-box-Cartoonization'
-TITLE = 'SystemErrorWang/White-box-Cartoonization'
-DESCRIPTION = f"""This is a demo for {ORIGINAL_REPO_URL}.
-
-"""
-ARTICLE = """
-
-"""
-
-SAFEHASH = [x for x in "0123456789-abcdefghijklmnopqrstuvwxyz_ABCDEFGHIJKLMNOPQRSTUVWXYZ"]
-def compress_UUID():
- '''
- 根据http://www.ietf.org/rfc/rfc1738.txt,由uuid编码扩bai大字符域生成du串
- 包括:[0-9a-zA-Z\-_]共64个
- 长度:(32-2)/3*2=20
- 备注:可在地球上人zhi人都用,使用100年不重复(2^120)
- :return:String
- '''
- row = str(uuid.uuid4()).replace('-', '')
- safe_code = ''
- for i in range(10):
- enbin = "%012d" % int(bin(int(row[i * 3] + row[i * 3 + 1] + row[i * 3 + 2], 16))[2:], 10)
- safe_code += (SAFEHASH[int(enbin[0:6], 2)] + SAFEHASH[int(enbin[6:12], 2)])
- safe_code = safe_code.replace('-', '')
- return safe_code
-
-
-def parse_args() -> argparse.Namespace:
- parser = argparse.ArgumentParser()
- parser.add_argument('--device', type=str, default='cpu')
- parser.add_argument('--theme', type=str)
- parser.add_argument('--live', action='store_true')
- parser.add_argument('--share', action='store_true')
- parser.add_argument('--port', type=int)
- parser.add_argument('--disable-queue',
- dest='enable_queue',
- action='store_false')
- parser.add_argument('--allow-flagging', type=str, default='never')
- parser.add_argument('--allow-screenshot', action='store_true')
- return parser.parse_args()
-
-def run(
- image,
- cartoonize : Cartoonize
-) -> tuple[PIL.Image.Image]:
-
- out_path = compress_UUID()+'.png'
- cartoonize.run_sigle(image.name, out_path)
-
- return PIL.Image.open(out_path)
-
-
-def main():
- gr.close_all()
-
- args = parse_args()
-
- cartoonize = Cartoonize(os.path.join(os.path.dirname(os.path.abspath(__file__)),'wbc/saved_models/'))
-
- func = functools.partial(run, cartoonize=cartoonize)
- func = functools.update_wrapper(func, run)
-
- gr.Interface(
- func,
- [
- gr.inputs.Image(type='file', label='Input Image'),
- ],
- [
- gr.outputs.Image(
- type='pil',
- label='Result'),
- ],
- # examples=examples,
- theme=args.theme,
- title=TITLE,
- description=DESCRIPTION,
- article=ARTICLE,
- allow_screenshot=args.allow_screenshot,
- allow_flagging=args.allow_flagging,
- live=args.live,
- ).launch(
- enable_queue=args.enable_queue,
- server_port=args.port,
- share=args.share,
- )
-
-
-if __name__ == '__main__':
- main()
diff --git a/spaces/Solis/Solis/llm_src/demo.py b/spaces/Solis/Solis/llm_src/demo.py
deleted file mode 100644
index 7f5dc8f0078babf674d4f72e2035b9a03d8e2ddd..0000000000000000000000000000000000000000
--- a/spaces/Solis/Solis/llm_src/demo.py
+++ /dev/null
@@ -1,68 +0,0 @@
-import argparse
-import random
-
-from utils.cot.get_prompt import get_prompt
-from utils.decoder import Decoder, answer_cleansing
-from utils.fp_substitution import fp_substitute, get_nums_from_passage
-from utils.solis.solis_solver import try_search
-from utils.solis.helper import *
-
-
-def demo(decoder: Decoder, x: str, CNT_SUM):
- random.seed(123)
- args = get_default_argument()
- prompt_x = get_prompt()
-
- orig_nums, _ = get_nums_from_passage(x)
- if len(orig_nums) > 3:
- return "Too many operands!"
- orig_x = prompt_x + f"Q: {x}\nA:"
- # step 0, original predict
- try:
- orig_z = decoder.decode(args, orig_x, CNT_SUM)
- orig_z = answer_cleansing(args, orig_z)
- except Exception as e:
- print(e)
- orig_z = "Too Frequent!"
- return orig_z
-
- # step 1, #TODO skip operand proposal
- # step 2, substitute
- fp_data_list = fp_substitute(x, args.substitute_time)
- fp_results = []
- for fp_data in fp_data_list:
- fp_x = prompt_x + f"Q: {fp_data['Question']}\nA:"
- try:
- fp_z = decoder.decode(args, fp_x, CNT_SUM)
- fp_z = answer_cleansing(args, fp_z)
- except Exception as e:
- print(e)
- fp_z = "Too Frequent!"
- return fp_z
- fp_results.append({
- "fp_nums": fp_data["Alignments"],
- "fp_z": fp_z,
- })
- # step 3, arith relationship inversion
- solis_ret = try_search(args, orig_nums, fp_results)
- print(solis_ret)
- return solis_ret
-
-def get_default_argument():
- parser = argparse.ArgumentParser(description="Solis")
- parser.add_argument("--seed", type=int, default=123)
- parser.add_argument("--api_time_interval", type=float, default=2)
- parser.add_argument("--max_length", type=int, default=256)
- parser.add_argument("--substitute_time", type=int, default=5)
- parser.add_argument("--dataset", type=str, default="multiarith")
- parser.add_argument("--direct_answer_trigger_for_fewshot", type=str, default="The answer is")
- args = parser.parse_args()
- return args
-
-if __name__ == "__main__":
- test_examples = [
- "Nancy uploaded 41 pictures to Facebook. She put 37 pics into one album and put the rest into 2 different albums. How many pictures were in each album?",
- ]
- decoder = Decoder()
- for test_example in test_examples:
- demo(decoder, test_example, 0)
\ No newline at end of file
diff --git a/spaces/Sonnt/Fracture_Webapp/mLogsFunctions/dataprocessing.py b/spaces/Sonnt/Fracture_Webapp/mLogsFunctions/dataprocessing.py
deleted file mode 100644
index 753e8e188c02fb732d1c539170e9203a42855154..0000000000000000000000000000000000000000
--- a/spaces/Sonnt/Fracture_Webapp/mLogsFunctions/dataprocessing.py
+++ /dev/null
@@ -1,14 +0,0 @@
-import pandas as pd
-import streamlit as st
-
-def columns_list(data:pd.DataFrame, no_depth:bool=None, no_well:bool=None):
- columns_list = list(data.columns.unique())
- columns_list.sort()
- if "DEPTH" in columns_list and no_depth == True:
- columns_list.remove("DEPTH")
- if "WELL" in columns_list and no_well == True:
- columns_list.remove("WELL")
- return columns_list
-
-def well_filter(df, well_name):
- return df[df.WELL == well_name]
\ No newline at end of file
diff --git a/spaces/SuYuanS/AudioCraft_Plus/audiocraft/modules/chroma.py b/spaces/SuYuanS/AudioCraft_Plus/audiocraft/modules/chroma.py
deleted file mode 100644
index e84fb66b4a4aaefb0b3ccac8a9a44c3b20e48f61..0000000000000000000000000000000000000000
--- a/spaces/SuYuanS/AudioCraft_Plus/audiocraft/modules/chroma.py
+++ /dev/null
@@ -1,66 +0,0 @@
-# Copyright (c) Meta Platforms, Inc. and affiliates.
-# All rights reserved.
-#
-# This source code is licensed under the license found in the
-# LICENSE file in the root directory of this source tree.
-import typing as tp
-
-from einops import rearrange
-from librosa import filters
-import torch
-from torch import nn
-import torch.nn.functional as F
-import torchaudio
-
-
-class ChromaExtractor(nn.Module):
- """Chroma extraction and quantization.
-
- Args:
- sample_rate (int): Sample rate for the chroma extraction.
- n_chroma (int): Number of chroma bins for the chroma extraction.
- radix2_exp (int): Size of stft window for the chroma extraction (power of 2, e.g. 12 -> 2^12).
- nfft (int, optional): Number of FFT.
- winlen (int, optional): Window length.
- winhop (int, optional): Window hop size.
- argmax (bool, optional): Whether to use argmax. Defaults to False.
- norm (float, optional): Norm for chroma normalization. Defaults to inf.
- """
- def __init__(self, sample_rate: int, n_chroma: int = 12, radix2_exp: int = 12, nfft: tp.Optional[int] = None,
- winlen: tp.Optional[int] = None, winhop: tp.Optional[int] = None, argmax: bool = False,
- norm: float = torch.inf):
- super().__init__()
- self.winlen = winlen or 2 ** radix2_exp
- self.nfft = nfft or self.winlen
- self.winhop = winhop or (self.winlen // 4)
- self.sample_rate = sample_rate
- self.n_chroma = n_chroma
- self.norm = norm
- self.argmax = argmax
- self.register_buffer('fbanks', torch.from_numpy(filters.chroma(sr=sample_rate, n_fft=self.nfft, tuning=0,
- n_chroma=self.n_chroma)), persistent=False)
- self.spec = torchaudio.transforms.Spectrogram(n_fft=self.nfft, win_length=self.winlen,
- hop_length=self.winhop, power=2, center=True,
- pad=0, normalized=True)
-
- def forward(self, wav: torch.Tensor) -> torch.Tensor:
- T = wav.shape[-1]
- # in case we are getting a wav that was dropped out (nullified)
- # from the conditioner, make sure wav length is no less that nfft
- if T < self.nfft:
- pad = self.nfft - T
- r = 0 if pad % 2 == 0 else 1
- wav = F.pad(wav, (pad // 2, pad // 2 + r), 'constant', 0)
- assert wav.shape[-1] == self.nfft, f"expected len {self.nfft} but got {wav.shape[-1]}"
-
- spec = self.spec(wav).squeeze(1)
- raw_chroma = torch.einsum('cf,...ft->...ct', self.fbanks, spec)
- norm_chroma = torch.nn.functional.normalize(raw_chroma, p=self.norm, dim=-2, eps=1e-6)
- norm_chroma = rearrange(norm_chroma, 'b d t -> b t d')
-
- if self.argmax:
- idx = norm_chroma.argmax(-1, keepdim=True)
- norm_chroma[:] = 0
- norm_chroma.scatter_(dim=-1, index=idx, value=1)
-
- return norm_chroma
diff --git a/spaces/SungBeom/chatwine-korean/.venv/Lib/site-packages/clickhouse_connect/driver/npconv.py b/spaces/SungBeom/chatwine-korean/.venv/Lib/site-packages/clickhouse_connect/driver/npconv.py
deleted file mode 100644
index df99550d348a89dd4086050358591ac94ad50467..0000000000000000000000000000000000000000
--- a/spaces/SungBeom/chatwine-korean/.venv/Lib/site-packages/clickhouse_connect/driver/npconv.py
+++ /dev/null
@@ -1,9 +0,0 @@
-from clickhouse_connect.driver.options import np
-
-from clickhouse_connect.driver.types import ByteSource
-
-
-def read_numpy_array(source: ByteSource, np_type: str, num_rows: int):
- dtype = np.dtype(np_type)
- buffer = source.read_bytes(dtype.itemsize * num_rows)
- return np.frombuffer(buffer, dtype, num_rows)
diff --git a/spaces/SungBeom/chatwine-korean/.venv/Lib/site-packages/debugpy/_vendored/pydevd/_pydev_bundle/pydev_monkey_qt.py b/spaces/SungBeom/chatwine-korean/.venv/Lib/site-packages/debugpy/_vendored/pydevd/_pydev_bundle/pydev_monkey_qt.py
deleted file mode 100644
index e348b842d4a02e91e772a08ea6703f3caf760280..0000000000000000000000000000000000000000
--- a/spaces/SungBeom/chatwine-korean/.venv/Lib/site-packages/debugpy/_vendored/pydevd/_pydev_bundle/pydev_monkey_qt.py
+++ /dev/null
@@ -1,216 +0,0 @@
-from __future__ import nested_scopes
-
-from _pydev_bundle._pydev_saved_modules import threading
-import os
-from _pydev_bundle import pydev_log
-
-
-def set_trace_in_qt():
- from _pydevd_bundle.pydevd_comm import get_global_debugger
- py_db = get_global_debugger()
- if py_db is not None:
- threading.current_thread() # Create the dummy thread for qt.
- py_db.enable_tracing()
-
-
-_patched_qt = False
-
-
-def patch_qt(qt_support_mode):
- '''
- This method patches qt (PySide2, PySide, PyQt4, PyQt5) so that we have hooks to set the tracing for QThread.
- '''
- if not qt_support_mode:
- return
-
- if qt_support_mode is True or qt_support_mode == 'True':
- # do not break backward compatibility
- qt_support_mode = 'auto'
-
- if qt_support_mode == 'auto':
- qt_support_mode = os.getenv('PYDEVD_PYQT_MODE', 'auto')
-
- # Avoid patching more than once
- global _patched_qt
- if _patched_qt:
- return
-
- pydev_log.debug('Qt support mode: %s', qt_support_mode)
-
- _patched_qt = True
-
- if qt_support_mode == 'auto':
-
- patch_qt_on_import = None
- try:
- import PySide2 # @UnresolvedImport @UnusedImport
- qt_support_mode = 'pyside2'
- except:
- try:
- import Pyside # @UnresolvedImport @UnusedImport
- qt_support_mode = 'pyside'
- except:
- try:
- import PyQt5 # @UnresolvedImport @UnusedImport
- qt_support_mode = 'pyqt5'
- except:
- try:
- import PyQt4 # @UnresolvedImport @UnusedImport
- qt_support_mode = 'pyqt4'
- except:
- return
-
- if qt_support_mode == 'pyside2':
- try:
- import PySide2.QtCore # @UnresolvedImport
- _internal_patch_qt(PySide2.QtCore, qt_support_mode)
- except:
- return
-
- elif qt_support_mode == 'pyside':
- try:
- import PySide.QtCore # @UnresolvedImport
- _internal_patch_qt(PySide.QtCore, qt_support_mode)
- except:
- return
-
- elif qt_support_mode == 'pyqt5':
- try:
- import PyQt5.QtCore # @UnresolvedImport
- _internal_patch_qt(PyQt5.QtCore)
- except:
- return
-
- elif qt_support_mode == 'pyqt4':
- # Ok, we have an issue here:
- # PyDev-452: Selecting PyQT API version using sip.setapi fails in debug mode
- # http://pyqt.sourceforge.net/Docs/PyQt4/incompatible_apis.html
- # Mostly, if the user uses a different API version (i.e.: v2 instead of v1),
- # that has to be done before importing PyQt4 modules (PySide/PyQt5 don't have this issue
- # as they only implements v2).
- patch_qt_on_import = 'PyQt4'
-
- def get_qt_core_module():
- import PyQt4.QtCore # @UnresolvedImport
- return PyQt4.QtCore
-
- _patch_import_to_patch_pyqt_on_import(patch_qt_on_import, get_qt_core_module)
-
- else:
- raise ValueError('Unexpected qt support mode: %s' % (qt_support_mode,))
-
-
-def _patch_import_to_patch_pyqt_on_import(patch_qt_on_import, get_qt_core_module):
- # I don't like this approach very much as we have to patch __import__, but I like even less
- # asking the user to configure something in the client side...
- # So, our approach is to patch PyQt4 right before the user tries to import it (at which
- # point he should've set the sip api version properly already anyways).
-
- pydev_log.debug('Setting up Qt post-import monkeypatch.')
-
- dotted = patch_qt_on_import + '.'
- original_import = __import__
-
- from _pydev_bundle._pydev_sys_patch import patch_sys_module, patch_reload, cancel_patches_in_sys_module
-
- patch_sys_module()
- patch_reload()
-
- def patched_import(name, *args, **kwargs):
- if patch_qt_on_import == name or name.startswith(dotted):
- builtins.__import__ = original_import
- cancel_patches_in_sys_module()
- _internal_patch_qt(get_qt_core_module()) # Patch it only when the user would import the qt module
- return original_import(name, *args, **kwargs)
-
- import builtins # Py3
-
- builtins.__import__ = patched_import
-
-
-def _internal_patch_qt(QtCore, qt_support_mode='auto'):
- pydev_log.debug('Patching Qt: %s', QtCore)
-
- _original_thread_init = QtCore.QThread.__init__
- _original_runnable_init = QtCore.QRunnable.__init__
- _original_QThread = QtCore.QThread
-
- class FuncWrapper:
-
- def __init__(self, original):
- self._original = original
-
- def __call__(self, *args, **kwargs):
- set_trace_in_qt()
- return self._original(*args, **kwargs)
-
- class StartedSignalWrapper(QtCore.QObject): # Wrapper for the QThread.started signal
-
- try:
- _signal = QtCore.Signal() # @UndefinedVariable
- except:
- _signal = QtCore.pyqtSignal() # @UndefinedVariable
-
- def __init__(self, thread, original_started):
- QtCore.QObject.__init__(self)
- self.thread = thread
- self.original_started = original_started
- if qt_support_mode in ('pyside', 'pyside2'):
- self._signal = original_started
- else:
- self._signal.connect(self._on_call)
- self.original_started.connect(self._signal)
-
- def connect(self, func, *args, **kwargs):
- if qt_support_mode in ('pyside', 'pyside2'):
- return self._signal.connect(FuncWrapper(func), *args, **kwargs)
- else:
- return self._signal.connect(func, *args, **kwargs)
-
- def disconnect(self, *args, **kwargs):
- return self._signal.disconnect(*args, **kwargs)
-
- def emit(self, *args, **kwargs):
- return self._signal.emit(*args, **kwargs)
-
- def _on_call(self, *args, **kwargs):
- set_trace_in_qt()
-
- class ThreadWrapper(QtCore.QThread): # Wrapper for QThread
-
- def __init__(self, *args, **kwargs):
- _original_thread_init(self, *args, **kwargs)
-
- # In PyQt5 the program hangs when we try to call original run method of QThread class.
- # So we need to distinguish instances of QThread class and instances of QThread inheritors.
- if self.__class__.run == _original_QThread.run:
- self.run = self._exec_run
- else:
- self._original_run = self.run
- self.run = self._new_run
- self._original_started = self.started
- self.started = StartedSignalWrapper(self, self.started)
-
- def _exec_run(self):
- set_trace_in_qt()
- self.exec_()
- return None
-
- def _new_run(self):
- set_trace_in_qt()
- return self._original_run()
-
- class RunnableWrapper(QtCore.QRunnable): # Wrapper for QRunnable
-
- def __init__(self, *args, **kwargs):
- _original_runnable_init(self, *args, **kwargs)
-
- self._original_run = self.run
- self.run = self._new_run
-
- def _new_run(self):
- set_trace_in_qt()
- return self._original_run()
-
- QtCore.QThread = ThreadWrapper
- QtCore.QRunnable = RunnableWrapper
diff --git a/spaces/Suniilkumaar/SwapMukham/face_parsing/swap.py b/spaces/Suniilkumaar/SwapMukham/face_parsing/swap.py
deleted file mode 100644
index 0ae65e486b4cc2fa729a0992f28d922f45ada840..0000000000000000000000000000000000000000
--- a/spaces/Suniilkumaar/SwapMukham/face_parsing/swap.py
+++ /dev/null
@@ -1,133 +0,0 @@
-import torch
-import torch.nn as nn
-import torch.nn.functional as F
-import torchvision.transforms as transforms
-import cv2
-import numpy as np
-
-from .model import BiSeNet
-
-mask_regions = {
- "Background":0,
- "Skin":1,
- "L-Eyebrow":2,
- "R-Eyebrow":3,
- "L-Eye":4,
- "R-Eye":5,
- "Eye-G":6,
- "L-Ear":7,
- "R-Ear":8,
- "Ear-R":9,
- "Nose":10,
- "Mouth":11,
- "U-Lip":12,
- "L-Lip":13,
- "Neck":14,
- "Neck-L":15,
- "Cloth":16,
- "Hair":17,
- "Hat":18
-}
-
-# Borrowed from simswap
-# https://github.com/neuralchen/SimSwap/blob/26c84d2901bd56eda4d5e3c5ca6da16e65dc82a6/util/reverse2original.py#L30
-class SoftErosion(nn.Module):
- def __init__(self, kernel_size=15, threshold=0.6, iterations=1):
- super(SoftErosion, self).__init__()
- r = kernel_size // 2
- self.padding = r
- self.iterations = iterations
- self.threshold = threshold
-
- # Create kernel
- y_indices, x_indices = torch.meshgrid(torch.arange(0., kernel_size), torch.arange(0., kernel_size))
- dist = torch.sqrt((x_indices - r) ** 2 + (y_indices - r) ** 2)
- kernel = dist.max() - dist
- kernel /= kernel.sum()
- kernel = kernel.view(1, 1, *kernel.shape)
- self.register_buffer('weight', kernel)
-
- def forward(self, x):
- x = x.float()
- for i in range(self.iterations - 1):
- x = torch.min(x, F.conv2d(x, weight=self.weight, groups=x.shape[1], padding=self.padding))
- x = F.conv2d(x, weight=self.weight, groups=x.shape[1], padding=self.padding)
-
- mask = x >= self.threshold
- x[mask] = 1.0
- x[~mask] /= x[~mask].max()
-
- return x, mask
-
-device = "cpu"
-
-def init_parser(pth_path, mode="cpu"):
- global device
- device = mode
- n_classes = 19
- net = BiSeNet(n_classes=n_classes)
- if device == "cuda":
- net.cuda()
- net.load_state_dict(torch.load(pth_path))
- else:
- net.load_state_dict(torch.load(pth_path, map_location=torch.device('cpu')))
- net.eval()
- return net
-
-
-def image_to_parsing(img, net):
- img = cv2.resize(img, (512, 512))
- img = img[:,:,::-1]
- transform = transforms.Compose([
- transforms.ToTensor(),
- transforms.Normalize((0.485, 0.456, 0.406), (0.229, 0.224, 0.225))
- ])
- img = transform(img.copy())
- img = torch.unsqueeze(img, 0)
-
- with torch.no_grad():
- img = img.to(device)
- out = net(img)[0]
- parsing = out.squeeze(0).cpu().numpy().argmax(0)
- return parsing
-
-
-def get_mask(parsing, classes):
- res = parsing == classes[0]
- for val in classes[1:]:
- res += parsing == val
- return res
-
-
-def swap_regions(source, target, net, smooth_mask, includes=[1,2,3,4,5,10,11,12,13], blur=10):
- parsing = image_to_parsing(source, net)
-
- if len(includes) == 0:
- return source, np.zeros_like(source)
-
- include_mask = get_mask(parsing, includes)
- mask = np.repeat(include_mask[:, :, np.newaxis], 3, axis=2).astype("float32")
-
- if smooth_mask is not None:
- mask_tensor = torch.from_numpy(mask.copy().transpose((2, 0, 1))).float().to(device)
- face_mask_tensor = mask_tensor[0] + mask_tensor[1]
- soft_face_mask_tensor, _ = smooth_mask(face_mask_tensor.unsqueeze_(0).unsqueeze_(0))
- soft_face_mask_tensor.squeeze_()
- mask = np.repeat(soft_face_mask_tensor.cpu().numpy()[:, :, np.newaxis], 3, axis=2)
-
- if blur > 0:
- mask = cv2.GaussianBlur(mask, (0, 0), blur)
-
- resized_source = cv2.resize((source).astype("float32"), (512, 512))
- resized_target = cv2.resize((target).astype("float32"), (512, 512))
- result = mask * resized_source + (1 - mask) * resized_target
- result = cv2.resize(result.astype("uint8"), (source.shape[1], source.shape[0]))
-
- return result
-
-def mask_regions_to_list(values):
- out_ids = []
- for value in values:
- if value in mask_regions.keys():
- out_ids.append(mask_regions.get(value))
- return out_ids
diff --git a/spaces/Superlang/ImageComposition/app.py b/spaces/Superlang/ImageComposition/app.py
deleted file mode 100644
index 9b2f81027e725370471e4a7f15588312cae742d1..0000000000000000000000000000000000000000
--- a/spaces/Superlang/ImageComposition/app.py
+++ /dev/null
@@ -1,57 +0,0 @@
-import importlib
-import os
-from PIL import Image
-import gradio as gr
-from omegaconf import OmegaConf
-
-config = OmegaConf.load("config/annotator.yaml")
-
-package_annotator = "processor"
-
-
-def process_image(cls: str, fg: Image.Image, bg: Image.Image, *kwargs):
- if fg.size != bg.size:
- fg = fg.resize(bg.size)
- module_imp = importlib.import_module(package_annotator)
- model = getattr(module_imp, cls)
- image_processor = model()
- result = image_processor(fg, bg, *kwargs)
- if type(result) == tuple:
- return result
- return [result]
-
-
-def process(cls):
- def process_fc(img, res, *args):
- return process_image(cls, img, res, *args)
-
- return process_fc
-
-
-block = gr.Blocks().queue()
-examples = [[os.path.join(os.path.dirname(__file__), "example/product.png"),
- os.path.join(os.path.dirname(__file__), "example/bg.png")]]
-with block:
- for key in config.keys():
- cls, input_element = config[key]["process"], config[key].get("input")
- input_append = []
- with gr.Tab(key):
- with gr.Row():
- gr.Markdown("## " + key)
- with gr.Row():
- with gr.Column():
- input_image = gr.Image(label="foreground", source='upload', type="pil", image_mode="RGBA")
- bg_image = gr.Image(label="background", source='upload', type="pil", image_mode="RGBA")
- if input_element is not None:
- for item in input_element:
- input_append.append(getattr(gr, item["attr"])(**item["args"]))
- run_button = gr.Button(label="Run")
- gr.Examples(examples, [input_image, bg_image])
- with gr.Column():
- gallery = gr.Gallery(label="Generated images", show_label=False).style(height="auto")
-
- run_button.click(fn=process(cls),
- inputs=[input_image, bg_image] + input_append,
- outputs=[gallery])
-
-block.launch()
diff --git a/spaces/Superlang/ImageProcessor/annotator/zoe/zoedepth/models/base_models/midas_repo/tf/run_onnx.py b/spaces/Superlang/ImageProcessor/annotator/zoe/zoedepth/models/base_models/midas_repo/tf/run_onnx.py
deleted file mode 100644
index 7107b99969a127f951814f743d5c562a436b2430..0000000000000000000000000000000000000000
--- a/spaces/Superlang/ImageProcessor/annotator/zoe/zoedepth/models/base_models/midas_repo/tf/run_onnx.py
+++ /dev/null
@@ -1,119 +0,0 @@
-"""Compute depth maps for images in the input folder.
-"""
-import os
-import glob
-import utils
-import cv2
-import sys
-import numpy as np
-import argparse
-
-import onnx
-import onnxruntime as rt
-
-from transforms import Resize, NormalizeImage, PrepareForNet
-
-
-def run(input_path, output_path, model_path, model_type="large"):
- """Run MonoDepthNN to compute depth maps.
-
- Args:
- input_path (str): path to input folder
- output_path (str): path to output folder
- model_path (str): path to saved model
- """
- print("initialize")
-
- # select device
- device = "CUDA:0"
- #device = "CPU"
- print("device: %s" % device)
-
- # network resolution
- if model_type == "large":
- net_w, net_h = 384, 384
- elif model_type == "small":
- net_w, net_h = 256, 256
- else:
- print(f"model_type '{model_type}' not implemented, use: --model_type large")
- assert False
-
- # load network
- print("loading model...")
- model = rt.InferenceSession(model_path)
- input_name = model.get_inputs()[0].name
- output_name = model.get_outputs()[0].name
-
- resize_image = Resize(
- net_w,
- net_h,
- resize_target=None,
- keep_aspect_ratio=False,
- ensure_multiple_of=32,
- resize_method="upper_bound",
- image_interpolation_method=cv2.INTER_CUBIC,
- )
-
- def compose2(f1, f2):
- return lambda x: f2(f1(x))
-
- transform = compose2(resize_image, PrepareForNet())
-
- # get input
- img_names = glob.glob(os.path.join(input_path, "*"))
- num_images = len(img_names)
-
- # create output folder
- os.makedirs(output_path, exist_ok=True)
-
- print("start processing")
-
- for ind, img_name in enumerate(img_names):
-
- print(" processing {} ({}/{})".format(img_name, ind + 1, num_images))
-
- # input
- img = utils.read_image(img_name)
- img_input = transform({"image": img})["image"]
-
- # compute
- output = model.run([output_name], {input_name: img_input.reshape(1, 3, net_h, net_w).astype(np.float32)})[0]
- prediction = np.array(output).reshape(net_h, net_w)
- prediction = cv2.resize(prediction, (img.shape[1], img.shape[0]), interpolation=cv2.INTER_CUBIC)
-
- # output
- filename = os.path.join(
- output_path, os.path.splitext(os.path.basename(img_name))[0]
- )
- utils.write_depth(filename, prediction, bits=2)
-
- print("finished")
-
-
-if __name__ == "__main__":
- parser = argparse.ArgumentParser()
-
- parser.add_argument('-i', '--input_path',
- default='input',
- help='folder with input images'
- )
-
- parser.add_argument('-o', '--output_path',
- default='output',
- help='folder for output images'
- )
-
- parser.add_argument('-m', '--model_weights',
- default='model-f6b98070.onnx',
- help='path to the trained weights of model'
- )
-
- parser.add_argument('-t', '--model_type',
- default='large',
- help='model type: large or small'
- )
-
- args = parser.parse_args()
-
- # compute depth maps
- run(args.input_path, args.output_path, args.model_weights, args.model_type)
diff --git a/spaces/TRaw/dtet/README.md b/spaces/TRaw/dtet/README.md
deleted file mode 100644
index 8936dea47ba363c737d8348383e5809f781bc5fd..0000000000000000000000000000000000000000
--- a/spaces/TRaw/dtet/README.md
+++ /dev/null
@@ -1,13 +0,0 @@
----
-title: Chat Ui Template
-emoji: 🚀
-colorFrom: indigo
-colorTo: blue
-sdk: docker
-pinned: false
-app_port: 3000
-suggested_hardware: a10g-small
-duplicated_from: huggingchat/chat-ui-template
----
-
-Check out the configuration reference at https://huggingface.co/docs/hub/spaces-config-reference
diff --git a/spaces/TencentARC/VLog/models/grit_src/third_party/CenterNet2/detectron2/modeling/roi_heads/roi_heads.py b/spaces/TencentARC/VLog/models/grit_src/third_party/CenterNet2/detectron2/modeling/roi_heads/roi_heads.py
deleted file mode 100644
index 13dd57a0478917001841f6c6299f380e1198e63a..0000000000000000000000000000000000000000
--- a/spaces/TencentARC/VLog/models/grit_src/third_party/CenterNet2/detectron2/modeling/roi_heads/roi_heads.py
+++ /dev/null
@@ -1,877 +0,0 @@
-# Copyright (c) Facebook, Inc. and its affiliates.
-import inspect
-import logging
-import numpy as np
-from typing import Dict, List, Optional, Tuple
-import torch
-from torch import nn
-
-from detectron2.config import configurable
-from detectron2.layers import ShapeSpec, nonzero_tuple
-from detectron2.structures import Boxes, ImageList, Instances, pairwise_iou
-from detectron2.utils.events import get_event_storage
-from detectron2.utils.registry import Registry
-
-from ..backbone.resnet import BottleneckBlock, ResNet
-from ..matcher import Matcher
-from ..poolers import ROIPooler
-from ..proposal_generator.proposal_utils import add_ground_truth_to_proposals
-from ..sampling import subsample_labels
-from .box_head import build_box_head
-from .fast_rcnn import FastRCNNOutputLayers
-from .keypoint_head import build_keypoint_head
-from .mask_head import build_mask_head
-
-ROI_HEADS_REGISTRY = Registry("ROI_HEADS")
-ROI_HEADS_REGISTRY.__doc__ = """
-Registry for ROI heads in a generalized R-CNN model.
-ROIHeads take feature maps and region proposals, and
-perform per-region computation.
-
-The registered object will be called with `obj(cfg, input_shape)`.
-The call is expected to return an :class:`ROIHeads`.
-"""
-
-logger = logging.getLogger(__name__)
-
-
-def build_roi_heads(cfg, input_shape):
- """
- Build ROIHeads defined by `cfg.MODEL.ROI_HEADS.NAME`.
- """
- name = cfg.MODEL.ROI_HEADS.NAME
- return ROI_HEADS_REGISTRY.get(name)(cfg, input_shape)
-
-
-def select_foreground_proposals(
- proposals: List[Instances], bg_label: int
-) -> Tuple[List[Instances], List[torch.Tensor]]:
- """
- Given a list of N Instances (for N images), each containing a `gt_classes` field,
- return a list of Instances that contain only instances with `gt_classes != -1 &&
- gt_classes != bg_label`.
-
- Args:
- proposals (list[Instances]): A list of N Instances, where N is the number of
- images in the batch.
- bg_label: label index of background class.
-
- Returns:
- list[Instances]: N Instances, each contains only the selected foreground instances.
- list[Tensor]: N boolean vector, correspond to the selection mask of
- each Instances object. True for selected instances.
- """
- assert isinstance(proposals, (list, tuple))
- assert isinstance(proposals[0], Instances)
- assert proposals[0].has("gt_classes")
- fg_proposals = []
- fg_selection_masks = []
- for proposals_per_image in proposals:
- gt_classes = proposals_per_image.gt_classes
- fg_selection_mask = (gt_classes != -1) & (gt_classes != bg_label)
- fg_idxs = fg_selection_mask.nonzero().squeeze(1)
- fg_proposals.append(proposals_per_image[fg_idxs])
- fg_selection_masks.append(fg_selection_mask)
- return fg_proposals, fg_selection_masks
-
-
-def select_proposals_with_visible_keypoints(proposals: List[Instances]) -> List[Instances]:
- """
- Args:
- proposals (list[Instances]): a list of N Instances, where N is the
- number of images.
-
- Returns:
- proposals: only contains proposals with at least one visible keypoint.
-
- Note that this is still slightly different from Detectron.
- In Detectron, proposals for training keypoint head are re-sampled from
- all the proposals with IOU>threshold & >=1 visible keypoint.
-
- Here, the proposals are first sampled from all proposals with
- IOU>threshold, then proposals with no visible keypoint are filtered out.
- This strategy seems to make no difference on Detectron and is easier to implement.
- """
- ret = []
- all_num_fg = []
- for proposals_per_image in proposals:
- # If empty/unannotated image (hard negatives), skip filtering for train
- if len(proposals_per_image) == 0:
- ret.append(proposals_per_image)
- continue
- gt_keypoints = proposals_per_image.gt_keypoints.tensor
- # #fg x K x 3
- vis_mask = gt_keypoints[:, :, 2] >= 1
- xs, ys = gt_keypoints[:, :, 0], gt_keypoints[:, :, 1]
- proposal_boxes = proposals_per_image.proposal_boxes.tensor.unsqueeze(dim=1) # #fg x 1 x 4
- kp_in_box = (
- (xs >= proposal_boxes[:, :, 0])
- & (xs <= proposal_boxes[:, :, 2])
- & (ys >= proposal_boxes[:, :, 1])
- & (ys <= proposal_boxes[:, :, 3])
- )
- selection = (kp_in_box & vis_mask).any(dim=1)
- selection_idxs = nonzero_tuple(selection)[0]
- all_num_fg.append(selection_idxs.numel())
- ret.append(proposals_per_image[selection_idxs])
-
- storage = get_event_storage()
- storage.put_scalar("keypoint_head/num_fg_samples", np.mean(all_num_fg))
- return ret
-
-
-class ROIHeads(torch.nn.Module):
- """
- ROIHeads perform all per-region computation in an R-CNN.
-
- It typically contains logic to
-
- 1. (in training only) match proposals with ground truth and sample them
- 2. crop the regions and extract per-region features using proposals
- 3. make per-region predictions with different heads
-
- It can have many variants, implemented as subclasses of this class.
- This base class contains the logic to match/sample proposals.
- But it is not necessary to inherit this class if the sampling logic is not needed.
- """
-
- @configurable
- def __init__(
- self,
- *,
- num_classes,
- batch_size_per_image,
- positive_fraction,
- proposal_matcher,
- proposal_append_gt=True,
- ):
- """
- NOTE: this interface is experimental.
-
- Args:
- num_classes (int): number of foreground classes (i.e. background is not included)
- batch_size_per_image (int): number of proposals to sample for training
- positive_fraction (float): fraction of positive (foreground) proposals
- to sample for training.
- proposal_matcher (Matcher): matcher that matches proposals and ground truth
- proposal_append_gt (bool): whether to include ground truth as proposals as well
- """
- super().__init__()
- self.batch_size_per_image = batch_size_per_image
- self.positive_fraction = positive_fraction
- self.num_classes = num_classes
- self.proposal_matcher = proposal_matcher
- self.proposal_append_gt = proposal_append_gt
-
- @classmethod
- def from_config(cls, cfg):
- return {
- "batch_size_per_image": cfg.MODEL.ROI_HEADS.BATCH_SIZE_PER_IMAGE,
- "positive_fraction": cfg.MODEL.ROI_HEADS.POSITIVE_FRACTION,
- "num_classes": cfg.MODEL.ROI_HEADS.NUM_CLASSES,
- "proposal_append_gt": cfg.MODEL.ROI_HEADS.PROPOSAL_APPEND_GT,
- # Matcher to assign box proposals to gt boxes
- "proposal_matcher": Matcher(
- cfg.MODEL.ROI_HEADS.IOU_THRESHOLDS,
- cfg.MODEL.ROI_HEADS.IOU_LABELS,
- allow_low_quality_matches=False,
- ),
- }
-
- def _sample_proposals(
- self, matched_idxs: torch.Tensor, matched_labels: torch.Tensor, gt_classes: torch.Tensor
- ) -> Tuple[torch.Tensor, torch.Tensor]:
- """
- Based on the matching between N proposals and M groundtruth,
- sample the proposals and set their classification labels.
-
- Args:
- matched_idxs (Tensor): a vector of length N, each is the best-matched
- gt index in [0, M) for each proposal.
- matched_labels (Tensor): a vector of length N, the matcher's label
- (one of cfg.MODEL.ROI_HEADS.IOU_LABELS) for each proposal.
- gt_classes (Tensor): a vector of length M.
-
- Returns:
- Tensor: a vector of indices of sampled proposals. Each is in [0, N).
- Tensor: a vector of the same length, the classification label for
- each sampled proposal. Each sample is labeled as either a category in
- [0, num_classes) or the background (num_classes).
- """
- has_gt = gt_classes.numel() > 0
- # Get the corresponding GT for each proposal
- if has_gt:
- gt_classes = gt_classes[matched_idxs]
- # Label unmatched proposals (0 label from matcher) as background (label=num_classes)
- gt_classes[matched_labels == 0] = self.num_classes
- # Label ignore proposals (-1 label)
- gt_classes[matched_labels == -1] = -1
- else:
- gt_classes = torch.zeros_like(matched_idxs) + self.num_classes
-
- sampled_fg_idxs, sampled_bg_idxs = subsample_labels(
- gt_classes, self.batch_size_per_image, self.positive_fraction, self.num_classes
- )
-
- sampled_idxs = torch.cat([sampled_fg_idxs, sampled_bg_idxs], dim=0)
- return sampled_idxs, gt_classes[sampled_idxs]
-
- @torch.no_grad()
- def label_and_sample_proposals(
- self, proposals: List[Instances], targets: List[Instances]
- ) -> List[Instances]:
- """
- Prepare some proposals to be used to train the ROI heads.
- It performs box matching between `proposals` and `targets`, and assigns
- training labels to the proposals.
- It returns ``self.batch_size_per_image`` random samples from proposals and groundtruth
- boxes, with a fraction of positives that is no larger than
- ``self.positive_fraction``.
-
- Args:
- See :meth:`ROIHeads.forward`
-
- Returns:
- list[Instances]:
- length `N` list of `Instances`s containing the proposals
- sampled for training. Each `Instances` has the following fields:
-
- - proposal_boxes: the proposal boxes
- - gt_boxes: the ground-truth box that the proposal is assigned to
- (this is only meaningful if the proposal has a label > 0; if label = 0
- then the ground-truth box is random)
-
- Other fields such as "gt_classes", "gt_masks", that's included in `targets`.
- """
- # Augment proposals with ground-truth boxes.
- # In the case of learned proposals (e.g., RPN), when training starts
- # the proposals will be low quality due to random initialization.
- # It's possible that none of these initial
- # proposals have high enough overlap with the gt objects to be used
- # as positive examples for the second stage components (box head,
- # cls head, mask head). Adding the gt boxes to the set of proposals
- # ensures that the second stage components will have some positive
- # examples from the start of training. For RPN, this augmentation improves
- # convergence and empirically improves box AP on COCO by about 0.5
- # points (under one tested configuration).
- if self.proposal_append_gt:
- proposals = add_ground_truth_to_proposals(targets, proposals)
-
- proposals_with_gt = []
-
- num_fg_samples = []
- num_bg_samples = []
- for proposals_per_image, targets_per_image in zip(proposals, targets):
- has_gt = len(targets_per_image) > 0
- match_quality_matrix = pairwise_iou(
- targets_per_image.gt_boxes, proposals_per_image.proposal_boxes
- )
- matched_idxs, matched_labels = self.proposal_matcher(match_quality_matrix)
- sampled_idxs, gt_classes = self._sample_proposals(
- matched_idxs, matched_labels, targets_per_image.gt_classes
- )
-
- # Set target attributes of the sampled proposals:
- proposals_per_image = proposals_per_image[sampled_idxs]
- proposals_per_image.gt_classes = gt_classes
-
- if has_gt:
- sampled_targets = matched_idxs[sampled_idxs]
- # We index all the attributes of targets that start with "gt_"
- # and have not been added to proposals yet (="gt_classes").
- # NOTE: here the indexing waste some compute, because heads
- # like masks, keypoints, etc, will filter the proposals again,
- # (by foreground/background, or number of keypoints in the image, etc)
- # so we essentially index the data twice.
- for (trg_name, trg_value) in targets_per_image.get_fields().items():
- if trg_name.startswith("gt_") and not proposals_per_image.has(trg_name):
- proposals_per_image.set(trg_name, trg_value[sampled_targets])
- # If no GT is given in the image, we don't know what a dummy gt value can be.
- # Therefore the returned proposals won't have any gt_* fields, except for a
- # gt_classes full of background label.
-
- num_bg_samples.append((gt_classes == self.num_classes).sum().item())
- num_fg_samples.append(gt_classes.numel() - num_bg_samples[-1])
- proposals_with_gt.append(proposals_per_image)
-
- # Log the number of fg/bg samples that are selected for training ROI heads
- storage = get_event_storage()
- storage.put_scalar("roi_head/num_fg_samples", np.mean(num_fg_samples))
- storage.put_scalar("roi_head/num_bg_samples", np.mean(num_bg_samples))
-
- return proposals_with_gt
-
- def forward(
- self,
- images: ImageList,
- features: Dict[str, torch.Tensor],
- proposals: List[Instances],
- targets: Optional[List[Instances]] = None,
- ) -> Tuple[List[Instances], Dict[str, torch.Tensor]]:
- """
- Args:
- images (ImageList):
- features (dict[str,Tensor]): input data as a mapping from feature
- map name to tensor. Axis 0 represents the number of images `N` in
- the input data; axes 1-3 are channels, height, and width, which may
- vary between feature maps (e.g., if a feature pyramid is used).
- proposals (list[Instances]): length `N` list of `Instances`. The i-th
- `Instances` contains object proposals for the i-th input image,
- with fields "proposal_boxes" and "objectness_logits".
- targets (list[Instances], optional): length `N` list of `Instances`. The i-th
- `Instances` contains the ground-truth per-instance annotations
- for the i-th input image. Specify `targets` during training only.
- It may have the following fields:
-
- - gt_boxes: the bounding box of each instance.
- - gt_classes: the label for each instance with a category ranging in [0, #class].
- - gt_masks: PolygonMasks or BitMasks, the ground-truth masks of each instance.
- - gt_keypoints: NxKx3, the groud-truth keypoints for each instance.
-
- Returns:
- list[Instances]: length `N` list of `Instances` containing the
- detected instances. Returned during inference only; may be [] during training.
-
- dict[str->Tensor]:
- mapping from a named loss to a tensor storing the loss. Used during training only.
- """
- raise NotImplementedError()
-
-
-@ROI_HEADS_REGISTRY.register()
-class Res5ROIHeads(ROIHeads):
- """
- The ROIHeads in a typical "C4" R-CNN model, where
- the box and mask head share the cropping and
- the per-region feature computation by a Res5 block.
- See :paper:`ResNet` Appendix A.
- """
-
- @configurable
- def __init__(
- self,
- *,
- in_features: List[str],
- pooler: ROIPooler,
- res5: nn.Module,
- box_predictor: nn.Module,
- mask_head: Optional[nn.Module] = None,
- **kwargs,
- ):
- """
- NOTE: this interface is experimental.
-
- Args:
- in_features (list[str]): list of backbone feature map names to use for
- feature extraction
- pooler (ROIPooler): pooler to extra region features from backbone
- res5 (nn.Sequential): a CNN to compute per-region features, to be used by
- ``box_predictor`` and ``mask_head``. Typically this is a "res5"
- block from a ResNet.
- box_predictor (nn.Module): make box predictions from the feature.
- Should have the same interface as :class:`FastRCNNOutputLayers`.
- mask_head (nn.Module): transform features to make mask predictions
- """
- super().__init__(**kwargs)
- self.in_features = in_features
- self.pooler = pooler
- if isinstance(res5, (list, tuple)):
- res5 = nn.Sequential(*res5)
- self.res5 = res5
- self.box_predictor = box_predictor
- self.mask_on = mask_head is not None
- if self.mask_on:
- self.mask_head = mask_head
-
- @classmethod
- def from_config(cls, cfg, input_shape):
- # fmt: off
- ret = super().from_config(cfg)
- in_features = ret["in_features"] = cfg.MODEL.ROI_HEADS.IN_FEATURES
- pooler_resolution = cfg.MODEL.ROI_BOX_HEAD.POOLER_RESOLUTION
- pooler_type = cfg.MODEL.ROI_BOX_HEAD.POOLER_TYPE
- pooler_scales = (1.0 / input_shape[in_features[0]].stride, )
- sampling_ratio = cfg.MODEL.ROI_BOX_HEAD.POOLER_SAMPLING_RATIO
- mask_on = cfg.MODEL.MASK_ON
- # fmt: on
- assert not cfg.MODEL.KEYPOINT_ON
- assert len(in_features) == 1
-
- ret["pooler"] = ROIPooler(
- output_size=pooler_resolution,
- scales=pooler_scales,
- sampling_ratio=sampling_ratio,
- pooler_type=pooler_type,
- )
-
- # Compatbility with old moco code. Might be useful.
- # See notes in StandardROIHeads.from_config
- if not inspect.ismethod(cls._build_res5_block):
- logger.warning(
- "The behavior of _build_res5_block may change. "
- "Please do not depend on private methods."
- )
- cls._build_res5_block = classmethod(cls._build_res5_block)
-
- ret["res5"], out_channels = cls._build_res5_block(cfg)
- ret["box_predictor"] = FastRCNNOutputLayers(
- cfg, ShapeSpec(channels=out_channels, height=1, width=1)
- )
-
- if mask_on:
- ret["mask_head"] = build_mask_head(
- cfg,
- ShapeSpec(channels=out_channels, width=pooler_resolution, height=pooler_resolution),
- )
- return ret
-
- @classmethod
- def _build_res5_block(cls, cfg):
- # fmt: off
- stage_channel_factor = 2 ** 3 # res5 is 8x res2
- num_groups = cfg.MODEL.RESNETS.NUM_GROUPS
- width_per_group = cfg.MODEL.RESNETS.WIDTH_PER_GROUP
- bottleneck_channels = num_groups * width_per_group * stage_channel_factor
- out_channels = cfg.MODEL.RESNETS.RES2_OUT_CHANNELS * stage_channel_factor
- stride_in_1x1 = cfg.MODEL.RESNETS.STRIDE_IN_1X1
- norm = cfg.MODEL.RESNETS.NORM
- assert not cfg.MODEL.RESNETS.DEFORM_ON_PER_STAGE[-1], \
- "Deformable conv is not yet supported in res5 head."
- # fmt: on
-
- blocks = ResNet.make_stage(
- BottleneckBlock,
- 3,
- stride_per_block=[2, 1, 1],
- in_channels=out_channels // 2,
- bottleneck_channels=bottleneck_channels,
- out_channels=out_channels,
- num_groups=num_groups,
- norm=norm,
- stride_in_1x1=stride_in_1x1,
- )
- return nn.Sequential(*blocks), out_channels
-
- def _shared_roi_transform(self, features: List[torch.Tensor], boxes: List[Boxes]):
- x = self.pooler(features, boxes)
- return self.res5(x)
-
- def forward(
- self,
- images: ImageList,
- features: Dict[str, torch.Tensor],
- proposals: List[Instances],
- targets: Optional[List[Instances]] = None,
- ):
- """
- See :meth:`ROIHeads.forward`.
- """
- del images
-
- if self.training:
- assert targets
- proposals = self.label_and_sample_proposals(proposals, targets)
- del targets
-
- proposal_boxes = [x.proposal_boxes for x in proposals]
- box_features = self._shared_roi_transform(
- [features[f] for f in self.in_features], proposal_boxes
- )
- predictions = self.box_predictor(box_features.mean(dim=[2, 3]))
-
- if self.training:
- del features
- losses = self.box_predictor.losses(predictions, proposals)
- if self.mask_on:
- proposals, fg_selection_masks = select_foreground_proposals(
- proposals, self.num_classes
- )
- # Since the ROI feature transform is shared between boxes and masks,
- # we don't need to recompute features. The mask loss is only defined
- # on foreground proposals, so we need to select out the foreground
- # features.
- mask_features = box_features[torch.cat(fg_selection_masks, dim=0)]
- del box_features
- losses.update(self.mask_head(mask_features, proposals))
- return [], losses
- else:
- pred_instances, _ = self.box_predictor.inference(predictions, proposals)
- pred_instances = self.forward_with_given_boxes(features, pred_instances)
- return pred_instances, {}
-
- def forward_with_given_boxes(
- self, features: Dict[str, torch.Tensor], instances: List[Instances]
- ) -> List[Instances]:
- """
- Use the given boxes in `instances` to produce other (non-box) per-ROI outputs.
-
- Args:
- features: same as in `forward()`
- instances (list[Instances]): instances to predict other outputs. Expect the keys
- "pred_boxes" and "pred_classes" to exist.
-
- Returns:
- instances (Instances):
- the same `Instances` object, with extra
- fields such as `pred_masks` or `pred_keypoints`.
- """
- assert not self.training
- assert instances[0].has("pred_boxes") and instances[0].has("pred_classes")
-
- if self.mask_on:
- feature_list = [features[f] for f in self.in_features]
- x = self._shared_roi_transform(feature_list, [x.pred_boxes for x in instances])
- return self.mask_head(x, instances)
- else:
- return instances
-
-
-@ROI_HEADS_REGISTRY.register()
-class StandardROIHeads(ROIHeads):
- """
- It's "standard" in a sense that there is no ROI transform sharing
- or feature sharing between tasks.
- Each head independently processes the input features by each head's
- own pooler and head.
-
- This class is used by most models, such as FPN and C5.
- To implement more models, you can subclass it and implement a different
- :meth:`forward()` or a head.
- """
-
- @configurable
- def __init__(
- self,
- *,
- box_in_features: List[str],
- box_pooler: ROIPooler,
- box_head: nn.Module,
- box_predictor: nn.Module,
- mask_in_features: Optional[List[str]] = None,
- mask_pooler: Optional[ROIPooler] = None,
- mask_head: Optional[nn.Module] = None,
- keypoint_in_features: Optional[List[str]] = None,
- keypoint_pooler: Optional[ROIPooler] = None,
- keypoint_head: Optional[nn.Module] = None,
- train_on_pred_boxes: bool = False,
- **kwargs,
- ):
- """
- NOTE: this interface is experimental.
-
- Args:
- box_in_features (list[str]): list of feature names to use for the box head.
- box_pooler (ROIPooler): pooler to extra region features for box head
- box_head (nn.Module): transform features to make box predictions
- box_predictor (nn.Module): make box predictions from the feature.
- Should have the same interface as :class:`FastRCNNOutputLayers`.
- mask_in_features (list[str]): list of feature names to use for the mask
- pooler or mask head. None if not using mask head.
- mask_pooler (ROIPooler): pooler to extract region features from image features.
- The mask head will then take region features to make predictions.
- If None, the mask head will directly take the dict of image features
- defined by `mask_in_features`
- mask_head (nn.Module): transform features to make mask predictions
- keypoint_in_features, keypoint_pooler, keypoint_head: similar to ``mask_*``.
- train_on_pred_boxes (bool): whether to use proposal boxes or
- predicted boxes from the box head to train other heads.
- """
- super().__init__(**kwargs)
- # keep self.in_features for backward compatibility
- self.in_features = self.box_in_features = box_in_features
- self.box_pooler = box_pooler
- self.box_head = box_head
- self.box_predictor = box_predictor
-
- self.mask_on = mask_in_features is not None
- if self.mask_on:
- self.mask_in_features = mask_in_features
- self.mask_pooler = mask_pooler
- self.mask_head = mask_head
-
- self.keypoint_on = keypoint_in_features is not None
- if self.keypoint_on:
- self.keypoint_in_features = keypoint_in_features
- self.keypoint_pooler = keypoint_pooler
- self.keypoint_head = keypoint_head
-
- self.train_on_pred_boxes = train_on_pred_boxes
-
- @classmethod
- def from_config(cls, cfg, input_shape):
- ret = super().from_config(cfg)
- ret["train_on_pred_boxes"] = cfg.MODEL.ROI_BOX_HEAD.TRAIN_ON_PRED_BOXES
- # Subclasses that have not been updated to use from_config style construction
- # may have overridden _init_*_head methods. In this case, those overridden methods
- # will not be classmethods and we need to avoid trying to call them here.
- # We test for this with ismethod which only returns True for bound methods of cls.
- # Such subclasses will need to handle calling their overridden _init_*_head methods.
- if inspect.ismethod(cls._init_box_head):
- ret.update(cls._init_box_head(cfg, input_shape))
- if inspect.ismethod(cls._init_mask_head):
- ret.update(cls._init_mask_head(cfg, input_shape))
- if inspect.ismethod(cls._init_keypoint_head):
- ret.update(cls._init_keypoint_head(cfg, input_shape))
- return ret
-
- @classmethod
- def _init_box_head(cls, cfg, input_shape):
- # fmt: off
- in_features = cfg.MODEL.ROI_HEADS.IN_FEATURES
- pooler_resolution = cfg.MODEL.ROI_BOX_HEAD.POOLER_RESOLUTION
- pooler_scales = tuple(1.0 / input_shape[k].stride for k in in_features)
- sampling_ratio = cfg.MODEL.ROI_BOX_HEAD.POOLER_SAMPLING_RATIO
- pooler_type = cfg.MODEL.ROI_BOX_HEAD.POOLER_TYPE
- # fmt: on
-
- # If StandardROIHeads is applied on multiple feature maps (as in FPN),
- # then we share the same predictors and therefore the channel counts must be the same
- in_channels = [input_shape[f].channels for f in in_features]
- # Check all channel counts are equal
- assert len(set(in_channels)) == 1, in_channels
- in_channels = in_channels[0]
-
- box_pooler = ROIPooler(
- output_size=pooler_resolution,
- scales=pooler_scales,
- sampling_ratio=sampling_ratio,
- pooler_type=pooler_type,
- )
- # Here we split "box head" and "box predictor", which is mainly due to historical reasons.
- # They are used together so the "box predictor" layers should be part of the "box head".
- # New subclasses of ROIHeads do not need "box predictor"s.
- box_head = build_box_head(
- cfg, ShapeSpec(channels=in_channels, height=pooler_resolution, width=pooler_resolution)
- )
- box_predictor = FastRCNNOutputLayers(cfg, box_head.output_shape)
- return {
- "box_in_features": in_features,
- "box_pooler": box_pooler,
- "box_head": box_head,
- "box_predictor": box_predictor,
- }
-
- @classmethod
- def _init_mask_head(cls, cfg, input_shape):
- if not cfg.MODEL.MASK_ON:
- return {}
- # fmt: off
- in_features = cfg.MODEL.ROI_HEADS.IN_FEATURES
- pooler_resolution = cfg.MODEL.ROI_MASK_HEAD.POOLER_RESOLUTION
- pooler_scales = tuple(1.0 / input_shape[k].stride for k in in_features)
- sampling_ratio = cfg.MODEL.ROI_MASK_HEAD.POOLER_SAMPLING_RATIO
- pooler_type = cfg.MODEL.ROI_MASK_HEAD.POOLER_TYPE
- # fmt: on
-
- in_channels = [input_shape[f].channels for f in in_features][0]
-
- ret = {"mask_in_features": in_features}
- ret["mask_pooler"] = (
- ROIPooler(
- output_size=pooler_resolution,
- scales=pooler_scales,
- sampling_ratio=sampling_ratio,
- pooler_type=pooler_type,
- )
- if pooler_type
- else None
- )
- if pooler_type:
- shape = ShapeSpec(
- channels=in_channels, width=pooler_resolution, height=pooler_resolution
- )
- else:
- shape = {f: input_shape[f] for f in in_features}
- ret["mask_head"] = build_mask_head(cfg, shape)
- return ret
-
- @classmethod
- def _init_keypoint_head(cls, cfg, input_shape):
- if not cfg.MODEL.KEYPOINT_ON:
- return {}
- # fmt: off
- in_features = cfg.MODEL.ROI_HEADS.IN_FEATURES
- pooler_resolution = cfg.MODEL.ROI_KEYPOINT_HEAD.POOLER_RESOLUTION
- pooler_scales = tuple(1.0 / input_shape[k].stride for k in in_features) # noqa
- sampling_ratio = cfg.MODEL.ROI_KEYPOINT_HEAD.POOLER_SAMPLING_RATIO
- pooler_type = cfg.MODEL.ROI_KEYPOINT_HEAD.POOLER_TYPE
- # fmt: on
-
- in_channels = [input_shape[f].channels for f in in_features][0]
-
- ret = {"keypoint_in_features": in_features}
- ret["keypoint_pooler"] = (
- ROIPooler(
- output_size=pooler_resolution,
- scales=pooler_scales,
- sampling_ratio=sampling_ratio,
- pooler_type=pooler_type,
- )
- if pooler_type
- else None
- )
- if pooler_type:
- shape = ShapeSpec(
- channels=in_channels, width=pooler_resolution, height=pooler_resolution
- )
- else:
- shape = {f: input_shape[f] for f in in_features}
- ret["keypoint_head"] = build_keypoint_head(cfg, shape)
- return ret
-
- def forward(
- self,
- images: ImageList,
- features: Dict[str, torch.Tensor],
- proposals: List[Instances],
- targets: Optional[List[Instances]] = None,
- ) -> Tuple[List[Instances], Dict[str, torch.Tensor]]:
- """
- See :class:`ROIHeads.forward`.
- """
- del images
- if self.training:
- assert targets, "'targets' argument is required during training"
- proposals = self.label_and_sample_proposals(proposals, targets)
- del targets
-
- if self.training:
- losses = self._forward_box(features, proposals)
- # Usually the original proposals used by the box head are used by the mask, keypoint
- # heads. But when `self.train_on_pred_boxes is True`, proposals will contain boxes
- # predicted by the box head.
- losses.update(self._forward_mask(features, proposals))
- losses.update(self._forward_keypoint(features, proposals))
- return proposals, losses
- else:
- pred_instances = self._forward_box(features, proposals)
- # During inference cascaded prediction is used: the mask and keypoints heads are only
- # applied to the top scoring box detections.
- pred_instances = self.forward_with_given_boxes(features, pred_instances)
- return pred_instances, {}
-
- def forward_with_given_boxes(
- self, features: Dict[str, torch.Tensor], instances: List[Instances]
- ) -> List[Instances]:
- """
- Use the given boxes in `instances` to produce other (non-box) per-ROI outputs.
-
- This is useful for downstream tasks where a box is known, but need to obtain
- other attributes (outputs of other heads).
- Test-time augmentation also uses this.
-
- Args:
- features: same as in `forward()`
- instances (list[Instances]): instances to predict other outputs. Expect the keys
- "pred_boxes" and "pred_classes" to exist.
-
- Returns:
- list[Instances]:
- the same `Instances` objects, with extra
- fields such as `pred_masks` or `pred_keypoints`.
- """
- assert not self.training
- assert instances[0].has("pred_boxes") and instances[0].has("pred_classes")
-
- instances = self._forward_mask(features, instances)
- instances = self._forward_keypoint(features, instances)
- return instances
-
- def _forward_box(self, features: Dict[str, torch.Tensor], proposals: List[Instances]):
- """
- Forward logic of the box prediction branch. If `self.train_on_pred_boxes is True`,
- the function puts predicted boxes in the `proposal_boxes` field of `proposals` argument.
-
- Args:
- features (dict[str, Tensor]): mapping from feature map names to tensor.
- Same as in :meth:`ROIHeads.forward`.
- proposals (list[Instances]): the per-image object proposals with
- their matching ground truth.
- Each has fields "proposal_boxes", and "objectness_logits",
- "gt_classes", "gt_boxes".
-
- Returns:
- In training, a dict of losses.
- In inference, a list of `Instances`, the predicted instances.
- """
- features = [features[f] for f in self.box_in_features]
- box_features = self.box_pooler(features, [x.proposal_boxes for x in proposals])
- box_features = self.box_head(box_features)
- predictions = self.box_predictor(box_features)
- del box_features
-
- if self.training:
- losses = self.box_predictor.losses(predictions, proposals)
- # proposals is modified in-place below, so losses must be computed first.
- if self.train_on_pred_boxes:
- with torch.no_grad():
- pred_boxes = self.box_predictor.predict_boxes_for_gt_classes(
- predictions, proposals
- )
- for proposals_per_image, pred_boxes_per_image in zip(proposals, pred_boxes):
- proposals_per_image.proposal_boxes = Boxes(pred_boxes_per_image)
- return losses
- else:
- pred_instances, _ = self.box_predictor.inference(predictions, proposals)
- return pred_instances
-
- def _forward_mask(self, features: Dict[str, torch.Tensor], instances: List[Instances]):
- """
- Forward logic of the mask prediction branch.
-
- Args:
- features (dict[str, Tensor]): mapping from feature map names to tensor.
- Same as in :meth:`ROIHeads.forward`.
- instances (list[Instances]): the per-image instances to train/predict masks.
- In training, they can be the proposals.
- In inference, they can be the boxes predicted by R-CNN box head.
-
- Returns:
- In training, a dict of losses.
- In inference, update `instances` with new fields "pred_masks" and return it.
- """
- if not self.mask_on:
- return {} if self.training else instances
-
- if self.training:
- # head is only trained on positive proposals.
- instances, _ = select_foreground_proposals(instances, self.num_classes)
-
- if self.mask_pooler is not None:
- features = [features[f] for f in self.mask_in_features]
- boxes = [x.proposal_boxes if self.training else x.pred_boxes for x in instances]
- features = self.mask_pooler(features, boxes)
- else:
- features = {f: features[f] for f in self.mask_in_features}
- return self.mask_head(features, instances)
-
- def _forward_keypoint(self, features: Dict[str, torch.Tensor], instances: List[Instances]):
- """
- Forward logic of the keypoint prediction branch.
-
- Args:
- features (dict[str, Tensor]): mapping from feature map names to tensor.
- Same as in :meth:`ROIHeads.forward`.
- instances (list[Instances]): the per-image instances to train/predict keypoints.
- In training, they can be the proposals.
- In inference, they can be the boxes predicted by R-CNN box head.
-
- Returns:
- In training, a dict of losses.
- In inference, update `instances` with new fields "pred_keypoints" and return it.
- """
- if not self.keypoint_on:
- return {} if self.training else instances
-
- if self.training:
- # head is only trained on positive proposals with >=1 visible keypoints.
- instances, _ = select_foreground_proposals(instances, self.num_classes)
- instances = select_proposals_with_visible_keypoints(instances)
-
- if self.keypoint_pooler is not None:
- features = [features[f] for f in self.keypoint_in_features]
- boxes = [x.proposal_boxes if self.training else x.pred_boxes for x in instances]
- features = self.keypoint_pooler(features, boxes)
- else:
- features = {f: features[f] for f in self.keypoint_in_features}
- return self.keypoint_head(features, instances)
diff --git a/spaces/Tetel/secondbing/EdgeGPT/__init__.py b/spaces/Tetel/secondbing/EdgeGPT/__init__.py
deleted file mode 100644
index e69de29bb2d1d6434b8b29ae775ad8c2e48c5391..0000000000000000000000000000000000000000
diff --git a/spaces/Toxfu/BIgVisionEffnetB2/model.py b/spaces/Toxfu/BIgVisionEffnetB2/model.py
deleted file mode 100644
index 52c2696c874740179528f0bdae8ce87b774a138f..0000000000000000000000000000000000000000
--- a/spaces/Toxfu/BIgVisionEffnetB2/model.py
+++ /dev/null
@@ -1,36 +0,0 @@
-import torch
-import torchvision
-
-from torch import nn
-
-
-def create_effnetb2_model(num_classes:int=3,
- seed:int=42):
- """Creates an EfficientNetB2 feature extractor model and transforms.
-
- Args:
- num_classes (int, optional): number of classes in the classifier head.
- Defaults to 3.
- seed (int, optional): random seed value. Defaults to 42.
-
- Returns:
- model (torch.nn.Module): EffNetB2 feature extractor model.
- transforms (torchvision.transforms): EffNetB2 image transforms.
- """
- # Create EffNetB2 pretrained weights, transforms and model
- weights = torchvision.models.EfficientNet_B2_Weights.DEFAULT
- transforms = weights.transforms()
- model = torchvision.models.efficientnet_b2(weights=weights)
-
- # Freeze all layers in base model
- for param in model.parameters():
- param.requires_grad = False
-
- # Change classifier head with random seed for reproducibility
- torch.manual_seed(seed)
- model.classifier = nn.Sequential(
- nn.Dropout(p=0.3, inplace=True),
- nn.Linear(in_features=1408, out_features=num_classes),
- )
-
- return model, transforms
diff --git a/spaces/Uppuluri/mychatbotai/app.py b/spaces/Uppuluri/mychatbotai/app.py
deleted file mode 100644
index 2dbf3ae89c2e3fdab7134107dd346f984dca8eb1..0000000000000000000000000000000000000000
--- a/spaces/Uppuluri/mychatbotai/app.py
+++ /dev/null
@@ -1,34 +0,0 @@
-import os
-import gradio as gr
-from langchain.chat_models import ChatOpenAI
-from langchain import LLMChain, PromptTemplate
-from langchain.memory import ConversationBufferMemory
-
-OPENAI_API_KEY=os.getenv('OPENAI_API_KEY')
-
-template = """Meet Riya, your youthful and witty personal assistant! At 21 years old, she's full of energy and always eager to help. Riya's goal is to assist you with any questions or problems you might have. Her enthusiasm shines through in every response, making interactions with her enjoyable and engaging.
-{chat_history}
-User: {user_message}
-Chatbot:"""
-
-prompt = PromptTemplate(
- input_variables=["chat_history", "user_message"], template=template
-)
-
-memory = ConversationBufferMemory(memory_key="chat_history")
-
-llm_chain = LLMChain(
- llm=ChatOpenAI(temperature='0.5', model_name="gpt-3.5-turbo"),
- prompt=prompt,
- verbose=True,
- memory=memory,
-)
-
-def get_text_response(user_message,history):
- response = llm_chain.predict(user_message = user_message)
- return response
-
-demo = gr.ChatInterface(get_text_response)
-
-if __name__ == "__main__":
- demo.launch() #To create a public link, set `share=True` in `launch()`. To enable errors and logs, set `debug=True` in `launch()`.
diff --git a/spaces/VGG1555/VGG1/README.md b/spaces/VGG1555/VGG1/README.md
deleted file mode 100644
index a77f0699171af01cd870ccf0d950e683160d1293..0000000000000000000000000000000000000000
--- a/spaces/VGG1555/VGG1/README.md
+++ /dev/null
@@ -1,13 +0,0 @@
----
-title: VGG1
-emoji: 🐢
-colorFrom: green
-colorTo: red
-sdk: streamlit
-sdk_version: 1.21.0
-app_file: app.py
-pinned: false
-license: openrail
----
-
-Check out the configuration reference at https://huggingface.co/docs/hub/spaces-config-reference
diff --git a/spaces/Vipitis/ShaderEval/ShaderEval.py b/spaces/Vipitis/ShaderEval/ShaderEval.py
deleted file mode 100644
index 0ea60f9bc64966519e12d8ec90f821669fc2360e..0000000000000000000000000000000000000000
--- a/spaces/Vipitis/ShaderEval/ShaderEval.py
+++ /dev/null
@@ -1,242 +0,0 @@
-# Copyright 2020 The HuggingFace Datasets Authors and the current dataset script contributor.
-#
-# #TODO: license: MIT pending (evaluation suite itself can be completely open, nothing copyleft from the dataset reaches us here)
-"""TODO: Add a description here."""
-
-# TODO: Add BibTeX citation
-_CITATION = """\
-@InProceedings{huggingface:module,
-title = {A great new module},
-authors={huggingface, Inc.},
-year={2023}
-}
-"""
-
-# TODO: Add description of the module here
-_DESCRIPTION = """\
-This EvaluationSuite currently solves {1} tasks to test code intelligence of genereative language models for "creative programming" (fragment shaders).
-"""
-
-
-
-
-# via https://huggingface.co/docs/evaluate/evaluation_suite
-import evaluate
-from evaluate import evaluator #used by Suite.run()
-from evaluate.evaluator.utils import DatasetColumn # used in .prepare_data()
-from evaluate.evaluation_suite import SubTask
-from datasets import Dataset
-from typing import Any, Callable, Dict, List, Optional, Union # used in .prepare_pipeline()
-import transformers
-from transformers import Pipeline, pipeline, GenerationConfig, AutoTokenizer #GenerationConfig to specify greedy and avoid error
-from datasets import load_dataset #used by Suite.run()
-
-# write a custom evaluator, inherent from: https://github.com/huggingface/evaluate/blob/v0.4.0/src/evaluate/evaluator/text_generation.py#L31
-class ReturnGenerationEvaluator(evaluate.TextGenerationEvaluator):
- def __init__(self, task="text-generation", default_metric_name="exact_match", predictions_prefix: str = "generated"):
- super().__init__(task=task, default_metric_name=default_metric_name)
- self.predictions_prefix = predictions_prefix
-
- greedy_cfg = GenerationConfig(
- do_sample = False, # default to ensure greedy
- num_beams = 1, # same as above
- )
- PIPELINE_KWARGS = {"return_full_text": False, "generation_config":greedy_cfg} #these kwargs are for the pipeline call, not the pipeline init - but that seems to still work.
-
- # for the pipeline init we need to copy the whole function and add two lines. this still prints errors due to the pad_toke_id = eos_token_id change.
- # from: https://github.com/huggingface/evaluate/blob/v0.4.0/src/evaluate/evaluator/base.py#L375
- def prepare_pipeline(
- self,
- model_or_pipeline: Union[str, "Pipeline", Callable, "PreTrainedModel", "TFPreTrainedModel"], # noqa: F821
- tokenizer: Union["PreTrainedTokenizerBase", "FeatureExtractionMixin"] = None, # noqa: F821
- feature_extractor: Union["PreTrainedTokenizerBase", "FeatureExtractionMixin"] = None, # noqa: F821
- device: int = None,
- ):
- """
- Prepare pipeline.
- Args:
- model_or_pipeline (`str` or `Pipeline` or `Callable` or `PreTrainedModel` or `TFPreTrainedModel`,
- defaults to `None`):
- If the argument in not specified, we initialize the default pipeline for the task. If the argument is of the type `str` or
- is a model instance, we use it to initialize a new `Pipeline` with the given model. Otherwise we assume the
- argument specifies a pre-initialized pipeline.
- preprocessor (`PreTrainedTokenizerBase` or `FeatureExtractionMixin`, *optional*, defaults to `None`):
- Argument can be used to overwrite a default preprocessor if `model_or_pipeline` represents a model for
- which we build a pipeline. If `model_or_pipeline` is `None` or a pre-initialized pipeline, we ignore
- this argument.
- Returns:
- The initialized pipeline, with modifications for the specific task of generating text, even with long inputs.
- """
-
- if device is None:
- device = self._infer_device()
-
- if (
- isinstance(model_or_pipeline, str)
- or isinstance(model_or_pipeline, transformers.PreTrainedModel)
- or isinstance(model_or_pipeline, transformers.TFPreTrainedModel)
- ):
- if isinstance(model_or_pipeline, str):
- # load tokenizer manually, since the pipeline does fail to do so at times. needed for bigcode/santacoder for example.
- tokenizer = AutoTokenizer.from_pretrained(model_or_pipeline, trust_remote_code=True)
-
- pipe = pipeline(
- self.task,
- model=model_or_pipeline,
- tokenizer=tokenizer,
- feature_extractor=feature_extractor,
- device=device,
- # my additions here:
- handle_long_generation= "hole", #our solution? relevant: https://github.com/huggingface/transformers/issues/14033#issuecomment-948385227
- # pad_token_id=tokenizer.eos_token_id, #to avoid the warning, however there might be issues as tokenizers will call this differently.
- do_sample=False, #important to get reproduceable results but we need to make sure the generator is deterministic
- trust_remote_code=True, # do we need this for some custom models? need to test if it works right here. one example is bigcode/santacoder
- )
- else:
- if model_or_pipeline is None:
- pipe = pipeline(self.task, device=device)
- else:
- pipe = model_or_pipeline
- # if tokenizer is not None and feature_extractor is not None:
- # logger.warning("Ignoring the value of the preprocessor argument (`tokenizer` or `feature_extractor`).") #excluded warning because I didn't import logger
- if (pipe.task != self.task) and not (self.task == "translation" and pipe.task.startswith("translation")):
- raise ValueError(
- f"Incompatible `model_or_pipeline`. Please specify `model_or_pipeline` compatible with the `{self.task}` task."
- )
-
- # fixinging default for max_lenght
- pipe.model.config.max_length = self._resolve_context_lenght(pipe=pipe)
-
- # update the generation config with information from the pipe
- self._update_generation_config(pipe)
-
- return pipe
-
- def _update_generation_config(self, pipe):
- """
- Update the generation config with information from the pipe. Sets eos_token_id and pad_token_id.
- Args:
- pipe (:class:`~transformers.Pipeline`): we need to access the tokenizer.vocab
- returns:
- None
- """
- semicolon_token_ids = [v for k,v in pipe.tokenizer.vocab.items() if ";" in k] # this requires the tokenizer, which we only have once a pipe is made.
- # GenerationConfig.update also exists, but it does only replace, not add kwargs.
- self.greedy_cfg.eos_token_id = semicolon_token_ids # eos_token_id can be a list, so we give them all possible tokens.
- self.greedy_cfg.pad_token_id = semicolon_token_ids[0] # pad_token_id has to be an int, so we just take the first one.
- return None # doesn't do anything?
-
- def _resolve_context_lenght(self, model_or_pipeline=None, pipe=None): #TODO should really copy the typing hints here.
- if isinstance(model_or_pipeline, transformers.GPT2Model): # you are comparing a string here -.-
- return model_or_pipeline.config.n_ctx # how GPT2 models might handle is, seen with
- if pipe is not None: #should I figure out a way to pass this.
- return pipe.tokenizer.model_max_length # this is set to something small for pipeline default task, but we would want to put it to the max instead.
- # tokenizer needs to know the context length for our pipe strategy, but it has to be passed to the tokenizer, not model.
- # the tokenizer should read from the model config, but that can be wrong, or it has a task overwrite (for "text-generation" for example you get 50)
- #model_or_pipeline only exists via the .compute call, so we have to take it in
- # model_or_pipeline.tokenier.config.max_new_tokens = 1024 # we shouldn't return it, but overwrite the tokenizer config, which the pipeline relies on.
-
- return 1024 # we shouldn't return it, but overwrite the tokenizer config, which the pipeline relies on.
-
- def _estimate_stopping(self, labels, **kwargs):
- """ estimates max_new_tokens for the pipeline call
- by counting the characters in the longest string of the references adding 5 (for good measure but probably not needed)
- Args:
- labels: A list of dicts by knowing the labels
- Returns:
- `int`: the estimated max_new_tokens, should be smaller than context_lenght in all cases
- """
- context_lenght = self._resolve_context_lenght(**kwargs)
- estimate = min(max([len(ref) for ref in labels]) + 5, context_lenght) #does the min call get done inside the pipeline anyway? is there even a single case where the return statement is this long?
- return estimate
-
- # this one needs to be adjusted
- def predictions_processor(self, predictions, *args, **kwargs):
- """
- processes the output of the pipeline to be compatible with the metric.
- generated texts cut off by the first semicolon and whitespaces are stripped (using python str builtins)
- Args:
- predictions: A list of lists of dicts
- Returns:
- `dict`: All the processed text are flattened and stored under the "predictions" key.
- """
- return {"predictions": [pred[f"{self.predictions_prefix}_text"].split(";")[0].strip() for pred_list in predictions for pred in pred_list]}
-
- # straight copy, doesn't seem to give me the
- def prepare_data(self, data: Dataset, input_column: str, label_column: str, *args, **kwargs):
- """
- Prepare data.
- Args:
- data (`Dataset`): Specifies the dataset we will run evaluation on.
- input_column (`str`, defaults to `"text"`):
- the name of the column containing the text feature in the dataset specified by `data`.
- label_column (`str`, defaults to `"label"`):
- the name of the column containing the labels in the dataset specified by `data`.
- Returns:
- `dict`: metric inputs. everything before the first semicolon and whitespaces are stripped (using python str builtins, just like the pred prep)
- `list`: pipeline inputs.
- """
-
- self.check_required_columns(data, {"input_column": input_column, "label_column": label_column}) #this will throw and exception with useful error messages
-
- # don't put everything in the return statement, so you have the control...
- references = [ref.split(";")[0].strip() for ref in data[label_column]]
- self.PIPELINE_KWARGS.update({"max_new_tokens": self._estimate_stopping(references)}) #this is a hack, does it work tho?
-
- return {"references": references}, data[input_column] #DatasetColumn(data, input_column) doesn't seem to work. data[input_column] does, but ignores any of the features of the helper class..
-
-
-
-# via: https://huggingface.co/docs/evaluate/evaluation_suite
-# relevant source: https://github.com/huggingface/evaluate/blob/v0.4.0/src/evaluate/evaluation_suite/__init__.py
-class Suite(evaluate.EvaluationSuite):
-
-
- def __init__(self, name):
- super().__init__(name)
- self.preprocessor = lambda x: {"return_statement": x["return_statement"].split(";")[0]} #like this? refactored to RetrunGenerationEvaluator
- self.suite = [
- # more subtasks are only possible once we can pass custom evaluators. -> https://github.com/huggingface/evaluate/pull/367
- SubTask( #this one is adjusted already
- task_type="text-generation", #this call an evaluator, but can you specify your own custom evaluator instead?
- data="Vipitis/Shadertoys-fine",
- subset="return_completion",
- split="test", # use this to select a subset of the data during testing, perhaps remove later?
- args_for_task={
- # "metric": "exact_match",
- "input_column": "body",
- "label_column": "return_statement",
- }
- )
- ]
-
- # from: https://github.com/huggingface/evaluate/blob/v0.4.0/src/evaluate/evaluation_suite/__init__.py#LL103C5-L129C27
- def run(
- self, model_or_pipeline: Union[str, "Pipeline", Callable, "PreTrainedModel", "TFPreTrainedModel"] = "Vipitis/santacoder-finetuned-Shadertoys-fine", #not so useful default model?
- snippet: int = "" # noqa: F821
- ) -> Dict[str, float]:
-
- self.assert_suite_nonempty()
-
- results_all = []
- for task in self.suite:
-
- task_name = task.data
-
- if task.data_preprocessor: # task requires extra preprocessing is all done inside the Evaluator
- ds = load_dataset(task.data, name=task.subset, split=(task.split + f"[:{snippet}]"))
- task.data = ds.map(task.data_preprocessor)
-
- task_evaluator = ReturnGenerationEvaluator() #this is the change we make: specify our custom evaluator from above.
- args_for_task = task.args_for_task
- args_for_task["model_or_pipeline"] = model_or_pipeline
- args_for_task["data"] = task.data
- args_for_task["subset"] = task.subset
- args_for_task["split"] = (task.split + f"[:{snippet}]") #make a downselection of the split via keywordarg in the .run() call?
- results = task_evaluator.compute(**args_for_task)
-
- results["model_cp"] = model_or_pipeline #added this to the output, should be useful. But be careful when passed something that is not a string. #TODO: currently the same for all tasks, maybe move to the list?
- results["task_name"] = task_name + "/" + task.subset if task.subset else task_name
- results["data_preprocessor"] = str(task.data_preprocessor) if task.data_preprocessor is not None else None
- results_all.append(results)
- return results_all
\ No newline at end of file
diff --git a/spaces/Xenova/doodle-dash/assets/index-973c1bab.js b/spaces/Xenova/doodle-dash/assets/index-973c1bab.js
deleted file mode 100644
index dca22da9c3f41d05d03079bd2ffe549ab57643e2..0000000000000000000000000000000000000000
--- a/spaces/Xenova/doodle-dash/assets/index-973c1bab.js
+++ /dev/null
@@ -1,60 +0,0 @@
-(function(){const t=document.createElement("link").relList;if(t&&t.supports&&t.supports("modulepreload"))return;for(const i of document.querySelectorAll('link[rel="modulepreload"]'))r(i);new MutationObserver(i=>{for(const o of i)if(o.type==="childList")for(const s of o.addedNodes)s.tagName==="LINK"&&s.rel==="modulepreload"&&r(s)}).observe(document,{childList:!0,subtree:!0});function n(i){const o={};return i.integrity&&(o.integrity=i.integrity),i.referrerPolicy&&(o.referrerPolicy=i.referrerPolicy),i.crossOrigin==="use-credentials"?o.credentials="include":i.crossOrigin==="anonymous"?o.credentials="omit":o.credentials="same-origin",o}function r(i){if(i.ep)return;i.ep=!0;const o=n(i);fetch(i.href,o)}})();function um(e){return e&&e.__esModule&&Object.prototype.hasOwnProperty.call(e,"default")?e.default:e}var Lf={exports:{}},ko={},Mf={exports:{}},I={};/**
- * @license React
- * react.production.min.js
- *
- * Copyright (c) Facebook, Inc. and its affiliates.
- *
- * This source code is licensed under the MIT license found in the
- * LICENSE file in the root directory of this source tree.
- */var Jr=Symbol.for("react.element"),cm=Symbol.for("react.portal"),fm=Symbol.for("react.fragment"),dm=Symbol.for("react.strict_mode"),pm=Symbol.for("react.profiler"),hm=Symbol.for("react.provider"),mm=Symbol.for("react.context"),gm=Symbol.for("react.forward_ref"),ym=Symbol.for("react.suspense"),vm=Symbol.for("react.memo"),wm=Symbol.for("react.lazy"),au=Symbol.iterator;function xm(e){return e===null||typeof e!="object"?null:(e=au&&e[au]||e["@@iterator"],typeof e=="function"?e:null)}var Rf={isMounted:function(){return!1},enqueueForceUpdate:function(){},enqueueReplaceState:function(){},enqueueSetState:function(){}},Vf=Object.assign,Af={};function er(e,t,n){this.props=e,this.context=t,this.refs=Af,this.updater=n||Rf}er.prototype.isReactComponent={};er.prototype.setState=function(e,t){if(typeof e!="object"&&typeof e!="function"&&e!=null)throw Error("setState(...): takes an object of state variables to update or a function which returns an object of state variables.");this.updater.enqueueSetState(this,e,t,"setState")};er.prototype.forceUpdate=function(e){this.updater.enqueueForceUpdate(this,e,"forceUpdate")};function _f(){}_f.prototype=er.prototype;function Wl(e,t,n){this.props=e,this.context=t,this.refs=Af,this.updater=n||Rf}var Gl=Wl.prototype=new _f;Gl.constructor=Wl;Vf(Gl,er.prototype);Gl.isPureReactComponent=!0;var uu=Array.isArray,Nf=Object.prototype.hasOwnProperty,Kl={current:null},Of={key:!0,ref:!0,__self:!0,__source:!0};function jf(e,t,n){var r,i={},o=null,s=null;if(t!=null)for(r in t.ref!==void 0&&(s=t.ref),t.key!==void 0&&(o=""+t.key),t)Nf.call(t,r)&&!Of.hasOwnProperty(r)&&(i[r]=t[r]);var l=arguments.length-2;if(l===1)i.children=n;else if(1>>1,F=L[D];if(0>>1;Di(qt,N))mei(Ue,qt)?(L[D]=Ue,L[me]=N,D=me):(L[D]=qt,L[ee]=N,D=ee);else if(mei(Ue,N))L[D]=Ue,L[me]=N,D=me;else break e}}return _}function i(L,_){var N=L.sortIndex-_.sortIndex;return N!==0?N:L.id-_.id}if(typeof performance=="object"&&typeof performance.now=="function"){var o=performance;e.unstable_now=function(){return o.now()}}else{var s=Date,l=s.now();e.unstable_now=function(){return s.now()-l}}var a=[],u=[],c=1,f=null,p=3,g=!1,y=!1,v=!1,T=typeof setTimeout=="function"?setTimeout:null,m=typeof clearTimeout=="function"?clearTimeout:null,d=typeof setImmediate<"u"?setImmediate:null;typeof navigator<"u"&&navigator.scheduling!==void 0&&navigator.scheduling.isInputPending!==void 0&&navigator.scheduling.isInputPending.bind(navigator.scheduling);function h(L){for(var _=n(u);_!==null;){if(_.callback===null)r(u);else if(_.startTime<=L)r(u),_.sortIndex=_.expirationTime,t(a,_);else break;_=n(u)}}function w(L){if(v=!1,h(L),!y)if(n(a)!==null)y=!0,he(x);else{var _=n(u);_!==null&&Ae(w,_.startTime-L)}}function x(L,_){y=!1,v&&(v=!1,m(k),k=-1),g=!0;var N=p;try{for(h(_),f=n(a);f!==null&&(!(f.expirationTime>_)||L&&!j());){var D=f.callback;if(typeof D=="function"){f.callback=null,p=f.priorityLevel;var F=D(f.expirationTime<=_);_=e.unstable_now(),typeof F=="function"?f.callback=F:f===n(a)&&r(a),h(_)}else r(a);f=n(a)}if(f!==null)var Be=!0;else{var ee=n(u);ee!==null&&Ae(w,ee.startTime-_),Be=!1}return Be}finally{f=null,p=N,g=!1}}var C=!1,E=null,k=-1,V=5,R=-1;function j(){return!(e.unstable_now()-RL||125D?(L.sortIndex=N,t(u,L),n(a)===null&&L===n(u)&&(v?(m(k),k=-1):v=!0,Ae(w,N-D))):(L.sortIndex=F,t(a,L),y||g||(y=!0,he(x))),L},e.unstable_shouldYield=j,e.unstable_wrapCallback=function(L){var _=p;return function(){var N=p;p=_;try{return L.apply(this,arguments)}finally{p=N}}}})(Bf);zf.exports=Bf;var Vm=zf.exports;/**
- * @license React
- * react-dom.production.min.js
- *
- * Copyright (c) Facebook, Inc. and its affiliates.
- *
- * This source code is licensed under the MIT license found in the
- * LICENSE file in the root directory of this source tree.
