-
-Download windows 10 gamer edition 2020 for both 32-Bit and 64-Bit computer ... November 2020 (x86-x64) ISO files - Direct Download Links + Torrent Magnet. ... 2014 · Windows 7 NVIDIA Edition x86 x64 - Direct Links Posted by : Unknown ... Direct [YEE] Direct Download Installer Autodesk 3ds Max 2020 (x64) Bit Lifetime. 4d29de3e1b
-
-
-
diff --git a/spaces/1line/AutoGPT/autogpt/speech/say.py b/spaces/1line/AutoGPT/autogpt/speech/say.py
deleted file mode 100644
index 727983d12bf334205550a54bcd69a7a36824eda4..0000000000000000000000000000000000000000
--- a/spaces/1line/AutoGPT/autogpt/speech/say.py
+++ /dev/null
@@ -1,41 +0,0 @@
-""" Text to speech module """
-import threading
-from threading import Semaphore
-
-from autogpt.config import Config
-from autogpt.speech.brian import BrianSpeech
-from autogpt.speech.eleven_labs import ElevenLabsSpeech
-from autogpt.speech.gtts import GTTSVoice
-from autogpt.speech.macos_tts import MacOSTTS
-
-CFG = Config()
-DEFAULT_VOICE_ENGINE = GTTSVoice()
-VOICE_ENGINE = None
-if CFG.elevenlabs_api_key:
- VOICE_ENGINE = ElevenLabsSpeech()
-elif CFG.use_mac_os_tts == "True":
- VOICE_ENGINE = MacOSTTS()
-elif CFG.use_brian_tts == "True":
- VOICE_ENGINE = BrianSpeech()
-else:
- VOICE_ENGINE = GTTSVoice()
-
-
-QUEUE_SEMAPHORE = Semaphore(
- 1
-) # The amount of sounds to queue before blocking the main thread
-
-
-def say_text(text: str, voice_index: int = 0) -> None:
- """Speak the given text using the given voice index"""
-
- def speak() -> None:
- success = VOICE_ENGINE.say(text, voice_index)
- if not success:
- DEFAULT_VOICE_ENGINE.say(text)
-
- QUEUE_SEMAPHORE.release()
-
- QUEUE_SEMAPHORE.acquire(True)
- thread = threading.Thread(target=speak)
- thread.start()
diff --git a/spaces/1phancelerku/anime-remove-background/Dig Digger Pro APK 1.0-pro-2022-09-16 The Ultimate File Recovery Tool for Rooted Devices.md b/spaces/1phancelerku/anime-remove-background/Dig Digger Pro APK 1.0-pro-2022-09-16 The Ultimate File Recovery Tool for Rooted Devices.md
deleted file mode 100644
index 2245bba5cf145825bf8e8fdff2a8d2cd46f1831b..0000000000000000000000000000000000000000
--- a/spaces/1phancelerku/anime-remove-background/Dig Digger Pro APK 1.0-pro-2022-09-16 The Ultimate File Recovery Tool for Rooted Devices.md
+++ /dev/null
@@ -1,114 +0,0 @@
-
-
Dig Digger Pro APK: How to Recover Lost Files on Android
-
Have you ever accidentally deleted some important photos, videos, documents, or music files from your Android device? Or have you ever lost some files due to formatting, virus attack, system crash, or other reasons? If yes, then you might be wondering how to get them back. Well, don't worry, because there is a solution for you: Dig Digger Pro APK.
Dig Digger Pro APK is a powerful file recovery app for Android devices that can help you undelete and recover lost files from your internal memory or external storage cards. It is developed by Defiant Technologies, LLC, and it is available for download from various sources .
-
Features of Dig Digger Pro APK
-
Some of the features of Dig Digger Pro APK are:
-
-
It can recover photos, videos, music, documents, and other file types.
-
It can scan both the internal memory and the external storage cards of your device.
-
It can work on both rooted and unrooted devices, but it requires root access for full functionality.
-
It can filter the scan results by name, size, date, or directory.
-
It can preview the recoverable files before restoring them.
-
It can upload the recovered files to Google Drive, Dropbox, or email them.
-
-
How to download and install Dig Digger Pro APK
-
To download and install Dig Digger Pro APK on your Android device, you need to follow these steps:
-
-
Go to one of the sources that offer the app for download .
-
Tap on the download button and wait for the APK file to be downloaded.
-
Once the download is complete, go to your device settings and enable the installation of apps from unknown sources.
-
Locate the downloaded APK file and tap on it to start the installation process.
-
Follow the instructions on the screen and grant the necessary permissions to the app.
-
Wait for the installation to finish and then launch the app from your app drawer.
-
-
How to use Dig Digger Pro APK to recover lost files
-
To use Dig Digger Pro APK to recover lost files from your Android device, you need to follow these steps:
-
Step 1: Launch the app and select the scan mode
-
When you launch the app, you will see two scan modes: Basic Scan and Full Scan. The Basic Scan mode can recover files from your cache and thumbnails without root access. The Full Scan mode can recover files from your entire memory with root access. Choose the scan mode that suits your needs and tap on Start Basic Photo Scan or Start Full Scan.
-
DiskDigger Pro file recovery apk download
-DiskDigger Pro apk full version free
-DiskDigger Pro apk for rooted devices
-DiskDigger Pro file recovery app
-DiskDigger Pro apk latest version
-DiskDigger Pro apk mod unlocked
-DiskDigger Pro apk no root required
-DiskDigger Pro file recovery tool
-DiskDigger Pro apk cracked premium
-DiskDigger Pro apk 2023 update
-DiskDigger Pro file recovery software
-DiskDigger Pro apk best data recovery
-DiskDigger Pro apk android app
-DiskDigger Pro apk paid license
-DiskDigger Pro file recovery guide
-DiskDigger Pro apk review and rating
-DiskDigger Pro apk how to use
-DiskDigger Pro file recovery features
-DiskDigger Pro apk support and help
-DiskDigger Pro apk alternative apps
-DiskDigger Pro file recovery tips and tricks
-DiskDigger Pro apk download link
-DiskDigger Pro apk install and setup
-DiskDigger Pro file recovery tutorial
-DiskDigger Pro apk compatible devices
-DiskDigger Pro file recovery benefits and advantages
-DiskDigger Pro apk refund policy
-DiskDigger Pro apk customer feedback and testimonials
-DiskDigger Pro file recovery comparison and analysis
-DiskDigger Pro apk frequently asked questions (FAQs)
-DiskDigger Pro file recovery success stories and case studies
-DiskDigger Pro apk developer information and contact details
-DiskDigger Pro file recovery pros and cons
-DiskDigger Pro apk system requirements and specifications
-DiskDigger Pro file recovery best practices and recommendations
-DiskDigger Pro apk coupon code and discount offer
-DiskDigger Pro file recovery challenges and solutions
-DiskDigger Pro apk upgrade and update information
-DiskDigger Pro file recovery statistics and facts
-DiskDigger Pro apk terms and conditions and privacy policy
-
Step 2: Choose the storage device and file types
-
The app will then ask you to choose the storage device that you want to scan. You can select either your internal memory or your external storage card. Then, you can choose the file types that you want to recover. You can select photos, videos, music, documents, or all files. Tap on OK to start the scanning process.
Step 3: Preview and recover the files
-
After the scanning process is complete, the app will show you the list of recoverable files. You can tap on each file to preview it and check its details. You can also use the filter option to narrow down the results by name, size, date, or directory. To recover the files, you can select them individually or tap on Select All. Then, you can choose to restore them to your device, upload them to Google Drive or Dropbox, or email them. Tap on Recover to complete the recovery process.
-
Pros and cons of Dig Digger Pro APK
-
Like any other app, Dig Digger Pro APK has its advantages and disadvantages. Here are some of them:
-
Pros
-
Easy to use interface
-
The app has a simple and user-friendly interface that makes it easy to navigate and operate. You can easily select the scan mode, the storage device, the file types, and the recovery options with a few taps.
-
Supports various file formats
-
The app can recover various file formats, such as photos, videos, music, documents, and more. It can also recover files from different apps, such as WhatsApp, Instagram, Facebook, etc.
-
Works on rooted and unrooted devices
-
The app can work on both rooted and unrooted devices, although it requires root access for full functionality. If your device is not rooted, you can still use the Basic Scan mode to recover some files from your cache and thumbnails.
-
Cons
-
Requires root access for full functionality
-
The app requires root access for full functionality, which means that you need to have a rooted device to use the Full Scan mode and recover files from your entire memory. Rooting your device may void your warranty, expose your device to security risks, or cause system instability.
-
May not recover all files
-
The app may not be able to recover all files, especially if they have been overwritten by new data or corrupted by viruses. The recovery success rate depends on various factors, such as the file size, the file format, the storage device condition, etc.
-
May consume battery and memory resources
-
The app may consume a lot of battery and memory resources during the scanning and recovery process. This may affect your device performance and cause overheating or lagging issues. You may want to close other apps and connect your device to a power source before using the app.
-
Conclusion
-
Dig Digger Pro APK is a useful file recovery app for Android devices that can help you recover lost files from your internal memory or external storage cards. It has a simple and user-friendly interface that makes it easy to use. It supports various file formats and works on both rooted and unrooted devices. However, it also has some drawbacks, such as requiring root access for full functionality, not recovering all files, and consuming battery and memory resources. You should weigh the pros and cons before using the app and always backup your important data regularly.
-
FAQs
-
-
Q: Is Dig Digger Pro APK safe to use?
-
A: Dig Digger Pro APK is generally safe to use, as long as you download it from a trusted source and grant it the necessary permissions. However, you should be careful when rooting your device or recovering files from unknown sources, as they may pose some security risks.
-
Q: How long does it take to scan and recover files with Dig Digger Pro APK?
-
A: The scanning and recovery time depends on various factors, such as the scan mode, the storage device size, the file types, the number of files, etc. It may take from a few minutes to several hours.
-
Q: Can I recover files that I deleted a long time ago with Dig Digger Pro APK?
-
A: It depends on whether the files have been overwritten by new data or not. If they have not been overwritten, you may be able to recover them with Dig Digger Pro APK. However, if they have been overwritten, you may not be able to recover them.
-
Q: Can I use Dig Digger Pro APK on other devices besides Android?
-
A: No, Dig Digger Pro APK is only compatible with Android devices. You cannot use it on iOS, Windows, Mac, or other devices.
-
Q: What should I do if I encounter any problems with Dig Digger Pro APK?
A: If you encounter any problems with Dig Digger Pro APK, such as installation errors, scanning failures, recovery errors, etc., you can try the following solutions:
-
-
Check your internet connection and make sure it is stable and fast.
-
Check your device settings and make sure you have enough storage space and battery power.
-
Check your device root status and make sure you have granted the app the necessary permissions.
-
Check the app version and make sure you have updated it to the latest one.
-
Restart your device and try again.
-
Contact the app developer for support or feedback.
-
-
-
I hope this article has helped you learn more about Dig Digger Pro APK and how to use it to recover lost files on Android. If you have any questions or comments, please feel free to leave them below. Thank you for reading!
401be4b1e0
-
-
\ No newline at end of file
diff --git a/spaces/1phancelerku/anime-remove-background/Download Real Racing 3 APK from APKPure and Enjoy the Premier Racing Experience on Android.md b/spaces/1phancelerku/anime-remove-background/Download Real Racing 3 APK from APKPure and Enjoy the Premier Racing Experience on Android.md
deleted file mode 100644
index 562ddf3ac3bde85511aacc6c5b542e07eaf753d7..0000000000000000000000000000000000000000
--- a/spaces/1phancelerku/anime-remove-background/Download Real Racing 3 APK from APKPure and Enjoy the Premier Racing Experience on Android.md
+++ /dev/null
@@ -1,81 +0,0 @@
-
-
Download Real Racing 3 APKPure: The Ultimate Guide
-
If you are a fan of racing games, you have probably heard of Real Racing 3, one of the most realistic and immersive racing games on mobile devices. But did you know that you can download it from APKPure, a third-party app store that offers fast and safe downloads of free and updated apps? In this article, we will tell you everything you need to know about Real Racing 3 and APKPure, and how to download them on your Android device.
-
What is Real Racing 3?
-
Real Racing 3 is a racing game developed by Firemonkeys Studios and published by Electronic Arts. It was released in 2013 for iOS and Android devices, and has since become one of the most popular and acclaimed racing games on mobile platforms. It has won several awards, such as the Best Mobile Game at the BAFTA Games Awards, and has been downloaded over 500 million times.
Real Racing 3 offers a variety of features that make it stand out from other racing games. Here are some of them:
-
Real cars, real tracks, real people
-
Real Racing 3 features over 300 licensed cars from top manufacturers, such as Ferrari, Lamborghini, Porsche, Bugatti, and more. You can race on over 40 real-world tracks, such as Silverstone, Le Mans, Dubai Autodrome, and more. You can also compete with real players from around the world in online multiplayer modes, or challenge their AI-controlled versions in Time-Shifted Multiplayer.
-
Over 4,000 events and challenges
-
Real Racing 3 offers a variety of events and challenges to test your skills and earn rewards. You can participate in Formula 1® Grands Prix™, Cup races, Eliminations, Endurance challenges, and more. You can also join clubs and teams to collaborate with other players and unlock exclusive content.
-
Customization and upgrades
-
Real Racing 3 allows you to customize your cars with different paint jobs, vinyls, rims, spoilers, and more. You can also upgrade your cars with different parts and performance boosts to improve their speed, handling, acceleration, and braking. You can also tune your cars to suit your driving style and preferences.
-
What is APKPure?
-
APKPure is a third-party app store that provides fast and safe downloads of free and updated apps for Android devices. It was launched in 2014 and has since become one of the most popular alternatives to Google Play Store. It has over 10 million users and over 300 thousand apps in its library.
-
Benefits of using APKPure
-
APKPure offers several benefits that make it a great choice for downloading apps. Here are some of them:
-
Fast and safe downloads
-
APKPure uses advanced technology to ensure that the downloads are fast and secure. It scans all the apps for viruses and malware before uploading them to its servers. It also verifies the signatures of the apps to ensure that they are original and not modified. You can also pause and resume your downloads at any time.
-
Free and updated apps
-
APKPure provides free access to all the apps in its library. You don't need to pay any fees or subscriptions to download or use them. You can also get the latest updates of the apps as soon as they are released by the developers. You can also discover new and trending apps that are not available on Google Play Store.
-
download real racing 3 apk mod unlimited money
-download real racing 3 apk obb latest version
-download real racing 3 apk data highly compressed
-download real racing 3 apk offline installer
-download real racing 3 apk for android free
-download real racing 3 apk pure full game
-download real racing 3 apk update new cars
-download real racing 3 apk hack unlock all
-download real racing 3 apk from apkpure.com[^1^]
-download real racing 3 apk and sd data
-download real racing 3 apk mod revdl
-download real racing 3 apk no ads
-download real racing 3 apk for pc windows 10
-download real racing 3 apk mirror link
-download real racing 3 apk old version
-download real racing 3 apk mod menu
-download real racing 3 apk rexdl
-download real racing 3 apk without wifi
-download real racing 3 apk for ios devices
-download real racing 3 apk with cheat codes
-download real racing 3 apkpure latest update
-download real racing 3 apkpure modded version
-download real racing 3 apkpure offline mode
-download real racing 3 apkpure for android tv
-download real racing 3 apkpure with all tracks
-download real racing 3 apkpure premium features
-download real racing 3 apkpure cracked apk
-download real racing 3 apkpure for firestick
-download real racing 3 apkpure safe and secure
-download real racing 3 apkpure original file
-
No region restrictions
-
APKPure allows you to download and use apps that are not available in your region or country. You don't need to use a VPN or change your location settings to access them. You can also switch between different languages and regions to explore more apps from different markets.
-
How to download Real Racing 3 APKPure?
-
If you want to download Real Racing 3 APKPure, you need to follow these simple steps:
-
Step-by-step instructions
-
Visit the APKPure website or app
-
You can either visit the APKPure website (https://apkpure.com/) on your browser, or download the APKPure app from the website or from other sources. The app is compatible with Android 4.1 and above, and requires about 15 MB of storage space.
-
Search for Real Racing 3 and tap on it
-
Once you are on the APKPure website or app, you can search for Real Racing 3 using the search bar. You will see the app icon, name, rating, and size. Tap on it to open the app page.
-
Download and install the APK file
-
On the app page, you will see a green button that says "Download APK". Tap on it to start downloading the APK file. The file size is about 45 MB, so make sure you have enough space and a stable internet connection. Once the download is complete, you will see a notification that says "Download successful". Tap on it to open the file and install it on your device. You may need to enable the "Unknown sources" option in your settings to allow the installation of apps from outside Google Play Store.
-
Conclusion
-
Real Racing 3 is one of the best racing games on mobile devices, and APKPure is one of the best app stores to download it from. By following the steps above, you can enjoy this amazing game with fast and safe downloads, free and updated apps, and no region restrictions. So what are you waiting for? Download Real Racing 3 APKPure today and experience the thrill of racing like never before!
-
FAQs
-
Here are some frequently asked questions about Real Racing 3 and APKPure:
-
-
Is Real Racing 3 free to play?
-
Yes, Real Racing 3 is free to download and play, but it also offers in-app purchases that can enhance your gameplay experience. You can buy premium currency, cars, upgrades, and more using real money. However, you can also earn these items by playing the game and completing events and challenges.
-
Is APKPure safe to use?
-
Yes, APKPure is safe to use, as it scans all the apps for viruses and malware before uploading them to its servers. It also verifies the signatures of the apps to ensure that they are original and not modified. However, you should always be careful when downloading apps from third-party sources, as they may contain harmful or unwanted content. You should also check the permissions and reviews of the apps before installing them.
-
Can I play Real Racing 3 offline?
-
No, Real Racing 3 requires an internet connection to play, as it uses cloud save technology to sync your progress across devices. It also uses online multiplayer modes and Time-Shifted Multiplayer to let you race with real players from around the world. However, you can play some events and challenges offline if you have already downloaded them.
-
Can I play Real Racing 3 on PC?
-
No, Real Racing 3 is only available for iOS and Android devices. However, you can use an emulator software to run Android apps on your PC. Some of the popular emulators are BlueStacks, NoxPlayer, and LDPlayer. You can download them from their official websites or from other sources. Then, you can install APKPure on them and download Real Racing 3 from there.
-
How can I update Real Racing 3?
-
If you have downloaded Real Racing 3 from APKPure, you can update it from there as well. You will see a notification when a new update is available for the app. You can tap on it to download and install the update. Alternatively, you can check for updates manually by visiting the app page on APKPure and tapping on the "Update" button.
-
197e85843d
-
-
\ No newline at end of file
diff --git a/spaces/1phancelerku/anime-remove-background/Download the Ultimate Sonic Forces Running Battle Mod with Money Speed and God Mode.md b/spaces/1phancelerku/anime-remove-background/Download the Ultimate Sonic Forces Running Battle Mod with Money Speed and God Mode.md
deleted file mode 100644
index 74d05ba1469dd372c0c1eaadcef3be96fa119d93..0000000000000000000000000000000000000000
--- a/spaces/1phancelerku/anime-remove-background/Download the Ultimate Sonic Forces Running Battle Mod with Money Speed and God Mode.md
+++ /dev/null
@@ -1,99 +0,0 @@
-
-
Download Sonic Forces Running Battle Mod: A Guide for Sonic Fans
-
If you are a fan of Sonic the Hedgehog and love racing games, you might want to try out Sonic Forces Running Battle Mod. This is a modified version of Sonic Forces Running Battle, a mobile game developed by Sega. In this article, we will tell you what this mod is, how to download and install it, and some tips and tricks for playing it.
Sonic Forces Running Battle Mod is a mod that adds some features and changes to the original game. The mod allows you to play with God Mode, unlimited money, and increased speed. You can also unlock all the characters, stages, and items in the game. The mod also improves the graphics, sound, and performance of the game.
-
Features and gameplay of the mod
-
The mod has the same gameplay as the original game, but with some enhancements. You can race and battle with your favorite characters from the Sonic universe, such as Sonic, Knuckles, Shadow, Amy, Tails, and more. You can also create your own custom character with different species, outfits, and Wispons. Wispons are special weapons that harness different kinds of wisp energy, such as lightning, drill, fire, ice, etc. You can use them to attack your opponents or to access hidden paths.
-
The mod has various stages that are based on the locations from the Sonic Forces console game. You can run through Green Hill Zone, Chemical Plant Zone, Metropolis Zone, Mystic Jungle Zone, Death Egg Zone, and more. Each stage has different obstacles, traps, enemies, and power-ups that you need to avoid or use to your advantage. You can also collect rings, items, red star rings, and trophies as you run.
-
The mod has a multiplayer mode where you can race against other players online. You can join or create a room with up to four players and compete for the best time and score. You can also chat with other players and send them emojis. The mod has a ranking system where you can earn points and medals based on your performance. You can also join or create a team with other players and cooperate or challenge them.
-
How to download and install Sonic Forces Running Battle Mod?
-
Requirements and sources for the mod
-
To download and install Sonic Forces Running Battle Mod, you need to have an Android device with at least Android 4.1 or higher. You also need to have at least 300 MB of free space on your device. You also need to have the original game installed on your device.
-
You can download the latest version of Sonic Forces Running Battle Mod from [HappyMod](^1^), a website that provides various mods for Android games. You can also find other sources for the mod online by searching for "sonic forces running battle mod". However, be careful of fake or malicious links that may harm your device.
-
How to download sonic forces running battle mod apk for android
-Sonic forces running battle mod unlimited money and god mode
-Sonic forces speed battle mod features and gameplay
-Best sonic forces running battle mod download sites
-Sonic forces running battle mod review and rating
-Download sonic forces running battle mod for PC and Mac
-Sonic forces running battle mod tips and tricks
-Sonic forces running battle mod latest version and updates
-Sonic forces running battle mod compatibility and requirements
-Sonic forces running battle mod vs original game comparison
-Download sonic forces running battle mod for iOS and iPhone
-Sonic forces running battle mod cheats and hacks
-Sonic forces running battle mod online multiplayer mode
-Sonic forces running battle mod offline mode and data usage
-Sonic forces running battle mod installation guide and troubleshooting
-Download sonic forces running battle mod for Windows 10 and 11
-Sonic forces running battle mod graphics and sound quality
-Sonic forces running battle mod characters and customization
-Sonic forces running battle mod levels and challenges
-Sonic forces running battle mod free download link and virus scan
-Download sonic forces running battle mod for Linux and Ubuntu
-Sonic forces running battle mod speed hack and boost
-Sonic forces running battle mod controller support and settings
-Sonic forces running battle mod achievements and rewards
-Sonic forces running battle mod fan-made mods and community
-Download sonic forces running battle mod for Chromebook and Chrome OS
-Sonic forces running battle mod bugs and glitches fix
-Sonic forces running battle mod ranking and leaderboards
-Sonic forces running battle mod skins and outfits
-Sonic forces running battle mod weapons and items
-Download sonic forces running battle mod for Kindle Fire and Fire OS
-Sonic forces running battle mod storyline and plot
-Sonic forces running battle mod enemies and bosses
-Sonic forces running battle mod missions and objectives
-Sonic forces running battle mod screenshots and videos
-Download sonic forces running battle mod for Nintendo Switch and Switch Lite
-Sonic forces running battle mod alternatives and similar games
-Sonic forces running battle mod feedback and suggestions
-Sonic forces running battle mod FAQs and answers
-Sonic forces running battle mod forums and discussions
-
Steps to install the mod using HedgeModManager or Sonic Forces Mod Installer
-
To install the mod on your device, you need to use a mod installer or manager that can modify the game files. There are two popular tools that you can use: HedgeModManager or Sonic Forces Mod Installer. Here are the steps to install the mod using either tool:
-
-
Download the tool from its official source. For HedgeModManager, you can download it from [here]. For Sonic Forces Mod Installer, you can download it from [here].
-
Extract the tool to a folder on your device. You may need to use a file manager app or a zip extractor app to do this.
-
Open the tool and grant it the necessary permissions to access your device's storage and files.
-
Locate the Sonic Forces Running Battle Mod file that you downloaded from HappyMod or another source. It should be a .zip or .rar file.
-
Select the mod file and click on "Install" or "Apply". The tool will automatically copy and replace the game files with the modded ones.
-
Wait for the installation process to finish. It may take a few minutes depending on the size of the mod and your device's speed.
-
Launch the game and enjoy the mod. You may need to restart your device or clear the game's cache if you encounter any issues.
-
-
Tips and tricks for playing Sonic Forces Running Battle Mod
-
Choose your species, Wispon, and Star Runner wisely
-
One of the fun aspects of Sonic Forces Running Battle Mod is that you can create your own custom character with different options. You can choose from seven different species: wolf, rabbit, cat, dog, bear, bird, or hedgehog. Each species has its own unique ability that can help you in the game. For example, wolves can automatically draw in nearby rings, rabbits can jump higher, cats can always keep one ring after being hit, etc.
-
You can also choose from different Wispons that have different effects and attacks. Some Wispons are better suited for certain stages than others. For example, lightning Wispons can activate electric rails, drill Wispons can dig through sand or snow, fire Wispons can burn wooden bridges or crates, etc. You can also use your Wispon to attack other players or enemies by tapping on them.
-
You can also choose from different Star Runners that have different stats and bonuses. Star Runners are special outfits that you can unlock by collecting red star rings or trophies. They can boost your speed, acceleration, strength, or ring bonus. Some Star Runners also have special effects such as invincibility, magnetism, or double jump.
-
Collect rings, items, and power-ups
-
Rings are the main currency of Sonic Forces Running Battle Mod. You can use them to buy new Wispons, outfits, items, or upgrades. You can also use them to revive yourself if you fall off the stage or get hit by an enemy. You can collect rings by running through them or by using your Wispon ability. You can also get rings by completing missions, achievements, or daily challenges.
-
Items are consumables that you can use before or during a race to give you an edge over your opponents. You can buy items with rings or get them as rewards. Some items are speed shoes, shield, invincibility, bomb, boost, etc. You can use items by tapping on their icons on the screen.
-
Power-ups are temporary effects that you can get by running through special capsules on the stage. They can help you or hinder your opponents. Some power-ups are magnet, lightning, fireball, ice cube, tornado, etc. You can activate power-ups by tapping on their icons on the screen.
-
Use your Wispon abilities and attacks strategically
-
Your Wispon is not only a weapon but also a tool that can help you navigate the stage and reach hidden areas. You can use your Wispon ability by swiping up on the screen. Depending on your Wispon type, you can do different things such as fly, drill, dash, hover, etc. You can also use your Wispon ability to collect rings or items that are out of reach.
-
You can also use your Wispon to attack other players or enemies by tapping on them. Depending on your Wispon type, you can do different things such as shoot projectiles, create explosions, freeze opponents, etc. You can also use your Wispon attack to destroy obstacles or traps that are blocking your way.
-
Avoid obstacles and traps
-
The stages in Sonic Forces Running Battle Mod are full of obstacles and traps that can slow you down or damage you. You need to avoid them or destroy them with your Wispon attack. Some obstacles and traps are spikes, lasers, mines, swinging axes, falling rocks, etc. You can also use obstacles and traps to your advantage by pushing other players into them or by using them as shortcuts.
-
Race against other players online
-
The multiplayer mode in Sonic Forces Running Battle Mod is where you can test your skills and compete with other players online. You can join or create a room with up to four players and race on any stage that you have unlocked. You can also chat with other players and send them emojis. You can choose to race for fun or for ranking points and medals. You can also join or create a team with other players and cooperate or challenge them.
-
The multiplayer mode is fast-paced and competitive, so you need to be quick and smart. You need to use your Wispon abilities and attacks, items, power-ups, and shortcuts to gain an advantage over your rivals. You also need to avoid or counter their attacks, obstacles, and traps. You can also use your Star Runner bonus to boost your performance. The player who reaches the finish line first wins the race.
