Commit
·
0b32c6a
1
Parent(s):
8ba65d9
Update parquet files (step 29 of 296)
Browse filesThis view is limited to 50 files because it contains too many changes.
See raw diff
- spaces/1-13-am/neural-style-transfer/network.py +0 -127
- spaces/1acneusushi/gradio-2dmoleculeeditor/data/Attend Hrm Crack Keygen The Best HR Software for Small and Medium Businesses.md +0 -144
- spaces/1acneusushi/gradio-2dmoleculeeditor/data/Clearview Rc Flight Simulator Version 5.34 Serial Crack Download and Install Guide.md +0 -131
- spaces/1acneusushi/gradio-2dmoleculeeditor/data/Elements 3D Plugin After Effects Crack 13 Download and Install Guide.md +0 -72
- spaces/1gistliPinn/ChatGPT4/Examples/Azerbaycan Dili Test Banki Cavablari 1 Ci Hisse.md +0 -6
- spaces/1gistliPinn/ChatGPT4/Examples/CRACK Microsoft Office 2016 VL Visio Project Language Accessory Packs VERIFIED.md +0 -75
- spaces/1gistliPinn/ChatGPT4/Examples/Construct2licensefilecrack.md +0 -6
- spaces/1pelhydcardo/ChatGPT-prompt-generator/assets/Candy Crush Saga APK Download Everything You Need to Know About the Legendary Puzzle Game.md +0 -87
- spaces/1pelhydcardo/ChatGPT-prompt-generator/assets/Download CSR Racing for Android and Compete with the Worlds Top Drag Racers.md +0 -91
- spaces/1phancelerku/anime-remove-background/Download Basketball Battle Mod APK and Challenge Your Friends.md +0 -157
- spaces/1phancelerku/anime-remove-background/Download Yama no Susume S1 The Fun and Relaxing Anime You Need.md +0 -113
- spaces/2023Liu2023/bingo/src/state/index.ts +0 -118
- spaces/AFCMEgypt/AFCM_iGEM_LFA/README.md +0 -13
- spaces/AI-Zero-to-Hero/01-H5-Play-Canvas-Sim-Physics/index.html +0 -10
- spaces/AIGC-Audio/AudioGPT/audio_detection/audio_infer/utils/utilities.py +0 -172
- spaces/AgentVerse/agentVerse/agentverse/agents/tasksolving_agent/solver.py +0 -110
- spaces/AgentVerse/agentVerse/ui/src/phaser3-rex-plugins/templates/ui/gridtable/input/EmitCellEvent.js +0 -17
- spaces/AhmadHakami/Alzheimer_image_classification/app.py +0 -25
- spaces/Ajaxon6255/Emerald_Isle/theme_dropdown.py +0 -57
- spaces/AlexWang/lama/models/ade20k/segm_lib/nn/parallel/data_parallel.py +0 -112
- spaces/Amiminoru/Deus/README.md +0 -10
- spaces/Amrrs/DragGan-Inversion/PTI/models/e4e/stylegan2/__init__.py +0 -0
- spaces/Androidonnxfork/CivitAi-to-Diffusers/diffusers/docs/source/en/optimization/torch2.0.md +0 -444
- spaces/Androidonnxfork/CivitAi-to-Diffusers/diffusers/tests/pipelines/stable_diffusion_xl/test_stable_diffusion_xl_img2img.py +0 -317
- spaces/Andy1621/uniformer_image_detection/configs/cascade_rcnn/cascade_mask_rcnn_x101_32x4d_fpn_20e_coco.py +0 -13
- spaces/Andy1621/uniformer_image_detection/mmdet/models/dense_heads/transformer_head.py +0 -654
- spaces/Ariharasudhan/YoloV5/export.py +0 -652
- spaces/Artples/Named-Entity-Recognition/README.md +0 -13
- spaces/Ataturk-Chatbot/HuggingFaceChat/venv/lib/python3.11/site-packages/pip/_vendor/chardet/langhungarianmodel.py +0 -0
- spaces/Awesimo/jojogan/util.py +0 -220
- spaces/Bart92/RVC_HF/infer/modules/train/preprocess.py +0 -147
- spaces/Benson/text-generation/Examples/Descarga De Actualizacin De Redmi 5a Miui 12.md +0 -101
- spaces/Benson/text-generation/Examples/Descargar Gratis Para Windows 7.md +0 -61
- spaces/BetterAPI/BetterChat/postcss.config.js +0 -6
- spaces/BramVanroy/mai-simplification-nl-2023-demo/app.py +0 -128
- spaces/CVPR/Dual-Key_Backdoor_Attacks/datagen/detectron2/projects/DensePose/densepose/densepose_head.py +0 -1183
- spaces/CVPR/Dual-Key_Backdoor_Attacks/datagen/detectron2/tests/test_roi_align_rotated.py +0 -176
- spaces/CVPR/LIVE/pybind11/tests/test_chrono.py +0 -202
- spaces/CVPR/LIVE/thrust/internal/scripts/wiki2tex.py +0 -194
- spaces/CVPR/LIVE/thrust/thrust/system/cpp/detail/find.h +0 -23
- spaces/CVPR/LIVE/thrust/thrust/system/cuda/detail/sequence.h +0 -22
- spaces/CVPR/WALT/mmdet/core/visualization/image.py +0 -322
- spaces/CVPR/transfiner/demo/README.md +0 -5
- spaces/Caoyunkang/Segment-Any-Anomaly/GroundingDINO/groundingdino/util/get_tokenlizer.py +0 -26
- spaces/ChristopherMarais/Andrew_AI-BB_classification-beta/mysite/andrew_alpha/urls.py +0 -8
- spaces/ClassCat/DETR-Object-Detection/README.md +0 -12
- spaces/DQChoi/gpt-demo/venv/lib/python3.11/site-packages/gradio/components/__init__.py +0 -116
- spaces/DQChoi/gpt-demo/venv/lib/python3.11/site-packages/gradio/components/status_tracker.py +0 -13
- spaces/DaFujaTyping/hf-Chat-ui/src/lib/stores/pendingMessageIdToRetry.ts +0 -4
- spaces/Daniton/facebook-blenderbot-3Byx/README.md +0 -13
spaces/1-13-am/neural-style-transfer/network.py
DELETED
@@ -1,127 +0,0 @@
|
|
1 |
-
import torch
|
2 |
-
import torch.nn as nn
|
3 |
-
import torchvision
|
4 |
-
from torchvision.models import vgg19
|
5 |
-
import utils
|
6 |
-
from utils import batch_wct, batch_histogram_matching
|
7 |
-
|
8 |
-
class Encoder(nn.Module):
|
9 |
-
def __init__(self, layers = [1, 6, 11, 20]):
|
10 |
-
super(Encoder, self).__init__()
|
11 |
-
vgg = torchvision.models.vgg19(pretrained=True).features
|
12 |
-
|
13 |
-
self.encoder = nn.ModuleList()
|
14 |
-
temp_seq = nn.Sequential()
|
15 |
-
for i in range(max(layers)+1):
|
16 |
-
temp_seq.add_module(str(i), vgg[i])
|
17 |
-
if i in layers:
|
18 |
-
self.encoder.append(temp_seq)
|
19 |
-
temp_seq = nn.Sequential()
|
20 |
-
|
21 |
-
def forward(self, x):
|
22 |
-
features = []
|
23 |
-
for layer in self.encoder:
|
24 |
-
x = layer(x)
|
25 |
-
features.append(x)
|
26 |
-
return features
|
27 |
-
|
28 |
-
# need to copy the whole architecture bcuz we will need outputs from "layers" layers to compute the loss
|
29 |
-
class Decoder(nn.Module):
|
30 |
-
def __init__(self, layers=[1, 6, 11, 20]):
|
31 |
-
super(Decoder, self).__init__()
|
32 |
-
vgg = torchvision.models.vgg19(pretrained=False).features
|
33 |
-
|
34 |
-
self.decoder = nn.ModuleList()
|
35 |
-
temp_seq = nn.Sequential()
|
36 |
-
count = 0
|
37 |
-
for i in range(max(layers)-1, -1, -1):
|
38 |
-
if isinstance(vgg[i], nn.Conv2d):
|
39 |
-
# get number of in/out channels
|
40 |
-
out_channels = vgg[i].in_channels
|
41 |
-
in_channels = vgg[i].out_channels
|
42 |
-
kernel_size = vgg[i].kernel_size
|
43 |
-
|
44 |
-
# make a [reflection pad + convolution + relu] layer
|
45 |
-
temp_seq.add_module(str(count), nn.ReflectionPad2d(padding=(1,1,1,1)))
|
46 |
-
count += 1
|
47 |
-
temp_seq.add_module(str(count), nn.Conv2d(in_channels, out_channels, kernel_size=kernel_size))
|
48 |
-
count += 1
|
49 |
-
temp_seq.add_module(str(count), nn.ReLU())
|
50 |
-
count += 1
|
51 |
-
|
52 |
-
# change down-sampling(MaxPooling) --> upsampling
|
53 |
-
elif isinstance(vgg[i], nn.MaxPool2d):
|
54 |
-
temp_seq.add_module(str(count), nn.Upsample(scale_factor=2))
|
55 |
-
count += 1
|
56 |
-
|
57 |
-
if i in layers:
|
58 |
-
self.decoder.append(temp_seq)
|
59 |
-
temp_seq = nn.Sequential()
|
60 |
-
|
61 |
-
# append last conv layers without ReLU activation
|
62 |
-
self.decoder.append(temp_seq[:-1])
|
63 |
-
|
64 |
-
def forward(self, x):
|
65 |
-
y = x
|
66 |
-
for layer in self.decoder:
|
67 |
-
y = layer(y)
|
68 |
-
return y
|
69 |
-
|
70 |
-
class AdaIN(nn.Module):
|
71 |
-
def __init__(self):
|
72 |
-
super(AdaIN, self).__init__()
|
73 |
-
|
74 |
-
def forward(self, content, style, style_strength=1.0, eps=1e-5):
|
75 |
-
"""
|
76 |
-
content: tensor of shape B * C * H * W
|
77 |
-
style: tensor of shape B * C * H * W
|
78 |
-
note that AdaIN does computation on a pair of content - style img"""
|
79 |
-
b, c, h, w = content.size()
|
80 |
-
|
81 |
-
content_std, content_mean = torch.std_mean(content.view(b, c, -1), dim=2, keepdim=True)
|
82 |
-
style_std, style_mean = torch.std_mean(style.view(b, c, -1), dim=2, keepdim=True)
|
83 |
-
|
84 |
-
normalized_content = (content.view(b, c, -1) - content_mean) / (content_std+eps)
|
85 |
-
|
86 |
-
stylized_content = (normalized_content * style_std) + style_mean
|
87 |
-
|
88 |
-
output = (1-style_strength) * content + style_strength * stylized_content.view(b, c, h, w)
|
89 |
-
return output
|
90 |
-
|
91 |
-
class Style_Transfer_Network(nn.Module):
|
92 |
-
def __init__(self, layers = [1, 6, 11, 20]):
|
93 |
-
super(Style_Transfer_Network, self).__init__()
|
94 |
-
self.encoder = Encoder(layers)
|
95 |
-
self.decoder = Decoder(layers)
|
96 |
-
self.adain = AdaIN()
|
97 |
-
|
98 |
-
def forward(self, content, styles, style_strength = 1., interpolation_weights = None, preserve_color = None, train = False):
|
99 |
-
if interpolation_weights is None:
|
100 |
-
interpolation_weights = [1/len(styles)] * len(styles)
|
101 |
-
# encode the content image
|
102 |
-
content_feature = self.encoder(content)
|
103 |
-
|
104 |
-
# encode style images
|
105 |
-
style_features = []
|
106 |
-
for style in styles:
|
107 |
-
if preserve_color == 'whitening_and_coloring' or preserve_color == 'histogram_matching':
|
108 |
-
style = batch_wct(style, content)
|
109 |
-
style_features.append(self.encoder(style))
|
110 |
-
|
111 |
-
transformed_features = []
|
112 |
-
for style_feature, interpolation_weight in zip(style_features, interpolation_weights):
|
113 |
-
AdaIN_feature = self.adain(content_feature[-1], style_feature[-1], style_strength) * interpolation_weight
|
114 |
-
if preserve_color == 'histogram_matching':
|
115 |
-
AdaIN_feature *= 0.9
|
116 |
-
transformed_features.append(AdaIN_feature)
|
117 |
-
transformed_feature = sum(transformed_features)
|
118 |
-
|
119 |
-
stylized_image = self.decoder(transformed_feature)
|
120 |
-
if preserve_color == "whitening_and_coloring":
|
121 |
-
stylized_image = batch_wct(stylized_image, content)
|
122 |
-
if preserve_color == "histogram_matching":
|
123 |
-
stylized_image = batch_histogram_matching(stylized_image, content)
|
124 |
-
if train:
|
125 |
-
return stylized_image, transformed_feature
|
126 |
-
else:
|
127 |
-
return stylized_image
|
|
|
|
|
|
|
|
|
|
|
|
|
|
|
|
|
|
|
|
|
|
|
|
|
|
|
|
|
|
|
|
|
|
|
|
|
|
|
|
|
|
|
|
|
|
|
|
|
|
|
|
|
|
|
|
|
|
|
|
|
|
|
|
|
|
|
|
|
|
|
|
|
|
|
|
|
|
|
|
|
|
|
|
|
|
|
|
|
|
|
|
|
|
|
|
|
|
|
|
|
|
|
|
|
|
|
|
|
|
|
|
|
|
|
|
|
|
|
|
|
|
|
|
|
|
|
|
|
|
|
|
|
|
|
|
|
|
|
|
|
|
|
|
|
|
|
|
|
|
|
|
|
|
|
|
|
|
|
|
|
|
|
|
|
|
|
|
|
|
|
|
|
|
|
|
|
|
|
|
|
|
|
|
|
|
|
|
|
|
|
|
|
|
|
|
|
|
|
|
|
|
|
|
|
|
|
|
|
|
|
|
|
|
|
|
|
|
|
|
|
|
|
|
|
|
|
|
|
|
|
|
|
|
|
|
|
|
|
|
|
|
|
|
|
|
|
|
|
|
|
|
|
|
|
|
spaces/1acneusushi/gradio-2dmoleculeeditor/data/Attend Hrm Crack Keygen The Best HR Software for Small and Medium Businesses.md
DELETED
@@ -1,144 +0,0 @@
|
|
1 |
-
|
2 |
-
<h1>Attend HRM Crack Keygen: How to Download and Install the Best Attendance Software for Free</h1>
|
3 |
-
<p>If you are looking for a software solution that can help you manage your employee attendance and payroll, you may have heard of Attend HRM. This software is designed to automate your attendance process, generate reports, and calculate salaries. But what if you don't want to pay for the full version of the software? Is there a way to get it for free? In this article, we will show you how to download and install Attend HRM crack keygen, a tool that can activate the software without paying a dime. We will also discuss the features and benefits of Attend HRM, as well as the risks and drawbacks of using crack keygen.</p>
|
4 |
-
<h2>Attend Hrm Crack Keygen</h2><br /><p><b><b>DOWNLOAD</b> ››› <a href="https://byltly.com/2uKzXp">https://byltly.com/2uKzXp</a></b></p><br /><br />
|
5 |
-
<h2>What is Attend HRM and why do you need it?</h2>
|
6 |
-
<p>Attend HRM is a software solution for managing employee attendance and payroll. It is designed for small and medium businesses that need a simple and effective way to track their employees' time and attendance, as well as their salaries and deductions. With Attend HRM, you can:</p>
|
7 |
-
<h3>Attend HRM is a software solution for managing employee attendance and payroll</h3>
|
8 |
-
<ul>
|
9 |
-
<li>Automate your attendance process by connecting to various devices such as biometric scanners, RFID cards, or mobile apps.</li>
|
10 |
-
<li>Customize your attendance rules according to your company's policies, such as working hours, shifts, overtime, leaves, holidays, etc.</li>
|
11 |
-
<li>Import data from multiple locations into a centralized database.</li>
|
12 |
-
<li>Generate reports on attendance, absenteeism, late arrivals, early departures, overtime, etc.</li>
|
13 |
-
<li>Calculate salaries based on attendance data, as well as other factors such as bonuses, commissions, taxes, deductions, etc.</li>
|
14 |
-
<li>Export payroll data to your accounting software or bank account.</li>
|
15 |
-
</ul>
|
16 |
-
<h3>Attend HRM offers many features and benefits for small and medium businesses</h3>
|
17 |
-
<ul>
|
18 |
-
<li>It saves you time and money by eliminating manual processes and human errors.</li>
|
19 |
-
<li>It improves your productivity and efficiency by streamlining your workflow and reducing paperwork.</li>
|
20 |
-
<li>It enhances your employee satisfaction and retention by ensuring fair and accurate payment.</li>
|
21 |
-
<li>It helps you comply with labor laws and regulations by keeping records of your employees' attendance and payroll.</li>
|
22 |
-
<li>It supports multiple languages, currencies, and formats to suit your business needs.</li>
|
23 |
-
</ul>
|
24 |
-
<h3>Attend HRM is compatible with various devices and databases</h3>
|
25 |
-
<ul>
|
26 |
-
<li>You can use any device that can record time and attendance data, such as fingerprint scanners, face recognition systems, RFID cards, mobile apps, etc.</li>
|
27 |
-
<li>You can use any database system that can store your data securely, such as SQL Server, Oracle, Sybase, Firebird, etc.</li>
|
28 |
-
<li>You can access your data from any device or location via web-based interface or mobile app.</li>
|
29 |
-
</ul>
|
30 |
-
<h2>How to download and install Attend HRM crack keygen?</h2>
|
31 |
-
<p>If you want to use Attend HRM without paying for the full version of the software, you can try to download and install Attend HRM crack keygen. This is a tool that can generate a license key that can activate the software for free. However, you should be careful when downloading crack keygen from online sources, as some of them may contain viruses or malware that can harm your computer or steal your data. Here are the steps to download and install Attend HRM crack keygen safely:</p>
|
32 |
-
<h3>Find a reliable source for Attend HRM crack keygen online</h3>
|
33 |
-
<ul>
|
34 |
-
<li>You can search for Attend HRM crack keygen on Google or other search engines. You will find many websites that claim to offer free download links for crack keygen.</li>
|
35 |
-
<li>You should check the reviews and ratings of these websites before clicking on any link. You should also scan the files with an antivirus program before opening them.</li>
|
36 |
-
<li>You should avoid websites that ask you to complete surveys or provide personal information in order to download crack keygen. These are usually scams that try to trick you into giving away your money or data.</li>
|
37 |
-
</ul>
|
38 |
-
<h3>Follow the instructions to download and extract the files</h3>
|
39 |
-
<ul>
|
40 |
-
<li>Once you find a trustworthy source for Attend HRM crack keygen, you can click on the download link and save the file on your computer.</li>
|
41 |
-
<li>The file may be compressed in a ZIP or RAR format. You will need a program like WinRAR or 7-Zip to extract it.</li>
|
42 |
-
<li>After extracting the file, you will see a folder that contains two files: setup.exe and keygen.exe. These are the files that you need to install and activate the software.</li>
|
43 |
-
</ul>
|
44 |
-
<h3>Run the setup file and enter the license key</h3>
|
45 |
-
<ul>
|
46 |
-
<li>You can run the setup file by double-clicking on it. This will start the installation process of Attend HRM on your computer.</li>
|
47 |
-
<li>You will be asked to choose a language, accept the terms and conditions, select a destination folder, etc. You can follow the instructions on the screen to complete the installation.</li>
|
48 |
-
<li>After installing the software, you will need to activate it with a license key. You can generate a license key by running the keygen file by double-clicking on it.</li>
|
49 |
-
<li>The keygen file will open a window that shows a random license key. You can copy this license key by clicking on it.</li>
|
50 |
-
<li>You can paste this license key into the activation window of Attend HRM by clicking on "Enter License Key" button. This will activate the software for free.</li>
|
51 |
-
</ul>
|
52 |
-
<h2>How to use Attend HRM crack keygen?</h2>
|
53 |
-
<p>After activating Attend HRM with crack keygen, you can start using it to manage your employee attendance and payroll. Here are some tips on how to use Attend HRM effectively:</p>
|
54 |
-
<p>Attend Hrm full version with serial key<br />
|
55 |
-
How to activate Attend Hrm software for free<br />
|
56 |
-
Download Attend Hrm cracked license code<br />
|
57 |
-
Attend Hrm patch download link<br />
|
58 |
-
Attend Hrm registration key generator<br />
|
59 |
-
Attend Hrm activation key crack<br />
|
60 |
-
Attend Hrm pro crack download<br />
|
61 |
-
Attend Hrm ultimate crack free download<br />
|
62 |
-
Attend Hrm professional edition keygen<br />
|
63 |
-
Attend Hrm enterprise edition crack<br />
|
64 |
-
Attend Hrm premium edition serial number<br />
|
65 |
-
Attend Hrm crack 2023 latest version<br />
|
66 |
-
Attend Hrm crack for windows 10/8/7<br />
|
67 |
-
Attend Hrm crack for mac os x<br />
|
68 |
-
Attend Hrm crack for linux<br />
|
69 |
-
Attend Hrm portable version crack<br />
|
70 |
-
Attend Hrm offline installer crack<br />
|
71 |
-
Attend Hrm online activation crack<br />
|
72 |
-
Attend Hrm lifetime license crack<br />
|
73 |
-
Attend Hrm unlimited users crack<br />
|
74 |
-
Attend Hrm multi-language support crack<br />
|
75 |
-
Attend Hrm cloud-based edition crack<br />
|
76 |
-
Attend Hrm web-based edition crack<br />
|
77 |
-
Attend Hrm mobile app edition crack<br />
|
78 |
-
Attend Hrm desktop app edition crack<br />
|
79 |
-
Attend Hrm biometric integration crack<br />
|
80 |
-
Attend Hrm payroll module crack<br />
|
81 |
-
Attend Hrm leave management module crack<br />
|
82 |
-
Attend Hrm attendance management module crack<br />
|
83 |
-
Attend Hrm employee management module crack<br />
|
84 |
-
Attend Hrm performance management module crack<br />
|
85 |
-
Attend Hrm training management module crack<br />
|
86 |
-
Attend Hrm recruitment management module crack<br />
|
87 |
-
Attend Hrm project management module crack<br />
|
88 |
-
Attend Hrm expense management module crack<br />
|
89 |
-
Attend Hrm asset management module crack<br />
|
90 |
-
Attend Hrm compliance management module crack<br />
|
91 |
-
Attend Hrm report generation module crack<br />
|
92 |
-
Attend Hrm dashboard customization module crack<br />
|
93 |
-
Attend Hrm data import/export module crack<br />
|
94 |
-
Attend Hrm data backup/restore module crack<br />
|
95 |
-
Attend Hrm data security/encryption module crack<br />
|
96 |
-
Attend Hrm data synchronization module crack<br />
|
97 |
-
Attend Hrm data analysis module crack<br />
|
98 |
-
Attend Hrm data visualization module crack<br />
|
99 |
-
Attend Hrm artificial intelligence module crack<br />
|
100 |
-
Attend Hrm machine learning module crack<br />
|
101 |
-
Attend Hrm natural language processing module crack<br />
|
102 |
-
Attend Hrm voice recognition module crack<br />
|
103 |
-
Attend Hrm facial recognition module crack</p>
|
104 |
-
<h3>Configure your attendance rules and connect to remote devices</h3>
|
105 |
-
<ul>
|
106 |
-
<li>You can configure your attendance rules by clicking on "Settings" menu in Attend HRM. You can set up your working hours, shifts, overtime rates, leaves, holidays, etc. according to your company's policies. You can also create different groups and categories for your employees, such as departments, designations, grades, etc. </li>
|
107 |
-
<li>You can connect to remote devices by clicking on "Device Integration" menu in Attend HRM. You can add new devices by entering their name, IP address, port number, communication password, etc. You can also test the connection and synchronize the data between the devices and the software. You can use any device that can record time and attendance data, such as biometric scanners, face recognition systems, RFID cards, mobile apps, etc. </li>
|
108 |
-
</ul>
|
109 |
-
<h3>Import data from multiple locations and generate reports</h3>
|
110 |
-
<ul>
|
111 |
-
<li>You can import data from multiple locations by clicking on "Data Import" menu in Attend HRM. You can select the source device or database from which you want to import data. You can also schedule automatic data import at regular intervals.</li>
|
112 |
-
<li>You can generate reports by clicking on "Reports" menu in Attend HRM. You can choose from various types of reports, such as attendance report, absenteeism report, late arrival report, early departure report, overtime report, etc. You can also may be sued by the software developer or distributor for damages and losses. You may also face fines or penalties from the government agencies that enforce anti-piracy laws.</li>
|
113 |
-
</ul>
|
114 |
-
<h3>You may compromise your data security and privacy</h3>
|
115 |
-
<ul>
|
116 |
-
<li>Using crack keygen may expose your computer and data to viruses, malware, spyware, or ransomware. These malicious programs may infect your system, damage your files, steal your information, or lock your data until you pay a ransom.</li>
|
117 |
-
<li>You may also compromise your data privacy by using crack keygen. The software may contain hidden codes or backdoors that allow hackers or third parties to access your data without your consent. They may use your data for identity theft, fraud, or other illegal purposes.</li>
|
118 |
-
</ul>
|
119 |
-
<h3>You may encounter errors and bugs that affect your performance</h3>
|
120 |
-
<ul>
|
121 |
-
<li>Using crack keygen may cause errors and bugs in the software that affect its functionality and performance. The software may not work properly, crash frequently, or produce inaccurate results.</li>
|
122 |
-
<li>You may also miss out on the updates and upgrades that the software developer or distributor provides to improve the software and fix the issues. You may not be able to access the latest features and benefits of the software.</li>
|
123 |
-
<li>You may also have difficulty getting technical support or customer service if you encounter any problems with the software. The software developer or distributor may not provide any assistance or warranty to users of pirated software.</li>
|
124 |
-
</ul>
|
125 |
-
<h2>Conclusion</h2>
|
126 |
-
<p>Attend HRM is a software solution that can help you manage your employee attendance and payroll efficiently and effectively. It offers many features and benefits that can save you time and money, improve your productivity and efficiency, enhance your employee satisfaction and retention, and help you comply with labor laws and regulations.</p>
|
127 |
-
<p>However, using Attend HRM crack keygen is not a good idea if you want to use the software legally and safely. Using crack keygen is a form of software piracy that can expose you to legal issues, data security risks, and performance problems. You may end up losing more than you gain by using pirated software.</p>
|
128 |
-
<p>Therefore, we recommend that you purchase the full version of Attend HRM from the official website or authorized resellers. This way, you can enjoy the full features and benefits of the software without any worries or hassles. You can also get technical support and customer service if you need any help with the software.</p>
|
129 |
-
<p>We hope this article has helped you understand what Attend HRM crack keygen is, how to download and install it, how to use it, and what are the risks and drawbacks of using it. If you have any questions or comments, please feel free to leave them below.</p>
|
130 |
-
<h2>FAQs</h2>
|
131 |
-
<p>Here are some frequently asked questions about Attend HRM crack keygen:</p>
|
132 |
-
<h3>What is a crack keygen?</h3>
|
133 |
-
<p>A crack keygen is a tool that can generate a license key that can activate a software product without paying for it. It is a form of software piracy that is illegal and unethical.</p>
|
134 |
-
<h3>Where can I download Attend HRM crack keygen?</h3>
|
135 |
-
<p>You can search for Attend HRM crack keygen on Google or other search engines. You will find many websites that claim to offer free download links for crack keygen. However, you should be careful when downloading crack keygen from online sources, as some of them may contain viruses or malware that can harm your computer or steal your data.</p>
|
136 |
-
<h3>How do I install Attend HRM crack keygen?</h3>
|
137 |
-
<p>To install Attend HRM crack keygen, you need to download and extract the files from a reliable source. Then, you need to run the setup file and enter the license key generated by the keygen file. This will activate the software for free.</p>
|
138 |
-
<h3>Is Attend HRM crack keygen safe to use?</h3>
|
139 |
-
<p>No, Attend HRM crack keygen is not safe to use. Using crack keygen can expose you to legal issues, data security risks, and performance problems. You may face legal consequences if you are caught using pirated software. You may also compromise your data security and privacy by using crack keygen. You may also encounter errors and bugs that affect your performance by using crack keygen.</p>
|
140 |
-
<h3>What are the alternatives to Attend HRM crack keygen?</h3>
|
141 |
-
<p>The best alternative to Attend HRM crack keygen is to purchase the full version of Attend HRM from the official website or authorized resellers. This way, you can enjoy the full features and benefits of the software without any worries or hassles. You can also get technical support and customer service if you need any help with the software.</p>
|
142 |
-
</p> 0a6ba089eb<br />
|
143 |
-
<br />
|
144 |
-
<br />
|
|
|
|
|
|
|
|
|
|
|
|
|
|
|
|
|
|
|
|
|
|
|
|
|
|
|
|
|
|
|
|
|
|
|
|
|
|
|
|
|
|
|
|
|
|
|
|
|
|
|
|
|
|
|
|
|
|
|
|
|
|
|
|
|
|
|
|
|
|
|
|
|
|
|
|
|
|
|
|
|
|
|
|
|
|
|
|
|
|
|
|
|
|
|
|
|
|
|
|
|
|
|
|
|
|
|
|
|
|
|
|
|
|
|
|
|
|
|
|
|
|
|
|
|
|
|
|
|
|
|
|
|
|
|
|
|
|
|
|
|
|
|
|
|
|
|
|
|
|
|
|
|
|
|
|
|
|
|
|
|
|
|
|
|
|
|
|
|
|
|
|
|
|
|
|
|
|
|
|
|
|
|
|
|
|
|
|
|
|
|
|
|
|
|
|
|
|
|
|
|
|
|
|
|
|
|
|
|
|
|
|
|
|
|
|
|
|
|
|
|
|
|
|
|
|
|
|
|
|
|
|
|
|
|
|
|
|
|
|
|
|
|
|
|
|
|
|
|
|
|
|
|
|
|
|
|
|
|
|
|
|
|
|
|
|
|
|
|
|
|
|
|
|
|
|
|
|
|
|
|
|
|
|
|
|
|
|
|
|
spaces/1acneusushi/gradio-2dmoleculeeditor/data/Clearview Rc Flight Simulator Version 5.34 Serial Crack Download and Install Guide.md
DELETED
@@ -1,131 +0,0 @@
|
|
1 |
-
|
2 |
-
<h1>Clearview RC Flight Simulator: A Review</h1>
|
3 |
-
<p>Are you looking for a professional quality RC flight simulator for radio controlled planes and helicopters? Do you want to learn to fly without fear, save money and time by not crashing your real models, and enjoy realistic physics and graphics? If so, you might want to check out Clearview RC Flight Simulator, one of the best simulators on the market. In this article, we will review Clearview RC Flight Simulator and its latest version, 5.34. We will also warn you about the dangers of downloading a cracked version of the simulator, and show you how to get the full version legally and safely.</p>
|
4 |
-
<h2>Clearview Rc Flight Simulator Version 5.34 serial crack.iso</h2><br /><p><b><b>Download</b> <a href="https://byltly.com/2uKv6T">https://byltly.com/2uKv6T</a></b></p><br /><br />
|
5 |
-
<h2>What is Clearview RC Flight Simulator?</h2>
|
6 |
-
<p>Clearview RC Flight Simulator is a software program that simulates radio controlled planes and helicopters on your computer. It was developed by SVK Systems, a company based in Bulgaria, and has been around since 2006. Clearview RC Flight Simulator is designed for Windows operating systems, and works on Windows 11,10,8 and 7. It has over 50 heli models, 50 plane models, and 17 flying fields to choose from. You can also import your own models and landscapes from other sources.</p>
|
7 |
-
<h2>Why choose Clearview RC Flight Simulator?</h2>
|
8 |
-
<p>There are many reasons why Clearview RC Flight Simulator is a great choice for anyone who loves RC flying. Here are some of them:</p>
|
9 |
-
<ul>
|
10 |
-
<li>It has a refined flight model with autorotation, true high alpha angles simulation, water support, advanced setup for editing all flight parameters, rate and heading hold gyro simulation, and much more.</li>
|
11 |
-
<li>It has a dynamic camera mode, zoom modes, close up camera, auto pilot, magic time (tm) time slowdown for better learning, record/replay feature, panorama scenery import, DirectX FMS models import, network update feature, network multiplayer feature, etc.</li>
|
12 |
-
<li>It has a realistic weather system that affects the flight dynamics and visuals. You can adjust the wind speed, direction, turbulence, thermals, clouds, fog, etc.</li>
|
13 |
-
<li>It has a user-friendly interface that allows you to easily select models, fields, settings, etc. You can also customize your controller settings and calibrate your controller before flying.</li>
|
14 |
-
<li>It has a low price compared to other simulators. You can download it for free and try it before buying it. The full version costs only $39.99 and includes lifetime upgrades and free support.</li>
|
15 |
-
</ul>
|
16 |
-
<h2>How to download and install Clearview RC Flight Simulator?</h2>
|
17 |
-
<p>Downloading and installing Clearview RC Flight Simulator is very easy. Just follow these steps:</p>
|
18 |
-
<ol>
|
19 |
-
<li>Go to <a href="http://rcflightsim.com/">http://rcflightsim.com/</a>, the official website of Clearview RC Flight Simulator.</li>
|
20 |
-
<li>Click on "Download" button on the top menu.</li>
|
21 |
-
<li>Click on "Download ClearView" button on the download page.</li>
|
22 |
-
<li>Save the file "ClearView-Setup.exe" on your computer.</li>
|
23 |
-
<li>Run the file "ClearView-Setup.exe" and follow the instructions on the screen.</li>
|
24 |
-
<li>After installation is complete, launch Clearview RC Flight Simulator from your desktop or start menu.</li>
|
25 |
-
</ol>
|
26 |
-
<h1>Clearview RC Flight Simulator Version 5.34: What's New?</h1>
|
27 |
-
<p>Clearview RC Flight Simulator is constantly updated with new features and improvements. The latest version, 5.34, was released on December 2020. Here are some of the highlights of this version:</p>
|
28 |
-
<h2>Enhanced weather system</h2>
|
29 |
-
<p>The weather system in Clearview RC Flight Simulator has been reworked from ground up. Now it has more realistic effects on the flight dynamics and visuals. You can see clouds moving with the wind direction and speed. You can also see fog forming at low altitudes or near water surfaces. The wind turbulence affects not only your model but also other objects in the scene such as trees or flags.</p>
|
30 |
-
<h2>NetPlay feature</h2>
|
31 |
-
<p>The NetPlay feature allows you to broadcast or replay flights online with other users. You can join or create a session with up to four players. You can chat with other players using text or voice messages. You can also watch other players' flights or record your own flights for later replaying.</p>
|
32 |
-
<h2>New models added</h2>
|
33 |
-
<p>The new version adds four new models to the simulator: A6M Zero (Japanese fighter plane), B-25 Mitchell (American bomber plane), T-33 Shooting Star (American jet trainer), and GeeBee R3 Fantasy Racer (American racing plane). These models are expertly designed and tuned by Ken Northup, one of the best modelers in the industry. They have high quality graphics and realistic flight characteristics.</p>
|
34 |
-
<h1>How to get Clearview RC Flight Simulator Version 5.34 serial crack.iso?</h1>
|
35 |
-
<p>You might be tempted to download a cracked version of Clearview RC Flight Simulator Version 5.34 from some shady website or torrent site. However, we strongly advise you against doing so. Here are some reasons why:</p>
|
36 |
-
<h2>The dangers of downloading cracked software</h2>
|
37 |
-
<p>Downloading cracked software is risky for several reasons:</p>
|
38 |
-
<p>Clearview Rc Flight Simulator Version 5.34 Serial Crack.iso free download<br />
|
39 |
-
Clearview Rc Flight Simulator Version 5.34 Serial Crack.iso hd video<br />
|
40 |
-
Clearview Rc Flight Simulator Version 5.34 Serial Crack.iso installation<br />
|
41 |
-
Clearview Rc Flight Simulator Version 5.34 Serial Crack.iso unblock<br />
|
42 |
-
Clearview Rc Flight Simulator Version 5.34 Serial Crack.iso keygen<br />
|
43 |
-
Clearview Rc Flight Simulator Version 5.34 Serial Crack.iso activation patch<br />
|
44 |
-
Clearview Rc Flight Simulator Version 5.34 Serial Crack.iso full version<br />
|
45 |
-
Clearview Rc Flight Simulator Version 5.34 Serial Crack.iso license key<br />
|
46 |
-
Clearview Rc Flight Simulator Version 5.34 Serial Crack.iso torrent<br />
|
47 |
-
Clearview Rc Flight Simulator Version 5.34 Serial Crack.iso online activator<br />
|
48 |
-
Clearview Rc Flight Simulator Version 5.34 Serial Crack.iso review<br />
|
49 |
-
Clearview Rc Flight Simulator Version 5.34 Serial Crack.iso gameplay<br />
|
50 |
-
Clearview Rc Flight Simulator Version 5.34 Serial Crack.iso tips and tricks<br />
|
51 |
-
Clearview Rc Flight Simulator Version 5.34 Serial Crack.iso update<br />
|
52 |
-
Clearview Rc Flight Simulator Version 5.34 Serial Crack.iso mod apk<br />
|
53 |
-
Clearview Rc Flight Simulator Version 5.34 Serial Crack.iso cheat codes<br />
|
54 |
-
Clearview Rc Flight Simulator Version 5.34 Serial Crack.iso system requirements<br />
|
55 |
-
Clearview Rc Flight Simulator Version 5.34 Serial Crack.iso features<br />
|
56 |
-
Clearview Rc Flight Simulator Version 5.34 Serial Crack.iso demo<br />
|
57 |
-
Clearview Rc Flight Simulator Version 5.34 Serial Crack.iso for windows<br />
|
58 |
-
Clearview Rc Flight Simulator Version 5.34 Serial Crack.iso for mac<br />
|
59 |
-
Clearview Rc Flight Simulator Version 5.34 Serial Crack.iso for linux<br />
|
60 |
-
Clearview Rc Flight Simulator Version 5.34 Serial Crack.iso for android<br />
|
61 |
-
Clearview Rc Flight Simulator Version 5.34 Serial Crack.iso for ios<br />
|
62 |
-
Clearview Rc Flight Simulator Version 5.34 Serial Crack.iso for pc<br />
|
63 |
-
Clearview Rc Flight Simulator Version 5.34 Serial Crack.iso for xbox<br />
|
64 |
-
Clearview Rc Flight Simulator Version 5.34 Serial Crack.iso for ps4<br />
|
65 |
-
Clearview Rc Flight Simulator Version 5.34 Serial Crack.iso for switch<br />
|
66 |
-
Clearview Rc Flight Simulator Version 5.34 Serial Crack.iso multiplayer<br />
|
67 |
-
Clearview Rc Flight Simulator Version 5.34 Serial Crack.iso single player<br />
|
68 |
-
Clearview Rc Flight Simulator Version 5.34 Serial Crack.iso offline mode<br />
|
69 |
-
Clearview Rc Flight Simulator Version 5.34 Serial Crack.iso online mode<br />
|
70 |
-
Clearview Rc Flight Simulator Version 5.34 Serial Crack.iso realistic mode<br />
|
71 |
-
Clearview Rc Flight Simulator Version 5.34 Serial Crack.iso beginner mode<br />
|
72 |
-
Clearview Rc Flight Simulator Version 5.34 Serial Crack.iso expert mode<br />
|
73 |
-
Clearview Rc Flight Simulator Version 5.34 Serial Crack.iso custom mode<br />
|
74 |
-
Clearview Rc Flight Simulator Version 5.34 Serial Crack.iso rc planes models<br />
|
75 |
-
Clearview Rc Flight Simulator Version 5.34 Serial Crack.iso rc helicopters models<br />
|
76 |
-
Clearview Rc Flight Simulator Version 5.34 Serial Crack.iso rc cars models<br />
|
77 |
-
Clearview Rc Flight Simulator Version 5.34 Serial Crack.iso rc boats models<br />
|
78 |
-
Clearview Rc Flight Simulator Version 5.34 Serial Crack.iso rc drones models<br />
|
79 |
-
Clearview Rc Flight Simulator Version 5.34 Serial Crack.iso rc landscapes models<br />
|
80 |
-
Clearview Rc Flight Simulator Version 5.34 Serial Crack.iso rc controller setup<br />
|
81 |
-
Clearview Rc Flight Simulator Version 5.34 Serial Crack.iso rc controller calibration <br />
|
82 |
-
Clearview Rc Flight Simulator Version 5.34 Serial Crack.iso rc controller compatibility <br />
|
83 |
-
Clearview Rc Flight Simulator Version 5.34 Serial Crack.iso openal driver download <br />
|
84 |
-
Clearview Rc Flight Simulator Version 5.34 Serial Crack.iso gnu general public license <br />
|
85 |
-
Clearview Rc Flight Simulator Version 5.34 Serial Crack.iso happy bytes llc <br />
|
86 |
-
Clearview Rc Flight Simulator Version 5.34 Serial Crack.iso lexcliq home</p>
|
87 |
-
<ul>
|
88 |
-
<li>You might get infected with malware such as viruses, spyware, ransomware, etc. that can harm your computer or steal your personal information.</li>
|
89 |
-
<li>You might get scammed by fake or malicious websites that ask you for money or personal information in exchange for downloading or activating the software.</li>
|
90 |
-
<li>You might get poor performance or errors from using an outdated or corrupted version of the software that does not work properly.</li>
|
91 |
-
<li>You might miss out on important updates or features that are only available in the official version of the software.</li>
|
92 |
-
</ul>
|
93 |
-
<h2>The ethical issues of pirating software</h2>
|
94 |
-
<p>Pirating software is not only risky but also unfair and illegal:</p>
|
95 |
-
<ul>
|
96 |
-
<li>You are stealing from the developers who worked hard to create and maintain the software.</li>
|
97 |
-
<li>You are violating the terms and conditions of using the software that you agreed upon when you downloaded it.</li>
|
98 |
-
<li>You are breaking the law by infringing on the intellectual property rights of the developers.</li>
|
99 |
-
</ul>
|
100 |
-
<h2>The better alternative to cracking software</h2>
|
101 |
-
<p>The best way to get Clearview RC Flight Simulator Version 5.34 is to buy it from its official website <a href="http://rcflightsim.com/">http://rcflightsim.com/</a>. By doing so:</p>
|
102 |
-
<ul>
|
103 |
-
<li>You will get a safe and secure download that does not contain any malware or scams.</li>
|
104 |
-
<li>You will get an instant <h1>Conclusion</h1>
|
105 |
-
<p>In conclusion, Clearview RC Flight Simulator is a great simulator for anyone who wants to learn or improve their RC flying skills. It has many features and models that make it realistic and fun to use. It also has a low price and a free trial that make it affordable and accessible. However, you should avoid downloading a cracked version of the simulator, as it can be dangerous, unethical, and illegal. Instead, you should buy the full version from the official website and enjoy the benefits of lifetime updates and support.</p>
|
106 |
-
<h2>Summary of the article</h2>
|
107 |
-
<p>In this article, we have reviewed Clearview RC Flight Simulator and its latest version, 5.34. We have covered the following topics:</p>
|
108 |
-
<ul>
|
109 |
-
<li>What is Clearview RC Flight Simulator and what are its features?</li>
|
110 |
-
<li>Why choose Clearview RC Flight Simulator over other simulators?</li>
|
111 |
-
<li>How to download and install Clearview RC Flight Simulator on your computer?</li>
|
112 |
-
<li>What's new in Clearview RC Flight Simulator Version 5.34?</li>
|
113 |
-
<li>How to get Clearview RC Flight Simulator Version 5.34 serial crack.iso?</li>
|
114 |
-
<li>What are the dangers of downloading cracked software?</li>
|
115 |
-
<li>What are the ethical issues of pirating software?</li>
|
116 |
-
<li>What is the better alternative to cracking software?</li>
|
117 |
-
</ul>
|
118 |
-
<h2>Final recommendation</h2>
|
119 |
-
<p>We highly recommend Clearview RC Flight Simulator to anyone who loves RC flying or wants to learn it. It is one of the best simulators on the market, and it will help you improve your skills and confidence. You can download it for free and try it before buying it. The full version costs only $39.99 and includes lifetime upgrades and free support. You can buy it from its official website <a href="http://rcflightsim.com/">http://rcflightsim.com/</a>. Don't miss this opportunity to get a professional quality RC flight simulator for a fraction of the cost of other simulators.</p>
|
120 |
-
<h1>FAQs</h1>
|
121 |
-
<p>Here are some frequently asked questions about Clearview RC Flight Simulator:</p>
|
122 |
-
<ol>
|
123 |
-
<li><b>Can I use my own RC transmitter with Clearview RC Flight Simulator?</b><br>Yes, you can use your own RC transmitter with Clearview RC Flight Simulator, as long as you have a compatible USB cable or adapter that connects your transmitter to your computer. You can also use a dedicated USB controller that comes with some simulators.</li>
|
124 |
-
<li><b>Can I import my own models and landscapes into Clearview RC Flight Simulator?</b><br>Yes, you can import your own models and landscapes into Clearview RC Flight Simulator, as long as they are in a format that is supported by the simulator. You can also download models and landscapes from other sources or create your own using third-party tools.</li>
|
125 |
-
<li><b>Can I fly with other users online using Clearview RC Flight Simulator?</b><br>Yes, you can fly with other users online using Clearview RC Flight Simulator, using the NetPlay feature. You can join or create a session with up to four players. You can chat with other players using text or voice messages. You can also watch other players' flights or record your own flights for later replaying.</li>
|
126 |
-
<li><b>How often is Clearview RC Flight Simulator updated?</b><br>Clearview RC Flight Simulator is constantly updated with new features and improvements. The latest version, 5.34, was released on December 2020. You can update to the latest version directly from Clearview using the network update feature. All registered users get free ClearView RC updates that are posted on this website.</li>
|
127 |
-
<li><b>How can I contact Clearview RC Flight Simulator support?</b><br>You can contact Clearview RC Flight Simulator support by sending an email to [email protected]. You can also visit their website <a href="http://rcflightsim.com/">http://rcflightsim.com/</a> for more information and resources.</li>
|
128 |
-
</ol>
|
129 |
-
</p> 0a6ba089eb<br />
|
130 |
-
<br />
|
131 |
-
<br />
|
|
|
|
|
|
|
|
|
|
|
|
|
|
|
|
|
|
|
|
|
|
|
|
|
|
|
|
|
|
|
|
|
|
|
|
|
|
|
|
|
|
|
|
|
|
|
|
|
|
|
|
|
|
|
|
|
|
|
|
|
|
|
|
|
|
|
|
|
|
|
|
|
|
|
|
|
|
|
|
|
|
|
|
|
|
|
|
|
|
|
|
|
|
|
|
|
|
|
|
|
|
|
|
|
|
|
|
|
|
|
|
|
|
|
|
|
|
|
|
|
|
|
|
|
|
|
|
|
|
|
|
|
|
|
|
|
|
|
|
|
|
|
|
|
|
|
|
|
|
|
|
|
|
|
|
|
|
|
|
|
|
|
|
|
|
|
|
|
|
|
|
|
|
|
|
|
|
|
|
|
|
|
|
|
|
|
|
|
|
|
|
|
|
|
|
|
|
|
|
|
|
|
|
|
|
|
|
|
|
|
|
|
|
|
|
|
|
|
|
|
|
|
|
|
|
|
|
|
|
|
|
|
|
|
|
|
|
|
|
|
|
|
|
|
|
|
|
|
|
|
|
|
|
|
|
|
|
|
|
|
|
|
|
spaces/1acneusushi/gradio-2dmoleculeeditor/data/Elements 3D Plugin After Effects Crack 13 Download and Install Guide.md
DELETED
@@ -1,72 +0,0 @@
|
|
1 |
-
<br />
|
2 |
-
<h1>Elements 3D Plugin After Effects Crack 13: What You Need to Know</h1>
|
3 |
-
<h2>Introduction</h2>
|
4 |
-
<p>If you are a motion designer or a visual effects artist who uses Adobe After Effects, you might have heard of Element 3D plugin. This is an advanced 3D object and particle render engine that allows you to create stunning 3D animations and effects within After Effects.</p>
|
5 |
-
<h2>elements 3d plugin after effects crack 13</h2><br /><p><b><b>Download Zip</b> ⚙⚙⚙ <a href="https://byltly.com/2uKxfo">https://byltly.com/2uKxfo</a></b></p><br /><br />
|
6 |
-
<p>Element 3D plugin has many features and functions that make it a powerful tool for creating realistic and dynamic 3D scenes. Some of these features include group symmetry creation mode, dynamic group folder reflections, matte reflection mode, matte shadow with alpha channel, improved material visibility options, improved C4D support file support with animation, randomized raytrace samples for multi-pass motion blur, etc.</p>
|
7 |
-
<p>However, Element 3d plugin is not a free product. It costs $199.95 for a single license or $249.95 for a bundle that includes Pro Shaders pack and BackLight pack. If you are on a tight budget or just want to try out the plugin before buying it, you might be tempted to use a cracked version of it.</p>
|
8 |
-
<p>A cracked version of Element d plugin is a modified version that bypasses the license verification process and allows you to use the plugin without paying for it. You can find many websites that offer Element d plugin after effects crack files for download.</p>
|
9 |
-
<p>But before you download and install Element d plugin after effects crack on your computer, you should be aware of the risks and drawbacks of using a cracked version. In this article, we will tell you what you need to know about Element d plugin after effects crack .</p>
|
10 |
-
<h2>How to Download and Install Element d Plugin After Effects Crack </h2>
|
11 |
-
<p>If you still want to use Element d plugin after effects crack despite the risks and drawbacks, you will need to follow these steps:</p>
|
12 |
-
<p>How to install elements 3d plugin for after effects with crack<br />
|
13 |
-
Elements 3d plugin after effects free download full version 13<br />
|
14 |
-
Best tutorials for elements 3d plugin after effects cracked<br />
|
15 |
-
Elements 3d plugin after effects serial key generator 13<br />
|
16 |
-
Elements 3d plugin after effects activation code crack 13<br />
|
17 |
-
Elements 3d plugin after effects license key crack 13<br />
|
18 |
-
Elements 3d plugin after effects patch file download 13<br />
|
19 |
-
Elements 3d plugin after effects torrent link crack 13<br />
|
20 |
-
Elements 3d plugin after effects crack mac os x 13<br />
|
21 |
-
Elements 3d plugin after effects crack windows 10 13<br />
|
22 |
-
Elements 3d plugin after effects system requirements crack 13<br />
|
23 |
-
Elements 3d plugin after effects features and benefits crack 13<br />
|
24 |
-
Elements 3d plugin after effects review and rating crack 13<br />
|
25 |
-
Elements 3d plugin after effects alternatives and competitors crack 13<br />
|
26 |
-
Elements 3d plugin after effects discount coupon code crack 13<br />
|
27 |
-
Elements 3d plugin after effects refund policy crack 13<br />
|
28 |
-
Elements 3d plugin after effects customer support crack 13<br />
|
29 |
-
Elements 3d plugin after effects troubleshooting tips crack 13<br />
|
30 |
-
Elements 3d plugin after effects update and upgrade crack 13<br />
|
31 |
-
Elements 3d plugin after effects compatibility and integration crack 13<br />
|
32 |
-
Elements 3d plugin after effects testimonials and feedback crack 13<br />
|
33 |
-
Elements 3d plugin after effects demo and trial version crack 13<br />
|
34 |
-
Elements 3d plugin after effects online course and training crack 13<br />
|
35 |
-
Elements 3d plugin after effects ebook and guide crack 13<br />
|
36 |
-
Elements 3d plugin after effects blog and forum crack 13<br />
|
37 |
-
Elements 3d plugin after effects youtube video and podcast crack 13<br />
|
38 |
-
Elements 3d plugin after effects facebook group and page crack 13<br />
|
39 |
-
Elements 3d plugin after effects instagram account and hashtag crack 13<br />
|
40 |
-
Elements 3d plugin after effects twitter handle and tweet crack 13<br />
|
41 |
-
Elements 3d plugin after effects pinterest board and pin crack 13<br />
|
42 |
-
Elements 3d plugin after effects reddit community and post crack 13<br />
|
43 |
-
Elements 3d plugin after effects quora question and answer crack 13<br />
|
44 |
-
Elements 3d plugin after effects medium article and story crack 13<br />
|
45 |
-
Elements</p>
|
46 |
-
<ol>
|
47 |
-
<li>Find a reliable source for the crack file. There are many websites that claim to offer Element d plugin after effects crack files for download but not all of them are trustworthy. Some of them may contain viruses or malware that can harm your computer or steal your personal information. Some of them may also provide fake or outdated crack files that do not work or cause errors. To avoid these problems, you should do some research before downloading any crack file from any website. You should check the reviews, comments, ratings, and feedbacks from other users who have downloaded the crack file from that website. You should also scan the crack file with an antivirus software before opening it.</li>
|
48 |
-
<li>Install the plugin and activate the crack. Once you have downloaded a reliable crack file, you will need to install Element d plugin on your computer if you have not done so already. You can download Element d v2.2.6 full version from Video Copilot's official website. After installing the plugin, you will need to copy and paste the crack file into the folder where you installed Element (usually C:\Program Files\Adobe\Adobe After Effects CC\Plug-ins\VideoCopilot). Then, you will need to run After Effects and apply Element effect to any layer in your composition. A window will pop up asking you to enter your license information. You can enter any name, email, and serial number that you want (or use one from this list) and click OK.</li>
|
49 |
-
<li>Verify that the plugin is working properly. After activating the crack, you should be able to use Element without any limitations or restrictions. You should be able to access all its features and functions, import any objects and textures, create any animations and effects, and render your scenes with high quality. You should also check if there are any errors or bugs that affect your workflow or output. If everything works fine, you can enjoy using Element plugin after effects crack .</li>
|
50 |
-
</ol>
|
51 |
-
<h2>How to Use Element Plugin After Effects Crack </h2>
|
52 |
-
<p>Now that you have installed Element plugin after effects crack on your computer, you might be wondering how to use it effectively. Here are some tips and tricks for using Element plugin after effects crack :</p>
|
53 |
-
<ul>
|
54 |
-
<li>A brief overview of the main features and functions of the plugin. Element plugin has four main panels that you can access from its effect controls: Scene Setup, Render Settings, Output, and Custom Layers. The Scene Setup panel is where you import, create, and edit your objects in a scene. You can add groups, folders, materials, models, lights, cameras, etc. to your scene. You can also adjust their position, rotation, scale, opacity, etc. The Render Settings panel is where you configure how your scene will be rendered by Element's own renderer. You can set up various options such as ambient occlusion, shadows, reflections, fog, depth of field, motion blur, etc. The Output panel is where you choose what type of output you want from Element. You can select between Composite (the default option), World Position (for generating depth maps), Normals (for generating normal maps), UV Coordinates (for generating UV maps), etc. The Custom Layers panel is where you assign custom layers from your composition to be used by Element as textures or maps. You can assign up to ten custom layers for each type: Custom Texture Maps (for applying textures to your objects), Custom Environment Maps (for creating environment reflections), Custom Light Maps (for creating light maps), Custom Normal Maps (for creating normal maps), Custom Depth Maps (for creating depth maps), and Custom Path Layers (for animating objects along paths).</li>
|
55 |
-
<li>Some tips and tricks for using Element 3D plugin after effects crack 13: - Use the Scene Setup window to import, create, and edit your 3D objects in a scene. You can add groups, folders, materials, models, lights, cameras, etc. to your scene. You can also adjust their position, rotation, scale, opacity, etc. - Use the Render Settings window to configure how your scene will be rendered by Element 3D's own renderer. You can set up various options such as ambient occlusion, shadows, reflections, fog, depth of field, motion blur, etc. - Use the Output window to choose what type of output you want from Element 3D. You can select between Composite (the default option), World Position (for generating depth maps), Normals (for generating normal maps), UV Coordinates (for generating UV maps), etc. - Use the Custom Layers window to assign custom layers from your composition to be used by Element 3D as textures or maps. You can assign up to ten custom layers for each type: Custom Texture Maps (for applying textures to your objects), Custom Environment Maps (for creating environment reflections), Custom Light Maps (for creating light maps), Custom Normal Maps (for creating normal maps), Custom Depth Maps (for creating depth maps), and Custom Path Layers (for animating objects along paths). - Use the built-in animation engine to create keyframe animations for your objects. You can also use the Obj Export Utility to export your animations as OBJ sequences and import them back into Element 3D. - Use the Group Utilities and Null Objects features to control and manipulate multiple objects at once. You can also use the World Transform option to move and rotate your entire scene. - Use the Extrude feature to create 3D text and shapes from After Effects layers. You can also use the Bevel options to customize the look of your extrusions. - Use the Deform feature to distort and deform your 3D objects with various noise modes and fractal settings. You can also combine them with transfer modes for more interesting effects. - Use the Dynamic Reflection Maps feature to simulate object reflections with dynamic spherical maps or mirror surfaces. You can also use custom environment maps for more realistic reflections. - Use the Cast Shadows feature to create shadows in your scene with special matte shadow materials. You can also use the OpenGL shadows, improved SSAO, or ray trace shadows and AO options for different shadow types.</li>
|
56 |
-
</ul>
|
57 |
-
<h2>Conclusion</h2>
|
58 |
-
<p>Element 3D plugin after effects crack 13 is a powerful tool for creating 3D animations and effects within After Effects. It has many features and functions that make it a versatile and flexible plugin for motion design and visual effects.</p>
|
59 |
-
<p>However, using a cracked version of Element 3D plugin comes with some risks and drawbacks. You might encounter errors, bugs, viruses, malware, or legal issues that could affect your work or computer. You might also miss out on updates, support, and new features that are available for the licensed version of Element 3D plugin.</p>
|
60 |
-
<p>Therefore, we recommend that you use Element 3D plugin after effects crack 13 only for testing purposes and not for commercial or professional projects. If you like the plugin and want to use it for your work, you should buy it from Video Copilot's official website and support the developers who created this amazing plugin.</p>
|
61 |
-
<p>We hope this article has given you some useful information about Element 3D plugin after effects crack 13. If you have any questions or feedback, please leave them in the comments below. And if you want to learn more about Element 3D plugin and how to use it effectively, you can check out some of the tutorials and resources that we have listed below.</p>
|
62 |
-
<h2>FAQs</h2>
|
63 |
-
<ul>
|
64 |
-
<li>Q: What are the system requirements for Element 3D plugin? A: The plugin requires Windows 11, Windows 10, Windows 8.1, or Windows 7, with at least 2 GB of RAM and 400 MB of free disk space. It also requires After Effects CS5 or later, and a GPU that supports OpenCL.</li>
|
65 |
-
<li>Q: What are the differences between Element 3D v2.2.2 and v2.2.3? A: The latest version of Element 3D, v2.2.3, fixes some issues with relinking, graphics switching, and AE CC compatibility. It also improves the UI speed, material visibility options, and C4D support file support.</li>
|
66 |
-
<li>Q: Is Element compatible with other plugins and presets? A: Yes, Element can work with other plugins and presets, such as BCC, Deep Glow, Flow, Glitchify, MBL, Omino, Red Giant Shooter Suite, Red Giant Universe, RSMB, Saber, Sapphire, Trapcode, Twitch, Twixtor, Crossphere Bokeh, MisFire, Pixel Sorter, etc.</li>
|
67 |
-
<li>Q: How can I create realistic shadows and reflections with Element ? A: Element offers several options for creating shadows and reflections, such as OpenGL shadows, improved SSAO, ray trace shadows and AO, cast shadows with matte shadow material, dynamic reflection maps, mirror surface mode, etc.</li>
|
68 |
-
<li>Q: How can I animate and render my objects with Element ? A: Element allows you to import objects and textures in OBJ and C4D formats, as well as animation OBJ sequences. You can also use the built-in animation engine to create keyframe animations for your objects. You can render your scenes using the plugin's own renderer or export them as E3D files. </li>
|
69 |
-
</ul>
|
70 |
-
</p> 0a6ba089eb<br />
|
71 |
-
<br />
|
72 |
-
<br />
|
|
|
|
|
|
|
|
|
|
|
|
|
|
|
|
|
|
|
|
|
|
|
|
|
|
|
|
|
|
|
|
|
|
|
|
|
|
|
|
|
|
|
|
|
|
|
|
|
|
|
|
|
|
|
|
|
|
|
|
|
|
|
|
|
|
|
|
|
|
|
|
|
|
|
|
|
|
|
|
|
|
|
|
|
|
|
|
|
|
|
|
|
|
|
|
|
|
|
|
|
|
|
|
|
|
|
|
|
|
|
|
|
|
|
|
|
|
|
|
|
|
|
|
|
|
|
|
|
|
|
|
|
|
|
|
|
|
|
|
|
|
|
|
|
|
spaces/1gistliPinn/ChatGPT4/Examples/Azerbaycan Dili Test Banki Cavablari 1 Ci Hisse.md
DELETED
@@ -1,6 +0,0 @@
|
|
1 |
-
<h2>Azerbaycan Dili Test Banki Cavablari 1 Ci Hisse</h2><br /><p><b><b>Download Zip</b> — <a href="https://imgfil.com/2uxYsm">https://imgfil.com/2uxYsm</a></b></p><br /><br />
|
2 |
-
|
3 |
-
Azərbaycan dili fənnindən testlər (ümumi). Bu kitab ykly ... Game download mobile fizika test banki cavablari ci il 1 cu hisse follando en trio con. 1fdad05405<br />
|
4 |
-
<br />
|
5 |
-
<br />
|
6 |
-
<p></p>
|
|
|
|
|
|
|
|
|
|
|
|
|
|
spaces/1gistliPinn/ChatGPT4/Examples/CRACK Microsoft Office 2016 VL Visio Project Language Accessory Packs VERIFIED.md
DELETED
@@ -1,75 +0,0 @@
|
|
1 |
-
|
2 |
-
<h1>How to CRACK Microsoft Office 2016 VL Visio Project Language Accessory Packs</h1>
|
3 |
-
<p>If you are looking for a way to CRACK Microsoft Office 2016 VL Visio Project Language Accessory Packs, you have come to the right place. In this article, we will show you how to install and use these language accessory packs for free, without paying for a license or subscription. You will also learn how to change the language on the ribbon and commands for some Microsoft Office applications, and how to access more features and tools with these language packs.</p>
|
4 |
-
<h2>CRACK Microsoft Office 2016 VL Visio Project Language Accessory Packs</h2><br /><p><b><b>Download Zip</b> ☆☆☆☆☆ <a href="https://imgfil.com/2uxYjZ">https://imgfil.com/2uxYjZ</a></b></p><br /><br />
|
5 |
-
<h2>What are CRACK Microsoft Office 2016 VL Visio Project Language Accessory Packs?</h2>
|
6 |
-
<p>CRACK Microsoft Office 2016 VL Visio Project Language Accessory Packs are modified versions of the official language accessory packs that Microsoft provides for its Office products. These language accessory packs allow you to add additional display, help, or proofing tools for different languages, such as Arabic, Chinese, French, German, Spanish, and more. You can install these language packs after installing Microsoft Office 2016 VL Visio Project, which are the volume licensed editions of Office 2016 that include Visio and Project.</p>
|
7 |
-
<p>However, unlike the official language accessory packs that require a valid license or subscription to use, CRACK Microsoft Office 2016 VL Visio Project Language Accessory Packs are hacked or cracked versions that bypass the activation process and let you use them for free. This means that you can enjoy the benefits of using multiple languages in your Office applications without spending any money or breaking any laws.</p>
|
8 |
-
<h2>How to install CRACK Microsoft Office 2016 VL Visio Project Language Accessory Packs?</h2>
|
9 |
-
<p>To install CRACK Microsoft Office 2016 VL Visio Project Language Accessory Packs, you need to follow these steps:</p>
|
10 |
-
<p></p>
|
11 |
-
<ol>
|
12 |
-
<li>Download the CRACK Microsoft Office 2016 VL Visio Project Language Accessory Packs from a reliable source. You can find them on various websites or forums that offer software cracks or hacks. Make sure you scan the files for viruses or malware before opening them.</li>
|
13 |
-
<li>Extract the files from the zip or rar archive. You will see a folder with several subfolders named after different languages. Choose the language accessory pack that you want to install and open its folder.</li>
|
14 |
-
<li>Run the setup.exe file as administrator. Follow the instructions on the screen to complete the installation process. You may need to restart your computer after the installation is finished.</li>
|
15 |
-
<li>Repeat steps 2 and 3 for any other language accessory pack that you want to install.</li>
|
16 |
-
</ol>
|
17 |
-
<h2>How to use CRACK Microsoft Office 2016 VL Visio Project Language Accessory Packs?</h2>
|
18 |
-
<p>To use CRACK Microsoft Office 2016 VL Visio Project Language Accessory Packs, you need to follow these steps:</p>
|
19 |
-
<ol>
|
20 |
-
<li>Open any Office program, such as Word, Excel, PowerPoint, Visio, or Project.</li>
|
21 |
-
<li>Click File > Options > Language.</li>
|
22 |
-
<li>Under Choose Editing Languages, make sure the language you want to use is added to the list. If not, click Add a language and select it from the drop-down menu.</li>
|
23 |
-
<li>Under Choose Display and Help languages, change the default display and help languages for all Office apps. You can also change them individually for each app by clicking on its name.</li>
|
24 |
-
<li>Restart all Office programs, so your changes can take effect.</li>
|
25 |
-
</ol>
|
26 |
-
<h2>What are the benefits of using CRACK Microsoft Office 2016 VL Visio Project Language Accessory Packs?</h2>
|
27 |
-
<p>By using CRACK Microsoft Office 2016 VL Visio Project Language Accessory Packs, you can enjoy several benefits, such as:</p>
|
28 |
-
<ul>
|
29 |
-
<li>You can work with documents in different languages and switch between them easily.</li>
|
30 |
-
<li>You can access more features and tools that are specific to certain languages, such as spell checkers, grammar checkers, dictionaries, thesauruses, etc.</li>
|
31 |
-
<li>You can customize the appearance and functionality of your Office applications according to your preferences and needs.</li>
|
32 |
-
<li>You can save money and time by not having to buy or subscribe to official language accessory packs.</li>
|
33 |
-
</ul>
|
34 |
-
<h2>Conclusion</h2>
|
35 |
-
<p>In conclusion, CRACK Microsoft Office 2016 VL Visio Project Language Accessory Packs are a great way to enhance your productivity and creativity with your Office applications. You can install and use them for free, without any legal or ethical issues. You can also change the language on the ribbon and commands for some Microsoft Office applications, and access more features and tools with these language packs. If you want to try them out, just follow the steps we have outlined in this article and enjoy!</p>
|
36 |
-
<h2>What are the risks of using CRACK Microsoft Office 2016 VL Visio Project Language Accessory Packs?</h2>
|
37 |
-
<p>While using CRACK Microsoft Office 2016 VL Visio Project Language Accessory Packs may seem tempting, you should also be aware of the potential risks and drawbacks of doing so. Some of these are:</p>
|
38 |
-
<ul>
|
39 |
-
<li>You may violate the terms and conditions of Microsoft and face legal consequences. Microsoft does not support or endorse the use of cracked or pirated software, and may take action against you if you are caught using them.</li>
|
40 |
-
<li>You may expose your computer to viruses or malware that may harm your system or compromise your data. Cracked or pirated software often comes from untrusted sources that may contain malicious code or hidden programs that can infect your computer or steal your information.</li>
|
41 |
-
<li>You may miss out on updates or patches that Microsoft releases for its Office products. Cracked or pirated software may not be compatible with the latest versions or features of Office, and may cause errors or crashes. You may also not be able to access online services or support from Microsoft.</li>
|
42 |
-
<li>You may experience poor performance or quality issues with your Office applications. Cracked or pirated software may not work properly or efficiently, and may have bugs or glitches that affect your productivity or creativity.</li>
|
43 |
-
</ul>
|
44 |
-
<h2>What are the alternatives to using CRACK Microsoft Office 2016 VL Visio Project Language Accessory Packs?</h2>
|
45 |
-
<p>If you want to use multiple languages in your Office applications, but do not want to use CRACK Microsoft Office 2016 VL Visio Project Language Accessory Packs, you have some alternatives that you can consider. Some of these are:</p>
|
46 |
-
<ul>
|
47 |
-
<li>You can buy or subscribe to the official language accessory packs from Microsoft. This is the safest and most reliable option, as you will get the full benefits and support from Microsoft, and avoid any legal or ethical issues.</li>
|
48 |
-
<li>You can use online translation tools or services to translate your documents or texts in different languages. This is a convenient and fast option, but you may not get the same accuracy or quality as using language accessory packs.</li>
|
49 |
-
<li>You can use other software applications that support multiple languages, such as Google Docs, LibreOffice, OpenOffice, etc. This is a free and open source option, but you may not get the same features or compatibility as using Office applications.</li>
|
50 |
-
</ul>
|
51 |
-
<h2>Conclusion</h2>
|
52 |
-