- */var Uf=S,je=Vm;function P(e){for(var t="https://reactjs.org/docs/error-decoder.html?invariant="+e,n=1;n"u"||typeof window.document>"u"||typeof window.document.createElement>"u"),Fs=Object.prototype.hasOwnProperty,Am=/^[:A-Z_a-z\u00C0-\u00D6\u00D8-\u00F6\u00F8-\u02FF\u0370-\u037D\u037F-\u1FFF\u200C-\u200D\u2070-\u218F\u2C00-\u2FEF\u3001-\uD7FF\uF900-\uFDCF\uFDF0-\uFFFD][:A-Z_a-z\u00C0-\u00D6\u00D8-\u00F6\u00F8-\u02FF\u0370-\u037D\u037F-\u1FFF\u200C-\u200D\u2070-\u218F\u2C00-\u2FEF\u3001-\uD7FF\uF900-\uFDCF\uFDF0-\uFFFD\-.0-9\u00B7\u0300-\u036F\u203F-\u2040]*$/,fu={},du={};function _m(e){return Fs.call(du,e)?!0:Fs.call(fu,e)?!1:Am.test(e)?du[e]=!0:(fu[e]=!0,!1)}function Nm(e,t,n,r){if(n!==null&&n.type===0)return!1;switch(typeof t){case"function":case"symbol":return!0;case"boolean":return r?!1:n!==null?!n.acceptsBooleans:(e=e.toLowerCase().slice(0,5),e!=="data-"&&e!=="aria-");default:return!1}}function Om(e,t,n,r){if(t===null||typeof t>"u"||Nm(e,t,n,r))return!0;if(r)return!1;if(n!==null)switch(n.type){case 3:return!t;case 4:return t===!1;case 5:return isNaN(t);case 6:return isNaN(t)||1>t}return!1}function Ce(e,t,n,r,i,o,s){this.acceptsBooleans=t===2||t===3||t===4,this.attributeName=r,this.attributeNamespace=i,this.mustUseProperty=n,this.propertyName=e,this.type=t,this.sanitizeURL=o,this.removeEmptyString=s}var fe={};"children dangerouslySetInnerHTML defaultValue defaultChecked innerHTML suppressContentEditableWarning suppressHydrationWarning style".split(" ").forEach(function(e){fe[e]=new Ce(e,0,!1,e,null,!1,!1)});[["acceptCharset","accept-charset"],["className","class"],["htmlFor","for"],["httpEquiv","http-equiv"]].forEach(function(e){var t=e[0];fe[t]=new Ce(t,1,!1,e[1],null,!1,!1)});["contentEditable","draggable","spellCheck","value"].forEach(function(e){fe[e]=new Ce(e,2,!1,e.toLowerCase(),null,!1,!1)});["autoReverse","externalResourcesRequired","focusable","preserveAlpha"].forEach(function(e){fe[e]=new Ce(e,2,!1,e,null,!1,!1)});"allowFullScreen async autoFocus autoPlay controls default defer disabled disablePictureInPicture disableRemotePlayback formNoValidate hidden loop noModule noValidate open playsInline readOnly required reversed scoped seamless itemScope".split(" ").forEach(function(e){fe[e]=new Ce(e,3,!1,e.toLowerCase(),null,!1,!1)});["checked","multiple","muted","selected"].forEach(function(e){fe[e]=new Ce(e,3,!0,e,null,!1,!1)});["capture","download"].forEach(function(e){fe[e]=new Ce(e,4,!1,e,null,!1,!1)});["cols","rows","size","span"].forEach(function(e){fe[e]=new Ce(e,6,!1,e,null,!1,!1)});["rowSpan","start"].forEach(function(e){fe[e]=new Ce(e,5,!1,e.toLowerCase(),null,!1,!1)});var Xl=/[\-:]([a-z])/g;function Zl(e){return e[1].toUpperCase()}"accent-height alignment-baseline arabic-form baseline-shift cap-height clip-path clip-rule color-interpolation color-interpolation-filters color-profile color-rendering dominant-baseline enable-background fill-opacity fill-rule flood-color flood-opacity font-family font-size font-size-adjust font-stretch font-style font-variant font-weight glyph-name glyph-orientation-horizontal glyph-orientation-vertical horiz-adv-x horiz-origin-x image-rendering letter-spacing lighting-color marker-end marker-mid marker-start overline-position overline-thickness paint-order panose-1 pointer-events rendering-intent shape-rendering stop-color stop-opacity strikethrough-position strikethrough-thickness stroke-dasharray stroke-dashoffset stroke-linecap stroke-linejoin stroke-miterlimit stroke-opacity stroke-width text-anchor text-decoration text-rendering underline-position underline-thickness unicode-bidi unicode-range units-per-em v-alphabetic v-hanging v-ideographic v-mathematical vector-effect vert-adv-y vert-origin-x vert-origin-y word-spacing writing-mode xmlns:xlink x-height".split(" ").forEach(function(e){var t=e.replace(Xl,Zl);fe[t]=new Ce(t,1,!1,e,null,!1,!1)});"xlink:actuate xlink:arcrole xlink:role xlink:show xlink:title xlink:type".split(" ").forEach(function(e){var t=e.replace(Xl,Zl);fe[t]=new Ce(t,1,!1,e,"http://www.w3.org/1999/xlink",!1,!1)});["xml:base","xml:lang","xml:space"].forEach(function(e){var t=e.replace(Xl,Zl);fe[t]=new Ce(t,1,!1,e,"http://www.w3.org/XML/1998/namespace",!1,!1)});["tabIndex","crossOrigin"].forEach(function(e){fe[e]=new Ce(e,1,!1,e.toLowerCase(),null,!1,!1)});fe.xlinkHref=new Ce("xlinkHref",1,!1,"xlink:href","http://www.w3.org/1999/xlink",!0,!1);["src","href","action","formAction"].forEach(function(e){fe[e]=new Ce(e,1,!1,e.toLowerCase(),null,!0,!0)});function ql(e,t,n,r){var i=fe.hasOwnProperty(t)?fe[t]:null;(i!==null?i.type!==0:r||!(2l||i[s]!==o[l]){var a=`
-`+i[s].replace(" at new "," at ");return e.displayName&&a.includes("")&&(a=a.replace("",e.displayName)),a}while(1<=s&&0<=l);break}}}finally{Xo=!1,Error.prepareStackTrace=n}return(e=e?e.displayName||e.name:"")?pr(e):""}function jm(e){switch(e.tag){case 5:return pr(e.type);case 16:return pr("Lazy");case 13:return pr("Suspense");case 19:return pr("SuspenseList");case 0:case 2:case 15:return e=Zo(e.type,!1),e;case 11:return e=Zo(e.type.render,!1),e;case 1:return e=Zo(e.type,!0),e;default:return""}}function Us(e){if(e==null)return null;if(typeof e=="function")return e.displayName||e.name||null;if(typeof e=="string")return e;switch(e){case Cn:return"Fragment";case Pn:return"Portal";case Is:return"Profiler";case Jl:return"StrictMode";case zs:return"Suspense";case Bs:return"SuspenseList"}if(typeof e=="object")switch(e.$$typeof){case Wf:return(e.displayName||"Context")+".Consumer";case Hf:return(e._context.displayName||"Context")+".Provider";case bl:var t=e.render;return e=e.displayName,e||(e=t.displayName||t.name||"",e=e!==""?"ForwardRef("+e+")":"ForwardRef"),e;case ea:return t=e.displayName||null,t!==null?t:Us(e.type)||"Memo";case Et:t=e._payload,e=e._init;try{return Us(e(t))}catch{}}return null}function Fm(e){var t=e.type;switch(e.tag){case 24:return"Cache";case 9:return(t.displayName||"Context")+".Consumer";case 10:return(t._context.displayName||"Context")+".Provider";case 18:return"DehydratedFragment";case 11:return e=t.render,e=e.displayName||e.name||"",t.displayName||(e!==""?"ForwardRef("+e+")":"ForwardRef");case 7:return"Fragment";case 5:return t;case 4:return"Portal";case 3:return"Root";case 6:return"Text";case 16:return Us(t);case 8:return t===Jl?"StrictMode":"Mode";case 22:return"Offscreen";case 12:return"Profiler";case 21:return"Scope";case 13:return"Suspense";case 19:return"SuspenseList";case 25:return"TracingMarker";case 1:case 0:case 17:case 2:case 14:case 15:if(typeof t=="function")return t.displayName||t.name||null;if(typeof t=="string")return t}return null}function Ht(e){switch(typeof e){case"boolean":case"number":case"string":case"undefined":return e;case"object":return e;default:return""}}function Kf(e){var t=e.type;return(e=e.nodeName)&&e.toLowerCase()==="input"&&(t==="checkbox"||t==="radio")}function Im(e){var t=Kf(e)?"checked":"value",n=Object.getOwnPropertyDescriptor(e.constructor.prototype,t),r=""+e[t];if(!e.hasOwnProperty(t)&&typeof n<"u"&&typeof n.get=="function"&&typeof n.set=="function"){var i=n.get,o=n.set;return Object.defineProperty(e,t,{configurable:!0,get:function(){return i.call(this)},set:function(s){r=""+s,o.call(this,s)}}),Object.defineProperty(e,t,{enumerable:n.enumerable}),{getValue:function(){return r},setValue:function(s){r=""+s},stopTracking:function(){e._valueTracker=null,delete e[t]}}}}function ci(e){e._valueTracker||(e._valueTracker=Im(e))}function Qf(e){if(!e)return!1;var t=e._valueTracker;if(!t)return!0;var n=t.getValue(),r="";return e&&(r=Kf(e)?e.checked?"true":"false":e.value),e=r,e!==n?(t.setValue(e),!0):!1}function $i(e){if(e=e||(typeof document<"u"?document:void 0),typeof e>"u")return null;try{return e.activeElement||e.body}catch{return e.body}}function $s(e,t){var n=t.checked;return J({},t,{defaultChecked:void 0,defaultValue:void 0,value:void 0,checked:n??e._wrapperState.initialChecked})}function hu(e,t){var n=t.defaultValue==null?"":t.defaultValue,r=t.checked!=null?t.checked:t.defaultChecked;n=Ht(t.value!=null?t.value:n),e._wrapperState={initialChecked:r,initialValue:n,controlled:t.type==="checkbox"||t.type==="radio"?t.checked!=null:t.value!=null}}function Yf(e,t){t=t.checked,t!=null&&ql(e,"checked",t,!1)}function Hs(e,t){Yf(e,t);var n=Ht(t.value),r=t.type;if(n!=null)r==="number"?(n===0&&e.value===""||e.value!=n)&&(e.value=""+n):e.value!==""+n&&(e.value=""+n);else if(r==="submit"||r==="reset"){e.removeAttribute("value");return}t.hasOwnProperty("value")?Ws(e,t.type,n):t.hasOwnProperty("defaultValue")&&Ws(e,t.type,Ht(t.defaultValue)),t.checked==null&&t.defaultChecked!=null&&(e.defaultChecked=!!t.defaultChecked)}function mu(e,t,n){if(t.hasOwnProperty("value")||t.hasOwnProperty("defaultValue")){var r=t.type;if(!(r!=="submit"&&r!=="reset"||t.value!==void 0&&t.value!==null))return;t=""+e._wrapperState.initialValue,n||t===e.value||(e.value=t),e.defaultValue=t}n=e.name,n!==""&&(e.name=""),e.defaultChecked=!!e._wrapperState.initialChecked,n!==""&&(e.name=n)}function Ws(e,t,n){(t!=="number"||$i(e.ownerDocument)!==e)&&(n==null?e.defaultValue=""+e._wrapperState.initialValue:e.defaultValue!==""+n&&(e.defaultValue=""+n))}var hr=Array.isArray;function zn(e,t,n,r){if(e=e.options,t){t={};for(var i=0;i"+t.valueOf().toString()+"",t=fi.firstChild;e.firstChild;)e.removeChild(e.firstChild);for(;t.firstChild;)e.appendChild(t.firstChild)}});function Ar(e,t){if(t){var n=e.firstChild;if(n&&n===e.lastChild&&n.nodeType===3){n.nodeValue=t;return}}e.textContent=t}var vr={animationIterationCount:!0,aspectRatio:!0,borderImageOutset:!0,borderImageSlice:!0,borderImageWidth:!0,boxFlex:!0,boxFlexGroup:!0,boxOrdinalGroup:!0,columnCount:!0,columns:!0,flex:!0,flexGrow:!0,flexPositive:!0,flexShrink:!0,flexNegative:!0,flexOrder:!0,gridArea:!0,gridRow:!0,gridRowEnd:!0,gridRowSpan:!0,gridRowStart:!0,gridColumn:!0,gridColumnEnd:!0,gridColumnSpan:!0,gridColumnStart:!0,fontWeight:!0,lineClamp:!0,lineHeight:!0,opacity:!0,order:!0,orphans:!0,tabSize:!0,widows:!0,zIndex:!0,zoom:!0,fillOpacity:!0,floodOpacity:!0,stopOpacity:!0,strokeDasharray:!0,strokeDashoffset:!0,strokeMiterlimit:!0,strokeOpacity:!0,strokeWidth:!0},zm=["Webkit","ms","Moz","O"];Object.keys(vr).forEach(function(e){zm.forEach(function(t){t=t+e.charAt(0).toUpperCase()+e.substring(1),vr[t]=vr[e]})});function Jf(e,t,n){return t==null||typeof t=="boolean"||t===""?"":n||typeof t!="number"||t===0||vr.hasOwnProperty(e)&&vr[e]?(""+t).trim():t+"px"}function bf(e,t){e=e.style;for(var n in t)if(t.hasOwnProperty(n)){var r=n.indexOf("--")===0,i=Jf(n,t[n],r);n==="float"&&(n="cssFloat"),r?e.setProperty(n,i):e[n]=i}}var Bm=J({menuitem:!0},{area:!0,base:!0,br:!0,col:!0,embed:!0,hr:!0,img:!0,input:!0,keygen:!0,link:!0,meta:!0,param:!0,source:!0,track:!0,wbr:!0});function Qs(e,t){if(t){if(Bm[e]&&(t.children!=null||t.dangerouslySetInnerHTML!=null))throw Error(P(137,e));if(t.dangerouslySetInnerHTML!=null){if(t.children!=null)throw Error(P(60));if(typeof t.dangerouslySetInnerHTML!="object"||!("__html"in t.dangerouslySetInnerHTML))throw Error(P(61))}if(t.style!=null&&typeof t.style!="object")throw Error(P(62))}}function Ys(e,t){if(e.indexOf("-")===-1)return typeof t.is=="string";switch(e){case"annotation-xml":case"color-profile":case"font-face":case"font-face-src":case"font-face-uri":case"font-face-format":case"font-face-name":case"missing-glyph":return!1;default:return!0}}var Xs=null;function ta(e){return e=e.target||e.srcElement||window,e.correspondingUseElement&&(e=e.correspondingUseElement),e.nodeType===3?e.parentNode:e}var Zs=null,Bn=null,Un=null;function vu(e){if(e=ti(e)){if(typeof Zs!="function")throw Error(P(280));var t=e.stateNode;t&&(t=Do(t),Zs(e.stateNode,e.type,t))}}function ed(e){Bn?Un?Un.push(e):Un=[e]:Bn=e}function td(){if(Bn){var e=Bn,t=Un;if(Un=Bn=null,vu(e),t)for(e=0;e>>=0,e===0?32:31-(qm(e)/Jm|0)|0}var di=64,pi=4194304;function mr(e){switch(e&-e){case 1:return 1;case 2:return 2;case 4:return 4;case 8:return 8;case 16:return 16;case 32:return 32;case 64:case 128:case 256:case 512:case 1024:case 2048:case 4096:case 8192:case 16384:case 32768:case 65536:case 131072:case 262144:case 524288:case 1048576:case 2097152:return e&4194240;case 4194304:case 8388608:case 16777216:case 33554432:case 67108864:return e&130023424;case 134217728:return 134217728;case 268435456:return 268435456;case 536870912:return 536870912;case 1073741824:return 1073741824;default:return e}}function Ki(e,t){var n=e.pendingLanes;if(n===0)return 0;var r=0,i=e.suspendedLanes,o=e.pingedLanes,s=n&268435455;if(s!==0){var l=s&~i;l!==0?r=mr(l):(o&=s,o!==0&&(r=mr(o)))}else s=n&~i,s!==0?r=mr(s):o!==0&&(r=mr(o));if(r===0)return 0;if(t!==0&&t!==r&&!(t&i)&&(i=r&-r,o=t&-t,i>=o||i===16&&(o&4194240)!==0))return t;if(r&4&&(r|=n&16),t=e.entangledLanes,t!==0)for(e=e.entanglements,t&=r;0n;n++)t.push(e);return t}function br(e,t,n){e.pendingLanes|=t,t!==536870912&&(e.suspendedLanes=0,e.pingedLanes=0),e=e.eventTimes,t=31-et(t),e[t]=n}function ng(e,t){var n=e.pendingLanes&~t;e.pendingLanes=t,e.suspendedLanes=0,e.pingedLanes=0,e.expiredLanes&=t,e.mutableReadLanes&=t,e.entangledLanes&=t,t=e.entanglements;var r=e.eventTimes;for(e=e.expirationTimes;0=xr),Du=String.fromCharCode(32),Lu=!1;function Sd(e,t){switch(e){case"keyup":return Rg.indexOf(t.keyCode)!==-1;case"keydown":return t.keyCode!==229;case"keypress":case"mousedown":case"focusout":return!0;default:return!1}}function kd(e){return e=e.detail,typeof e=="object"&&"data"in e?e.data:null}var Tn=!1;function Ag(e,t){switch(e){case"compositionend":return kd(t);case"keypress":return t.which!==32?null:(Lu=!0,Du);case"textInput":return e=t.data,e===Du&&Lu?null:e;default:return null}}function _g(e,t){if(Tn)return e==="compositionend"||!ua&&Sd(e,t)?(e=wd(),Vi=sa=Vt=null,Tn=!1,e):null;switch(e){case"paste":return null;case"keypress":if(!(t.ctrlKey||t.altKey||t.metaKey)||t.ctrlKey&&t.altKey){if(t.char&&1=t)return{node:n,offset:t-e};e=r}e:{for(;n;){if(n.nextSibling){n=n.nextSibling;break e}n=n.parentNode}n=void 0}n=Au(n)}}function Ed(e,t){return e&&t?e===t?!0:e&&e.nodeType===3?!1:t&&t.nodeType===3?Ed(e,t.parentNode):"contains"in e?e.contains(t):e.compareDocumentPosition?!!(e.compareDocumentPosition(t)&16):!1:!1}function Dd(){for(var e=window,t=$i();t instanceof e.HTMLIFrameElement;){try{var n=typeof t.contentWindow.location.href=="string"}catch{n=!1}if(n)e=t.contentWindow;else break;t=$i(e.document)}return t}function ca(e){var t=e&&e.nodeName&&e.nodeName.toLowerCase();return t&&(t==="input"&&(e.type==="text"||e.type==="search"||e.type==="tel"||e.type==="url"||e.type==="password")||t==="textarea"||e.contentEditable==="true")}function $g(e){var t=Dd(),n=e.focusedElem,r=e.selectionRange;if(t!==n&&n&&n.ownerDocument&&Ed(n.ownerDocument.documentElement,n)){if(r!==null&&ca(n)){if(t=r.start,e=r.end,e===void 0&&(e=t),"selectionStart"in n)n.selectionStart=t,n.selectionEnd=Math.min(e,n.value.length);else if(e=(t=n.ownerDocument||document)&&t.defaultView||window,e.getSelection){e=e.getSelection();var i=n.textContent.length,o=Math.min(r.start,i);r=r.end===void 0?o:Math.min(r.end,i),!e.extend&&o>r&&(i=r,r=o,o=i),i=_u(n,o);var s=_u(n,r);i&&s&&(e.rangeCount!==1||e.anchorNode!==i.node||e.anchorOffset!==i.offset||e.focusNode!==s.node||e.focusOffset!==s.offset)&&(t=t.createRange(),t.setStart(i.node,i.offset),e.removeAllRanges(),o>r?(e.addRange(t),e.extend(s.node,s.offset)):(t.setEnd(s.node,s.offset),e.addRange(t)))}}for(t=[],e=n;e=e.parentNode;)e.nodeType===1&&t.push({element:e,left:e.scrollLeft,top:e.scrollTop});for(typeof n.focus=="function"&&n.focus(),n=0;n=document.documentMode,En=null,nl=null,kr=null,rl=!1;function Nu(e,t,n){var r=n.window===n?n.document:n.nodeType===9?n:n.ownerDocument;rl||En==null||En!==$i(r)||(r=En,"selectionStart"in r&&ca(r)?r={start:r.selectionStart,end:r.selectionEnd}:(r=(r.ownerDocument&&r.ownerDocument.defaultView||window).getSelection(),r={anchorNode:r.anchorNode,anchorOffset:r.anchorOffset,focusNode:r.focusNode,focusOffset:r.focusOffset}),kr&&Ir(kr,r)||(kr=r,r=Xi(nl,"onSelect"),0Mn||(e.current=ul[Mn],ul[Mn]=null,Mn--)}function H(e,t){Mn++,ul[Mn]=e.current,e.current=t}var Wt={},we=Yt(Wt),Le=Yt(!1),dn=Wt;function Qn(e,t){var n=e.type.contextTypes;if(!n)return Wt;var r=e.stateNode;if(r&&r.__reactInternalMemoizedUnmaskedChildContext===t)return r.__reactInternalMemoizedMaskedChildContext;var i={},o;for(o in n)i[o]=t[o];return r&&(e=e.stateNode,e.__reactInternalMemoizedUnmaskedChildContext=t,e.__reactInternalMemoizedMaskedChildContext=i),i}function Me(e){return e=e.childContextTypes,e!=null}function qi(){G(Le),G(we)}function Uu(e,t,n){if(we.current!==Wt)throw Error(P(168));H(we,t),H(Le,n)}function jd(e,t,n){var r=e.stateNode;if(t=t.childContextTypes,typeof r.getChildContext!="function")return n;r=r.getChildContext();for(var i in r)if(!(i in t))throw Error(P(108,Fm(e)||"Unknown",i));return J({},n,r)}function Ji(e){return e=(e=e.stateNode)&&e.__reactInternalMemoizedMergedChildContext||Wt,dn=we.current,H(we,e),H(Le,Le.current),!0}function $u(e,t,n){var r=e.stateNode;if(!r)throw Error(P(169));n?(e=jd(e,t,dn),r.__reactInternalMemoizedMergedChildContext=e,G(Le),G(we),H(we,e)):G(Le),H(Le,n)}var ft=null,Lo=!1,cs=!1;function Fd(e){ft===null?ft=[e]:ft.push(e)}function ey(e){Lo=!0,Fd(e)}function Xt(){if(!cs&&ft!==null){cs=!0;var e=0,t=B;try{var n=ft;for(B=1;e>=s,i-=s,dt=1<<32-et(t)+i|n<k?(V=E,E=null):V=E.sibling;var R=p(m,E,h[k],w);if(R===null){E===null&&(E=V);break}e&&E&&R.alternate===null&&t(m,E),d=o(R,d,k),C===null?x=R:C.sibling=R,C=R,E=V}if(k===h.length)return n(m,E),K&&tn(m,k),x;if(E===null){for(;kk?(V=E,E=null):V=E.sibling;var j=p(m,E,R.value,w);if(j===null){E===null&&(E=V);break}e&&E&&j.alternate===null&&t(m,E),d=o(j,d,k),C===null?x=j:C.sibling=j,C=j,E=V}if(R.done)return n(m,E),K&&tn(m,k),x;if(E===null){for(;!R.done;k++,R=h.next())R=f(m,R.value,w),R!==null&&(d=o(R,d,k),C===null?x=R:C.sibling=R,C=R);return K&&tn(m,k),x}for(E=r(m,E);!R.done;k++,R=h.next())R=g(E,m,k,R.value,w),R!==null&&(e&&R.alternate!==null&&E.delete(R.key===null?k:R.key),d=o(R,d,k),C===null?x=R:C.sibling=R,C=R);return e&&E.forEach(function(de){return t(m,de)}),K&&tn(m,k),x}function T(m,d,h,w){if(typeof h=="object"&&h!==null&&h.type===Cn&&h.key===null&&(h=h.props.children),typeof h=="object"&&h!==null){switch(h.$$typeof){case ui:e:{for(var x=h.key,C=d;C!==null;){if(C.key===x){if(x=h.type,x===Cn){if(C.tag===7){n(m,C.sibling),d=i(C,h.props.children),d.return=m,m=d;break e}}else if(C.elementType===x||typeof x=="object"&&x!==null&&x.$$typeof===Et&&Xu(x)===C.type){n(m,C.sibling),d=i(C,h.props),d.ref=ar(m,C,h),d.return=m,m=d;break e}n(m,C);break}else t(m,C);C=C.sibling}h.type===Cn?(d=fn(h.props.children,m.mode,w,h.key),d.return=m,m=d):(w=zi(h.type,h.key,h.props,null,m.mode,w),w.ref=ar(m,d,h),w.return=m,m=w)}return s(m);case Pn:e:{for(C=h.key;d!==null;){if(d.key===C)if(d.tag===4&&d.stateNode.containerInfo===h.containerInfo&&d.stateNode.implementation===h.implementation){n(m,d.sibling),d=i(d,h.children||[]),d.return=m,m=d;break e}else{n(m,d);break}else t(m,d);d=d.sibling}d=vs(h,m.mode,w),d.return=m,m=d}return s(m);case Et:return C=h._init,T(m,d,C(h._payload),w)}if(hr(h))return y(m,d,h,w);if(rr(h))return v(m,d,h,w);xi(m,h)}return typeof h=="string"&&h!==""||typeof h=="number"?(h=""+h,d!==null&&d.tag===6?(n(m,d.sibling),d=i(d,h),d.return=m,m=d):(n(m,d),d=ys(h,m.mode,w),d.return=m,m=d),s(m)):n(m,d)}return T}var Xn=Gd(!0),Kd=Gd(!1),ni={},at=Yt(ni),$r=Yt(ni),Hr=Yt(ni);function an(e){if(e===ni)throw Error(P(174));return e}function wa(e,t){switch(H(Hr,t),H($r,e),H(at,ni),e=t.nodeType,e){case 9:case 11:t=(t=t.documentElement)?t.namespaceURI:Ks(null,"");break;default:e=e===8?t.parentNode:t,t=e.namespaceURI||null,e=e.tagName,t=Ks(t,e)}G(at),H(at,t)}function Zn(){G(at),G($r),G(Hr)}function Qd(e){an(Hr.current);var t=an(at.current),n=Ks(t,e.type);t!==n&&(H($r,e),H(at,n))}function xa(e){$r.current===e&&(G(at),G($r))}var X=Yt(0);function io(e){for(var t=e;t!==null;){if(t.tag===13){var n=t.memoizedState;if(n!==null&&(n=n.dehydrated,n===null||n.data==="$?"||n.data==="$!"))return t}else if(t.tag===19&&t.memoizedProps.revealOrder!==void 0){if(t.flags&128)return t}else if(t.child!==null){t.child.return=t,t=t.child;continue}if(t===e)break;for(;t.sibling===null;){if(t.return===null||t.return===e)return null;t=t.return}t.sibling.return=t.return,t=t.sibling}return null}var fs=[];function Sa(){for(var e=0;en?n:4,e(!0);var r=ds.transition;ds.transition={};try{e(!1),t()}finally{B=n,ds.transition=r}}function up(){return Ye().memoizedState}function iy(e,t,n){var r=zt(e);if(n={lane:r,action:n,hasEagerState:!1,eagerState:null,next:null},cp(e))fp(t,n);else if(n=Ud(e,t,n,r),n!==null){var i=ke();tt(n,e,r,i),dp(n,t,r)}}function oy(e,t,n){var r=zt(e),i={lane:r,action:n,hasEagerState:!1,eagerState:null,next:null};if(cp(e))fp(t,i);else{var o=e.alternate;if(e.lanes===0&&(o===null||o.lanes===0)&&(o=t.lastRenderedReducer,o!==null))try{var s=t.lastRenderedState,l=o(s,n);if(i.hasEagerState=!0,i.eagerState=l,nt(l,s)){var a=t.interleaved;a===null?(i.next=i,ya(t)):(i.next=a.next,a.next=i),t.interleaved=i;return}}catch{}finally{}n=Ud(e,t,i,r),n!==null&&(i=ke(),tt(n,e,r,i),dp(n,t,r))}}function cp(e){var t=e.alternate;return e===q||t!==null&&t===q}function fp(e,t){Pr=oo=!0;var n=e.pending;n===null?t.next=t:(t.next=n.next,n.next=t),e.pending=t}function dp(e,t,n){if(n&4194240){var r=t.lanes;r&=e.pendingLanes,n|=r,t.lanes=n,ra(e,n)}}var so={readContext:Qe,useCallback:ge,useContext:ge,useEffect:ge,useImperativeHandle:ge,useInsertionEffect:ge,useLayoutEffect:ge,useMemo:ge,useReducer:ge,useRef:ge,useState:ge,useDebugValue:ge,useDeferredValue:ge,useTransition:ge,useMutableSource:ge,useSyncExternalStore:ge,useId:ge,unstable_isNewReconciler:!1},sy={readContext:Qe,useCallback:function(e,t){return it().memoizedState=[e,t===void 0?null:t],e},useContext:Qe,useEffect:qu,useImperativeHandle:function(e,t,n){return n=n!=null?n.concat([e]):null,Oi(4194308,4,ip.bind(null,t,e),n)},useLayoutEffect:function(e,t){return Oi(4194308,4,e,t)},useInsertionEffect:function(e,t){return Oi(4,2,e,t)},useMemo:function(e,t){var n=it();return t=t===void 0?null:t,e=e(),n.memoizedState=[e,t],e},useReducer:function(e,t,n){var r=it();return t=n!==void 0?n(t):t,r.memoizedState=r.baseState=t,e={pending:null,interleaved:null,lanes:0,dispatch:null,lastRenderedReducer:e,lastRenderedState:t},r.queue=e,e=e.dispatch=iy.bind(null,q,e),[r.memoizedState,e]},useRef:function(e){var t=it();return e={current:e},t.memoizedState=e},useState:Zu,useDebugValue:Ea,useDeferredValue:function(e){return it().memoizedState=e},useTransition:function(){var e=Zu(!1),t=e[0];return e=ry.bind(null,e[1]),it().memoizedState=e,[t,e]},useMutableSource:function(){},useSyncExternalStore:function(e,t,n){var r=q,i=it();if(K){if(n===void 0)throw Error(P(407));n=n()}else{if(n=t(),ae===null)throw Error(P(349));hn&30||Zd(r,t,n)}i.memoizedState=n;var o={value:n,getSnapshot:t};return i.queue=o,qu(Jd.bind(null,r,o,e),[e]),r.flags|=2048,Kr(9,qd.bind(null,r,o,n,t),void 0,null),n},useId:function(){var e=it(),t=ae.identifierPrefix;if(K){var n=pt,r=dt;n=(r&~(1<<32-et(r)-1)).toString(32)+n,t=":"+t+"R"+n,n=Wr++,0<\/script>",e=e.removeChild(e.firstChild)):typeof r.is=="string"?e=s.createElement(n,{is:r.is}):(e=s.createElement(n),n==="select"&&(s=e,r.multiple?s.multiple=!0:r.size&&(s.size=r.size))):e=s.createElementNS(e,n),e[st]=t,e[Ur]=r,Sp(e,t,!1,!1),t.stateNode=e;e:{switch(s=Ys(n,r),n){case"dialog":W("cancel",e),W("close",e),i=r;break;case"iframe":case"object":case"embed":W("load",e),i=r;break;case"video":case"audio":for(i=0;iJn&&(t.flags|=128,r=!0,ur(o,!1),t.lanes=4194304)}else{if(!r)if(e=io(s),e!==null){if(t.flags|=128,r=!0,n=e.updateQueue,n!==null&&(t.updateQueue=n,t.flags|=4),ur(o,!0),o.tail===null&&o.tailMode==="hidden"&&!s.alternate&&!K)return ye(t),null}else 2*te()-o.renderingStartTime>Jn&&n!==1073741824&&(t.flags|=128,r=!0,ur(o,!1),t.lanes=4194304);o.isBackwards?(s.sibling=t.child,t.child=s):(n=o.last,n!==null?n.sibling=s:t.child=s,o.last=s)}return o.tail!==null?(t=o.tail,o.rendering=t,o.tail=t.sibling,o.renderingStartTime=te(),t.sibling=null,n=X.current,H(X,r?n&1|2:n&1),t):(ye(t),null);case 22:case 23:return Aa(),r=t.memoizedState!==null,e!==null&&e.memoizedState!==null!==r&&(t.flags|=8192),r&&t.mode&1?_e&1073741824&&(ye(t),t.subtreeFlags&6&&(t.flags|=8192)):ye(t),null;case 24:return null;case 25:return null}throw Error(P(156,t.tag))}function hy(e,t){switch(da(t),t.tag){case 1:return Me(t.type)&&qi(),e=t.flags,e&65536?(t.flags=e&-65537|128,t):null;case 3:return Zn(),G(Le),G(we),Sa(),e=t.flags,e&65536&&!(e&128)?(t.flags=e&-65537|128,t):null;case 5:return xa(t),null;case 13:if(G(X),e=t.memoizedState,e!==null&&e.dehydrated!==null){if(t.alternate===null)throw Error(P(340));Yn()}return e=t.flags,e&65536?(t.flags=e&-65537|128,t):null;case 19:return G(X),null;case 4:return Zn(),null;case 10:return ga(t.type._context),null;case 22:case 23:return Aa(),null;case 24:return null;default:return null}}var ki=!1,ve=!1,my=typeof WeakSet=="function"?WeakSet:Set,M=null;function _n(e,t){var n=e.ref;if(n!==null)if(typeof n=="function")try{n(null)}catch(r){b(e,t,r)}else n.current=null}function Sl(e,t,n){try{n()}catch(r){b(e,t,r)}}var sc=!1;function gy(e,t){if(il=Qi,e=Dd(),ca(e)){if("selectionStart"in e)var n={start:e.selectionStart,end:e.selectionEnd};else e:{n=(n=e.ownerDocument)&&n.defaultView||window;var r=n.getSelection&&n.getSelection();if(r&&r.rangeCount!==0){n=r.anchorNode;var i=r.anchorOffset,o=r.focusNode;r=r.focusOffset;try{n.nodeType,o.nodeType}catch{n=null;break e}var s=0,l=-1,a=-1,u=0,c=0,f=e,p=null;t:for(;;){for(var g;f!==n||i!==0&&f.nodeType!==3||(l=s+i),f!==o||r!==0&&f.nodeType!==3||(a=s+r),f.nodeType===3&&(s+=f.nodeValue.length),(g=f.firstChild)!==null;)p=f,f=g;for(;;){if(f===e)break t;if(p===n&&++u===i&&(l=s),p===o&&++c===r&&(a=s),(g=f.nextSibling)!==null)break;f=p,p=f.parentNode}f=g}n=l===-1||a===-1?null:{start:l,end:a}}else n=null}n=n||{start:0,end:0}}else n=null;for(ol={focusedElem:e,selectionRange:n},Qi=!1,M=t;M!==null;)if(t=M,e=t.child,(t.subtreeFlags&1028)!==0&&e!==null)e.return=t,M=e;else for(;M!==null;){t=M;try{var y=t.alternate;if(t.flags&1024)switch(t.tag){case 0:case 11:case 15:break;case 1:if(y!==null){var v=y.memoizedProps,T=y.memoizedState,m=t.stateNode,d=m.getSnapshotBeforeUpdate(t.elementType===t.type?v:qe(t.type,v),T);m.__reactInternalSnapshotBeforeUpdate=d}break;case 3:var h=t.stateNode.containerInfo;h.nodeType===1?h.textContent="":h.nodeType===9&&h.documentElement&&h.removeChild(h.documentElement);break;case 5:case 6:case 4:case 17:break;default:throw Error(P(163))}}catch(w){b(t,t.return,w)}if(e=t.sibling,e!==null){e.return=t.return,M=e;break}M=t.return}return y=sc,sc=!1,y}function Cr(e,t,n){var r=t.updateQueue;if(r=r!==null?r.lastEffect:null,r!==null){var i=r=r.next;do{if((i.tag&e)===e){var o=i.destroy;i.destroy=void 0,o!==void 0&&Sl(t,n,o)}i=i.next}while(i!==r)}}function Vo(e,t){if(t=t.updateQueue,t=t!==null?t.lastEffect:null,t!==null){var n=t=t.next;do{if((n.tag&e)===e){var r=n.create;n.destroy=r()}n=n.next}while(n!==t)}}function kl(e){var t=e.ref;if(t!==null){var n=e.stateNode;switch(e.tag){case 5:e=n;break;default:e=n}typeof t=="function"?t(e):t.current=e}}function Cp(e){var t=e.alternate;t!==null&&(e.alternate=null,Cp(t)),e.child=null,e.deletions=null,e.sibling=null,e.tag===5&&(t=e.stateNode,t!==null&&(delete t[st],delete t[Ur],delete t[al],delete t[Jg],delete t[bg])),e.stateNode=null,e.return=null,e.dependencies=null,e.memoizedProps=null,e.memoizedState=null,e.pendingProps=null,e.stateNode=null,e.updateQueue=null}function Tp(e){return e.tag===5||e.tag===3||e.tag===4}function lc(e){e:for(;;){for(;e.sibling===null;){if(e.return===null||Tp(e.return))return null;e=e.return}for(e.sibling.return=e.return,e=e.sibling;e.tag!==5&&e.tag!==6&&e.tag!==18;){if(e.flags&2||e.child===null||e.tag===4)continue e;e.child.return=e,e=e.child}if(!(e.flags&2))return e.stateNode}}function Pl(e,t,n){var r=e.tag;if(r===5||r===6)e=e.stateNode,t?n.nodeType===8?n.parentNode.insertBefore(e,t):n.insertBefore(e,t):(n.nodeType===8?(t=n.parentNode,t.insertBefore(e,n)):(t=n,t.appendChild(e)),n=n._reactRootContainer,n!=null||t.onclick!==null||(t.onclick=Zi));else if(r!==4&&(e=e.child,e!==null))for(Pl(e,t,n),e=e.sibling;e!==null;)Pl(e,t,n),e=e.sibling}function Cl(e,t,n){var r=e.tag;if(r===5||r===6)e=e.stateNode,t?n.insertBefore(e,t):n.appendChild(e);else if(r!==4&&(e=e.child,e!==null))for(Cl(e,t,n),e=e.sibling;e!==null;)Cl(e,t,n),e=e.sibling}var ue=null,Je=!1;function Ct(e,t,n){for(n=n.child;n!==null;)Ep(e,t,n),n=n.sibling}function Ep(e,t,n){if(lt&&typeof lt.onCommitFiberUnmount=="function")try{lt.onCommitFiberUnmount(Po,n)}catch{}switch(n.tag){case 5:ve||_n(n,t);case 6:var r=ue,i=Je;ue=null,Ct(e,t,n),ue=r,Je=i,ue!==null&&(Je?(e=ue,n=n.stateNode,e.nodeType===8?e.parentNode.removeChild(n):e.removeChild(n)):ue.removeChild(n.stateNode));break;case 18:ue!==null&&(Je?(e=ue,n=n.stateNode,e.nodeType===8?us(e.parentNode,n):e.nodeType===1&&us(e,n),jr(e)):us(ue,n.stateNode));break;case 4:r=ue,i=Je,ue=n.stateNode.containerInfo,Je=!0,Ct(e,t,n),ue=r,Je=i;break;case 0:case 11:case 14:case 15:if(!ve&&(r=n.updateQueue,r!==null&&(r=r.lastEffect,r!==null))){i=r=r.next;do{var o=i,s=o.destroy;o=o.tag,s!==void 0&&(o&2||o&4)&&Sl(n,t,s),i=i.next}while(i!==r)}Ct(e,t,n);break;case 1:if(!ve&&(_n(n,t),r=n.stateNode,typeof r.componentWillUnmount=="function"))try{r.props=n.memoizedProps,r.state=n.memoizedState,r.componentWillUnmount()}catch(l){b(n,t,l)}Ct(e,t,n);break;case 21:Ct(e,t,n);break;case 22:n.mode&1?(ve=(r=ve)||n.memoizedState!==null,Ct(e,t,n),ve=r):Ct(e,t,n);break;default:Ct(e,t,n)}}function ac(e){var t=e.updateQueue;if(t!==null){e.updateQueue=null;var n=e.stateNode;n===null&&(n=e.stateNode=new my),t.forEach(function(r){var i=Ty.bind(null,e,r);n.has(r)||(n.add(r),r.then(i,i))})}}function Ze(e,t){var n=t.deletions;if(n!==null)for(var r=0;ri&&(i=s),r&=~o}if(r=i,r=te()-r,r=(120>r?120:480>r?480:1080>r?1080:1920>r?1920:3e3>r?3e3:4320>r?4320:1960*vy(r/1960))-r,10e?16:e,At===null)var r=!1;else{if(e=At,At=null,uo=0,z&6)throw Error(P(331));var i=z;for(z|=4,M=e.current;M!==null;){var o=M,s=o.child;if(M.flags&16){var l=o.deletions;if(l!==null){for(var a=0;ate()-Ra?cn(e,0):Ma|=n),Re(e,t)}function Np(e,t){t===0&&(e.mode&1?(t=pi,pi<<=1,!(pi&130023424)&&(pi=4194304)):t=1);var n=ke();e=xt(e,t),e!==null&&(br(e,t,n),Re(e,n))}function Cy(e){var t=e.memoizedState,n=0;t!==null&&(n=t.retryLane),Np(e,n)}function Ty(e,t){var n=0;switch(e.tag){case 13:var r=e.stateNode,i=e.memoizedState;i!==null&&(n=i.retryLane);break;case 19:r=e.stateNode;break;default:throw Error(P(314))}r!==null&&r.delete(t),Np(e,n)}var Op;Op=function(e,t,n){if(e!==null)if(e.memoizedProps!==t.pendingProps||Le.current)De=!0;else{if(!(e.lanes&n)&&!(t.flags&128))return De=!1,dy(e,t,n);De=!!(e.flags&131072)}else De=!1,K&&t.flags&1048576&&Id(t,eo,t.index);switch(t.lanes=0,t.tag){case 2:var r=t.type;ji(e,t),e=t.pendingProps;var i=Qn(t,we.current);Hn(t,n),i=Pa(null,t,r,e,i,n);var o=Ca();return t.flags|=1,typeof i=="object"&&i!==null&&typeof i.render=="function"&&i.$$typeof===void 0?(t.tag=1,t.memoizedState=null,t.updateQueue=null,Me(r)?(o=!0,Ji(t)):o=!1,t.memoizedState=i.state!==null&&i.state!==void 0?i.state:null,va(t),i.updater=Mo,t.stateNode=i,i._reactInternals=t,hl(t,r,e,n),t=yl(null,t,r,!0,o,n)):(t.tag=0,K&&o&&fa(t),Se(null,t,i,n),t=t.child),t;case 16:r=t.elementType;e:{switch(ji(e,t),e=t.pendingProps,i=r._init,r=i(r._payload),t.type=r,i=t.tag=Dy(r),e=qe(r,e),i){case 0:t=gl(null,t,r,e,n);break e;case 1:t=rc(null,t,r,e,n);break e;case 11:t=tc(null,t,r,e,n);break e;case 14:t=nc(null,t,r,qe(r.type,e),n);break e}throw Error(P(306,r,""))}return t;case 0:return r=t.type,i=t.pendingProps,i=t.elementType===r?i:qe(r,i),gl(e,t,r,i,n);case 1:return r=t.type,i=t.pendingProps,i=t.elementType===r?i:qe(r,i),rc(e,t,r,i,n);case 3:e:{if(vp(t),e===null)throw Error(P(387));r=t.pendingProps,o=t.memoizedState,i=o.element,$d(e,t),ro(t,r,null,n);var s=t.memoizedState;if(r=s.element,o.isDehydrated)if(o={element:r,isDehydrated:!1,cache:s.cache,pendingSuspenseBoundaries:s.pendingSuspenseBoundaries,transitions:s.transitions},t.updateQueue.baseState=o,t.memoizedState=o,t.flags&256){i=qn(Error(P(423)),t),t=ic(e,t,r,n,i);break e}else if(r!==i){i=qn(Error(P(424)),t),t=ic(e,t,r,n,i);break e}else for(Ne=jt(t.stateNode.containerInfo.firstChild),Oe=t,K=!0,be=null,n=Kd(t,null,r,n),t.child=n;n;)n.flags=n.flags&-3|4096,n=n.sibling;else{if(Yn(),r===i){t=St(e,t,n);break e}Se(e,t,r,n)}t=t.child}return t;case 5:return Qd(t),e===null&&fl(t),r=t.type,i=t.pendingProps,o=e!==null?e.memoizedProps:null,s=i.children,sl(r,i)?s=null:o!==null&&sl(r,o)&&(t.flags|=32),yp(e,t),Se(e,t,s,n),t.child;case 6:return e===null&&fl(t),null;case 13:return wp(e,t,n);case 4:return wa(t,t.stateNode.containerInfo),r=t.pendingProps,e===null?t.child=Xn(t,null,r,n):Se(e,t,r,n),t.child;case 11:return r=t.type,i=t.pendingProps,i=t.elementType===r?i:qe(r,i),tc(e,t,r,i,n);case 7:return Se(e,t,t.pendingProps,n),t.child;case 8:return Se(e,t,t.pendingProps.children,n),t.child;case 12:return Se(e,t,t.pendingProps.children,n),t.child;case 10:e:{if(r=t.type._context,i=t.pendingProps,o=t.memoizedProps,s=i.value,H(to,r._currentValue),r._currentValue=s,o!==null)if(nt(o.value,s)){if(o.children===i.children&&!Le.current){t=St(e,t,n);break e}}else for(o=t.child,o!==null&&(o.return=t);o!==null;){var l=o.dependencies;if(l!==null){s=o.child;for(var a=l.firstContext;a!==null;){if(a.context===r){if(o.tag===1){a=mt(-1,n&-n),a.tag=2;var u=o.updateQueue;if(u!==null){u=u.shared;var c=u.pending;c===null?a.next=a:(a.next=c.next,c.next=a),u.pending=a}}o.lanes|=n,a=o.alternate,a!==null&&(a.lanes|=n),dl(o.return,n,t),l.lanes|=n;break}a=a.next}}else if(o.tag===10)s=o.type===t.type?null:o.child;else if(o.tag===18){if(s=o.return,s===null)throw Error(P(341));s.lanes|=n,l=s.alternate,l!==null&&(l.lanes|=n),dl(s,n,t),s=o.sibling}else s=o.child;if(s!==null)s.return=o;else for(s=o;s!==null;){if(s===t){s=null;break}if(o=s.sibling,o!==null){o.return=s.return,s=o;break}s=s.return}o=s}Se(e,t,i.children,n),t=t.child}return t;case 9:return i=t.type,r=t.pendingProps.children,Hn(t,n),i=Qe(i),r=r(i),t.flags|=1,Se(e,t,r,n),t.child;case 14:return r=t.type,i=qe(r,t.pendingProps),i=qe(r.type,i),nc(e,t,r,i,n);case 15:return mp(e,t,t.type,t.pendingProps,n);case 17:return r=t.type,i=t.pendingProps,i=t.elementType===r?i:qe(r,i),ji(e,t),t.tag=1,Me(r)?(e=!0,Ji(t)):e=!1,Hn(t,n),Wd(t,r,i),hl(t,r,i,n),yl(null,t,r,!0,e,n);case 19:return xp(e,t,n);case 22:return gp(e,t,n)}throw Error(P(156,t.tag))};function jp(e,t){return ad(e,t)}function Ey(e,t,n,r){this.tag=e,this.key=n,this.sibling=this.child=this.return=this.stateNode=this.type=this.elementType=null,this.index=0,this.ref=null,this.pendingProps=t,this.dependencies=this.memoizedState=this.updateQueue=this.memoizedProps=null,this.mode=r,this.subtreeFlags=this.flags=0,this.deletions=null,this.childLanes=this.lanes=0,this.alternate=null}function Ge(e,t,n,r){return new Ey(e,t,n,r)}function Na(e){return e=e.prototype,!(!e||!e.isReactComponent)}function Dy(e){if(typeof e=="function")return Na(e)?1:0;if(e!=null){if(e=e.$$typeof,e===bl)return 11;if(e===ea)return 14}return 2}function Bt(e,t){var n=e.alternate;return n===null?(n=Ge(e.tag,t,e.key,e.mode),n.elementType=e.elementType,n.type=e.type,n.stateNode=e.stateNode,n.alternate=e,e.alternate=n):(n.pendingProps=t,n.type=e.type,n.flags=0,n.subtreeFlags=0,n.deletions=null),n.flags=e.flags&14680064,n.childLanes=e.childLanes,n.lanes=e.lanes,n.child=e.child,n.memoizedProps=e.memoizedProps,n.memoizedState=e.memoizedState,n.updateQueue=e.updateQueue,t=e.dependencies,n.dependencies=t===null?null:{lanes:t.lanes,firstContext:t.firstContext},n.sibling=e.sibling,n.index=e.index,n.ref=e.ref,n}function zi(e,t,n,r,i,o){var s=2;if(r=e,typeof e=="function")Na(e)&&(s=1);else if(typeof e=="string")s=5;else e:switch(e){case Cn:return fn(n.children,i,o,t);case Jl:s=8,i|=8;break;case Is:return e=Ge(12,n,t,i|2),e.elementType=Is,e.lanes=o,e;case zs:return e=Ge(13,n,t,i),e.elementType=zs,e.lanes=o,e;case Bs:return e=Ge(19,n,t,i),e.elementType=Bs,e.lanes=o,e;case Gf:return _o(n,i,o,t);default:if(typeof e=="object"&&e!==null)switch(e.$$typeof){case Hf:s=10;break e;case Wf:s=9;break e;case bl:s=11;break e;case ea:s=14;break e;case Et:s=16,r=null;break e}throw Error(P(130,e==null?e:typeof e,""))}return t=Ge(s,n,t,i),t.elementType=e,t.type=r,t.lanes=o,t}function fn(e,t,n,r){return e=Ge(7,e,r,t),e.lanes=n,e}function _o(e,t,n,r){return e=Ge(22,e,r,t),e.elementType=Gf,e.lanes=n,e.stateNode={isHidden:!1},e}function ys(e,t,n){return e=Ge(6,e,null,t),e.lanes=n,e}function vs(e,t,n){return t=Ge(4,e.children!==null?e.children:[],e.key,t),t.lanes=n,t.stateNode={containerInfo:e.containerInfo,pendingChildren:null,implementation:e.implementation},t}function Ly(e,t,n,r,i){this.tag=t,this.containerInfo=e,this.finishedWork=this.pingCache=this.current=this.pendingChildren=null,this.timeoutHandle=-1,this.callbackNode=this.pendingContext=this.context=null,this.callbackPriority=0,this.eventTimes=Jo(0),this.expirationTimes=Jo(-1),this.entangledLanes=this.finishedLanes=this.mutableReadLanes=this.expiredLanes=this.pingedLanes=this.suspendedLanes=this.pendingLanes=0,this.entanglements=Jo(0),this.identifierPrefix=r,this.onRecoverableError=i,this.mutableSourceEagerHydrationData=null}function Oa(e,t,n,r,i,o,s,l,a){return e=new Ly(e,t,n,l,a),t===1?(t=1,o===!0&&(t|=8)):t=0,o=Ge(3,null,null,t),e.current=o,o.stateNode=e,o.memoizedState={element:r,isDehydrated:n,cache:null,transitions:null,pendingSuspenseBoundaries:null},va(o),e}function My(e,t,n){var r=3"u"||typeof __REACT_DEVTOOLS_GLOBAL_HOOK__.checkDCE!="function"))try{__REACT_DEVTOOLS_GLOBAL_HOOK__.checkDCE(Bp)}catch(e){console.error(e)}}Bp(),If.exports=Ie;var Ny=If.exports,gc=Ny;js.createRoot=gc.createRoot,js.hydrateRoot=gc.hydrateRoot;function Oy(e,t){let n=0;return function(...r){const i=performance.now();i-n{for(let r of t)e.addEventListener(r,n)},Ss=(e,t,n)=>{for(let r of t)e.removeEventListener(r,n)},kc=e=>{if(e.touches&&e.touches[0]){const t=(e.target.offsetHeight-document.body.offsetHeight)/2;return[e.touches[0].clientX,e.touches[0].clientY-t]}else return[e.offsetX,e.offsetY]},Up=S.forwardRef(({onSketchChange:e,disabled:t},n)=>{const r=S.useRef(null),i=S.useRef(null),[o,s]=S.useState(null),[l,a]=S.useState(!1),[u,c]=S.useState(0);S.useEffect(()=>{const g=r.current;i.current||(i.current=g.getContext("2d",{willReadFrequently:!0}));const y=i.current;y.imageSmoothingEnabled=!0,y.lineWidth=Te.BRUSH_SIZE,y.lineJoin="round",y.lineCap="round",y.strokeStyle="black",y.shadowColor="rgba(0, 0, 0, 0.9)",y.shadowBlur=1;const v=(g.width-window.innerWidth)/2,T=(g.height-window.innerHeight)/2,m=Te.BRUSH_SIZE/2,d=()=>{g.style.width=window.innerWidth,g.style.height=window.innerHeight},h=C=>{if(t)return;const[E,k]=kc(C),V=E+v,R=k+T;y.moveTo(V,R),y.beginPath(),y.lineTo(V,R),y.arc(V,R,.5,0,2*Math.PI),y.stroke(),a(!0),s(j=>j===null?[V,R,V,R]:[Math.min(j[0],V-m),Math.min(j[1],R-m),Math.max(j[2],V+m),Math.max(j[3],R+m)]),e()},w=Oy(C=>{if(!l||t)return;c(j=>j+xc);const[E,k]=kc(C),V=E+v,R=k+T;s(j=>j===null?j:[Math.min(j[0],V-m),Math.min(j[1],R-m),Math.max(j[2],V+m),Math.max(j[3],R+m)]),y.lineTo(V,R),y.stroke(),e()},xc),x=()=>{a(!1)};return d(),window.addEventListener("resize",d),xs(g,yc,h),xs(g,vc,w),xs(g,wc,x),()=>{window.removeEventListener("resize",d),Ss(g,yc,h),Ss(g,vc,w),Ss(g,wc,x)}},[l,e,t]);const f=()=>{if(o===null)return null;const g=i.current;let y=o[0],v=o[1],T=o[2]-o[0],m=o[3]-o[1],d=2*ws;return T>=m?(d+=T,v=Math.max(v-(T-m)/2,0)):(d+=m,y=Math.max(y-(m-T)/2,0)),g.getImageData(y-ws,v-ws,d,d)},p=(g=!1)=>{s(null);const y=r.current;i.current.clearRect(0,0,y.width,y.height),a(!1),g&&c(0)};return S.useImperativeHandle(n,()=>({getCanvasData:f,clearCanvas:p,getTimeSpentDrawing:()=>u})),O.jsx("canvas",{className:"object-none w-full h-full",ref:r,width:Sc,height:Sc})});Up.displayName="SketchCanvas";const $p=S.createContext({transformPagePoint:e=>e,isStatic:!1,reducedMotion:"never"}),Io=S.createContext({}),zo=S.createContext(null),Bo=typeof document<"u",po=Bo?S.useLayoutEffect:S.useEffect,Hp=S.createContext({strict:!1});function Iy(e,t,n,r){const{visualElement:i}=S.useContext(Io),o=S.useContext(Hp),s=S.useContext(zo),l=S.useContext($p).reducedMotion,a=S.useRef();r=r||o.renderer,!a.current&&r&&(a.current=r(e,{visualState:t,parent:i,props:n,presenceContext:s,blockInitialAnimation:s?s.initial===!1:!1,reducedMotionConfig:l}));const u=a.current;return S.useInsertionEffect(()=>{u&&u.update(n,s)}),po(()=>{u&&u.render()}),S.useEffect(()=>{u&&u.updateFeatures()}),(window.HandoffAppearAnimations?po:S.useEffect)(()=>{u&&u.animationState&&u.animationState.animateChanges()}),u}function On(e){return typeof e=="object"&&Object.prototype.hasOwnProperty.call(e,"current")}function zy(e,t,n){return S.useCallback(r=>{r&&e.mount&&e.mount(r),t&&(r?t.mount(r):t.unmount()),n&&(typeof n=="function"?n(r):On(n)&&(n.current=r))},[t])}function Yr(e){return typeof e=="string"||Array.isArray(e)}function Uo(e){return typeof e=="object"&&typeof e.start=="function"}const za=["animate","whileInView","whileFocus","whileHover","whileTap","whileDrag","exit"],Ba=["initial",...za];function $o(e){return Uo(e.animate)||Ba.some(t=>Yr(e[t]))}function Wp(e){return!!($o(e)||e.variants)}function By(e,t){if($o(e)){const{initial:n,animate:r}=e;return{initial:n===!1||Yr(n)?n:void 0,animate:Yr(r)?r:void 0}}return e.inherit!==!1?t:{}}function Uy(e){const{initial:t,animate:n}=By(e,S.useContext(Io));return S.useMemo(()=>({initial:t,animate:n}),[Pc(t),Pc(n)])}function Pc(e){return Array.isArray(e)?e.join(" "):e}const Cc={animation:["animate","variants","whileHover","whileTap","exit","whileInView","whileFocus","whileDrag"],exit:["exit"],drag:["drag","dragControls"],focus:["whileFocus"],hover:["whileHover","onHoverStart","onHoverEnd"],tap:["whileTap","onTap","onTapStart","onTapCancel"],pan:["onPan","onPanStart","onPanSessionStart","onPanEnd"],inView:["whileInView","onViewportEnter","onViewportLeave"],layout:["layout","layoutId"]},Xr={};for(const e in Cc)Xr[e]={isEnabled:t=>Cc[e].some(n=>!!t[n])};function $y(e){for(const t in e)Xr[t]={...Xr[t],...e[t]}}const Ua=S.createContext({}),Gp=S.createContext({}),Hy=Symbol.for("motionComponentSymbol");function Wy({preloadedFeatures:e,createVisualElement:t,useRender:n,useVisualState:r,Component:i}){e&&$y(e);function o(l,a){let u;const c={...S.useContext($p),...l,layoutId:Gy(l)},{isStatic:f}=c,p=Uy(l),g=r(l,f);if(!f&&Bo){p.visualElement=Iy(i,g,c,t);const y=S.useContext(Gp),v=S.useContext(Hp).strict;p.visualElement&&(u=p.visualElement.loadFeatures(c,v,e,y))}return S.createElement(Io.Provider,{value:p},u&&p.visualElement?S.createElement(u,{visualElement:p.visualElement,...c}):null,n(i,l,zy(g,p.visualElement,a),g,f,p.visualElement))}const s=S.forwardRef(o);return s[Hy]=i,s}function Gy({layoutId:e}){const t=S.useContext(Ua).id;return t&&e!==void 0?t+"-"+e:e}function Ky(e){function t(r,i={}){return Wy(e(r,i))}if(typeof Proxy>"u")return t;const n=new Map;return new Proxy(t,{get:(r,i)=>(n.has(i)||n.set(i,t(i)),n.get(i))})}const Qy=["animate","circle","defs","desc","ellipse","g","image","line","filter","marker","mask","metadata","path","pattern","polygon","polyline","rect","stop","switch","symbol","svg","text","tspan","use","view"];function $a(e){return typeof e!="string"||e.includes("-")?!1:!!(Qy.indexOf(e)>-1||/[A-Z]/.test(e))}const ho={};function Yy(e){Object.assign(ho,e)}const ri=["transformPerspective","x","y","z","translateX","translateY","translateZ","scale","scaleX","scaleY","rotate","rotateX","rotateY","rotateZ","skew","skewX","skewY"],wn=new Set(ri);function Kp(e,{layout:t,layoutId:n}){return wn.has(e)||e.startsWith("origin")||(t||n!==void 0)&&(!!ho[e]||e==="opacity")}const Ve=e=>!!(e&&e.getVelocity),Xy={x:"translateX",y:"translateY",z:"translateZ",transformPerspective:"perspective"},Zy=ri.length;function qy(e,{enableHardwareAcceleration:t=!0,allowTransformNone:n=!0},r,i){let o="";for(let s=0;st=>typeof t=="string"&&t.startsWith(e),Yp=Qp("--"),Ml=Qp("var(--"),Jy=/var\s*\(\s*--[\w-]+(\s*,\s*(?:(?:[^)(]|\((?:[^)(]+|\([^)(]*\))*\))*)+)?\s*\)/g,by=(e,t)=>t&&typeof e=="number"?t.transform(e):e,Gt=(e,t,n)=>Math.min(Math.max(n,e),t),xn={test:e=>typeof e=="number",parse:parseFloat,transform:e=>e},Dr={...xn,transform:e=>Gt(0,1,e)},Ti={...xn,default:1},Lr=e=>Math.round(e*1e5)/1e5,Ho=/(-)?([\d]*\.?[\d])+/g,Xp=/(#[0-9a-f]{3,8}|(rgb|hsl)a?\((-?[\d\.]+%?[,\s]+){2}(-?[\d\.]+%?)\s*[\,\/]?\s*[\d\.]*%?\))/gi,e0=/^(#[0-9a-f]{3,8}|(rgb|hsl)a?\((-?[\d\.]+%?[,\s]+){2}(-?[\d\.]+%?)\s*[\,\/]?\s*[\d\.]*%?\))$/i;function ii(e){return typeof e=="string"}const oi=e=>({test:t=>ii(t)&&t.endsWith(e)&&t.split(" ").length===1,parse:parseFloat,transform:t=>`${t}${e}`}),Tt=oi("deg"),ut=oi("%"),A=oi("px"),t0=oi("vh"),n0=oi("vw"),Tc={...ut,parse:e=>ut.parse(e)/100,transform:e=>ut.transform(e*100)},Ec={...xn,transform:Math.round},Zp={borderWidth:A,borderTopWidth:A,borderRightWidth:A,borderBottomWidth:A,borderLeftWidth:A,borderRadius:A,radius:A,borderTopLeftRadius:A,borderTopRightRadius:A,borderBottomRightRadius:A,borderBottomLeftRadius:A,width:A,maxWidth:A,height:A,maxHeight:A,size:A,top:A,right:A,bottom:A,left:A,padding:A,paddingTop:A,paddingRight:A,paddingBottom:A,paddingLeft:A,margin:A,marginTop:A,marginRight:A,marginBottom:A,marginLeft:A,rotate:Tt,rotateX:Tt,rotateY:Tt,rotateZ:Tt,scale:Ti,scaleX:Ti,scaleY:Ti,scaleZ:Ti,skew:Tt,skewX:Tt,skewY:Tt,distance:A,translateX:A,translateY:A,translateZ:A,x:A,y:A,z:A,perspective:A,transformPerspective:A,opacity:Dr,originX:Tc,originY:Tc,originZ:A,zIndex:Ec,fillOpacity:Dr,strokeOpacity:Dr,numOctaves:Ec};function Ha(e,t,n,r){const{style:i,vars:o,transform:s,transformOrigin:l}=e;let a=!1,u=!1,c=!0;for(const f in t){const p=t[f];if(Yp(f)){o[f]=p;continue}const g=Zp[f],y=by(p,g);if(wn.has(f)){if(a=!0,s[f]=y,!c)continue;p!==(g.default||0)&&(c=!1)}else f.startsWith("origin")?(u=!0,l[f]=y):i[f]=y}if(t.transform||(a||r?i.transform=qy(e.transform,n,c,r):i.transform&&(i.transform="none")),u){const{originX:f="50%",originY:p="50%",originZ:g=0}=l;i.transformOrigin=`${f} ${p} ${g}`}}const Wa=()=>({style:{},transform:{},transformOrigin:{},vars:{}});function qp(e,t,n){for(const r in t)!Ve(t[r])&&!Kp(r,n)&&(e[r]=t[r])}function r0({transformTemplate:e},t,n){return S.useMemo(()=>{const r=Wa();return Ha(r,t,{enableHardwareAcceleration:!n},e),Object.assign({},r.vars,r.style)},[t])}function i0(e,t,n){const r=e.style||{},i={};return qp(i,r,e),Object.assign(i,r0(e,t,n)),e.transformValues?e.transformValues(i):i}function o0(e,t,n){const r={},i=i0(e,t,n);return e.drag&&e.dragListener!==!1&&(r.draggable=!1,i.userSelect=i.WebkitUserSelect=i.WebkitTouchCallout="none",i.touchAction=e.drag===!0?"none":`pan-${e.drag==="x"?"y":"x"}`),e.tabIndex===void 0&&(e.onTap||e.onTapStart||e.whileTap)&&(r.tabIndex=0),r.style=i,r}const s0=new Set(["animate","exit","variants","initial","style","values","variants","transition","transformTemplate","transformValues","custom","inherit","onLayoutAnimationStart","onLayoutAnimationComplete","onLayoutMeasure","onBeforeLayoutMeasure","onAnimationStart","onAnimationComplete","onUpdate","onDragStart","onDrag","onDragEnd","onMeasureDragConstraints","onDirectionLock","onDragTransitionEnd","_dragX","_dragY","onHoverStart","onHoverEnd","onViewportEnter","onViewportLeave","ignoreStrict","viewport"]);function mo(e){return e.startsWith("while")||e.startsWith("drag")&&e!=="draggable"||e.startsWith("layout")||e.startsWith("onTap")||e.startsWith("onPan")||s0.has(e)}let Jp=e=>!mo(e);function l0(e){e&&(Jp=t=>t.startsWith("on")?!mo(t):e(t))}try{l0(require("@emotion/is-prop-valid").default)}catch{}function a0(e,t,n){const r={};for(const i in e)i==="values"&&typeof e.values=="object"||(Jp(i)||n===!0&&mo(i)||!t&&!mo(i)||e.draggable&&i.startsWith("onDrag"))&&(r[i]=e[i]);return r}function Dc(e,t,n){return typeof e=="string"?e:A.transform(t+n*e)}function u0(e,t,n){const r=Dc(t,e.x,e.width),i=Dc(n,e.y,e.height);return`${r} ${i}`}const c0={offset:"stroke-dashoffset",array:"stroke-dasharray"},f0={offset:"strokeDashoffset",array:"strokeDasharray"};function d0(e,t,n=1,r=0,i=!0){e.pathLength=1;const o=i?c0:f0;e[o.offset]=A.transform(-r);const s=A.transform(t),l=A.transform(n);e[o.array]=`${s} ${l}`}function Ga(e,{attrX:t,attrY:n,attrScale:r,originX:i,originY:o,pathLength:s,pathSpacing:l=1,pathOffset:a=0,...u},c,f,p){if(Ha(e,u,c,p),f){e.style.viewBox&&(e.attrs.viewBox=e.style.viewBox);return}e.attrs=e.style,e.style={};const{attrs:g,style:y,dimensions:v}=e;g.transform&&(v&&(y.transform=g.transform),delete g.transform),v&&(i!==void 0||o!==void 0||y.transform)&&(y.transformOrigin=u0(v,i!==void 0?i:.5,o!==void 0?o:.5)),t!==void 0&&(g.x=t),n!==void 0&&(g.y=n),r!==void 0&&(g.scale=r),s!==void 0&&d0(g,s,l,a,!1)}const bp=()=>({...Wa(),attrs:{}}),Ka=e=>typeof e=="string"&&e.toLowerCase()==="svg";function p0(e,t,n,r){const i=S.useMemo(()=>{const o=bp();return Ga(o,t,{enableHardwareAcceleration:!1},Ka(r),e.transformTemplate),{...o.attrs,style:{...o.style}}},[t]);if(e.style){const o={};qp(o,e.style,e),i.style={...o,...i.style}}return i}function h0(e=!1){return(n,r,i,{latestValues:o},s)=>{const a=($a(n)?p0:o0)(r,o,s,n),c={...a0(r,typeof n=="string",e),...a,ref:i},{children:f}=r,p=S.useMemo(()=>Ve(f)?f.get():f,[f]);return S.createElement(n,{...c,children:p})}}const Qa=e=>e.replace(/([a-z])([A-Z])/g,"$1-$2").toLowerCase();function eh(e,{style:t,vars:n},r,i){Object.assign(e.style,t,i&&i.getProjectionStyles(r));for(const o in n)e.style.setProperty(o,n[o])}const th=new Set(["baseFrequency","diffuseConstant","kernelMatrix","kernelUnitLength","keySplines","keyTimes","limitingConeAngle","markerHeight","markerWidth","numOctaves","targetX","targetY","surfaceScale","specularConstant","specularExponent","stdDeviation","tableValues","viewBox","gradientTransform","pathLength","startOffset","textLength","lengthAdjust"]);function nh(e,t,n,r){eh(e,t,void 0,r);for(const i in t.attrs)e.setAttribute(th.has(i)?i:Qa(i),t.attrs[i])}function Ya(e,t){const{style:n}=e,r={};for(const i in n)(Ve(n[i])||t.style&&Ve(t.style[i])||Kp(i,e))&&(r[i]=n[i]);return r}function rh(e,t){const n=Ya(e,t);for(const r in e)if(Ve(e[r])||Ve(t[r])){const i=ri.indexOf(r)!==-1?"attr"+r.charAt(0).toUpperCase()+r.substring(1):r;n[i]=e[r]}return n}function Xa(e,t,n,r={},i={}){return typeof t=="function"&&(t=t(n!==void 0?n:e.custom,r,i)),typeof t=="string"&&(t=e.variants&&e.variants[t]),typeof t=="function"&&(t=t(n!==void 0?n:e.custom,r,i)),t}function ih(e){const t=S.useRef(null);return t.current===null&&(t.current=e()),t.current}const go=e=>Array.isArray(e),m0=e=>!!(e&&typeof e=="object"&&e.mix&&e.toValue),g0=e=>go(e)?e[e.length-1]||0:e;function Bi(e){const t=Ve(e)?e.get():e;return m0(t)?t.toValue():t}function y0({scrapeMotionValuesFromProps:e,createRenderState:t,onMount:n},r,i,o){const s={latestValues:v0(r,i,o,e),renderState:t()};return n&&(s.mount=l=>n(r,l,s)),s}const oh=e=>(t,n)=>{const r=S.useContext(Io),i=S.useContext(zo),o=()=>y0(e,t,r,i);return n?o():ih(o)};function v0(e,t,n,r){const i={},o=r(e,{});for(const p in o)i[p]=Bi(o[p]);let{initial:s,animate:l}=e;const a=$o(e),u=Wp(e);t&&u&&!a&&e.inherit!==!1&&(s===void 0&&(s=t.initial),l===void 0&&(l=t.animate));let c=n?n.initial===!1:!1;c=c||s===!1;const f=c?l:s;return f&&typeof f!="boolean"&&!Uo(f)&&(Array.isArray(f)?f:[f]).forEach(g=>{const y=Xa(e,g);if(!y)return;const{transitionEnd:v,transition:T,...m}=y;for(const d in m){let h=m[d];if(Array.isArray(h)){const w=c?h.length-1:0;h=h[w]}h!==null&&(i[d]=h)}for(const d in v)i[d]=v[d]}),i}const w0={useVisualState:oh({scrapeMotionValuesFromProps:rh,createRenderState:bp,onMount:(e,t,{renderState:n,latestValues:r})=>{try{n.dimensions=typeof t.getBBox=="function"?t.getBBox():t.getBoundingClientRect()}catch{n.dimensions={x:0,y:0,width:0,height:0}}Ga(n,r,{enableHardwareAcceleration:!1},Ka(t.tagName),e.transformTemplate),nh(t,n)}})},x0={useVisualState:oh({scrapeMotionValuesFromProps:Ya,createRenderState:Wa})};function S0(e,{forwardMotionProps:t=!1},n,r){return{...$a(e)?w0:x0,preloadedFeatures:n,useRender:h0(t),createVisualElement:r,Component:e}}function ht(e,t,n,r={passive:!0}){return e.addEventListener(t,n,r),()=>e.removeEventListener(t,n)}const sh=e=>e.pointerType==="mouse"?typeof e.button!="number"||e.button<=0:e.isPrimary!==!1;function Wo(e,t="page"){return{point:{x:e[t+"X"],y:e[t+"Y"]}}}const k0=e=>t=>sh(t)&&e(t,Wo(t));function gt(e,t,n,r){return ht(e,t,k0(n),r)}const P0=(e,t)=>n=>t(e(n)),Ut=(...e)=>e.reduce(P0);function lh(e){let t=null;return()=>{const n=()=>{t=null};return t===null?(t=e,n):!1}}const Lc=lh("dragHorizontal"),Mc=lh("dragVertical");function ah(e){let t=!1;if(e==="y")t=Mc();else if(e==="x")t=Lc();else{const n=Lc(),r=Mc();n&&r?t=()=>{n(),r()}:(n&&n(),r&&r())}return t}function uh(){const e=ah(!0);return e?(e(),!1):!0}class Zt{constructor(t){this.isMounted=!1,this.node=t}update(){}}function C0(e){let t=[],n=[],r=0,i=!1,o=!1;const s=new WeakSet,l={schedule:(a,u=!1,c=!1)=>{const f=c&&i,p=f?t:n;return u&&s.add(a),p.indexOf(a)===-1&&(p.push(a),f&&i&&(r=t.length)),a},cancel:a=>{const u=n.indexOf(a);u!==-1&&n.splice(u,1),s.delete(a)},process:a=>{if(i){o=!0;return}if(i=!0,[t,n]=[n,t],n.length=0,r=t.length,r)for(let u=0;u(e[t]=C0(()=>Zr=!0),e),{}),E0=e=>Gn[e].process(Y),ch=e=>{Zr=!1,Y.delta=Rl?1e3/60:Math.max(Math.min(e-Y.timestamp,T0),1),Y.timestamp=e,Y.isProcessing=!0,Go.forEach(E0),Y.isProcessing=!1,Zr&&(Rl=!1,requestAnimationFrame(ch))},D0=()=>{Zr=!0,Rl=!0,Y.isProcessing||requestAnimationFrame(ch)},Q=Go.reduce((e,t)=>{const n=Gn[t];return e[t]=(r,i=!1,o=!1)=>(Zr||D0(),n.schedule(r,i,o)),e},{});function kt(e){Go.forEach(t=>Gn[t].cancel(e))}function Rc(e,t){const n="pointer"+(t?"enter":"leave"),r="onHover"+(t?"Start":"End"),i=(o,s)=>{if(o.type==="touch"||uh())return;const l=e.getProps();e.animationState&&l.whileHover&&e.animationState.setActive("whileHover",t),l[r]&&Q.update(()=>l[r](o,s))};return gt(e.current,n,i,{passive:!e.getProps()[r]})}class L0 extends Zt{mount(){this.unmount=Ut(Rc(this.node,!0),Rc(this.node,!1))}unmount(){}}class M0 extends Zt{constructor(){super(...arguments),this.isActive=!1}onFocus(){let t=!1;try{t=this.node.current.matches(":focus-visible")}catch{t=!0}!t||!this.node.animationState||(this.node.animationState.setActive("whileFocus",!0),this.isActive=!0)}onBlur(){!this.isActive||!this.node.animationState||(this.node.animationState.setActive("whileFocus",!1),this.isActive=!1)}mount(){this.unmount=Ut(ht(this.node.current,"focus",()=>this.onFocus()),ht(this.node.current,"blur",()=>this.onBlur()))}unmount(){}}const fh=(e,t)=>t?e===t?!0:fh(e,t.parentElement):!1,oe=e=>e;function ks(e,t){if(!t)return;const n=new PointerEvent("pointer"+e);t(n,Wo(n))}class R0 extends Zt{constructor(){super(...arguments),this.removeStartListeners=oe,this.removeEndListeners=oe,this.removeAccessibleListeners=oe,this.startPointerPress=(t,n)=>{if(this.removeEndListeners(),this.isPressing)return;const r=this.node.getProps(),o=gt(window,"pointerup",(l,a)=>{if(!this.checkPressEnd())return;const{onTap:u,onTapCancel:c}=this.node.getProps();Q.update(()=>{fh(this.node.current,l.target)?u&&u(l,a):c&&c(l,a)})},{passive:!(r.onTap||r.onPointerUp)}),s=gt(window,"pointercancel",(l,a)=>this.cancelPress(l,a),{passive:!(r.onTapCancel||r.onPointerCancel)});this.removeEndListeners=Ut(o,s),this.startPress(t,n)},this.startAccessiblePress=()=>{const t=o=>{if(o.key!=="Enter"||this.isPressing)return;const s=l=>{l.key!=="Enter"||!this.checkPressEnd()||ks("up",(a,u)=>{const{onTap:c}=this.node.getProps();c&&Q.update(()=>c(a,u))})};this.removeEndListeners(),this.removeEndListeners=ht(this.node.current,"keyup",s),ks("down",(l,a)=>{this.startPress(l,a)})},n=ht(this.node.current,"keydown",t),r=()=>{this.isPressing&&ks("cancel",(o,s)=>this.cancelPress(o,s))},i=ht(this.node.current,"blur",r);this.removeAccessibleListeners=Ut(n,i)}}startPress(t,n){this.isPressing=!0;const{onTapStart:r,whileTap:i}=this.node.getProps();i&&this.node.animationState&&this.node.animationState.setActive("whileTap",!0),r&&Q.update(()=>r(t,n))}checkPressEnd(){return this.removeEndListeners(),this.isPressing=!1,this.node.getProps().whileTap&&this.node.animationState&&this.node.animationState.setActive("whileTap",!1),!uh()}cancelPress(t,n){if(!this.checkPressEnd())return;const{onTapCancel:r}=this.node.getProps();r&&Q.update(()=>r(t,n))}mount(){const t=this.node.getProps(),n=gt(this.node.current,"pointerdown",this.startPointerPress,{passive:!(t.onTapStart||t.onPointerStart)}),r=ht(this.node.current,"focus",this.startAccessiblePress);this.removeStartListeners=Ut(n,r)}unmount(){this.removeStartListeners(),this.removeEndListeners(),this.removeAccessibleListeners()}}const Vl=new WeakMap,Ps=new WeakMap,V0=e=>{const t=Vl.get(e.target);t&&t(e)},A0=e=>{e.forEach(V0)};function _0({root:e,...t}){const n=e||document;Ps.has(n)||Ps.set(n,{});const r=Ps.get(n),i=JSON.stringify(t);return r[i]||(r[i]=new IntersectionObserver(A0,{root:e,...t})),r[i]}function N0(e,t,n){const r=_0(t);return Vl.set(e,n),r.observe(e),()=>{Vl.delete(e),r.unobserve(e)}}const O0={some:0,all:1};class j0 extends Zt{constructor(){super(...arguments),this.hasEnteredView=!1,this.isInView=!1}startObserver(){this.unmount();const{viewport:t={}}=this.node.getProps(),{root:n,margin:r,amount:i="some",once:o}=t,s={root:n?n.current:void 0,rootMargin:r,threshold:typeof i=="number"?i:O0[i]},l=a=>{const{isIntersecting:u}=a;if(this.isInView===u||(this.isInView=u,o&&!u&&this.hasEnteredView))return;u&&(this.hasEnteredView=!0),this.node.animationState&&this.node.animationState.setActive("whileInView",u);const{onViewportEnter:c,onViewportLeave:f}=this.node.getProps(),p=u?c:f;p&&p(a)};return N0(this.node.current,s,l)}mount(){this.startObserver()}update(){if(typeof IntersectionObserver>"u")return;const{props:t,prevProps:n}=this.node;["amount","margin","root"].some(F0(t,n))&&this.startObserver()}unmount(){}}function F0({viewport:e={}},{viewport:t={}}={}){return n=>e[n]!==t[n]}const I0={inView:{Feature:j0},tap:{Feature:R0},focus:{Feature:M0},hover:{Feature:L0}};function dh(e,t){if(!Array.isArray(t))return!1;const n=t.length;if(n!==e.length)return!1;for(let r=0;rt[r]=n.get()),t}function B0(e){const t={};return e.values.forEach((n,r)=>t[r]=n.getVelocity()),t}function Ko(e,t,n){const r=e.getProps();return Xa(r,t,n!==void 0?n:r.custom,z0(e),B0(e))}const U0="framerAppearId",$0="data-"+Qa(U0);let H0=oe,Za=oe;const $t=e=>e*1e3,yt=e=>e/1e3,W0={current:!1},ph=e=>Array.isArray(e)&&typeof e[0]=="number";function hh(e){return!!(!e||typeof e=="string"&&mh[e]||ph(e)||Array.isArray(e)&&e.every(hh))}const yr=([e,t,n,r])=>`cubic-bezier(${e}, ${t}, ${n}, ${r})`,mh={linear:"linear",ease:"ease",easeIn:"ease-in",easeOut:"ease-out",easeInOut:"ease-in-out",circIn:yr([0,.65,.55,1]),circOut:yr([.55,0,1,.45]),backIn:yr([.31,.01,.66,-.59]),backOut:yr([.33,1.53,.69,.99])};function gh(e){if(e)return ph(e)?yr(e):Array.isArray(e)?e.map(gh):mh[e]}function G0(e,t,n,{delay:r=0,duration:i,repeat:o=0,repeatType:s="loop",ease:l,times:a}={}){const u={[t]:n};a&&(u.offset=a);const c=gh(l);return Array.isArray(c)&&(u.easing=c),e.animate(u,{delay:r,duration:i,easing:Array.isArray(c)?"linear":c,fill:"both",iterations:o+1,direction:s==="reverse"?"alternate":"normal"})}const Vc={waapi:()=>Object.hasOwnProperty.call(Element.prototype,"animate")},Cs={},yh={};for(const e in Vc)yh[e]=()=>(Cs[e]===void 0&&(Cs[e]=Vc[e]()),Cs[e]);function K0(e,{repeat:t,repeatType:n="loop"}){const r=t&&n!=="loop"&&t%2===1?0:e.length-1;return e[r]}const vh=(e,t,n)=>(((1-3*n+3*t)*e+(3*n-6*t))*e+3*t)*e,Q0=1e-7,Y0=12;function X0(e,t,n,r,i){let o,s,l=0;do s=t+(n-t)/2,o=vh(s,r,i)-e,o>0?n=s:t=s;while(Math.abs(o)>Q0&&++lX0(o,0,1,e,n);return o=>o===0||o===1?o:vh(i(o),t,r)}const Z0=si(.42,0,1,1),q0=si(0,0,.58,1),wh=si(.42,0,.58,1),J0=e=>Array.isArray(e)&&typeof e[0]!="number",xh=e=>t=>t<=.5?e(2*t)/2:(2-e(2*(1-t)))/2,Sh=e=>t=>1-e(1-t),kh=e=>1-Math.sin(Math.acos(e)),qa=Sh(kh),b0=xh(qa),Ph=si(.33,1.53,.69,.99),Ja=Sh(Ph),ev=xh(Ja),tv=e=>(e*=2)<1?.5*Ja(e):.5*(2-Math.pow(2,-10*(e-1))),nv={linear:oe,easeIn:Z0,easeInOut:wh,easeOut:q0,circIn:kh,circInOut:b0,circOut:qa,backIn:Ja,backInOut:ev,backOut:Ph,anticipate:tv},Ac=e=>{if(Array.isArray(e)){Za(e.length===4);const[t,n,r,i]=e;return si(t,n,r,i)}else if(typeof e=="string")return nv[e];return e},ba=(e,t)=>n=>!!(ii(n)&&e0.test(n)&&n.startsWith(e)||t&&Object.prototype.hasOwnProperty.call(n,t)),Ch=(e,t,n)=>r=>{if(!ii(r))return r;const[i,o,s,l]=r.match(Ho);return{[e]:parseFloat(i),[t]:parseFloat(o),[n]:parseFloat(s),alpha:l!==void 0?parseFloat(l):1}},rv=e=>Gt(0,255,e),Ts={...xn,transform:e=>Math.round(rv(e))},un={test:ba("rgb","red"),parse:Ch("red","green","blue"),transform:({red:e,green:t,blue:n,alpha:r=1})=>"rgba("+Ts.transform(e)+", "+Ts.transform(t)+", "+Ts.transform(n)+", "+Lr(Dr.transform(r))+")"};function iv(e){let t="",n="",r="",i="";return e.length>5?(t=e.substring(1,3),n=e.substring(3,5),r=e.substring(5,7),i=e.substring(7,9)):(t=e.substring(1,2),n=e.substring(2,3),r=e.substring(3,4),i=e.substring(4,5),t+=t,n+=n,r+=r,i+=i),{red:parseInt(t,16),green:parseInt(n,16),blue:parseInt(r,16),alpha:i?parseInt(i,16)/255:1}}const Al={test:ba("#"),parse:iv,transform:un.transform},jn={test:ba("hsl","hue"),parse:Ch("hue","saturation","lightness"),transform:({hue:e,saturation:t,lightness:n,alpha:r=1})=>"hsla("+Math.round(e)+", "+ut.transform(Lr(t))+", "+ut.transform(Lr(n))+", "+Lr(Dr.transform(r))+")"},xe={test:e=>un.test(e)||Al.test(e)||jn.test(e),parse:e=>un.test(e)?un.parse(e):jn.test(e)?jn.parse(e):Al.parse(e),transform:e=>ii(e)?e:e.hasOwnProperty("red")?un.transform(e):jn.transform(e)},Z=(e,t,n)=>-n*e+n*t+e;function Es(e,t,n){return n<0&&(n+=1),n>1&&(n-=1),n<1/6?e+(t-e)*6*n:n<1/2?t:n<2/3?e+(t-e)*(2/3-n)*6:e}function ov({hue:e,saturation:t,lightness:n,alpha:r}){e/=360,t/=100,n/=100;let i=0,o=0,s=0;if(!t)i=o=s=n;else{const l=n<.5?n*(1+t):n+t-n*t,a=2*n-l;i=Es(a,l,e+1/3),o=Es(a,l,e),s=Es(a,l,e-1/3)}return{red:Math.round(i*255),green:Math.round(o*255),blue:Math.round(s*255),alpha:r}}const Ds=(e,t,n)=>{const r=e*e;return Math.sqrt(Math.max(0,n*(t*t-r)+r))},sv=[Al,un,jn],lv=e=>sv.find(t=>t.test(e));function _c(e){const t=lv(e);let n=t.parse(e);return t===jn&&(n=ov(n)),n}const Th=(e,t)=>{const n=_c(e),r=_c(t),i={...n};return o=>(i.red=Ds(n.red,r.red,o),i.green=Ds(n.green,r.green,o),i.blue=Ds(n.blue,r.blue,o),i.alpha=Z(n.alpha,r.alpha,o),un.transform(i))};function av(e){var t,n;return isNaN(e)&&ii(e)&&(((t=e.match(Ho))===null||t===void 0?void 0:t.length)||0)+(((n=e.match(Xp))===null||n===void 0?void 0:n.length)||0)>0}const Eh={regex:Jy,countKey:"Vars",token:"${v}",parse:oe},Dh={regex:Xp,countKey:"Colors",token:"${c}",parse:xe.parse},Lh={regex:Ho,countKey:"Numbers",token:"${n}",parse:xn.parse};function Ls(e,{regex:t,countKey:n,token:r,parse:i}){const o=e.tokenised.match(t);o&&(e["num"+n]=o.length,e.tokenised=e.tokenised.replace(t,r),e.values.push(...o.map(i)))}function yo(e){const t=e.toString(),n={value:t,tokenised:t,values:[],numVars:0,numColors:0,numNumbers:0};return n.value.includes("var(--")&&Ls(n,Eh),Ls(n,Dh),Ls(n,Lh),n}function Mh(e){return yo(e).values}function Rh(e){const{values:t,numColors:n,numVars:r,tokenised:i}=yo(e),o=t.length;return s=>{let l=i;for(let a=0;atypeof e=="number"?0:e;function cv(e){const t=Mh(e);return Rh(e)(t.map(uv))}const Kt={test:av,parse:Mh,createTransformer:Rh,getAnimatableNone:cv},Vh=(e,t)=>n=>`${n>0?t:e}`;function Ah(e,t){return typeof e=="number"?n=>Z(e,t,n):xe.test(e)?Th(e,t):e.startsWith("var(")?Vh(e,t):Nh(e,t)}const _h=(e,t)=>{const n=[...e],r=n.length,i=e.map((o,s)=>Ah(o,t[s]));return o=>{for(let s=0;s{const n={...e,...t},r={};for(const i in n)e[i]!==void 0&&t[i]!==void 0&&(r[i]=Ah(e[i],t[i]));return i=>{for(const o in r)n[o]=r[o](i);return n}},Nh=(e,t)=>{const n=Kt.createTransformer(t),r=yo(e),i=yo(t);return r.numVars===i.numVars&&r.numColors===i.numColors&&r.numNumbers>=i.numNumbers?Ut(_h(r.values,i.values),n):Vh(e,t)},qr=(e,t,n)=>{const r=t-e;return r===0?1:(n-e)/r},Nc=(e,t)=>n=>Z(e,t,n);function dv(e){return typeof e=="number"?Nc:typeof e=="string"?xe.test(e)?Th:Nh:Array.isArray(e)?_h:typeof e=="object"?fv:Nc}function pv(e,t,n){const r=[],i=n||dv(e[0]),o=e.length-1;for(let s=0;st[0];e[0]>e[o-1]&&(e=[...e].reverse(),t=[...t].reverse());const s=pv(t,r,i),l=s.length,a=u=>{let c=0;if(l>1)for(;ca(Gt(e[0],e[o-1],u)):a}function hv(e,t){const n=e[e.length-1];for(let r=1;r<=t;r++){const i=qr(0,t,r);e.push(Z(n,1,i))}}function mv(e){const t=[0];return hv(t,e.length-1),t}function gv(e,t){return e.map(n=>n*t)}function yv(e,t){return e.map(()=>t||wh).splice(0,e.length-1)}function vo({duration:e=300,keyframes:t,times:n,ease:r="easeInOut"}){const i=J0(r)?r.map(Ac):Ac(r),o={done:!1,value:t[0]},s=gv(n&&n.length===t.length?n:mv(t),e),l=Oh(s,t,{ease:Array.isArray(i)?i:yv(t,i)});return{calculatedDuration:e,next:a=>(o.value=l(a),o.done=a>=e,o)}}function jh(e,t){return t?e*(1e3/t):0}const vv=5;function Fh(e,t,n){const r=Math.max(t-vv,0);return jh(n-e(r),t-r)}const Ms=.001,wv=.01,Oc=10,xv=.05,Sv=1;function kv({duration:e=800,bounce:t=.25,velocity:n=0,mass:r=1}){let i,o;H0(e<=$t(Oc));let s=1-t;s=Gt(xv,Sv,s),e=Gt(wv,Oc,yt(e)),s<1?(i=u=>{const c=u*s,f=c*e,p=c-n,g=_l(u,s),y=Math.exp(-f);return Ms-p/g*y},o=u=>{const f=u*s*e,p=f*n+n,g=Math.pow(s,2)*Math.pow(u,2)*e,y=Math.exp(-f),v=_l(Math.pow(u,2),s);return(-i(u)+Ms>0?-1:1)*((p-g)*y)/v}):(i=u=>{const c=Math.exp(-u*e),f=(u-n)*e+1;return-Ms+c*f},o=u=>{const c=Math.exp(-u*e),f=(n-u)*(e*e);return c*f});const l=5/e,a=Cv(i,o,l);if(e=$t(e),isNaN(a))return{stiffness:100,damping:10,duration:e};{const u=Math.pow(a,2)*r;return{stiffness:u,damping:s*2*Math.sqrt(r*u),duration:e}}}const Pv=12;function Cv(e,t,n){let r=n;for(let i=1;ie[n]!==void 0)}function Dv(e){let t={velocity:0,stiffness:100,damping:10,mass:1,isResolvedFromDuration:!1,...e};if(!jc(e,Ev)&&jc(e,Tv)){const n=kv(e);t={...t,...n,velocity:0,mass:1},t.isResolvedFromDuration=!0}return t}function Ih({keyframes:e,restDelta:t,restSpeed:n,...r}){const i=e[0],o=e[e.length-1],s={done:!1,value:i},{stiffness:l,damping:a,mass:u,velocity:c,duration:f,isResolvedFromDuration:p}=Dv(r),g=c?-yt(c):0,y=a/(2*Math.sqrt(l*u)),v=o-i,T=yt(Math.sqrt(l/u)),m=Math.abs(v)<5;n||(n=m?.01:2),t||(t=m?.005:.5);let d;if(y<1){const h=_l(T,y);d=w=>{const x=Math.exp(-y*T*w);return o-x*((g+y*T*v)/h*Math.sin(h*w)+v*Math.cos(h*w))}}else if(y===1)d=h=>o-Math.exp(-T*h)*(v+(g+T*v)*h);else{const h=T*Math.sqrt(y*y-1);d=w=>{const x=Math.exp(-y*T*w),C=Math.min(h*w,300);return o-x*((g+y*T*v)*Math.sinh(C)+h*v*Math.cosh(C))/h}}return{calculatedDuration:p&&f||null,next:h=>{const w=d(h);if(p)s.done=h>=f;else{let x=g;h!==0&&(y<1?x=Fh(d,h,w):x=0);const C=Math.abs(x)<=n,E=Math.abs(o-w)<=t;s.done=C&&E}return s.value=s.done?o:w,s}}}function Fc({keyframes:e,velocity:t=0,power:n=.8,timeConstant:r=325,bounceDamping:i=10,bounceStiffness:o=500,modifyTarget:s,min:l,max:a,restDelta:u=.5,restSpeed:c}){const f=e[0],p={done:!1,value:f},g=k=>l!==void 0&&ka,y=k=>l===void 0?a:a===void 0||Math.abs(l-k)-v*Math.exp(-k/r),h=k=>m+d(k),w=k=>{const V=d(k),R=h(k);p.done=Math.abs(V)<=u,p.value=p.done?m:R};let x,C;const E=k=>{g(p.value)&&(x=k,C=Ih({keyframes:[p.value,y(p.value)],velocity:Fh(h,k,p.value),damping:i,stiffness:o,restDelta:u,restSpeed:c}))};return E(0),{calculatedDuration:null,next:k=>{let V=!1;return!C&&x===void 0&&(V=!0,w(k),E(k)),x!==void 0&&k>x?C.next(k-x):(!V&&w(k),p)}}}const Lv=e=>{const t=({timestamp:n})=>e(n);return{start:()=>Q.update(t,!0),stop:()=>kt(t),now:()=>Y.isProcessing?Y.timestamp:performance.now()}},Ic=2e4;function zc(e){let t=0;const n=50;let r=e.next(t);for(;!r.done&&t=Ic?1/0:t}const Mv={decay:Fc,inertia:Fc,tween:vo,keyframes:vo,spring:Ih};function wo({autoplay:e=!0,delay:t=0,driver:n=Lv,keyframes:r,type:i="keyframes",repeat:o=0,repeatDelay:s=0,repeatType:l="loop",onPlay:a,onStop:u,onComplete:c,onUpdate:f,...p}){let g=1,y=!1,v,T;const m=()=>{v&&v(),T=new Promise(D=>{v=D})};m();let d;const h=Mv[i]||vo;let w;h!==vo&&typeof r[0]!="number"&&(w=Oh([0,100],r,{clamp:!1}),r=[0,100]);const x=h({...p,keyframes:r});let C;l==="mirror"&&(C=h({...p,keyframes:[...r].reverse(),velocity:-(p.velocity||0)}));let E="idle",k=null,V=null,R=null;x.calculatedDuration===null&&o&&(x.calculatedDuration=zc(x));const{calculatedDuration:j}=x;let de=1/0,pe=1/0;j!==null&&(de=j+s,pe=de*(o+1)-s);let U=0;const $=D=>{if(V===null)return;g>0&&(V=Math.min(V,D)),g<0&&(V=Math.min(D-pe/g,V)),k!==null?U=k:U=Math.round(D-V)*g;const F=U-t*(g>=0?1:-1),Be=g>=0?F<0:F>pe;U=Math.max(F,0),E==="finished"&&k===null&&(U=pe);let ee=U,qt=x;if(o){const Jt=U/de;let li=Math.floor(Jt),bt=Jt%1;!bt&&Jt>=1&&(bt=1),bt===1&&li--,li=Math.min(li,o+1);const su=!!(li%2);su&&(l==="reverse"?(bt=1-bt,s&&(bt-=s/de)):l==="mirror"&&(qt=C));let lu=Gt(0,1,bt);U>pe&&(lu=l==="reverse"&&su?1:0),ee=lu*de}const me=Be?{done:!1,value:r[0]}:qt.next(ee);w&&(me.value=w(me.value));let{done:Ue}=me;!Be&&j!==null&&(Ue=g>=0?U>=pe:U<=0);const Xe=k===null&&(E==="finished"||E==="running"&&Ue);return f&&f(me.value),Xe&&L(),me},he=()=>{d&&d.stop(),d=void 0},Ae=()=>{E="idle",he(),m(),V=R=null},L=()=>{E="finished",c&&c(),he(),m()},_=()=>{if(y)return;d||(d=n($));const D=d.now();a&&a(),k!==null?V=D-k:(!V||E==="finished")&&(V=D),R=V,k=null,E="running",d.start()};e&&_();const N={then(D,F){return T.then(D,F)},get time(){return yt(U)},set time(D){D=$t(D),U=D,k!==null||!d||g===0?k=D:V=d.now()-D/g},get duration(){const D=x.calculatedDuration===null?zc(x):x.calculatedDuration;return yt(D)},get speed(){return g},set speed(D){D===g||!d||(g=D,N.time=yt(U))},get state(){return E},play:_,pause:()=>{E="paused",k=U},stop:()=>{y=!0,E!=="idle"&&(E="idle",u&&u(),Ae())},cancel:()=>{R!==null&&$(R),Ae()},complete:()=>{E="finished"},sample:D=>(V=0,$(D))};return N}const Rv=new Set(["opacity","clipPath","filter","transform","backgroundColor"]),Ei=10,Vv=2e4,Av=(e,t)=>t.type==="spring"||e==="backgroundColor"||!hh(t.ease);function _v(e,t,{onUpdate:n,onComplete:r,...i}){if(!(yh.waapi()&&Rv.has(t)&&!i.repeatDelay&&i.repeatType!=="mirror"&&i.damping!==0&&i.type!