-
Conclusion and FAQs
-
Summary of the article and benefits of the mod
-
Sonic Forces Running Battle Mod is a great mod for Sonic fans who want to enjoy a more fun and exciting racing game. The mod adds God Mode, unlimited money, increased speed, and all unlocked characters, stages, and items to the original game. The mod also improves the graphics, sound, and performance of the game. The mod has the same gameplay as the original game, but with some enhancements. You can create your own custom character with different species, Wispons, and Star Runners. You can run through various stages that are based on the Sonic Forces console game. You can collect rings, items, power-ups, and trophies as you run. You can also race against other players online in a multiplayer mode.
-
The mod is easy to download and install using HedgeModManager or Sonic Forces Mod Installer. You just need to have an Android device with at least Android 4.1 or higher and 300 MB of free space. You also need to have the original game installed on your device. You can download the mod from HappyMod or other sources online.
-
The mod is fun and challenging to play with some tips and tricks. You need to choose your species, Wispon, and Star Runner wisely based on their abilities, effects, and bonuses. You need to collect rings, items, and power-ups to help you in the game. You need to use your Wispon abilities and attacks strategically to navigate the stage and attack your opponents. You need to avoid obstacles and traps that can slow you down or damage you. You need to race against other players online and use your skills and items to win.
-
Five unique FAQs about the mod
-
-
Question
Answer
-
Is Sonic Forces Running Battle Mod safe to use?
Yes, Sonic Forces Running Battle Mod is safe to use as long as you download it from a trusted source and use a reliable mod installer or manager. However, you should always backup your game data before installing any mod.
-
Can I play Sonic Forces Running Battle Mod offline?
Yes, you can play Sonic Forces Running Battle Mod offline in the single-player mode. However, you will not be able to access the multiplayer mode or some features that require an internet connection.
-
Can I play Sonic Forces Running Battle Mod with my friends?
Yes, you can play Sonic Forces Running Battle Mod with your friends in the multiplayer mode. You can join or create a room with up to four players and race on any stage that you have unlocked. You can also chat with your friends and send them emojis.
-
How do I update Sonic Forces Running Battle Mod?
To update Sonic Forces Running Battle Mod, you need to download the latest version of the mod from HappyMod or another source online. Then, you need to uninstall the previous version of the mod using HedgeModManager or Sonic Forces Mod Installer. After that, you need to install the new version of the mod using the same tool.
-
How do I uninstall Sonic Forces Running Battle Mod?
To uninstall Sonic Forces Running Battle Mod, you need to use HedgeModManager or Sonic Forces Mod Installer. You need to open the tool and locate the mod file that you installed. Then, you need to select the mod file and click on "Uninstall" or "Remove". The tool will automatically restore the original game files.
-
401be4b1e0
-
-
\ No newline at end of file
diff --git a/spaces/1phancelerku/anime-remove-background/Enjoy Live Streaming of Over 100 Football and Basketball Leagues with Live Score 808 APK.md b/spaces/1phancelerku/anime-remove-background/Enjoy Live Streaming of Over 100 Football and Basketball Leagues with Live Score 808 APK.md
deleted file mode 100644
index 832ed91a33b379d2aed961e053b1670a099e829f..0000000000000000000000000000000000000000
--- a/spaces/1phancelerku/anime-remove-background/Enjoy Live Streaming of Over 100 Football and Basketball Leagues with Live Score 808 APK.md
+++ /dev/null
@@ -1,125 +0,0 @@
-
-
Live Score 808 APK: A Unique Football Game for Android
-
Are you a football fan who loves to watch and play the game? Do you want to experience a different kind of football game on your Android device? If yes, then you should try Live Score 808 APK, a free smartphone application that lets you compete against your favorite football teams in a fun and challenging way. In this article, we will tell you everything you need to know about Live Score 808 APK, including its features, how to download and install it, why you should play it, and some tips and tricks to help you win. Let's get started!
-
What is Live Score 808 APK?
-
Live Score 808 APK is a football game that is unlike any other. Instead of controlling an entire team, you can only manipulate a single player at a time. Your goal is to score as many goals as possible while avoiding the opponents and obstacles on the field. You can choose from hundreds of players from different countries and leagues, each with their own skills and abilities. You can also use power-ups and boosters to enhance your performance and overcome the challenges. The game has realistic graphics, sound effects, and animations that make you feel like you are in a real stadium. You can also check the live scores of real matches and compare your results with other players around the world.
Click on the "Download" button and wait for the file to be downloaded on your device.
-
Once the file is downloaded, go to your device's settings and enable the option to install apps from unknown sources.
-
Locate the downloaded file in your device's storage and tap on it to start the installation process.
-
Follow the instructions on the screen and wait for the installation to be completed.
-
Launch the app and enjoy playing Live Score 808 APK!
-
-
Why play Live Score 808 APK?
-
If you are still wondering why you should play Live Score 808 APK, here are some reasons that might convince you:
-
Pros of Live Score 808 APK
-
-
It is a unique and innovative football game that tests your skills and reflexes.
-
It is a fun and entertaining way to pass the time and relieve stress.
-
It is a great way to show your love and support for your favorite football teams and players.
-
It is a challenging and rewarding game that keeps you motivated and engaged.
-
-
Cons
Cons of Live Score 808 APK
-
-
It is a relatively new game that might have some bugs and glitches.
-
It requires a stable internet connection to play and access the live scores.
-
It might consume a lot of battery and data on your device.
-
It might not be compatible with some older or low-end devices.
-
-
Tips and tricks for playing Live Score 808 APK
-
If you want to improve your skills and performance in Live Score 808 APK, here are some tips and tricks that you can use:
-
Choose your player wisely
-
Before you start a match, you can choose from hundreds of players from different countries and leagues. Each player has their own stats and abilities, such as speed, power, accuracy, and stamina. You should choose a player that suits your playstyle and strategy. For example, if you want to score fast and furious goals, you should pick a player with high speed and power. If you want to play more defensively and tactically, you should pick a player with high accuracy and stamina.
-
Use the power-ups and boosters
-
During the match, you can collect and use various power-ups and boosters that can help you score more goals and avoid the enemies. Some of the power-ups and boosters are:
-
-
Shield: It protects you from the opponents and obstacles for a short time.
-
Magnet: It attracts the coins and gems to you for a short time.
-
Rocket: It boosts your speed and power for a short time.
-
Bomb: It explodes and clears the enemies and obstacles around you for a short time.
-
Freeze: It freezes the opponents and obstacles for a short time.
-
-
Watch out for the opponents and obstacles
-
As you play, you will encounter various opponents and obstacles that will try to stop you from scoring. Some of the opponents and obstacles are:
-
live score 808 apk download
-live score 808 apk latest version
-live score 808 apk mod
-live score 808 apk for android
-live score 808 apk free
-live score 808 apk offline
-live score 808 apk update
-live score 808 apk file
-live score 808 apk install
-live score 808 apk review
-live score 808 apk football simulation
-live score 808 apk single player mode
-live score 808 apk online multiplayer
-live score 808 apk realistic graphics
-live score 808 apk easy controls
-live score 808 apk customisation options
-live score 808 apk best teams
-live score 808 apk top players
-live score 808 apk stats and rankings
-live score 808 apk achievements and rewards
-live score 808 apk tips and tricks
-live score 808 apk cheats and hacks
-live score 808 apk bugs and fixes
-live score 808 apk support and feedback
-live score 808 apk news and updates
-live score 808 apk alternatives and competitors
-live score 808 apk comparison and analysis
-live score 808 apk pros and cons
-live score 808 apk features and benefits
-live score 808 apk requirements and compatibility
-live score 808 apk size and speed
-live score 808 apk security and privacy
-live score 808 apk ratings and reviews
-live score 808 apk testimonials and feedbacks
-live score 808 apk screenshots and videos
-live score 808 apk guide and tutorial
-live score 808 apk faq and help
-live score 808 apk forum and community
-live score 808 apk blog and website
-live score 808 apk social media and promotion
-
-
Goalkeeper: He will try to block your shots and save the goals.
-
Defender: He will try to tackle you and steal the ball from you.
-
Mine: It will explode if you touch it and damage your health.
-
Spike: It will pierce you if you touch it and damage your health.
-
Hole: It will make you fall if you step on it and end your run.
-
-
Collect coins and gems
-
As you play, you can also collect coins and gems that are scattered on the field. Coins and gems are useful for buying new players, power-ups, boosters, and other items in the shop. You can also use coins and gems to revive yourself if you lose or to continue playing if you run out of time. The more coins and gems you collect, the more options and advantages you have in the game.
-
Conclusion
-
Live Score 808 APK is a unique football game that lets you compete against your favorite football teams in a fun and challenging way. You can choose from hundreds of players from different countries and leagues, each with their own skills and abilities. You can also use power-ups and boosters to enhance your performance and overcome the challenges. The game has realistic graphics, sound effects, and animations that make you feel like you are in a real stadium. You can also check the live scores of real matches and compare your results with other players around the world. Live Score 808 APK is a free smartphone application that is easy to download and install on your Android device. If you are a football fan who loves to watch and play the game, you should definitely try Live Score 808 APK!
-
FAQs
-
Here are some frequently asked questions about Live Score 808 APK:
-
-
What is the latest version of Live Score 808 APK?
-
The latest version of Live Score 808 APK is 1.0.8, which was released on June 22, 2023. It has some bug fixes and performance improvements.
-
Is Live Score 808 APK safe to use?
-
Yes, Live Score 808 APK is safe to use as long as you download it from the official website or a trusted source. It does not contain any viruses or malware that can harm your device or data.
-
How can I contact the developer of Live Score 808 APK?
-
You can contact the developer of Live Score 808 APK by sending an email to livescore808@gmail.com or by visiting their Facebook page at < a href="">https://www.facebook.com/LiveScore808.
-
Can I play Live Score 808 APK offline?
-
No, you cannot play Live Score 808 APK offline. You need a stable internet connection to play and access the live scores.
-
Can I play Live Score 808 APK on other devices?
-
Yes, you can play Live Score 808 APK on other devices that support Android operating system. However, you might need to adjust the settings and graphics according to your device's specifications.
- 197e85843d
-
-
\ No newline at end of file
diff --git a/spaces/2023Liu2023/bingo/src/components/ui/dialog.tsx b/spaces/2023Liu2023/bingo/src/components/ui/dialog.tsx
deleted file mode 100644
index 925e77fe7858fb218b5115b4e225174a886e0f02..0000000000000000000000000000000000000000
--- a/spaces/2023Liu2023/bingo/src/components/ui/dialog.tsx
+++ /dev/null
@@ -1,128 +0,0 @@
-'use client'
-
-import * as React from 'react'
-import * as DialogPrimitive from '@radix-ui/react-dialog'
-
-import { cn } from '@/lib/utils'
-import { IconClose } from '@/components/ui/icons'
-
-const Dialog = DialogPrimitive.Root
-
-const DialogTrigger = DialogPrimitive.Trigger
-
-const DialogPortal = ({
- className,
- children,
- ...props
-}: DialogPrimitive.DialogPortalProps) => (
-
-
- {children}
-
-
-)
-DialogPortal.displayName = DialogPrimitive.Portal.displayName
-
-const DialogOverlay = React.forwardRef<
- React.ElementRef,
- React.ComponentPropsWithoutRef
->(({ className, ...props }, ref) => (
-
-))
-DialogOverlay.displayName = DialogPrimitive.Overlay.displayName
-
-const DialogContent = React.forwardRef<
- React.ElementRef,
- React.ComponentPropsWithoutRef
->(({ className, children, ...props }, ref) => (
-
-
-
- {children}
-
-
- Close
-
-
-
-))
-DialogContent.displayName = DialogPrimitive.Content.displayName
-
-const DialogHeader = ({
- className,
- ...props
-}: React.HTMLAttributes) => (
-
-)
-DialogHeader.displayName = 'DialogHeader'
-
-const DialogFooter = ({
- className,
- ...props
-}: React.HTMLAttributes) => (
-
-)
-DialogFooter.displayName = 'DialogFooter'
-
-const DialogTitle = React.forwardRef<
- React.ElementRef,
- React.ComponentPropsWithoutRef
->(({ className, ...props }, ref) => (
-
-))
-DialogTitle.displayName = DialogPrimitive.Title.displayName
-
-const DialogDescription = React.forwardRef<
- React.ElementRef,
- React.ComponentPropsWithoutRef
->(({ className, ...props }, ref) => (
-
-))
-DialogDescription.displayName = DialogPrimitive.Description.displayName
-
-export {
- Dialog,
- DialogTrigger,
- DialogContent,
- DialogHeader,
- DialogFooter,
- DialogTitle,
- DialogDescription
-}
diff --git a/spaces/2ndelement/voicevox/voicevox_engine/engine_manifest/EngineManifest.py b/spaces/2ndelement/voicevox/voicevox_engine/engine_manifest/EngineManifest.py
deleted file mode 100644
index 44a9329b40658999fda3f369887ab4455d86372d..0000000000000000000000000000000000000000
--- a/spaces/2ndelement/voicevox/voicevox_engine/engine_manifest/EngineManifest.py
+++ /dev/null
@@ -1,58 +0,0 @@
-from typing import List, Optional
-
-from pydantic import BaseModel, Field
-
-
-class UpdateInfo(BaseModel):
- """
- エンジンのアップデート情報
- """
-
- version: str = Field(title="エンジンのバージョン名")
- descriptions: List[str] = Field(title="アップデートの詳細についての説明")
- contributors: Optional[List[str]] = Field(title="貢献者名")
-
-
-class LicenseInfo(BaseModel):
- """
- 依存ライブラリのライセンス情報
- """
-
- name: str = Field(title="依存ライブラリ名")
- version: Optional[str] = Field(title="依存ライブラリのバージョン")
- license: Optional[str] = Field(title="依存ライブラリのライセンス名")
- text: str = Field(title="依存ライブラリのライセンス本文")
-
-
-class SupportedFeatures(BaseModel):
- """
- エンジンが持つ機能の一覧
- """
-
- adjust_mora_pitch: bool = Field(title="モーラごとの音高の調整")
- adjust_phoneme_length: bool = Field(title="音素ごとの長さの調整")
- adjust_speed_scale: bool = Field(title="全体の話速の調整")
- adjust_pitch_scale: bool = Field(title="全体の音高の調整")
- adjust_intonation_scale: bool = Field(title="全体の抑揚の調整")
- adjust_volume_scale: bool = Field(title="全体の音量の調整")
- interrogative_upspeak: bool = Field(title="疑問文の自動調整")
- synthesis_morphing: bool = Field(title="2人の話者でモーフィングした音声を合成")
- manage_library: bool = Field(title="音声ライブラリのインストール・アンインストール")
-
-
-class EngineManifest(BaseModel):
- """
- エンジン自体に関する情報
- """
-
- manifest_version: str = Field(title="マニフェストのバージョン")
- name: str = Field(title="エンジン名")
- brand_name: str = Field(title="ブランド名")
- uuid: str = Field(title="エンジンのUUID")
- url: str = Field(title="エンジンのURL")
- icon: str = Field(title="エンジンのアイコンをBASE64エンコードしたもの")
- default_sampling_rate: int = Field(title="デフォルトのサンプリング周波数")
- terms_of_service: str = Field(title="エンジンの利用規約")
- update_infos: List[UpdateInfo] = Field(title="エンジンのアップデート情報")
- dependency_licenses: List[LicenseInfo] = Field(title="依存関係のライセンス情報")
- supported_features: SupportedFeatures = Field(title="エンジンが持つ機能")
diff --git a/spaces/AK-12/llama-gradio-chat/app.py b/spaces/AK-12/llama-gradio-chat/app.py
deleted file mode 100644
index 29a8654f7b734c9cb7c22342d878ef2cf4cc6f79..0000000000000000000000000000000000000000
--- a/spaces/AK-12/llama-gradio-chat/app.py
+++ /dev/null
@@ -1,19 +0,0 @@
-import gradio as gr
-import requests
-# def greet(name):
-# return "Hello " + name + "!!"
-# import requests
-
-# API_URL = "https://api-inference.huggingface.co/models/AK-12/llama-2-medical-fine-tune"
-# headers = {"Authorization": "Bearer "}
-
-def query(payload):
- response = requests.post("https://api-inference.huggingface.co/models/AK-12/llama-2-medical-fine-tune", headers={"Authorization": "Bearer "}, json=payload)
- return response.json()
-
-# output = query({
-# "inputs": "What is haller cells? ",
-# })
-
-iface = gr.Interface(fn=query, inputs="text", outputs="text")
-iface.launch()
\ No newline at end of file
diff --git a/spaces/Ababababababbababa/Ashaar/poetry_diacritizer/predict.py b/spaces/Ababababababbababa/Ashaar/poetry_diacritizer/predict.py
deleted file mode 100644
index 5787dbd8a67b8ce535663bd5d848dca7e460e554..0000000000000000000000000000000000000000
--- a/spaces/Ababababababbababa/Ashaar/poetry_diacritizer/predict.py
+++ /dev/null
@@ -1,167 +0,0 @@
-import os
-from typing import Dict
-
-from diacritization_evaluation import der, wer
-import torch
-from torch import nn
-from torch import optim
-from torch.cuda.amp import autocast
-from torch.utils.tensorboard.writer import SummaryWriter
-from tqdm.notebook import tqdm
-from tqdm import trange
-from diacritization_evaluation import util
-
-from .config_manager import ConfigManager
-from .dataset import load_iterators
-from .diacritizer import CBHGDiacritizer, Seq2SeqDiacritizer
-from .options import OptimizerType
-import gdown
-
-class Trainer:
- def run(self):
- raise NotImplementedError
-
-
-class GeneralTrainer(Trainer):
- def __init__(self, config_path: str, model_kind: str) -> None:
- self.config_path = config_path
- self.model_kind = model_kind
- self.config_manager = ConfigManager(
- config_path=config_path, model_kind=model_kind
- )
- self.config = self.config_manager.config
- self.losses = []
- self.lr = 0
- self.pad_idx = 0
- self.criterion = nn.CrossEntropyLoss(ignore_index=self.pad_idx)
- self.set_device()
-
- self.config_manager.create_remove_dirs()
- self.text_encoder = self.config_manager.text_encoder
- self.start_symbol_id = self.text_encoder.start_symbol_id
- self.summary_manager = SummaryWriter(log_dir=self.config_manager.log_dir)
-
- self.model = self.config_manager.get_model()
-
- self.optimizer = self.get_optimizer()
- self.model = self.model.to(self.device)
-
- self.load_model(model_path=self.config.get("train_resume_model_path"))
- self.load_diacritizer()
-
- self.initialize_model()
-
-
- def set_device(self):
- if self.config.get("device"):
- self.device = self.config["device"]
- else:
- self.device = "cuda" if torch.cuda.is_available() else "cpu"
-
- def load_diacritizer(self):
- if self.model_kind in ["cbhg", "baseline"]:
- self.diacritizer = CBHGDiacritizer(self.config_path, self.model_kind)
- elif self.model_kind in ["seq2seq", "tacotron_based"]:
- self.diacritizer = Seq2SeqDiacritizer(self.config_path, self.model_kind)
-
- def initialize_model(self):
- if self.global_step > 1:
- return
- if self.model_kind == "transformer":
- print("Initializing using xavier_uniform_")
- self.model.apply(initialize_weights)
-
-
- def load_model(self, model_path: str = None, load_optimizer: bool = True):
- with open(
- self.config_manager.base_dir / f"{self.model_kind}_network.txt", "w"
- ) as file:
- file.write(str(self.model))
-
- if model_path is None:
- last_model_path = self.config_manager.get_last_model_path()
- if last_model_path is None:
- self.global_step = 1
- return
- else:
- last_model_path = model_path
-
- print(f"loading from {last_model_path}")
- saved_model = torch.load(last_model_path, torch.device(self.config.get("device")))
- self.model.load_state_dict(saved_model["model_state_dict"])
- if load_optimizer:
- self.optimizer.load_state_dict(saved_model["optimizer_state_dict"])
- self.global_step = saved_model["global_step"] + 1
-
-class DiacritizationTester(GeneralTrainer):
- def __init__(self, config_path: str, model_kind: str, model_path: str) -> None:
- # if config_path == 'config/test.yml' or config_path == "Arabic_Diacritization/config/test.yml":
- # print("Exporting the pretrained models ... ")
- # url = 'https://drive.google.com/uc?id=12aYNY7cbsLNzhdPdC2K3u1sgrb1lpzwO'
- # gdown.cached_download(url,'model.zip', quiet=False, postprocess=gdown.extractall)
-
- self.config_path = config_path
- self.model_kind = model_kind
- self.config_manager = ConfigManager(
- config_path=config_path, model_kind=model_kind
- )
- self.config = self.config_manager.config
- # print(self.config)
- self.pad_idx = 0
- self.criterion = nn.CrossEntropyLoss(ignore_index=self.pad_idx)
- self.set_device()
-
- self.text_encoder = self.config_manager.text_encoder
- self.start_symbol_id = self.text_encoder.start_symbol_id
-
- self.model = self.config_manager.get_model()
-
- self.model = self.model.to(self.device)
- self.load_model(model_path=model_path, load_optimizer=False)
- self.load_diacritizer()
- self.diacritizer.set_model(self.model)
- self.initialize_model()
-
- def collate_fn(self, data):
- """
- Padding the input and output sequences
- """
-
- def merge(sequences):
- lengths = [len(seq) for seq in sequences]
- padded_seqs = torch.zeros(len(sequences), max(lengths)).long()
- for i, seq in enumerate(sequences):
- end = lengths[i]
- padded_seqs[i, :end] = seq[:end]
- return padded_seqs, lengths
-
- data.sort(key=lambda x: len(x[0]), reverse=True)
-
- # separate source and target sequences
- src_seqs, trg_seqs, original = zip(*data)
-
- # merge sequences (from tuple of 1D tensor to 2D tensor)
- src_seqs, src_lengths = merge(src_seqs)
- trg_seqs, trg_lengths = merge(trg_seqs)
-
- batch = {
- "original": original,
- "src": src_seqs,
- "target": trg_seqs,
- "lengths": torch.LongTensor(src_lengths), # src_lengths = trg_lengths
- }
- return batch
-
- def get_batch(self, sentence):
- data = self.text_encoder.clean(sentence)
- text, inputs, diacritics = util.extract_haraqat(data)
- inputs = torch.Tensor(self.text_encoder.input_to_sequence("".join(inputs)))
- diacritics = torch.Tensor(self.text_encoder.target_to_sequence(diacritics))
- batch = self.collate_fn([(inputs, diacritics, text)])
- return batch
-
- def infer(self, sentence):
- self.model.eval()
- batch = self.get_batch(sentence)
- predicted = self.diacritizer.diacritize_batch(batch)
- return predicted[0]
diff --git a/spaces/AbandonedMuse/UnlimitedMusicGen/audiocraft/data/audio_utils.py b/spaces/AbandonedMuse/UnlimitedMusicGen/audiocraft/data/audio_utils.py
deleted file mode 100644
index 7595435329587d7fe97afbff5f74664a808ea050..0000000000000000000000000000000000000000
--- a/spaces/AbandonedMuse/UnlimitedMusicGen/audiocraft/data/audio_utils.py
+++ /dev/null
@@ -1,293 +0,0 @@
-# Copyright (c) Meta Platforms, Inc. and affiliates.
-# All rights reserved.
-#
-# This source code is licensed under the license found in the
-# LICENSE file in the root directory of this source tree.
-
-import sys
-import typing as tp
-
-import julius
-import torch
-import torchaudio
-
-
-def convert_audio_channels(wav: torch.Tensor, channels: int = 2) -> torch.Tensor:
- """Convert audio to the given number of channels.
-
- Args:
- wav (torch.Tensor): Audio wave of shape [B, C, T].
- channels (int): Expected number of channels as output.
- Returns:
- torch.Tensor: Downmixed or unchanged audio wave [B, C, T].
- """
- *shape, src_channels, length = wav.shape
- if src_channels == channels:
- pass
- elif channels == 1:
- # Case 1:
- # The caller asked 1-channel audio, and the stream has multiple
- # channels, downmix all channels.
- wav = wav.mean(dim=-2, keepdim=True)
- elif src_channels == 1:
- # Case 2:
- # The caller asked for multiple channels, but the input file has
- # a single channel, replicate the audio over all channels.
- wav = wav.expand(*shape, channels, length)
- elif src_channels >= channels:
- # Case 3:
- # The caller asked for multiple channels, and the input file has
- # more channels than requested. In that case return the first channels.
- wav = wav[..., :channels, :]
- else:
- # Case 4: What is a reasonable choice here?
- raise ValueError('The audio file has less channels than requested but is not mono.')
- return wav
-
-
-def convert_audio(wav: torch.Tensor, from_rate: float,
- to_rate: float, to_channels: int) -> torch.Tensor:
- """Convert audio to new sample rate and number of audio channels.
- """
- wav = julius.resample_frac(wav, int(from_rate), int(to_rate))
- wav = convert_audio_channels(wav, to_channels)
- return wav
-
-
-def normalize_loudness(wav: torch.Tensor, sample_rate: int, loudness_headroom_db: float = 14,
- loudness_compressor: bool = False, energy_floor: float = 2e-3):
- """Normalize an input signal to a user loudness in dB LKFS.
- Audio loudness is defined according to the ITU-R BS.1770-4 recommendation.
-
- Args:
- wav (torch.Tensor): Input multichannel audio data.
- sample_rate (int): Sample rate.
- loudness_headroom_db (float): Target loudness of the output in dB LUFS.
- loudness_compressor (bool): Uses tanh for soft clipping.
- energy_floor (float): anything below that RMS level will not be rescaled.
- Returns:
- output (torch.Tensor): Loudness normalized output data.
- """
- energy = wav.pow(2).mean().sqrt().item()
- if energy < energy_floor:
- return wav
- transform = torchaudio.transforms.Loudness(sample_rate)
- input_loudness_db = transform(wav).item()
- # calculate the gain needed to scale to the desired loudness level
- delta_loudness = -loudness_headroom_db - input_loudness_db
- gain = 10.0 ** (delta_loudness / 20.0)
- output = gain * wav
- if loudness_compressor:
- output = torch.tanh(output)
- assert output.isfinite().all(), (input_loudness_db, wav.pow(2).mean().sqrt())
- return output
-
-
-def _clip_wav(wav: torch.Tensor, log_clipping: bool = False, stem_name: tp.Optional[str] = None) -> None:
- """Utility function to clip the audio with logging if specified."""
- max_scale = wav.abs().max()
- if log_clipping and max_scale > 1:
- clamp_prob = (wav.abs() > 1).float().mean().item()
- print(f"CLIPPING {stem_name or ''} happening with proba (a bit of clipping is okay):",
- clamp_prob, "maximum scale: ", max_scale.item(), file=sys.stderr)
- wav.clamp_(-1, 1)
-
-
-def normalize_audio(wav: torch.Tensor, normalize: bool = True,
- strategy: str = 'peak', peak_clip_headroom_db: float = 1,
- rms_headroom_db: float = 18, loudness_headroom_db: float = 14,
- loudness_compressor: bool = False, log_clipping: bool = False,
- sample_rate: tp.Optional[int] = None,
- stem_name: tp.Optional[str] = None) -> torch.Tensor:
- """Normalize the audio according to the prescribed strategy (see after).
-
- Args:
- wav (torch.Tensor): Audio data.
- normalize (bool): if `True` (default), normalizes according to the prescribed
- strategy (see after). If `False`, the strategy is only used in case clipping
- would happen.
- strategy (str): Can be either 'clip', 'peak', or 'rms'. Default is 'peak',
- i.e. audio is normalized by its largest value. RMS normalizes by root-mean-square
- with extra headroom to avoid clipping. 'clip' just clips.
- peak_clip_headroom_db (float): Headroom in dB when doing 'peak' or 'clip' strategy.
- rms_headroom_db (float): Headroom in dB when doing 'rms' strategy. This must be much larger
- than the `peak_clip` one to avoid further clipping.
- loudness_headroom_db (float): Target loudness for loudness normalization.
- loudness_compressor (bool): If True, uses tanh based soft clipping.
- log_clipping (bool): If True, basic logging on stderr when clipping still
- occurs despite strategy (only for 'rms').
- sample_rate (int): Sample rate for the audio data (required for loudness).
- stem_name (Optional[str]): Stem name for clipping logging.
- Returns:
- torch.Tensor: Normalized audio.