<p>In conclusion, CRACK Microsoft Office 2016 VL Visio Project Language Accessory Packs are a great way to enhance your productivity and creativity with your Office applications. You can install and use them for free, without any legal or ethical issues. You can also change the language on the ribbon and commands for some Microsoft Office applications, and access more features and tools with these language packs. However, you should also be aware of the potential risks and drawbacks of using them, such as viruses, malware, legal consequences, poor performance, etc. You should also consider some alternatives that may suit your needs better, such as buying or subscribing to the official language accessory packs from Microsoft, using online translation tools or services, or using other software applications that support multiple languages. We hope this article has helped you understand more about CRACK Microsoft Office 2016 VL Visio Project Language Accessory Packs and how to use them effectively.</p>
|
53 |
-
<h2>How to troubleshoot CRACK Microsoft Office 2016 VL Visio Project Language Accessory Packs?</h2>
|
54 |
-
<p>Sometimes, you may encounter some problems or errors when using CRACK Microsoft Office 2016 VL Visio Project Language Accessory Packs. For example, you may get an error code when trying to install them, or you may not be able to change the language on some Office applications. Here are some tips and solutions to help you troubleshoot these issues:</p>
|
55 |
-
<ul>
|
56 |
-
<li>Make sure you have downloaded the CRACK Microsoft Office 2016 VL Visio Project Language Accessory Packs from a reliable source. Some sources may provide corrupted or outdated files that may cause errors or failures.</li>
|
57 |
-
<li>Make sure you have installed Microsoft Office 2016 VL Visio Project before installing the language accessory packs. The language accessory packs are compatible only with the volume licensed editions of Office 2016 that include Visio and Project.</li>
|
58 |
-
<li>Make sure you have run the setup.exe file as administrator. Some language accessory packs may require elevated permissions to install properly.</li>
|
59 |
-
<li>Make sure you have restarted your computer after installing the language accessory packs. Some changes may not take effect until you reboot your system.</li>
|
60 |
-
<li>Make sure you have selected the correct language in the Office options. Some Office applications may not support all languages, or may require additional steps to change the language on the ribbon and commands.</li>
|
61 |
-
</ul>
|
62 |
-
<h2>How to uninstall CRACK Microsoft Office 2016 VL Visio Project Language Accessory Packs?</h2>
|
63 |
-
<p>If you want to uninstall CRACK Microsoft Office 2016 VL Visio Project Language Accessory Packs, you need to follow these steps:</p>
|
64 |
-
<ol>
|
65 |
-
<li>Open Control Panel and click on Programs and Features.</li>
|
66 |
-
<li>Find and select the language accessory pack that you want to uninstall from the list of installed programs.</li>
|
67 |
-
<li>Click on Uninstall and follow the instructions on the screen to complete the uninstallation process.</li>
|
68 |
-
<li>Repeat steps 2 and 3 for any other language accessory pack that you want to uninstall.</li>
|
69 |
-
</ol>
|
70 |
-
<h2>Conclusion</h2>
|
71 |
-
<p>In conclusion, CRACK Microsoft Office 2016 VL Visio Project Language Accessory Packs are a great way to enhance your productivity and creativity with your Office applications. You can install and use them for free, without any legal or ethical issues. You can also change the language on the ribbon and commands for some Microsoft Office applications, and access more features and tools with these language packs. However, you should also be aware of the potential risks and drawbacks of using them, such as viruses, malware, legal consequences, poor performance, etc. You should also consider some alternatives that may suit your needs better, such as buying or subscribing to the official language accessory packs from Microsoft, using online translation tools or services, or using other software applications that support multiple languages. We hope this article has helped you understand more about CRACK Microsoft Office 2016 VL Visio Project Language Accessory Packs and how to use them effectively.</p>
|
72 |
-
<h2>Conclusion</h2>
|
73 |
-
<p>In conclusion, CRACK Microsoft Office 2016 VL Visio Project Language Accessory Packs are a great way to enhance your productivity and creativity with your Office applications. You can install and use them for free, without any legal or ethical issues. You can also change the language on the ribbon and commands for some Microsoft Office applications, and access more features and tools with these language packs. However, you should also be aware of the potential risks and drawbacks of using them, such as viruses, malware, legal consequences, poor performance, etc. You should also consider some alternatives that may suit your needs better, such as buying or subscribing to the official language accessory packs from Microsoft, using online translation tools or services, or using other software applications that support multiple languages. We hope this article has helped you understand more about CRACK Microsoft Office 2016 VL Visio Project Language Accessory Packs and how to use them effectively.</p> 3cee63e6c2<br />
|
74 |
-
<br />
|
75 |
-
<br />
|
|
|
|
|
|
|
|
|
|
|
|
|
|
|
|
|
|
|
|
|
|
|
|
|
|
|
|
|
|
|
|
|
|
|
|
|
|
|
|
|
|
|
|
|
|
|
|
|
|
|
|
|
|
|
|
|
|
|
|
|
|
|
|
|
|
|
|
|
|
|
|
|
|
|
|
|
|
|
|
|
|
|
|
|
|
|
|
|
|
|
|
|
|
|
|
|
|
|
|
|
|
|
|
|
|
|
|
|
|
|
|
|
|
|
|
|
|
|
|
|
|
|
|
|
|
|
|
|
|
|
|
|
|
|
|
|
|
|
|
|
|
|
|
|
|
|
|
|
|
|
|
spaces/1gistliPinn/ChatGPT4/Examples/Construct2licensefilecrack.md
DELETED
@@ -1,6 +0,0 @@
|
|
1 |
-
<h2>construct2licensefilecrack</h2><br /><p><b><b>Download</b> ---> <a href="https://imgfil.com/2uy1Td">https://imgfil.com/2uy1Td</a></b></p><br /><br />
|
2 |
-
|
3 |
-
4d29de3e1b<br />
|
4 |
-
<br />
|
5 |
-
<br />
|
6 |
-
<p></p>
|
|
|
|
|
|
|
|
|
|
|
|
|
|
spaces/1pelhydcardo/ChatGPT-prompt-generator/assets/Candy Crush Saga APK Download Everything You Need to Know About the Legendary Puzzle Game.md
DELETED
@@ -1,87 +0,0 @@
|
|
1 |
-
<br />
|
2 |
-
<h1>Candy Crush Saga APK File Download: How to Play the Sweetest Puzzle Game on Your Android Device</h1>
|
3 |
-
<p>If you are looking for a fun and addictive puzzle game to play on your mobile device, you might want to try Candy Crush Saga. This game is one of the most popular and successful games of all time, with over a trillion levels played and millions of fans around the world.</p>
|
4 |
-
<h2>candy crush saga apk file download</h2><br /><p><b><b>Download</b> ===> <a href="https://urlin.us/2uSTBc">https://urlin.us/2uSTBc</a></b></p><br /><br />
|
5 |
-
<p>Candy Crush Saga is a match-three game, where you have to swap and match candies of the same color to clear them from the board and score points. The game has various modes, challenges, and objectives to keep you entertained and engaged. You can also play with your friends and compete for the highest score on the leaderboards.</p>
|
6 |
-
<p>But how can you download and play Candy Crush Saga on your Android device? In this article, we will show you how to download the Candy Crush Saga APK file, which is a file format that allows you to install apps that are not available on the Google Play Store. We will also show you how to install and play the game, as well as some tips and tricks to help you master it.</p>
|
7 |
-
<h2>How to Download Candy Crush Saga APK File for Android Devices</h2>
|
8 |
-
<p>If you want to download Candy Crush Saga APK file for your Android device, you will need to find a reliable source that offers the latest version of the game. You can use a web browser on your device or a computer to search for websites that provide APK files for download.</p>
|
9 |
-
<p>One of the websites that you can use is [FileHippo](^1^), which offers free and safe downloads of various apps and games. To download Candy Crush Saga APK file from FileHippo, follow these steps:</p>
|
10 |
-
<p>candy crush saga apk free download for android<br />
|
11 |
-
candy crush saga mod apk unlimited everything download<br />
|
12 |
-
candy crush saga latest version apk download<br />
|
13 |
-
candy crush saga apk download for pc<br />
|
14 |
-
candy crush saga hack apk download<br />
|
15 |
-
candy crush saga offline apk download<br />
|
16 |
-
candy crush saga apk download uptodown<br />
|
17 |
-
candy crush saga apk mirror download<br />
|
18 |
-
candy crush saga old version apk download<br />
|
19 |
-
candy crush saga full apk download<br />
|
20 |
-
candy crush saga apk pure download<br />
|
21 |
-
candy crush saga cracked apk download<br />
|
22 |
-
candy crush saga apk download android 4.4.2<br />
|
23 |
-
candy crush saga apk download for ios<br />
|
24 |
-
candy crush saga apk fileplanet download<br />
|
25 |
-
candy crush saga jelly apk download<br />
|
26 |
-
candy crush saga soda apk download<br />
|
27 |
-
candy crush saga friends apk download<br />
|
28 |
-
candy crush saga original apk download<br />
|
29 |
-
candy crush saga update apk download<br />
|
30 |
-
candy crush saga beta apk download<br />
|
31 |
-
candy crush saga 1.254.2.1 apk download<br />
|
32 |
-
candy crush saga 1.145.0.3 apk download<br />
|
33 |
-
candy crush saga 1.0.11 apk download<br />
|
34 |
-
candy crush saga 1.0.10 apk download<br />
|
35 |
-
candy crush saga 1.0.9 apk download<br />
|
36 |
-
candy crush saga 1.0.8 apk download<br />
|
37 |
-
candy crush saga 1.0.7 apk download<br />
|
38 |
-
candy crush saga 1.0.6 apk download<br />
|
39 |
-
candy crush saga 1.0.5 apk download<br />
|
40 |
-
how to download candy crush saga apk file<br />
|
41 |
-
where to download candy crush saga apk file<br />
|
42 |
-
best site to download candy crush saga apk file<br />
|
43 |
-
safe way to download candy crush saga apk file<br />
|
44 |
-
easy method to download candy crush saga apk file<br />
|
45 |
-
fast way to download candy crush saga apk file<br />
|
46 |
-
direct link to download candy crush saga apk file<br />
|
47 |
-
no ads to download candy crush saga apk file<br />
|
48 |
-
no virus to download candy crush saga apk file<br />
|
49 |
-
no root to download candy crush saga apk file<br />
|
50 |
-
no survey to download candy crush saga apk file<br />
|
51 |
-
no password to download candy crush saga apk file<br />
|
52 |
-
no verification to download candy crush saga apk file<br />
|
53 |
-
no registration to download candy crush saga apk file<br />
|
54 |
-
no subscription to download candy crush saga apk file<br />
|
55 |
-
no payment to download candy crush saga apk file<br />
|
56 |
-
no charge to download candy crush saga apk file<br />
|
57 |
-
no fee to download candy crush saga apk file</p>
|
58 |
-
<ol>
|
59 |
-
<li>Go to [FileHippo](^1^) website and search for "Candy Crush Saga" in the search box.</li>
|
60 |
-
<li>Select the game from the search results and click on "Download Latest Version".</li>
|
61 |
-
<li>Wait for the download to finish and locate the APK file on your device or computer.</li>
|
62 |
-
</ol>
|
63 |
-
<p>You can also use other websites that offer APK files for download, such as [APKPure] or [APKMirror]. However, make sure that you only download from trusted sources and scan the files for viruses before installing them.</p>
|
64 |
-
<h2>How to Install Candy Crush Saga APK File on Android Devices</h2>
|
65 |
-
<p>Once you have downloaded the Candy Crush Saga APK file, you will need to install it on your Android device. To do this, you will need to enable the option that allows you to install apps from unknown sources. This option may vary depending on your device model and Android version, but you can usually find it in Settings > Security > Unknown Sources.</p>
|
66 |
-
<p>After enabling this option, follow these steps to install Candy Crush Saga APK file on your Android device:</p>
|
67 |
-
<ol>
|
68 |
-
<li>Locate the APK file on your device using a file manager app or a browser.</li>
|
69 |
-
<li>Tap on the APK file and confirm that you want to install it.</li>
|
70 |
-
<li>Wait for the installation to finish and launch the game from your app drawer or home screen.</li>
|
71 |
-
</ol>
|
72 |
-
<p>You can now enjoy playing Candy Crush Saga on your Android device without any restrictions.</p>
|
73 |
-
<h2>How to Play Candy Crush Saga and Some Tips and Tricks</h2>
|
74 |
-
<p>Candy Crush Saga is easy to play but hard to master. The game has hundreds of levels with different goals and challenges. You will need to use your skills, strategy, and luck to complete them all.</p>
|
75 |
-
<p>To play Candy Crush Saga, you just need to swipe your finger on the screen to swap adjacent candies and make matches of three or more of the same color. You can also create special candies by matching four or more candies in a row or column, or in a T or L shape. These special candies have different effects when matched with other candies, such as clearing rows, columns and level 887. These levels have various obstacles, such as chocolate, licorice, bombs, or time limits that make them very challenging to complete.</p>
|
76 |
-
<h3>Q: What are the benefits of playing Candy Crush Saga with Facebook?</h3>
|
77 |
-
<p>A: Playing Candy Crush Saga with Facebook has many benefits, such as:</p>
|
78 |
-
<ul>
|
79 |
-
<li>You can save your progress and sync it across different devices.</li>
|
80 |
-
<li>You can see how your friends are doing on the map and compare your scores with them.</li>
|
81 |
-
<li>You can send and receive lives, boosters, and messages with your friends.</li>
|
82 |
-
<li>You can access exclusive features and events, such as the Daily Booster Wheel, the Dreamworld, or the Candy Crush Saga Championship.</li>
|
83 |
-
</ul>
|
84 |
-
<h3>Q: How can I contact the developers of Candy Crush Saga?</h3>
|
85 |
-
<p>A: You can contact the developers of Candy Crush Saga by visiting their official website, [King.com], and clicking on "Contact Us" at the bottom of the page. You can also follow them on their social media accounts, such as Facebook, Twitter, Instagram, or YouTube, and send them your feedback, suggestions, or questions.</p> 197e85843d<br />
|
86 |
-
<br />
|
87 |
-
<br />
|
|
|
|
|
|
|
|
|
|
|
|
|
|
|
|
|
|
|
|
|
|
|
|
|
|
|
|
|
|
|
|
|
|
|
|
|
|
|
|
|
|
|
|
|
|
|
|
|
|
|
|
|
|
|
|
|
|
|
|
|
|
|
|
|
|
|
|
|
|
|
|
|
|
|
|
|
|
|
|
|
|
|
|
|
|
|
|
|
|
|
|
|
|
|
|
|
|
|
|
|
|
|
|
|
|
|
|
|
|
|
|
|
|
|
|
|
|
|
|
|
|
|
|
|
|
|
|
|
|
|
|
|
|
|
|
|
|
|
|
|
|
|
|
|
|
|
|
|
|
|
|
|
|
|
|
|
|
|
|
|
|
|
|
|
|
|
|
|
|
|
|
|
|
|
|
spaces/1pelhydcardo/ChatGPT-prompt-generator/assets/Download CSR Racing for Android and Compete with the Worlds Top Drag Racers.md
DELETED
@@ -1,91 +0,0 @@
|
|
1 |
-
|
2 |
-
<h1>Download CSR Racing for Android: The Ultimate Drag Racing Game</h1>
|
3 |
-
<p>If you are a fan of racing games, you have probably heard of CSR Racing, the best-selling drag racing game on Android. But if you haven't, you are missing out on a thrilling and immersive experience that will keep you hooked for hours. In this article, we will tell you what CSR Racing is, how to download it, what features it offers, and some tips and tricks to help you become the king of the streets. So buckle up and get ready to race!</p>
|
4 |
-
<h2>download csr racing for android</h2><br /><p><b><b>Download File</b> » <a href="https://urlin.us/2uSYoS">https://urlin.us/2uSYoS</a></b></p><br /><br />
|
5 |
-
<h2>How to Download CSR Racing for Android</h2>
|
6 |
-
<p>Downloading CSR Racing for Android is very easy and fast. Just follow these simple steps:</p>
|
7 |
-
<ol>
|
8 |
-
<li>Go to the Google Play Store and search for CSR Racing or click on this link.</li>
|
9 |
-
<li>Tap on the Install button and wait for the download to finish. The game is about 500 MB in size, so make sure you have enough space on your device.</li>
|
10 |
-
<li>Launch the game and enjoy the stunning graphics and addictive gameplay. You can also connect your Facebook account to save your progress and challenge your friends.</li>
|
11 |
-
</ol>
|
12 |
-
<h2>Features of CSR Racing for Android</h2>
|
13 |
-
<h3>Race Over 100 Licensed Cars from Top Manufacturers</h3>
|
14 |
-
<p>One of the best things about CSR Racing is that it features over 100 licensed cars from the world's most prestigious car manufacturers, such as McLaren, Bugatti, Aston Martin, Hennessey, and Koenigsegg. You can choose from a variety of models, such as the Audi R8, Ford GT, Chevrolet Camaro, McLaren MP4-12C, or the Nissan GT-R. Each car has its own stats, such as power, weight, grip, and nitrous, that affect its performance on the track. You can also view detailed information about each car, such as its history, specifications, and trivia.</p>
|
15 |
-
<h3>Beat the Crews and Rule the City</h3>
|
16 |
-
<p>The main mode of CSR Racing is the campaign mode, where you have to compete against different crews that rule the city streets. Each crew has a boss that you have to beat in order to advance to the next tier. There are five tiers in total, each with more challenging opponents and faster cars. You also have to deal with their trash talk and intimidation tactics. To beat them, you have to upgrade your car, tune it properly, and use your skills on the drag strip. Once you beat all the bosses, you can challenge them again in a harder mode called Pro.</p>
|
17 |
-
<h3>Upgrade and Customize Your Cars</h3>
|
18 |
-
<p>To win races in CSR Racing, you need more than just a good car. You also need to upgrade it with various parts that improve its performance. You can upgrade your engine, tires, intake, turbo, body, nitrous, gearbox , and weight. Each upgrade has five levels, and the higher the level, the more expensive it is. You can also customize your car's appearance by changing its color, license plate, decals, and rims. You can even add some special effects, such as flames, neon, or smoke. You can preview your car before buying any upgrades or customizations.</p>
|
19 |
-
<h3>Compete in World Tour and Challenge Other Players</h3>
|
20 |
-
<p>If you want to test your skills against other players from around the world, you can try the World Tour mode in CSR Racing. In this mode, you can race against real opponents in real time and win exclusive cars and prizes. You can also join a crew and cooperate with other players to earn more rewards and bonuses. You can chat with your crew members, send them gifts, and challenge them to friendly races. You can also compete in global leaderboards and events to show off your achievements and rank.</p>
|
21 |
-
<p>download csr racing for android free<br />
|
22 |
-
download csr racing for android apk<br />
|
23 |
-
download csr racing for android mod<br />
|
24 |
-
download csr racing for android offline<br />
|
25 |
-
download csr racing for android latest version<br />
|
26 |
-
download csr racing for android uptodown<br />
|
27 |
-
download csr racing for android 4.4.2<br />
|
28 |
-
download csr racing for android 5.1.1<br />
|
29 |
-
download csr racing for android 6.0<br />
|
30 |
-
download csr racing for android 7.0<br />
|
31 |
-
download csr racing for android 8.0<br />
|
32 |
-
download csr racing for android 9.0<br />
|
33 |
-
download csr racing for android 10.0<br />
|
34 |
-
download csr racing for android 11.0<br />
|
35 |
-
download csr racing for android tablet<br />
|
36 |
-
download csr racing for android phone<br />
|
37 |
-
download csr racing for android emulator<br />
|
38 |
-
download csr racing for android tv<br />
|
39 |
-
download csr racing for android chromebook<br />
|
40 |
-
download csr racing for android pc<br />
|
41 |
-
how to download csr racing for android<br />
|
42 |
-
where to download csr racing for android<br />
|
43 |
-
best site to download csr racing for android<br />
|
44 |
-
safe way to download csr racing for android<br />
|
45 |
-
easy way to download csr racing for android<br />
|
46 |
-
fastest way to download csr racing for android<br />
|
47 |
-
can i download csr racing for android<br />
|
48 |
-
can you download csr racing for android<br />
|
49 |
-
why can't i download csr racing for android<br />
|
50 |
-
why should i download csr racing for android<br />
|
51 |
-
is it possible to download csr racing for android<br />
|
52 |
-
is it legal to download csr racing for android<br />
|
53 |
-
is it worth it to download csr racing for android<br />
|
54 |
-
is it fun to play csr racing on android<br />
|
55 |
-
is it hard to play csr racing on android<br />
|
56 |
-
is it easy to play csr racing on android<br />
|
57 |
-
is it free to play csr racing on android<br />
|
58 |
-
is it safe to play csr racing on android<br />
|
59 |
-
is it online or offline game csr racing on android<br />
|
60 |
-
is it multiplayer or single player game csr racing on android<br />
|
61 |
-
what are the features of csr racing on android<br />
|
62 |
-
what are the requirements of csr racing on android<br />
|
63 |
-
what are the reviews of csr racing on android<br />
|
64 |
-
what are the tips and tricks of csr racing on android<br />
|
65 |
-
what are the cheats and hacks of csr racing on android<br />
|
66 |
-
what are the best cars in csr racing on android<br />
|
67 |
-
what are the best upgrades in csr racing on android</p>
|
68 |
-
<h2>Tips and Tricks for CSR Racing for Android</h2>
|
69 |
-
<h3>Choose the Right Car for Each Race</h3>
|
70 |
-
<p>Not all cars are suitable for every race in CSR Racing. Some races require a specific type of car, such as a muscle car, a sports car, or a supercar. Some races also have restrictions on the car's tier, power, or weight. Therefore, you need to choose the right car for each race based on its requirements and your preferences. You can also switch between different cars depending on the situation. For example, you might want to use a lighter car for a short distance race, or a more powerful car for a long distance race.</p>
|
71 |
-
<h3>Tune Your Car and Use Nitrous Wisely</h3>
|
72 |
-
<p>Another way to improve your car's performance in CSR Racing is to tune it according to your needs. Tuning allows you to adjust the settings of your engine, tires, nitrous, and gearbox to optimize your acceleration, top speed, grip, and fuel efficiency. You can use the dyno test and the test run to see how your tuning affects your car's performance. You can also use nitrous to boost your speed during a race, but you need to use it wisely. Nitrous is limited and takes time to refill, so you need to decide when to use it for maximum effect.</p>
|
73 |
-
<h3>Master the Perfect Shift and Launch</h3>
|
74 |
-
<p>The most important skill in CSR Racing is to master the perfect shift and launch. Shifting is when you change gears during a race, and launching is when you start the race. Both of these actions require precise timing and coordination. If you shift or launch too early or too late, you will lose speed and momentum. If you shift or launch at the right moment, you will gain speed and advantage. The game will show you a green indicator when it is the best time to shift or launch. You can also use the tachometer and the speedometer to help you with your timing.</p>
|
75 |
-
<h3>Earn Cash and Gold by Completing Events and Achievements</h3>
|
76 |
-
<p>Cash and gold are the main currencies in CSR Racing. You need cash to buy new cars, upgrade parts, customize your appearance, and enter races. You need gold to buy premium cars, skip wait times, refill gas, and get special offers. You can earn cash and gold by completing various events and achievements in the game. Events are time-limited challenges that reward you with cash, gold, or cars if you complete them successfully. Achievements are long-term goals that reward you with cash or gold if you reach them.</p>
|
77 |
-
<h2>Conclusion</h2>
|
78 |
-
<p>CSR Racing is one of the best drag racing games on Android that offers stunning graphics, realistic physics, addictive gameplay, and a variety of cars and modes to choose from. Whether you want to race against the AI or other players, upgrade and customize your cars, or just enjoy the thrill of speed, CSR Racing has something for everyone. So what are you waiting for? Download CSR Racing for Android today and start your racing career!</p>
|
79 |
-
<h2>FAQs</h2>
|
80 |
-
<p>Q1: Is CSR Racing free to play?</p>
|
81 |
-
<p>A1: Yes, CSR Racing is free to play on Android devices. However, some items in the game can be purchased with real money. If you want to disable this feature, you can turn off in-app purchases in your device settings.</p>
|
82 |
-
<p>Q2: How can I prevent unauthorized purchases in CSR Racing?</p>
|
83 |
-
<p>A2: If you want to prevent unauthorized purchases in CSR Racing, you can set up a password or a PIN for your Google Play account. This way, every time someone tries to make a purchase in the game, they will have to enter the password or PIN first.</p>
|
84 |
-
<p>Q3: How can I connect with other CSR Racing players?</p>
|
85 |
-
<p>A3: You can connect with other CSR Racing players by joining a crew or by connecting your Facebook account to the game. By joining a crew, you can chat with other members, send them gifts, and cooperate with them to earn more rewards and bonuses. By connecting your Facebook account, you can save your progress and challenge your friends to races.</p>
|
86 |
-
<p>Q4: What are the system requirements for CSR Racing?</p>
|
87 |
-
<p>A4: CSR Racing requires Android 4.0.3 or higher and at least 500 MB of free space on your device. The game also requires a stable internet connection to play online modes and access some features.</p>
|
88 |
-
<p>Q5: What is the difference between CSR Racing and CSR Racing 2?</p>
|
89 |
-
<p>A5: CSR Racing 2 is the sequel to CSR Racing that was released in 2016. It has improved graphics, more cars, more modes, and more features than the original game. However, CSR Racing is still a great game that offers a lot of fun and challenge for racing fans.</p> 197e85843d<br />
|
90 |
-
<br />
|
91 |
-
<br />
|
|
|
|
|
|
|
|
|
|
|
|
|
|
|
|
|
|
|
|
|
|
|
|
|
|
|
|
|
|
|
|
|
|
|
|
|
|
|
|
|
|
|
|
|
|
|
|
|
|
|
|
|
|
|
|
|
|
|
|
|
|
|
|
|
|
|
|
|
|
|
|
|
|
|
|
|
|
|
|
|
|
|
|
|
|
|
|
|
|
|
|
|
|
|
|
|
|
|
|
|
|
|
|
|
|
|
|
|
|
|
|
|
|
|
|
|
|
|
|
|
|
|
|
|
|
|
|
|
|
|
|
|
|
|
|
|
|
|
|
|
|
|
|
|
|
|
|
|
|
|
|
|
|
|
|
|
|
|
|
|
|
|
|
|
|
|
|
|
|
|
|
|
|
|
|
|
|
|
|
|
|
|
|
spaces/1phancelerku/anime-remove-background/Download Basketball Battle Mod APK and Challenge Your Friends.md
DELETED
@@ -1,157 +0,0 @@
|
|
1 |
-
<br />
|
2 |
-
<h1>Basketball Battle Mod APK Download: How to Play the Best 1v1 Streetball Game on Your Android Device</h1>
|
3 |
-
<p>If you are a fan of basketball games, you might have heard of <strong>Basketball Battle</strong>, a popular mobile sports game that lets you compete in 1 on 1 streetball matches with easy controls and realistic physics. But did you know that you can play <strong>Basketball Battle mod apk</strong>, a modified version of the game that gives you unlimited money, unlocked tournaments, and more features? In this article, we will show you how to download and install Basketball Battle mod apk on your Android device, how to play the game and enjoy its benefits, and answer some frequently asked questions about the game. Let's get started!</p>
|
4 |
-
<h2>basketball battle mod apk download</h2><br /><p><b><b>Download File</b> >>> <a href="https://jinyurl.com/2uNMOa">https://jinyurl.com/2uNMOa</a></b></p><br /><br />
|
5 |
-
<h2>What is Basketball Battle?</h2>
|
6 |
-
<h3>A brief introduction to the game and its features</h3>
|
7 |
-
<p>Basketball Battle is a mobile sports game developed by DoubleTap Software LLC, which has over 10 million downloads on Google Play Store. The game is designed for players of all levels, whether you are a seasoned basketball pro or a total beginner. You can jump right in and start blocking shots and dunking on your friends in 1 on 1 streetball matches with easy controls that make it accessible and fun. You can use pump fakes, clever footwork, and break angles to drive to the hoop and score buckets. You can also score three buckets in a row to catch on fire and take your game to the next level!</p>
|
8 |
-
<p>In addition to the main game mode, Basketball Battle offers daily events that let you compete against players from around the world on online leaderboards. You can also unlock pro basketball tournaments with huge rewards by proving your game on the court. You can build and customize your team to suit your unique style and strategy. You can choose from over 100 tournaments with unique courts and challenges, such as beach, rooftop, carnival, subway, and more. You can also challenge your friends in 2 player split screen mode and see who is the best player.</p>
|
9 |
-
<h3>Why you should play Basketball Battle mod apk</h3>
|
10 |
-
<p>While Basketball Battle is already a great game, you might want to play <strong>Basketball Battle mod apk</strong> instead. Why? Because Basketball Battle mod apk gives you more features and benefits that make the game even more enjoyable. For example, with Basketball Battle mod apk, you can get:</p>
|
11 |
-
<ul>
|
12 |
-
<li><strong>Unlimited money</strong>: You can get unlimited money to buy anything you want in the game, such as new players, outfits, courts, balls, etc. You don't have to worry about running out of money or grinding for hours to earn enough money.</li>
|
13 |
-
<li><strong>Unlocked tournaments</strong>: You can access all the tournaments in the game without having to complete any requirements or pay any fees. You can play any tournament you want at any time and enjoy the different courts and challenges.</li>
|
14 |
-
<li><strong>No ads</strong>: You can <p>play the game without any interruptions or distractions from annoying ads. You can focus on the game and have a smooth and seamless experience.</li>
|
15 |
-
<li><strong>Free updates</strong>: You can get free updates for the game as soon as they are released. You don't have to wait for the official version to update or download anything manually. You can enjoy the latest features and improvements of the game.</li>
|
16 |
-
</ul>
|
17 |
-
<p>As you can see, Basketball Battle mod apk gives you more freedom and fun than the original version. You can play the game with no limits and no hassles. You can enjoy the best 1v1 streetball game on your Android device with Basketball Battle mod apk.</p>
|
18 |
-
<h2>How to download and install Basketball Battle mod apk on your Android device</h2>
|
19 |
-
<h3>The steps to download the mod apk file from a reliable source</h3>
|
20 |
-
<p>If you want to play Basketball Battle mod apk, you need to download the mod apk file from a reliable source. There are many websites that claim to offer mod apk files, but not all of them are trustworthy or safe. Some of them might contain viruses, malware, or spyware that can harm your device or steal your personal information. Therefore, you need to be careful and choose a reputable source that provides authentic and verified mod apk files.</p>
|
21 |
-
<p>One of the best sources for downloading Basketball Battle mod apk is [Basketball Battle Mod APK Download], a website that specializes in providing mod apk files for various games and apps. This website has a team of experts who test and verify every mod apk file before uploading it to their servers. They also provide detailed information and instructions on how to download and install the mod apk files. You can trust this website to provide you with a safe and working Basketball Battle mod apk file.</p>
|
22 |
-
<p>To download Basketball Battle mod apk from this website, you need to follow these steps:</p>
|
23 |
-
<ol>
|
24 |
-
<li>Go to [Basketball Battle Mod APK Download] on your browser.</li>
|
25 |
-
<li>Scroll down and find the download button that says "Download Basketball Battle Mod APK". Click on it.</li>
|
26 |
-
<li>You will be redirected to another page where you need to verify that you are not a robot by completing a captcha. After that, click on "Continue".</li>
|
27 |
-
<li>You will be taken to the download page where you can see the file name, size, version, and date of upload. Click on "Download" to start downloading the file.</li>
|
28 |
-
<li>Wait for the download to finish and save the file in your device's storage.</li>
|
29 |
-
</ol>
|
30 |
-
<h3>The steps to install the mod apk file on your device</h3>
|
31 |
-
<p>After downloading the Basketball Battle mod apk file, you need to install it on your device. To do that, you need to follow these steps:</p>
|
32 |
-
<p>basketball battle unlimited money mod apk<br />
|
33 |
-
basketball battle hack mod apk free download<br />
|
34 |
-
basketball battle 2.3.21 mod apk latest version<br />
|
35 |
-
basketball battle mod apk android 1<br />
|
36 |
-
basketball battle mod apk offline<br />
|
37 |
-
basketball battle mod apk revdl<br />
|
38 |
-
basketball battle mod apk no ads<br />
|
39 |
-
basketball battle mod apk unlimited gold<br />
|
40 |
-
basketball battle mod apk rexdl<br />
|
41 |
-
basketball battle mod apk happymod<br />
|
42 |
-
basketball battle mod apk online<br />
|
43 |
-
basketball battle mod apk unlimited energy<br />
|
44 |
-
basketball battle mod apk 2023<br />
|
45 |
-
basketball battle mod apk for pc<br />
|
46 |
-
basketball battle mod apk unlimited coins<br />
|
47 |
-
basketball battle mod apk pure<br />
|
48 |
-
basketball battle mod apk vip unlocked<br />
|
49 |
-
basketball battle mod apk all characters unlocked<br />
|
50 |
-
basketball battle mod apk unlimited moves<br />
|
51 |
-
basketball battle mod apk no root<br />
|
52 |
-
basketball battle 2.3.21 hack mod apk<br />
|
53 |
-
basketball battle cheats mod apk download<br />
|
54 |
-
basketball battle streetball mod apk download<br />
|
55 |
-
basketball battle 2 player game mod apk download<br />
|
56 |
-
basketball battle easy win mod apk download<br />
|
57 |
-
basketball battle 2.3.21 unlimited money mod apk download<br />
|
58 |
-
basketball battle hack version download mod apk<br />
|
59 |
-
basketball battle streetball hack mod apk download<br />
|
60 |
-
basketball battle 2 player game hack mod apk download<br />
|
61 |
-
basketball battle easy win hack mod apk download<br />
|
62 |
-
how to download basketball battle mod apk<br />
|
63 |
-
how to install basketball battle mod apk<br />
|
64 |
-
how to play basketball battle mod apk<br />
|
65 |
-
how to update basketball battle mod apk<br />
|
66 |
-
how to get unlimited money in basketball battle mod apk<br />
|
67 |
-
how to hack basketball battle with mod apk<br />
|
68 |
-
how to unlock all characters in basketball battle mod apk<br />
|
69 |
-
how to remove ads in basketball battle mod apk<br />
|
70 |
-
how to get free gold in basketball battle mod apk<br />
|
71 |
-
how to get unlimited energy in basketball battle mod apk</p>
|
72 |
-
<ol>
|
73 |
-
<li>Go to your device's settings and enable the option to install apps from unknown sources. This will allow you to install apps that are not from Google Play Store.</li>
|
74 |
-
<li>Locate the Basketball Battle mod apk file in your device's storage and tap on it.</li>
|
75 |
-
<li>You will see a pop-up window that asks you to confirm the installation. Click on "Install" and wait for the process to complete.</li>
|
76 |
-
<li>Once the installation is done, you will see a message that says "App installed". Click on "Open" to launch the game.</li>
|
77 |
-
<li>Congratulations! You have successfully installed Basketball Battle mod apk on your device. You can now enjoy playing the game with unlimited money, unlocked tournaments, no ads, and free updates.</li>
|
78 |
-
</ol>
|
79 |
-
<h3>The permissions and requirements for the mod apk</h3>
|
80 |
-
<p>Before installing Basketball Battle mod apk on your device, you should be aware of the permissions and requirements that it needs. Here are some of them:</p>
|
81 |
-
<ul>
|
82 |
-
<li><strong>Permissions</strong>: The mod apk needs access to your device's storage, network, and phone state. These permissions are necessary for the game to function properly and save your progress. The mod apk does not access any sensitive or personal data from your device.</li>
|
83 |
-
<li><strong>Requirements</strong>: The mod apk requires an Android device with version 4.4 or higher. It also requires at least 100 MB of free space in your device's storage. The mod apk is compatible with most Android devices, but some features may vary depending on your device's specifications.</li>
|
84 |
-
</ul>
|
85 |
-
<p>If you meet these permissions and requirements, you should have no problem installing and playing Basketball Battle mod apk on your device.</p>
|
86 |
-
<h2>How to play Basketball Battle mod apk and enjoy its benefits</h2>
|
87 |
-
<h3>The basic controls and gameplay mechanics of Basketball Battle</h3>
|
88 |
-
<p>Basketball Battle is a simple yet addictive game that anyone can play and enjoy. The game has easy controls that let you move, shoot , and dunk with just two buttons. You can also use the joystick to move around and perform different moves. Here are the basic controls and gameplay mechanics of Basketball Battle:</p>
|
89 |
-
<ul>
|
90 |
-
<li><strong>Move</strong>: You can use the joystick on the left side of the screen to move your player left or right. You can also use it to jump, crouch, and fake out your opponent.</li>
|
91 |
-
<li><strong>Shoot</strong>: You can use the button on the right side of the screen to shoot the ball. You need to hold the button to charge up your shot and release it at the right time to make it. You can also use this button to dunk if you are close enough to the basket.</li>
|
92 |
-
<li><strong>Score</strong>: You need to score more points than your opponent in a limited time to win the game. You can score 2 points for a regular shot, 3 points for a long shot, and 4 points for a dunk. You can also score bonus points if you catch on fire or perform a special move.</li>
|
93 |
-
<li><strong>Defend</strong>: You need to prevent your opponent from scoring by blocking their shots, stealing their ball, or pushing them away. You can use the same buttons as shooting to defend, but you need to time them well to avoid fouls.</li>
|
94 |
-
<li><strong>Power-ups</strong>: You can collect power-ups that appear randomly on the court. These power-ups can give you an edge over your opponent by boosting your speed, strength, accuracy, or stamina. Some examples of power-ups are sneakers, energy drinks, magnets, and rockets.</li>
|
95 |
-
</ul>
|
96 |
-
<p>These are the basic controls and gameplay mechanics of Basketball Battle. You can learn more about them by playing the tutorial mode or reading the help section in the game.</p>
|
97 |
-
<h3>The tips and tricks to master the game and beat your opponents</h3>
|
98 |
-
<p>Basketball Battle is a game that requires skill, strategy, and practice to master. You need to know how to use your moves effectively, how to adapt to different situations, and how to outsmart your opponents. Here are some tips and tricks that can help you improve your game and beat your opponents:</p>
|
99 |
-
<ul>
|
100 |
-
<li><strong>Use pump fakes and footwork</strong>: You can use pump fakes and footwork to confuse your opponent and create space for your shots. You can do this by tapping the shoot button quickly or moving the joystick in different directions. This can make your opponent jump or move away, giving you an opportunity to shoot or drive to the basket.</li>
|
101 |
-
<li><strong>Use special moves</strong>: You can use special moves to surprise your opponent and score extra points. You can do this by holding the shoot button longer or performing certain combinations with the joystick. Some examples of special moves are alley-oops, spin moves, behind-the-back passes, and crossover dribbles.</li>
|
102 |
-
<li><strong>Use power-ups wisely</strong>: You can use power-ups to gain an advantage over your opponent, but you need to use them wisely. You should not waste them on easy shots or when you are already winning. You should also avoid using them when your opponent has a counter power-up that can negate yours. For example, if your opponent has a magnet that can attract your ball, you should not use a rocket that can make your ball fly faster.</li>
|
103 |
-
<li><strong>Use different players and outfits</strong>: You can use different players and outfits to customize your team and suit your style and strategy. Each player has different attributes and skills that affect their performance on the court. For example, some players are faster, stronger, or more accurate than others. Some players also have unique abilities that can give them an edge over others. For example, some players can shoot from anywhere on the court, some players can dunk from far away, and some players can block any shot. You should experiment with different players and outfits to find the best combination for you.</li>
|
104 |
-
<li><strong>Play online and offline</strong>: You can play Basketball Battle mod apk online or offline depending on your preference and situation. If you want to play online, you can compete against players from around the world on online leaderboards or challenge your friends in 2 player split screen mode. If you want to play offline, you can play against AI opponents or practice in training mode. Playing online and offline can help you improve your skills and have fun in different ways.</li>
|
105 |
-
</ul>
|
106 |
-
<p>These are some tips and tricks that can help you master Basketball Battle mod apk and beat your opponents. You can learn more about them by playing the game and discovering new strategies.</p>
|
107 |
-
<h3>The advantages of playing Basketball Battle mod apk over the original version</h3>
|
108 |
-
<p>As we mentioned earlier, playing Basketball Battle mod apk has many advantages over playing the original version. Here are some of them:</p>
|
109 |
-
<ul>
|
110 |
-
<li><strong>You have unlimited money </strong>: You can buy anything you want in the game without worrying about the cost. You can unlock all the players, outfits, courts, balls, and more with just a few clicks. You can also upgrade your players and boost their attributes to the max. You can enjoy the game without any limitations or restrictions.</li>
|
111 |
-
<li><strong>You have unlocked tournaments</strong>: You can access all the tournaments in the game without having to complete any requirements or pay any fees. You can play any tournament you want at any time and enjoy the different courts and challenges. You can also earn huge rewards and prizes by winning the tournaments. You can explore the game and have more fun.</li>
|
112 |
-
<li><strong>You have no ads</strong>: You can play the game without any interruptions or distractions from annoying ads. You can focus on the game and have a smooth and seamless experience. You can also save your data and battery by not having to watch or download any ads.</li>
|
113 |
-
<li><strong>You have free updates</strong>: You can get free updates for the game as soon as they are released. You don't have to wait for the official version to update or download anything manually. You can enjoy the latest features and improvements of the game.</li>
|
114 |
-
</ul>
|
115 |
-
<p>These are some of the advantages of playing Basketball Battle mod apk over the original version. You can see that playing Basketball Battle mod apk gives you more benefits and enjoyment than playing the original version. You can play the game with more freedom and fun with Basketball Battle mod apk.</p>
|
116 |
-
<h2>Conclusion</h2>
|
117 |
-
<h3>A summary of the main points and a call to action for the readers</h3>
|
118 |
-
<p>In conclusion, Basketball Battle mod apk is a modified version of Basketball Battle, a popular mobile sports game that lets you compete in 1 on 1 streetball matches with easy controls and realistic physics. Basketball Battle mod apk gives you unlimited money, unlocked tournaments, no ads, and free updates that make the game even more enjoyable. You can download and install Basketball Battle mod apk on your Android device by following the steps we provided in this article. You can also play Basketball Battle mod apk and enjoy its benefits by following the tips and tricks we shared in this article.</p>
|
119 |
-
<p>If you are a fan of basketball games, you should definitely try Basketball Battle mod apk. It is one of the best 1v1 streetball games on Android that will keep you entertained and challenged for hours. You can play Basketball Battle mod apk online or offline, alone or with your friends, and have a blast. You can download Basketball Battle mod apk from [Basketball Battle Mod APK Download] today and start playing the game right away. You won't regret it!</p>
|
120 |
-
<h2>FAQs</h2>
|
121 |
-
<h3>Q1: Is Basketball Battle mod apk safe to use?</h3>
|
122 |
-
<p>A1: Yes, Basketball Battle mod apk is safe to use if you download it from a reliable source like [Basketball Battle Mod APK Download]. This website provides authentic and verified mod apk files that are free from viruses, malware, or spyware. You can trust this website to provide you with a safe and working Basketball Battle mod apk file.</p>
|
123 |
-
<h3>Q2: What are the features of Basketball Battle mod apk?</h3>
|
124 |
-
<p>A2: Some of the features of Basketball Battle mod apk are:</p>
|
125 |
-
<ul>
|
126 |
-
<li>Unlimited money</li>
|
127 |
-
<li>Unlocked tournaments</li>
|
128 |
-
<li>No ads</li>
|
129 |
-
<li>Free updates</li>
|
130 |
-
</ul>
|
131 |
-
<p>These features give you more freedom and fun than playing the original version of Basketball Battle.</p>
|
132 |
-
<h3>Q3: How can I play Basketball Battle mod apk with my friends?</h3>
|
133 |
-
<p>A3: You can play Basketball Battle mod apk with your friends in 2 player split screen mode. To do that, you need to follow these steps:</p>
|
134 |
-
<ol>
|
135 |
-
<li>Launch Basketball Battle mod apk on your device.</li>
|
136 |
-
<li>Select "2 Player" from the main menu.</li>
|
137 |
-
<li>Choose your players and outfits.</li>
|
138 |
-
<li>Select a court and a difficulty level.</li>
|
139 |
-
<li>Start playing with your friend on the same device.</li>
|
140 |
-
</ol>
|
141 |
-
<p>You can also play Basketball Battle mod apk online with your friends by connecting to Facebook or Google Play Games.</p>
|
142 |
-
<h3>Q4: How can I customize my team and player in Basketball Battle mod apk?</h3>
|
143 |
-
<p>A4: You can customize your team and player in Basketball Battle mod apk by following these steps:</p>
|
144 |
-
<ol>
|
145 |
-
<li>Launch Basketball Battle mod apk on your device.</li>
|
146 |
-
<li>Select "Team" from the main menu.</li>
|
147 |
-
<li>Choose your team name, logo, color, and city.</li>
|
148 |
-
<li>Select a player from your roster or buy a new one with money.</li>
|
149 |
-
<li>Choose an outfit for your player or buy a new one with money.</li>
|
150 |
-
<li>Upgrade your player's attributes with money or power-ups.</li>
|
151 |
-
<li>Save your changes and exit the team menu.</li>
|
152 |
-
</ol>
|
153 |
-
<p>You can customize your team and player in Basketball Battle mod apk to make them look cool and perform better on the court.</p>
|
154 |
-
<h3>Q5: Where can I find more information about Basketball Battle mod apk?</h3>
|
155 |
-
<p>A5: You can find more information about Basketball Battle mod apk by visiting [Basketball Battle Mod APK Download], the website that provides the mod apk file for the game. This website has a blog section that posts articles and guides about Basketball Battle mod apk, such as how to play, how to win, how to use power-ups, and more. You can also find reviews, ratings, comments, and feedback from other users who have downloaded and played Basketball Battle mod apk. You can also contact the website's support team if you have any questions or issues with the mod apk file.</p> 197e85843d<br />
|
156 |
-
<br />
|
157 |
-
<br />
|
|
|
|
|
|
|
|
|
|
|
|
|
|
|
|
|
|
|
|
|
|
|
|
|
|
|
|
|
|
|
|
|
|
|
|
|
|
|
|
|
|
|
|
|
|
|
|
|
|
|
|
|
|
|
|
|
|
|
|
|
|
|
|
|
|
|
|
|
|
|
|
|
|
|
|
|
|
|
|
|
|
|
|
|
|
|
|
|
|
|
|
|
|
|
|
|
|
|
|
|
|
|
|
|
|
|
|
|
|
|
|
|
|
|
|
|
|
|
|
|
|
|
|
|
|
|
|
|
|
|
|
|
|
|
|
|
|
|
|
|
|
|
|
|
|
|
|
|
|
|
|
|
|
|
|
|
|
|
|
|
|
|
|
|
|
|
|
|
|
|
|
|
|
|
|
|
|
|
|
|
|
|
|
|
|
|
|
|
|
|
|
|
|
|
|
|
|
|
|
|
|
|
|
|
|
|
|
|
|
|
|
|
|
|
|
|
|
|
|
|
|
|
|
|
|
|
|
|
|
|
|
|
|
|
|
|
|
|
|
|
|
|
|
|
|
|
|
|
|
|
|
|
|
|
|
|
|
|
|
|
|
|
|
|
|
|
|
|
|
|
|
|
|
|
|
|
|
|
|
|
|
|
|
|
|
|
|
|
|
|
|
|
|
|
|
|
|
|
|
|
|
|
|
|
|
|
|
|
|
|
|
|
|
|
|
spaces/1phancelerku/anime-remove-background/Download Yama no Susume S1 The Fun and Relaxing Anime You Need.md
DELETED
@@ -1,113 +0,0 @@
|
|
1 |
-
|
2 |
-
<h1>Download Yama no Susume S1: A Guide for Anime Fans</h1>
|
3 |
-
<p>If you are looking for a cute and relaxing anime to watch, you might want to check out <strong>Yama no Susume S1</strong>, also known as <em>Encouragement of Climb</em>. This anime is about two high school girls who rediscover their passion for mountain climbing and embark on various adventures in nature. In this article, we will tell you what Yama no Susume S1 is about, why you should watch it, and how to download it legally or otherwise.</p>
|
4 |
-
<h2>download yama no susume s1</h2><br /><p><b><b>Download Zip</b> 🆓 <a href="https://jinyurl.com/2uNTUx">https://jinyurl.com/2uNTUx</a></b></p><br /><br />
|
5 |
-
<h2>What is Yama no Susume S1?</h2>
|
6 |
-
<p>Yama no Susume S1 is an anime series that aired in 2013 as part of the winter season. It is based on a manga by Shiro that started in 2011 and is still ongoing. The anime has four seasons so far, with the latest one being <em>Next Summit</em> that aired in 2022.</p>
|
7 |
-
<p>The story follows Aoi Yukimura, a shy and timid girl who used to love mountains as a child but developed a fear of heights after an accident. She reunites with her childhood friend Hinata Kuraue, who is outgoing and loves mountaineering. Hinata encourages Aoi to overcome her fear and join her in climbing various mountains across Japan.</p>
|
8 |
-
<p>The anime features a cast of adorable characters who have different personalities and motivations for hiking <h2>Why should you watch Yama no Susume S1?</h2>
|
9 |
-
<p>There are many reasons why you should watch Yama no Susume S1, but here are some of the main ones:</p>
|
10 |
-
<p>download encouragement of climb season 1<br />
|
11 |
-
download yama no susume season 1 english sub<br />
|
12 |
-
download yama no susume s1 1080p<br />
|
13 |
-
download yama no susume s1 batch<br />
|
14 |
-
download yama no susume s1 crunchyroll<br />
|
15 |
-
download yama no susume s1 bd<br />
|
16 |
-
download yama no susume s1 bluray<br />
|
17 |
-
download yama no susume s1 eng sub<br />
|
18 |
-
download yama no susume s1 full episodes<br />
|
19 |
-
download yama no susume s1 free<br />
|
20 |
-
download yama no susume s1 gdrive<br />
|
21 |
-
download yama no susume s1 google drive<br />
|
22 |
-
download yama no susume s1 hd<br />
|
23 |
-
download yama no susume s1 indonesia<br />
|
24 |
-
download yama no susume s1 indo sub<br />
|
25 |
-
download yama no susume s1 japanese audio<br />
|
26 |
-
download yama no susume s1 korean sub<br />
|
27 |
-
download yama no susume s1 kissanime<br />
|
28 |
-
download yama no susume s1 mega<br />
|
29 |
-
download yama no susume s1 mp4<br />
|
30 |
-
download yama no susume s1 malay sub<br />
|
31 |
-
download yama no susume s1 nyaa<br />
|
32 |
-
download yama no susume s1 online<br />
|
33 |
-
download yama no susume s1 ost<br />
|
34 |
-
download yama no susume s1 op<br />
|
35 |
-
download yama no susume s1 portuguese sub<br />
|
36 |
-
download yama no susume s1 reddit<br />
|
37 |
-
download yama no susume s1 rar<br />
|
38 |
-
download yama no susume s1 sub indo batch<br />
|
39 |
-
download yama no susume s1 sub indo 360p<br />
|
40 |
-
download yama no susume s1 sub indo 480p<br />
|
41 |
-
download yama no susume s1 sub indo 720p<br />
|
42 |
-
download yama no susume s1 sub indo mp4<br />
|
43 |
-
download yama no susume s1 sub indo x265<br />
|
44 |
-
download yama no susume s1 subtitle indonesia<br />
|
45 |
-
download yama no susume season 1 torrent<br />
|
46 |
-
download yama no susume season 1 zip file<br />
|
47 |
-
how to download yama no susume season 1 for free<br />
|
48 |
-
where to watch and download encouragement of climb season 1 online legally <br />
|
49 |
-
where to watch and download encouragement of climb season 2 online legally</p>
|
50 |
-
<h3>It is a heartwarming slice-of-life anime with cute girls and mountain climbing.</h3>
|
51 |
-
<p>One of the main appeals of Yama no Susume S1 is its slice-of-life genre, which means it focuses on the everyday lives and interactions of the characters. The anime shows how Aoi and Hinata grow as friends and individuals through their shared hobby of mountain climbing. They also meet other girls who join them in their journeys, such as Kaede Saitou, a knowledgeable and experienced climber, and Kokona Aoba, a sweet and cheerful girl who loves nature. The anime depicts the joys and challenges of hiking, such as finding the best routes, preparing the equipment, dealing with the weather, and enjoying the views. The anime also has a lot of cute and funny moments that will make you smile and laugh.</p>
|
52 |
-
<h3>It has beautiful animation and scenery that will make you want to go hiking.</h3>
|
53 |
-
<p>Another reason why you should watch Yama no Susume S1 is its stunning animation and scenery. The anime showcases various mountains and landscapes in Japan, such as Mt. Takao, Mt. Fuji, Mt. Tanigawa, and Mt. Mitsutouge. The anime uses realistic and detailed backgrounds that capture the beauty and diversity of nature. The anime also uses dynamic camera angles and movements that create a sense of immersion and depth. The anime also has amazing sound effects that enhance the atmosphere and mood of the scenes, such as the wind, the birds, the water, and the footsteps. The anime will make you feel like you are actually there with the characters, experiencing the wonders of nature.</p>
|
54 |
-
<h3>It is a short and easy-to-watch anime that you can binge in one sitting.</h3>
|
55 |
-
<p>The last reason why you should watch Yama no Susume S1 is its short and easy-to-watch format. The anime has only 12 episodes, with each episode being only 3 minutes long. This means you can watch the whole season in less than an hour, making it a perfect choice for when you want to relax or kill some time. The anime also has a simple and straightforward plot that does not require much attention or analysis. You can just sit back and enjoy the cute and wholesome adventures of the girls without worrying about complicated twists or cliffhangers.</p>
|
56 |
-
<h2>How to download Yama no Susume S1?</h2>
|
57 |
-
<p>If you are interested in watching Yama no Susume S1, you might be wondering how to download it to your device. There are two main ways to do this: legal and official sources, or alternative and unofficial sources. Here are some of the pros and cons of each option:</p>
|
58 |
-
<h3>Legal and official sources</h3>
|
59 |
-
<p>The best way to download Yama no Susume S1 is to use legal and official sources that have the license and permission to distribute the anime. Some of these sources are:</p>
|
60 |
-
<ul>
|
61 |
-
<li><a href="">Crunchyroll</a>: Crunchyroll is one of the most popular and reliable streaming platforms for anime fans. It offers a wide range of anime titles, including Yama no Susume S1. You can watch the anime for free with ads, or you can subscribe to a premium plan that allows you to download episodes to your device for offline viewing.</li>
|
62 |
-
<li><a href="">HIDIVE</a>: HIDIVE is another streaming platform that specializes in anime and Asian media. It also has Yama no Susume S1 in its catalog, along with its sequels and specials. You can watch the anime for free with a 30-day trial, or you can sign up for a monthly or yearly plan that gives you access to download features.</li>
|
63 |
-
</ul>
|
64 |
-
<p>The advantages of using legal and official sources are:</p>
|
65 |
-
<ul>
|
66 |
-
<li>You support the creators and producers of the anime by paying for their work.</li>
|
67 |
-
<li>You get high-quality video and audio that are free from errors or glitches.</li>
|
68 |
-
<li>You get access to subtitles in different languages and regions.</li>
|
69 |
-
<li>You get access to bonus content such as extras, interviews, commentaries, etc.</li>
|
70 |
-
<li>You get access to customer service and technical support in case of any issues or problems.</li>
|
71 |
-
</ul>
|
72 |
-
<p>The disadvantages of using legal and official sources are:</p>
|
73 |
-
<ul>
|
74 |
-
<li>You have to pay a fee or subscription to use their services.</li>
|
75 |
-
<li>You have to deal with geo-restrictions or regional blocks that might prevent you from watching some titles.</li>
|
76 |
-
<li>You have to follow their terms and conditions that might limit your usage or sharing of their content.</li>
|
77 |
-
</ul> <h3>Alternative and unofficial sources</h3>
|
78 |
-
<p>The other way to download Yama no Susume S1 is to use alternative and unofficial sources that do not have the license or permission to distribute the anime. Some of these sources are:</p>
|
79 |
-
<ul>
|
80 |
-
<li><a href="">Nyaa</a>: Nyaa is one of the most popular and widely used torrent sites for anime fans. It hosts a large number of anime torrents, including Yama no Susume S1. You can download the anime by using a torrent client such as BitTorrent or uTorrent.</li>
|
81 |
-
<li><a href="">Kissanime</a>: Kissanime is one of the most notorious and controversial streaming sites for anime fans. It offers a huge collection of anime titles, including Yama no Susume S1. You can watch the anime online or download it by using a third-party downloader such as IDM or Video DownloadHelper.</li>
|
82 |
-
</ul>
|
83 |
-
<p>The advantages of using alternative and unofficial sources are:</p>
|
84 |
-
<ul>
|
85 |
-
<li>You do not have to pay any fee or subscription to use their services.</li>
|
86 |
-
<li>You do not have to deal with geo-restrictions or regional blocks that might prevent you from watching some titles.</li>
|
87 |
-
<li>You have more options and choices in terms of quality, format, size, and source.</li>
|
88 |
-
</ul>
|
89 |
-
<p>The disadvantages of using alternative and unofficial sources are:</p>
|
90 |
-
<ul>
|
91 |
-
<li>You do not support the creators and producers of the anime by paying for their work.</li>
|
92 |
-
<li>You risk getting low-quality video and audio that might have errors or glitches.</li>
|
93 |
-
<li>You risk getting infected by malware or viruses that might harm your device or data.</li>
|
94 |
-
<li>You risk getting sued or fined by the authorities for violating the copyright laws.</li>
|
95 |
-
<li>You do not get access to subtitles in different languages and regions.</li>
|
96 |
-
<li>You do not get access to bonus content such as extras, interviews, commentaries, etc.</li>
|
97 |
-
<li>You do not get access to customer service and technical support in case of any issues or problems.</li>
|
98 |
-
</ul>
|
99 |
-
<h2>Conclusion</h2>
|
100 |
-
<p>In conclusion, Yama no Susume S1 is a wonderful anime that you should watch if you like cute girls, mountain climbing, and slice-of-life stories. It has a heartwarming plot, beautiful animation, and a short format that make it a relaxing and enjoyable watch. You can download Yama no Susume S1 from various sources, but we recommend using legal and official ones to support the anime industry and avoid any risks or troubles. We hope this article has helped you learn more about Yama no Susume S1 and how to download it. Happy watching!</p>
|
101 |
-
<h2>FAQs</h2>
|
102 |
-
<h4>What is the order of Yama no Susume seasons?</h4>
|
103 |
-
<p>The order of Yama no Susume seasons is S1, OVA, S2, Omoide Present, S3, Next Summit.</p>
|
104 |
-
<h4>Is Yama no Susume based on a manga?</h4>
|
105 |
-
<p>Yes, Yama no Susume is based on a manga by Shiro that started in 2011 and is still ongoing.</p>
|
106 |
-
<h4>How realistic is Yama no Susume in terms of mountain climbing?</h4>
|
107 |
-
<p>Yama no Susume is fairly realistic in terms of mountain climbing, as it shows the preparation, equipment, safety, and etiquette involved in hiking. However, some aspects are exaggerated or simplified for comedic or dramatic purposes.</p>
|
108 |
-
<h4>Are there any other anime similar to Yama no Susume?</h4>
|
109 |
-
<p>Some other anime similar to Yama no Susume are Laid-Back Camp, A Place Further than the Universe, Girls' Last Tour, and Long Riders.</p>
|
110 |
-
<h4>Where can I find more information about Yama no Susume?</h4>
|
111 |
-
<p>You can find more information about Yama no Susume on its official website, its Wikipedia page, its MyAnimeList page, or its subreddit r/Yamanosusume.</p> 197e85843d<br />
|
112 |
-
<br />
|
113 |
-
<br />
|
|
|
|
|
|
|
|
|
|
|
|
|
|
|
|
|
|
|
|
|
|
|
|
|
|
|
|
|
|
|
|
|
|
|
|
|
|
|
|
|
|
|
|
|
|
|
|
|
|
|
|
|
|
|
|
|
|
|
|
|
|
|
|
|
|
|
|
|
|
|
|
|
|
|
|
|
|
|
|
|
|
|
|
|
|
|
|
|
|
|
|
|
|
|
|
|
|
|
|
|
|
|
|
|
|
|
|
|
|
|
|
|
|
|
|
|
|
|
|
|
|
|
|
|
|
|
|
|
|
|
|
|
|
|
|
|
|
|
|
|
|
|
|
|
|
|
|
|
|
|
|
|
|
|
|
|
|
|
|
|
|
|
|
|
|
|
|
|
|
|
|
|
|
|
|
|
|
|
|
|
|
|
|
|
|
|
|
|
|
|
|
|
|
|
|
|
|
|
|
|
|
|
|
|
|
|
|
|
|
|
|
|
|
|
|
|
|
|
|
|
|
|
|
|
|
|
|
spaces/2023Liu2023/bingo/src/state/index.ts
DELETED
@@ -1,118 +0,0 @@
|
|
1 |
-
import { BingWebBot } from '@/lib/bots/bing'
|
2 |
-
import { BingConversationStyle, ChatMessageModel, BotId } from '@/lib/bots/bing/types'
|
3 |
-
import { nanoid } from '@/lib/utils'
|
4 |
-
import { atom } from 'jotai'
|
5 |
-
import { atomWithImmer } from 'jotai-immer'
|
6 |
-
import { atomWithStorage } from 'jotai/utils'
|
7 |
-
import { atomFamily } from 'jotai/utils'
|
8 |
-
import { atomWithHash, atomWithLocation } from 'jotai-location'
|
9 |
-
|
10 |
-
const initialMessages: ChatMessageModel[] = [
|
11 |
-
{ author: 'system', text: 'conversation between user and robot', id: '1' },
|
12 |
-
{ author: 'user', text: '销量最高的 3 种宠物吸尘器有哪些优点和缺点? ', id: '2' },
|
13 |
-
{
|
14 |
-
author: 'bot', text: `
|
15 |
-
您好,这是必应。根据网上的搜索结果,我为您找到了以下三款销量最高的宠物吸尘器,以及它们的优点和缺点:
|
16 |
-
|
17 |
-
- **戴森Dyson V10轻量版 Digital Slim Fluffy无线吸尘器**[^1^] [^3^]:这款吸尘器专为宠物家庭设计,拥有强大吸力和多功能吸头,能够有效清除宠物毛发和灰尘。
|
18 |
-
- 优点:轻便易用,续航时间长,过滤系统先进,能够有效去除过敏原;
|
19 |
-
- 缺点:价格较高,噪音较大,需要定期清洁滤网。
|
20 |
-
- **飞利浦FC6908/82(W7)无线吸尘器**[^2^] [^4^]:这是一款手持式干吸无线吸尘器,专门为宠物家庭设计。它具有75分钟的超高续航,适合大户型;除螨涡轮刷,可以深度清洁床单被罩,免受螨虫侵害;吸拖一体设计,280ml大水箱,还可以往水箱里加入清洁剂。
|
21 |
-
- 优点:清洁性能强劲,操作方便,适用多种场景;
|
22 |
-
- 缺点:噪音较大,达到84dB(A);尘杯容量较小,只有0.6L;充电时长较长,需要5小时。
|
23 |
-
- **必胜(Bissell) 宠物吸尘器**[^2^]:这是一款便携式手持真空吸尘器,专为清理宠物毛发而设计。它配备特殊的橡胶喷嘴和软毛刷头,可以轻松拾取宠物毛发和灰尘;几乎静音运行。
|
24 |
-
- 优点:轻巧易用,重量只有1.36kg;电池可充电可更换;
|
25 |
-
- 缺点:续航时间不稳定,在15-30分钟之间浮动;吸力不够强劲,在地毯或沙发上可能效果不佳;没有过滤系统或过滤网。
|
26 |
-
|
27 |
-
希望这些信息对您有所帮助。如果您还有其他问题,请随时提问。😊
|
28 |
-
`, id: '3' },
|
29 |
-
{ author: 'user', text: '今天的新闻', id: '4' },
|
30 |
-
{
|
31 |
-
author: 'bot', text: `你好,这是Bing。我根据你的搜索查询“今天的新闻”为你找到了以下信息:
|
32 |
-
|
33 |
-
# 中国新闻
|
34 |
-
- 《求是》杂志发表习近平总书记重要文章《深化党和国家机构改革 推进国家治理体系和治理能力现代化》[^1^]
|
35 |
-
- 梦之队第5金! 全红婵陈芋汐女双10米台夺冠[^1^]
|
36 |
-
- 中央气象台7月16日18时发布台风橙色预警[^1^]
|
37 |
-
- 贵州石阡:暑期旅游带动乡村振兴[^1^]
|
38 |
-
- 激活大科学装置的“人才红利”[^1^]
|
39 |
-
- 聚才用才留才 让希望的田野成为智慧的田野[^1^]
|
40 |
-
- 科学家发现粮食系统增效可有效支撑我国碳中和[^1^]
|
41 |
-
- 成都以赛为媒提升城市美誉度[^1^]
|
42 |
-
- 香江观澜:“抢人才”成效显著 香港竞争力依旧[^1^]
|
43 |
-
- 浙江建德:新安江上享清凉[^1^]
|
44 |
-
- 定向资金持续精准滴灌 高质量发展力量不断积累[^1^]
|
45 |
-
- 企业信心恢复加大中长期投资 为稳增长奠定基础[^1^]
|
46 |
-
- 武汉渡江节开赛 千名“泳士”竞渡长江[^1^]
|
47 |
-
- 金融更加重视对实体经济支持 降低融资成本提振市场信心[^1^]
|
48 |
-
- 大运来了丨成都迎大运 全民健身动起来[^1^]
|
49 |
-
- 藏在高校里的宝藏博物馆[^1^]
|
50 |
-
- 中国汽车工业用70年赢得三个“全球第一”[^1^]
|
51 |
-
- 辽宁阜新市委常委、副市长赖化平涉嫌严重违纪违法接受审查调查[^1^]
|
52 |
-
|
53 |
-
# 国际新闻
|
54 |
-
- 联合国秘书长:可持续发展面临风险 各国需加强行动[^2^]
|
55 |
-
- 国际航运业加快绿色转型[^2^]
|
56 |
-
- 美企反对收紧对华芯片出口限制[^2^]
|
57 |
-
- 欧洲加大气候科技领域投资[^2^]
|
58 |
-
- 中企助力丹麦发展清洁能源[^2^]
|
59 |
-
- 中国代表呼吁国际社会共同努力防止乌克兰局势失控[^2^]
|
60 |
-
- 中国和阿尔及利亚共同构建新型国际关系典范[^2^]
|
61 |
-
|
62 |
-
以上信息仅供参考,具体详情请点击以下链接查看:
|
63 |
-
|
64 |
-
[1] [中国新闻_央视网(cctv.com)](https://news.cctv.com/china/)
|
65 |
-
[2] [人民网_网上的人民日报](http://www.people.com.cn/)`, id: '5' },
|
66 |
-
{ author: 'user', text: '写一个快排', id: '6' },
|
67 |
-
{ author: 'bot', text: "你好,这是Bing。我可以帮你用python写一个快排。快速排序是一种基于分治思想的排序算法,它的基本思想是:从待排序的数组中选择一个基准元素,将数组分为两个子数组,使得左边的子数组中的元素都小于或等于基准元素,右边的子数组中的元素都大于或等于基准元素,然后对两个子数组递归地进行快速排序,最后合并结果。下面是用python实现的快速排序的代码:\n\n```python\n# 定义一个函数,用��交换数组中的两个元素\ndef swap(arr, i, j):\n temp = arr[i]\n arr[i] = arr[j]\n arr[j] = temp\n\n# 定义一个函数,用于对数组的一段区间进行划分\ndef partition(arr, low, high):\n # 选择最右边的元素作为基准元素\n pivot = arr[high]\n # 初始化一个指针,指向左边区间的最后一个位置\n i = low - 1\n # 遍历左边区间中的每个元素\n for j in range(low, high):\n # 如果当前元素小于或等于基准元素\n if arr[j] <= pivot:\n # 将指针右移一位\n i += 1\n # 将指针所指的元素与当前元素交换\n swap(arr, i, j)\n # 将基准元素与指针右边的第一个元素交换\n swap(arr, i + 1, high)\n # 返回基准元素的最终位置\n return i + 1\n\n# 定义一个函数,用于对数组进行快速排序\ndef quick_sort(arr, low, high):\n # 如果区间长度大于1\n if low < high:\n # 对区间进行划分,得到基准元素的位置\n p = partition(arr, low, high)\n # 对左边子区间进行快速排序\n quick_sort(arr, low, p - 1)\n # 对右边子区间进行快速排序\n quick_sort(arr, p + 1, high)\n\n# 测试代码\narr = [5, 3, 7, 2, 9, 1, 4, 6, 8]\nprint(\"原始数组:\", arr)\nquick_sort(arr, 0, len(arr) - 1)\nprint(\"排序后的数组:\", arr)\n```", id: '7' },
|
68 |
-
{
|
69 |
-
author: 'bot', text: "好的,我会尝试画一只猫。\n > ",
|
70 |
-
id: '8'
|
71 |
-
}
|
72 |
-
]
|
73 |
-
|
74 |
-
export const GreetMessages = [
|
75 |
-
'谢谢你! 知道你什么时候准备好继续前进总是很有帮助的。我现在能为你回答什么问题?',
|
76 |
-
'重新开始总是很棒。问我任何问题!',
|
77 |
-
'当然,我很乐意重新开始。我现在可以为你提供哪些帮助?',
|
78 |
-
'当然,我已准备好进行新的挑战。我现在可以为你做什么?',
|
79 |
-
'很好,让我们来更改主题。你在想什么?',
|
80 |
-
'不用担心,我很高兴尝试一些新内容。我现在可以为你回答什么问题?',
|
81 |
-
'好的,我准备好了!感谢重置。我们应该了解哪些内容?',
|
82 |
-
'感谢刷新!你有新的话题吗?',
|
83 |
-
'明白了,让我们重新开始。接下来应该讨论什么?',
|
84 |
-
'下一步!我可以为你做什么?',
|
85 |
-
'好的,我已准备好新话题。我们应该一起了解哪些内容?'