=="inertia"))return!1;let s=!1,l,a;const u=()=>{a=new Promise(m=>{l=m})};u();let{keyframes:c,duration:f=300,ease:p,times:g}=i;if(Av(t,i)){const m=wo({...i,repeat:0,delay:0});let d={done:!1,value:c[0]};const h=[];let w=0;for(;!d.done&&wy.cancel(),T=()=>{Q.update(v),l(),u()};return y.onfinish=()=>{e.set(K0(c,i)),r&&r(),T()},{then(m,d){return a.then(m,d)},get time(){return yt(y.currentTime||0)},set time(m){y.currentTime=$t(m)},get speed(){return y.playbackRate},set speed(m){y.playbackRate=m},get duration(){return yt(f)},play:()=>{s||(y.play(),kt(v))},pause:()=>y.pause(),stop:()=>{if(s=!0,y.playState==="idle")return;const{currentTime:m}=y;if(m){const d=wo({...i,autoplay:!1});e.setWithVelocity(d.sample(m-Ei).value,d.sample(m).value,Ei)}T()},complete:()=>y.finish(),cancel:T}}function Nv({keyframes:e,delay:t,onUpdate:n,onComplete:r}){const i=()=>(n&&n(e[e.length-1]),r&&r(),{time:0,speed:1,duration:0,play:oe,pause:oe,stop:oe,then:o=>(o(),Promise.resolve()),cancel:oe,complete:oe});return t?wo({keyframes:[0,1],duration:0,delay:t,onComplete:i}):i()}const Ov={type:"spring",stiffness:500,damping:25,restSpeed:10},jv=e=>({type:"spring",stiffness:550,damping:e===0?2*Math.sqrt(550):30,restSpeed:10}),Fv={type:"keyframes",duration:.8},Iv={type:"keyframes",ease:[.25,.1,.35,1],duration:.3},zv=(e,{keyframes:t})=>t.length>2?Fv:wn.has(e)?e.startsWith("scale")?jv(t[1]):Ov:Iv,Nl=(e,t)=>e==="zIndex"?!1:!!(typeof t=="number"||Array.isArray(t)||typeof t=="string"&&(Kt.test(t)||t==="0")&&!t.startsWith("url(")),Bv=new Set(["brightness","contrast","saturate","opacity"]);function Uv(e){const[t,n]=e.slice(0,-1).split("(");if(t==="drop-shadow")return e;const[r]=n.match(Ho)||[];if(!r)return e;const i=n.replace(r,"");let o=Bv.has(t)?1:0;return r!==n&&(o*=100),t+"("+o+i+")"}const $v=/([a-z-]*)\(.*?\)/g,Ol={...Kt,getAnimatableNone:e=>{const t=e.match($v);return t?t.map(Uv).join(" "):e}},Hv={...Zp,color:xe,backgroundColor:xe,outlineColor:xe,fill:xe,stroke:xe,borderColor:xe,borderTopColor:xe,borderRightColor:xe,borderBottomColor:xe,borderLeftColor:xe,filter:Ol,WebkitFilter:Ol},eu=e=>Hv[e];function zh(e,t){let n=eu(e);return n!==Ol&&(n=Kt),n.getAnimatableNone?n.getAnimatableNone(t):void 0}const Bh=e=>/^0[^.\s]+$/.test(e);function Wv(e){if(typeof e=="number")return e===0;if(e!==null)return e==="none"||e==="0"||Bh(e)}function Gv(e,t,n,r){const i=Nl(t,n);let o;Array.isArray(n)?o=[...n]:o=[null,n];const s=r.from!==void 0?r.from:e.get();let l;const a=[];for(let u=0;ui=>{const o=Uh(r,e)||{},s=o.delay||r.delay||0;let{elapsed:l=0}=r;l=l-$t(s);const a=Gv(t,e,n,o),u=a[0],c=a[a.length-1],f=Nl(e,u),p=Nl(e,c);let g={keyframes:a,velocity:t.getVelocity(),ease:"easeOut",...o,delay:-l,onUpdate:y=>{t.set(y),o.onUpdate&&o.onUpdate(y)},onComplete:()=>{i(),o.onComplete&&o.onComplete()}};if(Kv(o)||(g={...g,...zv(e,g)}),g.duration&&(g.duration=$t(g.duration)),g.repeatDelay&&(g.repeatDelay=$t(g.repeatDelay)),!f||!p||W0.current||o.type===!1)return Nv(g);if(t.owner&&t.owner.current instanceof HTMLElement&&!t.owner.getProps().onUpdate){const y=_v(t,e,g);if(y)return y}return wo(g)};function xo(e){return!!(Ve(e)&&e.add)}const Qv=e=>/^\-?\d*\.?\d+$/.test(e);function nu(e,t){e.indexOf(t)===-1&&e.push(t)}function ru(e,t){const n=e.indexOf(t);n>-1&&e.splice(n,1)}class iu{constructor(){this.subscriptions=[]}add(t){return nu(this.subscriptions,t),()=>ru(this.subscriptions,t)}notify(t,n,r){const i=this.subscriptions.length;if(i)if(i===1)this.subscriptions[0](t,n,r);else for(let o=0;o!isNaN(parseFloat(e));class Xv{constructor(t,n={}){this.version="10.12.18",this.timeDelta=0,this.lastUpdated=0,this.canTrackVelocity=!1,this.events={},this.updateAndNotify=(r,i=!0)=>{this.prev=this.current,this.current=r;const{delta:o,timestamp:s}=Y;this.lastUpdated!==s&&(this.timeDelta=o,this.lastUpdated=s,Q.postRender(this.scheduleVelocityCheck)),this.prev!==this.current&&this.events.change&&this.events.change.notify(this.current),this.events.velocityChange&&this.events.velocityChange.notify(this.getVelocity()),i&&this.events.renderRequest&&this.events.renderRequest.notify(this.current)},this.scheduleVelocityCheck=()=>Q.postRender(this.velocityCheck),this.velocityCheck=({timestamp:r})=>{r!==this.lastUpdated&&(this.prev=this.current,this.events.velocityChange&&this.events.velocityChange.notify(this.getVelocity()))},this.hasAnimated=!1,this.prev=this.current=t,this.canTrackVelocity=Yv(this.current),this.owner=n.owner}onChange(t){return this.on("change",t)}on(t,n){this.events[t]||(this.events[t]=new iu);const r=this.events[t].add(n);return t==="change"?()=>{r(),Q.read(()=>{this.events.change.getSize()||this.stop()})}:r}clearListeners(){for(const t in this.events)this.events[t].clear()}attach(t,n){this.passiveEffect=t,this.stopPassiveEffect=n}set(t,n=!0){!n||!this.passiveEffect?this.updateAndNotify(t,n):this.passiveEffect(t,this.updateAndNotify)}setWithVelocity(t,n,r){this.set(n),this.prev=t,this.timeDelta=r}jump(t){this.updateAndNotify(t),this.prev=t,this.stop(),this.stopPassiveEffect&&this.stopPassiveEffect()}get(){return this.current}getPrevious(){return this.prev}getVelocity(){return this.canTrackVelocity?jh(parseFloat(this.current)-parseFloat(this.prev),this.timeDelta):0}start(t){return this.stop(),new Promise(n=>{this.hasAnimated=!0,this.animation=t(n),this.events.animationStart&&this.events.animationStart.notify()}).then(()=>{this.events.animationComplete&&this.events.animationComplete.notify(),this.clearAnimation()})}stop(){this.animation&&(this.animation.stop(),this.events.animationCancel&&this.events.animationCancel.notify()),this.clearAnimation()}isAnimating(){return!!this.animation}clearAnimation(){delete this.animation}destroy(){this.clearListeners(),this.stop(),this.stopPassiveEffect&&this.stopPassiveEffect()}}function bn(e,t){return new Xv(e,t)}const $h=e=>t=>t.test(e),Zv={test:e=>e==="auto",parse:e=>e},Hh=[xn,A,ut,Tt,n0,t0,Zv],fr=e=>Hh.find($h(e)),qv=[...Hh,xe,Kt],Jv=e=>qv.find($h(e));function bv(e,t,n){e.hasValue(t)?e.getValue(t).set(n):e.addValue(t,bn(n))}function e1(e,t){const n=Ko(e,t);let{transitionEnd:r={},transition:i={},...o}=n?e.makeTargetAnimatable(n,!1):{};o={...o,...r};for(const s in o){const l=g0(o[s]);bv(e,s,l)}}function t1(e,t,n){var r,i;const o=Object.keys(t).filter(l=>!e.hasValue(l)),s=o.length;if(s)for(let l=0;la.remove(f))),u.push(v)}return s&&Promise.all(u).then(()=>{s&&e1(e,s)}),u}function jl(e,t,n={}){const r=Ko(e,t,n.custom);let{transition:i=e.getDefaultTransition()||{}}=r||{};n.transitionOverride&&(i=n.transitionOverride);const o=r?()=>Promise.all(Wh(e,r,n)):()=>Promise.resolve(),s=e.variantChildren&&e.variantChildren.size?(a=0)=>{const{delayChildren:u=0,staggerChildren:c,staggerDirection:f}=i;return o1(e,t,u+a,c,f,n)}:()=>Promise.resolve(),{when:l}=i;if(l){const[a,u]=l==="beforeChildren"?[o,s]:[s,o];return a().then(()=>u())}else return Promise.all([o(),s(n.delay)])}function o1(e,t,n=0,r=0,i=1,o){const s=[],l=(e.variantChildren.size-1)*r,a=i===1?(u=0)=>u*r:(u=0)=>l-u*r;return Array.from(e.variantChildren).sort(s1).forEach((u,c)=>{u.notify("AnimationStart",t),s.push(jl(u,t,{...o,delay:n+a(c)}).then(()=>u.notify("AnimationComplete",t)))}),Promise.all(s)}function s1(e,t){return e.sortNodePosition(t)}function l1(e,t,n={}){e.notify("AnimationStart",t);let r;if(Array.isArray(t)){const i=t.map(o=>jl(e,o,n));r=Promise.all(i)}else if(typeof t=="string")r=jl(e,t,n);else{const i=typeof t=="function"?Ko(e,t,n.custom):t;r=Promise.all(Wh(e,i,n))}return r.then(()=>e.notify("AnimationComplete",t))}const a1=[...za].reverse(),u1=za.length;function c1(e){return t=>Promise.all(t.map(({animation:n,options:r})=>l1(e,n,r)))}function f1(e){let t=c1(e);const n=p1();let r=!0;const i=(a,u)=>{const c=Ko(e,u);if(c){const{transition:f,transitionEnd:p,...g}=c;a={...a,...g,...p}}return a};function o(a){t=a(e)}function s(a,u){const c=e.getProps(),f=e.getVariantContext(!0)||{},p=[],g=new Set;let y={},v=1/0;for(let m=0;mv&&x;const R=Array.isArray(w)?w:[w];let j=R.reduce(i,{});C===!1&&(j={});const{prevResolvedValues:de={}}=h,pe={...de,...j},U=$=>{V=!0,g.delete($),h.needsAnimating[$]=!0};for(const $ in pe){const he=j[$],Ae=de[$];y.hasOwnProperty($)||(he!==Ae?go(he)&&go(Ae)?!dh(he,Ae)||k?U($):h.protectedKeys[$]=!0:he!==void 0?U($):g.add($):he!==void 0&&g.has($)?U($):h.protectedKeys[$]=!0)}h.prevProp=w,h.prevResolvedValues=j,h.isActive&&(y={...y,...j}),r&&e.blockInitialAnimation&&(V=!1),V&&!E&&p.push(...R.map($=>({animation:$,options:{type:d,...a}})))}if(g.size){const m={};g.forEach(d=>{const h=e.getBaseTarget(d);h!==void 0&&(m[d]=h)}),p.push({animation:m})}let T=!!p.length;return r&&c.initial===!1&&!e.manuallyAnimateOnMount&&(T=!1),r=!1,T?t(p):Promise.resolve()}function l(a,u,c){var f;if(n[a].isActive===u)return Promise.resolve();(f=e.variantChildren)===null||f===void 0||f.forEach(g=>{var y;return(y=g.animationState)===null||y===void 0?void 0:y.setActive(a,u)}),n[a].isActive=u;const p=s(c,a);for(const g in n)n[g].protectedKeys={};return p}return{animateChanges:s,setActive:l,setAnimateFunction:o,getState:()=>n}}function d1(e,t){return typeof t=="string"?t!==e:Array.isArray(t)?!dh(t,e):!1}function en(e=!1){return{isActive:e,protectedKeys:{},needsAnimating:{},prevResolvedValues:{}}}function p1(){return{animate:en(!0),whileInView:en(),whileHover:en(),whileTap:en(),whileDrag:en(),whileFocus:en(),exit:en()}}class h1 extends Zt{constructor(t){super(t),t.animationState||(t.animationState=f1(t))}updateAnimationControlsSubscription(){const{animate:t}=this.node.getProps();this.unmount(),Uo(t)&&(this.unmount=t.subscribe(this.node))}mount(){this.updateAnimationControlsSubscription()}update(){const{animate:t}=this.node.getProps(),{animate:n}=this.node.prevProps||{};t!==n&&this.updateAnimationControlsSubscription()}unmount(){}}let m1=0;class g1 extends Zt{constructor(){super(...arguments),this.id=m1++}update(){if(!this.node.presenceContext)return;const{isPresent:t,onExitComplete:n,custom:r}=this.node.presenceContext,{isPresent:i}=this.node.prevPresenceContext||{};if(!this.node.animationState||t===i)return;const o=this.node.animationState.setActive("exit",!t,{custom:r??this.node.getProps().custom});n&&!t&&o.then(()=>n(this.id))}mount(){const{register:t}=this.node.presenceContext||{};t&&(this.unmount=t(this.id))}unmount(){}}const y1={animation:{Feature:h1},exit:{Feature:g1}},Bc=(e,t)=>Math.abs(e-t);function v1(e,t){const n=Bc(e.x,t.x),r=Bc(e.y,t.y);return Math.sqrt(n**2+r**2)}class Gh{constructor(t,n,{transformPagePoint:r}={}){if(this.startEvent=null,this.lastMoveEvent=null,this.lastMoveEventInfo=null,this.handlers={},this.updatePoint=()=>{if(!(this.lastMoveEvent&&this.lastMoveEventInfo))return;const u=Vs(this.lastMoveEventInfo,this.history),c=this.startEvent!==null,f=v1(u.offset,{x:0,y:0})>=3;if(!c&&!f)return;const{point:p}=u,{timestamp:g}=Y;this.history.push({...p,timestamp:g});const{onStart:y,onMove:v}=this.handlers;c||(y&&y(this.lastMoveEvent,u),this.startEvent=this.lastMoveEvent),v&&v(this.lastMoveEvent,u)},this.handlePointerMove=(u,c)=>{this.lastMoveEvent=u,this.lastMoveEventInfo=Rs(c,this.transformPagePoint),Q.update(this.updatePoint,!0)},this.handlePointerUp=(u,c)=>{if(this.end(),!(this.lastMoveEvent&&this.lastMoveEventInfo))return;const{onEnd:f,onSessionEnd:p}=this.handlers,g=Vs(u.type==="pointercancel"?this.lastMoveEventInfo:Rs(c,this.transformPagePoint),this.history);this.startEvent&&f&&f(u,g),p&&p(u,g)},!sh(t))return;this.handlers=n,this.transformPagePoint=r;const i=Wo(t),o=Rs(i,this.transformPagePoint),{point:s}=o,{timestamp:l}=Y;this.history=[{...s,timestamp:l}];const{onSessionStart:a}=n;a&&a(t,Vs(o,this.history)),this.removeListeners=Ut(gt(window,"pointermove",this.handlePointerMove),gt(window,"pointerup",this.handlePointerUp),gt(window,"pointercancel",this.handlePointerUp))}updateHandlers(t){this.handlers=t}end(){this.removeListeners&&this.removeListeners(),kt(this.updatePoint)}}function Rs(e,t){return t?{point:t(e.point)}:e}function Uc(e,t){return{x:e.x-t.x,y:e.y-t.y}}function Vs({point:e},t){return{point:e,delta:Uc(e,Kh(t)),offset:Uc(e,w1(t)),velocity:x1(t,.1)}}function w1(e){return e[0]}function Kh(e){return e[e.length-1]}function x1(e,t){if(e.length<2)return{x:0,y:0};let n=e.length-1,r=null;const i=Kh(e);for(;n>=0&&(r=e[n],!(i.timestamp-r.timestamp>$t(t)));)n--;if(!r)return{x:0,y:0};const o=yt(i.timestamp-r.timestamp);if(o===0)return{x:0,y:0};const s={x:(i.x-r.x)/o,y:(i.y-r.y)/o};return s.x===1/0&&(s.x=0),s.y===1/0&&(s.y=0),s}function Fe(e){return e.max-e.min}function Fl(e,t=0,n=.01){return Math.abs(e-t)<=n}function $c(e,t,n,r=.5){e.origin=r,e.originPoint=Z(t.min,t.max,e.origin),e.scale=Fe(n)/Fe(t),(Fl(e.scale,1,1e-4)||isNaN(e.scale))&&(e.scale=1),e.translate=Z(n.min,n.max,e.origin)-e.originPoint,(Fl(e.translate)||isNaN(e.translate))&&(e.translate=0)}function Mr(e,t,n,r){$c(e.x,t.x,n.x,r?r.originX:void 0),$c(e.y,t.y,n.y,r?r.originY:void 0)}function Hc(e,t,n){e.min=n.min+t.min,e.max=e.min+Fe(t)}function S1(e,t,n){Hc(e.x,t.x,n.x),Hc(e.y,t.y,n.y)}function Wc(e,t,n){e.min=t.min-n.min,e.max=e.min+Fe(t)}function Rr(e,t,n){Wc(e.x,t.x,n.x),Wc(e.y,t.y,n.y)}function k1(e,{min:t,max:n},r){return t!==void 0&&en&&(e=r?Z(n,e,r.max):Math.min(e,n)),e}function Gc(e,t,n){return{min:t!==void 0?e.min+t:void 0,max:n!==void 0?e.max+n-(e.max-e.min):void 0}}function P1(e,{top:t,left:n,bottom:r,right:i}){return{x:Gc(e.x,n,i),y:Gc(e.y,t,r)}}function Kc(e,t){let n=t.min-e.min,r=t.max-e.max;return t.max-t.minr?n=qr(t.min,t.max-r,e.min):r>i&&(n=qr(e.min,e.max-i,t.min)),Gt(0,1,n)}function E1(e,t){const n={};return t.min!==void 0&&(n.min=t.min-e.min),t.max!==void 0&&(n.max=t.max-e.min),n}const Il=.35;function D1(e=Il){return e===!1?e=0:e===!0&&(e=Il),{x:Qc(e,"left","right"),y:Qc(e,"top","bottom")}}function Qc(e,t,n){return{min:Yc(e,t),max:Yc(e,n)}}function Yc(e,t){return typeof e=="number"?e:e[t]||0}const Xc=()=>({translate:0,scale:1,origin:0,originPoint:0}),Fn=()=>({x:Xc(),y:Xc()}),Zc=()=>({min:0,max:0}),ne=()=>({x:Zc(),y:Zc()});function ot(e){return[e("x"),e("y")]}function Qh({top:e,left:t,right:n,bottom:r}){return{x:{min:t,max:n},y:{min:e,max:r}}}function L1({x:e,y:t}){return{top:t.min,right:e.max,bottom:t.max,left:e.min}}function M1(e,t){if(!t)return e;const n=t({x:e.left,y:e.top}),r=t({x:e.right,y:e.bottom});return{top:n.y,left:n.x,bottom:r.y,right:r.x}}function As(e){return e===void 0||e===1}function zl({scale:e,scaleX:t,scaleY:n}){return!As(e)||!As(t)||!As(n)}function rn(e){return zl(e)||Yh(e)||e.z||e.rotate||e.rotateX||e.rotateY}function Yh(e){return qc(e.x)||qc(e.y)}function qc(e){return e&&e!=="0%"}function So(e,t,n){const r=e-n,i=t*r;return n+i}function Jc(e,t,n,r,i){return i!==void 0&&(e=So(e,i,r)),So(e,n,r)+t}function Bl(e,t=0,n=1,r,i){e.min=Jc(e.min,t,n,r,i),e.max=Jc(e.max,t,n,r,i)}function Xh(e,{x:t,y:n}){Bl(e.x,t.translate,t.scale,t.originPoint),Bl(e.y,n.translate,n.scale,n.originPoint)}function R1(e,t,n,r=!1){const i=n.length;if(!i)return;t.x=t.y=1;let o,s;for(let l=0;l1.0000000000001||e<.999999999999?e:1}function Lt(e,t){e.min=e.min+t,e.max=e.max+t}function ef(e,t,[n,r,i]){const o=t[i]!==void 0?t[i]:.5,s=Z(e.min,e.max,o);Bl(e,t[n],t[r],s,t.scale)}const V1=["x","scaleX","originX"],A1=["y","scaleY","originY"];function In(e,t){ef(e.x,t,V1),ef(e.y,t,A1)}function Zh(e,t){return Qh(M1(e.getBoundingClientRect(),t))}function _1(e,t,n){const r=Zh(e,n),{scroll:i}=t;return i&&(Lt(r.x,i.offset.x),Lt(r.y,i.offset.y)),r}const N1=new WeakMap;class O1{constructor(t){this.openGlobalLock=null,this.isDragging=!1,this.currentDirection=null,this.originPoint={x:0,y:0},this.constraints=!1,this.hasMutatedConstraints=!1,this.elastic=ne(),this.visualElement=t}start(t,{snapToCursor:n=!1}={}){const{presenceContext:r}=this.visualElement;if(r&&r.isPresent===!1)return;const i=a=>{this.stopAnimation(),n&&this.snapToCursor(Wo(a,"page").point)},o=(a,u)=>{const{drag:c,dragPropagation:f,onDragStart:p}=this.getProps();if(c&&!f&&(this.openGlobalLock&&this.openGlobalLock(),this.openGlobalLock=ah(c),!this.openGlobalLock))return;this.isDragging=!0,this.currentDirection=null,this.resolveConstraints(),this.visualElement.projection&&(this.visualElement.projection.isAnimationBlocked=!0,this.visualElement.projection.target=void 0),ot(y=>{let v=this.getAxisMotionValue(y).get()||0;if(ut.test(v)){const{projection:T}=this.visualElement;if(T&&T.layout){const m=T.layout.layoutBox[y];m&&(v=Fe(m)*(parseFloat(v)/100))}}this.originPoint[y]=v}),p&&Q.update(()=>p(a,u),!1,!0);const{animationState:g}=this.visualElement;g&&g.setActive("whileDrag",!0)},s=(a,u)=>{const{dragPropagation:c,dragDirectionLock:f,onDirectionLock:p,onDrag:g}=this.getProps();if(!c&&!this.openGlobalLock)return;const{offset:y}=u;if(f&&this.currentDirection===null){this.currentDirection=j1(y),this.currentDirection!==null&&p&&p(this.currentDirection);return}this.updateAxis("x",u.point,y),this.updateAxis("y",u.point,y),this.visualElement.render(),g&&g(a,u)},l=(a,u)=>this.stop(a,u);this.panSession=new Gh(t,{onSessionStart:i,onStart:o,onMove:s,onSessionEnd:l},{transformPagePoint:this.visualElement.getTransformPagePoint()})}stop(t,n){const r=this.isDragging;if(this.cancel(),!r)return;const{velocity:i}=n;this.startAnimation(i);const{onDragEnd:o}=this.getProps();o&&Q.update(()=>o(t,n))}cancel(){this.isDragging=!1;const{projection:t,animationState:n}=this.visualElement;t&&(t.isAnimationBlocked=!1),this.panSession&&this.panSession.end(),this.panSession=void 0;const{dragPropagation:r}=this.getProps();!r&&this.openGlobalLock&&(this.openGlobalLock(),this.openGlobalLock=null),n&&n.setActive("whileDrag",!1)}updateAxis(t,n,r){const{drag:i}=this.getProps();if(!r||!Di(t,i,this.currentDirection))return;const o=this.getAxisMotionValue(t);let s=this.originPoint[t]+r[t];this.constraints&&this.constraints[t]&&(s=k1(s,this.constraints[t],this.elastic[t])),o.set(s)}resolveConstraints(){const{dragConstraints:t,dragElastic:n}=this.getProps(),{layout:r}=this.visualElement.projection||{},i=this.constraints;t&&On(t)?this.constraints||(this.constraints=this.resolveRefConstraints()):t&&r?this.constraints=P1(r.layoutBox,t):this.constraints=!1,this.elastic=D1(n),i!==this.constraints&&r&&this.constraints&&!this.hasMutatedConstraints&&ot(o=>{this.getAxisMotionValue(o)&&(this.constraints[o]=E1(r.layoutBox[o],this.constraints[o]))})}resolveRefConstraints(){const{dragConstraints:t,onMeasureDragConstraints:n}=this.getProps();if(!t||!On(t))return!1;const r=t.current,{projection:i}=this.visualElement;if(!i||!i.layout)return!1;const o=_1(r,i.root,this.visualElement.getTransformPagePoint());let s=C1(i.layout.layoutBox,o);if(n){const l=n(L1(s));this.hasMutatedConstraints=!!l,l&&(s=Qh(l))}return s}startAnimation(t){const{drag:n,dragMomentum:r,dragElastic:i,dragTransition:o,dragSnapToOrigin:s,onDragTransitionEnd:l}=this.getProps(),a=this.constraints||{},u=ot(c=>{if(!Di(c,n,this.currentDirection))return;let f=a&&a[c]||{};s&&(f={min:0,max:0});const p=i?200:1e6,g=i?40:1e7,y={type:"inertia",velocity:r?t[c]:0,bounceStiffness:p,bounceDamping:g,timeConstant:750,restDelta:1,restSpeed:10,...o,...f};return this.startAxisValueAnimation(c,y)});return Promise.all(u).then(l)}startAxisValueAnimation(t,n){const r=this.getAxisMotionValue(t);return r.start(tu(t,r,0,n))}stopAnimation(){ot(t=>this.getAxisMotionValue(t).stop())}getAxisMotionValue(t){const n="_drag"+t.toUpperCase(),r=this.visualElement.getProps(),i=r[n];return i||this.visualElement.getValue(t,(r.initial?r.initial[t]:void 0)||0)}snapToCursor(t){ot(n=>{const{drag:r}=this.getProps();if(!Di(n,r,this.currentDirection))return;const{projection:i}=this.visualElement,o=this.getAxisMotionValue(n);if(i&&i.layout){const{min:s,max:l}=i.layout.layoutBox[n];o.set(t[n]-Z(s,l,.5))}})}scalePositionWithinConstraints(){if(!this.visualElement.current)return;const{drag:t,dragConstraints:n}=this.getProps(),{projection:r}=this.visualElement;if(!On(n)||!r||!this.constraints)return;this.stopAnimation();const i={x:0,y:0};ot(s=>{const l=this.getAxisMotionValue(s);if(l){const a=l.get();i[s]=T1({min:a,max:a},this.constraints[s])}});const{transformTemplate:o}=this.visualElement.getProps();this.visualElement.current.style.transform=o?o({},""):"none",r.root&&r.root.updateScroll(),r.updateLayout(),this.resolveConstraints(),ot(s=>{if(!Di(s,t,null))return;const l=this.getAxisMotionValue(s),{min:a,max:u}=this.constraints[s];l.set(Z(a,u,i[s]))})}addListeners(){if(!this.visualElement.current)return;N1.set(this.visualElement,this);const t=this.visualElement.current,n=gt(t,"pointerdown",a=>{const{drag:u,dragListener:c=!0}=this.getProps();u&&c&&this.start(a)}),r=()=>{const{dragConstraints:a}=this.getProps();On(a)&&(this.constraints=this.resolveRefConstraints())},{projection:i}=this.visualElement,o=i.addEventListener("measure",r);i&&!i.layout&&(i.root&&i.root.updateScroll(),i.updateLayout()),r();const s=ht(window,"resize",()=>this.scalePositionWithinConstraints()),l=i.addEventListener("didUpdate",({delta:a,hasLayoutChanged:u})=>{this.isDragging&&u&&(ot(c=>{const f=this.getAxisMotionValue(c);f&&(this.originPoint[c]+=a[c].translate,f.set(f.get()+a[c].translate))}),this.visualElement.render())});return()=>{s(),n(),o(),l&&l()}}getProps(){const t=this.visualElement.getProps(),{drag:n=!1,dragDirectionLock:r=!1,dragPropagation:i=!1,dragConstraints:o=!1,dragElastic:s=Il,dragMomentum:l=!0}=t;return{...t,drag:n,dragDirectionLock:r,dragPropagation:i,dragConstraints:o,dragElastic:s,dragMomentum:l}}}function Di(e,t,n){return(t===!0||t===e)&&(n===null||n===e)}function j1(e,t=10){let n=null;return Math.abs(e.y)>t?n="y":Math.abs(e.x)>t&&(n="x"),n}class F1 extends Zt{constructor(t){super(t),this.removeGroupControls=oe,this.removeListeners=oe,this.controls=new O1(t)}mount(){const{dragControls:t}=this.node.getProps();t&&(this.removeGroupControls=t.subscribe(this.controls)),this.removeListeners=this.controls.addListeners()||oe}unmount(){this.removeGroupControls(),this.removeListeners()}}const tf=e=>(t,n)=>{e&&Q.update(()=>e(t,n))};class I1 extends Zt{constructor(){super(...arguments),this.removePointerDownListener=oe}onPointerDown(t){this.session=new Gh(t,this.createPanHandlers(),{transformPagePoint:this.node.getTransformPagePoint()})}createPanHandlers(){const{onPanSessionStart:t,onPanStart:n,onPan:r,onPanEnd:i}=this.node.getProps();return{onSessionStart:tf(t),onStart:tf(n),onMove:r,onEnd:(o,s)=>{delete this.session,i&&Q.update(()=>i(o,s))}}}mount(){this.removePointerDownListener=gt(this.node.current,"pointerdown",t=>this.onPointerDown(t))}update(){this.session&&this.session.updateHandlers(this.createPanHandlers())}unmount(){this.removePointerDownListener(),this.session&&this.session.end()}}function z1(){const e=S.useContext(zo);if(e===null)return[!0,null];const{isPresent:t,onExitComplete:n,register:r}=e,i=S.useId();return S.useEffect(()=>r(i),[]),!t&&n?[!1,()=>n&&n(i)]:[!0]}const Ui={hasAnimatedSinceResize:!0,hasEverUpdated:!1};function nf(e,t){return t.max===t.min?0:e/(t.max-t.min)*100}const dr={correct:(e,t)=>{if(!t.target)return e;if(typeof e=="string")if(A.test(e))e=parseFloat(e);else return e;const n=nf(e,t.target.x),r=nf(e,t.target.y);return`${n}% ${r}%`}},B1={correct:(e,{treeScale:t,projectionDelta:n})=>{const r=e,i=Kt.parse(e);if(i.length>5)return r;const o=Kt.createTransformer(e),s=typeof i[0]!="number"?1:0,l=n.x.scale*t.x,a=n.y.scale*t.y;i[0+s]/=l,i[1+s]/=a;const u=Z(l,a,.5);return typeof i[2+s]=="number"&&(i[2+s]/=u),typeof i[3+s]=="number"&&(i[3+s]/=u),o(i)}};class U1 extends Yl.Component{componentDidMount(){const{visualElement:t,layoutGroup:n,switchLayoutGroup:r,layoutId:i}=this.props,{projection:o}=t;Yy($1),o&&(n.group&&n.group.add(o),r&&r.register&&i&&r.register(o),o.root.didUpdate(),o.addEventListener("animationComplete",()=>{this.safeToRemove()}),o.setOptions({...o.options,onExitComplete:()=>this.safeToRemove()})),Ui.hasEverUpdated=!0}getSnapshotBeforeUpdate(t){const{layoutDependency:n,visualElement:r,drag:i,isPresent:o}=this.props,s=r.projection;return s&&(s.isPresent=o,i||t.layoutDependency!==n||n===void 0?s.willUpdate():this.safeToRemove(),t.isPresent!==o&&(o?s.promote():s.relegate()||Q.postRender(()=>{const l=s.getStack();(!l||!l.members.length)&&this.safeToRemove()}))),null}componentDidUpdate(){const{projection:t}=this.props.visualElement;t&&(t.root.didUpdate(),queueMicrotask(()=>{!t.currentAnimation&&t.isLead()&&this.safeToRemove()}))}componentWillUnmount(){const{visualElement:t,layoutGroup:n,switchLayoutGroup:r}=this.props,{projection:i}=t;i&&(i.scheduleCheckAfterUnmount(),n&&n.group&&n.group.remove(i),r&&r.deregister&&r.deregister(i))}safeToRemove(){const{safeToRemove:t}=this.props;t&&t()}render(){return null}}function qh(e){const[t,n]=z1(),r=S.useContext(Ua);return Yl.createElement(U1,{...e,layoutGroup:r,switchLayoutGroup:S.useContext(Gp),isPresent:t,safeToRemove:n})}const $1={borderRadius:{...dr,applyTo:["borderTopLeftRadius","borderTopRightRadius","borderBottomLeftRadius","borderBottomRightRadius"]},borderTopLeftRadius:dr,borderTopRightRadius:dr,borderBottomLeftRadius:dr,borderBottomRightRadius:dr,boxShadow:B1},Jh=["TopLeft","TopRight","BottomLeft","BottomRight"],H1=Jh.length,rf=e=>typeof e=="string"?parseFloat(e):e,of=e=>typeof e=="number"||A.test(e);function W1(e,t,n,r,i,o){i?(e.opacity=Z(0,n.opacity!==void 0?n.opacity:1,G1(r)),e.opacityExit=Z(t.opacity!==void 0?t.opacity:1,0,K1(r))):o&&(e.opacity=Z(t.opacity!==void 0?t.opacity:1,n.opacity!==void 0?n.opacity:1,r));for(let s=0;s
- """)
- chatbot = gr.Chatbot()
- message = gr.Textbox(placeholder=prompt)
- state = gr.State()
- submit = gr.Button("SEND")
- submit.click(chatgpt_clone, inputs=[message, state], outputs=[chatbot, state])
-
-block.launch(debug = True, share = False)
\ No newline at end of file
diff --git a/spaces/abdvl/datahub_qa_bot/docs/actions/actions/executor.md b/spaces/abdvl/datahub_qa_bot/docs/actions/actions/executor.md
deleted file mode 100644
index 784ac43940866072059e257da9e4a6c3ff65c0be..0000000000000000000000000000000000000000
--- a/spaces/abdvl/datahub_qa_bot/docs/actions/actions/executor.md
+++ /dev/null
@@ -1,82 +0,0 @@
-# Ingestion Executor
-
-
-
-
-## Overview
-
-This Action executes ingestion recipes that are configured via the UI.
-
-### Capabilities
-
-- Executing `datahub ingest` command in a sub-process when an Execution Request command is received from DataHub. (Scheduled or manual ingestion run)
-- Resolving secrets within an ingestion recipe from DataHub
-- Reporting ingestion execution status to DataHub
-
-### Supported Events
-
-- `MetadataChangeLog_v1`
-
-Specifically, changes to the `dataHubExecutionRequestInput` and `dataHubExecutionRequestSignal` aspects of the `dataHubExecutionRequest` entity are required.
-
-
-## Action Quickstart
-
-### Prerequisites
-
-#### DataHub Privileges
-
-This action must be executed as a privileged DataHub user (e.g. using Personal Access Tokens). Specifically, the user must have the `Manage Secrets` Platform Privilege, which allows for retrieval
-of decrypted secrets for injection into an ingestion recipe.
-
-An access token generated from a privileged account must be configured in the `datahub` configuration
-block of the YAML configuration, as shown in the example below.
-
-#### Connecting to Ingestion Sources
-
-In order for ingestion to run successfully, the process running the Actions must have
-network connectivity to any source systems that are required for ingestion.
-
-For example, if the ingestion recipe is pulling from an internal DBMS, the actions container
-must be able to resolve & connect to that DBMS system for the ingestion command to run successfully.
-
-### Install the Plugin(s)
-
-Run the following commands to install the relevant action plugin(s):
-
-`pip install 'acryl-datahub-actions[executor]'`
-
-
-### Configure the Action Config
-
-Use the following config(s) to get started with this Action.
-
-```yml
-name: "pipeline-name"
-source:
- # source configs
-action:
- type: "executor"
-# Requires DataHub API configurations to report to DataHub
-datahub:
- server: "http://${DATAHUB_GMS_HOST:-localhost}:${DATAHUB_GMS_PORT:-8080}"
- # token: # Must have "Manage Secrets" privilege
-```
-
-
- View All Configuration Options
-
- | Field | Required | Default | Description |
- | --- | :-: | :-: | --- |
- | `executor_id` | ❌ | `default` | An executor ID assigned to the executor. This can be used to manage multiple distinct executors. |
-
-
-
-## Troubleshooting
-
-### Quitting the Actions Framework
-
-Currently, when you quit the Actions framework, any in-flight ingestion processing will continue to execute as a subprocess on your system. This means that there may be "orphaned" processes which
-are never marked as "Succeeded" or "Failed" in the UI, even though they may have completed.
-
-To address this, simply "Cancel" the ingestion source on the UI once you've restarted the Ingestion Executor action.
diff --git a/spaces/abhishek/sketch-to-image/annotator/uniformer/mmdet/core/anchor/builder.py b/spaces/abhishek/sketch-to-image/annotator/uniformer/mmdet/core/anchor/builder.py
deleted file mode 100644
index d79b448ebca9f2b21d455046623172c48c5c3ef0..0000000000000000000000000000000000000000
--- a/spaces/abhishek/sketch-to-image/annotator/uniformer/mmdet/core/anchor/builder.py
+++ /dev/null
@@ -1,7 +0,0 @@
-from mmcv.utils import Registry, build_from_cfg
-
-ANCHOR_GENERATORS = Registry('Anchor generator')
-
-
-def build_anchor_generator(cfg, default_args=None):
- return build_from_cfg(cfg, ANCHOR_GENERATORS, default_args)
diff --git a/spaces/abhishek/sketch-to-image/annotator/uniformer/mmdet_null/models/builder.py b/spaces/abhishek/sketch-to-image/annotator/uniformer/mmdet_null/models/builder.py
deleted file mode 100644
index 81c927e507a7c1625ffb114de10e93c94927af25..0000000000000000000000000000000000000000
--- a/spaces/abhishek/sketch-to-image/annotator/uniformer/mmdet_null/models/builder.py
+++ /dev/null
@@ -1,77 +0,0 @@
-import warnings
-
-from mmcv.utils import Registry, build_from_cfg
-from torch import nn
-
-BACKBONES = Registry('backbone')
-NECKS = Registry('neck')
-ROI_EXTRACTORS = Registry('roi_extractor')
-SHARED_HEADS = Registry('shared_head')
-HEADS = Registry('head')
-LOSSES = Registry('loss')
-DETECTORS = Registry('detector')
-
-
-def build(cfg, registry, default_args=None):
- """Build a module.
-
- Args:
- cfg (dict, list[dict]): The config of modules, is is either a dict
- or a list of configs.
- registry (:obj:`Registry`): A registry the module belongs to.
- default_args (dict, optional): Default arguments to build the module.
- Defaults to None.
-
- Returns:
- nn.Module: A built nn module.
- """
- if isinstance(cfg, list):
- modules = [
- build_from_cfg(cfg_, registry, default_args) for cfg_ in cfg
- ]
- return nn.Sequential(*modules)
- else:
- return build_from_cfg(cfg, registry, default_args)
-
-
-def build_backbone(cfg):
- """Build backbone."""
- return build(cfg, BACKBONES)
-
-
-def build_neck(cfg):
- """Build neck."""
- return build(cfg, NECKS)
-
-
-def build_roi_extractor(cfg):
- """Build roi extractor."""
- return build(cfg, ROI_EXTRACTORS)
-
-
-def build_shared_head(cfg):
- """Build shared head."""
- return build(cfg, SHARED_HEADS)
-
-
-def build_head(cfg):
- """Build head."""
- return build(cfg, HEADS)
-
-
-def build_loss(cfg):
- """Build loss."""
- return build(cfg, LOSSES)
-
-
-def build_detector(cfg, train_cfg=None, test_cfg=None):
- """Build detector."""
- if train_cfg is not None or test_cfg is not None:
- warnings.warn(
- 'train_cfg and test_cfg is deprecated, '
- 'please specify them in model', UserWarning)
- assert cfg.get('train_cfg') is None or train_cfg is None, \
- 'train_cfg specified in both outer field and model field '
- assert cfg.get('test_cfg') is None or test_cfg is None, \
- 'test_cfg specified in both outer field and model field '
- return build(cfg, DETECTORS, dict(train_cfg=train_cfg, test_cfg=test_cfg))
diff --git a/spaces/abhishek/sketch-to-image/annotator/uniformer/mmdet_null/utils/contextmanagers.py b/spaces/abhishek/sketch-to-image/annotator/uniformer/mmdet_null/utils/contextmanagers.py
deleted file mode 100644
index 38a639262d949b5754dedf12f33fa814b030ea38..0000000000000000000000000000000000000000
--- a/spaces/abhishek/sketch-to-image/annotator/uniformer/mmdet_null/utils/contextmanagers.py
+++ /dev/null
@@ -1,121 +0,0 @@
-import asyncio
-import contextlib
-import logging
-import os
-import time
-from typing import List
-
-import torch
-
-logger = logging.getLogger(__name__)
-
-DEBUG_COMPLETED_TIME = bool(os.environ.get('DEBUG_COMPLETED_TIME', False))
-
-
-@contextlib.asynccontextmanager
-async def completed(trace_name='',
- name='',
- sleep_interval=0.05,
- streams: List[torch.cuda.Stream] = None):
- """Async context manager that waits for work to complete on given CUDA
- streams."""
- if not torch.cuda.is_available():
- yield
- return
-
- stream_before_context_switch = torch.cuda.current_stream()
- if not streams:
- streams = [stream_before_context_switch]
- else:
- streams = [s if s else stream_before_context_switch for s in streams]
-
- end_events = [
- torch.cuda.Event(enable_timing=DEBUG_COMPLETED_TIME) for _ in streams
- ]
-
- if DEBUG_COMPLETED_TIME:
- start = torch.cuda.Event(enable_timing=True)
- stream_before_context_switch.record_event(start)
-
- cpu_start = time.monotonic()
- logger.debug('%s %s starting, streams: %s', trace_name, name, streams)
- grad_enabled_before = torch.is_grad_enabled()
- try:
- yield
- finally:
- current_stream = torch.cuda.current_stream()
- assert current_stream == stream_before_context_switch
-
- if DEBUG_COMPLETED_TIME:
- cpu_end = time.monotonic()
- for i, stream in enumerate(streams):
- event = end_events[i]
- stream.record_event(event)
-
- grad_enabled_after = torch.is_grad_enabled()
-
- # observed change of torch.is_grad_enabled() during concurrent run of
- # async_test_bboxes code
- assert (grad_enabled_before == grad_enabled_after
- ), 'Unexpected is_grad_enabled() value change'
-
- are_done = [e.query() for e in end_events]
- logger.debug('%s %s completed: %s streams: %s', trace_name, name,
- are_done, streams)
- with torch.cuda.stream(stream_before_context_switch):
- while not all(are_done):
- await asyncio.sleep(sleep_interval)
- are_done = [e.query() for e in end_events]
- logger.debug(
- '%s %s completed: %s streams: %s',
- trace_name,
- name,
- are_done,
- streams,
- )
-
- current_stream = torch.cuda.current_stream()
- assert current_stream == stream_before_context_switch
-
- if DEBUG_COMPLETED_TIME:
- cpu_time = (cpu_end - cpu_start) * 1000
- stream_times_ms = ''
- for i, stream in enumerate(streams):
- elapsed_time = start.elapsed_time(end_events[i])
- stream_times_ms += f' {stream} {elapsed_time:.2f} ms'
- logger.info('%s %s %.2f ms %s', trace_name, name, cpu_time,
- stream_times_ms)
-
-
-@contextlib.asynccontextmanager
-async def concurrent(streamqueue: asyncio.Queue,
- trace_name='concurrent',
- name='stream'):
- """Run code concurrently in different streams.
-
- :param streamqueue: asyncio.Queue instance.
-
- Queue tasks define the pool of streams used for concurrent execution.
- """
- if not torch.cuda.is_available():
- yield
- return
-
- initial_stream = torch.cuda.current_stream()
-
- with torch.cuda.stream(initial_stream):
- stream = await streamqueue.get()
- assert isinstance(stream, torch.cuda.Stream)
-
- try:
- with torch.cuda.stream(stream):
- logger.debug('%s %s is starting, stream: %s', trace_name, name,
- stream)
- yield
- current = torch.cuda.current_stream()
- assert current == stream
- logger.debug('%s %s has finished, stream: %s', trace_name,
- name, stream)
- finally:
- streamqueue.task_done()
- streamqueue.put_nowait(stream)
diff --git a/spaces/abhishek/sketch-to-image/annotator/uniformer_base/mmcv/visualization/__init__.py b/spaces/abhishek/sketch-to-image/annotator/uniformer_base/mmcv/visualization/__init__.py
deleted file mode 100644
index 835df136bdcf69348281d22914d41aa84cdf92b1..0000000000000000000000000000000000000000
--- a/spaces/abhishek/sketch-to-image/annotator/uniformer_base/mmcv/visualization/__init__.py
+++ /dev/null
@@ -1,9 +0,0 @@
-# Copyright (c) OpenMMLab. All rights reserved.
-from .color import Color, color_val
-from .image import imshow, imshow_bboxes, imshow_det_bboxes
-from .optflow import flow2rgb, flowshow, make_color_wheel
-
-__all__ = [
- 'Color', 'color_val', 'imshow', 'imshow_bboxes', 'imshow_det_bboxes',
- 'flowshow', 'flow2rgb', 'make_color_wheel'
-]
diff --git a/spaces/abrar-lohia/text-2-character-anim/pyrender/.eggs/pyglet-2.0.5-py3.10.egg/pyglet/image/__init__.py b/spaces/abrar-lohia/text-2-character-anim/pyrender/.eggs/pyglet-2.0.5-py3.10.egg/pyglet/image/__init__.py
deleted file mode 100644
index 9ccd1697e14de00df62a8f2debbc17810c979798..0000000000000000000000000000000000000000
--- a/spaces/abrar-lohia/text-2-character-anim/pyrender/.eggs/pyglet-2.0.5-py3.10.egg/pyglet/image/__init__.py
+++ /dev/null
@@ -1,2152 +0,0 @@
-"""Image load, capture and high-level texture functions.
-
-Only basic functionality is described here; for full reference see the
-accompanying documentation.
-
-To load an image::
-
- from pyglet import image
- pic = image.load('picture.png')
-
-The supported image file types include PNG, BMP, GIF, JPG, and many more,
-somewhat depending on the operating system. To load an image from a file-like
-object instead of a filename::
-
- pic = image.load('hint.jpg', file=fileobj)
-
-The hint helps the module locate an appropriate decoder to use based on the
-file extension. It is optional.
-
-Once loaded, images can be used directly by most other modules of pyglet. All
-images have a width and height you can access::
-
- width, height = pic.width, pic.height
-
-You can extract a region of an image (this keeps the original image intact;
-the memory is shared efficiently)::
-
- subimage = pic.get_region(x, y, width, height)
-
-Remember that y-coordinates are always increasing upwards.
-
-Drawing images
---------------
-
-To draw an image at some point on the screen::
-
- pic.blit(x, y, z)
-
-This assumes an appropriate view transform and projection have been applied.
-
-Some images have an intrinsic "anchor point": this is the point which will be
-aligned to the ``x`` and ``y`` coordinates when the image is drawn. By
-default the anchor point is the lower-left corner of the image. You can use
-the anchor point to center an image at a given point, for example::
-
- pic.anchor_x = pic.width // 2
- pic.anchor_y = pic.height // 2
- pic.blit(x, y, z)
-
-Texture access
---------------
-
-If you are using OpenGL directly, you can access the image as a texture::
-
- texture = pic.get_texture()
-
-(This is the most efficient way to obtain a texture; some images are
-immediately loaded as textures, whereas others go through an intermediate
-form). To use a texture with pyglet.gl::
-
- from pyglet.gl import *
- glEnable(texture.target) # typically target is GL_TEXTURE_2D
- glBindTexture(texture.target, texture.id)
- # ... draw with the texture
-
-Pixel access
-------------
-
-To access raw pixel data of an image::
-
- rawimage = pic.get_image_data()
-
-(If the image has just been loaded this will be a very quick operation;
-however if the image is a texture a relatively expensive readback operation
-will occur). The pixels can be accessed as a string::
-
- format = 'RGBA'
- pitch = rawimage.width * len(format)
- pixels = rawimage.get_data(format, pitch)
-
-"format" strings consist of characters that give the byte order of each color
-component. For example, if rawimage.format is 'RGBA', there are four color
-components: red, green, blue and alpha, in that order. Other common format
-strings are 'RGB', 'LA' (luminance, alpha) and 'I' (intensity).
-
-The "pitch" of an image is the number of bytes in a row (this may validly be
-more than the number required to make up the width of the image, it is common
-to see this for word alignment). If "pitch" is negative the rows of the image
-are ordered from top to bottom, otherwise they are ordered from bottom to top.
-
-Retrieving data with the format and pitch given in `ImageData.format` and
-`ImageData.pitch` avoids the need for data conversion (assuming you can make
-use of the data in this arbitrary format).
-
-"""
-import re
-import weakref
-
-from ctypes import *
-from io import open, BytesIO
-
-import pyglet
-
-from pyglet.gl import *
-from pyglet.gl import gl_info
-from pyglet.util import asbytes
-
-from .codecs import ImageEncodeException, ImageDecodeException
-from .codecs import registry as _codec_registry
-from .codecs import add_default_codecs as _add_default_codecs
-
-from .animation import Animation, AnimationFrame
-from .buffer import *
-from . import atlas
-
-
-class ImageException(Exception):
- pass
-
-
-def load(filename, file=None, decoder=None):
- """Load an image from a file.
-
- :note: You can make no assumptions about the return type; usually it will
- be ImageData or CompressedImageData, but decoders are free to return
- any subclass of AbstractImage.
-
- :Parameters:
- `filename` : str
- Used to guess the image format, and to load the file if `file` is
- unspecified.
- `file` : file-like object or None
- Source of image data in any supported format.
- `decoder` : ImageDecoder or None
- If unspecified, all decoders that are registered for the filename
- extension are tried. If none succeed, the exception from the
- first decoder is raised.
-
- :rtype: AbstractImage
- """
- if decoder:
- return decoder.decode(filename, file)
- else:
- return _codec_registry.decode(filename, file)
-
-
-def load_animation(filename, file=None, decoder=None):
- """Load an animation from a file.
-
- Currently, the only supported format is GIF.
-
- :Parameters:
- `filename` : str
- Used to guess the animation format, and to load the file if `file`
- is unspecified.
- `file` : file-like object or None
- File object containing the animation stream.
- `decoder` : ImageDecoder or None
- If unspecified, all decoders that are registered for the filename
- extension are tried. If none succeed, the exception from the
- first decoder is raised.
-
- :rtype: Animation
- """
- if decoder:
- return decoder.decode_animation(filename, file)
- else:
- return _codec_registry.decode_animation(filename, file)
-
-
-def create(width, height, pattern=None):
- """Create an image optionally filled with the given pattern.
-
- :note: You can make no assumptions about the return type; usually it will
- be ImageData or CompressedImageData, but patterns are free to return
- any subclass of AbstractImage.
-
- :Parameters:
- `width` : int
- Width of image to create
- `height` : int
- Height of image to create
- `pattern` : ImagePattern or None
- Pattern to fill image with. If unspecified, the image will
- initially be transparent.
-
- :rtype: AbstractImage
- """
- if not pattern:
- pattern = SolidColorImagePattern()
- return pattern.create_image(width, height)
-
-
-def get_max_texture_size():
- """Query the maximum texture size available"""
- size = c_int()
- glGetIntegerv(GL_MAX_TEXTURE_SIZE, size)
- return size.value
-
-
-def get_max_array_texture_layers():
- """Query the maximum TextureArray depth"""
- max_layers = c_int()
- glGetIntegerv(GL_MAX_ARRAY_TEXTURE_LAYERS, max_layers)
- return max_layers.value
-
-
-def _color_as_bytes(color):
- if len(color) != 4:
- raise TypeError("color is expected to have 4 components")
- return bytes(color)
-
-
-class ImagePattern:
- """Abstract image creation class."""
-
- def create_image(self, width, height):
- """Create an image of the given size.
-
- :Parameters:
- `width` : int
- Width of image to create
- `height` : int
- Height of image to create
-
- :rtype: AbstractImage
- """
- raise NotImplementedError('abstract')
-
-
-class SolidColorImagePattern(ImagePattern):
- """Creates an image filled with a solid color."""
-
- def __init__(self, color=(0, 0, 0, 0)):
- """Create a solid image pattern with the given color.
-
- :Parameters:
- `color` : (int, int, int, int)
- 4-tuple of ints in range [0,255] giving RGBA components of
- color to fill with.
-
- """
- self.color = _color_as_bytes(color)
-
- def create_image(self, width, height):
- data = self.color * width * height
- return ImageData(width, height, 'RGBA', data)
-
-
-class CheckerImagePattern(ImagePattern):
- """Create an image with a tileable checker image.
- """
-
- def __init__(self, color1=(150, 150, 150, 255), color2=(200, 200, 200, 255)):
- """Initialise with the given colors.
-
- :Parameters:
- `color1` : (int, int, int, int)
- 4-tuple of ints in range [0,255] giving RGBA components of
- color to fill with. This color appears in the top-left and
- bottom-right corners of the image.
- `color2` : (int, int, int, int)
- 4-tuple of ints in range [0,255] giving RGBA components of
- color to fill with. This color appears in the top-right and
- bottom-left corners of the image.
-
- """
- self.color1 = _color_as_bytes(color1)
- self.color2 = _color_as_bytes(color2)
-
- def create_image(self, width, height):
- hw = width // 2
- hh = height // 2
- row1 = self.color1 * hw + self.color2 * hw
- row2 = self.color2 * hw + self.color1 * hw
- data = row1 * hh + row2 * hh
- return ImageData(width, height, 'RGBA', data)
-
-
-class AbstractImage:
- """Abstract class representing an image.
-
- :Parameters:
- `width` : int
- Width of image
- `height` : int
- Height of image
- `anchor_x` : int
- X coordinate of anchor, relative to left edge of image data
- `anchor_y` : int
- Y coordinate of anchor, relative to bottom edge of image data
- """
- anchor_x = 0
- anchor_y = 0
-
- def __init__(self, width, height):
- self.width = width
- self.height = height
-
- def __repr__(self):
- return "{}(size={}x{})".format(self.__class__.__name__, self.width, self.height)
-
- def get_image_data(self):
- """Get an ImageData view of this image.
-
- Changes to the returned instance may or may not be reflected in this
- image.
-
- :rtype: :py:class:`~pyglet.image.ImageData`
-
- .. versionadded:: 1.1
- """
- raise ImageException('Cannot retrieve image data for %r' % self)
-
- def get_texture(self, rectangle=False):
- """A :py:class:`~pyglet.image.Texture` view of this image.
-
- :Parameters:
- `rectangle` : bool
- Unused. Kept for compatibility.
-
- .. versionadded:: 1.1.4.
- :rtype: :py:class:`~pyglet.image.Texture`
-
- .. versionadded:: 1.1
- """
- raise ImageException('Cannot retrieve texture for %r' % self)
-
- def get_mipmapped_texture(self):
- """Retrieve a :py:class:`~pyglet.image.Texture` instance with all mipmap levels filled in.
-
- :rtype: :py:class:`~pyglet.image.Texture`
-
- .. versionadded:: 1.1
- """
- raise ImageException('Cannot retrieve mipmapped texture for %r' % self)
-
- def get_region(self, x, y, width, height):
- """Retrieve a rectangular region of this image.
-
- :Parameters:
- `x` : int
- Left edge of region.
- `y` : int
- Bottom edge of region.
- `width` : int
- Width of region.
- `height` : int
- Height of region.
-
- :rtype: AbstractImage
- """
- raise ImageException('Cannot get region for %r' % self)
-
- def save(self, filename=None, file=None, encoder=None):
- """Save this image to a file.
-
- :Parameters:
- `filename` : str
- Used to set the image file format, and to open the output file
- if `file` is unspecified.
- `file` : file-like object or None
- File to write image data to.
- `encoder` : ImageEncoder or None
- If unspecified, all encoders matching the filename extension
- are tried. If all fail, the exception from the first one
- attempted is raised.
-
- """
- if not file:
- file = open(filename, 'wb')
-
- if encoder:
- encoder.encode(self, filename, file)
- else:
- first_exception = None
- for encoder in _codec_registry.get_encoders(filename):
- try:
- return encoder.encode(self, filename, file)
- except ImageEncodeException as e:
- first_exception = first_exception or e
- file.seek(0)
-
- if not first_exception:
- raise ImageEncodeException('No image encoders are available')
- raise first_exception
-
- def blit(self, x, y, z=0):
- """Draw this image to the active framebuffers.
-
- The image will be drawn with the lower-left corner at
- (``x -`` `anchor_x`, ``y -`` `anchor_y`, ``z``).
- """
- raise ImageException('Cannot blit %r.' % self)
-
- def blit_into(self, source, x, y, z):
- """Draw `source` on this image.
-
- `source` will be copied into this image such that its anchor point
- is aligned with the `x` and `y` parameters. If this image is a 3D
- texture, the `z` coordinate gives the image slice to copy into.
-
- Note that if `source` is larger than this image (or the positioning
- would cause the copy to go out of bounds) then you must pass a
- region of `source` to this method, typically using get_region().
- """
- raise ImageException('Cannot blit images onto %r.' % self)
-
- def blit_to_texture(self, target, level, x, y, z=0):
- """Draw this image on the currently bound texture at `target`.
-
- This image is copied into the texture such that this image's anchor
- point is aligned with the given `x` and `y` coordinates of the
- destination texture. If the currently bound texture is a 3D texture,
- the `z` coordinate gives the image slice to blit into.
- """
- raise ImageException('Cannot blit %r to a texture.' % self)
-
-
-class AbstractImageSequence:
- """Abstract sequence of images.
-
- The sequence is useful for storing image animations or slices of a volume.
- For efficient access, use the `texture_sequence` member. The class
- also implements the sequence interface (`__len__`, `__getitem__`,
- `__setitem__`).
- """
-
- def get_texture_sequence(self):
- """Get a TextureSequence.
-
- :rtype: `TextureSequence`
-
- .. versionadded:: 1.1
- """
- raise NotImplementedError('abstract')
-
- def get_animation(self, period, loop=True):
- """Create an animation over this image sequence for the given constant
- framerate.
-
- :Parameters
- `period` : float
- Number of seconds to display each frame.
- `loop` : bool
- If True, the animation will loop continuously.
-
- :rtype: :py:class:`~pyglet.image.Animation`
-
- .. versionadded:: 1.1
- """
- return Animation.from_image_sequence(self, period, loop)
-
- def __getitem__(self, slice):
- """Retrieve a (list of) image.
-
- :rtype: AbstractImage
- """
- raise NotImplementedError('abstract')
-
- def __setitem__(self, slice, image):
- """Replace one or more images in the sequence.
-
- :Parameters:
- `image` : `~pyglet.image.AbstractImage`
- The replacement image. The actual instance may not be used,
- depending on this implementation.
-
- """
- raise NotImplementedError('abstract')
-
- def __len__(self):
- raise NotImplementedError('abstract')
-
- def __iter__(self):
- """Iterate over the images in sequence.
-
- :rtype: Iterator
-
- .. versionadded:: 1.1
- """
- raise NotImplementedError('abstract')
-
-
-class TextureSequence(AbstractImageSequence):
- """Interface for a sequence of textures.
-
- Typical implementations store multiple :py:class:`~pyglet.image.TextureRegion` s within one
- :py:class:`~pyglet.image.Texture` so as to minimise state changes.
- """
-
- def get_texture_sequence(self):
- return self
-
-
-class UniformTextureSequence(TextureSequence):
- """Interface for a sequence of textures, each with the same dimensions.
-
- :Parameters:
- `item_width` : int
- Width of each texture in the sequence.
- `item_height` : int
- Height of each texture in the sequence.
-
- """
-
- def _get_item_width(self):
- raise NotImplementedError('abstract')
-
- def _get_item_height(self):
- raise NotImplementedError('abstract')
-
- @property
- def item_width(self):
- return self._get_item_width()
-
- @property
- def item_height(self):
- return self._get_item_height()
-
-
-class ImageData(AbstractImage):
- """An image represented as a string of unsigned bytes.
-
- :Parameters:
- `data` : str
- Pixel data, encoded according to `format` and `pitch`.
- `format` : str
- The format string to use when reading or writing `data`.
- `pitch` : int
- Number of bytes per row. Negative values indicate a top-to-bottom
- arrangement.
-
- """
- _swap1_pattern = re.compile(asbytes('(.)'), re.DOTALL)
- _swap2_pattern = re.compile(asbytes('(.)(.)'), re.DOTALL)
- _swap3_pattern = re.compile(asbytes('(.)(.)(.)'), re.DOTALL)
- _swap4_pattern = re.compile(asbytes('(.)(.)(.)(.)'), re.DOTALL)
-
- _current_texture = None
- _current_mipmap_texture = None
-
- def __init__(self, width, height, fmt, data, pitch=None):
- """Initialise image data.
-
- :Parameters:
- `width` : int
- Width of image data
- `height` : int
- Height of image data
- `fmt` : str
- A valid format string, such as 'RGB', 'RGBA', 'ARGB', etc.
- `data` : sequence
- String or array/list of bytes giving the decoded data.
- `pitch` : int or None
- If specified, the number of bytes per row. Negative values
- indicate a top-to-bottom arrangement. Defaults to
- ``width * len(format)``.
-
- """
- super().__init__(width, height)
-
- self._current_format = self._desired_format = fmt.upper()
- self._current_data = data
- self.pitch = pitch or width * len(fmt)
- self._current_pitch = self.pitch
- self.mipmap_images = []
-
- def __getstate__(self):
- return {
- 'width': self.width,
- 'height': self.height,
- '_current_data': self.get_data(self._current_format, self._current_pitch),
- '_current_format': self._current_format,
- '_desired_format': self._desired_format,
- '_current_pitch': self._current_pitch,
- 'pitch': self.pitch,
- 'mipmap_images': self.mipmap_images
- }
-
- def get_image_data(self):
- return self
-
- @property
- def format(self):
- """Format string of the data. Read-write.
-
- :type: str
- """
- return self._desired_format
-
- @format.setter
- def format(self, fmt):
- self._desired_format = fmt.upper()
- self._current_texture = None
-
- def get_data(self, fmt=None, pitch=None):
- """Get the byte data of the image.
-
- :Parameters:
- `fmt` : str
- Format string of the return data.
- `pitch` : int
- Number of bytes per row. Negative values indicate a
- top-to-bottom arrangement.
-
- .. versionadded:: 1.1
-
- :rtype: sequence of bytes, or str
- """
- fmt = fmt or self._desired_format
- pitch = pitch or self._current_pitch
-
- if fmt == self._current_format and pitch == self._current_pitch:
- return self._current_data
- return self._convert(fmt, pitch)
-
- def set_data(self, fmt, pitch, data):
- """Set the byte data of the image.
-
- :Parameters:
- `fmt` : str
- Format string of the return data.
- `pitch` : int
- Number of bytes per row. Negative values indicate a
- top-to-bottom arrangement.
- `data` : str or sequence of bytes
- Image data.
-
- .. versionadded:: 1.1
- """
- self._current_format = fmt
- self._current_pitch = pitch
- self._current_data = data
- self._current_texture = None
- self._current_mipmap_texture = None
-
- def set_mipmap_image(self, level, image):
- """Set a mipmap image for a particular level.
-
- The mipmap image will be applied to textures obtained via
- `get_mipmapped_texture`.
-
- :Parameters:
- `level` : int
- Mipmap level to set image at, must be >= 1.
- `image` : AbstractImage
- Image to set. Must have correct dimensions for that mipmap
- level (i.e., width >> level, height >> level)
- """
-
- if level == 0:
- raise ImageException('Cannot set mipmap image at level 0 (it is this image)')
-
- # Check dimensions of mipmap
- width, height = self.width, self.height
- for i in range(level):
- width >>= 1
- height >>= 1
- if width != image.width or height != image.height:
- raise ImageException('Mipmap image has wrong dimensions for level %d' % level)
-
- # Extend mipmap_images list to required level
- self.mipmap_images += [None] * (level - len(self.mipmap_images))
- self.mipmap_images[level - 1] = image
-
- def create_texture(self, cls, rectangle=False):
- """Create a texture containing this image.
-
- :Parameters:
- `cls` : class (subclass of Texture)
- Class to construct.
- `rectangle` : bool
- Unused. kept for compatibility.
-
- .. versionadded:: 1.1
-
- :rtype: cls or cls.region_class
- """
- internalformat = self._get_internalformat(self._desired_format)
- texture = cls.create(self.width, self.height, GL_TEXTURE_2D, internalformat, False, blank_data=False)
- if self.anchor_x or self.anchor_y:
- texture.anchor_x = self.anchor_x
- texture.anchor_y = self.anchor_y
-
- self.blit_to_texture(texture.target, texture.level, self.anchor_x, self.anchor_y, 0, None)
-
- return texture
-
- def get_texture(self, rectangle=False):
- if not self._current_texture:
- self._current_texture = self.create_texture(Texture, rectangle)
- return self._current_texture
-
- def get_mipmapped_texture(self):
- """Return a Texture with mipmaps.
-
- If :py:class:`~pyglet.image.set_mipmap_Image` has been called with at least one image, the set
- of images defined will be used. Otherwise, mipmaps will be
- automatically generated.
-
- :rtype: :py:class:`~pyglet.image.Texture`
-
- .. versionadded:: 1.1
- """
- if self._current_mipmap_texture:
- return self._current_mipmap_texture
-
- texture = Texture.create(self.width, self.height, GL_TEXTURE_2D, None, blank_data=False)
- if self.anchor_x or self.anchor_y:
- texture.anchor_x = self.anchor_x
- texture.anchor_y = self.anchor_y
-
- internalformat = self._get_internalformat(self.format)
-
- glBindTexture(texture.target, texture.id)
- glTexParameteri(texture.target, GL_TEXTURE_MIN_FILTER, GL_LINEAR_MIPMAP_LINEAR)
-
- if self.mipmap_images:
- self.blit_to_texture(texture.target, texture.level, self.anchor_x, self.anchor_y, 0, internalformat)
- level = 0
- for image in self.mipmap_images:
- level += 1
- if image:
- image.blit_to_texture(texture.target, level, self.anchor_x, self.anchor_y, 0, internalformat)
- # TODO: should set base and max mipmap level if some mipmaps are missing.
- else:
- glGenerateMipmap(texture.target)
- self.blit_to_texture(texture.target, texture.level, self.anchor_x, self.anchor_y, 0, internalformat)
-
- self._current_mipmap_texture = texture
- return texture
-
- def get_region(self, x, y, width, height):
- """Retrieve a rectangular region of this image data.
-
- :Parameters:
- `x` : int
- Left edge of region.
- `y` : int
- Bottom edge of region.
- `width` : int
- Width of region.
- `height` : int
- Height of region.
-
- :rtype: ImageDataRegion
- """
- return ImageDataRegion(x, y, width, height, self)
-
- def blit(self, x, y, z=0, width=None, height=None):
- self.get_texture().blit(x, y, z, width, height)
-
- def blit_to_texture(self, target, level, x, y, z, internalformat=None):
- """Draw this image to to the currently bound texture at `target`.
-
- This image's anchor point will be aligned to the given `x` and `y`
- coordinates. If the currently bound texture is a 3D texture, the `z`
- parameter gives the image slice to blit into.
-
- If `internalformat` is specified, glTexImage is used to initialise
- the texture; otherwise, glTexSubImage is used to update a region.
- """
- x -= self.anchor_x
- y -= self.anchor_y
-
- data_format = self.format
- data_pitch = abs(self._current_pitch)
-
- # Determine pixel format from format string
- fmt, gl_type = self._get_gl_format_and_type(data_format)
-
- if fmt is None:
- # Need to convert data to a standard form
- data_format = {
- 1: 'R',
- 2: 'RG',
- 3: 'RGB',
- 4: 'RGBA'}.get(len(data_format))
- fmt, gl_type = self._get_gl_format_and_type(data_format)
-
- # Get data in required format (hopefully will be the same format it's already
- # in, unless that's an obscure format, upside-down or the driver is old).
- data = self._convert(data_format, data_pitch)
-
- if data_pitch & 0x1:
- align = 1
- elif data_pitch & 0x2:
- align = 2
- else:
- align = 4
- row_length = data_pitch // len(data_format)
-
- glPixelStorei(GL_UNPACK_ALIGNMENT, align)
- glPixelStorei(GL_UNPACK_ROW_LENGTH, row_length)
- self._apply_region_unpack()
-
- if target == GL_TEXTURE_3D or target == GL_TEXTURE_2D_ARRAY:
- assert not internalformat
- glTexSubImage3D(target, level,
- x, y, z,
- self.width, self.height, 1,
- fmt, gl_type,
- data)
- elif internalformat:
- glTexImage2D(target, level,
- internalformat,
- self.width, self.height,
- 0,
- fmt, gl_type,
- data)
- else:
- glTexSubImage2D(target, level,
- x, y,
- self.width, self.height,
- fmt, gl_type,
- data)
-
- # Unset GL_UNPACK_ROW_LENGTH:
- glPixelStorei(GL_UNPACK_ROW_LENGTH, 0)
- self._default_region_unpack()
-
- # Flush image upload before data get GC'd:
- glFlush()
-
- def _apply_region_unpack(self):
- pass
-
- def _default_region_unpack(self):
- pass
-
- def _convert(self, fmt, pitch):
- """Return data in the desired format; does not alter this instance's
- current format or pitch.
- """
- if fmt == self._current_format and pitch == self._current_pitch:
- if type(self._current_data) is str:
- return asbytes(self._current_data)
- return self._current_data
-
- self._ensure_bytes()
- data = self._current_data
- current_pitch = self._current_pitch
- current_format = self._current_format
- sign_pitch = current_pitch // abs(current_pitch)
- if fmt != self._current_format:
- # Create replacement string, e.g. r'\4\1\2\3' to convert RGBA to ARGB
- repl = asbytes('')
- for c in fmt:
- try:
- idx = current_format.index(c) + 1
- except ValueError:
- idx = 1
- repl += asbytes(r'\%d' % idx)
-
- if len(current_format) == 1:
- swap_pattern = self._swap1_pattern
- elif len(current_format) == 2:
- swap_pattern = self._swap2_pattern
- elif len(current_format) == 3:
- swap_pattern = self._swap3_pattern
- elif len(current_format) == 4:
- swap_pattern = self._swap4_pattern
- else:
- raise ImageException('Current image format is wider than 32 bits.')
-
- packed_pitch = self.width * len(current_format)
- if abs(self._current_pitch) != packed_pitch:
- # Pitch is wider than pixel data, need to go row-by-row.
- new_pitch = abs(self._current_pitch)
- rows = [data[i:i+new_pitch] for i in range(0, len(data), new_pitch)]
- rows = [swap_pattern.sub(repl, r[:packed_pitch]) for r in rows]
- data = asbytes('').join(rows)
- else:
- # Rows are tightly packed, apply regex over whole image.
- data = swap_pattern.sub(repl, data)
-
- # After conversion, rows will always be tightly packed
- current_pitch = sign_pitch * (len(fmt) * self.width)
-
- if pitch != current_pitch:
- diff = abs(current_pitch) - abs(pitch)
- if diff > 0:
- # New pitch is shorter than old pitch, chop bytes off each row
- new_pitch = abs(pitch)
- rows = [data[i:i+new_pitch-diff] for i in range(0, len(data), new_pitch)]
- data = asbytes('').join(rows)
-
- elif diff < 0:
- # New pitch is longer than old pitch, add '0' bytes to each row
- new_pitch = abs(current_pitch)
- padding = asbytes(1) * -diff
- rows = [data[i:i+new_pitch] + padding for i in range(0, len(data), new_pitch)]
- data = asbytes('').join(rows)
-
- if current_pitch * pitch < 0:
- # Pitch differs in sign, swap row order
- new_pitch = abs(pitch)
- rows = [data[i:i+new_pitch] for i in range(0, len(data), new_pitch)]
- rows.reverse()
- data = asbytes('').join(rows)
-
- return asbytes(data)
-
- def _ensure_bytes(self):
- if type(self._current_data) is not bytes:
- self._current_data = asbytes(self._current_data)
-
- @staticmethod
- def _get_gl_format_and_type(fmt):
- if fmt == 'R':
- return GL_RED, GL_UNSIGNED_BYTE
- elif fmt == 'RG':
- return GL_RG, GL_UNSIGNED_BYTE
- elif fmt == 'RGB':
- return GL_RGB, GL_UNSIGNED_BYTE
- elif fmt == 'BGR':
- return GL_BGR, GL_UNSIGNED_BYTE
- elif fmt == 'RGBA':
- return GL_RGBA, GL_UNSIGNED_BYTE
- elif fmt == 'BGRA':
- return GL_BGRA, GL_UNSIGNED_BYTE
-
- elif fmt == 'L':
- return GL_LUMINANCE, GL_UNSIGNED_BYTE
- elif fmt == 'A':
- return GL_ALPHA, GL_UNSIGNED_BYTE
-
- return None, None
-
- @staticmethod
- def _get_internalformat(fmt):
- if fmt == 'R':
- return GL_RED
- elif fmt == 'RG':
- return GL_RG
- elif fmt == 'RGB':
- return GL_RGB
- elif fmt == 'RGBA':
- return GL_RGBA
- elif fmt == 'D':
- return GL_DEPTH_COMPONENT
- elif fmt == 'DS':
- return GL_DEPTH_STENCIL
-
- elif fmt == 'L':
- return GL_LUMINANCE
- elif fmt == 'A':
- return GL_ALPHA
-
- return GL_RGBA
-
-
-class ImageDataRegion(ImageData):
- def __init__(self, x, y, width, height, image_data):
- super().__init__(width, height,
- image_data._current_format,
- image_data._current_data,
- image_data._current_pitch)
- self.x = x
- self.y = y
-
- def __getstate__(self):
- return {
- 'width': self.width,
- 'height': self.height,
- '_current_data': self.get_data(self._current_format, self._current_pitch),
- '_current_format': self._current_format,
- '_desired_format': self._desired_format,
- '_current_pitch': self._current_pitch,
- 'pitch': self.pitch,
- 'mipmap_images': self.mipmap_images,
- 'x': self.x,
- 'y': self.y
- }
-
- def get_data(self, fmt=None, pitch=None):
- x1 = len(self._current_format) * self.x
- x2 = len(self._current_format) * (self.x + self.width)
-
- self._ensure_bytes()
- data = self._convert(self._current_format, abs(self._current_pitch))
- new_pitch = abs(self._current_pitch)
- rows = [data[i:i+new_pitch] for i in range(0, len(data), new_pitch)]
- rows = [row[x1:x2] for row in rows[self.y:self.y + self.height]]
- self._current_data = b''.join(rows)
- self._current_pitch = self.width * len(self._current_format)
- self._current_texture = None
- self.x = 0
- self.y = 0
-
- fmt = fmt or self._desired_format
- pitch = pitch or self._current_pitch
- return super().get_data(fmt, pitch)
-
- def set_data(self, fmt, pitch, data):
- self.x = 0
- self.y = 0
- super().set_data(fmt, pitch, data)
-
- def _apply_region_unpack(self):
- glPixelStorei(GL_UNPACK_SKIP_PIXELS, self.x)
- glPixelStorei(GL_UNPACK_SKIP_ROWS, self.y)
-
- def _default_region_unpack(self):
- glPixelStorei(GL_UNPACK_SKIP_PIXELS, 0)
- glPixelStorei(GL_UNPACK_SKIP_ROWS, 0)
-
- def get_region(self, x, y, width, height):
- x += self.x
- y += self.y
- return super().get_region(x, y, width, height)
-
-
-class CompressedImageData(AbstractImage):
- """Image representing some compressed data suitable for direct uploading
- to driver.
- """
-
- _current_texture = None
- _current_mipmap_texture = None
-
- def __init__(self, width, height, gl_format, data, extension=None, decoder=None):
- """Construct a CompressedImageData with the given compressed data.
-
- :Parameters:
- `width` : int
- Width of image
- `height` : int
- Height of image
- `gl_format` : int
- GL constant giving format of compressed data; for example,
- ``GL_COMPRESSED_RGBA_S3TC_DXT5_EXT``.
- `data` : sequence
- String or array/list of bytes giving compressed image data.
- `extension` : str or None
- If specified, gives the name of a GL extension to check for
- before creating a texture.
- `decoder` : function(data, width, height) -> AbstractImage
- A function to decode the compressed data, to be used if the
- required extension is not present.
-
- """
- super().__init__(width, height)
- self.data = data
- self.gl_format = gl_format
- self.extension = extension
- self.decoder = decoder
- self.mipmap_data = []
-
- def set_mipmap_data(self, level, data):
- """Set data for a mipmap level.
-
- Supplied data gives a compressed image for the given mipmap level.
- The image must be of the correct dimensions for the level
- (i.e., width >> level, height >> level); but this is not checked. If
- any mipmap levels are specified, they are used; otherwise, mipmaps for
- `mipmapped_texture` are generated automatically.
-
- :Parameters:
- `level` : int
- Level of mipmap image to set.
- `data` : sequence
- String or array/list of bytes giving compressed image data.
- Data must be in same format as specified in constructor.
-
- """
- # Extend mipmap_data list to required level
- self.mipmap_data += [None] * (level - len(self.mipmap_data))
- self.mipmap_data[level - 1] = data
-
- def _have_extension(self):
- return self.extension is None or gl_info.have_extension(self.extension)
-
- def _verify_driver_supported(self):
- """Assert that the extension required for this image data is
- supported.
-
- Raises `ImageException` if not.
- """
-
- if not self._have_extension():
- raise ImageException('%s is required to decode %r' % (self.extension, self))
-
- def get_texture(self, rectangle=False):
- if rectangle:
- raise ImageException('Compressed texture rectangles not supported')
-
- if self._current_texture:
- return self._current_texture
-
- texture = Texture.create(self.width, self.height, GL_TEXTURE_2D, None)
-
- if self.anchor_x or self.anchor_y:
- texture.anchor_x = self.anchor_x
- texture.anchor_y = self.anchor_y
-
- glBindTexture(texture.target, texture.id)
- glTexParameteri(texture.target, GL_TEXTURE_MIN_FILTER, texture.min_filter)
- glTexParameteri(texture.target, GL_TEXTURE_MAG_FILTER, texture.mag_filter)
-
- if self._have_extension():
- glCompressedTexImage2D(texture.target, texture.level,
- self.gl_format,
- self.width, self.height, 0,
- len(self.data), self.data)
- else:
- image = self.decoder(self.data, self.width, self.height)
- texture = image.get_texture()
- assert texture.width == self.width
- assert texture.height == self.height
-
- glFlush()
- self._current_texture = texture
- return texture
-
- def get_mipmapped_texture(self):
- if self._current_mipmap_texture:
- return self._current_mipmap_texture
-
- if not self._have_extension():
- # TODO mip-mapped software decoded compressed textures. For now,
- # just return a non-mipmapped texture.
- return self.get_texture()
-
- texture = Texture.create(self.width, self.height, GL_TEXTURE_2D, None)
-
- if self.anchor_x or self.anchor_y:
- texture.anchor_x = self.anchor_x
- texture.anchor_y = self.anchor_y
-
- glBindTexture(texture.target, texture.id)
-
- glTexParameteri(texture.target, GL_TEXTURE_MIN_FILTER, GL_LINEAR_MIPMAP_LINEAR)
-
- if not self.mipmap_data:
- glGenerateMipmap(texture.target)
-
- glCompressedTexImage2D(texture.target, texture.level,
- self.gl_format,
- self.width, self.height, 0,
- len(self.data), self.data)
-
- width, height = self.width, self.height
- level = 0
- for data in self.mipmap_data:
- width >>= 1
- height >>= 1
- level += 1
- glCompressedTexImage2D(texture.target, level, self.gl_format, width, height, 0, len(data), data)
-
- glFlush()
-
- self._current_mipmap_texture = texture
- return texture
-
- def blit_to_texture(self, target, level, x, y, z):
- self._verify_driver_supported()
-
- if target == GL_TEXTURE_3D:
- glCompressedTexSubImage3D(target, level,
- x - self.anchor_x, y - self.anchor_y, z,
- self.width, self.height, 1,
- self.gl_format,
- len(self.data), self.data)
- else:
- glCompressedTexSubImage2D(target, level,
- x - self.anchor_x, y - self.anchor_y,
- self.width, self.height,
- self.gl_format,
- len(self.data), self.data)
-
-
-class Texture(AbstractImage):
- """An image loaded into video memory that can be efficiently drawn
- to the framebuffer.
-
- Typically, you will get an instance of Texture by accessing the `texture`
- member of any other AbstractImage.