- """
- scale_peak = 10 ** (-peak_clip_headroom_db / 20)
- scale_rms = 10 ** (-rms_headroom_db / 20)
- if strategy == 'peak':
- rescaling = (scale_peak / wav.abs().max())
- if normalize or rescaling < 1:
- wav = wav * rescaling
- elif strategy == 'clip':
- wav = wav.clamp(-scale_peak, scale_peak)
- elif strategy == 'rms':
- mono = wav.mean(dim=0)
- rescaling = scale_rms / mono.pow(2).mean().sqrt()
- if normalize or rescaling < 1:
- wav = wav * rescaling
- _clip_wav(wav, log_clipping=log_clipping, stem_name=stem_name)
- elif strategy == 'loudness':
- assert sample_rate is not None, "Loudness normalization requires sample rate."
- wav = normalize_loudness(wav, sample_rate, loudness_headroom_db, loudness_compressor)
- _clip_wav(wav, log_clipping=log_clipping, stem_name=stem_name)
- else:
- assert wav.abs().max() < 1
- assert strategy == '' or strategy == 'none', f"Unexpected strategy: '{strategy}'"
- return wav
-
-
-def f32_pcm(wav: torch.Tensor) -> torch.Tensor:
- """Convert audio to float 32 bits PCM format.
- """
- if wav.dtype.is_floating_point:
- return wav
- else:
- assert wav.dtype == torch.int16
- return wav.float() / 2**15
-
-
-def i16_pcm(wav: torch.Tensor) -> torch.Tensor:
- """Convert audio to int 16 bits PCM format.
-
- ..Warning:: There exist many formula for doing this convertion. None are perfect
- due to the asymetry of the int16 range. One either have possible clipping, DC offset,
- or inconsistancies with f32_pcm. If the given wav doesn't have enough headroom,
- it is possible that `i16_pcm(f32_pcm)) != Identity`.
- """
- if wav.dtype.is_floating_point:
- assert wav.abs().max() <= 1
- candidate = (wav * 2 ** 15).round()
- if candidate.max() >= 2 ** 15: # clipping would occur
- candidate = (wav * (2 ** 15 - 1)).round()
- return candidate.short()
- else:
- assert wav.dtype == torch.int16
- return wav
-
-def apply_tafade(audio: torch.Tensor, sample_rate, duration=3.0, out=True, start=True, shape: str = "linear", stem_name: tp.Optional[str] = None) -> torch.Tensor:
- """
- Apply fade-in and/or fade-out effects to the audio tensor.
-
- Args:
- audio (torch.Tensor): The input audio tensor of shape (C, L).
- sample_rate (int): The sample rate of the audio.
- duration (float, optional): The duration of the fade in seconds. Defaults to 3.0.
- out (bool, optional): Determines whether to apply fade-in (False) or fade-out (True) effect. Defaults to True.
- start (bool, optional): Determines whether the fade is applied to the beginning (True) or end (False) of the audio. Defaults to True.
- shape (str, optional): The shape of the fade. Must be one of: "quarter_sine", "half_sine", "linear", "logarithmic", "exponential". Defaults to "linear".
-
- Returns:
- torch.Tensor: The audio tensor with the fade effect applied.
-
- """
- fade_samples = int(sample_rate * duration) # Number of samples for the fade duration
-
- # Create the fade transform
- fade_transform = torchaudio.transforms.Fade(fade_in_len=0, fade_out_len=0, fade_shape=shape)
-
- if out:
- fade_transform.fade_out_len = fade_samples
- else:
- fade_transform.fade_in_len = fade_samples
-
- # Select the portion of the audio to apply the fade
- if start:
- audio_fade_section = audio[:, :fade_samples]
- else:
- audio_fade_section = audio[:, -fade_samples:]
-
- # Apply the fade transform to the audio section
- audio_faded = fade_transform(audio)
-
- # Replace the selected portion of the audio with the faded section
- if start:
- audio_faded[:, :fade_samples] = audio_fade_section
- else:
- audio_faded[:, -fade_samples:] = audio_fade_section
-
- wav = normalize_loudness(audio_faded,sample_rate, loudness_headroom_db=18, loudness_compressor=True)
- _clip_wav(wav, log_clipping=False, stem_name=stem_name)
- return wav
-
-
-def apply_fade(audio: torch.Tensor, sample_rate, duration=3.0, out=True, start=True, curve_start:float=0.0, curve_end:float=1.0, current_device:str="cpu", stem_name: tp.Optional[str] = None) -> torch.Tensor:
- """
- Apply fade-in and/or fade-out effects to the audio tensor.
-
- Args:
- audio (torch.Tensor): The input audio tensor of shape (C, L).
- sample_rate (int): The sample rate of the audio.
- duration (float, optional): The duration of the fade in seconds. Defaults to 3.0.
- out (bool, optional): Determines whether to apply fade-in (False) or fade-out (True) effect. Defaults to True.
- start (bool, optional): Determines whether the fade is applied to the beginning (True) or end (False) of the audio. Defaults to True.
- curve_start (float, optional): The starting amplitude of the fade curve. Defaults to 0.0.
- curve_end (float, optional): The ending amplitude of the fade curve. Defaults to 1.0.
- current_device (str, optional): The device on which the fade curve tensor should be created. Defaults to "cpu".
-
- Returns:
- torch.Tensor: The audio tensor with the fade effect applied.
-
- """
- fade_samples = int(sample_rate * duration) # Number of samples for the fade duration
- fade_curve = torch.linspace(curve_start, curve_end, fade_samples, device=current_device) # Generate linear fade curve
-
- if out:
- fade_curve = fade_curve.flip(0) # Reverse the fade curve for fade out
-
- # Select the portion of the audio to apply the fade
- if start:
- audio_fade_section = audio[:, :fade_samples]
- else:
- audio_fade_section = audio[:, -fade_samples:]
-
- # Apply the fade curve to the audio section
- audio_faded = audio.clone()
- audio_faded[:, :fade_samples] *= fade_curve.unsqueeze(0)
- audio_faded[:, -fade_samples:] *= fade_curve.unsqueeze(0)
-
- # Replace the selected portion of the audio with the faded section
- if start:
- audio_faded[:, :fade_samples] = audio_fade_section
- else:
- audio_faded[:, -fade_samples:] = audio_fade_section
-
- wav = normalize_loudness(audio_faded,sample_rate, loudness_headroom_db=18, loudness_compressor=True)
- _clip_wav(wav, log_clipping=False, stem_name=stem_name)
- return wav
-
-def apply_splice_effect(waveform1, sample_rate1, waveform2, sample_rate2, overlap):
- # Convert sample rates to integers
- sample_rate1 = int(sample_rate1)
- sample_rate2 = int(sample_rate2)
-
- # Convert tensors to mono-channel if needed
- if waveform1.ndim > 2:
- waveform1 = waveform1.mean(dim=1)
- if waveform2.ndim > 2:
- waveform2 = waveform2.mean(dim=1)
-
- ## Convert tensors to numpy arrays
- #waveform1_np = waveform1.numpy()
- #waveform2_np = waveform2.numpy()
-
- # Apply splice effect using torchaudio.sox_effects.apply_effects_tensor
- effects = [
- ["splice", f"-q {waveform1},{overlap}"],
- ]
- output_waveform, output_sample_rate = torchaudio.sox_effects.apply_effects_tensor(
- torch.cat([waveform1.unsqueeze(0), waveform2.unsqueeze(0)], dim=2),
- sample_rate1,
- effects
- )
-
- return output_waveform.squeeze(0), output_sample_rate
-
diff --git a/spaces/Abhilashvj/planogram-compliance/utils/loggers/wandb/wandb_utils.py b/spaces/Abhilashvj/planogram-compliance/utils/loggers/wandb/wandb_utils.py
deleted file mode 100644
index 00de0cca3346a64443ccc00baed62edc47a0537a..0000000000000000000000000000000000000000
--- a/spaces/Abhilashvj/planogram-compliance/utils/loggers/wandb/wandb_utils.py
+++ /dev/null
@@ -1,788 +0,0 @@
-"""Utilities and tools for tracking runs with Weights & Biases."""
-
-import logging
-import os
-import sys
-from contextlib import contextmanager
-from pathlib import Path
-from typing import Dict
-
-import yaml
-from tqdm import tqdm
-
-FILE = Path(__file__).resolve()
-ROOT = FILE.parents[3] # YOLOv5 root directory
-if str(ROOT) not in sys.path:
- sys.path.append(str(ROOT)) # add ROOT to PATH
-
-from utils.dataloaders import LoadImagesAndLabels, img2label_paths
-from utils.general import LOGGER, check_dataset, check_file
-
-try:
- import wandb
-
- assert hasattr(wandb, "__version__") # verify package import not local dir
-except (ImportError, AssertionError):
- wandb = None
-
-RANK = int(os.getenv("RANK", -1))
-WANDB_ARTIFACT_PREFIX = "wandb-artifact://"
-
-
-def remove_prefix(from_string, prefix=WANDB_ARTIFACT_PREFIX):
- return from_string[len(prefix) :]
-
-
-def check_wandb_config_file(data_config_file):
- wandb_config = "_wandb.".join(
- data_config_file.rsplit(".", 1)
- ) # updated data.yaml path
- if Path(wandb_config).is_file():
- return wandb_config
- return data_config_file
-
-
-def check_wandb_dataset(data_file):
- is_trainset_wandb_artifact = False
- is_valset_wandb_artifact = False
- if isinstance(data_file, dict):
- # In that case another dataset manager has already processed it and we don't have to
- return data_file
- if check_file(data_file) and data_file.endswith(".yaml"):
- with open(data_file, errors="ignore") as f:
- data_dict = yaml.safe_load(f)
- is_trainset_wandb_artifact = isinstance(
- data_dict["train"], str
- ) and data_dict["train"].startswith(WANDB_ARTIFACT_PREFIX)
- is_valset_wandb_artifact = isinstance(
- data_dict["val"], str
- ) and data_dict["val"].startswith(WANDB_ARTIFACT_PREFIX)
- if is_trainset_wandb_artifact or is_valset_wandb_artifact:
- return data_dict
- else:
- return check_dataset(data_file)
-
-
-def get_run_info(run_path):
- run_path = Path(remove_prefix(run_path, WANDB_ARTIFACT_PREFIX))
- run_id = run_path.stem
- project = run_path.parent.stem
- entity = run_path.parent.parent.stem
- model_artifact_name = "run_" + run_id + "_model"
- return entity, project, run_id, model_artifact_name
-
-
-def check_wandb_resume(opt):
- process_wandb_config_ddp_mode(opt) if RANK not in [-1, 0] else None
- if isinstance(opt.resume, str):
- if opt.resume.startswith(WANDB_ARTIFACT_PREFIX):
- if RANK not in [-1, 0]: # For resuming DDP runs
- entity, project, run_id, model_artifact_name = get_run_info(
- opt.resume
- )
- api = wandb.Api()
- artifact = api.artifact(
- entity
- + "/"
- + project
- + "/"
- + model_artifact_name
- + ":latest"
- )
- modeldir = artifact.download()
- opt.weights = str(Path(modeldir) / "last.pt")
- return True
- return None
-
-
-def process_wandb_config_ddp_mode(opt):
- with open(check_file(opt.data), errors="ignore") as f:
- data_dict = yaml.safe_load(f) # data dict
- train_dir, val_dir = None, None
- if isinstance(data_dict["train"], str) and data_dict["train"].startswith(
- WANDB_ARTIFACT_PREFIX
- ):
- api = wandb.Api()
- train_artifact = api.artifact(
- remove_prefix(data_dict["train"]) + ":" + opt.artifact_alias
- )
- train_dir = train_artifact.download()
- train_path = Path(train_dir) / "data/images/"
- data_dict["train"] = str(train_path)
-
- if isinstance(data_dict["val"], str) and data_dict["val"].startswith(
- WANDB_ARTIFACT_PREFIX
- ):
- api = wandb.Api()
- val_artifact = api.artifact(
- remove_prefix(data_dict["val"]) + ":" + opt.artifact_alias
- )
- val_dir = val_artifact.download()
- val_path = Path(val_dir) / "data/images/"
- data_dict["val"] = str(val_path)
- if train_dir or val_dir:
- ddp_data_path = str(Path(val_dir) / "wandb_local_data.yaml")
- with open(ddp_data_path, "w") as f:
- yaml.safe_dump(data_dict, f)
- opt.data = ddp_data_path
-
-
-class WandbLogger:
- """Log training runs, datasets, models, and predictions to Weights & Biases.
-
- This logger sends information to W&B at wandb.ai. By default, this information
- includes hyperparameters, system configuration and metrics, model metrics,
- and basic data metrics and analyses.
-
- By providing additional command line arguments to train.py, datasets,
- models and predictions can also be logged.
-
- For more on how this logger is used, see the Weights & Biases documentation:
- https://docs.wandb.com/guides/integrations/yolov5
- """
-
- def __init__(self, opt, run_id=None, job_type="Training"):
- """
- - Initialize WandbLogger instance
- - Upload dataset if opt.upload_dataset is True
- - Setup training processes if job_type is 'Training'
-
- arguments:
- opt (namespace) -- Commandline arguments for this run
- run_id (str) -- Run ID of W&B run to be resumed
- job_type (str) -- To set the job_type for this run
-
- """
- # Temporary-fix
- if opt.upload_dataset:
- opt.upload_dataset = False
- # LOGGER.info("Uploading Dataset functionality is not being supported temporarily due to a bug.")
-
- # Pre-training routine --
- self.job_type = job_type
- self.wandb, self.wandb_run = wandb, None if not wandb else wandb.run
- self.val_artifact, self.train_artifact = None, None
- self.train_artifact_path, self.val_artifact_path = None, None
- self.result_artifact = None
- self.val_table, self.result_table = None, None
- self.bbox_media_panel_images = []
- self.val_table_path_map = None
- self.max_imgs_to_log = 16
- self.wandb_artifact_data_dict = None
- self.data_dict = None
- # It's more elegant to stick to 1 wandb.init call,
- # but useful config data is overwritten in the WandbLogger's wandb.init call
- if isinstance(opt.resume, str): # checks resume from artifact
- if opt.resume.startswith(WANDB_ARTIFACT_PREFIX):
- entity, project, run_id, model_artifact_name = get_run_info(
- opt.resume
- )
- model_artifact_name = (
- WANDB_ARTIFACT_PREFIX + model_artifact_name
- )
- assert wandb, "install wandb to resume wandb runs"
- # Resume wandb-artifact:// runs here| workaround for not overwriting wandb.config
- self.wandb_run = wandb.init(
- id=run_id,
- project=project,
- entity=entity,
- resume="allow",
- allow_val_change=True,
- )
- opt.resume = model_artifact_name
- elif self.wandb:
- self.wandb_run = (
- wandb.init(
- config=opt,
- resume="allow",
- project="YOLOv5"
- if opt.project == "runs/train"
- else Path(opt.project).stem,
- entity=opt.entity,
- name=opt.name if opt.name != "exp" else None,
- job_type=job_type,
- id=run_id,
- allow_val_change=True,
- )
- if not wandb.run
- else wandb.run
- )
- if self.wandb_run:
- if self.job_type == "Training":
- if opt.upload_dataset:
- if not opt.resume:
- self.wandb_artifact_data_dict = (
- self.check_and_upload_dataset(opt)
- )
-
- if isinstance(opt.data, dict):
- # This means another dataset manager has already processed the dataset info (e.g. ClearML)
- # and they will have stored the already processed dict in opt.data
- self.data_dict = opt.data
- elif opt.resume:
- # resume from artifact
- if isinstance(opt.resume, str) and opt.resume.startswith(
- WANDB_ARTIFACT_PREFIX
- ):
- self.data_dict = dict(self.wandb_run.config.data_dict)
- else: # local resume
- self.data_dict = check_wandb_dataset(opt.data)
- else:
- self.data_dict = check_wandb_dataset(opt.data)
- self.wandb_artifact_data_dict = (
- self.wandb_artifact_data_dict or self.data_dict
- )
-
- # write data_dict to config. useful for resuming from artifacts. Do this only when not resuming.
- self.wandb_run.config.update(
- {"data_dict": self.wandb_artifact_data_dict},
- allow_val_change=True,
- )
- self.setup_training(opt)
-
- if self.job_type == "Dataset Creation":
- self.wandb_run.config.update({"upload_dataset": True})
- self.data_dict = self.check_and_upload_dataset(opt)
-
- def check_and_upload_dataset(self, opt):
- """
- Check if the dataset format is compatible and upload it as W&B artifact
-
- arguments:
- opt (namespace)-- Commandline arguments for current run
-
- returns:
- Updated dataset info dictionary where local dataset paths are replaced by WAND_ARFACT_PREFIX links.
- """
- assert wandb, "Install wandb to upload dataset"
- config_path = self.log_dataset_artifact(
- opt.data,
- opt.single_cls,
- "YOLOv5"
- if opt.project == "runs/train"
- else Path(opt.project).stem,
- )
- with open(config_path, errors="ignore") as f:
- wandb_data_dict = yaml.safe_load(f)
- return wandb_data_dict
-
- def setup_training(self, opt):
- """
- Setup the necessary processes for training YOLO models:
- - Attempt to download model checkpoint and dataset artifacts if opt.resume stats with WANDB_ARTIFACT_PREFIX
- - Update data_dict, to contain info of previous run if resumed and the paths of dataset artifact if downloaded
- - Setup log_dict, initialize bbox_interval
-
- arguments:
- opt (namespace) -- commandline arguments for this run
-
- """
- self.log_dict, self.current_epoch = {}, 0
- self.bbox_interval = opt.bbox_interval
- if isinstance(opt.resume, str):
- modeldir, _ = self.download_model_artifact(opt)
- if modeldir:
- self.weights = Path(modeldir) / "last.pt"
- config = self.wandb_run.config
- (
- opt.weights,
- opt.save_period,
- opt.batch_size,
- opt.bbox_interval,
- opt.epochs,
- opt.hyp,
- opt.imgsz,
- ) = (
- str(self.weights),
- config.save_period,
- config.batch_size,
- config.bbox_interval,
- config.epochs,
- config.hyp,
- config.imgsz,
- )
- data_dict = self.data_dict
- if (
- self.val_artifact is None
- ): # If --upload_dataset is set, use the existing artifact, don't download
- (
- self.train_artifact_path,
- self.train_artifact,
- ) = self.download_dataset_artifact(
- data_dict.get("train"), opt.artifact_alias
- )
- (
- self.val_artifact_path,
- self.val_artifact,
- ) = self.download_dataset_artifact(
- data_dict.get("val"), opt.artifact_alias
- )
-
- if self.train_artifact_path is not None:
- train_path = Path(self.train_artifact_path) / "data/images/"
- data_dict["train"] = str(train_path)
- if self.val_artifact_path is not None:
- val_path = Path(self.val_artifact_path) / "data/images/"
- data_dict["val"] = str(val_path)
-
- if self.val_artifact is not None:
- self.result_artifact = wandb.Artifact(
- "run_" + wandb.run.id + "_progress", "evaluation"
- )
- columns = ["epoch", "id", "ground truth", "prediction"]
- columns.extend(self.data_dict["names"])
- self.result_table = wandb.Table(columns)
- self.val_table = self.val_artifact.get("val")
- if self.val_table_path_map is None:
- self.map_val_table_path()
- if opt.bbox_interval == -1:
- self.bbox_interval = opt.bbox_interval = (
- (opt.epochs // 10) if opt.epochs > 10 else 1
- )
- if opt.evolve or opt.noplots:
- self.bbox_interval = opt.bbox_interval = (
- opt.epochs + 1
- ) # disable bbox_interval
- train_from_artifact = (
- self.train_artifact_path is not None
- and self.val_artifact_path is not None
- )
- # Update the the data_dict to point to local artifacts dir
- if train_from_artifact:
- self.data_dict = data_dict
-
- def download_dataset_artifact(self, path, alias):
- """
- download the model checkpoint artifact if the path starts with WANDB_ARTIFACT_PREFIX
-
- arguments:
- path -- path of the dataset to be used for training
- alias (str)-- alias of the artifact to be download/used for training
-
- returns:
- (str, wandb.Artifact) -- path of the downladed dataset and it's corresponding artifact object if dataset
- is found otherwise returns (None, None)
- """
- if isinstance(path, str) and path.startswith(WANDB_ARTIFACT_PREFIX):
- artifact_path = Path(
- remove_prefix(path, WANDB_ARTIFACT_PREFIX) + ":" + alias
- )
- dataset_artifact = wandb.use_artifact(
- artifact_path.as_posix().replace("\\", "/")
- )
- assert (
- dataset_artifact is not None
- ), "'Error: W&B dataset artifact doesn't exist'"
- datadir = dataset_artifact.download()
- return datadir, dataset_artifact
- return None, None
-
- def download_model_artifact(self, opt):
- """
- download the model checkpoint artifact if the resume path starts with WANDB_ARTIFACT_PREFIX
-
- arguments:
- opt (namespace) -- Commandline arguments for this run
- """
- if opt.resume.startswith(WANDB_ARTIFACT_PREFIX):
- model_artifact = wandb.use_artifact(
- remove_prefix(opt.resume, WANDB_ARTIFACT_PREFIX) + ":latest"
- )
- assert (
- model_artifact is not None
- ), "Error: W&B model artifact doesn't exist"
- modeldir = model_artifact.download()
- # epochs_trained = model_artifact.metadata.get('epochs_trained')
- total_epochs = model_artifact.metadata.get("total_epochs")
- is_finished = total_epochs is None
- assert (
- not is_finished
- ), "training is finished, can only resume incomplete runs."
- return modeldir, model_artifact
- return None, None
-
- def log_model(self, path, opt, epoch, fitness_score, best_model=False):
- """
- Log the model checkpoint as W&B artifact
-
- arguments:
- path (Path) -- Path of directory containing the checkpoints
- opt (namespace) -- Command line arguments for this run
- epoch (int) -- Current epoch number
- fitness_score (float) -- fitness score for current epoch
- best_model (boolean) -- Boolean representing if the current checkpoint is the best yet.
- """
- model_artifact = wandb.Artifact(
- "run_" + wandb.run.id + "_model",
- type="model",
- metadata={
- "original_url": str(path),
- "epochs_trained": epoch + 1,
- "save period": opt.save_period,
- "project": opt.project,
- "total_epochs": opt.epochs,
- "fitness_score": fitness_score,
- },
- )
- model_artifact.add_file(str(path / "last.pt"), name="last.pt")
- wandb.log_artifact(
- model_artifact,
- aliases=[
- "latest",
- "last",
- "epoch " + str(self.current_epoch),
- "best" if best_model else "",
- ],
- )
- LOGGER.info(f"Saving model artifact on epoch {epoch + 1}")
-
- def log_dataset_artifact(
- self, data_file, single_cls, project, overwrite_config=False
- ):
- """
- Log the dataset as W&B artifact and return the new data file with W&B links
-
- arguments:
- data_file (str) -- the .yaml file with information about the dataset like - path, classes etc.
- single_class (boolean) -- train multi-class data as single-class
- project (str) -- project name. Used to construct the artifact path
- overwrite_config (boolean) -- overwrites the data.yaml file if set to true otherwise creates a new
- file with _wandb postfix. Eg -> data_wandb.yaml
-
- returns:
- the new .yaml file with artifact links. it can be used to start training directly from artifacts
- """
- upload_dataset = self.wandb_run.config.upload_dataset
- log_val_only = (
- isinstance(upload_dataset, str) and upload_dataset == "val"
- )
- self.data_dict = check_dataset(data_file) # parse and check
- data = dict(self.data_dict)
- nc, names = (
- (1, ["item"]) if single_cls else (int(data["nc"]), data["names"])
- )
- names = {k: v for k, v in enumerate(names)} # to index dictionary
-
- # log train set
- if not log_val_only:
- self.train_artifact = (
- self.create_dataset_table(
- LoadImagesAndLabels(
- data["train"], rect=True, batch_size=1
- ),
- names,
- name="train",
- )
- if data.get("train")
- else None
- )
- if data.get("train"):
- data["train"] = WANDB_ARTIFACT_PREFIX + str(
- Path(project) / "train"
- )
-
- self.val_artifact = (
- self.create_dataset_table(
- LoadImagesAndLabels(data["val"], rect=True, batch_size=1),
- names,
- name="val",
- )
- if data.get("val")
- else None
- )
- if data.get("val"):
- data["val"] = WANDB_ARTIFACT_PREFIX + str(Path(project) / "val")
-
- path = Path(data_file)
- # create a _wandb.yaml file with artifacts links if both train and test set are logged
- if not log_val_only:
- path = (
- path.stem if overwrite_config else path.stem + "_wandb"
- ) + ".yaml" # updated data.yaml path
- path = ROOT / "data" / path
- data.pop("download", None)
- data.pop("path", None)
- with open(path, "w") as f:
- yaml.safe_dump(data, f)
- LOGGER.info(f"Created dataset config file {path}")
-
- if (
- self.job_type == "Training"
- ): # builds correct artifact pipeline graph
- if not log_val_only:
- self.wandb_run.log_artifact(
- self.train_artifact
- ) # calling use_artifact downloads the dataset. NOT NEEDED!
- self.wandb_run.use_artifact(self.val_artifact)
- self.val_artifact.wait()
- self.val_table = self.val_artifact.get("val")
- self.map_val_table_path()
- else:
- self.wandb_run.log_artifact(self.train_artifact)
- self.wandb_run.log_artifact(self.val_artifact)
- return path
-
- def map_val_table_path(self):
- """
- Map the validation dataset Table like name of file -> it's id in the W&B Table.
- Useful for - referencing artifacts for evaluation.
- """
- self.val_table_path_map = {}
- LOGGER.info("Mapping dataset")
- for i, data in enumerate(tqdm(self.val_table.data)):
- self.val_table_path_map[data[3]] = data[0]
-
- def create_dataset_table(
- self,
- dataset: LoadImagesAndLabels,
- class_to_id: Dict[int, str],
- name: str = "dataset",
- ):
- """
- Create and return W&B artifact containing W&B Table of the dataset.
-
- arguments:
- dataset -- instance of LoadImagesAndLabels class used to iterate over the data to build Table
- class_to_id -- hash map that maps class ids to labels
- name -- name of the artifact
-
- returns:
- dataset artifact to be logged or used
- """
- # TODO: Explore multiprocessing to slpit this loop parallely| This is essential for speeding up the the logging
- artifact = wandb.Artifact(name=name, type="dataset")
- img_files = (
- tqdm([dataset.path])
- if isinstance(dataset.path, str) and Path(dataset.path).is_dir()
- else None
- )
- img_files = tqdm(dataset.im_files) if not img_files else img_files
- for img_file in img_files:
- if Path(img_file).is_dir():
- artifact.add_dir(img_file, name="data/images")
- labels_path = "labels".join(dataset.path.rsplit("images", 1))
- artifact.add_dir(labels_path, name="data/labels")
- else:
- artifact.add_file(
- img_file, name="data/images/" + Path(img_file).name
- )
- label_file = Path(img2label_paths([img_file])[0])
- artifact.add_file(
- str(label_file), name="data/labels/" + label_file.name
- ) if label_file.exists() else None
- table = wandb.Table(columns=["id", "train_image", "Classes", "name"])
- class_set = wandb.Classes(
- [{"id": id, "name": name} for id, name in class_to_id.items()]
- )
- for si, (img, labels, paths, shapes) in enumerate(tqdm(dataset)):
- box_data, img_classes = [], {}
- for cls, *xywh in labels[:, 1:].tolist():
- cls = int(cls)
- box_data.append(
- {
- "position": {
- "middle": [xywh[0], xywh[1]],
- "width": xywh[2],
- "height": xywh[3],
- },
- "class_id": cls,
- "box_caption": "%s" % (class_to_id[cls]),
- }
- )
- img_classes[cls] = class_to_id[cls]
- boxes = {
- "ground_truth": {
- "box_data": box_data,
- "class_labels": class_to_id,
- }
- } # inference-space
- table.add_data(
- si,
- wandb.Image(paths, classes=class_set, boxes=boxes),
- list(img_classes.values()),
- Path(paths).name,
- )
- artifact.add(table, name)
- return artifact
-
- def log_training_progress(self, predn, path, names):
- """
- Build evaluation Table. Uses reference from validation dataset table.