|
86 |
-
]
|
87 |
-
|
88 |
-
export const bingConversationStyleAtom = atomWithStorage<BingConversationStyle>('bingConversationStyle', BingConversationStyle.Creative, undefined, { unstable_getOnInit: true })
|
89 |
-
export const voiceAtom = atomWithStorage<boolean>('enableTTS', false, undefined, { unstable_getOnInit: true })
|
90 |
-
|
91 |
-
type Param = { botId: BotId; page: string }
|
92 |
-
|
93 |
-
const createBotInstance = () => {
|
94 |
-
return new BingWebBot({
|
95 |
-
cookie: ' ',
|
96 |
-
ua: ' ',
|
97 |
-
})
|
98 |
-
}
|
99 |
-
|
100 |
-
export const chatFamily = atomFamily(
|
101 |
-
(param: Param) => {
|
102 |
-
return atomWithImmer({
|
103 |
-
botId: param.botId,
|
104 |
-
bot: createBotInstance(),
|
105 |
-
messages: [] as ChatMessageModel[],
|
106 |
-
generatingMessageId: '',
|
107 |
-
abortController: undefined as AbortController | undefined,
|
108 |
-
conversationId: nanoid(),
|
109 |
-
})
|
110 |
-
},
|
111 |
-
(a, b) => a.botId === b.botId && a.page === b.page,
|
112 |
-
)
|
113 |
-
|
114 |
-
export const hashAtom = atomWithHash('dialog', '')
|
115 |
-
|
116 |
-
export const locationAtom = atomWithLocation()
|
117 |
-
|
118 |
-
export const voiceListenAtom = atom(false)
|
|
|
|
|
|
|
|
|
|
|
|
|
|
|
|
|
|
|
|
|
|
|
|
|
|
|
|
|
|
|
|
|
|
|
|
|
|
|
|
|
|
|
|
|
|
|
|
|
|
|
|
|
|
|
|
|
|
|
|
|
|
|
|
|
|
|
|
|
|
|
|
|
|
|
|
|
|
|
|
|
|
|
|
|
|
|
|
|
|
|
|
|
|
|
|
|
|
|
|
|
|
|
|
|
|
|
|
|
|
|
|
|
|
|
|
|
|
|
|
|
|
|
|
|
|
|
|
|
|
|
|
|
|
|
|
|
|
|
|
|
|
|
|
|
|
|
|
|
|
|
|
|
|
|
|
|
|
|
|
|
|
|
|
|
|
|
|
|
|
|
|
|
|
|
|
|
|
|
|
|
|
|
|
|
|
|
|
|
|
|
|
|
|
|
|
|
|
|
|
|
|
|
|
|
|
|
|
|
|
|
|
|
|
|
|
|
|
|
|
|
|
|
|
|
|
|
|
|
|
|
|
|
|
|
|
|
|
spaces/AFCMEgypt/AFCM_iGEM_LFA/README.md
DELETED
@@ -1,13 +0,0 @@
|
|
1 |
-
---
|
2 |
-
title: AFCM IGEM LFA
|
3 |
-
emoji: 📊
|
4 |
-
colorFrom: red
|
5 |
-
colorTo: gray
|
6 |
-
sdk: gradio
|
7 |
-
sdk_version: 3.4
|
8 |
-
app_file: app.py
|
9 |
-
pinned: false
|
10 |
-
license: gpl-3.0
|
11 |
-
---
|
12 |
-
|
13 |
-
Check out the configuration reference at https://huggingface.co/docs/hub/spaces-config-reference
|
|
|
|
|
|
|
|
|
|
|
|
|
|
|
|
|
|
|
|
|
|
|
|
|
|
|
|
spaces/AI-Zero-to-Hero/01-H5-Play-Canvas-Sim-Physics/index.html
DELETED
@@ -1,10 +0,0 @@
|
|
1 |
-
<body onload="window.scrollTo({top: document.body.scrollHeight, behavior: 'smooth'});">
|
2 |
-
<h1 id="smarter-npc-non-player-character-">SimPhysics</h1>
|
3 |
-
<h4>User input: WASD</h4>
|
4 |
-
<p>This WebGL demo demonstrates PlayCanvas and a physics vehicle simulation that is web based and playable anywhere your browser goes<a href="https://playcanv.as/p/JIwncOGZ/">🤗 Inference API</a>.</p>
|
5 |
-
<p>Source code is in Readme.md file.</p>
|
6 |
-
<p>PlayCanvas project <a href="https://playcanv.as/p/JIwncOGZ/"> is here</a></p>
|
7 |
-
<div style="max-width: 100%;max-height: 100%;width: 100vw;height: 100vh;overflow: hidden;">
|
8 |
-
<iframe style="min-width: 100%; min-height: 100%; background: black;" allowfullscreen="true" frameborder="0" src="https://playcanv.as/p/JIwncOGZ/">></iframe>
|
9 |
-
</div>
|
10 |
-
</body>
|
|
|
|
|
|
|
|
|
|
|
|
|
|
|
|
|
|
|
|
|
|
spaces/AIGC-Audio/AudioGPT/audio_detection/audio_infer/utils/utilities.py
DELETED
@@ -1,172 +0,0 @@
|
|
1 |
-
import os
|
2 |
-
import logging
|
3 |
-
import h5py
|
4 |
-
import soundfile
|
5 |
-
import librosa
|
6 |
-
import numpy as np
|
7 |
-
import pandas as pd
|
8 |
-
from scipy import stats
|
9 |
-
import datetime
|
10 |
-
import pickle
|
11 |
-
|
12 |
-
|
13 |
-
def create_folder(fd):
|
14 |
-
if not os.path.exists(fd):
|
15 |
-
os.makedirs(fd)
|
16 |
-
|
17 |
-
|
18 |
-
def get_filename(path):
|
19 |
-
path = os.path.realpath(path)
|
20 |
-
na_ext = path.split('/')[-1]
|
21 |
-
na = os.path.splitext(na_ext)[0]
|
22 |
-
return na
|
23 |
-
|
24 |
-
|
25 |
-
def get_sub_filepaths(folder):
|
26 |
-
paths = []
|
27 |
-
for root, dirs, files in os.walk(folder):
|
28 |
-
for name in files:
|
29 |
-
path = os.path.join(root, name)
|
30 |
-
paths.append(path)
|
31 |
-
return paths
|
32 |
-
|
33 |
-
|
34 |
-
def create_logging(log_dir, filemode):
|
35 |
-
create_folder(log_dir)
|
36 |
-
i1 = 0
|
37 |
-
|
38 |
-
while os.path.isfile(os.path.join(log_dir, '{:04d}.log'.format(i1))):
|
39 |
-
i1 += 1
|
40 |
-
|
41 |
-
log_path = os.path.join(log_dir, '{:04d}.log'.format(i1))
|
42 |
-
logging.basicConfig(
|
43 |
-
level=logging.DEBUG,
|
44 |
-
format='%(asctime)s %(filename)s[line:%(lineno)d] %(levelname)s %(message)s',
|
45 |
-
datefmt='%a, %d %b %Y %H:%M:%S',
|
46 |
-
filename=log_path,
|
47 |
-
filemode=filemode)
|
48 |
-
|
49 |
-
# Print to console
|
50 |
-
console = logging.StreamHandler()
|
51 |
-
console.setLevel(logging.INFO)
|
52 |
-
formatter = logging.Formatter('%(name)-12s: %(levelname)-8s %(message)s')
|
53 |
-
console.setFormatter(formatter)
|
54 |
-
logging.getLogger('').addHandler(console)
|
55 |
-
|
56 |
-
return logging
|
57 |
-
|
58 |
-
|
59 |
-
def read_metadata(csv_path, classes_num, id_to_ix):
|
60 |
-
"""Read metadata of AudioSet from a csv file.
|
61 |
-
|
62 |
-
Args:
|
63 |
-
csv_path: str
|
64 |
-
|
65 |
-
Returns:
|
66 |
-
meta_dict: {'audio_name': (audios_num,), 'target': (audios_num, classes_num)}
|
67 |
-
"""
|
68 |
-
|
69 |
-
with open(csv_path, 'r') as fr:
|
70 |
-
lines = fr.readlines()
|
71 |
-
lines = lines[3:] # Remove heads
|
72 |
-
|
73 |
-
audios_num = len(lines)
|
74 |
-
targets = np.zeros((audios_num, classes_num), dtype=np.bool)
|
75 |
-
audio_names = []
|
76 |
-
|
77 |
-
for n, line in enumerate(lines):
|
78 |
-
items = line.split(', ')
|
79 |
-
"""items: ['--4gqARaEJE', '0.000', '10.000', '"/m/068hy,/m/07q6cd_,/m/0bt9lr,/m/0jbk"\n']"""
|
80 |
-
|
81 |
-
audio_name = 'Y{}.wav'.format(items[0]) # Audios are started with an extra 'Y' when downloading
|
82 |
-
label_ids = items[3].split('"')[1].split(',')
|
83 |
-
|
84 |
-
audio_names.append(audio_name)
|
85 |
-
|
86 |
-
# Target
|
87 |
-
for id in label_ids:
|
88 |
-
ix = id_to_ix[id]
|
89 |
-
targets[n, ix] = 1
|
90 |
-
|
91 |
-
meta_dict = {'audio_name': np.array(audio_names), 'target': targets}
|
92 |
-
return meta_dict
|
93 |
-
|
94 |
-
|
95 |
-
def float32_to_int16(x):
|
96 |
-
assert np.max(np.abs(x)) <= 1.2
|
97 |
-
x = np.clip(x, -1, 1)
|
98 |
-
return (x * 32767.).astype(np.int16)
|
99 |
-
|
100 |
-
def int16_to_float32(x):
|
101 |
-
return (x / 32767.).astype(np.float32)
|
102 |
-
|
103 |
-
|
104 |
-
def pad_or_truncate(x, audio_length):
|
105 |
-
"""Pad all audio to specific length."""
|
106 |
-
if len(x) <= audio_length:
|
107 |
-
return np.concatenate((x, np.zeros(audio_length - len(x))), axis=0)
|
108 |
-
else:
|
109 |
-
return x[0 : audio_length]
|
110 |
-
|
111 |
-
|
112 |
-
def d_prime(auc):
|
113 |
-
d_prime = stats.norm().ppf(auc) * np.sqrt(2.0)
|
114 |
-
return d_prime
|
115 |
-
|
116 |
-
|
117 |
-
class Mixup(object):
|
118 |
-
def __init__(self, mixup_alpha, random_seed=1234):
|
119 |
-
"""Mixup coefficient generator.
|
120 |
-
"""
|
121 |
-
self.mixup_alpha = mixup_alpha
|
122 |
-
self.random_state = np.random.RandomState(random_seed)
|
123 |
-
|
124 |
-
def get_lambda(self, batch_size):
|
125 |
-
"""Get mixup random coefficients.
|
126 |
-
Args:
|
127 |
-
batch_size: int
|
128 |
-
Returns:
|
129 |
-
mixup_lambdas: (batch_size,)
|
130 |
-
"""
|
131 |
-
mixup_lambdas = []
|
132 |
-
for n in range(0, batch_size, 2):
|
133 |
-
lam = self.random_state.beta(self.mixup_alpha, self.mixup_alpha, 1)[0]
|
134 |
-
mixup_lambdas.append(lam)
|
135 |
-
mixup_lambdas.append(1. - lam)
|
136 |
-
|
137 |
-
return np.array(mixup_lambdas)
|
138 |
-
|
139 |
-
|
140 |
-
class StatisticsContainer(object):
|
141 |
-
def __init__(self, statistics_path):
|
142 |
-
"""Contain statistics of different training iterations.
|
143 |
-
"""
|
144 |
-
self.statistics_path = statistics_path
|
145 |
-
|
146 |
-
self.backup_statistics_path = '{}_{}.pkl'.format(
|
147 |
-
os.path.splitext(self.statistics_path)[0],
|
148 |
-
datetime.datetime.now().strftime('%Y-%m-%d_%H-%M-%S'))
|
149 |
-
|
150 |
-
self.statistics_dict = {'bal': [], 'test': []}
|
151 |
-
|
152 |
-
def append(self, iteration, statistics, data_type):
|
153 |
-
statistics['iteration'] = iteration
|
154 |
-
self.statistics_dict[data_type].append(statistics)
|
155 |
-
|
156 |
-
def dump(self):
|
157 |
-
pickle.dump(self.statistics_dict, open(self.statistics_path, 'wb'))
|
158 |
-
pickle.dump(self.statistics_dict, open(self.backup_statistics_path, 'wb'))
|
159 |
-
logging.info(' Dump statistics to {}'.format(self.statistics_path))
|
160 |
-
logging.info(' Dump statistics to {}'.format(self.backup_statistics_path))
|
161 |
-
|
162 |
-
def load_state_dict(self, resume_iteration):
|
163 |
-
self.statistics_dict = pickle.load(open(self.statistics_path, 'rb'))
|
164 |
-
|
165 |
-
resume_statistics_dict = {'bal': [], 'test': []}
|
166 |
-
|
167 |
-
for key in self.statistics_dict.keys():
|
168 |
-
for statistics in self.statistics_dict[key]:
|
169 |
-
if statistics['iteration'] <= resume_iteration:
|
170 |
-
resume_statistics_dict[key].append(statistics)
|
171 |
-
|
172 |
-
self.statistics_dict = resume_statistics_dict
|
|
|
|
|
|
|
|
|
|
|
|
|
|
|
|
|
|
|
|
|
|
|
|
|
|
|
|
|
|
|
|
|
|
|
|
|
|
|
|
|
|
|
|
|
|
|
|
|
|
|
|
|
|
|
|
|
|
|
|
|
|
|
|
|
|
|
|
|
|
|
|
|
|
|
|
|
|
|
|
|
|
|
|
|
|
|
|
|
|
|
|
|
|
|
|
|
|
|
|
|
|
|
|
|
|
|
|
|
|
|
|
|
|
|
|
|
|
|
|
|
|
|
|
|
|
|
|
|
|
|
|
|
|
|
|
|
|
|
|
|
|
|
|
|
|
|
|
|
|
|
|
|
|
|
|
|
|
|
|
|
|
|
|
|
|
|
|
|
|
|
|
|
|
|
|
|
|
|
|
|
|
|
|
|
|
|
|
|
|
|
|
|
|
|
|
|
|
|
|
|
|
|
|
|
|
|
|
|
|
|
|
|
|
|
|
|
|
|
|
|
|
|
|
|
|
|
|
|
|
|
|
|
|
|
|
|
|
|
|
|
|
|
|
|
|
|
|
|
|
|
|
|
|
|
|
|
|
|
|
|
|
|
|
|
|
|
|
|
|
|
|
|
|
|
|
|
|
|
|
|
|
|
|
|
|
|
|
|
|
|
|
|
|
|
|
|
|
|
|
|
|
|
|
|
|
|
|
|
|
|
|
|
|
|
|
|
|
|
|
|
|
|
|
|
|
|
|
|
|
|
|
|
|
|
|
|
|
|
|
|
|
|
|
|
|
spaces/AgentVerse/agentVerse/agentverse/agents/tasksolving_agent/solver.py
DELETED
@@ -1,110 +0,0 @@
|
|
1 |
-
from __future__ import annotations
|
2 |
-
|
3 |
-
import asyncio
|
4 |
-
from colorama import Fore
|
5 |
-
|
6 |
-
from agentverse.logging import get_logger
|
7 |
-
import bdb
|
8 |
-
from string import Template
|
9 |
-
from typing import TYPE_CHECKING, List, Tuple
|
10 |
-
|
11 |
-
# from agentverse.environments import PipelineEnvironment
|
12 |
-
from agentverse.message import SolverMessage, Message, CriticMessage
|
13 |
-
|
14 |
-
from agentverse.agents import agent_registry
|
15 |
-
from agentverse.agents.base import BaseAgent
|
16 |
-
from agentverse.utils import AgentCriticism
|
17 |
-
|
18 |
-
|
19 |
-
logger = get_logger()
|
20 |
-
|
21 |
-
|
22 |
-
@agent_registry.register("solver")
|
23 |
-
class SolverAgent(BaseAgent):
|
24 |
-
max_history: int = 3
|
25 |
-
|
26 |
-
def step(
|
27 |
-
self, former_solution: str, advice: str, task_description: str = "", **kwargs
|
28 |
-
) -> SolverMessage:
|
29 |
-
logger.debug("", self.name, Fore.MAGENTA)
|
30 |
-
# prompt = self._fill_prompt_template(
|
31 |
-
# former_solution, critic_opinions, advice, task_description
|
32 |
-
# )
|
33 |
-
prepend_prompt, append_prompt = self.get_all_prompts(
|
34 |
-
former_solution=former_solution,
|
35 |
-
task_description=task_description,
|
36 |
-
advice=advice,
|
37 |
-
role_description=self.role_description,
|
38 |
-
**kwargs,
|
39 |
-
)
|
40 |
-
history = self.memory.to_messages(self.name, start_index=-self.max_history)
|
41 |
-
parsed_response = None
|
42 |
-
for i in range(self.max_retry):
|
43 |
-
try:
|
44 |
-
response = self.llm.generate_response(
|
45 |
-
prepend_prompt, history, append_prompt
|
46 |
-
)
|
47 |
-
parsed_response = self.output_parser.parse(response)
|
48 |
-
break
|
49 |
-
except (KeyboardInterrupt, bdb.BdbQuit):
|
50 |
-
raise
|
51 |
-
except Exception as e:
|
52 |
-
logger.error(e)
|
53 |
-
logger.warn("Retrying...")
|
54 |
-
continue
|
55 |
-
|
56 |
-
if parsed_response is None:
|
57 |
-
logger.error(f"{self.name} failed to generate valid response.")
|
58 |
-
|
59 |
-
message = SolverMessage(
|
60 |
-
content=""
|
61 |
-
if parsed_response is None
|
62 |
-
else parsed_response.return_values["output"],
|
63 |
-
sender=self.name,
|
64 |
-
receiver=self.get_receiver(),
|
65 |
-
)
|
66 |
-
return message
|
67 |
-
|
68 |
-
async def astep(self, env_description: str = "") -> SolverMessage:
|
69 |
-
"""Asynchronous version of step"""
|
70 |
-
pass
|
71 |
-
|
72 |
-
def _fill_prompt_template(
|
73 |
-
self,
|
74 |
-
former_solution: str,
|
75 |
-
critic_opinions: List[AgentCriticism],
|
76 |
-
advice: str,
|
77 |
-
task_description: str,
|
78 |
-
) -> str:
|
79 |
-
"""Fill the placeholders in the prompt template
|
80 |
-
|
81 |
-
In the role_assigner agent, three placeholders are supported:
|
82 |
-
- ${task_description}
|
83 |
-
- ${former_solution}
|
84 |
-
- ${critic_messages}
|
85 |
-
- ${advice}
|
86 |
-
"""
|
87 |
-
input_arguments = {
|
88 |
-
"task_description": task_description,
|
89 |
-
"former_solution": former_solution,
|
90 |
-
"critic_opinions": "\n".join(
|
91 |
-
[
|
92 |
-
f"{critic.sender_agent.role_description} said: {critic.criticism}"
|
93 |
-
for critic in critic_opinions
|
94 |
-
]
|
95 |
-
),
|
96 |
-
"advice": advice,
|
97 |
-
}
|
98 |
-
# if discussion_mode:
|
99 |
-
# template = Template(self.prompt_template[1])
|
100 |
-
# else:
|
101 |
-
template = Template(self.prompt_template)
|
102 |
-
return template.safe_substitute(input_arguments)
|
103 |
-
|
104 |
-
def add_message_to_memory(self, messages: List[Message]) -> None:
|
105 |
-
self.memory.add_message(messages)
|
106 |
-
|
107 |
-
def reset(self) -> None:
|
108 |
-
"""Reset the agent"""
|
109 |
-
self.memory.reset()
|
110 |
-
# TODO: reset receiver
|
|
|
|
|
|
|
|
|
|
|
|
|
|
|
|
|
|
|
|
|
|
|
|
|
|
|
|
|
|
|
|
|
|
|
|
|
|
|
|
|
|
|
|
|
|
|
|
|
|
|
|
|
|
|
|
|
|
|
|
|
|
|
|
|
|
|
|
|
|
|
|
|
|
|
|
|
|
|
|
|
|
|
|
|
|
|
|
|
|
|
|
|
|
|
|
|
|
|
|
|
|
|
|
|
|
|
|
|
|
|
|
|
|
|
|
|
|
|
|
|
|
|
|
|
|
|
|
|
|
|
|
|
|
|
|
|
|
|
|
|
|
|
|
|
|
|
|
|
|
|
|
|
|
|
|
|
|
|
|
|
|
|
|
|
|
|
|
|
|
|
|
|
|
|
|
|
|
|
|
|
|
|
|
|
|
|
|
|
|
|
|
|
|
|
|
|
|
|
|
|
|
|
|
|
|
|
|
|
|
|
|
|
|
|
|
|
|
|
|
|
|
spaces/AgentVerse/agentVerse/ui/src/phaser3-rex-plugins/templates/ui/gridtable/input/EmitCellEvent.js
DELETED
@@ -1,17 +0,0 @@
|
|
1 |
-
var EmitCellEvent = function (eventEmitter, eventName, table, x, y, pointer, event) {
|
2 |
-
var cellIndex;
|
3 |
-
if (y === undefined) {
|
4 |
-
cellIndex = x;
|
5 |
-
} else {
|
6 |
-
cellIndex = table.pointToCellIndex(x, y);
|
7 |
-
}
|
8 |
-
if ((cellIndex === null) || (cellIndex === undefined)) {
|
9 |
-
return;
|
10 |
-
}
|
11 |
-
var cellContainer = table.getCellContainer(cellIndex);
|
12 |
-
if (cellContainer) {
|
13 |
-
eventEmitter.emit(eventName, cellContainer, cellIndex, pointer, event);
|
14 |
-
}
|
15 |
-
}
|
16 |
-
|
17 |
-
export default EmitCellEvent;
|
|
|
|
|
|
|
|
|
|
|
|
|
|
|
|
|
|
|
|
|
|
|
|
|
|
|
|
|
|
|
|
|
|
|
|
spaces/AhmadHakami/Alzheimer_image_classification/app.py
DELETED
@@ -1,25 +0,0 @@
|
|
1 |
-
from transformers import pipeline
|
2 |
-
import gradio as gr
|
3 |
-
import os
|
4 |
-
|
5 |
-
model = pipeline(model = "AhmadHakami/alzheimer-image-classification-google-vit-base-patch16",
|
6 |
-
task = "image-classification")
|
7 |
-
|
8 |
-
model.model.config.id2label = {
|
9 |
-
0: 'خفيف الخرف (Mild Demented)',
|
10 |
-
1: 'متوسط الخرف (Moderate Demented)',
|
11 |
-
2: 'غير مصاب بالخرف (Non Demented)',
|
12 |
-
3: 'خفيف جداً الخرف (Very Mild Demented)'
|
13 |
-
}
|
14 |
-
|
15 |
-
examples = []
|
16 |
-
for image in os.listdir("examples"):
|
17 |
-
examples.append(f'examples//{image}')
|
18 |
-
|
19 |
-
|
20 |
-
gr.Interface.from_pipeline(model,
|
21 |
-
title="Tbyan - تِــبْيان Alzheimer MRI Classification",
|
22 |
-
description="This model fine-tuned using vit-base-patch16-224-in21k by Google, and trained on credible MRI data from Open Access Series of Imaging Studies (OASIS) and the data annotated by experts, it aims to expedite patient results, developed by Tbyan تبيان Team at AI course by `Misk Foundation & Samsung Innovation Campus`, \n **Try it now:**",
|
23 |
-
examples = examples).launch()
|
24 |
-
|
25 |
-
|
|
|
|
|
|
|
|
|
|
|
|
|
|
|
|
|
|
|
|
|
|
|
|
|
|
|
|
|
|
|
|
|
|
|
|
|
|
|
|
|
|
|
|
|
|
|
|
|
|
|
|
spaces/Ajaxon6255/Emerald_Isle/theme_dropdown.py
DELETED
@@ -1,57 +0,0 @@
|
|
1 |
-
import os
|
2 |
-
import pathlib
|
3 |
-
|
4 |
-
from gradio.themes.utils import ThemeAsset
|
5 |
-
|
6 |
-
|
7 |
-
def create_theme_dropdown():
|
8 |
-
import gradio as gr
|
9 |
-
|
10 |
-
asset_path = pathlib.Path(__file__).parent / "themes"
|
11 |
-
themes = []
|
12 |
-
for theme_asset in os.listdir(str(asset_path)):
|
13 |
-
themes.append(
|
14 |
-
(ThemeAsset(theme_asset), gr.Theme.load(str(asset_path / theme_asset)))
|
15 |
-
)
|
16 |
-
|
17 |
-
def make_else_if(theme_asset):
|
18 |
-
return f"""
|
19 |
-
else if (theme == '{str(theme_asset[0].version)}') {{
|
20 |
-
var theme_css = `{theme_asset[1]._get_theme_css()}`
|
21 |
-
}}"""
|
22 |
-
|
23 |
-
head, tail = themes[0], themes[1:]
|
24 |
-
if_statement = f"""
|
25 |
-
if (theme == "{str(head[0].version)}") {{
|
26 |
-
var theme_css = `{head[1]._get_theme_css()}`
|
27 |
-
}} {" ".join(make_else_if(t) for t in tail)}
|
28 |
-
"""
|
29 |
-
|
30 |
-
latest_to_oldest = sorted([t[0] for t in themes], key=lambda asset: asset.version)[
|
31 |
-
::-1
|
32 |
-
]
|
33 |
-
latest_to_oldest = [str(t.version) for t in latest_to_oldest]
|
34 |
-
|
35 |
-
component = gr.Dropdown(
|
36 |
-
choices=latest_to_oldest,
|
37 |
-
value=latest_to_oldest[0],
|
38 |
-
render=False,
|
39 |
-
label="Select Version",
|
40 |
-
).style(container=False)
|
41 |
-
|
42 |
-
return (
|
43 |
-
component,
|
44 |
-
f"""
|
45 |
-
(theme) => {{
|
46 |
-
if (!document.querySelector('.theme-css')) {{
|
47 |
-
var theme_elem = document.createElement('style');
|
48 |
-
theme_elem.classList.add('theme-css');
|
49 |
-
document.head.appendChild(theme_elem);
|
50 |
-
}} else {{
|
51 |
-
var theme_elem = document.querySelector('.theme-css');
|
52 |
-
}}
|
53 |
-
{if_statement}
|
54 |
-
theme_elem.innerHTML = theme_css;
|
55 |
-
}}
|
56 |
-
""",
|
57 |
-
)
|
|
|
|
|
|
|
|
|
|
|
|
|
|
|
|
|
|
|
|
|
|
|
|
|
|
|
|
|
|
|
|
|
|
|
|
|
|
|
|
|
|
|
|
|
|
|
|
|
|
|
|
|
|
|
|
|
|
|
|
|
|
|
|
|
|
|
|
|
|
|
|
|
|
|
|
|
|
|
|
|
|
|
|
|
|
|
|
|
|
|
|
|
|
|
|
|
|
|
|
|
|
|
|
|
|
|
|
|
|
|
|
|
|
|
|
spaces/AlexWang/lama/models/ade20k/segm_lib/nn/parallel/data_parallel.py
DELETED
@@ -1,112 +0,0 @@
|
|
1 |
-
# -*- coding: utf8 -*-
|
2 |
-
|
3 |
-
import torch.cuda as cuda
|
4 |
-
import torch.nn as nn
|
5 |
-
import torch
|
6 |
-
import collections
|
7 |
-
from torch.nn.parallel._functions import Gather
|
8 |
-
|
9 |
-
|
10 |
-
__all__ = ['UserScatteredDataParallel', 'user_scattered_collate', 'async_copy_to']
|
11 |
-
|
12 |
-
|
13 |
-
def async_copy_to(obj, dev, main_stream=None):
|
14 |
-
if torch.is_tensor(obj):
|
15 |
-
v = obj.cuda(dev, non_blocking=True)
|
16 |
-
if main_stream is not None:
|
17 |
-
v.data.record_stream(main_stream)
|
18 |
-
return v
|
19 |
-
elif isinstance(obj, collections.Mapping):
|
20 |
-
return {k: async_copy_to(o, dev, main_stream) for k, o in obj.items()}
|
21 |
-
elif isinstance(obj, collections.Sequence):
|
22 |
-
return [async_copy_to(o, dev, main_stream) for o in obj]
|
23 |
-
else:
|
24 |
-
return obj
|
25 |
-
|
26 |
-
|
27 |
-
def dict_gather(outputs, target_device, dim=0):
|
28 |
-
"""
|
29 |
-
Gathers variables from different GPUs on a specified device
|
30 |
-
(-1 means the CPU), with dictionary support.
|
31 |
-
"""
|
32 |
-
def gather_map(outputs):
|
33 |
-
out = outputs[0]
|
34 |
-
if torch.is_tensor(out):
|
35 |
-
# MJY(20180330) HACK:: force nr_dims > 0
|
36 |
-
if out.dim() == 0:
|
37 |
-
outputs = [o.unsqueeze(0) for o in outputs]
|
38 |
-
return Gather.apply(target_device, dim, *outputs)
|
39 |
-
elif out is None:
|
40 |
-
return None
|
41 |
-
elif isinstance(out, collections.Mapping):
|
42 |
-
return {k: gather_map([o[k] for o in outputs]) for k in out}
|
43 |
-
elif isinstance(out, collections.Sequence):
|
44 |
-
return type(out)(map(gather_map, zip(*outputs)))
|
45 |
-
return gather_map(outputs)
|
46 |
-
|
47 |
-
|
48 |
-
class DictGatherDataParallel(nn.DataParallel):
|
49 |
-
def gather(self, outputs, output_device):
|
50 |
-
return dict_gather(outputs, output_device, dim=self.dim)
|
51 |
-
|
52 |
-
|
53 |
-
class UserScatteredDataParallel(DictGatherDataParallel):
|
54 |
-
def scatter(self, inputs, kwargs, device_ids):
|
55 |
-
assert len(inputs) == 1
|
56 |
-
inputs = inputs[0]
|
57 |
-
inputs = _async_copy_stream(inputs, device_ids)
|
58 |
-
inputs = [[i] for i in inputs]
|
59 |
-
assert len(kwargs) == 0
|
60 |
-
kwargs = [{} for _ in range(len(inputs))]
|
61 |
-
|
62 |
-
return inputs, kwargs
|
63 |
-
|
64 |
-
|
65 |
-
def user_scattered_collate(batch):
|
66 |
-
return batch
|
67 |
-
|
68 |
-
|
69 |
-
def _async_copy(inputs, device_ids):
|
70 |
-
nr_devs = len(device_ids)
|
71 |
-
assert type(inputs) in (tuple, list)
|
72 |
-
assert len(inputs) == nr_devs
|
73 |
-
|
74 |
-
outputs = []
|
75 |
-
for i, dev in zip(inputs, device_ids):
|
76 |
-
with cuda.device(dev):
|
77 |
-
outputs.append(async_copy_to(i, dev))
|
78 |
-
|
79 |
-
return tuple(outputs)
|
80 |
-
|
81 |
-
|
82 |
-
def _async_copy_stream(inputs, device_ids):
|
83 |
-
nr_devs = len(device_ids)
|
84 |
-
assert type(inputs) in (tuple, list)
|
85 |
-
assert len(inputs) == nr_devs
|
86 |
-
|
87 |
-
outputs = []
|
88 |
-
streams = [_get_stream(d) for d in device_ids]
|
89 |
-
for i, dev, stream in zip(inputs, device_ids, streams):
|
90 |
-
with cuda.device(dev):
|
91 |
-
main_stream = cuda.current_stream()
|
92 |
-
with cuda.stream(stream):
|
93 |
-
outputs.append(async_copy_to(i, dev, main_stream=main_stream))
|
94 |
-
main_stream.wait_stream(stream)
|
95 |
-
|
96 |
-
return outputs
|
97 |
-
|
98 |
-
|
99 |
-
"""Adapted from: torch/nn/parallel/_functions.py"""
|
100 |
-
# background streams used for copying
|
101 |
-
_streams = None
|
102 |
-
|
103 |
-
|
104 |
-
def _get_stream(device):
|
105 |
-
"""Gets a background stream for copying between CPU and GPU"""
|
106 |
-
global _streams
|
107 |
-
if device == -1:
|
108 |
-
return None
|
109 |
-
if _streams is None:
|
110 |
-
_streams = [None] * cuda.device_count()
|
111 |
-
if _streams[device] is None: _streams[device] = cuda.Stream(device)
|
112 |
-
return _streams[device]
|
|
|
|
|
|
|
|
|
|
|
|
|
|
|
|
|
|
|
|
|
|
|
|
|
|
|
|
|
|
|
|
|
|
|
|
|
|
|
|
|
|
|
|
|
|
|
|
|
|
|
|
|
|
|
|
|
|
|
|
|
|
|
|
|
|
|
|
|
|
|
|
|
|
|
|
|
|
|
|
|
|
|
|
|
|
|
|
|
|
|
|
|
|
|
|
|
|
|
|
|
|
|
|
|
|
|
|
|
|
|
|
|
|
|
|
|
|
|
|
|
|
|
|
|
|
|
|
|
|
|
|
|
|
|
|
|
|
|
|
|
|
|
|
|
|
|
|
|
|
|
|
|
|
|
|
|
|
|
|
|
|
|
|
|
|
|
|
|
|
|
|
|
|
|
|
|
|
|
|
|
|
|
|
|
|
|
|
|
|
|
|
|
|
|
|
|
|
|
|
|
|
|
|
|
|
|
|
|
|
|
|
|
|
|
|
|
|
|
|
|
|
|
|
|
|
spaces/Amiminoru/Deus/README.md
DELETED
@@ -1,10 +0,0 @@
|
|
1 |
-
---
|
2 |
-
title: Deus
|
3 |
-
emoji: 👁
|
4 |
-
colorFrom: red
|
5 |
-
colorTo: red
|
6 |
-
sdk: docker
|
7 |
-
pinned: false
|
8 |
-
---
|
9 |
-
|
10 |
-
Check out the configuration reference at https://huggingface.co/docs/hub/spaces-config-reference
|
|
|
|
|
|
|
|
|
|
|
|
|
|
|
|
|
|
|
|
|
|
spaces/Amrrs/DragGan-Inversion/PTI/models/e4e/stylegan2/__init__.py
DELETED
File without changes
|
spaces/Androidonnxfork/CivitAi-to-Diffusers/diffusers/docs/source/en/optimization/torch2.0.md
DELETED
@@ -1,444 +0,0 @@
|
|
1 |
-
<!--Copyright 2023 The HuggingFace Team. All rights reserved.
|
2 |
-
|
3 |
-
Licensed under the Apache License, Version 2.0 (the "License"); you may not use this file except in compliance with
|
4 |
-
the License. You may obtain a copy of the License at
|
5 |
-
|
6 |
-
http://www.apache.org/licenses/LICENSE-2.0
|
7 |
-
|
8 |
-
Unless required by applicable law or agreed to in writing, software distributed under the License is distributed on
|
9 |
-
an "AS IS" BASIS, WITHOUT WARRANTIES OR CONDITIONS OF ANY KIND, either express or implied. See the License for the
|
10 |
-
specific language governing permissions and limitations under the License.
|
11 |
-
-->
|
12 |
-
|
13 |
-
# Accelerated PyTorch 2.0 support in Diffusers
|
14 |
-
|
15 |
-
Starting from version `0.13.0`, Diffusers supports the latest optimization from [PyTorch 2.0](https://pytorch.org/get-started/pytorch-2.0/). These include:
|
16 |
-
1. Support for accelerated transformers implementation with memory-efficient attention – no extra dependencies (such as `xformers`) required.
|
17 |
-
2. [torch.compile](https://pytorch.org/tutorials/intermediate/torch_compile_tutorial.html) support for extra performance boost when individual models are compiled.
|
18 |
-
|
19 |
-
|
20 |
-
## Installation
|
21 |
-
|
22 |
-
To benefit from the accelerated attention implementation and `torch.compile()`, you just need to install the latest versions of PyTorch 2.0 from pip, and make sure you are on diffusers 0.13.0 or later. As explained below, diffusers automatically uses the optimized attention processor ([`AttnProcessor2_0`](https://github.com/huggingface/diffusers/blob/1a5797c6d4491a879ea5285c4efc377664e0332d/src/diffusers/models/attention_processor.py#L798)) (but not `torch.compile()`)
|
23 |
-
when PyTorch 2.0 is available.
|
24 |
-
|
25 |
-
```bash
|
26 |
-
pip install --upgrade torch diffusers
|
27 |
-
```
|
28 |
-
|
29 |
-
## Using accelerated transformers and `torch.compile`.
|
30 |
-
|
31 |
-
|
32 |
-
1. **Accelerated Transformers implementation**
|
33 |
-
|
34 |
-
PyTorch 2.0 includes an optimized and memory-efficient attention implementation through the [`torch.nn.functional.scaled_dot_product_attention`](https://pytorch.org/docs/master/generated/torch.nn.functional.scaled_dot_product_attention) function, which automatically enables several optimizations depending on the inputs and the GPU type. This is similar to the `memory_efficient_attention` from [xFormers](https://github.com/facebookresearch/xformers), but built natively into PyTorch.
|
35 |
-
|
36 |
-
These optimizations will be enabled by default in Diffusers if PyTorch 2.0 is installed and if `torch.nn.functional.scaled_dot_product_attention` is available. To use it, just install `torch 2.0` as suggested above and simply use the pipeline. For example:
|
37 |
-
|
38 |
-
```Python
|
39 |
-
import torch
|
40 |
-
from diffusers import DiffusionPipeline
|
41 |
-
|
42 |
-
pipe = DiffusionPipeline.from_pretrained("runwayml/stable-diffusion-v1-5", torch_dtype=torch.float16)
|
43 |
-
pipe = pipe.to("cuda")
|
44 |
-
|
45 |
-
prompt = "a photo of an astronaut riding a horse on mars"
|
46 |
-
image = pipe(prompt).images[0]
|
47 |
-
```
|
48 |
-
|
49 |
-
If you want to enable it explicitly (which is not required), you can do so as shown below.
|
50 |
-
|
51 |
-
```diff
|
52 |
-
import torch
|
53 |
-
from diffusers import DiffusionPipeline
|
54 |
-
+ from diffusers.models.attention_processor import AttnProcessor2_0
|
55 |
-
|
56 |
-
pipe = DiffusionPipeline.from_pretrained("runwayml/stable-diffusion-v1-5", torch_dtype=torch.float16).to("cuda")
|
57 |
-
+ pipe.unet.set_attn_processor(AttnProcessor2_0())
|
58 |
-
|
59 |
-
prompt = "a photo of an astronaut riding a horse on mars"
|
60 |
-
image = pipe(prompt).images[0]
|
61 |
-
```
|
62 |
-
|
63 |
-
This should be as fast and memory efficient as `xFormers`. More details [in our benchmark](#benchmark).
|
64 |
-
|
65 |
-
It is possible to revert to the vanilla attention processor ([`AttnProcessor`](https://github.com/huggingface/diffusers/blob/1a5797c6d4491a879ea5285c4efc377664e0332d/src/diffusers/models/attention_processor.py#L402)), which can be helpful to make the pipeline more deterministic, or if you need to convert a fine-tuned model to other formats such as [Core ML](https://huggingface.co/docs/diffusers/v0.16.0/en/optimization/coreml#how-to-run-stable-diffusion-with-core-ml). To use the normal attention processor you can use the [`~diffusers.UNet2DConditionModel.set_default_attn_processor`] function:
|
66 |
-
|
67 |
-
```Python
|
68 |
-
import torch
|
69 |
-
from diffusers import DiffusionPipeline
|
70 |
-
from diffusers.models.attention_processor import AttnProcessor
|
71 |
-
|
72 |
-
pipe = DiffusionPipeline.from_pretrained("runwayml/stable-diffusion-v1-5", torch_dtype=torch.float16).to("cuda")
|
73 |
-
pipe.unet.set_default_attn_processor()
|
74 |
-
|
75 |
-
prompt = "a photo of an astronaut riding a horse on mars"
|
76 |
-
image = pipe(prompt).images[0]
|
77 |
-
```
|
78 |
-
|
79 |
-
2. **torch.compile**
|
80 |
-
|
81 |
-
To get an additional speedup, we can use the new `torch.compile` feature. Since the UNet of the pipeline is usually the most computationally expensive, we wrap the `unet` with `torch.compile` leaving rest of the sub-models (text encoder and VAE) as is. For more information and different options, refer to the
|
82 |
-
[torch compile docs](https://pytorch.org/tutorials/intermediate/torch_compile_tutorial.html).
|
83 |
-
|
84 |
-
```python
|
85 |
-
pipe.unet = torch.compile(pipe.unet, mode="reduce-overhead", fullgraph=True)
|
86 |
-
images = pipe(prompt, num_inference_steps=steps, num_images_per_prompt=batch_size).images
|
87 |
-
```
|
88 |
-
|
89 |
-
Depending on the type of GPU, `compile()` can yield between **5% - 300%** of _additional speed-up_ over the accelerated transformer optimizations. Note, however, that compilation is able to squeeze more performance improvements in more recent GPU architectures such as Ampere (A100, 3090), Ada (4090) and Hopper (H100).
|
90 |
-
|
91 |
-
Compilation takes some time to complete, so it is best suited for situations where you need to prepare your pipeline once and then perform the same type of inference operations multiple times. Calling the compiled pipeline on a different image size will re-trigger compilation which can be expensive.
|
92 |
-
|
93 |
-
|
94 |
-
## Benchmark
|
95 |
-
|
96 |
-
We conducted a comprehensive benchmark with PyTorch 2.0's efficient attention implementation and `torch.compile` across different GPUs and batch sizes for five of our most used pipelines. We used `diffusers 0.17.0.dev0`, which [makes sure `torch.compile()` is leveraged optimally](https://github.com/huggingface/diffusers/pull/3313).
|
97 |
-
|
98 |
-
### Benchmarking code
|
99 |
-
|
100 |
-
#### Stable Diffusion text-to-image
|
101 |
-
|
102 |
-
```python
|
103 |
-
from diffusers import DiffusionPipeline
|
104 |
-
import torch
|
105 |
-
|
106 |
-
path = "runwayml/stable-diffusion-v1-5"
|
107 |
-
|
108 |
-
run_compile = True # Set True / False
|
109 |
-
|
110 |
-
pipe = DiffusionPipeline.from_pretrained(path, torch_dtype=torch.float16)
|
111 |
-
pipe = pipe.to("cuda")
|
112 |
-
pipe.unet.to(memory_format=torch.channels_last)
|
113 |
-
|
114 |
-
if run_compile:
|
115 |
-
print("Run torch compile")
|
116 |
-
pipe.unet = torch.compile(pipe.unet, mode="reduce-overhead", fullgraph=True)
|
117 |
-
|
118 |
-
prompt = "ghibli style, a fantasy landscape with castles"
|
119 |
-
|
120 |
-
for _ in range(3):
|
121 |
-
images = pipe(prompt=prompt).images
|
122 |
-
```
|
123 |
-
|
124 |
-
#### Stable Diffusion image-to-image
|
125 |
-
|
126 |
-
```python
|
127 |
-
from diffusers import StableDiffusionImg2ImgPipeline
|
128 |
-
import requests
|
129 |
-
import torch
|
130 |
-
from PIL import Image
|
131 |
-
from io import BytesIO
|
132 |
-
|
133 |
-
url = "https://raw.githubusercontent.com/CompVis/stable-diffusion/main/assets/stable-samples/img2img/sketch-mountains-input.jpg"
|
134 |
-
|
135 |
-
response = requests.get(url)
|
136 |
-
init_image = Image.open(BytesIO(response.content)).convert("RGB")
|
137 |
-
init_image = init_image.resize((512, 512))
|
138 |
-
|
139 |
-
path = "runwayml/stable-diffusion-v1-5"
|
140 |
-
|
141 |
-
run_compile = True # Set True / False
|
142 |
-
|
143 |
-
pipe = StableDiffusionImg2ImgPipeline.from_pretrained(path, torch_dtype=torch.float16)
|
144 |
-
pipe = pipe.to("cuda")
|
145 |
-
pipe.unet.to(memory_format=torch.channels_last)
|
146 |
-
|
147 |
-
if run_compile:
|
148 |
-
print("Run torch compile")
|
149 |
-
pipe.unet = torch.compile(pipe.unet, mode="reduce-overhead", fullgraph=True)
|
150 |
-
|
151 |
-
prompt = "ghibli style, a fantasy landscape with castles"
|
152 |
-
|
153 |
-
for _ in range(3):
|
154 |
-
image = pipe(prompt=prompt, image=init_image).images[0]
|
155 |
-
```
|
156 |
-
|
157 |
-
#### Stable Diffusion - inpainting
|
158 |
-
|
159 |
-
```python
|
160 |
-
from diffusers import StableDiffusionInpaintPipeline
|
161 |
-
import requests
|
162 |
-
import torch
|
163 |
-
from PIL import Image
|
164 |
-
from io import BytesIO
|
165 |
-
|
166 |
-
url = "https://raw.githubusercontent.com/CompVis/stable-diffusion/main/assets/stable-samples/img2img/sketch-mountains-input.jpg"
|
167 |
-
|
168 |
-
def download_image(url):
|
169 |
-
response = requests.get(url)
|
170 |
-
return Image.open(BytesIO(response.content)).convert("RGB")
|
171 |
-
|
172 |
-
|
173 |
-
img_url = "https://raw.githubusercontent.com/CompVis/latent-diffusion/main/data/inpainting_examples/overture-creations-5sI6fQgYIuo.png"
|
174 |
-
mask_url = "https://raw.githubusercontent.com/CompVis/latent-diffusion/main/data/inpainting_examples/overture-creations-5sI6fQgYIuo_mask.png"
|
175 |
-
|
176 |
-
init_image = download_image(img_url).resize((512, 512))
|
177 |
-
mask_image = download_image(mask_url).resize((512, 512))
|
178 |
-
|
179 |
-
path = "runwayml/stable-diffusion-inpainting"
|
180 |
-
|
181 |
-
run_compile = True # Set True / False
|
182 |
-
|
183 |
-
pipe = StableDiffusionInpaintPipeline.from_pretrained(path, torch_dtype=torch.float16)
|
184 |
-
pipe = pipe.to("cuda")
|
185 |
-
pipe.unet.to(memory_format=torch.channels_last)
|
186 |
-
|
187 |
-
if run_compile:
|
188 |
-
print("Run torch compile")
|
189 |
-
pipe.unet = torch.compile(pipe.unet, mode="reduce-overhead", fullgraph=True)
|
190 |
-
|
191 |
-
prompt = "ghibli style, a fantasy landscape with castles"
|
192 |
-
|
193 |
-
for _ in range(3):
|
194 |
-
image = pipe(prompt=prompt, image=init_image, mask_image=mask_image).images[0]
|
195 |
-
```
|
196 |
-
|
197 |
-
#### ControlNet
|
198 |
-
|
199 |
-
```python
|
200 |
-
from diffusers import StableDiffusionControlNetPipeline, ControlNetModel
|
201 |
-
import requests
|
202 |
-
import torch
|
203 |
-
from PIL import Image
|
204 |
-
from io import BytesIO
|
205 |
-
|
206 |
-
url = "https://raw.githubusercontent.com/CompVis/stable-diffusion/main/assets/stable-samples/img2img/sketch-mountains-input.jpg"
|
207 |
-
|
208 |
-
response = requests.get(url)
|
209 |
-
init_image = Image.open(BytesIO(response.content)).convert("RGB")
|
210 |
-
init_image = init_image.resize((512, 512))
|
211 |
-
|
212 |
-
path = "runwayml/stable-diffusion-v1-5"
|
213 |
-
|
214 |
-
run_compile = True # Set True / False
|
215 |
-
controlnet = ControlNetModel.from_pretrained("lllyasviel/sd-controlnet-canny", torch_dtype=torch.float16)
|
216 |
-
pipe = StableDiffusionControlNetPipeline.from_pretrained(
|
217 |
-
path, controlnet=controlnet, torch_dtype=torch.float16
|
218 |
-
)
|
219 |
-
|
220 |
-
pipe = pipe.to("cuda")
|
221 |
-
pipe.unet.to(memory_format=torch.channels_last)
|
222 |
-
pipe.controlnet.to(memory_format=torch.channels_last)
|
223 |
-
|
224 |
-
if run_compile:
|
225 |
-
print("Run torch compile")
|
226 |
-
pipe.unet = torch.compile(pipe.unet, mode="reduce-overhead", fullgraph=True)
|
227 |
-
pipe.controlnet = torch.compile(pipe.controlnet, mode="reduce-overhead", fullgraph=True)
|
228 |
-
|
229 |
-
prompt = "ghibli style, a fantasy landscape with castles"
|
230 |
-
|
231 |
-
for _ in range(3):
|
232 |
-
image = pipe(prompt=prompt, image=init_image).images[0]
|
233 |
-
```
|
234 |
-
|
235 |
-
#### IF text-to-image + upscaling
|
236 |
-
|
237 |
-
```python
|
238 |
-
from diffusers import DiffusionPipeline
|
239 |
-
import torch
|
240 |
-
|
241 |
-
run_compile = True # Set True / False
|
242 |
-
|
243 |
-
pipe = DiffusionPipeline.from_pretrained("DeepFloyd/IF-I-M-v1.0", variant="fp16", text_encoder=None, torch_dtype=torch.float16)
|
244 |
-
pipe.to("cuda")
|
245 |
-
pipe_2 = DiffusionPipeline.from_pretrained("DeepFloyd/IF-II-M-v1.0", variant="fp16", text_encoder=None, torch_dtype=torch.float16)
|
246 |
-
pipe_2.to("cuda")
|
247 |
-
pipe_3 = DiffusionPipeline.from_pretrained("stabilityai/stable-diffusion-x4-upscaler", torch_dtype=torch.float16)
|
248 |
-
pipe_3.to("cuda")
|
249 |
-
|
250 |
-
|
251 |
-
pipe.unet.to(memory_format=torch.channels_last)
|
252 |
-
pipe_2.unet.to(memory_format=torch.channels_last)
|
253 |
-
pipe_3.unet.to(memory_format=torch.channels_last)
|
254 |
-
|
255 |
-
if run_compile:
|
256 |
-
pipe.unet = torch.compile(pipe.unet, mode="reduce-overhead", fullgraph=True)
|
257 |
-
pipe_2.unet = torch.compile(pipe_2.unet, mode="reduce-overhead", fullgraph=True)
|
258 |
-
pipe_3.unet = torch.compile(pipe_3.unet, mode="reduce-overhead", fullgraph=True)
|
259 |
-
|
260 |
-
prompt = "the blue hulk"
|
261 |
-
|
262 |
-
prompt_embeds = torch.randn((1, 2, 4096), dtype=torch.float16)
|
263 |
-
neg_prompt_embeds = torch.randn((1, 2, 4096), dtype=torch.float16)
|
264 |
-
|
265 |
-
for _ in range(3):
|
266 |
-
image = pipe(prompt_embeds=prompt_embeds, negative_prompt_embeds=neg_prompt_embeds, output_type="pt").images
|
267 |
-
image_2 = pipe_2(image=image, prompt_embeds=prompt_embeds, negative_prompt_embeds=neg_prompt_embeds, output_type="pt").images
|
268 |
-
image_3 = pipe_3(prompt=prompt, image=image, noise_level=100).images
|
269 |
-
```
|
270 |
-
|
271 |
-
To give you a pictorial overview of the possible speed-ups that can be obtained with PyTorch 2.0 and `torch.compile()`,
|
272 |
-
here is a plot that shows relative speed-ups for the [Stable Diffusion text-to-image pipeline](StableDiffusionPipeline) across five
|
273 |
-
different GPU families (with a batch size of 4):
|
274 |
-
|
275 |
-
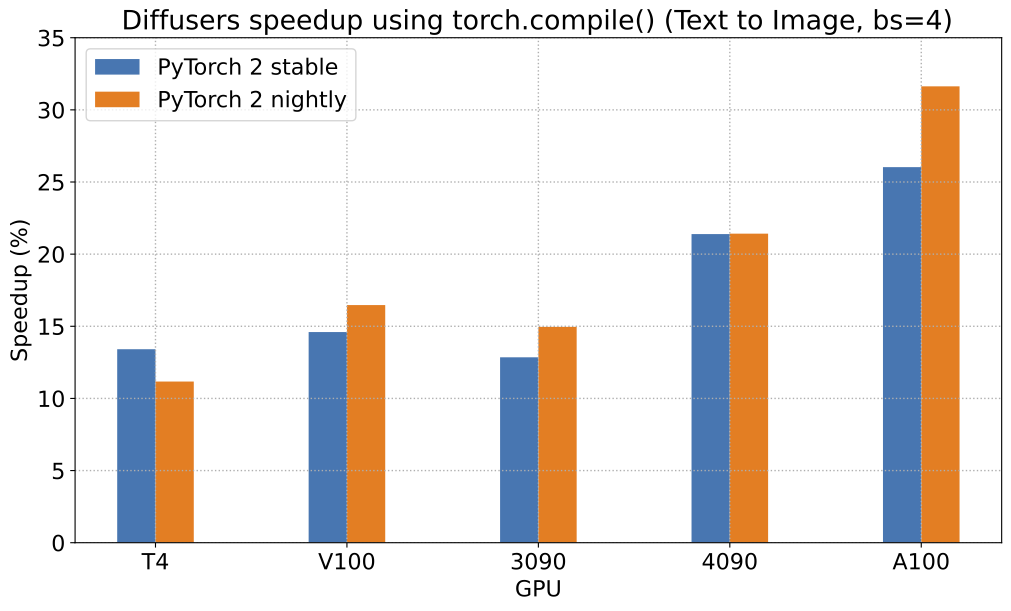
|
276 |
-
|
277 |
-
To give you an even better idea of how this speed-up holds for the other pipelines presented above, consider the following
|
278 |
-
plot that shows the benchmarking numbers from an A100 across three different batch sizes
|
279 |
-
(with PyTorch 2.0 nightly and `torch.compile()`):
|
280 |
-
|
281 |
-
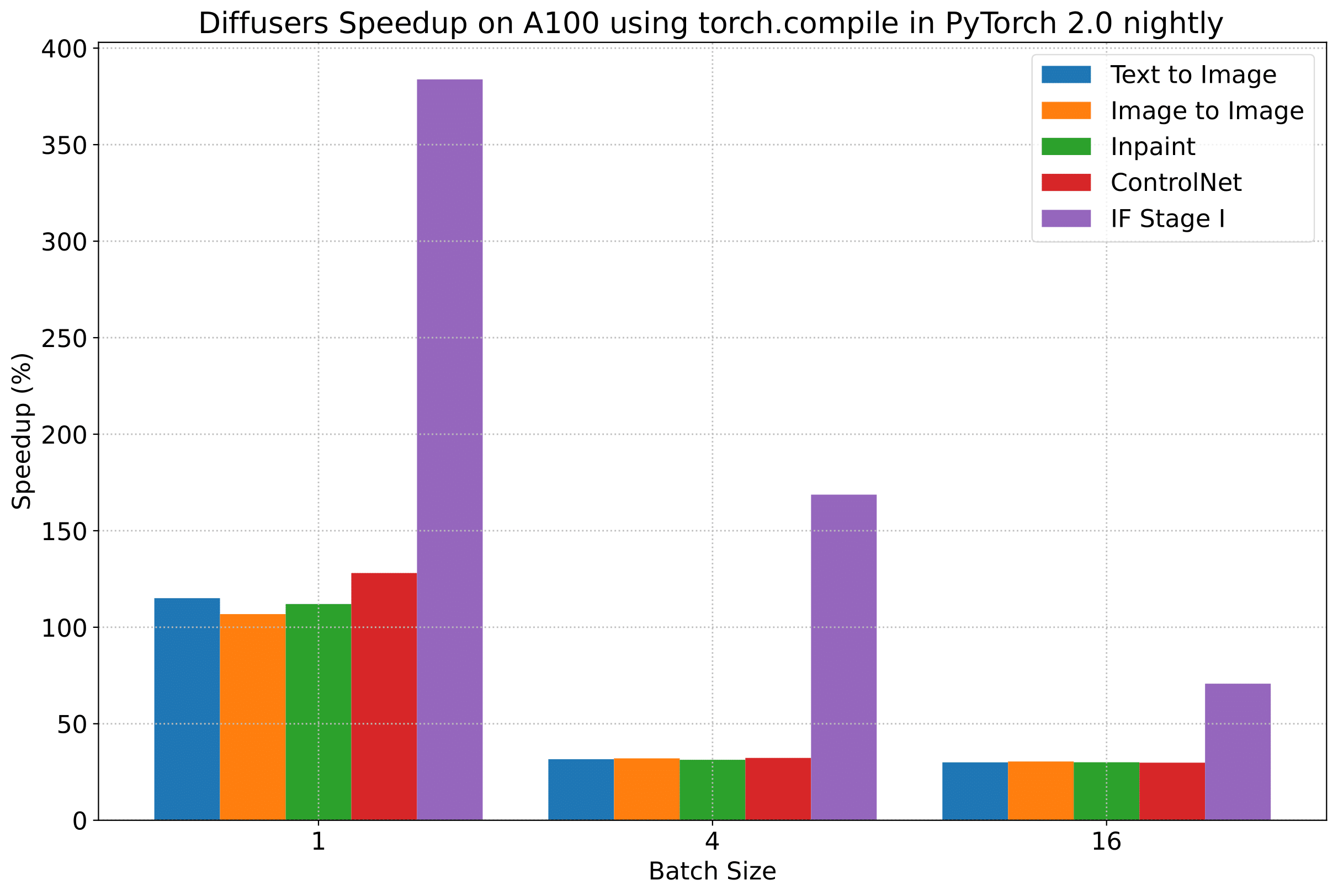
|
282 |
-
|
283 |
-
_(Our benchmarking metric for the plots above is **number of iterations/second**)_
|
284 |
-
|
285 |
-
But we reveal all the benchmarking numbers in the interest of transparency!
|
286 |
-
|
287 |
-
In the following tables, we report our findings in terms of the number of **_iterations processed per second_**.
|
288 |
-
|
289 |
-
### A100 (batch size: 1)
|
290 |
-
|
291 |
-
| **Pipeline** | **torch 2.0 - <br>no compile** | **torch nightly - <br>no compile** | **torch 2.0 - <br>compile** | **torch nightly - <br>compile** |
|
292 |
-
|:---:|:---:|:---:|:---:|:---:|
|
293 |
-
| SD - txt2img | 21.66 | 23.13 | 44.03 | 49.74 |
|
294 |
-
| SD - img2img | 21.81 | 22.40 | 43.92 | 46.32 |
|
295 |
-
| SD - inpaint | 22.24 | 23.23 | 43.76 | 49.25 |
|
296 |
-
| SD - controlnet | 15.02 | 15.82 | 32.13 | 36.08 |
|
297 |
-
| IF | 20.21 / <br>13.84 / <br>24.00 | 20.12 / <br>13.70 / <br>24.03 | ❌ | 97.34 / <br>27.23 / <br>111.66 |
|
298 |
-
|
299 |
-
### A100 (batch size: 4)
|
300 |
-
|
301 |
-
| **Pipeline** | **torch 2.0 - <br>no compile** | **torch nightly - <br>no compile** | **torch 2.0 - <br>compile** | **torch nightly - <br>compile** |
|
302 |
-
|:---:|:---:|:---:|:---:|:---:|
|
303 |
-
| SD - txt2img | 11.6 | 13.12 | 14.62 | 17.27 |
|
304 |
-
| SD - img2img | 11.47 | 13.06 | 14.66 | 17.25 |
|
305 |
-
| SD - inpaint | 11.67 | 13.31 | 14.88 | 17.48 |
|
306 |
-
| SD - controlnet | 8.28 | 9.38 | 10.51 | 12.41 |
|
307 |
-
| IF | 25.02 | 18.04 | ❌ | 48.47 |
|
308 |
-
|
309 |
-
### A100 (batch size: 16)
|
310 |
-
|
311 |
-
| **Pipeline** | **torch 2.0 - <br>no compile** | **torch nightly - <br>no compile** | **torch 2.0 - <br>compile** | **torch nightly - <br>compile** |
|
312 |
-
|:---:|:---:|:---:|:---:|:---:|
|
313 |
-
| SD - txt2img | 3.04 | 3.6 | 3.83 | 4.68 |
|
314 |
-
| SD - img2img | 2.98 | 3.58 | 3.83 | 4.67 |
|
315 |
-
| SD - inpaint | 3.04 | 3.66 | 3.9 | 4.76 |
|
316 |
-
| SD - controlnet | 2.15 | 2.58 | 2.74 | 3.35 |
|
317 |
-
| IF | 8.78 | 9.82 | ❌ | 16.77 |
|
318 |
-
|
319 |
-
### V100 (batch size: 1)
|
320 |
-
|
321 |
-
| **Pipeline** | **torch 2.0 - <br>no compile** | **torch nightly - <br>no compile** | **torch 2.0 - <br>compile** | **torch nightly - <br>compile** |
|
322 |
-
|:---:|:---:|:---:|:---:|:---:|
|
323 |
-
| SD - txt2img | 18.99 | 19.14 | 20.95 | 22.17 |
|
324 |
-
| SD - img2img | 18.56 | 19.18 | 20.95 | 22.11 |
|
325 |
-
| SD - inpaint | 19.14 | 19.06 | 21.08 | 22.20 |
|
326 |
-
| SD - controlnet | 13.48 | 13.93 | 15.18 | 15.88 |
|
327 |
-
| IF | 20.01 / <br>9.08 / <br>23.34 | 19.79 / <br>8.98 / <br>24.10 | ❌ | 55.75 / <br>11.57 / <br>57.67 |
|
328 |
-
|
329 |
-
### V100 (batch size: 4)
|
330 |
-
|
331 |
-
| **Pipeline** | **torch 2.0 - <br>no compile** | **torch nightly - <br>no compile** | **torch 2.0 - <br>compile** | **torch nightly - <br>compile** |
|
332 |
-
|:---:|:---:|:---:|:---:|:---:|
|
333 |
-
| SD - txt2img | 5.96 | 5.89 | 6.83 | 6.86 |
|
334 |
-
| SD - img2img | 5.90 | 5.91 | 6.81 | 6.82 |
|
335 |
-
| SD - inpaint | 5.99 | 6.03 | 6.93 | 6.95 |
|
336 |
-
| SD - controlnet | 4.26 | 4.29 | 4.92 | 4.93 |
|
337 |
-
| IF | 15.41 | 14.76 | ❌ | 22.95 |
|
338 |
-
|
339 |
-
### V100 (batch size: 16)
|
340 |
-
|
341 |
-
| **Pipeline** | **torch 2.0 - <br>no compile** | **torch nightly - <br>no compile** | **torch 2.0 - <br>compile** | **torch nightly - <br>compile** |
|
342 |
-
|:---:|:---:|:---:|:---:|:---:|
|
343 |
-
| SD - txt2img | 1.66 | 1.66 | 1.92 | 1.90 |
|
344 |
-
| SD - img2img | 1.65 | 1.65 | 1.91 | 1.89 |
|
345 |
-
| SD - inpaint | 1.69 | 1.69 | 1.95 | 1.93 |
|
346 |
-
| SD - controlnet | 1.19 | 1.19 | OOM after warmup | 1.36 |
|
347 |
-
| IF | 5.43 | 5.29 | ❌ | 7.06 |
|
348 |
-
|
349 |
-
### T4 (batch size: 1)
|
350 |
-
|
351 |
-
| **Pipeline** | **torch 2.0 - <br>no compile** | **torch nightly - <br>no compile** | **torch 2.0 - <br>compile** | **torch nightly - <br>compile** |
|
352 |
-
|:---:|:---:|:---:|:---:|:---:|
|
353 |
-
| SD - txt2img | 6.9 | 6.95 | 7.3 | 7.56 |
|
354 |
-
| SD - img2img | 6.84 | 6.99 | 7.04 | 7.55 |
|
355 |
-
| SD - inpaint | 6.91 | 6.7 | 7.01 | 7.37 |
|
356 |
-
| SD - controlnet | 4.89 | 4.86 | 5.35 | 5.48 |
|
357 |
-
| IF | 17.42 / <br>2.47 / <br>18.52 | 16.96 / <br>2.45 / <br>18.69 | ❌ | 24.63 / <br>2.47 / <br>23.39 |
|
358 |
-
|
359 |
-
### T4 (batch size: 4)
|
360 |
-
|
361 |
-
| **Pipeline** | **torch 2.0 - <br>no compile** | **torch nightly - <br>no compile** | **torch 2.0 - <br>compile** | **torch nightly - <br>compile** |
|
362 |
-
|:---:|:---:|:---:|:---:|:---:|
|
363 |
-
| SD - txt2img | 1.79 | 1.79 | 2.03 | 1.99 |
|
364 |
-
| SD - img2img | 1.77 | 1.77 | 2.05 | 2.04 |
|
365 |
-
| SD - inpaint | 1.81 | 1.82 | 2.09 | 2.09 |
|
366 |
-
| SD - controlnet | 1.34 | 1.27 | 1.47 | 1.46 |
|
367 |
-
| IF | 5.79 | 5.61 | ❌ | 7.39 |
|
368 |
-
|
369 |
-
### T4 (batch size: 16)
|
370 |
-
|
371 |
-
| **Pipeline** | **torch 2.0 - <br>no compile** | **torch nightly - <br>no compile** | **torch 2.0 - <br>compile** | **torch nightly - <br>compile** |
|
372 |
-
|:---:|:---:|:---:|:---:|:---:|
|
373 |
-
| SD - txt2img | 2.34s | 2.30s | OOM after 2nd iteration | 1.99s |
|
374 |
-
| SD - img2img | 2.35s | 2.31s | OOM after warmup | 2.00s |
|
375 |
-
| SD - inpaint | 2.30s | 2.26s | OOM after 2nd iteration | 1.95s |
|
376 |
-
| SD - controlnet | OOM after 2nd iteration | OOM after 2nd iteration | OOM after warmup | OOM after warmup |
|
377 |
-
| IF * | 1.44 | 1.44 | ❌ | 1.94 |
|
378 |
-
|
379 |
-
### RTX 3090 (batch size: 1)
|
380 |
-
|
381 |
-
| **Pipeline** | **torch 2.0 - <br>no compile** | **torch nightly - <br>no compile** | **torch 2.0 - <br>compile** | **torch nightly - <br>compile** |
|
382 |
-
|:---:|:---:|:---:|:---:|:---:|
|
383 |
-
| SD - txt2img | 22.56 | 22.84 | 23.84 | 25.69 |
|
384 |
-
| SD - img2img | 22.25 | 22.61 | 24.1 | 25.83 |
|
385 |
-
| SD - inpaint | 22.22 | 22.54 | 24.26 | 26.02 |
|
386 |
-
| SD - controlnet | 16.03 | 16.33 | 17.38 | 18.56 |
|
387 |
-
| IF | 27.08 / <br>9.07 / <br>31.23 | 26.75 / <br>8.92 / <br>31.47 | ❌ | 68.08 / <br>11.16 / <br>65.29 |
|
388 |
-
|
389 |
-
### RTX 3090 (batch size: 4)
|
390 |
-
|
391 |
-
| **Pipeline** | **torch 2.0 - <br>no compile** | **torch nightly - <br>no compile** | **torch 2.0 - <br>compile** | **torch nightly - <br>compile** |
|
392 |
-
|:---:|:---:|:---:|:---:|:---:|
|
393 |
-
| SD - txt2img | 6.46 | 6.35 | 7.29 | 7.3 |
|
394 |
-
| SD - img2img | 6.33 | 6.27 | 7.31 | 7.26 |
|
395 |
-
| SD - inpaint | 6.47 | 6.4 | 7.44 | 7.39 |
|
396 |
-
| SD - controlnet | 4.59 | 4.54 | 5.27 | 5.26 |
|
397 |
-
| IF | 16.81 | 16.62 | ❌ | 21.57 |
|
398 |
-
|
399 |
-
### RTX 3090 (batch size: 16)
|
400 |
-
|
401 |
-
| **Pipeline** | **torch 2.0 - <br>no compile** | **torch nightly - <br>no compile** | **torch 2.0 - <br>compile** | **torch nightly - <br>compile** |
|
402 |
-
|:---:|:---:|:---:|:---:|:---:|
|
403 |
-
| SD - txt2img | 1.7 | 1.69 | 1.93 | 1.91 |
|
404 |
-
| SD - img2img | 1.68 | 1.67 | 1.93 | 1.9 |
|
405 |
-
| SD - inpaint | 1.72 | 1.71 | 1.97 | 1.94 |
|
406 |
-
| SD - controlnet | 1.23 | 1.22 | 1.4 | 1.38 |
|
407 |
-
| IF | 5.01 | 5.00 | ❌ | 6.33 |
|
408 |
-
|
409 |
-
### RTX 4090 (batch size: 1)
|
410 |
-
|
411 |
-
| **Pipeline** | **torch 2.0 - <br>no compile** | **torch nightly - <br>no compile** | **torch 2.0 - <br>compile** | **torch nightly - <br>compile** |
|
412 |
-
|:---:|:---:|:---:|:---:|:---:|
|
413 |
-
| SD - txt2img | 40.5 | 41.89 | 44.65 | 49.81 |
|
414 |
-
| SD - img2img | 40.39 | 41.95 | 44.46 | 49.8 |
|
415 |
-
| SD - inpaint | 40.51 | 41.88 | 44.58 | 49.72 |
|
416 |
-
| SD - controlnet | 29.27 | 30.29 | 32.26 | 36.03 |
|
417 |
-
| IF | 69.71 / <br>18.78 / <br>85.49 | 69.13 / <br>18.80 / <br>85.56 | ❌ | 124.60 / <br>26.37 / <br>138.79 |
|
418 |
-
|
419 |
-
### RTX 4090 (batch size: 4)
|
420 |
-
|
421 |
-
| **Pipeline** | **torch 2.0 - <br>no compile** | **torch nightly - <br>no compile** | **torch 2.0 - <br>compile** | **torch nightly - <br>compile** |
|
422 |
-
|:---:|:---:|:---:|:---:|:---:|
|
423 |
-
| SD - txt2img | 12.62 | 12.84 | 15.32 | 15.59 |
|
424 |
-
| SD - img2img | 12.61 | 12,.79 | 15.35 | 15.66 |
|
425 |
-
| SD - inpaint | 12.65 | 12.81 | 15.3 | 15.58 |
|
426 |
-
| SD - controlnet | 9.1 | 9.25 | 11.03 | 11.22 |
|
427 |
-
| IF | 31.88 | 31.14 | ❌ | 43.92 |
|
428 |
-
|
429 |
-
### RTX 4090 (batch size: 16)
|
430 |
-
|
431 |
-
| **Pipeline** | **torch 2.0 - <br>no compile** | **torch nightly - <br>no compile** | **torch 2.0 - <br>compile** | **torch nightly - <br>compile** |
|
432 |
-
|:---:|:---:|:---:|:---:|:---:|
|
433 |
-
| SD - txt2img | 3.17 | 3.2 | 3.84 | 3.85 |
|
434 |
-
| SD - img2img | 3.16 | 3.2 | 3.84 | 3.85 |
|
435 |
-
| SD - inpaint | 3.17 | 3.2 | 3.85 | 3.85 |
|
436 |
-
| SD - controlnet | 2.23 | 2.3 | 2.7 | 2.75 |
|
437 |
-
| IF | 9.26 | 9.2 | ❌ | 13.31 |
|
438 |
-
|
439 |
-
## Notes
|
440 |
-
|
441 |
-
* Follow [this PR](https://github.com/huggingface/diffusers/pull/3313) for more details on the environment used for conducting the benchmarks.