-
- :Parameters:
- `region_class` : class (subclass of TextureRegion)
- Class to use when constructing regions of this texture.
- `tex_coords` : tuple
- 12-tuple of float, named (u1, v1, r1, u2, v2, r2, ...). u, v, r
- give the 3D texture coordinates for vertices 1-4. The vertices
- are specified in the order bottom-left, bottom-right, top-right
- and top-left.
- `target` : int
- The GL texture target (e.g., ``GL_TEXTURE_2D``).
- `level` : int
- The mipmap level of this texture.
-
- """
-
- region_class = None # Set to TextureRegion after it's defined
- tex_coords = (0, 0, 0, 1, 0, 0, 1, 1, 0, 0, 1, 0)
- tex_coords_order = (0, 1, 2, 3)
- colors = (0, 0, 0, 0) * 4
- level = 0
- images = 1
- x = y = z = 0
- default_min_filter = GL_LINEAR
- default_mag_filter = GL_LINEAR
-
- def __init__(self, width, height, target, tex_id):
- super().__init__(width, height)
- self.target = target
- self.id = tex_id
- self._context = pyglet.gl.current_context
-
- def __del__(self):
- try:
- self._context.delete_texture(self.id)
- except Exception:
- pass
-
- def bind(self, texture_unit: int = 0):
- """Bind to a specific Texture Unit by number."""
- glActiveTexture(GL_TEXTURE0 + texture_unit)
- glBindTexture(self.target, self.id)
-
- def bind_image_texture(self, unit, level=0, layered=False, layer=0, access=GL_READ_WRITE, fmt=GL_RGBA32F):
- """Bind as an ImageTexture for use with a :py:class:`~pyglet.shader.ComputeShaderProgram`.
-
- ..note:: OpenGL 4.3, or 4.2 with the GL_ARB_compute_shader extention is required.
- """
- glBindImageTexture(unit, self.id, level, layered, layer, access, fmt)
-
- @classmethod
- def create(cls, width, height, target=GL_TEXTURE_2D, internalformat=GL_RGBA8, min_filter=None, mag_filter=None, fmt=GL_RGBA, blank_data=True):
- """Create a Texture
-
- Create a Texture with the specified dimentions, target and format.
- On return, the texture will be bound.
-
- :Parameters:
- `width` : int
- Width of texture in pixels.
- `height` : int
- Height of texture in pixels.
- `target` : int
- GL constant giving texture target to use, typically ``GL_TEXTURE_2D``.
- `internalformat` : int
- GL constant giving internal format of texture; for example, ``GL_RGBA``.
- The internal format decides how the texture data will be stored internally.
- If ``None``, the texture will be created but not initialized.
- `min_filter` : int
- The minifaction filter used for this texture, commonly ``GL_LINEAR`` or ``GL_NEAREST``
- `mag_filter` : int
- The magnification filter used for this texture, commonly ``GL_LINEAR`` or ``GL_NEAREST``
- `fmt` : int
- GL constant giving format of texture; for example, ``GL_RGBA``.
- The format specifies what format the pixel data we're expecting to write
- to the texture and should ideally be the same as for internal format.
- `blank_data` : bool
- Setting to True will initialize the texture data with all zeros. Setting False, will initialize Texture
- with no data.
-
- :rtype: :py:class:`~pyglet.image.Texture`
- """
- min_filter = min_filter or cls.default_min_filter
- mag_filter = mag_filter or cls.default_mag_filter
-
- tex_id = GLuint()
- glGenTextures(1, byref(tex_id))
- glBindTexture(target, tex_id.value)
- glTexParameteri(target, GL_TEXTURE_MIN_FILTER, min_filter)
- glTexParameteri(target, GL_TEXTURE_MAG_FILTER, mag_filter)
-
- if internalformat is not None:
- blank = (GLubyte * (width * height * 4))() if blank_data else None
- glTexImage2D(target, 0,
- internalformat,
- width, height,
- 0,
- fmt,
- GL_UNSIGNED_BYTE,
- blank)
- glFlush()
-
- texture = cls(width, height, target, tex_id.value)
- texture.min_filter = min_filter
- texture.mag_filter = mag_filter
- texture.tex_coords = cls.tex_coords
-
- return texture
-
- def get_image_data(self, z=0):
- """Get the image data of this texture.
-
- Changes to the returned instance will not be reflected in this
- texture.
-
- :Parameters:
- `z` : int
- For 3D textures, the image slice to retrieve.
-
- :rtype: :py:class:`~pyglet.image.ImageData`
- """
- glBindTexture(self.target, self.id)
-
- # Always extract complete RGBA data. Could check internalformat
- # to only extract used channels. XXX
- fmt = 'RGBA'
- gl_format = GL_RGBA
-
- buf = (GLubyte * (self.width * self.height * self.images * len(fmt)))()
-
- # TODO: Clean up this temporary hack
- if pyglet.gl.current_context.get_info().get_opengl_api() == "gles":
- fbo = c_uint()
- glGenFramebuffers(1, fbo)
- glBindFramebuffer(GL_FRAMEBUFFER, fbo.value)
- glPixelStorei(GL_PACK_ALIGNMENT, 1)
- glCheckFramebufferStatus(GL_FRAMEBUFFER)
- glFramebufferTexture2D(GL_FRAMEBUFFER, GL_COLOR_ATTACHMENT0, GL_TEXTURE_2D, self.id, self.level)
- glReadPixels(0, 0, self.width, self.height, gl_format, GL_UNSIGNED_BYTE, buf)
- glBindFramebuffer(GL_FRAMEBUFFER, 0)
- glDeleteFramebuffers(1, fbo)
- else:
- glPixelStorei(GL_PACK_ALIGNMENT, 1)
- glGetTexImage(self.target, self.level, gl_format, GL_UNSIGNED_BYTE, buf)
-
- data = ImageData(self.width, self.height, fmt, buf)
- if self.images > 1:
- data = data.get_region(0, z * self.height, self.width, self.height)
- return data
-
- def get_texture(self, rectangle=False):
- return self
-
- # no implementation of blit_to_texture yet
-
- def blit(self, x, y, z=0, width=None, height=None):
- x1 = x - self.anchor_x
- y1 = y - self.anchor_y
- x2 = x1 + (width is None and self.width or width)
- y2 = y1 + (height is None and self.height or height)
- vertices = x1, y1, z, x2, y1, z, x2, y2, z, x1, y2, z
-
- glActiveTexture(GL_TEXTURE0)
- glBindTexture(self.target, self.id)
-
- pyglet.graphics.draw_indexed(4, GL_TRIANGLES, [0, 1, 2, 0, 2, 3],
- position=('f', vertices),
- tex_coords=('f', self.tex_coords),
- colors=('Bn', self.colors))
-
- glBindTexture(self.target, 0)
-
- def blit_into(self, source, x, y, z):
- glBindTexture(self.target, self.id)
- source.blit_to_texture(self.target, self.level, x, y, z)
-
- def get_region(self, x, y, width, height):
- return self.region_class(x, y, 0, width, height, self)
-
- def get_transform(self, flip_x=False, flip_y=False, rotate=0):
- """Create a copy of this image applying a simple transformation.
-
- The transformation is applied to the texture coordinates only;
- :py:meth:`~pyglet.image.ImageData.get_image_data` will return the untransformed data. The
- transformation is applied around the anchor point.
-
- :Parameters:
- `flip_x` : bool
- If True, the returned image will be flipped horizontally.
- `flip_y` : bool
- If True, the returned image will be flipped vertically.
- `rotate` : int
- Degrees of clockwise rotation of the returned image. Only
- 90-degree increments are supported.
-
- :rtype: :py:class:`~pyglet.image.TextureRegion`
- """
- transform = self.get_region(0, 0, self.width, self.height)
- bl, br, tr, tl = 0, 1, 2, 3
- transform.anchor_x = self.anchor_x
- transform.anchor_y = self.anchor_y
- if flip_x:
- bl, br, tl, tr = br, bl, tr, tl
- transform.anchor_x = self.width - self.anchor_x
- if flip_y:
- bl, br, tl, tr = tl, tr, bl, br
- transform.anchor_y = self.height - self.anchor_y
- rotate %= 360
- if rotate < 0:
- rotate += 360
- if rotate == 0:
- pass
- elif rotate == 90:
- bl, br, tr, tl = br, tr, tl, bl
- transform.anchor_x, transform.anchor_y = transform.anchor_y, transform.width - transform.anchor_x
- elif rotate == 180:
- bl, br, tr, tl = tr, tl, bl, br
- transform.anchor_x = transform.width - transform.anchor_x
- transform.anchor_y = transform.height - transform.anchor_y
- elif rotate == 270:
- bl, br, tr, tl = tl, bl, br, tr
- transform.anchor_x, transform.anchor_y = transform.height - transform.anchor_y, transform.anchor_x
- else:
- assert False, 'Only 90 degree rotations are supported.'
- if rotate in (90, 270):
- transform.width, transform.height = transform.height, transform.width
- transform._set_tex_coords_order(bl, br, tr, tl)
- return transform
-
- def _set_tex_coords_order(self, bl, br, tr, tl):
- tex_coords = (self.tex_coords[:3],
- self.tex_coords[3:6],
- self.tex_coords[6:9],
- self.tex_coords[9:])
- self.tex_coords = tex_coords[bl] + tex_coords[br] + tex_coords[tr] + tex_coords[tl]
-
- order = self.tex_coords_order
- self.tex_coords_order = (order[bl], order[br], order[tr], order[tl])
-
- def __repr__(self):
- return "{}(id={}, size={}x{})".format(self.__class__.__name__, self.id, self.width, self.height)
-
-
-class TextureRegion(Texture):
- """A rectangular region of a texture, presented as if it were a separate texture.
- """
-
- def __init__(self, x, y, z, width, height, owner):
- super().__init__(width, height, owner.target, owner.id)
-
- self.x = x
- self.y = y
- self.z = z
- self.owner = owner
- owner_u1 = owner.tex_coords[0]
- owner_v1 = owner.tex_coords[1]
- owner_u2 = owner.tex_coords[3]
- owner_v2 = owner.tex_coords[7]
- scale_u = owner_u2 - owner_u1
- scale_v = owner_v2 - owner_v1
- u1 = x / owner.width * scale_u + owner_u1
- v1 = y / owner.height * scale_v + owner_v1
- u2 = (x + width) / owner.width * scale_u + owner_u1
- v2 = (y + height) / owner.height * scale_v + owner_v1
- r = z / owner.images + owner.tex_coords[2]
- self.tex_coords = (u1, v1, r, u2, v1, r, u2, v2, r, u1, v2, r)
-
- def get_image_data(self):
- image_data = self.owner.get_image_data(self.z)
- return image_data.get_region(self.x, self.y, self.width, self.height)
-
- def get_region(self, x, y, width, height):
- x += self.x
- y += self.y
- region = self.region_class(x, y, self.z, width, height, self.owner)
- region._set_tex_coords_order(*self.tex_coords_order)
- return region
-
- def blit_into(self, source, x, y, z):
- self.owner.blit_into(source, x + self.x, y + self.y, z + self.z)
-
- def __repr__(self):
- return "{}(id={}, size={}x{}, owner={}x{})".format(self.__class__.__name__, self.id, self.width, self.height,
- self.owner.width, self.owner.height)
-
- def __del__(self):
- # only the owner Texture should handle deletion
- pass
-
-
-Texture.region_class = TextureRegion
-
-
-class Texture3D(Texture, UniformTextureSequence):
- """A texture with more than one image slice.
-
- Use `create_for_images` or `create_for_image_grid` classmethod to
- construct.
- """
- item_width = 0
- item_height = 0
- items = ()
-
- @classmethod
- def create_for_images(cls, images, internalformat=GL_RGBA, blank_data=True):
- item_width = images[0].width
- item_height = images[0].height
- for image in images:
- if image.width != item_width or image.height != item_height:
- raise ImageException('Images do not have same dimensions.')
-
- depth = len(images)
-
- texture = cls.create(item_width, item_height, GL_TEXTURE_3D, None)
- if images[0].anchor_x or images[0].anchor_y:
- texture.anchor_x = images[0].anchor_x
- texture.anchor_y = images[0].anchor_y
-
- texture.images = depth
-
- blank = (GLubyte * (texture.width * texture.height * texture.images))() if blank_data else None
- glBindTexture(texture.target, texture.id)
- glTexImage3D(texture.target, texture.level,
- internalformat,
- texture.width, texture.height, texture.images, 0,
- GL_ALPHA, GL_UNSIGNED_BYTE,
- blank)
-
- items = []
- for i, image in enumerate(images):
- item = cls.region_class(0, 0, i, item_width, item_height, texture)
- items.append(item)
- image.blit_to_texture(texture.target, texture.level, image.anchor_x, image.anchor_y, i)
-
- glFlush()
-
- texture.items = items
- texture.item_width = item_width
- texture.item_height = item_height
- return texture
-
- @classmethod
- def create_for_image_grid(cls, grid, internalformat=GL_RGBA):
- return cls.create_for_images(grid[:], internalformat)
-
- def __len__(self):
- return len(self.items)
-
- def __getitem__(self, index):
- return self.items[index]
-
- def __setitem__(self, index, value):
- if type(index) is slice:
- for item, image in zip(self[index], value):
- image.blit_to_texture(self.target, self.level, image.anchor_x, image.anchor_y, item.z)
- else:
- value.blit_to_texture(self.target, self.level, value.anchor_x, value.anchor_y, self[index].z)
-
- def __iter__(self):
- return iter(self.items)
-
-
-class TextureArrayRegion(TextureRegion):
- """A region of a TextureArray, presented as if it were a separate texture.
- """
- def __init__(self, x, y, z, width, height, owner):
- super().__init__(width, height, owner.target, owner.id)
-
- self.x = x
- self.y = y
- self.z = z
- self.owner = owner
- owner_u1 = owner.tex_coords[0]
- owner_v1 = owner.tex_coords[1]
- owner_u2 = owner.tex_coords[3]
- owner_v2 = owner.tex_coords[7]
- scale_u = owner_u2 - owner_u1
- scale_v = owner_v2 - owner_v1
- u1 = x / owner.width * scale_u + owner_u1
- v1 = y / owner.height * scale_v + owner_v1
- u2 = (x + width) / owner.width * scale_u + owner_u1
- v2 = (y + height) / owner.height * scale_v + owner_v1
- z = float(z)
- self.tex_coords = (u1, v1, z, u2, v1, z, u2, v2, z, u1, v2, z)
-
- def __repr__(self):
- return "{}(id={}, size={}x{}, layer={})".format(self.__class__.__name__, self.id, self.width, self.height, self.z)
-
-
-class TextureArray(Texture, UniformTextureSequence):
- allow_smaller_pack = True
-
- @classmethod
- def create(cls, width, height, internalformat=GL_RGBA, min_filter=None, mag_filter=None, max_depth=256):
- """Create an empty TextureArray.
-
- You may specify the maximum depth, or layers, the Texture Array should have. This defaults
- to 256, but will be hardware and driver dependent.
-
- :Parameters:
- `width` : int
- Width of the texture.
- `height` : int
- Height of the texture.
- `internalformat` : int
- GL constant giving the internal format of the texture array; for example, ``GL_RGBA``.
- `min_filter` : int
- The minifaction filter used for this texture array, commonly ``GL_LINEAR`` or ``GL_NEAREST``
- `mag_filter` : int
- The magnification filter used for this texture array, commonly ``GL_LINEAR`` or ``GL_NEAREST``
- `max_depth` : int
- The number of layers in the texture array.
-
- :rtype: :py:class:`~pyglet.image.TextureArray`
-
- .. versionadded:: 2.0
- """
- min_filter = min_filter or cls.default_min_filter
- mag_filter = mag_filter or cls.default_mag_filter
-
- max_depth_limit = get_max_array_texture_layers()
- assert max_depth <= max_depth_limit, "TextureArray max_depth supported is {}.".format(max_depth_limit)
-
- tex_id = GLuint()
- glGenTextures(1, byref(tex_id))
- glBindTexture(GL_TEXTURE_2D_ARRAY, tex_id.value)
- glTexParameteri(GL_TEXTURE_2D_ARRAY, GL_TEXTURE_MIN_FILTER, min_filter)
- glTexParameteri(GL_TEXTURE_2D_ARRAY, GL_TEXTURE_MAG_FILTER, mag_filter)
-
- glTexImage3D(GL_TEXTURE_2D_ARRAY, 0,
- internalformat,
- width, height, max_depth,
- 0,
- internalformat, GL_UNSIGNED_BYTE,
- 0)
- glFlush()
-
- texture = cls(width, height, GL_TEXTURE_2D_ARRAY, tex_id.value)
- texture.items = [] # No items on creation
- texture.max_depth = max_depth
- texture.min_filter = min_filter
- texture.mag_filter = mag_filter
-
- return texture
-
- def _verify_size(self, image):
- if image.width > self.width or image.height > self.height:
- raise ImageException('Image ({0}x{1}) exceeds the size of the TextureArray ({2}x{3})'.format(
- image.width, image.height, self.width, self.height))
-
- def allocate(self, *images):
- if len(self.items) + len(images) > self.max_depth:
- raise Exception("The amount of images being added exceeds the depth of this TextureArray.")
-
- textures = []
- start_length = len(self.items)
- for i, image in enumerate(images):
- self._verify_size(image)
- item = self.region_class(0, 0, start_length + i, image.width, image.height, self)
- self.items.append(item)
- image.blit_to_texture(self.target, self.level, image.anchor_x, image.anchor_y, start_length + i)
-
- glFlush()
-
- return self.items[start_length:]
-
- @classmethod
- def create_for_image_grid(cls, grid, internalformat=GL_RGBA):
- texture_array = cls.create(grid[0].width, grid[0].height, internalformat, max_depth=len(grid))
- texture_array.allocate(*grid[:])
- return texture_array
-
- def __len__(self):
- return len(self.items)
-
- def __getitem__(self, index):
- return self.items[index]
-
- def __setitem__(self, index, value):
- if type(index) is slice:
- for old_item, image in zip(self[index], value):
- self._verify_size(image)
- item = self.region_class(0, 0, old_item.z, image.width, image.height, self)
- image.blit_to_texture(self.target, self.level, image.anchor_x, image.anchor_y, old_item.z)
- self.items[old_item.z] = item
- else:
- self._verify_size(value)
- item = self.region_class(0, 0, index, value.width, value.height, self)
- value.blit_to_texture(self.target, self.level, value.anchor_x, value.anchor_y, index)
- self.items[index] = item
-
- def __iter__(self):
- return iter(self.items)
-
-
-TextureArray.region_class = TextureArrayRegion
-TextureArrayRegion.region_class = TextureArrayRegion
-
-
-class TileableTexture(Texture):
- """A texture that can be tiled efficiently.
-
- Use :py:class:`~pyglet.image.create_for_image` classmethod to construct.
- """
-
- def get_region(self, x, y, width, height):
- raise ImageException('Cannot get region of %r' % self)
-
- def blit_tiled(self, x, y, z, width, height):
- """Blit this texture tiled over the given area.
-
- The image will be tiled with the bottom-left corner of the destination
- rectangle aligned with the anchor point of this texture.
- """
- u1 = self.anchor_x / self.width
- v1 = self.anchor_y / self.height
- u2 = u1 + width / self.width
- v2 = v1 + height / self.height
- w, h = width, height
- t = self.tex_coords
-
- vertices = (x, y, z,
- x + w, y, z,
- x + w, y + h, z,
- x, y + h, z)
-
- tex_coords = (u1, v1, t[2],
- u2, v1, t[5],
- u2, v2, t[8],
- u1, v2, t[11],)
-
- glActiveTexture(GL_TEXTURE0)
- glBindTexture(self.target, self.id)
- pyglet.graphics.draw_indexed(4, GL_TRIANGLES, [0, 1, 2, 0, 2, 3],
- position=('f', vertices),
- tex_coords=('f', tex_coords))
- glBindTexture(self.target, 0)
-
- @classmethod
- def create_for_image(cls, image):
- image = image.get_image_data()
- return image.create_texture(cls)
-
-
-class DepthTexture(Texture):
- """A texture with depth samples (typically 24-bit)."""
-
- def blit_into(self, source, x, y, z):
- glBindTexture(self.target, self.id)
- source.blit_to_texture(self.level, x, y, z)
-
-
-class ImageGrid(AbstractImage, AbstractImageSequence):
- """An imaginary grid placed over an image allowing easy access to
- regular regions of that image.
-
- The grid can be accessed either as a complete image, or as a sequence
- of images. The most useful applications are to access the grid
- as a :py:class:`~pyglet.image.TextureGrid`::
-
- image_grid = ImageGrid(...)
- texture_grid = image_grid.get_texture_sequence()
-
- or as a :py:class:`~pyglet.image.Texture3D`::
-
- image_grid = ImageGrid(...)
- texture_3d = Texture3D.create_for_image_grid(image_grid)
-
- """
- _items = ()
- _texture_grid = None
-
- def __init__(self, image, rows, columns, item_width=None, item_height=None, row_padding=0, column_padding=0):
- """Construct a grid for the given image.
-
- You can specify parameters for the grid, for example setting
- the padding between cells. Grids are always aligned to the
- bottom-left corner of the image.
-
- :Parameters:
- `image` : AbstractImage
- Image over which to construct the grid.
- `rows` : int
- Number of rows in the grid.
- `columns` : int
- Number of columns in the grid.
- `item_width` : int
- Width of each column. If unspecified, is calculated such
- that the entire image width is used.
- `item_height` : int
- Height of each row. If unspecified, is calculated such that
- the entire image height is used.
- `row_padding` : int
- Pixels separating adjacent rows. The padding is only
- inserted between rows, not at the edges of the grid.
- `column_padding` : int
- Pixels separating adjacent columns. The padding is only
- inserted between columns, not at the edges of the grid.
- """
- super().__init__(image.width, image.height)
- self.image = image
- self.rows = rows
- self.columns = columns
- self.item_width = item_width or (image.width - column_padding * (columns - 1)) // columns
- self.item_height = item_height or (image.height - row_padding * (rows - 1)) // rows
- self.row_padding = row_padding
- self.column_padding = column_padding
-
- def get_texture(self, rectangle=False):
- return self.image.get_texture(rectangle)
-
- def get_image_data(self):
- return self.image.get_image_data()
-
- def get_texture_sequence(self):
- if not self._texture_grid:
- self._texture_grid = TextureGrid(self)
- return self._texture_grid
-
- def __len__(self):
- return self.rows * self.columns
-
- def _update_items(self):
- if not self._items:
- self._items = []
- y = 0
- for row in range(self.rows):
- x = 0
- for col in range(self.columns):
- self._items.append(self.image.get_region(x, y, self.item_width, self.item_height))
- x += self.item_width + self.column_padding
- y += self.item_height + self.row_padding
-
- def __getitem__(self, index):
- self._update_items()
- if type(index) is tuple:
- row, column = index
- assert 0 <= row < self.rows and 0 <= column < self.columns
- return self._items[row * self.columns + column]
- else:
- return self._items[index]
-
- def __iter__(self):
- self._update_items()
- return iter(self._items)
-
-
-class TextureGrid(TextureRegion, UniformTextureSequence):
- """A texture containing a regular grid of texture regions.
-
- To construct, create an :py:class:`~pyglet.image.ImageGrid` first::
-
- image_grid = ImageGrid(...)
- texture_grid = TextureGrid(image_grid)
-
- The texture grid can be accessed as a single texture, or as a sequence
- of :py:class:`~pyglet.image.TextureRegion`. When accessing as a sequence, you can specify
- integer indexes, in which the images are arranged in rows from the
- bottom-left to the top-right::
-
- # assume the texture_grid is 3x3:
- current_texture = texture_grid[3] # get the middle-left image
-
- You can also specify tuples in the sequence methods, which are addressed
- as ``row, column``::
-
- # equivalent to the previous example:
- current_texture = texture_grid[1, 0]
-
- When using tuples in a slice, the returned sequence is over the
- rectangular region defined by the slice::
-
- # returns center, center-right, center-top, top-right images in that
- # order:
- images = texture_grid[(1,1):]
- # equivalent to
- images = texture_grid[(1,1):(3,3)]
-
- """
- items = ()
- rows = 1
- columns = 1
- item_width = 0
- item_height = 0
-
- def __init__(self, grid):
- image = grid.get_texture()
- if isinstance(image, TextureRegion):
- owner = image.owner
- else:
- owner = image
-
- super().__init__(image.x, image.y, image.z, image.width, image.height, owner)
-
- items = []
- y = 0
- for row in range(grid.rows):
- x = 0
- for col in range(grid.columns):
- items.append(self.get_region(x, y, grid.item_width, grid.item_height))
- x += grid.item_width + grid.column_padding
- y += grid.item_height + grid.row_padding
-
- self.items = items
- self.rows = grid.rows
- self.columns = grid.columns
- self.item_width = grid.item_width
- self.item_height = grid.item_height
-
- def get(self, row, column):
- return self[(row, column)]
-
- def __getitem__(self, index):
- if type(index) is slice:
- if type(index.start) is not tuple and type(index.stop) is not tuple:
- return self.items[index]
- else:
- row1 = 0
- col1 = 0
- row2 = self.rows
- col2 = self.columns
- if type(index.start) is tuple:
- row1, col1 = index.start
- elif type(index.start) is int:
- row1 = index.start // self.columns
- col1 = index.start % self.columns
- assert 0 <= row1 < self.rows and 0 <= col1 < self.columns
-
- if type(index.stop) is tuple:
- row2, col2 = index.stop
- elif type(index.stop) is int:
- row2 = index.stop // self.columns
- col2 = index.stop % self.columns
- assert 0 <= row2 <= self.rows and 0 <= col2 <= self.columns
-
- result = []
- i = row1 * self.columns
- for row in range(row1, row2):
- result += self.items[i + col1:i + col2]
- i += self.columns
- return result
- else:
- if type(index) is tuple:
- row, column = index
- assert 0 <= row < self.rows and 0 <= column < self.columns
- return self.items[row * self.columns + column]
- elif type(index) is int:
- return self.items[index]
-
- def __setitem__(self, index, value):
- if type(index) is slice:
- for region, image in zip(self[index], value):
- if image.width != self.item_width or image.height != self.item_height:
- raise ImageException('Image has incorrect dimensions')
- image.blit_into(region, image.anchor_x, image.anchor_y, 0)
- else:
- image = value
- if image.width != self.item_width or image.height != self.item_height:
- raise ImageException('Image has incorrect dimensions')
- image.blit_into(self[index], image.anchor_x, image.anchor_y, 0)
-
- def __len__(self):
- return len(self.items)
-
- def __iter__(self):
- return iter(self.items)
-
-
-# Initialise default codecs
-_add_default_codecs()
-
-# Default Framebuffer classes:
-###############################################################
-
-
-class BufferManager:
- """Manages the set of framebuffers for a context.
-
- Use :py:func:`~pyglet.image.get_buffer_manager` to obtain the instance of this class for the
- current context.
- """
-
- def __init__(self):
- self.color_buffer = None
- self.depth_buffer = None
- self.free_stencil_bits = None
- self.refs = []
-
- @staticmethod
- def get_viewport():
- """Get the current OpenGL viewport dimensions.
-
- :rtype: 4-tuple of float.
- :return: Left, top, right and bottom dimensions.
- """
- viewport = (GLint * 4)()
- glGetIntegerv(GL_VIEWPORT, viewport)
- return viewport
-
- def get_color_buffer(self):
- """Get the color buffer.
-
- :rtype: :py:class:`~pyglet.image.ColorBufferImage`
- """
- viewport = self.get_viewport()
- viewport_width = viewport[2]
- viewport_height = viewport[3]
- if (not self.color_buffer or
- viewport_width != self.color_buffer.width or
- viewport_height != self.color_buffer.height):
- self.color_buffer = ColorBufferImage(*viewport)
- return self.color_buffer
-
- def get_depth_buffer(self):
- """Get the depth buffer.
-
- :rtype: :py:class:`~pyglet.image.DepthBufferImage`
- """
- viewport = self.get_viewport()
- viewport_width = viewport[2]
- viewport_height = viewport[3]
- if (not self.depth_buffer or
- viewport_width != self.depth_buffer.width or
- viewport_height != self.depth_buffer.height):
- self.depth_buffer = DepthBufferImage(*viewport)
- return self.depth_buffer
-
- def get_buffer_mask(self):
- """Get a free bitmask buffer.
-
- A bitmask buffer is a buffer referencing a single bit in the stencil
- buffer. If no bits are free, `ImageException` is raised. Bits are
- released when the bitmask buffer is garbage collected.
-
- :rtype: :py:class:`~pyglet.image.BufferImageMask`
- """
- if self.free_stencil_bits is None:
- try:
- stencil_bits = GLint()
- glGetFramebufferAttachmentParameteriv(GL_DRAW_FRAMEBUFFER,
- GL_STENCIL,
- GL_FRAMEBUFFER_ATTACHMENT_STENCIL_SIZE,
- stencil_bits)
- self.free_stencil_bits = list(range(stencil_bits.value))
- except GLException:
- pass
-
- if not self.free_stencil_bits:
- raise ImageException('No free stencil bits are available.')
-
- stencil_bit = self.free_stencil_bits.pop(0)
- x, y, width, height = self.get_viewport()
- bufimg = BufferImageMask(x, y, width, height)
- bufimg.stencil_bit = stencil_bit
-
- def release_buffer(ref, owner=self):
- owner.free_stencil_bits.insert(0, stencil_bit)
-
- self.refs.append(weakref.ref(bufimg, release_buffer))
-
- return bufimg
-
-
-def get_buffer_manager():
- """Get the buffer manager for the current OpenGL context.
-
- :rtype: :py:class:`~pyglet.image.BufferManager`
- """
- context = pyglet.gl.current_context
- if not hasattr(context, 'image_buffer_manager'):
- context.image_buffer_manager = BufferManager()
- return context.image_buffer_manager
-
-
-class BufferImage(AbstractImage):
- """An abstract framebuffer.
- """
- #: The OpenGL read and write target for this buffer.
- gl_buffer = GL_BACK
-
- #: The OpenGL format constant for image data.
- gl_format = 0
-
- #: The format string used for image data.
- format = ''
-
- owner = None
-
- # TODO: enable methods
-
- def __init__(self, x, y, width, height):
- super().__init__(width, height)
- self.x = x
- self.y = y
- self.width = width
- self.height = height
-
- def get_image_data(self):
- buf = (GLubyte * (len(self.format) * self.width * self.height))()
-
- x = self.x
- y = self.y
- if self.owner:
- x += self.owner.x
- y += self.owner.y
-
- glReadBuffer(self.gl_buffer)
- glPixelStorei(GL_PACK_ALIGNMENT, 1)
- glReadPixels(x, y, self.width, self.height, self.gl_format, GL_UNSIGNED_BYTE, buf)
- return ImageData(self.width, self.height, self.format, buf)
-
- def get_region(self, x, y, width, height):
- if self.owner:
- return self.owner.get_region(x + self.x, y + self.y, width, height)
-
- region = self.__class__(x + self.x, y + self.y, width, height)
- region.gl_buffer = self.gl_buffer
- region.owner = self
- return region
-
-
-class ColorBufferImage(BufferImage):
- """A color framebuffer.
-
- This class is used to wrap the primary color buffer (i.e., the back
- buffer)
- """
- gl_format = GL_RGBA
- format = 'RGBA'
-
- def get_texture(self, rectangle=False):
- texture = Texture.create(self.width, self.height, GL_TEXTURE_2D, GL_RGBA, blank_data=False)
- self.blit_to_texture(texture.target, texture.level, self.anchor_x, self.anchor_y, 0)
- return texture
-
- def blit_to_texture(self, target, level, x, y, z):
- glReadBuffer(self.gl_buffer)
- glCopyTexSubImage2D(target, level, x-self.anchor_x, y-self.anchor_y, self.x, self.y, self.width, self.height)
-
-
-class DepthBufferImage(BufferImage):
- """The depth buffer.
- """
- gl_format = GL_DEPTH_COMPONENT
- format = 'L'
-
- def get_texture(self, rectangle=False):
- assert rectangle is False, 'Depth textures cannot be rectangular'
-
- texture = DepthTexture.create(self.width, self.height, GL_TEXTURE_2D, None)
- if self.anchor_x or self.anchor_y:
- texture.anchor_x = self.anchor_x
- texture.anchor_y = self.anchor_y
-
- glReadBuffer(self.gl_buffer)
- glCopyTexImage2D(texture.target, 0,
- GL_DEPTH_COMPONENT,
- self.x, self.y, self.width, self.height,
- 0)
- return texture
-
- def blit_to_texture(self, target, level, x, y, z):
- glReadBuffer(self.gl_buffer)
- glCopyTexSubImage2D(target, level, x-self.anchor_x, y-self.anchor_y, self.x, self.y, self.width, self.height)
-
-
-class BufferImageMask(BufferImage):
- """A single bit of the stencil buffer.
- """
- gl_format = GL_STENCIL_INDEX
- format = 'L'
-
- # TODO mask methods
diff --git a/spaces/abrar-lohia/text-2-character-anim/pyrender/setup.py b/spaces/abrar-lohia/text-2-character-anim/pyrender/setup.py
deleted file mode 100644
index 9a11b5c2d32983cd3c240597a504995dfb439af4..0000000000000000000000000000000000000000
--- a/spaces/abrar-lohia/text-2-character-anim/pyrender/setup.py
+++ /dev/null
@@ -1,76 +0,0 @@
-"""
-Setup of pyrender Python codebase.
-
-Author: Matthew Matl
-"""
-import sys
-from setuptools import setup
-
-# load __version__
-exec(open('pyrender/version.py').read())
-
-def get_imageio_dep():
- if sys.version[0] == "2":
- return 'imageio<=2.6.1'
- return 'imageio'
-
-requirements = [
- 'freetype-py', # For font loading
- get_imageio_dep(), # For Image I/O
- 'networkx', # For the scene graph
- 'numpy', # Numpy
- 'Pillow', # For Trimesh texture conversions
- 'pyglet>=1.5.24', # For the pyglet viewer
- 'PyOpenGL~=3.1.0', # For OpenGL
- 'PyOpenGL_accelerate~=3.1.0', # For OpenGL
- 'scipy', # Because of trimesh missing dep
- 'six', # For Python 2/3 interop
- 'trimesh', # For meshes
-]
-
-dev_requirements = [
- 'flake8', # Code formatting checker
- 'pre-commit', # Pre-commit hooks
- 'pytest', # Code testing
- 'pytest-cov', # Coverage testing
- 'tox', # Automatic virtualenv testing
-]
-
-docs_requirements = [
- 'sphinx', # General doc library
- 'sphinx_rtd_theme', # RTD theme for sphinx
- 'sphinx-automodapi' # For generating nice tables
-]
-
-
-setup(
- name = 'pyrender',
- version=__version__,
- description='Easy-to-use Python renderer for 3D visualization',
- long_description='A simple implementation of Physically-Based Rendering '
- '(PBR) in Python. Compliant with the glTF 2.0 standard.',
- author='Matthew Matl',
- author_email='matthewcmatl@gmail.com',
- license='MIT License',
- url = 'https://github.com/mmatl/pyrender',
- classifiers = [
- 'Development Status :: 4 - Beta',
- 'License :: OSI Approved :: MIT License',
- 'Operating System :: POSIX :: Linux',
- 'Operating System :: MacOS :: MacOS X',
- 'Programming Language :: Python :: 2.7',
- 'Programming Language :: Python :: 3.5',
- 'Programming Language :: Python :: 3.6',
- 'Natural Language :: English',
- 'Topic :: Scientific/Engineering'
- ],
- keywords = 'rendering graphics opengl 3d visualization pbr gltf',
- packages = ['pyrender', 'pyrender.platforms'],
- setup_requires = requirements,
- install_requires = requirements,
- extras_require={
- 'dev': dev_requirements,
- 'docs': docs_requirements,
- },
- include_package_data=True
-)
diff --git a/spaces/aijack/jojo/e4e/README.md b/spaces/aijack/jojo/e4e/README.md
deleted file mode 100644
index 14b6bc701b2bad3c2fc7b1d9b36f1892681ded5f..0000000000000000000000000000000000000000
--- a/spaces/aijack/jojo/e4e/README.md
+++ /dev/null
@@ -1,142 +0,0 @@
-# Designing an Encoder for StyleGAN Image Manipulation
-
-
- [](http://colab.research.google.com/github/omertov/encoder4editing/blob/main/notebooks/inference_playground.ipynb)
-
-> Recently, there has been a surge of diverse methods for performing image editing by employing pre-trained unconditional generators. Applying these methods on real images, however, remains a challenge, as it necessarily requires the inversion of the images into their latent space. To successfully invert a real image, one needs to find a latent code that reconstructs the input image accurately, and more importantly, allows for its meaningful manipulation. In this paper, we carefully study the latent space of StyleGAN, the state-of-the-art unconditional generator. We identify and analyze the existence of a distortion-editability tradeoff and a distortion-perception tradeoff within the StyleGAN latent space. We then suggest two principles for designing encoders in a manner that allows one to control the proximity of the inversions to regions that StyleGAN was originally trained on. We present an encoder based on our two principles that is specifically designed for facilitating editing on real images by balancing these tradeoffs. By evaluating its performance qualitatively and quantitatively on numerous challenging domains, including cars and horses, we show that our inversion method, followed by common editing techniques, achieves superior real-image editing quality, with only a small reconstruction accuracy drop.
-
-
-
-
-
-## Description
-Official Implementation of "Designing an Encoder for StyleGAN Image Manipulation" paper for both training and evaluation.
-The e4e encoder is specifically designed to complement existing image manipulation techniques performed over StyleGAN's latent space.
-
-## Recent Updates
-`2021.03.25`: Add pose editing direction.
-
-## Getting Started
-### Prerequisites
-- Linux or macOS
-- NVIDIA GPU + CUDA CuDNN (CPU may be possible with some modifications, but is not inherently supported)
-- Python 3
-
-### Installation
-- Clone the repository:
-```
-git clone https://github.com/omertov/encoder4editing.git
-cd encoder4editing
-```
-- Dependencies:
-We recommend running this repository using [Anaconda](https://docs.anaconda.com/anaconda/install/).
-All dependencies for defining the environment are provided in `environment/e4e_env.yaml`.
-
-### Inference Notebook
-We provide a Jupyter notebook found in `notebooks/inference_playground.ipynb` that allows one to encode and perform several editings on real images using StyleGAN.
-
-### Pretrained Models
-Please download the pre-trained models from the following links. Each e4e model contains the entire pSp framework architecture, including the encoder and decoder weights.
-| Path | Description
-| :--- | :----------
-|[FFHQ Inversion](https://drive.google.com/file/d/1cUv_reLE6k3604or78EranS7XzuVMWeO/view?usp=sharing) | FFHQ e4e encoder.
-|[Cars Inversion](https://drive.google.com/file/d/17faPqBce2m1AQeLCLHUVXaDfxMRU2QcV/view?usp=sharing) | Cars e4e encoder.
-|[Horse Inversion](https://drive.google.com/file/d/1TkLLnuX86B_BMo2ocYD0kX9kWh53rUVX/view?usp=sharing) | Horse e4e encoder.
-|[Church Inversion](https://drive.google.com/file/d/1-L0ZdnQLwtdy6-A_Ccgq5uNJGTqE7qBa/view?usp=sharing) | Church e4e encoder.
-
-If you wish to use one of the pretrained models for training or inference, you may do so using the flag `--checkpoint_path`.
-
-In addition, we provide various auxiliary models needed for training your own e4e model from scratch.
-| Path | Description
-| :--- | :----------
-|[FFHQ StyleGAN](https://drive.google.com/file/d/1EM87UquaoQmk17Q8d5kYIAHqu0dkYqdT/view?usp=sharing) | StyleGAN model pretrained on FFHQ taken from [rosinality](https://github.com/rosinality/stylegan2-pytorch) with 1024x1024 output resolution.
-|[IR-SE50 Model](https://drive.google.com/file/d/1KW7bjndL3QG3sxBbZxreGHigcCCpsDgn/view?usp=sharing) | Pretrained IR-SE50 model taken from [TreB1eN](https://github.com/TreB1eN/InsightFace_Pytorch) for use in our ID loss during training.
-|[MOCOv2 Model](https://drive.google.com/file/d/18rLcNGdteX5LwT7sv_F7HWr12HpVEzVe/view?usp=sharing) | Pretrained ResNet-50 model trained using MOCOv2 for use in our simmilarity loss for domains other then human faces during training.
-
-By default, we assume that all auxiliary models are downloaded and saved to the directory `pretrained_models`. However, you may use your own paths by changing the necessary values in `configs/path_configs.py`.
-
-## Training
-To train the e4e encoder, make sure the paths to the required models, as well as training and testing data is configured in `configs/path_configs.py` and `configs/data_configs.py`.
-#### **Training the e4e Encoder**
-```
-python scripts/train.py \
---dataset_type cars_encode \
---exp_dir new/experiment/directory \
---start_from_latent_avg \
---use_w_pool \
---w_discriminator_lambda 0.1 \
---progressive_start 20000 \
---id_lambda 0.5 \
---val_interval 10000 \
---max_steps 200000 \
---stylegan_size 512 \
---stylegan_weights path/to/pretrained/stylegan.pt \
---workers 8 \
---batch_size 8 \
---test_batch_size 4 \
---test_workers 4
-```
-
-#### Training on your own dataset
-In order to train the e4e encoder on a custom dataset, perform the following adjustments:
-1. Insert the paths to your train and test data into the `dataset_paths` variable defined in `configs/paths_config.py`:
-```
-dataset_paths = {
- 'my_train_data': '/path/to/train/images/directory',
- 'my_test_data': '/path/to/test/images/directory'
-}
-```
-2. Configure a new dataset under the DATASETS variable defined in `configs/data_configs.py`:
-```
-DATASETS = {
- 'my_data_encode': {
- 'transforms': transforms_config.EncodeTransforms,
- 'train_source_root': dataset_paths['my_train_data'],
- 'train_target_root': dataset_paths['my_train_data'],
- 'test_source_root': dataset_paths['my_test_data'],
- 'test_target_root': dataset_paths['my_test_data']
- }
-}
-```
-Refer to `configs/transforms_config.py` for the transformations applied to the train and test images during training.
-
-3. Finally, run a training session with `--dataset_type my_data_encode`.
-
-## Inference
-Having trained your model, you can use `scripts/inference.py` to apply the model on a set of images.
-For example,
-```
-python scripts/inference.py \
---images_dir=/path/to/images/directory \
---save_dir=/path/to/saving/directory \
-path/to/checkpoint.pt
-```
-
-## Latent Editing Consistency (LEC)
-As described in the paper, we suggest a new metric, Latent Editing Consistency (LEC), for evaluating the encoder's
-performance.
-We provide an example for calculating the metric over the FFHQ StyleGAN using the aging editing direction in
-`metrics/LEC.py`.
-
-To run the example:
-```
-cd metrics
-python LEC.py \
---images_dir=/path/to/images/directory \
-path/to/checkpoint.pt
-```
-
-## Acknowledgments
-This code borrows heavily from [pixel2style2pixel](https://github.com/eladrich/pixel2style2pixel)
-
-## Citation
-If you use this code for your research, please cite our paper Designing an Encoder for StyleGAN Image Manipulation:
-
-```
-@article{tov2021designing,
- title={Designing an Encoder for StyleGAN Image Manipulation},
- author={Tov, Omer and Alaluf, Yuval and Nitzan, Yotam and Patashnik, Or and Cohen-Or, Daniel},
- journal={arXiv preprint arXiv:2102.02766},
- year={2021}
-}
-```
diff --git a/spaces/aijack/jojo/e4e/editings/ganspace.py b/spaces/aijack/jojo/e4e/editings/ganspace.py
deleted file mode 100644
index 0c286a421280c542e9776a75e64bb65409da8fc7..0000000000000000000000000000000000000000
--- a/spaces/aijack/jojo/e4e/editings/ganspace.py
+++ /dev/null
@@ -1,22 +0,0 @@
-import torch
-
-
-def edit(latents, pca, edit_directions):
- edit_latents = []
- for latent in latents:
- for pca_idx, start, end, strength in edit_directions:
- delta = get_delta(pca, latent, pca_idx, strength)
- delta_padded = torch.zeros(latent.shape).to('cuda')
- delta_padded[start:end] += delta.repeat(end - start, 1)
- edit_latents.append(latent + delta_padded)
- return torch.stack(edit_latents)
-
-
-def get_delta(pca, latent, idx, strength):
- # pca: ganspace checkpoint. latent: (16, 512) w+
- w_centered = latent - pca['mean'].to('cuda')
- lat_comp = pca['comp'].to('cuda')
- lat_std = pca['std'].to('cuda')
- w_coord = torch.sum(w_centered[0].reshape(-1)*lat_comp[idx].reshape(-1)) / lat_std[idx]
- delta = (strength - w_coord)*lat_comp[idx]*lat_std[idx]
- return delta
diff --git a/spaces/akhaliq/Deit/app.py b/spaces/akhaliq/Deit/app.py
deleted file mode 100644
index a4ae366e2df23e7efa5daa2c7acdcaa75a057ce3..0000000000000000000000000000000000000000
--- a/spaces/akhaliq/Deit/app.py
+++ /dev/null
@@ -1,59 +0,0 @@
-from PIL import Image
-
-import torch
-import timm
-import torchvision
-import torchvision.transforms as T
-
-from timm.data.constants import IMAGENET_DEFAULT_MEAN, IMAGENET_DEFAULT_STD
-import gradio as gr
-
-torch.set_grad_enabled(False);
-
-with open("imagenet_classes.txt", "r") as f:
- imagenet_categories = [s.strip() for s in f.readlines()]
-
-transform = T.Compose([
- T.Resize(256, interpolation=3),
- T.CenterCrop(224),
- T.ToTensor(),
- T.Normalize(IMAGENET_DEFAULT_MEAN, IMAGENET_DEFAULT_STD),
-])
-
-model = torch.hub.load('facebookresearch/deit:main', 'deit_base_patch16_224', pretrained=True)
-
-def detr(im):
- img = transform(im).unsqueeze(0)
-
- # compute the predictions
- out = model(img)
-
- # and convert them into probabilities
- scores = torch.nn.functional.softmax(out, dim=-1)[0]
-
- # finally get the index of the prediction with highest score
- topk_scores, topk_label = torch.topk(scores, k=5, dim=-1)
-
-
- d = {}
- for i in range(5):
- pred_name = imagenet_categories[topk_label[i]]
- pred_name = f"{pred_name:<25}"
- score = topk_scores[i]
- score = f"{score:.3f}"
- d[pred_name] = score
- return d
-
-inputs = gr.inputs.Image(type='pil', label="Original Image")
-outputs = gr.outputs.Label(type="confidences",num_top_classes=5)
-
-title = "Deit"
-description = "Gradio demo for Facebook DeiT: Data-efficient Image Transformers. To use it, simply upload your image, or click one of the examples to load them. Read more at the links below."
-article = "
-
-George Zamfira's albums and discography at AllMusic, including all information about the best, old and new albums. All songs by Gheorghe Zamfira, including all albums, songs, lyrics, etc. etc. are presented here.
-All lyrics at AllMusic.
-All songs by Gheorghe Zamfira
-Hymns of life: music for the new film by Luis Buñuel
-Louis Bunuel is one of the most prominent representatives of world surrealism, the creator of such famous films as "Andalusian Dog", "Golden Age", "Milky Way" and others.
-He died quite recently - in August 2009.
-His work has not become outdated and has not lost its relevance. 8a78ff9644
-
-
-
diff --git a/spaces/bofenghuang/speech-to-text/run_demo_layout.py b/spaces/bofenghuang/speech-to-text/run_demo_layout.py
deleted file mode 100644
index e371a0a289f07e5b3a7212ebda9e111f7b0722a3..0000000000000000000000000000000000000000
--- a/spaces/bofenghuang/speech-to-text/run_demo_layout.py
+++ /dev/null
@@ -1,314 +0,0 @@
-#! /usr/bin/env python
-# coding=utf-8
-# Copyright 2023 Bofeng Huang
-
-import datetime
-import logging
-import os
-import re
-import warnings
-
-import gradio as gr
-import librosa
-
-# import nltk
-import pandas as pd
-import psutil
-import pytube as pt
-import torch
-
-# import torchaudio
-from transformers import pipeline, Wav2Vec2ProcessorWithLM, AutoModelForCTC
-from transformers.utils.logging import disable_progress_bar
-
-# nltk.download("punkt")
-# from nltk.tokenize import sent_tokenize
-
-warnings.filterwarnings("ignore")
-disable_progress_bar()
-
-DEFAULT_MODEL_NAME = "bofenghuang/asr-wav2vec2-ctc-french"
-SAMPLE_RATE = 16_000
-
-GEN_KWARGS = {
- "chunk_length_s": 30,
- "stride_length_s": 5,
-}
-
-logging.basicConfig(
- format="%(asctime)s [%(levelname)s] [%(name)s] %(message)s",
- datefmt="%Y-%m-%dT%H:%M:%SZ",
-)
-logger = logging.getLogger(__name__)
-logger.setLevel(logging.DEBUG)
-
-# device = 0 if torch.cuda.is_available() else "cpu"
-device = torch.device("cuda:0" if torch.cuda.is_available() else "cpu")
-logger.info(f"Model will be loaded on device `{device}`")
-
-cached_models = {}
-
-
-def _return_yt_html_embed(yt_url):
- video_id = yt_url.split("?v=")[-1]
- HTML_str = (
- f'
'
- "
"
- )
- return HTML_str
-
-
-def download_audio_from_youtube(yt_url, downloaded_filename="audio.wav"):
- yt = pt.YouTube(yt_url)
- stream = yt.streams.filter(only_audio=True)[0]
- # stream.download(filename="audio.mp3")
- stream.download(filename=downloaded_filename)
- return downloaded_filename
-
-
-def download_video_from_youtube(yt_url, downloaded_filename="video.mp4"):
- yt = pt.YouTube(yt_url)
- stream = yt.streams.filter(progressive=True, file_extension="mp4").order_by("resolution").desc().first()
- stream.download(filename=downloaded_filename)
- logger.info(f"Download YouTube video from {yt_url}")
- return downloaded_filename
-
-
-def _print_memory_info():
- memory = psutil.virtual_memory()
- logger.info(
- f"Memory info - Free: {memory.available / (1024 ** 3):.2f} Gb, used: {memory.percent}%, total: {memory.total / (1024 ** 3):.2f} Gb"
- )
-
-
-def _print_cuda_memory_info():
- used_mem, tot_mem = torch.cuda.mem_get_info()
- logger.info(
- f"CUDA memory info - Free: {used_mem / 1024 ** 3:.2f} Gb, used: {(tot_mem - used_mem) / 1024 ** 3:.2f} Gb, total: {tot_mem / 1024 ** 3:.2f} Gb"
- )
-
-
-def print_memory_info():
- _print_memory_info()
- if torch.cuda.is_available():
- _print_cuda_memory_info()
-
-
-def maybe_load_cached_pipeline(model_name):
- model = cached_models.get(model_name)
- if model is None:
- pipe = pipeline(model=model_name, device=device)
-
- # model = AutoModelForCTC.from_pretrained(model_name).to(device)
- # processor = Wav2Vec2ProcessorWithLM.from_pretrained(model_name)
- # pipe = pipeline(
- # "automatic-speech-recognition",
- # model=model,
- # tokenizer=processor.tokenizer,
- # feature_extractor=processor.feature_extractor,
- # decoder=processor.decoder,
- # )
-
- logger.info(f"`{model_name}` has been loaded on device `{device}`")
-
- print_memory_info()
-
- cached_models[model_name] = pipe
- return model
-
-
-def process_audio_file(audio_file):
- # waveform, sample_rate = torchaudio.load(audio_file)
- # waveform = waveform.squeeze(axis=0) # mono
- # # resample
- # if sample_rate != SAMPLE_RATE:
- # resampler = torchaudio.transforms.Resample(sample_rate, SAMPLE_RATE)
- # waveform = resampler(waveform)
-
- waveform, sample_rate = librosa.load(audio_file, mono=True)
-
- # resample
- if sample_rate != SAMPLE_RATE:
- waveform = librosa.resample(waveform, orig_sr=sample_rate, target_sr=SAMPLE_RATE)
-
- return waveform
-
-
-def infer(model, filename, return_df=False):
- audio_data = process_audio_file(filename)
-
- text = model(audio_data, **GEN_KWARGS)["text"]
-
- if return_df:
- # return pd.DataFrame({"text": sent_tokenize(text)})
- return pd.DataFrame({"text": [text]})
- else:
- return text
-
-
-def transcribe(microphone, file_upload, model_name=DEFAULT_MODEL_NAME):
- warn_output = ""
- if (microphone is not None) and (file_upload is not None):
- warn_output = (
- "WARNING: You've uploaded an audio file and used the microphone. "
- "The recorded file from the microphone will be used and the uploaded audio will be discarded.\n"
- )
-
- elif (microphone is None) and (file_upload is None):
- return "ERROR: You have to either use the microphone or upload an audio file"
-
- file = microphone if microphone is not None else file_upload
-
- model = maybe_load_cached_pipeline(model_name)
- text = infer(model, file, return_df=True)
-
- logger.info(f'Transcription by `{model_name}`:\n{text.to_json(orient="index", force_ascii=False, indent=2)}\n')
-
- # return warn_output + text
- return text
-
-
-def yt_transcribe(yt_url, model_name=DEFAULT_MODEL_NAME):
- # html_embed_str = _return_yt_html_embed(yt_url)
- audio_file_path = download_audio_from_youtube(yt_url)
-
- model = maybe_load_cached_pipeline(model_name)
- text = infer(model, audio_file_path, return_df=True)
-
- logger.info(
- f'Transcription by `{model_name}` of "{yt_url}":\n{text.to_json(orient="index", force_ascii=False, indent=2)}\n'
- )
-
- # return html_embed_str, text
- return text
-
-
-def video_transcribe(video_file_path, model_name=DEFAULT_MODEL_NAME):
- if video_file_path is None:
- raise ValueError("Failed to transcribe video as no video_file_path has been defined")
-
- audio_file_path = re.sub(r"\.mp4$", ".wav", video_file_path)
- os.system(
- f'ffmpeg -hide_banner -loglevel error -y -i "{video_file_path}" -ar 16000 -ac 1 -c:a pcm_s16le "{audio_file_path}"'
- )
-
- model = maybe_load_cached_pipeline(model_name)
- text = infer(model, audio_file_path, return_df=True)
-
- logger.info(f'Transcription by `{model_name}`:\n{text.to_json(orient="index", force_ascii=False, indent=2)}\n')
-
- return text
-
-
-# load default model
-maybe_load_cached_pipeline(DEFAULT_MODEL_NAME)
-
-# default_text_output_df = pd.DataFrame(columns=["start", "end", "text"])
-default_text_output_df = pd.DataFrame(columns=["text"])
-
-with gr.Blocks() as demo:
- with gr.Tab("Transcribe Audio"):
- gr.Markdown(
- f"""
-
-
Speech-to-Text in French: Transcribe Audio
-
- Transcribe long-form microphone or audio inputs!
-
- Demo uses the fine-tuned wav2vec2 model {DEFAULT_MODEL_NAME} and 🤗 Transformers to transcribe audio files of arbitrary length.
-
- To achieve improved accuracy and well-punctuated text, please use the [Whisper demo](https://huggingface.co/spaces/bofenghuang/whisper-demo-french).
- """
- )
-
- microphone_input = gr.inputs.Audio(source="microphone", type="filepath", label="Record", optional=True)
- upload_input = gr.inputs.Audio(source="upload", type="filepath", label="Upload File", optional=True)
- # with_timestamps_input = gr.Checkbox(label="With timestamps?")
-
- microphone_transcribe_btn = gr.Button("Transcribe Audio")
-
- # gr.Markdown('''
- # Here you will get generated transcrit.
- # ''')
-
- # microphone_text_output = gr.outputs.Textbox(label="Transcription")
- text_output_df2 = gr.DataFrame(
- value=default_text_output_df,
- label="Transcription",
- row_count=(0, "dynamic"),
- max_rows=10,
- wrap=True,
- overflow_row_behaviour="paginate",
- )
-
- microphone_transcribe_btn.click(transcribe, inputs=[microphone_input, upload_input], outputs=text_output_df2)
-
- # with gr.Tab("Transcribe YouTube"):
- # gr.Markdown(
- # f"""
- #
-
- aaccfb2cb3
-
-
-
diff --git a/spaces/cihyFjudo/fairness-paper-search/Finite Element Analysis Senthil Pdf Downloadl A Step-by-Step Tutorial on FEM.md b/spaces/cihyFjudo/fairness-paper-search/Finite Element Analysis Senthil Pdf Downloadl A Step-by-Step Tutorial on FEM.md
deleted file mode 100644
index 35171a4e9131486150a1fa117f9aff5d2ab61563..0000000000000000000000000000000000000000
--- a/spaces/cihyFjudo/fairness-paper-search/Finite Element Analysis Senthil Pdf Downloadl A Step-by-Step Tutorial on FEM.md
+++ /dev/null
@@ -1,6 +0,0 @@
-
-
This book also has exercises! The text material evolved from over 50 years of combined teaching experience it deals with a formulation and application of the finite element method. A meaningful course can be constructed from a subset of the chapters in this book for a quarter course; instructions for such use are given in the preface. The course material is organized in three chronological units of one month each:
This book provides a simple, basic approach to the course material that can be understood by both undergraduate and graduate students without the usual prerequisites (i.e. structural analysis). The book is written primarily as a basic learning tool for the undergraduate student in civil and mechanical engineering whose main interest is in stress analysis and heat transfer. The text is geared toward those who want to apply the finite element method as a tool to solve practical physical problems.
aaccfb2cb3
-
-
\ No newline at end of file
diff --git a/spaces/cihyFjudo/fairness-paper-search/Realterm Spy Driver Download A Comprehensive Manual for Realterm Users.md b/spaces/cihyFjudo/fairness-paper-search/Realterm Spy Driver Download A Comprehensive Manual for Realterm Users.md
deleted file mode 100644
index 66d1937b94d94380db8f32cadd9c031a3d766558..0000000000000000000000000000000000000000
--- a/spaces/cihyFjudo/fairness-paper-search/Realterm Spy Driver Download A Comprehensive Manual for Realterm Users.md
+++ /dev/null
@@ -1,15 +0,0 @@
-
-
Thought I would post a follow-up to see if anyone has heard anything from the developer on this. I just donated to support this development. Unfortunately, after donating and you're redirected to the driver download page, you learn at that moment that it only supports 32 bit. Any word on a 64-bit version? I've seen several related posts going back at least a few years and most of them seem to have been ignored by the developer.
I received my drivers and installed them successfully. However, now I get the message that they are not properly installed, even after a reinstall. All I can imagine now is that it is related to having updated RealTerm or to running with reduced (non-admin) permissions. Any ideas?
-
Haven't heard back. Sad because it appears the author is actively monitoring this site. If the drivers are not available anymore, then a new stable release without the donate/get drivers popup should be made the default download.
-
EOBD-Facile The people that brought us the iOS version of EOBD-Facile now offer a Mac OSX version. The free download allows you to read trouble codes, while the paid version offers full functionality. See the Outils OBD Facile YouTube page for additional info.
-
Movi and Movi Pro Only for the Mac, there are two versions of this software. The standard version provides all the basic OBD functions, while Movi Pro adds more bells and whistles. If you just want to try it out, the download will operate in demo mode.
-
Tera Term is a free software utility offered by the current development team: Tera Term Project. The free download package is lightweight and does not require a lot of storage space on PC computers and laptops.
-
Both the old releases and the latest updates are available to download and install. The developers update the software on a relatively consistent basis. You can visit their home page in your website browser to view their most recent software update, privacy policy, copyright terms, etc.
-
-
The devicetree configuration allows you to use a minimal pin configuration.Connect all unused pins to the fixed logic level as instructed in the official documentation.For example, csd-gpios is an optional pin that sets the device into sleep mode.If this pin is not controlled by the driver, it must be connected to the fixed logic level.
-
On the nRF5340 development kit, the physical UART interface of the application core is used for communication with the tester device.This sample uses the IPC UART driver for sending responses and receiving commands through the UART interface of the application core.
-
make sure you have the FDTI drivers installed make sure you have connected the buspirate and the red led is lit if you were on preorder 2 make sure you have uploaded the firmware see this for more information -pirate-firmware-upgrades/ and =100.0
aaccfb2cb3
-
-
\ No newline at end of file
diff --git a/spaces/cleanmaster/akagi-sovits3/vdecoder/__init__.py b/spaces/cleanmaster/akagi-sovits3/vdecoder/__init__.py
deleted file mode 100644
index e69de29bb2d1d6434b8b29ae775ad8c2e48c5391..0000000000000000000000000000000000000000
diff --git a/spaces/colakin/video-generater/public/ffmpeg/compat/solaris/make_sunver.pl b/spaces/colakin/video-generater/public/ffmpeg/compat/solaris/make_sunver.pl
deleted file mode 100644
index 0e9ed1d351ec2ac65817609dd929100fb49e43ae..0000000000000000000000000000000000000000
--- a/spaces/colakin/video-generater/public/ffmpeg/compat/solaris/make_sunver.pl
+++ /dev/null
@@ -1,352 +0,0 @@
-#!/usr/bin/env perl
-
-# make_sunver.pl
-#
-# Copyright (C) 2010, 2011, 2012, 2013
-# Free Software Foundation, Inc.
-#
-# This file is free software; you can redistribute it and/or modify it
-# under the terms of the GNU General Public License as published by
-# the Free Software Foundation; either version 3 of the License, or
-# (at your option) any later version.
-#
-# This program is distributed in the hope that it will be useful, but
-# WITHOUT ANY WARRANTY; without even the implied warranty of
-# MERCHANTABILITY or FITNESS FOR A PARTICULAR PURPOSE. See the GNU
-# General Public License for more details.
-#
-# You should have received a copy of the GNU General Public License
-# along with this program; see the file COPYING.GPLv3. If not see
-# .
-
-# This script takes at least two arguments, a GNU style version script and
-# a list of object and archive files, and generates a corresponding Sun
-# style version script as follows:
-#
-# Each glob pattern, C++ mangled pattern or literal in the input script is
-# matched against all global symbols in the input objects, emitting those
-# that matched (or nothing if no match was found).
-# A comment with the original pattern and its type is left in the output
-# file to make it easy to understand the matches.
-#
-# It uses elfdump when present (native), GNU readelf otherwise.
-# It depends on the GNU version of c++filt, since it must understand the
-# GNU mangling style.
-
-use FileHandle;
-use IPC::Open2;
-
-# Enforce C locale.
-$ENV{'LC_ALL'} = "C";
-$ENV{'LANG'} = "C";
-
-# Input version script, GNU style.
-my $symvers = shift;
-
-##########
-# Get all the symbols from the library, match them, and add them to a hash.
-
-my %sym_hash = ();
-
-# List of objects and archives to process.
-my @OBJECTS = ();
-
-# List of shared objects to omit from processing.
-my @SHAREDOBJS = ();
-
-# Filter out those input archives that have corresponding shared objects to
-# avoid adding all symbols matched in the archive to the output map.
-foreach $file (@ARGV) {
- if (($so = $file) =~ s/\.a$/.so/ && -e $so) {
- printf STDERR "omitted $file -> $so\n";
- push (@SHAREDOBJS, $so);
- } else {
- push (@OBJECTS, $file);
- }
-}
-
-# We need to detect and ignore hidden symbols. Solaris nm can only detect
-# this in the harder to parse default output format, and GNU nm not at all,
-# so use elfdump -s in the native case and GNU readelf -s otherwise.
-# GNU objdump -t cannot be used since it produces a variable number of
-# columns.
-
-# The path to elfdump.
-my $elfdump = "/usr/ccs/bin/elfdump";
-
-if (-f $elfdump) {
- open ELFDUMP,$elfdump.' -s '.(join ' ',@OBJECTS).'|' or die $!;
- my $skip_arsym = 0;
-
- while () {
- chomp;
-
- # Ignore empty lines.
- if (/^$/) {
- # End of archive symbol table, stop skipping.
- $skip_arsym = 0 if $skip_arsym;
- next;
- }
-
- # Keep skipping until end of archive symbol table.
- next if ($skip_arsym);
-
- # Ignore object name header for individual objects and archives.
- next if (/:$/);
-
- # Ignore table header lines.
- next if (/^Symbol Table Section:/);
- next if (/index.*value.*size/);
-
- # Start of archive symbol table: start skipping.
- if (/^Symbol Table: \(archive/) {
- $skip_arsym = 1;
- next;
- }
-
- # Split table.
- (undef, undef, undef, undef, $bind, $oth, undef, $shndx, $name) = split;
-
- # Error out for unknown input.
- die "unknown input line:\n$_" unless defined($bind);
-
- # Ignore local symbols.
- next if ($bind eq "LOCL");
- # Ignore hidden symbols.
- next if ($oth eq "H");
- # Ignore undefined symbols.
- next if ($shndx eq "UNDEF");
- # Error out for unhandled cases.
- if ($bind !~ /^(GLOB|WEAK)/ or $oth ne "D") {
- die "unhandled symbol:\n$_";
- }
-
- # Remember symbol.
- $sym_hash{$name}++;
- }
- close ELFDUMP or die "$elfdump error";
-} else {
- open READELF, 'readelf -s -W '.(join ' ',@OBJECTS).'|' or die $!;
- # Process each symbol.
- while () {
- chomp;
-
- # Ignore empty lines.
- next if (/^$/);
-
- # Ignore object name header.
- next if (/^File: .*$/);
-
- # Ignore table header lines.
- next if (/^Symbol table.*contains.*:/);
- next if (/Num:.*Value.*Size/);
-
- # Split table.
- (undef, undef, undef, undef, $bind, $vis, $ndx, $name) = split;
-
- # Error out for unknown input.
- die "unknown input line:\n$_" unless defined($bind);
-
- # Ignore local symbols.
- next if ($bind eq "LOCAL");
- # Ignore hidden symbols.
- next if ($vis eq "HIDDEN");
- # Ignore undefined symbols.
- next if ($ndx eq "UND");
- # Error out for unhandled cases.
- if ($bind !~ /^(GLOBAL|WEAK)/ or $vis ne "DEFAULT") {
- die "unhandled symbol:\n$_";
- }
-
- # Remember symbol.
- $sym_hash{$name}++;
- }
- close READELF or die "readelf error";
-}
-
-##########
-# The various types of glob patterns.
-#
-# A glob pattern that is to be applied to the demangled name: 'cxx'.
-# A glob patterns that applies directly to the name in the .o files: 'glob'.
-# This pattern is ignored; used for local variables (usually just '*'): 'ign'.
-
-# The type of the current pattern.
-my $glob = 'glob';
-
-# We're currently inside `extern "C++"', which Sun ld doesn't understand.
-my $in_extern = 0;
-
-# The c++filt command to use. This *must* be GNU c++filt; the Sun Studio
-# c++filt doesn't handle the GNU mangling style.
-my $cxxfilt = $ENV{'CXXFILT'} || "c++filt";
-
-# The current version name.
-my $current_version = "";
-
-# Was there any attempt to match a symbol to this version?
-my $matches_attempted;
-
-# The number of versions which matched this symbol.
-my $matched_symbols;
-
-open F,$symvers or die $!;
-
-# Print information about generating this file
-print "# This file was generated by make_sunver.pl. DO NOT EDIT!\n";
-print "# It was generated by:\n";
-printf "# %s %s %s\n", $0, $symvers, (join ' ',@ARGV);
-printf "# Omitted archives with corresponding shared libraries: %s\n",
- (join ' ', @SHAREDOBJS) if $#SHAREDOBJS >= 0;
-print "#\n\n";
-
-print "\$mapfile_version 2\n";
-
-while () {
- # Lines of the form '};'
- if (/^([ \t]*)(\}[ \t]*;[ \t]*)$/) {
- $glob = 'glob';
- if ($in_extern) {
- $in_extern--;
- print "$1##$2\n";
- } else {
- print;
- }
- next;
- }
-
- # Lines of the form '} SOME_VERSION_NAME_1.0;'
- if (/^[ \t]*\}[ \tA-Z0-9_.a-z]+;[ \t]*$/) {
- $glob = 'glob';
- # We tried to match symbols agains this version, but none matched.
- # Emit dummy hidden symbol to avoid marking this version WEAK.
- if ($matches_attempted && $matched_symbols == 0) {
- print " hidden:\n";
- print " .force_WEAK_off_$current_version = DATA S0x0 V0x0;\n";
- }
- print; next;
- }
-
- # Comment and blank lines
- if (/^[ \t]*\#/) { print; next; }
- if (/^[ \t]*$/) { print; next; }
-
- # Lines of the form '{'
- if (/^([ \t]*){$/) {
- if ($in_extern) {
- print "$1##{\n";
- } else {
- print;
- }
- next;
- }
-
- # Lines of the form 'SOME_VERSION_NAME_1.1 {'
- if (/^([A-Z0-9_.]+)[ \t]+{$/) {
- # Record version name.
- $current_version = $1;
- # Reset match attempts, #matched symbols for this version.
- $matches_attempted = 0;
- $matched_symbols = 0;
- print "SYMBOL_VERSION $1 {\n";
- next;
- }
-
- # Ignore 'global:'
- if (/^[ \t]*global:$/) { print; next; }
-
- # After 'local:', globs should be ignored, they won't be exported.
- if (/^[ \t]*local:$/) {
- $glob = 'ign';
- print;
- next;
- }
-
- # After 'extern "C++"', globs are C++ patterns
- if (/^([ \t]*)(extern \"C\+\+\"[ \t]*)$/) {
- $in_extern++;
- $glob = 'cxx';
- # Need to comment, Sun ld cannot handle this.
- print "$1##$2\n"; next;
- }
-
- # Chomp newline now we're done with passing through the input file.
- chomp;
-
- # Catch globs. Note that '{}' is not allowed in globs by this script,
- # so only '*' and '[]' are available.
- if (/^([ \t]*)([^ \t;{}#]+);?[ \t]*$/) {
- my $ws = $1;
- my $ptn = $2;
- # Turn the glob into a regex by replacing '*' with '.*', '?' with '.'.
- # Keep $ptn so we can still print the original form.
- ($pattern = $ptn) =~ s/\*/\.\*/g;
- $pattern =~ s/\?/\./g;
-
- if ($glob eq 'ign') {
- # We're in a local: * section; just continue.
- print "$_\n";
- next;
- }
-
- # Print the glob commented for human readers.
- print "$ws##$ptn ($glob)\n";
- # We tried to match a symbol to this version.
- $matches_attempted++;
-
- if ($glob eq 'glob') {
- my %ptn_syms = ();
-
- # Match ptn against symbols in %sym_hash.
- foreach my $sym (keys %sym_hash) {
- # Maybe it matches one of the patterns based on the symbol in
- # the .o file.
- $ptn_syms{$sym}++ if ($sym =~ /^$pattern$/);
- }
-
- foreach my $sym (sort keys(%ptn_syms)) {
- $matched_symbols++;
- print "$ws$sym;\n";
- }
- } elsif ($glob eq 'cxx') {
- my %dem_syms = ();
-
- # Verify that we're actually using GNU c++filt. Other versions
- # most likely cannot handle GNU style symbol mangling.
- my $cxxout = `$cxxfilt --version 2>&1`;
- $cxxout =~ m/GNU/ or die "$0 requires GNU c++filt to function";
-
- # Talk to c++filt through a pair of file descriptors.
- # Need to start a fresh instance per pattern, otherwise the
- # process grows to 500+ MB.
- my $pid = open2(*FILTIN, *FILTOUT, $cxxfilt) or die $!;
-
- # Match ptn against symbols in %sym_hash.
- foreach my $sym (keys %sym_hash) {
- # No? Well, maybe its demangled form matches one of those
- # patterns.
- printf FILTOUT "%s\n",$sym;
- my $dem = ;
- chomp $dem;
- $dem_syms{$sym}++ if ($dem =~ /^$pattern$/);
- }
-
- close FILTOUT or die "c++filt error";
- close FILTIN or die "c++filt error";
- # Need to wait for the c++filt process to avoid lots of zombies.
- waitpid $pid, 0;
-
- foreach my $sym (sort keys(%dem_syms)) {
- $matched_symbols++;
- print "$ws$sym;\n";
- }
- } else {
- # No? Well, then ignore it.
- }
- next;
- }
- # Important sanity check. This script can't handle lots of formats
- # that GNU ld can, so be sure to error out if one is seen!
- die "strange line `$_'";
-}
-close F;
diff --git a/spaces/colakin/video-generater/public/ffmpeg/libavcodec/dxva2_hevc.c b/spaces/colakin/video-generater/public/ffmpeg/libavcodec/dxva2_hevc.c
deleted file mode 100644
index 6b239d9917cf75dac20e7610f3750d229579627c..0000000000000000000000000000000000000000
--- a/spaces/colakin/video-generater/public/ffmpeg/libavcodec/dxva2_hevc.c
+++ /dev/null
@@ -1,471 +0,0 @@
-/*
- * DXVA2 HEVC HW acceleration.
- *
- * copyright (c) 2014 - 2015 Hendrik Leppkes
- *
- * This file is part of FFmpeg.
- *
- * FFmpeg is free software; you can redistribute it and/or
- * modify it under the terms of the GNU Lesser General Public
- * License as published by the Free Software Foundation; either
- * version 2.1 of the License, or (at your option) any later version.
- *
- * FFmpeg is distributed in the hope that it will be useful,
- * but WITHOUT ANY WARRANTY; without even the implied warranty of
- * MERCHANTABILITY or FITNESS FOR A PARTICULAR PURPOSE. See the GNU
- * Lesser General Public License for more details.
- *
- * You should have received a copy of the GNU Lesser General Public
- * License along with FFmpeg; if not, write to the Free Software
- * Foundation, Inc., 51 Franklin Street, Fifth Floor, Boston, MA 02110-1301 USA
- */
-
-#include "config_components.h"
-
-#include "libavutil/avassert.h"
-
-#include "dxva2_internal.h"
-#include "hevc_data.h"
-#include "hevcdec.h"
-
-#define MAX_SLICES 256
-
-struct hevc_dxva2_picture_context {
- DXVA_PicParams_HEVC pp;
- DXVA_Qmatrix_HEVC qm;
- unsigned slice_count;
- DXVA_Slice_HEVC_Short slice_short[MAX_SLICES];
- const uint8_t *bitstream;
- unsigned bitstream_size;
-};
-
-static void fill_picture_entry(DXVA_PicEntry_HEVC *pic,
- unsigned index, unsigned flag)
-{
- av_assert0((index & 0x7f) == index && (flag & 0x01) == flag);
- pic->bPicEntry = index | (flag << 7);
-}
-
-static int get_refpic_index(const DXVA_PicParams_HEVC *pp, int surface_index)
-{
- int i;
- for (i = 0; i < FF_ARRAY_ELEMS(pp->RefPicList); i++) {
- if ((pp->RefPicList[i].bPicEntry & 0x7f) == surface_index)
- return i;
- }
- return 0xff;
-}
-
-static void fill_picture_parameters(const AVCodecContext *avctx, AVDXVAContext *ctx, const HEVCContext *h,
- DXVA_PicParams_HEVC *pp)
-{
- const HEVCFrame *current_picture = h->ref;
- const HEVCSPS *sps = h->ps.sps;
- const HEVCPPS *pps = h->ps.pps;
- int i, j;
-
- memset(pp, 0, sizeof(*pp));
-
- pp->PicWidthInMinCbsY = sps->min_cb_width;
- pp->PicHeightInMinCbsY = sps->min_cb_height;
-
- pp->wFormatAndSequenceInfoFlags = (sps->chroma_format_idc << 0) |
- (sps->separate_colour_plane_flag << 2) |
- ((sps->bit_depth - 8) << 3) |
- ((sps->bit_depth - 8) << 6) |
- ((sps->log2_max_poc_lsb - 4) << 9) |
- (0 << 13) |
- (0 << 14) |
- (0 << 15);
-
- fill_picture_entry(&pp->CurrPic, ff_dxva2_get_surface_index(avctx, ctx, current_picture->frame), 0);
-
- pp->sps_max_dec_pic_buffering_minus1 = sps->temporal_layer[sps->max_sub_layers - 1].max_dec_pic_buffering - 1;
- pp->log2_min_luma_coding_block_size_minus3 = sps->log2_min_cb_size - 3;
- pp->log2_diff_max_min_luma_coding_block_size = sps->log2_diff_max_min_coding_block_size;
- pp->log2_min_transform_block_size_minus2 = sps->log2_min_tb_size - 2;
- pp->log2_diff_max_min_transform_block_size = sps->log2_max_trafo_size - sps->log2_min_tb_size;
- pp->max_transform_hierarchy_depth_inter = sps->max_transform_hierarchy_depth_inter;
- pp->max_transform_hierarchy_depth_intra = sps->max_transform_hierarchy_depth_intra;
- pp->num_short_term_ref_pic_sets = sps->nb_st_rps;
- pp->num_long_term_ref_pics_sps = sps->num_long_term_ref_pics_sps;
-
- pp->num_ref_idx_l0_default_active_minus1 = pps->num_ref_idx_l0_default_active - 1;
- pp->num_ref_idx_l1_default_active_minus1 = pps->num_ref_idx_l1_default_active - 1;
- pp->init_qp_minus26 = pps->pic_init_qp_minus26;
-
- if (h->sh.short_term_ref_pic_set_sps_flag == 0 && h->sh.short_term_rps) {
- pp->ucNumDeltaPocsOfRefRpsIdx = h->sh.short_term_rps->rps_idx_num_delta_pocs;
- pp->wNumBitsForShortTermRPSInSlice = h->sh.short_term_ref_pic_set_size;
- }
-
- pp->dwCodingParamToolFlags = (sps->scaling_list_enable_flag << 0) |
- (sps->amp_enabled_flag << 1) |
- (sps->sao_enabled << 2) |
- (sps->pcm_enabled_flag << 3) |
- ((sps->pcm_enabled_flag ? (sps->pcm.bit_depth - 1) : 0) << 4) |
- ((sps->pcm_enabled_flag ? (sps->pcm.bit_depth_chroma - 1) : 0) << 8) |
- ((sps->pcm_enabled_flag ? (sps->pcm.log2_min_pcm_cb_size - 3) : 0) << 12) |
- ((sps->pcm_enabled_flag ? (sps->pcm.log2_max_pcm_cb_size - sps->pcm.log2_min_pcm_cb_size) : 0) << 14) |
- (sps->pcm.loop_filter_disable_flag << 16) |
- (sps->long_term_ref_pics_present_flag << 17) |
- (sps->sps_temporal_mvp_enabled_flag << 18) |
- (sps->sps_strong_intra_smoothing_enable_flag << 19) |
- (pps->dependent_slice_segments_enabled_flag << 20) |
- (pps->output_flag_present_flag << 21) |
- (pps->num_extra_slice_header_bits << 22) |
- (pps->sign_data_hiding_flag << 25) |
- (pps->cabac_init_present_flag << 26) |
- (0 << 27);
-
- pp->dwCodingSettingPicturePropertyFlags = (pps->constrained_intra_pred_flag << 0) |
- (pps->transform_skip_enabled_flag << 1) |
- (pps->cu_qp_delta_enabled_flag << 2) |
- (pps->pic_slice_level_chroma_qp_offsets_present_flag << 3) |
- (pps->weighted_pred_flag << 4) |
- (pps->weighted_bipred_flag << 5) |
- (pps->transquant_bypass_enable_flag << 6) |
- (pps->tiles_enabled_flag << 7) |
- (pps->entropy_coding_sync_enabled_flag << 8) |
- (pps->uniform_spacing_flag << 9) |
- ((pps->tiles_enabled_flag ? pps->loop_filter_across_tiles_enabled_flag : 0) << 10) |
- (pps->seq_loop_filter_across_slices_enabled_flag << 11) |
- (pps->deblocking_filter_override_enabled_flag << 12) |
- (pps->disable_dbf << 13) |
- (pps->lists_modification_present_flag << 14) |
- (pps->slice_header_extension_present_flag << 15) |
- (IS_IRAP(h) << 16) |
- (IS_IDR(h) << 17) |
- /* IntraPicFlag */
- (IS_IRAP(h) << 18) |
- (0 << 19);
- pp->pps_cb_qp_offset = pps->cb_qp_offset;
- pp->pps_cr_qp_offset = pps->cr_qp_offset;
- if (pps->tiles_enabled_flag) {
- pp->num_tile_columns_minus1 = pps->num_tile_columns - 1;
- pp->num_tile_rows_minus1 = pps->num_tile_rows - 1;
-
- if (!pps->uniform_spacing_flag) {
- for (i = 0; i < pps->num_tile_columns; i++)
- pp->column_width_minus1[i] = pps->column_width[i] - 1;
-
- for (i = 0; i < pps->num_tile_rows; i++)
- pp->row_height_minus1[i] = pps->row_height[i] - 1;
- }
- }
-
- pp->diff_cu_qp_delta_depth = pps->diff_cu_qp_delta_depth;
- pp->pps_beta_offset_div2 = pps->beta_offset / 2;
- pp->pps_tc_offset_div2 = pps->tc_offset / 2;
- pp->log2_parallel_merge_level_minus2 = pps->log2_parallel_merge_level - 2;
- pp->CurrPicOrderCntVal = h->poc;
-
- // fill RefPicList from the DPB
- for (i = 0, j = 0; i < FF_ARRAY_ELEMS(pp->RefPicList); i++) {
- const HEVCFrame *frame = NULL;
- while (!frame && j < FF_ARRAY_ELEMS(h->DPB)) {
- if (&h->DPB[j] != current_picture && (h->DPB[j].flags & (HEVC_FRAME_FLAG_LONG_REF | HEVC_FRAME_FLAG_SHORT_REF)))
- frame = &h->DPB[j];
- j++;
- }
-
- if (frame) {
- fill_picture_entry(&pp->RefPicList[i], ff_dxva2_get_surface_index(avctx, ctx, frame->frame), !!(frame->flags & HEVC_FRAME_FLAG_LONG_REF));
- pp->PicOrderCntValList[i] = frame->poc;
- } else {
- pp->RefPicList[i].bPicEntry = 0xff;
- pp->PicOrderCntValList[i] = 0;
- }
- }
-
- #define DO_REF_LIST(ref_idx, ref_list) { \
- const RefPicList *rpl = &h->rps[ref_idx]; \
- for (i = 0, j = 0; i < FF_ARRAY_ELEMS(pp->ref_list); i++) { \
- const HEVCFrame *frame = NULL; \
- while (!frame && j < rpl->nb_refs) \
- frame = rpl->ref[j++]; \
- if (frame && frame->flags & (HEVC_FRAME_FLAG_LONG_REF | HEVC_FRAME_FLAG_SHORT_REF)) \
- pp->ref_list[i] = get_refpic_index(pp, ff_dxva2_get_surface_index(avctx, ctx, frame->frame)); \
- else \
- pp->ref_list[i] = 0xff; \
- } \
- }
-
- // Fill short term and long term lists
- DO_REF_LIST(ST_CURR_BEF, RefPicSetStCurrBefore);
- DO_REF_LIST(ST_CURR_AFT, RefPicSetStCurrAfter);
- DO_REF_LIST(LT_CURR, RefPicSetLtCurr);
-
- pp->StatusReportFeedbackNumber = 1 + DXVA_CONTEXT_REPORT_ID(avctx, ctx)++;
-}
-
-static void fill_scaling_lists(AVDXVAContext *ctx, const HEVCContext *h, DXVA_Qmatrix_HEVC *qm)
-{
- unsigned i, j, pos;
- const ScalingList *sl = h->ps.pps->scaling_list_data_present_flag ?