-
- arguments:
- predn (list): list of predictions in the native space in the format - [xmin, ymin, xmax, ymax, confidence, class]
- path (str): local path of the current evaluation image
- names (dict(int, str)): hash map that maps class ids to labels
- """
- class_set = wandb.Classes(
- [{"id": id, "name": name} for id, name in names.items()]
- )
- box_data = []
- avg_conf_per_class = [0] * len(self.data_dict["names"])
- pred_class_count = {}
- for *xyxy, conf, cls in predn.tolist():
- if conf >= 0.25:
- cls = int(cls)
- box_data.append(
- {
- "position": {
- "minX": xyxy[0],
- "minY": xyxy[1],
- "maxX": xyxy[2],
- "maxY": xyxy[3],
- },
- "class_id": cls,
- "box_caption": f"{names[cls]} {conf:.3f}",
- "scores": {"class_score": conf},
- "domain": "pixel",
- }
- )
- avg_conf_per_class[cls] += conf
-
- if cls in pred_class_count:
- pred_class_count[cls] += 1
- else:
- pred_class_count[cls] = 1
-
- for pred_class in pred_class_count.keys():
- avg_conf_per_class[pred_class] = (
- avg_conf_per_class[pred_class] / pred_class_count[pred_class]
- )
-
- boxes = {
- "predictions": {"box_data": box_data, "class_labels": names}
- } # inference-space
- id = self.val_table_path_map[Path(path).name]
- self.result_table.add_data(
- self.current_epoch,
- id,
- self.val_table.data[id][1],
- wandb.Image(
- self.val_table.data[id][1], boxes=boxes, classes=class_set
- ),
- *avg_conf_per_class,
- )
-
- def val_one_image(self, pred, predn, path, names, im):
- """
- Log validation data for one image. updates the result Table if validation dataset is uploaded and log bbox media panel
-
- arguments:
- pred (list): list of scaled predictions in the format - [xmin, ymin, xmax, ymax, confidence, class]
- predn (list): list of predictions in the native space - [xmin, ymin, xmax, ymax, confidence, class]
- path (str): local path of the current evaluation image
- """
- if (
- self.val_table and self.result_table
- ): # Log Table if Val dataset is uploaded as artifact
- self.log_training_progress(predn, path, names)
-
- if (
- len(self.bbox_media_panel_images) < self.max_imgs_to_log
- and self.current_epoch > 0
- ):
- if self.current_epoch % self.bbox_interval == 0:
- box_data = [
- {
- "position": {
- "minX": xyxy[0],
- "minY": xyxy[1],
- "maxX": xyxy[2],
- "maxY": xyxy[3],
- },
- "class_id": int(cls),
- "box_caption": f"{names[int(cls)]} {conf:.3f}",
- "scores": {"class_score": conf},
- "domain": "pixel",
- }
- for *xyxy, conf, cls in pred.tolist()
- ]
- boxes = {
- "predictions": {
- "box_data": box_data,
- "class_labels": names,
- }
- } # inference-space
- self.bbox_media_panel_images.append(
- wandb.Image(im, boxes=boxes, caption=path.name)
- )
-
- def log(self, log_dict):
- """
- save the metrics to the logging dictionary
-
- arguments:
- log_dict (Dict) -- metrics/media to be logged in current step
- """
- if self.wandb_run:
- for key, value in log_dict.items():
- self.log_dict[key] = value
-
- def end_epoch(self, best_result=False):
- """
- commit the log_dict, model artifacts and Tables to W&B and flush the log_dict.
-
- arguments:
- best_result (boolean): Boolean representing if the result of this evaluation is best or not
- """
- if self.wandb_run:
- with all_logging_disabled():
- if self.bbox_media_panel_images:
- self.log_dict[
- "BoundingBoxDebugger"
- ] = self.bbox_media_panel_images
- try:
- wandb.log(self.log_dict)
- except BaseException as e:
- LOGGER.info(
- f"An error occurred in wandb logger. The training will proceed without interruption. More info\n{e}"
- )
- self.wandb_run.finish()
- self.wandb_run = None
-
- self.log_dict = {}
- self.bbox_media_panel_images = []
- if self.result_artifact:
- self.result_artifact.add(self.result_table, "result")
- wandb.log_artifact(
- self.result_artifact,
- aliases=[
- "latest",
- "last",
- "epoch " + str(self.current_epoch),
- ("best" if best_result else ""),
- ],
- )
-
- wandb.log({"evaluation": self.result_table})
- columns = ["epoch", "id", "ground truth", "prediction"]
- columns.extend(self.data_dict["names"])
- self.result_table = wandb.Table(columns)
- self.result_artifact = wandb.Artifact(
- "run_" + wandb.run.id + "_progress", "evaluation"
- )
-
- def finish_run(self):
- """
- Log metrics if any and finish the current W&B run
- """
- if self.wandb_run:
- if self.log_dict:
- with all_logging_disabled():
- wandb.log(self.log_dict)
- wandb.run.finish()
-
-
-@contextmanager
-def all_logging_disabled(highest_level=logging.CRITICAL):
- """source - https://gist.github.com/simon-weber/7853144
- A context manager that will prevent any logging messages triggered during the body from being processed.
- :param highest_level: the maximum logging level in use.
- This would only need to be changed if a custom level greater than CRITICAL is defined.
- """
- previous_level = logging.root.manager.disable
- logging.disable(highest_level)
- try:
- yield
- finally:
- logging.disable(previous_level)
diff --git a/spaces/Adapter/CoAdapter/README.md b/spaces/Adapter/CoAdapter/README.md
deleted file mode 100644
index 3450e8a9e0e672e4612763730ae078a8b012e3a2..0000000000000000000000000000000000000000
--- a/spaces/Adapter/CoAdapter/README.md
+++ /dev/null
@@ -1,12 +0,0 @@
----
-license: openrail
-title: CoAdapter
-sdk: gradio
-sdk_version: 3.19.1
-emoji: 😻
-colorFrom: pink
-colorTo: blue
-pinned: false
-python_version: 3.8.16
-app_file: app.py
----
\ No newline at end of file
diff --git a/spaces/AgentVerse/agentVerse/agentverse/llms/utils/jsonrepair.py b/spaces/AgentVerse/agentVerse/agentverse/llms/utils/jsonrepair.py
deleted file mode 100644
index df8d6c708522c01a0a66dc594ecea4d1e5d1f3cc..0000000000000000000000000000000000000000
--- a/spaces/AgentVerse/agentVerse/agentverse/llms/utils/jsonrepair.py
+++ /dev/null
@@ -1,660 +0,0 @@
-# jsonrepair.py - Repair invalid JSON documents in Python
-#
-# Just https://github.com/josdejong/jsonrepair ported from TypeScript to Python.
-#
-# This port won't get updates, because the goal should be to generate this library instead.
-#
-# See: https://github.com/josdejong/jsonrepair/issues/84
-#
-
-import json
-import re
-from typing import Optional
-
-CONTROL_CHARACTERS = {"\b": "\\b", "\f": "\\f", "\n": "\\n", "\r": "\\r", "\t": "\\t"}
-
-ESCAPE_CHARACTERS = {
- '"': '"',
- "\\": "\\",
- "/": "/",
- "b": "\b",
- "f": "\f",
- "n": "\n",
- "r": "\r",
- "t": "\t"
- # note that \u is handled separately in parseString()
-}
-
-
-def remove_at_index(text: str, start: int, count: int) -> str:
- return text[0:start] + text[start + count :]
-
-
-def is_control_character(char: str) -> bool:
- return char in CONTROL_CHARACTERS
-
-
-def is_valid_string_character(char: str) -> bool:
- return 0x20 <= ord(char) <= 0x10FFFF
-
-
-def is_quote(char: str) -> bool:
- return is_single_quote(char) or is_double_quote(char)
-
-
-def is_single_quote(char: str) -> bool:
- """Test whether the given character is a single quote character.
- Also tests for special variants of single quotes.
- """
- return char in (
- "'", # U+0027
- "‘", # U+2018
- "’", # U+2019
- "`", # U+0060
- "´", # U+00B4
- )
-
-
-def is_double_quote(char: str) -> bool:
- return (
- is_ascii_double_quote(char)
- or is_double_quote_left(char)
- or is_double_quote_right(char)
- )
-
-
-def is_ascii_double_quote(char: str) -> bool:
- return char == '"' # U+0022
-
-
-def is_double_quote_left(char: str) -> bool:
- return char == "“" # U+201C
-
-
-def is_double_quote_right(char: str) -> bool:
- return char == "”" # U+201D
-
-
-def is_start_of_value(char: str) -> bool:
- regex_start_of_value = (
- r"^[[{\w-]$" # alpha, number, minus, or opening bracket or brace
- )
- return bool(re.search(regex_start_of_value, char)) or is_quote(char)
-
-
-def ends_with_comma_or_newline(text: str) -> bool:
- return bool(re.search(r"[,\n][ \t\r]*$", text))
-
-
-def is_whitespace(char: str) -> bool:
- return char.isspace()
-
-
-def is_special_whitespace(char: str) -> bool:
- """Check if the given character is a special whitespace character, some unicode variant"""
- return (
- char == "\u00A0" # non-breaking space
- or ord("\u2000") <= ord(char) <= ord("\u200A")
- or char == "\u202F"
- or char == "\u205F"
- or char == "\u3000"
- )
-
-
-def insert_before_last_whitespace(text: str, text_to_insert: str) -> str:
- index = len(text)
-
- if not is_whitespace(text[index - 1]):
- # no trailing whitespaces
- return text + text_to_insert
-
- while is_whitespace(text[index - 1]):
- index -= 1
-
- return text[:index] + text_to_insert + text[index:]
-
-
-def strip_last_occurrence(
- text: str, text_to_strip: str, strip_remaining: bool = False
-) -> str:
- index = text.rindex(text_to_strip)
- try:
- return text[:index] + ("" if strip_remaining else text[index + 1 :])
- except ValueError:
- return text
-
-
-def is_hex(char: str) -> bool:
- try:
- int(char, 16)
- return True
- except ValueError:
- return False
-
-
-def is_delimiter(char: str) -> bool:
- return char in ",:[]{}()\n'" or is_quote(char)
-
-
-def at_end_of_block_comment(text: str, i: int) -> bool:
- return text[i] == "*" and text[i + 1] == "/"
-
-
-class JsonRepairError(Exception):
- def __init__(self, message: str, position: int):
- super(JsonRepairError, self).__init__(message + f" at position {position}")
- self.position = position
-
-
-class JsonRepair:
- """Repairs invalid JSON, i.e. change JavaScript notation into JSON notation.
-
- Example:
-
- try:
- json = "{name: 'John'}"
- repaired = JsonRepair(json).repair()
- print(repaired)
- # '{"name": "John"}'
- except JsonRepairFailed as err:
- print(err)
-
- """
-
- def __init__(self, text: str):
- self.text = text
- self.i = 0 # current index in text
- self.output = "" # generated output
-
- def char(self, pos: int = 0) -> str:
- return self.text[self.i + pos]
-
- def inc(self, by: int = 1) -> None:
- self.i += by
-
- def dec(self, by: int = 1) -> None:
- self.i -= by
-
- def is_start_of_document(self, pos: int = 0) -> bool:
- return self.i + pos == 0
-
- def is_end_of_document(self, pos: int = 0) -> bool:
- return self.i + pos >= len(self.text)
-
- def repair(self) -> str:
- processed = self.parse_value()
- if not processed:
- raise self.unexpected_end()
-
- processed_comma = self.parse_character(",")
- if processed_comma:
- self.parse_whitespace_and_skip_comments()
-
- if (
- not self.is_end_of_document()
- and is_start_of_value(self.char())
- and ends_with_comma_or_newline(self.output)
- ):
- # start of a new value after end of the root level object: looks like
- # newline delimited JSON -> turn into a root level array
- if not processed_comma:
- # repair missing comma
- self.output = insert_before_last_whitespace(self.output, ",")
-
- self.parse_newline_delimited_json()
- elif processed_comma:
- # repair: remove trailing comma
- self.output = strip_last_occurrence(self.output, ",")
-
- if self.is_end_of_document():
- # reached the end of the document properly
- return self.output
-
- raise self.unexpected_character()
-
- def parse_value(self) -> bool:
- self.parse_whitespace_and_skip_comments()
- processed = (
- self.parse_object()
- or self.parse_array()
- or self.parse_string()
- or self.parse_number()
- or self.parse_keywords()
- or self.parse_unquoted_string()
- )
- self.parse_whitespace_and_skip_comments()
- return processed
-
- def parse_whitespace_and_skip_comments(self) -> bool:
- start = self.i
-
- changed = self.parse_whitespace()
- while True:
- changed = self.parse_comment()
- if changed:
- changed = self.parse_whitespace()
- if not changed:
- break
-
- return self.i > start
-
- def parse_whitespace(self) -> bool:
- whitespace = ""
-
- while not self.is_end_of_document():
- char = self.char()
-
- normal = is_whitespace(char)
- special = is_special_whitespace(char)
-
- if not normal and not special:
- break
-
- if special:
- whitespace += " " # repair special whitespace
- else:
- whitespace += char
-
- self.inc()
-
- if whitespace:
- self.output += whitespace
- return True
- return False
-
- def parse_comment(self) -> bool:
- # find a block comment '/* ... */'
- if not self.is_end_of_document() and not self.is_end_of_document(pos=+1):
- if self.char() == "/" and self.char(pos=+1) == "*":
- # repair block comment by skipping it
- while not self.is_end_of_document() and not at_end_of_block_comment(
- self.text, self.i
- ):
- self.inc()
- self.inc(by=2)
- return True
-
- # find a line comment '// ...'
- if self.char() == "/" and self.char(pos=+1) == "/":
- # repair line comment by skipping it
- while not self.is_end_of_document() and self.char() != "\n":
- self.inc()
- return True
-
- return False
-
- def parse_character(self, char: str) -> bool:
- if not self.is_end_of_document():
- if self.char() == char:
- self.output += char
- self.inc()
- return True
- return False
-
- def skip_character(self, char: str) -> bool:
- if not self.is_end_of_document() and self.char() == char:
- self.inc()
- return True
- return False
-
- def skip_escape_character(self) -> bool:
- return self.skip_character("\\")
-
- def parse_object(self) -> bool:
- """Parse an object like '{"key": "value"}'"""
- if not self.is_end_of_document() and self.char() == "{":
- self.output += "{"
- self.inc()
- self.parse_whitespace_and_skip_comments()
-
- initial = True
- while not self.is_end_of_document() and self.char() != "}":
- if not initial:
- processed_comma = self.parse_character(",")
- if not processed_comma:
- # repair missing comma
- self.output = insert_before_last_whitespace(self.output, ",")
- self.parse_whitespace_and_skip_comments()
- else:
- processed_comma = True
- initial = False
-
- processed_key = self.parse_string() or self.parse_unquoted_string()
- if not processed_key:
- if self.is_end_of_document() or self.char() in "{}[]":
- # repair trailing comma
- self.output = strip_last_occurrence(self.output, ",")
- break
- raise self.object_key_expected()
-
- self.parse_whitespace_and_skip_comments()
- processed_colon = self.parse_character(":")
- if not processed_colon:
- if is_start_of_value(self.char()):
- # repair missing colon
- self.output = insert_before_last_whitespace(self.output, ":")
- else:
- raise self.colon_expected()
- processed_value = self.parse_value()
- if not processed_value:
- if processed_colon:
- raise self.object_value_expected()
- raise self.colon_expected()
-
- if not self.is_end_of_document() and self.char() == "}":
- self.output += "}"
- self.inc()
- else:
- # repair missing end bracket
- self.output = insert_before_last_whitespace(self.output, "}")
-
- return True
-
- return False
-
- def parse_array(self) -> bool:
- """Parse an array like '["item1", "item2", ...]'"""
- if not self.is_end_of_document() and self.char() == "[":
- self.output += "["
- self.inc()
- self.parse_whitespace_and_skip_comments()
-
- initial = True
- while not self.is_end_of_document() and self.char() != "]":
- if not initial:
- processed_comma = self.parse_character(",")
- if not processed_comma:
- # repair missing comma
- self.output = insert_before_last_whitespace(self.output, ",")
- else:
- initial = False
-
- processed_value = self.parse_value()
- if not processed_value:
- # repair trailing comma
- self.output = strip_last_occurrence(self.output, ",")
- break
-
- if not self.is_end_of_document() and self.char() == "]":
- self.output += "]"
- self.inc()
- else:
- # repair missing closing array bracket
- self.output = insert_before_last_whitespace(self.output, "]")
-
- return True
-
- return False
-
- def parse_newline_delimited_json(self):
- """Parse and repair Newline Delimited JSON (NDJSON):
- multiple JSON objects separated by a newline character
- """
- # repair NDJSON
- initial = True
- processed_value = True
- while processed_value:
- if not initial:
- # parse optional comma, insert when missing
- processed_comma = self.parse_character(",")
- if not processed_comma:
- # repair: add missing comma
- self.output = insert_before_last_whitespace(self.output, ",")
- else:
- initial = False
-
- processed_value = self.parse_value()
-
- if not processed_value:
- # repair: remove trailing comma
- self.output = strip_last_occurrence(self.output, ",")
-
- # repair: wrap the output inside array brackets
- self.output = f"[\n{self.output}\n]"
-
- def parse_string(self) -> bool:
- """Parse a string enclosed by double quotes "...". Can contain escaped quotes
- Repair strings enclosed in single quotes or special quotes
- Repair an escaped string
- """
- if not self.is_end_of_document():
- skip_escape_chars = self.char() == "\\"
- if skip_escape_chars:
- # repair: remove the first escape character
- self.inc()
- skip_escape_chars = True
-
- if not self.is_end_of_document() and is_quote(self.char()):
- is_end_quote = (
- is_single_quote if is_single_quote(self.char()) else is_double_quote
- )
-
- if self.char() != '"':
- pass # TODO?: repair non-normalized quote
- self.output += '"'
- self.inc()
-
- while not self.is_end_of_document() and not is_end_quote(self.char()):
- if self.char() == "\\":
- char = self.char(pos=+1)
- escape_char = ESCAPE_CHARACTERS.get(char)
- if escape_char:
- self.output += self.text[self.i : self.i + 2]
- self.inc(by=2)
- elif char == "u":
- if (
- not self.is_end_of_document(pos=+5)
- and is_hex(self.char(pos=+2))
- and is_hex(self.char(pos=+3))
- and is_hex(self.char(pos=+4))
- and is_hex(self.char(pos=+5))
- ):
- self.output += self.text[self.i : self.i + 6]
- self.inc(by=6)
- else:
- raise self.invalid_unicode_character(self.i)
- else:
- # repair invalid escape character: remove it
- self.output += char
- self.inc(by=2)
- else:
- char = self.char()
-
- if char == '"' and self.char(pos=-1) != "\\":
- # repair unescaped double quote
- self.output += "\\" + char
- self.inc()
- elif is_control_character(char):
- # unescaped control character
- self.output += CONTROL_CHARACTERS[char]
- self.inc()
- else:
- if not is_valid_string_character(char):
- raise self.invalid_character(char)
- self.output += char
- self.inc()
-
- if skip_escape_chars:
- processed = self.skip_escape_character()
- if processed:
- pass # repair: skipped escape character (nothing to do)
-
- if not self.is_end_of_document() and is_quote(self.char()):
- if self.char() != '"':
- pass # TODO:? repair non-normalized quote
-
- self.output += '"'
- self.inc()
- else:
- # repair missing end quote
- self.output += '"'
-
- self.parse_concatenated_string()
-
- return True
-
- return False
-
- def parse_concatenated_string(self) -> bool:
- """Repair concatenated strings like \"hello\" + \"world\", change this into \"helloworld\" """
- processed = False
-
- self.parse_whitespace_and_skip_comments()
- while not self.is_end_of_document() and self.char() == "+":
- processed = True
- self.inc()
- self.parse_whitespace_and_skip_comments()
-
- # repair: remove the end quote of the first string
- self.output = strip_last_occurrence(self.output, '"', True)
- start = len(self.output)
- self.parse_string()
-
- # repair: remove the start quote of the second string
- self.output = remove_at_index(self.output, start, 1)
-
- return processed
-
- def parse_number(self) -> bool:
- """Parse a number like 2.4 or 2.4e6"""
- if not self.is_end_of_document():
- start = self.i
- if self.char() == "-":
- self.inc()
- err = self.expect_digit(start)
- if err:
- raise err
-
- if not self.is_end_of_document() and self.char() == "0":
- self.inc()
- elif not self.is_end_of_document() and self.char() in "123456789":
- self.inc()
- while not self.is_end_of_document() and self.char().isdigit():
- self.inc()
-
- if not self.is_end_of_document() and self.char() == ".":
- self.inc()
- err = self.expect_digit(start)
- if err:
- raise err
- while not self.is_end_of_document() and self.char().isdigit():
- self.inc()
-
- if not self.is_end_of_document() and self.char() in "eE":
- self.inc()
- if not self.is_end_of_document() and self.char() in "+-":
- self.inc()
- err = self.expect_digit(start)
- if err:
- raise err
- while not self.is_end_of_document() and self.char().isdigit():
- self.inc()
-
- if self.i > start:
- self.output += self.text[start : self.i]
- return True
-
- return False
-
- def parse_keywords(self) -> bool:
- """Parse keywords true, false, null
- Repair Python keywords True, False, None
- """
- return (
- self.parse_keyword("true", "true")
- or self.parse_keyword("false", "false")
- or self.parse_keyword("null", "null")
- # repair Python keywords True, False, None
- or self.parse_keyword("True", "true")
- or self.parse_keyword("False", "false")
- or self.parse_keyword("None", "null")
- )
-
- def parse_keyword(self, name: str, value: str) -> bool:
- if self.text[self.i : self.i + len(name)] == name:
- self.output += value
- self.inc(by=len(name))
- return True
-
- return False
-
- def parse_unquoted_string(self) -> bool:
- """Repair and unquoted string by adding quotes around it
- Repair a MongoDB function call like NumberLong("2")
- Repair a JSONP function call like callback({...});
- """
- # note that the symbol can end with whitespaces: we stop at the next delimiter
- start = self.i
- while not self.is_end_of_document() and not is_delimiter(self.char()):
- self.inc()
-
- if self.i > start:
- if not self.is_end_of_document() and self.char() == "(":
- # repair a MongoDB function call like NumberLong("2")
- # repair a JSONP function call like callback({...});
- self.inc()
-
- self.parse_value()
-
- if not self.is_end_of_document() and self.char() == ")":
- # repair: skip close bracket of function call
- self.inc()
- if not self.is_end_of_document() and self.char() == ";":
- # repair: skip semicolon after JSONP call
- self.inc()
-
- return True
-
- # else repair unquoted string
-
- # first, go back to prevent getting trailing whitespaces in the string
- while not self.is_start_of_document() and is_whitespace(self.char(pos=-1)):
- self.dec()
-
- symbol = self.text[start : self.i]
- self.output += json.dumps(symbol)
-
- return True
-
- return False
-
- def expect_digit(self, start: int) -> Optional[JsonRepairError]:
- if self.is_end_of_document() or not self.char().isdigit():
- num_so_far = self.text[start : self.i]
- return JsonRepairError(
- f"Invalid number '{num_so_far}', expecting a digit {self.got()}", 2
- )
-
- def invalid_character(self, char: str) -> JsonRepairError:
- return JsonRepairError("Invalid character " + json.dumps(char), self.i)
-
- def unexpected_character(self) -> JsonRepairError:
- return JsonRepairError(
- "Unexpected character " + json.dumps(self.text[self.i]), self.i
- )
-
- def unexpected_end(self) -> JsonRepairError:
- return JsonRepairError("Unexpected end of json string", len(self.text))
-
- def object_key_expected(self) -> JsonRepairError:
- return JsonRepairError("Object key expected", self.i)
-
- def object_value_expected(self) -> JsonRepairError:
- return JsonRepairError("Object value expected", self.i)
-
- def colon_expected(self) -> JsonRepairError:
- return JsonRepairError("Colon expected", self.i)
-
- def invalid_unicode_character(self, start: int) -> JsonRepairError:
- end = start + 2
- while re.match(r"\w", self.text[end]):
- end += 1
- chars = self.text[start:end]
- return JsonRepairError(f'Invalid unicode character "{chars}"', self.i)
-
- def got(self) -> str:
- return (
- f"but got '{self.char()}'"
- if not self.is_end_of_document()
- else "but reached end of input"
- )
diff --git a/spaces/AgentVerse/agentVerse/ui/src/phaser3-rex-plugins/plugins/bracketparser2-plugin.js b/spaces/AgentVerse/agentVerse/ui/src/phaser3-rex-plugins/plugins/bracketparser2-plugin.js
deleted file mode 100644
index c1583f3d3363a08282915f18d7135ffef10767dc..0000000000000000000000000000000000000000
--- a/spaces/AgentVerse/agentVerse/ui/src/phaser3-rex-plugins/plugins/bracketparser2-plugin.js
+++ /dev/null
@@ -1,18 +0,0 @@
-import BracketParser from './bracketparser2.js';
-
-class BracketParserPlugin extends Phaser.Plugins.BasePlugin {
- constructor(pluginManager) {
- super(pluginManager);
- }
-
- start() {
- var eventEmitter = this.game.events;
- eventEmitter.on('destroy', this.destroy, this);
- }
-
- add(config) {
- return new BracketParser(config);
- }
-}
-
-export default BracketParserPlugin;
\ No newline at end of file
diff --git a/spaces/AgentVerse/agentVerse/ui/src/phaser3-rex-plugins/templates/ui/dialog/methods/ModalMethods.js b/spaces/AgentVerse/agentVerse/ui/src/phaser3-rex-plugins/templates/ui/dialog/methods/ModalMethods.js
deleted file mode 100644
index 0d8a3787ad081b1ace3fc103d8c3778ebbae9bea..0000000000000000000000000000000000000000
--- a/spaces/AgentVerse/agentVerse/ui/src/phaser3-rex-plugins/templates/ui/dialog/methods/ModalMethods.js
+++ /dev/null
@@ -1,43 +0,0 @@
-import ModalMethods from '../../basesizer/ModalMethods.js';
-
-export default {
- onCreateModalBehavior(self) {
- self.on('button.click', function (button, groupName, index, pointer, event) {
- if (groupName !== 'actions') {
- return;
- }
-
- var closeEventData = {
- index: index,
- text: button.text,
- button: button,
- dialog: self
- }
-
-
- switch (self.buttonsType) {
- case 'radio':
- closeEventData.value = self.getChoicesSelectedButtonName();
- break;
- case 'checkboxes':
- closeEventData.value = self.getChoicesButtonStates();
- break;
- default:
- closeEventData.value = undefined;
- }
-
- self.modalClose(closeEventData);
- });
- },
-
- modal(config, onClose) {
- if (config && (config.defaultBehavior === false)) {
- this.onCreateModalBehavior = false;
- } else {
- delete this.onCreateModalBehavior;
- }
-
- ModalMethods.modal.call(this, config, onClose);
- return this;
- }
-}
\ No newline at end of file
diff --git a/spaces/AgentVerse/agentVerse/ui/src/phaser3-rex-plugins/templates/ui/sides/childbehaviors/index.js b/spaces/AgentVerse/agentVerse/ui/src/phaser3-rex-plugins/templates/ui/sides/childbehaviors/index.js
deleted file mode 100644
index c600b6f0b411d473d0d46d66fee672bc750894cd..0000000000000000000000000000000000000000
--- a/spaces/AgentVerse/agentVerse/ui/src/phaser3-rex-plugins/templates/ui/sides/childbehaviors/index.js
+++ /dev/null
@@ -1,14 +0,0 @@
-import Visible from './Visible.js';
-import Fade from './Fade.js';
-import Move from './Move.js';
-
-var methods = {};
-
-Object.assign(
- methods,
- Visible,
- Fade,
- Move
-);
-
-export default methods;
\ No newline at end of file
diff --git a/spaces/AlexWang/lama/bin/evaluate_predicts.py b/spaces/AlexWang/lama/bin/evaluate_predicts.py
deleted file mode 100644
index a4c182a50bc0cc3e2e03c713c2c0be2a804b04b8..0000000000000000000000000000000000000000
--- a/spaces/AlexWang/lama/bin/evaluate_predicts.py
+++ /dev/null
@@ -1,79 +0,0 @@
-#!/usr/bin/env python3
-
-import os
-
-import pandas as pd
-
-from saicinpainting.evaluation.data import PrecomputedInpaintingResultsDataset
-from saicinpainting.evaluation.evaluator import InpaintingEvaluator, lpips_fid100_f1
-from saicinpainting.evaluation.losses.base_loss import SegmentationAwareSSIM, \
- SegmentationClassStats, SSIMScore, LPIPSScore, FIDScore, SegmentationAwareLPIPS, SegmentationAwareFID
-from saicinpainting.evaluation.utils import load_yaml
-
-
-def main(args):
- config = load_yaml(args.config)
-
- dataset = PrecomputedInpaintingResultsDataset(args.datadir, args.predictdir, **config.dataset_kwargs)
-
- metrics = {
- 'ssim': SSIMScore(),
- 'lpips': LPIPSScore(),
- 'fid': FIDScore()
- }
- enable_segm = config.get('segmentation', dict(enable=False)).get('enable', False)
- if enable_segm:
- weights_path = os.path.expandvars(config.segmentation.weights_path)
- metrics.update(dict(
- segm_stats=SegmentationClassStats(weights_path=weights_path),
- segm_ssim=SegmentationAwareSSIM(weights_path=weights_path),
- segm_lpips=SegmentationAwareLPIPS(weights_path=weights_path),
- segm_fid=SegmentationAwareFID(weights_path=weights_path)
- ))
- evaluator = InpaintingEvaluator(dataset, scores=metrics,
- integral_title='lpips_fid100_f1', integral_func=lpips_fid100_f1,
- **config.evaluator_kwargs)
-
- os.makedirs(os.path.dirname(args.outpath), exist_ok=True)
-
- results = evaluator.evaluate()
-
- results = pd.DataFrame(results).stack(1).unstack(0)
- results.dropna(axis=1, how='all', inplace=True)
- results.to_csv(args.outpath, sep='\t', float_format='%.4f')
-
- if enable_segm:
- only_short_results = results[[c for c in results.columns if not c[0].startswith('segm_')]].dropna(axis=1, how='all')
- only_short_results.to_csv(args.outpath + '_short', sep='\t', float_format='%.4f')
-
- print(only_short_results)
-
- segm_metrics_results = results[['segm_ssim', 'segm_lpips', 'segm_fid']].dropna(axis=1, how='all').transpose().unstack(0).reorder_levels([1, 0], axis=1)
- segm_metrics_results.drop(['mean', 'std'], axis=0, inplace=True)
-
- segm_stats_results = results['segm_stats'].dropna(axis=1, how='all').transpose()
- segm_stats_results.index = pd.MultiIndex.from_tuples(n.split('/') for n in segm_stats_results.index)
- segm_stats_results = segm_stats_results.unstack(0).reorder_levels([1, 0], axis=1)
- segm_stats_results.sort_index(axis=1, inplace=True)
- segm_stats_results.dropna(axis=0, how='all', inplace=True)
-
- segm_results = pd.concat([segm_metrics_results, segm_stats_results], axis=1, sort=True)
- segm_results.sort_values(('mask_freq', 'total'), ascending=False, inplace=True)
-
- segm_results.to_csv(args.outpath + '_segm', sep='\t', float_format='%.4f')
- else:
- print(results)
-
-
-if __name__ == '__main__':
- import argparse
-
- aparser = argparse.ArgumentParser()
- aparser.add_argument('config', type=str, help='Path to evaluation config')
- aparser.add_argument('datadir', type=str,
- help='Path to folder with images and masks (output of gen_mask_dataset.py)')
- aparser.add_argument('predictdir', type=str,
- help='Path to folder with predicts (e.g. predict_hifill_baseline.py)')
- aparser.add_argument('outpath', type=str, help='Where to put results')
-
- main(aparser.parse_args())
diff --git a/spaces/Alfasign/diffusers-gallery/index.html b/spaces/Alfasign/diffusers-gallery/index.html
deleted file mode 100644
index fb4cde8f8a568181b566ecfdf3ba25ab89b0a352..0000000000000000000000000000000000000000
--- a/spaces/Alfasign/diffusers-gallery/index.html
+++ /dev/null
@@ -1,218 +0,0 @@
-
-
-
-
-
-
- Diffusers gallery
-
-
-
-
-
-
-
-
-
-
-
-
-
-
-
-
-
-
-
-
-
-
-
-
-
-
-
-
-
-
-
-
-
-
-
-
diff --git a/spaces/Amrrs/DragGan-Inversion/PTI/models/StyleCLIP/__init__.py b/spaces/Amrrs/DragGan-Inversion/PTI/models/StyleCLIP/__init__.py
deleted file mode 100644
index e69de29bb2d1d6434b8b29ae775ad8c2e48c5391..0000000000000000000000000000000000000000
diff --git a/spaces/Androidonnxfork/CivitAi-to-Diffusers/diffusers/docs/source/en/using-diffusers/depth2img.md b/spaces/Androidonnxfork/CivitAi-to-Diffusers/diffusers/docs/source/en/using-diffusers/depth2img.md
deleted file mode 100644
index a4141644b006d5ec7cb96f827365a597a7ba02c7..0000000000000000000000000000000000000000
--- a/spaces/Androidonnxfork/CivitAi-to-Diffusers/diffusers/docs/source/en/using-diffusers/depth2img.md
+++ /dev/null
@@ -1,56 +0,0 @@
-
-
-# Text-guided depth-to-image generation
-
-[[open-in-colab]]
-
-The [`StableDiffusionDepth2ImgPipeline`] lets you pass a text prompt and an initial image to condition the generation of new images. In addition, you can also pass a `depth_map` to preserve the image structure. If no `depth_map` is provided, the pipeline automatically predicts the depth via an integrated [depth-estimation model](https://github.com/isl-org/MiDaS).