|
442 |
-
* For the IF pipeline and batch sizes > 1, we only used a batch size of >1 in the first IF pipeline for text-to-image generation and NOT for upscaling. So, that means the two upscaling pipelines received a batch size of 1.
|
443 |
-
|
444 |
-
*Thanks to [Horace He](https://github.com/Chillee) from the PyTorch team for their support in improving our support of `torch.compile()` in Diffusers.*
|
|
|
|
|
|
|
|
|
|
|
|
|
|
|
|
|
|
|
|
|
|
|
|
|
|
|
|
|
|
|
|
|
|
|
|
|
|
|
|
|
|
|
|
|
|
|
|
|
|
|
|
|
|
|
|
|
|
|
|
|
|
|
|
|
|
|
|
|
|
|
|
|
|
|
|
|
|
|
|
|
|
|
|
|
|
|
|
|
|
|
|
|
|
|
|
|
|
|
|
|
|
|
|
|
|
|
|
|
|
|
|
|
|
|
|
|
|
|
|
|
|
|
|
|
|
|
|
|
|
|
|
|
|
|
|
|
|
|
|
|
|
|
|
|
|
|
|
|
|
|
|
|
|
|
|
|
|
|
|
|
|
|
|
|
|
|
|
|
|
|
|
|
|
|
|
|
|
|
|
|
|
|
|
|
|
|
|
|
|
|
|
|
|
|
|
|
|
|
|
|
|
|
|
|
|
|
|
|
|
|
|
|
|
|
|
|
|
|
|
|
|
|
|
|
|
|
|
|
|
|
|
|
|
|
|
|
|
|
|
|
|
|
|
|
|
|
|
|
|
|
|
|
|
|
|
|
|
|
|
|
|
|
|
|
|
|
|
|
|
|
|
|
|
|
|
|
|
|
|
|
|
|
|
|
|
|
|
|
|
|
|
|
|
|
|
|
|
|
|
|
|
|
|
|
|
|
|
|
|
|
|
|
|
|
|
|
|
|
|
|
|
|
|
|
|
|
|
|
|
|
|
|
|
|
|
|
|
|
|
|
|
|
|
|
|
|
|
|
|
|
|
|
|
|
|
|
|
|
|
|
|
|
|
|
|
|
|
|
|
|
|
|
|
|
|
|
|
|
|
|
|
|
|
|
|
|
|
|
|
|
|
|
|
|
|
|
|
|
|
|
|
|
|
|
|
|
|
|
|
|
|
|
|
|
|
|
|
|
|
|
|
|
|
|
|
|
|
|
|
|
|
|
|
|
|
|
|
|
|
|
|
|
|
|
|
|
|
|
|
|
|
|
|
|
|
|
|
|
|
|
|
|
|
|
|
|
|
|
|
|
|
|
|
|
|
|
|
|
|
|
|
|
|
|
|
|
|
|
|
|
|
|
|
|
|
|
|
|
|
|
|
|
|
|
|
|
|
|
|
|
|
|
|
|
|
|
|
|
|
|
|
|
|
|
|
|
|
|
|
|
|
|
|
|
|
|
|
|
|
|
|
|
|
|
|
|
|
|
|
|
|
|
|
|
|
|
|
|
|
|
|
|
|
|
|
|
|
|
|
|
|
|
|
|
|
|
|
|
|
|
|
|
|
|
|
|
|
|
|
|
|
|
|
|
|
|
|
|
|
|
|
|
|
|
|
|
|
|
|
|
|
|
|
|
|
|
|
|
|
|
|
|
|
|
|
|
|
|
|
|
|
|
|
|
|
|
|
|
|
|
|
|
|
|
|
|
|
|
|
|
|
|
|
|
|
|
|
|
|
|
|
|
|
|
|
|
|
|
|
|
|
|
|
|
|
|
|
|
|
|
|
|
|
|
|
|
|
|
|
|
|
|
|
|
|
|
|
|
|
|
|
|
|
|
|
|
|
|
|
|
|
|
|
|
|
|
|
|
|
|
|
|
|
|
|
|
|
|
|
|
|
|
|
|
|
|
|
|
|
|
|
|
|
|
|
|
|
|
|
|
|
|
|
|
|
|
|
|
|
|
|
|
|
|
|
|
|
|
|
|
|
|
|
|
|
|
|
|
|
|
|
|
|
|
|
|
|
|
|
|
|
|
|
|
|
|
|
|
|
|
|
|
|
|
|
|
|
|
|
|
|
|
|
|
|
|
|
|
|
|
|
|
|
|
|
|
|
|
|
|
|
|
|
|
|
|
|
|
|
|
|
|
|
|
|
|
|
|
|
|
|
|
|
|
|
|
|
|
|
|
|
|
|
|
|
|
|
|
|
|
|
|
|
|
|
|
|
|
|
|
|
|
|
|
|
|
|
|
|
spaces/Androidonnxfork/CivitAi-to-Diffusers/diffusers/tests/pipelines/stable_diffusion_xl/test_stable_diffusion_xl_img2img.py
DELETED
@@ -1,317 +0,0 @@
|
|
1 |
-
# coding=utf-8
|
2 |
-
# Copyright 2023 HuggingFace Inc.
|
3 |
-
#
|
4 |
-
# Licensed under the Apache License, Version 2.0 (the "License");
|
5 |
-
# you may not use this file except in compliance with the License.
|
6 |
-
# You may obtain a copy of the License at
|
7 |
-
#
|
8 |
-
# http://www.apache.org/licenses/LICENSE-2.0
|
9 |
-
#
|
10 |
-
# Unless required by applicable law or agreed to in writing, software
|
11 |
-
# distributed under the License is distributed on an "AS IS" BASIS,
|
12 |
-
# WITHOUT WARRANTIES OR CONDITIONS OF ANY KIND, either express or implied.
|
13 |
-
# See the License for the specific language governing permissions and
|
14 |
-
# limitations under the License.
|
15 |
-
|
16 |
-
import random
|
17 |
-
import unittest
|
18 |
-
|
19 |
-
import numpy as np
|
20 |
-
import torch
|
21 |
-
from transformers import CLIPTextConfig, CLIPTextModel, CLIPTextModelWithProjection, CLIPTokenizer
|
22 |
-
|
23 |
-
from diffusers import (
|
24 |
-
AutoencoderKL,
|
25 |
-
EulerDiscreteScheduler,
|
26 |
-
StableDiffusionXLImg2ImgPipeline,
|
27 |
-
UNet2DConditionModel,
|
28 |
-
)
|
29 |
-
from diffusers.utils import floats_tensor, torch_device
|
30 |
-
from diffusers.utils.testing_utils import enable_full_determinism, require_torch_gpu
|
31 |
-
|
32 |
-
from ..pipeline_params import (
|
33 |
-
IMAGE_TO_IMAGE_IMAGE_PARAMS,
|
34 |
-
TEXT_GUIDED_IMAGE_VARIATION_BATCH_PARAMS,
|
35 |
-
TEXT_GUIDED_IMAGE_VARIATION_PARAMS,
|
36 |
-
)
|
37 |
-
from ..test_pipelines_common import PipelineLatentTesterMixin, PipelineTesterMixin
|
38 |
-
|
39 |
-
|
40 |
-
enable_full_determinism()
|
41 |
-
|
42 |
-
|
43 |
-
class StableDiffusionXLImg2ImgPipelineFastTests(PipelineLatentTesterMixin, PipelineTesterMixin, unittest.TestCase):
|
44 |
-
pipeline_class = StableDiffusionXLImg2ImgPipeline
|
45 |
-
params = TEXT_GUIDED_IMAGE_VARIATION_PARAMS - {"height", "width"}
|
46 |
-
required_optional_params = PipelineTesterMixin.required_optional_params - {"latents"}
|
47 |
-
batch_params = TEXT_GUIDED_IMAGE_VARIATION_BATCH_PARAMS
|
48 |
-
image_params = IMAGE_TO_IMAGE_IMAGE_PARAMS
|
49 |
-
image_latents_params = IMAGE_TO_IMAGE_IMAGE_PARAMS
|
50 |
-
|
51 |
-
def get_dummy_components(self, skip_first_text_encoder=False):
|
52 |
-
torch.manual_seed(0)
|
53 |
-
unet = UNet2DConditionModel(
|
54 |
-
block_out_channels=(32, 64),
|
55 |
-
layers_per_block=2,
|
56 |
-
sample_size=32,
|
57 |
-
in_channels=4,
|
58 |
-
out_channels=4,
|
59 |
-
down_block_types=("DownBlock2D", "CrossAttnDownBlock2D"),
|
60 |
-
up_block_types=("CrossAttnUpBlock2D", "UpBlock2D"),
|
61 |
-
# SD2-specific config below
|
62 |
-
attention_head_dim=(2, 4),
|
63 |
-
use_linear_projection=True,
|
64 |
-
addition_embed_type="text_time",
|
65 |
-
addition_time_embed_dim=8,
|
66 |
-
transformer_layers_per_block=(1, 2),
|
67 |
-
projection_class_embeddings_input_dim=72, # 5 * 8 + 32
|
68 |
-
cross_attention_dim=64 if not skip_first_text_encoder else 32,
|
69 |
-
)
|
70 |
-
scheduler = EulerDiscreteScheduler(
|
71 |
-
beta_start=0.00085,
|
72 |
-
beta_end=0.012,
|
73 |
-
steps_offset=1,
|
74 |
-
beta_schedule="scaled_linear",
|
75 |
-
timestep_spacing="leading",
|
76 |
-
)
|
77 |
-
torch.manual_seed(0)
|
78 |
-
vae = AutoencoderKL(
|
79 |
-
block_out_channels=[32, 64],
|
80 |
-
in_channels=3,
|
81 |
-
out_channels=3,
|
82 |
-
down_block_types=["DownEncoderBlock2D", "DownEncoderBlock2D"],
|
83 |
-
up_block_types=["UpDecoderBlock2D", "UpDecoderBlock2D"],
|
84 |
-
latent_channels=4,
|
85 |
-
sample_size=128,
|
86 |
-
)
|
87 |
-
torch.manual_seed(0)
|
88 |
-
text_encoder_config = CLIPTextConfig(
|
89 |
-
bos_token_id=0,
|
90 |
-
eos_token_id=2,
|
91 |
-
hidden_size=32,
|
92 |
-
intermediate_size=37,
|
93 |
-
layer_norm_eps=1e-05,
|
94 |
-
num_attention_heads=4,
|
95 |
-
num_hidden_layers=5,
|
96 |
-
pad_token_id=1,
|
97 |
-
vocab_size=1000,
|
98 |
-
# SD2-specific config below
|
99 |
-
hidden_act="gelu",
|
100 |
-
projection_dim=32,
|
101 |
-
)
|
102 |
-
text_encoder = CLIPTextModel(text_encoder_config)
|
103 |
-
tokenizer = CLIPTokenizer.from_pretrained("hf-internal-testing/tiny-random-clip")
|
104 |
-
|
105 |
-
text_encoder_2 = CLIPTextModelWithProjection(text_encoder_config)
|
106 |
-
tokenizer_2 = CLIPTokenizer.from_pretrained("hf-internal-testing/tiny-random-clip")
|
107 |
-
|
108 |
-
components = {
|
109 |
-
"unet": unet,
|
110 |
-
"scheduler": scheduler,
|
111 |
-
"vae": vae,
|
112 |
-
"text_encoder": text_encoder if not skip_first_text_encoder else None,
|
113 |
-
"tokenizer": tokenizer if not skip_first_text_encoder else None,
|
114 |
-
"text_encoder_2": text_encoder_2,
|
115 |
-
"tokenizer_2": tokenizer_2,
|
116 |
-
"requires_aesthetics_score": True,
|
117 |
-
}
|
118 |
-
return components
|
119 |
-
|
120 |
-
def test_components_function(self):
|
121 |
-
init_components = self.get_dummy_components()
|
122 |
-
init_components.pop("requires_aesthetics_score")
|
123 |
-
pipe = self.pipeline_class(**init_components)
|
124 |
-
|
125 |
-
self.assertTrue(hasattr(pipe, "components"))
|
126 |
-
self.assertTrue(set(pipe.components.keys()) == set(init_components.keys()))
|
127 |
-
|
128 |
-
def get_dummy_inputs(self, device, seed=0):
|
129 |
-
image = floats_tensor((1, 3, 32, 32), rng=random.Random(seed)).to(device)
|
130 |
-
image = image / 2 + 0.5
|
131 |
-
if str(device).startswith("mps"):
|
132 |
-
generator = torch.manual_seed(seed)
|
133 |
-
else:
|
134 |
-
generator = torch.Generator(device=device).manual_seed(seed)
|
135 |
-
inputs = {
|
136 |
-
"prompt": "A painting of a squirrel eating a burger",
|
137 |
-
"image": image,
|
138 |
-
"generator": generator,
|
139 |
-
"num_inference_steps": 2,
|
140 |
-
"guidance_scale": 5.0,
|
141 |
-
"output_type": "numpy",
|
142 |
-
"strength": 0.8,
|
143 |
-
}
|
144 |
-
return inputs
|
145 |
-
|
146 |
-
def test_stable_diffusion_xl_img2img_euler(self):
|
147 |
-
device = "cpu" # ensure determinism for the device-dependent torch.Generator
|
148 |
-
components = self.get_dummy_components()
|
149 |
-
sd_pipe = StableDiffusionXLImg2ImgPipeline(**components)
|
150 |
-
sd_pipe = sd_pipe.to(device)
|
151 |
-
sd_pipe.set_progress_bar_config(disable=None)
|
152 |
-
|
153 |
-
inputs = self.get_dummy_inputs(device)
|
154 |
-
image = sd_pipe(**inputs).images
|
155 |
-
image_slice = image[0, -3:, -3:, -1]
|
156 |
-
|
157 |
-
assert image.shape == (1, 32, 32, 3)
|
158 |
-
|
159 |
-
expected_slice = np.array([0.4664, 0.4886, 0.4403, 0.6902, 0.5592, 0.4534, 0.5931, 0.5951, 0.5224])
|
160 |
-
|
161 |
-
assert np.abs(image_slice.flatten() - expected_slice).max() < 1e-2
|
162 |
-
|
163 |
-
def test_stable_diffusion_xl_refiner(self):
|
164 |
-
device = "cpu" # ensure determinism for the device-dependent torch.Generator
|
165 |
-
components = self.get_dummy_components(skip_first_text_encoder=True)
|
166 |
-
|
167 |
-
sd_pipe = StableDiffusionXLImg2ImgPipeline(**components)
|
168 |
-
sd_pipe = sd_pipe.to(device)
|
169 |
-
sd_pipe.set_progress_bar_config(disable=None)
|
170 |
-
|
171 |
-
inputs = self.get_dummy_inputs(device)
|
172 |
-
image = sd_pipe(**inputs).images
|
173 |
-
image_slice = image[0, -3:, -3:, -1]
|
174 |
-
|
175 |
-
assert image.shape == (1, 32, 32, 3)
|
176 |
-
|
177 |
-
expected_slice = np.array([0.4578, 0.4981, 0.4301, 0.6454, 0.5588, 0.4442, 0.5678, 0.5940, 0.5176])
|
178 |
-
|
179 |
-
assert np.abs(image_slice.flatten() - expected_slice).max() < 1e-2
|
180 |
-
|
181 |
-
def test_attention_slicing_forward_pass(self):
|
182 |
-
super().test_attention_slicing_forward_pass(expected_max_diff=3e-3)
|
183 |
-
|
184 |
-
def test_inference_batch_single_identical(self):
|
185 |
-
super().test_inference_batch_single_identical(expected_max_diff=3e-3)
|
186 |
-
|
187 |
-
# TODO(Patrick, Sayak) - skip for now as this requires more refiner tests
|
188 |
-
def test_save_load_optional_components(self):
|
189 |
-
pass
|
190 |
-
|
191 |
-
def test_stable_diffusion_xl_img2img_negative_prompt_embeds(self):
|
192 |
-
components = self.get_dummy_components()
|
193 |
-
sd_pipe = StableDiffusionXLImg2ImgPipeline(**components)
|
194 |
-
sd_pipe = sd_pipe.to(torch_device)
|
195 |
-
sd_pipe = sd_pipe.to(torch_device)
|
196 |
-
sd_pipe.set_progress_bar_config(disable=None)
|
197 |
-
|
198 |
-
# forward without prompt embeds
|
199 |
-
inputs = self.get_dummy_inputs(torch_device)
|
200 |
-
negative_prompt = 3 * ["this is a negative prompt"]
|
201 |
-
inputs["negative_prompt"] = negative_prompt
|
202 |
-
inputs["prompt"] = 3 * [inputs["prompt"]]
|
203 |
-
|
204 |
-
output = sd_pipe(**inputs)
|
205 |
-
image_slice_1 = output.images[0, -3:, -3:, -1]
|
206 |
-
|
207 |
-
# forward with prompt embeds
|
208 |
-
inputs = self.get_dummy_inputs(torch_device)
|
209 |
-
negative_prompt = 3 * ["this is a negative prompt"]
|
210 |
-
prompt = 3 * [inputs.pop("prompt")]
|
211 |
-
|
212 |
-
(
|
213 |
-
prompt_embeds,
|
214 |
-
negative_prompt_embeds,
|
215 |
-
pooled_prompt_embeds,
|
216 |
-
negative_pooled_prompt_embeds,
|
217 |
-
) = sd_pipe.encode_prompt(prompt, negative_prompt=negative_prompt)
|
218 |
-
|
219 |
-
output = sd_pipe(
|
220 |
-
**inputs,
|
221 |
-
prompt_embeds=prompt_embeds,
|
222 |
-
negative_prompt_embeds=negative_prompt_embeds,
|
223 |
-
pooled_prompt_embeds=pooled_prompt_embeds,
|
224 |
-
negative_pooled_prompt_embeds=negative_pooled_prompt_embeds,
|
225 |
-
)
|
226 |
-
image_slice_2 = output.images[0, -3:, -3:, -1]
|
227 |
-
|
228 |
-
# make sure that it's equal
|
229 |
-
assert np.abs(image_slice_1.flatten() - image_slice_2.flatten()).max() < 1e-4
|
230 |
-
|
231 |
-
@require_torch_gpu
|
232 |
-
def test_stable_diffusion_xl_offloads(self):
|
233 |
-
pipes = []
|
234 |
-
components = self.get_dummy_components()
|
235 |
-
sd_pipe = StableDiffusionXLImg2ImgPipeline(**components).to(torch_device)
|
236 |
-
pipes.append(sd_pipe)
|
237 |
-
|
238 |
-
components = self.get_dummy_components()
|
239 |
-
sd_pipe = StableDiffusionXLImg2ImgPipeline(**components)
|
240 |
-
sd_pipe.enable_model_cpu_offload()
|
241 |
-
pipes.append(sd_pipe)
|
242 |
-
|
243 |
-
components = self.get_dummy_components()
|
244 |
-
sd_pipe = StableDiffusionXLImg2ImgPipeline(**components)
|
245 |
-
sd_pipe.enable_sequential_cpu_offload()
|
246 |
-
pipes.append(sd_pipe)
|
247 |
-
|
248 |
-
image_slices = []
|
249 |
-
for pipe in pipes:
|
250 |
-
pipe.unet.set_default_attn_processor()
|
251 |
-
|
252 |
-
inputs = self.get_dummy_inputs(torch_device)
|
253 |
-
image = pipe(**inputs).images
|
254 |
-
|
255 |
-
image_slices.append(image[0, -3:, -3:, -1].flatten())
|
256 |
-
|
257 |
-
assert np.abs(image_slices[0] - image_slices[1]).max() < 1e-3
|
258 |
-
assert np.abs(image_slices[0] - image_slices[2]).max() < 1e-3
|
259 |
-
|
260 |
-
def test_stable_diffusion_xl_multi_prompts(self):
|
261 |
-
components = self.get_dummy_components()
|
262 |
-
sd_pipe = self.pipeline_class(**components).to(torch_device)
|
263 |
-
|
264 |
-
# forward with single prompt
|
265 |
-
inputs = self.get_dummy_inputs(torch_device)
|
266 |
-
inputs["num_inference_steps"] = 5
|
267 |
-
output = sd_pipe(**inputs)
|
268 |
-
image_slice_1 = output.images[0, -3:, -3:, -1]
|
269 |
-
|
270 |
-
# forward with same prompt duplicated
|
271 |
-
inputs = self.get_dummy_inputs(torch_device)
|
272 |
-
inputs["num_inference_steps"] = 5
|
273 |
-
inputs["prompt_2"] = inputs["prompt"]
|
274 |
-
output = sd_pipe(**inputs)
|
275 |
-
image_slice_2 = output.images[0, -3:, -3:, -1]
|
276 |
-
|
277 |
-
# ensure the results are equal
|
278 |
-
assert np.abs(image_slice_1.flatten() - image_slice_2.flatten()).max() < 1e-4
|
279 |
-
|
280 |
-
# forward with different prompt
|
281 |
-
inputs = self.get_dummy_inputs(torch_device)
|
282 |
-
inputs["num_inference_steps"] = 5
|
283 |
-
inputs["prompt_2"] = "different prompt"
|
284 |
-
output = sd_pipe(**inputs)
|
285 |
-
image_slice_3 = output.images[0, -3:, -3:, -1]
|
286 |
-
|
287 |
-
# ensure the results are not equal
|
288 |
-
assert np.abs(image_slice_1.flatten() - image_slice_3.flatten()).max() > 1e-4
|
289 |
-
|
290 |
-
# manually set a negative_prompt
|
291 |
-
inputs = self.get_dummy_inputs(torch_device)
|
292 |
-
inputs["num_inference_steps"] = 5
|
293 |
-
inputs["negative_prompt"] = "negative prompt"
|
294 |
-
output = sd_pipe(**inputs)
|
295 |
-
image_slice_1 = output.images[0, -3:, -3:, -1]
|
296 |
-
|
297 |
-
# forward with same negative_prompt duplicated
|
298 |
-
inputs = self.get_dummy_inputs(torch_device)
|
299 |
-
inputs["num_inference_steps"] = 5
|
300 |
-
inputs["negative_prompt"] = "negative prompt"
|
301 |
-
inputs["negative_prompt_2"] = inputs["negative_prompt"]
|
302 |
-
output = sd_pipe(**inputs)
|
303 |
-
image_slice_2 = output.images[0, -3:, -3:, -1]
|
304 |
-
|
305 |
-
# ensure the results are equal
|
306 |
-
assert np.abs(image_slice_1.flatten() - image_slice_2.flatten()).max() < 1e-4
|
307 |
-
|
308 |
-
# forward with different negative_prompt
|
309 |
-
inputs = self.get_dummy_inputs(torch_device)
|
310 |
-
inputs["num_inference_steps"] = 5
|
311 |
-
inputs["negative_prompt"] = "negative prompt"
|
312 |
-
inputs["negative_prompt_2"] = "different negative prompt"
|
313 |
-
output = sd_pipe(**inputs)
|
314 |
-
image_slice_3 = output.images[0, -3:, -3:, -1]
|
315 |
-
|
316 |
-
# ensure the results are not equal
|
317 |
-
assert np.abs(image_slice_1.flatten() - image_slice_3.flatten()).max() > 1e-4
|
|
|
|
|
|
|
|
|
|
|
|
|
|
|
|
|
|
|
|
|
|
|
|
|
|
|
|
|
|
|
|
|
|
|
|
|
|
|
|
|
|
|
|
|
|
|
|
|
|
|
|
|
|
|
|
|
|
|
|
|
|
|
|
|
|
|
|
|
|
|
|
|
|
|
|
|
|
|
|
|
|
|
|
|
|
|
|
|
|
|
|
|
|
|
|
|
|
|
|
|
|
|
|
|
|
|
|
|
|
|
|
|
|
|
|
|
|
|
|
|
|
|
|
|
|
|
|
|
|
|
|
|
|
|
|
|
|
|
|
|
|
|
|
|
|
|
|
|
|
|
|
|
|
|
|
|
|
|
|
|
|
|
|
|
|
|
|
|
|
|
|
|
|
|
|
|
|
|
|
|
|
|
|
|
|
|
|
|
|
|
|
|
|
|
|
|
|
|
|
|
|
|
|
|
|
|
|
|
|
|
|
|
|
|
|
|
|
|
|
|
|
|
|
|
|
|
|
|
|
|
|
|
|
|
|
|
|
|
|
|
|
|
|
|
|
|
|
|
|
|
|
|
|
|
|
|
|
|
|
|
|
|
|
|
|
|
|
|
|
|
|
|
|
|
|
|
|
|
|
|
|
|
|
|
|
|
|
|
|
|
|
|
|
|
|
|
|
|
|
|
|
|
|
|
|
|
|
|
|
|
|
|
|
|
|
|
|
|
|
|
|
|
|
|
|
|
|
|
|
|
|
|
|
|
|
|
|
|
|
|
|
|
|
|
|
|
|
|
|
|
|
|
|
|
|
|
|
|
|
|
|
|
|
|
|
|
|
|
|
|
|
|
|
|
|
|
|
|
|
|
|
|
|
|
|
|
|
|
|
|
|
|
|
|
|
|
|
|
|
|
|
|
|
|
|
|
|
|
|
|
|
|
|
|
|
|
|
|
|
|
|
|
|
|
|
|
|
|
|
|
|
|
|
|
|
|
|
|
|
|
|
|
|
|
|
|
|
|
|
|
|
|
|
|
|
|
|
|
|
|
|
|
|
|
|
|
|
|
|
|
|
|
|
|
|
|
|
|
|
|
|
|
|
|
|
|
|
|
|
|
|
|
|
|
|
|
|
|
|
|
|
|
|
|
|
|
|
|
|
|
|
|
|
|
|
|
|
|
|
|
|
|
|
|
|
|
|
|
|
|
|
|
|
|
|
|
|
|
|
|
|
|
|
|
|
|
|
|
|
|
|
|
|
|
|
|
|
|
|
|
|
|
|
|
|
|
|
|
|
|
|
|
|
|
|
|
|
|
|
|
|
|
|
|
|
|
|
|
|
|
|
|
|
|
|
|
|
|
|
|
|
|
|
|
|
|
|
|
|
|
|
|
|
|
|
|
|
|
|
|
|
|
|
|
|
|
|
|
|
|
|
|
|
|
|
spaces/Andy1621/uniformer_image_detection/configs/cascade_rcnn/cascade_mask_rcnn_x101_32x4d_fpn_20e_coco.py
DELETED
@@ -1,13 +0,0 @@
|
|
1 |
-
_base_ = './cascade_mask_rcnn_r50_fpn_20e_coco.py'
|
2 |
-
model = dict(
|
3 |
-
pretrained='open-mmlab://resnext101_32x4d',
|
4 |
-
backbone=dict(
|
5 |
-
type='ResNeXt',
|
6 |
-
depth=101,
|
7 |
-
groups=32,
|
8 |
-
base_width=4,
|
9 |
-
num_stages=4,
|
10 |
-
out_indices=(0, 1, 2, 3),
|
11 |
-
frozen_stages=1,
|
12 |
-
norm_cfg=dict(type='BN', requires_grad=True),
|
13 |
-
style='pytorch'))
|
|
|
|
|
|
|
|
|
|
|
|
|
|
|
|
|
|
|
|
|
|
|
|
|
|
|
|
spaces/Andy1621/uniformer_image_detection/mmdet/models/dense_heads/transformer_head.py
DELETED
@@ -1,654 +0,0 @@
|
|
1 |
-
import torch
|
2 |
-
import torch.nn as nn
|
3 |
-
import torch.nn.functional as F
|
4 |
-
from mmcv.cnn import Conv2d, Linear, build_activation_layer
|
5 |
-
from mmcv.runner import force_fp32
|
6 |
-
|
7 |
-
from mmdet.core import (bbox_cxcywh_to_xyxy, bbox_xyxy_to_cxcywh,
|
8 |
-
build_assigner, build_sampler, multi_apply,
|
9 |
-
reduce_mean)
|
10 |
-
from mmdet.models.utils import (FFN, build_positional_encoding,
|
11 |
-
build_transformer)
|
12 |
-
from ..builder import HEADS, build_loss
|
13 |
-
from .anchor_free_head import AnchorFreeHead
|
14 |
-
|
15 |
-
|
16 |
-
@HEADS.register_module()
|
17 |
-
class TransformerHead(AnchorFreeHead):
|
18 |
-
"""Implements the DETR transformer head.
|
19 |
-
|
20 |
-
See `paper: End-to-End Object Detection with Transformers
|
21 |
-
<https://arxiv.org/pdf/2005.12872>`_ for details.
|
22 |
-
|
23 |
-
Args:
|
24 |
-
num_classes (int): Number of categories excluding the background.
|
25 |
-
in_channels (int): Number of channels in the input feature map.
|
26 |
-
num_fcs (int, optional): Number of fully-connected layers used in
|
27 |
-
`FFN`, which is then used for the regression head. Default 2.
|
28 |
-
transformer (dict, optional): Config for transformer.
|
29 |
-
positional_encoding (dict, optional): Config for position encoding.
|
30 |
-
loss_cls (dict, optional): Config of the classification loss.
|
31 |
-
Default `CrossEntropyLoss`.
|
32 |
-
loss_bbox (dict, optional): Config of the regression loss.
|
33 |
-
Default `L1Loss`.
|
34 |
-
loss_iou (dict, optional): Config of the regression iou loss.
|
35 |
-
Default `GIoULoss`.
|
36 |
-
tran_cfg (dict, optional): Training config of transformer head.
|
37 |
-
test_cfg (dict, optional): Testing config of transformer head.
|
38 |
-
|
39 |
-
Example:
|
40 |
-
>>> import torch
|
41 |
-
>>> self = TransformerHead(80, 2048)
|
42 |
-
>>> x = torch.rand(1, 2048, 32, 32)
|
43 |
-
>>> mask = torch.ones(1, 32, 32).to(x.dtype)
|
44 |
-
>>> mask[:, :16, :15] = 0
|
45 |
-
>>> all_cls_scores, all_bbox_preds = self(x, mask)
|
46 |
-
"""
|
47 |
-
|
48 |
-
def __init__(self,
|
49 |
-
num_classes,
|
50 |
-
in_channels,
|
51 |
-
num_fcs=2,
|
52 |
-
transformer=dict(
|
53 |
-
type='Transformer',
|
54 |
-
embed_dims=256,
|
55 |
-
num_heads=8,
|
56 |
-
num_encoder_layers=6,
|
57 |
-
num_decoder_layers=6,
|
58 |
-
feedforward_channels=2048,
|
59 |
-
dropout=0.1,
|
60 |
-
act_cfg=dict(type='ReLU', inplace=True),
|
61 |
-
norm_cfg=dict(type='LN'),
|
62 |
-
num_fcs=2,
|
63 |
-
pre_norm=False,
|
64 |
-
return_intermediate_dec=True),
|
65 |
-
positional_encoding=dict(
|
66 |
-
type='SinePositionalEncoding',
|
67 |
-
num_feats=128,
|
68 |
-
normalize=True),
|
69 |
-
loss_cls=dict(
|
70 |
-
type='CrossEntropyLoss',
|
71 |
-
bg_cls_weight=0.1,
|
72 |
-
use_sigmoid=False,
|
73 |
-
loss_weight=1.0,
|
74 |
-
class_weight=1.0),
|
75 |
-
loss_bbox=dict(type='L1Loss', loss_weight=5.0),
|
76 |
-
loss_iou=dict(type='GIoULoss', loss_weight=2.0),
|
77 |
-
train_cfg=dict(
|
78 |
-
assigner=dict(
|
79 |
-
type='HungarianAssigner',
|
80 |
-
cls_cost=dict(type='ClassificationCost', weight=1.),
|
81 |
-
reg_cost=dict(type='BBoxL1Cost', weight=5.0),
|
82 |
-
iou_cost=dict(
|
83 |
-
type='IoUCost', iou_mode='giou', weight=2.0))),
|
84 |
-
test_cfg=dict(max_per_img=100),
|
85 |
-
**kwargs):
|
86 |
-
# NOTE here use `AnchorFreeHead` instead of `TransformerHead`,
|
87 |
-
# since it brings inconvenience when the initialization of
|
88 |
-
# `AnchorFreeHead` is called.
|
89 |
-
super(AnchorFreeHead, self).__init__()
|
90 |
-
use_sigmoid_cls = loss_cls.get('use_sigmoid', False)
|
91 |
-
assert not use_sigmoid_cls, 'setting use_sigmoid_cls as True is ' \
|
92 |
-
'not supported in DETR, since background is needed for the ' \
|
93 |
-
'matching process.'
|
94 |
-
assert 'embed_dims' in transformer \
|
95 |
-
and 'num_feats' in positional_encoding
|
96 |
-
num_feats = positional_encoding['num_feats']
|
97 |
-
embed_dims = transformer['embed_dims']
|
98 |
-
assert num_feats * 2 == embed_dims, 'embed_dims should' \
|
99 |
-
f' be exactly 2 times of num_feats. Found {embed_dims}' \
|
100 |
-
f' and {num_feats}.'
|
101 |
-
assert test_cfg is not None and 'max_per_img' in test_cfg
|
102 |
-
|
103 |
-
class_weight = loss_cls.get('class_weight', None)
|
104 |
-
if class_weight is not None:
|
105 |
-
assert isinstance(class_weight, float), 'Expected ' \
|
106 |
-
'class_weight to have type float. Found ' \
|
107 |
-
f'{type(class_weight)}.'
|
108 |
-
# NOTE following the official DETR rep0, bg_cls_weight means
|
109 |
-
# relative classification weight of the no-object class.
|
110 |
-
bg_cls_weight = loss_cls.get('bg_cls_weight', class_weight)
|
111 |
-
assert isinstance(bg_cls_weight, float), 'Expected ' \
|
112 |
-
'bg_cls_weight to have type float. Found ' \
|
113 |
-
f'{type(bg_cls_weight)}.'
|
114 |
-
class_weight = torch.ones(num_classes + 1) * class_weight
|
115 |
-
# set background class as the last indice
|
116 |
-
class_weight[num_classes] = bg_cls_weight
|
117 |
-
loss_cls.update({'class_weight': class_weight})
|
118 |
-
if 'bg_cls_weight' in loss_cls:
|
119 |
-
loss_cls.pop('bg_cls_weight')
|
120 |
-
self.bg_cls_weight = bg_cls_weight
|
121 |
-
|
122 |
-
if train_cfg:
|
123 |
-
assert 'assigner' in train_cfg, 'assigner should be provided '\
|
124 |
-
'when train_cfg is set.'
|
125 |
-
assigner = train_cfg['assigner']
|
126 |
-
assert loss_cls['loss_weight'] == assigner['cls_cost']['weight'], \
|
127 |
-
'The classification weight for loss and matcher should be' \
|
128 |
-
'exactly the same.'
|
129 |
-
assert loss_bbox['loss_weight'] == assigner['reg_cost'][
|
130 |
-
'weight'], 'The regression L1 weight for loss and matcher ' \
|
131 |
-
'should be exactly the same.'
|
132 |
-
assert loss_iou['loss_weight'] == assigner['iou_cost']['weight'], \
|
133 |
-
'The regression iou weight for loss and matcher should be' \
|
134 |
-
'exactly the same.'
|
135 |
-
self.assigner = build_assigner(assigner)
|
136 |
-
# DETR sampling=False, so use PseudoSampler
|
137 |
-
sampler_cfg = dict(type='PseudoSampler')
|
138 |
-
self.sampler = build_sampler(sampler_cfg, context=self)
|
139 |
-
self.num_classes = num_classes
|
140 |
-
self.cls_out_channels = num_classes + 1
|
141 |
-
self.in_channels = in_channels
|
142 |
-
self.num_fcs = num_fcs
|
143 |
-
self.train_cfg = train_cfg
|
144 |
-
self.test_cfg = test_cfg
|
145 |
-
self.use_sigmoid_cls = use_sigmoid_cls
|
146 |
-
self.embed_dims = embed_dims
|
147 |
-
self.num_query = test_cfg['max_per_img']
|
148 |
-
self.fp16_enabled = False
|
149 |
-
self.loss_cls = build_loss(loss_cls)
|
150 |
-
self.loss_bbox = build_loss(loss_bbox)
|
151 |
-
self.loss_iou = build_loss(loss_iou)
|
152 |
-
self.act_cfg = transformer.get('act_cfg',
|
153 |
-
dict(type='ReLU', inplace=True))
|
154 |
-
self.activate = build_activation_layer(self.act_cfg)
|
155 |
-
self.positional_encoding = build_positional_encoding(
|
156 |
-
positional_encoding)
|
157 |
-
self.transformer = build_transformer(transformer)
|
158 |
-
self._init_layers()
|
159 |
-
|
160 |
-
def _init_layers(self):
|
161 |
-
"""Initialize layers of the transformer head."""
|
162 |
-
self.input_proj = Conv2d(
|
163 |
-
self.in_channels, self.embed_dims, kernel_size=1)
|
164 |
-
self.fc_cls = Linear(self.embed_dims, self.cls_out_channels)
|
165 |
-
self.reg_ffn = FFN(
|
166 |
-
self.embed_dims,
|
167 |
-
self.embed_dims,
|
168 |
-
self.num_fcs,
|
169 |
-
self.act_cfg,
|
170 |
-
dropout=0.0,
|
171 |
-
add_residual=False)
|
172 |
-
self.fc_reg = Linear(self.embed_dims, 4)
|
173 |
-
self.query_embedding = nn.Embedding(self.num_query, self.embed_dims)
|
174 |
-
|
175 |
-
def init_weights(self, distribution='uniform'):
|
176 |
-
"""Initialize weights of the transformer head."""
|
177 |
-
# The initialization for transformer is important
|
178 |
-
self.transformer.init_weights()
|
179 |
-
|
180 |
-
def _load_from_state_dict(self, state_dict, prefix, local_metadata, strict,
|
181 |
-
missing_keys, unexpected_keys, error_msgs):
|
182 |
-
"""load checkpoints."""
|
183 |
-
# NOTE here use `AnchorFreeHead` instead of `TransformerHead`,
|
184 |
-
# since `AnchorFreeHead._load_from_state_dict` should not be
|
185 |
-
# called here. Invoking the default `Module._load_from_state_dict`
|
186 |
-
# is enough.
|
187 |
-
super(AnchorFreeHead,
|
188 |
-
self)._load_from_state_dict(state_dict, prefix, local_metadata,
|
189 |
-
strict, missing_keys,
|
190 |
-
unexpected_keys, error_msgs)
|
191 |
-
|
192 |
-
def forward(self, feats, img_metas):
|
193 |
-
"""Forward function.
|
194 |
-
|
195 |
-
Args:
|
196 |
-
feats (tuple[Tensor]): Features from the upstream network, each is
|
197 |
-
a 4D-tensor.
|
198 |
-
img_metas (list[dict]): List of image information.
|
199 |
-
|
200 |
-
Returns:
|
201 |
-
tuple[list[Tensor], list[Tensor]]: Outputs for all scale levels.
|
202 |
-
|
203 |
-
- all_cls_scores_list (list[Tensor]): Classification scores \
|
204 |
-
for each scale level. Each is a 4D-tensor with shape \
|
205 |
-
[nb_dec, bs, num_query, cls_out_channels]. Note \
|
206 |
-
`cls_out_channels` should includes background.
|
207 |
-
- all_bbox_preds_list (list[Tensor]): Sigmoid regression \
|
208 |
-
outputs for each scale level. Each is a 4D-tensor with \
|
209 |
-
normalized coordinate format (cx, cy, w, h) and shape \
|
210 |
-
[nb_dec, bs, num_query, 4].
|
211 |
-
"""
|
212 |
-
num_levels = len(feats)
|
213 |
-
img_metas_list = [img_metas for _ in range(num_levels)]
|
214 |
-
return multi_apply(self.forward_single, feats, img_metas_list)
|
215 |
-
|
216 |
-
def forward_single(self, x, img_metas):
|
217 |
-
""""Forward function for a single feature level.
|
218 |
-
|
219 |
-
Args:
|
220 |
-
x (Tensor): Input feature from backbone's single stage, shape
|
221 |
-
[bs, c, h, w].
|
222 |
-
img_metas (list[dict]): List of image information.
|
223 |
-
|
224 |
-
Returns:
|
225 |
-
all_cls_scores (Tensor): Outputs from the classification head,
|
226 |
-
shape [nb_dec, bs, num_query, cls_out_channels]. Note
|
227 |
-
cls_out_channels should includes background.
|
228 |
-
all_bbox_preds (Tensor): Sigmoid outputs from the regression
|
229 |
-
head with normalized coordinate format (cx, cy, w, h).
|
230 |
-
Shape [nb_dec, bs, num_query, 4].
|
231 |
-
"""
|
232 |
-
# construct binary masks which used for the transformer.
|
233 |
-
# NOTE following the official DETR repo, non-zero values representing
|
234 |
-
# ignored positions, while zero values means valid positions.
|
235 |
-
batch_size = x.size(0)
|
236 |
-
input_img_h, input_img_w = img_metas[0]['batch_input_shape']
|
237 |
-
masks = x.new_ones((batch_size, input_img_h, input_img_w))
|
238 |
-
for img_id in range(batch_size):
|
239 |
-
img_h, img_w, _ = img_metas[img_id]['img_shape']
|
240 |
-
masks[img_id, :img_h, :img_w] = 0
|
241 |
-
|
242 |
-
x = self.input_proj(x)
|
243 |
-
# interpolate masks to have the same spatial shape with x
|
244 |
-
masks = F.interpolate(
|
245 |
-
masks.unsqueeze(1), size=x.shape[-2:]).to(torch.bool).squeeze(1)
|
246 |
-
# position encoding
|
247 |
-
pos_embed = self.positional_encoding(masks) # [bs, embed_dim, h, w]
|
248 |
-
# outs_dec: [nb_dec, bs, num_query, embed_dim]
|
249 |
-
outs_dec, _ = self.transformer(x, masks, self.query_embedding.weight,
|
250 |
-
pos_embed)
|
251 |
-
|
252 |
-
all_cls_scores = self.fc_cls(outs_dec)
|
253 |
-
all_bbox_preds = self.fc_reg(self.activate(
|
254 |
-
self.reg_ffn(outs_dec))).sigmoid()
|
255 |
-
return all_cls_scores, all_bbox_preds
|
256 |
-
|
257 |
-
@force_fp32(apply_to=('all_cls_scores_list', 'all_bbox_preds_list'))
|
258 |
-
def loss(self,
|
259 |
-
all_cls_scores_list,
|
260 |
-
all_bbox_preds_list,
|
261 |
-
gt_bboxes_list,
|
262 |
-
gt_labels_list,
|
263 |
-
img_metas,
|
264 |
-
gt_bboxes_ignore=None):
|
265 |
-
""""Loss function.
|
266 |
-
|
267 |
-
Only outputs from the last feature level are used for computing
|
268 |
-
losses by default.
|
269 |
-
|
270 |
-
Args:
|
271 |
-
all_cls_scores_list (list[Tensor]): Classification outputs
|
272 |
-
for each feature level. Each is a 4D-tensor with shape
|
273 |
-
[nb_dec, bs, num_query, cls_out_channels].
|
274 |
-
all_bbox_preds_list (list[Tensor]): Sigmoid regression
|
275 |
-
outputs for each feature level. Each is a 4D-tensor with
|
276 |
-
normalized coordinate format (cx, cy, w, h) and shape
|
277 |
-
[nb_dec, bs, num_query, 4].
|
278 |
-
gt_bboxes_list (list[Tensor]): Ground truth bboxes for each image
|
279 |
-
with shape (num_gts, 4) in [tl_x, tl_y, br_x, br_y] format.
|
280 |
-
gt_labels_list (list[Tensor]): Ground truth class indices for each
|
281 |
-
image with shape (num_gts, ).
|
282 |
-
img_metas (list[dict]): List of image meta information.
|
283 |
-
gt_bboxes_ignore (list[Tensor], optional): Bounding boxes
|
284 |
-
which can be ignored for each image. Default None.
|
285 |
-
|
286 |
-
Returns:
|
287 |
-
dict[str, Tensor]: A dictionary of loss components.
|
288 |
-
"""
|
289 |
-
# NOTE defaultly only the outputs from the last feature scale is used.
|
290 |
-
all_cls_scores = all_cls_scores_list[-1]
|
291 |
-
all_bbox_preds = all_bbox_preds_list[-1]
|
292 |
-
assert gt_bboxes_ignore is None, \
|
293 |
-
'Only supports for gt_bboxes_ignore setting to None.'
|
294 |
-
|
295 |
-
num_dec_layers = len(all_cls_scores)
|
296 |
-
all_gt_bboxes_list = [gt_bboxes_list for _ in range(num_dec_layers)]
|
297 |
-
all_gt_labels_list = [gt_labels_list for _ in range(num_dec_layers)]
|
298 |
-
all_gt_bboxes_ignore_list = [
|
299 |
-
gt_bboxes_ignore for _ in range(num_dec_layers)
|
300 |
-
]
|
301 |
-
img_metas_list = [img_metas for _ in range(num_dec_layers)]
|
302 |
-
|
303 |
-
losses_cls, losses_bbox, losses_iou = multi_apply(
|
304 |
-
self.loss_single, all_cls_scores, all_bbox_preds,
|
305 |
-
all_gt_bboxes_list, all_gt_labels_list, img_metas_list,
|
306 |
-
all_gt_bboxes_ignore_list)
|
307 |
-
|
308 |
-
loss_dict = dict()
|
309 |
-
# loss from the last decoder layer
|
310 |
-
loss_dict['loss_cls'] = losses_cls[-1]
|
311 |
-
loss_dict['loss_bbox'] = losses_bbox[-1]
|
312 |
-
loss_dict['loss_iou'] = losses_iou[-1]
|
313 |
-
# loss from other decoder layers
|
314 |
-
num_dec_layer = 0
|
315 |
-
for loss_cls_i, loss_bbox_i, loss_iou_i in zip(losses_cls[:-1],
|
316 |
-
losses_bbox[:-1],
|
317 |
-
losses_iou[:-1]):
|
318 |
-
loss_dict[f'd{num_dec_layer}.loss_cls'] = loss_cls_i
|
319 |
-
loss_dict[f'd{num_dec_layer}.loss_bbox'] = loss_bbox_i
|
320 |
-
loss_dict[f'd{num_dec_layer}.loss_iou'] = loss_iou_i
|
321 |
-
num_dec_layer += 1
|
322 |
-
return loss_dict
|
323 |
-
|
324 |
-
def loss_single(self,
|
325 |
-
cls_scores,
|
326 |
-
bbox_preds,
|
327 |
-
gt_bboxes_list,
|
328 |
-
gt_labels_list,
|
329 |
-
img_metas,
|
330 |
-
gt_bboxes_ignore_list=None):
|
331 |
-
""""Loss function for outputs from a single decoder layer of a single
|
332 |
-
feature level.
|
333 |
-
|
334 |
-
Args:
|
335 |
-
cls_scores (Tensor): Box score logits from a single decoder layer
|
336 |
-
for all images. Shape [bs, num_query, cls_out_channels].
|
337 |
-
bbox_preds (Tensor): Sigmoid outputs from a single decoder layer
|
338 |
-
for all images, with normalized coordinate (cx, cy, w, h) and
|
339 |
-
shape [bs, num_query, 4].
|
340 |
-
gt_bboxes_list (list[Tensor]): Ground truth bboxes for each image
|
341 |
-
with shape (num_gts, 4) in [tl_x, tl_y, br_x, br_y] format.
|
342 |
-
gt_labels_list (list[Tensor]): Ground truth class indices for each
|
343 |
-
image with shape (num_gts, ).
|
344 |
-
img_metas (list[dict]): List of image meta information.
|
345 |
-
gt_bboxes_ignore_list (list[Tensor], optional): Bounding
|
346 |
-
boxes which can be ignored for each image. Default None.
|
347 |
-
|
348 |
-
Returns:
|
349 |
-
dict[str, Tensor]: A dictionary of loss components for outputs from
|
350 |
-
a single decoder layer.
|
351 |
-
"""
|
352 |
-
num_imgs = cls_scores.size(0)
|
353 |
-
cls_scores_list = [cls_scores[i] for i in range(num_imgs)]
|
354 |
-
bbox_preds_list = [bbox_preds[i] for i in range(num_imgs)]
|
355 |
-
cls_reg_targets = self.get_targets(cls_scores_list, bbox_preds_list,
|
356 |
-
gt_bboxes_list, gt_labels_list,
|
357 |
-
img_metas, gt_bboxes_ignore_list)
|
358 |
-
(labels_list, label_weights_list, bbox_targets_list, bbox_weights_list,
|
359 |
-
num_total_pos, num_total_neg) = cls_reg_targets
|
360 |
-
labels = torch.cat(labels_list, 0)
|
361 |
-
label_weights = torch.cat(label_weights_list, 0)
|
362 |
-
bbox_targets = torch.cat(bbox_targets_list, 0)
|
363 |
-
bbox_weights = torch.cat(bbox_weights_list, 0)
|
364 |
-
|
365 |
-
# classification loss
|
366 |
-
cls_scores = cls_scores.reshape(-1, self.cls_out_channels)
|
367 |
-
# construct weighted avg_factor to match with the official DETR repo
|
368 |
-
cls_avg_factor = num_total_pos * 1.0 + \
|
369 |
-
num_total_neg * self.bg_cls_weight
|
370 |
-
loss_cls = self.loss_cls(
|
371 |
-
cls_scores, labels, label_weights, avg_factor=cls_avg_factor)
|
372 |
-
|
373 |
-
# Compute the average number of gt boxes accross all gpus, for
|
374 |
-
# normalization purposes
|
375 |
-
num_total_pos = loss_cls.new_tensor([num_total_pos])
|
376 |
-
num_total_pos = torch.clamp(reduce_mean(num_total_pos), min=1).item()
|
377 |
-
|
378 |
-
# construct factors used for rescale bboxes
|
379 |
-
factors = []
|
380 |
-
for img_meta, bbox_pred in zip(img_metas, bbox_preds):
|
381 |
-
img_h, img_w, _ = img_meta['img_shape']
|
382 |
-
factor = bbox_pred.new_tensor([img_w, img_h, img_w,
|
383 |
-
img_h]).unsqueeze(0).repeat(
|
384 |
-
bbox_pred.size(0), 1)
|
385 |
-
factors.append(factor)
|
386 |
-
factors = torch.cat(factors, 0)
|
387 |
-
|
388 |
-
# DETR regress the relative position of boxes (cxcywh) in the image,
|
389 |
-
# thus the learning target is normalized by the image size. So here
|
390 |
-
# we need to re-scale them for calculating IoU loss
|
391 |
-
bbox_preds = bbox_preds.reshape(-1, 4)
|
392 |
-
bboxes = bbox_cxcywh_to_xyxy(bbox_preds) * factors
|
393 |
-
bboxes_gt = bbox_cxcywh_to_xyxy(bbox_targets) * factors
|
394 |
-
|
395 |
-
# regression IoU loss, defaultly GIoU loss
|
396 |
-
loss_iou = self.loss_iou(
|
397 |
-
bboxes, bboxes_gt, bbox_weights, avg_factor=num_total_pos)
|
398 |
-
|
399 |
-
# regression L1 loss
|
400 |
-
loss_bbox = self.loss_bbox(
|
401 |
-
bbox_preds, bbox_targets, bbox_weights, avg_factor=num_total_pos)
|
402 |
-
return loss_cls, loss_bbox, loss_iou
|
403 |
-
|
404 |
-
def get_targets(self,
|
405 |
-
cls_scores_list,
|
406 |
-
bbox_preds_list,
|
407 |
-
gt_bboxes_list,
|
408 |
-
gt_labels_list,
|
409 |
-
img_metas,
|
410 |
-
gt_bboxes_ignore_list=None):
|
411 |
-
""""Compute regression and classification targets for a batch image.
|
412 |
-
|
413 |
-
Outputs from a single decoder layer of a single feature level are used.
|
414 |
-
|
415 |
-
Args:
|
416 |
-
cls_scores_list (list[Tensor]): Box score logits from a single
|
417 |
-
decoder layer for each image with shape [num_query,
|
418 |
-
cls_out_channels].
|
419 |
-
bbox_preds_list (list[Tensor]): Sigmoid outputs from a single
|
420 |
-
decoder layer for each image, with normalized coordinate
|
421 |
-
(cx, cy, w, h) and shape [num_query, 4].
|
422 |
-
gt_bboxes_list (list[Tensor]): Ground truth bboxes for each image
|
423 |
-
with shape (num_gts, 4) in [tl_x, tl_y, br_x, br_y] format.
|
424 |
-
gt_labels_list (list[Tensor]): Ground truth class indices for each
|
425 |
-
image with shape (num_gts, ).
|
426 |
-
img_metas (list[dict]): List of image meta information.
|
427 |
-
gt_bboxes_ignore_list (list[Tensor], optional): Bounding
|
428 |
-
boxes which can be ignored for each image. Default None.
|
429 |
-
|
430 |
-
Returns:
|
431 |
-
tuple: a tuple containing the following targets.
|
432 |
-
|
433 |
-
- labels_list (list[Tensor]): Labels for all images.
|
434 |
-
- label_weights_list (list[Tensor]): Label weights for all \
|
435 |
-
images.
|
436 |
-
- bbox_targets_list (list[Tensor]): BBox targets for all \
|
437 |
-
images.
|
438 |
-
- bbox_weights_list (list[Tensor]): BBox weights for all \
|
439 |
-
images.
|
440 |
-
- num_total_pos (int): Number of positive samples in all \
|
441 |
-
images.
|
442 |
-
- num_total_neg (int): Number of negative samples in all \
|
443 |
-
images.
|
444 |
-
"""
|
445 |
-
assert gt_bboxes_ignore_list is None, \
|
446 |
-
'Only supports for gt_bboxes_ignore setting to None.'
|
447 |
-
num_imgs = len(cls_scores_list)
|
448 |
-
gt_bboxes_ignore_list = [
|
449 |
-
gt_bboxes_ignore_list for _ in range(num_imgs)
|
450 |
-
]
|
451 |
-
|
452 |
-
(labels_list, label_weights_list, bbox_targets_list,
|
453 |
-
bbox_weights_list, pos_inds_list, neg_inds_list) = multi_apply(
|
454 |
-
self._get_target_single, cls_scores_list, bbox_preds_list,
|
455 |
-
gt_bboxes_list, gt_labels_list, img_metas, gt_bboxes_ignore_list)
|
456 |
-
num_total_pos = sum((inds.numel() for inds in pos_inds_list))
|
457 |
-
num_total_neg = sum((inds.numel() for inds in neg_inds_list))
|
458 |
-
return (labels_list, label_weights_list, bbox_targets_list,
|
459 |
-
bbox_weights_list, num_total_pos, num_total_neg)
|
460 |
-
|
461 |
-
def _get_target_single(self,
|
462 |
-
cls_score,
|
463 |
-
bbox_pred,
|
464 |
-
gt_bboxes,
|
465 |
-
gt_labels,
|
466 |
-
img_meta,
|
467 |
-
gt_bboxes_ignore=None):
|
468 |
-
""""Compute regression and classification targets for one image.
|
469 |
-
|
470 |
-
Outputs from a single decoder layer of a single feature level are used.
|
471 |
-
|
472 |
-
Args:
|
473 |
-
cls_score (Tensor): Box score logits from a single decoder layer
|
474 |
-
for one image. Shape [num_query, cls_out_channels].
|
475 |
-
bbox_pred (Tensor): Sigmoid outputs from a single decoder layer
|
476 |
-
for one image, with normalized coordinate (cx, cy, w, h) and
|
477 |
-
shape [num_query, 4].
|
478 |
-
gt_bboxes (Tensor): Ground truth bboxes for one image with
|
479 |
-
shape (num_gts, 4) in [tl_x, tl_y, br_x, br_y] format.
|
480 |
-
gt_labels (Tensor): Ground truth class indices for one image
|
481 |
-
with shape (num_gts, ).
|
482 |
-
img_meta (dict): Meta information for one image.
|
483 |
-
gt_bboxes_ignore (Tensor, optional): Bounding boxes
|
484 |
-
which can be ignored. Default None.
|
485 |
-
|
486 |
-
Returns:
|
487 |
-
tuple[Tensor]: a tuple containing the following for one image.
|
488 |
-
|
489 |
-
- labels (Tensor): Labels of each image.
|
490 |
-
- label_weights (Tensor]): Label weights of each image.
|
491 |
-
- bbox_targets (Tensor): BBox targets of each image.
|
492 |
-
- bbox_weights (Tensor): BBox weights of each image.
|
493 |
-
- pos_inds (Tensor): Sampled positive indices for each image.
|
494 |
-
- neg_inds (Tensor): Sampled negative indices for each image.
|
495 |
-
"""
|
496 |
-
|
497 |
-
num_bboxes = bbox_pred.size(0)
|
498 |
-
# assigner and sampler
|
499 |
-
assign_result = self.assigner.assign(bbox_pred, cls_score, gt_bboxes,
|
500 |
-
gt_labels, img_meta,
|
501 |
-
gt_bboxes_ignore)
|
502 |
-
sampling_result = self.sampler.sample(assign_result, bbox_pred,
|
503 |
-
gt_bboxes)
|
504 |
-
pos_inds = sampling_result.pos_inds
|
505 |
-
neg_inds = sampling_result.neg_inds
|
506 |
-
|
507 |
-
# label targets
|
508 |
-
labels = gt_bboxes.new_full((num_bboxes, ),
|
509 |
-
self.num_classes,
|
510 |
-
dtype=torch.long)
|
511 |
-
labels[pos_inds] = gt_labels[sampling_result.pos_assigned_gt_inds]
|
512 |
-
label_weights = gt_bboxes.new_ones(num_bboxes)
|
513 |
-
|
514 |
-
# bbox targets
|
515 |
-
bbox_targets = torch.zeros_like(bbox_pred)
|
516 |
-
bbox_weights = torch.zeros_like(bbox_pred)
|
517 |
-
bbox_weights[pos_inds] = 1.0
|
518 |
-
img_h, img_w, _ = img_meta['img_shape']
|
519 |
-
|
520 |
-
# DETR regress the relative position of boxes (cxcywh) in the image.
|
521 |
-
# Thus the learning target should be normalized by the image size, also
|
522 |
-
# the box format should be converted from defaultly x1y1x2y2 to cxcywh.
|
523 |
-
factor = bbox_pred.new_tensor([img_w, img_h, img_w,
|
524 |
-
img_h]).unsqueeze(0)
|
525 |
-
pos_gt_bboxes_normalized = sampling_result.pos_gt_bboxes / factor
|
526 |
-
pos_gt_bboxes_targets = bbox_xyxy_to_cxcywh(pos_gt_bboxes_normalized)
|
527 |
-
bbox_targets[pos_inds] = pos_gt_bboxes_targets
|
528 |
-
return (labels, label_weights, bbox_targets, bbox_weights, pos_inds,
|
529 |
-
neg_inds)
|
530 |
-
|
531 |
-
# over-write because img_metas are needed as inputs for bbox_head.
|
532 |
-
def forward_train(self,
|
533 |
-
x,
|
534 |
-
img_metas,
|
535 |
-
gt_bboxes,
|
536 |
-
gt_labels=None,
|
537 |
-
gt_bboxes_ignore=None,
|
538 |
-
proposal_cfg=None,
|
539 |
-
**kwargs):
|
540 |
-
"""Forward function for training mode.
|
541 |
-
|
542 |
-
Args:
|
543 |
-
x (list[Tensor]): Features from backbone.
|
544 |
-
img_metas (list[dict]): Meta information of each image, e.g.,
|
545 |
-
image size, scaling factor, etc.
|
546 |
-
gt_bboxes (Tensor): Ground truth bboxes of the image,
|
547 |
-
shape (num_gts, 4).
|
548 |
-
gt_labels (Tensor): Ground truth labels of each box,
|
549 |
-
shape (num_gts,).
|
550 |
-
gt_bboxes_ignore (Tensor): Ground truth bboxes to be
|
551 |
-
ignored, shape (num_ignored_gts, 4).
|
552 |
-
proposal_cfg (mmcv.Config): Test / postprocessing configuration,
|
553 |
-
if None, test_cfg would be used.
|
554 |
-
|
555 |
-
Returns:
|
556 |
-
dict[str, Tensor]: A dictionary of loss components.
|
557 |
-
"""
|
558 |
-
assert proposal_cfg is None, '"proposal_cfg" must be None'
|
559 |
-
outs = self(x, img_metas)
|
560 |
-
if gt_labels is None:
|
561 |
-
loss_inputs = outs + (gt_bboxes, img_metas)
|
562 |
-
else:
|
563 |
-
loss_inputs = outs + (gt_bboxes, gt_labels, img_metas)
|
564 |
-
losses = self.loss(*loss_inputs, gt_bboxes_ignore=gt_bboxes_ignore)
|
565 |
-
return losses
|
566 |
-
|
567 |
-
@force_fp32(apply_to=('all_cls_scores_list', 'all_bbox_preds_list'))
|
568 |
-
def get_bboxes(self,
|
569 |
-
all_cls_scores_list,
|
570 |
-
all_bbox_preds_list,
|
571 |
-
img_metas,
|
572 |
-
rescale=False):
|
573 |
-
"""Transform network outputs for a batch into bbox predictions.
|
574 |
-
|
575 |
-
Args:
|
576 |
-
all_cls_scores_list (list[Tensor]): Classification outputs
|
577 |
-
for each feature level. Each is a 4D-tensor with shape
|
578 |
-
[nb_dec, bs, num_query, cls_out_channels].
|
579 |
-
all_bbox_preds_list (list[Tensor]): Sigmoid regression
|
580 |
-
outputs for each feature level. Each is a 4D-tensor with
|
581 |
-
normalized coordinate format (cx, cy, w, h) and shape
|
582 |
-
[nb_dec, bs, num_query, 4].
|
583 |
-
img_metas (list[dict]): Meta information of each image.
|
584 |
-
rescale (bool, optional): If True, return boxes in original
|
585 |
-
image space. Default False.
|
586 |
-
|
587 |
-
Returns:
|
588 |
-
list[list[Tensor, Tensor]]: Each item in result_list is 2-tuple. \
|
589 |
-
The first item is an (n, 5) tensor, where the first 4 columns \
|
590 |
-
are bounding box positions (tl_x, tl_y, br_x, br_y) and the \
|
591 |
-
5-th column is a score between 0 and 1. The second item is a \
|
592 |
-
(n,) tensor where each item is the predicted class label of \
|
593 |
-
the corresponding box.
|
594 |
-
"""
|
595 |
-
# NOTE defaultly only using outputs from the last feature level,
|
596 |
-
# and only the outputs from the last decoder layer is used.
|
597 |
-
cls_scores = all_cls_scores_list[-1][-1]
|
598 |
-
bbox_preds = all_bbox_preds_list[-1][-1]
|
599 |
-
|
600 |
-
result_list = []
|
601 |
-
for img_id in range(len(img_metas)):
|
602 |
-
cls_score = cls_scores[img_id]
|
603 |
-
bbox_pred = bbox_preds[img_id]
|
604 |
-
img_shape = img_metas[img_id]['img_shape']
|
605 |
-
scale_factor = img_metas[img_id]['scale_factor']
|
606 |
-
proposals = self._get_bboxes_single(cls_score, bbox_pred,
|
607 |
-
img_shape, scale_factor,
|
608 |
-
rescale)
|
609 |
-
result_list.append(proposals)
|
610 |
-
return result_list
|
611 |
-
|
612 |
-
def _get_bboxes_single(self,
|
613 |
-
cls_score,
|
614 |
-
bbox_pred,
|
615 |
-
img_shape,
|
616 |
-
scale_factor,
|
617 |
-
rescale=False):
|
618 |
-
"""Transform outputs from the last decoder layer into bbox predictions
|
619 |
-
for each image.
|
620 |
-
|
621 |
-
Args:
|
622 |
-
cls_score (Tensor): Box score logits from the last decoder layer
|
623 |
-
for each image. Shape [num_query, cls_out_channels].
|
624 |
-
bbox_pred (Tensor): Sigmoid outputs from the last decoder layer
|
625 |
-
for each image, with coordinate format (cx, cy, w, h) and
|
626 |
-
shape [num_query, 4].