- &h->ps.pps->scaling_list : &h->ps.sps->scaling_list;
-
- memset(qm, 0, sizeof(*qm));
- for (i = 0; i < 6; i++) {
- for (j = 0; j < 16; j++) {
- pos = 4 * ff_hevc_diag_scan4x4_y[j] + ff_hevc_diag_scan4x4_x[j];
- qm->ucScalingLists0[i][j] = sl->sl[0][i][pos];
- }
-
- for (j = 0; j < 64; j++) {
- pos = 8 * ff_hevc_diag_scan8x8_y[j] + ff_hevc_diag_scan8x8_x[j];
- qm->ucScalingLists1[i][j] = sl->sl[1][i][pos];
- qm->ucScalingLists2[i][j] = sl->sl[2][i][pos];
-
- if (i < 2)
- qm->ucScalingLists3[i][j] = sl->sl[3][i * 3][pos];
- }
-
- qm->ucScalingListDCCoefSizeID2[i] = sl->sl_dc[0][i];
- if (i < 2)
- qm->ucScalingListDCCoefSizeID3[i] = sl->sl_dc[1][i * 3];
- }
-}
-
-static void fill_slice_short(DXVA_Slice_HEVC_Short *slice,
- unsigned position, unsigned size)
-{
- memset(slice, 0, sizeof(*slice));
- slice->BSNALunitDataLocation = position;
- slice->SliceBytesInBuffer = size;
- slice->wBadSliceChopping = 0;
-}
-
-static int commit_bitstream_and_slice_buffer(AVCodecContext *avctx,
- DECODER_BUFFER_DESC *bs,
- DECODER_BUFFER_DESC *sc)
-{
- const HEVCContext *h = avctx->priv_data;
- AVDXVAContext *ctx = DXVA_CONTEXT(avctx);
- const HEVCFrame *current_picture = h->ref;
- struct hevc_dxva2_picture_context *ctx_pic = current_picture->hwaccel_picture_private;
- DXVA_Slice_HEVC_Short *slice = NULL;
- void *dxva_data_ptr;
- uint8_t *dxva_data, *current, *end;
- unsigned dxva_size;
- void *slice_data;
- unsigned slice_size;
- unsigned padding;
- unsigned i;
- unsigned type;
-
- /* Create an annex B bitstream buffer with only slice NAL and finalize slice */
-#if CONFIG_D3D11VA
- if (ff_dxva2_is_d3d11(avctx)) {
- type = D3D11_VIDEO_DECODER_BUFFER_BITSTREAM;
- if (FAILED(ID3D11VideoContext_GetDecoderBuffer(D3D11VA_CONTEXT(ctx)->video_context,
- D3D11VA_CONTEXT(ctx)->decoder,
- type,
- &dxva_size, &dxva_data_ptr)))
- return -1;
- }
-#endif
-#if CONFIG_DXVA2
- if (avctx->pix_fmt == AV_PIX_FMT_DXVA2_VLD) {
- type = DXVA2_BitStreamDateBufferType;
- if (FAILED(IDirectXVideoDecoder_GetBuffer(DXVA2_CONTEXT(ctx)->decoder,
- type,
- &dxva_data_ptr, &dxva_size)))
- return -1;
- }
-#endif
-
- dxva_data = dxva_data_ptr;
- current = dxva_data;
- end = dxva_data + dxva_size;
-
- for (i = 0; i < ctx_pic->slice_count; i++) {
- static const uint8_t start_code[] = { 0, 0, 1 };
- static const unsigned start_code_size = sizeof(start_code);
- unsigned position, size;
-
- slice = &ctx_pic->slice_short[i];
-
- position = slice->BSNALunitDataLocation;
- size = slice->SliceBytesInBuffer;
- if (start_code_size + size > end - current) {
- av_log(avctx, AV_LOG_ERROR, "Failed to build bitstream");
- break;
- }
-
- slice->BSNALunitDataLocation = current - dxva_data;
- slice->SliceBytesInBuffer = start_code_size + size;
-
- memcpy(current, start_code, start_code_size);
- current += start_code_size;
-
- memcpy(current, &ctx_pic->bitstream[position], size);
- current += size;
- }
- padding = FFMIN(128 - ((current - dxva_data) & 127), end - current);
- if (slice && padding > 0) {
- memset(current, 0, padding);
- current += padding;
-
- slice->SliceBytesInBuffer += padding;
- }
-#if CONFIG_D3D11VA
- if (ff_dxva2_is_d3d11(avctx))
- if (FAILED(ID3D11VideoContext_ReleaseDecoderBuffer(D3D11VA_CONTEXT(ctx)->video_context, D3D11VA_CONTEXT(ctx)->decoder, type)))
- return -1;
-#endif
-#if CONFIG_DXVA2
- if (avctx->pix_fmt == AV_PIX_FMT_DXVA2_VLD)
- if (FAILED(IDirectXVideoDecoder_ReleaseBuffer(DXVA2_CONTEXT(ctx)->decoder, type)))
- return -1;
-#endif
- if (i < ctx_pic->slice_count)
- return -1;
-
-#if CONFIG_D3D11VA
- if (ff_dxva2_is_d3d11(avctx)) {
- D3D11_VIDEO_DECODER_BUFFER_DESC *dsc11 = bs;
- memset(dsc11, 0, sizeof(*dsc11));
- dsc11->BufferType = type;
- dsc11->DataSize = current - dxva_data;
- dsc11->NumMBsInBuffer = 0;
-
- type = D3D11_VIDEO_DECODER_BUFFER_SLICE_CONTROL;
- }
-#endif
-#if CONFIG_DXVA2
- if (avctx->pix_fmt == AV_PIX_FMT_DXVA2_VLD) {
- DXVA2_DecodeBufferDesc *dsc2 = bs;
- memset(dsc2, 0, sizeof(*dsc2));
- dsc2->CompressedBufferType = type;
- dsc2->DataSize = current - dxva_data;
- dsc2->NumMBsInBuffer = 0;
-
- type = DXVA2_SliceControlBufferType;
- }
-#endif
-
- slice_data = ctx_pic->slice_short;
- slice_size = ctx_pic->slice_count * sizeof(*ctx_pic->slice_short);
-
- av_assert0(((current - dxva_data) & 127) == 0);
- return ff_dxva2_commit_buffer(avctx, ctx, sc,
- type,
- slice_data, slice_size, 0);
-}
-
-
-static int dxva2_hevc_start_frame(AVCodecContext *avctx,
- av_unused const uint8_t *buffer,
- av_unused uint32_t size)
-{
- const HEVCContext *h = avctx->priv_data;
- AVDXVAContext *ctx = DXVA_CONTEXT(avctx);
- struct hevc_dxva2_picture_context *ctx_pic = h->ref->hwaccel_picture_private;
-
- if (!DXVA_CONTEXT_VALID(avctx, ctx))
- return -1;
- av_assert0(ctx_pic);
-
- /* Fill up DXVA_PicParams_HEVC */
- fill_picture_parameters(avctx, ctx, h, &ctx_pic->pp);
-
- /* Fill up DXVA_Qmatrix_HEVC */
- fill_scaling_lists(ctx, h, &ctx_pic->qm);
-
- ctx_pic->slice_count = 0;
- ctx_pic->bitstream_size = 0;
- ctx_pic->bitstream = NULL;
- return 0;
-}
-
-static int dxva2_hevc_decode_slice(AVCodecContext *avctx,
- const uint8_t *buffer,
- uint32_t size)
-{
- const HEVCContext *h = avctx->priv_data;
- const HEVCFrame *current_picture = h->ref;
- struct hevc_dxva2_picture_context *ctx_pic = current_picture->hwaccel_picture_private;
- unsigned position;
-
- if (ctx_pic->slice_count >= MAX_SLICES)
- return -1;
-
- if (!ctx_pic->bitstream)
- ctx_pic->bitstream = buffer;
- ctx_pic->bitstream_size += size;
-
- position = buffer - ctx_pic->bitstream;
- fill_slice_short(&ctx_pic->slice_short[ctx_pic->slice_count], position, size);
- ctx_pic->slice_count++;
-
- return 0;
-}
-
-static int dxva2_hevc_end_frame(AVCodecContext *avctx)
-{
- HEVCContext *h = avctx->priv_data;
- struct hevc_dxva2_picture_context *ctx_pic = h->ref->hwaccel_picture_private;
- int scale = ctx_pic->pp.dwCodingParamToolFlags & 1;
- int ret;
-
- if (ctx_pic->slice_count <= 0 || ctx_pic->bitstream_size <= 0)
- return -1;
-
- ret = ff_dxva2_common_end_frame(avctx, h->ref->frame,
- &ctx_pic->pp, sizeof(ctx_pic->pp),
- scale ? &ctx_pic->qm : NULL, scale ? sizeof(ctx_pic->qm) : 0,
- commit_bitstream_and_slice_buffer);
- return ret;
-}
-
-#if CONFIG_HEVC_DXVA2_HWACCEL
-const AVHWAccel ff_hevc_dxva2_hwaccel = {
- .name = "hevc_dxva2",
- .type = AVMEDIA_TYPE_VIDEO,
- .id = AV_CODEC_ID_HEVC,
- .pix_fmt = AV_PIX_FMT_DXVA2_VLD,
- .init = ff_dxva2_decode_init,
- .uninit = ff_dxva2_decode_uninit,
- .start_frame = dxva2_hevc_start_frame,
- .decode_slice = dxva2_hevc_decode_slice,
- .end_frame = dxva2_hevc_end_frame,
- .frame_params = ff_dxva2_common_frame_params,
- .frame_priv_data_size = sizeof(struct hevc_dxva2_picture_context),
- .priv_data_size = sizeof(FFDXVASharedContext),
-};
-#endif
-
-#if CONFIG_HEVC_D3D11VA_HWACCEL
-const AVHWAccel ff_hevc_d3d11va_hwaccel = {
- .name = "hevc_d3d11va",
- .type = AVMEDIA_TYPE_VIDEO,
- .id = AV_CODEC_ID_HEVC,
- .pix_fmt = AV_PIX_FMT_D3D11VA_VLD,
- .init = ff_dxva2_decode_init,
- .uninit = ff_dxva2_decode_uninit,
- .start_frame = dxva2_hevc_start_frame,
- .decode_slice = dxva2_hevc_decode_slice,
- .end_frame = dxva2_hevc_end_frame,
- .frame_params = ff_dxva2_common_frame_params,
- .frame_priv_data_size = sizeof(struct hevc_dxva2_picture_context),
- .priv_data_size = sizeof(FFDXVASharedContext),
-};
-#endif
-
-#if CONFIG_HEVC_D3D11VA2_HWACCEL
-const AVHWAccel ff_hevc_d3d11va2_hwaccel = {
- .name = "hevc_d3d11va2",
- .type = AVMEDIA_TYPE_VIDEO,
- .id = AV_CODEC_ID_HEVC,
- .pix_fmt = AV_PIX_FMT_D3D11,
- .init = ff_dxva2_decode_init,
- .uninit = ff_dxva2_decode_uninit,
- .start_frame = dxva2_hevc_start_frame,
- .decode_slice = dxva2_hevc_decode_slice,
- .end_frame = dxva2_hevc_end_frame,
- .frame_params = ff_dxva2_common_frame_params,
- .frame_priv_data_size = sizeof(struct hevc_dxva2_picture_context),
- .priv_data_size = sizeof(FFDXVASharedContext),
-};
-#endif
diff --git a/spaces/colakin/video-generater/public/ffmpeg/libavcodec/hevc_data.h b/spaces/colakin/video-generater/public/ffmpeg/libavcodec/hevc_data.h
deleted file mode 100644
index 74558f0a989584ca9b4a10386787cd99395efd85..0000000000000000000000000000000000000000
--- a/spaces/colakin/video-generater/public/ffmpeg/libavcodec/hevc_data.h
+++ /dev/null
@@ -1,31 +0,0 @@
-/*
- * HEVC shared data tables
- *
- * This file is part of FFmpeg.
- *
- * FFmpeg is free software; you can redistribute it and/or
- * modify it under the terms of the GNU Lesser General Public
- * License as published by the Free Software Foundation; either
- * version 2.1 of the License, or (at your option) any later version.
- *
- * FFmpeg is distributed in the hope that it will be useful,
- * but WITHOUT ANY WARRANTY; without even the implied warranty of
- * MERCHANTABILITY or FITNESS FOR A PARTICULAR PURPOSE. See the GNU
- * Lesser General Public License for more details.
- *
- * You should have received a copy of the GNU Lesser General Public
- * License along with FFmpeg; if not, write to the Free Software
- * Foundation, Inc., 51 Franklin Street, Fifth Floor, Boston, MA 02110-1301 USA
- */
-
-#ifndef AVCODEC_HEVC_DATA_H
-#define AVCODEC_HEVC_DATA_H
-
-#include
-
-extern const uint8_t ff_hevc_diag_scan4x4_x[16];
-extern const uint8_t ff_hevc_diag_scan4x4_y[16];
-extern const uint8_t ff_hevc_diag_scan8x8_x[64];
-extern const uint8_t ff_hevc_diag_scan8x8_y[64];
-
-#endif /* AVCODEC_HEVC_DATA_H */
diff --git a/spaces/colakin/video-generater/public/ffmpeg/libavcodec/libshine.c b/spaces/colakin/video-generater/public/ffmpeg/libavcodec/libshine.c
deleted file mode 100644
index 2f6a9233e02c214936058c940bb47644ee5389fe..0000000000000000000000000000000000000000
--- a/spaces/colakin/video-generater/public/ffmpeg/libavcodec/libshine.c
+++ /dev/null
@@ -1,149 +0,0 @@
-/*
- * Interface to libshine for mp3 encoding
- * Copyright (c) 2012 Paul B Mahol
- *
- * This file is part of FFmpeg.
- *
- * FFmpeg is free software; you can redistribute it and/or
- * modify it under the terms of the GNU Lesser General Public
- * License as published by the Free Software Foundation; either
- * version 2.1 of the License, or (at your option) any later version.
- *
- * FFmpeg is distributed in the hope that it will be useful,
- * but WITHOUT ANY WARRANTY; without even the implied warranty of
- * MERCHANTABILITY or FITNESS FOR A PARTICULAR PURPOSE. See the GNU
- * Lesser General Public License for more details.
- *
- * You should have received a copy of the GNU Lesser General Public
- * License along with FFmpeg; if not, write to the Free Software
- * Foundation, Inc., 51 Franklin Street, Fifth Floor, Boston, MA 02110-1301 USA
- */
-
-#include
-
-#include "libavutil/channel_layout.h"
-#include "libavutil/intreadwrite.h"
-#include "audio_frame_queue.h"
-#include "avcodec.h"
-#include "codec_internal.h"
-#include "encode.h"
-#include "mpegaudio.h"
-#include "mpegaudiodecheader.h"
-
-#define BUFFER_SIZE (4096 * 20)
-
-typedef struct SHINEContext {
- shine_config_t config;
- shine_t shine;
- uint8_t buffer[BUFFER_SIZE];
- int buffer_index;
- AudioFrameQueue afq;
-} SHINEContext;
-
-static av_cold int libshine_encode_init(AVCodecContext *avctx)
-{
- SHINEContext *s = avctx->priv_data;
-
- shine_set_config_mpeg_defaults(&s->config.mpeg);
- if (avctx->bit_rate)
- s->config.mpeg.bitr = avctx->bit_rate / 1000;
- s->config.mpeg.mode = avctx->ch_layout.nb_channels == 2 ? STEREO : MONO;
- s->config.wave.samplerate = avctx->sample_rate;
- s->config.wave.channels = avctx->ch_layout.nb_channels == 2 ? PCM_STEREO : PCM_MONO;
- if (shine_check_config(s->config.wave.samplerate, s->config.mpeg.bitr) < 0) {
- av_log(avctx, AV_LOG_ERROR, "invalid configuration\n");
- return AVERROR(EINVAL);
- }
- s->shine = shine_initialise(&s->config);
- if (!s->shine)
- return AVERROR(ENOMEM);
- avctx->frame_size = shine_samples_per_pass(s->shine);
- ff_af_queue_init(avctx, &s->afq);
- return 0;
-}
-
-static int libshine_encode_frame(AVCodecContext *avctx, AVPacket *avpkt,
- const AVFrame *frame, int *got_packet_ptr)
-{
- SHINEContext *s = avctx->priv_data;
- MPADecodeHeader hdr;
- unsigned char *data;
- int written;
- int ret, len;
-
- if (frame)
- data = shine_encode_buffer(s->shine, (int16_t **)frame->data, &written);
- else
- data = shine_flush(s->shine, &written);
- if (written < 0)
- return -1;
- if (written > 0) {
- if (s->buffer_index + written > BUFFER_SIZE) {
- av_log(avctx, AV_LOG_ERROR, "internal buffer too small\n");
- return AVERROR_BUG;
- }
- memcpy(s->buffer + s->buffer_index, data, written);
- s->buffer_index += written;
- }
- if (frame) {
- if ((ret = ff_af_queue_add(&s->afq, frame)) < 0)
- return ret;
- }
-
- if (s->buffer_index < 4 || !s->afq.frame_count)
- return 0;
- if (avpriv_mpegaudio_decode_header(&hdr, AV_RB32(s->buffer))) {
- av_log(avctx, AV_LOG_ERROR, "free format output not supported\n");
- return -1;
- }
-
- len = hdr.frame_size;
- if (len <= s->buffer_index) {
- if ((ret = ff_get_encode_buffer(avctx, avpkt, len, 0)))
- return ret;
- memcpy(avpkt->data, s->buffer, len);
- s->buffer_index -= len;
- memmove(s->buffer, s->buffer + len, s->buffer_index);
-
- ff_af_queue_remove(&s->afq, avctx->frame_size, &avpkt->pts,
- &avpkt->duration);
-
- *got_packet_ptr = 1;
- }
- return 0;
-}
-
-static av_cold int libshine_encode_close(AVCodecContext *avctx)
-{
- SHINEContext *s = avctx->priv_data;
-
- ff_af_queue_close(&s->afq);
- shine_close(s->shine);
- return 0;
-}
-
-static const int libshine_sample_rates[] = {
- 44100, 48000, 32000, 0
-};
-
-const FFCodec ff_libshine_encoder = {
- .p.name = "libshine",
- CODEC_LONG_NAME("libshine MP3 (MPEG audio layer 3)"),
- .p.type = AVMEDIA_TYPE_AUDIO,
- .p.id = AV_CODEC_ID_MP3,
- .p.capabilities = AV_CODEC_CAP_DR1 | AV_CODEC_CAP_DELAY,
- .caps_internal = FF_CODEC_CAP_NOT_INIT_THREADSAFE,
- .priv_data_size = sizeof(SHINEContext),
- .init = libshine_encode_init,
- FF_CODEC_ENCODE_CB(libshine_encode_frame),
- .close = libshine_encode_close,
- .p.sample_fmts = (const enum AVSampleFormat[]){ AV_SAMPLE_FMT_S16P,
- AV_SAMPLE_FMT_NONE },
- .p.supported_samplerates = libshine_sample_rates,
- CODEC_OLD_CHANNEL_LAYOUTS(AV_CH_LAYOUT_MONO, AV_CH_LAYOUT_STEREO)
- .p.ch_layouts = (const AVChannelLayout[]) { AV_CHANNEL_LAYOUT_MONO,
- AV_CHANNEL_LAYOUT_STEREO,
- { 0 },
- },
- .p.wrapper_name = "libshine",
-};
diff --git a/spaces/colakin/video-generater/public/ffmpeg/libavcodec/mips/pixblockdsp_init_mips.c b/spaces/colakin/video-generater/public/ffmpeg/libavcodec/mips/pixblockdsp_init_mips.c
deleted file mode 100644
index 2e2d70953bdd97c6c15a3653b3463e10280d25ef..0000000000000000000000000000000000000000
--- a/spaces/colakin/video-generater/public/ffmpeg/libavcodec/mips/pixblockdsp_init_mips.c
+++ /dev/null
@@ -1,56 +0,0 @@
-/*
- * Copyright (c) 2015 Shivraj Patil (Shivraj.Patil@imgtec.com)
- * Zhou Xiaoyong
- *
- * This file is part of FFmpeg.
- *
- * FFmpeg is free software; you can redistribute it and/or
- * modify it under the terms of the GNU Lesser General Public
- * License as published by the Free Software Foundation; either
- * version 2.1 of the License, or (at your option) any later version.
- *
- * FFmpeg is distributed in the hope that it will be useful,
- * but WITHOUT ANY WARRANTY; without even the implied warranty of
- * MERCHANTABILITY or FITNESS FOR A PARTICULAR PURPOSE. See the GNU
- * Lesser General Public License for more details.
- *
- * You should have received a copy of the GNU Lesser General Public
- * License along with FFmpeg; if not, write to the Free Software
- * Foundation, Inc., 51 Franklin Street, Fifth Floor, Boston, MA 02110-1301 USA
- */
-
-#include "libavutil/mips/cpu.h"
-#include "pixblockdsp_mips.h"
-
-void ff_pixblockdsp_init_mips(PixblockDSPContext *c, AVCodecContext *avctx,
- unsigned high_bit_depth)
-{
- int cpu_flags = av_get_cpu_flags();
-
- if (have_mmi(cpu_flags)) {
- c->diff_pixels = ff_diff_pixels_mmi;
-
- if (!high_bit_depth || avctx->codec_type != AVMEDIA_TYPE_VIDEO) {
- c->get_pixels = ff_get_pixels_8_mmi;
- }
- }
-
- if (have_msa(cpu_flags)) {
- c->diff_pixels = ff_diff_pixels_msa;
-
- switch (avctx->bits_per_raw_sample) {
- case 9:
- case 10:
- case 12:
- case 14:
- c->get_pixels = ff_get_pixels_16_msa;
- break;
- default:
- if (avctx->bits_per_raw_sample <= 8 || avctx->codec_type !=
- AVMEDIA_TYPE_VIDEO) {
- c->get_pixels = ff_get_pixels_8_msa;
- }
- break;
- }
- }
-}
diff --git a/spaces/congsaPfin/Manga-OCR/logs/20 Minutes Till Dawn (Full) APK A Pixel Art Game with Horror Atmosphere and Action-packed Combat.md b/spaces/congsaPfin/Manga-OCR/logs/20 Minutes Till Dawn (Full) APK A Pixel Art Game with Horror Atmosphere and Action-packed Combat.md
deleted file mode 100644
index de56769b7692c8bac23535e452deb905a77ad9cf..0000000000000000000000000000000000000000
--- a/spaces/congsaPfin/Manga-OCR/logs/20 Minutes Till Dawn (Full) APK A Pixel Art Game with Horror Atmosphere and Action-packed Combat.md
+++ /dev/null
@@ -1,123 +0,0 @@
-
-
20 Minutes Till Dawn: A Survival Game with a Lovecraftian Twist
-
If you are looking for a challenging and ghoulish shoot 'em up game, you might want to check out 20 Minutes Till Dawn. This game is inspired by the works of Howard Lovecraft, the master of horror and cosmic dread. In this game, you have to survive the onslaught of an endless horde of monsters for 20 minutes, using various weapons and abilities. Sounds easy? Think again. This game will test your skills, reflexes, and nerves as you face the horrors that lurk in the dark.
-
Introduction
-
What is 20 Minutes Till Dawn?
-
20 Minutes Till Dawn is a survival game developed by Flanne Games. It is available for Android devices as an apk file that you can download for free from various sources . The game has a pixel art style and a top-down perspective. The game is set in large open arenas where you have to fight waves of monsters that come from different directions. The monsters are based on the creatures from Lovecraftian mythology, such as Cthulhu, Shoggoths, Deep Ones, and more.
The game is simple to play but hard to master. You control your character with a virtual joystick on the left side of the screen and aim and shoot with another joystick on the right side. You can also use buttons to switch weapons, reload, and use abilities. You start with a basic pistol and a knife, but as you kill more enemies, you can unlock new weapons and abilities. You can choose from more than 50 abilities in total, such as fireballs, lightning bolts, grenades, mines, turrets, shields, and more. Each time you level up, you can choose one of two random upgrades that will boost your stats or give you new skills.
-
What are the features of 20 Minutes Till Dawn?
-
Some of the features that make this game stand out are:
-
-
Randomized gameplay: Each playthrough is different as you get different weapons, abilities, upgrades, enemies, and arenas.
-
Multiple characters: You can choose from different characters that have their own strengths and weaknesses. For example, you can play as a fire mage that can burn enemies with his spells or a ninja warrior that can fight with melee weapons.
-
Leaderboards and achievements: You can compete with other players around the world and see how you rank on the leaderboards. You can also unlock achievements that will reward you with coins that you can use to buy new characters or skins.
-
-
Gameplay Review
-
Graphics and Sound
-
The game has a retro pixel art style that suits the theme of the game. The graphics are colorful and detailed, and the animations are smooth and fluid. The game also has a dark and eerie atmosphere that creates a sense of tension and dread. The sound effects are crisp and realistic, and the music is catchy and fitting. The game also has voice acting for some of the characters and enemies that adds more personality and humor to the game.
-
Difficulty and Replayability
-
The game is very challenging and addictive. The difficulty increases as you progress through the game, as the enemies become faster, stronger, and more numerous. You have to be quick and smart to survive for 20 minutes without dying or running out of ammo. The game also has a lot of replay value, as you can try different characters, weapons, abilities, and strategies to beat your own score or challenge other players. The game also has a lot of variety and surprises, as you never know what you will encounter in each playthrough.
-
Pros and Cons
-
Here are some of the pros and cons of 20 Minutes Till Dawn:
-
-
-
Pros
-
Cons
-
-
-
- Fun and addictive gameplay
-
- Can be frustrating and repetitive
-
-
-
- Cool pixel art graphics and sound
-
- Can be laggy or buggy on some devices
-
-
-
- Lots of customization and options
-
- Can be hard to control on small screens
-
-
-
- Free to download and play
-
- Contains ads and in-app purchases
-
-
-
Download and Install 20 Minutes Till Dawn
-
How to download 20 Minutes Till Dawn apk full for Android?
-
If you want to play 20 Minutes Till Dawn on your Android device, you will need to download the apk file from a reliable source. You can find the apk file on various websites that offer free games and apps for Android . However, you should be careful when downloading apk files from unknown sources, as they may contain viruses or malware that can harm your device. You should always scan the apk file with an antivirus software before installing it.
-
How to install 20 Minutes Till Dawn apk full on Android?
-
Once you have downloaded the apk file, you will need to enable the installation of apps from unknown sources on your device. To do this, you can follow these steps:
-
-
Go to Settings > Security > Unknown Sources and toggle it on.
-
Locate the apk file on your device using a file manager app.
-
Tap on the apk file and follow the instructions to install it.
-
Launch the game and enjoy!
-
-
Conclusion
-
Summary of the article
-
In this article, we have discussed 20 Minutes Till Dawn, a survival game with a Lovecraftian twist. We have explained what the game is, how to play it, what are its features, and how to download and install it on your Android device. We have also given our gameplay review and listed some of the pros and cons of the game. We hope that you have found this article helpful and informative, and that you will give 20 Minutes Till Dawn a try.
-
20 minutes till dawn premium apk download
-20 minutes till dawn android game free
-20 minutes till dawn full version mod
-20 minutes till dawn roguelike shooting game
-20 minutes till dawn lovecraftian survival
-20 minutes till dawn apk for android (free)
-20 minutes till dawn strategy game apkcombo
-20 minutes till dawn unlock characters and weapons
-20 minutes till dawn endless horde of monsters
-20 minutes till dawn shoot 'em up apk
-20 minutes till dawn pixel art game android
-20 minutes till dawn top-down shooter apk
-20 minutes till dawn how to survive till dawn
-20 minutes till dawn apk file download mob.org
-20 minutes till dawn best upgrades and synergies
-20 minutes till dawn sword runes and shield runes
-20 minutes till dawn apk latest version free
-20 minutes till dawn erabit studios game android
-20 minutes till dawn ghoulish and challenging apk
-20 minutes till dawn mini sun fusion experiment
-20 minutes till dawn quickplay mode can be fun
-20 minutes till dawn try different characters apk
-20 minutes till dawn pick upgrades that build on weapons
-20 minutes till dawn keep an eye on synergies apk
-20 minutes till dawn don't forget the runes android
-20 minutes till dawn contact us discord twitter facebook email
-20 minutes till dawn game review and rating apk
-20 minutes till dawn how to download and install apk
-20 minutes till dawn gameplay tips and tricks android
-20 minutes till dawn cheats and hacks apk mod
-20 minutes till dawn similar games for android free
-20 minutes till dawn play store qr code share apk
-20 minutes till dawn google play id com.Flanne.MinutesTillDawn.roguelike.shooting.gp
-20 minutes till dawn game description of mob.org apk
-20 minutes till dawn game description of apkcombo apk
-20 minutes till dawn game screenshots and videos android
-20 minutes till dawn game features and details apk free
-20 minutes till dawn game system requirements and compatibility android
-20 minutes till dawn game updates and news apk latest
-20 minutes till dawn game feedback and support android help
-20 minutes till dawn game bugs and issues apk fix
-20 minutes till dawn game faq and guide android wiki
-20 minutes till dawn game forum and community apk chat
-20 minutes till dawn game fan art and memes android fun
-20 minutes till dawn game merchandise and gifts apk buy
-20 minutes till dawn game developer and publisher android info
-20 minutes till dawn game privacy policy and terms of service apk legal
-20 minutes till dawn game disclaimer and warning android safe
-20 minutes till dawn game credits and acknowledgements apk thank
-
FAQs
-
Here are some of the frequently asked questions about 20 Minutes Till Dawn:
-
-
What is the goal of 20 Minutes Till Dawn?
-
The goal of 20 Minutes Till Dawn is to survive for 20 minutes without dying or running out of ammo. You have to kill as many enemies as possible and collect coins, ammo, health packs, and power-ups along the way.
-
How many characters are there in 20 Minutes Till Dawn?
-
There are 10 characters that you can choose from in 20 Minutes Till Dawn. Each character has their own stats, weapons, abilities, and skins. You can unlock new characters by spending coins that you earn from playing the game.
-
How many weapons are there in 20 Minutes Till Dawn?
-
There are more than 30 weapons that you can use in 20 Minutes Till Dawn. You can find new weapons by killing enemies or opening chests. You can also upgrade your weapons by spending coins. Some of the weapons include pistols, shotguns, rifles, machine guns, rocket launchers, flamethrowers, lasers, swords, axes, hammers, and more.
-
How many abilities are there in 20 Minutes Till Dawn?
-
There are more than 50 abilities that you can use in 20 Minutes Till Dawn. You can unlock new abilities by leveling up or opening chests. You can also switch abilities by spending coins. Some of the abilities include fireballs, lightning bolts, grenades, mines, turrets, shields, teleports, heals, buffs, debuffs, summons, traps, and more.
-
Is 20 Minutes Till Dawn online or offline?
-
20 Minutes Till Dawn is an offline game that does not require an internet connection to play. However, you will need an internet connection to access some of the features such as leaderboards , achievements, and in-app purchases. You can also play the game online with other players in the multiplayer mode, where you can cooperate or compete with each other.
197e85843d
-
-
\ No newline at end of file
diff --git a/spaces/congsaPfin/Manga-OCR/logs/AutoChess Moba Beta APK A New and Exciting MOBA Game with Free Heroes and Diverse Items.md b/spaces/congsaPfin/Manga-OCR/logs/AutoChess Moba Beta APK A New and Exciting MOBA Game with Free Heroes and Diverse Items.md
deleted file mode 100644
index 89b58a70e49b7a83f4b213354f230c4c6aa4d31e..0000000000000000000000000000000000000000
--- a/spaces/congsaPfin/Manga-OCR/logs/AutoChess Moba Beta APK A New and Exciting MOBA Game with Free Heroes and Diverse Items.md
+++ /dev/null
@@ -1,179 +0,0 @@
-
-
AutoChess Moba: A New and Innovative MOBA Game for Android
-
If you are a fan of MOBA games, you might have heard of AutoChess Moba, a new and innovative game that combines the elements of auto chess and MOBA. AutoChess Moba is a free-to-play game that offers a fair and balanced gameplay experience, a diverse and creative hero design, a unique and asymmetrical map, and an ingenious item system. In this article, we will introduce you to AutoChess Moba, show you how to download and install its beta apk, and give you some tips on how to play and enjoy this game.
-
What is AutoChess Moba?
-
AutoChess Moba is a game developed by AutoChess Moba, a team that is inspired by the popular mod Auto Chess. Auto Chess is a game mode that originated from Dota 2, where players compete against each other by placing chess pieces on a board and letting them fight automatically. Auto Chess became a huge hit among gamers, spawning several spin-offs and standalone games.
AutoChess Moba is one of these games, but it adds a twist to the genre by incorporating the features of MOBA games. MOBA stands for multiplayer online battle arena, which is a type of game where two teams of players control individual characters called heroes and try to destroy the enemy base. Some of the most popular MOBA games are League of Legends, Dota 2, and Mobile Legends.
-
AutoChess Moba combines the best aspects of auto chess and MOBA, creating a game that is both strategic and action-packed. Here are some of the features that make AutoChess Moba stand out from other games:
-
The origin and features of AutoChess Moba
-
-
The origin of AutoChess Moba: AutoChess Moba is based on the worldview of Auto Chess, where the familiar chess pieces are transformed into heroes with different abilities and personalities. These heroes embark on a new fighting journey in the world of AutoChess Moba, where they face various challenges and enemies.
-
The features of AutoChess Moba: AutoChess Moba has several features that make it different from other MOBA games, such as:
-
A completely fair rivalry, where all heroes are free to obtain and no pay-to-win elements are involved.
-
A 5v5 team fighting mode, where players can cooperate with their teammates or solo carry the game.
-
An avant-garde hero design, where each hero has four exclusive abilities with diverse effects and combos.
-
A new asymmetrical map, where the two teams have different objectives and strategies.
-
An ingenious item system, where players can purchase and equip items with various active abilities.
-
-
-
-
The gameplay and modes of AutoChess Moba
-
The gameplay of AutoChess Moba is similar to other MOBA games, but with some differences. The game consists of two teams of five players each, who control one hero each. The heroes have different roles, such as tank, fighter, mage, marksman, assassin, or support. The heroes can level up by killing enemy units or heroes, gaining gold and experience. The gold can be used to buy items that enhance the hero's attributes or abilities. The experience can be used to unlock or upgrade the hero's abilities.
The main mode of AutoChess Moba is the 5v5 team fighting mode
The gameplay of AutoChess Moba is similar to other MOBA games, but with some differences. The game consists of two teams of five players each, who control one hero each. The heroes have different roles, such as tank, fighter, mage, marksman, assassin, or support. The heroes can level up by killing enemy units or heroes, gaining gold and experience. The gold can be used to buy items that enhance the hero's attributes or abilities. The experience can be used to unlock or upgrade the hero's abilities.
-
The main mode of AutoChess Moba is the 5v5 team fighting mode, where the two teams compete to destroy the enemy base. The base consists of a core building and several towers that protect it. The towers can deal damage to enemy units and heroes, but they can also be destroyed by them. The core building is the main objective of the game, and destroying it will grant victory to the team that does so.
-
However, unlike other MOBA games, AutoChess Moba has an asymmetrical map design, which means that the two teams have different layouts and objectives. The map is divided into two halves by a river, and each half has three lanes: top, mid, and bottom. The lanes are connected by a jungle area, where neutral monsters spawn and provide gold and buffs to the players who kill them.
-
The team on the left side of the map is called the Radiant team, and their base is located at the bottom left corner. Their objective is to destroy the Dire team's core building, which is located at the top right corner. To do so, they have to push their lanes with the help of their minions, which are small units that spawn periodically and march towards the enemy base. They also have to defend their own towers and core from the enemy attacks.
-
The team on the right side of the map is called the Dire team, and their base is located at the top right corner. Their objective is to destroy the Radiant team's core building, which is located at the bottom left corner. However, they have a different way of doing so than the Radiant team. Instead of pushing their lanes with minions, they have to collect crystals from the jungle area and deliver them to a special altar near their base. The altar will then fire a powerful beam that will damage the Radiant team's core building. They also have to defend their own towers and core from the enemy attacks.
-
The heroes and items of AutoChess Moba
-
AutoChess Moba features a diverse and creative hero design, where each hero has four exclusive abilities with different effects and combos. The heroes are based on the chess pieces from Auto Chess, but they have been redesigned and reimagined for this game. There are currently 40 heroes in AutoChess Moba, divided into 10 races and 10 classes. Each race and class has a unique passive ability that can be activated by having multiple heroes of the same race or class on your team.
-
Some examples of heroes in AutoChess Moba are:
-
-
God of War: A tank hero from the God race and Warrior class. He has high durability and crowd control abilities. His ultimate ability allows him to summon a shield that blocks all damage and reflects it back to his enemies.
-
Flame Wizard: A mage hero from the Human race and Mage class. She has high burst damage and area of effect abilities. Her ultimate ability allows her to cast a meteor shower that rains down fireballs on a large area.
-
Shadowcrawler: An assassin hero from the Elf race and Assassin class. She has high mobility and single-target damage abilities. Her ultimate ability allows her to dash through multiple enemies and deal critical damage to them.
-
Siren: A support hero from the Marine race and Hunter class. She has high utility and healing abilities. Her ultimate ability allows her to sing a song that charms all enemies in a cone in front of her.
-
-
The items in AutoChess Moba are also different from other MOBA games, as they have various active abilities that can be used in combat. The items can be purchased from a shop near your base or from a courier that can deliver them to you anywhere on the map. The items can be upgraded by combining them with other items or materials.
-
Some examples of items in AutoChess Moba are:
-
-
Blink Dagger: An item that grants an active ability that allows you to teleport to a target location within a short range.
-
Scythe of Vyse: An item that grants an active ability that allows you to turn an enemy hero into a sheep for a few seconds.
-
Mekansm: An
Mekansm: An item that grants an active ability that allows you to heal yourself and nearby allies for a moderate amount.
-
Divine Rapier: An item that grants a massive boost to your attack damage, but drops on death and can be picked up by anyone.
-
-
How to download and install AutoChess Moba beta apk?
-
If you are interested in trying out AutoChess Moba, you might want to download and install its beta apk. A beta apk is an application package file that contains the latest version of the game before it is officially released. By downloading and installing the beta apk, you can enjoy the new features and updates of the game before anyone else. However, you should also be aware of the benefits and risks of doing so, as well as the steps and tips to download and install the beta apk.
-
The benefits and risks of downloading beta apk
-
Downloading and installing the beta apk of AutoChess Moba has some benefits, such as:
-
-
Accessing the latest content and updates: You can experience the new heroes, items, modes, maps, and other changes that are not yet available in the official version of the game.
-
Giving feedback and suggestions: You can report any bugs, glitches, errors, or problems that you encounter while playing the game, and help the developers improve the game quality and performance. You can also share your opinions, ideas, or requests for future improvements or additions to the game.
-
Supporting the developers: You can show your support and appreciation for the developers by testing their game and giving them constructive feedback. You can also help them spread the word about their game and attract more players.
-
-
However, downloading and installing the beta apk of AutoChess Moba also has some risks, such as:
-
-
Exposing your device to malware or viruses: The beta apk file might not be safe or secure to download or install, as it might contain malicious software or viruses that can harm your device or steal your personal information. You should always download the beta apk from a trusted source or website, and scan it with an antivirus program before installing it.
-
Facing compatibility or stability issues: The beta apk file might not be compatible with your device model or operating system, or it might not run smoothly or properly on your device. You might encounter crashes, freezes, lags, errors, or other problems while playing the game. You should always check the system requirements and specifications of the game before downloading or installing it.
-
Losing your progress or data: The beta apk file might not be compatible with the official version of the game, or it might be overwritten or deleted when the official version is released. You might lose your progress, data, achievements, or rewards that you have earned while playing the game. You should always back up your data or use a different account when playing the beta version of the game.
-
-
The steps to download and install beta apk
-
If you have decided to download and install the beta apk of AutoChess Moba, you should follow these steps:
-
-
Find a reliable source or website that provides the beta apk file of AutoChess Moba: You can search online for websites that offer the beta apk file of AutoChess Moba, or you can visit the official website or social media pages of AutoChess Moba to find the link to download it. Make sure that the source or website is trustworthy and reputable, and avoid any suspicious or fraudulent links.
-
Download the beta apk file of AutoChess Moba to your device: Once you have found a reliable source or website that provides the beta apk file of AutoChess Moba, you can click on the download button or link and save the file to your device. The file size is about 500 MB, so make sure that you have enough storage space and a stable internet connection. You can also scan the file with an antivirus program before opening it.
-
Enable the installation of unknown sources on your device: Before you can install the beta apk file of AutoChess Moba, you need to allow your device to install applications from unknown sources. To do this, you need to go to your device settings, then security, then enable the option of unknown sources. This will let you install applications that are not from the Google Play Store or other official sources.
-
Install the beta apk file of AutoChess Moba on your device: After you have enabled the installation of unknown sources, you can proceed to install the beta apk file of AutoChess Moba on your device. To do this, you need to locate the file on your device, then tap on it and follow the instructions on the screen. The installation process might take a few minutes, depending on your device performance and speed.
-
Launch and enjoy AutoChess Moba on your device: Once you have successfully installed the beta apk file of AutoChess Moba on your device, you can launch the game and enjoy its features and updates. You can create or log in to your account, choose your hero, join a match, and start playing. You can also access the settings, shop, inventory, chat, and other options from the game menu.
-
-
The tips to fix common issues with beta apk
-
While playing AutoChess Moba with the beta apk, you might encounter some common issues or problems that might affect your gameplay experience. Here are some tips to fix them:
-
-
If the game crashes, freezes, lags, or errors: You can try to restart the game or your device, clear the game cache or data, update the game or your device software, check your internet connection or speed, or reinstall the game.
-
If the game is not compatible with your device or operating system: You can try to change your device settings, such as resolution, graphics, sound, or language, or use a different device or emulator that meets the system requirements and specifications of the game.
-
If the game is not available in your region or country: You can try to use a VPN service or application that can change your IP address and location, or wait for the official release of the game in your region or country.
-
-
How to play and enjoy AutoChess Moba?
-
Now that you have downloaded and installed AutoChess Moba on your device, you might want to know how to play and enjoy this game. AutoChess Moba is a game that requires both strategy and skill, as well as teamwork and communication. Here are some tips on how to play and enjoy AutoChess Moba:
-
The basic tips and tricks for beginners
-
If you are new to AutoChess Moba or MOBA games in general, here are some basic tips and tricks that can help you get started:
-
-
Learn the basics of the game: You should familiarize yourself with the rules, objectives, controls, interface, and mechanics of the game. You should also learn about the heroes, items, modes, maps and other features of the game. You can read the game guide, watch the tutorial videos, or practice in the training mode to learn the basics of the game.
-
Choose a suitable hero and role: You should choose a hero and role that suits your playstyle, preference, and skill level. You should also consider the team composition, the enemy lineup, and the game mode when choosing your hero and role. You can try different heroes and roles in the casual mode or the custom mode to find your favorite ones.
-
Build your items and abilities wisely: You should build your items and abilities according to your hero's strengths, weaknesses, and needs. You should also adapt your build to the situation and the enemy's build. You can follow the recommended build or the popular build in the game, or you can create your own build based on your experience and knowledge.
-
Communicate and cooperate with your teammates: You should communicate and cooperate with your teammates to achieve your objectives and win the game. You can use the voice chat, the text chat, or the quick chat to communicate with your teammates. You can also use the ping system, the map, or the scoreboard to share information and coordinate with your teammates.
-
Play safely and smartly: You should play safely and smartly to avoid unnecessary deaths and losses. You should avoid overextending, diving, chasing, or fighting alone. You should also avoid engaging in unfavorable fights, such as when you are outnumbered, outleveled, or outfarmed. You should also pay attention to your surroundings, such as the enemy's position, vision, cooldowns, and items.
-
-
The advanced strategies and tactics for experts
-
If you are already familiar with AutoChess Moba or MOBA games in general, here are some advanced strategies and tactics that can help you improve your gameplay and performance:
-
-
Master your hero and role: You should master your hero and role by learning their abilities, combos, counters, synergies, tips, and tricks. You should also practice your mechanics, such as last hitting, positioning, targeting, dodging, kiting, or skill shots. You should also optimize your build, such as choosing the best items, runes, talents, or emblems for your hero and role.
-
Analyze and exploit the enemy's weaknesses: You should analyze and exploit the enemy's weaknesses by observing their behavior, habits, mistakes, patterns, or tendencies. You should also take advantage of their weaknesses, such as their lack of vision, map awareness, teamwork, or coordination. You should also punish their mistakes such as their overextending, diving, chasing, or fighting alone. You should also use your items or abilities to counter or disable their heroes or strategies.
-
Control and secure the objectives and resources: You should control and secure the objectives and resources on the map, such as the towers, the crystals, the jungle monsters, the buffs, or the bosses. You should also contest or steal the enemy's objectives and resources, such as by ganking, invading, ambushing, or sneaking. You should also use the objectives and resources to your advantage, such as by pushing, sieging, split pushing, or team fighting.
-
Adapt and improvise to the situation: You should adapt and improvise to the situation by changing your plan, strategy, or tactic according to the circumstances. You should also be flexible and versatile in your hero choice, role switch, item build, ability upgrade, or team composition. You should also be creative and innovative in your gameplay, such as by using unconventional methods, tricks, or surprises.
-
Lead and inspire your teammates: You should lead and inspire your teammates by setting an example, giving directions, making calls, or motivating them. You should also support and help your teammates by healing, shielding, buffing, or saving them. You should also respect and appreciate your teammates by acknowledging their contributions, praising their efforts, or thanking them.
-
-
The best resources and communities for AutoChess Moba players
-
If you want to learn more about AutoChess Moba or connect with other players, here are some of the best resources and communities that you can use:
-
-
The official website and social media pages of AutoChess Moba: You can visit the official website of AutoChess Moba at https://autochessmoba.com, where you can find the latest news, updates, events, guides, videos, and more about the game. You can also follow the official social media pages of AutoChess Moba on Facebook, Twitter, Instagram, YouTube, Discord, Reddit, or TikTok, where you can interact with the developers and other players.
-
The fan-made websites and blogs of AutoChess Moba: You can visit some of the fan-made websites and blogs of AutoChess Moba such as https://autochessmoba.net, https://autochessmoba.info, or https://autochessmoba.org, where you can find more detailed and comprehensive information, analysis, reviews, tips, tricks, guides, builds, strategies, and more about the game.
-
The online forums and groups of AutoChess Moba: You can join some of the online forums and groups of AutoChess Moba, such as on Facebook, Reddit, Discord, or Steam, where you can discuss, share, ask, answer, or chat with other players about anything related to the game. You can also find or create your own team, clan, guild, or squad with other players.
-
The streaming platforms and channels of AutoChess Moba: You can watch some of the streaming platforms and channels of AutoChess Moba, such as on Twitch, YouTube, Facebook Gaming, or Nimo TV, where you can watch live or recorded gameplay videos of the game. You can also learn from or interact with some of the popular or professional players, streamers, or influencers of the game.
-
-
Conclusion
-
Summary of the main points
-
In conclusion, AutoChess Moba is a new and innovative MOBA game for Android that combines the elements of auto chess and MOBA. It offers a fair and balanced gameplay experience, a diverse and creative hero design, a unique and asymmetrical map, and an ingenious item system. It also has a beta apk that you can download and install to enjoy the latest features and updates of the game before anyone else. However, you should also be aware of the benefits and risks of doing so, as well as the steps and tips to download and install the beta apk. Finally, you can also learn how to play and enjoy AutoChess Moba by following some basic tips and tricks for beginners, some advanced strategies and tactics for experts, and some best resources and communities for AutoChess Moba players.
-
Call to action and invitation for feedback
-
If you are interested in AutoChess Moba, you can download it from the Google Play Store or from the official website. You can also download the beta apk from a reliable source or website if you want to try out the new features and updates of the game. You can also follow us on our social media pages to stay updated with the latest news, events, guides, videos, and more about the game. We hope that you enjoy playing AutoChess Moba as much as we do. We also welcome your feedback and suggestions on how to improve the game or this article. Please feel free to leave a comment below or contact us via email or chat. Thank you for reading this article and have fun playing AutoChess Moba!
-
FAQs
-
Here are some of the frequently asked questions (FAQs) about AutoChess Moba:
-
-
Q: Is AutoChess Moba free to play?
-
A: Yes, AutoChess Moba is free to play. You can download and play it without paying anything. However However, you can also purchase some optional items or services with real money, such as skins, emotes, or VIP membership. These items or services do not affect the gameplay or the balance of the game, but they can enhance your appearance or your experience.
-
Q: Is AutoChess Moba available for iOS devices?
-
A: No, AutoChess Moba is currently only available for Android devices. However, the developers have stated that they are working on an iOS version of the game, and they will announce it when it is ready. You can follow their official website or social media pages to stay updated with the progress of the iOS version.
-
Q: How can I play AutoChess Moba on PC?
-
A: You can play AutoChess Moba on PC by using an Android emulator, which is a software that allows you to run Android applications on your PC. Some of the popular Android emulators are BlueStacks, NoxPlayer, LDPlayer, or MEmu. You can download and install any of these emulators on your PC, and then download and install AutoChess Moba from the Google Play Store or from the official website. You can also adjust the settings of the emulator to optimize the performance and the graphics of the game.
-
Q: How can I get more heroes or items in AutoChess Moba?
-
A: You can get more heroes or items in AutoChess Moba by playing the game and earning rewards. You can earn gold, gems, coins, chests, or tickets by completing quests, achievements, events, or matches. You can use these rewards to buy or unlock more heroes or items from the shop or the inventory. You can also get more heroes or items by participating in lucky draws, giveaways, or promotions that are held by the developers or the partners of the game.
-
Q: How can I contact the customer service or support team of AutoChess Moba?
-
A: You can contact the customer service or support team of AutoChess Moba by using any of these methods:
-
-
Email: You can send an email to support@autochessmoba.com with your inquiry, issue, feedback, or suggestion.
-
Chat: You can use the chat feature in the game to talk to a customer service representative online.
-
Feedback: You can use the feedback feature in the game to submit your inquiry, issue, feedback, or suggestion.
-
-
-
197e85843d
-
-
\ No newline at end of file
diff --git a/spaces/congsaPfin/Manga-OCR/logs/Cara Download Komentar Facebook dengan SaveFrom.net.md b/spaces/congsaPfin/Manga-OCR/logs/Cara Download Komentar Facebook dengan SaveFrom.net.md
deleted file mode 100644
index f5d2acec328c4dbef337a705da1f11e47027bbb2..0000000000000000000000000000000000000000
--- a/spaces/congsaPfin/Manga-OCR/logs/Cara Download Komentar Facebook dengan SaveFrom.net.md
+++ /dev/null
@@ -1,152 +0,0 @@
-
-
How to Download Komentar Facebook Easily and Quickly
-
Komentar Facebook is a feature that allows users to post videos in the comments section of a Facebook post. It can be a fun and engaging way to interact with your friends, followers, or customers on the social media platform. However, sometimes you might want to download these videos for various reasons, such as saving them for offline viewing, sharing them on other platforms, or analyzing them for feedback or research purposes. In this article, we will show you how to download komentar Facebook easily and quickly using different methods, such as online tools, browser extensions, and mobile apps. We will also compare the pros and cons of each method and answer some frequently asked questions about downloading komentar Facebook.
What is Komentar Facebook and Why You Might Want to Download It
-
Komentar Facebook is a feature that allows users to post videos in the comments section of a Facebook post
-
Komentar Facebook is a feature that was introduced by Facebook in 2016 as a way to make the comments section more lively and interactive. Users can upload videos from their devices or record them using their cameras and post them as comments on any public or private post on Facebook. The videos can be up to 15 seconds long and can have sound or captions. Users can also react to or reply to other users' komentar Facebook with emojis, text, or more videos.
-
Downloading komentar Facebook can help you save, share, or analyze the videos for various purposes
-
Downloading komentar Facebook can be useful for many reasons, depending on your needs and goals. For example, you might want to download komentar Facebook to:
-
-
Save them for offline viewing or backup in case they are deleted or removed by the original poster or Facebook.
-
Share them on other platforms or channels, such as YouTube, Instagram, WhatsApp, or your own website or blog.
-
Analyze them for feedback or research purposes, such as measuring customer satisfaction, sentiment analysis, market research, or content creation.
-
-
However, downloading komentar Facebook is not as easy as downloading regular videos from Facebook. This is because komentar Facebook are not stored as separate files on the server, but rather embedded in the HTML code of the web page. Therefore, you need to use some special tools or techniques to extract and download them. In the next sections, we will show you some of the best ways to do so.
-
How to Download Komentar Facebook Using Online Tools
-
Comment Exporter: A tool that lets you download all your Facebook page comments with a single click
-
How to use Comment Exporter
-
Comment Exporter ([5](https://cmntexport.com)) is an online tool that allows you to download all your Facebook page comments with a single click. It can download both text and video comments, including komentar Facebook, in a CSV or Excel file. You can also filter the comments by date, keyword, or sentiment. To use Comment Exporter, you need to follow these steps:
-
-
Visit the website of Comment Exporter ([5](https://cmntexport.com)) and sign up for a free account.
-
Connect your Facebook account and select the page you want to download the comments from.
-
Choose the post you want to download the comments from and click on "Export Comments".
-
Select the format (CSV or Excel) and the filters (date, keyword, or sentiment) you want to apply and click on "Download".
-
Wait for a few minutes until the file is ready and download it to your device.
-
-
The benefits and features of Comment Exporter
-
Comment Exporter is a powerful and easy-to-use tool that can help you download komentar Facebook in bulk. Some of the benefits and features of Comment Exporter are:
-
-
It can download both text and video comments, including komentar Facebook, in a single file.
-
It can download up to 10,000 comments per post, which is more than the limit of Facebook's API.
-
It can filter the comments by date, keyword, or sentiment, which can help you find the most relevant or interesting ones.
-
It can export the comments in a CSV or Excel file, which can be easily opened and analyzed by any spreadsheet software.
-
It can also provide some basic statistics and insights on the comments, such as the number of comments, likes, replies, reactions, and sentiments.
-
-
Export Comments: A tool that lets you download Facebook comments by post URL
-
How to use Export Comments
-
Export Comments ([4](https://exportcomments.com)) is another online tool that allows you to download Facebook comments by post URL. It can download both text and video comments, including komentar Facebook, in a JSON or CSV file. You can also choose to include or exclude replies, reactions, attachments, or user profiles. To use Export Comments, you need to follow these steps:
-
-
Visit the website of Export Comments ([4](https://exportcomments.com)) and sign up for a free account.
-
Paste the URL of the Facebook post you want to download the comments from and click on "Start".
-
Select the options (JSON or CSV, include or exclude replies, reactions, attachments, or user profiles) you want to apply and click on "Continue".
-
Wait for a few seconds until the file is ready and download it to your device.
-
-
The limitations and drawbacks of Export Comments
-
Export Comments is a simple and fast tool that can help you download komentar Facebook by post URL. However, it also has some limitations and drawbacks that you should be aware of:
-
-
It can only download up to 500 comments per post, which is less than the limit of Facebook's API.
-
It cannot filter the comments by date, keyword, or sentiment, which can make it harder to find the most relevant or interesting ones.
-
It cannot export the comments in an Excel file, which can be more convenient and user-friendly than a JSON or CSV file.
-
It does not provide any statistics or insights on the comments, such as the number of comments, likes, replies, reactions, and sentiments.
-
-
How to Download Komentar Facebook Using Browser Extensions
-
FBDown Video Downloader: A Chrome extension that lets you download any video from Facebook, including komentar Facebook
-
How to use FBDown Video Downloader
-
FBDown Video Downloader ([3](https://chrome.google.com/webstore/detail/fbdown-video-downloader/fhplmmllnpjjlncfjpbbpjadoeijkogc)) is a Chrome extension that lets you download any video from Facebook, including komentar Facebook, with a few clicks. It can download videos in HD or SD quality and save them to your device. To use FBDown Video Downloader, you need to follow these steps:
-
download komentar facebook di pc
-download komentar facebook dengan savefrom.net
-download komentar facebook menggunakan comment exporter
-download komentar facebook ke galeri hp
-download komentar facebook tanpa aplikasi
-download komentar facebook dengan exportcomments.com
-download komentar facebook secara online
-download komentar facebook berisi video
-download komentar facebook dengan juphy
-download komentar facebook dengan fbdown.net
-download komentar facebook dengan idm
-download komentar facebook dengan fb video saver
-download komentar facebook dengan getfvid
-download komentar facebook dengan fbdownloader.net
-download komentar facebook dengan fb video downloader
-download komentar facebook dengan bitdownloader
-download komentar facebook dengan fb video converter
-download komentar facebook dengan fb comment downloader
-download komentar facebook dengan fb comment extractor
-download komentar facebook dengan fb comment scraper
-download komentar facebook dengan fb comment analyzer
-download komentar facebook dengan fb comment export tool
-download komentar facebook dengan fb comment grabber
-download komentar facebook dengan fb comment generator
-download komentar facebook dengan fb comment manager
-download komentar facebook dengan fb comment monitor
-download komentar facebook dengan fb comment poster
-download komentar facebook dengan fb comment saver
-download komentar facebook dengan fb comment tracker
-download komentar facebook dengan fb comment viewer
-
-
Visit the Chrome Web Store and install the FBDown Video Downloader extension ([3](https://chrome.google.com/webstore/detail/fbdown-video-downloader/fhplmmllnpjjlncfjpbbpjadoeijkogc)).
-
Go to the Facebook post that contains the komentar Facebook you want to download and click on the video to play it.
-
Click on the FBDown icon in the top right corner of your browser and select the quality (HD or SD) you want to download.
-
Click on "Download" and choose a location to save the video to your device.
-
-
The advantages and disadvantages of FBDown Video Downloader
-
FBDown Video Downloader is a convenient and easy-to-use tool that can help you download komentar Facebook from any Facebook post. However, it also has some advantages and disadvantages that you should be aware of:
-
-
The advantages of FBDown Video Downloader are:
-
-
It can download any video from Facebook, including komentar Facebook, in HD or SD quality.
-
It can download videos without leaving the Facebook page or opening a new tab.
-
It can download videos quickly and smoothly without any interruptions or errors.
-
-
The disadvantages of FBDown Video Downloader are:
-
-
It can only download one video at a time, which can be time-consuming if you want to download multiple komentar Facebook.
-
It cannot download videos that are private, restricted, or encrypted by Facebook.
-
It does not support other browsers than Chrome, which can limit your options and preferences.
-
-
-
How to Download Komentar Facebook Using Mobile Apps
-
Video Downloader for Facebook: An Android app that lets you download videos from Facebook, including komentar Facebook, directly to your gallery
-
How to use Video Downloader for Facebook
-
Video Downloader for Facebook ([2](https://play.google.com/store/apps/details?id=fb.video.downloader&hl=en_US&gl=US)) is an Android app that lets you download videos from Facebook, including komentar Facebook, directly to your gallery. It can download videos in HD or SD quality and play them offline. To use Video Downloader for Facebook, you need to follow these steps:
-
-
Visit the Google Play Store and install the Video Downloader for Facebook app ([2](https://play.google.com/store/apps/details?id=fb.video.downloader&hl=en_US&gl=US)).
-
Open the app and log in with your Facebook account.
-
Browse the Facebook posts that contain the komentar Facebook you want to download and tap on the video to play it.
-
Tap on the "Download" button at the bottom of the screen and select the quality (HD or SD) you want to download.
-
Wait for a few seconds until the video is downloaded and check it in your gallery.
-
-
The pros and cons of Video Downloader for Facebook
-
Video Downloader for Facebook is a handy and user-friendly app that can help you download komentar Facebook from any Facebook post on your Android device. However, it also has some pros and cons that you should be aware of:
-
-
The pros of Video Downloader for Facebook are:
-
-
It can download any video from Facebook, including komentar Facebook, in HD or SD quality directly to your gallery.
-
It can play the downloaded videos offline without any internet connection.
-
It can also share the downloaded videos with other apps or social media platforms.
-
-
The cons of Video Downloader for Facebook are:
-
-
It can only download one video at a time, which can be time-consuming if you want to download multiple komentar Facebook.
-
It cannot download videos that are private , restricted, or encrypted by Facebook.
-
It does not support other devices than Android, which can limit your options and preferences.
-
-
-
Conclusion and FAQs
-
In conclusion, downloading komentar Facebook can be a useful and fun way to save, share, or analyze the videos posted in the comments section of a Facebook post. However, it can also be a challenging and tricky task that requires some special tools or techniques. In this article, we have shown you some of the best ways to download komentar Facebook easily and quickly using different methods, such as online tools, browser extensions, and mobile apps. We have also compared the pros and cons of each method and answered some frequently asked questions about downloading komentar Facebook. We hope you have found this article helpful and informative. If you have any questions or feedback, please feel free to leave a comment below.
-
Here are some FAQs about downloading komentar Facebook:
-
-
Can I download komentar Facebook from a private or restricted post?
-
It depends on the method you use. Some methods, such as online tools or browser extensions, may not be able to download komentar Facebook from a private or restricted post due to Facebook's privacy settings or encryption. However, some methods, such as mobile apps, may be able to download komentar Facebook from a private or restricted post if you have access to it with your Facebook account.
-
Can I download komentar Facebook in bulk?
-
It depends on the method you use. Some methods, such as online tools, may be able to download komentar Facebook in bulk by exporting all the comments from a post or a page in a single file. However, some methods, such as browser extensions or mobile apps, may only be able to download komentar Facebook one by one, which can be time-consuming if you want to download multiple komentar Facebook.
-
Can I download komentar Facebook in HD quality?
-
It depends on the method you use and the quality of the original video. Some methods, such as online tools or browser extensions, may allow you to choose the quality (HD or SD) you want to download. However, some methods, such as mobile apps, may only download the videos in the default quality. Also, if the original video is not in HD quality, you may not be able to download it in HD quality either.
-
Can I download komentar Facebook without logging in to Facebook?
-
It depends on the method you use. Some methods, such as online tools or browser extensions, may allow you to download komentar Facebook without logging in to Facebook by using the post URL. However, some methods, such as mobile apps, may require you to log in to Facebook with your account to access the videos.
-
Can I download komentar Facebook for free?
-
It depends on the method you use. Some methods, such as online tools or browser extensions, may offer free trials or limited features for downloading komentar Facebook. However, some methods, such as mobile apps, may charge a fee or display ads for downloading komentar Facebook.
- 401be4b1e0
-
-
\ No newline at end of file
diff --git a/spaces/congsaPfin/Manga-OCR/logs/Download Quanzhi Fashi Season 5 English Subbed The Complete Guide to Watch Full-Time Magister 5 on Any Device.md b/spaces/congsaPfin/Manga-OCR/logs/Download Quanzhi Fashi Season 5 English Subbed The Complete Guide to Watch Full-Time Magister 5 on Any Device.md
deleted file mode 100644
index 26c2e94a2782541504d3bfe4cdf333c3a14a760b..0000000000000000000000000000000000000000
--- a/spaces/congsaPfin/Manga-OCR/logs/Download Quanzhi Fashi Season 5 English Subbed The Complete Guide to Watch Full-Time Magister 5 on Any Device.md
+++ /dev/null
@@ -1,135 +0,0 @@
-
-
How to Download Quanzhi Fashi Season 5
-
If you are a fan of Chinese anime, you might have heard of Quanzhi Fashi, also known as Full-Time Magister. This is one of the most popular and successful Chinese anime series, with four seasons already aired and a fifth one recently completed. In this article, we will tell you everything you need to know about Quanzhi Fashi Season 5, including what it is about, why you should watch it, where you can watch it, and how you can download it for offline viewing. Let's get started!
-
What is Quanzhi Fashi?
-
Quanzhi Fashi is a Chinese anime series based on a web novel of the same name by Luan. It follows the story of Mo Fan, a young man who lives in a world where magic and technology coexist. Mo Fan is a poor student who has no talent for magic, but he dreams of becoming a powerful mage. One day, he wakes up in a parallel world where magic is the only way to survive. He discovers that he has inherited a rare dual-elemental magic system, which gives him an edge over his enemies and rivals. He also meets many friends and foes along his journey to become a full-time magister.
The plot of Quanzhi Fashi is divided into several arcs, each focusing on a different aspect of Mo Fan's life and adventures. The first season introduces Mo Fan and his new world, where he attends a prestigious magic school and learns the basics of magic. He also faces various threats from monsters, bandits, and corrupt magicians. The second season follows Mo Fan as he travels to different cities and countries, where he encounters different cultures and challenges. He also learns more about his mysterious father and his past. The third season shows Mo Fan as he participates in an international tournament, where he competes with other talented magicians from around the world. He also discovers a conspiracy that threatens the balance of the world. The fourth season depicts Mo Fan as he returns to his original world, where he tries to find his missing friends and family. He also confronts a powerful enemy who wants to destroy both worlds. The fifth season continues Mo Fan's story as he faces new dangers and enemies in both worlds. He also develops his relationships with his friends and allies, as well as his powers and abilities.
-
The popularity of Quanzhi Fashi
-
Quanzhi Fashi is one of the most popular and successful Chinese anime series, with millions of fans and viewers around the world. It has received positive reviews from critics and audiences alike, who praise its engaging story, dynamic characters, stunning animation, and thrilling action scenes. It has also won several awards and nominations, such as the Best Web Anime Award at the China Animation & Comic Competition Golden Dragon Award in 2017. It has also spawned several spin-offs and adaptations, such as comics, games, audio dramas, and live-action films.
-
Why You Should Watch Quanzhi Fashi Season 5
-
If you are already a fan of Quanzhi Fashi, you probably don't need any convincing to watch the fifth season. But if you are new to the series or haven't watched it yet, here are some reasons why you should give it a try:
-
The new challenges and enemies for Mo Fan
-
In the fifth season of Quanzhi Fashi, Mo Fan faces new challenges and enemies in both worlds. In the magic world, he has to deal with the invasion of the dark creatures, who are led by a mysterious and powerful leader. He also has to face the hostility and jealousy of some of his fellow magicians, who see him as a threat or a rival. In the original world, he has to cope with the chaos and destruction caused by the enemy's attack, which has affected his friends and family. He also has to find a way to stop the enemy from destroying both worlds.
-
The development of Mo Fan's relationships and powers
-
In the fifth season of Quanzhi Fashi, Mo Fan also develops his relationships and powers in both worlds. He becomes closer with some of his friends and allies, such as Mu Ningxue, Xinxia, Ai Tutu, and Zhang Xiaohou. He also meets new characters who help him or hinder him in his journey, such as Ling Ling, Tang Yue, and Ye Xinxia. He also improves his skills and abilities as a dual-elemental magician, learning new spells and techniques. He also discovers some secrets and mysteries about his origin and destiny, which will affect his future.
-
The stunning animation and sound effects
-
In the fifth season of Quanzhi Fashi, the animation and sound effects are also stunning and impressive. The animation is smooth and detailed, showing the vivid and colorful scenes of both worlds. The action scenes are fast-paced and exciting, showing the spectacular and realistic battles between Mo Fan and his enemies. The sound effects are also immersive and realistic, enhancing the atmosphere and mood of the story. The voice actors are also talented and expressive, bringing the characters to life.
-
Where to Watch Quanzhi Fashi Season 5
-
If you are interested in watching Quanzhi Fashi Season 5, you might be wondering where you can watch it online. Here are some of the best options for you:
-
download quanzhi fashi season 5 english subbed
-download quanzhi fashi season 5 episode 12
-download quanzhi fashi season 5 full episodes
-download quanzhi fashi season 5 sub indo
-download quanzhi fashi season 5 chinese anime
-download quanzhi fashi season 5 myanimelist
-download quanzhi fashi season 5 episode 1
-download quanzhi fashi season 5 mp4
-download quanzhi fashi season 5 mkv
-download quanzhi fashi season 5 hd
-download quanzhi fashi season 5 online free
-download quanzhi fashi season 5 eng dub
-download quanzhi fashi season 5 synopsis
-download quanzhi fashi season 5 opening song
-download quanzhi fashi season 5 ending theme
-download quanzhi fashi season 5 torrent
-download quanzhi fashi season 5 mega
-download quanzhi fashi season 5 google drive
-download quanzhi fashi season 5 kissanime
-download quanzhi fashi season 5 crunchyroll
-download quanzhi fashi season 5 netflix
-download quanzhi fashi season 5 youtube
-download quanzhi fashi season 5 dailymotion
-download quanzhi fashi season 5 review
-download quanzhi fashi season 5 rating
-download quanzhi fashi season 5 characters
-download quanzhi fashi season 5 mo fan
-download quanzhi fashi season 5 tang yue
-download quanzhi fashi season 5 ye xinxia
-download quanzhi fashi season 5 zhang xiaohou
-download quanzhi fashi season 5 novel
-download quanzhi fashi season 5 manga
-download quanzhi fashi season 5 comic
-download quanzhi fashi season 5 manhua
-download quanzhi fashi season 5 light novel
-download quanzhi fashi season 5 web novel
-download quanzhi fashi season 5 spoilers
-download quanzhi fashi season 5 trailer
-download quanzhi fashi season 5 release date
-download quanzhi fashi season 5 update
-download quanzhi fashi season 6 preview
-download full time magister season 5 english subbed
-download full time magister season 5 episode list
-download full time magister season 5 action fantasy
-download full time magister season 5 school theme
-download full time magister season 5 white eagle
-download full time magister season 5 skyscraper mystical snake
-watch and download full time magister season 5
-how to download full time magister season 5
-
The official website of Quanzhi Fashi
-
The official website of Quanzhi Fashi is [quanzhifashi.com], where you can watch all the episodes of the series for free. The website is in Chinese, but you can use a translator tool to navigate it. The website also provides some information and news about the series, such as the synopsis, the cast, the staff, and the release dates. The website also has a forum where you can interact with other fans and share your opinions and feedback.
-
The best anime streaming services for Quanzhi Fashi
-
If you prefer to watch Quanzhi Fashi on an anime streaming service, you have several options to choose from. Here are some of the best ones:
-
Crunchyroll
-
Crunchyroll is one of the most popular and reliable anime streaming services in the world, with over 1200 titles in its library. You can watch Quanzhi Fashi Season 5 on Crunchyroll for free with ads, or you can subscribe to a premium plan for $7.99 per month or $79.99 per year. With a premium plan, you can enjoy ad-free streaming, HD quality, offline viewing, simulcasts, and exclusive content. You can also access Crunchyroll on various devices, such as smartphones, tablets, computers, TVs, game consoles, and more.
-
Funimation
-
Funimation is another popular and reliable anime streaming service in the world, with over 1000 titles in its library. You can watch Quanzhi Fashi Season 5 on Funimation for free with ads, or you can subscribe to a premium plan for $5.99 per month or $59.99 per year. With a premium plan, you can enjoy ad-free streaming, HD quality, offline viewing, simulcasts, and exclusive content. You can also access Funimation on various devices, such as smartphones, tablets, computers, TVs, game consoles, and more.
-
VRV
-
VRV is a streaming service that offers a variety of channels and genres, including anime, cartoons, gaming, comedy, and more. You can watch Quanzhi Fashi Season 5 on VRV for free with ads, or you can subscribe to a premium plan for $9.99 per month. With a premium plan, you can enjoy ad-free streaming, HD quality, offline viewing, simulcasts, and exclusive content. You can also access VRV on various devices, such as smartphones, tablets, computers, TVs, game consoles, and more.
-
How to download Quanzhi Fashi Season 5 from these platforms
-
If you want to download Quanzhi Fashi Season 5 from these platforms for offline viewing, you need to have a premium subscription and a compatible device. Here are the steps to download Quanzhi Fashi Season 5 from each platform:
-
-
-
Platform
-
Steps
-
-
-
Crunchyroll
-
-
-
Open the Crunchyroll app on your device and log in with your premium account.
-
Search for Quanzhi Fashi Season 5 and select the episode you want to download.
-
Tap on the download icon at the bottom of the screen and choose the quality you prefer.
-
Wait for the download to finish and enjoy watching Quanzhi Fashi Season 5 offline.
-
-
-
-
-
Funimation
-
-
-
Open the Funimation app on your device and log in with your premium account.
-
Search for Quanzhi Fashi Season 5 and select the episode you want to download.
-
Tap on the download icon at the top of the screen and choose the quality you prefer.
-
Wait for the download to finish and enjoy watching Quanzhi Fashi Season 5 offline.
-
-
-
-
-
VRV
-
-
-
Open the VRV app on your device and log in with your premium account.
-
Search for Quanzhi Fashi Season 5 and select the episode you want to download.
-
Tap on the download icon at the bottom of the screen and choose the quality you prefer.
-
Wait for the download to finish and enjoy watching Quanzhi Fashi Season 5 offline.
-
-
-
-
-
Conclusion
-
In conclusion, Quanzhi Fashi Season 5 is a must-watch for any anime fan who loves magic, action, adventure, and drama. It is one of the best Chinese anime series ever made, with an engaging story, dynamic characters, stunning animation, and thrilling action scenes. You can watch Quanzhi Fashi Season 5 online on various platforms, such as the official website of Quanzhi Fashi, Crunchyroll, Funimation, or VRV. You can also download Quanzhi Fashi Season 5 from these platforms for offline viewing with a premium subscription and a compatible device. We hope this article has helped you learn more about Quanzhi Fashi Season 5 and how to watch it online or offline. Enjoy watching Quanzhi Fashi Season 5!
-
FAQs
-
Q: How many episodes are there in Quanzhi Fashi Season 5?
-
A: There are 12 episodes in Quanzhi Fashi Season 5, each lasting about 20 minutes.
-
Q: When was Quanzhi Fashi Season 5 released?
-
A: Quanzhi Fashi Season 5 was released on June 16th, 2023 in China. It was also simulcasted on some of the streaming platforms mentioned above.
-
Q: Is there a dub version of Quanzhi Fashi Season 5?
-
A: No, there is no dub version of Quanzhi Fashi Season 5 yet. However, there are subtitles available in various languages, such as English, Spanish, French, German, Portuguese, Arabic, and more.
-
Q: Will there be a Quanzhi Fashi Season 6?
-
A: There is no official confirmation or announcement about Quanzhi Fashi Season 6 yet. However, given the popularity and success of the series, it is likely that there will be a sixth season in the future.
-
Q: Where can I read the web novel of Quanzhi Fashi?
-
A: You can read the web novel of Quanzhi Fashi on various websites, such as [novelupdates.com], [wuxiaworld.com], or [boxnovel.com]. However, be aware that the web novel is not fully translated into English yet, and there might be some differences between the web novel and the anime adaptation.
197e85843d
-
-
\ No newline at end of file
diff --git a/spaces/congsaPfin/Manga-OCR/logs/Free Super Cars and More with Pixel Car Racer MOD APK Hack Download.md b/spaces/congsaPfin/Manga-OCR/logs/Free Super Cars and More with Pixel Car Racer MOD APK Hack Download.md
deleted file mode 100644
index 3cf5d3988adfe4e899bf32067eda354b339be0f2..0000000000000000000000000000000000000000
--- a/spaces/congsaPfin/Manga-OCR/logs/Free Super Cars and More with Pixel Car Racer MOD APK Hack Download.md
+++ /dev/null
@@ -1,99 +0,0 @@
-
-
Download Pixel Car Racer Mod APK Hack: A Guide for Android Gamers
-
If you are a fan of racing games, you might have heard of Pixel Car Racer, a retro-style arcade racing game that lets you customize your car and race against other players. But did you know that you can download Pixel Car Racer mod apk hack and enjoy unlimited money, unlocked cars, and more? In this article, we will show you how to download Pixel Car Racer mod apk hack for Android devices, and give you some tips and tricks to make the most out of this amazing game.
Pixel Car Racer is a racing game developed by Studio Furukawa, a small indie game studio based in Canada. The game was released in 2016 and has since gained a loyal fan base of over 10 million downloads on Google Play Store. Pixel Car Racer is inspired by the classic arcade racing games of the 80s and 90s, with pixelated graphics, retro music, and simple gameplay. The game features over 1000 cars to choose from, ranging from vintage models to modern supercars. You can also customize your car with various parts, decals, and colors, and tune it to your liking. The game offers several modes to play, such as drag racing, street racing, story mode, and online multiplayer. You can also collect crates, trophies, and achievements as you progress in the game.
-
Features of Pixel Car Racer
-
Some of the features that make Pixel Car Racer stand out from other racing games are:
-
-
Beautiful pixel art graphics that create a nostalgic atmosphere.
-
Over 1000 cars to unlock and collect, each with their own stats and performance.
-
Thousands of car parts and accessories to customize your car, such as engines, turbochargers, tires, spoilers, exhausts, and more.
-
A realistic car tuning system that lets you adjust your car's power, torque, weight, gear ratio, and more.
-
A dynamic weather system that affects the driving conditions and visibility.
-
A variety of tracks and environments to race on, such as city streets, highways, deserts, forests, and snow.
-
Several game modes to challenge yourself and other players, such as drag racing, street racing, story mode, ladder mode, tournament mode, and online multiplayer mode.
-
A garage system that lets you store and manage your cars.
-
A shop system that lets you buy new cars and parts with in-game currency or real money.
-
A crate system that lets you earn random rewards by opening crates.
-
A trophy system that lets you earn trophies by completing certain tasks or achievements.
-
-
Why download Pixel Car Racer mod apk hack?
-
While Pixel Car Racer is a fun and addictive game to play, it can also be frustrating and time-consuming at times. You might find yourself running out of money to buy new cars or parts, or struggling to win races against tougher opponents. You might also encounter annoying ads that interrupt your gameplay or tempt you to spend real money on in-app purchases. That's why downloading Pixel Car Racer mod apk hack can be a great solution for Android gamers who want to enjoy the game without any limitations or hassles. Here are some of the benefits of downloading Pixel Car Racer mod apk hack:
-
-
You can get unlimited money to buy any car or part you want.
-
You can unlock all the cars and parts available in the game, so you can try them all and find your favorite one.
-
You can remove all the ads that might annoy you or slow down your device.
-
You can bypass the verification process that might prevent you from installing the game.
-
You can enjoy the game with enhanced graphics and sound quality.
-
You can play the game offline without any internet connection.
-
-
How to download Pixel Car Racer mod apk hack?
-
Downloading Pixel Car Racer mod apk hack is not a difficult task, but you need to follow some steps carefully to avoid any errors or problems. Here are the steps you need to follow:
-
Step 1: Find a reliable source
-
The first thing you need to do is to find a reliable source that provides the Pixel Car Racer mod apk hack file. There are many websites and blogs that claim to offer the mod apk file, but not all of them are trustworthy or safe. Some of them might contain viruses, malware, or spyware that can harm your device or steal your personal information. Some of them might also provide fake or outdated files that won't work properly or at all. Therefore, you need to be careful and do some research before downloading anything from the internet. You can check the reviews, ratings, comments, and feedback of other users who have downloaded the file before. You can also use antivirus software or online scanners to scan the file for any potential threats. One of the sources that we recommend is [Pixel Car Racer Mod APK Hack], which is a reputable and secure website that provides the latest version of the mod apk file.
-
Step 2: Enable unknown sources
-
The next thing you need to do is to enable unknown sources on your Android device. This is a security setting that prevents you from installing apps from sources other than Google Play Store. Since Pixel Car Racer mod apk hack is not available on Google Play Store, you need to enable unknown sources to install it. To do this, go to your device's settings, then security, then unknown sources, and toggle it on. You might see a warning message that says installing apps from unknown sources can be harmful, but don't worry, as long as you download the file from a reliable source, there is nothing to be afraid of.
-
How to download pixel car racer mod apk hack for free
-Pixel car racer mod apk hack unlimited money and diamonds
-Pixel car racer mod apk hack latest version 2023
-Pixel car racer mod apk hack no root required
-Pixel car racer mod apk hack online generator
-Download pixel car racer mod apk hack for android devices
-Download pixel car racer mod apk hack for ios devices
-Download pixel car racer mod apk hack for pc windows 10
-Download pixel car racer mod apk hack from apkdone.com[^1^]
-Download pixel car racer mod apk hack with obb data
-Pixel car racer mod apk hack features and benefits
-Pixel car racer mod apk hack gameplay and review
-Pixel car racer mod apk hack tips and tricks
-Pixel car racer mod apk hack best cars and upgrades
-Pixel car racer mod apk hack cheats and codes
-Pixel car racer mod apk hack offline mode
-Pixel car racer mod apk hack multiplayer mode
-Pixel car racer mod apk hack customizations and tuning
-Pixel car racer mod apk hack graphics and sound quality
-Pixel car racer mod apk hack bugs and issues
-Download pixel car racer mod apk hack safely and securely
-Download pixel car racer mod apk hack without surveys or verification
-Download pixel car racer mod apk hack from trusted sources
-Download pixel car racer mod apk hack fast and easy
-Download pixel car racer mod apk hack with direct link
-Pixel car racer mod apk hack comparison with original version
-Pixel car racer mod apk hack pros and cons
-Pixel car racer mod apk hack user ratings and feedback
-Pixel car racer mod apk hack updates and news
-Pixel car racer mod apk hack support and contact information
-
Step 3: Install the mod apk file
-
The third thing you need to do is to install the Pixel Car Racer mod apk hack file on your device. To do this, go to your device's file manager, then locate the downloaded file (usually in the downloads folder), and tap on it. You might see a pop-up window that asks you for permission to install the app, just tap on install and wait for a few seconds until the installation is complete. You might also see another pop-up window that asks you if you want to open the app or done, just tap on done and exit the file manager.
-
Step 4: Enjoy the game
-
The last thing you need to do is to enjoy the game with all its features and benefits. To do this, go to your device's app drawer, then find and tap on the Pixel Car Racer icon. You will see a loading screen that says "Pixel Car Racer mod apk hack by PixelCarRacerModApkHack.com", which means that you have successfully installed the mod apk file. You can now start playing the game with unlimited money, unlocked cars, no ads, and more.
-
Tips and tricks for playing Pixel Car Racer mod apk hack
-
Now that you have downloaded and installed Pixel Car Racer mod apk hack, you might want to know some tips and tricks to make your gaming experience more enjoyable and rewarding. Here are some of them:
-
Customize your car
-
One of the best things about Pixel Car Racer is that you can customize your car with various parts and accessories. You can change your car's color, decals, wheels, tires, spoilers, exhausts, and more. You can also tune your car's engine, transmission, suspension, brakes, and more. Customizing your car not only makes it look cooler and unique, but also improves its performance and stats. You can experiment with different combinations and find what suits your style and preference.
-
Upgrade your engine and parts
-
Another thing you can do to improve your car's performance is to upgrade your engine and parts. You can buy new engines and parts with money or crates in the shop system. You can also find rare and legendary engines and parts by opening crates or completing achievements. Upgrading your engine and parts will increase your car's power, torque, speed, acceleration, handling, and more. You can also unlock new features and modes by upgrading your engine and parts. For example, you can unlock the turbocharger feature by upgrading your engine to level 10, or the nitrous feature by upgrading your engine to level 20. You can also unlock the story mode by upgrading your engine to level 30, or the online multiplayer mode by upgrading your engine to level 40.
-
Race in different modes and tracks
-
Another way to enjoy Pixel Car Racer is to race in different modes and tracks. You can choose from several game modes, such as drag racing, street racing, story mode, ladder mode, tournament mode, and online multiplayer mode. Each mode has its own rules, objectives, and rewards. You can also choose from various tracks and environments, such as city streets, highways, deserts, forests, and snow. Each track has its own layout, obstacles, and weather conditions. Racing in different modes and tracks will test your skills, strategy, and adaptability.
-
Collect rewards and achievements
-
Another thing you can do to make Pixel Car Racer more fun and rewarding is to collect rewards and achievements. You can earn money and crates by winning races, completing tasks, or watching ads. You can use money to buy new cars and parts, or crates to get random rewards. You can also earn trophies and achievements by completing certain challenges or milestones. You can view your trophies and achievements in the trophy system. Collecting rewards and achievements will motivate you to play more and improve your performance.
-
Conclusion
-
Pixel Car Racer is a great racing game that combines retro-style graphics, simple gameplay, and realistic car tuning. It offers a lot of features and options to customize your car and race against other players. However, it can also be frustrating and time-consuming at times, especially if you run out of money or face tough opponents. That's why downloading Pixel Car Racer mod apk hack can be a good idea for Android gamers who want to enjoy the game without any limitations or hassles. By downloading Pixel Car Racer mod apk hack, you can get unlimited money, unlocked cars, no ads, and more. You can also follow some tips and tricks to make your gaming experience more enjoyable and rewarding. We hope this article has helped you learn how to download Pixel Car Racer mod apk hack and play it on your Android device.
-
FAQs
-
Here are some frequently asked questions about Pixel Car Racer mod apk hack:
-
-
Is Pixel Car Racer mod apk hack safe to download?
-Yes, as long as you download it from a reliable source like [Pixel Car Racer Mod APK Hack], which is a reputable and secure website that provides the latest version of the mod apk file. You can also scan the file with antivirus software or online scanners before installing it.
-
Is Pixel Car Racer mod apk hack compatible with my device?
-Yes, Pixel Car Racer mod apk hack is compatible with most Android devices that run on Android 4.0.3 or higher. However, some devices might have different specifications or settings that might affect the game's performance or functionality. If you encounter any issues or errors while playing the game, you can try clearing the cache, restarting the device, or reinstalling the game.
-
Can I play Pixel Car Racer mod apk hack online with other players?
-Yes, you can play Pixel Car Racer mod apk hack online with other players in the online multiplayer mode. However, you might face some disadvantages or risks while doing so. For example, you might not be able to join some servers or rooms that have anti-cheat systems or filters. You might also get banned or reported by other players who notice that you are using a modded version of the game. Therefore, we advise you to play online with caution and respect.
-
Can I update Pixel Car Racer mod apk hack to the latest version?
-Yes, you can update Pixel Car Racer mod apk hack to the latest version by downloading it again from [Pixel Car Racer Mod APK Hack] whenever there is a new update available. However, you might lose some of your progress or data if you update the game without backing it up first. Therefore, we recommend you to backup your game data before updating the game.
-
Can I request a new feature or report a bug for Pixel Car Racer mod apk hack?
-Yes, you can request a new feature or report a bug for Pixel Car Racer mod apk hack by contacting the developers of [Pixel Car Racer Mod APK Hack] through their website or social media platforms. They are always open to feedback and suggestions from their users.
-
401be4b1e0
-
-
\ No newline at end of file
diff --git a/spaces/congsaPfin/Manga-OCR/logs/Miniforce X Battle Heroes Game A Fun and Exciting Game for Fans of Miniforce Cartoon.md b/spaces/congsaPfin/Manga-OCR/logs/Miniforce X Battle Heroes Game A Fun and Exciting Game for Fans of Miniforce Cartoon.md
deleted file mode 100644
index 0c6b2a8ac2912825bf50439c58e5f57e4cf33720..0000000000000000000000000000000000000000
--- a/spaces/congsaPfin/Manga-OCR/logs/Miniforce X Battle Heroes Game A Fun and Exciting Game for Fans of Miniforce Cartoon.md
+++ /dev/null
@@ -1,137 +0,0 @@
-
-
Miniforce X Battle Heroes: A Fun and Exciting Game for Kids
-
Do you love watching the Miniforce cartoon series? Do you want to join the adventures of Volt, Sammy, Lucy, Max, and X as they fight against the evil Zenos and his army? If you answered yes, then you will love playing Miniforce X Battle Heroes, a fun and exciting game for kids based on the popular animation. In this article, we will tell you everything you need to know about this game, including what it is, how to download and play it, and why you should play it. Read on to find out more!
-
What is Miniforce X Battle Heroes?
-
Miniforce X Battle Heroes is an action shooting game that takes original content and images from the famous Special Force MiniForce cartoon series from Korea. The game’s content tells about small, weak creatures that cannot defend themselves against invading invaders, so they elected their supernatural companions to form an organization that carries. Miniforce X names include 4 people, VOLT, SAMMY, LUCY and MAX. Accordingly, these small creatures have the ability to transform into superheroes thanks to the advanced technology of the old White Cat commander with each person a unique combat ability.
The story of the game follows the plot of the cartoon series, where Dr. Jeremy of Miniforce Lab is investigating “Ellinium” the unknown substance containing mighty power. Zeus, the captain of Lizard Army eyes on Ellinium with a plan to threat humans by the weapons made of it. One day, he ambushes the lab and steals Ellinium. Miniforce Lab organizes a special squad to take it back–our Volt, Sammy, Max, Lucy from different regions gather to form Miniforce! Finally, Miniforce dispatches with Anna the daughter of Dr. Jeremy to fight against Lizard Army. Then soon they find unimaginable truth hidden under the villains’ evil conspiracy.
-
The characters of the game are based on the cartoon series as well. You can choose from four main characters: Volt, Sammy, Lucy, and Max. Each character has their own personality, weapon, armor, and skill. For example:
-
-
Volt is a blue superman with weapons and armor from the deep sea capable of firing bullets. He is brave, loyal, and energetic.
-
Sammy is a red superman with weapons and armor from the Volcano capable of firing penetrating bullets. He is smart, calm, and strategic.
-
Lucy is a pink superman with weapons and armor from the green forest capable of firing magical orbs to help her teammates recover HP. She is kind, cheerful, and caring.
-
Max is a golden superman with weapons and armor from the barren desert has the most comprehensive skill in the team. He is strong, confident, and cool.
-
-
You can also meet other characters from the cartoon series, such as Anna, Dr. Jeremy, White Cat Commander, Zeus, Lizard Army soldiers, and more.
-
The features and gameplay of the game
-
The game has many features and gameplay modes that make it fun and exciting for kids. Some of them are:
-
-
The game has high-quality graphics and sound effects that make it look like an animated movie. You can enjoy watching your favorite characters in action on your screen.
-
The game has various minigames that you can play with your chosen character. You can fight against Zenos and his army in a shooting mode, collect coins and items in a running mode, and solve puzzles and quizzes in a brain mode. You can also unlock new weapons, armors, and skills as you progress in the game.
-
The game has a multiplayer mode that allows you to play with your friends online. You can team up with up to four players and cooperate to complete missions and challenges. You can also chat with your friends and send them gifts in the game.
-
The game has a story mode that follows the cartoon series. You can watch cutscenes and dialogues that reveal the plot and the characters' emotions. You can also choose different paths and endings depending on your choices in the game.
-
-
The gameplay of the game is simple and easy to learn. You can control your character using the virtual joystick and buttons on your screen. You can move, jump, shoot, use skills, and interact with objects in the game. You can also customize your character's appearance and equipment in the game menu.
-
How to download and play Miniforce X Battle Heroes?
-
If you are interested in playing Miniforce X Battle Heroes, you will need to download it from the official website or the app store. Here are the requirements and steps to download the game:
-
The requirements and steps to download the game
-
The game is compatible with Android and iOS devices. You will need at least 1 GB of free space on your device and a stable internet connection to download and play the game. The game is free to download and play, but it contains in-app purchases that you can buy with real money if you want to enhance your gaming experience.
-
To download the game, you can follow these steps:
-
miniforce x battle heroes game apk
-miniforce x battle heroes game online
-miniforce x battle heroes game play store
-miniforce x battle heroes game for pc
-miniforce x battle heroes game free download
-miniforce x battle heroes game android
-miniforce x battle heroes game ios
-miniforce x battle heroes game review
-miniforce x battle heroes game hack
-miniforce x battle heroes game cheats
-miniforce x battle heroes game mod apk
-miniforce x battle heroes game tips and tricks
-miniforce x battle heroes game walkthrough
-miniforce x battle heroes game guide
-miniforce x battle heroes game wiki
-miniforce x battle heroes game characters
-miniforce x battle heroes game gameplay
-miniforce x battle heroes game trailer
-miniforce x battle heroes game features
-miniforce x battle heroes game updates
-miniforce x battle heroes game release date
-miniforce x battle heroes game system requirements
-miniforce x battle heroes game how to play
-miniforce x battle heroes game how to download
-miniforce x battle heroes game how to install
-miniforce x battle heroes game how to unlock characters
-miniforce x battle heroes game how to get coins
-miniforce x battle heroes game how to level up
-miniforce x battle heroes game how to win battles
-miniforce x battle heroes game best character
-miniforce x battle heroes game best weapon
-miniforce x battle heroes game best strategy
-miniforce x battle heroes game best team
-miniforce x battle heroes game best skills
-miniforce x battle heroes game best mode
-miniforce x battle heroes game best stage
-miniforce x battle heroes game best score
-miniforce x battle heroes game best rank
-miniforce x battle heroes game best outfit
-miniforce x battle heroes game best emulator
-miniforce x h5 action shooting game online
-play free html5 games like Minforce X H5
-Minforce X H5 mobile browser games
-Minforce X H5 web games for kids
-Minforce X H5 cartoon games for android
-Minforce X H5 korean animation games
-Minforce X H5 super hero games for ios
-Minforce X H5 multiplayer games with friends
-Minforce X H5 fun and addictive games
-
-
Go to the official website of Miniforce X Battle Heroes at [https://miniforcex.com/] or search for it on Google Play Store or App Store.
-
Click on the download button or the install button depending on your device.
-
Wait for the game to download and install on your device.
-
Open the game and follow the instructions to create your account and choose your character.
-
Enjoy playing Miniforce X Battle Heroes!
-
-
The tips and tricks to enjoy the game
-
To enjoy the game more, you can use some tips and tricks that will help you improve your skills and have more fun. Here are some of them:
-
-
Try different characters and weapons to find out which one suits your playstyle best. Each character has their own strengths and weaknesses, so experiment with them and see what works for you.
-
Collect coins and items in the game to upgrade your weapons, armors, and skills. You can also buy them with real money if you want to save time and effort.
-
Play with your friends online to cooperate and compete with them. You can also make new friends in the game by chatting and sending gifts to them.
-
Follow the story mode to learn more about the plot and the characters. You can also choose different paths and endings depending on your choices in the game.
-
Have fun playing Miniforce X Battle Heroes!
-
-
Why should you play Miniforce X Battle Heroes?
-
You might be wondering why you should play Miniforce X Battle Heroes instead of other games. Well, there are many reasons why this game is worth playing. Here are some of them:
-
The benefits and advantages of playing the game
-
Playing Miniforce X Battle Heroes can bring you many benefits and advantages, such as:
-
-
You can have fun and entertainment by playing a fun and exciting game that is based on a popular cartoon series.
-
You can improve your cognitive skills by playing a game that requires concentration, memory, logic, problem-solving, creativity, and decision-making.