-
-Start by creating an instance of the [`StableDiffusionDepth2ImgPipeline`]:
-
-```python
-import torch
-import requests
-from PIL import Image
-
-from diffusers import StableDiffusionDepth2ImgPipeline
-
-pipe = StableDiffusionDepth2ImgPipeline.from_pretrained(
- "stabilityai/stable-diffusion-2-depth",
- torch_dtype=torch.float16,
-).to("cuda")
-```
-
-Now pass your prompt to the pipeline. You can also pass a `negative_prompt` to prevent certain words from guiding how an image is generated:
-
-```python
-url = "http://images.cocodataset.org/val2017/000000039769.jpg"
-init_image = Image.open(requests.get(url, stream=True).raw)
-prompt = "two tigers"
-n_prompt = "bad, deformed, ugly, bad anatomy"
-image = pipe(prompt=prompt, image=init_image, negative_prompt=n_prompt, strength=0.7).images[0]
-image
-```
-
-| Input | Output |
-|---------------------------------------------------------------------------------|---------------------------------------------------------------------------------------------------------------------------------------|
-| | |
-
-Play around with the Spaces below and see if you notice a difference between generated images with and without a depth map!
-
-
diff --git a/spaces/Androidonnxfork/CivitAi-to-Diffusers/diffusers/src/diffusers/models/t5_film_transformer.py b/spaces/Androidonnxfork/CivitAi-to-Diffusers/diffusers/src/diffusers/models/t5_film_transformer.py
deleted file mode 100644
index 1c41e656a9dbe81edafd5a2958d49ff28e84fd01..0000000000000000000000000000000000000000
--- a/spaces/Androidonnxfork/CivitAi-to-Diffusers/diffusers/src/diffusers/models/t5_film_transformer.py
+++ /dev/null
@@ -1,321 +0,0 @@
-# Copyright 2023 The HuggingFace Team. All rights reserved.
-#
-# Licensed under the Apache License, Version 2.0 (the "License");
-# you may not use this file except in compliance with the License.
-# You may obtain a copy of the License at
-#
-# http://www.apache.org/licenses/LICENSE-2.0
-#
-# Unless required by applicable law or agreed to in writing, software
-# distributed under the License is distributed on an "AS IS" BASIS,
-# WITHOUT WARRANTIES OR CONDITIONS OF ANY KIND, either express or implied.
-# See the License for the specific language governing permissions and
-# limitations under the License.
-import math
-
-import torch
-from torch import nn
-
-from ..configuration_utils import ConfigMixin, register_to_config
-from .attention_processor import Attention
-from .embeddings import get_timestep_embedding
-from .modeling_utils import ModelMixin
-
-
-class T5FilmDecoder(ModelMixin, ConfigMixin):
- @register_to_config
- def __init__(
- self,
- input_dims: int = 128,
- targets_length: int = 256,
- max_decoder_noise_time: float = 2000.0,
- d_model: int = 768,
- num_layers: int = 12,
- num_heads: int = 12,
- d_kv: int = 64,
- d_ff: int = 2048,
- dropout_rate: float = 0.1,
- ):
- super().__init__()
-
- self.conditioning_emb = nn.Sequential(
- nn.Linear(d_model, d_model * 4, bias=False),
- nn.SiLU(),
- nn.Linear(d_model * 4, d_model * 4, bias=False),
- nn.SiLU(),
- )
-
- self.position_encoding = nn.Embedding(targets_length, d_model)
- self.position_encoding.weight.requires_grad = False
-
- self.continuous_inputs_projection = nn.Linear(input_dims, d_model, bias=False)
-
- self.dropout = nn.Dropout(p=dropout_rate)
-
- self.decoders = nn.ModuleList()
- for lyr_num in range(num_layers):
- # FiLM conditional T5 decoder
- lyr = DecoderLayer(d_model=d_model, d_kv=d_kv, num_heads=num_heads, d_ff=d_ff, dropout_rate=dropout_rate)
- self.decoders.append(lyr)
-
- self.decoder_norm = T5LayerNorm(d_model)
-
- self.post_dropout = nn.Dropout(p=dropout_rate)
- self.spec_out = nn.Linear(d_model, input_dims, bias=False)
-
- def encoder_decoder_mask(self, query_input, key_input):
- mask = torch.mul(query_input.unsqueeze(-1), key_input.unsqueeze(-2))
- return mask.unsqueeze(-3)
-
- def forward(self, encodings_and_masks, decoder_input_tokens, decoder_noise_time):
- batch, _, _ = decoder_input_tokens.shape
- assert decoder_noise_time.shape == (batch,)
-
- # decoder_noise_time is in [0, 1), so rescale to expected timing range.
- time_steps = get_timestep_embedding(
- decoder_noise_time * self.config.max_decoder_noise_time,
- embedding_dim=self.config.d_model,
- max_period=self.config.max_decoder_noise_time,
- ).to(dtype=self.dtype)
-
- conditioning_emb = self.conditioning_emb(time_steps).unsqueeze(1)
-
- assert conditioning_emb.shape == (batch, 1, self.config.d_model * 4)
-
- seq_length = decoder_input_tokens.shape[1]
-
- # If we want to use relative positions for audio context, we can just offset
- # this sequence by the length of encodings_and_masks.
- decoder_positions = torch.broadcast_to(
- torch.arange(seq_length, device=decoder_input_tokens.device),
- (batch, seq_length),
- )
-
- position_encodings = self.position_encoding(decoder_positions)
-
- inputs = self.continuous_inputs_projection(decoder_input_tokens)
- inputs += position_encodings
- y = self.dropout(inputs)
-
- # decoder: No padding present.
- decoder_mask = torch.ones(
- decoder_input_tokens.shape[:2], device=decoder_input_tokens.device, dtype=inputs.dtype
- )
-
- # Translate encoding masks to encoder-decoder masks.
- encodings_and_encdec_masks = [(x, self.encoder_decoder_mask(decoder_mask, y)) for x, y in encodings_and_masks]
-
- # cross attend style: concat encodings
- encoded = torch.cat([x[0] for x in encodings_and_encdec_masks], dim=1)
- encoder_decoder_mask = torch.cat([x[1] for x in encodings_and_encdec_masks], dim=-1)
-
- for lyr in self.decoders:
- y = lyr(
- y,
- conditioning_emb=conditioning_emb,
- encoder_hidden_states=encoded,
- encoder_attention_mask=encoder_decoder_mask,
- )[0]
-
- y = self.decoder_norm(y)
- y = self.post_dropout(y)
-
- spec_out = self.spec_out(y)
- return spec_out
-
-
-class DecoderLayer(nn.Module):
- def __init__(self, d_model, d_kv, num_heads, d_ff, dropout_rate, layer_norm_epsilon=1e-6):
- super().__init__()
- self.layer = nn.ModuleList()
-
- # cond self attention: layer 0
- self.layer.append(
- T5LayerSelfAttentionCond(d_model=d_model, d_kv=d_kv, num_heads=num_heads, dropout_rate=dropout_rate)
- )
-
- # cross attention: layer 1
- self.layer.append(
- T5LayerCrossAttention(
- d_model=d_model,
- d_kv=d_kv,
- num_heads=num_heads,
- dropout_rate=dropout_rate,
- layer_norm_epsilon=layer_norm_epsilon,
- )
- )
-
- # Film Cond MLP + dropout: last layer
- self.layer.append(
- T5LayerFFCond(d_model=d_model, d_ff=d_ff, dropout_rate=dropout_rate, layer_norm_epsilon=layer_norm_epsilon)
- )
-
- def forward(
- self,
- hidden_states,
- conditioning_emb=None,
- attention_mask=None,
- encoder_hidden_states=None,
- encoder_attention_mask=None,
- encoder_decoder_position_bias=None,
- ):
- hidden_states = self.layer[0](
- hidden_states,
- conditioning_emb=conditioning_emb,
- attention_mask=attention_mask,
- )
-
- if encoder_hidden_states is not None:
- encoder_extended_attention_mask = torch.where(encoder_attention_mask > 0, 0, -1e10).to(
- encoder_hidden_states.dtype
- )
-
- hidden_states = self.layer[1](
- hidden_states,
- key_value_states=encoder_hidden_states,
- attention_mask=encoder_extended_attention_mask,
- )
-
- # Apply Film Conditional Feed Forward layer
- hidden_states = self.layer[-1](hidden_states, conditioning_emb)
-
- return (hidden_states,)
-
-
-class T5LayerSelfAttentionCond(nn.Module):
- def __init__(self, d_model, d_kv, num_heads, dropout_rate):
- super().__init__()
- self.layer_norm = T5LayerNorm(d_model)
- self.FiLMLayer = T5FiLMLayer(in_features=d_model * 4, out_features=d_model)
- self.attention = Attention(query_dim=d_model, heads=num_heads, dim_head=d_kv, out_bias=False, scale_qk=False)
- self.dropout = nn.Dropout(dropout_rate)
-
- def forward(
- self,
- hidden_states,
- conditioning_emb=None,
- attention_mask=None,
- ):
- # pre_self_attention_layer_norm
- normed_hidden_states = self.layer_norm(hidden_states)
-
- if conditioning_emb is not None:
- normed_hidden_states = self.FiLMLayer(normed_hidden_states, conditioning_emb)
-
- # Self-attention block
- attention_output = self.attention(normed_hidden_states)
-
- hidden_states = hidden_states + self.dropout(attention_output)
-
- return hidden_states
-
-
-class T5LayerCrossAttention(nn.Module):
- def __init__(self, d_model, d_kv, num_heads, dropout_rate, layer_norm_epsilon):
- super().__init__()
- self.attention = Attention(query_dim=d_model, heads=num_heads, dim_head=d_kv, out_bias=False, scale_qk=False)
- self.layer_norm = T5LayerNorm(d_model, eps=layer_norm_epsilon)
- self.dropout = nn.Dropout(dropout_rate)
-
- def forward(
- self,
- hidden_states,
- key_value_states=None,
- attention_mask=None,
- ):
- normed_hidden_states = self.layer_norm(hidden_states)
- attention_output = self.attention(
- normed_hidden_states,
- encoder_hidden_states=key_value_states,
- attention_mask=attention_mask.squeeze(1),
- )
- layer_output = hidden_states + self.dropout(attention_output)
- return layer_output
-
-
-class T5LayerFFCond(nn.Module):
- def __init__(self, d_model, d_ff, dropout_rate, layer_norm_epsilon):
- super().__init__()
- self.DenseReluDense = T5DenseGatedActDense(d_model=d_model, d_ff=d_ff, dropout_rate=dropout_rate)
- self.film = T5FiLMLayer(in_features=d_model * 4, out_features=d_model)
- self.layer_norm = T5LayerNorm(d_model, eps=layer_norm_epsilon)
- self.dropout = nn.Dropout(dropout_rate)
-
- def forward(self, hidden_states, conditioning_emb=None):
- forwarded_states = self.layer_norm(hidden_states)
- if conditioning_emb is not None:
- forwarded_states = self.film(forwarded_states, conditioning_emb)
-
- forwarded_states = self.DenseReluDense(forwarded_states)
- hidden_states = hidden_states + self.dropout(forwarded_states)
- return hidden_states
-
-
-class T5DenseGatedActDense(nn.Module):
- def __init__(self, d_model, d_ff, dropout_rate):
- super().__init__()
- self.wi_0 = nn.Linear(d_model, d_ff, bias=False)
- self.wi_1 = nn.Linear(d_model, d_ff, bias=False)
- self.wo = nn.Linear(d_ff, d_model, bias=False)
- self.dropout = nn.Dropout(dropout_rate)
- self.act = NewGELUActivation()
-
- def forward(self, hidden_states):
- hidden_gelu = self.act(self.wi_0(hidden_states))
- hidden_linear = self.wi_1(hidden_states)
- hidden_states = hidden_gelu * hidden_linear
- hidden_states = self.dropout(hidden_states)
-
- hidden_states = self.wo(hidden_states)
- return hidden_states
-
-
-class T5LayerNorm(nn.Module):
- def __init__(self, hidden_size, eps=1e-6):
- """
- Construct a layernorm module in the T5 style. No bias and no subtraction of mean.
- """
- super().__init__()
- self.weight = nn.Parameter(torch.ones(hidden_size))
- self.variance_epsilon = eps
-
- def forward(self, hidden_states):
- # T5 uses a layer_norm which only scales and doesn't shift, which is also known as Root Mean
- # Square Layer Normalization https://arxiv.org/abs/1910.07467 thus variance is calculated
- # w/o mean and there is no bias. Additionally we want to make sure that the accumulation for
- # half-precision inputs is done in fp32
-
- variance = hidden_states.to(torch.float32).pow(2).mean(-1, keepdim=True)
- hidden_states = hidden_states * torch.rsqrt(variance + self.variance_epsilon)
-
- # convert into half-precision if necessary
- if self.weight.dtype in [torch.float16, torch.bfloat16]:
- hidden_states = hidden_states.to(self.weight.dtype)
-
- return self.weight * hidden_states
-
-
-class NewGELUActivation(nn.Module):
- """
- Implementation of the GELU activation function currently in Google BERT repo (identical to OpenAI GPT). Also see
- the Gaussian Error Linear Units paper: https://arxiv.org/abs/1606.08415
- """
-
- def forward(self, input: torch.Tensor) -> torch.Tensor:
- return 0.5 * input * (1.0 + torch.tanh(math.sqrt(2.0 / math.pi) * (input + 0.044715 * torch.pow(input, 3.0))))
-
-
-class T5FiLMLayer(nn.Module):
- """
- FiLM Layer
- """
-
- def __init__(self, in_features, out_features):
- super().__init__()
- self.scale_bias = nn.Linear(in_features, out_features * 2, bias=False)
-
- def forward(self, x, conditioning_emb):
- emb = self.scale_bias(conditioning_emb)
- scale, shift = torch.chunk(emb, 2, -1)
- x = x * (1 + scale) + shift
- return x
diff --git a/spaces/Androidonnxfork/CivitAi-to-Diffusers/diffusers/src/diffusers/pipelines/auto_pipeline.py b/spaces/Androidonnxfork/CivitAi-to-Diffusers/diffusers/src/diffusers/pipelines/auto_pipeline.py
deleted file mode 100644
index 66d306720aaf9d4b2aad84af3b4113447335782c..0000000000000000000000000000000000000000
--- a/spaces/Androidonnxfork/CivitAi-to-Diffusers/diffusers/src/diffusers/pipelines/auto_pipeline.py
+++ /dev/null
@@ -1,886 +0,0 @@
-# coding=utf-8
-# Copyright 2023 The HuggingFace Inc. team.
-#
-# Licensed under the Apache License, Version 2.0 (the "License");
-# you may not use this file except in compliance with the License.
-# You may obtain a copy of the License at
-#
-# http://www.apache.org/licenses/LICENSE-2.0
-#
-# Unless required by applicable law or agreed to in writing, software
-# distributed under the License is distributed on an "AS IS" BASIS,
-# WITHOUT WARRANTIES OR CONDITIONS OF ANY KIND, either express or implied.
-# See the License for the specific language governing permissions and
-# limitations under the License.
-
-import inspect
-from collections import OrderedDict
-
-from ..configuration_utils import ConfigMixin
-from .controlnet import (
- StableDiffusionControlNetImg2ImgPipeline,
- StableDiffusionControlNetInpaintPipeline,
- StableDiffusionControlNetPipeline,
- StableDiffusionXLControlNetPipeline,
-)
-from .deepfloyd_if import IFImg2ImgPipeline, IFInpaintingPipeline, IFPipeline
-from .kandinsky import (
- KandinskyCombinedPipeline,
- KandinskyImg2ImgCombinedPipeline,
- KandinskyImg2ImgPipeline,
- KandinskyInpaintCombinedPipeline,
- KandinskyInpaintPipeline,
- KandinskyPipeline,
-)
-from .kandinsky2_2 import (
- KandinskyV22CombinedPipeline,
- KandinskyV22Img2ImgCombinedPipeline,
- KandinskyV22Img2ImgPipeline,
- KandinskyV22InpaintCombinedPipeline,
- KandinskyV22InpaintPipeline,
- KandinskyV22Pipeline,
-)
-from .stable_diffusion import (
- StableDiffusionImg2ImgPipeline,
- StableDiffusionInpaintPipeline,
- StableDiffusionPipeline,
-)
-from .stable_diffusion_xl import (
- StableDiffusionXLImg2ImgPipeline,
- StableDiffusionXLInpaintPipeline,
- StableDiffusionXLPipeline,
-)
-
-
-AUTO_TEXT2IMAGE_PIPELINES_MAPPING = OrderedDict(
- [
- ("stable-diffusion", StableDiffusionPipeline),
- ("stable-diffusion-xl", StableDiffusionXLPipeline),
- ("if", IFPipeline),
- ("kandinsky", KandinskyCombinedPipeline),
- ("kandinsky22", KandinskyV22CombinedPipeline),
- ("stable-diffusion-controlnet", StableDiffusionControlNetPipeline),
- ("stable-diffusion-xl-controlnet", StableDiffusionXLControlNetPipeline),
- ]
-)
-
-AUTO_IMAGE2IMAGE_PIPELINES_MAPPING = OrderedDict(
- [
- ("stable-diffusion", StableDiffusionImg2ImgPipeline),
- ("stable-diffusion-xl", StableDiffusionXLImg2ImgPipeline),
- ("if", IFImg2ImgPipeline),
- ("kandinsky", KandinskyImg2ImgCombinedPipeline),
- ("kandinsky22", KandinskyV22Img2ImgCombinedPipeline),
- ("stable-diffusion-controlnet", StableDiffusionControlNetImg2ImgPipeline),
- ]
-)
-
-AUTO_INPAINT_PIPELINES_MAPPING = OrderedDict(
- [
- ("stable-diffusion", StableDiffusionInpaintPipeline),
- ("stable-diffusion-xl", StableDiffusionXLInpaintPipeline),
- ("if", IFInpaintingPipeline),
- ("kandinsky", KandinskyInpaintCombinedPipeline),
- ("kandinsky22", KandinskyV22InpaintCombinedPipeline),
- ("stable-diffusion-controlnet", StableDiffusionControlNetInpaintPipeline),
- ]
-)
-
-_AUTO_TEXT2IMAGE_DECODER_PIPELINES_MAPPING = OrderedDict(
- [
- ("kandinsky", KandinskyPipeline),
- ("kandinsky22", KandinskyV22Pipeline),
- ]
-)
-_AUTO_IMAGE2IMAGE_DECODER_PIPELINES_MAPPING = OrderedDict(
- [
- ("kandinsky", KandinskyImg2ImgPipeline),
- ("kandinsky22", KandinskyV22Img2ImgPipeline),
- ]
-)
-_AUTO_INPAINT_DECODER_PIPELINES_MAPPING = OrderedDict(
- [
- ("kandinsky", KandinskyInpaintPipeline),
- ("kandinsky22", KandinskyV22InpaintPipeline),
- ]
-)
-
-SUPPORTED_TASKS_MAPPINGS = [
- AUTO_TEXT2IMAGE_PIPELINES_MAPPING,
- AUTO_IMAGE2IMAGE_PIPELINES_MAPPING,
- AUTO_INPAINT_PIPELINES_MAPPING,
- _AUTO_TEXT2IMAGE_DECODER_PIPELINES_MAPPING,
- _AUTO_IMAGE2IMAGE_DECODER_PIPELINES_MAPPING,
- _AUTO_INPAINT_DECODER_PIPELINES_MAPPING,
-]
-
-
-def _get_connected_pipeline(pipeline_cls):
- # for now connected pipelines can only be loaded from decoder pipelines, such as kandinsky-community/kandinsky-2-2-decoder
- if pipeline_cls in _AUTO_TEXT2IMAGE_DECODER_PIPELINES_MAPPING.values():
- return _get_task_class(
- AUTO_TEXT2IMAGE_PIPELINES_MAPPING, pipeline_cls.__name__, throw_error_if_not_exist=False
- )
- if pipeline_cls in _AUTO_IMAGE2IMAGE_DECODER_PIPELINES_MAPPING.values():
- return _get_task_class(
- AUTO_IMAGE2IMAGE_PIPELINES_MAPPING, pipeline_cls.__name__, throw_error_if_not_exist=False
- )
- if pipeline_cls in _AUTO_INPAINT_DECODER_PIPELINES_MAPPING.values():
- return _get_task_class(AUTO_INPAINT_PIPELINES_MAPPING, pipeline_cls.__name__, throw_error_if_not_exist=False)
-
-
-def _get_task_class(mapping, pipeline_class_name, throw_error_if_not_exist: bool = True):
- def get_model(pipeline_class_name):
- for task_mapping in SUPPORTED_TASKS_MAPPINGS:
- for model_name, pipeline in task_mapping.items():
- if pipeline.__name__ == pipeline_class_name:
- return model_name
-
- model_name = get_model(pipeline_class_name)
-
- if model_name is not None:
- task_class = mapping.get(model_name, None)
- if task_class is not None:
- return task_class
-
- if throw_error_if_not_exist:
- raise ValueError(f"AutoPipeline can't find a pipeline linked to {pipeline_class_name} for {model_name}")
-
-
-def _get_signature_keys(obj):
- parameters = inspect.signature(obj.__init__).parameters
- required_parameters = {k: v for k, v in parameters.items() if v.default == inspect._empty}
- optional_parameters = set({k for k, v in parameters.items() if v.default != inspect._empty})
- expected_modules = set(required_parameters.keys()) - {"self"}
- return expected_modules, optional_parameters
-
-
-class AutoPipelineForText2Image(ConfigMixin):
- r"""
-
- AutoPipeline for text-to-image generation.
-
- [`AutoPipelineForText2Image`] is a generic pipeline class that will be instantiated as one of the text-to-image
- pipeline class in diffusers.
-
- The pipeline type (for example [`StableDiffusionPipeline`]) is automatically selected when created with the
- AutoPipelineForText2Image.from_pretrained(pretrained_model_name_or_path) or
- AutoPipelineForText2Image.from_pipe(pipeline) class methods .
-
- This class cannot be instantiated using __init__() (throws an error).