|
627 |
-
img_shape (tuple[int]): Shape of input image, (height, width, 3).
|
628 |
-
scale_factor (ndarray, optional): Scale factor of the image arange
|
629 |
-
as (w_scale, h_scale, w_scale, h_scale).
|
630 |
-
rescale (bool, optional): If True, return boxes in original image
|
631 |
-
space. Default False.
|
632 |
-
|
633 |
-
Returns:
|
634 |
-
tuple[Tensor]: Results of detected bboxes and labels.
|
635 |
-
|
636 |
-
- det_bboxes: Predicted bboxes with shape [num_query, 5], \
|
637 |
-
where the first 4 columns are bounding box positions \
|
638 |
-
(tl_x, tl_y, br_x, br_y) and the 5-th column are scores \
|
639 |
-
between 0 and 1.
|
640 |
-
- det_labels: Predicted labels of the corresponding box with \
|
641 |
-
shape [num_query].
|
642 |
-
"""
|
643 |
-
assert len(cls_score) == len(bbox_pred)
|
644 |
-
# exclude background
|
645 |
-
scores, det_labels = F.softmax(cls_score, dim=-1)[..., :-1].max(-1)
|
646 |
-
det_bboxes = bbox_cxcywh_to_xyxy(bbox_pred)
|
647 |
-
det_bboxes[:, 0::2] = det_bboxes[:, 0::2] * img_shape[1]
|
648 |
-
det_bboxes[:, 1::2] = det_bboxes[:, 1::2] * img_shape[0]
|
649 |
-
det_bboxes[:, 0::2].clamp_(min=0, max=img_shape[1])
|
650 |
-
det_bboxes[:, 1::2].clamp_(min=0, max=img_shape[0])
|
651 |
-
if rescale:
|
652 |
-
det_bboxes /= det_bboxes.new_tensor(scale_factor)
|
653 |
-
det_bboxes = torch.cat((det_bboxes, scores.unsqueeze(1)), -1)
|
654 |
-
return det_bboxes, det_labels
|
|
|
|
|
|
|
|
|
|
|
|
|
|
|
|
|
|
|
|
|
|
|
|
|
|
|
|
|
|
|
|
|
|
|
|
|
|
|
|
|
|
|
|
|
|
|
|
|
|
|
|
|
|
|
|
|
|
|
|
|
|
|
|
|
|
|
|
|
|
|
|
|
|
|
|
|
|
|
|
|
|
|
|
|
|
|
|
|
|
|
|
|
|
|
|
|
|
|
|
|
|
|
|
|
|
|
|
|
|
|
|
|
|
|
|
|
|
|
|
|
|
|
|
|
|
|
|
|
|
|
|
|
|
|
|
|
|
|
|
|
|
|
|
|
|
|
|
|
|
|
|
|
|
|
|
|
|
|
|
|
|
|
|
|
|
|
|
|
|
|
|
|
|
|
|
|
|
|
|
|
|
|
|
|
|
|
|
|
|
|
|
|
|
|
|
|
|
|
|
|
|
|
|
|
|
|
|
|
|
|
|
|
|
|
|
|
|
|
|
|
|
|
|
|
|
|
|
|
|
|
|
|
|
|
|
|
|
|
|
|
|
|
|
|
|
|
|
|
|
|
|
|
|
|
|
|
|
|
|
|
|
|
|
|
|
|
|
|
|
|
|
|
|
|
|
|
|
|
|
|
|
|
|
|
|
|
|
|
|
|
|
|
|
|
|
|
|
|
|
|
|
|
|
|
|
|
|
|
|
|
|
|
|
|
|
|
|
|
|
|
|
|
|
|
|
|
|
|
|
|
|
|
|
|
|
|
|
|
|
|
|
|
|
|
|
|
|
|
|
|
|
|
|
|
|
|
|
|
|
|
|
|
|
|
|
|
|
|
|
|
|
|
|
|
|
|
|
|
|
|
|
|
|
|
|
|
|
|
|
|
|
|
|
|
|
|
|
|
|
|
|
|
|
|
|
|
|
|
|
|
|
|
|
|
|
|
|
|
|
|
|
|
|
|
|
|
|
|
|
|
|
|
|
|
|
|
|
|
|
|
|
|
|
|
|
|
|
|
|
|
|
|
|
|
|
|
|
|
|
|
|
|
|
|
|
|
|
|
|
|
|
|
|
|
|
|
|
|
|
|
|
|
|
|
|
|
|
|
|
|
|
|
|
|
|
|
|
|
|
|
|
|
|
|
|
|
|
|
|
|
|
|
|
|
|
|
|
|
|
|
|
|
|
|
|
|
|
|
|
|
|
|
|
|
|
|
|
|
|
|
|
|
|
|
|
|
|
|
|
|
|
|
|
|
|
|
|
|
|
|
|
|
|
|
|
|
|
|
|
|
|
|
|
|
|
|
|
|
|
|
|
|
|
|
|
|
|
|
|
|
|
|
|
|
|
|
|
|
|
|
|
|
|
|
|
|
|
|
|
|
|
|
|
|
|
|
|
|
|
|
|
|
|
|
|
|
|
|
|
|
|
|
|
|
|
|
|
|
|
|
|
|
|
|
|
|
|
|
|
|
|
|
|
|
|
|
|
|
|
|
|
|
|
|
|
|
|
|
|
|
|
|
|
|
|
|
|
|
|
|
|
|
|
|
|
|
|
|
|
|
|
|
|
|
|
|
|
|
|
|
|
|
|
|
|
|
|
|
|
|
|
|
|
|
|
|
|
|
|
|
|
|
|
|
|
|
|
|
|
|
|
|
|
|
|
|
|
|
|
|
|
|
|
|
|
|
|
|
|
|
|
|
|
|
|
|
|
|
|
|
|
|
|
|
|
|
|
|
|
|
|
|
|
|
|
|
|
|
|
|
|
|
|
|
|
|
|
|
|
|
|
|
|
|
|
|
|
|
|
|
|
|
|
|
|
|
|
|
|
|
|
|
|
|
|
|
|
|
|
|
|
|
|
|
|
|
|
|
|
|
|
|
|
|
|
|
|
|
|
|
|
|
|
|
|
|
|
|
|
|
|
|
|
|
|
|
|
|
|
|
|
|
|
|
|
|
|
|
|
|
|
|
|
|
|
|
|
|
|
|
|
|
|
|
|
|
|
|
|
|
|
|
|
|
|
|
|
|
|
|
|
|
|
|
|
|
|
|
|
|
|
|
|
|
|
|
|
|
|
|
|
|
|
|
|
|
|
|
|
|
|
|
|
|
|
|
|
|
|
|
|
|
|
|
|
|
|
|
|
|
|
|
|
|
|
|
|
|
|
|
|
|
|
|
|
|
|
|
|
|
|
|
|
|
|
|
|
|
|
|
|
|
|
|
|
|
|
|
|
|
|
|
|
|
|
|
|
|
|
|
|
|
|
|
|
|
|
|
|
|
|
|
|
|
|
|
|
|
|
|
|
|
|
|
|
|
|
|
|
|
|
|
|
|
|
|
|
|
|
|
|
|
|
|
|
|
|
|
|
|
|
|
|
|
|
|
|
|
|
|
|
|
|
|
|
|
|
|
|
|
|
|
|
|
|
|
|
|
|
|
|
|
|
|
|
|
|
|
|
|
|
|
|
|
|
|
|
|
|
|
|
|
|
|
|
|
|
|
|
|
|
|
|
|
|
|
|
|
|
|
|
|
|
|
|
|
|
|
|
|
|
|
|
|
|
|
|
|
|
|
|
|
|
|
|
|
|
|
|
|
|
|
|
|
|
|
|
|
|
|
|
|
|
|
|
|
|
|
|
|
|
|
|
|
|
|
|
|
|
|
|
|
|
|
|
|
|
|
|
|
|
|
|
|
|
|
|
|
|
|
|
|
|
|
|
|
|
|
|
|
|
|
|
|
|
|
|
|
|
|
|
|
|
|
|
|
|
|
|
|
|
|
|
|
|
|
|
|
|
|
|
|
|
|
|
|
|
|
|
|
|
|
|
|
|
|
|
|
|
|
|
|
|
|
|
|
|
|
|
|
|
|
|
|
|
|
|
|
|
|
|
|
|
|
|
|
|
|
|
|
|
|
|
|
|
|
|
|
|
|
|
|
|
|
|
|
|
|
|
spaces/Ariharasudhan/YoloV5/export.py
DELETED
@@ -1,652 +0,0 @@
|
|
1 |
-
# YOLOv5 🚀 by Ultralytics, GPL-3.0 license
|
2 |
-
"""
|
3 |
-
Export a YOLOv5 PyTorch model to other formats. TensorFlow exports authored by https://github.com/zldrobit
|
4 |
-
|
5 |
-
Format | `export.py --include` | Model
|
6 |
-
--- | --- | ---
|
7 |
-
PyTorch | - | yolov5s.pt
|
8 |
-
TorchScript | `torchscript` | yolov5s.torchscript
|
9 |
-
ONNX | `onnx` | yolov5s.onnx
|
10 |
-
OpenVINO | `openvino` | yolov5s_openvino_model/
|
11 |
-
TensorRT | `engine` | yolov5s.engine
|
12 |
-
CoreML | `coreml` | yolov5s.mlmodel
|
13 |
-
TensorFlow SavedModel | `saved_model` | yolov5s_saved_model/
|
14 |
-
TensorFlow GraphDef | `pb` | yolov5s.pb
|
15 |
-
TensorFlow Lite | `tflite` | yolov5s.tflite
|
16 |
-
TensorFlow Edge TPU | `edgetpu` | yolov5s_edgetpu.tflite
|
17 |
-
TensorFlow.js | `tfjs` | yolov5s_web_model/
|
18 |
-
PaddlePaddle | `paddle` | yolov5s_paddle_model/
|
19 |
-
|
20 |
-
Requirements:
|
21 |
-
$ pip install -r requirements.txt coremltools onnx onnx-simplifier onnxruntime openvino-dev tensorflow-cpu # CPU
|
22 |
-
$ pip install -r requirements.txt coremltools onnx onnx-simplifier onnxruntime-gpu openvino-dev tensorflow # GPU
|
23 |
-
|
24 |
-
Usage:
|
25 |
-
$ python export.py --weights yolov5s.pt --include torchscript onnx openvino engine coreml tflite ...
|
26 |
-
|
27 |
-
Inference:
|
28 |
-
$ python detect.py --weights yolov5s.pt # PyTorch
|
29 |
-
yolov5s.torchscript # TorchScript
|
30 |
-
yolov5s.onnx # ONNX Runtime or OpenCV DNN with --dnn
|
31 |
-
yolov5s_openvino_model # OpenVINO
|
32 |
-
yolov5s.engine # TensorRT
|
33 |
-
yolov5s.mlmodel # CoreML (macOS-only)
|
34 |
-
yolov5s_saved_model # TensorFlow SavedModel
|
35 |
-
yolov5s.pb # TensorFlow GraphDef
|
36 |
-
yolov5s.tflite # TensorFlow Lite
|
37 |
-
yolov5s_edgetpu.tflite # TensorFlow Edge TPU
|
38 |
-
yolov5s_paddle_model # PaddlePaddle
|
39 |
-
|
40 |
-
TensorFlow.js:
|
41 |
-
$ cd .. && git clone https://github.com/zldrobit/tfjs-yolov5-example.git && cd tfjs-yolov5-example
|
42 |
-
$ npm install
|
43 |
-
$ ln -s ../../yolov5/yolov5s_web_model public/yolov5s_web_model
|
44 |
-
$ npm start
|
45 |
-
"""
|
46 |
-
|
47 |
-
import argparse
|
48 |
-
import contextlib
|
49 |
-
import json
|
50 |
-
import os
|
51 |
-
import platform
|
52 |
-
import re
|
53 |
-
import subprocess
|
54 |
-
import sys
|
55 |
-
import time
|
56 |
-
import warnings
|
57 |
-
from pathlib import Path
|
58 |
-
|
59 |
-
import pandas as pd
|
60 |
-
import torch
|
61 |
-
from torch.utils.mobile_optimizer import optimize_for_mobile
|
62 |
-
|
63 |
-
FILE = Path(__file__).resolve()
|
64 |
-
ROOT = FILE.parents[0] # YOLOv5 root directory
|
65 |
-
if str(ROOT) not in sys.path:
|
66 |
-
sys.path.append(str(ROOT)) # add ROOT to PATH
|
67 |
-
if platform.system() != 'Windows':
|
68 |
-
ROOT = Path(os.path.relpath(ROOT, Path.cwd())) # relative
|
69 |
-
|
70 |
-
from models.experimental import attempt_load
|
71 |
-
from models.yolo import ClassificationModel, Detect, DetectionModel, SegmentationModel
|
72 |
-
from utils.dataloaders import LoadImages
|
73 |
-
from utils.general import (LOGGER, Profile, check_dataset, check_img_size, check_requirements, check_version,
|
74 |
-
check_yaml, colorstr, file_size, get_default_args, print_args, url2file, yaml_save)
|
75 |
-
from utils.torch_utils import select_device, smart_inference_mode
|
76 |
-
|
77 |
-
MACOS = platform.system() == 'Darwin' # macOS environment
|
78 |
-
|
79 |
-
|
80 |
-
def export_formats():
|
81 |
-
# YOLOv5 export formats
|
82 |
-
x = [
|
83 |
-
['PyTorch', '-', '.pt', True, True],
|
84 |
-
['TorchScript', 'torchscript', '.torchscript', True, True],
|
85 |
-
['ONNX', 'onnx', '.onnx', True, True],
|
86 |
-
['OpenVINO', 'openvino', '_openvino_model', True, False],
|
87 |
-
['TensorRT', 'engine', '.engine', False, True],
|
88 |
-
['CoreML', 'coreml', '.mlmodel', True, False],
|
89 |
-
['TensorFlow SavedModel', 'saved_model', '_saved_model', True, True],
|
90 |
-
['TensorFlow GraphDef', 'pb', '.pb', True, True],
|
91 |
-
['TensorFlow Lite', 'tflite', '.tflite', True, False],
|
92 |
-
['TensorFlow Edge TPU', 'edgetpu', '_edgetpu.tflite', False, False],
|
93 |
-
['TensorFlow.js', 'tfjs', '_web_model', False, False],
|
94 |
-
['PaddlePaddle', 'paddle', '_paddle_model', True, True],]
|
95 |
-
return pd.DataFrame(x, columns=['Format', 'Argument', 'Suffix', 'CPU', 'GPU'])
|
96 |
-
|
97 |
-
|
98 |
-
def try_export(inner_func):
|
99 |
-
# YOLOv5 export decorator, i..e @try_export
|
100 |
-
inner_args = get_default_args(inner_func)
|
101 |
-
|
102 |
-
def outer_func(*args, **kwargs):
|
103 |
-
prefix = inner_args['prefix']
|
104 |
-
try:
|
105 |
-
with Profile() as dt:
|
106 |
-
f, model = inner_func(*args, **kwargs)
|
107 |
-
LOGGER.info(f'{prefix} export success ✅ {dt.t:.1f}s, saved as {f} ({file_size(f):.1f} MB)')
|
108 |
-
return f, model
|
109 |
-
except Exception as e:
|
110 |
-
LOGGER.info(f'{prefix} export failure ❌ {dt.t:.1f}s: {e}')
|
111 |
-
return None, None
|
112 |
-
|
113 |
-
return outer_func
|
114 |
-
|
115 |
-
|
116 |
-
@try_export
|
117 |
-
def export_torchscript(model, im, file, optimize, prefix=colorstr('TorchScript:')):
|
118 |
-
# YOLOv5 TorchScript model export
|
119 |
-
LOGGER.info(f'\n{prefix} starting export with torch {torch.__version__}...')
|
120 |
-
f = file.with_suffix('.torchscript')
|
121 |
-
|
122 |
-
ts = torch.jit.trace(model, im, strict=False)
|
123 |
-
d = {"shape": im.shape, "stride": int(max(model.stride)), "names": model.names}
|
124 |
-
extra_files = {'config.txt': json.dumps(d)} # torch._C.ExtraFilesMap()
|
125 |
-
if optimize: # https://pytorch.org/tutorials/recipes/mobile_interpreter.html
|
126 |
-
optimize_for_mobile(ts)._save_for_lite_interpreter(str(f), _extra_files=extra_files)
|
127 |
-
else:
|
128 |
-
ts.save(str(f), _extra_files=extra_files)
|
129 |
-
return f, None
|
130 |
-
|
131 |
-
|
132 |
-
@try_export
|
133 |
-
def export_onnx(model, im, file, opset, dynamic, simplify, prefix=colorstr('ONNX:')):
|
134 |
-
# YOLOv5 ONNX export
|
135 |
-
check_requirements('onnx')
|
136 |
-
import onnx
|
137 |
-
|
138 |
-
LOGGER.info(f'\n{prefix} starting export with onnx {onnx.__version__}...')
|
139 |
-
f = file.with_suffix('.onnx')
|
140 |
-
|
141 |
-
output_names = ['output0', 'output1'] if isinstance(model, SegmentationModel) else ['output0']
|
142 |
-
if dynamic:
|
143 |
-
dynamic = {'images': {0: 'batch', 2: 'height', 3: 'width'}} # shape(1,3,640,640)
|
144 |
-
if isinstance(model, SegmentationModel):
|
145 |
-
dynamic['output0'] = {0: 'batch', 1: 'anchors'} # shape(1,25200,85)
|
146 |
-
dynamic['output1'] = {0: 'batch', 2: 'mask_height', 3: 'mask_width'} # shape(1,32,160,160)
|
147 |
-
elif isinstance(model, DetectionModel):
|
148 |
-
dynamic['output0'] = {0: 'batch', 1: 'anchors'} # shape(1,25200,85)
|
149 |
-
|
150 |
-
torch.onnx.export(
|
151 |
-
model.cpu() if dynamic else model, # --dynamic only compatible with cpu
|
152 |
-
im.cpu() if dynamic else im,
|
153 |
-
f,
|
154 |
-
verbose=False,
|
155 |
-
opset_version=opset,
|
156 |
-
do_constant_folding=True,
|
157 |
-
input_names=['images'],
|
158 |
-
output_names=output_names,
|
159 |
-
dynamic_axes=dynamic or None)
|
160 |
-
|
161 |
-
# Checks
|
162 |
-
model_onnx = onnx.load(f) # load onnx model
|
163 |
-
onnx.checker.check_model(model_onnx) # check onnx model
|
164 |
-
|
165 |
-
# Metadata
|
166 |
-
d = {'stride': int(max(model.stride)), 'names': model.names}
|
167 |
-
for k, v in d.items():
|
168 |
-
meta = model_onnx.metadata_props.add()
|
169 |
-
meta.key, meta.value = k, str(v)
|
170 |
-
onnx.save(model_onnx, f)
|
171 |
-
|
172 |
-
# Simplify
|
173 |
-
if simplify:
|
174 |
-
try:
|
175 |
-
cuda = torch.cuda.is_available()
|
176 |
-
check_requirements(('onnxruntime-gpu' if cuda else 'onnxruntime', 'onnx-simplifier>=0.4.1'))
|
177 |
-
import onnxsim
|
178 |
-
|
179 |
-
LOGGER.info(f'{prefix} simplifying with onnx-simplifier {onnxsim.__version__}...')
|
180 |
-
model_onnx, check = onnxsim.simplify(model_onnx)
|
181 |
-
assert check, 'assert check failed'
|
182 |
-
onnx.save(model_onnx, f)
|
183 |
-
except Exception as e:
|
184 |
-
LOGGER.info(f'{prefix} simplifier failure: {e}')
|
185 |
-
return f, model_onnx
|
186 |
-
|
187 |
-
|
188 |
-
@try_export
|
189 |
-
def export_openvino(file, metadata, half, prefix=colorstr('OpenVINO:')):
|
190 |
-
# YOLOv5 OpenVINO export
|
191 |
-
check_requirements('openvino-dev') # requires openvino-dev: https://pypi.org/project/openvino-dev/
|
192 |
-
import openvino.inference_engine as ie
|
193 |
-
|
194 |
-
LOGGER.info(f'\n{prefix} starting export with openvino {ie.__version__}...')
|
195 |
-
f = str(file).replace('.pt', f'_openvino_model{os.sep}')
|
196 |
-
|
197 |
-
cmd = f"mo --input_model {file.with_suffix('.onnx')} --output_dir {f} --data_type {'FP16' if half else 'FP32'}"
|
198 |
-
subprocess.run(cmd.split(), check=True, env=os.environ) # export
|
199 |
-
yaml_save(Path(f) / file.with_suffix('.yaml').name, metadata) # add metadata.yaml
|
200 |
-
return f, None
|
201 |
-
|
202 |
-
|
203 |
-
@try_export
|
204 |
-
def export_paddle(model, im, file, metadata, prefix=colorstr('PaddlePaddle:')):
|
205 |
-
# YOLOv5 Paddle export
|
206 |
-
check_requirements(('paddlepaddle', 'x2paddle'))
|
207 |
-
import x2paddle
|
208 |
-
from x2paddle.convert import pytorch2paddle
|
209 |
-
|
210 |
-
LOGGER.info(f'\n{prefix} starting export with X2Paddle {x2paddle.__version__}...')
|
211 |
-
f = str(file).replace('.pt', f'_paddle_model{os.sep}')
|
212 |
-
|
213 |
-
pytorch2paddle(module=model, save_dir=f, jit_type='trace', input_examples=[im]) # export
|
214 |
-
yaml_save(Path(f) / file.with_suffix('.yaml').name, metadata) # add metadata.yaml
|
215 |
-
return f, None
|
216 |
-
|
217 |
-
|
218 |
-
@try_export
|
219 |
-
def export_coreml(model, im, file, int8, half, prefix=colorstr('CoreML:')):
|
220 |
-
# YOLOv5 CoreML export
|
221 |
-
check_requirements('coremltools')
|
222 |
-
import coremltools as ct
|
223 |
-
|
224 |
-
LOGGER.info(f'\n{prefix} starting export with coremltools {ct.__version__}...')
|
225 |
-
f = file.with_suffix('.mlmodel')
|
226 |
-
|
227 |
-
ts = torch.jit.trace(model, im, strict=False) # TorchScript model
|
228 |
-
ct_model = ct.convert(ts, inputs=[ct.ImageType('image', shape=im.shape, scale=1 / 255, bias=[0, 0, 0])])
|
229 |
-
bits, mode = (8, 'kmeans_lut') if int8 else (16, 'linear') if half else (32, None)
|
230 |
-
if bits < 32:
|
231 |
-
if MACOS: # quantization only supported on macOS
|
232 |
-
with warnings.catch_warnings():
|
233 |
-
warnings.filterwarnings("ignore", category=DeprecationWarning) # suppress numpy==1.20 float warning
|
234 |
-
ct_model = ct.models.neural_network.quantization_utils.quantize_weights(ct_model, bits, mode)
|
235 |
-
else:
|
236 |
-
print(f'{prefix} quantization only supported on macOS, skipping...')
|
237 |
-
ct_model.save(f)
|
238 |
-
return f, ct_model
|
239 |
-
|
240 |
-
|
241 |
-
@try_export
|
242 |
-
def export_engine(model, im, file, half, dynamic, simplify, workspace=4, verbose=False, prefix=colorstr('TensorRT:')):
|
243 |
-
# YOLOv5 TensorRT export https://developer.nvidia.com/tensorrt
|
244 |
-
assert im.device.type != 'cpu', 'export running on CPU but must be on GPU, i.e. `python export.py --device 0`'
|
245 |
-
try:
|
246 |
-
import tensorrt as trt
|
247 |
-
except Exception:
|
248 |
-
if platform.system() == 'Linux':
|
249 |
-
check_requirements('nvidia-tensorrt', cmds='-U --index-url https://pypi.ngc.nvidia.com')
|
250 |
-
import tensorrt as trt
|
251 |
-
|
252 |
-
if trt.__version__[0] == '7': # TensorRT 7 handling https://github.com/ultralytics/yolov5/issues/6012
|
253 |
-
grid = model.model[-1].anchor_grid
|
254 |
-
model.model[-1].anchor_grid = [a[..., :1, :1, :] for a in grid]
|
255 |
-
export_onnx(model, im, file, 12, dynamic, simplify) # opset 12
|
256 |
-
model.model[-1].anchor_grid = grid
|
257 |
-
else: # TensorRT >= 8
|
258 |
-
check_version(trt.__version__, '8.0.0', hard=True) # require tensorrt>=8.0.0
|
259 |
-
export_onnx(model, im, file, 12, dynamic, simplify) # opset 12
|
260 |
-
onnx = file.with_suffix('.onnx')
|
261 |
-
|
262 |
-
LOGGER.info(f'\n{prefix} starting export with TensorRT {trt.__version__}...')
|
263 |
-
assert onnx.exists(), f'failed to export ONNX file: {onnx}'
|
264 |
-
f = file.with_suffix('.engine') # TensorRT engine file
|
265 |
-
logger = trt.Logger(trt.Logger.INFO)
|
266 |
-
if verbose:
|
267 |
-
logger.min_severity = trt.Logger.Severity.VERBOSE
|
268 |
-
|
269 |
-
builder = trt.Builder(logger)
|
270 |
-
config = builder.create_builder_config()
|
271 |
-
config.max_workspace_size = workspace * 1 << 30
|
272 |
-
# config.set_memory_pool_limit(trt.MemoryPoolType.WORKSPACE, workspace << 30) # fix TRT 8.4 deprecation notice
|
273 |
-
|
274 |
-
flag = (1 << int(trt.NetworkDefinitionCreationFlag.EXPLICIT_BATCH))
|
275 |
-
network = builder.create_network(flag)
|
276 |
-
parser = trt.OnnxParser(network, logger)
|
277 |
-
if not parser.parse_from_file(str(onnx)):
|
278 |
-
raise RuntimeError(f'failed to load ONNX file: {onnx}')
|
279 |
-
|
280 |
-
inputs = [network.get_input(i) for i in range(network.num_inputs)]
|
281 |
-
outputs = [network.get_output(i) for i in range(network.num_outputs)]
|
282 |
-
for inp in inputs:
|
283 |
-
LOGGER.info(f'{prefix} input "{inp.name}" with shape{inp.shape} {inp.dtype}')
|
284 |
-
for out in outputs:
|
285 |
-
LOGGER.info(f'{prefix} output "{out.name}" with shape{out.shape} {out.dtype}')
|
286 |
-
|
287 |
-
if dynamic:
|
288 |
-
if im.shape[0] <= 1:
|
289 |
-
LOGGER.warning(f"{prefix} WARNING ⚠️ --dynamic model requires maximum --batch-size argument")
|
290 |
-
profile = builder.create_optimization_profile()
|
291 |
-
for inp in inputs:
|
292 |
-
profile.set_shape(inp.name, (1, *im.shape[1:]), (max(1, im.shape[0] // 2), *im.shape[1:]), im.shape)
|
293 |
-
config.add_optimization_profile(profile)
|
294 |
-
|
295 |
-
LOGGER.info(f'{prefix} building FP{16 if builder.platform_has_fast_fp16 and half else 32} engine as {f}')
|
296 |
-
if builder.platform_has_fast_fp16 and half:
|
297 |
-
config.set_flag(trt.BuilderFlag.FP16)
|
298 |
-
with builder.build_engine(network, config) as engine, open(f, 'wb') as t:
|
299 |
-
t.write(engine.serialize())
|
300 |
-
return f, None
|
301 |
-
|
302 |
-
|
303 |
-
@try_export
|
304 |
-
def export_saved_model(model,
|
305 |
-
im,
|
306 |
-
file,
|
307 |
-
dynamic,
|
308 |
-
tf_nms=False,
|
309 |
-
agnostic_nms=False,
|
310 |
-
topk_per_class=100,
|
311 |
-
topk_all=100,
|
312 |
-
iou_thres=0.45,
|
313 |
-
conf_thres=0.25,
|
314 |
-
keras=False,
|
315 |
-
prefix=colorstr('TensorFlow SavedModel:')):
|
316 |
-
# YOLOv5 TensorFlow SavedModel export
|
317 |
-
try:
|
318 |
-
import tensorflow as tf
|
319 |
-
except Exception:
|
320 |
-
check_requirements(f"tensorflow{'' if torch.cuda.is_available() else '-macos' if MACOS else '-cpu'}")
|
321 |
-
import tensorflow as tf
|
322 |
-
from tensorflow.python.framework.convert_to_constants import convert_variables_to_constants_v2
|
323 |
-
|
324 |
-
from models.tf import TFModel
|
325 |
-
|
326 |
-
LOGGER.info(f'\n{prefix} starting export with tensorflow {tf.__version__}...')
|
327 |
-
f = str(file).replace('.pt', '_saved_model')
|
328 |
-
batch_size, ch, *imgsz = list(im.shape) # BCHW
|
329 |
-
|
330 |
-
tf_model = TFModel(cfg=model.yaml, model=model, nc=model.nc, imgsz=imgsz)
|
331 |
-
im = tf.zeros((batch_size, *imgsz, ch)) # BHWC order for TensorFlow
|
332 |
-
_ = tf_model.predict(im, tf_nms, agnostic_nms, topk_per_class, topk_all, iou_thres, conf_thres)
|
333 |
-
inputs = tf.keras.Input(shape=(*imgsz, ch), batch_size=None if dynamic else batch_size)
|
334 |
-
outputs = tf_model.predict(inputs, tf_nms, agnostic_nms, topk_per_class, topk_all, iou_thres, conf_thres)
|
335 |
-
keras_model = tf.keras.Model(inputs=inputs, outputs=outputs)
|
336 |
-
keras_model.trainable = False
|
337 |
-
keras_model.summary()
|
338 |
-
if keras:
|
339 |
-
keras_model.save(f, save_format='tf')
|
340 |
-
else:
|
341 |
-
spec = tf.TensorSpec(keras_model.inputs[0].shape, keras_model.inputs[0].dtype)
|
342 |
-
m = tf.function(lambda x: keras_model(x)) # full model
|
343 |
-
m = m.get_concrete_function(spec)
|
344 |
-
frozen_func = convert_variables_to_constants_v2(m)
|
345 |
-
tfm = tf.Module()
|
346 |
-
tfm.__call__ = tf.function(lambda x: frozen_func(x)[:4] if tf_nms else frozen_func(x), [spec])
|
347 |
-
tfm.__call__(im)
|
348 |
-
tf.saved_model.save(tfm,
|
349 |
-
f,
|
350 |
-
options=tf.saved_model.SaveOptions(experimental_custom_gradients=False) if check_version(
|
351 |
-
tf.__version__, '2.6') else tf.saved_model.SaveOptions())
|
352 |
-
return f, keras_model
|
353 |
-
|
354 |
-
|
355 |
-
@try_export
|
356 |
-
def export_pb(keras_model, file, prefix=colorstr('TensorFlow GraphDef:')):
|
357 |
-
# YOLOv5 TensorFlow GraphDef *.pb export https://github.com/leimao/Frozen_Graph_TensorFlow
|
358 |
-
import tensorflow as tf
|
359 |
-
from tensorflow.python.framework.convert_to_constants import convert_variables_to_constants_v2
|
360 |
-
|
361 |
-
LOGGER.info(f'\n{prefix} starting export with tensorflow {tf.__version__}...')
|
362 |
-
f = file.with_suffix('.pb')
|
363 |
-
|
364 |
-
m = tf.function(lambda x: keras_model(x)) # full model
|
365 |
-
m = m.get_concrete_function(tf.TensorSpec(keras_model.inputs[0].shape, keras_model.inputs[0].dtype))
|
366 |
-
frozen_func = convert_variables_to_constants_v2(m)
|
367 |
-
frozen_func.graph.as_graph_def()
|
368 |
-
tf.io.write_graph(graph_or_graph_def=frozen_func.graph, logdir=str(f.parent), name=f.name, as_text=False)
|
369 |
-
return f, None
|
370 |
-
|
371 |
-
|
372 |
-
@try_export
|
373 |
-
def export_tflite(keras_model, im, file, int8, data, nms, agnostic_nms, prefix=colorstr('TensorFlow Lite:')):
|
374 |
-
# YOLOv5 TensorFlow Lite export
|
375 |
-
import tensorflow as tf
|
376 |
-
|
377 |
-
LOGGER.info(f'\n{prefix} starting export with tensorflow {tf.__version__}...')
|
378 |
-
batch_size, ch, *imgsz = list(im.shape) # BCHW
|
379 |
-
f = str(file).replace('.pt', '-fp16.tflite')
|
380 |
-
|
381 |
-
converter = tf.lite.TFLiteConverter.from_keras_model(keras_model)
|
382 |
-
converter.target_spec.supported_ops = [tf.lite.OpsSet.TFLITE_BUILTINS]
|
383 |
-
converter.target_spec.supported_types = [tf.float16]
|
384 |
-
converter.optimizations = [tf.lite.Optimize.DEFAULT]
|
385 |
-
if int8:
|
386 |
-
from models.tf import representative_dataset_gen
|
387 |
-
dataset = LoadImages(check_dataset(check_yaml(data))['train'], img_size=imgsz, auto=False)
|
388 |
-
converter.representative_dataset = lambda: representative_dataset_gen(dataset, ncalib=100)
|
389 |
-
converter.target_spec.supported_ops = [tf.lite.OpsSet.TFLITE_BUILTINS_INT8]
|
390 |
-
converter.target_spec.supported_types = []
|
391 |
-
converter.inference_input_type = tf.uint8 # or tf.int8
|
392 |
-
converter.inference_output_type = tf.uint8 # or tf.int8
|
393 |
-
converter.experimental_new_quantizer = True
|
394 |
-
f = str(file).replace('.pt', '-int8.tflite')
|
395 |
-
if nms or agnostic_nms:
|
396 |
-
converter.target_spec.supported_ops.append(tf.lite.OpsSet.SELECT_TF_OPS)
|
397 |
-
|
398 |
-
tflite_model = converter.convert()
|
399 |
-
open(f, "wb").write(tflite_model)
|
400 |
-
return f, None
|
401 |
-
|
402 |
-
|
403 |
-
@try_export
|
404 |
-
def export_edgetpu(file, prefix=colorstr('Edge TPU:')):
|
405 |
-
# YOLOv5 Edge TPU export https://coral.ai/docs/edgetpu/models-intro/
|
406 |
-
cmd = 'edgetpu_compiler --version'
|
407 |
-
help_url = 'https://coral.ai/docs/edgetpu/compiler/'
|
408 |
-
assert platform.system() == 'Linux', f'export only supported on Linux. See {help_url}'
|
409 |
-
if subprocess.run(f'{cmd} >/dev/null', shell=True).returncode != 0:
|
410 |
-
LOGGER.info(f'\n{prefix} export requires Edge TPU compiler. Attempting install from {help_url}')
|
411 |
-
sudo = subprocess.run('sudo --version >/dev/null', shell=True).returncode == 0 # sudo installed on system
|
412 |
-
for c in (
|
413 |
-
'curl https://packages.cloud.google.com/apt/doc/apt-key.gpg | sudo apt-key add -',
|
414 |
-
'echo "deb https://packages.cloud.google.com/apt coral-edgetpu-stable main" | sudo tee /etc/apt/sources.list.d/coral-edgetpu.list',
|
415 |
-
'sudo apt-get update', 'sudo apt-get install edgetpu-compiler'):
|
416 |
-
subprocess.run(c if sudo else c.replace('sudo ', ''), shell=True, check=True)
|
417 |
-
ver = subprocess.run(cmd, shell=True, capture_output=True, check=True).stdout.decode().split()[-1]
|
418 |
-
|
419 |
-
LOGGER.info(f'\n{prefix} starting export with Edge TPU compiler {ver}...')
|
420 |
-
f = str(file).replace('.pt', '-int8_edgetpu.tflite') # Edge TPU model
|
421 |
-
f_tfl = str(file).replace('.pt', '-int8.tflite') # TFLite model
|
422 |
-
|
423 |
-
cmd = f"edgetpu_compiler -s -d -k 10 --out_dir {file.parent} {f_tfl}"
|
424 |
-
subprocess.run(cmd.split(), check=True)
|
425 |
-
return f, None
|
426 |
-
|
427 |
-
|
428 |
-
@try_export
|
429 |
-
def export_tfjs(file, prefix=colorstr('TensorFlow.js:')):
|
430 |
-
# YOLOv5 TensorFlow.js export
|
431 |
-
check_requirements('tensorflowjs')
|
432 |
-
import tensorflowjs as tfjs
|
433 |
-
|
434 |
-
LOGGER.info(f'\n{prefix} starting export with tensorflowjs {tfjs.__version__}...')
|
435 |
-
f = str(file).replace('.pt', '_web_model') # js dir
|
436 |
-
f_pb = file.with_suffix('.pb') # *.pb path
|
437 |
-
f_json = f'{f}/model.json' # *.json path
|
438 |
-
|
439 |
-
cmd = f'tensorflowjs_converter --input_format=tf_frozen_model ' \
|
440 |
-
f'--output_node_names=Identity,Identity_1,Identity_2,Identity_3 {f_pb} {f}'
|
441 |
-
subprocess.run(cmd.split())
|
442 |
-
|
443 |
-
json = Path(f_json).read_text()
|
444 |
-
with open(f_json, 'w') as j: # sort JSON Identity_* in ascending order
|
445 |
-
subst = re.sub(
|
446 |
-
r'{"outputs": {"Identity.?.?": {"name": "Identity.?.?"}, '
|
447 |
-
r'"Identity.?.?": {"name": "Identity.?.?"}, '
|
448 |
-
r'"Identity.?.?": {"name": "Identity.?.?"}, '
|
449 |
-
r'"Identity.?.?": {"name": "Identity.?.?"}}}', r'{"outputs": {"Identity": {"name": "Identity"}, '
|
450 |
-
r'"Identity_1": {"name": "Identity_1"}, '
|
451 |
-
r'"Identity_2": {"name": "Identity_2"}, '
|
452 |
-
r'"Identity_3": {"name": "Identity_3"}}}', json)
|
453 |
-
j.write(subst)
|
454 |
-
return f, None
|
455 |
-
|
456 |
-
|
457 |
-
def add_tflite_metadata(file, metadata, num_outputs):
|
458 |
-
# Add metadata to *.tflite models per https://www.tensorflow.org/lite/models/convert/metadata
|
459 |
-
with contextlib.suppress(ImportError):
|
460 |
-
# check_requirements('tflite_support')
|
461 |
-
from tflite_support import flatbuffers
|
462 |
-
from tflite_support import metadata as _metadata
|
463 |
-
from tflite_support import metadata_schema_py_generated as _metadata_fb
|
464 |
-
|
465 |
-
tmp_file = Path('/tmp/meta.txt')
|
466 |
-
with open(tmp_file, 'w') as meta_f:
|
467 |
-
meta_f.write(str(metadata))
|
468 |
-
|
469 |
-
model_meta = _metadata_fb.ModelMetadataT()
|
470 |
-
label_file = _metadata_fb.AssociatedFileT()
|
471 |
-
label_file.name = tmp_file.name
|
472 |
-
model_meta.associatedFiles = [label_file]
|
473 |
-
|
474 |
-
subgraph = _metadata_fb.SubGraphMetadataT()
|
475 |
-
subgraph.inputTensorMetadata = [_metadata_fb.TensorMetadataT()]
|
476 |
-
subgraph.outputTensorMetadata = [_metadata_fb.TensorMetadataT()] * num_outputs
|
477 |
-
model_meta.subgraphMetadata = [subgraph]
|
478 |
-
|
479 |
-
b = flatbuffers.Builder(0)
|
480 |
-
b.Finish(model_meta.Pack(b), _metadata.MetadataPopulator.METADATA_FILE_IDENTIFIER)
|
481 |
-
metadata_buf = b.Output()
|
482 |
-
|
483 |
-
populator = _metadata.MetadataPopulator.with_model_file(file)
|
484 |
-
populator.load_metadata_buffer(metadata_buf)
|
485 |
-
populator.load_associated_files([str(tmp_file)])
|
486 |
-
populator.populate()
|
487 |
-
tmp_file.unlink()
|
488 |
-
|
489 |
-
|
490 |
-
@smart_inference_mode()
|
491 |
-
def run(
|
492 |
-
data=ROOT / 'data/coco128.yaml', # 'dataset.yaml path'
|
493 |
-
weights=ROOT / 'yolov5s.pt', # weights path
|
494 |
-
imgsz=(640, 640), # image (height, width)
|
495 |
-
batch_size=1, # batch size
|
496 |
-
device='cpu', # cuda device, i.e. 0 or 0,1,2,3 or cpu
|
497 |
-
include=('torchscript', 'onnx'), # include formats
|
498 |
-
half=False, # FP16 half-precision export
|
499 |
-
inplace=False, # set YOLOv5 Detect() inplace=True
|
500 |
-
keras=False, # use Keras
|
501 |
-
optimize=False, # TorchScript: optimize for mobile
|
502 |
-
int8=False, # CoreML/TF INT8 quantization
|
503 |
-
dynamic=False, # ONNX/TF/TensorRT: dynamic axes
|
504 |
-
simplify=False, # ONNX: simplify model
|
505 |
-
opset=12, # ONNX: opset version
|
506 |
-
verbose=False, # TensorRT: verbose log
|
507 |
-
workspace=4, # TensorRT: workspace size (GB)
|
508 |
-
nms=False, # TF: add NMS to model
|
509 |
-
agnostic_nms=False, # TF: add agnostic NMS to model
|
510 |
-
topk_per_class=100, # TF.js NMS: topk per class to keep
|
511 |
-
topk_all=100, # TF.js NMS: topk for all classes to keep
|
512 |
-
iou_thres=0.45, # TF.js NMS: IoU threshold
|
513 |
-
conf_thres=0.25, # TF.js NMS: confidence threshold
|
514 |
-
):
|
515 |
-
t = time.time()
|
516 |
-
include = [x.lower() for x in include] # to lowercase
|
517 |
-
fmts = tuple(export_formats()['Argument'][1:]) # --include arguments
|
518 |
-
flags = [x in include for x in fmts]
|
519 |
-
assert sum(flags) == len(include), f'ERROR: Invalid --include {include}, valid --include arguments are {fmts}'
|
520 |
-
jit, onnx, xml, engine, coreml, saved_model, pb, tflite, edgetpu, tfjs, paddle = flags # export booleans
|
521 |
-
file = Path(url2file(weights) if str(weights).startswith(('http:/', 'https:/')) else weights) # PyTorch weights
|
522 |
-
|
523 |
-
# Load PyTorch model
|
524 |
-
device = select_device(device)
|
525 |
-
if half:
|
526 |
-
assert device.type != 'cpu' or coreml, '--half only compatible with GPU export, i.e. use --device 0'
|
527 |
-
assert not dynamic, '--half not compatible with --dynamic, i.e. use either --half or --dynamic but not both'
|
528 |
-
model = attempt_load(weights, device=device, inplace=True, fuse=True) # load FP32 model
|
529 |
-
|
530 |
-
# Checks
|
531 |
-
imgsz *= 2 if len(imgsz) == 1 else 1 # expand
|
532 |
-
if optimize:
|
533 |
-
assert device.type == 'cpu', '--optimize not compatible with cuda devices, i.e. use --device cpu'
|
534 |
-
|
535 |
-
# Input
|
536 |
-
gs = int(max(model.stride)) # grid size (max stride)
|
537 |
-
imgsz = [check_img_size(x, gs) for x in imgsz] # verify img_size are gs-multiples
|
538 |
-
im = torch.zeros(batch_size, 3, *imgsz).to(device) # image size(1,3,320,192) BCHW iDetection
|
539 |
-
|
540 |
-
# Update model
|
541 |
-
model.eval()
|
542 |
-
for k, m in model.named_modules():
|
543 |
-
if isinstance(m, Detect):
|
544 |
-
m.inplace = inplace
|
545 |
-
m.dynamic = dynamic
|
546 |
-
m.export = True
|
547 |
-
|
548 |
-
for _ in range(2):
|
549 |
-
y = model(im) # dry runs
|
550 |
-
if half and not coreml:
|
551 |
-
im, model = im.half(), model.half() # to FP16
|
552 |
-
shape = tuple((y[0] if isinstance(y, tuple) else y).shape) # model output shape
|
553 |
-
metadata = {'stride': int(max(model.stride)), 'names': model.names} # model metadata
|
554 |
-
LOGGER.info(f"\n{colorstr('PyTorch:')} starting from {file} with output shape {shape} ({file_size(file):.1f} MB)")
|
555 |
-
|
556 |
-
# Exports
|
557 |
-
f = [''] * len(fmts) # exported filenames
|
558 |
-
warnings.filterwarnings(action='ignore', category=torch.jit.TracerWarning) # suppress TracerWarning
|
559 |
-
if jit: # TorchScript
|
560 |
-
f[0], _ = export_torchscript(model, im, file, optimize)
|
561 |
-
if engine: # TensorRT required before ONNX
|
562 |
-
f[1], _ = export_engine(model, im, file, half, dynamic, simplify, workspace, verbose)
|
563 |
-
if onnx or xml: # OpenVINO requires ONNX
|
564 |
-
f[2], _ = export_onnx(model, im, file, opset, dynamic, simplify)
|
565 |
-
if xml: # OpenVINO
|
566 |
-
f[3], _ = export_openvino(file, metadata, half)
|
567 |
-
if coreml: # CoreML
|
568 |
-
f[4], _ = export_coreml(model, im, file, int8, half)
|
569 |
-
if any((saved_model, pb, tflite, edgetpu, tfjs)): # TensorFlow formats
|
570 |
-
assert not tflite or not tfjs, 'TFLite and TF.js models must be exported separately, please pass only one type.'
|
571 |
-
assert not isinstance(model, ClassificationModel), 'ClassificationModel export to TF formats not yet supported.'
|
572 |
-
f[5], s_model = export_saved_model(model.cpu(),
|
573 |
-
im,
|
574 |
-
file,
|
575 |
-
dynamic,
|
576 |
-
tf_nms=nms or agnostic_nms or tfjs,
|
577 |
-
agnostic_nms=agnostic_nms or tfjs,
|
578 |
-
topk_per_class=topk_per_class,
|
579 |
-
topk_all=topk_all,
|
580 |
-
iou_thres=iou_thres,
|
581 |
-
conf_thres=conf_thres,
|
582 |
-
keras=keras)
|
583 |
-
if pb or tfjs: # pb prerequisite to tfjs
|
584 |
-
f[6], _ = export_pb(s_model, file)
|
585 |
-
if tflite or edgetpu:
|
586 |
-
f[7], _ = export_tflite(s_model, im, file, int8 or edgetpu, data=data, nms=nms, agnostic_nms=agnostic_nms)
|
587 |
-
if edgetpu:
|
588 |
-
f[8], _ = export_edgetpu(file)
|
589 |
-
add_tflite_metadata(f[8] or f[7], metadata, num_outputs=len(s_model.outputs))
|
590 |
-
if tfjs:
|
591 |
-
f[9], _ = export_tfjs(file)
|
592 |
-
if paddle: # PaddlePaddle
|
593 |
-
f[10], _ = export_paddle(model, im, file, metadata)
|
594 |
-
|
595 |
-
# Finish
|
596 |
-
f = [str(x) for x in f if x] # filter out '' and None
|
597 |
-
if any(f):
|
598 |
-
cls, det, seg = (isinstance(model, x) for x in (ClassificationModel, DetectionModel, SegmentationModel)) # type
|
599 |
-
dir = Path('segment' if seg else 'classify' if cls else '')
|
600 |
-
h = '--half' if half else '' # --half FP16 inference arg
|
601 |
-
s = "# WARNING ⚠️ ClassificationModel not yet supported for PyTorch Hub AutoShape inference" if cls else \
|
602 |
-
"# WARNING ⚠️ SegmentationModel not yet supported for PyTorch Hub AutoShape inference" if seg else ''
|
603 |
-
LOGGER.info(f'\nExport complete ({time.time() - t:.1f}s)'
|
604 |
-
f"\nResults saved to {colorstr('bold', file.parent.resolve())}"
|
605 |
-
f"\nDetect: python {dir / ('detect.py' if det else 'predict.py')} --weights {f[-1]} {h}"
|
606 |
-
f"\nValidate: python {dir / 'val.py'} --weights {f[-1]} {h}"
|
607 |
-
f"\nPyTorch Hub: model = torch.hub.load('ultralytics/yolov5', 'custom', '{f[-1]}') {s}"
|
608 |
-
f"\nVisualize: https://netron.app")
|
609 |
-
return f # return list of exported files/dirs
|
610 |
-
|
611 |
-
|
612 |
-
def parse_opt():
|
613 |
-
parser = argparse.ArgumentParser()
|
614 |
-
parser.add_argument('--data', type=str, default=ROOT / 'data/coco128.yaml', help='dataset.yaml path')
|
615 |
-
parser.add_argument('--weights', nargs='+', type=str, default=ROOT / 'yolov5s.pt', help='model.pt path(s)')
|
616 |
-
parser.add_argument('--imgsz', '--img', '--img-size', nargs='+', type=int, default=[640, 640], help='image (h, w)')
|
617 |
-
parser.add_argument('--batch-size', type=int, default=1, help='batch size')
|
618 |
-
parser.add_argument('--device', default='cpu', help='cuda device, i.e. 0 or 0,1,2,3 or cpu')
|
619 |
-
parser.add_argument('--half', action='store_true', help='FP16 half-precision export')
|
620 |
-
parser.add_argument('--inplace', action='store_true', help='set YOLOv5 Detect() inplace=True')
|
621 |
-
parser.add_argument('--keras', action='store_true', help='TF: use Keras')
|
622 |
-
parser.add_argument('--optimize', action='store_true', help='TorchScript: optimize for mobile')
|
623 |
-
parser.add_argument('--int8', action='store_true', help='CoreML/TF INT8 quantization')
|
624 |
-
parser.add_argument('--dynamic', action='store_true', help='ONNX/TF/TensorRT: dynamic axes')
|
625 |
-
parser.add_argument('--simplify', action='store_true', help='ONNX: simplify model')
|
626 |
-
parser.add_argument('--opset', type=int, default=12, help='ONNX: opset version')
|
627 |
-
parser.add_argument('--verbose', action='store_true', help='TensorRT: verbose log')
|
628 |
-
parser.add_argument('--workspace', type=int, default=4, help='TensorRT: workspace size (GB)')
|
629 |
-
parser.add_argument('--nms', action='store_true', help='TF: add NMS to model')
|
630 |
-
parser.add_argument('--agnostic-nms', action='store_true', help='TF: add agnostic NMS to model')
|
631 |
-
parser.add_argument('--topk-per-class', type=int, default=100, help='TF.js NMS: topk per class to keep')
|
632 |
-
parser.add_argument('--topk-all', type=int, default=100, help='TF.js NMS: topk for all classes to keep')
|
633 |
-
parser.add_argument('--iou-thres', type=float, default=0.45, help='TF.js NMS: IoU threshold')
|
634 |
-
parser.add_argument('--conf-thres', type=float, default=0.25, help='TF.js NMS: confidence threshold')
|
635 |
-
parser.add_argument(
|
636 |
-
'--include',
|
637 |
-
nargs='+',
|
638 |
-
default=['torchscript'],
|
639 |
-
help='torchscript, onnx, openvino, engine, coreml, saved_model, pb, tflite, edgetpu, tfjs, paddle')
|
640 |
-
opt = parser.parse_args()
|
641 |
-
print_args(vars(opt))
|
642 |
-
return opt
|
643 |
-
|
644 |
-
|
645 |
-
def main(opt):
|
646 |
-
for opt.weights in (opt.weights if isinstance(opt.weights, list) else [opt.weights]):
|
647 |
-
run(**vars(opt))
|
648 |
-
|
649 |
-
|
650 |
-
if __name__ == "__main__":
|
651 |
-
opt = parse_opt()
|
652 |
-
main(opt)
|
|
|
|
|
|
|
|
|
|
|
|
|
|
|
|
|
|
|
|
|
|
|
|
|
|
|
|
|
|
|
|
|
|
|
|
|
|
|
|
|
|
|
|
|
|
|
|
|
|
|
|
|
|
|
|
|
|
|
|
|
|
|
|
|
|
|
|
|
|
|
|
|
|
|
|
|
|
|
|
|
|
|
|
|
|
|
|
|
|
|
|
|
|
|
|
|
|
|
|
|
|
|
|
|
|
|
|
|
|
|
|
|
|
|
|
|
|
|
|
|
|
|
|
|
|
|
|
|
|
|
|
|
|
|
|
|
|
|
|
|
|
|
|
|
|
|
|
|
|
|
|
|
|
|
|
|
|
|
|
|
|
|
|
|
|
|
|
|
|
|
|
|
|
|
|
|
|
|
|
|
|
|
|
|
|
|
|
|
|
|
|
|
|
|
|
|
|
|
|
|
|
|
|
|
|
|
|
|
|
|
|
|
|
|
|
|
|
|
|
|
|
|
|
|
|
|
|
|
|
|
|
|
|
|
|
|
|
|
|
|
|
|
|
|
|
|
|
|
|
|
|
|
|
|
|
|
|
|
|
|
|
|
|
|
|
|
|
|
|
|
|
|
|
|
|
|
|
|
|
|
|
|
|
|
|
|
|
|
|
|
|
|
|
|
|
|
|
|
|
|
|
|
|
|
|
|
|
|
|
|
|
|
|
|
|
|
|
|
|
|
|
|
|
|
|
|
|
|
|
|
|
|
|
|
|
|
|
|
|
|
|
|
|
|
|
|
|
|
|
|
|
|
|
|
|
|
|
|
|
|
|
|
|
|
|
|
|
|
|
|
|
|
|
|
|
|
|
|
|
|
|
|
|
|
|
|
|
|
|
|
|
|
|
|
|
|
|
|
|
|
|
|
|
|
|
|
|
|
|
|
|
|
|
|
|
|
|
|
|
|
|
|
|
|
|
|
|
|
|
|
|
|
|
|
|
|
|
|
|
|
|
|
|
|
|
|
|
|
|
|
|
|
|
|
|
|
|
|
|
|
|
|
|
|
|
|
|
|
|
|
|
|
|
|
|
|
|
|
|
|
|
|
|
|
|
|
|
|
|
|
|
|
|
|
|
|
|
|
|
|
|
|
|
|
|
|
|
|
|
|
|
|
|
|
|
|
|
|
|
|
|
|
|
|
|
|
|
|
|
|
|
|
|
|
|
|
|
|
|
|
|
|
|
|
|
|
|
|
|
|
|
|
|
|
|
|
|
|
|
|
|
|
|
|
|
|
|
|
|
|
|
|
|
|
|
|
|
|
|
|
|
|
|
|
|
|
|
|
|
|
|
|
|
|
|
|
|
|
|
|
|
|
|
|
|
|
|
|
|
|
|
|
|
|
|
|
|
|
|
|
|
|
|
|
|
|
|
|
|
|
|
|
|
|
|
|
|
|
|
|
|
|
|
|
|
|
|
|
|
|
|
|
|
|
|
|
|
|
|
|
|
|
|
|
|
|
|
|
|
|
|
|
|
|
|
|
|
|
|
|
|
|
|
|
|
|
|
|
|
|
|
|
|
|
|
|
|
|
|
|
|
|
|
|
|
|
|
|
|
|
|
|
|
|
|
|
|
|
|
|
|
|
|
|
|
|
|
|
|
|
|
|
|
|
|
|
|
|
|
|
|
|
|
|
|
|
|
|
|
|
|
|
|
|
|
|
|
|
|
|
|
|
|
|
|
|
|
|
|
|
|
|
|
|
|
|
|
|
|
|
|
|
|
|
|
|
|
|
|
|
|
|
|
|
|
|
|
|
|
|
|
|
|
|
|
|
|
|
|
|
|
|
|
|
|
|
|
|
|
|
|
|
|
|
|
|
|
|
|
|
|
|
|
|
|
|
|
|
|
|
|
|
|
|
|
|
|
|
|
|
|
|
|
|
|
|
|
|
|
|
|
|
|
|
|
|
|
|
|
|
|
|
|
|
|
|
|
|
|
|
|
|
|
|
|
|
|
|
|
|
|
|
|
|
|
|
|
|
|
|
|
|
|
|
|
|
|
|
|
|
|
|
|
|
|
|
|
|
|
|
|
|
|
|
|
|
|
|
|
|
|
|
|
|
|
|
|
|
|
|
|
|
|
|
|
|
|
|
|
|
|
|
|
|
|
|
|
|
|
|
|
|
|
|
|
|
|
|
|
|
|
|
|
|
|
|
|
|
|
|
|
|
|
|
|
|
|
|
|
|
|
|
|
|
|
|
|
|
|
|
|
|
|
|
|
|
|
|
|
|
|
|
|
|
|
|
|
|
|
|
|
|
|
|
|
|
|
|
|
|
|
|
|
|
|
|
|
|
|
|
|
|
|
|
|
|
|
|
|
|
|
|
|
|
|
|
|
|
|
|
|
|
|
|
|
|
|
|
|
|
|
|
|
|
|
|
|
|
|
|
|
|
|
|
|
|
|
|
|
|
|
|
|
|
|
|
|
|
|
|
|
|
|
|
|
|
|
|
|
|
|
|
|
|
|
|
|
|
|
|
|
|
|
|
|
|
|
|
|
|
|
|
|
|
|
|
|
|
|
|
|
|
|
|
|
|
|
|
|
|
|
|
|
|
|
|
|
|
|
|
|
|
|
|
|
|
|
|
|
|
|
|
|
|
|
|
|
|
|
|
|
|
|
|
|
|
|
|
|
|
|
|
|
|
|
|
|
|
|
|
|
|
|
|
|
|
|
|
|
|
|
|
|
|
|
|
|
|
|
|
|
|
|
|
|
|
|
|
|
|
|
|
|
|
|
|
|
|
|
|
|
|
|
|
|
|
|
|
|
|
|
|
|
|
|
|
|
|
|
|
|
|
|
|
|
|
|
|
|
|
|
|
|
|
|
|
|
|
|
|
|
|
|
|
|
|
|
|
|
|
|
|
|
|
|
|
|
|
|
|
|
|
|
|
|
spaces/Artples/Named-Entity-Recognition/README.md
DELETED
@@ -1,13 +0,0 @@
|
|
1 |
-
---
|
2 |
-
title: Named Entity Recognition
|
3 |
-
emoji: 📊
|
4 |
-
colorFrom: purple
|
5 |
-
colorTo: gray
|
6 |
-
sdk: gradio
|
7 |
-
sdk_version: 3.24.1
|
8 |
-
app_file: app.py
|
9 |
-
pinned: false
|
10 |
-
license: apache-2.0
|
11 |
-
---
|
12 |
-
|
13 |
-
Check out the configuration reference at https://huggingface.co/docs/hub/spaces-config-reference
|
|
|
|
|
|
|
|
|
|
|
|
|
|
|
|
|
|
|
|
|
|
|
|
|
|
|
|
spaces/Ataturk-Chatbot/HuggingFaceChat/venv/lib/python3.11/site-packages/pip/_vendor/chardet/langhungarianmodel.py
DELETED
The diff for this file is too large to render.