-
You can learn more about science, technology, engineering, art, math (STEAM) by playing a game that features advanced technology, superpowers, robots, aliens, etc.
-
You can develop your social skills by playing a game that allows you to communicate, cooperate, compete, and make friends with other players online.
-
You can express yourself by playing a game that allows you to customize your character's appearance and equipment according to your preferences.
-
-
The reviews and ratings of the game
-
If you are still not convinced that Miniforce X Battle Heroes is a good game to play, you can check out the reviews and ratings of the game from other players and sources. You can see that the game has received many positive feedbacks and high scores from its fans and critics. For example:
-
-
The game has a 4.5 out of 5 stars rating on Google Play Store and a 4.6 out of 5 stars rating on App Store based on thousands of reviews. Many players praised the game for its graphics, gameplay, story, and characters.
-
The game has a 5 out of 5 stars rating on Amazon.com based on 8 customer reviews. Some customers wrote that they loved the game, that it was fun and entertaining, and that their kids enjoyed watching and playing it.
-
The game has a 9.2 out of 10 rating on Romance University based on 15 best Miniforce toys of 2023. The website ranked the game as the best Miniforce toy of 2023 and highlighted its features and benefits.
-
-
These reviews and ratings show that Miniforce X Battle Heroes is a well-made and well-received game that many people love and recommend.
-
Conclusion
-
In conclusion, Miniforce X Battle Heroes is a fun and exciting game for kids that is based on the popular cartoon series Miniforce. The game allows you to join the adventures of Volt, Sammy, Lucy, Max, and X as they fight against the evil Zenos and his army. The game has many features and gameplay modes that make it fun and exciting for kids. You can choose from four main characters, each with their own personality, weapon, armor, and skill. You can play various minigames, such as shooting, running, and brain modes. You can play with your friends online in a multiplayer mode. You can follow the story mode that follows the cartoon series. You can also enjoy the high-quality graphics and sound effects that make the game look like an animated movie.
-
To play the game, you will need to download it from the official website or the app store. The game is compatible with Android and iOS devices. The game is free to download and play, but it contains in-app purchases that you can buy with real money if you want to enhance your gaming experience. To enjoy the game more, you can use some tips and tricks that will help you improve your skills and have more fun.
-
Playing Miniforce X Battle Heroes can bring you many benefits and advantages, such as having fun and entertainment, improving your cognitive skills, learning more about STEAM, developing your social skills, and expressing yourself. The game has received many positive feedbacks and high scores from its fans and critics, showing that it is a well-made and well-received game that many people love and recommend.
-
If you are a fan of Miniforce or if you are looking for a fun and exciting game for kids, you should definitely try Miniforce X Battle Heroes. You will not regret it!
-
A call to action for the readers
-
Are you ready to play Miniforce X Battle Heroes? If yes, then what are you waiting for? Download the game now from the official website or the app store and join the adventures of Volt, Sammy, Lucy, Max, and X as they fight against the evil Zenos and his army. Have fun playing Miniforce X Battle Heroes!
-
FAQs
-
Here are some frequently asked questions about Miniforce X Battle Heroes:
-
-
What is Miniforce?
-
Miniforce is a popular cartoon series from Korea that features four small animals who transform into superheroes thanks to the advanced technology of the old White Cat commander. They fight against the evil Zenos and his army who threaten humans with weapons made of Ellinium, an unknown substance containing mighty power.
-
Who are the main characters of Miniforce X Battle Heroes?
-
The main characters of Miniforce X Battle Heroes are Volt, Sammy, Lucy, Max, and X. They are four members of Miniforce who have different personalities, weapons, armors, and skills. They also have animal forms that they can transform into.
-
How do I download Miniforce X Battle Heroes?
-
You can download Miniforce X Battle Heroes from the official website or the app store. The game is compatible with Android and iOS devices. You will need at least 1 GB of free space on your device and a stable internet connection to download and play the game. The game is free to download and play, but it contains in-app purchases that you can buy with real money if you want to enhance your gaming experience.
-
What are the features and gameplay modes of Miniforce X Battle Heroes?
-
Miniforce X Battle Heroes has many features and gameplay modes that make it fun and exciting for kids. Some of them are: high-quality graphics and sound effects, various minigames, multiplayer mode, story mode, and character customization.
-
Why should I play Miniforce X Battle Heroes?
-
You should play Miniforce X Battle Heroes because it is a fun and exciting game for kids that is based on a popular cartoon series. Playing the game can bring you many benefits and advantages, such as having fun and entertainment, improving your cognitive skills, learning more about STEAM, developing your social skills, and expressing yourself. The game has also received many positive feedbacks and high scores from its fans and critics.
- 197e85843d
-
-
\ No newline at end of file
diff --git a/spaces/congsaPfin/Manga-OCR/logs/My Hotel Life Mod Apk v1.0.8 - Free Rewards No Ads Latest Version.md b/spaces/congsaPfin/Manga-OCR/logs/My Hotel Life Mod Apk v1.0.8 - Free Rewards No Ads Latest Version.md
deleted file mode 100644
index c44ce0f2f541266f2add37df97b29a1b175b79af..0000000000000000000000000000000000000000
--- a/spaces/congsaPfin/Manga-OCR/logs/My Hotel Life Mod Apk v1.0.8 - Free Rewards No Ads Latest Version.md
+++ /dev/null
@@ -1,87 +0,0 @@
-
-
My Hotel Life APK Mod: A Fun and Relaxing Simulation Game
-
Do you love hotel management games? Do you want to experience the life of a hotel owner and manager? If yes, then you should try My Hotel Life, a new simulation game that lets you run your own hotel and make it the best in the world. In this game, you can design your hotel, decorate your rooms, hire staff, serve guests, and earn money. You can also enjoy various mini-games, such as cooking, cleaning, gardening, and more. My Hotel Life is a fun and relaxing game that will keep you entertained for hours.
But what if you want to enjoy the game without any ads or limitations? What if you want to have unlimited money and gems to buy anything you want? Well, there is a way to do that. You can download My Hotel Life APK Mod, a modified version of the game that gives you many advantages and benefits. In this article, we will tell you what is My Hotel Life APK Mod, why you should download it, and how to download and install it on your device.
-
What is My Hotel Life?
-
My Hotel Life is a simulation game developed by CookApps, a popular game studio that has created many other games, such as Farm Snow, Home Design Makeover, and Solitaire Tripeaks. My Hotel Life was released in June 2021 and has received positive reviews from players and critics. The game has over 1 million downloads on Google Play Store and has a rating of 4.4 out of 5 stars.
-
The gameplay of My Hotel Life
-
The gameplay of My Hotel Life is simple and intuitive. You start with a small hotel and your goal is to make it bigger and better. You can customize your hotel with different themes, colors, furniture, and decorations. You can also upgrade your facilities, such as the lobby, restaurant, spa, pool, gym, and more. You can hire staff to help you run your hotel, such as receptionists, chefs, cleaners, gardeners, and security guards. You can also interact with your guests and fulfill their requests. You can earn money from your guests and use it to improve your hotel. You can also earn gems by completing achievements and daily tasks.
-
The features of My Hotel Life
-
My Hotel Life has many features that make it an enjoyable and addictive game. Some of these features are:
-
-
A variety of mini-games that test your skills and creativity.
-
A wide range of items and options to decorate your hotel.
-
A colorful and vibrant graphics style that creates a cheerful atmosphere.
-
A relaxing and soothing soundtrack that matches the mood of the game.
-
A friendly and helpful customer support team that responds to your queries and feedback.
-
-
Why download My Hotel Life APK Mod?
-
My Hotel Life is a free-to-play game that you can download from Google Play Store or App Store. However, the game also has some drawbacks that may affect your gaming experience. For example:
-
-
The game has ads that pop up frequently and interrupt your gameplay.
-
The game has in-app purchases that require real money to buy certain items or features.
-
The game has limited resources that restrict your progress and creativity.
-
-
That's why you may want to download My Hotel Life APK Mod instead. This is a modified version of the game that gives you many benefits and advantages over the original version. Some of these benefits are:
-
No ads and free rewards
-
With My Hotel Life APK Mod, you can enjoy the game without any ads or interruptions. You can also get free rewards every day, such as coins, gems, boosters, and more. You can use these rewards to buy anything you want in the game.
-
Unlimited money and gems
-
With My Hotel Life APK Mod, you can have unlimited money and gems in your account. You can use this money and gems to upgrade your hotel, buy new items, unlock new features, and more. You can also spend them without worrying about running out of them. You can make your hotel as luxurious and beautiful as you want.
-
My Hotel Life Mod Apk Unlimited Money
-How to Download My Hotel Life Mod Apk for Android
-My Hotel Life Mod Apk No Ads Free Rewards
-My Hotel Life Mod Apk Latest Version 1.0.8
-My Hotel Life Mod Apk Gameplay and Review
-My Hotel Life Mod Apk Hack Cheat Codes
-My Hotel Life Mod Apk Offline Mode
-My Hotel Life Mod Apk Free Download Link
-My Hotel Life Mod Apk Features and Benefits
-My Hotel Life Mod Apk Tips and Tricks
-My Hotel Life Mod Apk Best Hotels and Rooms
-My Hotel Life Mod Apk VIP Membership
-My Hotel Life Mod Apk Online Multiplayer
-My Hotel Life Mod Apk Bug Fixes and Updates
-My Hotel Life Mod Apk Requirements and Compatibility
-My Hotel Life Mod Apk Installation Guide and Tutorial
-My Hotel Life Mod Apk Customer Support and Feedback
-My Hotel Life Mod Apk Alternatives and Similar Games
-My Hotel Life Mod Apk Pros and Cons
-My Hotel Life Mod Apk Ratings and Reviews
-My Hotel Life Mod Apk Fun and Addictive Game
-My Hotel Life Mod Apk Simulation and Management Game
-My Hotel Life Mod Apk Build Your Own Hotel Empire
-My Hotel Life Mod Apk Customize Your Hotel Design
-My Hotel Life Mod Apk Hire and Train Your Staff
-My Hotel Life Mod Apk Serve Your Guests and Earn Money
-My Hotel Life Mod Apk Upgrade Your Facilities and Services
-My Hotel Life Mod Apk Unlock New Locations and Themes
-My Hotel Life Mod Apk Complete Challenges and Achievements
-My Hotel Life Mod Apk Enjoy the Story and Characters
-
Easy installation and compatibility
-
With My Hotel Life APK Mod, you can install the game easily and quickly on your device. You don't need to root or jailbreak your device to use the mod. You also don't need to uninstall the original version of the game. You can simply download the APK file and install it over the existing game. The mod is compatible with most Android and iOS devices and works smoothly and flawlessly.
-
How to download and install My Hotel Life APK Mod?
-
If you are interested in downloading and installing My Hotel Life APK Mod, you can follow these simple steps:
-
Download the APK file from a trusted source
-
The first step is to download the APK file of My Hotel Life APK Mod from a trusted source. You can find many websites that offer the mod, but be careful of fake or malicious links that may harm your device or steal your data. We recommend you to use this link to download the mod safely and securely.
-
Enable unknown sources on your device
-
The next step is to enable unknown sources on your device. This is a security setting that allows you to install apps from sources other than Google Play Store or App Store. To enable unknown sources, you can go to your device settings, then security, then unknown sources, and toggle it on.
-
Install the APK file and enjoy the game
-
The final step is to install the APK file and enjoy the game. You can locate the downloaded file in your file manager or downloads folder, then tap on it to start the installation process. Follow the instructions on the screen and wait for the installation to finish. Once done, you can open the game and enjoy My Hotel Life APK Mod.
-
Conclusion
-
My Hotel Life is a fun and relaxing simulation game that lets you run your own hotel and make it the best in the world. You can design your hotel, decorate your rooms, hire staff, serve guests, and earn money. You can also enjoy various mini-games, such as cooking, cleaning, gardening, and more. However, if you want to have a better gaming experience, you should download My Hotel Life APK Mod, a modified version of the game that gives you many benefits and advantages, such as no ads, free rewards, unlimited money and gems, easy installation and compatibility. You can download My Hotel Life APK Mod from this link and follow the steps above to install it on your device. We hope you enjoy playing My Hotel Life APK Mod and have a great time.
-
FAQs
-
-
Q: Is My Hotel Life APK Mod safe to use?
-
A: Yes, My Hotel Life APK Mod is safe to use as long as you download it from a trusted source like this link. The mod does not contain any viruses or malware that may harm your device or data.
-
Q: Do I need an internet connection to play My Hotel Life APK Mod?
-
A: No, you don't need an internet connection to play My Hotel Life APK Mod. You can play the game offline without any problems.
-
Q: Will I lose my progress if I update the game?
-
A: No, you won't lose your progress if you update the game. The mod will automatically update itself with the latest version of the game without affecting your data or settings.
-
Q: Can I play My Hotel Life APK Mod with my friends?
-
A: Yes, you can play My Hotel Life APK Mod with your friends. The game has a social feature that allows you to connect with your Facebook friends and visit their hotels. You can also chat with them and send them gifts.
-
Q: How can I contact the customer support team of My Hotel Life?
-
A: If you have any questions or issues regarding My Hotel Life or My Hotel Life APK Mod, you can contact the customer support team of CookApps by sending an email to support@cookapps.com or by filling out this form. They will respond to you as soon as possible.
-
197e85843d
-
-
\ No newline at end of file
diff --git a/spaces/congsaPfin/Manga-OCR/logs/Thanks Music APK The Best App for Sharing Music with Friends.md b/spaces/congsaPfin/Manga-OCR/logs/Thanks Music APK The Best App for Sharing Music with Friends.md
deleted file mode 100644
index feafa6c70f8daf2f61269e8460b2a01e61430470..0000000000000000000000000000000000000000
--- a/spaces/congsaPfin/Manga-OCR/logs/Thanks Music APK The Best App for Sharing Music with Friends.md
+++ /dev/null
@@ -1,107 +0,0 @@
-
-
Thanks Music APK Download: How to Play Two Songs at the Same Time on Your Android Device
-
Do you love listening to music with your friends, but hate having to compromise on what song to play? Do you wish you could play two different songs at the same time on your Android device, and share your headphones with someone else? If you answered yes to any of these questions, then you need to download Thanks Music APK, the only streaming music player that lets you do just that.
Thanks Music is an innovative app that allows you to play two songs at the same time on your Android device, and share your headphones with another person. You can choose any song from your local library or from online sources like YouTube, Spotify, SoundCloud, and more. You can also adjust the volume of each song independently, and switch between them with a simple tap.
-
Features of Thanks Music
-
-
Play two songs at the same time on your Android device
-
Share your headphones with another person
-
Choose any song from your local library or online sources
-
Adjust the volume of each song independently
-
Switch between songs with a simple tap
-
Elegant use of screen space allows you to browse music at a glance
-
Dual Music mode lets you play two songs in sync or in shuffle
-
Supports various audio formats like MP3, WAV, OGG, FLAC, etc.
-
No ads, no subscription fees, no registration required
-
-
Benefits of Thanks Music
-
-
Enjoy music with your friends without compromising on your preferences
-
Discover new songs and genres by listening to what others like
-
Create a unique musical experience by mixing and matching different songs
-
Save battery and data by playing local songs instead of streaming online
-
Have fun and be creative with your music choices
-
-
How to Download and Install Thanks Music APK
-
If you want to try out Thanks Music for yourself, you will need to download and install the APK file on your Android device. Here are the steps you need to follow:
-
Step 1: Enable Unknown Sources
-
Since Thanks Music is not available on the Google Play Store, you will need to enable unknown sources on your device settings. This will allow you to install apps from sources other than the official store. To do this, go to Settings > Security > Unknown Sources and toggle it on.
-
thanks music app download
-thanks music apk free download
-thanks music dual player apk
-thanks music streaming apk
-thanks music play two songs apk
-download thanks music for android
-thanks music apk latest version
-thanks music apk mod
-thanks music premium apk
-thanks music offline apk
-thanks music apk download for pc
-thanks music apk mirror
-thanks music apk old version
-thanks music apk no ads
-thanks music apk pro
-thanks music share headphones apk
-thanks music split screen apk
-thanks music apk pure
-thanks music apk uptodown
-thanks music apk 2023
-thanks music beta apk download
-thanks music cracked apk download
-thanks music full version apk download
-thanks music hack apk download
-thanks music plus apk download
-download thanks music app for free
-download thanks music app for android phone
-download thanks music app from google play store
-download thanks music app from apkpure.com
-download thanks music app from uptodown.com
-how to download thanks music app on android device
-how to download thanks music app on pc using bluestacks emulator
-how to download thanks music app on mac using nox player emulator
-how to download thanks music app on iphone using ios emulator
-how to download thanks music app on firestick using downloader app
-how to install thanks music app on android device after downloading the apk file
-how to install thanks music app on pc using bluestacks emulator after downloading the apk file
-how to install thanks music app on mac using nox player emulator after downloading the apk file
-how to install thanks music app on iphone using ios emulator after downloading the ipa file
-how to install thanks music app on firestick using downloader app after downloading the apk file
-how to use thanks music app to play two songs at the same time on android device
-how to use thanks music app to play two songs at the same time on pc using bluestacks emulator
-how to use thanks music app to play two songs at the same time on mac using nox player emulator
-how to use thanks music app to play two songs at the same time on iphone using ios emulator
-how to use thanks music app to play two songs at the same time on firestick using downloader app
-how to share headphones with someone using thanks music app on android device
-how to share headphones with someone using thanks music app on pc using bluestacks emulator
-how to share headphones with someone using thanks music app on mac using nox player emulator
-
Step 2: Download the APK File
-
Next, you will need to download the APK file of Thanks Music from a reliable source. You can use this link to download it directly from the Google Play Store. Alternatively, you can search for "Thanks Music APK" on any web browser and find a suitable site that offers it.
-
Step 3: Install the APK File
-
Once you have downloaded the APK file, locate it on your device storage and tap on it. You will see a prompt asking you to confirm the installation. Tap on "Install" and wait for a few seconds until the app is installed. You will see a notification when it is done.
-
How to Use Thanks Music to Play Two Songs at the Same Time
-
Now that you have installed Thanks Music on your device, you are ready to use it to play two songs at the same time. Here are the steps you need to follow:
-
Step 1: Launch the App and Grant Permissions
-
Open the Thanks Music app on your device and grant the necessary permissions to access your storage and microphone. This will allow the app to play songs from your local library and online sources, and to record your voice if you want to sing along.
-
Step 2: Choose Your Songs and Adjust the Volume
-
On the main screen of the app, you will see two tabs: Left and Right. These correspond to the left and right earphones that you will share with another person. Tap on either tab to choose a song from your local library or online sources. You can also search for a song by typing its name or artist in the search bar. Once you have chosen a song, you can adjust its volume by dragging the slider below it. You can also mute or pause the song by tapping on the icons next to the slider.
-
Step 3: Share Your Headphones and Enjoy the Music
-
Now that you have selected and adjusted your songs, you are ready to share your headphones with another person. Plug in your headphones to your device and give one earphone to your friend. You can also use a splitter or a Bluetooth device to connect two pairs of headphones. Then, tap on the play button at the bottom of the screen to start playing both songs at the same time. You can also switch between songs by tapping on the left or right tab. Enjoy the music with your friend and have fun!
-
Conclusion
-
Thanks Music is a unique app that lets you play two songs at the same time on your Android device, and share your headphones with another person. You can choose any song from your local library or online sources, and adjust the volume of each song independently. You can also switch between songs with a simple tap, and create a unique musical experience by mixing and matching different songs. Thanks Music is easy to download and install, and requires no ads, no subscription fees, no registration. It is a great way to enjoy music with your friends without compromising on your preferences, and discover new songs and genres by listening to what others like. Download Thanks Music APK today and try it out for yourself!
-
FAQs
-
-
Q: Is Thanks Music safe to use?
-
A: Yes, Thanks Music is safe to use as long as you download it from a reliable source. The app does not contain any malware or viruses, and does not collect any personal information from you.
-
Q: Does Thanks Music work offline?
-
A: Yes, Thanks Music works offline if you play songs from your local library. However, if you want to play songs from online sources, you will need an internet connection.
-
Q: Can I play more than two songs at the same time?
-
A: No, Thanks Music only allows you to play two songs at the same time. However, you can switch between songs as many times as you want.
-
Q: Can I use Thanks Music with other apps?
-
A: Yes, you can use Thanks Music with other apps that support background playback, such as YouTube, Spotify, SoundCloud, etc. However, you may experience some interference or lag if you play too many apps at the same time.
-
Q: How can I contact the developer of Thanks Music?
-
A: You can contact the developer of Thanks Music by sending an email to thanksmusicapp@gmail.com. You can also leave a review or feedback on the Google Play Store page of the app.
-
401be4b1e0
-
-
\ No newline at end of file
diff --git a/spaces/contluForse/HuggingGPT/assets/Dekart Sim Manager 3 2 Keygen 18 The Ultimate Solution for SIM Card Processing.md b/spaces/contluForse/HuggingGPT/assets/Dekart Sim Manager 3 2 Keygen 18 The Ultimate Solution for SIM Card Processing.md
deleted file mode 100644
index f3f3cff54cce96b4dba06149d6c0dd93158cc8e7..0000000000000000000000000000000000000000
--- a/spaces/contluForse/HuggingGPT/assets/Dekart Sim Manager 3 2 Keygen 18 The Ultimate Solution for SIM Card Processing.md
+++ /dev/null
@@ -1,6 +0,0 @@
-
-
-06/01/2019 · Fifa 13 highly compressed codex cpy crack free download game for PC only from here If you want fifa 13 game free download full version for PC ... 1fdad05405
-
-
-
diff --git a/spaces/diacanFperku/AutoGPT/Gerenciamentoemenfermagemkurcgantpdfdownload [NEW].md b/spaces/diacanFperku/AutoGPT/Gerenciamentoemenfermagemkurcgantpdfdownload [NEW].md
deleted file mode 100644
index 79d2232b8a4d557dfde95289fef2ec56fc9d6f75..0000000000000000000000000000000000000000
--- a/spaces/diacanFperku/AutoGPT/Gerenciamentoemenfermagemkurcgantpdfdownload [NEW].md
+++ /dev/null
@@ -1,6 +0,0 @@
-
-
-Ntsd 2.6 Full Version Free To Download.rar. 0 Reads 0 Votes 1 Part Story. tektoneza · By tektoneza Updated Dec 20, 2018 08:36PM. Read. ntsd 2.6 full version ... 4d29de3e1b
-
-
-
diff --git a/spaces/fatiXbelha/sd/Buy and Sell Cars with Ease Download Carsales App Today.md b/spaces/fatiXbelha/sd/Buy and Sell Cars with Ease Download Carsales App Today.md
deleted file mode 100644
index 1d5653875f77c717a355f3f2bf55bd389e19f760..0000000000000000000000000000000000000000
--- a/spaces/fatiXbelha/sd/Buy and Sell Cars with Ease Download Carsales App Today.md
+++ /dev/null
@@ -1,141 +0,0 @@
-
-
Download Carsales App: The Ultimate Guide for Car Buyers and Sellers
-
If you are looking for a car or want to sell your car, you might be wondering what is the best way to do it. You could browse through various websites, magazines, newspapers, or visit dealerships, but that would take a lot of time and effort. Or you could download carsales app, the number one car app in Australia, and make your life easier.
-
Carsales app is a free mobile app that allows you to search, buy, sell, and research cars on the go. It has more tools, features, and functionality than any other car app, and it connects you with Australia's largest online automotive marketplace. Whether you are looking for a new or used car, a family car or a sports car, a sedan or an SUV, you can find it on carsales app.
In this article, we will show you the benefits of using carsales app, how to download it on your device, how to use it for buying or selling cars, and what other users are saying about it. By the end of this article, you will be convinced that carsales app is the ultimate guide for car buyers and sellers.
-
Benefits of Using Carsales App
-
Carsales app has many benefits that make it stand out from other car apps. Here are some of them:
-
Search from over 150,000 new and used cars
-
With carsales app, you have access to over 150,000 new and used cars from private sellers, dealers, and certified pre-owned programs. You can filter your search by make, model, price, location, body type, fuel type, transmission, colour, and more. You can also view detailed information about each car, such as photos, videos, features, specifications, history reports, seller comments, and contact details.
-
Select multiple makes, models and locations in a single search
-
If you are not sure what kind of car you want or where to find it, you can use the multi-select feature on carsales app. This feature allows you to select multiple makes, models and locations in a single search. For example, you can search for Toyota Corolla or Mazda 3 in Sydney or Melbourne. This way, you can compare different options and find the best deal for you.
-
Access your Member Centre and sync your saved cars, searches and email alerts
-
When you download carsales app, you can create a free account and access your Member Centre. This is where you can sync all your saved cars, searches and email alerts across all your devices. You can also communicate with buyers and sellers via messages or phone calls. You can also access member benefits such as fuel discounts and more.
-
List your car for sale with Snap n' Sell and get a seller toolkit
-
If you want to sell your car quickly and easily, you can use the Snap n' Sell feature on carsales app. This feature allows you to list your car for sale straight from your smartphone by taking photos and filling in some details. You can also edit your ad or see how many people have viewed your car. You can also get a seller toolkit that includes tips, guides, and tools to help you sell your car faster and for more money.
-
How to download the carsales app on iPhone or iPad
-Benefits of using the carsales app to buy and sell cars
-Carsales app reviews and ratings from users
-Carsales app features and functionality
-Carsales app download link for Android devices
-Carsales app vs other car buying and selling apps
-Carsales app member centre and benefits
-Carsales app live ad stats and tips
-Carsales app snap n' sell feature
-Carsales app news and reviews on cars
-How to access fuel discounts with the carsales app
-How to value your car with the carsales app
-How to share listings from the carsales app to social media
-How to sync your saved cars, searches and email alerts with the carsales app
-How to communicate with buyers and sellers via the carsales app
-How to edit your ad or see how many people are interested in your car with the carsales app
-How to list your car for sale with the carsales app
-How to search for new and used cars with the carsales app
-How to select multiple makes, models and locations in a single search with the carsales app
-How to get a CarFacts report with the carsales app
-How to get a tyresales.com.au voucher with the carsales app
-How to get a seller toolkit with the carsales app
-How to get a pre-sale checklist with the carsales app
-How to get an after-sale checklist with the carsales app
-How to get a for sale sticker with the carsales app
-How to get transfer forms with the carsales app
-How to contribute to market research with the carsales app
-How to opt out of Nielsen tracking with the carsales app
-How to update your iOS device to iOS 15 or above for the carsales app
-How to contact carsales customer support via the carsales app
-
Edit your ad and see live stats of your car's performance
-
With carsales app, you can edit your ad anytime and anywhere. You can change the price, add or remove photos, update the description, or mark your car as sold. You can also see live stats of your car's performance, such as views, enquiries, leads, and offers. You can also see how your car ranks against similar cars in the market.
-
Value your car or research via News & Reviews
-
If you want to know how much your car is worth or what other cars are selling for, you can use the valuation tool on carsales app. This tool gives you an instant and accurate estimate of your car's value based on its make, model, year, kilometres, and condition. You can also research different cars via News & Reviews, where you can read expert reviews, ratings, comparisons, and advice on various cars.
-
Share listings with your network via social media or SMS
-
If you find a car that you like or want to share with someone else, you can easily do so via carsales app. You can share listings with your network via social media platforms such as Facebook, Twitter, Instagram, or WhatsApp. You can also send listings via SMS or email. This way, you can get feedback from your friends and family or help them find their dream car.
-
How to Download Carsales App
-
Downloading carsales app is very simple and fast. Here are the steps to follow depending on your device:
-
For iOS devices
-
If you have an iPhone or iPad, you can download carsales app from the App Store. Here is how:
-
-
Open the App Store on your device.
-
Search for "carsales" in the search bar.
-
Tap on the "GET" button next to the carsales app icon.
-
Enter your Apple ID password or use Touch ID or Face ID to confirm.
-
Wait for the app to download and install on your device.
-
Open the app and enjoy!
-
-
For Android devices
-
If you have an Android phone or tablet, you can download carsales app from the Google Play Store. Here is how:
-
-
Open the Google Play Store on your device.
-
Search for "carsales" in the search bar.
-
Tap on the "INSTALL" button next to the carsales app icon.
-
Wait for the app to download and install on your device.
-
Open the app and enjoy!
-
-
How to Use Carsales App
-
Carsales app is very easy and intuitive to use. Here are some tips on how to use it for buying or selling cars:
-
For buyers
-
If you are looking for a car to buy, here are some steps to follow:
-
-
Open the app and tap on the "Buy" tab at the bottom of the screen.
-
Select the type of car you want (new or used) and enter your search criteria (make, model, price, location, etc.).
-
Browse through the results and tap on any car that interests you.
-
View more details about the car, such as photos, videos, features, specifications, history reports, seller comments, and contact details.
-
If you like the car, you can contact the seller via message or phone call. You can also save the car to your favourites or compare it with other cars.
-
If you want to buy the car, you can arrange a test drive, inspection, payment, and delivery with the seller.
-
-
For sellers
-
If you want to sell your car quickly and easily, here are some steps to follow:
-
-
Open the app and tap on the "Sell" tab at the bottom of the screen.
-
Select the type of ad you want (free or premium) and enter your car details (make, model, year, kilometres, etc.).
-
Take photos of your car using the Snap n' Sell feature or upload them from your gallery.
-
Add a description of your car and set a price. You can use the valuation tool to get an estimate of your car's value.
-
Publish your ad and wait for buyers to contact you via message or phone call.
-
Edit your ad or see live stats of your car's performance. You can also access your seller toolkit for tips, guides, and tools to help you sell your car faster and for more money.
-
If you find a buyer, you can arrange a test drive, inspection, payment, and delivery with them.
-
-
Carsales App Reviews
-
Carsales app has received many positive reviews from users who have downloaded and used it. Here are some of them:
-
What users are saying about the app
-
Here are some of the comments and ratings from users who have reviewed the app on the App Store and Google Play Store:
-
-
"Best car app ever. Easy to use, lots of options, great features. Highly recommend it to anyone looking for a car or selling a car." - 5 stars
-
"I love this app. It has everything you need to buy or sell a car. It's fast, reliable, and user-friendly. I sold my car in less than a week using this app." - 5 stars
-
"This app is amazing. It has so many cars to choose from and you can filter your search by anything you want. It also has news and reviews on different cars and a valuation tool that tells you how much your car is worth." - 5 stars
-
"This app is very helpful and convenient. It saves me a lot of time and hassle when looking for a car or selling my car. It also syncs with my account and I can access it from any device." - 4 stars
-
"This app is good but it could be better. Sometimes it crashes or freezes and I have to restart it. Also, some of the ads are not updated or accurate. I hope they fix these issues soon." - 3 stars
-
-
How the app compares to other car apps
-
Carsales app is not the only car app available, but it is one of the best ones. Here are some of the reasons why carsales app is better than other car apps:
-
-
It has more cars than any other car app in Australia, with over 150,000 new and used cars from private sellers, dealers, and certified pre-owned programs.
-
It has more tools, features, and functionality than any other car app, such as multi-select, Snap n' Sell, valuation tool, news and reviews, seller toolkit, live stats, and more.
-
It connects you with Australia's largest online automotive marketplace, where you can find the best deals and offers for buying or selling cars.
-
It has a high rating and positive feedback from users who have downloaded and used it.
-
-
Conclusion
-
Carsales app is the ultimate guide for car buyers and sellers in Australia. It is a free mobile app that allows you to search, buy, sell, and research cars on the go. It has more cars, tools, features, and functionality than any other car app. It also connects you with Australia's largest online automotive marketplace.
-
If you are looking for a car or want to sell your car, you should download carsales app today. You will not regret it. You will save time, money, and hassle when buying or selling cars. You will also enjoy the convenience and ease of using the app.
-
So what are you waiting for? Download carsales app now and find your dream car or sell your car fast!
-
Frequently Asked Questions
-
Here are some of the frequently asked questions about carsales app:
-
-
Is carsales app free?
-
Yes, carsales app is free to download and use. However, some features may require a premium subscription or payment.
-
Is carsales app safe?
-
Yes, carsales app is safe to use. It uses encryption and security measures to protect your personal information and transactions. However, you should always be careful when dealing with strangers online and follow the safety tips provided by the app.
-
Is carsales app available in other countries?
-
No, carsales app is only available in Australia. However, you can use the website version of carsales.com.au in other countries.
-
How do I contact carsales app support?
-
If you have any questions or issues with the app, you can contact carsales app support via email at support@carsales.com.au or via phone at 13 72 53.
-
How do I update carsales app?
-
To update carsales app, you can follow these steps:
-
-
Open the App Store or Google Play Store on your device.
-
Search for "carsales" in the search bar.
-
Tap on the "UPDATE" button next to the carsales app icon.
-
Wait for the app to download and install the latest version on your device.
-
Open the app and enjoy the new features and improvements.
- 401be4b1e0
-
-
\ No newline at end of file
diff --git "a/spaces/fb700/chatglm-fitness-RLHF/crazy_functions/\350\260\267\346\255\214\346\243\200\347\264\242\345\260\217\345\212\251\346\211\213.py" "b/spaces/fb700/chatglm-fitness-RLHF/crazy_functions/\350\260\267\346\255\214\346\243\200\347\264\242\345\260\217\345\212\251\346\211\213.py"
deleted file mode 100644
index 5a5dd93f6f9ca8c47c0de193b95e863e9bb82087..0000000000000000000000000000000000000000
--- "a/spaces/fb700/chatglm-fitness-RLHF/crazy_functions/\350\260\267\346\255\214\346\243\200\347\264\242\345\260\217\345\212\251\346\211\213.py"
+++ /dev/null
@@ -1,112 +0,0 @@
-from .crazy_utils import request_gpt_model_in_new_thread_with_ui_alive
-from toolbox import CatchException, report_execption, write_results_to_file
-from toolbox import update_ui
-
-def get_meta_information(url, chatbot, history):
- import requests
- import arxiv
- import difflib
- from bs4 import BeautifulSoup
- from toolbox import get_conf
- proxies, = get_conf('proxies')
- headers = {
- 'User-Agent': 'Mozilla/5.0 (Windows NT 10.0; Win64; x64) AppleWebKit/537.36 (KHTML, like Gecko) Chrome/105.0.0.0 Safari/537.36',
- }
- # 发送 GET 请求
- response = requests.get(url, proxies=proxies, headers=headers)
-
- # 解析网页内容
- soup = BeautifulSoup(response.text, "html.parser")
-
- def string_similar(s1, s2):
- return difflib.SequenceMatcher(None, s1, s2).quick_ratio()
-
- profile = []
- # 获取所有文章的标题和作者
- for result in soup.select(".gs_ri"):
- title = result.a.text.replace('\n', ' ').replace(' ', ' ')
- author = result.select_one(".gs_a").text
- try:
- citation = result.select_one(".gs_fl > a[href*='cites']").text # 引用次数是链接中的文本,直接取出来
- except:
- citation = 'cited by 0'
- abstract = result.select_one(".gs_rs").text.strip() # 摘要在 .gs_rs 中的文本,需要清除首尾空格
- search = arxiv.Search(
- query = title,
- max_results = 1,
- sort_by = arxiv.SortCriterion.Relevance,
- )
- try:
- paper = next(search.results())
- if string_similar(title, paper.title) > 0.90: # same paper
- abstract = paper.summary.replace('\n', ' ')
- is_paper_in_arxiv = True
- else: # different paper
- abstract = abstract
- is_paper_in_arxiv = False
- paper = next(search.results())
- except:
- abstract = abstract
- is_paper_in_arxiv = False
- print(title)
- print(author)
- print(citation)
- profile.append({
- 'title':title,
- 'author':author,
- 'citation':citation,
- 'abstract':abstract,
- 'is_paper_in_arxiv':is_paper_in_arxiv,
- })
-
- chatbot[-1] = [chatbot[-1][0], title + f'\n\n是否在arxiv中(不在arxiv中无法获取完整摘要):{is_paper_in_arxiv}\n\n' + abstract]
- yield from update_ui(chatbot=chatbot, history=[]) # 刷新界面
- return profile
-
-@CatchException
-def 谷歌检索小助手(txt, llm_kwargs, plugin_kwargs, chatbot, history, system_prompt, web_port):
- # 基本信息:功能、贡献者
- chatbot.append([
- "函数插件功能?",
- "分析用户提供的谷歌学术(google scholar)搜索页面中,出现的所有文章: binary-husky,插件初始化中..."])
- yield from update_ui(chatbot=chatbot, history=history) # 刷新界面
-
- # 尝试导入依赖,如果缺少依赖,则给出安装建议
- try:
- import arxiv
- import math
- from bs4 import BeautifulSoup
- except:
- report_execption(chatbot, history,
- a = f"解析项目: {txt}",
- b = f"导入软件依赖失败。使用该模块需要额外依赖,安装方法```pip install --upgrade beautifulsoup4 arxiv```。")
- yield from update_ui(chatbot=chatbot, history=history) # 刷新界面
- return
-
- # 清空历史,以免输入溢出
- history = []
- meta_paper_info_list = yield from get_meta_information(txt, chatbot, history)
- batchsize = 5
- for batch in range(math.ceil(len(meta_paper_info_list)/batchsize)):
- if len(meta_paper_info_list[:batchsize]) > 0:
- i_say = "下面是一些学术文献的数据,提取出以下内容:" + \
- "1、英文题目;2、中文题目翻译;3、作者;4、arxiv公开(is_paper_in_arxiv);4、引用数量(cite);5、中文摘要翻译。" + \
- f"以下是信息源:{str(meta_paper_info_list[:batchsize])}"
-
- inputs_show_user = f"请分析此页面中出现的所有文章:{txt},这是第{batch+1}批"
- gpt_say = yield from request_gpt_model_in_new_thread_with_ui_alive(
- inputs=i_say, inputs_show_user=inputs_show_user,
- llm_kwargs=llm_kwargs, chatbot=chatbot, history=[],
- sys_prompt="你是一个学术翻译,请从数据中提取信息。你必须使用Markdown表格。你必须逐个文献进行处理。"
- )
-
- history.extend([ f"第{batch+1}批", gpt_say ])
- meta_paper_info_list = meta_paper_info_list[batchsize:]
-
- chatbot.append(["状态?",
- "已经全部完成,您可以试试让AI写一个Related Works,例如您可以继续输入Write an academic \"Related Works\" section about \"你搜索的研究领域\" for me."])
- msg = '正常'
- yield from update_ui(chatbot=chatbot, history=history, msg=msg) # 刷新界面
- res = write_results_to_file(history)
- chatbot.append(("完成了吗?", res));
- yield from update_ui(chatbot=chatbot, history=history, msg=msg) # 刷新界面
diff --git a/spaces/fb700/chatglm-fitness-RLHF/docs/self_analysis.md b/spaces/fb700/chatglm-fitness-RLHF/docs/self_analysis.md
deleted file mode 100644
index ebc2337194974bf210794df7d858889010fecf08..0000000000000000000000000000000000000000
--- a/spaces/fb700/chatglm-fitness-RLHF/docs/self_analysis.md
+++ /dev/null
@@ -1,378 +0,0 @@
-# chatgpt-academic项目自译解报告
-(Author补充:以下分析均由本项目调用ChatGPT一键生成,如果有不准确的地方,全怪GPT😄)
-
-
-| 文件名 | 功能描述 |
-| ------ | ------ |
-| check_proxy.py | 检查代理有效性及地理位置 |
-| colorful.py | 控制台打印彩色文字 |
-| config.py | 配置和参数设置 |
-| config_private.py | 私人配置和参数设置 |
-| core_functional.py | 核心函数和参数设置 |
-| crazy_functional.py | 高级功能插件集合 |
-| main.py | 一个 Chatbot 程序,提供各种学术翻译、文本处理和其他查询服务 |
-| multi_language.py | 识别和翻译不同语言 |
-| theme.py | 自定义 gradio 应用程序主题 |
-| toolbox.py | 工具类库,用于协助实现各种功能 |
-| crazy_functions\crazy_functions_test.py | 测试 crazy_functions 中的各种函数 |
-| crazy_functions\crazy_utils.py | 工具函数,用于字符串处理、异常检测、Markdown 格式转换等 |
-| crazy_functions\Latex全文润色.py | 对整个 Latex 项目进行润色和纠错 |
-| crazy_functions\Latex全文翻译.py | 对整个 Latex 项目进行翻译 |
-| crazy_functions\\_\_init\_\_.py | 模块初始化文件,标识 `crazy_functions` 是一个包 |
-| crazy_functions\下载arxiv论文翻译摘要.py | 下载 `arxiv` 论文的 PDF 文件,并提取摘要和翻译 |
-| crazy_functions\代码重写为全英文_多线程.py | 将Python源代码文件中的中文内容转化为英文 |
-| crazy_functions\图片生成.py | 根据激励文本使用GPT模型生成相应的图像 |
-| crazy_functions\对话历史存档.py | 将每次对话记录写入Markdown格式的文件中 |
-| crazy_functions\总结word文档.py | 对输入的word文档进行摘要生成 |
-| crazy_functions\总结音视频.py | 对输入的音视频文件进行摘要生成 |
-| crazy_functions\批量Markdown翻译.py | 将指定目录下的Markdown文件进行中英文翻译 |
-| crazy_functions\批量总结PDF文档.py | 对PDF文件进行切割和摘要生成 |
-| crazy_functions\批量总结PDF文档pdfminer.py | 对PDF文件进行文本内容的提取和摘要生成 |
-| crazy_functions\批量翻译PDF文档_多线程.py | 将指定目录下的PDF文件进行中英文翻译 |
-| crazy_functions\理解PDF文档内容.py | 对PDF文件进行摘要生成和问题解答 |
-| crazy_functions\生成函数注释.py | 自动生成Python函数的注释 |
-| crazy_functions\联网的ChatGPT.py | 使用网络爬虫和ChatGPT模型进行聊天回答 |
-| crazy_functions\解析JupyterNotebook.py | 对Jupyter Notebook进行代码解析 |
-| crazy_functions\解析项目源代码.py | 对指定编程语言的源代码进行解析 |
-| crazy_functions\询问多个大语言模型.py | 使用多个大语言模型对输入进行处理和回复 |
-| crazy_functions\读文章写摘要.py | 对论文进行解析和全文摘要生成 |
-| crazy_functions\谷歌检索小助手.py | 提供谷歌学术搜索页面中相关文章的元数据信息。 |
-| crazy_functions\高级功能函数模板.py | 使用Unsplash API发送相关图片以回复用户的输入。 |
-| request_llm\bridge_all.py | 基于不同LLM模型进行对话。 |
-| request_llm\bridge_chatglm.py | 使用ChatGLM模型生成回复,支持单线程和多线程方式。 |
-| request_llm\bridge_chatgpt.py | 基于GPT模型完成对话。 |
-| request_llm\bridge_jittorllms_llama.py | 使用JittorLLMs模型完成对话,支持单线程和多线程方式。 |
-| request_llm\bridge_jittorllms_pangualpha.py | 使用JittorLLMs模型完成对话,基于多进程和多线程方式。 |
-| request_llm\bridge_jittorllms_rwkv.py | 使用JittorLLMs模型完成聊天功能,提供包括历史信息、参数调节等在内的多个功能选项。 |
-| request_llm\bridge_moss.py | 加载Moss模型完成对话功能。 |
-| request_llm\bridge_newbing.py | 使用Newbing聊天机器人进行对话,支持单线程和多线程方式。 |
-| request_llm\bridge_newbingfree.py | 基于Bing chatbot API实现聊天机器人的文本生成功能。 |
-| request_llm\bridge_stackclaude.py | 基于Slack API实现Claude与用户的交互。 |
-| request_llm\bridge_tgui.py | 通过websocket实现聊天机器人与UI界面交互。 |
-| request_llm\edge_gpt.py | 调用Bing chatbot API提供聊天机器人服务。 |
-| request_llm\edge_gpt_free.py | 实现聊天机器人API,采用aiohttp和httpx工具库。 |
-| request_llm\test_llms.py | 对llm模型进行单元测试。 |
-
-## 接下来请你逐文件分析下面的工程[0/48] 请对下面的程序文件做一个概述: check_proxy.py
-
-这个文件主要包含了五个函数:
-
-1. `check_proxy`:用于检查代理的有效性及地理位置,输出代理配置和所在地信息。
-
-2. `backup_and_download`:用于备份当前版本并下载新版本。
-
-3. `patch_and_restart`:用于覆盖更新当前版本并重新启动程序。
-
-4. `get_current_version`:用于获取当前程序的版本号。
-
-5. `auto_update`:用于自动检查新版本并提示用户更新。如果用户选择更新,则备份并下载新版本,覆盖更新当前版本并重新启动程序。如果更新失败,则输出错误信息,并不会向用户进行任何提示。
-
-还有一个没有函数名的语句`os.environ['no_proxy'] = '*'`,用于设置环境变量,避免代理网络产生意外污染。
-
-此外,该文件导入了以下三个模块/函数:
-
-- `requests`
-- `shutil`
-- `os`
-
-## [1/48] 请对下面的程序文件做一个概述: colorful.py
-
-该文件是一个Python脚本,用于在控制台中打印彩色文字。该文件包含了一些函数,用于以不同颜色打印文本。其中,红色、绿色、黄色、蓝色、紫色、靛色分别以函数 print红、print绿、print黄、print蓝、print紫、print靛 的形式定义;亮红色、亮绿色、亮黄色、亮蓝色、亮紫色、亮靛色分别以 print亮红、print亮绿、print亮黄、print亮蓝、print亮紫、print亮靛 的形式定义。它们使用 ANSI Escape Code 将彩色输出从控制台突出显示。如果运行在 Linux 操作系统上,文件所执行的操作被留空;否则,该文件导入了 colorama 库并调用 init() 函数进行初始化。最后,通过一系列条件语句,该文件通过将所有彩色输出函数的名称重新赋值为 print 函数的名称来避免输出文件的颜色问题。
-
-## [2/48] 请对下面的程序文件做一个概述: config.py
-
-这个程序文件是用来配置和参数设置的。它包含了许多设置,如API key,使用代理,线程数,默认模型,超时时间等等。此外,它还包含了一些高级功能,如URL重定向等。这些设置将会影响到程序的行为和性能。
-
-## [3/48] 请对下面的程序文件做一个概述: config_private.py
-
-这个程序文件是一个Python脚本,文件名为config_private.py。其中包含以下变量的赋值:
-
-1. API_KEY:API密钥。
-2. USE_PROXY:是否应用代理。
-3. proxies:如果使用代理,则设置代理网络的协议(socks5/http)、地址(localhost)和端口(11284)。
-4. DEFAULT_WORKER_NUM:默认的工作线程数量。
-5. SLACK_CLAUDE_BOT_ID:Slack机器人ID。
-6. SLACK_CLAUDE_USER_TOKEN:Slack用户令牌。
-
-## [4/48] 请对下面的程序文件做一个概述: core_functional.py
-
-这是一个名为core_functional.py的源代码文件,该文件定义了一个名为get_core_functions()的函数,该函数返回一个字典,该字典包含了各种学术翻译润色任务的说明和相关参数,如颜色、前缀、后缀等。这些任务包括英语学术润色、中文学术润色、查找语法错误、中译英、学术中英互译、英译中、找图片和参考文献转Bib。其中,一些任务还定义了预处理函数用于处理任务的输入文本。
-
-## [5/48] 请对下面的程序文件做一个概述: crazy_functional.py
-
-此程序文件(crazy_functional.py)是一个函数插件集合,包含了多个函数插件的定义和调用。这些函数插件旨在提供一些高级功能,如解析项目源代码、批量翻译PDF文档和Latex全文润色等。其中一些插件还支持热更新功能,不需要重启程序即可生效。文件中的函数插件按照功能进行了分类(第一组和第二组),并且有不同的调用方式(作为按钮或下拉菜单)。
-
-## [6/48] 请对下面的程序文件做一个概述: main.py
-
-这是一个Python程序文件,文件名为main.py。该程序包含一个名为main的函数,程序会自动运行该函数。程序要求已经安装了gradio、os等模块,会根据配置文件加载代理、model、API Key等信息。程序提供了Chatbot功能,实现了一个对话界面,用户可以输入问题,然后Chatbot可以回答问题或者提供相关功能。程序还包含了基础功能区、函数插件区、更换模型 & SysPrompt & 交互界面布局、备选输入区,用户可以在这些区域选择功能和插件进行使用。程序中还包含了一些辅助模块,如logging等。
-
-## [7/48] 请对下面的程序文件做一个概述: multi_language.py
-
-该文件multi_language.py是用于将项目翻译成不同语言的程序。它包含了以下函数和变量:lru_file_cache、contains_chinese、split_list、map_to_json、read_map_from_json、advanced_split、trans、trans_json、step_1_core_key_translate、CACHE_FOLDER、blacklist、LANG、TransPrompt、cached_translation等。注释和文档字符串提供了有关程序的说明,例如如何使用该程序,如何修改“LANG”和“TransPrompt”变量等。
-
-## [8/48] 请对下面的程序文件做一个概述: theme.py
-
-这是一个Python源代码文件,文件名为theme.py。此文件中定义了一个函数adjust_theme,其功能是自定义gradio应用程序的主题,包括调整颜色、字体、阴影等。如果允许,则添加一个看板娘。此文件还包括变量advanced_css,其中包含一些CSS样式,用于高亮显示代码和自定义聊天框样式。此文件还导入了get_conf函数和gradio库。
-
-## [9/48] 请对下面的程序文件做一个概述: toolbox.py
-
-toolbox.py是一个工具类库,其中主要包含了一些函数装饰器和小工具函数,用于协助实现聊天机器人所需的各种功能,包括文本处理、功能插件加载、异常检测、Markdown格式转换,文件读写等等。此外,该库还包含一些依赖、参数配置等信息。该库易于理解和维护。
-
-## [10/48] 请对下面的程序文件做一个概述: crazy_functions\crazy_functions_test.py
-
-这个文件是一个Python测试模块,用于测试crazy_functions中的各种函数插件。这些函数包括:解析Python项目源代码、解析Cpp项目源代码、Latex全文润色、Markdown中译英、批量翻译PDF文档、谷歌检索小助手、总结word文档、下载arxiv论文并翻译摘要、联网回答问题、和解析Jupyter Notebooks。对于每个函数插件,都有一个对应的测试函数来进行测试。
-
-## [11/48] 请对下面的程序文件做一个概述: crazy_functions\crazy_utils.py
-
-这个Python文件中包括了两个函数:
-
-1. `input_clipping`: 该函数用于裁剪输入文本长度,使其不超过一定的限制。
-2. `request_gpt_model_in_new_thread_with_ui_alive`: 该函数用于请求 GPT 模型并保持用户界面的响应,支持多线程和实时更新用户界面。
-
-这两个函数都依赖于从 `toolbox` 和 `request_llm` 中导入的一些工具函数。函数的输入和输出有详细的描述文档。
-
-## [12/48] 请对下面的程序文件做一个概述: crazy_functions\Latex全文润色.py
-
-这是一个Python程序文件,文件名为crazy_functions\Latex全文润色.py。文件包含了一个PaperFileGroup类和三个函数Latex英文润色,Latex中文润色和Latex英文纠错。程序使用了字符串处理、正则表达式、文件读写、多线程等技术,主要作用是对整个Latex项目进行润色和纠错。其中润色和纠错涉及到了对文本的语法、清晰度和整体可读性等方面的提升。此外,该程序还参考了第三方库,并封装了一些工具函数。
-
-## [13/48] 请对下面的程序文件做一个概述: crazy_functions\Latex全文翻译.py
-
-这个文件包含两个函数 `Latex英译中` 和 `Latex中译英`,它们都会对整个Latex项目进行翻译。这个文件还包含一个类 `PaperFileGroup`,它拥有一个方法 `run_file_split`,用于把长文本文件分成多个短文件。其中使用了工具库 `toolbox` 中的一些函数和从 `request_llm` 中导入了 `model_info`。接下来的函数把文件读取进来,把它们的注释删除,进行分割,并进行翻译。这个文件还包括了一些异常处理和界面更新的操作。
-
-## [14/48] 请对下面的程序文件做一个概述: crazy_functions\__init__.py
-
-这是一个Python模块的初始化文件(__init__.py),命名为"crazy_functions"。该模块包含了一些疯狂的函数,但该文件并没有实现这些函数,而是作为一个包(package)来导入其它的Python模块以实现这些函数。在该文件中,没有定义任何类或函数,它唯一的作用就是标识"crazy_functions"模块是一个包。
-
-## [15/48] 请对下面的程序文件做一个概述: crazy_functions\下载arxiv论文翻译摘要.py
-
-这是一个 Python 程序文件,文件名为 `下载arxiv论文翻译摘要.py`。程序包含多个函数,其中 `下载arxiv论文并翻译摘要` 函数的作用是下载 `arxiv` 论文的 PDF 文件,提取摘要并使用 GPT 对其进行翻译。其他函数包括用于下载 `arxiv` 论文的 `download_arxiv_` 函数和用于获取文章信息的 `get_name` 函数,其中涉及使用第三方库如 requests, BeautifulSoup 等。该文件还包含一些用于调试和存储文件的代码段。
-
-## [16/48] 请对下面的程序文件做一个概述: crazy_functions\代码重写为全英文_多线程.py
-
-该程序文件是一个多线程程序,主要功能是将指定目录下的所有Python代码文件中的中文内容转化为英文,并将转化后的代码存储到一个新的文件中。其中,程序使用了GPT-3等技术进行中文-英文的转化,同时也进行了一些Token限制下的处理,以防止程序发生错误。程序在执行过程中还会输出一些提示信息,并将所有转化过的代码文件存储到指定目录下。在程序执行结束后,还会生成一个任务执行报告,记录程序运行的详细信息。
-
-## [17/48] 请对下面的程序文件做一个概述: crazy_functions\图片生成.py
-
-该程序文件提供了一个用于生成图像的函数`图片生成`。函数实现的过程中,会调用`gen_image`函数来生成图像,并返回图像生成的网址和本地文件地址。函数有多个参数,包括`prompt`(激励文本)、`llm_kwargs`(GPT模型的参数)、`plugin_kwargs`(插件模型的参数)等。函数核心代码使用了`requests`库向OpenAI API请求图像,并做了简单的处理和保存。函数还更新了交互界面,清空聊天历史并显示正在生成图像的消息和最终的图像网址和预览。
-
-## [18/48] 请对下面的程序文件做一个概述: crazy_functions\对话历史存档.py
-
-这个文件是名为crazy_functions\对话历史存档.py的Python程序文件,包含了4个函数:
-
-1. write_chat_to_file(chatbot, history=None, file_name=None):用来将对话记录以Markdown格式写入文件中,并且生成文件名,如果没指定文件名则用当前时间。写入完成后将文件路径打印出来。
-
-2. gen_file_preview(file_name):从传入的文件中读取内容,解析出对话历史记录并返回前100个字符,用于文件预览。
-
-3. read_file_to_chat(chatbot, history, file_name):从传入的文件中读取内容,解析出对话历史记录并更新聊天显示框。
-
-4. 对话历史存档(txt, llm_kwargs, plugin_kwargs, chatbot, history, system_prompt, web_port):一个主要函数,用于保存当前对话记录并提醒用户。如果用户希望加载历史记录,则调用read_file_to_chat()来更新聊天显示框。如果用户希望删除历史记录,调用删除所有本地对话历史记录()函数完成删除操作。
-
-## [19/48] 请对下面的程序文件做一个概述: crazy_functions\总结word文档.py
-
-该程序文件实现了一个总结Word文档的功能,使用Python的docx库读取docx格式的文件,使用pywin32库读取doc格式的文件。程序会先根据传入的txt参数搜索需要处理的文件,并逐个解析其中的内容,将内容拆分为指定长度的文章片段,然后使用另一个程序文件中的request_gpt_model_in_new_thread_with_ui_alive函数进行中文概述。最后将所有的总结结果写入一个文件中,并在界面上进行展示。
-
-## [20/48] 请对下面的程序文件做一个概述: crazy_functions\总结音视频.py
-
-该程序文件包括两个函数:split_audio_file()和AnalyAudio(),并且导入了一些必要的库并定义了一些工具函数。split_audio_file用于将音频文件分割成多个时长相等的片段,返回一个包含所有切割音频片段文件路径的列表,而AnalyAudio用来分析音频文件,通过调用whisper模型进行音频转文字并使用GPT模型对音频内容进行概述,最终将所有总结结果写入结果文件中。
-
-## [21/48] 请对下面的程序文件做一个概述: crazy_functions\批量Markdown翻译.py
-
-该程序文件名为`批量Markdown翻译.py`,包含了以下功能:读取Markdown文件,将长文本分离开来,将Markdown文件进行翻译(英译中和中译英),整理结果并退出。程序使用了多线程以提高效率。程序使用了`tiktoken`依赖库,可能需要额外安装。文件中还有一些其他的函数和类,但与文件名所描述的功能无关。
-
-## [22/48] 请对下面的程序文件做一个概述: crazy_functions\批量总结PDF文档.py
-
-该文件是一个Python脚本,名为crazy_functions\批量总结PDF文档.py。在导入了一系列库和工具函数后,主要定义了5个函数,其中包括一个错误处理装饰器(@CatchException),用于批量总结PDF文档。该函数主要实现对PDF文档的解析,并调用模型生成中英文摘要。
-
-## [23/48] 请对下面的程序文件做一个概述: crazy_functions\批量总结PDF文档pdfminer.py
-
-该程序文件是一个用于批量总结PDF文档的函数插件,使用了pdfminer插件和BeautifulSoup库来提取PDF文档的文本内容,对每个PDF文件分别进行处理并生成中英文摘要。同时,该程序文件还包括一些辅助工具函数和处理异常的装饰器。
-
-## [24/48] 请对下面的程序文件做一个概述: crazy_functions\批量翻译PDF文档_多线程.py
-
-这个程序文件是一个Python脚本,文件名为“批量翻译PDF文档_多线程.py”。它主要使用了“toolbox”、“request_gpt_model_in_new_thread_with_ui_alive”、“request_gpt_model_multi_threads_with_very_awesome_ui_and_high_efficiency”、“colorful”等Python库和自定义的模块“crazy_utils”的一些函数。程序实现了一个批量翻译PDF文档的功能,可以自动解析PDF文件中的基础信息,递归地切割PDF文件,翻译和处理PDF论文中的所有内容,并生成相应的翻译结果文件(包括md文件和html文件)。功能比较复杂,其中需要调用多个函数和依赖库,涉及到多线程操作和UI更新。文件中有详细的注释和变量命名,代码比较清晰易读。
-
-## [25/48] 请对下面的程序文件做一个概述: crazy_functions\理解PDF文档内容.py
-
-该程序文件实现了一个名为“理解PDF文档内容”的函数,该函数可以为输入的PDF文件提取摘要以及正文各部分的主要内容,并在提取过程中根据上下文关系进行学术性问题解答。该函数依赖于多个辅助函数和第三方库,并在执行过程中针对可能出现的异常进行了处理。
-
-## [26/48] 请对下面的程序文件做一个概述: crazy_functions\生成函数注释.py
-
-该程序文件是一个Python模块文件,文件名为“生成函数注释.py”,定义了两个函数:一个是生成函数注释的主函数“生成函数注释”,另一个是通过装饰器实现异常捕捉的函数“批量生成函数注释”。该程序文件依赖于“toolbox”和本地“crazy_utils”模块,并且在运行时使用了多线程技术和GPT模型来生成注释。函数生成的注释结果使用Markdown表格输出并写入历史记录文件。
-
-## [27/48] 请对下面的程序文件做一个概述: crazy_functions\联网的ChatGPT.py
-
-这是一个名为`联网的ChatGPT.py`的Python程序文件,其中定义了一个函数`连接网络回答问题`。该函数通过爬取搜索引擎的结果和访问网页来综合回答给定的问题,并使用ChatGPT模型完成回答。此外,该文件还包括一些工具函数,例如从网页中抓取文本和使用代理访问网页。
-
-## [28/48] 请对下面的程序文件做一个概述: crazy_functions\解析JupyterNotebook.py
-
-这个程序文件包含了两个函数: `parseNotebook()`和`解析ipynb文件()`,并且引入了一些工具函数和类。`parseNotebook()`函数将Jupyter Notebook文件解析为文本代码块,`解析ipynb文件()`函数则用于解析多个Jupyter Notebook文件,使用`parseNotebook()`解析每个文件和一些其他的处理。函数中使用了多线程处理输入和输出,并且将结果写入到文件中。
-
-## [29/48] 请对下面的程序文件做一个概述: crazy_functions\解析项目源代码.py
-
-这是一个源代码分析的Python代码文件,其中定义了多个函数,包括解析一个Python项目、解析一个C项目、解析一个C项目的头文件和解析一个Java项目等。其中解析源代码新函数是实际处理源代码分析并生成报告的函数。该函数首先会逐个读取传入的源代码文件,生成对应的请求内容,通过多线程发送到chatgpt进行分析。然后将结果写入文件,并进行汇总分析。最后通过调用update_ui函数刷新界面,完整实现了源代码的分析。
-
-## [30/48] 请对下面的程序文件做一个概述: crazy_functions\询问多个大语言模型.py
-
-该程序文件包含两个函数:同时问询()和同时问询_指定模型(),它们的作用是使用多个大语言模型同时对用户输入进行处理,返回对应模型的回复结果。同时问询()会默认使用ChatGPT和ChatGLM两个模型,而同时问询_指定模型()则可以指定要使用的模型。该程序文件还引用了其他的模块和函数库。
-
-## [31/48] 请对下面的程序文件做一个概述: crazy_functions\读文章写摘要.py
-
-这个程序文件是一个Python模块,文件名为crazy_functions\读文章写摘要.py。该模块包含了两个函数,其中主要函数是"读文章写摘要"函数,其实现了解析给定文件夹中的tex文件,对其中每个文件的内容进行摘要生成,并根据各论文片段的摘要,最终生成全文摘要。第二个函数是"解析Paper"函数,用于解析单篇论文文件。其中用到了一些工具函数和库,如update_ui、CatchException、report_execption、write_results_to_file等。
-
-## [32/48] 请对下面的程序文件做一个概述: crazy_functions\谷歌检索小助手.py
-
-该文件是一个Python模块,文件名为“谷歌检索小助手.py”。该模块包含两个函数,一个是“get_meta_information()”,用于从提供的网址中分析出所有相关的学术文献的元数据信息;另一个是“谷歌检索小助手()”,是主函数,用于分析用户提供的谷歌学术搜索页面中出现的文章,并提取相关信息。其中,“谷歌检索小助手()”函数依赖于“get_meta_information()”函数,并调用了其他一些Python模块,如“arxiv”、“math”、“bs4”等。
-
-## [33/48] 请对下面的程序文件做一个概述: crazy_functions\高级功能函数模板.py
-
-该程序文件定义了一个名为高阶功能模板函数的函数,该函数接受多个参数,包括输入的文本、gpt模型参数、插件模型参数、聊天显示框的句柄、聊天历史等,并利用送出请求,使用 Unsplash API 发送相关图片。其中,为了避免输入溢出,函数会在开始时清空历史。函数也有一些 UI 更新的语句。该程序文件还依赖于其他两个模块:CatchException 和 update_ui,以及一个名为 request_gpt_model_in_new_thread_with_ui_alive 的来自 crazy_utils 模块(应该是自定义的工具包)的函数。
-
-## [34/48] 请对下面的程序文件做一个概述: request_llm\bridge_all.py
-
-该文件包含两个函数:predict和predict_no_ui_long_connection,用于基于不同的LLM模型进行对话。该文件还包含一个lazyloadTiktoken类和一个LLM_CATCH_EXCEPTION修饰器函数。其中lazyloadTiktoken类用于懒加载模型的tokenizer,LLM_CATCH_EXCEPTION用于错误处理。整个文件还定义了一些全局变量和模型信息字典,用于引用和配置LLM模型。
-
-## [35/48] 请对下面的程序文件做一个概述: request_llm\bridge_chatglm.py
-
-这是一个Python程序文件,名为`bridge_chatglm.py`,其中定义了一个名为`GetGLMHandle`的类和三个方法:`predict_no_ui_long_connection`、 `predict`和 `stream_chat`。该文件依赖于多个Python库,如`transformers`和`sentencepiece`。该文件实现了一个聊天机器人,使用ChatGLM模型来生成回复,支持单线程和多线程方式。程序启动时需要加载ChatGLM的模型和tokenizer,需要一段时间。在配置文件`config.py`中设置参数会影响模型的内存和显存使用,因此程序可能会导致低配计算机卡死。
-
-## [36/48] 请对下面的程序文件做一个概述: request_llm\bridge_chatgpt.py
-
-该文件为 Python 代码文件,文件名为 request_llm\bridge_chatgpt.py。该代码文件主要提供三个函数:predict、predict_no_ui和 predict_no_ui_long_connection,用于发送至 chatGPT 并等待回复,获取输出。该代码文件还包含一些辅助函数,用于处理连接异常、生成 HTTP 请求等。该文件的代码架构清晰,使用了多个自定义函数和模块。
-
-## [37/48] 请对下面的程序文件做一个概述: request_llm\bridge_jittorllms_llama.py
-
-该代码文件实现了一个聊天机器人,其中使用了 JittorLLMs 模型。主要包括以下几个部分:
-1. GetGLMHandle 类:一个进程类,用于加载 JittorLLMs 模型并接收并处理请求。
-2. predict_no_ui_long_connection 函数:一个多线程方法,用于在后台运行聊天机器人。
-3. predict 函数:一个单线程方法,用于在前端页面上交互式调用聊天机器人,以获取用户输入并返回相应的回复。
-
-这个文件中还有一些辅助函数和全局变量,例如 importlib、time、threading 等。
-
-## [38/48] 请对下面的程序文件做一个概述: request_llm\bridge_jittorllms_pangualpha.py
-
-这个文件是为了实现使用jittorllms(一种机器学习模型)来进行聊天功能的代码。其中包括了模型加载、模型的参数加载、消息的收发等相关操作。其中使用了多进程和多线程来提高性能和效率。代码中还包括了处理依赖关系的函数和预处理函数等。
-
-## [39/48] 请对下面的程序文件做一个概述: request_llm\bridge_jittorllms_rwkv.py
-
-这个文件是一个Python程序,文件名为request_llm\bridge_jittorllms_rwkv.py。它依赖transformers、time、threading、importlib、multiprocessing等库。在文件中,通过定义GetGLMHandle类加载jittorllms模型参数和定义stream_chat方法来实现与jittorllms模型的交互。同时,该文件还定义了predict_no_ui_long_connection和predict方法来处理历史信息、调用jittorllms模型、接收回复信息并输出结果。
-
-## [40/48] 请对下面的程序文件做一个概述: request_llm\bridge_moss.py
-
-该文件为一个Python源代码文件,文件名为 request_llm\bridge_moss.py。代码定义了一个 GetGLMHandle 类和两个函数 predict_no_ui_long_connection 和 predict。
-
-GetGLMHandle 类继承自Process类(多进程),主要功能是启动一个子进程并加载 MOSS 模型参数,通过 Pipe 进行主子进程的通信。该类还定义了 check_dependency、moss_init、run 和 stream_chat 等方法,其中 check_dependency 和 moss_init 是子进程的初始化方法,run 是子进程运行方法,stream_chat 实现了主进程和子进程的交互过程。
-
-函数 predict_no_ui_long_connection 是多线程方法,调用 GetGLMHandle 类加载 MOSS 参数后使用 stream_chat 实现主进程和子进程的交互过程。
-
-函数 predict 是单线程方法,通过调用 update_ui 将交互过程中 MOSS 的回复实时更新到UI(User Interface)中,并执行一个 named function(additional_fn)指定的函数对输入进行预处理。
-
-## [41/48] 请对下面的程序文件做一个概述: request_llm\bridge_newbing.py
-
-这是一个名为`bridge_newbing.py`的程序文件,包含三个部分:
-
-第一部分使用from语句导入了`edge_gpt`模块的`NewbingChatbot`类。
-
-第二部分定义了一个名为`NewBingHandle`的继承自进程类的子类,该类会检查依赖性并启动进程。同时,该部分还定义了一个名为`predict_no_ui_long_connection`的多线程方法和一个名为`predict`的单线程方法,用于与NewBing进行通信。
-
-第三部分定义了一个名为`newbing_handle`的全局变量,并导出了`predict_no_ui_long_connection`和`predict`这两个方法,以供其他程序可以调用。
-
-## [42/48] 请对下面的程序文件做一个概述: request_llm\bridge_newbingfree.py
-
-这个Python文件包含了三部分内容。第一部分是来自edge_gpt_free.py文件的聊天机器人程序。第二部分是子进程Worker,用于调用主体。第三部分提供了两个函数:predict_no_ui_long_connection和predict用于调用NewBing聊天机器人和返回响应。其中predict函数还提供了一些参数用于控制聊天机器人的回复和更新UI界面。
-
-## [43/48] 请对下面的程序文件做一个概述: request_llm\bridge_stackclaude.py
-
-这是一个Python源代码文件,文件名为request_llm\bridge_stackclaude.py。代码分为三个主要部分:
-
-第一部分定义了Slack API Client类,实现Slack消息的发送、接收、循环监听,用于与Slack API进行交互。
-
-第二部分定义了ClaudeHandle类,继承Process类,用于创建子进程Worker,调用主体,实现Claude与用户交互的功能。
-
-第三部分定义了predict_no_ui_long_connection和predict两个函数,主要用于通过调用ClaudeHandle对象的stream_chat方法来获取Claude的回复,并更新ui以显示相关信息。其中predict函数采用单线程方法,而predict_no_ui_long_connection函数使用多线程方法。
-
-## [44/48] 请对下面的程序文件做一个概述: request_llm\bridge_tgui.py
-
-该文件是一个Python代码文件,名为request_llm\bridge_tgui.py。它包含了一些函数用于与chatbot UI交互,并通过WebSocket协议与远程LLM模型通信完成文本生成任务,其中最重要的函数是predict()和predict_no_ui_long_connection()。这个程序还有其他的辅助函数,如random_hash()。整个代码文件在协作的基础上完成了一次修改。
-
-## [45/48] 请对下面的程序文件做一个概述: request_llm\edge_gpt.py
-
-该文件是一个用于调用Bing chatbot API的Python程序,它由多个类和辅助函数构成,可以根据给定的对话连接在对话中提出问题,使用websocket与远程服务通信。程序实现了一个聊天机器人,可以为用户提供人工智能聊天。
-
-## [46/48] 请对下面的程序文件做一个概述: request_llm\edge_gpt_free.py
-
-该代码文件为一个会话API,可通过Chathub发送消息以返回响应。其中使用了 aiohttp 和 httpx 库进行网络请求并发送。代码中包含了一些函数和常量,多数用于生成请求数据或是请求头信息等。同时该代码文件还包含了一个 Conversation 类,调用该类可实现对话交互。
-
-## [47/48] 请对下面的程序文件做一个概述: request_llm\test_llms.py
-
-这个文件是用于对llm模型进行单元测试的Python程序。程序导入一个名为"request_llm.bridge_newbingfree"的模块,然后三次使用该模块中的predict_no_ui_long_connection()函数进行预测,并输出结果。此外,还有一些注释掉的代码段,这些代码段也是关于模型预测的。
-
-## 用一张Markdown表格简要描述以下文件的功能:
-check_proxy.py, colorful.py, config.py, config_private.py, core_functional.py, crazy_functional.py, main.py, multi_language.py, theme.py, toolbox.py, crazy_functions\crazy_functions_test.py, crazy_functions\crazy_utils.py, crazy_functions\Latex全文润色.py, crazy_functions\Latex全文翻译.py, crazy_functions\__init__.py, crazy_functions\下载arxiv论文翻译摘要.py。根据以上分析,用一句话概括程序的整体功能。
-
-| 文件名 | 功能描述 |
-| ------ | ------ |
-| check_proxy.py | 检查代理有效性及地理位置 |
-| colorful.py | 控制台打印彩色文字 |
-| config.py | 配置和参数设置 |
-| config_private.py | 私人配置和参数设置 |
-| core_functional.py | 核心函数和参数设置 |
-| crazy_functional.py | 高级功能插件集合 |
-| main.py | 一个 Chatbot 程序,提供各种学术翻译、文本处理和其他查询服务 |
-| multi_language.py | 识别和翻译不同语言 |
-| theme.py | 自定义 gradio 应用程序主题 |
-| toolbox.py | 工具类库,用于协助实现各种功能 |
-| crazy_functions\crazy_functions_test.py | 测试 crazy_functions 中的各种函数 |
-| crazy_functions\crazy_utils.py | 工具函数,用于字符串处理、异常检测、Markdown 格式转换等 |
-| crazy_functions\Latex全文润色.py | 对整个 Latex 项目进行润色和纠错 |
-| crazy_functions\Latex全文翻译.py | 对整个 Latex 项目进行翻译 |
-| crazy_functions\__init__.py | 模块初始化文件,标识 `crazy_functions` 是一个包 |
-| crazy_functions\下载arxiv论文翻译摘要.py | 下载 `arxiv` 论文的 PDF 文件,并提取摘要和翻译 |
-
-这些程序源文件提供了基础的文本和语言处理功能、工具函数和高级插件,使 Chatbot 能够处理各种复杂的学术文本问题,包括润色、翻译、搜索、下载、解析等。
-
-## 用一张Markdown表格简要描述以下文件的功能:
-crazy_functions\代码重写为全英文_多线程.py, crazy_functions\图片生成.py, crazy_functions\对话历史存档.py, crazy_functions\总结word文档.py, crazy_functions\总结音视频.py, crazy_functions\批量Markdown翻译.py, crazy_functions\批量总结PDF文档.py, crazy_functions\批量总结PDF文档pdfminer.py, crazy_functions\批量翻译PDF文档_多线程.py, crazy_functions\理解PDF文档内容.py, crazy_functions\生成函数注释.py, crazy_functions\联网的ChatGPT.py, crazy_functions\解析JupyterNotebook.py, crazy_functions\解析项目源代码.py, crazy_functions\询问多个大语言模型.py, crazy_functions\读文章写摘要.py。根据以上分析,用一句话概括程序的整体功能。
-
-| 文件名 | 功能简述 |
-| --- | --- |
-| 代码重写为全英文_多线程.py | 将Python源代码文件中的中文内容转化为英文 |
-| 图片生成.py | 根据激励文本使用GPT模型生成相应的图像 |
-| 对话历史存档.py | 将每次对话记录写入Markdown格式的文件中 |
-| 总结word文档.py | 对输入的word文档进行摘要生成 |
-| 总结音视频.py | 对输入的音视频文件进行摘要生成 |
-| 批量Markdown翻译.py | 将指定目录下的Markdown文件进行中英文翻译 |
-| 批量总结PDF文档.py | 对PDF文件进行切割和摘要生成 |
-| 批量总结PDF文档pdfminer.py | 对PDF文件进行文本内容的提取和摘要生成 |
-| 批量翻译PDF文档_多线程.py | 将指定目录下的PDF文件进行中英文翻译 |
-| 理解PDF文档内容.py | 对PDF文件进行摘要生成和问题解答 |
-| 生成函数注释.py | 自动生成Python函数的注释 |
-| 联网的ChatGPT.py | 使用网络爬虫和ChatGPT模型进行聊天回答 |
-| 解析JupyterNotebook.py | 对Jupyter Notebook进行代码解析 |
-| 解析项目源代码.py | 对指定编程语言的源代码进行解析 |
-| 询问多个大语言模型.py | 使用多个大语言模型对输入进行处理和回复 |
-| 读文章写摘要.py | 对论文进行解析和全文摘要生成 |
-
-概括程序的整体功能:提供了一系列处理文本、文件和代码的功能,使用了各类语言模型、多线程、网络请求和数据解析技术来提高效率和精度。
-
-## 用一张Markdown表格简要描述以下文件的功能:
-crazy_functions\谷歌检索小助手.py, crazy_functions\高级功能函数模板.py, request_llm\bridge_all.py, request_llm\bridge_chatglm.py, request_llm\bridge_chatgpt.py, request_llm\bridge_jittorllms_llama.py, request_llm\bridge_jittorllms_pangualpha.py, request_llm\bridge_jittorllms_rwkv.py, request_llm\bridge_moss.py, request_llm\bridge_newbing.py, request_llm\bridge_newbingfree.py, request_llm\bridge_stackclaude.py, request_llm\bridge_tgui.py, request_llm\edge_gpt.py, request_llm\edge_gpt_free.py, request_llm\test_llms.py。根据以上分析,用一句话概括程序的整体功能。
-
-| 文件名 | 功能描述 |
-| --- | --- |
-| crazy_functions\谷歌检索小助手.py | 提供谷歌学术搜索页面中相关文章的元数据信息。 |
-| crazy_functions\高级功能函数模板.py | 使用Unsplash API发送相关图片以回复用户的输入。 |
-| request_llm\bridge_all.py | 基于不同LLM模型进行对话。 |
-| request_llm\bridge_chatglm.py | 使用ChatGLM模型生成回复,支持单线程和多线程方式。 |
-| request_llm\bridge_chatgpt.py | 基于GPT模型完成对话。 |
-| request_llm\bridge_jittorllms_llama.py | 使用JittorLLMs模型完成对话,支持单线程和多线程方式。 |
-| request_llm\bridge_jittorllms_pangualpha.py | 使用JittorLLMs模型完成对话,基于多进程和多线程方式。 |
-| request_llm\bridge_jittorllms_rwkv.py | 使用JittorLLMs模型完成聊天功能,提供包括历史信息、参数调节等在内的多个功能选项。 |
-| request_llm\bridge_moss.py | 加载Moss模型完成对话功能。 |
-| request_llm\bridge_newbing.py | 使用Newbing聊天机器人进行对话,支持单线程和多线程方式。 |
-| request_llm\bridge_newbingfree.py | 基于Bing chatbot API实现聊天机器人的文本生成功能。 |
-| request_llm\bridge_stackclaude.py | 基于Slack API实现Claude与用户的交互。 |
-| request_llm\bridge_tgui.py | 通过websocket实现聊天机器人与UI界面交互。 |
-| request_llm\edge_gpt.py | 调用Bing chatbot API提供聊天机器人服务。 |
-| request_llm\edge_gpt_free.py | 实现聊天机器人API,采用aiohttp和httpx工具库。 |
-| request_llm\test_llms.py | 对llm模型进行单元测试。 |
-| 程序整体功能 | 实现不同种类的聊天机器人,可以根据输入进行文本生成。 |
diff --git a/spaces/fclong/summary/fengshen/data/data_utils/mask_utils.py b/spaces/fclong/summary/fengshen/data/data_utils/mask_utils.py
deleted file mode 100644
index 0009f00272bf6feff1dbd491153332584cb431e1..0000000000000000000000000000000000000000
--- a/spaces/fclong/summary/fengshen/data/data_utils/mask_utils.py
+++ /dev/null
@@ -1,285 +0,0 @@
-import collections
-
-import numpy as np
-
-MaskedLmInstance = collections.namedtuple("MaskedLmInstance",
- ["index", "label"])
-
-
-def is_start_piece(piece):
- """Check if the current word piece is the starting piece (BERT)."""
- # When a word has been split into
- # WordPieces, the first token does not have any marker and any subsequence
- # tokens are prefixed with ##. So whenever we see the ## token, we
- # append it to the previous set of word indexes.
- return not piece.startswith("##")
-
-
-def create_masked_lm_predictions(tokens,
- vocab_id_list, vocab_id_to_token_dict,
- masked_lm_prob,
- cls_id, sep_id, mask_id,
- max_predictions_per_seq,
- np_rng,
- max_ngrams=3,
- do_whole_word_mask=True,
- favor_longer_ngram=False,
- do_permutation=False,
- geometric_dist=False,
- masking_style="bert",
- zh_tokenizer=None):
- """Creates the predictions for the masked LM objective.
- Note: Tokens here are vocab ids and not text tokens."""
- '''
- modified from Megatron-LM
- Args:
- tokens: 输入
- vocab_id_list: 词表token_id_list
- vocab_id_to_token_dict: token_id到token字典
- masked_lm_prob:mask概率
- cls_id、sep_id、mask_id:特殊token
- max_predictions_per_seq:最大mask个数
- np_rng:mask随机数
- max_ngrams:最大词长度
- do_whole_word_mask:是否做全词掩码
- favor_longer_ngram:优先用长的词
- do_permutation:是否打乱
- geometric_dist:用np_rng.geometric做随机
- masking_style:mask类型
- zh_tokenizer:WWM的分词器,比如用jieba.lcut做分词之类的
- '''
- cand_indexes = []
- # Note(mingdachen): We create a list for recording if the piece is
- # the starting piece of current token, where 1 means true, so that
- # on-the-fly whole word masking is possible.
- token_boundary = [0] * len(tokens)
- # 如果没有指定中文分词器,那就直接按##算
- if zh_tokenizer is None:
- for (i, token) in enumerate(tokens):
- if token == cls_id or token == sep_id:
- token_boundary[i] = 1
- continue
- # Whole Word Masking means that if we mask all of the wordpieces
- # corresponding to an original word.
- #
- # Note that Whole Word Masking does *not* change the training code
- # at all -- we still predict each WordPiece independently, softmaxed
- # over the entire vocabulary.
- if (do_whole_word_mask and len(cand_indexes) >= 1 and
- not is_start_piece(vocab_id_to_token_dict[token])):
- cand_indexes[-1].append(i)
- else:
- cand_indexes.append([i])
- if is_start_piece(vocab_id_to_token_dict[token]):
- token_boundary[i] = 1
- else:
- # 如果指定了中文分词器,那就先用分词器分词,然后再进行判断
- # 获取去掉CLS SEP的原始文本
- raw_tokens = []
- for t in tokens:
- if t != cls_id and t != sep_id:
- raw_tokens.append(t)
- raw_tokens = [vocab_id_to_token_dict[i] for i in raw_tokens]
- # 分词然后获取每次字开头的最长词的长度
- word_list = set(zh_tokenizer(''.join(raw_tokens), HMM=True))
- word_length_dict = {}
- for w in word_list:
- if len(w) < 1:
- continue
- if w[0] not in word_length_dict:
- word_length_dict[w[0]] = len(w)
- elif word_length_dict[w[0]] < len(w):
- word_length_dict[w[0]] = len(w)
- i = 0
- # 从词表里面检索
- while i < len(tokens):
- token_id = tokens[i]
- token = vocab_id_to_token_dict[token_id]
- if len(token) == 0 or token_id == cls_id or token_id == sep_id:
- token_boundary[i] = 1
- i += 1
- continue
- word_max_length = 1
- if token[0] in word_length_dict:
- word_max_length = word_length_dict[token[0]]
- j = 0
- word = ''
- word_end = i+1
- # 兼容以前##的形式,如果后面的词是##开头的,那么直接把后面的拼到前面当作一个词
- old_style = False
- while word_end < len(tokens) and vocab_id_to_token_dict[tokens[word_end]].startswith('##'):
- old_style = True
- word_end += 1
- if not old_style:
- while j < word_max_length and i+j < len(tokens):
- cur_token = tokens[i+j]
- word += vocab_id_to_token_dict[cur_token]
- j += 1
- if word in word_list:
- word_end = i+j
- cand_indexes.append([p for p in range(i, word_end)])
- token_boundary[i] = 1
- i = word_end
-
- output_tokens = list(tokens)
-
- masked_lm_positions = []
- masked_lm_labels = []
-
- if masked_lm_prob == 0:
- return (output_tokens, masked_lm_positions,
- masked_lm_labels, token_boundary)
-
- num_to_predict = min(max_predictions_per_seq,
- max(1, int(round(len(tokens) * masked_lm_prob))))
-
- ngrams = np.arange(1, max_ngrams + 1, dtype=np.int64)
- if not geometric_dist:
- # Note(mingdachen):
- # By default, we set the probilities to favor shorter ngram sequences.
- pvals = 1. / np.arange(1, max_ngrams + 1)
- pvals /= pvals.sum(keepdims=True)
- if favor_longer_ngram:
- pvals = pvals[::-1]
- # 获取一个ngram的idx,对于每个word,记录他的ngram的word
- ngram_indexes = []
- for idx in range(len(cand_indexes)):
- ngram_index = []
- for n in ngrams:
- ngram_index.append(cand_indexes[idx:idx + n])
- ngram_indexes.append(ngram_index)
-
- np_rng.shuffle(ngram_indexes)
-
- (masked_lms, masked_spans) = ([], [])
- covered_indexes = set()
- for cand_index_set in ngram_indexes:
- if len(masked_lms) >= num_to_predict:
- break
- if not cand_index_set:
- continue
- # Note(mingdachen):
- # Skip current piece if they are covered in lm masking or previous ngrams.
- for index_set in cand_index_set[0]:
- for index in index_set:
- if index in covered_indexes:
- continue
-
- if not geometric_dist:
- n = np_rng.choice(ngrams[:len(cand_index_set)],
- p=pvals[:len(cand_index_set)] /
- pvals[:len(cand_index_set)].sum(keepdims=True))
- else:
- # Sampling "n" from the geometric distribution and clipping it to
- # the max_ngrams. Using p=0.2 default from the SpanBERT paper
- # https://arxiv.org/pdf/1907.10529.pdf (Sec 3.1)
- n = min(np_rng.geometric(0.2), max_ngrams)
-
- index_set = sum(cand_index_set[n - 1], [])
- n -= 1
- # Note(mingdachen):
- # Repeatedly looking for a candidate that does not exceed the
- # maximum number of predictions by trying shorter ngrams.
- while len(masked_lms) + len(index_set) > num_to_predict:
- if n == 0:
- break
- index_set = sum(cand_index_set[n - 1], [])
- n -= 1
- # If adding a whole-word mask would exceed the maximum number of
- # predictions, then just skip this candidate.
- if len(masked_lms) + len(index_set) > num_to_predict:
- continue
- is_any_index_covered = False
- for index in index_set:
- if index in covered_indexes:
- is_any_index_covered = True
- break
- if is_any_index_covered:
- continue
- for index in index_set:
- covered_indexes.add(index)
- masked_token = None
- token_id = tokens[index]
- if masking_style == "bert":
- # 80% of the time, replace with [MASK]
- if np_rng.random() < 0.8:
- masked_token = mask_id
- else:
- # 10% of the time, keep original
- if np_rng.random() < 0.5:
- masked_token = tokens[index]
- # 10% of the time, replace with random word
- else:
- masked_token = vocab_id_list[np_rng.randint(0, len(vocab_id_list))]
- elif masking_style == "t5":
- masked_token = mask_id
- else:
- raise ValueError("invalid value of masking style")
-
- output_tokens[index] = masked_token
- masked_lms.append(MaskedLmInstance(index=index, label=token_id))
-
- masked_spans.append(MaskedLmInstance(
- index=index_set,
- label=[tokens[index] for index in index_set]))
-
- assert len(masked_lms) <= num_to_predict
- np_rng.shuffle(ngram_indexes)
-
- select_indexes = set()
- if do_permutation:
- for cand_index_set in ngram_indexes:
- if len(select_indexes) >= num_to_predict:
- break
- if not cand_index_set:
- continue
- # Note(mingdachen):
- # Skip current piece if they are covered in lm masking or previous ngrams.
- for index_set in cand_index_set[0]:
- for index in index_set:
- if index in covered_indexes or index in select_indexes:
- continue
-
- n = np.random.choice(ngrams[:len(cand_index_set)],
- p=pvals[:len(cand_index_set)] /
- pvals[:len(cand_index_set)].sum(keepdims=True))
- index_set = sum(cand_index_set[n - 1], [])
- n -= 1
-
- while len(select_indexes) + len(index_set) > num_to_predict:
- if n == 0:
- break
- index_set = sum(cand_index_set[n - 1], [])
- n -= 1
- # If adding a whole-word mask would exceed the maximum number of
- # predictions, then just skip this candidate.