-
- Class attributes:
-
- - **config_name** (`str`) -- The configuration filename that stores the class and module names of all the
- diffusion pipeline's components.
-
- """
- config_name = "model_index.json"
-
- def __init__(self, *args, **kwargs):
- raise EnvironmentError(
- f"{self.__class__.__name__} is designed to be instantiated "
- f"using the `{self.__class__.__name__}.from_pretrained(pretrained_model_name_or_path)` or "
- f"`{self.__class__.__name__}.from_pipe(pipeline)` methods."
- )
-
- @classmethod
- def from_pretrained(cls, pretrained_model_or_path, **kwargs):
- r"""
- Instantiates a text-to-image Pytorch diffusion pipeline from pretrained pipeline weight.
-
- The from_pretrained() method takes care of returning the correct pipeline class instance by:
- 1. Detect the pipeline class of the pretrained_model_or_path based on the _class_name property of its
- config object
- 2. Find the text-to-image pipeline linked to the pipeline class using pattern matching on pipeline class
- name.
-
- If a `controlnet` argument is passed, it will instantiate a [`StableDiffusionControlNetPipeline`] object.
-
- The pipeline is set in evaluation mode (`model.eval()`) by default.
-
- If you get the error message below, you need to finetune the weights for your downstream task:
-
- ```
- Some weights of UNet2DConditionModel were not initialized from the model checkpoint at runwayml/stable-diffusion-v1-5 and are newly initialized because the shapes did not match:
- - conv_in.weight: found shape torch.Size([320, 4, 3, 3]) in the checkpoint and torch.Size([320, 9, 3, 3]) in the model instantiated
- You should probably TRAIN this model on a down-stream task to be able to use it for predictions and inference.
- ```
-
- Parameters:
- pretrained_model_name_or_path (`str` or `os.PathLike`, *optional*):
- Can be either:
-
- - A string, the *repo id* (for example `CompVis/ldm-text2im-large-256`) of a pretrained pipeline
- hosted on the Hub.
- - A path to a *directory* (for example `./my_pipeline_directory/`) containing pipeline weights
- saved using
- [`~DiffusionPipeline.save_pretrained`].
- torch_dtype (`str` or `torch.dtype`, *optional*):
- Override the default `torch.dtype` and load the model with another dtype. If "auto" is passed, the
- dtype is automatically derived from the model's weights.
- force_download (`bool`, *optional*, defaults to `False`):
- Whether or not to force the (re-)download of the model weights and configuration files, overriding the
- cached versions if they exist.
- cache_dir (`Union[str, os.PathLike]`, *optional*):
- Path to a directory where a downloaded pretrained model configuration is cached if the standard cache
- is not used.
- resume_download (`bool`, *optional*, defaults to `False`):
- Whether or not to resume downloading the model weights and configuration files. If set to `False`, any
- incompletely downloaded files are deleted.
- proxies (`Dict[str, str]`, *optional*):
- A dictionary of proxy servers to use by protocol or endpoint, for example, `{'http': 'foo.bar:3128',
- 'http://hostname': 'foo.bar:4012'}`. The proxies are used on each request.
- output_loading_info(`bool`, *optional*, defaults to `False`):
- Whether or not to also return a dictionary containing missing keys, unexpected keys and error messages.
- local_files_only (`bool`, *optional*, defaults to `False`):
- Whether to only load local model weights and configuration files or not. If set to `True`, the model
- won't be downloaded from the Hub.
- use_auth_token (`str` or *bool*, *optional*):
- The token to use as HTTP bearer authorization for remote files. If `True`, the token generated from
- `diffusers-cli login` (stored in `~/.huggingface`) is used.
- revision (`str`, *optional*, defaults to `"main"`):
- The specific model version to use. It can be a branch name, a tag name, a commit id, or any identifier
- allowed by Git.
- custom_revision (`str`, *optional*, defaults to `"main"`):
- The specific model version to use. It can be a branch name, a tag name, or a commit id similar to
- `revision` when loading a custom pipeline from the Hub. It can be a 🤗 Diffusers version when loading a
- custom pipeline from GitHub, otherwise it defaults to `"main"` when loading from the Hub.
- mirror (`str`, *optional*):
- Mirror source to resolve accessibility issues if you’re downloading a model in China. We do not
- guarantee the timeliness or safety of the source, and you should refer to the mirror site for more
- information.
- device_map (`str` or `Dict[str, Union[int, str, torch.device]]`, *optional*):
- A map that specifies where each submodule should go. It doesn’t need to be defined for each
- parameter/buffer name; once a given module name is inside, every submodule of it will be sent to the
- same device.
-
- Set `device_map="auto"` to have 🤗 Accelerate automatically compute the most optimized `device_map`. For
- more information about each option see [designing a device
- map](https://hf.co/docs/accelerate/main/en/usage_guides/big_modeling#designing-a-device-map).
- max_memory (`Dict`, *optional*):
- A dictionary device identifier for the maximum memory. Will default to the maximum memory available for
- each GPU and the available CPU RAM if unset.
- offload_folder (`str` or `os.PathLike`, *optional*):
- The path to offload weights if device_map contains the value `"disk"`.
- offload_state_dict (`bool`, *optional*):
- If `True`, temporarily offloads the CPU state dict to the hard drive to avoid running out of CPU RAM if
- the weight of the CPU state dict + the biggest shard of the checkpoint does not fit. Defaults to `True`
- when there is some disk offload.
- low_cpu_mem_usage (`bool`, *optional*, defaults to `True` if torch version >= 1.9.0 else `False`):
- Speed up model loading only loading the pretrained weights and not initializing the weights. This also
- tries to not use more than 1x model size in CPU memory (including peak memory) while loading the model.
- Only supported for PyTorch >= 1.9.0. If you are using an older version of PyTorch, setting this
- argument to `True` will raise an error.
- use_safetensors (`bool`, *optional*, defaults to `None`):
- If set to `None`, the safetensors weights are downloaded if they're available **and** if the
- safetensors library is installed. If set to `True`, the model is forcibly loaded from safetensors
- weights. If set to `False`, safetensors weights are not loaded.
- kwargs (remaining dictionary of keyword arguments, *optional*):
- Can be used to overwrite load and saveable variables (the pipeline components of the specific pipeline
- class). The overwritten components are passed directly to the pipelines `__init__` method. See example
- below for more information.
- variant (`str`, *optional*):
- Load weights from a specified variant filename such as `"fp16"` or `"ema"`. This is ignored when
- loading `from_flax`.
-
-
-
- To use private or [gated](https://huggingface.co/docs/hub/models-gated#gated-models) models, log-in with
- `huggingface-cli login`.
-
-
-
- Examples:
-
- ```py
- >>> from diffusers import AutoPipelineForTextToImage
-
- >>> pipeline = AutoPipelineForTextToImage.from_pretrained("runwayml/stable-diffusion-v1-5")
- >>> print(pipeline.__class__)
- ```
- """
- config = cls.load_config(pretrained_model_or_path)
- orig_class_name = config["_class_name"]
-
- if "controlnet" in kwargs:
- orig_class_name = config["_class_name"].replace("Pipeline", "ControlNetPipeline")
-
- text_2_image_cls = _get_task_class(AUTO_TEXT2IMAGE_PIPELINES_MAPPING, orig_class_name)
-
- return text_2_image_cls.from_pretrained(pretrained_model_or_path, **kwargs)
-
- @classmethod
- def from_pipe(cls, pipeline, **kwargs):
- r"""
- Instantiates a text-to-image Pytorch diffusion pipeline from another instantiated diffusion pipeline class.
-
- The from_pipe() method takes care of returning the correct pipeline class instance by finding the text-to-image
- pipeline linked to the pipeline class using pattern matching on pipeline class name.
-
- All the modules the pipeline contains will be used to initialize the new pipeline without reallocating
- additional memoery.
-
- The pipeline is set in evaluation mode (`model.eval()`) by default.
-
- Parameters:
- pipeline (`DiffusionPipeline`):
- an instantiated `DiffusionPipeline` object
-
- ```py
- >>> from diffusers import AutoPipelineForTextToImage, AutoPipelineForImageToImage
-
- >>> pipe_i2i = AutoPipelineForImage2Image.from_pretrained(
- ... "runwayml/stable-diffusion-v1-5", requires_safety_checker=False
- ... )
-
- >>> pipe_t2i = AutoPipelineForTextToImage.from_pipe(pipe_t2i)
- ```
- """
-
- original_config = dict(pipeline.config)
- original_cls_name = pipeline.__class__.__name__
-
- # derive the pipeline class to instantiate
- text_2_image_cls = _get_task_class(AUTO_TEXT2IMAGE_PIPELINES_MAPPING, original_cls_name)
-
- # define expected module and optional kwargs given the pipeline signature
- expected_modules, optional_kwargs = _get_signature_keys(text_2_image_cls)
-
- pretrained_model_name_or_path = original_config.pop("_name_or_path", None)
-
- # allow users pass modules in `kwargs` to override the original pipeline's components
- passed_class_obj = {k: kwargs.pop(k) for k in expected_modules if k in kwargs}
- original_class_obj = {
- k: pipeline.components[k]
- for k, v in pipeline.components.items()
- if k in expected_modules and k not in passed_class_obj
- }
-
- # allow users pass optional kwargs to override the original pipelines config attribute
- passed_pipe_kwargs = {k: kwargs.pop(k) for k in optional_kwargs if k in kwargs}
- original_pipe_kwargs = {
- k: original_config[k]
- for k, v in original_config.items()
- if k in optional_kwargs and k not in passed_pipe_kwargs
- }
-
- # config that were not expected by original pipeline is stored as private attribute
- # we will pass them as optional arguments if they can be accepted by the pipeline
- additional_pipe_kwargs = [
- k[1:]
- for k in original_config.keys()
- if k.startswith("_") and k[1:] in optional_kwargs and k[1:] not in passed_pipe_kwargs
- ]
- for k in additional_pipe_kwargs:
- original_pipe_kwargs[k] = original_config.pop(f"_{k}")
-
- text_2_image_kwargs = {**passed_class_obj, **original_class_obj, **passed_pipe_kwargs, **original_pipe_kwargs}
-
- # store unused config as private attribute
- unused_original_config = {
- f"{'' if k.startswith('_') else '_'}{k}": original_config[k]
- for k, v in original_config.items()
- if k not in text_2_image_kwargs
- }
-
- missing_modules = set(expected_modules) - set(pipeline._optional_components) - set(text_2_image_kwargs.keys())
-
- if len(missing_modules) > 0:
- raise ValueError(
- f"Pipeline {text_2_image_cls} expected {expected_modules}, but only {set(list(passed_class_obj.keys()) + list(original_class_obj.keys()))} were passed"
- )
-
- model = text_2_image_cls(**text_2_image_kwargs)
- model.register_to_config(_name_or_path=pretrained_model_name_or_path)
- model.register_to_config(**unused_original_config)
-
- return model
-
-
-class AutoPipelineForImage2Image(ConfigMixin):
- r"""
-
- AutoPipeline for image-to-image generation.
-
- [`AutoPipelineForImage2Image`] is a generic pipeline class that will be instantiated as one of the image-to-image
- pipeline classes in diffusers.
-
- The pipeline type (for example [`StableDiffusionImg2ImgPipeline`]) is automatically selected when created with the
- `AutoPipelineForImage2Image.from_pretrained(pretrained_model_name_or_path)` or
- `AutoPipelineForImage2Image.from_pipe(pipeline)` class methods.
-
- This class cannot be instantiated using __init__() (throws an error).
-
- Class attributes:
-
- - **config_name** (`str`) -- The configuration filename that stores the class and module names of all the
- diffusion pipeline's components.
-
- """
- config_name = "model_index.json"
-
- def __init__(self, *args, **kwargs):
- raise EnvironmentError(
- f"{self.__class__.__name__} is designed to be instantiated "
- f"using the `{self.__class__.__name__}.from_pretrained(pretrained_model_name_or_path)` or "
- f"`{self.__class__.__name__}.from_pipe(pipeline)` methods."
- )
-
- @classmethod
- def from_pretrained(cls, pretrained_model_or_path, **kwargs):
- r"""
- Instantiates a image-to-image Pytorch diffusion pipeline from pretrained pipeline weight.
-
- The from_pretrained() method takes care of returning the correct pipeline class instance by:
- 1. Detect the pipeline class of the pretrained_model_or_path based on the _class_name property of its
- config object
- 2. Find the image-to-image pipeline linked to the pipeline class using pattern matching on pipeline class
- name.
-
- If a `controlnet` argument is passed, it will instantiate a StableDiffusionControlNetImg2ImgPipeline object.
-
- The pipeline is set in evaluation mode (`model.eval()`) by default.
-
- If you get the error message below, you need to finetune the weights for your downstream task:
-
- ```
- Some weights of UNet2DConditionModel were not initialized from the model checkpoint at runwayml/stable-diffusion-v1-5 and are newly initialized because the shapes did not match:
- - conv_in.weight: found shape torch.Size([320, 4, 3, 3]) in the checkpoint and torch.Size([320, 9, 3, 3]) in the model instantiated
- You should probably TRAIN this model on a down-stream task to be able to use it for predictions and inference.
- ```
-
- Parameters:
- pretrained_model_name_or_path (`str` or `os.PathLike`, *optional*):
- Can be either:
-
- - A string, the *repo id* (for example `CompVis/ldm-text2im-large-256`) of a pretrained pipeline
- hosted on the Hub.
- - A path to a *directory* (for example `./my_pipeline_directory/`) containing pipeline weights
- saved using
- [`~DiffusionPipeline.save_pretrained`].
- torch_dtype (`str` or `torch.dtype`, *optional*):
- Override the default `torch.dtype` and load the model with another dtype. If "auto" is passed, the
- dtype is automatically derived from the model's weights.
- force_download (`bool`, *optional*, defaults to `False`):
- Whether or not to force the (re-)download of the model weights and configuration files, overriding the
- cached versions if they exist.
- cache_dir (`Union[str, os.PathLike]`, *optional*):
- Path to a directory where a downloaded pretrained model configuration is cached if the standard cache
- is not used.
- resume_download (`bool`, *optional*, defaults to `False`):
- Whether or not to resume downloading the model weights and configuration files. If set to `False`, any
- incompletely downloaded files are deleted.
- proxies (`Dict[str, str]`, *optional*):
- A dictionary of proxy servers to use by protocol or endpoint, for example, `{'http': 'foo.bar:3128',
- 'http://hostname': 'foo.bar:4012'}`. The proxies are used on each request.
- output_loading_info(`bool`, *optional*, defaults to `False`):
- Whether or not to also return a dictionary containing missing keys, unexpected keys and error messages.
- local_files_only (`bool`, *optional*, defaults to `False`):
- Whether to only load local model weights and configuration files or not. If set to `True`, the model
- won't be downloaded from the Hub.
- use_auth_token (`str` or *bool*, *optional*):
- The token to use as HTTP bearer authorization for remote files. If `True`, the token generated from
- `diffusers-cli login` (stored in `~/.huggingface`) is used.
- revision (`str`, *optional*, defaults to `"main"`):
- The specific model version to use. It can be a branch name, a tag name, a commit id, or any identifier
- allowed by Git.
- custom_revision (`str`, *optional*, defaults to `"main"`):
- The specific model version to use. It can be a branch name, a tag name, or a commit id similar to
- `revision` when loading a custom pipeline from the Hub. It can be a 🤗 Diffusers version when loading a
- custom pipeline from GitHub, otherwise it defaults to `"main"` when loading from the Hub.
- mirror (`str`, *optional*):
- Mirror source to resolve accessibility issues if you’re downloading a model in China. We do not
- guarantee the timeliness or safety of the source, and you should refer to the mirror site for more
- information.
- device_map (`str` or `Dict[str, Union[int, str, torch.device]]`, *optional*):
- A map that specifies where each submodule should go. It doesn’t need to be defined for each
- parameter/buffer name; once a given module name is inside, every submodule of it will be sent to the
- same device.
-
- Set `device_map="auto"` to have 🤗 Accelerate automatically compute the most optimized `device_map`. For
- more information about each option see [designing a device
- map](https://hf.co/docs/accelerate/main/en/usage_guides/big_modeling#designing-a-device-map).
- max_memory (`Dict`, *optional*):
- A dictionary device identifier for the maximum memory. Will default to the maximum memory available for
- each GPU and the available CPU RAM if unset.
- offload_folder (`str` or `os.PathLike`, *optional*):
- The path to offload weights if device_map contains the value `"disk"`.
- offload_state_dict (`bool`, *optional*):
- If `True`, temporarily offloads the CPU state dict to the hard drive to avoid running out of CPU RAM if
- the weight of the CPU state dict + the biggest shard of the checkpoint does not fit. Defaults to `True`
- when there is some disk offload.
- low_cpu_mem_usage (`bool`, *optional*, defaults to `True` if torch version >= 1.9.0 else `False`):
- Speed up model loading only loading the pretrained weights and not initializing the weights. This also
- tries to not use more than 1x model size in CPU memory (including peak memory) while loading the model.
- Only supported for PyTorch >= 1.9.0. If you are using an older version of PyTorch, setting this
- argument to `True` will raise an error.
- use_safetensors (`bool`, *optional*, defaults to `None`):
- If set to `None`, the safetensors weights are downloaded if they're available **and** if the
- safetensors library is installed. If set to `True`, the model is forcibly loaded from safetensors
- weights. If set to `False`, safetensors weights are not loaded.
- kwargs (remaining dictionary of keyword arguments, *optional*):
- Can be used to overwrite load and saveable variables (the pipeline components of the specific pipeline
- class). The overwritten components are passed directly to the pipelines `__init__` method. See example
- below for more information.
- variant (`str`, *optional*):
- Load weights from a specified variant filename such as `"fp16"` or `"ema"`. This is ignored when
- loading `from_flax`.
-
-
-
- To use private or [gated](https://huggingface.co/docs/hub/models-gated#gated-models) models, log-in with
- `huggingface-cli login`.
-
-
-
- Examples:
-
- ```py
- >>> from diffusers import AutoPipelineForTextToImage
-
- >>> pipeline = AutoPipelineForImageToImage.from_pretrained("runwayml/stable-diffusion-v1-5")
- >>> print(pipeline.__class__)
- ```
- """
- config = cls.load_config(pretrained_model_or_path)
- orig_class_name = config["_class_name"]
-
- if "controlnet" in kwargs:
- orig_class_name = config["_class_name"].replace("Pipeline", "ControlNetPipeline")
-
- image_2_image_cls = _get_task_class(AUTO_IMAGE2IMAGE_PIPELINES_MAPPING, orig_class_name)
-
- return image_2_image_cls.from_pretrained(pretrained_model_or_path, **kwargs)
-
- @classmethod
- def from_pipe(cls, pipeline, **kwargs):
- r"""
- Instantiates a image-to-image Pytorch diffusion pipeline from another instantiated diffusion pipeline class.
-
- The from_pipe() method takes care of returning the correct pipeline class instance by finding the
- image-to-image pipeline linked to the pipeline class using pattern matching on pipeline class name.
-
- All the modules the pipeline contains will be used to initialize the new pipeline without reallocating
- additional memoery.
-
- The pipeline is set in evaluation mode (`model.eval()`) by default.
-
- Parameters:
- pipeline (`DiffusionPipeline`):
- an instantiated `DiffusionPipeline` object
-
- Examples:
-
- ```py
- >>> from diffusers import AutoPipelineForTextToImage, AutoPipelineForImageToImage
-
- >>> pipe_t2i = AutoPipelineForText2Image.from_pretrained(
- ... "runwayml/stable-diffusion-v1-5", requires_safety_checker=False
- ... )
-
- >>> pipe_i2i = AutoPipelineForImageToImage.from_pipe(pipe_t2i)
- ```
- """
-
- original_config = dict(pipeline.config)
- original_cls_name = pipeline.__class__.__name__
-
- # derive the pipeline class to instantiate
- image_2_image_cls = _get_task_class(AUTO_IMAGE2IMAGE_PIPELINES_MAPPING, original_cls_name)
-
- # define expected module and optional kwargs given the pipeline signature
- expected_modules, optional_kwargs = _get_signature_keys(image_2_image_cls)
-
- pretrained_model_name_or_path = original_config.pop("_name_or_path", None)
-
- # allow users pass modules in `kwargs` to override the original pipeline's components
- passed_class_obj = {k: kwargs.pop(k) for k in expected_modules if k in kwargs}
- original_class_obj = {
- k: pipeline.components[k]
- for k, v in pipeline.components.items()
- if k in expected_modules and k not in passed_class_obj
- }
-
- # allow users pass optional kwargs to override the original pipelines config attribute
- passed_pipe_kwargs = {k: kwargs.pop(k) for k in optional_kwargs if k in kwargs}
- original_pipe_kwargs = {
- k: original_config[k]
- for k, v in original_config.items()
- if k in optional_kwargs and k not in passed_pipe_kwargs
- }
-
- # config attribute that were not expected by original pipeline is stored as its private attribute
- # we will pass them as optional arguments if they can be accepted by the pipeline
- additional_pipe_kwargs = [
- k[1:]
- for k in original_config.keys()
- if k.startswith("_") and k[1:] in optional_kwargs and k[1:] not in passed_pipe_kwargs
- ]
- for k in additional_pipe_kwargs:
- original_pipe_kwargs[k] = original_config.pop(f"_{k}")
-
- image_2_image_kwargs = {**passed_class_obj, **original_class_obj, **passed_pipe_kwargs, **original_pipe_kwargs}
-
- # store unused config as private attribute
- unused_original_config = {
- f"{'' if k.startswith('_') else '_'}{k}": original_config[k]
- for k, v in original_config.items()
- if k not in image_2_image_kwargs
- }
-
- missing_modules = set(expected_modules) - set(pipeline._optional_components) - set(image_2_image_kwargs.keys())
-
- if len(missing_modules) > 0:
- raise ValueError(
- f"Pipeline {image_2_image_cls} expected {expected_modules}, but only {set(list(passed_class_obj.keys()) + list(original_class_obj.keys()))} were passed"
- )
-
- model = image_2_image_cls(**image_2_image_kwargs)
- model.register_to_config(_name_or_path=pretrained_model_name_or_path)
- model.register_to_config(**unused_original_config)
-
- return model
-
-
-class AutoPipelineForInpainting(ConfigMixin):
- r"""
-
- AutoPipeline for inpainting generation.
-
- [`AutoPipelineForInpainting`] is a generic pipeline class that will be instantiated as one of the inpainting
- pipeline class in diffusers.
-
- The pipeline type (for example [`IFInpaintingPipeline`]) is automatically selected when created with the
- AutoPipelineForInpainting.from_pretrained(pretrained_model_name_or_path) or
- AutoPipelineForInpainting.from_pipe(pipeline) class methods .
-
- This class cannot be instantiated using __init__() (throws an error).
-
- Class attributes:
-
- - **config_name** (`str`) -- The configuration filename that stores the class and module names of all the
- diffusion pipeline's components.
-
- """
- config_name = "model_index.json"
-
- def __init__(self, *args, **kwargs):
- raise EnvironmentError(
- f"{self.__class__.__name__} is designed to be instantiated "
- f"using the `{self.__class__.__name__}.from_pretrained(pretrained_model_name_or_path)` or "
- f"`{self.__class__.__name__}.from_pipe(pipeline)` methods."
- )
-
- @classmethod
- def from_pretrained(cls, pretrained_model_or_path, **kwargs):
- r"""
- Instantiates a inpainting Pytorch diffusion pipeline from pretrained pipeline weight.
-
- The from_pretrained() method takes care of returning the correct pipeline class instance by:
- 1. Detect the pipeline class of the pretrained_model_or_path based on the _class_name property of its
- config object
- 2. Find the inpainting pipeline linked to the pipeline class using pattern matching on pipeline class name.
-
- If a `controlnet` argument is passed, it will instantiate a StableDiffusionControlNetInpaintPipeline object.
-
- The pipeline is set in evaluation mode (`model.eval()`) by default.
-
- If you get the error message below, you need to finetune the weights for your downstream task:
-
- ```
- Some weights of UNet2DConditionModel were not initialized from the model checkpoint at runwayml/stable-diffusion-v1-5 and are newly initialized because the shapes did not match:
- - conv_in.weight: found shape torch.Size([320, 4, 3, 3]) in the checkpoint and torch.Size([320, 9, 3, 3]) in the model instantiated
- You should probably TRAIN this model on a down-stream task to be able to use it for predictions and inference.
- ```
-
- Parameters:
- pretrained_model_name_or_path (`str` or `os.PathLike`, *optional*):
- Can be either:
-
- - A string, the *repo id* (for example `CompVis/ldm-text2im-large-256`) of a pretrained pipeline
- hosted on the Hub.
- - A path to a *directory* (for example `./my_pipeline_directory/`) containing pipeline weights
- saved using
- [`~DiffusionPipeline.save_pretrained`].
- torch_dtype (`str` or `torch.dtype`, *optional*):
- Override the default `torch.dtype` and load the model with another dtype. If "auto" is passed, the
- dtype is automatically derived from the model's weights.
- force_download (`bool`, *optional*, defaults to `False`):
- Whether or not to force the (re-)download of the model weights and configuration files, overriding the
- cached versions if they exist.
- cache_dir (`Union[str, os.PathLike]`, *optional*):
- Path to a directory where a downloaded pretrained model configuration is cached if the standard cache
- is not used.
- resume_download (`bool`, *optional*, defaults to `False`):
- Whether or not to resume downloading the model weights and configuration files. If set to `False`, any
- incompletely downloaded files are deleted.
- proxies (`Dict[str, str]`, *optional*):
- A dictionary of proxy servers to use by protocol or endpoint, for example, `{'http': 'foo.bar:3128',
- 'http://hostname': 'foo.bar:4012'}`. The proxies are used on each request.
- output_loading_info(`bool`, *optional*, defaults to `False`):
- Whether or not to also return a dictionary containing missing keys, unexpected keys and error messages.
- local_files_only (`bool`, *optional*, defaults to `False`):
- Whether to only load local model weights and configuration files or not. If set to `True`, the model
- won't be downloaded from the Hub.
- use_auth_token (`str` or *bool*, *optional*):
- The token to use as HTTP bearer authorization for remote files. If `True`, the token generated from
- `diffusers-cli login` (stored in `~/.huggingface`) is used.
- revision (`str`, *optional*, defaults to `"main"`):
- The specific model version to use. It can be a branch name, a tag name, a commit id, or any identifier
- allowed by Git.
- custom_revision (`str`, *optional*, defaults to `"main"`):
- The specific model version to use. It can be a branch name, a tag name, or a commit id similar to
- `revision` when loading a custom pipeline from the Hub. It can be a 🤗 Diffusers version when loading a
- custom pipeline from GitHub, otherwise it defaults to `"main"` when loading from the Hub.
- mirror (`str`, *optional*):
- Mirror source to resolve accessibility issues if you’re downloading a model in China. We do not
- guarantee the timeliness or safety of the source, and you should refer to the mirror site for more
- information.
- device_map (`str` or `Dict[str, Union[int, str, torch.device]]`, *optional*):
- A map that specifies where each submodule should go. It doesn’t need to be defined for each
- parameter/buffer name; once a given module name is inside, every submodule of it will be sent to the
- same device.
-
- Set `device_map="auto"` to have 🤗 Accelerate automatically compute the most optimized `device_map`. For
- more information about each option see [designing a device
- map](https://hf.co/docs/accelerate/main/en/usage_guides/big_modeling#designing-a-device-map).
- max_memory (`Dict`, *optional*):
- A dictionary device identifier for the maximum memory. Will default to the maximum memory available for
- each GPU and the available CPU RAM if unset.
- offload_folder (`str` or `os.PathLike`, *optional*):
- The path to offload weights if device_map contains the value `"disk"`.
- offload_state_dict (`bool`, *optional*):
- If `True`, temporarily offloads the CPU state dict to the hard drive to avoid running out of CPU RAM if
- the weight of the CPU state dict + the biggest shard of the checkpoint does not fit. Defaults to `True`
- when there is some disk offload.