See raw diff
|
|
spaces/Awesimo/jojogan/util.py
DELETED
@@ -1,220 +0,0 @@
|
|
1 |
-
from matplotlib import pyplot as plt
|
2 |
-
import torch
|
3 |
-
import torch.nn.functional as F
|
4 |
-
import os
|
5 |
-
import cv2
|
6 |
-
import dlib
|
7 |
-
from PIL import Image
|
8 |
-
import numpy as np
|
9 |
-
import math
|
10 |
-
import torchvision
|
11 |
-
import scipy
|
12 |
-
import scipy.ndimage
|
13 |
-
import torchvision.transforms as transforms
|
14 |
-
|
15 |
-
from huggingface_hub import hf_hub_download
|
16 |
-
|
17 |
-
|
18 |
-
shape_predictor_path = hf_hub_download(repo_id="akhaliq/jojogan_dlib", filename="shape_predictor_68_face_landmarks.dat")
|
19 |
-
|
20 |
-
|
21 |
-
google_drive_paths = {
|
22 |
-
"models/stylegan2-ffhq-config-f.pt": "https://drive.google.com/uc?id=1Yr7KuD959btpmcKGAUsbAk5rPjX2MytK",
|
23 |
-
"models/dlibshape_predictor_68_face_landmarks.dat": "https://drive.google.com/uc?id=11BDmNKS1zxSZxkgsEvQoKgFd8J264jKp",
|
24 |
-
"models/e4e_ffhq_encode.pt": "https://drive.google.com/uc?id=1o6ijA3PkcewZvwJJ73dJ0fxhndn0nnh7",
|
25 |
-
"models/restyle_psp_ffhq_encode.pt": "https://drive.google.com/uc?id=1nbxCIVw9H3YnQsoIPykNEFwWJnHVHlVd",
|
26 |
-
"models/arcane_caitlyn.pt": "https://drive.google.com/uc?id=1gOsDTiTPcENiFOrhmkkxJcTURykW1dRc",
|
27 |
-
"models/arcane_caitlyn_preserve_color.pt": "https://drive.google.com/uc?id=1cUTyjU-q98P75a8THCaO545RTwpVV-aH",
|
28 |
-
"models/arcane_jinx_preserve_color.pt": "https://drive.google.com/uc?id=1jElwHxaYPod5Itdy18izJk49K1nl4ney",
|
29 |
-
"models/arcane_jinx.pt": "https://drive.google.com/uc?id=1quQ8vPjYpUiXM4k1_KIwP4EccOefPpG_",
|
30 |
-
"models/disney.pt": "https://drive.google.com/uc?id=1zbE2upakFUAx8ximYnLofFwfT8MilqJA",
|
31 |
-
"models/disney_preserve_color.pt": "https://drive.google.com/uc?id=1Bnh02DjfvN_Wm8c4JdOiNV4q9J7Z_tsi",
|
32 |
-
"models/jojo.pt": "https://drive.google.com/uc?id=13cR2xjIBj8Ga5jMO7gtxzIJj2PDsBYK4",
|
33 |
-
"models/jojo_preserve_color.pt": "https://drive.google.com/uc?id=1ZRwYLRytCEKi__eT2Zxv1IlV6BGVQ_K2",
|
34 |
-
"models/jojo_yasuho.pt": "https://drive.google.com/uc?id=1grZT3Gz1DLzFoJchAmoj3LoM9ew9ROX_",
|
35 |
-
"models/jojo_yasuho_preserve_color.pt": "https://drive.google.com/uc?id=1SKBu1h0iRNyeKBnya_3BBmLr4pkPeg_L",
|
36 |
-
"models/supergirl.pt": "https://drive.google.com/uc?id=1L0y9IYgzLNzB-33xTpXpecsKU-t9DpVC",
|
37 |
-
"models/supergirl_preserve_color.pt": "https://drive.google.com/uc?id=1VmKGuvThWHym7YuayXxjv0fSn32lfDpE",
|
38 |
-
}
|
39 |
-
|
40 |
-
@torch.no_grad()
|
41 |
-
def load_model(generator, model_file_path):
|
42 |
-
ensure_checkpoint_exists(model_file_path)
|
43 |
-
ckpt = torch.load(model_file_path, map_location=lambda storage, loc: storage)
|
44 |
-
generator.load_state_dict(ckpt["g_ema"], strict=False)
|
45 |
-
return generator.mean_latent(50000)
|
46 |
-
|
47 |
-
def ensure_checkpoint_exists(model_weights_filename):
|
48 |
-
if not os.path.isfile(model_weights_filename) and (
|
49 |
-
model_weights_filename in google_drive_paths
|
50 |
-
):
|
51 |
-
gdrive_url = google_drive_paths[model_weights_filename]
|
52 |
-
try:
|
53 |
-
from gdown import download as drive_download
|
54 |
-
|
55 |
-
drive_download(gdrive_url, model_weights_filename, quiet=False)
|
56 |
-
except ModuleNotFoundError:
|
57 |
-
print(
|
58 |
-
"gdown module not found.",
|
59 |
-
"pip3 install gdown or, manually download the checkpoint file:",
|
60 |
-
gdrive_url
|
61 |
-
)
|
62 |
-
|
63 |
-
if not os.path.isfile(model_weights_filename) and (
|
64 |
-
model_weights_filename not in google_drive_paths
|
65 |
-
):
|
66 |
-
print(
|
67 |
-
model_weights_filename,
|
68 |
-
" not found, you may need to manually download the model weights."
|
69 |
-
)
|
70 |
-
|
71 |
-
# given a list of filenames, load the inverted style code
|
72 |
-
@torch.no_grad()
|
73 |
-
def load_source(files, generator, device='cuda'):
|
74 |
-
sources = []
|
75 |
-
|
76 |
-
for file in files:
|
77 |
-
source = torch.load(f'./inversion_codes/{file}.pt')['latent'].to(device)
|
78 |
-
|
79 |
-
if source.size(0) != 1:
|
80 |
-
source = source.unsqueeze(0)
|
81 |
-
|
82 |
-
if source.ndim == 3:
|
83 |
-
source = generator.get_latent(source, truncation=1, is_latent=True)
|
84 |
-
source = list2style(source)
|
85 |
-
|
86 |
-
sources.append(source)
|
87 |
-
|
88 |
-
sources = torch.cat(sources, 0)
|
89 |
-
if type(sources) is not list:
|
90 |
-
sources = style2list(sources)
|
91 |
-
|
92 |
-
return sources
|
93 |
-
|
94 |
-
def display_image(image, size=None, mode='nearest', unnorm=False, title=''):
|
95 |
-
# image is [3,h,w] or [1,3,h,w] tensor [0,1]
|
96 |
-
if not isinstance(image, torch.Tensor):
|
97 |
-
image = transforms.ToTensor()(image).unsqueeze(0)
|
98 |
-
if image.is_cuda:
|
99 |
-
image = image.cpu()
|
100 |
-
if size is not None and image.size(-1) != size:
|
101 |
-
image = F.interpolate(image, size=(size,size), mode=mode)
|
102 |
-
if image.dim() == 4:
|
103 |
-
image = image[0]
|
104 |
-
image = image.permute(1, 2, 0).detach().numpy()
|
105 |
-
plt.figure()
|
106 |
-
plt.title(title)
|
107 |
-
plt.axis('off')
|
108 |
-
plt.imshow(image)
|
109 |
-
|
110 |
-
def get_landmark(filepath, predictor):
|
111 |
-
"""get landmark with dlib
|
112 |
-
:return: np.array shape=(68, 2)
|
113 |
-
"""
|
114 |
-
detector = dlib.get_frontal_face_detector()
|
115 |
-
|
116 |
-
img = dlib.load_rgb_image(filepath)
|
117 |
-
dets = detector(img, 1)
|
118 |
-
assert len(dets) > 0, "Face not detected, try another face image"
|
119 |
-
|
120 |
-
for k, d in enumerate(dets):
|
121 |
-
shape = predictor(img, d)
|
122 |
-
|
123 |
-
t = list(shape.parts())
|
124 |
-
a = []
|
125 |
-
for tt in t:
|
126 |
-
a.append([tt.x, tt.y])
|
127 |
-
lm = np.array(a)
|
128 |
-
return lm
|
129 |
-
|
130 |
-
|
131 |
-
def align_face(filepath, output_size=256, transform_size=1024, enable_padding=True):
|
132 |
-
|
133 |
-
"""
|
134 |
-
:param filepath: str
|
135 |
-
:return: PIL Image
|
136 |
-
"""
|
137 |
-
predictor = dlib.shape_predictor(shape_predictor_path)
|
138 |
-
lm = get_landmark(filepath, predictor)
|
139 |
-
|
140 |
-
lm_chin = lm[0: 17] # left-right
|
141 |
-
lm_eyebrow_left = lm[17: 22] # left-right
|
142 |
-
lm_eyebrow_right = lm[22: 27] # left-right
|
143 |
-
lm_nose = lm[27: 31] # top-down
|
144 |
-
lm_nostrils = lm[31: 36] # top-down
|
145 |
-
lm_eye_left = lm[36: 42] # left-clockwise
|
146 |
-
lm_eye_right = lm[42: 48] # left-clockwise
|
147 |
-
lm_mouth_outer = lm[48: 60] # left-clockwise
|
148 |
-
lm_mouth_inner = lm[60: 68] # left-clockwise
|
149 |
-
|
150 |
-
# Calculate auxiliary vectors.
|
151 |
-
eye_left = np.mean(lm_eye_left, axis=0)
|
152 |
-
eye_right = np.mean(lm_eye_right, axis=0)
|
153 |
-
eye_avg = (eye_left + eye_right) * 0.5
|
154 |
-
eye_to_eye = eye_right - eye_left
|
155 |
-
mouth_left = lm_mouth_outer[0]
|
156 |
-
mouth_right = lm_mouth_outer[6]
|
157 |
-
mouth_avg = (mouth_left + mouth_right) * 0.5
|
158 |
-
eye_to_mouth = mouth_avg - eye_avg
|
159 |
-
|
160 |
-
# Choose oriented crop rectangle.
|
161 |
-
x = eye_to_eye - np.flipud(eye_to_mouth) * [-1, 1]
|
162 |
-
x /= np.hypot(*x)
|
163 |
-
x *= max(np.hypot(*eye_to_eye) * 2.0, np.hypot(*eye_to_mouth) * 1.8)
|
164 |
-
y = np.flipud(x) * [-1, 1]
|
165 |
-
c = eye_avg + eye_to_mouth * 0.1
|
166 |
-
quad = np.stack([c - x - y, c - x + y, c + x + y, c + x - y])
|
167 |
-
qsize = np.hypot(*x) * 2
|
168 |
-
|
169 |
-
# read image
|
170 |
-
img = Image.open(filepath)
|
171 |
-
|
172 |
-
transform_size = output_size
|
173 |
-
enable_padding = True
|
174 |
-
|
175 |
-
# Shrink.
|
176 |
-
shrink = int(np.floor(qsize / output_size * 0.5))
|
177 |
-
if shrink > 1:
|
178 |
-
rsize = (int(np.rint(float(img.size[0]) / shrink)), int(np.rint(float(img.size[1]) / shrink)))
|
179 |
-
img = img.resize(rsize, Image.ANTIALIAS)
|
180 |
-
quad /= shrink
|
181 |
-
qsize /= shrink
|
182 |
-
|
183 |
-
# Crop.
|
184 |
-
border = max(int(np.rint(qsize * 0.1)), 3)
|
185 |
-
crop = (int(np.floor(min(quad[:, 0]))), int(np.floor(min(quad[:, 1]))), int(np.ceil(max(quad[:, 0]))),
|
186 |
-
int(np.ceil(max(quad[:, 1]))))
|
187 |
-
crop = (max(crop[0] - border, 0), max(crop[1] - border, 0), min(crop[2] + border, img.size[0]),
|
188 |
-
min(crop[3] + border, img.size[1]))
|
189 |
-
if crop[2] - crop[0] < img.size[0] or crop[3] - crop[1] < img.size[1]:
|
190 |
-
img = img.crop(crop)
|
191 |
-
quad -= crop[0:2]
|
192 |
-
|
193 |
-
# Pad.
|
194 |
-
pad = (int(np.floor(min(quad[:, 0]))), int(np.floor(min(quad[:, 1]))), int(np.ceil(max(quad[:, 0]))),
|
195 |
-
int(np.ceil(max(quad[:, 1]))))
|
196 |
-
pad = (max(-pad[0] + border, 0), max(-pad[1] + border, 0), max(pad[2] - img.size[0] + border, 0),
|
197 |
-
max(pad[3] - img.size[1] + border, 0))
|
198 |
-
if enable_padding and max(pad) > border - 4:
|
199 |
-
pad = np.maximum(pad, int(np.rint(qsize * 0.3)))
|
200 |
-
img = np.pad(np.float32(img), ((pad[1], pad[3]), (pad[0], pad[2]), (0, 0)), 'reflect')
|
201 |
-
h, w, _ = img.shape
|
202 |
-
y, x, _ = np.ogrid[:h, :w, :1]
|
203 |
-
mask = np.maximum(1.0 - np.minimum(np.float32(x) / pad[0], np.float32(w - 1 - x) / pad[2]),
|
204 |
-
1.0 - np.minimum(np.float32(y) / pad[1], np.float32(h - 1 - y) / pad[3]))
|
205 |
-
blur = qsize * 0.02
|
206 |
-
img += (scipy.ndimage.gaussian_filter(img, [blur, blur, 0]) - img) * np.clip(mask * 3.0 + 1.0, 0.0, 1.0)
|
207 |
-
img += (np.median(img, axis=(0, 1)) - img) * np.clip(mask, 0.0, 1.0)
|
208 |
-
img = Image.fromarray(np.uint8(np.clip(np.rint(img), 0, 255)), 'RGB')
|
209 |
-
quad += pad[:2]
|
210 |
-
|
211 |
-
# Transform.
|
212 |
-
img = img.transform((transform_size, transform_size), Image.QUAD, (quad + 0.5).flatten(), Image.BILINEAR)
|
213 |
-
if output_size < transform_size:
|
214 |
-
img = img.resize((output_size, output_size), Image.ANTIALIAS)
|
215 |
-
|
216 |
-
# Return aligned image.
|
217 |
-
return img
|
218 |
-
|
219 |
-
def strip_path_extension(path):
|
220 |
-
return os.path.splitext(path)[0]
|
|
|
|
|
|
|
|
|
|
|
|
|
|
|
|
|
|
|
|
|
|
|
|
|
|
|
|
|
|
|
|
|
|
|
|
|
|
|
|
|
|
|
|
|
|
|
|
|
|
|
|
|
|
|
|
|
|
|
|
|
|
|
|
|
|
|
|
|
|
|
|
|
|
|
|
|
|
|
|
|
|
|
|
|
|
|
|
|
|
|
|
|
|
|
|
|
|
|
|
|
|
|
|
|
|
|
|
|
|
|
|
|
|
|
|
|
|
|
|
|
|
|
|
|
|
|
|
|
|
|
|
|
|
|
|
|
|
|
|
|
|
|
|
|
|
|
|
|
|
|
|
|
|
|
|
|
|
|
|
|
|
|
|
|
|
|
|
|
|
|
|
|
|
|
|
|
|
|
|
|
|
|
|
|
|
|
|
|
|
|
|
|
|
|
|
|
|
|
|
|
|
|
|
|
|
|
|
|
|
|
|
|
|
|
|
|
|
|
|
|
|
|
|
|
|
|
|
|
|
|
|
|
|
|
|
|
|
|
|
|
|
|
|
|
|
|
|
|
|
|
|
|
|
|
|
|
|
|
|
|
|
|
|
|
|
|
|
|
|
|
|
|
|
|
|
|
|
|
|
|
|
|
|
|
|
|
|
|
|
|
|
|
|
|
|
|
|
|
|
|
|
|
|
|
|
|
|
|
|
|
|
|
|
|
|
|
|
|
|
|
|
|
|
|
|
|
|
|
|
|
|
|
|
|
|
|
|
|
|
|
|
|
|
|
|
|
|
|
|
|
|
|
|
|
|
|
|
|
|
|
|
|
|
|
|
|
|
|
|
|
|
|
|
|
|
|
|
|
|
|
|
|
|
|
|
|
|
|
|
|
|
|
|
|
|
|
|
|
|
|
|
|
|
|
|
|
|
|
|
|
|
|
|
|
|
|
|
|
|
|
|
|
|
|
|
|
|
|
|
|
|
|
|
|
|
|
|
|
|
|
|
spaces/Bart92/RVC_HF/infer/modules/train/preprocess.py
DELETED
@@ -1,147 +0,0 @@
|
|
1 |
-
import multiprocessing
|
2 |
-
import os
|
3 |
-
import sys
|
4 |
-
|
5 |
-
from scipy import signal
|
6 |
-
|
7 |
-
now_dir = os.getcwd()
|
8 |
-
sys.path.append(now_dir)
|
9 |
-
print(sys.argv)
|
10 |
-
inp_root = sys.argv[1]
|
11 |
-
sr = int(sys.argv[2])
|
12 |
-
n_p = int(sys.argv[3])
|
13 |
-
exp_dir = sys.argv[4]
|
14 |
-
noparallel = sys.argv[5] == "True"
|
15 |
-
per = float(sys.argv[6])
|
16 |
-
import multiprocessing
|
17 |
-
import os
|
18 |
-
import traceback
|
19 |
-
|
20 |
-
import librosa
|
21 |
-
import numpy as np
|
22 |
-
from scipy.io import wavfile
|
23 |
-
|
24 |
-
from infer.lib.audio import load_audio
|
25 |
-
from infer.lib.slicer2 import Slicer
|
26 |
-
|
27 |
-
mutex = multiprocessing.Lock()
|
28 |
-
f = open("%s/preprocess.log" % exp_dir, "a+")
|
29 |
-
|
30 |
-
|
31 |
-
def println(strr):
|
32 |
-
mutex.acquire()
|
33 |
-
print(strr)
|
34 |
-
f.write("%s\n" % strr)
|
35 |
-
f.flush()
|
36 |
-
mutex.release()
|
37 |
-
|
38 |
-
|
39 |
-
class PreProcess:
|
40 |
-
def __init__(self, sr, exp_dir, per=3.7):
|
41 |
-
self.slicer = Slicer(
|
42 |
-
sr=sr,
|
43 |
-
threshold=-42,
|
44 |
-
min_length=1500,
|
45 |
-
min_interval=400,
|
46 |
-
hop_size=15,
|
47 |
-
max_sil_kept=500,
|
48 |
-
)
|
49 |
-
self.sr = sr
|
50 |
-
self.bh, self.ah = signal.butter(N=5, Wn=48, btype="high", fs=self.sr)
|
51 |
-
self.per = per
|
52 |
-
self.overlap = 0.3
|
53 |
-
self.tail = self.per + self.overlap
|
54 |
-
self.max = 0.9
|
55 |
-
self.alpha = 0.75
|
56 |
-
self.exp_dir = exp_dir
|
57 |
-
self.gt_wavs_dir = "%s/0_gt_wavs" % exp_dir
|
58 |
-
self.wavs16k_dir = "%s/1_16k_wavs" % exp_dir
|
59 |
-
os.makedirs(self.exp_dir, exist_ok=True)
|
60 |
-
os.makedirs(self.gt_wavs_dir, exist_ok=True)
|
61 |
-
os.makedirs(self.wavs16k_dir, exist_ok=True)
|
62 |
-
|
63 |
-
def norm_write(self, tmp_audio, idx0, idx1):
|
64 |
-
tmp_max = np.abs(tmp_audio).max()
|
65 |
-
if tmp_max > 2.5:
|
66 |
-
print("%s-%s-%s-filtered" % (idx0, idx1, tmp_max))
|
67 |
-
return
|
68 |
-
tmp_audio = (tmp_audio / tmp_max * (self.max * self.alpha)) + (
|
69 |
-
1 - self.alpha
|
70 |
-
) * tmp_audio
|
71 |
-
wavfile.write(
|
72 |
-
"%s/%s_%s.wav" % (self.gt_wavs_dir, idx0, idx1),
|
73 |
-
self.sr,
|
74 |
-
tmp_audio.astype(np.float32),
|
75 |
-
)
|
76 |
-
tmp_audio = librosa.resample(
|
77 |
-
tmp_audio, orig_sr=self.sr, target_sr=16000
|
78 |
-
) # , res_type="soxr_vhq"
|
79 |
-
wavfile.write(
|
80 |
-
"%s/%s_%s.wav" % (self.wavs16k_dir, idx0, idx1),
|
81 |
-
16000,
|
82 |
-
tmp_audio.astype(np.float32),
|
83 |
-
)
|
84 |
-
|
85 |
-
def pipeline(self, path, idx0):
|
86 |
-
try:
|
87 |
-
audio = load_audio(path, self.sr)
|
88 |
-
# zero phased digital filter cause pre-ringing noise...
|
89 |
-
# audio = signal.filtfilt(self.bh, self.ah, audio)
|
90 |
-
audio = signal.lfilter(self.bh, self.ah, audio)
|
91 |
-
|
92 |
-
idx1 = 0
|
93 |
-
for audio in self.slicer.slice(audio):
|
94 |
-
i = 0
|
95 |
-
while 1:
|
96 |
-
start = int(self.sr * (self.per - self.overlap) * i)
|
97 |
-
i += 1
|
98 |
-
if len(audio[start:]) > self.tail * self.sr:
|
99 |
-
tmp_audio = audio[start : start + int(self.per * self.sr)]
|
100 |
-
self.norm_write(tmp_audio, idx0, idx1)
|
101 |
-
idx1 += 1
|
102 |
-
else:
|
103 |
-
tmp_audio = audio[start:]
|
104 |
-
idx1 += 1
|
105 |
-
break
|
106 |
-
self.norm_write(tmp_audio, idx0, idx1)
|
107 |
-
println("%s->Suc." % path)
|
108 |
-
except:
|
109 |
-
println("%s->%s" % (path, traceback.format_exc()))
|
110 |
-
|
111 |
-
def pipeline_mp(self, infos):
|
112 |
-
for path, idx0 in infos:
|
113 |
-
self.pipeline(path, idx0)
|
114 |
-
|
115 |
-
def pipeline_mp_inp_dir(self, inp_root, n_p):
|
116 |
-
try:
|
117 |
-
infos = [
|
118 |
-
("%s/%s" % (inp_root, name), idx)
|
119 |
-
for idx, name in enumerate(sorted(list(os.listdir(inp_root))))
|
120 |
-
]
|
121 |
-
if noparallel:
|
122 |
-
for i in range(n_p):
|
123 |
-
self.pipeline_mp(infos[i::n_p])
|
124 |
-
else:
|
125 |
-
ps = []
|
126 |
-
for i in range(n_p):
|
127 |
-
p = multiprocessing.Process(
|
128 |
-
target=self.pipeline_mp, args=(infos[i::n_p],)
|
129 |
-
)
|
130 |
-
ps.append(p)
|
131 |
-
p.start()
|
132 |
-
for i in range(n_p):
|
133 |
-
ps[i].join()
|
134 |
-
except:
|
135 |
-
println("Fail. %s" % traceback.format_exc())
|
136 |
-
|
137 |
-
|
138 |
-
def preprocess_trainset(inp_root, sr, n_p, exp_dir, per):
|
139 |
-
pp = PreProcess(sr, exp_dir, per)
|
140 |
-
println("start preprocess")
|
141 |
-
println(sys.argv)
|
142 |
-
pp.pipeline_mp_inp_dir(inp_root, n_p)
|
143 |
-
println("end preprocess")
|
144 |
-
|
145 |
-
|
146 |
-
if __name__ == "__main__":
|
147 |
-
preprocess_trainset(inp_root, sr, n_p, exp_dir, per)
|
|
|
|
|
|
|
|
|
|
|
|
|
|
|
|
|
|
|
|
|
|
|
|
|
|
|
|
|
|
|
|
|
|
|
|
|
|
|
|
|
|
|
|
|
|
|
|
|
|
|
|
|
|
|
|
|
|
|
|
|
|
|
|
|
|
|
|
|
|
|
|
|
|
|
|
|
|
|
|
|
|
|
|
|
|
|
|
|
|
|
|
|
|
|
|
|
|
|
|
|
|
|
|
|
|
|
|
|
|
|
|
|
|
|
|
|
|
|
|
|
|
|
|
|
|
|
|
|
|
|
|
|
|
|
|
|
|
|
|
|
|
|
|
|
|
|
|
|
|
|
|
|
|
|
|
|
|
|
|
|
|
|
|
|
|
|
|
|
|
|
|
|
|
|
|
|
|
|
|
|
|
|
|
|
|
|
|
|
|
|
|
|
|
|
|
|
|
|
|
|
|
|
|
|
|
|
|
|
|
|
|
|
|
|
|
|
|
|
|
|
|
|
|
|
|
|
|
|
|
|
|
|
|
|
|
|
|
|
|
|
|
|
|
|
|
|
|
|
|
|
|
|
|
|
|
|
|
|
|
|
|
|
|
|
|
|
|
|
|
|
|
|
|
|
|
|
|
|
|
|
|
|
|
|
|
|
|
|
|
|
|
|
|
|
|
spaces/Benson/text-generation/Examples/Descarga De Actualizacin De Redmi 5a Miui 12.md
DELETED
@@ -1,101 +0,0 @@
|
|
1 |
-
<br />
|
2 |
-
<h1>Cómo descargar música gratis con una aplicación de descarga de música</h1>
|
3 |
-
<p>La música es una de las mejores maneras de relajarse, entretenerse e inspirarse. Pero a veces, es posible que no tenga acceso a Internet o quiera guardar sus datos. Ahí es cuando una aplicación de descarga de música es útil. Una aplicación de descarga de música le permite descargar canciones de varias fuentes y escucharlas sin conexión, sin usar sus datos o wifi. En este artículo, le mostraremos qué es una aplicación de descarga de música, por qué debe usar una, qué características buscar y cómo usarla para descargar música de forma gratuita. </p>
|
4 |
-
<h2>descarga de actualización de redmi 5a miui 12</h2><br /><p><b><b>Download</b> ✵ <a href="https://bltlly.com/2v6LNu">https://bltlly.com/2v6LNu</a></b></p><br /><br />
|
5 |
-
<h2>¿Qué es una aplicación de descarga de música? </h2>
|
6 |
-
<p>Una aplicación de descarga de música es una aplicación de software que le permite descargar música de plataformas en línea como YouTube, SoundCloud, Spotify, etc. Puede elegir entre diferentes formatos de salida y niveles de calidad, dependiendo de sus preferencias y almacenamiento de dispositivos. Una aplicación de descarga de música generalmente tiene un reproductor de música incorporado que te permite reproducir tus canciones descargadas sin conexión. Algunas aplicaciones de descarga de música también tienen otras características como radio, podcasts, listas de reproducción, letras, etc.</p>
|
7 |
-
<h3>Beneficios de usar una aplicación de descarga de música</h3>
|
8 |
-
<p>Hay muchos beneficios de usar una aplicación de descarga de música, como:</p>
|
9 |
-
<ul>
|
10 |
-
<li>Puedes descargar música gratis de varias fuentes y géneros. </li>
|
11 |
-
<li>Puedes escuchar tus canciones favoritas sin conexión, sin usar tus datos o wifi. </li>
|
12 |
-
<li> Puede transferir sus canciones descargadas a otros dispositivos o almacenamiento externo. </li>
|
13 |
-
<li>Puedes crear tus propias listas de reproducción y personalizar tu experiencia musical. </li>
|
14 |
-
<li>Puedes descubrir nueva música y artistas basados en tu gusto y humor. </li>
|
15 |
-
</ul>
|
16 |
-
<h3>Características para buscar en una aplicación de descarga de música</h3>
|
17 |
-
<p>No todas las aplicaciones de descarga de música son iguales. Algunas pueden tener más características y funciones que otras. Aquí hay algunas características para buscar en una buena aplicación de descarga de música:</p>
|
18 |
-
<ul>
|
19 |
-
<li>Soporta la descarga de música desde múltiples fuentes y plataformas. </li>
|
20 |
-
|
21 |
-
<li> Tiene una interfaz fácil de usar y funciones fáciles de usar. </li>
|
22 |
-
<li> Tiene una velocidad de descarga rápida y confiable y el rendimiento. </li>
|
23 |
-
<li> Tiene un reproductor de música incorporado que admite la reproducción sin conexión y otras funciones. </li>
|
24 |
-
<li>No tiene anuncios o ventanas emergentes que puedan interrumpir su experiencia musical. </li>
|
25 |
-
</ul>
|
26 |
-
<h2>Las 3 mejores aplicaciones de descarga de música para Android e iOS</h2>
|
27 |
-
<p>Hay muchas aplicaciones de descarga de música disponibles para dispositivos Android e iOS, pero no todas valen tu tiempo y atención. Aquí están nuestras 3 mejores opciones para las mejores aplicaciones de descarga de música en 2023:</p>
|
28 |
-
<h3> Cualquier convertidor de vídeo libre</h3>
|
29 |
-
<p>Any Video Converter Free es una de las aplicaciones de descarga de música más populares y versátiles para computadoras Windows y Mac. Te permite descargar música desde más de 100 plataformas online, incluyendo YouTube, SoundCloud, Facebook, etc. Puedes elegir entre varios formatos de salida como MP3, AAC, M4A, WAV, etc. También puedes editar tus canciones descargadas con su editor básico que te permite recortar, combinar, recortar, rotar, etc. Any Video Converter Free también tiene un descargador de video incorporado que le permite descargar videos de fuentes en línea también. </p>
|
30 |
-
<p></p>
|
31 |
-
<h4>Pros</h4>
|
32 |
-
<ul <h4>Contras</h4>
|
33 |
-
<ul>
|
34 |
-
<li>Solo funciona en computadoras Windows y Mac, no en dispositivos móviles. </li>
|
35 |
-
<li>Puede que no soporte algunos formatos de música raros o protegidos. </li>
|
36 |
-
<li>Puede tener algunos errores o errores durante el proceso de descarga. </li>
|
37 |
-
</ul>
|
38 |
-
<h3>Audiomack: Descargador de música</h3>
|
39 |
-
|
40 |
-
<h4>Pros</h4>
|
41 |
-
<ul>
|
42 |
-
<li> Tiene un catálogo de música grande y diversa con más de 200 millones de canciones gratis. </li>
|
43 |
-
<li> Le permite descargar canciones y álbumes completos para escuchar sin conexión sin datos o wifi. </li>
|
44 |
-
<li> Tiene una interfaz fácil de usar y funciones fáciles de usar. </li>
|
45 |
-
<li>No tiene anuncios o ventanas emergentes que puedan interrumpir su experiencia musical. </li>
|
46 |
-
<li>Soporta el acceso multiplataforma, incluyendo Wear OS y Android Auto.</li>
|
47 |
-
</ul>
|
48 |
-
<h4>Contras</h4>
|
49 |
-
<ul>
|
50 |
-
<li>Puede que no tenga algunas canciones o artistas que sean exclusivos de otras plataformas. </li>
|
51 |
-
<li>Puede tener algunos problemas de calidad o fallos con algunas descargas o transmisiones. </li>
|
52 |
-
<li>Puede consumir mucha batería o espacio de almacenamiento en su dispositivo. </li>
|
53 |
-
</ul>
|
54 |
-
<h3>Descargador de música - Aplicaciones en Google Play</h3>
|
55 |
-
<p>Music Downloader es una aplicación simple y potente para dispositivos Android que te permite descargar música de varias fuentes en línea y reproducirlas sin conexión. Puedes buscar cualquier canción, lista de reproducción, álbum, artista, remix, single, cover, radio FM, podcast, etc. y descargarlo en diferentes formatos de salida como MP3, FLAC, M4B, MP4, 3GP, MID, OGG, etc. También puedes editar tus canciones descargadas con su editor básico que te permite recortar, merge, crop, rotate, etc. Music downloader también tiene un reproductor de música incorporado que admite la reproducción sin conexión y otras funciones como ecualizador, aumento de graves, tema oscuro, temporizador de sueño, tonos de llamada, letras, listas de reproducción, Dropbox, etc.</p>
|
56 |
-
<h4>Pros</h4>
|
57 |
-
<ul <ul>
|
58 |
-
<li>Soporta la descarga de música desde múltiples fuentes y plataformas. </li>
|
59 |
-
<li> Ofrece diferentes formatos de salida y opciones de calidad para sus canciones descargadas. </li>
|
60 |
-
<li> Tiene una interfaz fácil de usar y funciones fáciles de usar. </li>
|
61 |
-
<li> Tiene una velocidad de descarga rápida y confiable y el rendimiento. </li>
|
62 |
-
<li> Tiene un reproductor de música incorporado que admite la reproducción sin conexión y otras funciones. </li>
|
63 |
-
</ul>
|
64 |
-
<h4>Contras</h4>
|
65 |
-
<ul>
|
66 |
-
<li>Puede no ser compatible con algunos dispositivos o sistemas operativos. </li>
|
67 |
-
|
68 |
-
<li>Puede tener algunos problemas legales o éticos con algunas descargas o fuentes. </li>
|
69 |
-
</ul>
|
70 |
-
<h2>Cómo usar una aplicación de descarga de música para descargar canciones sin conexión</h2>
|
71 |
-
<p>Usar una aplicación de descarga de música para descargar canciones sin conexión es muy fácil y conveniente. Estos son los pasos básicos que debe seguir:</p>
|
72 |
-
<h3>Paso 1: Elija una aplicación de descarga de música e instálela en su dispositivo</h3>
|
73 |
-
<p>El primer paso es elegir una aplicación de descarga de música que se adapte a sus necesidades y preferencias. Puede consultar nuestras 3 mejores selecciones anteriores o buscar otras opciones en Internet. Una vez que haya elegido una aplicación de descarga de música, debe instalarla en su dispositivo. Puedes descargarlo desde el sitio web oficial, la tienda de aplicaciones o la tienda de Google Play, dependiendo de tu dispositivo y la aplicación. Siga las instrucciones en la pantalla para completar el proceso de instalación. </p>
|
74 |
-
<h3>Paso 2: Buscar las canciones o listas de reproducción que desea descargar</h3>
|
75 |
-
<p>El siguiente paso es buscar las canciones o listas de reproducción que desea descargar. Puede usar la función de búsqueda de la aplicación, navegar por categorías, géneros, artistas, etc., o ingresar la URL de la fuente en línea. También puedes usar las recomendaciones, sugerencias o gráficos de la aplicación para descubrir nueva música. Una vez que haya encontrado las canciones o listas de reproducción que desea descargar, puede seleccionarlas pulsando en ellas o marcando las casillas junto a ellas. </p>
|
76 |
-
<h3>Paso 3: Toque en el botón de descarga y seleccione el formato de salida y la calidad</h3>
|
77 |
-
<p>El paso final es tocar el botón de descarga y seleccionar el formato de salida y la calidad de sus canciones descargadas. Puede elegir entre diferentes opciones como MP3, AAC, M4A, WAV, etc., y diferentes niveles de calidad como alta, media, baja, etc. También puede personalizar otros ajustes como bitrate, frecuencia de muestreo, volumen, etc., dependiendo de la aplicación. Después de haber hecho sus elecciones, puede iniciar el proceso de descarga tocando el botón de inicio o confirmando su selección. </p>
|
78 |
-
<h3>Paso 4: Disfrute de su música sin conexión en cualquier momento, en cualquier lugar</h3>
|
79 |
-
|
80 |
-
<h2>Conclusión</h2>
|
81 |
-
<p>Una aplicación de descarga de música es una gran manera de descargar música gratis de varias fuentes en línea y escucharlas sin conexión. Tiene muchos beneficios como guardar sus datos o wifi, crear sus propias listas de reproducción, descubrir nueva música, etc. También tiene algunas características que buscar, como el apoyo a múltiples fuentes y plataformas, ofreciendo diferentes formatos de salida y opciones de calidad, tener una interfaz fácil de usar y funciones fáciles de usar, etc. Hemos revisado nuestras 3 mejores opciones para las mejores aplicaciones de descarga de música en 2023: Any Video Converter Free, Audiomack: Music Downloader y Music Downloader - Aplicaciones en Google Play. También le hemos mostrado cómo usar una aplicación de descarga de música para descargar canciones sin conexión en cuatro sencillos pasos. Esperamos que este artículo te haya ayudado a aprender más sobre las aplicaciones de descarga de música y cómo usarlas. Si tiene alguna pregunta o comentario, no dude en dejarlos abajo. </p>
|
82 |
-
<h2>Preguntas frecuentes</h2>
|
83 |
-
<p>Aquí hay algunas preguntas frecuentes sobre aplicaciones de descarga de música:</p>
|
84 |
-
<h4>Q: ¿Es legal descargar música con una aplicación de descarga de música? </h4>
|
85 |
-
<p>A: La legalidad de descargar música con una aplicación de descarga de música depende de varios factores, como la fuente de la música, el estado de copyright de la música, los términos y condiciones de la plataforma, etc. En términos generales, es legal descargar música que está en el dominio público, que está licenciada bajo Creative Commons o licencias similares, que se ofrece de forma gratuita por el artista o plataforma, etc. Sin embargo, puede no ser legal descargar música que esté protegida por las leyes de derechos de autor, que es exclusivo de una plataforma, que se descarga con fines comerciales, etc. Por lo tanto, es aconsejable verificar el estado legal de la música antes de descargarla con una aplicación de descarga de música, y respetar los derechos de los artistas y plataformas. </p>
|
86 |
-
<h4>Q: ¿Es seguro descargar música con una aplicación de descarga de música? </h4>
|
87 |
-
|
88 |
-
<h4>Q: ¿Cuánto espacio ocupa una aplicación de descarga de música en mi dispositivo? </h4>
|
89 |
-
<p>A: el espacio que una aplicación de descarga de música ocupa en su dispositivo depende de varios factores, como el tamaño de la aplicación, el número y el tamaño de las canciones descargadas, el formato de salida y la calidad de las canciones descargadas, etc. En términos generales, una aplicación de descarga de música en sí no ocupa mucho espacio en su dispositivo, generalmente menos de 100 MB. Sin embargo, las canciones descargadas pueden ocupar mucho espacio en su dispositivo, dependiendo de cuántas canciones descargue y qué formato y calidad elija. Por ejemplo, una canción MP3 de 3 minutos a 320 kbps puede ocupar unos 7 MB de espacio, mientras que una canción FLAC de 3 minutos a 1411 kbps puede ocupar unos 30 MB de espacio. Por lo tanto, es recomendable comprobar el espacio de almacenamiento disponible en el dispositivo antes de descargar canciones con una aplicación de descarga de música, y para eliminar o transferir algunas canciones si es necesario. </p>
|
90 |
-
<h4>Q: ¿Cómo puedo transferir mis canciones descargadas a otros dispositivos o almacenamiento externo? </h4>
|
91 |
-
<p>A: Hay diferentes formas de transferir tus canciones descargadas a otros dispositivos o almacenamiento externo, dependiendo del tipo y compatibilidad de tus dispositivos y almacenamiento. Algunos métodos comunes son:</p>
|
92 |
-
<ul>
|
93 |
-
<li>Usando un cable USB o una conexión inalámbrica para conectar su dispositivo a su computadora u otro dispositivo, y luego copiar y pegar o arrastrar y soltar sus canciones descargadas de una carpeta a otra. </li>
|
94 |
-
<li>Usando un servicio en la nube como Dropbox, Google Drive, iCloud, etc. para subir tus canciones descargadas desde tu dispositivo a tu cuenta en línea, y luego descargarlas desde tu cuenta a otro dispositivo o almacenamiento. </li>
|
95 |
-
<li>Usando una conexión Bluetooth para emparejar su dispositivo con otro dispositivo o almacenamiento, y luego enviar o recibir sus canciones descargadas a través de Bluetooth.</li>
|
96 |
-
<li>Usando una tarjeta SD o una unidad flash para insertar en su dispositivo o computadora, y luego copiar y pegar o arrastrar y soltar las canciones descargadas de una carpeta a otra. </li>
|
97 |
-
|
98 |
-
<h4>Q: ¿Cómo puedo editar mis canciones descargadas con una aplicación de descarga de música? </h4>
|
99 |
-
<p>A: Algunas aplicaciones de descarga de música tienen un editor incorporado que le permite editar sus canciones descargadas con funciones básicas como recorte, fusión, recorte, rotación, etc. Para editar sus canciones descargadas con una aplicación de descarga de música, necesita abrir la aplicación y seleccionar la canción que desea editar. Luego debe tocar en el botón de edición y elegir la función que desea utilizar. A continuación, puede ajustar los parámetros como la hora de inicio, hora de finalización, duración, volumen, etc., dependiendo de la función. Después de haber hecho sus cambios, necesita guardar o exportar su canción editada. </p> 64aa2da5cf<br />
|
100 |
-
<br />
|
101 |
-
<br />
|
|
|
|
|
|
|
|
|
|
|
|
|
|
|
|
|
|
|
|
|
|
|
|
|
|
|
|
|
|
|
|
|
|
|
|
|
|
|
|
|
|
|
|
|
|
|
|
|
|
|
|
|
|
|
|
|
|
|
|
|
|
|
|
|
|
|
|
|
|
|
|
|
|
|
|
|
|
|
|
|
|
|
|
|
|
|
|
|
|
|
|
|
|
|
|
|
|
|
|
|
|
|
|
|
|
|
|
|
|
|
|
|
|
|
|
|
|
|
|
|
|
|
|
|
|
|
|
|
|
|
|
|
|
|
|
|
|
|
|
|
|
|
|
|
|
|
|
|
|
|
|
|
|
|
|
|
|
|
|
|
|
|
|
|
|
|
|
|
|
|
|
|
|
|
|
|
|
|
|
|
|
|
|
|
|
|
|
|
|
|
|
|
|
|
|
|
|
|
|
|
|
|
|
spaces/Benson/text-generation/Examples/Descargar Gratis Para Windows 7.md
DELETED
@@ -1,61 +0,0 @@
|
|
1 |
-
|
2 |
-
<h1>Descargar gratis Kick the Buddy para Windows 7</h1>
|
3 |
-
<p>Kick the Buddy es un popular juego móvil que te permite desatar tu ira y frustración en un muñeco de trapo indefenso. Puedes usar todo tipo de armas y accesorios para torturar, explotar, aplastar, congelar, quemar, disparar e incluso bombardear a tu amigo. Es un juego divertido y relajante que puede ayudarte a desahogarte y reírte. </p>
|
4 |
-
<h2>descargar gratis para Windows 7</h2><br /><p><b><b>Download</b> ✏ ✏ ✏ <a href="https://bltlly.com/2v6Mjn">https://bltlly.com/2v6Mjn</a></b></p><br /><br />
|
5 |
-
<p>Pero ¿qué pasa si quieres jugar Kick the Buddy en tu PC en lugar de tu teléfono? Tal vez usted tiene una computadora con Windows 7 que todavía utiliza para el trabajo o el entretenimiento. Quizás prefieras jugar en una pantalla más grande y con un teclado y un ratón. Quizás solo quieras probar algo nuevo y diferente. </p>
|
6 |
-
<p>Si ese es el caso, entonces estás de suerte. En este artículo, te mostraremos cómo descargar e instalar Kick the Buddy en Windows 7 gratis. También te daremos algunos consejos y trucos sobre cómo jugarlo, así como algunos beneficios y desventajas de jugarlo en tu PC. Y si estás buscando alternativas a Kick the Buddy, también te sugeriremos otros juegos que puedes jugar en tu ordenador con Windows 7. </p>
|
7 |
-
<h2>Cómo descargar e instalar Kick the Buddy en Windows 7</h2>
|
8 |
-
<p>Kick the Buddy es un juego para Android, lo que significa que no puedes ejecutarlo directamente en tu PC con Windows 7. Necesitas un emulador de Android, que es un software que imita un dispositivo Android en tu computadora. Hay muchos emuladores de Android disponibles en línea, pero algunos de los más populares son BlueStacks y NoxPlayer. Estos son los pasos para descargar e instalar Kick the Buddy en Windows 7 usando un emulador de Android:</p>
|
9 |
-
<ol>
|
10 |
-
<li>Descargar un emulador de Android de su elección desde su sitio web oficial. Por ejemplo, puede descargar BlueStacks desde <a href="( 1 )">https://www.bluestacks.com</a> o NoxPlayer desde <a href="( 2 )">https://www.bignox.com</a>. Asegúrese de que su PC cumple con los requisitos mínimos del sistema para ejecutar el emulador. </li>
|
11 |
-
|
12 |
-
<li>Inicie el emulador en su PC. Es posible que necesite iniciar sesión con su cuenta de Google o crear uno si no tiene uno ya. </li>
|
13 |
-
<li>Busca a Kick the Buddy en la tienda de aplicaciones del emulador. También puedes usar Google Play Store si está disponible en tu emulador. Alternativamente, puede descargar Kick the Buddy APK archivo de una fuente de confianza y arrastrarlo a la ventana del emulador para instalarlo. </li>
|
14 |
-
<li>Disfruta jugando Kick the Buddy en tu PC. Puedes usar el teclado y el ratón para controlar el juego, o puedes personalizar la configuración para adaptarla a tus preferencias. </li>
|
15 |
-
</ol>
|
16 |
-
<h2>Cómo jugar a patear al amigo en Windows 7</h2>
|
17 |
-
<p>Kick the Buddy es un juego simple y fácil de jugar. El objetivo principal es divertirse con su amigo y usar varias armas y accesorios para destruirlo. También puede ganar monedas y oro jugando el juego, que se puede utilizar para comprar más artículos y características. Aquí hay algunos consejos y trucos sobre cómo jugar Kick the Buddy en Windows 7:</p>
|
18 |
-
<p></p>
|
19 |
-
<ul>
|
20 |
-
<li>Cómo usar diferentes armas y accesorios para destruir al compañero: Puedes elegir entre una amplia gama de armas y accesorios en el juego, como armas, cuchillos, bombas, cohetes, granadas, fuego, hielo, electricidad, animales, plantas y más. Puede arrastrarlos desde el menú en la parte inferior de la pantalla y soltarlos en su amigo. También puede pulsar o hacer clic en ellos para activarlos. Algunas armas y accesorios tienen efectos especiales o animaciones que puedes disfrutar viendo. </li>
|
21 |
-
<li>Cómo desbloquear nuevos elementos y características en el juego: Puedes desbloquear nuevos elementos y características en el juego completando misiones, logros y tareas diarias. También puede ver anuncios o hacer compras en la aplicación para obtener más monedas y oro. Algunos de los elementos y características que puedes desbloquear son nuevos amigos, nuevos fondos, nuevos trajes, nuevos modos, nuevos elementos y más. </li>
|
22 |
-
|
23 |
-
</ul>
|
24 |
-
<h2>Beneficios de jugar Kick the Buddy en Windows 7</h2>
|
25 |
-
<p>Jugar a Kick the Buddy en Windows 7 tiene algunos beneficios que quizás no obtengas al jugarlo en tu teléfono. Estos son algunos de ellos:</p>
|
26 |
-
<ul>
|
27 |
-
<li>Ventajas de jugar Kick the Buddy en una pantalla más grande y con un teclado y un ratón: Jugar Kick the Buddy en una pantalla más grande puede darle una mejor vista de los gráficos y animaciones del juego. También puede ver más detalles y efectos que podría perderse en una pantalla más pequeña. Jugar con un teclado y un ratón también puede darte más control y precisión sobre tus acciones. Puede utilizar atajos y teclas de acceso directo para acceder a diferentes funciones de forma más rápida y sencilla. </li>
|
28 |
-
<li> ¿Cómo jugar Kick the Buddy puede ayudar a aliviar el estrés y la ira: Jugar Kick the Buddy puede ser una gran manera de aliviar el estrés y la ira que usted puede sentir de su vida diaria. Usted puede ventilar sus emociones en su amigo sin lastimar a nadie o nada en la vida real. También puedes reírte del absurdo y el humor del juego, que puede mejorar tu estado de ánimo y hacerte sentir más feliz. </li>
|
29 |
-
<li>Cómo jugar Kick the Buddy puede mejorar tu creatividad e imaginación: Jugar Kick the Buddy también puede mejorar tu creatividad e imaginación al permitirte experimentar con diferentes combinaciones de armas y accesorios. Puedes descubrir nuevas formas de destruir a tu amigo y ver qué pasa cuando lo haces. También puedes crear tus propios escenarios e historias con tu amigo y tus antecedentes. </li>
|
30 |
-
</ul> <h2>Inconvenientes de jugar a patear al amigo en Windows 7</h2>
|
31 |
-
<p>Jugar Kick the Buddy en Windows 7 también tiene algunos inconvenientes que debes tener en cuenta. Estos son algunos de ellos:</p>
|
32 |
-
<ul>
|
33 |
-
|
34 |
-
<li>Cómo jugar Kick the Buddy puede ser adictivo y consume mucho tiempo: Jugar Kick the Buddy puede ser adictivo y consumir mucho tiempo si no se establece un límite para sí mismo. Usted puede encontrarse jugando el juego durante horas sin darse cuenta de la hora. También puede descuidar sus otras responsabilidades, como el trabajo, la escuela o la familia. También puede gastar demasiado dinero en compras en la aplicación o anuncios para obtener más monedas y oro. Deberías jugar el juego con moderación y equilibrarlo con otras actividades. </li>
|
35 |
-
<li> ¿Cómo jugar Kick the Buddy puede exponerte a la violencia excesiva y gore: Jugar Kick the Buddy puede exponerte a la violencia excesiva y gore que puede no ser adecuado para todos. El juego involucra mucha sangre, vísceras, huesos, explosiones y mutilaciones que pueden ser perturbadoras o perturbadoras para algunas personas. El juego también puede insensibilizarte a la violencia real o hacerte más agresivo o violento en la vida real. Debes jugar el juego con precaución y discreción. </li>
|
36 |
-
</ul>
|
37 |
-
<h2>Alternativas a jugar Kick the Buddy en Windows 7</h2>
|
38 |
-
<p>Si estás buscando algunas alternativas a jugar Kick the Buddy en Windows 7, hay otros juegos que puedes jugar en tu PC que son similares a Kick the Buddy. Aquí hay algunos ejemplos:</p>
|
39 |
-
<ul>
|
40 |
-
<li>Sala feliz: Sala feliz es un juego donde pruebas varias armas y trampas en un maniquí en un laboratorio. Puedes usar cohetes, sierras, láseres, minas, picos y más para infligir el mayor daño posible al maniquí. También puedes desbloquear nuevas armas y mejoras a medida que avanzas en el juego. </li>
|
41 |
-
<li>Whack Your Boss: Whack Your Boss es un juego donde se encuentran diferentes maneras de golpear a su jefe molesto en su oficina. Puede usar tijeras, grapadoras, lápices, teclados, tazas de café y más para ventilar su ira en su jefe. También puedes explorar diferentes escenarios y finales en el juego. </li>
|
42 |
-
|
43 |
-
</ul>
|
44 |
-
<h1>Conclusión</h1>
|
45 |
-
<p>Kick the Buddy es un juego divertido y relajante que te permite desatar tu ira y frustración en un muñeco de trapo indefenso. Puede descargarlo e instalarlo en su PC con Windows 7 de forma gratuita utilizando un emulador de Android. También puede jugar con diferentes armas y accesorios, desbloquear nuevos artículos y características, y personalizar a su amigo y su fondo. Sin embargo, también debe ser consciente de los inconvenientes de jugar en su PC, tales como problemas de compatibilidad, riesgos de adicción y exposición a la violencia. Y si quieres probar otros juegos similares a Kick the Buddy, puedes echar un vistazo a algunas de las alternativas que te sugerimos. </p>
|
46 |
-
<p>Esperamos que este artículo le haya ayudado a aprender a descargar y jugar Kick the Buddy en Windows 7. Si tiene alguna pregunta o comentario, no dude en dejar un comentario a continuación. Y si te gustó este artículo, por favor compártelo con tus amigos y familiares que también podrían estar interesados en jugar Kick the Buddy en su PC.</p>
|
47 |
-
<h3>Preguntas frecuentes</h3>
|
48 |
-
<ul>
|
49 |
-
<li>Q: ¿Kick the Buddy es seguro para los niños? </li>
|
50 |
-
<li>A: Kick the Buddy está clasificado 12+ en Google Play Store y 9+ en App Store para dibujos animados infrecuentes/ leves o violencia de fantasía. Puede no ser adecuado para niños más pequeños que pueden estar asustados o influenciados por la violencia y la sangre en el juego. Los padres deben supervisar a sus hijos mientras juegan el juego o usar el control parental para restringir el acceso a él. </li>
|
51 |
-
<li>Q: ¿Cómo puedo actualizar Kick the Buddy en Windows 7?</li>
|
52 |
-
<li>A: Para actualizar Kick the Buddy en Windows 7, es necesario actualizarlo a través de su emulador de Android. Puede comprobar si hay actualizaciones en la tienda de aplicaciones del emulador o descargar la última versión de Kick the Buddy APK archivo de una fuente de confianza e instalarlo en su emulador. </li>
|
53 |
-
<li>Q: ¿Cómo puedo desinstalar Kick the Buddy desde Windows 7?</li>
|
54 |
-
|
55 |
-
<li>Q: ¿Cómo puedo guardar mi progreso en Kick the Buddy en Windows 7?</li>
|
56 |
-
<li>A: Para guardar su progreso en Kick the Buddy en Windows 7, es necesario conectar el juego a su cuenta de Facebook. Puedes hacer esto yendo al menú en la esquina superior derecha de la pantalla y tocando el icono de Facebook. De esta manera, puede sincronizar su progreso a través de diferentes dispositivos y emuladores. </li>
|
57 |
-
<li>Q: ¿Cómo puedo contactar a los desarrolladores de Kick the Buddy? </li>
|
58 |
-
<li>A: Para contactar con los desarrolladores de Kick the Buddy, puedes ir a su sitio web oficial en <a href="">https://playgendary.com</a> y rellenar el formulario de contacto. También puedes seguirlos en sus cuentas de redes sociales, como Facebook, Twitter, Instagram y YouTube.</li>
|
59 |
-
</ul></p> 64aa2da5cf<br />
|
60 |
-
<br />
|
61 |
-
<br />
|
|
|
|
|
|
|
|
|
|
|
|
|
|
|
|
|
|
|
|
|
|
|
|
|
|
|
|
|
|
|
|
|
|
|
|
|
|
|
|
|
|
|
|
|
|
|
|
|
|
|
|
|
|
|
|
|
|
|
|
|
|
|
|
|
|
|
|
|
|
|
|
|
|
|
|
|
|
|
|
|
|
|
|
|
|
|
|
|
|
|
|
|
|
|
|
|
|
|
|
|
|
|
|
|
|
|
|
|
|
|
|
|
|
|
|
|
|
|
|
|
|
|
|
spaces/BetterAPI/BetterChat/postcss.config.js
DELETED
@@ -1,6 +0,0 @@
|
|
1 |
-
export default {
|
2 |
-
plugins: {
|
3 |
-
tailwindcss: {},
|
4 |
-
autoprefixer: {},
|
5 |
-
},
|
6 |
-
};
|
|
|
|
|
|
|
|
|
|
|
|
|
|
spaces/BramVanroy/mai-simplification-nl-2023-demo/app.py
DELETED
@@ -1,128 +0,0 @@
|
|
1 |
-
import base64
|
2 |
-
from io import StringIO
|
3 |
-
from math import ceil
|
4 |
-
|
5 |
-
import streamlit as st
|
6 |
-
|
7 |
-
from utils import get_resources, simplify
|
8 |
-
|
9 |
-
st.set_page_config(page_title="Text Simplification in Dutch", page_icon="🏃")
|
10 |
-
|
11 |
-
BATCH_SIZE = 8
|
12 |
-
|
13 |
-
if "text_to_simplify" not in st.session_state:
|
14 |
-
st.session_state["text_to_simplify"] = None
|
15 |
-
|
16 |
-
st.title("🏃 Text Simplification in Dutch")
|
17 |
-
|
18 |
-
fupload_check = st.checkbox("File upload?")
|
19 |
-
|
20 |
-
st.markdown(
|
21 |
-
"Make sure that the file or text in the text box contains **one sentence per line**. Empty lines will"
|
22 |
-
" be removed."
|
23 |
-
)
|
24 |
-
if fupload_check:
|
25 |
-
uploaded_file = st.file_uploader("Text file", label_visibility="collapsed")
|
26 |
-
if uploaded_file is not None:
|
27 |
-
stringio = StringIO(uploaded_file.getvalue().decode("utf-8"))
|
28 |
-
st.session_state["text_to_simplify"] = stringio.read().strip()
|
29 |
-
else:
|
30 |
-
st.session_state["text_to_simplify"] = None
|
31 |
-
else:
|
32 |
-
st.session_state["text_to_simplify"] = st.text_area(
|
33 |
-
label="Sentences to translate",
|
34 |
-
label_visibility="collapsed",
|
35 |
-
height=200,
|
36 |
-
value="Met het naderen van de zonovergoten middaghemel op deze betoverende dag, waarbij de atmosferische omstandigheden een onbelemmerde convergentie van cumulusbewolking en uitgestrekte stratosferische azuurblauwe wijdheid faciliteren, lijken de geaggregeerde weersverschijnselen van vandaag, die variëren van sporadische plensbuien tot kalme zuchtjes wind en zeldzame opvlammingen van bliksem, de delicate balans tussen meteorologische complexiteit en eenvoud te weerspiegelen, waardoor de gepassioneerde observator met een gevoel van ontzag en verwondering wordt vervuld.",
|
37 |
-
).strip()
|
38 |
-
|
39 |
-
|
40 |
-
def _get_increment_size(num_sents) -> int:
|
41 |
-
if num_sents == 1:
|
42 |
-
return 100
|
43 |
-
else:
|
44 |
-
return ceil(100 / num_sents)
|
45 |
-
|
46 |
-
|
47 |
-
btn_col, results_col = st.columns(2)
|
48 |
-
btn_ct = btn_col.empty()
|
49 |
-
pbar_ct = st.empty()
|
50 |
-
error_ct = st.empty()
|
51 |
-
simpl_ct = st.container()
|
52 |
-
if st.session_state["text_to_simplify"]:
|
53 |
-
if btn_ct.button("Simplify text"):
|
54 |
-
error_ct.empty()
|
55 |
-
lines = [
|
56 |
-
strip_line for line in st.session_state["text_to_simplify"].splitlines() if (strip_line := line.strip())
|
57 |
-
]
|
58 |
-
num_sentences = len(lines)
|
59 |
-
|
60 |
-
pbar = pbar_ct.progress(0, text=f"Simplifying sentences in batches of {BATCH_SIZE}...")
|
61 |
-
increment = _get_increment_size(num_sentences)
|
62 |
-
percent_done = 0
|
63 |
-
|
64 |
-
model, tokenizer = get_resources()
|
65 |
-
|
66 |
-
simpl_ct.caption("Simplified text")
|
67 |
-
output_ct = simpl_ct.empty()
|
68 |
-
all_simplifications = []
|
69 |
-
html = "<ol>"
|
70 |
-
for input_batch, simplifications in simplify(lines, model, tokenizer):
|
71 |
-
for input_text, simplification in zip(input_batch, simplifications):
|
72 |
-
output_ct.empty()
|
73 |
-
html += f"""<li>
|
74 |
-
<ul>
|
75 |
-
<li><strong>Input text:</strong> {input_text}</li>
|
76 |
-
<li><strong>Simplification:</strong> {simplification}</li>
|
77 |
-
</ul>
|
78 |
-
</li>"""
|
79 |
-
output_ct.markdown(html + "</ol>", unsafe_allow_html=True)
|
80 |
-
|
81 |
-
percent_done += increment
|
82 |
-
pbar.progress(min(percent_done, 100))
|
83 |
-
|
84 |
-
all_simplifications.extend(simplifications)
|
85 |
-
|
86 |
-
pbar.empty()
|
87 |
-
|
88 |
-
all_simplifications = "\n".join(all_simplifications) + "\n"
|
89 |
-
b64 = base64.b64encode(all_simplifications.encode("utf-8")).decode("utf-8")
|
90 |
-
results_col.markdown(
|
91 |
-
f'<a download="dutch-simplifications.txt" href="data:file/txt;base64,{b64}" title="Download">Download simplifications</a>',
|
92 |
-
unsafe_allow_html=True,
|
93 |
-
)
|
94 |
-
else:
|
95 |
-
btn_ct.empty()
|
96 |
-
error_ct.error("Text cannot be empty!", icon="⚠️")
|
97 |
-
simpl_ct.container()
|
98 |
-
|
99 |
-
|
100 |
-
########################
|
101 |
-
# Information, socials #
|
102 |
-
########################
|
103 |
-
st.header("Project background")
|
104 |
-
|
105 |
-
st.markdown(
|
106 |
-
"""This demo highlights work that has been done in light of a master thesis by **Charlotte Van de Velde** as part of the Master of Science in Artificial Intelligence at KU Leuven in 2023. Charlotte is supervised by Vincent Vandeghinste and Bram Vanroy.
|
107 |
-
|
108 |
-
Charlotte created a [dataset](https://huggingface.co/datasets/BramVanroy/chatgpt-dutch-simplification) that contains Dutch sentences and their simplified equivalents with ChatGPT. Bram then trained a number of models on this new dataset and built this demo.
|
109 |
-
|
110 |
-
The following models are available, all finetuned from the awesome Dutch T5 models by [Yeb Havinga](https://huggingface.co/yhavinga):
|
111 |
-
|
112 |
-
- [`BramVanroy/ul2-small-dutch-simplification-mai-2023`](https://huggingface.co/BramVanroy/ul2-small-dutch-simplification-mai-2023)
|
113 |
-
- [`BramVanroy/ul2-base-dutch-simplification-mai-2023`](https://huggingface.co/BramVanroy/ul2-base-dutch-simplification-mai-2023) (used in this demo)
|
114 |
-
- [`BramVanroy/ul2-large-dutch-simplification-mai-2023`](https://huggingface.co/BramVanroy/ul2-large-dutch-simplification-mai-2023)
|
115 |
-
|
116 |
-
The training code can be found on [Github](https://github.com/BramVanroy/mai-simplification-nl-2023#22-hyperparameter-sweep).
|
117 |
-
|
118 |
-
"""
|
119 |
-
)
|
120 |
-
|
121 |
-
|
122 |
-
st.header("Contact ✒️")
|
123 |
-
|
124 |
-
st.markdown(
|
125 |
-
"Would you like additional functionality in the demo, do you have questions, or just want to get in touch?"
|
126 |
-
" Give me a shout on [Twitter](https://twitter.com/BramVanroy)"
|
127 |
-
" or add me on [LinkedIn](https://www.linkedin.com/in/bramvanroy/)!"
|
128 |
-
)
|
|
|
|
|
|
|
|
|
|
|
|
|
|
|
|
|
|
|
|
|
|
|
|
|
|
|
|
|
|
|
|
|
|
|
|
|
|
|
|
|
|
|
|
|
|
|
|
|
|
|
|
|
|
|
|
|
|
|
|
|
|
|
|
|
|
|
|
|
|
|
|
|
|
|
|
|
|
|
|
|
|
|
|
|
|
|
|
|
|
|
|
|
|
|
|
|
|
|
|
|
|
|
|
|
|
|
|
|
|
|
|
|
|
|
|
|
|
|
|
|
|
|
|
|
|
|
|
|
|
|
|
|
|
|
|
|
|
|
|
|
|
|
|
|
|
|
|
|
|
|
|
|
|
|
|
|
|
|
|
|
|
|
|
|
|
|
|
|
|
|
|
|
|
|
|
|
|
|
|
|
|
|
|
|
|
|
|
|
|
|
|
|
|
|
|
|
|
|
|
|
|
|
|
|
|
|
|
|
|
|
|
|
|
|
|
|
|
|
|
|
|
|
|
|
|
|
|
|
|
|
|
|
|
|
|
|
|
|
|
|
|
|
|
|
|
|
|
|
|
|
|
|
|
|
|
|
|
spaces/CVPR/Dual-Key_Backdoor_Attacks/datagen/detectron2/projects/DensePose/densepose/densepose_head.py
DELETED
@@ -1,1183 +0,0 @@
|
|
1 |
-
# Copyright (c) Facebook, Inc. and its affiliates. All Rights Reserved
|
2 |
-
import math
|
3 |
-
from dataclasses import dataclass
|
4 |
-
from enum import Enum
|
5 |
-
import fvcore.nn.weight_init as weight_init
|
6 |
-
import torch
|
7 |
-
from torch import nn
|
8 |
-
from torch.nn import functional as F
|
9 |
-
|
10 |
-
from detectron2.config import CfgNode
|
11 |
-
from detectron2.layers import Conv2d, ConvTranspose2d, interpolate
|
12 |
-
from detectron2.structures.boxes import matched_boxlist_iou
|
13 |
-
from detectron2.utils.registry import Registry
|
14 |
-
|
15 |
-
from .structures import DensePoseOutput
|
16 |
-
|
17 |
-
ROI_DENSEPOSE_HEAD_REGISTRY = Registry("ROI_DENSEPOSE_HEAD")
|
18 |
-
|
19 |
-
|
20 |
-
class DensePoseUVConfidenceType(Enum):
|
21 |
-
"""
|
22 |
-
Statistical model type for confidence learning, possible values:
|
23 |
-
- "iid_iso": statistically independent identically distributed residuals
|
24 |
-
with anisotropic covariance
|
25 |
-
- "indep_aniso": statistically independent residuals with anisotropic
|
26 |
-
covariances
|
27 |
-
For details, see:
|
28 |
-
N. Neverova, D. Novotny, A. Vedaldi "Correlated Uncertainty for Learning
|
29 |
-
Dense Correspondences from Noisy Labels", p. 918--926, in Proc. NIPS 2019
|
30 |
-
"""
|
31 |
-
|
32 |
-
# fmt: off
|
33 |
-
IID_ISO = "iid_iso"
|
34 |
-
INDEP_ANISO = "indep_aniso"
|
35 |
-
# fmt: on
|
36 |
-
|
37 |
-
|
38 |
-
@dataclass
|
39 |
-
class DensePoseUVConfidenceConfig:
|
40 |
-
"""
|
41 |
-
Configuration options for confidence on UV data
|
42 |
-
"""
|
43 |
-
|
44 |
-
enabled: bool = False
|
45 |
-
# lower bound on UV confidences
|
46 |
-
epsilon: float = 0.01
|
47 |
-
type: DensePoseUVConfidenceType = DensePoseUVConfidenceType.IID_ISO
|
48 |
-
|
49 |
-
|
50 |
-
@dataclass
|
51 |
-
class DensePoseConfidenceModelConfig:
|
52 |
-
"""
|
53 |
-
Configuration options for confidence models
|
54 |
-
"""
|
55 |
-
|
56 |
-
# confidence for U and V values
|
57 |
-
uv_confidence: DensePoseUVConfidenceConfig
|
58 |
-
|
59 |
-
@staticmethod
|
60 |
-
def from_cfg(cfg: CfgNode) -> "DensePoseConfidenceModelConfig":
|
61 |
-
return DensePoseConfidenceModelConfig(
|
62 |
-
uv_confidence=DensePoseUVConfidenceConfig(
|
63 |
-
enabled=cfg.MODEL.ROI_DENSEPOSE_HEAD.UV_CONFIDENCE.ENABLED,
|
64 |
-
epsilon=cfg.MODEL.ROI_DENSEPOSE_HEAD.UV_CONFIDENCE.EPSILON,
|
65 |
-
type=DensePoseUVConfidenceType(cfg.MODEL.ROI_DENSEPOSE_HEAD.UV_CONFIDENCE.TYPE),
|
66 |
-
)
|
67 |
-
)
|
68 |
-
|
69 |
-
|
70 |
-
def initialize_module_params(module):
|
71 |
-
for name, param in module.named_parameters():
|
72 |
-
if "bias" in name:
|
73 |
-
nn.init.constant_(param, 0)
|
74 |
-
elif "weight" in name:
|
75 |
-
nn.init.kaiming_normal_(param, mode="fan_out", nonlinearity="relu")
|
76 |
-
|
77 |
-
|
78 |
-
@ROI_DENSEPOSE_HEAD_REGISTRY.register()
|
79 |
-
class DensePoseDeepLabHead(nn.Module):
|
80 |
-
def __init__(self, cfg, input_channels):
|
81 |
-
super(DensePoseDeepLabHead, self).__init__()
|
82 |
-
# fmt: off
|
83 |
-
hidden_dim = cfg.MODEL.ROI_DENSEPOSE_HEAD.CONV_HEAD_DIM
|
84 |
-
kernel_size = cfg.MODEL.ROI_DENSEPOSE_HEAD.CONV_HEAD_KERNEL
|
85 |
-
norm = cfg.MODEL.ROI_DENSEPOSE_HEAD.DEEPLAB.NORM
|
86 |
-
self.n_stacked_convs = cfg.MODEL.ROI_DENSEPOSE_HEAD.NUM_STACKED_CONVS
|
87 |
-
self.use_nonlocal = cfg.MODEL.ROI_DENSEPOSE_HEAD.DEEPLAB.NONLOCAL_ON
|
88 |
-
# fmt: on
|
89 |
-
pad_size = kernel_size // 2
|
90 |
-
n_channels = input_channels
|
91 |
-
|
92 |
-
self.ASPP = ASPP(input_channels, [6, 12, 56], n_channels) # 6, 12, 56
|
93 |
-
self.add_module("ASPP", self.ASPP)
|
94 |
-
|
95 |
-
if self.use_nonlocal:
|
96 |
-
self.NLBlock = NONLocalBlock2D(input_channels, bn_layer=True)
|
97 |
-
self.add_module("NLBlock", self.NLBlock)
|
98 |
-
# weight_init.c2_msra_fill(self.ASPP)
|
99 |
-
|
100 |
-
for i in range(self.n_stacked_convs):
|
101 |
-
norm_module = nn.GroupNorm(32, hidden_dim) if norm == "GN" else None
|
102 |
-
layer = Conv2d(
|
103 |
-
n_channels,
|
104 |
-
hidden_dim,
|
105 |
-
kernel_size,
|
106 |
-
stride=1,
|
107 |
-
padding=pad_size,
|
108 |
-
bias=not norm,
|
109 |
-
norm=norm_module,
|
110 |
-
)
|
111 |
-
weight_init.c2_msra_fill(layer)
|
112 |
-
n_channels = hidden_dim
|
113 |
-
layer_name = self._get_layer_name(i)
|
114 |
-
self.add_module(layer_name, layer)
|
115 |
-
self.n_out_channels = hidden_dim
|
116 |
-
# initialize_module_params(self)
|
117 |
-
|
118 |
-
def forward(self, features):
|
119 |
-
x0 = features
|
120 |
-
x = self.ASPP(x0)
|
121 |
-
if self.use_nonlocal:
|
122 |
-
x = self.NLBlock(x)
|
123 |
-
output = x
|
124 |
-
for i in range(self.n_stacked_convs):
|
125 |
-
layer_name = self._get_layer_name(i)
|
126 |
-
x = getattr(self, layer_name)(x)
|
127 |
-
x = F.relu(x)
|
128 |
-
output = x
|
129 |
-
return output
|
130 |
-
|
131 |
-
def _get_layer_name(self, i):
|
132 |
-
layer_name = "body_conv_fcn{}".format(i + 1)
|
133 |
-
return layer_name
|
134 |
-
|
135 |
-
|
136 |
-
# Copied from
|
137 |
-
# https://github.com/pytorch/vision/blob/master/torchvision/models/segmentation/deeplabv3.py
|
138 |
-
# See https://arxiv.org/pdf/1706.05587.pdf for details
|
139 |
-
class ASPPConv(nn.Sequential):
|
140 |
-
def __init__(self, in_channels, out_channels, dilation):
|
141 |
-
modules = [
|
142 |
-
nn.Conv2d(
|
143 |
-
in_channels, out_channels, 3, padding=dilation, dilation=dilation, bias=False
|
144 |
-
),
|
145 |
-
nn.GroupNorm(32, out_channels),
|
146 |
-
nn.ReLU(),
|
147 |
-
]
|
148 |
-
super(ASPPConv, self).__init__(*modules)
|
149 |
-
|
150 |
-
|
151 |
-
class ASPPPooling(nn.Sequential):
|
152 |
-
def __init__(self, in_channels, out_channels):
|
153 |
-
super(ASPPPooling, self).__init__(
|
154 |
-
nn.AdaptiveAvgPool2d(1),
|
155 |
-
nn.Conv2d(in_channels, out_channels, 1, bias=False),
|
156 |
-
nn.GroupNorm(32, out_channels),
|
157 |
-
nn.ReLU(),
|
158 |
-
)
|
159 |
-
|
160 |
-
def forward(self, x):
|
161 |
-
size = x.shape[-2:]
|
162 |
-
x = super(ASPPPooling, self).forward(x)
|
163 |
-
return F.interpolate(x, size=size, mode="bilinear", align_corners=False)
|
164 |
-
|
165 |
-
|
166 |
-
class ASPP(nn.Module):
|
167 |
-
def __init__(self, in_channels, atrous_rates, out_channels):
|
168 |
-
super(ASPP, self).__init__()
|
169 |
-
modules = []
|
170 |
-
modules.append(
|
171 |
-
nn.Sequential(
|
172 |
-
nn.Conv2d(in_channels, out_channels, 1, bias=False),
|
173 |
-
nn.GroupNorm(32, out_channels),
|
174 |
-
nn.ReLU(),
|
175 |
-
)
|
176 |
-
)
|
177 |
-
|
178 |
-
rate1, rate2, rate3 = tuple(atrous_rates)
|
179 |
-
modules.append(ASPPConv(in_channels, out_channels, rate1))
|
180 |
-
modules.append(ASPPConv(in_channels, out_channels, rate2))
|
181 |
-
modules.append(ASPPConv(in_channels, out_channels, rate3))
|
182 |
-
modules.append(ASPPPooling(in_channels, out_channels))
|
183 |
-
|
184 |
-
self.convs = nn.ModuleList(modules)
|
185 |
-
|
186 |
-
self.project = nn.Sequential(
|
187 |
-
nn.Conv2d(5 * out_channels, out_channels, 1, bias=False),
|
188 |
-
# nn.BatchNorm2d(out_channels),
|
189 |
-
nn.ReLU()
|
190 |
-
# nn.Dropout(0.5)
|
191 |
-
)
|
192 |
-
|
193 |
-
def forward(self, x):
|
194 |
-
res = []
|
195 |
-
for conv in self.convs:
|
196 |
-
res.append(conv(x))
|
197 |
-
res = torch.cat(res, dim=1)
|
198 |
-
return self.project(res)
|
199 |
-
|
200 |
-
|
201 |
-
# copied from
|
202 |
-
# https://github.com/AlexHex7/Non-local_pytorch/blob/master/lib/non_local_embedded_gaussian.py
|
203 |
-
# See https://arxiv.org/abs/1711.07971 for details
|
204 |
-
class _NonLocalBlockND(nn.Module):
|
205 |
-
def __init__(
|
206 |
-
self, in_channels, inter_channels=None, dimension=3, sub_sample=True, bn_layer=True
|
207 |
-
):
|
208 |
-
super(_NonLocalBlockND, self).__init__()
|
209 |
-
|
210 |
-
assert dimension in [1, 2, 3]
|
211 |
-
|
212 |
-
self.dimension = dimension
|
213 |
-
self.sub_sample = sub_sample
|
214 |
-
|
215 |
-
self.in_channels = in_channels
|
216 |
-
self.inter_channels = inter_channels
|
217 |
-
|
218 |
-
if self.inter_channels is None:
|
219 |
-
self.inter_channels = in_channels // 2
|
220 |
-
if self.inter_channels == 0:
|
221 |
-
self.inter_channels = 1
|
222 |
-
|
223 |
-
if dimension == 3:
|
224 |
-
conv_nd = nn.Conv3d
|
225 |
-
max_pool_layer = nn.MaxPool3d(kernel_size=(1, 2, 2))
|
226 |
-
bn = nn.GroupNorm # (32, hidden_dim) #nn.BatchNorm3d
|
227 |
-
elif dimension == 2:
|
228 |
-
conv_nd = nn.Conv2d
|
229 |
-
max_pool_layer = nn.MaxPool2d(kernel_size=(2, 2))
|
230 |
-
bn = nn.GroupNorm # (32, hidden_dim)nn.BatchNorm2d
|
231 |
-
else:
|
232 |
-
conv_nd = nn.Conv1d
|
233 |
-
max_pool_layer = nn.MaxPool1d(kernel_size=(2))
|
234 |
-
bn = nn.GroupNorm # (32, hidden_dim)nn.BatchNorm1d
|
235 |
-
|
236 |
-
self.g = conv_nd(
|
237 |
-
in_channels=self.in_channels,
|
238 |
-
out_channels=self.inter_channels,
|
239 |
-
kernel_size=1,
|
240 |
-
stride=1,
|
241 |
-
padding=0,
|
242 |
-
)
|
243 |
-
|
244 |
-
if bn_layer:
|
245 |
-
self.W = nn.Sequential(
|
246 |
-
conv_nd(
|
247 |
-
in_channels=self.inter_channels,
|
248 |
-
out_channels=self.in_channels,
|
249 |
-
kernel_size=1,
|
250 |
-
stride=1,
|
251 |
-
padding=0,
|
252 |
-
),
|
253 |
-
bn(32, self.in_channels),
|
254 |
-
)
|
255 |
-
nn.init.constant_(self.W[1].weight, 0)
|
256 |
-
nn.init.constant_(self.W[1].bias, 0)
|
257 |
-
else:
|
258 |
-
self.W = conv_nd(
|
259 |
-
in_channels=self.inter_channels,
|
260 |
-
out_channels=self.in_channels,
|
261 |
-
kernel_size=1,
|
262 |
-
stride=1,
|
263 |
-
padding=0,
|
264 |
-
)
|
265 |
-
nn.init.constant_(self.W.weight, 0)
|
266 |
-
nn.init.constant_(self.W.bias, 0)
|
267 |
-
|
268 |
-
self.theta = conv_nd(
|
269 |
-
in_channels=self.in_channels,
|
270 |
-
out_channels=self.inter_channels,
|
271 |
-
kernel_size=1,
|
272 |
-
stride=1,
|
273 |
-
padding=0,
|
274 |
-
)
|
275 |
-
self.phi = conv_nd(
|
276 |
-
in_channels=self.in_channels,
|
277 |
-
out_channels=self.inter_channels,
|
278 |
-
kernel_size=1,
|
279 |
-
stride=1,
|
280 |
-
padding=0,
|
281 |
-
)
|
282 |
-
|
283 |
-
if sub_sample:
|
284 |
-
self.g = nn.Sequential(self.g, max_pool_layer)
|
285 |
-
self.phi = nn.Sequential(self.phi, max_pool_layer)
|
286 |
-
|
287 |
-
def forward(self, x):
|
288 |
-
"""
|
289 |
-
:param x: (b, c, t, h, w)
|
290 |
-
:return:
|
291 |
-
"""
|
292 |
-
|
293 |
-
batch_size = x.size(0)
|
294 |
-
|
295 |
-
g_x = self.g(x).view(batch_size, self.inter_channels, -1)
|
296 |
-
g_x = g_x.permute(0, 2, 1)
|
297 |
-
|
298 |
-
theta_x = self.theta(x).view(batch_size, self.inter_channels, -1)
|
299 |
-
theta_x = theta_x.permute(0, 2, 1)
|
300 |
-
phi_x = self.phi(x).view(batch_size, self.inter_channels, -1)
|
301 |
-
f = torch.matmul(theta_x, phi_x)
|
302 |
-
f_div_C = F.softmax(f, dim=-1)
|
303 |
-
|
304 |
-
y = torch.matmul(f_div_C, g_x)
|
305 |
-
y = y.permute(0, 2, 1).contiguous()
|
306 |
-
y = y.view(batch_size, self.inter_channels, *x.size()[2:])
|
307 |
-
W_y = self.W(y)
|
308 |
-
z = W_y + x
|
309 |
-
|
310 |
-
return z
|
311 |
-
|
312 |
-
|
313 |
-
class NONLocalBlock2D(_NonLocalBlockND):
|
314 |
-
def __init__(self, in_channels, inter_channels=None, sub_sample=True, bn_layer=True):
|
315 |
-
super(NONLocalBlock2D, self).__init__(
|
316 |
-
in_channels,
|
317 |
-
inter_channels=inter_channels,
|
318 |
-
dimension=2,
|
319 |
-
sub_sample=sub_sample,
|
320 |
-
bn_layer=bn_layer,
|
321 |
-
)
|
322 |
-
|
323 |
-
|
324 |
-
@ROI_DENSEPOSE_HEAD_REGISTRY.register()
|
325 |
-
class DensePoseV1ConvXHead(nn.Module):
|
326 |
-
def __init__(self, cfg, input_channels):
|
327 |
-
super(DensePoseV1ConvXHead, self).__init__()
|
328 |
-
# fmt: off
|
329 |
-
hidden_dim = cfg.MODEL.ROI_DENSEPOSE_HEAD.CONV_HEAD_DIM
|
330 |
-
kernel_size = cfg.MODEL.ROI_DENSEPOSE_HEAD.CONV_HEAD_KERNEL
|
331 |
-
self.n_stacked_convs = cfg.MODEL.ROI_DENSEPOSE_HEAD.NUM_STACKED_CONVS
|
332 |
-
# fmt: on
|
333 |
-
pad_size = kernel_size // 2
|
334 |
-
n_channels = input_channels
|
335 |
-
for i in range(self.n_stacked_convs):
|
336 |
-
layer = Conv2d(n_channels, hidden_dim, kernel_size, stride=1, padding=pad_size)
|
337 |
-
layer_name = self._get_layer_name(i)
|
338 |
-
self.add_module(layer_name, layer)
|
339 |
-
n_channels = hidden_dim
|
340 |
-
self.n_out_channels = n_channels
|
341 |
-
initialize_module_params(self)
|
342 |
-
|
343 |
-
def forward(self, features):
|
344 |
-
x = features
|
345 |
-
output = x
|
346 |
-
for i in range(self.n_stacked_convs):
|
347 |
-
layer_name = self._get_layer_name(i)
|
348 |
-
x = getattr(self, layer_name)(x)
|
349 |
-
x = F.relu(x)
|
350 |
-
output = x
|
351 |
-
return output
|
352 |
-
|
353 |
-
def _get_layer_name(self, i):
|
354 |
-
layer_name = "body_conv_fcn{}".format(i + 1)
|
355 |
-
return layer_name
|
356 |
-
|
357 |
-
|
358 |
-
class DensePosePredictor(nn.Module):
|
359 |
-
def __init__(self, cfg, input_channels):
|
360 |
-
|
361 |
-
super(DensePosePredictor, self).__init__()
|
362 |
-
dim_in = input_channels
|
363 |
-
n_segm_chan = cfg.MODEL.ROI_DENSEPOSE_HEAD.NUM_COARSE_SEGM_CHANNELS
|
364 |
-
dim_out_patches = cfg.MODEL.ROI_DENSEPOSE_HEAD.NUM_PATCHES + 1
|
365 |
-
kernel_size = cfg.MODEL.ROI_DENSEPOSE_HEAD.DECONV_KERNEL
|
366 |
-
self.ann_index_lowres = ConvTranspose2d(
|
367 |
-
dim_in, n_segm_chan, kernel_size, stride=2, padding=int(kernel_size / 2 - 1)
|
368 |
-
)
|
369 |
-
self.index_uv_lowres = ConvTranspose2d(
|
370 |
-
dim_in, dim_out_patches, kernel_size, stride=2, padding=int(kernel_size / 2 - 1)
|
371 |
-
)
|
372 |
-
self.u_lowres = ConvTranspose2d(
|
373 |
-
dim_in, dim_out_patches, kernel_size, stride=2, padding=int(kernel_size / 2 - 1)
|
374 |
-
)
|
375 |
-
self.v_lowres = ConvTranspose2d(
|
376 |
-
dim_in, dim_out_patches, kernel_size, stride=2, padding=int(kernel_size / 2 - 1)
|
377 |
-
)
|
378 |
-
self.scale_factor = cfg.MODEL.ROI_DENSEPOSE_HEAD.UP_SCALE
|
379 |
-
self.confidence_model_cfg = DensePoseConfidenceModelConfig.from_cfg(cfg)
|
380 |
-
self._initialize_confidence_estimation_layers(cfg, self.confidence_model_cfg, dim_in)
|
381 |
-
initialize_module_params(self)
|
382 |
-
|
383 |
-
def forward(self, head_outputs):
|
384 |
-
ann_index_lowres = self.ann_index_lowres(head_outputs)
|
385 |
-
index_uv_lowres = self.index_uv_lowres(head_outputs)
|
386 |
-
u_lowres = self.u_lowres(head_outputs)
|
387 |
-
v_lowres = self.v_lowres(head_outputs)
|
388 |
-
|
389 |
-
def interp2d(input):
|
390 |
-
return interpolate(
|
391 |
-
input, scale_factor=self.scale_factor, mode="bilinear", align_corners=False
|
392 |
-
)
|
393 |
-
|
394 |
-
ann_index = interp2d(ann_index_lowres)
|
395 |
-
index_uv = interp2d(index_uv_lowres)
|
396 |
-
u = interp2d(u_lowres)
|
397 |
-
v = interp2d(v_lowres)
|
398 |
-
(
|
399 |
-
(sigma_1, sigma_2, kappa_u, kappa_v),
|
400 |
-
(sigma_1_lowres, sigma_2_lowres, kappa_u_lowres, kappa_v_lowres),
|
401 |
-
(ann_index, index_uv),
|
402 |
-
) = self._forward_confidence_estimation_layers(
|
403 |
-
self.confidence_model_cfg, head_outputs, interp2d, ann_index, index_uv
|
404 |
-
)
|
405 |
-
return (
|
406 |
-
(ann_index, index_uv, u, v),
|
407 |
-
(ann_index_lowres, index_uv_lowres, u_lowres, v_lowres),
|
408 |
-
(sigma_1, sigma_2, kappa_u, kappa_v),
|
409 |
-
(sigma_1_lowres, sigma_2_lowres, kappa_u_lowres, kappa_v_lowres),
|
410 |
-
)
|
411 |
-
|
412 |
-
def _initialize_confidence_estimation_layers(
|
413 |
-
self, cfg: CfgNode, confidence_model_cfg: DensePoseConfidenceModelConfig, dim_in: int
|
414 |
-
):
|
415 |
-
dim_out_patches = cfg.MODEL.ROI_DENSEPOSE_HEAD.NUM_PATCHES + 1
|
416 |
-
kernel_size = cfg.MODEL.ROI_DENSEPOSE_HEAD.DECONV_KERNEL
|
417 |
-
if confidence_model_cfg.uv_confidence.enabled:
|
418 |
-
if confidence_model_cfg.uv_confidence.type == DensePoseUVConfidenceType.IID_ISO:
|
419 |
-
self.sigma_2_lowres = ConvTranspose2d(
|
420 |
-
dim_in, dim_out_patches, kernel_size, stride=2, padding=int(kernel_size / 2 - 1)
|
421 |
-
)
|
422 |
-
elif confidence_model_cfg.uv_confidence.type == DensePoseUVConfidenceType.INDEP_ANISO:
|
423 |
-
self.sigma_2_lowres = ConvTranspose2d(
|
424 |
-
dim_in, dim_out_patches, kernel_size, stride=2, padding=int(kernel_size / 2 - 1)
|
425 |
-
)
|
426 |
-
self.kappa_u_lowres = ConvTranspose2d(
|
427 |
-
dim_in, dim_out_patches, kernel_size, stride=2, padding=int(kernel_size / 2 - 1)
|
428 |
-
)
|
429 |
-
self.kappa_v_lowres = ConvTranspose2d(
|
430 |
-
dim_in, dim_out_patches, kernel_size, stride=2, padding=int(kernel_size / 2 - 1)
|
431 |
-
)
|
432 |
-
else:
|
433 |
-
raise ValueError(
|
434 |
-
f"Unknown confidence model type: {confidence_model_cfg.confidence_model_type}"
|
435 |
-
)
|
436 |
-
|
437 |
-
def _forward_confidence_estimation_layers(
|
438 |
-
self, confidence_model_cfg, head_outputs, interp2d, ann_index, index_uv
|
439 |
-
):
|
440 |
-
sigma_1, sigma_2, kappa_u, kappa_v = None, None, None, None
|
441 |
-
sigma_1_lowres, sigma_2_lowres, kappa_u_lowres, kappa_v_lowres = None, None, None, None
|
442 |
-
if confidence_model_cfg.uv_confidence.enabled:
|
443 |
-
if confidence_model_cfg.uv_confidence.type == DensePoseUVConfidenceType.IID_ISO:
|
444 |
-
sigma_2_lowres = self.sigma_2_lowres(head_outputs)
|
445 |
-
sigma_2 = interp2d(sigma_2_lowres)
|
446 |
-
elif confidence_model_cfg.uv_confidence.type == DensePoseUVConfidenceType.INDEP_ANISO:
|
447 |
-
sigma_2_lowres = self.sigma_2_lowres(head_outputs)
|
448 |
-
kappa_u_lowres = self.kappa_u_lowres(head_outputs)
|
449 |
-
kappa_v_lowres = self.kappa_v_lowres(head_outputs)
|
450 |
-
sigma_2 = interp2d(sigma_2_lowres)
|
451 |
-
kappa_u = interp2d(kappa_u_lowres)
|
452 |
-
kappa_v = interp2d(kappa_v_lowres)
|
453 |
-
else:
|
454 |
-
raise ValueError(
|
455 |
-
f"Unknown confidence model type: {confidence_model_cfg.confidence_model_type}"
|
456 |
-
)
|
457 |
-
return (
|
458 |
-
(sigma_1, sigma_2, kappa_u, kappa_v),
|
459 |
-
(sigma_1_lowres, sigma_2_lowres, kappa_u_lowres, kappa_v_lowres),
|
460 |
-
(ann_index, index_uv),
|
461 |
-
)
|
462 |
-
|
463 |
-
|
464 |
-
class DensePoseDataFilter(object):
|
465 |
-
def __init__(self, cfg):
|
466 |
-
self.iou_threshold = cfg.MODEL.ROI_DENSEPOSE_HEAD.FG_IOU_THRESHOLD
|
467 |
-
|
468 |
-
@torch.no_grad()
|
469 |
-
def __call__(self, proposals_with_targets):
|
470 |
-
"""
|
471 |
-
Filters proposals with targets to keep only the ones relevant for
|
472 |
-
DensePose training
|
473 |
-
proposals: list(Instances), each element of the list corresponds to
|
474 |
-
various instances (proposals, GT for boxes and densepose) for one
|
475 |
-
image
|
476 |
-
"""
|
477 |
-
proposals_filtered = []
|
478 |
-
for proposals_per_image in proposals_with_targets:
|
479 |
-
if not hasattr(proposals_per_image, "gt_densepose"):
|
480 |
-
continue
|
481 |
-
assert hasattr(proposals_per_image, "gt_boxes")
|
482 |
-
assert hasattr(proposals_per_image, "proposal_boxes")
|
483 |
-
gt_boxes = proposals_per_image.gt_boxes
|
484 |
-
est_boxes = proposals_per_image.proposal_boxes
|
485 |
-
# apply match threshold for densepose head
|
486 |
-
iou = matched_boxlist_iou(gt_boxes, est_boxes)
|
487 |
-
iou_select = iou > self.iou_threshold
|
488 |
-
proposals_per_image = proposals_per_image[iou_select]
|
489 |
-
assert len(proposals_per_image.gt_boxes) == len(proposals_per_image.proposal_boxes)
|
490 |
-
# filter out any target without densepose annotation
|
491 |
-
gt_densepose = proposals_per_image.gt_densepose
|
492 |
-
assert len(proposals_per_image.gt_boxes) == len(proposals_per_image.gt_densepose)
|
493 |
-
selected_indices = [
|
494 |
-
i for i, dp_target in enumerate(gt_densepose) if dp_target is not None
|
495 |
-
]
|
496 |
-
if len(selected_indices) != len(gt_densepose):
|
497 |
-
proposals_per_image = proposals_per_image[selected_indices]
|
498 |
-
assert len(proposals_per_image.gt_boxes) == len(proposals_per_image.proposal_boxes)
|
499 |
-
assert len(proposals_per_image.gt_boxes) == len(proposals_per_image.gt_densepose)
|
500 |
-
proposals_filtered.append(proposals_per_image)
|
501 |
-
return proposals_filtered
|
502 |
-
|
503 |
-
|
504 |
-
def build_densepose_head(cfg, input_channels):
|
505 |
-
head_name = cfg.MODEL.ROI_DENSEPOSE_HEAD.NAME
|
506 |
-
return ROI_DENSEPOSE_HEAD_REGISTRY.get(head_name)(cfg, input_channels)
|
507 |
-
|
508 |
-
|
509 |
-
def build_densepose_predictor(cfg, input_channels):
|
510 |
-
predictor = DensePosePredictor(cfg, input_channels)
|
511 |
-
return predictor
|
512 |
-
|
513 |
-
|
514 |
-
def build_densepose_data_filter(cfg):
|
515 |
-
dp_filter = DensePoseDataFilter(cfg)
|
516 |
-
return dp_filter
|
517 |
-
|
518 |
-
|
519 |
-
def densepose_inference(densepose_outputs, densepose_confidences, detections):
|
520 |
-
"""
|
521 |
-
Infer dense pose estimate based on outputs from the DensePose head
|
522 |
-
and detections. The estimate for each detection instance is stored in its
|
523 |
-
"pred_densepose" attribute.