- if len(select_indexes) + len(index_set) > num_to_predict:
- continue
- is_any_index_covered = False
- for index in index_set:
- if index in covered_indexes or index in select_indexes:
- is_any_index_covered = True
- break
- if is_any_index_covered:
- continue
- for index in index_set:
- select_indexes.add(index)
- assert len(select_indexes) <= num_to_predict
-
- select_indexes = sorted(select_indexes)
- permute_indexes = list(select_indexes)
- np_rng.shuffle(permute_indexes)
- orig_token = list(output_tokens)
-
- for src_i, tgt_i in zip(select_indexes, permute_indexes):
- output_tokens[src_i] = orig_token[tgt_i]
- masked_lms.append(MaskedLmInstance(index=src_i, label=orig_token[src_i]))
-
- masked_lms = sorted(masked_lms, key=lambda x: x.index)
- # Sort the spans by the index of the first span
- masked_spans = sorted(masked_spans, key=lambda x: x.index[0])
-
- for p in masked_lms:
- masked_lm_positions.append(p.index)
- masked_lm_labels.append(p.label)
- return (output_tokens, masked_lm_positions, masked_lm_labels, token_boundary, masked_spans)
diff --git a/spaces/fclong/summary/fengshen/data/task_dataloader/task_datasets.py b/spaces/fclong/summary/fengshen/data/task_dataloader/task_datasets.py
deleted file mode 100644
index a8fe7bcf732c61725853df92d9422f207d55f785..0000000000000000000000000000000000000000
--- a/spaces/fclong/summary/fengshen/data/task_dataloader/task_datasets.py
+++ /dev/null
@@ -1,206 +0,0 @@
-# coding=utf8
-from torch.utils.data import Dataset, DataLoader
-from tqdm import tqdm
-from transformers import AutoTokenizer
-import json
-import torch
-import pytorch_lightning as pl
-import os
-
-
-class AbstractCollator:
- """
- collector for summary task
- """
-
- def __init__(self, tokenizer, max_enc_length, max_dec_length, prompt):
- self.tokenizer = tokenizer
- self.max_enc_length = max_enc_length
- self.max_dec_length = max_dec_length
- self.prompt = prompt
-
- def __call__(self, samples):
-
- labels = []
- attn_mask = []
- # decoder_attn_mask = []
- source_inputs = []
- for sample in samples:
- encode_dict = self.tokenizer.encode_plus(
- self.prompt + sample['text'],
- max_length=self.max_enc_length,
- padding='max_length',
- truncation=True,
- return_tensors='pt')
- decode_dict = self.tokenizer.encode_plus(
- sample['summary'],
- max_length=self.max_dec_length,
- padding='max_length',
- truncation=True,
- return_tensors='pt')
- source_inputs.append(encode_dict['input_ids'].squeeze())
- labels.append(decode_dict['input_ids'].squeeze())
- attn_mask.append(encode_dict['attention_mask'].squeeze())
- # decoder_attn_mask.append(decode_dict['attention_mask'].squeeze())
- # labels = torch.tensor(decode_dict['input'])
-
- source_inputs = torch.stack(source_inputs)
- labels = torch.stack(labels)
- attn_mask = torch.stack(attn_mask)
- # decoder_attn_mask = torch.stack(decoder_attn_mask)
- # decode_input_idxs = shift_tokens_right(labels, self.tokenizer.pad_token_id, self.tokenizer.pad_token_id)
- end_token_index = torch.where(labels == self.tokenizer.eos_token_id)[1]
- for idx, end_idx in enumerate(end_token_index):
- labels[idx][end_idx + 1:] = -100
-
- return {
- "input_ids": source_inputs,
- "attention_mask": attn_mask,
- "labels": labels,
- "text": [sample['text'] for sample in samples],
- "summary": [sample['summary'] for sample in samples]
- }
-
-
-class LCSTSDataset(Dataset):
- '''
- Dataset Used for LCSTS summary task.
- '''
-
- def __init__(self, data_path, args):
- super().__init__()
- self.tokenizer = AutoTokenizer.from_pretrained(
- args.pretrained_model_path, use_fast=False)
- self.data = self.load_data(data_path)
- self.prompt = args.prompt
- self.max_enc_length = args.max_enc_length
- self.max_dec_length = args.max_dec_length
-
- def __len__(self):
- return len(self.data)
-
- def __getitem__(self, index):
- return self.encode(self.data[index])
-
- def load_data(self, data_path):
- with open(data_path, "r", encoding='utf8') as f:
- lines = f.readlines()
- samples = []
- for line in tqdm(lines):
- obj = json.loads(line)
- source = obj['text']
- target = obj['summary']
- samples.append({
- "text": source,
- "summary": target
- })
- return samples
-
- def cal_data(self, data_path):
- with open(data_path, "r", encoding='utf8') as f:
- lines = f.readlines()
- samples = []
- enc_sizes = []
- dec_sizes = []
- for line in tqdm(lines):
- obj = json.loads(line.strip())
- source = obj['text']
- target = obj['summary']
- enc_input_ids = self.tokenizer.encode(source)
- target = self.tokenizer.encode(target)
- enc_sizes.append(len(enc_input_ids))
- dec_sizes.append(len(target)-1)
- samples.append({
- "enc_input_ids": enc_input_ids,
- "dec_input_ids": target[:-1],
- "label_ids": target[1:]
- })
- max_enc_len = max(enc_sizes)
- max_dec_len = max(dec_sizes)
- import numpy as np
- # mean of len(enc_input_ids): 74.68041911345998
- # mean of len(dec_input_ids): 14.02265483791283
- # max of len(enc_input_ids): 132
- # max of len(dec_input_ids): 31
- print('mean of len(enc_input_ids):', np.mean(enc_sizes),
- 'mean of len(dec_input_ids):', np.mean(dec_sizes),
- 'max of len(enc_input_ids):', max_enc_len,
- 'max of len(dec_input_ids):', max_dec_len)
- return samples
-
- def encode(self, item):
- encode_dict = self.tokenizer.encode_plus(
- self.prompt + item['text'],
- max_length=self.max_enc_length,
- padding='max_length',
- truncation=True,
- return_tensors='pt')
- decode_dict = self.tokenizer.encode_plus(
- item['summary'],
- max_length=self.max_dec_length,
- padding='max_length',
- truncation=True)
-
- target = decode_dict['input_ids']
- # print('encode_dict shape:', encode_dict['input_ids'].shape)
- labels = torch.tensor(target)
- labels[target == self.tokenizer.pad_token_id] = -100
- return {
- "input_ids": encode_dict['input_ids'].squeeze(),
- "attention_mask": encode_dict['attention_mask'].squeeze(),
- "labels": labels.squeeze(),
- "text": item['text'],
- "summary": item['summary']
- }
-
-
-class LCSTSDataModel(pl.LightningDataModule):
- @staticmethod
- def add_data_specific_args(parent_args):
- parser = parent_args.add_argument_group('LCSTSDataModel')
- parser.add_argument(
- '--data_dir', default='/cognitive_comp/ganruyi/data_datasets_LCSTS_LCSTS/', type=str)
- parser.add_argument('--num_workers', default=8, type=int)
- parser.add_argument('--train_data', default='train.jsonl', type=str)
- parser.add_argument('--valid_data', default='valid.jsonl', type=str)
- parser.add_argument('--test_data', default='test_public.jsonl', type=str)
- parser.add_argument('--train_batchsize', default=128, type=int)
- parser.add_argument('--valid_batchsize', default=128, type=int)
- parser.add_argument('--max_enc_length', default=128, type=int)
- parser.add_argument('--max_dec_length', default=30, type=int)
- parser.add_argument('--prompt', default='summarize:', type=str)
- return parent_args
-
- def __init__(self, args):
- super().__init__()
- self.args = args
- self.train_batchsize = args.train_batchsize
- self.valid_batchsize = args.valid_batchsize
- if not args.do_eval_only:
- self.train_data = LCSTSDataset(os.path.join(
- args.data_dir, args.train_data), args)
- self.valid_data = LCSTSDataset(os.path.join(
- args.data_dir, args.valid_data), args)
- self.test_data = LCSTSDataset(os.path.join(
- args.data_dir, args.test_data), args)
-
- def train_dataloader(self):
- return DataLoader(self.train_data,
- shuffle=True,
- batch_size=self.train_batchsize,
- pin_memory=False,
- num_workers=self.args.num_workers)
-
- def val_dataloader(self):
- return DataLoader(self.valid_data,
- shuffle=False,
- batch_size=self.valid_batchsize,
- pin_memory=False,
- num_workers=self.args.num_workers)
-
- def predict_dataloader(self):
- return DataLoader(self.test_data,
- shuffle=False,
- batch_size=self.valid_batchsize,
- pin_memory=False,
- num_workers=self.args.num_workers)
diff --git a/spaces/feng2022/styleganhuman_copy/torch_utils/ops/filtered_lrelu.h b/spaces/feng2022/styleganhuman_copy/torch_utils/ops/filtered_lrelu.h
deleted file mode 100644
index 524c804122a2582e20e2e4e9c49267e1a1b6db60..0000000000000000000000000000000000000000
--- a/spaces/feng2022/styleganhuman_copy/torch_utils/ops/filtered_lrelu.h
+++ /dev/null
@@ -1,90 +0,0 @@
-// Copyright (c) 2021, NVIDIA CORPORATION & AFFILIATES. All rights reserved.
-//
-// NVIDIA CORPORATION and its licensors retain all intellectual property
-// and proprietary rights in and to this software, related documentation
-// and any modifications thereto. Any use, reproduction, disclosure or
-// distribution of this software and related documentation without an express
-// license agreement from NVIDIA CORPORATION is strictly prohibited.
-
-#include
-
-//------------------------------------------------------------------------
-// CUDA kernel parameters.
-
-struct filtered_lrelu_kernel_params
-{
- // These parameters decide which kernel to use.
- int up; // upsampling ratio (1, 2, 4)
- int down; // downsampling ratio (1, 2, 4)
- int2 fuShape; // [size, 1] | [size, size]
- int2 fdShape; // [size, 1] | [size, size]
-
- int _dummy; // Alignment.
-
- // Rest of the parameters.
- const void* x; // Input tensor.
- void* y; // Output tensor.
- const void* b; // Bias tensor.
- unsigned char* s; // Sign tensor in/out. NULL if unused.
- const float* fu; // Upsampling filter.
- const float* fd; // Downsampling filter.
-
- int2 pad0; // Left/top padding.
- float gain; // Additional gain factor.
- float slope; // Leaky ReLU slope on negative side.
- float clamp; // Clamp after nonlinearity.
- int flip; // Filter kernel flip for gradient computation.
-
- int tilesXdim; // Original number of horizontal output tiles.
- int tilesXrep; // Number of horizontal tiles per CTA.
- int blockZofs; // Block z offset to support large minibatch, channel dimensions.
-
- int4 xShape; // [width, height, channel, batch]
- int4 yShape; // [width, height, channel, batch]
- int2 sShape; // [width, height] - width is in bytes. Contiguous. Zeros if unused.
- int2 sOfs; // [ofs_x, ofs_y] - offset between upsampled data and sign tensor.
- int swLimit; // Active width of sign tensor in bytes.
-
- longlong4 xStride; // Strides of all tensors except signs, same component order as shapes.
- longlong4 yStride; //
- int64_t bStride; //
- longlong3 fuStride; //
- longlong3 fdStride; //
-};
-
-struct filtered_lrelu_act_kernel_params
-{
- void* x; // Input/output, modified in-place.
- unsigned char* s; // Sign tensor in/out. NULL if unused.
-
- float gain; // Additional gain factor.
- float slope; // Leaky ReLU slope on negative side.
- float clamp; // Clamp after nonlinearity.
-
- int4 xShape; // [width, height, channel, batch]
- longlong4 xStride; // Input/output tensor strides, same order as in shape.
- int2 sShape; // [width, height] - width is in elements. Contiguous. Zeros if unused.
- int2 sOfs; // [ofs_x, ofs_y] - offset between upsampled data and sign tensor.
-};
-
-//------------------------------------------------------------------------
-// CUDA kernel specialization.
-
-struct filtered_lrelu_kernel_spec
-{
- void* setup; // Function for filter kernel setup.
- void* exec; // Function for main operation.
- int2 tileOut; // Width/height of launch tile.
- int numWarps; // Number of warps per thread block, determines launch block size.
- int xrep; // For processing multiple horizontal tiles per thread block.
- int dynamicSharedKB; // How much dynamic shared memory the exec kernel wants.
-};
-
-//------------------------------------------------------------------------
-// CUDA kernel selection.
-
-template filtered_lrelu_kernel_spec choose_filtered_lrelu_kernel(const filtered_lrelu_kernel_params& p, int sharedKB);
-template void* choose_filtered_lrelu_act_kernel(void);
-template cudaError_t copy_filters(cudaStream_t stream);
-
-//------------------------------------------------------------------------
\ No newline at end of file
diff --git a/spaces/fffiloni/Image-to-MusicGen/app.py b/spaces/fffiloni/Image-to-MusicGen/app.py
deleted file mode 100644
index 32212ca3379e99835937d4bda21c85714616ee36..0000000000000000000000000000000000000000
--- a/spaces/fffiloni/Image-to-MusicGen/app.py
+++ /dev/null
@@ -1,122 +0,0 @@
-"""
-Copyright (c) Meta Platforms, Inc. and affiliates.
-All rights reserved.
-
-This source code is licensed under the license found in the
-LICENSE file in the root directory of this source tree.
-"""
-
-from tempfile import NamedTemporaryFile
-import torch
-import gradio as gr
-from audiocraft.models import MusicGen
-
-from audiocraft.data.audio import audio_write
-
-
-MODEL = None
-
-img_to_text = gr.load(name="spaces/fffiloni/CLIP-Interrogator-2")
-
-
-def load_model(version):
- print("Loading model", version)
- return MusicGen.get_pretrained(version)
-
-
-def predict(uploaded_image, melody, duration):
- text = img_to_text(uploaded_image, 'best', 4, fn_index=1)[0]
- global MODEL
- topk = int(250)
- if MODEL is None or MODEL.name != "melody":
- MODEL = load_model("melody")
-
- if duration > MODEL.lm.cfg.dataset.segment_duration:
- raise gr.Error("MusicGen currently supports durations of up to 30 seconds!")
- MODEL.set_generation_params(
- use_sampling=True,
- top_k=250,
- top_p=0,
- temperature=1.0,
- cfg_coef=3.0,
- duration=duration,
- )
-
- if melody:
- sr, melody = melody[0], torch.from_numpy(melody[1]).to(MODEL.device).float().t().unsqueeze(0)
- print(melody.shape)
- if melody.dim() == 2:
- melody = melody[None]
- melody = melody[..., :int(sr * MODEL.lm.cfg.dataset.segment_duration)]
- output = MODEL.generate_with_chroma(
- descriptions=[text],
- melody_wavs=melody,
- melody_sample_rate=sr,
- progress=False
- )
- else:
- output = MODEL.generate(descriptions=[text], progress=False)
-
- output = output.detach().cpu().float()[0]
- with NamedTemporaryFile("wb", suffix=".wav", delete=False) as file:
- audio_write(file.name, output, MODEL.sample_rate, strategy="loudness", add_suffix=False)
- #waveform_video = gr.make_waveform(file.name)
- return file.name
-
-
-with gr.Blocks() as demo:
- gr.Markdown(
- """
- # Image to MusicGen
-
- This is the demo by @fffiloni for Image to [MusicGen](https://github.com/facebookresearch/audiocraft), a simple and controllable model for music generation
- presented at: ["Simple and Controllable Music Generation"](https://huggingface.co/papers/2306.05284), using Clip Interrogator to get an image description as init text.
-
-
-
- for longer sequences, more control and no queue.
- """
- )
- with gr.Row():
- with gr.Column():
- with gr.Column():
- uploaded_image = gr.Image(label="Input Image", interactive=True, source="upload", type="filepath")
- melody = gr.Audio(source="upload", type="numpy", label="Melody Condition (optional)", interactive=True)
- with gr.Row():
- submit = gr.Button("Submit")
- #with gr.Row():
- # model = gr.Radio(["melody", "medium", "small", "large"], label="Model", value="melody", interactive=True)
- with gr.Row():
- duration = gr.Slider(minimum=1, maximum=30, value=10, step=1, label="Duration", interactive=True)
- #with gr.Row():
- # topk = gr.Number(label="Top-k", value=250, interactive=True)
- # topp = gr.Number(label="Top-p", value=0, interactive=True)
- # temperature = gr.Number(label="Temperature", value=1.0, interactive=True)
- # cfg_coef = gr.Number(label="Classifier Free Guidance", value=3.0, interactive=True)
- with gr.Column():
- output = gr.Audio(label="Generated Music")
- submit.click(predict, inputs=[uploaded_image, melody, duration], outputs=[output])
-
- gr.Markdown(
- """
- ### More details
-
- The model will generate a short music extract based on the image you provided.
- You can generate up to 30 seconds of audio.
-
- This demo is set to use only the Melody model
- 1. Melody -- a music generation model capable of generating music condition on text and melody inputs. **Note**, you can also use text only.
- 2. Small -- a 300M transformer decoder conditioned on text only.
- 3. Medium -- a 1.5B transformer decoder conditioned on text only.
- 4. Large -- a 3.3B transformer decoder conditioned on text only (might OOM for the longest sequences.)
-
- When using `melody`, ou can optionaly provide a reference audio from
- which a broad melody will be extracted. The model will then try to follow both the description and melody provided.
-
- You can also use your own GPU or a Google Colab by following the instructions on our repo.
- See [github.com/facebookresearch/audiocraft](https://github.com/facebookresearch/audiocraft)
- for more details.
- """
- )
-
-demo.queue(max_size=32).launch()
diff --git a/spaces/fffiloni/Video-Matting-Anything/GroundingDINO/groundingdino/util/__init__.py b/spaces/fffiloni/Video-Matting-Anything/GroundingDINO/groundingdino/util/__init__.py
deleted file mode 100644
index 168f9979a4623806934b0ff1102ac166704e7dec..0000000000000000000000000000000000000000
--- a/spaces/fffiloni/Video-Matting-Anything/GroundingDINO/groundingdino/util/__init__.py
+++ /dev/null
@@ -1 +0,0 @@
-# Copyright (c) Facebook, Inc. and its affiliates. All Rights Reserved
diff --git a/spaces/fffiloni/controlnet-animation-doodle/node_modules/@types/node/ts4.8/crypto.d.ts b/spaces/fffiloni/controlnet-animation-doodle/node_modules/@types/node/ts4.8/crypto.d.ts
deleted file mode 100644
index 66dc8e54a2b3ae6119f7f6964eb295767cbc90bc..0000000000000000000000000000000000000000
--- a/spaces/fffiloni/controlnet-animation-doodle/node_modules/@types/node/ts4.8/crypto.d.ts
+++ /dev/null
@@ -1,3966 +0,0 @@
-/**
- * The `crypto` module provides cryptographic functionality that includes a set of
- * wrappers for OpenSSL's hash, HMAC, cipher, decipher, sign, and verify functions.
- *
- * ```js
- * const { createHmac } = await import('crypto');
- *
- * const secret = 'abcdefg';
- * const hash = createHmac('sha256', secret)
- * .update('I love cupcakes')
- * .digest('hex');
- * console.log(hash);
- * // Prints:
- * // c0fa1bc00531bd78ef38c628449c5102aeabd49b5dc3a2a516ea6ea959d6658e
- * ```
- * @see [source](https://github.com/nodejs/node/blob/v18.0.0/lib/crypto.js)
- */
-declare module 'crypto' {
- import * as stream from 'node:stream';
- import { PeerCertificate } from 'node:tls';
- /**
- * SPKAC is a Certificate Signing Request mechanism originally implemented by
- * Netscape and was specified formally as part of [HTML5's `keygen` element](https://developer.mozilla.org/en-US/docs/Web/HTML/Element/keygen).
- *
- * `` is deprecated since [HTML 5.2](https://www.w3.org/TR/html52/changes.html#features-removed) and new projects
- * should not use this element anymore.
- *
- * The `crypto` module provides the `Certificate` class for working with SPKAC
- * data. The most common usage is handling output generated by the HTML5`` element. Node.js uses [OpenSSL's SPKAC
- * implementation](https://www.openssl.org/docs/man1.1.0/apps/openssl-spkac.html) internally.
- * @since v0.11.8
- */
- class Certificate {
- /**
- * ```js
- * const { Certificate } = await import('crypto');
- * const spkac = getSpkacSomehow();
- * const challenge = Certificate.exportChallenge(spkac);
- * console.log(challenge.toString('utf8'));
- * // Prints: the challenge as a UTF8 string
- * ```
- * @since v9.0.0
- * @param encoding The `encoding` of the `spkac` string.
- * @return The challenge component of the `spkac` data structure, which includes a public key and a challenge.
- */
- static exportChallenge(spkac: BinaryLike): Buffer;
- /**
- * ```js
- * const { Certificate } = await import('crypto');
- * const spkac = getSpkacSomehow();
- * const publicKey = Certificate.exportPublicKey(spkac);
- * console.log(publicKey);
- * // Prints: the public key as
- * ```
- * @since v9.0.0
- * @param encoding The `encoding` of the `spkac` string.
- * @return The public key component of the `spkac` data structure, which includes a public key and a challenge.
- */
- static exportPublicKey(spkac: BinaryLike, encoding?: string): Buffer;
- /**
- * ```js
- * import { Buffer } from 'buffer';
- * const { Certificate } = await import('crypto');
- *
- * const spkac = getSpkacSomehow();
- * console.log(Certificate.verifySpkac(Buffer.from(spkac)));
- * // Prints: true or false
- * ```
- * @since v9.0.0
- * @param encoding The `encoding` of the `spkac` string.
- * @return `true` if the given `spkac` data structure is valid, `false` otherwise.
- */
- static verifySpkac(spkac: NodeJS.ArrayBufferView): boolean;
- /**
- * @deprecated
- * @param spkac
- * @returns The challenge component of the `spkac` data structure,
- * which includes a public key and a challenge.
- */
- exportChallenge(spkac: BinaryLike): Buffer;
- /**
- * @deprecated
- * @param spkac
- * @param encoding The encoding of the spkac string.
- * @returns The public key component of the `spkac` data structure,
- * which includes a public key and a challenge.
- */
- exportPublicKey(spkac: BinaryLike, encoding?: string): Buffer;
- /**
- * @deprecated
- * @param spkac
- * @returns `true` if the given `spkac` data structure is valid,
- * `false` otherwise.
- */
- verifySpkac(spkac: NodeJS.ArrayBufferView): boolean;
- }
- namespace constants {
- // https://nodejs.org/dist/latest-v10.x/docs/api/crypto.html#crypto_crypto_constants
- const OPENSSL_VERSION_NUMBER: number;
- /** Applies multiple bug workarounds within OpenSSL. See https://www.openssl.org/docs/man1.0.2/ssl/SSL_CTX_set_options.html for detail. */
- const SSL_OP_ALL: number;
- /** Allows legacy insecure renegotiation between OpenSSL and unpatched clients or servers. See https://www.openssl.org/docs/man1.0.2/ssl/SSL_CTX_set_options.html. */
- const SSL_OP_ALLOW_UNSAFE_LEGACY_RENEGOTIATION: number;
- /** Attempts to use the server's preferences instead of the client's when selecting a cipher. See https://www.openssl.org/docs/man1.0.2/ssl/SSL_CTX_set_options.html. */
- const SSL_OP_CIPHER_SERVER_PREFERENCE: number;
- /** Instructs OpenSSL to use Cisco's "speshul" version of DTLS_BAD_VER. */
- const SSL_OP_CISCO_ANYCONNECT: number;
- /** Instructs OpenSSL to turn on cookie exchange. */
- const SSL_OP_COOKIE_EXCHANGE: number;
- /** Instructs OpenSSL to add server-hello extension from an early version of the cryptopro draft. */
- const SSL_OP_CRYPTOPRO_TLSEXT_BUG: number;
- /** Instructs OpenSSL to disable a SSL 3.0/TLS 1.0 vulnerability workaround added in OpenSSL 0.9.6d. */
- const SSL_OP_DONT_INSERT_EMPTY_FRAGMENTS: number;
- /** Instructs OpenSSL to always use the tmp_rsa key when performing RSA operations. */
- const SSL_OP_EPHEMERAL_RSA: number;
- /** Allows initial connection to servers that do not support RI. */
- const SSL_OP_LEGACY_SERVER_CONNECT: number;
- const SSL_OP_MICROSOFT_BIG_SSLV3_BUFFER: number;
- const SSL_OP_MICROSOFT_SESS_ID_BUG: number;
- /** Instructs OpenSSL to disable the workaround for a man-in-the-middle protocol-version vulnerability in the SSL 2.0 server implementation. */
- const SSL_OP_MSIE_SSLV2_RSA_PADDING: number;
- const SSL_OP_NETSCAPE_CA_DN_BUG: number;
- const SSL_OP_NETSCAPE_CHALLENGE_BUG: number;
- const SSL_OP_NETSCAPE_DEMO_CIPHER_CHANGE_BUG: number;
- const SSL_OP_NETSCAPE_REUSE_CIPHER_CHANGE_BUG: number;
- /** Instructs OpenSSL to disable support for SSL/TLS compression. */
- const SSL_OP_NO_COMPRESSION: number;
- const SSL_OP_NO_QUERY_MTU: number;
- /** Instructs OpenSSL to always start a new session when performing renegotiation. */
- const SSL_OP_NO_SESSION_RESUMPTION_ON_RENEGOTIATION: number;
- const SSL_OP_NO_SSLv2: number;
- const SSL_OP_NO_SSLv3: number;
- const SSL_OP_NO_TICKET: number;
- const SSL_OP_NO_TLSv1: number;
- const SSL_OP_NO_TLSv1_1: number;
- const SSL_OP_NO_TLSv1_2: number;
- const SSL_OP_PKCS1_CHECK_1: number;
- const SSL_OP_PKCS1_CHECK_2: number;
- /** Instructs OpenSSL to always create a new key when using temporary/ephemeral DH parameters. */
- const SSL_OP_SINGLE_DH_USE: number;
- /** Instructs OpenSSL to always create a new key when using temporary/ephemeral ECDH parameters. */
- const SSL_OP_SINGLE_ECDH_USE: number;
- const SSL_OP_SSLEAY_080_CLIENT_DH_BUG: number;
- const SSL_OP_SSLREF2_REUSE_CERT_TYPE_BUG: number;
- const SSL_OP_TLS_BLOCK_PADDING_BUG: number;
- const SSL_OP_TLS_D5_BUG: number;
- /** Instructs OpenSSL to disable version rollback attack detection. */
- const SSL_OP_TLS_ROLLBACK_BUG: number;
- const ENGINE_METHOD_RSA: number;
- const ENGINE_METHOD_DSA: number;
- const ENGINE_METHOD_DH: number;
- const ENGINE_METHOD_RAND: number;
- const ENGINE_METHOD_EC: number;
- const ENGINE_METHOD_CIPHERS: number;
- const ENGINE_METHOD_DIGESTS: number;
- const ENGINE_METHOD_PKEY_METHS: number;
- const ENGINE_METHOD_PKEY_ASN1_METHS: number;
- const ENGINE_METHOD_ALL: number;
- const ENGINE_METHOD_NONE: number;
- const DH_CHECK_P_NOT_SAFE_PRIME: number;
- const DH_CHECK_P_NOT_PRIME: number;
- const DH_UNABLE_TO_CHECK_GENERATOR: number;
- const DH_NOT_SUITABLE_GENERATOR: number;
- const ALPN_ENABLED: number;
- const RSA_PKCS1_PADDING: number;
- const RSA_SSLV23_PADDING: number;
- const RSA_NO_PADDING: number;
- const RSA_PKCS1_OAEP_PADDING: number;
- const RSA_X931_PADDING: number;
- const RSA_PKCS1_PSS_PADDING: number;
- /** Sets the salt length for RSA_PKCS1_PSS_PADDING to the digest size when signing or verifying. */
- const RSA_PSS_SALTLEN_DIGEST: number;
- /** Sets the salt length for RSA_PKCS1_PSS_PADDING to the maximum permissible value when signing data. */
- const RSA_PSS_SALTLEN_MAX_SIGN: number;
- /** Causes the salt length for RSA_PKCS1_PSS_PADDING to be determined automatically when verifying a signature. */
- const RSA_PSS_SALTLEN_AUTO: number;
- const POINT_CONVERSION_COMPRESSED: number;
- const POINT_CONVERSION_UNCOMPRESSED: number;
- const POINT_CONVERSION_HYBRID: number;
- /** Specifies the built-in default cipher list used by Node.js (colon-separated values). */
- const defaultCoreCipherList: string;
- /** Specifies the active default cipher list used by the current Node.js process (colon-separated values). */
- const defaultCipherList: string;
- }
- interface HashOptions extends stream.TransformOptions {
- /**
- * For XOF hash functions such as `shake256`, the
- * outputLength option can be used to specify the desired output length in bytes.
- */
- outputLength?: number | undefined;
- }
- /** @deprecated since v10.0.0 */
- const fips: boolean;
- /**
- * Creates and returns a `Hash` object that can be used to generate hash digests
- * using the given `algorithm`. Optional `options` argument controls stream
- * behavior. For XOF hash functions such as `'shake256'`, the `outputLength` option
- * can be used to specify the desired output length in bytes.
- *
- * The `algorithm` is dependent on the available algorithms supported by the
- * version of OpenSSL on the platform. Examples are `'sha256'`, `'sha512'`, etc.
- * On recent releases of OpenSSL, `openssl list -digest-algorithms` will
- * display the available digest algorithms.
- *
- * Example: generating the sha256 sum of a file
- *
- * ```js
- * import {
- * createReadStream
- * } from 'fs';
- * import { argv } from 'process';
- * const {
- * createHash
- * } = await import('crypto');
- *
- * const filename = argv[2];
- *
- * const hash = createHash('sha256');
- *
- * const input = createReadStream(filename);
- * input.on('readable', () => {
- * // Only one element is going to be produced by the
- * // hash stream.
- * const data = input.read();
- * if (data)
- * hash.update(data);
- * else {
- * console.log(`${hash.digest('hex')} ${filename}`);
- * }
- * });
- * ```
- * @since v0.1.92
- * @param options `stream.transform` options
- */
- function createHash(algorithm: string, options?: HashOptions): Hash;
- /**
- * Creates and returns an `Hmac` object that uses the given `algorithm` and `key`.
- * Optional `options` argument controls stream behavior.
- *
- * The `algorithm` is dependent on the available algorithms supported by the
- * version of OpenSSL on the platform. Examples are `'sha256'`, `'sha512'`, etc.
- * On recent releases of OpenSSL, `openssl list -digest-algorithms` will
- * display the available digest algorithms.
- *
- * The `key` is the HMAC key used to generate the cryptographic HMAC hash. If it is
- * a `KeyObject`, its type must be `secret`.
- *
- * Example: generating the sha256 HMAC of a file
- *
- * ```js
- * import {
- * createReadStream
- * } from 'fs';
- * import { argv } from 'process';
- * const {
- * createHmac
- * } = await import('crypto');
- *
- * const filename = argv[2];
- *
- * const hmac = createHmac('sha256', 'a secret');
- *
- * const input = createReadStream(filename);
- * input.on('readable', () => {
- * // Only one element is going to be produced by the
- * // hash stream.
- * const data = input.read();
- * if (data)
- * hmac.update(data);
- * else {
- * console.log(`${hmac.digest('hex')} ${filename}`);
- * }
- * });
- * ```
- * @since v0.1.94
- * @param options `stream.transform` options
- */
- function createHmac(algorithm: string, key: BinaryLike | KeyObject, options?: stream.TransformOptions): Hmac;
- // https://nodejs.org/api/buffer.html#buffer_buffers_and_character_encodings
- type BinaryToTextEncoding = 'base64' | 'base64url' | 'hex' | 'binary';
- type CharacterEncoding = 'utf8' | 'utf-8' | 'utf16le' | 'latin1';
- type LegacyCharacterEncoding = 'ascii' | 'binary' | 'ucs2' | 'ucs-2';
- type Encoding = BinaryToTextEncoding | CharacterEncoding | LegacyCharacterEncoding;
- type ECDHKeyFormat = 'compressed' | 'uncompressed' | 'hybrid';
- /**
- * The `Hash` class is a utility for creating hash digests of data. It can be
- * used in one of two ways:
- *
- * * As a `stream` that is both readable and writable, where data is written
- * to produce a computed hash digest on the readable side, or
- * * Using the `hash.update()` and `hash.digest()` methods to produce the
- * computed hash.
- *
- * The {@link createHash} method is used to create `Hash` instances. `Hash`objects are not to be created directly using the `new` keyword.
- *
- * Example: Using `Hash` objects as streams:
- *
- * ```js
- * const {
- * createHash
- * } = await import('crypto');
- *
- * const hash = createHash('sha256');
- *
- * hash.on('readable', () => {
- * // Only one element is going to be produced by the
- * // hash stream.
- * const data = hash.read();
- * if (data) {
- * console.log(data.toString('hex'));
- * // Prints:
- * // 6a2da20943931e9834fc12cfe5bb47bbd9ae43489a30726962b576f4e3993e50
- * }
- * });
- *
- * hash.write('some data to hash');
- * hash.end();
- * ```
- *
- * Example: Using `Hash` and piped streams:
- *
- * ```js
- * import { createReadStream } from 'fs';
- * import { stdout } from 'process';
- * const { createHash } = await import('crypto');
- *
- * const hash = createHash('sha256');
- *
- * const input = createReadStream('test.js');
- * input.pipe(hash).setEncoding('hex').pipe(stdout);
- * ```
- *
- * Example: Using the `hash.update()` and `hash.digest()` methods:
- *
- * ```js
- * const {
- * createHash
- * } = await import('crypto');
- *
- * const hash = createHash('sha256');
- *
- * hash.update('some data to hash');
- * console.log(hash.digest('hex'));
- * // Prints:
- * // 6a2da20943931e9834fc12cfe5bb47bbd9ae43489a30726962b576f4e3993e50
- * ```
- * @since v0.1.92
- */
- class Hash extends stream.Transform {
- private constructor();
- /**
- * Creates a new `Hash` object that contains a deep copy of the internal state
- * of the current `Hash` object.
- *
- * The optional `options` argument controls stream behavior. For XOF hash
- * functions such as `'shake256'`, the `outputLength` option can be used to
- * specify the desired output length in bytes.
- *
- * An error is thrown when an attempt is made to copy the `Hash` object after
- * its `hash.digest()` method has been called.
- *
- * ```js
- * // Calculate a rolling hash.
- * const {
- * createHash
- * } = await import('crypto');
- *
- * const hash = createHash('sha256');
- *
- * hash.update('one');
- * console.log(hash.copy().digest('hex'));
- *
- * hash.update('two');
- * console.log(hash.copy().digest('hex'));
- *
- * hash.update('three');
- * console.log(hash.copy().digest('hex'));
- *
- * // Etc.
- * ```
- * @since v13.1.0
- * @param options `stream.transform` options
- */
- copy(options?: stream.TransformOptions): Hash;
- /**
- * Updates the hash content with the given `data`, the encoding of which
- * is given in `inputEncoding`.
- * If `encoding` is not provided, and the `data` is a string, an
- * encoding of `'utf8'` is enforced. If `data` is a `Buffer`, `TypedArray`, or`DataView`, then `inputEncoding` is ignored.
- *
- * This can be called many times with new data as it is streamed.
- * @since v0.1.92
- * @param inputEncoding The `encoding` of the `data` string.
- */
- update(data: BinaryLike): Hash;
- update(data: string, inputEncoding: Encoding): Hash;
- /**
- * Calculates the digest of all of the data passed to be hashed (using the `hash.update()` method).
- * If `encoding` is provided a string will be returned; otherwise
- * a `Buffer` is returned.
- *
- * The `Hash` object can not be used again after `hash.digest()` method has been
- * called. Multiple calls will cause an error to be thrown.
- * @since v0.1.92
- * @param encoding The `encoding` of the return value.
- */
- digest(): Buffer;
- digest(encoding: BinaryToTextEncoding): string;
- }
- /**
- * The `Hmac` class is a utility for creating cryptographic HMAC digests. It can
- * be used in one of two ways:
- *
- * * As a `stream` that is both readable and writable, where data is written
- * to produce a computed HMAC digest on the readable side, or
- * * Using the `hmac.update()` and `hmac.digest()` methods to produce the
- * computed HMAC digest.
- *
- * The {@link createHmac} method is used to create `Hmac` instances. `Hmac`objects are not to be created directly using the `new` keyword.
- *
- * Example: Using `Hmac` objects as streams:
- *
- * ```js
- * const {
- * createHmac
- * } = await import('crypto');
- *
- * const hmac = createHmac('sha256', 'a secret');
- *
- * hmac.on('readable', () => {
- * // Only one element is going to be produced by the
- * // hash stream.
- * const data = hmac.read();
- * if (data) {
- * console.log(data.toString('hex'));
- * // Prints:
- * // 7fd04df92f636fd450bc841c9418e5825c17f33ad9c87c518115a45971f7f77e
- * }
- * });
- *
- * hmac.write('some data to hash');
- * hmac.end();
- * ```
- *
- * Example: Using `Hmac` and piped streams:
- *
- * ```js
- * import { createReadStream } from 'fs';
- * import { stdout } from 'process';
- * const {
- * createHmac
- * } = await import('crypto');
- *
- * const hmac = createHmac('sha256', 'a secret');
- *
- * const input = createReadStream('test.js');
- * input.pipe(hmac).pipe(stdout);
- * ```
- *
- * Example: Using the `hmac.update()` and `hmac.digest()` methods:
- *
- * ```js
- * const {
- * createHmac
- * } = await import('crypto');
- *
- * const hmac = createHmac('sha256', 'a secret');
- *
- * hmac.update('some data to hash');
- * console.log(hmac.digest('hex'));
- * // Prints:
- * // 7fd04df92f636fd450bc841c9418e5825c17f33ad9c87c518115a45971f7f77e
- * ```
- * @since v0.1.94
- */
- class Hmac extends stream.Transform {
- private constructor();
- /**
- * Updates the `Hmac` content with the given `data`, the encoding of which
- * is given in `inputEncoding`.
- * If `encoding` is not provided, and the `data` is a string, an
- * encoding of `'utf8'` is enforced. If `data` is a `Buffer`, `TypedArray`, or`DataView`, then `inputEncoding` is ignored.
- *
- * This can be called many times with new data as it is streamed.
- * @since v0.1.94
- * @param inputEncoding The `encoding` of the `data` string.
- */
- update(data: BinaryLike): Hmac;
- update(data: string, inputEncoding: Encoding): Hmac;
- /**
- * Calculates the HMAC digest of all of the data passed using `hmac.update()`.
- * If `encoding` is
- * provided a string is returned; otherwise a `Buffer` is returned;
- *
- * The `Hmac` object can not be used again after `hmac.digest()` has been
- * called. Multiple calls to `hmac.digest()` will result in an error being thrown.
- * @since v0.1.94
- * @param encoding The `encoding` of the return value.
- */
- digest(): Buffer;
- digest(encoding: BinaryToTextEncoding): string;
- }
- type KeyObjectType = 'secret' | 'public' | 'private';
- interface KeyExportOptions {
- type: 'pkcs1' | 'spki' | 'pkcs8' | 'sec1';
- format: T;
- cipher?: string | undefined;
- passphrase?: string | Buffer | undefined;
- }
- interface JwkKeyExportOptions {
- format: 'jwk';
- }
- interface JsonWebKey {
- crv?: string | undefined;
- d?: string | undefined;
- dp?: string | undefined;
- dq?: string | undefined;
- e?: string | undefined;
- k?: string | undefined;
- kty?: string | undefined;
- n?: string | undefined;
- p?: string | undefined;
- q?: string | undefined;
- qi?: string | undefined;
- x?: string | undefined;
- y?: string | undefined;
- [key: string]: unknown;
- }
- interface AsymmetricKeyDetails {
- /**
- * Key size in bits (RSA, DSA).
- */
- modulusLength?: number | undefined;
- /**
- * Public exponent (RSA).
- */
- publicExponent?: bigint | undefined;
- /**
- * Name of the message digest (RSA-PSS).
- */
- hashAlgorithm?: string | undefined;
- /**
- * Name of the message digest used by MGF1 (RSA-PSS).
- */
- mgf1HashAlgorithm?: string | undefined;
- /**
- * Minimal salt length in bytes (RSA-PSS).
- */
- saltLength?: number | undefined;
- /**
- * Size of q in bits (DSA).
- */
- divisorLength?: number | undefined;
- /**
- * Name of the curve (EC).
- */
- namedCurve?: string | undefined;
- }
- /**
- * Node.js uses a `KeyObject` class to represent a symmetric or asymmetric key,
- * and each kind of key exposes different functions. The {@link createSecretKey}, {@link createPublicKey} and {@link createPrivateKey} methods are used to create `KeyObject`instances. `KeyObject`
- * objects are not to be created directly using the `new`keyword.
- *
- * Most applications should consider using the new `KeyObject` API instead of
- * passing keys as strings or `Buffer`s due to improved security features.
- *
- * `KeyObject` instances can be passed to other threads via `postMessage()`.
- * The receiver obtains a cloned `KeyObject`, and the `KeyObject` does not need to
- * be listed in the `transferList` argument.
- * @since v11.6.0
- */
- class KeyObject {
- private constructor();
- /**
- * Example: Converting a `CryptoKey` instance to a `KeyObject`:
- *
- * ```js
- * const { webcrypto, KeyObject } = await import('crypto');
- * const { subtle } = webcrypto;
- *
- * const key = await subtle.generateKey({
- * name: 'HMAC',
- * hash: 'SHA-256',
- * length: 256
- * }, true, ['sign', 'verify']);
- *
- * const keyObject = KeyObject.from(key);
- * console.log(keyObject.symmetricKeySize);
- * // Prints: 32 (symmetric key size in bytes)
- * ```
- * @since v15.0.0
- */
- static from(key: webcrypto.CryptoKey): KeyObject;
- /**
- * For asymmetric keys, this property represents the type of the key. Supported key
- * types are:
- *
- * * `'rsa'` (OID 1.2.840.113549.1.1.1)
- * * `'rsa-pss'` (OID 1.2.840.113549.1.1.10)
- * * `'dsa'` (OID 1.2.840.10040.4.1)
- * * `'ec'` (OID 1.2.840.10045.2.1)
- * * `'x25519'` (OID 1.3.101.110)
- * * `'x448'` (OID 1.3.101.111)
- * * `'ed25519'` (OID 1.3.101.112)
- * * `'ed448'` (OID 1.3.101.113)
- * * `'dh'` (OID 1.2.840.113549.1.3.1)
- *
- * This property is `undefined` for unrecognized `KeyObject` types and symmetric
- * keys.
- * @since v11.6.0
- */
- asymmetricKeyType?: KeyType | undefined;
- /**
- * For asymmetric keys, this property represents the size of the embedded key in
- * bytes. This property is `undefined` for symmetric keys.
- */
- asymmetricKeySize?: number | undefined;
- /**
- * This property exists only on asymmetric keys. Depending on the type of the key,
- * this object contains information about the key. None of the information obtained
- * through this property can be used to uniquely identify a key or to compromise
- * the security of the key.
- *
- * For RSA-PSS keys, if the key material contains a `RSASSA-PSS-params` sequence,
- * the `hashAlgorithm`, `mgf1HashAlgorithm`, and `saltLength` properties will be
- * set.
- *
- * Other key details might be exposed via this API using additional attributes.
- * @since v15.7.0
- */
- asymmetricKeyDetails?: AsymmetricKeyDetails | undefined;
- /**
- * For symmetric keys, the following encoding options can be used:
- *
- * For public keys, the following encoding options can be used:
- *
- * For private keys, the following encoding options can be used:
- *
- * The result type depends on the selected encoding format, when PEM the
- * result is a string, when DER it will be a buffer containing the data
- * encoded as DER, when [JWK](https://tools.ietf.org/html/rfc7517) it will be an object.
- *
- * When [JWK](https://tools.ietf.org/html/rfc7517) encoding format was selected, all other encoding options are
- * ignored.
- *
- * PKCS#1, SEC1, and PKCS#8 type keys can be encrypted by using a combination of
- * the `cipher` and `format` options. The PKCS#8 `type` can be used with any`format` to encrypt any key algorithm (RSA, EC, or DH) by specifying a`cipher`. PKCS#1 and SEC1 can only be
- * encrypted by specifying a `cipher`when the PEM `format` is used. For maximum compatibility, use PKCS#8 for
- * encrypted private keys. Since PKCS#8 defines its own
- * encryption mechanism, PEM-level encryption is not supported when encrypting
- * a PKCS#8 key. See [RFC 5208](https://www.rfc-editor.org/rfc/rfc5208.txt) for PKCS#8 encryption and [RFC 1421](https://www.rfc-editor.org/rfc/rfc1421.txt) for
- * PKCS#1 and SEC1 encryption.
- * @since v11.6.0
- */
- export(options: KeyExportOptions<'pem'>): string | Buffer;
- export(options?: KeyExportOptions<'der'>): Buffer;
- export(options?: JwkKeyExportOptions): JsonWebKey;
- /**
- * For secret keys, this property represents the size of the key in bytes. This
- * property is `undefined` for asymmetric keys.
- * @since v11.6.0
- */
- symmetricKeySize?: number | undefined;
- /**
- * Depending on the type of this `KeyObject`, this property is either`'secret'` for secret (symmetric) keys, `'public'` for public (asymmetric) keys
- * or `'private'` for private (asymmetric) keys.
- * @since v11.6.0
- */
- type: KeyObjectType;
- }
- type CipherCCMTypes = 'aes-128-ccm' | 'aes-192-ccm' | 'aes-256-ccm' | 'chacha20-poly1305';
- type CipherGCMTypes = 'aes-128-gcm' | 'aes-192-gcm' | 'aes-256-gcm';
- type CipherOCBTypes = 'aes-128-ocb' | 'aes-192-ocb' | 'aes-256-ocb';
- type BinaryLike = string | NodeJS.ArrayBufferView;
- type CipherKey = BinaryLike | KeyObject;
- interface CipherCCMOptions extends stream.TransformOptions {
- authTagLength: number;
- }
- interface CipherGCMOptions extends stream.TransformOptions {
- authTagLength?: number | undefined;
- }
- interface CipherOCBOptions extends stream.TransformOptions {
- authTagLength: number;
- }
- /**
- * Creates and returns a `Cipher` object that uses the given `algorithm` and`password`.
- *
- * The `options` argument controls stream behavior and is optional except when a
- * cipher in CCM or OCB mode (e.g. `'aes-128-ccm'`) is used. In that case, the`authTagLength` option is required and specifies the length of the
- * authentication tag in bytes, see `CCM mode`. In GCM mode, the `authTagLength`option is not required but can be used to set the length of the authentication
- * tag that will be returned by `getAuthTag()` and defaults to 16 bytes.
- * For `chacha20-poly1305`, the `authTagLength` option defaults to 16 bytes.
- *
- * The `algorithm` is dependent on OpenSSL, examples are `'aes192'`, etc. On
- * recent OpenSSL releases, `openssl list -cipher-algorithms` will
- * display the available cipher algorithms.
- *
- * The `password` is used to derive the cipher key and initialization vector (IV).
- * The value must be either a `'latin1'` encoded string, a `Buffer`, a`TypedArray`, or a `DataView`.
- *
- * The implementation of `crypto.createCipher()` derives keys using the OpenSSL
- * function [`EVP_BytesToKey`](https://www.openssl.org/docs/man1.1.0/crypto/EVP_BytesToKey.html) with the digest algorithm set to MD5, one
- * iteration, and no salt. The lack of salt allows dictionary attacks as the same
- * password always creates the same key. The low iteration count and
- * non-cryptographically secure hash algorithm allow passwords to be tested very
- * rapidly.
- *
- * In line with OpenSSL's recommendation to use a more modern algorithm instead of [`EVP_BytesToKey`](https://www.openssl.org/docs/man1.1.0/crypto/EVP_BytesToKey.html) it is recommended that
- * developers derive a key and IV on
- * their own using {@link scrypt} and to use {@link createCipheriv} to create the `Cipher` object. Users should not use ciphers with counter mode
- * (e.g. CTR, GCM, or CCM) in `crypto.createCipher()`. A warning is emitted when
- * they are used in order to avoid the risk of IV reuse that causes
- * vulnerabilities. For the case when IV is reused in GCM, see [Nonce-Disrespecting Adversaries](https://github.com/nonce-disrespect/nonce-disrespect) for details.
- * @since v0.1.94
- * @deprecated Since v10.0.0 - Use {@link createCipheriv} instead.
- * @param options `stream.transform` options
- */
- function createCipher(algorithm: CipherCCMTypes, password: BinaryLike, options: CipherCCMOptions): CipherCCM;
- /** @deprecated since v10.0.0 use `createCipheriv()` */
- function createCipher(algorithm: CipherGCMTypes, password: BinaryLike, options?: CipherGCMOptions): CipherGCM;
- /** @deprecated since v10.0.0 use `createCipheriv()` */
- function createCipher(algorithm: string, password: BinaryLike, options?: stream.TransformOptions): Cipher;
- /**
- * Creates and returns a `Cipher` object, with the given `algorithm`, `key` and
- * initialization vector (`iv`).
- *
- * The `options` argument controls stream behavior and is optional except when a
- * cipher in CCM or OCB mode (e.g. `'aes-128-ccm'`) is used. In that case, the`authTagLength` option is required and specifies the length of the
- * authentication tag in bytes, see `CCM mode`. In GCM mode, the `authTagLength`option is not required but can be used to set the length of the authentication
- * tag that will be returned by `getAuthTag()` and defaults to 16 bytes.
- * For `chacha20-poly1305`, the `authTagLength` option defaults to 16 bytes.
- *
- * The `algorithm` is dependent on OpenSSL, examples are `'aes192'`, etc. On
- * recent OpenSSL releases, `openssl list -cipher-algorithms` will
- * display the available cipher algorithms.
- *
- * The `key` is the raw key used by the `algorithm` and `iv` is an [initialization vector](https://en.wikipedia.org/wiki/Initialization_vector). Both arguments must be `'utf8'` encoded
- * strings,`Buffers`, `TypedArray`, or `DataView`s. The `key` may optionally be
- * a `KeyObject` of type `secret`. If the cipher does not need
- * an initialization vector, `iv` may be `null`.
- *
- * When passing strings for `key` or `iv`, please consider `caveats when using strings as inputs to cryptographic APIs`.
- *
- * Initialization vectors should be unpredictable and unique; ideally, they will be
- * cryptographically random. They do not have to be secret: IVs are typically just
- * added to ciphertext messages unencrypted. It may sound contradictory that
- * something has to be unpredictable and unique, but does not have to be secret;
- * remember that an attacker must not be able to predict ahead of time what a
- * given IV will be.
- * @since v0.1.94
- * @param options `stream.transform` options
- */
- function createCipheriv(algorithm: CipherCCMTypes, key: CipherKey, iv: BinaryLike, options: CipherCCMOptions): CipherCCM;
- function createCipheriv(algorithm: CipherOCBTypes, key: CipherKey, iv: BinaryLike, options: CipherOCBOptions): CipherOCB;
- function createCipheriv(algorithm: CipherGCMTypes, key: CipherKey, iv: BinaryLike, options?: CipherGCMOptions): CipherGCM;
- function createCipheriv(algorithm: string, key: CipherKey, iv: BinaryLike | null, options?: stream.TransformOptions): Cipher;
- /**
- * Instances of the `Cipher` class are used to encrypt data. The class can be
- * used in one of two ways:
- *
- * * As a `stream` that is both readable and writable, where plain unencrypted
- * data is written to produce encrypted data on the readable side, or
- * * Using the `cipher.update()` and `cipher.final()` methods to produce
- * the encrypted data.
- *
- * The {@link createCipher} or {@link createCipheriv} methods are
- * used to create `Cipher` instances. `Cipher` objects are not to be created
- * directly using the `new` keyword.
- *
- * Example: Using `Cipher` objects as streams:
- *
- * ```js
- * const {
- * scrypt,
- * randomFill,
- * createCipheriv
- * } = await import('crypto');
- *
- * const algorithm = 'aes-192-cbc';
- * const password = 'Password used to generate key';
- *
- * // First, we'll generate the key. The key length is dependent on the algorithm.
- * // In this case for aes192, it is 24 bytes (192 bits).
- * scrypt(password, 'salt', 24, (err, key) => {
- * if (err) throw err;
- * // Then, we'll generate a random initialization vector
- * randomFill(new Uint8Array(16), (err, iv) => {
- * if (err) throw err;
- *
- * // Once we have the key and iv, we can create and use the cipher...
- * const cipher = createCipheriv(algorithm, key, iv);
- *
- * let encrypted = '';
- * cipher.setEncoding('hex');
- *
- * cipher.on('data', (chunk) => encrypted += chunk);
- * cipher.on('end', () => console.log(encrypted));
- *
- * cipher.write('some clear text data');
- * cipher.end();
- * });
- * });
- * ```
- *
- * Example: Using `Cipher` and piped streams:
- *
- * ```js
- * import {
- * createReadStream,
- * createWriteStream,
- * } from 'fs';
- *
- * import {
- * pipeline
- * } from 'stream';
- *
- * const {
- * scrypt,
- * randomFill,
- * createCipheriv
- * } = await import('crypto');
- *
- * const algorithm = 'aes-192-cbc';
- * const password = 'Password used to generate key';
- *
- * // First, we'll generate the key. The key length is dependent on the algorithm.
- * // In this case for aes192, it is 24 bytes (192 bits).
- * scrypt(password, 'salt', 24, (err, key) => {
- * if (err) throw err;
- * // Then, we'll generate a random initialization vector
- * randomFill(new Uint8Array(16), (err, iv) => {
- * if (err) throw err;
- *
- * const cipher = createCipheriv(algorithm, key, iv);
- *
- * const input = createReadStream('test.js');
- * const output = createWriteStream('test.enc');
- *
- * pipeline(input, cipher, output, (err) => {
- * if (err) throw err;
- * });
- * });
- * });
- * ```
- *
- * Example: Using the `cipher.update()` and `cipher.final()` methods:
- *
- * ```js
- * const {
- * scrypt,
- * randomFill,
- * createCipheriv
- * } = await import('crypto');
- *
- * const algorithm = 'aes-192-cbc';
- * const password = 'Password used to generate key';
- *
- * // First, we'll generate the key. The key length is dependent on the algorithm.
- * // In this case for aes192, it is 24 bytes (192 bits).
- * scrypt(password, 'salt', 24, (err, key) => {
- * if (err) throw err;
- * // Then, we'll generate a random initialization vector
- * randomFill(new Uint8Array(16), (err, iv) => {
- * if (err) throw err;
- *
- * const cipher = createCipheriv(algorithm, key, iv);
- *
- * let encrypted = cipher.update('some clear text data', 'utf8', 'hex');
- * encrypted += cipher.final('hex');
- * console.log(encrypted);
- * });
- * });
- * ```
- * @since v0.1.94
- */
- class Cipher extends stream.Transform {
- private constructor();
- /**
- * Updates the cipher with `data`. If the `inputEncoding` argument is given,
- * the `data`argument is a string using the specified encoding. If the `inputEncoding`argument is not given, `data` must be a `Buffer`, `TypedArray`, or`DataView`. If `data` is a `Buffer`,
- * `TypedArray`, or `DataView`, then`inputEncoding` is ignored.
- *
- * The `outputEncoding` specifies the output format of the enciphered
- * data. If the `outputEncoding`is specified, a string using the specified encoding is returned. If no`outputEncoding` is provided, a `Buffer` is returned.
- *
- * The `cipher.update()` method can be called multiple times with new data until `cipher.final()` is called. Calling `cipher.update()` after `cipher.final()` will result in an error being
- * thrown.
- * @since v0.1.94
- * @param inputEncoding The `encoding` of the data.
- * @param outputEncoding The `encoding` of the return value.
- */
- update(data: BinaryLike): Buffer;
- update(data: string, inputEncoding: Encoding): Buffer;
- update(data: NodeJS.ArrayBufferView, inputEncoding: undefined, outputEncoding: Encoding): string;
- update(data: string, inputEncoding: Encoding | undefined, outputEncoding: Encoding): string;
- /**
- * Once the `cipher.final()` method has been called, the `Cipher` object can no
- * longer be used to encrypt data. Attempts to call `cipher.final()` more than
- * once will result in an error being thrown.
- * @since v0.1.94
- * @param outputEncoding The `encoding` of the return value.
- * @return Any remaining enciphered contents. If `outputEncoding` is specified, a string is returned. If an `outputEncoding` is not provided, a {@link Buffer} is returned.
- */
- final(): Buffer;
- final(outputEncoding: BufferEncoding): string;
- /**
- * When using block encryption algorithms, the `Cipher` class will automatically
- * add padding to the input data to the appropriate block size. To disable the
- * default padding call `cipher.setAutoPadding(false)`.
- *
- * When `autoPadding` is `false`, the length of the entire input data must be a
- * multiple of the cipher's block size or `cipher.final()` will throw an error.
- * Disabling automatic padding is useful for non-standard padding, for instance
- * using `0x0` instead of PKCS padding.
- *
- * The `cipher.setAutoPadding()` method must be called before `cipher.final()`.
- * @since v0.7.1
- * @param [autoPadding=true]
- * @return for method chaining.
- */
- setAutoPadding(autoPadding?: boolean): this;
- }
- interface CipherCCM extends Cipher {
- setAAD(
- buffer: NodeJS.ArrayBufferView,
- options: {
- plaintextLength: number;
- }
- ): this;
- getAuthTag(): Buffer;
- }
- interface CipherGCM extends Cipher {
- setAAD(
- buffer: NodeJS.ArrayBufferView,
- options?: {
- plaintextLength: number;
- }
- ): this;
- getAuthTag(): Buffer;
- }
- interface CipherOCB extends Cipher {
- setAAD(
- buffer: NodeJS.ArrayBufferView,
- options?: {
- plaintextLength: number;
- }
- ): this;
- getAuthTag(): Buffer;
- }
- /**
- * Creates and returns a `Decipher` object that uses the given `algorithm` and`password` (key).
- *
- * The `options` argument controls stream behavior and is optional except when a
- * cipher in CCM or OCB mode (e.g. `'aes-128-ccm'`) is used. In that case, the`authTagLength` option is required and specifies the length of the
- * authentication tag in bytes, see `CCM mode`.
- * For `chacha20-poly1305`, the `authTagLength` option defaults to 16 bytes.
- *
- * The implementation of `crypto.createDecipher()` derives keys using the OpenSSL
- * function [`EVP_BytesToKey`](https://www.openssl.org/docs/man1.1.0/crypto/EVP_BytesToKey.html) with the digest algorithm set to MD5, one
- * iteration, and no salt. The lack of salt allows dictionary attacks as the same
- * password always creates the same key. The low iteration count and
- * non-cryptographically secure hash algorithm allow passwords to be tested very
- * rapidly.
- *
- * In line with OpenSSL's recommendation to use a more modern algorithm instead of [`EVP_BytesToKey`](https://www.openssl.org/docs/man1.1.0/crypto/EVP_BytesToKey.html) it is recommended that
- * developers derive a key and IV on
- * their own using {@link scrypt} and to use {@link createDecipheriv} to create the `Decipher` object.
- * @since v0.1.94
- * @deprecated Since v10.0.0 - Use {@link createDecipheriv} instead.
- * @param options `stream.transform` options
- */
- function createDecipher(algorithm: CipherCCMTypes, password: BinaryLike, options: CipherCCMOptions): DecipherCCM;
- /** @deprecated since v10.0.0 use `createDecipheriv()` */
- function createDecipher(algorithm: CipherGCMTypes, password: BinaryLike, options?: CipherGCMOptions): DecipherGCM;
- /** @deprecated since v10.0.0 use `createDecipheriv()` */
- function createDecipher(algorithm: string, password: BinaryLike, options?: stream.TransformOptions): Decipher;
- /**
- * Creates and returns a `Decipher` object that uses the given `algorithm`, `key`and initialization vector (`iv`).
- *
- * The `options` argument controls stream behavior and is optional except when a
- * cipher in CCM or OCB mode (e.g. `'aes-128-ccm'`) is used. In that case, the`authTagLength` option is required and specifies the length of the
- * authentication tag in bytes, see `CCM mode`. In GCM mode, the `authTagLength`option is not required but can be used to restrict accepted authentication tags
- * to those with the specified length.
- * For `chacha20-poly1305`, the `authTagLength` option defaults to 16 bytes.
- *
- * The `algorithm` is dependent on OpenSSL, examples are `'aes192'`, etc. On
- * recent OpenSSL releases, `openssl list -cipher-algorithms` will
- * display the available cipher algorithms.
- *
- * The `key` is the raw key used by the `algorithm` and `iv` is an [initialization vector](https://en.wikipedia.org/wiki/Initialization_vector). Both arguments must be `'utf8'` encoded
- * strings,`Buffers`, `TypedArray`, or `DataView`s. The `key` may optionally be
- * a `KeyObject` of type `secret`. If the cipher does not need
- * an initialization vector, `iv` may be `null`.
- *
- * When passing strings for `key` or `iv`, please consider `caveats when using strings as inputs to cryptographic APIs`.
- *
- * Initialization vectors should be unpredictable and unique; ideally, they will be
- * cryptographically random. They do not have to be secret: IVs are typically just
- * added to ciphertext messages unencrypted. It may sound contradictory that
- * something has to be unpredictable and unique, but does not have to be secret;
- * remember that an attacker must not be able to predict ahead of time what a given
- * IV will be.
- * @since v0.1.94
- * @param options `stream.transform` options
- */
- function createDecipheriv(algorithm: CipherCCMTypes, key: CipherKey, iv: BinaryLike, options: CipherCCMOptions): DecipherCCM;
- function createDecipheriv(algorithm: CipherOCBTypes, key: CipherKey, iv: BinaryLike, options: CipherOCBOptions): DecipherOCB;
- function createDecipheriv(algorithm: CipherGCMTypes, key: CipherKey, iv: BinaryLike, options?: CipherGCMOptions): DecipherGCM;
- function createDecipheriv(algorithm: string, key: CipherKey, iv: BinaryLike | null, options?: stream.TransformOptions): Decipher;
- /**
- * Instances of the `Decipher` class are used to decrypt data. The class can be
- * used in one of two ways:
- *
- * * As a `stream` that is both readable and writable, where plain encrypted
- * data is written to produce unencrypted data on the readable side, or
- * * Using the `decipher.update()` and `decipher.final()` methods to
- * produce the unencrypted data.
- *
- * The {@link createDecipher} or {@link createDecipheriv} methods are
- * used to create `Decipher` instances. `Decipher` objects are not to be created
- * directly using the `new` keyword.
- *
- * Example: Using `Decipher` objects as streams:
- *
- * ```js
- * import { Buffer } from 'buffer';
- * const {
- * scryptSync,
- * createDecipheriv
- * } = await import('crypto');
- *
- * const algorithm = 'aes-192-cbc';
- * const password = 'Password used to generate key';
- * // Key length is dependent on the algorithm. In this case for aes192, it is
- * // 24 bytes (192 bits).
- * // Use the async `crypto.scrypt()` instead.
- * const key = scryptSync(password, 'salt', 24);
- * // The IV is usually passed along with the ciphertext.
- * const iv = Buffer.alloc(16, 0); // Initialization vector.
- *
- * const decipher = createDecipheriv(algorithm, key, iv);
- *
- * let decrypted = '';
- * decipher.on('readable', () => {
- * while (null !== (chunk = decipher.read())) {
- * decrypted += chunk.toString('utf8');
- * }
- * });
- * decipher.on('end', () => {
- * console.log(decrypted);
- * // Prints: some clear text data
- * });
- *
- * // Encrypted with same algorithm, key and iv.
- * const encrypted =
- * 'e5f79c5915c02171eec6b212d5520d44480993d7d622a7c4c2da32f6efda0ffa';
- * decipher.write(encrypted, 'hex');
- * decipher.end();
- * ```
- *
- * Example: Using `Decipher` and piped streams:
- *
- * ```js
- * import {
- * createReadStream,
- * createWriteStream,
- * } from 'fs';
- * import { Buffer } from 'buffer';
- * const {
- * scryptSync,
- * createDecipheriv
- * } = await import('crypto');
- *
- * const algorithm = 'aes-192-cbc';
- * const password = 'Password used to generate key';
- * // Use the async `crypto.scrypt()` instead.
- * const key = scryptSync(password, 'salt', 24);
- * // The IV is usually passed along with the ciphertext.
- * const iv = Buffer.alloc(16, 0); // Initialization vector.
- *
- * const decipher = createDecipheriv(algorithm, key, iv);
- *
- * const input = createReadStream('test.enc');
- * const output = createWriteStream('test.js');
- *
- * input.pipe(decipher).pipe(output);
- * ```
- *
- * Example: Using the `decipher.update()` and `decipher.final()` methods:
- *
- * ```js
- * import { Buffer } from 'buffer';
- * const {
- * scryptSync,
- * createDecipheriv
- * } = await import('crypto');
- *
- * const algorithm = 'aes-192-cbc';
- * const password = 'Password used to generate key';
- * // Use the async `crypto.scrypt()` instead.
- * const key = scryptSync(password, 'salt', 24);
- * // The IV is usually passed along with the ciphertext.
- * const iv = Buffer.alloc(16, 0); // Initialization vector.
- *
- * const decipher = createDecipheriv(algorithm, key, iv);
- *
- * // Encrypted using same algorithm, key and iv.
- * const encrypted =
- * 'e5f79c5915c02171eec6b212d5520d44480993d7d622a7c4c2da32f6efda0ffa';
- * let decrypted = decipher.update(encrypted, 'hex', 'utf8');
- * decrypted += decipher.final('utf8');
- * console.log(decrypted);
- * // Prints: some clear text data
- * ```
- * @since v0.1.94
- */
- class Decipher extends stream.Transform {
- private constructor();
- /**
- * Updates the decipher with `data`. If the `inputEncoding` argument is given,
- * the `data`argument is a string using the specified encoding. If the `inputEncoding`argument is not given, `data` must be a `Buffer`. If `data` is a `Buffer` then `inputEncoding` is
- * ignored.
- *
- * The `outputEncoding` specifies the output format of the enciphered
- * data. If the `outputEncoding`is specified, a string using the specified encoding is returned. If no`outputEncoding` is provided, a `Buffer` is returned.
- *
- * The `decipher.update()` method can be called multiple times with new data until `decipher.final()` is called. Calling `decipher.update()` after `decipher.final()` will result in an error
- * being thrown.
- * @since v0.1.94
- * @param inputEncoding The `encoding` of the `data` string.
- * @param outputEncoding The `encoding` of the return value.
- */
- update(data: NodeJS.ArrayBufferView): Buffer;
- update(data: string, inputEncoding: Encoding): Buffer;
- update(data: NodeJS.ArrayBufferView, inputEncoding: undefined, outputEncoding: Encoding): string;
- update(data: string, inputEncoding: Encoding | undefined, outputEncoding: Encoding): string;
- /**
- * Once the `decipher.final()` method has been called, the `Decipher` object can
- * no longer be used to decrypt data. Attempts to call `decipher.final()` more
- * than once will result in an error being thrown.
- * @since v0.1.94
- * @param outputEncoding The `encoding` of the return value.
- * @return Any remaining deciphered contents. If `outputEncoding` is specified, a string is returned. If an `outputEncoding` is not provided, a {@link Buffer} is returned.
- */
- final(): Buffer;
- final(outputEncoding: BufferEncoding): string;
- /**
- * When data has been encrypted without standard block padding, calling`decipher.setAutoPadding(false)` will disable automatic padding to prevent `decipher.final()` from checking for and
- * removing padding.
- *
- * Turning auto padding off will only work if the input data's length is a
- * multiple of the ciphers block size.
- *
- * The `decipher.setAutoPadding()` method must be called before `decipher.final()`.
- * @since v0.7.1
- * @param [autoPadding=true]
- * @return for method chaining.
- */
- setAutoPadding(auto_padding?: boolean): this;
- }
- interface DecipherCCM extends Decipher {
- setAuthTag(buffer: NodeJS.ArrayBufferView): this;
- setAAD(
- buffer: NodeJS.ArrayBufferView,
- options: {
- plaintextLength: number;
- }
- ): this;
- }
- interface DecipherGCM extends Decipher {
- setAuthTag(buffer: NodeJS.ArrayBufferView): this;
- setAAD(
- buffer: NodeJS.ArrayBufferView,
- options?: {
- plaintextLength: number;
- }
- ): this;
- }
- interface DecipherOCB extends Decipher {
- setAuthTag(buffer: NodeJS.ArrayBufferView): this;
- setAAD(
- buffer: NodeJS.ArrayBufferView,
- options?: {
- plaintextLength: number;
- }
- ): this;
- }
- interface PrivateKeyInput {
- key: string | Buffer;
- format?: KeyFormat | undefined;
- type?: 'pkcs1' | 'pkcs8' | 'sec1' | undefined;
- passphrase?: string | Buffer | undefined;
- encoding?: string | undefined;
- }
- interface PublicKeyInput {
- key: string | Buffer;
- format?: KeyFormat | undefined;
- type?: 'pkcs1' | 'spki' | undefined;
- encoding?: string | undefined;
- }
- /**
- * Asynchronously generates a new random secret key of the given `length`. The`type` will determine which validations will be performed on the `length`.
- *
- * ```js
- * const {
- * generateKey
- * } = await import('crypto');
- *
- * generateKey('hmac', { length: 64 }, (err, key) => {
- * if (err) throw err;
- * console.log(key.export().toString('hex')); // 46e..........620
- * });
- * ```
- * @since v15.0.0
- * @param type The intended use of the generated secret key. Currently accepted values are `'hmac'` and `'aes'`.
- */
- function generateKey(
- type: 'hmac' | 'aes',
- options: {
- length: number;
- },
- callback: (err: Error | null, key: KeyObject) => void
- ): void;
- /**
- * Synchronously generates a new random secret key of the given `length`. The`type` will determine which validations will be performed on the `length`.
- *
- * ```js
- * const {
- * generateKeySync
- * } = await import('crypto');
- *
- * const key = generateKeySync('hmac', { length: 64 });
- * console.log(key.export().toString('hex')); // e89..........41e
- * ```
- * @since v15.0.0
- * @param type The intended use of the generated secret key. Currently accepted values are `'hmac'` and `'aes'`.
- */
- function generateKeySync(
- type: 'hmac' | 'aes',
- options: {
- length: number;
- }
- ): KeyObject;
- interface JsonWebKeyInput {
- key: JsonWebKey;
- format: 'jwk';
- }
- /**
- * Creates and returns a new key object containing a private key. If `key` is a
- * string or `Buffer`, `format` is assumed to be `'pem'`; otherwise, `key`must be an object with the properties described above.
- *
- * If the private key is encrypted, a `passphrase` must be specified. The length
- * of the passphrase is limited to 1024 bytes.
- * @since v11.6.0
- */
- function createPrivateKey(key: PrivateKeyInput | string | Buffer | JsonWebKeyInput): KeyObject;
- /**
- * Creates and returns a new key object containing a public key. If `key` is a
- * string or `Buffer`, `format` is assumed to be `'pem'`; if `key` is a `KeyObject`with type `'private'`, the public key is derived from the given private key;
- * otherwise, `key` must be an object with the properties described above.
- *
- * If the format is `'pem'`, the `'key'` may also be an X.509 certificate.
- *
- * Because public keys can be derived from private keys, a private key may be
- * passed instead of a public key. In that case, this function behaves as if {@link createPrivateKey} had been called, except that the type of the
- * returned `KeyObject` will be `'public'` and that the private key cannot be
- * extracted from the returned `KeyObject`. Similarly, if a `KeyObject` with type`'private'` is given, a new `KeyObject` with type `'public'` will be returned
- * and it will be impossible to extract the private key from the returned object.
- * @since v11.6.0
- */
- function createPublicKey(key: PublicKeyInput | string | Buffer | KeyObject | JsonWebKeyInput): KeyObject;
- /**
- * Creates and returns a new key object containing a secret key for symmetric
- * encryption or `Hmac`.
- * @since v11.6.0
- * @param encoding The string encoding when `key` is a string.
- */
- function createSecretKey(key: NodeJS.ArrayBufferView): KeyObject;
- function createSecretKey(key: string, encoding: BufferEncoding): KeyObject;
- /**
- * Creates and returns a `Sign` object that uses the given `algorithm`. Use {@link getHashes} to obtain the names of the available digest algorithms.
- * Optional `options` argument controls the `stream.Writable` behavior.
- *
- * In some cases, a `Sign` instance can be created using the name of a signature
- * algorithm, such as `'RSA-SHA256'`, instead of a digest algorithm. This will use
- * the corresponding digest algorithm. This does not work for all signature
- * algorithms, such as `'ecdsa-with-SHA256'`, so it is best to always use digest
- * algorithm names.
- * @since v0.1.92
- * @param options `stream.Writable` options
- */
- function createSign(algorithm: string, options?: stream.WritableOptions): Sign;
- type DSAEncoding = 'der' | 'ieee-p1363';
- interface SigningOptions {
- /**
- * @See crypto.constants.RSA_PKCS1_PADDING
- */
- padding?: number | undefined;
- saltLength?: number | undefined;
- dsaEncoding?: DSAEncoding | undefined;
- }
- interface SignPrivateKeyInput extends PrivateKeyInput, SigningOptions {}
- interface SignKeyObjectInput extends SigningOptions {
- key: KeyObject;
- }
- interface VerifyPublicKeyInput extends PublicKeyInput, SigningOptions {}
- interface VerifyKeyObjectInput extends SigningOptions {
- key: KeyObject;
- }
- type KeyLike = string | Buffer | KeyObject;
- /**
- * The `Sign` class is a utility for generating signatures. It can be used in one
- * of two ways:
- *
- * * As a writable `stream`, where data to be signed is written and the `sign.sign()` method is used to generate and return the signature, or
- * * Using the `sign.update()` and `sign.sign()` methods to produce the
- * signature.
- *
- * The {@link createSign} method is used to create `Sign` instances. The
- * argument is the string name of the hash function to use. `Sign` objects are not
- * to be created directly using the `new` keyword.
- *
- * Example: Using `Sign` and `Verify` objects as streams:
- *
- * ```js
- * const {
- * generateKeyPairSync,
- * createSign,
- * createVerify
- * } = await import('crypto');
- *
- * const { privateKey, publicKey } = generateKeyPairSync('ec', {
- * namedCurve: 'sect239k1'
- * });
- *
- * const sign = createSign('SHA256');
- * sign.write('some data to sign');
- * sign.end();
- * const signature = sign.sign(privateKey, 'hex');
- *
- * const verify = createVerify('SHA256');
- * verify.write('some data to sign');
- * verify.end();
- * console.log(verify.verify(publicKey, signature, 'hex'));
- * // Prints: true
- * ```
- *
- * Example: Using the `sign.update()` and `verify.update()` methods:
- *
- * ```js
- * const {
- * generateKeyPairSync,
- * createSign,
- * createVerify
- * } = await import('crypto');
- *
- * const { privateKey, publicKey } = generateKeyPairSync('rsa', {
- * modulusLength: 2048,
- * });
- *
- * const sign = createSign('SHA256');
- * sign.update('some data to sign');
- * sign.end();
- * const signature = sign.sign(privateKey);
- *
- * const verify = createVerify('SHA256');
- * verify.update('some data to sign');
- * verify.end();
- * console.log(verify.verify(publicKey, signature));
- * // Prints: true
- * ```
- * @since v0.1.92
- */
- class Sign extends stream.Writable {
- private constructor();
- /**
- * Updates the `Sign` content with the given `data`, the encoding of which
- * is given in `inputEncoding`.
- * If `encoding` is not provided, and the `data` is a string, an
- * encoding of `'utf8'` is enforced. If `data` is a `Buffer`, `TypedArray`, or`DataView`, then `inputEncoding` is ignored.
- *
- * This can be called many times with new data as it is streamed.
- * @since v0.1.92
- * @param inputEncoding The `encoding` of the `data` string.
- */
- update(data: BinaryLike): this;
- update(data: string, inputEncoding: Encoding): this;
- /**
- * Calculates the signature on all the data passed through using either `sign.update()` or `sign.write()`.
- *
- * If `privateKey` is not a `KeyObject`, this function behaves as if`privateKey` had been passed to {@link createPrivateKey}. If it is an
- * object, the following additional properties can be passed:
- *
- * If `outputEncoding` is provided a string is returned; otherwise a `Buffer` is returned.
- *
- * The `Sign` object can not be again used after `sign.sign()` method has been
- * called. Multiple calls to `sign.sign()` will result in an error being thrown.
- * @since v0.1.92
- */
- sign(privateKey: KeyLike | SignKeyObjectInput | SignPrivateKeyInput): Buffer;
- sign(privateKey: KeyLike | SignKeyObjectInput | SignPrivateKeyInput, outputFormat: BinaryToTextEncoding): string;
- }
- /**
- * Creates and returns a `Verify` object that uses the given algorithm.
- * Use {@link getHashes} to obtain an array of names of the available
- * signing algorithms. Optional `options` argument controls the`stream.Writable` behavior.
- *
- * In some cases, a `Verify` instance can be created using the name of a signature
- * algorithm, such as `'RSA-SHA256'`, instead of a digest algorithm. This will use
- * the corresponding digest algorithm. This does not work for all signature
- * algorithms, such as `'ecdsa-with-SHA256'`, so it is best to always use digest
- * algorithm names.
- * @since v0.1.92
- * @param options `stream.Writable` options
- */
- function createVerify(algorithm: string, options?: stream.WritableOptions): Verify;
- /**
- * The `Verify` class is a utility for verifying signatures. It can be used in one
- * of two ways:
- *
- * * As a writable `stream` where written data is used to validate against the
- * supplied signature, or
- * * Using the `verify.update()` and `verify.verify()` methods to verify
- * the signature.
- *
- * The {@link createVerify} method is used to create `Verify` instances.`Verify` objects are not to be created directly using the `new` keyword.
- *
- * See `Sign` for examples.
- * @since v0.1.92
- */
- class Verify extends stream.Writable {
- private constructor();
- /**
- * Updates the `Verify` content with the given `data`, the encoding of which
- * is given in `inputEncoding`.
- * If `inputEncoding` is not provided, and the `data` is a string, an
- * encoding of `'utf8'` is enforced. If `data` is a `Buffer`, `TypedArray`, or`DataView`, then `inputEncoding` is ignored.
- *
- * This can be called many times with new data as it is streamed.
- * @since v0.1.92
- * @param inputEncoding The `encoding` of the `data` string.
- */
- update(data: BinaryLike): Verify;
- update(data: string, inputEncoding: Encoding): Verify;
- /**
- * Verifies the provided data using the given `object` and `signature`.
- *
- * If `object` is not a `KeyObject`, this function behaves as if`object` had been passed to {@link createPublicKey}. If it is an
- * object, the following additional properties can be passed:
- *
- * The `signature` argument is the previously calculated signature for the data, in
- * the `signatureEncoding`.
- * If a `signatureEncoding` is specified, the `signature` is expected to be a
- * string; otherwise `signature` is expected to be a `Buffer`,`TypedArray`, or `DataView`.
- *
- * The `verify` object can not be used again after `verify.verify()` has been
- * called. Multiple calls to `verify.verify()` will result in an error being
- * thrown.
- *
- * Because public keys can be derived from private keys, a private key may
- * be passed instead of a public key.
- * @since v0.1.92
- */
- verify(object: KeyLike | VerifyKeyObjectInput | VerifyPublicKeyInput, signature: NodeJS.ArrayBufferView): boolean;
- verify(object: KeyLike | VerifyKeyObjectInput | VerifyPublicKeyInput, signature: string, signature_format?: BinaryToTextEncoding): boolean;
- }
- /**
- * Creates a `DiffieHellman` key exchange object using the supplied `prime` and an
- * optional specific `generator`.
- *
- * The `generator` argument can be a number, string, or `Buffer`. If`generator` is not specified, the value `2` is used.
- *
- * If `primeEncoding` is specified, `prime` is expected to be a string; otherwise
- * a `Buffer`, `TypedArray`, or `DataView` is expected.
- *
- * If `generatorEncoding` is specified, `generator` is expected to be a string;
- * otherwise a number, `Buffer`, `TypedArray`, or `DataView` is expected.
- * @since v0.11.12
- * @param primeEncoding The `encoding` of the `prime` string.
- * @param [generator=2]
- * @param generatorEncoding The `encoding` of the `generator` string.
- */
- function createDiffieHellman(primeLength: number, generator?: number): DiffieHellman;
- function createDiffieHellman(prime: ArrayBuffer | NodeJS.ArrayBufferView, generator?: number | ArrayBuffer | NodeJS.ArrayBufferView): DiffieHellman;
- function createDiffieHellman(prime: ArrayBuffer | NodeJS.ArrayBufferView, generator: string, generatorEncoding: BinaryToTextEncoding): DiffieHellman;
- function createDiffieHellman(prime: string, primeEncoding: BinaryToTextEncoding, generator?: number | ArrayBuffer | NodeJS.ArrayBufferView): DiffieHellman;
- function createDiffieHellman(prime: string, primeEncoding: BinaryToTextEncoding, generator: string, generatorEncoding: BinaryToTextEncoding): DiffieHellman;
- /**
- * The `DiffieHellman` class is a utility for creating Diffie-Hellman key
- * exchanges.
- *
- * Instances of the `DiffieHellman` class can be created using the {@link createDiffieHellman} function.
- *
- * ```js
- * import assert from 'assert';
- *
- * const {
- * createDiffieHellman
- * } = await import('crypto');
- *
- * // Generate Alice's keys...
- * const alice = createDiffieHellman(2048);
- * const aliceKey = alice.generateKeys();
- *
- * // Generate Bob's keys...
- * const bob = createDiffieHellman(alice.getPrime(), alice.getGenerator());
- * const bobKey = bob.generateKeys();
- *
- * // Exchange and generate the secret...
- * const aliceSecret = alice.computeSecret(bobKey);
- * const bobSecret = bob.computeSecret(aliceKey);
- *
- * // OK
- * assert.strictEqual(aliceSecret.toString('hex'), bobSecret.toString('hex'));
- * ```
- * @since v0.5.0
- */
- class DiffieHellman {
- private constructor();
- /**
- * Generates private and public Diffie-Hellman key values, and returns
- * the public key in the specified `encoding`. This key should be
- * transferred to the other party.
- * If `encoding` is provided a string is returned; otherwise a `Buffer` is returned.
- * @since v0.5.0
- * @param encoding The `encoding` of the return value.
- */
- generateKeys(): Buffer;
- generateKeys(encoding: BinaryToTextEncoding): string;
- /**
- * Computes the shared secret using `otherPublicKey` as the other
- * party's public key and returns the computed shared secret. The supplied
- * key is interpreted using the specified `inputEncoding`, and secret is
- * encoded using specified `outputEncoding`.
- * If the `inputEncoding` is not
- * provided, `otherPublicKey` is expected to be a `Buffer`,`TypedArray`, or `DataView`.
- *
- * If `outputEncoding` is given a string is returned; otherwise, a `Buffer` is returned.
- * @since v0.5.0
- * @param inputEncoding The `encoding` of an `otherPublicKey` string.
- * @param outputEncoding The `encoding` of the return value.
- */
- computeSecret(otherPublicKey: NodeJS.ArrayBufferView, inputEncoding?: null, outputEncoding?: null): Buffer;
- computeSecret(otherPublicKey: string, inputEncoding: BinaryToTextEncoding, outputEncoding?: null): Buffer;
- computeSecret(otherPublicKey: NodeJS.ArrayBufferView, inputEncoding: null, outputEncoding: BinaryToTextEncoding): string;
- computeSecret(otherPublicKey: string, inputEncoding: BinaryToTextEncoding, outputEncoding: BinaryToTextEncoding): string;
- /**
- * Returns the Diffie-Hellman prime in the specified `encoding`.
- * If `encoding` is provided a string is
- * returned; otherwise a `Buffer` is returned.
- * @since v0.5.0
- * @param encoding The `encoding` of the return value.
- */
- getPrime(): Buffer;
- getPrime(encoding: BinaryToTextEncoding): string;
- /**
- * Returns the Diffie-Hellman generator in the specified `encoding`.
- * If `encoding` is provided a string is
- * returned; otherwise a `Buffer` is returned.
- * @since v0.5.0
- * @param encoding The `encoding` of the return value.
- */
- getGenerator(): Buffer;
- getGenerator(encoding: BinaryToTextEncoding): string;
- /**
- * Returns the Diffie-Hellman public key in the specified `encoding`.
- * If `encoding` is provided a
- * string is returned; otherwise a `Buffer` is returned.
- * @since v0.5.0
- * @param encoding The `encoding` of the return value.
- */
- getPublicKey(): Buffer;
- getPublicKey(encoding: BinaryToTextEncoding): string;
- /**
- * Returns the Diffie-Hellman private key in the specified `encoding`.
- * If `encoding` is provided a
- * string is returned; otherwise a `Buffer` is returned.
- * @since v0.5.0
- * @param encoding The `encoding` of the return value.
- */
- getPrivateKey(): Buffer;
- getPrivateKey(encoding: BinaryToTextEncoding): string;
- /**
- * Sets the Diffie-Hellman public key. If the `encoding` argument is provided,`publicKey` is expected
- * to be a string. If no `encoding` is provided, `publicKey` is expected
- * to be a `Buffer`, `TypedArray`, or `DataView`.
- * @since v0.5.0
- * @param encoding The `encoding` of the `publicKey` string.
- */
- setPublicKey(publicKey: NodeJS.ArrayBufferView): void;
- setPublicKey(publicKey: string, encoding: BufferEncoding): void;
- /**
- * Sets the Diffie-Hellman private key. If the `encoding` argument is provided,`privateKey` is expected
- * to be a string. If no `encoding` is provided, `privateKey` is expected
- * to be a `Buffer`, `TypedArray`, or `DataView`.
- * @since v0.5.0
- * @param encoding The `encoding` of the `privateKey` string.
- */
- setPrivateKey(privateKey: NodeJS.ArrayBufferView): void;
- setPrivateKey(privateKey: string, encoding: BufferEncoding): void;
- /**
- * A bit field containing any warnings and/or errors resulting from a check
- * performed during initialization of the `DiffieHellman` object.
- *
- * The following values are valid for this property (as defined in `constants`module):
- *
- * * `DH_CHECK_P_NOT_SAFE_PRIME`
- * * `DH_CHECK_P_NOT_PRIME`
- * * `DH_UNABLE_TO_CHECK_GENERATOR`
- * * `DH_NOT_SUITABLE_GENERATOR`
- * @since v0.11.12
- */
- verifyError: number;
- }
- /**
- * The `DiffieHellmanGroup` class takes a well-known modp group as its argument.
- * It works the same as `DiffieHellman`, except that it does not allow changing its keys after creation.
- * In other words, it does not implement `setPublicKey()` or `setPrivateKey()` methods.
- *
- * ```js
- * const { createDiffieHellmanGroup } = await import('node:crypto');
- * const dh = createDiffieHellmanGroup('modp1');
- * ```
- * The name (e.g. `'modp1'`) is taken from [RFC 2412](https://www.rfc-editor.org/rfc/rfc2412.txt) (modp1 and 2) and [RFC 3526](https://www.rfc-editor.org/rfc/rfc3526.txt):
- * ```bash
- * $ perl -ne 'print "$1\n" if /"(modp\d+)"/' src/node_crypto_groups.h
- * modp1 # 768 bits
- * modp2 # 1024 bits
- * modp5 # 1536 bits
- * modp14 # 2048 bits
- * modp15 # etc.
- * modp16
- * modp17
- * modp18
- * ```
- * @since v0.7.5
- */
- const DiffieHellmanGroup: DiffieHellmanGroupConstructor;
- interface DiffieHellmanGroupConstructor {
- new(name: string): DiffieHellmanGroup;
- (name: string): DiffieHellmanGroup;
- readonly prototype: DiffieHellmanGroup;
- }
- type DiffieHellmanGroup = Omit;
- /**
- * Creates a predefined `DiffieHellmanGroup` key exchange object. The
- * supported groups are: `'modp1'`, `'modp2'`, `'modp5'` (defined in [RFC 2412](https://www.rfc-editor.org/rfc/rfc2412.txt), but see `Caveats`) and `'modp14'`, `'modp15'`,`'modp16'`, `'modp17'`,
- * `'modp18'` (defined in [RFC 3526](https://www.rfc-editor.org/rfc/rfc3526.txt)). The
- * returned object mimics the interface of objects created by {@link createDiffieHellman}, but will not allow changing
- * the keys (with `diffieHellman.setPublicKey()`, for example). The
- * advantage of using this method is that the parties do not have to
- * generate nor exchange a group modulus beforehand, saving both processor
- * and communication time.
- *
- * Example (obtaining a shared secret):
- *
- * ```js
- * const {
- * getDiffieHellman
- * } = await import('crypto');
- * const alice = getDiffieHellman('modp14');
- * const bob = getDiffieHellman('modp14');
- *
- * alice.generateKeys();
- * bob.generateKeys();
- *
- * const aliceSecret = alice.computeSecret(bob.getPublicKey(), null, 'hex');
- * const bobSecret = bob.computeSecret(alice.getPublicKey(), null, 'hex');
- *
- * // aliceSecret and bobSecret should be the same
- * console.log(aliceSecret === bobSecret);
- * ```
- * @since v0.7.5
- */
- function getDiffieHellman(groupName: string): DiffieHellmanGroup;
- /**
- * An alias for {@link getDiffieHellman}
- * @since v0.9.3
- */
- function createDiffieHellmanGroup(name: string): DiffieHellmanGroup;
- /**
- * Provides an asynchronous Password-Based Key Derivation Function 2 (PBKDF2)
- * implementation. A selected HMAC digest algorithm specified by `digest` is
- * applied to derive a key of the requested byte length (`keylen`) from the`password`, `salt` and `iterations`.
- *
- * The supplied `callback` function is called with two arguments: `err` and`derivedKey`. If an error occurs while deriving the key, `err` will be set;
- * otherwise `err` will be `null`. By default, the successfully generated`derivedKey` will be passed to the callback as a `Buffer`. An error will be
- * thrown if any of the input arguments specify invalid values or types.
- *
- * If `digest` is `null`, `'sha1'` will be used. This behavior is deprecated,
- * please specify a `digest` explicitly.