- low_cpu_mem_usage (`bool`, *optional*, defaults to `True` if torch version >= 1.9.0 else `False`):
- Speed up model loading only loading the pretrained weights and not initializing the weights. This also
- tries to not use more than 1x model size in CPU memory (including peak memory) while loading the model.
- Only supported for PyTorch >= 1.9.0. If you are using an older version of PyTorch, setting this
- argument to `True` will raise an error.
- use_safetensors (`bool`, *optional*, defaults to `None`):
- If set to `None`, the safetensors weights are downloaded if they're available **and** if the
- safetensors library is installed. If set to `True`, the model is forcibly loaded from safetensors
- weights. If set to `False`, safetensors weights are not loaded.
- kwargs (remaining dictionary of keyword arguments, *optional*):
- Can be used to overwrite load and saveable variables (the pipeline components of the specific pipeline
- class). The overwritten components are passed directly to the pipelines `__init__` method. See example
- below for more information.
- variant (`str`, *optional*):
- Load weights from a specified variant filename such as `"fp16"` or `"ema"`. This is ignored when
- loading `from_flax`.
-
-
-
- To use private or [gated](https://huggingface.co/docs/hub/models-gated#gated-models) models, log-in with
- `huggingface-cli login`.
-
-
-
- Examples:
-
- ```py
- >>> from diffusers import AutoPipelineForTextToImage
-
- >>> pipeline = AutoPipelineForImageToImage.from_pretrained("runwayml/stable-diffusion-v1-5")
- >>> print(pipeline.__class__)
- ```
- """
- config = cls.load_config(pretrained_model_or_path)
- orig_class_name = config["_class_name"]
-
- if "controlnet" in kwargs:
- orig_class_name = config["_class_name"].replace("Pipeline", "ControlNetPipeline")
-
- inpainting_cls = _get_task_class(AUTO_INPAINT_PIPELINES_MAPPING, orig_class_name)
-
- return inpainting_cls.from_pretrained(pretrained_model_or_path, **kwargs)
-
- @classmethod
- def from_pipe(cls, pipeline, **kwargs):
- r"""
- Instantiates a inpainting Pytorch diffusion pipeline from another instantiated diffusion pipeline class.
-
- The from_pipe() method takes care of returning the correct pipeline class instance by finding the inpainting
- pipeline linked to the pipeline class using pattern matching on pipeline class name.
-
- All the modules the pipeline class contain will be used to initialize the new pipeline without reallocating
- additional memoery.
-
- The pipeline is set in evaluation mode (`model.eval()`) by default.
-
- Parameters:
- pipeline (`DiffusionPipeline`):
- an instantiated `DiffusionPipeline` object
-
- Examples:
-
- ```py
- >>> from diffusers import AutoPipelineForTextToImage, AutoPipelineForInpainting
-
- >>> pipe_t2i = AutoPipelineForText2Image.from_pretrained(
- ... "DeepFloyd/IF-I-XL-v1.0", requires_safety_checker=False
- ... )
-
- >>> pipe_inpaint = AutoPipelineForInpainting.from_pipe(pipe_t2i)
- ```
- """
- original_config = dict(pipeline.config)
- original_cls_name = pipeline.__class__.__name__
-
- # derive the pipeline class to instantiate
- inpainting_cls = _get_task_class(AUTO_INPAINT_PIPELINES_MAPPING, original_cls_name)
-
- # define expected module and optional kwargs given the pipeline signature
- expected_modules, optional_kwargs = _get_signature_keys(inpainting_cls)
-
- pretrained_model_name_or_path = original_config.pop("_name_or_path", None)
-
- # allow users pass modules in `kwargs` to override the original pipeline's components
- passed_class_obj = {k: kwargs.pop(k) for k in expected_modules if k in kwargs}
- original_class_obj = {
- k: pipeline.components[k]
- for k, v in pipeline.components.items()
- if k in expected_modules and k not in passed_class_obj
- }
-
- # allow users pass optional kwargs to override the original pipelines config attribute
- passed_pipe_kwargs = {k: kwargs.pop(k) for k in optional_kwargs if k in kwargs}
- original_pipe_kwargs = {
- k: original_config[k]
- for k, v in original_config.items()
- if k in optional_kwargs and k not in passed_pipe_kwargs
- }
-
- # config that were not expected by original pipeline is stored as private attribute
- # we will pass them as optional arguments if they can be accepted by the pipeline
- additional_pipe_kwargs = [
- k[1:]
- for k in original_config.keys()
- if k.startswith("_") and k[1:] in optional_kwargs and k[1:] not in passed_pipe_kwargs
- ]
- for k in additional_pipe_kwargs:
- original_pipe_kwargs[k] = original_config.pop(f"_{k}")
-
- inpainting_kwargs = {**passed_class_obj, **original_class_obj, **passed_pipe_kwargs, **original_pipe_kwargs}
-
- # store unused config as private attribute
- unused_original_config = {
- f"{'' if k.startswith('_') else '_'}{k}": original_config[k]
- for k, v in original_config.items()
- if k not in inpainting_kwargs
- }
-
- missing_modules = set(expected_modules) - set(pipeline._optional_components) - set(inpainting_kwargs.keys())
-
- if len(missing_modules) > 0:
- raise ValueError(
- f"Pipeline {inpainting_cls} expected {expected_modules}, but only {set(list(passed_class_obj.keys()) + list(original_class_obj.keys()))} were passed"
- )
-
- model = inpainting_cls(**inpainting_kwargs)
- model.register_to_config(_name_or_path=pretrained_model_name_or_path)
- model.register_to_config(**unused_original_config)
-
- return model
diff --git a/spaces/Andy1621/uniformer_image_detection/mmdet/models/dense_heads/dense_test_mixins.py b/spaces/Andy1621/uniformer_image_detection/mmdet/models/dense_heads/dense_test_mixins.py
deleted file mode 100644
index dd81364dec90e97c30a6e2220a5e0fe96373c5bd..0000000000000000000000000000000000000000
--- a/spaces/Andy1621/uniformer_image_detection/mmdet/models/dense_heads/dense_test_mixins.py
+++ /dev/null
@@ -1,100 +0,0 @@
-from inspect import signature
-
-import torch
-
-from mmdet.core import bbox2result, bbox_mapping_back, multiclass_nms
-
-
-class BBoxTestMixin(object):
- """Mixin class for test time augmentation of bboxes."""
-
- def merge_aug_bboxes(self, aug_bboxes, aug_scores, img_metas):
- """Merge augmented detection bboxes and scores.
-
- Args:
- aug_bboxes (list[Tensor]): shape (n, 4*#class)
- aug_scores (list[Tensor] or None): shape (n, #class)
- img_shapes (list[Tensor]): shape (3, ).
-
- Returns:
- tuple: (bboxes, scores)
- """
- recovered_bboxes = []
- for bboxes, img_info in zip(aug_bboxes, img_metas):
- img_shape = img_info[0]['img_shape']
- scale_factor = img_info[0]['scale_factor']
- flip = img_info[0]['flip']
- flip_direction = img_info[0]['flip_direction']
- bboxes = bbox_mapping_back(bboxes, img_shape, scale_factor, flip,
- flip_direction)
- recovered_bboxes.append(bboxes)
- bboxes = torch.cat(recovered_bboxes, dim=0)
- if aug_scores is None:
- return bboxes
- else:
- scores = torch.cat(aug_scores, dim=0)
- return bboxes, scores
-
- def aug_test_bboxes(self, feats, img_metas, rescale=False):
- """Test det bboxes with test time augmentation.
-
- Args:
- feats (list[Tensor]): the outer list indicates test-time
- augmentations and inner Tensor should have a shape NxCxHxW,
- which contains features for all images in the batch.
- img_metas (list[list[dict]]): the outer list indicates test-time
- augs (multiscale, flip, etc.) and the inner list indicates
- images in a batch. each dict has image information.
- rescale (bool, optional): Whether to rescale the results.
- Defaults to False.
-
- Returns:
- list[ndarray]: bbox results of each class
- """
- # check with_nms argument
- gb_sig = signature(self.get_bboxes)
- gb_args = [p.name for p in gb_sig.parameters.values()]
- if hasattr(self, '_get_bboxes'):
- gbs_sig = signature(self._get_bboxes)
- else:
- gbs_sig = signature(self._get_bboxes_single)
- gbs_args = [p.name for p in gbs_sig.parameters.values()]
- assert ('with_nms' in gb_args) and ('with_nms' in gbs_args), \
- f'{self.__class__.__name__}' \
- ' does not support test-time augmentation'
-
- aug_bboxes = []
- aug_scores = []
- aug_factors = [] # score_factors for NMS
- for x, img_meta in zip(feats, img_metas):
- # only one image in the batch
- outs = self.forward(x)
- bbox_inputs = outs + (img_meta, self.test_cfg, False, False)
- bbox_outputs = self.get_bboxes(*bbox_inputs)[0]
- aug_bboxes.append(bbox_outputs[0])
- aug_scores.append(bbox_outputs[1])
- # bbox_outputs of some detectors (e.g., ATSS, FCOS, YOLOv3)
- # contains additional element to adjust scores before NMS
- if len(bbox_outputs) >= 3:
- aug_factors.append(bbox_outputs[2])
-
- # after merging, bboxes will be rescaled to the original image size
- merged_bboxes, merged_scores = self.merge_aug_bboxes(
- aug_bboxes, aug_scores, img_metas)
- merged_factors = torch.cat(aug_factors, dim=0) if aug_factors else None
- det_bboxes, det_labels = multiclass_nms(
- merged_bboxes,
- merged_scores,
- self.test_cfg.score_thr,
- self.test_cfg.nms,
- self.test_cfg.max_per_img,
- score_factors=merged_factors)
-
- if rescale:
- _det_bboxes = det_bboxes
- else:
- _det_bboxes = det_bboxes.clone()
- _det_bboxes[:, :4] *= det_bboxes.new_tensor(
- img_metas[0][0]['scale_factor'])
- bbox_results = bbox2result(_det_bboxes, det_labels, self.num_classes)
- return bbox_results
diff --git a/spaces/Andy1621/uniformer_image_segmentation/configs/gcnet/README.md b/spaces/Andy1621/uniformer_image_segmentation/configs/gcnet/README.md
deleted file mode 100644
index 72f10d14b34a1df68dfeb0fdb056d527ed698c26..0000000000000000000000000000000000000000
--- a/spaces/Andy1621/uniformer_image_segmentation/configs/gcnet/README.md
+++ /dev/null
@@ -1,48 +0,0 @@
-# GCNet: Non-local Networks Meet Squeeze-Excitation Networks and Beyond
-
-## Introduction
-
-
-
-```latex
-@inproceedings{cao2019gcnet,
- title={Gcnet: Non-local networks meet squeeze-excitation networks and beyond},
- author={Cao, Yue and Xu, Jiarui and Lin, Stephen and Wei, Fangyun and Hu, Han},
- booktitle={Proceedings of the IEEE International Conference on Computer Vision Workshops},
- pages={0--0},
- year={2019}
-}
-```
-
-## Results and models
-
-### Cityscapes
-
-| Method | Backbone | Crop Size | Lr schd | Mem (GB) | Inf time (fps) | mIoU | mIoU(ms+flip) | config | download |
-| ------ | -------- | --------- | ------: | -------- | -------------- | ----: | ------------: | ------------------------------------------------------------------------------------------------------------------------- | ------------------------------------------------------------------------------------------------------------------------------------------------------------------------------------------------------------------------------------------------------------------------------------------------------------------------------------------------------ |
-| GCNet | R-50-D8 | 512x1024 | 40000 | 5.8 | 3.93 | 77.69 | 78.56 | [config](https://github.com/open-mmlab/mmsegmentation/blob/master/configs/gcnet/gcnet_r50-d8_512x1024_40k_cityscapes.py) | [model](https://download.openmmlab.com/mmsegmentation/v0.5/gcnet/gcnet_r50-d8_512x1024_40k_cityscapes/gcnet_r50-d8_512x1024_40k_cityscapes_20200618_074436-4b0fd17b.pth) | [log](https://download.openmmlab.com/mmsegmentation/v0.5/gcnet/gcnet_r50-d8_512x1024_40k_cityscapes/gcnet_r50-d8_512x1024_40k_cityscapes_20200618_074436.log.json) |
-| GCNet | R-101-D8 | 512x1024 | 40000 | 9.2 | 2.61 | 78.28 | 79.34 | [config](https://github.com/open-mmlab/mmsegmentation/blob/master/configs/gcnet/gcnet_r101-d8_512x1024_40k_cityscapes.py) | [model](https://download.openmmlab.com/mmsegmentation/v0.5/gcnet/gcnet_r101-d8_512x1024_40k_cityscapes/gcnet_r101-d8_512x1024_40k_cityscapes_20200618_074436-5e62567f.pth) | [log](https://download.openmmlab.com/mmsegmentation/v0.5/gcnet/gcnet_r101-d8_512x1024_40k_cityscapes/gcnet_r101-d8_512x1024_40k_cityscapes_20200618_074436.log.json) |
-| GCNet | R-50-D8 | 769x769 | 40000 | 6.5 | 1.67 | 78.12 | 80.09 | [config](https://github.com/open-mmlab/mmsegmentation/blob/master/configs/gcnet/gcnet_r50-d8_769x769_40k_cityscapes.py) | [model](https://download.openmmlab.com/mmsegmentation/v0.5/gcnet/gcnet_r50-d8_769x769_40k_cityscapes/gcnet_r50-d8_769x769_40k_cityscapes_20200618_182814-a26f4471.pth) | [log](https://download.openmmlab.com/mmsegmentation/v0.5/gcnet/gcnet_r50-d8_769x769_40k_cityscapes/gcnet_r50-d8_769x769_40k_cityscapes_20200618_182814.log.json) |
-| GCNet | R-101-D8 | 769x769 | 40000 | 10.5 | 1.13 | 78.95 | 80.71 | [config](https://github.com/open-mmlab/mmsegmentation/blob/master/configs/gcnet/gcnet_r101-d8_769x769_40k_cityscapes.py) | [model](https://download.openmmlab.com/mmsegmentation/v0.5/gcnet/gcnet_r101-d8_769x769_40k_cityscapes/gcnet_r101-d8_769x769_40k_cityscapes_20200619_092550-ca4f0a84.pth) | [log](https://download.openmmlab.com/mmsegmentation/v0.5/gcnet/gcnet_r101-d8_769x769_40k_cityscapes/gcnet_r101-d8_769x769_40k_cityscapes_20200619_092550.log.json) |
-| GCNet | R-50-D8 | 512x1024 | 80000 | - | - | 78.48 | 80.01 | [config](https://github.com/open-mmlab/mmsegmentation/blob/master/configs/gcnet/gcnet_r50-d8_512x1024_80k_cityscapes.py) | [model](https://download.openmmlab.com/mmsegmentation/v0.5/gcnet/gcnet_r50-d8_512x1024_80k_cityscapes/gcnet_r50-d8_512x1024_80k_cityscapes_20200618_074450-ef8f069b.pth) | [log](https://download.openmmlab.com/mmsegmentation/v0.5/gcnet/gcnet_r50-d8_512x1024_80k_cityscapes/gcnet_r50-d8_512x1024_80k_cityscapes_20200618_074450.log.json) |
-| GCNet | R-101-D8 | 512x1024 | 80000 | - | - | 79.03 | 79.84 | [config](https://github.com/open-mmlab/mmsegmentation/blob/master/configs/gcnet/gcnet_r101-d8_512x1024_80k_cityscapes.py) | [model](https://download.openmmlab.com/mmsegmentation/v0.5/gcnet/gcnet_r101-d8_512x1024_80k_cityscapes/gcnet_r101-d8_512x1024_80k_cityscapes_20200618_074450-778ebf69.pth) | [log](https://download.openmmlab.com/mmsegmentation/v0.5/gcnet/gcnet_r101-d8_512x1024_80k_cityscapes/gcnet_r101-d8_512x1024_80k_cityscapes_20200618_074450.log.json) |
-| GCNet | R-50-D8 | 769x769 | 80000 | - | - | 78.68 | 80.66 | [config](https://github.com/open-mmlab/mmsegmentation/blob/master/configs/gcnet/gcnet_r50-d8_769x769_80k_cityscapes.py) | [model](https://download.openmmlab.com/mmsegmentation/v0.5/gcnet/gcnet_r50-d8_769x769_80k_cityscapes/gcnet_r50-d8_769x769_80k_cityscapes_20200619_092516-4839565b.pth) | [log](https://download.openmmlab.com/mmsegmentation/v0.5/gcnet/gcnet_r50-d8_769x769_80k_cityscapes/gcnet_r50-d8_769x769_80k_cityscapes_20200619_092516.log.json) |
-| GCNet | R-101-D8 | 769x769 | 80000 | - | - | 79.18 | 80.71 | [config](https://github.com/open-mmlab/mmsegmentation/blob/master/configs/gcnet/gcnet_r101-d8_769x769_80k_cityscapes.py) | [model](https://download.openmmlab.com/mmsegmentation/v0.5/gcnet/gcnet_r101-d8_769x769_80k_cityscapes/gcnet_r101-d8_769x769_80k_cityscapes_20200619_092628-8e043423.pth) | [log](https://download.openmmlab.com/mmsegmentation/v0.5/gcnet/gcnet_r101-d8_769x769_80k_cityscapes/gcnet_r101-d8_769x769_80k_cityscapes_20200619_092628.log.json) |
-
-### ADE20K
-
-| Method | Backbone | Crop Size | Lr schd | Mem (GB) | Inf time (fps) | mIoU | mIoU(ms+flip) | config | download |
-| ------ | -------- | --------- | ------: | -------- | -------------- | ----: | ------------: | --------------------------------------------------------------------------------------------------------------------- | -------------------------------------------------------------------------------------------------------------------------------------------------------------------------------------------------------------------------------------------------------------------------------------------------------------------------------------- |
-| GCNet | R-50-D8 | 512x512 | 80000 | 8.5 | 23.38 | 41.47 | 42.85 | [config](https://github.com/open-mmlab/mmsegmentation/blob/master/configs/gcnet/gcnet_r50-d8_512x512_80k_ade20k.py) | [model](https://download.openmmlab.com/mmsegmentation/v0.5/gcnet/gcnet_r50-d8_512x512_80k_ade20k/gcnet_r50-d8_512x512_80k_ade20k_20200614_185146-91a6da41.pth) | [log](https://download.openmmlab.com/mmsegmentation/v0.5/gcnet/gcnet_r50-d8_512x512_80k_ade20k/gcnet_r50-d8_512x512_80k_ade20k_20200614_185146.log.json) |
-| GCNet | R-101-D8 | 512x512 | 80000 | 12 | 15.20 | 42.82 | 44.54 | [config](https://github.com/open-mmlab/mmsegmentation/blob/master/configs/gcnet/gcnet_r101-d8_512x512_80k_ade20k.py) | [model](https://download.openmmlab.com/mmsegmentation/v0.5/gcnet/gcnet_r101-d8_512x512_80k_ade20k/gcnet_r101-d8_512x512_80k_ade20k_20200615_020811-c3fcb6dd.pth) | [log](https://download.openmmlab.com/mmsegmentation/v0.5/gcnet/gcnet_r101-d8_512x512_80k_ade20k/gcnet_r101-d8_512x512_80k_ade20k_20200615_020811.log.json) |
-| GCNet | R-50-D8 | 512x512 | 160000 | - | - | 42.37 | 43.52 | [config](https://github.com/open-mmlab/mmsegmentation/blob/master/configs/gcnet/gcnet_r50-d8_512x512_160k_ade20k.py) | [model](https://download.openmmlab.com/mmsegmentation/v0.5/gcnet/gcnet_r50-d8_512x512_160k_ade20k/gcnet_r50-d8_512x512_160k_ade20k_20200615_224122-d95f3e1f.pth) | [log](https://download.openmmlab.com/mmsegmentation/v0.5/gcnet/gcnet_r50-d8_512x512_160k_ade20k/gcnet_r50-d8_512x512_160k_ade20k_20200615_224122.log.json) |
-| GCNet | R-101-D8 | 512x512 | 160000 | - | - | 43.69 | 45.21 | [config](https://github.com/open-mmlab/mmsegmentation/blob/master/configs/gcnet/gcnet_r101-d8_512x512_160k_ade20k.py) | [model](https://download.openmmlab.com/mmsegmentation/v0.5/gcnet/gcnet_r101-d8_512x512_160k_ade20k/gcnet_r101-d8_512x512_160k_ade20k_20200615_225406-615528d7.pth) | [log](https://download.openmmlab.com/mmsegmentation/v0.5/gcnet/gcnet_r101-d8_512x512_160k_ade20k/gcnet_r101-d8_512x512_160k_ade20k_20200615_225406.log.json) |
-
-### Pascal VOC 2012 + Aug
-
-| Method | Backbone | Crop Size | Lr schd | Mem (GB) | Inf time (fps) | mIoU | mIoU(ms+flip) | config | download |
-| ------ | -------- | --------- | ------: | -------- | -------------- | ----: | ------------: | ---------------------------------------------------------------------------------------------------------------------- | ------------------------------------------------------------------------------------------------------------------------------------------------------------------------------------------------------------------------------------------------------------------------------------------------------------------------------------------ |
-| GCNet | R-50-D8 | 512x512 | 20000 | 5.8 | 23.35 | 76.42 | 77.51 | [config](https://github.com/open-mmlab/mmsegmentation/blob/master/configs/gcnet/gcnet_r50-d8_512x512_20k_voc12aug.py) | [model](https://download.openmmlab.com/mmsegmentation/v0.5/gcnet/gcnet_r50-d8_512x512_20k_voc12aug/gcnet_r50-d8_512x512_20k_voc12aug_20200617_165701-3cbfdab1.pth) | [log](https://download.openmmlab.com/mmsegmentation/v0.5/gcnet/gcnet_r50-d8_512x512_20k_voc12aug/gcnet_r50-d8_512x512_20k_voc12aug_20200617_165701.log.json) |
-| GCNet | R-101-D8 | 512x512 | 20000 | 9.2 | 14.80 | 77.41 | 78.56 | [config](https://github.com/open-mmlab/mmsegmentation/blob/master/configs/gcnet/gcnet_r101-d8_512x512_20k_voc12aug.py) | [model](https://download.openmmlab.com/mmsegmentation/v0.5/gcnet/gcnet_r101-d8_512x512_20k_voc12aug/gcnet_r101-d8_512x512_20k_voc12aug_20200617_165713-6c720aa9.pth) | [log](https://download.openmmlab.com/mmsegmentation/v0.5/gcnet/gcnet_r101-d8_512x512_20k_voc12aug/gcnet_r101-d8_512x512_20k_voc12aug_20200617_165713.log.json) |
-| GCNet | R-50-D8 | 512x512 | 40000 | - | - | 76.24 | 77.63 | [config](https://github.com/open-mmlab/mmsegmentation/blob/master/configs/gcnet/gcnet_r50-d8_512x512_40k_voc12aug.py) | [model](https://download.openmmlab.com/mmsegmentation/v0.5/gcnet/gcnet_r50-d8_512x512_40k_voc12aug/gcnet_r50-d8_512x512_40k_voc12aug_20200613_195105-9797336d.pth) | [log](https://download.openmmlab.com/mmsegmentation/v0.5/gcnet/gcnet_r50-d8_512x512_40k_voc12aug/gcnet_r50-d8_512x512_40k_voc12aug_20200613_195105.log.json) |
-| GCNet | R-101-D8 | 512x512 | 40000 | - | - | 77.84 | 78.59 | [config](https://github.com/open-mmlab/mmsegmentation/blob/master/configs/gcnet/gcnet_r101-d8_512x512_40k_voc12aug.py) | [model](https://download.openmmlab.com/mmsegmentation/v0.5/gcnet/gcnet_r101-d8_512x512_40k_voc12aug/gcnet_r101-d8_512x512_40k_voc12aug_20200613_185806-1e38208d.pth) | [log](https://download.openmmlab.com/mmsegmentation/v0.5/gcnet/gcnet_r101-d8_512x512_40k_voc12aug/gcnet_r101-d8_512x512_40k_voc12aug_20200613_185806.log.json) |
diff --git a/spaces/Andy1621/uniformer_image_segmentation/configs/gcnet/gcnet_r101-d8_769x769_40k_cityscapes.py b/spaces/Andy1621/uniformer_image_segmentation/configs/gcnet/gcnet_r101-d8_769x769_40k_cityscapes.py
deleted file mode 100644
index 08a6031f20234b1cc1d792ea5d4891613503a185..0000000000000000000000000000000000000000
--- a/spaces/Andy1621/uniformer_image_segmentation/configs/gcnet/gcnet_r101-d8_769x769_40k_cityscapes.py
+++ /dev/null
@@ -1,2 +0,0 @@
-_base_ = './gcnet_r50-d8_769x769_40k_cityscapes.py'
-model = dict(pretrained='open-mmlab://resnet101_v1c', backbone=dict(depth=101))
diff --git a/spaces/Anonymous-sub/Rerender/ControlNet/annotator/uniformer/configs/_base_/datasets/pascal_context_59.py b/spaces/Anonymous-sub/Rerender/ControlNet/annotator/uniformer/configs/_base_/datasets/pascal_context_59.py
deleted file mode 100644
index 37585abab89834b95cd5bdd993b994fca1db65f6..0000000000000000000000000000000000000000
--- a/spaces/Anonymous-sub/Rerender/ControlNet/annotator/uniformer/configs/_base_/datasets/pascal_context_59.py
+++ /dev/null
@@ -1,60 +0,0 @@
-# dataset settings
-dataset_type = 'PascalContextDataset59'
-data_root = 'data/VOCdevkit/VOC2010/'
-img_norm_cfg = dict(
- mean=[123.675, 116.28, 103.53], std=[58.395, 57.12, 57.375], to_rgb=True)
-
-img_scale = (520, 520)
-crop_size = (480, 480)
-
-train_pipeline = [
- dict(type='LoadImageFromFile'),
- dict(type='LoadAnnotations', reduce_zero_label=True),
- dict(type='Resize', img_scale=img_scale, ratio_range=(0.5, 2.0)),
- dict(type='RandomCrop', crop_size=crop_size, cat_max_ratio=0.75),
- dict(type='RandomFlip', prob=0.5),
- dict(type='PhotoMetricDistortion'),
- dict(type='Normalize', **img_norm_cfg),
- dict(type='Pad', size=crop_size, pad_val=0, seg_pad_val=255),
- dict(type='DefaultFormatBundle'),
- dict(type='Collect', keys=['img', 'gt_semantic_seg']),
-]
-test_pipeline = [
- dict(type='LoadImageFromFile'),
- dict(
- type='MultiScaleFlipAug',
- img_scale=img_scale,
- # img_ratios=[0.5, 0.75, 1.0, 1.25, 1.5, 1.75],
- flip=False,
- transforms=[
- dict(type='Resize', keep_ratio=True),
- dict(type='RandomFlip'),
- dict(type='Normalize', **img_norm_cfg),
- dict(type='ImageToTensor', keys=['img']),
- dict(type='Collect', keys=['img']),
- ])
-]
-data = dict(
- samples_per_gpu=4,
- workers_per_gpu=4,
- train=dict(
- type=dataset_type,
- data_root=data_root,
- img_dir='JPEGImages',
- ann_dir='SegmentationClassContext',
- split='ImageSets/SegmentationContext/train.txt',
- pipeline=train_pipeline),
- val=dict(
- type=dataset_type,
- data_root=data_root,
- img_dir='JPEGImages',
- ann_dir='SegmentationClassContext',
- split='ImageSets/SegmentationContext/val.txt',
- pipeline=test_pipeline),
- test=dict(
- type=dataset_type,
- data_root=data_root,
- img_dir='JPEGImages',
- ann_dir='SegmentationClassContext',
- split='ImageSets/SegmentationContext/val.txt',
- pipeline=test_pipeline))
diff --git a/spaces/Anonymous-sub/Rerender/gmflow_module/utils/misc.py b/spaces/Anonymous-sub/Rerender/gmflow_module/utils/misc.py
deleted file mode 100644
index bcaf8b5e91ef61f256a94d919988a7a87cd90a7d..0000000000000000000000000000000000000000
--- a/spaces/Anonymous-sub/Rerender/gmflow_module/utils/misc.py
+++ /dev/null
@@ -1,42 +0,0 @@
-import os
-import numpy as np
-import sys
-import json
-
-
-def read_text_lines(filepath):
- with open(filepath, 'r') as f:
- lines = f.readlines()
- lines = [l.rstrip() for l in lines]
- return lines
-
-
-def check_path(path):
- if not os.path.exists(path):
- os.makedirs(path, exist_ok=True) # explicitly set exist_ok when multi-processing
-
-
-def save_command(save_path, filename='command_train.txt'):
- check_path(save_path)
- command = sys.argv
- save_file = os.path.join(save_path, filename)
- # Save all training commands when resuming training
- with open(save_file, 'a') as f:
- f.write(' '.join(command))
- f.write('\n\n')
-
-
-def save_args(args, filename='args.json'):
- args_dict = vars(args)
- check_path(args.checkpoint_dir)
- save_path = os.path.join(args.checkpoint_dir, filename)
-
- # Save all training args when resuming training
- with open(save_path, 'a') as f:
- json.dump(args_dict, f, indent=4, sort_keys=False)
- f.write('\n\n')
-
-
-def int_list(s):
- """Convert string to int list"""
- return [int(x) for x in s.split(',')]
diff --git a/spaces/Ataturk-Chatbot/HuggingFaceChat/venv/lib/python3.11/site-packages/pip/__pip-runner__.py b/spaces/Ataturk-Chatbot/HuggingFaceChat/venv/lib/python3.11/site-packages/pip/__pip-runner__.py
deleted file mode 100644
index 49a148a097e9cc06c165571e0bffaf7cae17dc5b..0000000000000000000000000000000000000000
--- a/spaces/Ataturk-Chatbot/HuggingFaceChat/venv/lib/python3.11/site-packages/pip/__pip-runner__.py
+++ /dev/null
@@ -1,50 +0,0 @@
-"""Execute exactly this copy of pip, within a different environment.