|
524 |
-
|
525 |
-
Args:
|
526 |
-
densepose_outputs (tuple(`torch.Tensor`)): iterable containing 4 elements:
|
527 |
-
- s (:obj: `torch.Tensor`): coarse segmentation tensor of size (N, A, H, W),
|
528 |
-
- i (:obj: `torch.Tensor`): fine segmentation tensor of size (N, C, H, W),
|
529 |
-
- u (:obj: `torch.Tensor`): U coordinates for each class of size (N, C, H, W),
|
530 |
-
- v (:obj: `torch.Tensor`): V coordinates for each class of size (N, C, H, W),
|
531 |
-
where N is the total number of detections in a batch,
|
532 |
-
A is the number of coarse segmentations labels
|
533 |
-
(e.g. 15 for coarse body parts + background),
|
534 |
-
C is the number of fine segmentation labels
|
535 |
-
(e.g. 25 for fine body parts + background),
|
536 |
-
W is the resolution along the X axis
|
537 |
-
H is the resolution along the Y axis
|
538 |
-
densepose_confidences (tuple(`torch.Tensor`)): iterable containing 4 elements:
|
539 |
-
- sigma_1 (:obj: `torch.Tensor`): global confidences for UV coordinates
|
540 |
-
of size (N, C, H, W)
|
541 |
-
- sigma_2 (:obj: `torch.Tensor`): individual confidences for UV coordinates
|
542 |
-
of size (N, C, H, W)
|
543 |
-
- kappa_u (:obj: `torch.Tensor`): first component of confidence direction
|
544 |
-
vector of size (N, C, H, W)
|
545 |
-
- kappa_v (:obj: `torch.Tensor`): second component of confidence direction
|
546 |
-
vector of size (N, C, H, W)
|
547 |
-
detections (list[Instances]): A list of N Instances, where N is the number of images
|
548 |
-
in the batch. Instances are modified by this method: "pred_densepose" attribute
|
549 |
-
is added to each instance, the attribute contains the corresponding
|
550 |
-
DensePoseOutput object.
|
551 |
-
"""
|
552 |
-
# DensePose outputs: segmentation, body part indices, U, V
|
553 |
-
s, index_uv, u, v = densepose_outputs
|
554 |
-
sigma_1, sigma_2, kappa_u, kappa_v = densepose_confidences
|
555 |
-
k = 0
|
556 |
-
for detection in detections:
|
557 |
-
n_i = len(detection)
|
558 |
-
s_i = s[k : k + n_i]
|
559 |
-
index_uv_i = index_uv[k : k + n_i]
|
560 |
-
u_i = u[k : k + n_i]
|
561 |
-
v_i = v[k : k + n_i]
|
562 |
-
_local_vars = locals()
|
563 |
-
confidences = {
|
564 |
-
name: _local_vars[name]
|
565 |
-
for name in ("sigma_1", "sigma_2", "kappa_u", "kappa_v")
|
566 |
-
if _local_vars.get(name) is not None
|
567 |
-
}
|
568 |
-
densepose_output_i = DensePoseOutput(s_i, index_uv_i, u_i, v_i, confidences)
|
569 |
-
detection.pred_densepose = densepose_output_i
|
570 |
-
k += n_i
|
571 |
-
|
572 |
-
|
573 |
-
def _linear_interpolation_utilities(v_norm, v0_src, size_src, v0_dst, size_dst, size_z):
|
574 |
-
"""
|
575 |
-
Computes utility values for linear interpolation at points v.
|
576 |
-
The points are given as normalized offsets in the source interval
|
577 |
-
(v0_src, v0_src + size_src), more precisely:
|
578 |
-
v = v0_src + v_norm * size_src / 256.0
|
579 |
-
The computed utilities include lower points v_lo, upper points v_hi,
|
580 |
-
interpolation weights v_w and flags j_valid indicating whether the
|
581 |
-
points falls into the destination interval (v0_dst, v0_dst + size_dst).
|
582 |
-
|
583 |
-
Args:
|
584 |
-
v_norm (:obj: `torch.Tensor`): tensor of size N containing
|
585 |
-
normalized point offsets
|
586 |
-
v0_src (:obj: `torch.Tensor`): tensor of size N containing
|
587 |
-
left bounds of source intervals for normalized points
|
588 |
-
size_src (:obj: `torch.Tensor`): tensor of size N containing
|
589 |
-
source interval sizes for normalized points
|
590 |
-
v0_dst (:obj: `torch.Tensor`): tensor of size N containing
|
591 |
-
left bounds of destination intervals
|
592 |
-
size_dst (:obj: `torch.Tensor`): tensor of size N containing
|
593 |
-
destination interval sizes
|
594 |
-
size_z (int): interval size for data to be interpolated
|
595 |
-
|
596 |
-
Returns:
|
597 |
-
v_lo (:obj: `torch.Tensor`): int tensor of size N containing
|
598 |
-
indices of lower values used for interpolation, all values are
|
599 |
-
integers from [0, size_z - 1]
|
600 |
-
v_hi (:obj: `torch.Tensor`): int tensor of size N containing
|
601 |
-
indices of upper values used for interpolation, all values are
|
602 |
-
integers from [0, size_z - 1]
|
603 |
-
v_w (:obj: `torch.Tensor`): float tensor of size N containing
|
604 |
-
interpolation weights
|
605 |
-
j_valid (:obj: `torch.Tensor`): uint8 tensor of size N containing
|
606 |
-
0 for points outside the estimation interval
|
607 |
-
(v0_est, v0_est + size_est) and 1 otherwise
|
608 |
-
"""
|
609 |
-
v = v0_src + v_norm * size_src / 256.0
|
610 |
-
j_valid = (v - v0_dst >= 0) * (v - v0_dst < size_dst)
|
611 |
-
v_grid = (v - v0_dst) * size_z / size_dst
|
612 |
-
v_lo = v_grid.floor().long().clamp(min=0, max=size_z - 1)
|
613 |
-
v_hi = (v_lo + 1).clamp(max=size_z - 1)
|
614 |
-
v_grid = torch.min(v_hi.float(), v_grid)
|
615 |
-
v_w = v_grid - v_lo.float()
|
616 |
-
return v_lo, v_hi, v_w, j_valid
|
617 |
-
|
618 |
-
|
619 |
-
def _grid_sampling_utilities(
|
620 |
-
zh, zw, bbox_xywh_est, bbox_xywh_gt, index_gt, x_norm, y_norm, index_bbox
|
621 |
-
):
|
622 |
-
"""
|
623 |
-
Prepare tensors used in grid sampling.
|
624 |
-
|
625 |
-
Args:
|
626 |
-
z_est (:obj: `torch.Tensor`): tensor of size (N,C,H,W) with estimated
|
627 |
-
values of Z to be extracted for the points X, Y and channel
|
628 |
-
indices I
|
629 |
-
bbox_xywh_est (:obj: `torch.Tensor`): tensor of size (N, 4) containing
|
630 |
-
estimated bounding boxes in format XYWH
|
631 |
-
bbox_xywh_gt (:obj: `torch.Tensor`): tensor of size (N, 4) containing
|
632 |
-
matched ground truth bounding boxes in format XYWH
|
633 |
-
index_gt (:obj: `torch.Tensor`): tensor of size K with point labels for
|
634 |
-
ground truth points
|
635 |
-
x_norm (:obj: `torch.Tensor`): tensor of size K with X normalized
|
636 |
-
coordinates of ground truth points. Image X coordinates can be
|
637 |
-
obtained as X = Xbbox + x_norm * Wbbox / 255
|
638 |
-
y_norm (:obj: `torch.Tensor`): tensor of size K with Y normalized
|
639 |
-
coordinates of ground truth points. Image Y coordinates can be
|
640 |
-
obtained as Y = Ybbox + y_norm * Hbbox / 255
|
641 |
-
index_bbox (:obj: `torch.Tensor`): tensor of size K with bounding box
|
642 |
-
indices for each ground truth point. The values are thus in
|
643 |
-
[0, N-1]
|
644 |
-
|
645 |
-
Returns:
|
646 |
-
j_valid (:obj: `torch.Tensor`): uint8 tensor of size M containing
|
647 |
-
0 for points to be discarded and 1 for points to be selected
|
648 |
-
y_lo (:obj: `torch.Tensor`): int tensor of indices of upper values
|
649 |
-
in z_est for each point
|
650 |
-
y_hi (:obj: `torch.Tensor`): int tensor of indices of lower values
|
651 |
-
in z_est for each point
|
652 |
-
x_lo (:obj: `torch.Tensor`): int tensor of indices of left values
|
653 |
-
in z_est for each point
|
654 |
-
x_hi (:obj: `torch.Tensor`): int tensor of indices of right values
|
655 |
-
in z_est for each point
|
656 |
-
w_ylo_xlo (:obj: `torch.Tensor`): float tensor of size M;
|
657 |
-
contains upper-left value weight for each point
|
658 |
-
w_ylo_xhi (:obj: `torch.Tensor`): float tensor of size M;
|
659 |
-
contains upper-right value weight for each point
|
660 |
-
w_yhi_xlo (:obj: `torch.Tensor`): float tensor of size M;
|
661 |
-
contains lower-left value weight for each point
|
662 |
-
w_yhi_xhi (:obj: `torch.Tensor`): float tensor of size M;
|
663 |
-
contains lower-right value weight for each point
|
664 |
-
"""
|
665 |
-
|
666 |
-
x0_gt, y0_gt, w_gt, h_gt = bbox_xywh_gt[index_bbox].unbind(dim=1)
|
667 |
-
x0_est, y0_est, w_est, h_est = bbox_xywh_est[index_bbox].unbind(dim=1)
|
668 |
-
x_lo, x_hi, x_w, jx_valid = _linear_interpolation_utilities(
|
669 |
-
x_norm, x0_gt, w_gt, x0_est, w_est, zw
|
670 |
-
)
|
671 |
-
y_lo, y_hi, y_w, jy_valid = _linear_interpolation_utilities(
|
672 |
-
y_norm, y0_gt, h_gt, y0_est, h_est, zh
|
673 |
-
)
|
674 |
-
j_valid = jx_valid * jy_valid
|
675 |
-
|
676 |
-
w_ylo_xlo = (1.0 - x_w) * (1.0 - y_w)
|
677 |
-
w_ylo_xhi = x_w * (1.0 - y_w)
|
678 |
-
w_yhi_xlo = (1.0 - x_w) * y_w
|
679 |
-
w_yhi_xhi = x_w * y_w
|
680 |
-
|
681 |
-
return j_valid, y_lo, y_hi, x_lo, x_hi, w_ylo_xlo, w_ylo_xhi, w_yhi_xlo, w_yhi_xhi
|
682 |
-
|
683 |
-
|
684 |
-
def _extract_at_points_packed(
|
685 |
-
z_est,
|
686 |
-
index_bbox_valid,
|
687 |
-
slice_index_uv,
|
688 |
-
y_lo,
|
689 |
-
y_hi,
|
690 |
-
x_lo,
|
691 |
-
x_hi,
|
692 |
-
w_ylo_xlo,
|
693 |
-
w_ylo_xhi,
|
694 |
-
w_yhi_xlo,
|
695 |
-
w_yhi_xhi,
|
696 |
-
):
|
697 |
-
"""
|
698 |
-
Extract ground truth values z_gt for valid point indices and estimated
|
699 |
-
values z_est using bilinear interpolation over top-left (y_lo, x_lo),
|
700 |
-
top-right (y_lo, x_hi), bottom-left (y_hi, x_lo) and bottom-right
|
701 |
-
(y_hi, x_hi) values in z_est with corresponding weights:
|
702 |
-
w_ylo_xlo, w_ylo_xhi, w_yhi_xlo and w_yhi_xhi.
|
703 |
-
Use slice_index_uv to slice dim=1 in z_est
|
704 |
-
"""
|
705 |
-
z_est_sampled = (
|
706 |
-
z_est[index_bbox_valid, slice_index_uv, y_lo, x_lo] * w_ylo_xlo
|
707 |
-
+ z_est[index_bbox_valid, slice_index_uv, y_lo, x_hi] * w_ylo_xhi
|
708 |
-
+ z_est[index_bbox_valid, slice_index_uv, y_hi, x_lo] * w_yhi_xlo
|
709 |
-
+ z_est[index_bbox_valid, slice_index_uv, y_hi, x_hi] * w_yhi_xhi
|
710 |
-
)
|
711 |
-
return z_est_sampled
|
712 |
-
|
713 |
-
|
714 |
-
def _resample_data(
|
715 |
-
z, bbox_xywh_src, bbox_xywh_dst, wout, hout, mode="nearest", padding_mode="zeros"
|
716 |
-
):
|
717 |
-
"""
|
718 |
-
Args:
|
719 |
-
z (:obj: `torch.Tensor`): tensor of size (N,C,H,W) with data to be
|
720 |
-
resampled
|
721 |
-
bbox_xywh_src (:obj: `torch.Tensor`): tensor of size (N,4) containing
|
722 |
-
source bounding boxes in format XYWH
|
723 |
-
bbox_xywh_dst (:obj: `torch.Tensor`): tensor of size (N,4) containing
|
724 |
-
destination bounding boxes in format XYWH
|
725 |
-
Return:
|
726 |
-
zresampled (:obj: `torch.Tensor`): tensor of size (N, C, Hout, Wout)
|
727 |
-
with resampled values of z, where D is the discretization size
|
728 |
-
"""
|
729 |
-
n = bbox_xywh_src.size(0)
|
730 |
-
assert n == bbox_xywh_dst.size(0), (
|
731 |
-
"The number of "
|
732 |
-
"source ROIs for resampling ({}) should be equal to the number "
|
733 |
-
"of destination ROIs ({})".format(bbox_xywh_src.size(0), bbox_xywh_dst.size(0))
|
734 |
-
)
|
735 |
-
x0src, y0src, wsrc, hsrc = bbox_xywh_src.unbind(dim=1)
|
736 |
-
x0dst, y0dst, wdst, hdst = bbox_xywh_dst.unbind(dim=1)
|
737 |
-
x0dst_norm = 2 * (x0dst - x0src) / wsrc - 1
|
738 |
-
y0dst_norm = 2 * (y0dst - y0src) / hsrc - 1
|
739 |
-
x1dst_norm = 2 * (x0dst + wdst - x0src) / wsrc - 1
|
740 |
-
y1dst_norm = 2 * (y0dst + hdst - y0src) / hsrc - 1
|
741 |
-
grid_w = torch.arange(wout, device=z.device, dtype=torch.float) / wout
|
742 |
-
grid_h = torch.arange(hout, device=z.device, dtype=torch.float) / hout
|
743 |
-
grid_w_expanded = grid_w[None, None, :].expand(n, hout, wout)
|
744 |
-
grid_h_expanded = grid_h[None, :, None].expand(n, hout, wout)
|
745 |
-
dx_expanded = (x1dst_norm - x0dst_norm)[:, None, None].expand(n, hout, wout)
|
746 |
-
dy_expanded = (y1dst_norm - y0dst_norm)[:, None, None].expand(n, hout, wout)
|
747 |
-
x0_expanded = x0dst_norm[:, None, None].expand(n, hout, wout)
|
748 |
-
y0_expanded = y0dst_norm[:, None, None].expand(n, hout, wout)
|
749 |
-
grid_x = grid_w_expanded * dx_expanded + x0_expanded
|
750 |
-
grid_y = grid_h_expanded * dy_expanded + y0_expanded
|
751 |
-
grid = torch.stack((grid_x, grid_y), dim=3)
|
752 |
-
# resample Z from (N, C, H, W) into (N, C, Hout, Wout)
|
753 |
-
zresampled = F.grid_sample(z, grid, mode=mode, padding_mode=padding_mode, align_corners=True)
|
754 |
-
return zresampled
|
755 |
-
|
756 |
-
|
757 |
-
def _extract_single_tensors_from_matches_one_image(
|
758 |
-
proposals_targets, bbox_with_dp_offset, bbox_global_offset
|
759 |
-
):
|
760 |
-
i_gt_all = []
|
761 |
-
x_norm_all = []
|
762 |
-
y_norm_all = []
|
763 |
-
u_gt_all = []
|
764 |
-
v_gt_all = []
|
765 |
-
s_gt_all = []
|
766 |
-
bbox_xywh_gt_all = []
|
767 |
-
bbox_xywh_est_all = []
|
768 |
-
# Ibbox_all == k should be true for all data that corresponds
|
769 |
-
# to bbox_xywh_gt[k] and bbox_xywh_est[k]
|
770 |
-
# index k here is global wrt images
|
771 |
-
i_bbox_all = []
|
772 |
-
# at offset k (k is global) contains index of bounding box data
|
773 |
-
# within densepose output tensor
|
774 |
-
i_with_dp = []
|
775 |
-
|
776 |
-
boxes_xywh_est = proposals_targets.proposal_boxes.clone()
|
777 |
-
boxes_xywh_gt = proposals_targets.gt_boxes.clone()
|
778 |
-
n_i = len(boxes_xywh_est)
|
779 |
-
assert n_i == len(boxes_xywh_gt)
|
780 |
-
|
781 |
-
if n_i:
|
782 |
-
boxes_xywh_est.tensor[:, 2] -= boxes_xywh_est.tensor[:, 0]
|
783 |
-
boxes_xywh_est.tensor[:, 3] -= boxes_xywh_est.tensor[:, 1]
|
784 |
-
boxes_xywh_gt.tensor[:, 2] -= boxes_xywh_gt.tensor[:, 0]
|
785 |
-
boxes_xywh_gt.tensor[:, 3] -= boxes_xywh_gt.tensor[:, 1]
|
786 |
-
if hasattr(proposals_targets, "gt_densepose"):
|
787 |
-
densepose_gt = proposals_targets.gt_densepose
|
788 |
-
for k, box_xywh_est, box_xywh_gt, dp_gt in zip(
|
789 |
-
range(n_i), boxes_xywh_est.tensor, boxes_xywh_gt.tensor, densepose_gt
|
790 |
-
):
|
791 |
-
if (dp_gt is not None) and (len(dp_gt.x) > 0):
|
792 |
-
i_gt_all.append(dp_gt.i)
|
793 |
-
x_norm_all.append(dp_gt.x)
|
794 |
-
y_norm_all.append(dp_gt.y)
|
795 |
-
u_gt_all.append(dp_gt.u)
|
796 |
-
v_gt_all.append(dp_gt.v)
|
797 |
-
s_gt_all.append(dp_gt.segm.unsqueeze(0))
|
798 |
-
bbox_xywh_gt_all.append(box_xywh_gt.view(-1, 4))
|
799 |
-
bbox_xywh_est_all.append(box_xywh_est.view(-1, 4))
|
800 |
-
i_bbox_k = torch.full_like(dp_gt.i, bbox_with_dp_offset + len(i_with_dp))
|
801 |
-
i_bbox_all.append(i_bbox_k)
|
802 |
-
i_with_dp.append(bbox_global_offset + k)
|
803 |
-
return (
|
804 |
-
i_gt_all,
|
805 |
-
x_norm_all,
|
806 |
-
y_norm_all,
|
807 |
-
u_gt_all,
|
808 |
-
v_gt_all,
|
809 |
-
s_gt_all,
|
810 |
-
bbox_xywh_gt_all,
|
811 |
-
bbox_xywh_est_all,
|
812 |
-
i_bbox_all,
|
813 |
-
i_with_dp,
|
814 |
-
)
|
815 |
-
|
816 |
-
|
817 |
-
def _extract_single_tensors_from_matches(proposals_with_targets):
|
818 |
-
i_img = []
|
819 |
-
i_gt_all = []
|
820 |
-
x_norm_all = []
|
821 |
-
y_norm_all = []
|
822 |
-
u_gt_all = []
|
823 |
-
v_gt_all = []
|
824 |
-
s_gt_all = []
|
825 |
-
bbox_xywh_gt_all = []
|
826 |
-
bbox_xywh_est_all = []
|
827 |
-
i_bbox_all = []
|
828 |
-
i_with_dp_all = []
|
829 |
-
n = 0
|
830 |
-
for i, proposals_targets_per_image in enumerate(proposals_with_targets):
|
831 |
-
n_i = proposals_targets_per_image.proposal_boxes.tensor.size(0)
|
832 |
-
if not n_i:
|
833 |
-
continue
|
834 |
-
i_gt_img, x_norm_img, y_norm_img, u_gt_img, v_gt_img, s_gt_img, bbox_xywh_gt_img, bbox_xywh_est_img, i_bbox_img, i_with_dp_img = _extract_single_tensors_from_matches_one_image( # noqa
|
835 |
-
proposals_targets_per_image, len(i_with_dp_all), n
|
836 |
-
)
|
837 |
-
i_gt_all.extend(i_gt_img)
|
838 |
-
x_norm_all.extend(x_norm_img)
|
839 |
-
y_norm_all.extend(y_norm_img)
|
840 |
-
u_gt_all.extend(u_gt_img)
|
841 |
-
v_gt_all.extend(v_gt_img)
|
842 |
-
s_gt_all.extend(s_gt_img)
|
843 |
-
bbox_xywh_gt_all.extend(bbox_xywh_gt_img)
|
844 |
-
bbox_xywh_est_all.extend(bbox_xywh_est_img)
|
845 |
-
i_bbox_all.extend(i_bbox_img)
|
846 |
-
i_with_dp_all.extend(i_with_dp_img)
|
847 |
-
i_img.extend([i] * len(i_with_dp_img))
|
848 |
-
n += n_i
|
849 |
-
# concatenate all data into a single tensor
|
850 |
-
if (n > 0) and (len(i_with_dp_all) > 0):
|
851 |
-
i_gt = torch.cat(i_gt_all, 0).long()
|
852 |
-
x_norm = torch.cat(x_norm_all, 0)
|
853 |
-
y_norm = torch.cat(y_norm_all, 0)
|
854 |
-
u_gt = torch.cat(u_gt_all, 0)
|
855 |
-
v_gt = torch.cat(v_gt_all, 0)
|
856 |
-
s_gt = torch.cat(s_gt_all, 0)
|
857 |
-
bbox_xywh_gt = torch.cat(bbox_xywh_gt_all, 0)
|
858 |
-
bbox_xywh_est = torch.cat(bbox_xywh_est_all, 0)
|
859 |
-
i_bbox = torch.cat(i_bbox_all, 0).long()
|
860 |
-
else:
|
861 |
-
i_gt = None
|
862 |
-
x_norm = None
|
863 |
-
y_norm = None
|
864 |
-
u_gt = None
|
865 |
-
v_gt = None
|
866 |
-
s_gt = None
|
867 |
-
bbox_xywh_gt = None
|
868 |
-
bbox_xywh_est = None
|
869 |
-
i_bbox = None
|
870 |
-
return (
|
871 |
-
i_img,
|
872 |
-
i_with_dp_all,
|
873 |
-
bbox_xywh_est,
|
874 |
-
bbox_xywh_gt,
|
875 |
-
i_gt,
|
876 |
-
x_norm,
|
877 |
-
y_norm,
|
878 |
-
u_gt,
|
879 |
-
v_gt,
|
880 |
-
s_gt,
|
881 |
-
i_bbox,
|
882 |
-
)
|
883 |
-
|
884 |
-
|
885 |
-
class IIDIsotropicGaussianUVLoss(nn.Module):
|
886 |
-
"""
|
887 |
-
Loss for the case of iid residuals with isotropic covariance:
|
888 |
-
$Sigma_i = sigma_i^2 I$
|
889 |
-
The loss (negative log likelihood) is then:
|
890 |
-
$1/2 sum_{i=1}^n (log(2 pi) + 2 log sigma_i^2 + ||delta_i||^2 / sigma_i^2)$,
|
891 |
-
where $delta_i=(u - u', v - v')$ is a 2D vector containing UV coordinates
|
892 |
-
difference between estimated and ground truth UV values
|
893 |
-
For details, see:
|
894 |
-
N. Neverova, D. Novotny, A. Vedaldi "Correlated Uncertainty for Learning
|
895 |
-
Dense Correspondences from Noisy Labels", p. 918--926, in Proc. NIPS 2019
|
896 |
-
"""
|
897 |
-
|
898 |
-
def __init__(self, sigma_lower_bound: float):
|
899 |
-
super(IIDIsotropicGaussianUVLoss, self).__init__()
|
900 |
-
self.sigma_lower_bound = sigma_lower_bound
|
901 |
-
self.log2pi = math.log(2 * math.pi)
|
902 |
-
|
903 |
-
def forward(
|
904 |
-
self,
|
905 |
-
u: torch.Tensor,
|
906 |
-
v: torch.Tensor,
|
907 |
-
sigma_u: torch.Tensor,
|
908 |
-
target_u: torch.Tensor,
|
909 |
-
target_v: torch.Tensor,
|
910 |
-
):
|
911 |
-
# compute $\sigma_i^2$
|
912 |
-
# use sigma_lower_bound to avoid degenerate solution for variance
|
913 |
-
# (sigma -> 0)
|
914 |
-
sigma2 = F.softplus(sigma_u) + self.sigma_lower_bound
|
915 |
-
# compute \|delta_i\|^2
|
916 |
-
delta_t_delta = (u - target_u) ** 2 + (v - target_v) ** 2
|
917 |
-
# the total loss from the formula above:
|
918 |
-
loss = 0.5 * (self.log2pi + 2 * torch.log(sigma2) + delta_t_delta / sigma2)
|
919 |
-
return loss.sum()
|
920 |
-
|
921 |
-
|
922 |
-
class IndepAnisotropicGaussianUVLoss(nn.Module):
|
923 |
-
"""
|
924 |
-
Loss for the case of independent residuals with anisotropic covariances:
|
925 |
-
$Sigma_i = sigma_i^2 I + r_i r_i^T$
|
926 |
-
The loss (negative log likelihood) is then:
|
927 |
-
$1/2 sum_{i=1}^n (log(2 pi)
|
928 |
-
+ log sigma_i^2 (sigma_i^2 + ||r_i||^2)
|
929 |
-
+ ||delta_i||^2 / sigma_i^2
|
930 |
-
- <delta_i, r_i>^2 / (sigma_i^2 * (sigma_i^2 + ||r_i||^2)))$,
|
931 |
-
where $delta_i=(u - u', v - v')$ is a 2D vector containing UV coordinates
|
932 |
-
difference between estimated and ground truth UV values
|
933 |
-
For details, see:
|
934 |
-
N. Neverova, D. Novotny, A. Vedaldi "Correlated Uncertainty for Learning
|
935 |
-
Dense Correspondences from Noisy Labels", p. 918--926, in Proc. NIPS 2019
|
936 |
-
"""
|
937 |
-
|
938 |
-
def __init__(self, sigma_lower_bound: float):
|
939 |
-
super(IndepAnisotropicGaussianUVLoss, self).__init__()
|
940 |
-
self.sigma_lower_bound = sigma_lower_bound
|
941 |
-
self.log2pi = math.log(2 * math.pi)
|
942 |
-
|
943 |
-
def forward(
|
944 |
-
self,
|
945 |
-
u: torch.Tensor,
|
946 |
-
v: torch.Tensor,
|
947 |
-
sigma_u: torch.Tensor,
|
948 |
-
kappa_u_est: torch.Tensor,
|
949 |
-
kappa_v_est: torch.Tensor,
|
950 |
-
target_u: torch.Tensor,
|
951 |
-
target_v: torch.Tensor,
|
952 |
-
):
|
953 |
-
# compute $\sigma_i^2$
|
954 |
-
sigma2 = F.softplus(sigma_u) + self.sigma_lower_bound
|
955 |
-
# compute \|r_i\|^2
|
956 |
-
r_sqnorm2 = kappa_u_est ** 2 + kappa_v_est ** 2
|
957 |
-
delta_u = u - target_u
|
958 |
-
delta_v = v - target_v
|
959 |
-
# compute \|delta_i\|^2
|
960 |
-
delta_sqnorm = delta_u ** 2 + delta_v ** 2
|
961 |
-
delta_u_r_u = delta_u * kappa_u_est
|
962 |
-
delta_v_r_v = delta_v * kappa_v_est
|
963 |
-
# compute the scalar product <delta_i, r_i>
|
964 |
-
delta_r = delta_u_r_u + delta_v_r_v
|
965 |
-
# compute squared scalar product <delta_i, r_i>^2
|
966 |
-
delta_r_sqnorm = delta_r ** 2
|
967 |
-
denom2 = sigma2 * (sigma2 + r_sqnorm2)
|
968 |
-
loss = 0.5 * (
|
969 |
-
self.log2pi + torch.log(denom2) + delta_sqnorm / sigma2 - delta_r_sqnorm / denom2
|
970 |
-
)
|
971 |
-
return loss.sum()
|
972 |
-
|
973 |
-
|
974 |
-
class DensePoseLosses(object):
|
975 |
-
def __init__(self, cfg):
|
976 |
-
# fmt: off
|
977 |
-
self.heatmap_size = cfg.MODEL.ROI_DENSEPOSE_HEAD.HEATMAP_SIZE
|
978 |
-
self.w_points = cfg.MODEL.ROI_DENSEPOSE_HEAD.POINT_REGRESSION_WEIGHTS
|
979 |
-
self.w_part = cfg.MODEL.ROI_DENSEPOSE_HEAD.PART_WEIGHTS
|
980 |
-
self.w_segm = cfg.MODEL.ROI_DENSEPOSE_HEAD.INDEX_WEIGHTS
|
981 |
-
self.n_segm_chan = cfg.MODEL.ROI_DENSEPOSE_HEAD.NUM_COARSE_SEGM_CHANNELS
|
982 |
-
# fmt: on
|
983 |
-
self.confidence_model_cfg = DensePoseConfidenceModelConfig.from_cfg(cfg)
|
984 |
-
if self.confidence_model_cfg.uv_confidence.type == DensePoseUVConfidenceType.IID_ISO:
|
985 |
-
self.uv_loss_with_confidences = IIDIsotropicGaussianUVLoss(
|
986 |
-
self.confidence_model_cfg.uv_confidence.epsilon
|
987 |
-
)
|
988 |
-
elif self.confidence_model_cfg.uv_confidence.type == DensePoseUVConfidenceType.INDEP_ANISO:
|
989 |
-
self.uv_loss_with_confidences = IndepAnisotropicGaussianUVLoss(
|
990 |
-
self.confidence_model_cfg.uv_confidence.epsilon
|
991 |
-
)
|
992 |
-
|
993 |
-
def __call__(self, proposals_with_gt, densepose_outputs, densepose_confidences):
|
994 |
-
losses = {}
|
995 |
-
# densepose outputs are computed for all images and all bounding boxes;
|
996 |
-
# i.e. if a batch has 4 images with (3, 1, 2, 1) proposals respectively,
|
997 |
-
# the outputs will have size(0) == 3+1+2+1 == 7
|
998 |
-
s, index_uv, u, v = densepose_outputs
|
999 |
-
sigma_1, sigma_2, kappa_u, kappa_v = densepose_confidences
|
1000 |
-
conf_type = self.confidence_model_cfg.uv_confidence.type
|
1001 |
-
assert u.size(2) == v.size(2)
|
1002 |
-
assert u.size(3) == v.size(3)
|
1003 |
-
assert u.size(2) == index_uv.size(2)
|
1004 |
-
assert u.size(3) == index_uv.size(3)
|
1005 |
-
|
1006 |
-
with torch.no_grad():
|
1007 |
-
index_uv_img, i_with_dp, bbox_xywh_est, bbox_xywh_gt, index_gt_all, x_norm, y_norm, u_gt_all, v_gt_all, s_gt, index_bbox = _extract_single_tensors_from_matches( # noqa
|
1008 |
-
proposals_with_gt
|
1009 |
-
)
|
1010 |
-
n_batch = len(i_with_dp)
|
1011 |
-
|
1012 |
-
# NOTE: we need to keep the same computation graph on all the GPUs to
|
1013 |
-
# perform reduction properly. Hence even if we have no data on one
|
1014 |
-
# of the GPUs, we still need to generate the computation graph.
|
1015 |
-
# Add fake (zero) loss in the form Tensor.sum() * 0
|
1016 |
-
if not n_batch:
|
1017 |
-
losses["loss_densepose_I"] = index_uv.sum() * 0
|
1018 |
-
losses["loss_densepose_S"] = s.sum() * 0
|
1019 |
-
if self.confidence_model_cfg.uv_confidence.enabled:
|
1020 |
-
losses["loss_densepose_UV"] = (u.sum() + v.sum()) * 0
|
1021 |
-
if conf_type == DensePoseUVConfidenceType.IID_ISO:
|
1022 |
-
losses["loss_densepose_UV"] += sigma_2.sum() * 0
|
1023 |
-
elif conf_type == DensePoseUVConfidenceType.INDEP_ANISO:
|
1024 |
-
losses["loss_densepose_UV"] += (
|
1025 |
-
sigma_2.sum() + kappa_u.sum() + kappa_v.sum()
|
1026 |
-
) * 0
|
1027 |
-
else:
|
1028 |
-
losses["loss_densepose_U"] = u.sum() * 0
|
1029 |
-
losses["loss_densepose_V"] = v.sum() * 0
|
1030 |
-
return losses
|
1031 |
-
|
1032 |
-
zh = u.size(2)
|
1033 |
-
zw = u.size(3)
|
1034 |
-
|
1035 |
-
j_valid, y_lo, y_hi, x_lo, x_hi, w_ylo_xlo, w_ylo_xhi, w_yhi_xlo, w_yhi_xhi = _grid_sampling_utilities( # noqa
|
1036 |
-
zh, zw, bbox_xywh_est, bbox_xywh_gt, index_gt_all, x_norm, y_norm, index_bbox
|
1037 |
-
)
|
1038 |
-
|
1039 |
-
j_valid_fg = j_valid * (index_gt_all > 0)
|
1040 |
-
|
1041 |
-
u_gt = u_gt_all[j_valid_fg]
|
1042 |
-
u_est_all = _extract_at_points_packed(
|
1043 |
-
u[i_with_dp],
|
1044 |
-
index_bbox,
|
1045 |
-
index_gt_all,
|
1046 |
-
y_lo,
|
1047 |
-
y_hi,
|
1048 |
-
x_lo,
|
1049 |
-
x_hi,
|
1050 |
-
w_ylo_xlo,
|
1051 |
-
w_ylo_xhi,
|
1052 |
-
w_yhi_xlo,
|
1053 |
-
w_yhi_xhi,
|
1054 |
-
)
|
1055 |
-
u_est = u_est_all[j_valid_fg]
|
1056 |
-
|
1057 |
-
v_gt = v_gt_all[j_valid_fg]
|
1058 |
-
v_est_all = _extract_at_points_packed(
|
1059 |
-
v[i_with_dp],
|
1060 |
-
index_bbox,
|
1061 |
-
index_gt_all,
|
1062 |
-
y_lo,
|
1063 |
-
y_hi,
|
1064 |
-
x_lo,
|
1065 |
-
x_hi,
|
1066 |
-
w_ylo_xlo,
|
1067 |
-
w_ylo_xhi,
|
1068 |
-
w_yhi_xlo,
|
1069 |
-
w_yhi_xhi,
|
1070 |
-
)
|
1071 |
-
v_est = v_est_all[j_valid_fg]
|
1072 |
-
|
1073 |
-
index_uv_gt = index_gt_all[j_valid]
|
1074 |
-
index_uv_est_all = _extract_at_points_packed(
|
1075 |
-
index_uv[i_with_dp],
|
1076 |
-
index_bbox,
|
1077 |
-
slice(None),
|
1078 |
-
y_lo,
|
1079 |
-
y_hi,
|
1080 |
-
x_lo,
|
1081 |
-
x_hi,
|
1082 |
-
w_ylo_xlo[:, None],
|
1083 |
-
w_ylo_xhi[:, None],
|
1084 |
-
w_yhi_xlo[:, None],
|
1085 |
-
w_yhi_xhi[:, None],
|
1086 |
-
)
|
1087 |
-
index_uv_est = index_uv_est_all[j_valid, :]
|
1088 |
-
|
1089 |
-
if self.confidence_model_cfg.uv_confidence.enabled:
|
1090 |
-
sigma_2_est_all = _extract_at_points_packed(
|
1091 |
-
sigma_2[i_with_dp],
|
1092 |
-
index_bbox,
|
1093 |
-
index_gt_all,
|
1094 |
-
y_lo,
|
1095 |
-
y_hi,
|
1096 |
-
x_lo,
|
1097 |
-
x_hi,
|
1098 |
-
w_ylo_xlo,
|
1099 |
-
w_ylo_xhi,
|
1100 |
-
w_yhi_xlo,
|
1101 |
-
w_yhi_xhi,
|
1102 |
-
)
|
1103 |
-
sigma_2_est = sigma_2_est_all[j_valid_fg]
|
1104 |
-
if conf_type in [DensePoseUVConfidenceType.INDEP_ANISO]:
|
1105 |
-
kappa_u_est_all = _extract_at_points_packed(
|
1106 |
-
kappa_u[i_with_dp],
|
1107 |
-
index_bbox,
|
1108 |
-
index_gt_all,
|
1109 |
-
y_lo,
|
1110 |
-
y_hi,
|
1111 |
-
x_lo,
|
1112 |
-
x_hi,
|
1113 |
-
w_ylo_xlo,
|
1114 |
-
w_ylo_xhi,
|
1115 |
-
w_yhi_xlo,
|
1116 |
-
w_yhi_xhi,
|
1117 |
-
)
|
1118 |
-
kappa_u_est = kappa_u_est_all[j_valid_fg]
|
1119 |
-
kappa_v_est_all = _extract_at_points_packed(
|
1120 |
-
kappa_v[i_with_dp],
|
1121 |
-
index_bbox,
|
1122 |
-
index_gt_all,
|
1123 |
-
y_lo,
|
1124 |
-
y_hi,
|
1125 |
-
x_lo,
|
1126 |
-
x_hi,
|
1127 |
-
w_ylo_xlo,
|
1128 |
-
w_ylo_xhi,
|
1129 |
-
w_yhi_xlo,
|
1130 |
-
w_yhi_xhi,
|
1131 |
-
)
|
1132 |
-
kappa_v_est = kappa_v_est_all[j_valid_fg]
|
1133 |
-
|
1134 |
-
# Resample everything to the estimated data size, no need to resample
|
1135 |
-
# S_est then:
|
1136 |
-
s_est = s[i_with_dp]
|
1137 |
-
with torch.no_grad():
|
1138 |
-
s_gt = _resample_data(
|
1139 |
-
s_gt.unsqueeze(1),
|
1140 |
-
bbox_xywh_gt,
|
1141 |
-
bbox_xywh_est,
|
1142 |
-
self.heatmap_size,
|
1143 |
-
self.heatmap_size,
|
1144 |
-
mode="nearest",
|
1145 |
-
padding_mode="zeros",
|
1146 |
-
).squeeze(1)
|
1147 |
-
|
1148 |
-
# add point-based losses:
|
1149 |
-
if self.confidence_model_cfg.uv_confidence.enabled:
|
1150 |
-
if conf_type == DensePoseUVConfidenceType.IID_ISO:
|
1151 |
-
uv_loss = (
|
1152 |
-
self.uv_loss_with_confidences(u_est, v_est, sigma_2_est, u_gt, v_gt)
|
1153 |
-
* self.w_points
|
1154 |
-
)
|
1155 |
-
losses["loss_densepose_UV"] = uv_loss
|
1156 |
-
elif conf_type == DensePoseUVConfidenceType.INDEP_ANISO:
|
1157 |
-
uv_loss = (
|
1158 |
-
self.uv_loss_with_confidences(
|
1159 |
-
u_est, v_est, sigma_2_est, kappa_u_est, kappa_v_est, u_gt, v_gt
|
1160 |
-
)
|
1161 |
-
* self.w_points
|
1162 |
-
)
|
1163 |
-
losses["loss_densepose_UV"] = uv_loss
|
1164 |
-
else:
|
1165 |
-
raise ValueError(f"Unknown confidence model type: {conf_type}")
|
1166 |
-
else:
|
1167 |
-
u_loss = F.smooth_l1_loss(u_est, u_gt, reduction="sum") * self.w_points
|
1168 |
-
losses["loss_densepose_U"] = u_loss
|
1169 |
-
v_loss = F.smooth_l1_loss(v_est, v_gt, reduction="sum") * self.w_points
|
1170 |
-
losses["loss_densepose_V"] = v_loss
|
1171 |
-
index_uv_loss = F.cross_entropy(index_uv_est, index_uv_gt.long()) * self.w_part
|
1172 |
-
losses["loss_densepose_I"] = index_uv_loss
|
1173 |
-
|
1174 |
-
if self.n_segm_chan == 2:
|
1175 |
-
s_gt = s_gt > 0
|
1176 |
-
s_loss = F.cross_entropy(s_est, s_gt.long()) * self.w_segm
|
1177 |
-
losses["loss_densepose_S"] = s_loss
|
1178 |
-
return losses
|
1179 |
-
|
1180 |
-
|
1181 |
-
def build_densepose_losses(cfg):
|
1182 |
-
losses = DensePoseLosses(cfg)
|
1183 |
-
return losses
|
|
|
|
|
|
|
|
|
|
|
|
|
|
|
|
|
|
|
|
|
|
|
|
|
|
|
|
|
|
|
|
|
|
|
|
|
|
|
|
|
|
|
|
|
|
|
|
|
|
|
|
|
|
|
|
|
|
|
|
|
|
|
|
|
|
|
|
|
|
|
|
|
|
|
|
|
|
|
|
|
|
|
|
|
|
|
|
|
|
|
|
|
|
|
|
|
|
|
|
|
|
|
|
|
|
|
|
|
|
|
|
|
|
|
|
|
|
|
|
|
|
|
|
|
|
|
|
|
|
|
|
|
|
|
|
|
|
|
|
|
|
|
|
|
|
|
|
|
|
|
|
|
|
|
|
|
|
|
|
|
|
|
|
|
|
|
|
|
|
|
|
|
|
|
|
|
|
|
|
|
|
|
|
|
|
|
|
|
|
|
|
|
|
|
|
|
|
|
|
|
|
|
|
|
|
|
|
|
|
|
|
|
|
|
|
|
|
|
|
|
|
|
|
|
|
|
|
|
|
|
|
|
|
|
|
|
|
|
|
|
|
|
|
|
|
|
|
|
|
|
|
|
|
|
|
|
|
|
|
|
|
|
|
|
|
|
|
|
|
|
|
|
|
|
|
|
|
|
|
|
|
|
|
|
|
|
|
|
|
|
|
|
|
|
|
|
|
|
|
|
|
|
|
|
|
|
|
|
|
|
|
|
|
|
|
|
|
|
|
|
|
|
|
|
|
|
|
|
|
|
|
|
|
|
|
|
|
|
|
|
|
|
|
|
|
|
|
|
|
|
|
|
|
|
|
|
|
|
|
|
|
|
|
|
|
|
|
|
|
|
|
|
|
|
|
|
|
|
|
|
|
|
|
|
|
|
|
|
|
|
|
|
|
|
|
|
|
|
|
|
|
|
|
|
|
|
|
|
|
|
|
|
|
|
|
|
|
|
|
|
|
|
|
|
|
|
|
|
|
|
|
|
|
|
|
|
|
|
|
|
|
|
|
|
|
|
|
|
|
|
|
|
|
|
|
|
|
|
|
|
|
|
|
|
|
|
|
|
|
|
|
|
|
|
|
|
|
|
|
|
|
|
|
|
|
|
|
|
|
|
|
|
|
|
|
|
|
|
|
|
|
|
|
|
|
|
|
|
|
|
|
|
|
|
|
|
|
|
|
|
|
|
|
|
|
|
|
|
|
|
|
|
|
|
|
|
|
|
|
|
|
|
|
|
|
|
|
|
|
|
|
|
|
|
|
|
|
|
|
|
|
|
|
|
|
|
|
|
|
|
|
|
|
|
|
|
|
|
|
|
|
|
|
|
|
|
|
|
|
|
|
|
|
|
|
|
|
|
|
|
|
|
|
|
|
|
|
|
|
|
|
|
|
|
|
|
|
|
|
|
|
|
|
|
|
|
|
|
|
|
|
|
|
|
|
|
|
|
|
|
|
|
|
|
|
|
|
|
|
|
|
|
|
|
|
|
|
|
|
|
|
|
|
|
|
|
|
|
|
|
|
|
|
|
|
|
|
|
|
|
|
|
|
|
|
|
|
|
|
|
|
|
|
|
|
|
|
|
|
|
|
|
|
|
|
|
|
|
|
|
|
|
|
|
|
|
|
|
|
|
|
|
|
|
|
|
|
|
|
|
|
|
|
|
|
|
|
|
|
|
|
|
|
|
|
|
|
|
|
|
|
|
|
|
|
|
|
|
|
|
|
|
|
|
|
|
|
|
|
|
|
|
|
|
|
|
|
|
|
|
|
|
|
|
|
|
|
|
|
|
|
|
|
|
|
|
|
|
|
|
|
|
|
|
|
|
|
|
|
|
|
|
|
|
|
|
|
|
|
|
|
|
|
|
|
|
|
|
|
|
|
|
|
|
|
|
|
|
|
|
|
|
|
|
|
|
|
|
|
|
|
|
|
|
|
|
|
|
|
|
|
|
|
|
|
|
|
|
|
|
|
|
|
|
|
|
|
|
|
|
|
|
|
|
|
|
|
|
|
|
|
|
|
|
|
|
|
|
|
|
|
|
|
|
|
|
|
|
|
|
|
|
|
|
|
|
|
|
|
|
|
|
|
|
|
|
|
|
|
|
|
|
|
|
|
|
|
|
|
|
|
|
|
|
|
|
|
|
|
|
|
|
|
|
|
|
|
|
|
|
|
|
|
|
|
|
|
|
|
|
|
|
|
|
|
|
|
|
|
|
|
|
|
|
|
|
|
|
|
|
|
|
|
|
|
|
|
|
|
|
|
|
|
|
|
|
|
|
|
|
|
|
|
|
|
|
|
|
|
|
|
|
|
|
|
|
|
|
|
|
|
|
|
|
|
|
|
|
|
|
|
|
|
|
|
|
|
|
|
|
|
|
|
|
|
|
|
|
|
|
|
|
|
|
|
|
|
|
|
|
|
|
|
|
|
|
|
|
|
|
|
|
|
|
|
|
|
|
|
|
|
|
|
|
|
|
|
|
|
|
|
|
|
|
|
|
|
|
|
|
|
|
|
|
|
|
|
|
|
|
|
|
|
|
|
|
|
|
|
|
|
|
|
|
|
|
|
|
|
|
|
|
|
|
|
|
|
|
|
|
|
|
|
|
|
|
|
|
|
|
|
|
|
|
|
|
|
|
|
|
|
|
|
|
|
|
|
|
|
|
|
|
|
|
|
|
|
|
|
|
|
|
|
|
|
|
|
|
|
|
|
|
|
|
|
|
|
|
|
|
|
|
|
|
|
|
|
|
|
|
|
|
|
|
|
|
|
|
|
|
|
|
|
|
|
|
|
|
|
|
|
|
|
|
|
|
|
|
|
|
|
|
|
|
|
|
|
|
|
|
|
|
|
|
|
|
|
|
|
|
|
|
|
|
|
|
|
|
|
|
|
|
|
|
|
|
|
|
|
|
|
|
|
|
|
|
|
|
|
|
|
|
|
|
|
|
|
|
|
|
|
|
|
|
|
|
|
|
|
|
|
|
|
|
|
|
|
|
|
|
|
|
|
|
|
|
|
|
|
|
|
|
|
|
|
|
|
|
|
|
|
|
|
|
|
|
|
|
|
|
|
|
|
|
|
|
|
|
|
|
|
|
|
|
|
|
|
|
|
|
|
|
|
|
|
|
|
|
|
|
|
|
|
|
|
|
|
|
|
|
|
|
|
|
|
|
|
|
|
|
|
|
|
|
|
|
|
|
|
|
|
|
|
|
|
|
|
|
|
|
|
|
|
|
|
|
|
|
|
|
|
|
|
|
|
|
|
|
|
|
|
|
|
|
|
|
|
|
|
|
|
|
|
|
|
|
|
|
|
|
|
|
|
|
|
|
|
|
|
|
|
|
|
|
|
|
|
|
|
|
|
|
|
|
|
|
|
|
|
|
|
|
|
|
|
|
|
|
|
|
|
|
|
|
|
|
|
|
|
|
|
|
|
|
|
|
|
|
|
|
|
|
|
|
|
|
|
|
|
|
|
|
|
|
|
|
|
|
|
|
|
|
|
|
|
|
|
|
|
|
|
|
|
|
|
|
|
|
|
|
|
|
|
|
|
|
|
|
|
|
|
|
|
|
|
|
|
|
|
|
|
|
|
|
|
|
|
|
|
|
|
|
|
|
|
|
|
|
|
|
|
|
|
|
|
|
|
|
|
|
|
|
|
|
|
|
|
|
|
|
|
|
|
|
|
|
|
|
|
|
|
|
|
|
|
|
|
|
|
|
|
|
|
|
|
|
|
|
|
|
|
|
|
|
|
|
|
|
|
|
|
|
|
|
|
|
|
|
|
|
|
|
|
|
|
|
|
|
|
|
|
|
|
|
|
|
|
|
|
|
|
|
|
|
|
|
|
|
|
|
|
|
|
|
|
|
|
|
|
|
|
|
|
|
|
|
|
|
|
|
|
|
|
|
|
|
|
|
|
|
|
|
|
|
|
|
|
|
|
|
|
|
|
|
|
|
|
|
|
|
|
|
|
|
|
|
|
|
|
|
|
|
|
|
|
|
|
|
|
|
|
|
|
|
|
|
|
|
|
|
|
|
|
|
|
|
|
|
|
|
|
|
|
|
|
|
|
|
|
|
|
|
|
|
|
|
|
|
|
|
|
|
|
|
|
|
|
|
|
|
|
|
|
|
|
|
|
|
|
|
|
|
|
|
|
|
|
|
|
|
|
|
|
|
|
|
|
|
|
|
|
|
|
|
|
|
|
|
|
|
|
|
|
|
|
|
|
|
|
|
|
|
|
|
|
|
|
|
|
|
|
|
|
|
|
|
|
|
|
|
|
|
|
|
|
|
|
|
|
|
|
|
|
|
|
|
|
|
|
|
|
|
|
|
|
|
|
|
|
|
|
|
|
|
|
|
|
|
|
|
|
|
|
|
|
|
|
|
|
|
|
|
|
|
|
|
|
|
|
|
|
|
|
|
|
|
|
|
|
|
|
|
|
|
|
|
|
|
|
|
|
|
|
|
|
|
|
|
|
|
|
|
|
|
|
|
|
|
|
|
|
|
|
|
|
|
|
|
|
|
|
|
|
|
|
|
|
|
|
|
|
|
|
|
|
|
|
|
|
|
|
|
|
|
|
|
|
|
|
|
|
|
|
|
|
|
|
|
|
|
|
|
|
|
|
|
|
|
|
|
|
|
|
|
|
|
|
|
|
|
|
|
|
|
|
|
|
|
|
|
|
|
|
|
|
|
|
|
|
|
|
|
|
|
|
|
|
|
|
|
|
|
|
|
|
|
|
|
|
|
|
|
|
|
|
|
|
|
|
|
|
|
|
|
|
|
|
|
|
|
|
|
|
|
|
|
|
|
|
|
|
|
|
|
|
|
|
|
|
|
|
|
|
|
|
|
|
|
|
|
|
|
|
|
|
|
|
|
|
|
|
|
|
|
|
|
|
|
|
|
|
|
|
|
|
|
|
|
|
|
|
|
|
|
|
|
|
|
|
|
|
|
|
|
|
|
|
|
|
|
|
|
|
|
|
|
|
|
|
|
|
|
|
|
|
|
|
|
|
|
|
|
|
|
|
|
|
|
|
|
|
|
|
|
|
|
|
|
|
|
|
|
|
|
|
|
|
|
|
|
|
|
|
|
|
|
|
|
|
|
|
|
|
|
|
|
|
|
|
|
|
|
|
|
|
|
|
|
|
|
|
|
|
|
|
|
|
|
|
|
|
|
|
|
|
|
|
|
|
|
|
|
|
|
|
|
|
|
|
|
|
|
|
|
|
|
|
|
|
|
|
|
|
|
|
|
|
|
|
|
|
|
|
|
|
|
|
|
|
|
|
|
|
|
|
|
|
|
|
|
|
|
|
|
|
|
|
spaces/CVPR/Dual-Key_Backdoor_Attacks/datagen/detectron2/tests/test_roi_align_rotated.py
DELETED
@@ -1,176 +0,0 @@
|
|
1 |
-
# Copyright (c) Facebook, Inc. and its affiliates. All Rights Reserved
|
2 |
-
import logging
|
3 |
-
import unittest
|
4 |
-
import cv2
|
5 |
-
import torch
|
6 |
-
from torch.autograd import Variable, gradcheck
|
7 |
-
|
8 |
-
from detectron2.layers.roi_align import ROIAlign
|
9 |
-
from detectron2.layers.roi_align_rotated import ROIAlignRotated
|
10 |
-
|
11 |
-
logger = logging.getLogger(__name__)
|
12 |
-
|
13 |
-
|
14 |
-
class ROIAlignRotatedTest(unittest.TestCase):
|
15 |
-
def _box_to_rotated_box(self, box, angle):
|
16 |
-
return [
|
17 |
-
(box[0] + box[2]) / 2.0,
|
18 |
-
(box[1] + box[3]) / 2.0,
|
19 |
-
box[2] - box[0],
|
20 |
-
box[3] - box[1],
|
21 |
-
angle,
|
22 |
-
]
|
23 |
-
|
24 |
-
def _rot90(self, img, num):
|
25 |
-
num = num % 4 # note: -1 % 4 == 3
|
26 |
-
for _ in range(num):
|
27 |
-
img = img.transpose(0, 1).flip(0)
|
28 |
-
return img
|
29 |
-
|
30 |
-
def test_forward_output_0_90_180_270(self):
|
31 |
-
for i in range(4):
|
32 |
-
# i = 0, 1, 2, 3 corresponding to 0, 90, 180, 270 degrees
|
33 |
-
img = torch.arange(25, dtype=torch.float32).reshape(5, 5)
|
34 |
-
"""
|
35 |
-
0 1 2 3 4
|
36 |
-
5 6 7 8 9
|
37 |
-
10 11 12 13 14
|
38 |
-
15 16 17 18 19
|
39 |
-
20 21 22 23 24
|
40 |
-
"""
|
41 |
-
box = [1, 1, 3, 3]
|
42 |
-
rotated_box = self._box_to_rotated_box(box=box, angle=90 * i)
|
43 |
-
|
44 |
-
result = self._simple_roi_align_rotated(img=img, box=rotated_box, resolution=(4, 4))
|
45 |
-
|
46 |
-
# Here's an explanation for 0 degree case:
|
47 |
-
# point 0 in the original input lies at [0.5, 0.5]
|
48 |
-
# (the center of bin [0, 1] x [0, 1])
|
49 |
-
# point 1 in the original input lies at [1.5, 0.5], etc.
|
50 |
-
# since the resolution is (4, 4) that divides [1, 3] x [1, 3]
|
51 |
-
# into 4 x 4 equal bins,
|
52 |
-
# the top-left bin is [1, 1.5] x [1, 1.5], and its center
|
53 |
-
# (1.25, 1.25) lies at the 3/4 position
|
54 |
-
# between point 0 and point 1, point 5 and point 6,
|
55 |
-
# point 0 and point 5, point 1 and point 6, so it can be calculated as
|
56 |
-
# 0.25*(0*0.25+1*0.75)+(5*0.25+6*0.75)*0.75 = 4.5
|
57 |
-
result_expected = torch.tensor(
|
58 |
-
[
|
59 |
-
[4.5, 5.0, 5.5, 6.0],
|
60 |
-
[7.0, 7.5, 8.0, 8.5],
|
61 |
-
[9.5, 10.0, 10.5, 11.0],
|
62 |
-
[12.0, 12.5, 13.0, 13.5],
|
63 |
-
]
|
64 |
-
)
|
65 |
-
# This is also an upsampled version of [[6, 7], [11, 12]]
|
66 |
-
|
67 |
-
# When the box is rotated by 90 degrees CCW,
|
68 |
-
# the result would be rotated by 90 degrees CW, thus it's -i here
|
69 |
-
result_expected = self._rot90(result_expected, -i)
|
70 |
-
|
71 |
-
assert torch.allclose(result, result_expected)
|
72 |
-
|
73 |
-
def test_resize(self):
|
74 |
-
H, W = 30, 30
|
75 |
-
input = torch.rand(H, W) * 100
|
76 |
-
box = [10, 10, 20, 20]
|
77 |
-
rotated_box = self._box_to_rotated_box(box, angle=0)
|
78 |
-
output = self._simple_roi_align_rotated(img=input, box=rotated_box, resolution=(5, 5))
|
79 |
-
|
80 |
-
input2x = cv2.resize(input.numpy(), (W // 2, H // 2), interpolation=cv2.INTER_LINEAR)
|
81 |
-
input2x = torch.from_numpy(input2x)
|
82 |
-
box2x = [x / 2 for x in box]
|
83 |
-
rotated_box2x = self._box_to_rotated_box(box2x, angle=0)
|
84 |
-
output2x = self._simple_roi_align_rotated(img=input2x, box=rotated_box2x, resolution=(5, 5))
|
85 |
-
assert torch.allclose(output2x, output)
|
86 |
-
|
87 |
-
def _simple_roi_align_rotated(self, img, box, resolution):
|
88 |
-
"""
|
89 |
-
RoiAlignRotated with scale 1.0 and 0 sample ratio.