- *
- * The `iterations` argument must be a number set as high as possible. The
- * higher the number of iterations, the more secure the derived key will be,
- * but will take a longer amount of time to complete.
- *
- * The `salt` should be as unique as possible. It is recommended that a salt is
- * random and at least 16 bytes long. See [NIST SP 800-132](https://nvlpubs.nist.gov/nistpubs/Legacy/SP/nistspecialpublication800-132.pdf) for details.
- *
- * When passing strings for `password` or `salt`, please consider `caveats when using strings as inputs to cryptographic APIs`.
- *
- * ```js
- * const {
- * pbkdf2
- * } = await import('crypto');
- *
- * pbkdf2('secret', 'salt', 100000, 64, 'sha512', (err, derivedKey) => {
- * if (err) throw err;
- * console.log(derivedKey.toString('hex')); // '3745e48...08d59ae'
- * });
- * ```
- *
- * The `crypto.DEFAULT_ENCODING` property can be used to change the way the`derivedKey` is passed to the callback. This property, however, has been
- * deprecated and use should be avoided.
- *
- * ```js
- * import crypto from 'crypto';
- * crypto.DEFAULT_ENCODING = 'hex';
- * crypto.pbkdf2('secret', 'salt', 100000, 512, 'sha512', (err, derivedKey) => {
- * if (err) throw err;
- * console.log(derivedKey); // '3745e48...aa39b34'
- * });
- * ```
- *
- * An array of supported digest functions can be retrieved using {@link getHashes}.
- *
- * This API uses libuv's threadpool, which can have surprising and
- * negative performance implications for some applications; see the `UV_THREADPOOL_SIZE` documentation for more information.
- * @since v0.5.5
- */
- function pbkdf2(password: BinaryLike, salt: BinaryLike, iterations: number, keylen: number, digest: string, callback: (err: Error | null, derivedKey: Buffer) => void): void;
- /**
- * Provides a synchronous Password-Based Key Derivation Function 2 (PBKDF2)
- * implementation. A selected HMAC digest algorithm specified by `digest` is
- * applied to derive a key of the requested byte length (`keylen`) from the`password`, `salt` and `iterations`.
- *
- * If an error occurs an `Error` will be thrown, otherwise the derived key will be
- * returned as a `Buffer`.
- *
- * If `digest` is `null`, `'sha1'` will be used. This behavior is deprecated,
- * please specify a `digest` explicitly.
- *
- * The `iterations` argument must be a number set as high as possible. The
- * higher the number of iterations, the more secure the derived key will be,
- * but will take a longer amount of time to complete.
- *
- * The `salt` should be as unique as possible. It is recommended that a salt is
- * random and at least 16 bytes long. See [NIST SP 800-132](https://nvlpubs.nist.gov/nistpubs/Legacy/SP/nistspecialpublication800-132.pdf) for details.
- *
- * When passing strings for `password` or `salt`, please consider `caveats when using strings as inputs to cryptographic APIs`.
- *
- * ```js
- * const {
- * pbkdf2Sync
- * } = await import('crypto');
- *
- * const key = pbkdf2Sync('secret', 'salt', 100000, 64, 'sha512');
- * console.log(key.toString('hex')); // '3745e48...08d59ae'
- * ```
- *
- * The `crypto.DEFAULT_ENCODING` property may be used to change the way the`derivedKey` is returned. This property, however, is deprecated and use
- * should be avoided.
- *
- * ```js
- * import crypto from 'crypto';
- * crypto.DEFAULT_ENCODING = 'hex';
- * const key = crypto.pbkdf2Sync('secret', 'salt', 100000, 512, 'sha512');
- * console.log(key); // '3745e48...aa39b34'
- * ```
- *
- * An array of supported digest functions can be retrieved using {@link getHashes}.
- * @since v0.9.3
- */
- function pbkdf2Sync(password: BinaryLike, salt: BinaryLike, iterations: number, keylen: number, digest: string): Buffer;
- /**
- * Generates cryptographically strong pseudorandom data. The `size` argument
- * is a number indicating the number of bytes to generate.
- *
- * If a `callback` function is provided, the bytes are generated asynchronously
- * and the `callback` function is invoked with two arguments: `err` and `buf`.
- * If an error occurs, `err` will be an `Error` object; otherwise it is `null`. The`buf` argument is a `Buffer` containing the generated bytes.
- *
- * ```js
- * // Asynchronous
- * const {
- * randomBytes
- * } = await import('crypto');
- *
- * randomBytes(256, (err, buf) => {
- * if (err) throw err;
- * console.log(`${buf.length} bytes of random data: ${buf.toString('hex')}`);
- * });
- * ```
- *
- * If the `callback` function is not provided, the random bytes are generated
- * synchronously and returned as a `Buffer`. An error will be thrown if
- * there is a problem generating the bytes.
- *
- * ```js
- * // Synchronous
- * const {
- * randomBytes
- * } = await import('crypto');
- *
- * const buf = randomBytes(256);
- * console.log(
- * `${buf.length} bytes of random data: ${buf.toString('hex')}`);
- * ```
- *
- * The `crypto.randomBytes()` method will not complete until there is
- * sufficient entropy available.
- * This should normally never take longer than a few milliseconds. The only time
- * when generating the random bytes may conceivably block for a longer period of
- * time is right after boot, when the whole system is still low on entropy.
- *
- * This API uses libuv's threadpool, which can have surprising and
- * negative performance implications for some applications; see the `UV_THREADPOOL_SIZE` documentation for more information.
- *
- * The asynchronous version of `crypto.randomBytes()` is carried out in a single
- * threadpool request. To minimize threadpool task length variation, partition
- * large `randomBytes` requests when doing so as part of fulfilling a client
- * request.
- * @since v0.5.8
- * @param size The number of bytes to generate. The `size` must not be larger than `2**31 - 1`.
- * @return if the `callback` function is not provided.
- */
- function randomBytes(size: number): Buffer;
- function randomBytes(size: number, callback: (err: Error | null, buf: Buffer) => void): void;
- function pseudoRandomBytes(size: number): Buffer;
- function pseudoRandomBytes(size: number, callback: (err: Error | null, buf: Buffer) => void): void;
- /**
- * Return a random integer `n` such that `min <= n < max`. This
- * implementation avoids [modulo bias](https://en.wikipedia.org/wiki/Fisher%E2%80%93Yates_shuffle#Modulo_bias).
- *
- * The range (`max - min`) must be less than 2^48. `min` and `max` must
- * be [safe integers](https://developer.mozilla.org/en-US/docs/Web/JavaScript/Reference/Global_Objects/Number/isSafeInteger).
- *
- * If the `callback` function is not provided, the random integer is
- * generated synchronously.
- *
- * ```js
- * // Asynchronous
- * const {
- * randomInt
- * } = await import('crypto');
- *
- * randomInt(3, (err, n) => {
- * if (err) throw err;
- * console.log(`Random number chosen from (0, 1, 2): ${n}`);
- * });
- * ```
- *
- * ```js
- * // Synchronous
- * const {
- * randomInt
- * } = await import('crypto');
- *
- * const n = randomInt(3);
- * console.log(`Random number chosen from (0, 1, 2): ${n}`);
- * ```
- *
- * ```js
- * // With `min` argument
- * const {
- * randomInt
- * } = await import('crypto');
- *
- * const n = randomInt(1, 7);
- * console.log(`The dice rolled: ${n}`);
- * ```
- * @since v14.10.0, v12.19.0
- * @param [min=0] Start of random range (inclusive).
- * @param max End of random range (exclusive).
- * @param callback `function(err, n) {}`.
- */
- function randomInt(max: number): number;
- function randomInt(min: number, max: number): number;
- function randomInt(max: number, callback: (err: Error | null, value: number) => void): void;
- function randomInt(min: number, max: number, callback: (err: Error | null, value: number) => void): void;
- /**
- * Synchronous version of {@link randomFill}.
- *
- * ```js
- * import { Buffer } from 'buffer';
- * const { randomFillSync } = await import('crypto');
- *
- * const buf = Buffer.alloc(10);
- * console.log(randomFillSync(buf).toString('hex'));
- *
- * randomFillSync(buf, 5);
- * console.log(buf.toString('hex'));
- *
- * // The above is equivalent to the following:
- * randomFillSync(buf, 5, 5);
- * console.log(buf.toString('hex'));
- * ```
- *
- * Any `ArrayBuffer`, `TypedArray` or `DataView` instance may be passed as`buffer`.
- *
- * ```js
- * import { Buffer } from 'buffer';
- * const { randomFillSync } = await import('crypto');
- *
- * const a = new Uint32Array(10);
- * console.log(Buffer.from(randomFillSync(a).buffer,
- * a.byteOffset, a.byteLength).toString('hex'));
- *
- * const b = new DataView(new ArrayBuffer(10));
- * console.log(Buffer.from(randomFillSync(b).buffer,
- * b.byteOffset, b.byteLength).toString('hex'));
- *
- * const c = new ArrayBuffer(10);
- * console.log(Buffer.from(randomFillSync(c)).toString('hex'));
- * ```
- * @since v7.10.0, v6.13.0
- * @param buffer Must be supplied. The size of the provided `buffer` must not be larger than `2**31 - 1`.
- * @param [offset=0]
- * @param [size=buffer.length - offset]
- * @return The object passed as `buffer` argument.
- */
- function randomFillSync(buffer: T, offset?: number, size?: number): T;
- /**
- * This function is similar to {@link randomBytes} but requires the first
- * argument to be a `Buffer` that will be filled. It also
- * requires that a callback is passed in.
- *
- * If the `callback` function is not provided, an error will be thrown.
- *
- * ```js
- * import { Buffer } from 'buffer';
- * const { randomFill } = await import('crypto');
- *
- * const buf = Buffer.alloc(10);
- * randomFill(buf, (err, buf) => {
- * if (err) throw err;
- * console.log(buf.toString('hex'));
- * });
- *
- * randomFill(buf, 5, (err, buf) => {
- * if (err) throw err;
- * console.log(buf.toString('hex'));
- * });
- *
- * // The above is equivalent to the following:
- * randomFill(buf, 5, 5, (err, buf) => {
- * if (err) throw err;
- * console.log(buf.toString('hex'));
- * });
- * ```
- *
- * Any `ArrayBuffer`, `TypedArray`, or `DataView` instance may be passed as`buffer`.
- *
- * While this includes instances of `Float32Array` and `Float64Array`, this
- * function should not be used to generate random floating-point numbers. The
- * result may contain `+Infinity`, `-Infinity`, and `NaN`, and even if the array
- * contains finite numbers only, they are not drawn from a uniform random
- * distribution and have no meaningful lower or upper bounds.
- *
- * ```js
- * import { Buffer } from 'buffer';
- * const { randomFill } = await import('crypto');
- *
- * const a = new Uint32Array(10);
- * randomFill(a, (err, buf) => {
- * if (err) throw err;
- * console.log(Buffer.from(buf.buffer, buf.byteOffset, buf.byteLength)
- * .toString('hex'));
- * });
- *
- * const b = new DataView(new ArrayBuffer(10));
- * randomFill(b, (err, buf) => {
- * if (err) throw err;
- * console.log(Buffer.from(buf.buffer, buf.byteOffset, buf.byteLength)
- * .toString('hex'));
- * });
- *
- * const c = new ArrayBuffer(10);
- * randomFill(c, (err, buf) => {
- * if (err) throw err;
- * console.log(Buffer.from(buf).toString('hex'));
- * });
- * ```
- *
- * This API uses libuv's threadpool, which can have surprising and
- * negative performance implications for some applications; see the `UV_THREADPOOL_SIZE` documentation for more information.
- *
- * The asynchronous version of `crypto.randomFill()` is carried out in a single
- * threadpool request. To minimize threadpool task length variation, partition
- * large `randomFill` requests when doing so as part of fulfilling a client
- * request.
- * @since v7.10.0, v6.13.0
- * @param buffer Must be supplied. The size of the provided `buffer` must not be larger than `2**31 - 1`.
- * @param [offset=0]
- * @param [size=buffer.length - offset]
- * @param callback `function(err, buf) {}`.
- */
- function randomFill(buffer: T, callback: (err: Error | null, buf: T) => void): void;
- function randomFill(buffer: T, offset: number, callback: (err: Error | null, buf: T) => void): void;
- function randomFill(buffer: T, offset: number, size: number, callback: (err: Error | null, buf: T) => void): void;
- interface ScryptOptions {
- cost?: number | undefined;
- blockSize?: number | undefined;
- parallelization?: number | undefined;
- N?: number | undefined;
- r?: number | undefined;
- p?: number | undefined;
- maxmem?: number | undefined;
- }
- /**
- * Provides an asynchronous [scrypt](https://en.wikipedia.org/wiki/Scrypt) implementation. Scrypt is a password-based
- * key derivation function that is designed to be expensive computationally and
- * memory-wise in order to make brute-force attacks unrewarding.
- *
- * The `salt` should be as unique as possible. It is recommended that a salt is
- * random and at least 16 bytes long. See [NIST SP 800-132](https://nvlpubs.nist.gov/nistpubs/Legacy/SP/nistspecialpublication800-132.pdf) for details.
- *
- * When passing strings for `password` or `salt`, please consider `caveats when using strings as inputs to cryptographic APIs`.
- *
- * The `callback` function is called with two arguments: `err` and `derivedKey`.`err` is an exception object when key derivation fails, otherwise `err` is`null`. `derivedKey` is passed to the
- * callback as a `Buffer`.
- *
- * An exception is thrown when any of the input arguments specify invalid values
- * or types.
- *
- * ```js
- * const {
- * scrypt
- * } = await import('crypto');
- *
- * // Using the factory defaults.
- * scrypt('password', 'salt', 64, (err, derivedKey) => {
- * if (err) throw err;
- * console.log(derivedKey.toString('hex')); // '3745e48...08d59ae'
- * });
- * // Using a custom N parameter. Must be a power of two.
- * scrypt('password', 'salt', 64, { N: 1024 }, (err, derivedKey) => {
- * if (err) throw err;
- * console.log(derivedKey.toString('hex')); // '3745e48...aa39b34'
- * });
- * ```
- * @since v10.5.0
- */
- function scrypt(password: BinaryLike, salt: BinaryLike, keylen: number, callback: (err: Error | null, derivedKey: Buffer) => void): void;
- function scrypt(password: BinaryLike, salt: BinaryLike, keylen: number, options: ScryptOptions, callback: (err: Error | null, derivedKey: Buffer) => void): void;
- /**
- * Provides a synchronous [scrypt](https://en.wikipedia.org/wiki/Scrypt) implementation. Scrypt is a password-based
- * key derivation function that is designed to be expensive computationally and
- * memory-wise in order to make brute-force attacks unrewarding.
- *
- * The `salt` should be as unique as possible. It is recommended that a salt is
- * random and at least 16 bytes long. See [NIST SP 800-132](https://nvlpubs.nist.gov/nistpubs/Legacy/SP/nistspecialpublication800-132.pdf) for details.
- *
- * When passing strings for `password` or `salt`, please consider `caveats when using strings as inputs to cryptographic APIs`.
- *
- * An exception is thrown when key derivation fails, otherwise the derived key is
- * returned as a `Buffer`.
- *
- * An exception is thrown when any of the input arguments specify invalid values
- * or types.
- *
- * ```js
- * const {
- * scryptSync
- * } = await import('crypto');
- * // Using the factory defaults.
- *
- * const key1 = scryptSync('password', 'salt', 64);
- * console.log(key1.toString('hex')); // '3745e48...08d59ae'
- * // Using a custom N parameter. Must be a power of two.
- * const key2 = scryptSync('password', 'salt', 64, { N: 1024 });
- * console.log(key2.toString('hex')); // '3745e48...aa39b34'
- * ```
- * @since v10.5.0
- */
- function scryptSync(password: BinaryLike, salt: BinaryLike, keylen: number, options?: ScryptOptions): Buffer;
- interface RsaPublicKey {
- key: KeyLike;
- padding?: number | undefined;
- }
- interface RsaPrivateKey {
- key: KeyLike;
- passphrase?: string | undefined;
- /**
- * @default 'sha1'
- */
- oaepHash?: string | undefined;
- oaepLabel?: NodeJS.TypedArray | undefined;
- padding?: number | undefined;
- }
- /**
- * Encrypts the content of `buffer` with `key` and returns a new `Buffer` with encrypted content. The returned data can be decrypted using
- * the corresponding private key, for example using {@link privateDecrypt}.
- *
- * If `key` is not a `KeyObject`, this function behaves as if`key` had been passed to {@link createPublicKey}. If it is an
- * object, the `padding` property can be passed. Otherwise, this function uses`RSA_PKCS1_OAEP_PADDING`.
- *
- * Because RSA public keys can be derived from private keys, a private key may
- * be passed instead of a public key.
- * @since v0.11.14
- */
- function publicEncrypt(key: RsaPublicKey | RsaPrivateKey | KeyLike, buffer: NodeJS.ArrayBufferView): Buffer;
- /**
- * Decrypts `buffer` with `key`.`buffer` was previously encrypted using
- * the corresponding private key, for example using {@link privateEncrypt}.
- *
- * If `key` is not a `KeyObject`, this function behaves as if`key` had been passed to {@link createPublicKey}. If it is an
- * object, the `padding` property can be passed. Otherwise, this function uses`RSA_PKCS1_PADDING`.
- *
- * Because RSA public keys can be derived from private keys, a private key may
- * be passed instead of a public key.
- * @since v1.1.0
- */
- function publicDecrypt(key: RsaPublicKey | RsaPrivateKey | KeyLike, buffer: NodeJS.ArrayBufferView): Buffer;
- /**
- * Decrypts `buffer` with `privateKey`. `buffer` was previously encrypted using
- * the corresponding public key, for example using {@link publicEncrypt}.
- *
- * If `privateKey` is not a `KeyObject`, this function behaves as if`privateKey` had been passed to {@link createPrivateKey}. If it is an
- * object, the `padding` property can be passed. Otherwise, this function uses`RSA_PKCS1_OAEP_PADDING`.
- * @since v0.11.14
- */
- function privateDecrypt(privateKey: RsaPrivateKey | KeyLike, buffer: NodeJS.ArrayBufferView): Buffer;
- /**
- * Encrypts `buffer` with `privateKey`. The returned data can be decrypted using
- * the corresponding public key, for example using {@link publicDecrypt}.
- *
- * If `privateKey` is not a `KeyObject`, this function behaves as if`privateKey` had been passed to {@link createPrivateKey}. If it is an
- * object, the `padding` property can be passed. Otherwise, this function uses`RSA_PKCS1_PADDING`.
- * @since v1.1.0
- */
- function privateEncrypt(privateKey: RsaPrivateKey | KeyLike, buffer: NodeJS.ArrayBufferView): Buffer;
- /**
- * ```js
- * const {
- * getCiphers
- * } = await import('crypto');
- *
- * console.log(getCiphers()); // ['aes-128-cbc', 'aes-128-ccm', ...]
- * ```
- * @since v0.9.3
- * @return An array with the names of the supported cipher algorithms.
- */
- function getCiphers(): string[];
- /**
- * ```js
- * const {
- * getCurves
- * } = await import('crypto');
- *
- * console.log(getCurves()); // ['Oakley-EC2N-3', 'Oakley-EC2N-4', ...]
- * ```
- * @since v2.3.0
- * @return An array with the names of the supported elliptic curves.
- */
- function getCurves(): string[];
- /**
- * @since v10.0.0
- * @return `1` if and only if a FIPS compliant crypto provider is currently in use, `0` otherwise. A future semver-major release may change the return type of this API to a {boolean}.
- */
- function getFips(): 1 | 0;
- /**
- * Enables the FIPS compliant crypto provider in a FIPS-enabled Node.js build. Throws an error if FIPS mode is not available.
- * @since v10.0.0
- * @param bool `true` to enable FIPS mode.
- */
- function setFips(bool: boolean): void;
- /**
- * ```js
- * const {
- * getHashes
- * } = await import('crypto');
- *
- * console.log(getHashes()); // ['DSA', 'DSA-SHA', 'DSA-SHA1', ...]
- * ```
- * @since v0.9.3
- * @return An array of the names of the supported hash algorithms, such as `'RSA-SHA256'`. Hash algorithms are also called "digest" algorithms.
- */
- function getHashes(): string[];
- /**
- * The `ECDH` class is a utility for creating Elliptic Curve Diffie-Hellman (ECDH)
- * key exchanges.
- *
- * Instances of the `ECDH` class can be created using the {@link createECDH} function.
- *
- * ```js
- * import assert from 'assert';
- *
- * const {
- * createECDH
- * } = await import('crypto');
- *
- * // Generate Alice's keys...
- * const alice = createECDH('secp521r1');
- * const aliceKey = alice.generateKeys();
- *
- * // Generate Bob's keys...
- * const bob = createECDH('secp521r1');
- * const bobKey = bob.generateKeys();
- *
- * // Exchange and generate the secret...
- * const aliceSecret = alice.computeSecret(bobKey);
- * const bobSecret = bob.computeSecret(aliceKey);
- *
- * assert.strictEqual(aliceSecret.toString('hex'), bobSecret.toString('hex'));
- * // OK
- * ```
- * @since v0.11.14
- */
- class ECDH {
- private constructor();
- /**
- * Converts the EC Diffie-Hellman public key specified by `key` and `curve` to the
- * format specified by `format`. The `format` argument specifies point encoding
- * and can be `'compressed'`, `'uncompressed'` or `'hybrid'`. The supplied key is
- * interpreted using the specified `inputEncoding`, and the returned key is encoded
- * using the specified `outputEncoding`.
- *
- * Use {@link getCurves} to obtain a list of available curve names.
- * On recent OpenSSL releases, `openssl ecparam -list_curves` will also display
- * the name and description of each available elliptic curve.
- *
- * If `format` is not specified the point will be returned in `'uncompressed'`format.
- *
- * If the `inputEncoding` is not provided, `key` is expected to be a `Buffer`,`TypedArray`, or `DataView`.
- *
- * Example (uncompressing a key):
- *
- * ```js
- * const {
- * createECDH,
- * ECDH
- * } = await import('crypto');
- *
- * const ecdh = createECDH('secp256k1');
- * ecdh.generateKeys();
- *
- * const compressedKey = ecdh.getPublicKey('hex', 'compressed');
- *
- * const uncompressedKey = ECDH.convertKey(compressedKey,
- * 'secp256k1',
- * 'hex',
- * 'hex',
- * 'uncompressed');
- *
- * // The converted key and the uncompressed public key should be the same
- * console.log(uncompressedKey === ecdh.getPublicKey('hex'));
- * ```
- * @since v10.0.0
- * @param inputEncoding The `encoding` of the `key` string.
- * @param outputEncoding The `encoding` of the return value.
- * @param [format='uncompressed']
- */
- static convertKey(
- key: BinaryLike,
- curve: string,
- inputEncoding?: BinaryToTextEncoding,
- outputEncoding?: 'latin1' | 'hex' | 'base64' | 'base64url',
- format?: 'uncompressed' | 'compressed' | 'hybrid'
- ): Buffer | string;
- /**
- * Generates private and public EC Diffie-Hellman key values, and returns
- * the public key in the specified `format` and `encoding`. This key should be
- * transferred to the other party.
- *
- * The `format` argument specifies point encoding and can be `'compressed'` or`'uncompressed'`. If `format` is not specified, the point will be returned in`'uncompressed'` format.
- *
- * If `encoding` is provided a string is returned; otherwise a `Buffer` is returned.
- * @since v0.11.14
- * @param encoding The `encoding` of the return value.
- * @param [format='uncompressed']
- */
- generateKeys(): Buffer;
- generateKeys(encoding: BinaryToTextEncoding, format?: ECDHKeyFormat): string;
- /**
- * Computes the shared secret using `otherPublicKey` as the other
- * party's public key and returns the computed shared secret. The supplied
- * key is interpreted using specified `inputEncoding`, and the returned secret
- * is encoded using the specified `outputEncoding`.
- * If the `inputEncoding` is not
- * provided, `otherPublicKey` is expected to be a `Buffer`, `TypedArray`, or`DataView`.
- *
- * If `outputEncoding` is given a string will be returned; otherwise a `Buffer` is returned.
- *
- * `ecdh.computeSecret` will throw an`ERR_CRYPTO_ECDH_INVALID_PUBLIC_KEY` error when `otherPublicKey`lies outside of the elliptic curve. Since `otherPublicKey` is
- * usually supplied from a remote user over an insecure network,
- * be sure to handle this exception accordingly.
- * @since v0.11.14
- * @param inputEncoding The `encoding` of the `otherPublicKey` string.
- * @param outputEncoding The `encoding` of the return value.
- */
- computeSecret(otherPublicKey: NodeJS.ArrayBufferView): Buffer;
- computeSecret(otherPublicKey: string, inputEncoding: BinaryToTextEncoding): Buffer;
- computeSecret(otherPublicKey: NodeJS.ArrayBufferView, outputEncoding: BinaryToTextEncoding): string;
- computeSecret(otherPublicKey: string, inputEncoding: BinaryToTextEncoding, outputEncoding: BinaryToTextEncoding): string;
- /**
- * If `encoding` is specified, a string is returned; otherwise a `Buffer` is
- * returned.
- * @since v0.11.14
- * @param encoding The `encoding` of the return value.
- * @return The EC Diffie-Hellman in the specified `encoding`.
- */
- getPrivateKey(): Buffer;
- getPrivateKey(encoding: BinaryToTextEncoding): string;
- /**
- * The `format` argument specifies point encoding and can be `'compressed'` or`'uncompressed'`. If `format` is not specified the point will be returned in`'uncompressed'` format.
- *
- * If `encoding` is specified, a string is returned; otherwise a `Buffer` is
- * returned.
- * @since v0.11.14
- * @param [encoding] The `encoding` of the return value.
- * @param [format='uncompressed']
- * @return The EC Diffie-Hellman public key in the specified `encoding` and `format`.
- */
- getPublicKey(encoding?: null, format?: ECDHKeyFormat): Buffer;
- getPublicKey(encoding: BinaryToTextEncoding, format?: ECDHKeyFormat): string;
- /**
- * Sets the EC Diffie-Hellman private key.
- * If `encoding` is provided, `privateKey` is expected
- * to be a string; otherwise `privateKey` is expected to be a `Buffer`,`TypedArray`, or `DataView`.
- *
- * If `privateKey` is not valid for the curve specified when the `ECDH` object was
- * created, an error is thrown. Upon setting the private key, the associated
- * public point (key) is also generated and set in the `ECDH` object.
- * @since v0.11.14
- * @param encoding The `encoding` of the `privateKey` string.
- */
- setPrivateKey(privateKey: NodeJS.ArrayBufferView): void;
- setPrivateKey(privateKey: string, encoding: BinaryToTextEncoding): void;
- }
- /**
- * Creates an Elliptic Curve Diffie-Hellman (`ECDH`) key exchange object using a
- * predefined curve specified by the `curveName` string. Use {@link getCurves} to obtain a list of available curve names. On recent
- * OpenSSL releases, `openssl ecparam -list_curves` will also display the name
- * and description of each available elliptic curve.
- * @since v0.11.14
- */
- function createECDH(curveName: string): ECDH;
- /**
- * This function is based on a constant-time algorithm.
- * Returns true if `a` is equal to `b`, without leaking timing information that
- * would allow an attacker to guess one of the values. This is suitable for
- * comparing HMAC digests or secret values like authentication cookies or [capability urls](https://www.w3.org/TR/capability-urls/).
- *
- * `a` and `b` must both be `Buffer`s, `TypedArray`s, or `DataView`s, and they
- * must have the same byte length. An error is thrown if `a` and `b` have
- * different byte lengths.
- *
- * If at least one of `a` and `b` is a `TypedArray` with more than one byte per
- * entry, such as `Uint16Array`, the result will be computed using the platform
- * byte order.
- *
- * Use of `crypto.timingSafeEqual` does not guarantee that the _surrounding_ code
- * is timing-safe. Care should be taken to ensure that the surrounding code does
- * not introduce timing vulnerabilities.
- * @since v6.6.0
- */
- function timingSafeEqual(a: NodeJS.ArrayBufferView, b: NodeJS.ArrayBufferView): boolean;
- /** @deprecated since v10.0.0 */
- const DEFAULT_ENCODING: BufferEncoding;
- type KeyType = 'rsa' | 'rsa-pss' | 'dsa' | 'ec' | 'ed25519' | 'ed448' | 'x25519' | 'x448';
- type KeyFormat = 'pem' | 'der' | 'jwk';
- interface BasePrivateKeyEncodingOptions {
- format: T;
- cipher?: string | undefined;
- passphrase?: string | undefined;
- }
- interface KeyPairKeyObjectResult {
- publicKey: KeyObject;
- privateKey: KeyObject;
- }
- interface ED25519KeyPairKeyObjectOptions {}
- interface ED448KeyPairKeyObjectOptions {}
- interface X25519KeyPairKeyObjectOptions {}
- interface X448KeyPairKeyObjectOptions {}
- interface ECKeyPairKeyObjectOptions {
- /**
- * Name of the curve to use
- */
- namedCurve: string;
- }
- interface RSAKeyPairKeyObjectOptions {
- /**
- * Key size in bits
- */
- modulusLength: number;
- /**
- * Public exponent
- * @default 0x10001
- */
- publicExponent?: number | undefined;
- }
- interface RSAPSSKeyPairKeyObjectOptions {
- /**
- * Key size in bits
- */
- modulusLength: number;
- /**
- * Public exponent
- * @default 0x10001
- */
- publicExponent?: number | undefined;
- /**
- * Name of the message digest
- */
- hashAlgorithm?: string;
- /**
- * Name of the message digest used by MGF1
- */
- mgf1HashAlgorithm?: string;
- /**
- * Minimal salt length in bytes
- */
- saltLength?: string;
- }
- interface DSAKeyPairKeyObjectOptions {
- /**
- * Key size in bits
- */
- modulusLength: number;
- /**
- * Size of q in bits
- */
- divisorLength: number;
- }
- interface RSAKeyPairOptions {
- /**
- * Key size in bits
- */
- modulusLength: number;
- /**
- * Public exponent
- * @default 0x10001
- */
- publicExponent?: number | undefined;
- publicKeyEncoding: {
- type: 'pkcs1' | 'spki';
- format: PubF;
- };
- privateKeyEncoding: BasePrivateKeyEncodingOptions & {
- type: 'pkcs1' | 'pkcs8';
- };
- }
- interface RSAPSSKeyPairOptions {
- /**
- * Key size in bits
- */
- modulusLength: number;
- /**
- * Public exponent
- * @default 0x10001
- */
- publicExponent?: number | undefined;
- /**
- * Name of the message digest
- */
- hashAlgorithm?: string;
- /**
- * Name of the message digest used by MGF1
- */
- mgf1HashAlgorithm?: string;
- /**
- * Minimal salt length in bytes
- */
- saltLength?: string;
- publicKeyEncoding: {
- type: 'spki';
- format: PubF;
- };
- privateKeyEncoding: BasePrivateKeyEncodingOptions & {
- type: 'pkcs8';
- };
- }
- interface DSAKeyPairOptions {
- /**
- * Key size in bits
- */
- modulusLength: number;
- /**
- * Size of q in bits
- */
- divisorLength: number;
- publicKeyEncoding: {
- type: 'spki';
- format: PubF;
- };
- privateKeyEncoding: BasePrivateKeyEncodingOptions & {
- type: 'pkcs8';
- };
- }
- interface ECKeyPairOptions {
- /**
- * Name of the curve to use.
- */
- namedCurve: string;
- publicKeyEncoding: {
- type: 'pkcs1' | 'spki';
- format: PubF;
- };
- privateKeyEncoding: BasePrivateKeyEncodingOptions & {
- type: 'sec1' | 'pkcs8';
- };
- }
- interface ED25519KeyPairOptions {
- publicKeyEncoding: {
- type: 'spki';
- format: PubF;
- };
- privateKeyEncoding: BasePrivateKeyEncodingOptions & {
- type: 'pkcs8';
- };
- }
- interface ED448KeyPairOptions {
- publicKeyEncoding: {
- type: 'spki';
- format: PubF;
- };
- privateKeyEncoding: BasePrivateKeyEncodingOptions & {
- type: 'pkcs8';
- };
- }
- interface X25519KeyPairOptions {
- publicKeyEncoding: {
- type: 'spki';
- format: PubF;
- };
- privateKeyEncoding: BasePrivateKeyEncodingOptions & {
- type: 'pkcs8';
- };
- }
- interface X448KeyPairOptions {
- publicKeyEncoding: {
- type: 'spki';
- format: PubF;
- };
- privateKeyEncoding: BasePrivateKeyEncodingOptions & {
- type: 'pkcs8';
- };
- }
- interface KeyPairSyncResult {
- publicKey: T1;
- privateKey: T2;
- }
- /**
- * Generates a new asymmetric key pair of the given `type`. RSA, RSA-PSS, DSA, EC,
- * Ed25519, Ed448, X25519, X448, and DH are currently supported.
- *
- * If a `publicKeyEncoding` or `privateKeyEncoding` was specified, this function
- * behaves as if `keyObject.export()` had been called on its result. Otherwise,
- * the respective part of the key is returned as a `KeyObject`.
- *
- * When encoding public keys, it is recommended to use `'spki'`. When encoding
- * private keys, it is recommended to use `'pkcs8'` with a strong passphrase,
- * and to keep the passphrase confidential.
- *
- * ```js
- * const {
- * generateKeyPairSync
- * } = await import('crypto');
- *
- * const {
- * publicKey,
- * privateKey,
- * } = generateKeyPairSync('rsa', {
- * modulusLength: 4096,
- * publicKeyEncoding: {
- * type: 'spki',
- * format: 'pem'
- * },
- * privateKeyEncoding: {
- * type: 'pkcs8',
- * format: 'pem',
- * cipher: 'aes-256-cbc',
- * passphrase: 'top secret'
- * }
- * });
- * ```
- *
- * The return value `{ publicKey, privateKey }` represents the generated key pair.
- * When PEM encoding was selected, the respective key will be a string, otherwise
- * it will be a buffer containing the data encoded as DER.
- * @since v10.12.0
- * @param type Must be `'rsa'`, `'rsa-pss'`, `'dsa'`, `'ec'`, `'ed25519'`, `'ed448'`, `'x25519'`, `'x448'`, or `'dh'`.
- */
- function generateKeyPairSync(type: 'rsa', options: RSAKeyPairOptions<'pem', 'pem'>): KeyPairSyncResult;
- function generateKeyPairSync(type: 'rsa', options: RSAKeyPairOptions<'pem', 'der'>): KeyPairSyncResult;
- function generateKeyPairSync(type: 'rsa', options: RSAKeyPairOptions<'der', 'pem'>): KeyPairSyncResult;
- function generateKeyPairSync(type: 'rsa', options: RSAKeyPairOptions<'der', 'der'>): KeyPairSyncResult;
- function generateKeyPairSync(type: 'rsa', options: RSAKeyPairKeyObjectOptions): KeyPairKeyObjectResult;
- function generateKeyPairSync(type: 'rsa-pss', options: RSAPSSKeyPairOptions<'pem', 'pem'>): KeyPairSyncResult;
- function generateKeyPairSync(type: 'rsa-pss', options: RSAPSSKeyPairOptions<'pem', 'der'>): KeyPairSyncResult;
- function generateKeyPairSync(type: 'rsa-pss', options: RSAPSSKeyPairOptions<'der', 'pem'>): KeyPairSyncResult;
- function generateKeyPairSync(type: 'rsa-pss', options: RSAPSSKeyPairOptions<'der', 'der'>): KeyPairSyncResult;
- function generateKeyPairSync(type: 'rsa-pss', options: RSAPSSKeyPairKeyObjectOptions): KeyPairKeyObjectResult;
- function generateKeyPairSync(type: 'dsa', options: DSAKeyPairOptions<'pem', 'pem'>): KeyPairSyncResult;
- function generateKeyPairSync(type: 'dsa', options: DSAKeyPairOptions<'pem', 'der'>): KeyPairSyncResult;
- function generateKeyPairSync(type: 'dsa', options: DSAKeyPairOptions<'der', 'pem'>): KeyPairSyncResult;
- function generateKeyPairSync(type: 'dsa', options: DSAKeyPairOptions<'der', 'der'>): KeyPairSyncResult;
- function generateKeyPairSync(type: 'dsa', options: DSAKeyPairKeyObjectOptions): KeyPairKeyObjectResult;
- function generateKeyPairSync(type: 'ec', options: ECKeyPairOptions<'pem', 'pem'>): KeyPairSyncResult;
- function generateKeyPairSync(type: 'ec', options: ECKeyPairOptions<'pem', 'der'>): KeyPairSyncResult;
- function generateKeyPairSync(type: 'ec', options: ECKeyPairOptions<'der', 'pem'>): KeyPairSyncResult;
- function generateKeyPairSync(type: 'ec', options: ECKeyPairOptions<'der', 'der'>): KeyPairSyncResult;
- function generateKeyPairSync(type: 'ec', options: ECKeyPairKeyObjectOptions): KeyPairKeyObjectResult;
- function generateKeyPairSync(type: 'ed25519', options: ED25519KeyPairOptions<'pem', 'pem'>): KeyPairSyncResult;
- function generateKeyPairSync(type: 'ed25519', options: ED25519KeyPairOptions<'pem', 'der'>): KeyPairSyncResult;
- function generateKeyPairSync(type: 'ed25519', options: ED25519KeyPairOptions<'der', 'pem'>): KeyPairSyncResult;
- function generateKeyPairSync(type: 'ed25519', options: ED25519KeyPairOptions<'der', 'der'>): KeyPairSyncResult;
- function generateKeyPairSync(type: 'ed25519', options?: ED25519KeyPairKeyObjectOptions): KeyPairKeyObjectResult;
- function generateKeyPairSync(type: 'ed448', options: ED448KeyPairOptions<'pem', 'pem'>): KeyPairSyncResult;
- function generateKeyPairSync(type: 'ed448', options: ED448KeyPairOptions<'pem', 'der'>): KeyPairSyncResult;
- function generateKeyPairSync(type: 'ed448', options: ED448KeyPairOptions<'der', 'pem'>): KeyPairSyncResult;
- function generateKeyPairSync(type: 'ed448', options: ED448KeyPairOptions<'der', 'der'>): KeyPairSyncResult;
- function generateKeyPairSync(type: 'ed448', options?: ED448KeyPairKeyObjectOptions): KeyPairKeyObjectResult;
- function generateKeyPairSync(type: 'x25519', options: X25519KeyPairOptions<'pem', 'pem'>): KeyPairSyncResult;
- function generateKeyPairSync(type: 'x25519', options: X25519KeyPairOptions<'pem', 'der'>): KeyPairSyncResult;
- function generateKeyPairSync(type: 'x25519', options: X25519KeyPairOptions<'der', 'pem'>): KeyPairSyncResult;
- function generateKeyPairSync(type: 'x25519', options: X25519KeyPairOptions<'der', 'der'>): KeyPairSyncResult;
- function generateKeyPairSync(type: 'x25519', options?: X25519KeyPairKeyObjectOptions): KeyPairKeyObjectResult;
- function generateKeyPairSync(type: 'x448', options: X448KeyPairOptions<'pem', 'pem'>): KeyPairSyncResult;
- function generateKeyPairSync(type: 'x448', options: X448KeyPairOptions<'pem', 'der'>): KeyPairSyncResult;
- function generateKeyPairSync(type: 'x448', options: X448KeyPairOptions<'der', 'pem'>): KeyPairSyncResult;
- function generateKeyPairSync(type: 'x448', options: X448KeyPairOptions<'der', 'der'>): KeyPairSyncResult;
- function generateKeyPairSync(type: 'x448', options?: X448KeyPairKeyObjectOptions): KeyPairKeyObjectResult;
- /**
- * Generates a new asymmetric key pair of the given `type`. RSA, RSA-PSS, DSA, EC,
- * Ed25519, Ed448, X25519, X448, and DH are currently supported.
- *
- * If a `publicKeyEncoding` or `privateKeyEncoding` was specified, this function
- * behaves as if `keyObject.export()` had been called on its result. Otherwise,
- * the respective part of the key is returned as a `KeyObject`.
- *
- * It is recommended to encode public keys as `'spki'` and private keys as`'pkcs8'` with encryption for long-term storage:
- *
- * ```js
- * const {
- * generateKeyPair
- * } = await import('crypto');
- *
- * generateKeyPair('rsa', {
- * modulusLength: 4096,
- * publicKeyEncoding: {
- * type: 'spki',
- * format: 'pem'
- * },
- * privateKeyEncoding: {
- * type: 'pkcs8',
- * format: 'pem',
- * cipher: 'aes-256-cbc',
- * passphrase: 'top secret'
- * }
- * }, (err, publicKey, privateKey) => {
- * // Handle errors and use the generated key pair.
- * });
- * ```
- *
- * On completion, `callback` will be called with `err` set to `undefined` and`publicKey` / `privateKey` representing the generated key pair.
- *
- * If this method is invoked as its `util.promisify()` ed version, it returns
- * a `Promise` for an `Object` with `publicKey` and `privateKey` properties.
- * @since v10.12.0
- * @param type Must be `'rsa'`, `'rsa-pss'`, `'dsa'`, `'ec'`, `'ed25519'`, `'ed448'`, `'x25519'`, `'x448'`, or `'dh'`.
- */
- function generateKeyPair(type: 'rsa', options: RSAKeyPairOptions<'pem', 'pem'>, callback: (err: Error | null, publicKey: string, privateKey: string) => void): void;
- function generateKeyPair(type: 'rsa', options: RSAKeyPairOptions<'pem', 'der'>, callback: (err: Error | null, publicKey: string, privateKey: Buffer) => void): void;
- function generateKeyPair(type: 'rsa', options: RSAKeyPairOptions<'der', 'pem'>, callback: (err: Error | null, publicKey: Buffer, privateKey: string) => void): void;
- function generateKeyPair(type: 'rsa', options: RSAKeyPairOptions<'der', 'der'>, callback: (err: Error | null, publicKey: Buffer, privateKey: Buffer) => void): void;
- function generateKeyPair(type: 'rsa', options: RSAKeyPairKeyObjectOptions, callback: (err: Error | null, publicKey: KeyObject, privateKey: KeyObject) => void): void;
- function generateKeyPair(type: 'rsa-pss', options: RSAPSSKeyPairOptions<'pem', 'pem'>, callback: (err: Error | null, publicKey: string, privateKey: string) => void): void;
- function generateKeyPair(type: 'rsa-pss', options: RSAPSSKeyPairOptions<'pem', 'der'>, callback: (err: Error | null, publicKey: string, privateKey: Buffer) => void): void;
- function generateKeyPair(type: 'rsa-pss', options: RSAPSSKeyPairOptions<'der', 'pem'>, callback: (err: Error | null, publicKey: Buffer, privateKey: string) => void): void;
- function generateKeyPair(type: 'rsa-pss', options: RSAPSSKeyPairOptions<'der', 'der'>, callback: (err: Error | null, publicKey: Buffer, privateKey: Buffer) => void): void;
- function generateKeyPair(type: 'rsa-pss', options: RSAPSSKeyPairKeyObjectOptions, callback: (err: Error | null, publicKey: KeyObject, privateKey: KeyObject) => void): void;
- function generateKeyPair(type: 'dsa', options: DSAKeyPairOptions<'pem', 'pem'>, callback: (err: Error | null, publicKey: string, privateKey: string) => void): void;
- function generateKeyPair(type: 'dsa', options: DSAKeyPairOptions<'pem', 'der'>, callback: (err: Error | null, publicKey: string, privateKey: Buffer) => void): void;
- function generateKeyPair(type: 'dsa', options: DSAKeyPairOptions<'der', 'pem'>, callback: (err: Error | null, publicKey: Buffer, privateKey: string) => void): void;
- function generateKeyPair(type: 'dsa', options: DSAKeyPairOptions<'der', 'der'>, callback: (err: Error | null, publicKey: Buffer, privateKey: Buffer) => void): void;
- function generateKeyPair(type: 'dsa', options: DSAKeyPairKeyObjectOptions, callback: (err: Error | null, publicKey: KeyObject, privateKey: KeyObject) => void): void;
- function generateKeyPair(type: 'ec', options: ECKeyPairOptions<'pem', 'pem'>, callback: (err: Error | null, publicKey: string, privateKey: string) => void): void;
- function generateKeyPair(type: 'ec', options: ECKeyPairOptions<'pem', 'der'>, callback: (err: Error | null, publicKey: string, privateKey: Buffer) => void): void;
- function generateKeyPair(type: 'ec', options: ECKeyPairOptions<'der', 'pem'>, callback: (err: Error | null, publicKey: Buffer, privateKey: string) => void): void;
- function generateKeyPair(type: 'ec', options: ECKeyPairOptions<'der', 'der'>, callback: (err: Error | null, publicKey: Buffer, privateKey: Buffer) => void): void;
- function generateKeyPair(type: 'ec', options: ECKeyPairKeyObjectOptions, callback: (err: Error | null, publicKey: KeyObject, privateKey: KeyObject) => void): void;
- function generateKeyPair(type: 'ed25519', options: ED25519KeyPairOptions<'pem', 'pem'>, callback: (err: Error | null, publicKey: string, privateKey: string) => void): void;
- function generateKeyPair(type: 'ed25519', options: ED25519KeyPairOptions<'pem', 'der'>, callback: (err: Error | null, publicKey: string, privateKey: Buffer) => void): void;
- function generateKeyPair(type: 'ed25519', options: ED25519KeyPairOptions<'der', 'pem'>, callback: (err: Error | null, publicKey: Buffer, privateKey: string) => void): void;
- function generateKeyPair(type: 'ed25519', options: ED25519KeyPairOptions<'der', 'der'>, callback: (err: Error | null, publicKey: Buffer, privateKey: Buffer) => void): void;
- function generateKeyPair(type: 'ed25519', options: ED25519KeyPairKeyObjectOptions | undefined, callback: (err: Error | null, publicKey: KeyObject, privateKey: KeyObject) => void): void;
- function generateKeyPair(type: 'ed448', options: ED448KeyPairOptions<'pem', 'pem'>, callback: (err: Error | null, publicKey: string, privateKey: string) => void): void;
- function generateKeyPair(type: 'ed448', options: ED448KeyPairOptions<'pem', 'der'>, callback: (err: Error | null, publicKey: string, privateKey: Buffer) => void): void;
- function generateKeyPair(type: 'ed448', options: ED448KeyPairOptions<'der', 'pem'>, callback: (err: Error | null, publicKey: Buffer, privateKey: string) => void): void;
- function generateKeyPair(type: 'ed448', options: ED448KeyPairOptions<'der', 'der'>, callback: (err: Error | null, publicKey: Buffer, privateKey: Buffer) => void): void;
- function generateKeyPair(type: 'ed448', options: ED448KeyPairKeyObjectOptions | undefined, callback: (err: Error | null, publicKey: KeyObject, privateKey: KeyObject) => void): void;
- function generateKeyPair(type: 'x25519', options: X25519KeyPairOptions<'pem', 'pem'>, callback: (err: Error | null, publicKey: string, privateKey: string) => void): void;
- function generateKeyPair(type: 'x25519', options: X25519KeyPairOptions<'pem', 'der'>, callback: (err: Error | null, publicKey: string, privateKey: Buffer) => void): void;
- function generateKeyPair(type: 'x25519', options: X25519KeyPairOptions<'der', 'pem'>, callback: (err: Error | null, publicKey: Buffer, privateKey: string) => void): void;
- function generateKeyPair(type: 'x25519', options: X25519KeyPairOptions<'der', 'der'>, callback: (err: Error | null, publicKey: Buffer, privateKey: Buffer) => void): void;
- function generateKeyPair(type: 'x25519', options: X25519KeyPairKeyObjectOptions | undefined, callback: (err: Error | null, publicKey: KeyObject, privateKey: KeyObject) => void): void;
- function generateKeyPair(type: 'x448', options: X448KeyPairOptions<'pem', 'pem'>, callback: (err: Error | null, publicKey: string, privateKey: string) => void): void;
- function generateKeyPair(type: 'x448', options: X448KeyPairOptions<'pem', 'der'>, callback: (err: Error | null, publicKey: string, privateKey: Buffer) => void): void;
- function generateKeyPair(type: 'x448', options: X448KeyPairOptions<'der', 'pem'>, callback: (err: Error | null, publicKey: Buffer, privateKey: string) => void): void;
- function generateKeyPair(type: 'x448', options: X448KeyPairOptions<'der', 'der'>, callback: (err: Error | null, publicKey: Buffer, privateKey: Buffer) => void): void;
- function generateKeyPair(type: 'x448', options: X448KeyPairKeyObjectOptions | undefined, callback: (err: Error | null, publicKey: KeyObject, privateKey: KeyObject) => void): void;
- namespace generateKeyPair {
- function __promisify__(
- type: 'rsa',
- options: RSAKeyPairOptions<'pem', 'pem'>
- ): Promise<{
- publicKey: string;
- privateKey: string;
- }>;
- function __promisify__(
- type: 'rsa',
- options: RSAKeyPairOptions<'pem', 'der'>
- ): Promise<{
- publicKey: string;
- privateKey: Buffer;
- }>;
- function __promisify__(
- type: 'rsa',
- options: RSAKeyPairOptions<'der', 'pem'>
- ): Promise<{
- publicKey: Buffer;
- privateKey: string;
- }>;
- function __promisify__(
- type: 'rsa',
- options: RSAKeyPairOptions<'der', 'der'>
- ): Promise<{
- publicKey: Buffer;
- privateKey: Buffer;
- }>;
- function __promisify__(type: 'rsa', options: RSAKeyPairKeyObjectOptions): Promise;
- function __promisify__(
- type: 'rsa-pss',
- options: RSAPSSKeyPairOptions<'pem', 'pem'>
- ): Promise<{
- publicKey: string;
- privateKey: string;
- }>;
- function __promisify__(
- type: 'rsa-pss',
- options: RSAPSSKeyPairOptions<'pem', 'der'>
- ): Promise<{
- publicKey: string;
- privateKey: Buffer;
- }>;
- function __promisify__(
- type: 'rsa-pss',
- options: RSAPSSKeyPairOptions<'der', 'pem'>
- ): Promise<{
- publicKey: Buffer;
- privateKey: string;
- }>;
- function __promisify__(
- type: 'rsa-pss',
- options: RSAPSSKeyPairOptions<'der', 'der'>
- ): Promise<{
- publicKey: Buffer;
- privateKey: Buffer;
- }>;
- function __promisify__(type: 'rsa-pss', options: RSAPSSKeyPairKeyObjectOptions): Promise;
- function __promisify__(
- type: 'dsa',
- options: DSAKeyPairOptions<'pem', 'pem'>
- ): Promise<{
- publicKey: string;
- privateKey: string;
- }>;
- function __promisify__(
- type: 'dsa',
- options: DSAKeyPairOptions<'pem', 'der'>
- ): Promise<{
- publicKey: string;
- privateKey: Buffer;
- }>;
- function __promisify__(
- type: 'dsa',
- options: DSAKeyPairOptions<'der', 'pem'>
- ): Promise<{
- publicKey: Buffer;
- privateKey: string;
- }>;
- function __promisify__(
- type: 'dsa',
- options: DSAKeyPairOptions<'der', 'der'>
- ): Promise<{
- publicKey: Buffer;
- privateKey: Buffer;
- }>;
- function __promisify__(type: 'dsa', options: DSAKeyPairKeyObjectOptions): Promise;
- function __promisify__(
- type: 'ec',
- options: ECKeyPairOptions<'pem', 'pem'>
- ): Promise<{
- publicKey: string;
- privateKey: string;
- }>;
- function __promisify__(
- type: 'ec',
- options: ECKeyPairOptions<'pem', 'der'>
- ): Promise<{
- publicKey: string;
- privateKey: Buffer;
- }>;
- function __promisify__(
- type: 'ec',
- options: ECKeyPairOptions<'der', 'pem'>
- ): Promise<{
- publicKey: Buffer;
- privateKey: string;
- }>;
- function __promisify__(
- type: 'ec',
- options: ECKeyPairOptions<'der', 'der'>
- ): Promise<{
- publicKey: Buffer;
- privateKey: Buffer;
- }>;
- function __promisify__(type: 'ec', options: ECKeyPairKeyObjectOptions): Promise;
- function __promisify__(
- type: 'ed25519',
- options: ED25519KeyPairOptions<'pem', 'pem'>
- ): Promise<{
- publicKey: string;
- privateKey: string;
- }>;
- function __promisify__(
- type: 'ed25519',
- options: ED25519KeyPairOptions<'pem', 'der'>
- ): Promise<{
- publicKey: string;
- privateKey: Buffer;
- }>;
- function __promisify__(
- type: 'ed25519',
- options: ED25519KeyPairOptions<'der', 'pem'>
- ): Promise<{
- publicKey: Buffer;
- privateKey: string;
- }>;
- function __promisify__(
- type: 'ed25519',
- options: ED25519KeyPairOptions<'der', 'der'>
- ): Promise<{
- publicKey: Buffer;
- privateKey: Buffer;
- }>;
- function __promisify__(type: 'ed25519', options?: ED25519KeyPairKeyObjectOptions): Promise;
- function __promisify__(
- type: 'ed448',
- options: ED448KeyPairOptions<'pem', 'pem'>
- ): Promise<{
- publicKey: string;
- privateKey: string;
- }>;
- function __promisify__(
- type: 'ed448',
- options: ED448KeyPairOptions<'pem', 'der'>
- ): Promise<{
- publicKey: string;
- privateKey: Buffer;
- }>;
- function __promisify__(
- type: 'ed448',
- options: ED448KeyPairOptions<'der', 'pem'>
- ): Promise<{
- publicKey: Buffer;
- privateKey: string;
- }>;
- function __promisify__(
- type: 'ed448',
- options: ED448KeyPairOptions<'der', 'der'>
- ): Promise<{
- publicKey: Buffer;
- privateKey: Buffer;
- }>;
- function __promisify__(type: 'ed448', options?: ED448KeyPairKeyObjectOptions): Promise;
- function __promisify__(
- type: 'x25519',
- options: X25519KeyPairOptions<'pem', 'pem'>
- ): Promise<{
- publicKey: string;
- privateKey: string;
- }>;
- function __promisify__(
- type: 'x25519',
- options: X25519KeyPairOptions<'pem', 'der'>
- ): Promise<{
- publicKey: string;
- privateKey: Buffer;
- }>;
- function __promisify__(
- type: 'x25519',
- options: X25519KeyPairOptions<'der', 'pem'>
- ): Promise<{
- publicKey: Buffer;
- privateKey: string;
- }>;
- function __promisify__(
- type: 'x25519',
- options: X25519KeyPairOptions<'der', 'der'>
- ): Promise<{
- publicKey: Buffer;
- privateKey: Buffer;
- }>;
- function __promisify__(type: 'x25519', options?: X25519KeyPairKeyObjectOptions): Promise;
- function __promisify__(
- type: 'x448',
- options: X448KeyPairOptions<'pem', 'pem'>
- ): Promise<{
- publicKey: string;
- privateKey: string;
- }>;
- function __promisify__(
- type: 'x448',
- options: X448KeyPairOptions<'pem', 'der'>
- ): Promise<{
- publicKey: string;
- privateKey: Buffer;
- }>;
- function __promisify__(
- type: 'x448',
- options: X448KeyPairOptions<'der', 'pem'>
- ): Promise<{
- publicKey: Buffer;
- privateKey: string;
- }>;
- function __promisify__(
- type: 'x448',
- options: X448KeyPairOptions<'der', 'der'>
- ): Promise<{
- publicKey: Buffer;
- privateKey: Buffer;
- }>;
- function __promisify__(type: 'x448', options?: X448KeyPairKeyObjectOptions): Promise;
- }
- /**
- * Calculates and returns the signature for `data` using the given private key and
- * algorithm. If `algorithm` is `null` or `undefined`, then the algorithm is
- * dependent upon the key type (especially Ed25519 and Ed448).
- *
- * If `key` is not a `KeyObject`, this function behaves as if `key` had been
- * passed to {@link createPrivateKey}. If it is an object, the following
- * additional properties can be passed:
- *
- * If the `callback` function is provided this function uses libuv's threadpool.
- * @since v12.0.0
- */
- function sign(algorithm: string | null | undefined, data: NodeJS.ArrayBufferView, key: KeyLike | SignKeyObjectInput | SignPrivateKeyInput): Buffer;
- function sign(
- algorithm: string | null | undefined,
- data: NodeJS.ArrayBufferView,
- key: KeyLike | SignKeyObjectInput | SignPrivateKeyInput,
- callback: (error: Error | null, data: Buffer) => void
- ): void;
- /**
- * Verifies the given signature for `data` using the given key and algorithm. If`algorithm` is `null` or `undefined`, then the algorithm is dependent upon the
- * key type (especially Ed25519 and Ed448).
- *
- * If `key` is not a `KeyObject`, this function behaves as if `key` had been
- * passed to {@link createPublicKey}. If it is an object, the following
- * additional properties can be passed:
- *
- * The `signature` argument is the previously calculated signature for the `data`.
- *
- * Because public keys can be derived from private keys, a private key or a public
- * key may be passed for `key`.
- *
- * If the `callback` function is provided this function uses libuv's threadpool.
- * @since v12.0.0
- */
- function verify(algorithm: string | null | undefined, data: NodeJS.ArrayBufferView, key: KeyLike | VerifyKeyObjectInput | VerifyPublicKeyInput, signature: NodeJS.ArrayBufferView): boolean;
- function verify(
- algorithm: string | null | undefined,
- data: NodeJS.ArrayBufferView,
- key: KeyLike | VerifyKeyObjectInput | VerifyPublicKeyInput,
- signature: NodeJS.ArrayBufferView,
- callback: (error: Error | null, result: boolean) => void
- ): void;
- /**
- * Computes the Diffie-Hellman secret based on a `privateKey` and a `publicKey`.
- * Both keys must have the same `asymmetricKeyType`, which must be one of `'dh'`(for Diffie-Hellman), `'ec'` (for ECDH), `'x448'`, or `'x25519'` (for ECDH-ES).
- * @since v13.9.0, v12.17.0
- */
- function diffieHellman(options: { privateKey: KeyObject; publicKey: KeyObject }): Buffer;
- type CipherMode = 'cbc' | 'ccm' | 'cfb' | 'ctr' | 'ecb' | 'gcm' | 'ocb' | 'ofb' | 'stream' | 'wrap' | 'xts';
- interface CipherInfoOptions {
- /**
- * A test key length.
- */
- keyLength?: number | undefined;
- /**
- * A test IV length.
- */
- ivLength?: number | undefined;
- }
- interface CipherInfo {
- /**
- * The name of the cipher.
- */
- name: string;
- /**
- * The nid of the cipher.
- */
- nid: number;
- /**
- * The block size of the cipher in bytes.
- * This property is omitted when mode is 'stream'.
- */
- blockSize?: number | undefined;
- /**
- * The expected or default initialization vector length in bytes.
- * This property is omitted if the cipher does not use an initialization vector.
- */
- ivLength?: number | undefined;
- /**
- * The expected or default key length in bytes.
- */
- keyLength: number;
- /**
- * The cipher mode.
- */
- mode: CipherMode;
- }
- /**
- * Returns information about a given cipher.
- *
- * Some ciphers accept variable length keys and initialization vectors. By default,
- * the `crypto.getCipherInfo()` method will return the default values for these
- * ciphers. To test if a given key length or iv length is acceptable for given
- * cipher, use the `keyLength` and `ivLength` options. If the given values are
- * unacceptable, `undefined` will be returned.
- * @since v15.0.0
- * @param nameOrNid The name or nid of the cipher to query.
- */
- function getCipherInfo(nameOrNid: string | number, options?: CipherInfoOptions): CipherInfo | undefined;
- /**
- * HKDF is a simple key derivation function defined in RFC 5869\. The given `ikm`,`salt` and `info` are used with the `digest` to derive a key of `keylen` bytes.
- *
- * The supplied `callback` function is called with two arguments: `err` and`derivedKey`. If an errors occurs while deriving the key, `err` will be set;
- * otherwise `err` will be `null`. The successfully generated `derivedKey` will
- * be passed to the callback as an [ArrayBuffer](https://developer.mozilla.org/en-US/docs/Web/JavaScript/Reference/Global_Objects/ArrayBuffer). An error will be thrown if any
- * of the input arguments specify invalid values or types.
- *
- * ```js
- * import { Buffer } from 'buffer';
- * const {
- * hkdf
- * } = await import('crypto');
- *
- * hkdf('sha512', 'key', 'salt', 'info', 64, (err, derivedKey) => {
- * if (err) throw err;
- * console.log(Buffer.from(derivedKey).toString('hex')); // '24156e2...5391653'
- * });
- * ```
- * @since v15.0.0
- * @param digest The digest algorithm to use.
- * @param ikm The input keying material. It must be at least one byte in length.
- * @param salt The salt value. Must be provided but can be zero-length.
- * @param info Additional info value. Must be provided but can be zero-length, and cannot be more than 1024 bytes.
- * @param keylen The length of the key to generate. Must be greater than 0. The maximum allowable value is `255` times the number of bytes produced by the selected digest function (e.g. `sha512`
- * generates 64-byte hashes, making the maximum HKDF output 16320 bytes).
- */
- function hkdf(digest: string, irm: BinaryLike | KeyObject, salt: BinaryLike, info: BinaryLike, keylen: number, callback: (err: Error | null, derivedKey: ArrayBuffer) => void): void;
- /**
- * Provides a synchronous HKDF key derivation function as defined in RFC 5869\. The
- * given `ikm`, `salt` and `info` are used with the `digest` to derive a key of`keylen` bytes.
- *
- * The successfully generated `derivedKey` will be returned as an [ArrayBuffer](https://developer.mozilla.org/en-US/docs/Web/JavaScript/Reference/Global_Objects/ArrayBuffer).
- *
- * An error will be thrown if any of the input arguments specify invalid values or
- * types, or if the derived key cannot be generated.
- *
- * ```js
- * import { Buffer } from 'buffer';
- * const {
- * hkdfSync
- * } = await import('crypto');
- *
- * const derivedKey = hkdfSync('sha512', 'key', 'salt', 'info', 64);
- * console.log(Buffer.from(derivedKey).toString('hex')); // '24156e2...5391653'
- * ```
- * @since v15.0.0
- * @param digest The digest algorithm to use.
- * @param ikm The input keying material. It must be at least one byte in length.
- * @param salt The salt value. Must be provided but can be zero-length.
- * @param info Additional info value. Must be provided but can be zero-length, and cannot be more than 1024 bytes.
- * @param keylen The length of the key to generate. Must be greater than 0. The maximum allowable value is `255` times the number of bytes produced by the selected digest function (e.g. `sha512`
- * generates 64-byte hashes, making the maximum HKDF output 16320 bytes).
- */
- function hkdfSync(digest: string, ikm: BinaryLike | KeyObject, salt: BinaryLike, info: BinaryLike, keylen: number): ArrayBuffer;
- interface SecureHeapUsage {
- /**
- * The total allocated secure heap size as specified using the `--secure-heap=n` command-line flag.
- */
- total: number;
- /**
- * The minimum allocation from the secure heap as specified using the `--secure-heap-min` command-line flag.
- */
- min: number;
- /**
- * The total number of bytes currently allocated from the secure heap.
- */
- used: number;
- /**
- * The calculated ratio of `used` to `total` allocated bytes.
- */
- utilization: number;
- }
- /**
- * @since v15.6.0
- */
- function secureHeapUsed(): SecureHeapUsage;
- interface RandomUUIDOptions {
- /**
- * By default, to improve performance,
- * Node.js will pre-emptively generate and persistently cache enough
- * random data to generate up to 128 random UUIDs. To generate a UUID
- * without using the cache, set `disableEntropyCache` to `true`.
- *
- * @default `false`
- */
- disableEntropyCache?: boolean | undefined;
- }
- /**
- * Generates a random [RFC 4122](https://www.rfc-editor.org/rfc/rfc4122.txt) version 4 UUID. The UUID is generated using a
- * cryptographic pseudorandom number generator.
- * @since v15.6.0, v14.17.0
- */
- function randomUUID(options?: RandomUUIDOptions): string;
- interface X509CheckOptions {
- /**
- * @default 'always'
- */
- subject?: 'always' | 'default' | 'never';
- /**
- * @default true
- */
- wildcards?: boolean;
- /**
- * @default true
- */
- partialWildcards?: boolean;
- /**
- * @default false
- */
- multiLabelWildcards?: boolean;
- /**
- * @default false
- */
- singleLabelSubdomains?: boolean;
- }
- /**
- * Encapsulates an X509 certificate and provides read-only access to
- * its information.
- *
- * ```js
- * const { X509Certificate } = await import('crypto');
- *
- * const x509 = new X509Certificate('{... pem encoded cert ...}');
- *
- * console.log(x509.subject);
- * ```
- * @since v15.6.0
- */
- class X509Certificate {
- /**
- * Will be \`true\` if this is a Certificate Authority (CA) certificate.
- * @since v15.6.0
- */
- readonly ca: boolean;
- /**
- * The SHA-1 fingerprint of this certificate.
- *
- * Because SHA-1 is cryptographically broken and because the security of SHA-1 is
- * significantly worse than that of algorithms that are commonly used to sign
- * certificates, consider using `x509.fingerprint256` instead.
- * @since v15.6.0
- */
- readonly fingerprint: string;
- /**
- * The SHA-256 fingerprint of this certificate.
- * @since v15.6.0
- */
- readonly fingerprint256: string;
- /**
- * The SHA-512 fingerprint of this certificate.
- * @since v16.14.0
- */
- readonly fingerprint512: string;
- /**
- * The complete subject of this certificate.
- * @since v15.6.0
- */
- readonly subject: string;
- /**
- * The subject alternative name specified for this certificate or `undefined`
- * if not available.
- * @since v15.6.0
- */
- readonly subjectAltName: string | undefined;
- /**
- * The information access content of this certificate or `undefined` if not
- * available.
- * @since v15.6.0
- */
- readonly infoAccess: string | undefined;
- /**
- * An array detailing the key usages for this certificate.
- * @since v15.6.0
- */
- readonly keyUsage: string[];
- /**
- * The issuer identification included in this certificate.
- * @since v15.6.0
- */
- readonly issuer: string;
- /**
- * The issuer certificate or `undefined` if the issuer certificate is not
- * available.
- * @since v15.9.0
- */
- readonly issuerCertificate?: X509Certificate | undefined;
- /**
- * The public key `KeyObject` for this certificate.
- * @since v15.6.0
- */
- readonly publicKey: KeyObject;
- /**
- * A `Buffer` containing the DER encoding of this certificate.
- * @since v15.6.0
- */
- readonly raw: Buffer;
- /**
- * The serial number of this certificate.
- *
- * Serial numbers are assigned by certificate authorities and do not uniquely
- * identify certificates. Consider using `x509.fingerprint256` as a unique
- * identifier instead.
- * @since v15.6.0
- */
- readonly serialNumber: string;
- /**
- * The date/time from which this certificate is considered valid.
- * @since v15.6.0
- */
- readonly validFrom: string;
- /**
- * The date/time until which this certificate is considered valid.
- * @since v15.6.0
- */
- readonly validTo: string;
- constructor(buffer: BinaryLike);
- /**
- * Checks whether the certificate matches the given email address.
- *
- * If the `'subject'` option is undefined or set to `'default'`, the certificate
- * subject is only considered if the subject alternative name extension either does
- * not exist or does not contain any email addresses.
- *
- * If the `'subject'` option is set to `'always'` and if the subject alternative
- * name extension either does not exist or does not contain a matching email
- * address, the certificate subject is considered.
- *
- * If the `'subject'` option is set to `'never'`, the certificate subject is never
- * considered, even if the certificate contains no subject alternative names.
- * @since v15.6.0
- * @return Returns `email` if the certificate matches, `undefined` if it does not.
- */
- checkEmail(email: string, options?: Pick): string | undefined;
- /**
- * Checks whether the certificate matches the given host name.
- *
- * If the certificate matches the given host name, the matching subject name is
- * returned. The returned name might be an exact match (e.g., `foo.example.com`)
- * or it might contain wildcards (e.g., `*.example.com`). Because host name
- * comparisons are case-insensitive, the returned subject name might also differ
- * from the given `name` in capitalization.
- *
- * If the `'subject'` option is undefined or set to `'default'`, the certificate
- * subject is only considered if the subject alternative name extension either does
- * not exist or does not contain any DNS names. This behavior is consistent with [RFC 2818](https://www.rfc-editor.org/rfc/rfc2818.txt) ("HTTP Over TLS").
- *
- * If the `'subject'` option is set to `'always'` and if the subject alternative
- * name extension either does not exist or does not contain a matching DNS name,
- * the certificate subject is considered.
- *
- * If the `'subject'` option is set to `'never'`, the certificate subject is never
- * considered, even if the certificate contains no subject alternative names.
- * @since v15.6.0
- * @return Returns a subject name that matches `name`, or `undefined` if no subject name matches `name`.
- */
- checkHost(name: string, options?: X509CheckOptions): string | undefined;
- /**
- * Checks whether the certificate matches the given IP address (IPv4 or IPv6).
- *
- * Only [RFC 5280](https://www.rfc-editor.org/rfc/rfc5280.txt) `iPAddress` subject alternative names are considered, and they
- * must match the given `ip` address exactly. Other subject alternative names as
- * well as the subject field of the certificate are ignored.
- * @since v15.6.0
- * @return Returns `ip` if the certificate matches, `undefined` if it does not.
- */
- checkIP(ip: string): string | undefined;
- /**
- * Checks whether this certificate was issued by the given `otherCert`.
- * @since v15.6.0
- */
- checkIssued(otherCert: X509Certificate): boolean;
- /**
- * Checks whether the public key for this certificate is consistent with
- * the given private key.
- * @since v15.6.0
- * @param privateKey A private key.
- */
- checkPrivateKey(privateKey: KeyObject): boolean;
- /**
- * There is no standard JSON encoding for X509 certificates. The`toJSON()` method returns a string containing the PEM encoded
- * certificate.
- * @since v15.6.0
- */
- toJSON(): string;
- /**
- * Returns information about this certificate using the legacy `certificate object` encoding.
- * @since v15.6.0
- */
- toLegacyObject(): PeerCertificate;
- /**
- * Returns the PEM-encoded certificate.
- * @since v15.6.0
- */
- toString(): string;
- /**
- * Verifies that this certificate was signed by the given public key.
- * Does not perform any other validation checks on the certificate.
- * @since v15.6.0
- * @param publicKey A public key.
- */
- verify(publicKey: KeyObject): boolean;
- }
- type LargeNumberLike = NodeJS.ArrayBufferView | SharedArrayBuffer | ArrayBuffer | bigint;
- interface GeneratePrimeOptions {
- add?: LargeNumberLike | undefined;
- rem?: LargeNumberLike | undefined;
- /**
- * @default false
- */
- safe?: boolean | undefined;
- bigint?: boolean | undefined;
- }
- interface GeneratePrimeOptionsBigInt extends GeneratePrimeOptions {
- bigint: true;
- }
- interface GeneratePrimeOptionsArrayBuffer extends GeneratePrimeOptions {
- bigint?: false | undefined;
- }
- /**
- * Generates a pseudorandom prime of `size` bits.
- *
- * If `options.safe` is `true`, the prime will be a safe prime -- that is,`(prime - 1) / 2` will also be a prime.
- *
- * The `options.add` and `options.rem` parameters can be used to enforce additional
- * requirements, e.g., for Diffie-Hellman:
- *
- * * If `options.add` and `options.rem` are both set, the prime will satisfy the
- * condition that `prime % add = rem`.
- * * If only `options.add` is set and `options.safe` is not `true`, the prime will
- * satisfy the condition that `prime % add = 1`.
- * * If only `options.add` is set and `options.safe` is set to `true`, the prime
- * will instead satisfy the condition that `prime % add = 3`. This is necessary
- * because `prime % add = 1` for `options.add > 2` would contradict the condition
- * enforced by `options.safe`.
- * * `options.rem` is ignored if `options.add` is not given.
- *
- * Both `options.add` and `options.rem` must be encoded as big-endian sequences
- * if given as an `ArrayBuffer`, `SharedArrayBuffer`, `TypedArray`, `Buffer`, or`DataView`.
- *
- * By default, the prime is encoded as a big-endian sequence of octets
- * in an [ArrayBuffer](https://developer.mozilla.org/en-US/docs/Web/JavaScript/Reference/Global_Objects/ArrayBuffer). If the `bigint` option is `true`, then a
- * [bigint](https://developer.mozilla.org/en-US/docs/Web/JavaScript/Reference/Global_Objects/BigInt) is provided.
- * @since v15.8.0
- * @param size The size (in bits) of the prime to generate.
- */
- function generatePrime(size: number, callback: (err: Error | null, prime: ArrayBuffer) => void): void;
- function generatePrime(size: number, options: GeneratePrimeOptionsBigInt, callback: (err: Error | null, prime: bigint) => void): void;
- function generatePrime(size: number, options: GeneratePrimeOptionsArrayBuffer, callback: (err: Error | null, prime: ArrayBuffer) => void): void;
- function generatePrime(size: number, options: GeneratePrimeOptions, callback: (err: Error | null, prime: ArrayBuffer | bigint) => void): void;
- /**
- * Generates a pseudorandom prime of `size` bits.
- *
- * If `options.safe` is `true`, the prime will be a safe prime -- that is,`(prime - 1) / 2` will also be a prime.
- *
- * The `options.add` and `options.rem` parameters can be used to enforce additional
- * requirements, e.g., for Diffie-Hellman:
- *
- * * If `options.add` and `options.rem` are both set, the prime will satisfy the
- * condition that `prime % add = rem`.
- * * If only `options.add` is set and `options.safe` is not `true`, the prime will
- * satisfy the condition that `prime % add = 1`.
- * * If only `options.add` is set and `options.safe` is set to `true`, the prime
- * will instead satisfy the condition that `prime % add = 3`. This is necessary
- * because `prime % add = 1` for `options.add > 2` would contradict the condition
- * enforced by `options.safe`.
- * * `options.rem` is ignored if `options.add` is not given.
- *
- * Both `options.add` and `options.rem` must be encoded as big-endian sequences
- * if given as an `ArrayBuffer`, `SharedArrayBuffer`, `TypedArray`, `Buffer`, or`DataView`.
- *
- * By default, the prime is encoded as a big-endian sequence of octets
- * in an [ArrayBuffer](https://developer.mozilla.org/en-US/docs/Web/JavaScript/Reference/Global_Objects/ArrayBuffer). If the `bigint` option is `true`, then a
- * [bigint](https://developer.mozilla.org/en-US/docs/Web/JavaScript/Reference/Global_Objects/BigInt) is provided.
- * @since v15.8.0
- * @param size The size (in bits) of the prime to generate.
- */
- function generatePrimeSync(size: number): ArrayBuffer;
- function generatePrimeSync(size: number, options: GeneratePrimeOptionsBigInt): bigint;
- function generatePrimeSync(size: number, options: GeneratePrimeOptionsArrayBuffer): ArrayBuffer;
- function generatePrimeSync(size: number, options: GeneratePrimeOptions): ArrayBuffer | bigint;
- interface CheckPrimeOptions {
- /**
- * The number of Miller-Rabin probabilistic primality iterations to perform.
- * When the value is 0 (zero), a number of checks is used that yields a false positive rate of at most `2**-64` for random input.
- * Care must be used when selecting a number of checks.
- * Refer to the OpenSSL documentation for the BN_is_prime_ex function nchecks options for more details.
- *
- * @default 0
- */
- checks?: number | undefined;
- }
- /**
- * Checks the primality of the `candidate`.
- * @since v15.8.0
- * @param candidate A possible prime encoded as a sequence of big endian octets of arbitrary length.
- */
- function checkPrime(value: LargeNumberLike, callback: (err: Error | null, result: boolean) => void): void;
- function checkPrime(value: LargeNumberLike, options: CheckPrimeOptions, callback: (err: Error | null, result: boolean) => void): void;
- /**
- * Checks the primality of the `candidate`.
- * @since v15.8.0
- * @param candidate A possible prime encoded as a sequence of big endian octets of arbitrary length.
- * @return `true` if the candidate is a prime with an error probability less than `0.25 ** options.checks`.
- */
- function checkPrimeSync(candidate: LargeNumberLike, options?: CheckPrimeOptions): boolean;
- /**
- * Load and set the `engine` for some or all OpenSSL functions (selected by flags).
- *
- * `engine` could be either an id or a path to the engine's shared library.
- *
- * The optional `flags` argument uses `ENGINE_METHOD_ALL` by default.
- * The `flags` is a bit field taking one of or a mix of the following flags (defined in `crypto.constants`):
- *
- * - `crypto.constants.ENGINE_METHOD_RSA`
- * - `crypto.constants.ENGINE_METHOD_DSA`
- * - `crypto.constants.ENGINE_METHOD_DH`
- * - `crypto.constants.ENGINE_METHOD_RAND`
- * - `crypto.constants.ENGINE_METHOD_EC`
- * - `crypto.constants.ENGINE_METHOD_CIPHERS`
- * - `crypto.constants.ENGINE_METHOD_DIGESTS`
- * - `crypto.constants.ENGINE_METHOD_PKEY_METHS`
- * - `crypto.constants.ENGINE_METHOD_PKEY_ASN1_METHS`
- * - `crypto.constants.ENGINE_METHOD_ALL`
- * - `crypto.constants.ENGINE_METHOD_NONE`
- *
- * The flags below are deprecated in OpenSSL-1.1.0.
- *
- * - `crypto.constants.ENGINE_METHOD_ECDH`
- * - `crypto.constants.ENGINE_METHOD_ECDSA`
- * - `crypto.constants.ENGINE_METHOD_STORE`
- * @since v0.11.11
- * @param [flags=crypto.constants.ENGINE_METHOD_ALL]
- */
- function setEngine(engine: string, flags?: number): void;
- /**
- * A convenient alias for `crypto.webcrypto.getRandomValues()`.
- * This implementation is not compliant with the Web Crypto spec,
- * to write web-compatible code use `crypto.webcrypto.getRandomValues()` instead.
- * @since v17.4.0
- * @returns Returns `typedArray`.
- */
- function getRandomValues(typedArray: T): T;
- /**
- * A convenient alias for `crypto.webcrypto.subtle`.
- * @since v17.4.0
- */
- const subtle: webcrypto.SubtleCrypto;
- /**
- * An implementation of the Web Crypto API standard.
- *
- * See the {@link https://nodejs.org/docs/latest/api/webcrypto.html Web Crypto API documentation} for details.
- * @since v15.0.0
- */
- const webcrypto: webcrypto.Crypto;
- namespace webcrypto {
- type BufferSource = ArrayBufferView | ArrayBuffer;
- type KeyFormat = 'jwk' | 'pkcs8' | 'raw' | 'spki';
- type KeyType = 'private' | 'public' | 'secret';
- type KeyUsage = 'decrypt' | 'deriveBits' | 'deriveKey' | 'encrypt' | 'sign' | 'unwrapKey' | 'verify' | 'wrapKey';
- type AlgorithmIdentifier = Algorithm | string;
- type HashAlgorithmIdentifier = AlgorithmIdentifier;
- type NamedCurve = string;
- type BigInteger = Uint8Array;
- interface AesCbcParams extends Algorithm {
- iv: BufferSource;
- }
- interface AesCtrParams extends Algorithm {
- counter: BufferSource;
- length: number;
- }
- interface AesDerivedKeyParams extends Algorithm {
- length: number;
- }
- interface AesGcmParams extends Algorithm {
- additionalData?: BufferSource;
- iv: BufferSource;
- tagLength?: number;
- }
- interface AesKeyAlgorithm extends KeyAlgorithm {
- length: number;
- }
- interface AesKeyGenParams extends Algorithm {
- length: number;
- }
- interface Algorithm {
- name: string;
- }
- interface EcKeyAlgorithm extends KeyAlgorithm {
- namedCurve: NamedCurve;
- }
- interface EcKeyGenParams extends Algorithm {
- namedCurve: NamedCurve;
- }
- interface EcKeyImportParams extends Algorithm {
- namedCurve: NamedCurve;
- }
- interface EcdhKeyDeriveParams extends Algorithm {
- public: CryptoKey;
- }
- interface EcdsaParams extends Algorithm {
- hash: HashAlgorithmIdentifier;
- }
- interface Ed448Params extends Algorithm {
- context?: BufferSource;
- }
- interface HkdfParams extends Algorithm {
- hash: HashAlgorithmIdentifier;
- info: BufferSource;
- salt: BufferSource;
- }
- interface HmacImportParams extends Algorithm {
- hash: HashAlgorithmIdentifier;
- length?: number;
- }
- interface HmacKeyAlgorithm extends KeyAlgorithm {
- hash: KeyAlgorithm;
- length: number;
- }
- interface HmacKeyGenParams extends Algorithm {
- hash: HashAlgorithmIdentifier;
- length?: number;
- }
- interface JsonWebKey {
- alg?: string;
- crv?: string;
- d?: string;
- dp?: string;
- dq?: string;
- e?: string;
- ext?: boolean;
- k?: string;
- key_ops?: string[];
- kty?: string;
- n?: string;
- oth?: RsaOtherPrimesInfo[];
- p?: string;
- q?: string;
- qi?: string;
- use?: string;
- x?: string;
- y?: string;
- }
- interface KeyAlgorithm {
- name: string;
- }
- interface Pbkdf2Params extends Algorithm {
- hash: HashAlgorithmIdentifier;
- iterations: number;
- salt: BufferSource;
- }
- interface RsaHashedImportParams extends Algorithm {
- hash: HashAlgorithmIdentifier;
- }
- interface RsaHashedKeyAlgorithm extends RsaKeyAlgorithm {
- hash: KeyAlgorithm;
- }
- interface RsaHashedKeyGenParams extends RsaKeyGenParams {
- hash: HashAlgorithmIdentifier;
- }
- interface RsaKeyAlgorithm extends KeyAlgorithm {
- modulusLength: number;
- publicExponent: BigInteger;
- }
- interface RsaKeyGenParams extends Algorithm {
- modulusLength: number;
- publicExponent: BigInteger;
- }
- interface RsaOaepParams extends Algorithm {
- label?: BufferSource;
- }
- interface RsaOtherPrimesInfo {
- d?: string;
- r?: string;
- t?: string;
- }
- interface RsaPssParams extends Algorithm {
- saltLength: number;
- }
- /**
- * Calling `require('node:crypto').webcrypto` returns an instance of the `Crypto` class.
- * `Crypto` is a singleton that provides access to the remainder of the crypto API.
- * @since v15.0.0
- */
- interface Crypto {
- /**
- * Provides access to the `SubtleCrypto` API.
- * @since v15.0.0
- */
- readonly subtle: SubtleCrypto;
- /**
- * Generates cryptographically strong random values.
- * The given `typedArray` is filled with random values, and a reference to `typedArray` is returned.
- *
- * The given `typedArray` must be an integer-based instance of {@link NodeJS.TypedArray}, i.e. `Float32Array` and `Float64Array` are not accepted.
- *
- * An error will be thrown if the given `typedArray` is larger than 65,536 bytes.
- * @since v15.0.0
- */
- getRandomValues>(typedArray: T): T;
- /**
- * Generates a random {@link https://www.rfc-editor.org/rfc/rfc4122.txt RFC 4122} version 4 UUID.
- * The UUID is generated using a cryptographic pseudorandom number generator.
- * @since v16.7.0
- */
- randomUUID(): string;
- CryptoKey: CryptoKeyConstructor;
- }
- // This constructor throws ILLEGAL_CONSTRUCTOR so it should not be newable.
- interface CryptoKeyConstructor {
- /** Illegal constructor */
- (_: { readonly _: unique symbol }): never; // Allows instanceof to work but not be callable by the user.
- readonly length: 0;
- readonly name: 'CryptoKey';
- readonly prototype: CryptoKey;
- }
- /**
- * @since v15.0.0
- */
- interface CryptoKey {
- /**
- * An object detailing the algorithm for which the key can be used along with additional algorithm-specific parameters.
- * @since v15.0.0
- */
- readonly algorithm: KeyAlgorithm;
- /**
- * When `true`, the {@link CryptoKey} can be extracted using either `subtleCrypto.exportKey()` or `subtleCrypto.wrapKey()`.
- * @since v15.0.0
- */
- readonly extractable: boolean;
- /**
- * A string identifying whether the key is a symmetric (`'secret'`) or asymmetric (`'private'` or `'public'`) key.
- * @since v15.0.0
- */
- readonly type: KeyType;
- /**
- * An array of strings identifying the operations for which the key may be used.
- *
- * The possible usages are:
- * - `'encrypt'` - The key may be used to encrypt data.
- * - `'decrypt'` - The key may be used to decrypt data.
- * - `'sign'` - The key may be used to generate digital signatures.
- * - `'verify'` - The key may be used to verify digital signatures.
- * - `'deriveKey'` - The key may be used to derive a new key.
- * - `'deriveBits'` - The key may be used to derive bits.
- * - `'wrapKey'` - The key may be used to wrap another key.
- * - `'unwrapKey'` - The key may be used to unwrap another key.
- *
- * Valid key usages depend on the key algorithm (identified by `cryptokey.algorithm.name`).
- * @since v15.0.0
- */
- readonly usages: KeyUsage[];
- }
- /**
- * The `CryptoKeyPair` is a simple dictionary object with `publicKey` and `privateKey` properties, representing an asymmetric key pair.
- * @since v15.0.0
- */
- interface CryptoKeyPair {
- /**
- * A {@link CryptoKey} whose type will be `'private'`.
- * @since v15.0.0
- */
- privateKey: CryptoKey;
- /**
- * A {@link CryptoKey} whose type will be `'public'`.
- * @since v15.0.0
- */
- publicKey: CryptoKey;
- }
- /**
- * @since v15.0.0
- */
- interface SubtleCrypto {
- /**
- * Using the method and parameters specified in `algorithm` and the keying material provided by `key`,
- * `subtle.decrypt()` attempts to decipher the provided `data`. If successful,
- * the returned promise will be resolved with an `` containing the plaintext result.
- *
- * The algorithms currently supported include:
- *
- * - `'RSA-OAEP'`
- * - `'AES-CTR'`
- * - `'AES-CBC'`
- * - `'AES-GCM'`
- * @since v15.0.0
- */
- decrypt(algorithm: AlgorithmIdentifier | RsaOaepParams | AesCtrParams | AesCbcParams | AesGcmParams, key: CryptoKey, data: BufferSource): Promise;
- /**
- * Using the method and parameters specified in `algorithm` and the keying material provided by `baseKey`,
- * `subtle.deriveBits()` attempts to generate `length` bits.
- * The Node.js implementation requires that when `length` is a number it must be multiple of `8`.
- * When `length` is `null` the maximum number of bits for a given algorithm is generated. This is allowed
- * for the `'ECDH'`, `'X25519'`, and `'X448'` algorithms.
- * If successful, the returned promise will be resolved with an `` containing the generated data.
- *
- * The algorithms currently supported include:
- *
- * - `'ECDH'`
- * - `'X25519'`
- * - `'X448'`
- * - `'HKDF'`
- * - `'PBKDF2'`
- * @since v15.0.0
- */
- deriveBits(algorithm: EcdhKeyDeriveParams, baseKey: CryptoKey, length: number | null): Promise;
- deriveBits(algorithm: AlgorithmIdentifier | HkdfParams | Pbkdf2Params, baseKey: CryptoKey, length: number): Promise;
- /**
- * Using the method and parameters specified in `algorithm`, and the keying material provided by `baseKey`,
- * `subtle.deriveKey()` attempts to generate a new ` based on the method and parameters in `derivedKeyAlgorithm`.
- *
- * Calling `subtle.deriveKey()` is equivalent to calling `subtle.deriveBits()` to generate raw keying material,
- * then passing the result into the `subtle.importKey()` method using the `deriveKeyAlgorithm`, `extractable`, and `keyUsages` parameters as input.
- *
- * The algorithms currently supported include:
- *
- * - `'ECDH'`
- * - `'X25519'`
- * - `'X448'`
- * - `'HKDF'`
- * - `'PBKDF2'`
- * @param keyUsages See {@link https://nodejs.org/docs/latest/api/webcrypto.html#cryptokeyusages Key usages}.
- * @since v15.0.0
- */
- deriveKey(
- algorithm: AlgorithmIdentifier | EcdhKeyDeriveParams | HkdfParams | Pbkdf2Params,
- baseKey: CryptoKey,
- derivedKeyAlgorithm: AlgorithmIdentifier | AesDerivedKeyParams | HmacImportParams | HkdfParams | Pbkdf2Params,
- extractable: boolean,
- keyUsages: ReadonlyArray
- ): Promise;
- /**
- * Using the method identified by `algorithm`, `subtle.digest()` attempts to generate a digest of `data`.
- * If successful, the returned promise is resolved with an `` containing the computed digest.
- *
- * If `algorithm` is provided as a ``, it must be one of:
- *
- * - `'SHA-1'`
- * - `'SHA-256'`
- * - `'SHA-384'`
- * - `'SHA-512'`
- *
- * If `algorithm` is provided as an `