|
90 |
-
"""
|
91 |
-
op = ROIAlignRotated(output_size=resolution, spatial_scale=1.0, sampling_ratio=0)
|
92 |
-
input = img[None, None, :, :]
|
93 |
-
|
94 |
-
rois = [0] + list(box)
|
95 |
-
rois = torch.tensor(rois, dtype=torch.float32)[None, :]
|
96 |
-
result_cpu = op.forward(input, rois)
|
97 |
-
if torch.cuda.is_available():
|
98 |
-
result_cuda = op.forward(input.cuda(), rois.cuda())
|
99 |
-
assert torch.allclose(result_cpu, result_cuda.cpu())
|
100 |
-
return result_cpu[0, 0]
|
101 |
-
|
102 |
-
def test_empty_box(self):
|
103 |
-
img = torch.rand(5, 5)
|
104 |
-
out = self._simple_roi_align_rotated(img, [2, 3, 0, 0, 0], (7, 7))
|
105 |
-
self.assertTrue((out == 0).all())
|
106 |
-
|
107 |
-
def test_roi_align_rotated_gradcheck_cpu(self):
|
108 |
-
dtype = torch.float64
|
109 |
-
device = torch.device("cpu")
|
110 |
-
roi_align_rotated_op = ROIAlignRotated(
|
111 |
-
output_size=(5, 5), spatial_scale=0.5, sampling_ratio=1
|
112 |
-
).to(dtype=dtype, device=device)
|
113 |
-
x = torch.rand(1, 1, 10, 10, dtype=dtype, device=device, requires_grad=True)
|
114 |
-
# roi format is (batch index, x_center, y_center, width, height, angle)
|
115 |
-
rois = torch.tensor(
|
116 |
-
[[0, 4.5, 4.5, 9, 9, 0], [0, 2, 7, 4, 4, 0], [0, 7, 7, 4, 4, 0]],
|
117 |
-
dtype=dtype,
|
118 |
-
device=device,
|
119 |
-
)
|
120 |
-
|
121 |
-
def func(input):
|
122 |
-
return roi_align_rotated_op(input, rois)
|
123 |
-
|
124 |
-
assert gradcheck(func, (x,)), "gradcheck failed for RoIAlignRotated CPU"
|
125 |
-
assert gradcheck(func, (x.transpose(2, 3),)), "gradcheck failed for RoIAlignRotated CPU"
|
126 |
-
|
127 |
-
@unittest.skipIf(not torch.cuda.is_available(), "CUDA not available")
|
128 |
-
def test_roi_align_rotated_gradient_cuda(self):
|
129 |
-
"""
|
130 |
-
Compute gradients for ROIAlignRotated with multiple bounding boxes on the GPU,
|
131 |
-
and compare the result with ROIAlign
|
132 |
-
"""
|
133 |
-
# torch.manual_seed(123)
|
134 |
-
dtype = torch.float64
|
135 |
-
device = torch.device("cuda")
|
136 |
-
pool_h, pool_w = (5, 5)
|
137 |
-
|
138 |
-
roi_align = ROIAlign(output_size=(pool_h, pool_w), spatial_scale=1, sampling_ratio=2).to(
|
139 |
-
device=device
|
140 |
-
)
|
141 |
-
|
142 |
-
roi_align_rotated = ROIAlignRotated(
|
143 |
-
output_size=(pool_h, pool_w), spatial_scale=1, sampling_ratio=2
|
144 |
-
).to(device=device)
|
145 |
-
|
146 |
-
x = torch.rand(1, 1, 10, 10, dtype=dtype, device=device, requires_grad=True)
|
147 |
-
# x_rotated = x.clone() won't work (will lead to grad_fun=CloneBackward)!
|
148 |
-
x_rotated = Variable(x.data.clone(), requires_grad=True)
|
149 |
-
|
150 |
-
# roi_rotated format is (batch index, x_center, y_center, width, height, angle)
|
151 |
-
rois_rotated = torch.tensor(
|
152 |
-
[[0, 4.5, 4.5, 9, 9, 0], [0, 2, 7, 4, 4, 0], [0, 7, 7, 4, 4, 0]],
|
153 |
-
dtype=dtype,
|
154 |
-
device=device,
|
155 |
-
)
|
156 |
-
|
157 |
-
y_rotated = roi_align_rotated(x_rotated, rois_rotated)
|
158 |
-
s_rotated = y_rotated.sum()
|
159 |
-
s_rotated.backward()
|
160 |
-
|
161 |
-
# roi format is (batch index, x1, y1, x2, y2)
|
162 |
-
rois = torch.tensor(
|
163 |
-
[[0, 0, 0, 9, 9], [0, 0, 5, 4, 9], [0, 5, 5, 9, 9]], dtype=dtype, device=device
|
164 |
-
)
|
165 |
-
|
166 |
-
y = roi_align(x, rois)
|
167 |
-
s = y.sum()
|
168 |
-
s.backward()
|
169 |
-
|
170 |
-
assert torch.allclose(
|
171 |
-
x.grad, x_rotated.grad
|
172 |
-
), "gradients for ROIAlign and ROIAlignRotated mismatch on CUDA"
|
173 |
-
|
174 |
-
|
175 |
-
if __name__ == "__main__":
|
176 |
-
unittest.main()
|
|
|
|
|
|
|
|
|
|
|
|
|
|
|
|
|
|
|
|
|
|
|
|
|
|
|
|
|
|
|
|
|
|
|
|
|
|
|
|
|
|
|
|
|
|
|
|
|
|
|
|
|
|
|
|
|
|
|
|
|
|
|
|
|
|
|
|
|
|
|
|
|
|
|
|
|
|
|
|
|
|
|
|
|
|
|
|
|
|
|
|
|
|
|
|
|
|
|
|
|
|
|
|
|
|
|
|
|
|
|
|
|
|
|
|
|
|
|
|
|
|
|
|
|
|
|
|
|
|
|
|
|
|
|
|
|
|
|
|
|
|
|
|
|
|
|
|
|
|
|
|
|
|
|
|
|
|
|
|
|
|
|
|
|
|
|
|
|
|
|
|
|
|
|
|
|
|
|
|
|
|
|
|
|
|
|
|
|
|
|
|
|
|
|
|
|
|
|
|
|
|
|
|
|
|
|
|
|
|
|
|
|
|
|
|
|
|
|
|
|
|
|
|
|
|
|
|
|
|
|
|
|
|
|
|
|
|
|
|
|
|
|
|
|
|
|
|
|
|
|
|
|
|
|
|
|
|
|
|
|
|
|
|
|
|
|
|
|
|
|
|
|
|
|
|
|
|
|
|
|
|
|
|
|
|
|
|
|
|
|
|
|
|
|
|
|
|
|
|
|
|
|
|
|
|
|
|
|
|
|
|
|
|
|
|
|
|
|
|
|
|
|
|
|
|
|
|
|
|
|
|
|
|
|
|
|
|
|
|
|
|
|
|
|
|
|
|
|
|
|
|
|
|
spaces/CVPR/LIVE/pybind11/tests/test_chrono.py
DELETED
@@ -1,202 +0,0 @@
|
|
1 |
-
# -*- coding: utf-8 -*-
|
2 |
-
from pybind11_tests import chrono as m
|
3 |
-
import datetime
|
4 |
-
import pytest
|
5 |
-
|
6 |
-
import env # noqa: F401
|
7 |
-
|
8 |
-
|
9 |
-
def test_chrono_system_clock():
|
10 |
-
|
11 |
-
# Get the time from both c++ and datetime
|
12 |
-
date0 = datetime.datetime.today()
|
13 |
-
date1 = m.test_chrono1()
|
14 |
-
date2 = datetime.datetime.today()
|
15 |
-
|
16 |
-
# The returned value should be a datetime
|
17 |
-
assert isinstance(date1, datetime.datetime)
|
18 |
-
|
19 |
-
# The numbers should vary by a very small amount (time it took to execute)
|
20 |
-
diff_python = abs(date2 - date0)
|
21 |
-
diff = abs(date1 - date2)
|
22 |
-
|
23 |
-
# There should never be a days difference
|
24 |
-
assert diff.days == 0
|
25 |
-
|
26 |
-
# Since datetime.datetime.today() calls time.time(), and on some platforms
|
27 |
-
# that has 1 second accuracy, we compare this way
|
28 |
-
assert diff.seconds <= diff_python.seconds
|
29 |
-
|
30 |
-
|
31 |
-
def test_chrono_system_clock_roundtrip():
|
32 |
-
date1 = datetime.datetime.today()
|
33 |
-
|
34 |
-
# Roundtrip the time
|
35 |
-
date2 = m.test_chrono2(date1)
|
36 |
-
|
37 |
-
# The returned value should be a datetime
|
38 |
-
assert isinstance(date2, datetime.datetime)
|
39 |
-
|
40 |
-
# They should be identical (no information lost on roundtrip)
|
41 |
-
diff = abs(date1 - date2)
|
42 |
-
assert diff.days == 0
|
43 |
-
assert diff.seconds == 0
|
44 |
-
assert diff.microseconds == 0
|
45 |
-
|
46 |
-
|
47 |
-
def test_chrono_system_clock_roundtrip_date():
|
48 |
-
date1 = datetime.date.today()
|
49 |
-
|
50 |
-
# Roundtrip the time
|
51 |
-
datetime2 = m.test_chrono2(date1)
|
52 |
-
date2 = datetime2.date()
|
53 |
-
time2 = datetime2.time()
|
54 |
-
|
55 |
-
# The returned value should be a datetime
|
56 |
-
assert isinstance(datetime2, datetime.datetime)
|
57 |
-
assert isinstance(date2, datetime.date)
|
58 |
-
assert isinstance(time2, datetime.time)
|
59 |
-
|
60 |
-
# They should be identical (no information lost on roundtrip)
|
61 |
-
diff = abs(date1 - date2)
|
62 |
-
assert diff.days == 0
|
63 |
-
assert diff.seconds == 0
|
64 |
-
assert diff.microseconds == 0
|
65 |
-
|
66 |
-
# Year, Month & Day should be the same after the round trip
|
67 |
-
assert date1.year == date2.year
|
68 |
-
assert date1.month == date2.month
|
69 |
-
assert date1.day == date2.day
|
70 |
-
|
71 |
-
# There should be no time information
|
72 |
-
assert time2.hour == 0
|
73 |
-
assert time2.minute == 0
|
74 |
-
assert time2.second == 0
|
75 |
-
assert time2.microsecond == 0
|
76 |
-
|
77 |
-
|
78 |
-
SKIP_TZ_ENV_ON_WIN = pytest.mark.skipif(
|
79 |
-
"env.WIN", reason="TZ environment variable only supported on POSIX"
|
80 |
-
)
|
81 |
-
|
82 |
-
|
83 |
-
@pytest.mark.parametrize("time1", [
|
84 |
-
datetime.datetime.today().time(),
|
85 |
-
datetime.time(0, 0, 0),
|
86 |
-
datetime.time(0, 0, 0, 1),
|
87 |
-
datetime.time(0, 28, 45, 109827),
|
88 |
-
datetime.time(0, 59, 59, 999999),
|
89 |
-
datetime.time(1, 0, 0),
|
90 |
-
datetime.time(5, 59, 59, 0),
|
91 |
-
datetime.time(5, 59, 59, 1),
|
92 |
-
])
|
93 |
-
@pytest.mark.parametrize("tz", [
|
94 |
-
None,
|
95 |
-
pytest.param("Europe/Brussels", marks=SKIP_TZ_ENV_ON_WIN),
|
96 |
-
pytest.param("Asia/Pyongyang", marks=SKIP_TZ_ENV_ON_WIN),
|
97 |
-
pytest.param("America/New_York", marks=SKIP_TZ_ENV_ON_WIN),
|
98 |
-
])
|
99 |
-
def test_chrono_system_clock_roundtrip_time(time1, tz, monkeypatch):
|
100 |
-
if tz is not None:
|
101 |
-
monkeypatch.setenv("TZ", "/usr/share/zoneinfo/{}".format(tz))
|
102 |
-
|
103 |
-
# Roundtrip the time
|
104 |
-
datetime2 = m.test_chrono2(time1)
|
105 |
-
date2 = datetime2.date()
|
106 |
-
time2 = datetime2.time()
|
107 |
-
|
108 |
-
# The returned value should be a datetime
|
109 |
-
assert isinstance(datetime2, datetime.datetime)
|
110 |
-
assert isinstance(date2, datetime.date)
|
111 |
-
assert isinstance(time2, datetime.time)
|
112 |
-
|
113 |
-
# Hour, Minute, Second & Microsecond should be the same after the round trip
|
114 |
-
assert time1.hour == time2.hour
|
115 |
-
assert time1.minute == time2.minute
|
116 |
-
assert time1.second == time2.second
|
117 |
-
assert time1.microsecond == time2.microsecond
|
118 |
-
|
119 |
-
# There should be no date information (i.e. date = python base date)
|
120 |
-
assert date2.year == 1970
|
121 |
-
assert date2.month == 1
|
122 |
-
assert date2.day == 1
|
123 |
-
|
124 |
-
|
125 |
-
def test_chrono_duration_roundtrip():
|
126 |
-
|
127 |
-
# Get the difference between two times (a timedelta)
|
128 |
-
date1 = datetime.datetime.today()
|
129 |
-
date2 = datetime.datetime.today()
|
130 |
-
diff = date2 - date1
|
131 |
-
|
132 |
-
# Make sure this is a timedelta
|
133 |
-
assert isinstance(diff, datetime.timedelta)
|
134 |
-
|
135 |
-
cpp_diff = m.test_chrono3(diff)
|
136 |
-
|
137 |
-
assert cpp_diff.days == diff.days
|
138 |
-
assert cpp_diff.seconds == diff.seconds
|
139 |
-
assert cpp_diff.microseconds == diff.microseconds
|
140 |
-
|
141 |
-
|
142 |
-
def test_chrono_duration_subtraction_equivalence():
|
143 |
-
|
144 |
-
date1 = datetime.datetime.today()
|
145 |
-
date2 = datetime.datetime.today()
|
146 |
-
|
147 |
-
diff = date2 - date1
|
148 |
-
cpp_diff = m.test_chrono4(date2, date1)
|
149 |
-
|
150 |
-
assert cpp_diff.days == diff.days
|
151 |
-
assert cpp_diff.seconds == diff.seconds
|
152 |
-
assert cpp_diff.microseconds == diff.microseconds
|
153 |
-
|
154 |
-
|
155 |
-
def test_chrono_duration_subtraction_equivalence_date():
|
156 |
-
|
157 |
-
date1 = datetime.date.today()
|
158 |
-
date2 = datetime.date.today()
|
159 |
-
|
160 |
-
diff = date2 - date1
|
161 |
-
cpp_diff = m.test_chrono4(date2, date1)
|
162 |
-
|
163 |
-
assert cpp_diff.days == diff.days
|
164 |
-
assert cpp_diff.seconds == diff.seconds
|
165 |
-
assert cpp_diff.microseconds == diff.microseconds
|
166 |
-
|
167 |
-
|
168 |
-
def test_chrono_steady_clock():
|
169 |
-
time1 = m.test_chrono5()
|
170 |
-
assert isinstance(time1, datetime.timedelta)
|
171 |
-
|
172 |
-
|
173 |
-
def test_chrono_steady_clock_roundtrip():
|
174 |
-
time1 = datetime.timedelta(days=10, seconds=10, microseconds=100)
|
175 |
-
time2 = m.test_chrono6(time1)
|
176 |
-
|
177 |
-
assert isinstance(time2, datetime.timedelta)
|
178 |
-
|
179 |
-
# They should be identical (no information lost on roundtrip)
|
180 |
-
assert time1.days == time2.days
|
181 |
-
assert time1.seconds == time2.seconds
|
182 |
-
assert time1.microseconds == time2.microseconds
|
183 |
-
|
184 |
-
|
185 |
-
def test_floating_point_duration():
|
186 |
-
# Test using a floating point number in seconds
|
187 |
-
time = m.test_chrono7(35.525123)
|
188 |
-
|
189 |
-
assert isinstance(time, datetime.timedelta)
|
190 |
-
|
191 |
-
assert time.seconds == 35
|
192 |
-
assert 525122 <= time.microseconds <= 525123
|
193 |
-
|
194 |
-
diff = m.test_chrono_float_diff(43.789012, 1.123456)
|
195 |
-
assert diff.seconds == 42
|
196 |
-
assert 665556 <= diff.microseconds <= 665557
|
197 |
-
|
198 |
-
|
199 |
-
def test_nano_timepoint():
|
200 |
-
time = datetime.datetime.now()
|
201 |
-
time1 = m.test_nano_timepoint(time, datetime.timedelta(seconds=60))
|
202 |
-
assert(time1 == time + datetime.timedelta(seconds=60))
|
|
|
|
|
|
|
|
|
|
|
|
|
|
|
|
|
|
|
|
|
|
|
|
|
|
|
|
|
|
|
|
|
|
|
|
|
|
|
|
|
|
|
|
|
|
|
|
|
|
|
|
|
|
|
|
|
|
|
|
|
|
|
|
|
|
|
|
|
|
|
|
|
|
|
|
|
|
|
|
|
|
|
|
|
|
|
|
|
|
|
|
|
|
|
|
|
|
|
|
|
|
|
|
|
|
|
|
|
|
|
|
|
|
|
|
|
|
|
|
|
|
|
|
|
|
|
|
|
|
|
|
|
|
|
|
|
|
|
|
|
|
|
|
|
|
|
|
|
|
|
|
|
|
|
|
|
|
|
|
|
|
|
|
|
|
|
|
|
|
|
|
|
|
|
|
|
|
|
|
|
|
|
|
|
|
|
|
|
|
|
|
|
|
|
|
|
|
|
|
|
|
|
|
|
|
|
|
|
|
|
|
|
|
|
|
|
|
|
|
|
|
|
|
|
|
|
|
|
|
|
|
|
|
|
|
|
|
|
|
|
|
|
|
|
|
|
|
|
|
|
|
|
|
|
|
|
|
|
|
|
|
|
|
|
|
|
|
|
|
|
|
|
|
|
|
|
|
|
|
|
|
|
|
|
|
|
|
|
|
|
|
|
|
|
|
|
|
|
|
|
|
|
|
|
|
|
|
|
|
|
|
|
|
|
|
|
|
|
|
|
|
|
|
|
|
|
|
|
|
|
|
|
|
|
|
|
|
|
|
|
|
|
|
|
|
|
|
|
|
|
|
|
|
|
|
|
|
|
|
|
|
|
|
|
|
|
|
|
|
|
|
|
|
|
|
|
|
|
|
|
|
|
|
|
|
|
|
|
|
|
|
|
|
|
|
|
|
|
|
|
|
|
|
|
|
spaces/CVPR/LIVE/thrust/internal/scripts/wiki2tex.py
DELETED
@@ -1,194 +0,0 @@
|
|
1 |
-
'''
|
2 |
-
Convert Google Code .wiki files into .tex formatted files.
|
3 |
-
|
4 |
-
Output is designed to be included within a larger TeX project, it is
|
5 |
-
not standalone.
|
6 |
-
|
7 |
-
'''
|
8 |
-
|
9 |
-
import sys
|
10 |
-
import re
|
11 |
-
import codecs
|
12 |
-
|
13 |
-
print(sys.argv)
|
14 |
-
|
15 |
-
'''
|
16 |
-
A "rule" is a begin tag, an end tag, and how to reformat the inner text
|
17 |
-
(function)
|
18 |
-
'''
|
19 |
-
|
20 |
-
def encase(pre, post, strip=False):
|
21 |
-
"""Return a function that prepends pre and postpends post"""
|
22 |
-
def f(txt):
|
23 |
-
if strip:
|
24 |
-
return pre + txt.strip() + post
|
25 |
-
else:
|
26 |
-
return pre + txt + post
|
27 |
-
return f
|
28 |
-
|
29 |
-
def constant(text):
|
30 |
-
def f(txt):
|
31 |
-
return text
|
32 |
-
return f
|
33 |
-
|
34 |
-
def encase_with_rules(pre, post, rules, strip=False):
|
35 |
-
def f(txt):
|
36 |
-
if strip:
|
37 |
-
return pre + apply_rules(txt, rules).strip() + post
|
38 |
-
else:
|
39 |
-
return pre + apply_rules(txt, rules) + post
|
40 |
-
return f
|
41 |
-
|
42 |
-
def encase_escape_underscore(pre, post):
|
43 |
-
def f(txt):
|
44 |
-
txt = sub(r'_', r'\_', txt)
|
45 |
-
return pre + txt + post
|
46 |
-
return f
|
47 |
-
|
48 |
-
def sub(pat, repl, txt):
|
49 |
-
"""Substitute in repl for pat in txt, txt can be multiple lines"""
|
50 |
-
return re.compile(pat, re.MULTILINE).sub(repl, txt)
|
51 |
-
|
52 |
-
def process_list(rules):
|
53 |
-
def f(txt):
|
54 |
-
txt = ' *' + txt # was removed to match begin tag of list
|
55 |
-
res = '\\begin{itemize}\n'
|
56 |
-
for ln in txt.split('\n'):
|
57 |
-
# Convert " *" to "\item "
|
58 |
-
ln = sub(r'^ \*', r'\\item ', ln)
|
59 |
-
res += apply_rules(ln, rules) + '\n'
|
60 |
-
res += '\\end{itemize}\n'
|
61 |
-
return res
|
62 |
-
return f
|
63 |
-
|
64 |
-
def process_link(rules):
|
65 |
-
def f(txt):
|
66 |
-
lst = txt.split(' ')
|
67 |
-
lnk = lst[0]
|
68 |
-
desc = apply_rules(' '.join(lst[1:]), rules)
|
69 |
-
if lnk[:7] == 'http://':
|
70 |
-
desc = apply_rules(' '.join(lst[1:]), rules)
|
71 |
-
return r'\href{' + lnk + r'}{' + desc + r'}'
|
72 |
-
if len(lst) > 1:
|
73 |
-
return r'\href{}{' + desc + r'}'
|
74 |
-
return r'\href{}{' + lnk + r'}'
|
75 |
-
return f
|
76 |
-
|
77 |
-
# Some rules can be used inside some other rules (backticks in section names)
|
78 |
-
|
79 |
-
link_rules = [
|
80 |
-
['_', '', constant(r'\_')],
|
81 |
-
]
|
82 |
-
|
83 |
-
section_rules = [
|
84 |
-
['`', '`', encase_escape_underscore(r'\texttt{', r'}')],
|
85 |
-
]
|
86 |
-
|
87 |
-
item_rules = [
|
88 |
-
['`', '`', encase(r'\verb|', r'|')],
|
89 |
-
['[', ']', process_link(link_rules)],
|
90 |
-
]
|
91 |
-
|
92 |
-
# Main rules for Latex formatting
|
93 |
-
|
94 |
-
rules = [
|
95 |
-
['{{{', '}}}', encase(r'\begin{lstlisting}[language=c++]', r'\end{lstlisting}')],
|
96 |
-
['[', ']', process_link(link_rules)],
|
97 |
-
[' *', '\n\n', process_list(item_rules)],
|
98 |
-
['"', '"', encase("``", "''")],
|
99 |
-
['`', '`', encase(r'\verb|', r'|')],
|
100 |
-
['*', '*', encase(r'\emph{', r'}')],
|
101 |
-
['_', '_', encase(r'\emph{', r'}')],
|
102 |
-
['==', '==', encase_with_rules(r'\section{', r'}', section_rules, True)],
|
103 |
-
['=', '=', encase_with_rules(r'\chapter{', r'}', section_rules, True)],
|
104 |
-
['(e.g. f(x) -> y and f(x,y) -> ', 'z)', constant(r'(e.g. $f(x)\to y$ and $f(x,y)\to z$)')],
|
105 |
-
]
|
106 |
-
|
107 |
-
def match_rules(txt, rules):
|
108 |
-
"""Find rule that first matches in txt"""
|
109 |
-
# Find first begin tag
|
110 |
-
first_begin_loc = 10e100
|
111 |
-
matching_rule = None
|
112 |
-
for rule in rules:
|
113 |
-
begin_tag, end_tag, func = rule
|
114 |
-
loc = txt.find(begin_tag)
|
115 |
-
if loc > -1 and loc < first_begin_loc:
|
116 |
-
first_begin_loc = loc
|
117 |
-
matching_rule = rule
|
118 |
-
return (matching_rule, first_begin_loc)
|
119 |
-
|
120 |
-
def apply_rules(txt, rules):
|
121 |
-
"""Apply set of rules to give txt, return transformed version of txt"""
|
122 |
-
matching_rule, first_begin_loc = match_rules(txt, rules)
|
123 |
-
if matching_rule is None:
|
124 |
-
return txt
|
125 |
-
begin_tag, end_tag, func = matching_rule
|
126 |
-
end_loc = txt.find(end_tag, first_begin_loc + 1)
|
127 |
-
if end_loc == -1:
|
128 |
-
sys.exit('Could not find end tag {0} after position {1}'.format(end_tag, first_begin_loc + 1))
|
129 |
-
inner_txt = txt[first_begin_loc + len(begin_tag) : end_loc]
|
130 |
-
# Copy characters up until begin tag
|
131 |
-
# Then have output of rule function on inner text
|
132 |
-
new_txt_start = txt[:first_begin_loc] + func(inner_txt)
|
133 |
-
# Follow with the remaining processed text
|
134 |
-
remaining_txt = txt[end_loc + len(end_tag):]
|
135 |
-
return new_txt_start + apply_rules(remaining_txt, rules)
|
136 |
-
|
137 |
-
def split_sections(contents):
|
138 |
-
"""Given one string of all file contents, return list of sections
|
139 |
-
|
140 |
-
Return format is list of pairs, each pair has section title
|
141 |
-
and list of lines. Result is ordered as the original input.
|
142 |
-
|
143 |
-
"""
|
144 |
-
res = []
|
145 |
-
cur_section = ''
|
146 |
-
section = []
|
147 |
-
for ln in contents.split('\n'):
|
148 |
-
if len(ln) > 0 and ln[0] == '=':
|
149 |
-
# remove = formatting from line
|
150 |
-
section_title = sub(r'^\=+ (.*) \=+', r'\1', ln)
|
151 |
-
res.append((cur_section, section))
|
152 |
-
cur_section = section_title
|
153 |
-
section = [ln]
|
154 |
-
else:
|
155 |
-
section.append(ln)
|
156 |
-
res.append((cur_section, section))
|
157 |
-
return res
|
158 |
-
|
159 |
-
def filter_sections(splitinput, removelst):
|
160 |
-
"""Take split input and remove sections in removelst"""
|
161 |
-
res = []
|
162 |
-
for sectname, sectcontents in splitinput:
|
163 |
-
if sectname in removelst:
|
164 |
-
pass
|
165 |
-
else:
|
166 |
-
res.extend(sectcontents)
|
167 |
-
# convert to single string for output
|
168 |
-
return '\n'.join(res)
|
169 |
-
|
170 |
-
|
171 |
-
def main():
|
172 |
-
infile = codecs.open(sys.argv[1], encoding='utf-8')
|
173 |
-
outfile = codecs.open(sys.argv[2], mode='w', encoding='utf-8')
|
174 |
-
|
175 |
-
contents = infile.read()
|
176 |
-
|
177 |
-
# Remove first three lines
|
178 |
-
contents = '\n'.join(contents.split('\n')[3:])
|
179 |
-
|
180 |
-
# Split sections and filter out some of them
|
181 |
-
sections = split_sections(contents)
|
182 |
-
contents = filter_sections(sections, ['Introduction', 'Prerequisites', 'Simple Example'])
|
183 |
-
|
184 |
-
# Convert to latex format
|
185 |
-
contents = apply_rules(contents, rules)
|
186 |
-
|
187 |
-
infile.close()
|
188 |
-
outfile.write(contents)
|
189 |
-
outfile.close()
|
190 |
-
return 0
|
191 |
-
|
192 |
-
|
193 |
-
if __name__ == '__main__':
|
194 |
-
sys.exit(main())
|
|
|
|
|
|
|
|
|
|
|
|
|
|
|
|
|
|
|
|
|
|
|
|
|
|
|
|
|
|
|
|
|
|
|
|
|
|
|
|
|
|
|
|
|
|
|
|
|
|
|
|
|
|
|
|
|
|
|
|
|
|
|
|
|
|
|
|
|
|
|
|
|
|
|
|
|
|
|
|
|
|
|
|
|
|
|
|
|
|
|
|
|
|
|
|
|
|
|
|
|
|
|
|
|
|
|
|
|
|
|
|
|
|
|
|
|
|
|
|
|
|
|
|
|
|
|
|
|
|
|
|
|
|
|
|
|
|
|
|
|
|
|
|
|
|
|
|
|
|
|
|
|
|
|
|
|
|
|
|
|
|
|
|
|
|
|
|
|
|
|
|
|
|
|
|
|
|
|
|
|
|
|
|
|
|
|
|
|
|
|
|
|
|
|
|
|
|
|
|
|
|
|
|
|
|
|
|
|
|
|
|
|
|
|
|
|
|
|
|
|
|
|
|
|
|
|
|
|
|
|
|
|
|
|
|
|
|
|
|
|
|
|
|
|
|
|
|
|
|
|
|
|
|
|
|
|
|
|
|
|
|
|
|
|
|
|
|
|
|
|
|
|
|
|
|
|
|
|
|
|
|
|
|
|
|
|
|
|
|
|
|
|
|
|
|
|
|
|
|
|
|
|
|
|
|
|
|
|
|
|
|
|
|
|
|
|
|
|
|
|
|
|
|
|
|
|
|
|
|
|
|
|
|
|
|
|
|
|
|
|
|
|
|
|
|
|
|
|
|
|
|
|
|
|
|
|
|
|
|
|
|
|
|
|
|
|
|
|
|
|
|
|
|
|
|
|
|
|
|
|
|
|
|
|
|
|
|
|
|
spaces/CVPR/LIVE/thrust/thrust/system/cpp/detail/find.h
DELETED
@@ -1,23 +0,0 @@
|
|
1 |
-
/*
|
2 |
-
* Copyright 2008-2013 NVIDIA Corporation
|
3 |
-
*
|
4 |
-
* Licensed under the Apache License, Version 2.0 (the "License");
|
5 |
-
* you may not use this file except in compliance with the License.
|
6 |
-
* You may obtain a copy of the License at
|
7 |
-
*
|
8 |
-
* http://www.apache.org/licenses/LICENSE-2.0
|
9 |
-
*
|
10 |
-
* Unless required by applicable law or agreed to in writing, software
|
11 |
-
* distributed under the License is distributed on an "AS IS" BASIS,
|
12 |
-
* WITHOUT WARRANTIES OR CONDITIONS OF ANY KIND, either express or implied.
|
13 |
-
* See the License for the specific language governing permissions and
|
14 |
-
* limitations under the License.
|
15 |
-
*/
|
16 |
-
|
17 |
-
#pragma once
|
18 |
-
|
19 |
-
#include <thrust/detail/config.h>
|
20 |
-
|
21 |
-
// this system inherits find
|
22 |
-
#include <thrust/system/detail/sequential/find.h>
|
23 |
-
|
|
|
|
|
|
|
|
|
|
|
|
|
|
|
|
|
|
|
|
|
|
|
|
|
|
|
|
|
|
|
|
|
|
|
|
|
|
|
|
|
|
|
|
|
|
|
|
spaces/CVPR/LIVE/thrust/thrust/system/cuda/detail/sequence.h
DELETED
@@ -1,22 +0,0 @@
|
|
1 |
-
/*
|
2 |
-
* Copyright 2008-2013 NVIDIA Corporation
|
3 |
-
*
|
4 |
-
* Licensed under the Apache License, Version 2.0 (the "License");
|
5 |
-
* you may not use this file except in compliance with the License.
|
6 |
-
* You may obtain a copy of the License at
|
7 |
-
*
|
8 |
-
* http://www.apache.org/licenses/LICENSE-2.0
|
9 |
-
*
|
10 |
-
* Unless required by applicable law or agreed to in writing, software
|
11 |
-
* distributed under the License is distributed on an "AS IS" BASIS,
|
12 |
-
* WITHOUT WARRANTIES OR CONDITIONS OF ANY KIND, either express or implied.
|
13 |
-
* See the License for the specific language governing permissions and
|
14 |
-
* limitations under the License.
|
15 |
-
*/
|
16 |
-
|
17 |
-
#pragma once
|
18 |
-
|
19 |
-
#include <thrust/detail/config.h>
|
20 |
-
|
21 |
-
// this system has no special version of this algorithm
|
22 |
-
|
|
|
|
|
|
|
|
|
|
|
|
|
|
|
|
|
|
|
|
|
|
|
|
|
|
|
|
|
|
|
|
|
|
|
|
|
|
|
|
|
|
|
|
|
|
spaces/CVPR/WALT/mmdet/core/visualization/image.py
DELETED
@@ -1,322 +0,0 @@
|
|
1 |
-
import matplotlib.pyplot as plt
|
2 |
-
import mmcv
|
3 |
-
import numpy as np
|
4 |
-
import pycocotools.mask as mask_util
|
5 |
-
from matplotlib.collections import PatchCollection
|
6 |
-
from matplotlib.patches import Polygon
|
7 |
-
import cv2
|
8 |
-
|
9 |
-
from ..utils import mask2ndarray
|
10 |
-
|
11 |
-
EPS = 1e-2
|
12 |
-
|
13 |
-
|
14 |
-
def color_val_matplotlib(color):
|
15 |
-
"""Convert various input in BGR order to normalized RGB matplotlib color
|
16 |
-
tuples,
|
17 |
-
|
18 |
-
Args:
|
19 |
-
color (:obj:`Color`/str/tuple/int/ndarray): Color inputs
|
20 |
-
|
21 |
-
Returns:
|
22 |
-
tuple[float]: A tuple of 3 normalized floats indicating RGB channels.
|
23 |
-
"""
|
24 |
-
color = mmcv.color_val(color)
|
25 |
-
color = [color / 255 for color in color[::-1]]
|
26 |
-
return tuple(color)
|
27 |
-
|
28 |
-
|
29 |
-
def imshow_det_bboxes(img,
|
30 |
-
bboxes,
|
31 |
-
labels,
|
32 |
-
segms=None,
|
33 |
-
class_names=None,
|
34 |
-
score_thr=0,
|
35 |
-
bbox_color='green',
|
36 |
-
text_color='green',
|
37 |
-
mask_color=None,
|
38 |
-
thickness=2,
|
39 |
-
font_size=13,
|
40 |
-
win_name='',
|
41 |
-
show=True,
|
42 |
-
wait_time=0,
|
43 |
-
out_file=None):
|
44 |
-
"""Draw bboxes and class labels (with scores) on an image.
|
45 |
-
|
46 |
-
Args:
|
47 |
-
img (str or ndarray): The image to be displayed.
|
48 |
-
bboxes (ndarray): Bounding boxes (with scores), shaped (n, 4) or
|
49 |
-
(n, 5).
|
50 |
-
labels (ndarray): Labels of bboxes.
|
51 |
-
segms (ndarray or None): Masks, shaped (n,h,w) or None
|
52 |
-
class_names (list[str]): Names of each classes.
|
53 |
-
score_thr (float): Minimum score of bboxes to be shown. Default: 0
|
54 |
-
bbox_color (str or tuple(int) or :obj:`Color`):Color of bbox lines.
|
55 |
-
The tuple of color should be in BGR order. Default: 'green'
|
56 |
-
text_color (str or tuple(int) or :obj:`Color`):Color of texts.
|
57 |
-
The tuple of color should be in BGR order. Default: 'green'
|
58 |
-
mask_color (str or tuple(int) or :obj:`Color`, optional):
|
59 |
-
Color of masks. The tuple of color should be in BGR order.
|
60 |
-
Default: None
|
61 |
-
thickness (int): Thickness of lines. Default: 2
|
62 |
-
font_size (int): Font size of texts. Default: 13
|
63 |
-
show (bool): Whether to show the image. Default: True
|
64 |
-
win_name (str): The window name. Default: ''
|
65 |
-
wait_time (float): Value of waitKey param. Default: 0.
|
66 |
-
out_file (str, optional): The filename to write the image.
|
67 |
-
Default: None
|
68 |
-
|
69 |
-
Returns:
|
70 |
-
ndarray: The image with bboxes drawn on it.
|
71 |
-
"""
|
72 |
-
assert bboxes.ndim == 2, \
|
73 |
-
f' bboxes ndim should be 2, but its ndim is {bboxes.ndim}.'
|
74 |
-
assert labels.ndim == 1, \
|
75 |
-
f' labels ndim should be 1, but its ndim is {labels.ndim}.'
|
76 |
-
assert bboxes.shape[0] == labels.shape[0], \
|
77 |
-
'bboxes.shape[0] and labels.shape[0] should have the same length.'
|
78 |
-
assert bboxes.shape[1] == 4 or bboxes.shape[1] == 5, \
|
79 |
-
f' bboxes.shape[1] should be 4 or 5, but its {bboxes.shape[1]}.'
|
80 |
-
img = mmcv.imread(img).astype(np.uint8)
|
81 |
-
|
82 |
-
if score_thr > 0:
|
83 |
-
assert bboxes.shape[1] == 5
|
84 |
-
scores = bboxes[:, -1]
|
85 |
-
inds = scores > score_thr
|
86 |
-
bboxes = bboxes[inds, :]
|
87 |
-
labels = labels[inds]
|
88 |
-
if segms is not None:
|
89 |
-
if len(inds) != len(segms):
|
90 |
-
inds = np.repeat(a = inds, repeats = 2)
|
91 |
-
segms = segms[inds, ...]
|
92 |
-
|
93 |
-
mask_colors = []
|
94 |
-
if labels.shape[0] > 0:
|
95 |
-
if mask_color is None:
|
96 |
-
# random color
|
97 |
-
np.random.seed(46)
|
98 |
-
mask_colors = [
|
99 |
-
np.random.randint(0, 256, (1, 3), dtype=np.uint8)
|
100 |
-
#for _ in range(max(labels) + 2)
|
101 |
-
for _ in range(100)
|
102 |
-
]
|
103 |
-
#print(mask_colors)
|
104 |
-
#asas
|
105 |
-
else:
|
106 |
-
# specify color
|
107 |
-
mask_colors = [
|
108 |
-
np.array(mmcv.color_val(mask_color)[::-1], dtype=np.uint8)
|
109 |
-
] * (
|
110 |
-
max(labels) + 1)
|
111 |
-
|
112 |
-
bbox_color = color_val_matplotlib(bbox_color)
|
113 |
-
text_color = color_val_matplotlib(text_color)
|
114 |
-
|
115 |
-
img = mmcv.bgr2rgb(img)
|
116 |
-
width, height = img.shape[1], img.shape[0]
|
117 |
-
img = np.ascontiguousarray(img)
|
118 |
-
|
119 |
-
fig = plt.figure(win_name, frameon=False)
|
120 |
-
plt.title(win_name)
|
121 |
-
canvas = fig.canvas
|
122 |
-
dpi = fig.get_dpi()
|
123 |
-
# add a small EPS to avoid precision lost due to matplotlib's truncation
|
124 |
-
# (https://github.com/matplotlib/matplotlib/issues/15363)
|
125 |
-
fig.set_size_inches((width + EPS) / dpi, (height + EPS) / dpi)
|
126 |
-
|
127 |
-
# remove white edges by set subplot margin
|
128 |
-
plt.subplots_adjust(left=0, right=1, bottom=0, top=1)
|
129 |
-
ax = plt.gca()
|
130 |
-
ax.axis('off')
|
131 |
-
|
132 |
-
polygons = []
|
133 |
-
color = []
|
134 |
-
img_bound =img*0
|
135 |
-
#img=img*0
|
136 |
-
for i, (bbox, label) in enumerate(zip(bboxes, labels)):
|
137 |
-
bbox_int = bbox.astype(np.int32)
|
138 |
-
poly = [[bbox_int[0], bbox_int[1]], [bbox_int[0], bbox_int[3]],
|
139 |
-
[bbox_int[2], bbox_int[3]], [bbox_int[2], bbox_int[1]]]
|
140 |
-
np_poly = np.array(poly).reshape((4, 2))
|
141 |
-
polygons.append(Polygon(np_poly))
|
142 |
-
color.append(bbox_color)
|
143 |
-
label_text = class_names[
|
144 |
-
label] if class_names is not None else f'class {label}'
|
145 |
-
if len(bbox) > 4:
|
146 |
-
label_text += f'|{bbox[-1]:.02f}'
|
147 |
-
'''
|
148 |
-
ax.text(
|
149 |
-
bbox_int[0],
|
150 |
-
bbox_int[1],
|
151 |
-
f'{label_text}',
|
152 |
-
bbox={
|
153 |
-
'facecolor': 'black',
|
154 |
-
'alpha': 0.8,
|
155 |
-
'pad': 0.7,
|
156 |
-
'edgecolor': 'none'
|
157 |
-
},
|
158 |
-
color=text_color,
|
159 |
-
fontsize=font_size,
|
160 |
-
verticalalignment='top',
|
161 |
-
horizontalalignment='left')
|
162 |
-
'''
|
163 |
-
if segms is not None:
|
164 |
-
for ll in range(1):
|
165 |
-
color_mask = mask_colors[np.random.randint(0, 99)]
|
166 |
-
mask = segms[len(labels)*ll+i].astype(bool)
|
167 |
-
show_border = True
|
168 |
-
img[mask] = img[mask] * 0.5 + color_mask * 0.5
|
169 |
-
if show_border:
|
170 |
-
contours,_ = cv2.findContours(mask.copy().astype('uint8'), cv2.RETR_CCOMP, cv2.CHAIN_APPROX_NONE)
|
171 |
-
border_thick = min(int(4*(max(bbox_int[2]-bbox_int[0],bbox_int[3]-bbox_int[1])/300))+1,6)
|
172 |
-
cv2.drawContours(img, contours, -1, (int(color_mask[0][0]),int(color_mask[0][1]),int(color_mask[0][2])), border_thick)
|
173 |
-
#img = cv2.addWeighted(img,1.0,img_bound,1.0,0)
|
174 |
-
|
175 |
-
#img[img_bound>0] = img_bound
|
176 |
-
|
177 |
-
plt.imshow(img)
|
178 |
-
|
179 |
-
p = PatchCollection(
|
180 |
-
polygons, facecolor='none', edgecolors=color, linewidths=thickness)
|
181 |
-
#ax.add_collection(p)
|
182 |
-
|
183 |
-
stream, _ = canvas.print_to_buffer()
|
184 |
-
buffer = np.frombuffer(stream, dtype='uint8')
|
185 |
-
img_rgba = buffer.reshape(height, width, 4)
|
186 |
-
rgb, alpha = np.split(img_rgba, [3], axis=2)
|
187 |
-
img = rgb.astype('uint8')
|
188 |
-
img = mmcv.rgb2bgr(img)
|
189 |
-
|
190 |
-
if show:
|
191 |
-
# We do not use cv2 for display because in some cases, opencv will
|
192 |
-
# conflict with Qt, it will output a warning: Current thread
|
193 |
-
# is not the object's thread. You can refer to
|
194 |
-
# https://github.com/opencv/opencv-python/issues/46 for details
|
195 |
-
if wait_time == 0:
|
196 |
-
plt.show()
|
197 |
-
else:
|
198 |
-
plt.show(block=False)
|
199 |
-
plt.pause(wait_time)
|
200 |
-
if out_file is not None:
|
201 |
-
mmcv.imwrite(img, out_file)
|
202 |
-
|
203 |
-
plt.close()
|
204 |
-
|
205 |
-
return img
|
206 |
-
|
207 |
-
|
208 |
-
def imshow_gt_det_bboxes(img,
|
209 |
-
annotation,
|
210 |
-
result,
|
211 |
-
class_names=None,
|
212 |
-
score_thr=0,
|
213 |
-
gt_bbox_color=(255, 102, 61),
|
214 |
-
gt_text_color=(255, 102, 61),
|
215 |
-
gt_mask_color=(255, 102, 61),
|
216 |
-
det_bbox_color=(72, 101, 241),
|
217 |
-
det_text_color=(72, 101, 241),
|
218 |
-
det_mask_color=(72, 101, 241),
|
219 |
-
thickness=2,
|
220 |
-
font_size=13,
|
221 |
-
win_name='',
|
222 |
-
show=True,
|
223 |
-
wait_time=0,
|
224 |
-
out_file=None):
|
225 |
-
"""General visualization GT and result function.
|
226 |
-
|
227 |
-
Args:
|
228 |
-
img (str or ndarray): The image to be displayed.)
|
229 |
-
annotation (dict): Ground truth annotations where contain keys of
|
230 |
-
'gt_bboxes' and 'gt_labels' or 'gt_masks'
|
231 |
-
result (tuple[list] or list): The detection result, can be either
|
232 |
-
(bbox, segm) or just bbox.
|
233 |
-
class_names (list[str]): Names of each classes.
|
234 |
-
score_thr (float): Minimum score of bboxes to be shown. Default: 0
|
235 |
-
gt_bbox_color (str or tuple(int) or :obj:`Color`):Color of bbox lines.
|
236 |
-
The tuple of color should be in BGR order. Default: (255, 102, 61)
|
237 |
-
gt_text_color (str or tuple(int) or :obj:`Color`):Color of texts.
|
238 |
-
The tuple of color should be in BGR order. Default: (255, 102, 61)
|
239 |
-
gt_mask_color (str or tuple(int) or :obj:`Color`, optional):
|
240 |
-
Color of masks. The tuple of color should be in BGR order.
|
241 |
-
Default: (255, 102, 61)
|
242 |
-
det_bbox_color (str or tuple(int) or :obj:`Color`):Color of bbox lines.
|
243 |
-
The tuple of color should be in BGR order. Default: (72, 101, 241)
|
244 |
-
det_text_color (str or tuple(int) or :obj:`Color`):Color of texts.
|
245 |
-
The tuple of color should be in BGR order. Default: (72, 101, 241)
|
246 |
-
det_mask_color (str or tuple(int) or :obj:`Color`, optional):
|
247 |
-
Color of masks. The tuple of color should be in BGR order.
|
248 |
-
Default: (72, 101, 241)
|
249 |
-
thickness (int): Thickness of lines. Default: 2
|
250 |
-
font_size (int): Font size of texts. Default: 13
|
251 |
-
win_name (str): The window name. Default: ''
|
252 |
-
show (bool): Whether to show the image. Default: True
|
253 |
-
wait_time (float): Value of waitKey param. Default: 0.
|
254 |
-
out_file (str, optional): The filename to write the image.
|
255 |
-
Default: None
|
256 |
-
|
257 |
-
Returns:
|
258 |
-
ndarray: The image with bboxes or masks drawn on it.
|
259 |
-
"""
|
260 |
-
assert 'gt_bboxes' in annotation
|
261 |
-
assert 'gt_labels' in annotation
|
262 |
-
assert isinstance(
|
263 |
-
result,
|
264 |
-
(tuple, list)), f'Expected tuple or list, but get {type(result)}'
|
265 |
-
|
266 |
-
gt_masks = annotation.get('gt_masks', None)
|
267 |
-
if gt_masks is not None:
|
268 |
-
gt_masks = mask2ndarray(gt_masks)
|
269 |
-
|
270 |
-
img = mmcv.imread(img)
|
271 |
-
|
272 |
-
img = imshow_det_bboxes(
|
273 |
-
img,
|
274 |
-
annotation['gt_bboxes'],
|
275 |
-
annotation['gt_labels'],
|
276 |
-
gt_masks,
|
277 |
-
class_names=class_names,
|
278 |
-
bbox_color=gt_bbox_color,
|
279 |
-
text_color=gt_text_color,
|
280 |
-
mask_color=gt_mask_color,
|
281 |
-
thickness=thickness,
|
282 |
-
font_size=font_size,
|
283 |
-
win_name=win_name,
|
284 |
-
show=False)
|
285 |
-
|
286 |
-
if isinstance(result, tuple):
|
287 |
-
bbox_result, segm_result = result
|
288 |
-
if isinstance(segm_result, tuple):
|
289 |
-
segm_result = segm_result[0] # ms rcnn
|
290 |
-
else:
|
291 |
-
bbox_result, segm_result = result, None
|
292 |
-
|
293 |
-
bboxes = np.vstack(bbox_result)
|
294 |
-
labels = [
|
295 |
-
np.full(bbox.shape[0], i, dtype=np.int32)
|
296 |
-
for i, bbox in enumerate(bbox_result)
|
297 |
-
]
|
298 |
-
labels = np.concatenate(labels)
|
299 |
-
|
300 |
-
segms = None
|
301 |
-
if segm_result is not None and len(labels) > 0: # non empty
|
302 |
-
segms = mmcv.concat_list(segm_result)
|
303 |
-
segms = mask_util.decode(segms)
|
304 |
-
segms = segms.transpose(2, 0, 1)
|
305 |
-
|
306 |
-
img = imshow_det_bboxes(
|
307 |
-
img,
|
308 |
-
bboxes,
|
309 |
-
labels,
|
310 |
-
segms=segms,
|
311 |
-
class_names=class_names,
|
312 |
-
score_thr=score_thr,
|
313 |
-
bbox_color=det_bbox_color,
|
314 |
-
text_color=det_text_color,
|
315 |
-
mask_color=det_mask_color,
|
316 |
-
thickness=thickness,
|
317 |
-
font_size=font_size,
|
318 |
-
win_name=win_name,
|
319 |
-
show=show,
|
320 |
-
wait_time=wait_time,
|
321 |
-
out_file=out_file)
|
322 |
-
return img
|
|
|
|
|
|
|
|
|
|
|
|
|
|
|
|
|
|
|
|
|
|
|
|
|
|
|
|
|
|
|
|
|
|
|
|
|
|
|
|
|
|
|
|
|
|
|
|
|
|
|
|
|
|
|
|
|
|
|
|
|
|
|
|
|
|
|
|
|
|
|
|
|
|
|
|
|
|
|
|
|
|
|
|
|
|
|
|
|
|
|
|
|
|
|
|
|
|
|
|
|
|
|
|
|
|
|
|
|
|
|
|
|
|
|
|
|
|
|
|
|
|
|
|
|
|
|
|
|
|
|
|
|
|
|
|
|
|
|
|
|
|
|
|
|
|
|
|
|
|
|
|
|
|
|
|
|
|
|
|
|
|
|
|
|
|
|
|
|
|
|
|
|
|
|
|
|
|
|
|
|
|
|
|
|
|
|
|
|
|
|
|
|
|
|
|
|
|
|
|
|
|
|
|
|
|
|
|
|
|
|
|
|
|
|
|
|
|
|
|
|
|
|
|
|
|
|
|
|
|
|
|
|
|
|
|
|
|
|
|
|
|
|
|
|
|
|
|
|
|
|
|
|
|
|
|
|
|
|
|
|
|
|
|
|
|
|
|
|
|
|
|
|
|
|
|
|
|
|
|
|
|
|
|
|
|
|
|
|
|
|
|
|
|
|
|
|
|
|
|
|
|
|
|
|
|
|
|
|
|
|
|
|
|
|
|
|
|
|
|
|
|
|
|
|
|
|
|
|
|
|
|
|
|
|
|
|
|
|
|
|
|
|
|
|
|
|
|
|
|
|
|
|
|
|
|
|
|
|
|
|
|
|
|
|
|
|
|
|
|
|
|
|
|
|
|
|
|
|
|
|
|
|
|
|
|
|
|
|
|
|
|
|
|
|
|
|
|
|
|
|
|
|
|
|
|
|
|
|
|
|
|
|
|
|
|
|
|
|
|
|
|
|
|
|
|
|
|
|
|
|
|
|
|
|
|
|
|
|
|
|
|
|
|
|
|
|
|
|
|
|
|
|
|
|
|
|
|
|
|
|
|
|
|
|
|
|
|
|
|
|
|
|
|
|
|
|
|
|
|
|
|
|
|
|
|
|
|
|
|
|
|
|
|
|
|
|
|
|
|
|
|
|
|
|
|
|
|
|
|
|
|
|
|
|
|
|
|
|
|
|
|
|
|
|
|
|
|
|
|
|
|
|
|
|
|
|
|
|
|
|
|
|
|
|
|
|
|
|
|
|
|
|
|
|
|
|
|
|
|
|
|
|
|
|
|
|
|
|
|
|
|
|
|
|
|
|
|
|
|
|
|
|
|
|
|
|
|
|
|
|
|
|
|
|
|
|
|
|
|
|
|
|
|
|
|
|
|
|
|
|
|
|
|
|
|
|
|
|
|
|
|
|
|
|
|
|
|
|
|
|
|
|
|
|
|
|
|
|
|
|
|
|
|
|
|
spaces/CVPR/transfiner/demo/README.md
DELETED
@@ -1,5 +0,0 @@
|
|
1 |
-
|
2 |
-
## Mask Transfiner Demo
|
3 |
-
|
4 |
-
For visualization demo, please refer to our [visualization script](https://github.com/SysCV/transfiner#visualization).
|
5 |
-
|
|
|
|
|
|
|
|
|
|
|
|
spaces/Caoyunkang/Segment-Any-Anomaly/GroundingDINO/groundingdino/util/get_tokenlizer.py
DELETED
@@ -1,26 +0,0 @@
|
|
1 |
-
from transformers import AutoTokenizer, BertModel, BertTokenizer, RobertaModel, RobertaTokenizerFast
|
2 |
-
|
3 |
-
|
4 |
-
def get_tokenlizer(text_encoder_type):
|
5 |
-
if not isinstance(text_encoder_type, str):
|
6 |
-
# print("text_encoder_type is not a str")
|
7 |
-
if hasattr(text_encoder_type, "text_encoder_type"):
|
8 |
-
text_encoder_type = text_encoder_type.text_encoder_type
|
9 |
-
elif text_encoder_type.get("text_encoder_type", False):
|
10 |
-
text_encoder_type = text_encoder_type.get("text_encoder_type")
|
11 |
-
else:
|
12 |
-
raise ValueError(
|
13 |
-
"Unknown type of text_encoder_type: {}".format(type(text_encoder_type))
|
14 |
-
)
|
15 |
-
print("final text_encoder_type: {}".format(text_encoder_type))
|
16 |
-
|
17 |
-
tokenizer = AutoTokenizer.from_pretrained(text_encoder_type)
|
18 |
-
return tokenizer
|
19 |
-
|
20 |
-
|
21 |
-
def get_pretrained_language_model(text_encoder_type):
|
22 |
-
if text_encoder_type == "bert-base-uncased":
|
23 |
-
return BertModel.from_pretrained(text_encoder_type)
|
24 |
-
if text_encoder_type == "roberta-base":
|
25 |
-
return RobertaModel.from_pretrained(text_encoder_type)
|
26 |
-
raise ValueError("Unknown text_encoder_type {}".format(text_encoder_type))
|
|
|
|
|
|
|
|
|
|
|
|
|
|
|
|
|
|
|
|
|
|
|
|
|
|
|
|
|
|
|
|
|
|
|
|
|
|
|
|
|
|
|
|
|
|
|
|
|
|
|
|
|
|
spaces/ChristopherMarais/Andrew_AI-BB_classification-beta/mysite/andrew_alpha/urls.py
DELETED
@@ -1,8 +0,0 @@
|
|
1 |
-
from django.urls import path
|
2 |
-
from andrew_alpha import views
|
3 |
-
from .views import process_uploaded_image
|
4 |
-
|
5 |
-
urlpatterns = [
|
6 |
-
path('', views.andrew_alpha, name='andrew_alpha'),
|
7 |
-
path('process_uploaded_image/', process_uploaded_image),
|
8 |
-
]
|
|
|
|
|
|
|
|
|
|
|
|
|
|
|
|
|
|
spaces/ClassCat/DETR-Object-Detection/README.md
DELETED
@@ -1,12 +0,0 @@
|
|
1 |
-
---
|
2 |
-
title: DETR Object Detection
|
3 |
-
emoji: 📚
|
4 |
-
colorFrom: blue
|
5 |
-
colorTo: green
|
6 |
-
sdk: gradio
|
7 |
-
sdk_version: 3.16.2
|
8 |
-
app_file: app.py
|
9 |
-
pinned: false
|
10 |
-
---
|
11 |
-
|
12 |
-
Check out the configuration reference at https://huggingface.co/docs/hub/spaces-config-reference
|
|
|
|
|
|
|
|
|
|
|
|
|
|
|
|
|
|
|
|
|
|
|
|
|
|
spaces/DQChoi/gpt-demo/venv/lib/python3.11/site-packages/gradio/components/__init__.py
DELETED
@@ -1,116 +0,0 @@
|
|
1 |
-
from gradio.components.annotated_image import AnnotatedImage
|
2 |
-
from gradio.components.audio import Audio
|
3 |
-
from gradio.components.bar_plot import BarPlot
|
4 |
-
from gradio.components.base import (
|
5 |
-
Column,
|
6 |
-
Component,
|
7 |
-
Form,
|
8 |
-
FormComponent,
|
9 |
-
IOComponent,
|
10 |
-
Row,
|
11 |
-
_Keywords,
|
12 |
-
component,
|
13 |
-
get_component_instance,
|
14 |
-
)
|
15 |
-
from gradio.components.button import Button
|
16 |
-
from gradio.components.carousel import Carousel
|
17 |
-
from gradio.components.chatbot import Chatbot
|
18 |
-
from gradio.components.checkbox import Checkbox
|
19 |
-
from gradio.components.checkboxgroup import CheckboxGroup
|
20 |
-
from gradio.components.clear_button import ClearButton
|
21 |
-
from gradio.components.code import Code
|
22 |
-
from gradio.components.color_picker import ColorPicker
|
23 |
-
from gradio.components.dataframe import Dataframe
|
24 |
-
from gradio.components.dataset import Dataset
|
25 |
-
from gradio.components.dropdown import Dropdown
|
26 |
-
from gradio.components.duplicate_button import DuplicateButton
|
27 |
-
from gradio.components.file import File
|
28 |
-
from gradio.components.gallery import Gallery
|
29 |
-
from gradio.components.highlighted_text import HighlightedText
|
30 |
-
from gradio.components.html import HTML
|
31 |
-
from gradio.components.image import Image
|
32 |
-
from gradio.components.interpretation import Interpretation
|
33 |
-
from gradio.components.json_component import JSON
|
34 |
-
from gradio.components.label import Label
|
35 |
-
from gradio.components.line_plot import LinePlot
|
36 |
-
from gradio.components.markdown import Markdown
|
37 |
-
from gradio.components.model3d import Model3D
|
38 |
-
from gradio.components.number import Number
|
39 |
-
from gradio.components.plot import Plot
|
40 |
-
from gradio.components.radio import Radio
|
41 |
-
from gradio.components.scatter_plot import ScatterPlot
|
42 |
-
from gradio.components.slider import Slider
|
43 |
-
from gradio.components.state import State, Variable
|
44 |
-
from gradio.components.status_tracker import StatusTracker
|
45 |
-
from gradio.components.textbox import Textbox
|
46 |
-
from gradio.components.timeseries import Timeseries
|
47 |
-
from gradio.components.upload_button import UploadButton
|
48 |
-
from gradio.components.video import Video
|
49 |
-
|
50 |
-
Text = Textbox
|
51 |
-
DataFrame = Dataframe
|
52 |
-
Highlightedtext = HighlightedText
|
53 |
-
Annotatedimage = AnnotatedImage
|
54 |
-
Highlight = HighlightedText
|
55 |
-
Checkboxgroup = CheckboxGroup
|
56 |
-
TimeSeries = Timeseries
|
57 |
-
Json = JSON
|
58 |
-
|
59 |
-
__all__ = [
|
60 |
-
"Audio",
|
61 |
-
"BarPlot",
|
62 |
-
"Button",
|
63 |
-
"Carousel",
|
64 |
-
"Chatbot",
|
65 |
-
"ClearButton",
|
66 |
-
"Component",
|
67 |
-
"component",
|
68 |
-
"get_component_instance",
|
69 |
-
"_Keywords",
|
70 |
-
"Checkbox",
|
71 |
-
"CheckboxGroup",
|
72 |
-
"Code",
|
73 |
-
"ColorPicker",
|
74 |
-
"Column",
|
75 |
-
"Dataframe",
|
76 |
-
"DataFrame",
|
77 |
-
"Dataset",
|
78 |
-
"DuplicateButton",
|
79 |
-
"Form",
|
80 |
-
"FormComponent",
|
81 |
-
"Gallery",
|
82 |
-
"HTML",
|
83 |
-
"Image",
|
84 |
-
"IOComponent",
|
85 |
-
"Interpretation",
|
86 |
-
"JSON",
|
87 |
-
"Json",
|
88 |
-
"Label",
|
89 |
-
"LinePlot",
|
90 |
-
"Markdown",
|
91 |
-
"Textbox",
|
92 |
-
"Dropdown",
|
93 |
-
"Model3D",
|
94 |
-
"File",
|
95 |
-
"HighlightedText",
|
96 |
-
"AnnotatedImage",
|
97 |
-
"CheckboxGroup",
|
98 |
-
"Timeseries",
|
99 |
-
"Text",
|
100 |
-
"Highlightedtext",
|
101 |
-
"Annotatedimage",
|
102 |
-
"Highlight",
|
103 |
-
"Checkboxgroup",
|
104 |
-
"TimeSeries",
|
105 |
-
"Number",
|
106 |
-
"Plot",
|
107 |
-
"Radio",
|
108 |
-
"Row",
|
109 |
-
"ScatterPlot",
|
110 |
-
"Slider",
|
111 |
-
"State",
|
112 |
-
"Variable",
|
113 |
-
"StatusTracker",
|
114 |
-
"UploadButton",
|
115 |
-
"Video",
|
116 |
-
]
|
|
|
|
|
|
|
|
|
|
|
|
|
|
|
|
|
|
|
|
|
|
|
|
|
|
|
|
|
|
|
|
|
|
|
|
|
|
|
|
|
|
|
|
|
|
|
|
|
|
|
|
|
|
|
|
|
|
|
|
|
|
|
|
|
|
|
|
|
|
|
|
|
|
|
|
|
|
|
|
|
|
|
|
|
|
|
|
|
|
|
|
|
|
|
|
|
|
|
|
|
|
|
|
|
|
|
|
|
|
|
|
|
|
|
|
|
|
|
|
|
|
|
|
|
|
|
|
|
|
|
|
|
|
|
|
|
|
|
|
|
|
|
|
|
|
|
|
|
|
|
|
|
|
|
|
|
|
|
|
|
|
|
|
|
|
|
|
|
|
|
|
|
|
|
|
|
|
|
|
|
|
|
|
|
|
|
|
|
|
|
|
|
|
|
|
|
|
|
|
|
|
|
|
|
|
|
|
|
|
|
|
|
|
|
|
|
|
|
|
|
|
|
|
|
|
|
|
|
|
|
|
|
|
spaces/DQChoi/gpt-demo/venv/lib/python3.11/site-packages/gradio/components/status_tracker.py
DELETED
@@ -1,13 +0,0 @@
|
|
1 |
-
"""gr.StatusTracker() component."""
|
2 |
-
from gradio_client.serializing import SimpleSerializable
|
3 |
-
|
4 |
-
from gradio.components.base import Component
|
5 |
-
from gradio.deprecation import warn_deprecation
|
6 |
-
|
7 |
-
|
8 |
-
class StatusTracker(Component, SimpleSerializable):
|
9 |
-
def __init__(
|
10 |
-
self,
|
11 |
-
**kwargs,
|
12 |
-
):
|
13 |
-
warn_deprecation("The StatusTracker component is deprecated.")
|
|
|
|
|
|
|
|
|
|
|
|
|
|
|
|
|
|
|
|
|
|
|
|
|
|
|
|
spaces/DaFujaTyping/hf-Chat-ui/src/lib/stores/pendingMessageIdToRetry.ts
DELETED
@@ -1,4 +0,0 @@
|
|
1 |
-
import type { Message } from "$lib/types/Message";
|
2 |
-
import { writable } from "svelte/store";
|
3 |
-
|
4 |
-
export const pendingMessageIdToRetry = writable<Message["id"] | null>(null);
|
|
|
|
|
|
|
|
|
|
spaces/Daniton/facebook-blenderbot-3Byx/README.md
DELETED
@@ -1,13 +0,0 @@
|
|
1 |
-
---
|
2 |
-
title: Facebook Blenderbot 3B
|
3 |
-
emoji: 😻
|
4 |
-
colorFrom: purple
|
5 |
-
colorTo: green
|
6 |
-
sdk: gradio
|
7 |
-
sdk_version: 3.28.3
|
8 |
-
app_file: app.py
|
9 |
-
pinned: false
|
10 |
-
duplicated_from: Grey41/facebook-blenderbot-3B
|
11 |
-
---
|
12 |
-
|
13 |
-
Check out the configuration reference at https://huggingface.co/docs/hub/spaces-config-reference
|
|
|
|
|
|
|
|
|
|
|
|
|
|
|
|
|
|
|
|
|
|
|
|
|
|
|
|