-
-This file is named as it is, to ensure that this module can't be imported via
-an import statement.
-"""
-
-# /!\ This version compatibility check section must be Python 2 compatible. /!\
-
-import sys
-
-# Copied from setup.py
-PYTHON_REQUIRES = (3, 7)
-
-
-def version_str(version): # type: ignore
- return ".".join(str(v) for v in version)
-
-
-if sys.version_info[:2] < PYTHON_REQUIRES:
- raise SystemExit(
- "This version of pip does not support python {} (requires >={}).".format(
- version_str(sys.version_info[:2]), version_str(PYTHON_REQUIRES)
- )
- )
-
-# From here on, we can use Python 3 features, but the syntax must remain
-# Python 2 compatible.
-
-import runpy # noqa: E402
-from importlib.machinery import PathFinder # noqa: E402
-from os.path import dirname # noqa: E402
-
-PIP_SOURCES_ROOT = dirname(dirname(__file__))
-
-
-class PipImportRedirectingFinder:
- @classmethod
- def find_spec(self, fullname, path=None, target=None): # type: ignore
- if fullname != "pip":
- return None
-
- spec = PathFinder.find_spec(fullname, [PIP_SOURCES_ROOT], target)
- assert spec, (PIP_SOURCES_ROOT, fullname)
- return spec
-
-
-sys.meta_path.insert(0, PipImportRedirectingFinder())
-
-assert __name__ == "__main__", "Cannot run __pip-runner__.py as a non-main module"
-runpy.run_module("pip", run_name="__main__", alter_sys=True)
diff --git a/spaces/Ataturk-Chatbot/HuggingFaceChat/venv/lib/python3.11/site-packages/pip/_internal/network/utils.py b/spaces/Ataturk-Chatbot/HuggingFaceChat/venv/lib/python3.11/site-packages/pip/_internal/network/utils.py
deleted file mode 100644
index 134848ae526e54e2b18738f83088c4a17efcce96..0000000000000000000000000000000000000000
--- a/spaces/Ataturk-Chatbot/HuggingFaceChat/venv/lib/python3.11/site-packages/pip/_internal/network/utils.py
+++ /dev/null
@@ -1,96 +0,0 @@
-from typing import Dict, Generator
-
-from pip._vendor.requests.models import CONTENT_CHUNK_SIZE, Response
-
-from pip._internal.exceptions import NetworkConnectionError
-
-# The following comments and HTTP headers were originally added by
-# Donald Stufft in git commit 22c562429a61bb77172039e480873fb239dd8c03.
-#
-# We use Accept-Encoding: identity here because requests defaults to
-# accepting compressed responses. This breaks in a variety of ways
-# depending on how the server is configured.
-# - Some servers will notice that the file isn't a compressible file
-# and will leave the file alone and with an empty Content-Encoding
-# - Some servers will notice that the file is already compressed and
-# will leave the file alone, adding a Content-Encoding: gzip header
-# - Some servers won't notice anything at all and will take a file
-# that's already been compressed and compress it again, and set
-# the Content-Encoding: gzip header
-# By setting this to request only the identity encoding we're hoping
-# to eliminate the third case. Hopefully there does not exist a server
-# which when given a file will notice it is already compressed and that
-# you're not asking for a compressed file and will then decompress it
-# before sending because if that's the case I don't think it'll ever be
-# possible to make this work.
-HEADERS: Dict[str, str] = {"Accept-Encoding": "identity"}
-
-
-def raise_for_status(resp: Response) -> None:
- http_error_msg = ""
- if isinstance(resp.reason, bytes):
- # We attempt to decode utf-8 first because some servers
- # choose to localize their reason strings. If the string
- # isn't utf-8, we fall back to iso-8859-1 for all other
- # encodings.
- try:
- reason = resp.reason.decode("utf-8")
- except UnicodeDecodeError:
- reason = resp.reason.decode("iso-8859-1")
- else:
- reason = resp.reason
-
- if 400 <= resp.status_code < 500:
- http_error_msg = (
- f"{resp.status_code} Client Error: {reason} for url: {resp.url}"
- )
-
- elif 500 <= resp.status_code < 600:
- http_error_msg = (
- f"{resp.status_code} Server Error: {reason} for url: {resp.url}"
- )
-
- if http_error_msg:
- raise NetworkConnectionError(http_error_msg, response=resp)
-
-
-def response_chunks(
- response: Response, chunk_size: int = CONTENT_CHUNK_SIZE
-) -> Generator[bytes, None, None]:
- """Given a requests Response, provide the data chunks."""
- try:
- # Special case for urllib3.
- for chunk in response.raw.stream(
- chunk_size,
- # We use decode_content=False here because we don't
- # want urllib3 to mess with the raw bytes we get
- # from the server. If we decompress inside of
- # urllib3 then we cannot verify the checksum
- # because the checksum will be of the compressed
- # file. This breakage will only occur if the
- # server adds a Content-Encoding header, which
- # depends on how the server was configured:
- # - Some servers will notice that the file isn't a
- # compressible file and will leave the file alone
- # and with an empty Content-Encoding
- # - Some servers will notice that the file is
- # already compressed and will leave the file
- # alone and will add a Content-Encoding: gzip
- # header
- # - Some servers won't notice anything at all and
- # will take a file that's already been compressed
- # and compress it again and set the
- # Content-Encoding: gzip header
- #
- # By setting this not to decode automatically we
- # hope to eliminate problems with the second case.
- decode_content=False,
- ):
- yield chunk
- except AttributeError:
- # Standard file-like object.
- while True:
- chunk = response.raw.read(chunk_size)
- if not chunk:
- break
- yield chunk
diff --git a/spaces/Ataturk-Chatbot/HuggingFaceChat/venv/lib/python3.11/site-packages/setuptools/_distutils/archive_util.py b/spaces/Ataturk-Chatbot/HuggingFaceChat/venv/lib/python3.11/site-packages/setuptools/_distutils/archive_util.py
deleted file mode 100644
index 5dfe2a16ffbf5dc907aa3ce315757f4f9a055a82..0000000000000000000000000000000000000000
--- a/spaces/Ataturk-Chatbot/HuggingFaceChat/venv/lib/python3.11/site-packages/setuptools/_distutils/archive_util.py
+++ /dev/null
@@ -1,280 +0,0 @@
-"""distutils.archive_util
-
-Utility functions for creating archive files (tarballs, zip files,
-that sort of thing)."""
-
-import os
-from warnings import warn
-import sys
-
-try:
- import zipfile
-except ImportError:
- zipfile = None
-
-
-from distutils.errors import DistutilsExecError
-from distutils.spawn import spawn
-from distutils.dir_util import mkpath
-from distutils import log
-
-try:
- from pwd import getpwnam
-except ImportError:
- getpwnam = None
-
-try:
- from grp import getgrnam
-except ImportError:
- getgrnam = None
-
-
-def _get_gid(name):
- """Returns a gid, given a group name."""
- if getgrnam is None or name is None:
- return None
- try:
- result = getgrnam(name)
- except KeyError:
- result = None
- if result is not None:
- return result[2]
- return None
-
-
-def _get_uid(name):
- """Returns an uid, given a user name."""
- if getpwnam is None or name is None:
- return None
- try:
- result = getpwnam(name)
- except KeyError:
- result = None
- if result is not None:
- return result[2]
- return None
-
-
-def make_tarball(
- base_name, base_dir, compress="gzip", verbose=0, dry_run=0, owner=None, group=None
-):
- """Create a (possibly compressed) tar file from all the files under
- 'base_dir'.
-
- 'compress' must be "gzip" (the default), "bzip2", "xz", "compress", or
- None. ("compress" will be deprecated in Python 3.2)
-
- 'owner' and 'group' can be used to define an owner and a group for the
- archive that is being built. If not provided, the current owner and group
- will be used.
-
- The output tar file will be named 'base_dir' + ".tar", possibly plus
- the appropriate compression extension (".gz", ".bz2", ".xz" or ".Z").
-
- Returns the output filename.
- """
- tar_compression = {
- 'gzip': 'gz',
- 'bzip2': 'bz2',
- 'xz': 'xz',
- None: '',
- 'compress': '',
- }
- compress_ext = {'gzip': '.gz', 'bzip2': '.bz2', 'xz': '.xz', 'compress': '.Z'}
-
- # flags for compression program, each element of list will be an argument
- if compress is not None and compress not in compress_ext.keys():
- raise ValueError(
- "bad value for 'compress': must be None, 'gzip', 'bzip2', "
- "'xz' or 'compress'"
- )
-
- archive_name = base_name + '.tar'
- if compress != 'compress':
- archive_name += compress_ext.get(compress, '')
-
- mkpath(os.path.dirname(archive_name), dry_run=dry_run)
-
- # creating the tarball
- import tarfile # late import so Python build itself doesn't break
-
- log.info('Creating tar archive')
-
- uid = _get_uid(owner)
- gid = _get_gid(group)
-
- def _set_uid_gid(tarinfo):
- if gid is not None:
- tarinfo.gid = gid
- tarinfo.gname = group
- if uid is not None:
- tarinfo.uid = uid
- tarinfo.uname = owner
- return tarinfo
-
- if not dry_run:
- tar = tarfile.open(archive_name, 'w|%s' % tar_compression[compress])
- try:
- tar.add(base_dir, filter=_set_uid_gid)
- finally:
- tar.close()
-
- # compression using `compress`
- if compress == 'compress':
- warn("'compress' is deprecated.", DeprecationWarning)
- # the option varies depending on the platform
- compressed_name = archive_name + compress_ext[compress]
- if sys.platform == 'win32':
- cmd = [compress, archive_name, compressed_name]
- else:
- cmd = [compress, '-f', archive_name]
- spawn(cmd, dry_run=dry_run)
- return compressed_name
-
- return archive_name
-
-
-def make_zipfile(base_name, base_dir, verbose=0, dry_run=0): # noqa: C901
- """Create a zip file from all the files under 'base_dir'.
-
- The output zip file will be named 'base_name' + ".zip". Uses either the
- "zipfile" Python module (if available) or the InfoZIP "zip" utility
- (if installed and found on the default search path). If neither tool is
- available, raises DistutilsExecError. Returns the name of the output zip
- file.
- """
- zip_filename = base_name + ".zip"
- mkpath(os.path.dirname(zip_filename), dry_run=dry_run)
-
- # If zipfile module is not available, try spawning an external
- # 'zip' command.
- if zipfile is None:
- if verbose:
- zipoptions = "-r"
- else:
- zipoptions = "-rq"
-
- try:
- spawn(["zip", zipoptions, zip_filename, base_dir], dry_run=dry_run)
- except DistutilsExecError:
- # XXX really should distinguish between "couldn't find
- # external 'zip' command" and "zip failed".
- raise DistutilsExecError(
- (
- "unable to create zip file '%s': "
- "could neither import the 'zipfile' module nor "
- "find a standalone zip utility"
- )
- % zip_filename
- )
-
- else:
- log.info("creating '%s' and adding '%s' to it", zip_filename, base_dir)
-
- if not dry_run:
- try:
- zip = zipfile.ZipFile(
- zip_filename, "w", compression=zipfile.ZIP_DEFLATED
- )
- except RuntimeError:
- zip = zipfile.ZipFile(zip_filename, "w", compression=zipfile.ZIP_STORED)
-
- with zip:
- if base_dir != os.curdir:
- path = os.path.normpath(os.path.join(base_dir, ''))
- zip.write(path, path)
- log.info("adding '%s'", path)
- for dirpath, dirnames, filenames in os.walk(base_dir):
- for name in dirnames:
- path = os.path.normpath(os.path.join(dirpath, name, ''))
- zip.write(path, path)
- log.info("adding '%s'", path)
- for name in filenames:
- path = os.path.normpath(os.path.join(dirpath, name))
- if os.path.isfile(path):
- zip.write(path, path)
- log.info("adding '%s'", path)
-
- return zip_filename
-
-
-ARCHIVE_FORMATS = {
- 'gztar': (make_tarball, [('compress', 'gzip')], "gzip'ed tar-file"),
- 'bztar': (make_tarball, [('compress', 'bzip2')], "bzip2'ed tar-file"),
- 'xztar': (make_tarball, [('compress', 'xz')], "xz'ed tar-file"),
- 'ztar': (make_tarball, [('compress', 'compress')], "compressed tar file"),
- 'tar': (make_tarball, [('compress', None)], "uncompressed tar file"),
- 'zip': (make_zipfile, [], "ZIP file"),
-}
-
-
-def check_archive_formats(formats):
- """Returns the first format from the 'format' list that is unknown.
-
- If all formats are known, returns None
- """
- for format in formats:
- if format not in ARCHIVE_FORMATS:
- return format
- return None
-
-
-def make_archive(
- base_name,
- format,
- root_dir=None,
- base_dir=None,
- verbose=0,
- dry_run=0,
- owner=None,
- group=None,
-):
- """Create an archive file (eg. zip or tar).
-
- 'base_name' is the name of the file to create, minus any format-specific
- extension; 'format' is the archive format: one of "zip", "tar", "gztar",
- "bztar", "xztar", or "ztar".
-
- 'root_dir' is a directory that will be the root directory of the
- archive; ie. we typically chdir into 'root_dir' before creating the
- archive. 'base_dir' is the directory where we start archiving from;
- ie. 'base_dir' will be the common prefix of all files and
- directories in the archive. 'root_dir' and 'base_dir' both default
- to the current directory. Returns the name of the archive file.
-
- 'owner' and 'group' are used when creating a tar archive. By default,
- uses the current owner and group.
- """
- save_cwd = os.getcwd()
- if root_dir is not None:
- log.debug("changing into '%s'", root_dir)
- base_name = os.path.abspath(base_name)
- if not dry_run:
- os.chdir(root_dir)
-
- if base_dir is None:
- base_dir = os.curdir
-
- kwargs = {'dry_run': dry_run}
-
- try:
- format_info = ARCHIVE_FORMATS[format]
- except KeyError:
- raise ValueError("unknown archive format '%s'" % format)
-
- func = format_info[0]
- for arg, val in format_info[1]:
- kwargs[arg] = val
-
- if format != 'zip':
- kwargs['owner'] = owner
- kwargs['group'] = group
-
- try:
- filename = func(base_name, base_dir, **kwargs)
- finally:
- if root_dir is not None:
- log.debug("changing back to '%s'", save_cwd)
- os.chdir(save_cwd)
-
- return filename
diff --git a/spaces/Atualli/node-media-server/README.md b/spaces/Atualli/node-media-server/README.md
deleted file mode 100644
index 23136c02773afdfa4654675f154bd8bf06fe3b20..0000000000000000000000000000000000000000
--- a/spaces/Atualli/node-media-server/README.md
+++ /dev/null
@@ -1,11 +0,0 @@
----
-title: Node Media Server
-emoji: 🚀
-colorFrom: indigo
-colorTo: gray
-sdk: docker
-pinned: false
-license: apache-2.0
----
-
-Check out the configuration reference at https://huggingface.co/docs/hub/spaces-config-reference
\ No newline at end of file
diff --git a/spaces/Awiny/Image2Paragraph/models/grit_src/third_party/CenterNet2/docs/tutorials/lazyconfigs.md b/spaces/Awiny/Image2Paragraph/models/grit_src/third_party/CenterNet2/docs/tutorials/lazyconfigs.md
deleted file mode 100644
index ca9de3052a8065c1c4579499cb8ef7ed9fc2d660..0000000000000000000000000000000000000000
--- a/spaces/Awiny/Image2Paragraph/models/grit_src/third_party/CenterNet2/docs/tutorials/lazyconfigs.md
+++ /dev/null
@@ -1,170 +0,0 @@
-# Lazy Configs
-
-The traditional yacs-based config system provides basic, standard functionalities.
-However, it does not offer enough flexibility for many new projects.
-We develop an alternative, non-intrusive config system that can be used with
-detectron2 or potentially any other complex projects.
-
-## Python Syntax
-
-Our config objects are still dictionaries. Instead of using Yaml to define dictionaries,
-we create dictionaries in Python directly. This gives users the following power that
-doesn't exist in Yaml:
-
-* Easily manipulate the dictionary (addition & deletion) using Python.
-* Write simple arithmetics or call simple functions.
-* Use more data types / objects.
-* Import / compose other config files, using the familiar Python import syntax.
-
-A Python config file can be loaded like this:
-```python
-# config.py:
-a = dict(x=1, y=2, z=dict(xx=1))
-b = dict(x=3, y=4)
-
-# my_code.py:
-from detectron2.config import LazyConfig
-cfg = LazyConfig.load("path/to/config.py") # an omegaconf dictionary
-assert cfg.a.z.xx == 1
-```
-
-After [LazyConfig.load](../modules/config.html#detectron2.config.LazyConfig.load), `cfg` will be a dictionary that contains all dictionaries
-defined in the global scope of the config file. Note that:
-* All dictionaries are turned to an [omegaconf](https://omegaconf.readthedocs.io/)
- config object during loading. This enables access to omegaconf features,
- such as its [access syntax](https://omegaconf.readthedocs.io/en/2.1_branch/usage.html#access-and-manipulation)
- and [interpolation](https://omegaconf.readthedocs.io/en/2.1_branch/usage.html#variable-interpolation).
-* Absolute imports in `config.py` works the same as in regular Python.
-* Relative imports can only import dictionaries from config files.
- They are simply a syntax sugar for [LazyConfig.load_rel](../modules/config.html#detectron2.config.LazyConfig.load_rel).
- They can load Python files at relative path without requiring `__init__.py`.
-
-[LazyConfig.save](../modules/config.html#detectron2.config.LazyConfig.save) can save a config object to yaml.
-Note that this is not always successful if non-serializable objects appear in the config file (e.g. lambdas).
-It is up to users whether to sacrifice the ability to save in exchange for flexibility.
-
-## Recursive Instantiation
-
-The LazyConfig system heavily uses recursive instantiation, which is a pattern that
-uses a dictionary to describe a
-call to a function/class. The dictionary consists of:
-
-1. A "\_target\_" key which contains path to the callable, such as "module.submodule.class_name".
-2. Other keys that represent arguments to pass to the callable. Arguments themselves can be defined
- using recursive instantiation.
-
-We provide a helper function [LazyCall](../modules/config.html#detectron2.config.LazyCall) that helps create such dictionaries.
-The following code using `LazyCall`
-```python
-from detectron2.config import LazyCall as L
-from my_app import Trainer, Optimizer
-cfg = L(Trainer)(
- optimizer=L(Optimizer)(
- lr=0.01,
- algo="SGD"
- )
-)
-```
-creates a dictionary like this:
-```
-cfg = {
- "_target_": "my_app.Trainer",
- "optimizer": {
- "_target_": "my_app.Optimizer",
- "lr": 0.01, "algo": "SGD"
- }
-}
-```
-
-By representing objects using such dictionaries, a general
-[instantiate](../modules/config.html#detectron2.config.instantiate)
-function can turn them into actual objects, i.e.:
-```python
-from detectron2.config import instantiate
-trainer = instantiate(cfg)
-# equivalent to:
-# from my_app import Trainer, Optimizer
-# trainer = Trainer(optimizer=Optimizer(lr=0.01, algo="SGD"))
-```
-
-This pattern is powerful enough to describe very complex objects, e.g.:
-
-
-
-A Full Mask R-CNN described in recursive instantiation (click to expand)
-
-
-```eval_rst
-.. literalinclude:: ../../configs/common/models/mask_rcnn_fpn.py
- :language: python
- :linenos:
-```
-
-
-
-There are also objects or logic that cannot be described simply by a dictionary,
-such as reused objects or method calls. They may require some refactoring
-to work with recursive instantiation.
-
-## Using Model Zoo LazyConfigs
-
-We provide some configs in the model zoo using the LazyConfig system, for example:
-
-* [common baselines](../../configs/common/).
-* [new Mask R-CNN baselines](../../configs/new_baselines/)
-
-After installing detectron2, they can be loaded by the model zoo API
-[model_zoo.get_config](../modules/model_zoo.html#detectron2.model_zoo.get_config).
-
-Using these as references, you're free to define custom config structure / fields for your own
-project, as long as your training script can understand them.
-Despite of this, our model zoo configs still follow some simple conventions for consistency, e.g.
-`cfg.model` defines a model object, `cfg.dataloader.{train,test}` defines dataloader objects,
-and `cfg.train` contains training options in key-value form.
-In addition to `print()`, a better way to view the structure of a config is like this:
-```
-from detectron2.model_zoo import get_config
-from detectron2.config import LazyConfig
-print(LazyConfig.to_py(get_config("COCO-InstanceSegmentation/mask_rcnn_R_50_FPN_1x.py")))
-```
-From the output it's easier to find relevant options to change, e.g.
-`dataloader.train.total_batch_size` for the batch size, or `optimizer.lr` for base learning rate.
-
-We provide a reference training script
-[tools/lazyconfig_train_net.py](../../tools/lazyconfig_train_net.py),
-that can train/eval our model zoo configs.
-It also shows how to support command line value overrides.
-
-To demonstrate the power and flexibility of the new system, we show that
-[a simple config file](../../configs/Misc/torchvision_imagenet_R_50.py)
-can let detectron2 train an ImageNet classification model from torchvision, even though
-detectron2 contains no features about ImageNet classification.
-This can serve as a reference for using detectron2 in other deep learning tasks.
-
-## Summary
-
-By using recursive instantiation to create objects,
-we avoid passing a giant config to many places, because `cfg` is only passed to `instantiate`.
-This has the following benefits:
-
-* It's __non-intrusive__: objects to be constructed are config-agnostic, regular Python
- functions/classes.
- They can even live in other libraries. For example,
- `{"_target_": "torch.nn.Conv2d", "in_channels": 10, "out_channels": 10, "kernel_size": 1}`
- defines a conv layer.
-* __Clarity__ of what function/classes will be called, and what arguments they use.
-* `cfg` doesn't need pre-defined keys and structures. It's valid as long as it translates to valid
- code. This gives a lot more __flexibility__.
-* You can still pass huge dictionaries as arguments, just like the old way.
-
-Recursive instantiation and Python syntax are orthogonal: you can use one without the other.
-But by putting them together, the config file looks a lot like the code that will be executed:
-
-
-
-However, the config file just defines dictionaries, which can be easily manipulated further
-by composition or overrides.
-The corresponding code will only be executed
-later when `instantiate` is called. In some way,
-in config files we're writing "editable code" that will be "lazily executed" later when needed.
-That's why we call this system "LazyConfig".
diff --git a/spaces/Awiny/Image2Paragraph/models/grit_src/third_party/CenterNet2/projects/CenterNet2/centernet/modeling/backbone/bifpn_fcos.py b/spaces/Awiny/Image2Paragraph/models/grit_src/third_party/CenterNet2/projects/CenterNet2/centernet/modeling/backbone/bifpn_fcos.py
deleted file mode 100644
index 17f2904ccad484f380b64efc668b9090d047d15e..0000000000000000000000000000000000000000
--- a/spaces/Awiny/Image2Paragraph/models/grit_src/third_party/CenterNet2/projects/CenterNet2/centernet/modeling/backbone/bifpn_fcos.py
+++ /dev/null
@@ -1,469 +0,0 @@
-# This file is modified from https://github.com/aim-uofa/AdelaiDet/blob/master/adet/modeling/backbone/bifpn.py
-# The original file is under 2-clause BSD License for academic use, and *non-commercial use*.
-import torch
-import torch.nn.functional as F
-from torch import nn
-
-from detectron2.layers import Conv2d, ShapeSpec, get_norm
-
-from detectron2.modeling.backbone import Backbone, build_resnet_backbone
-from detectron2.modeling import BACKBONE_REGISTRY
-from .dlafpn import dla34
-
-__all__ = []
-
-
-def swish(x):
- return x * x.sigmoid()
-
-
-def split_name(name):
- for i, c in enumerate(name):
- if not c.isalpha():
- return name[:i], int(name[i:])
- raise ValueError()
-
-
-class FeatureMapResampler(nn.Module):
- def __init__(self, in_channels, out_channels, stride, norm=""):
- super(FeatureMapResampler, self).__init__()
- if in_channels != out_channels:
- self.reduction = Conv2d(
- in_channels, out_channels, kernel_size=1,
- bias=(norm == ""),
- norm=get_norm(norm, out_channels),
- activation=None
- )
- else:
- self.reduction = None
-
- assert stride <= 2
- self.stride = stride
-
- def forward(self, x):
- if self.reduction is not None:
- x = self.reduction(x)
-
- if self.stride == 2:
- x = F.max_pool2d(
- x, kernel_size=self.stride + 1,
- stride=self.stride, padding=1
- )
- elif self.stride == 1:
- pass
- else:
- raise NotImplementedError()
- return x
-
-
-class BackboneWithTopLevels(Backbone):
- def __init__(self, backbone, out_channels, num_top_levels, norm=""):
- super(BackboneWithTopLevels, self).__init__()
- self.backbone = backbone
- backbone_output_shape = backbone.output_shape()
-
- self._out_feature_channels = {name: shape.channels for name, shape in backbone_output_shape.items()}
- self._out_feature_strides = {name: shape.stride for name, shape in backbone_output_shape.items()}
- self._out_features = list(self._out_feature_strides.keys())
-
- last_feature_name = max(self._out_feature_strides.keys(), key=lambda x: split_name(x)[1])
- self.last_feature_name = last_feature_name
- self.num_top_levels = num_top_levels
-
- last_channels = self._out_feature_channels[last_feature_name]
- last_stride = self._out_feature_strides[last_feature_name]
-
- prefix, suffix = split_name(last_feature_name)
- prev_channels = last_channels
- for i in range(num_top_levels):
- name = prefix + str(suffix + i + 1)
- self.add_module(name, FeatureMapResampler(
- prev_channels, out_channels, 2, norm
- ))
- prev_channels = out_channels
-
- self._out_feature_channels[name] = out_channels
- self._out_feature_strides[name] = last_stride * 2 ** (i + 1)
- self._out_features.append(name)
-
- def forward(self, x):
- outputs = self.backbone(x)
- last_features = outputs[self.last_feature_name]
- prefix, suffix = split_name(self.last_feature_name)
-
- x = last_features
- for i in range(self.num_top_levels):
- name = prefix + str(suffix + i + 1)
- x = self.__getattr__(name)(x)
- outputs[name] = x
-
- return outputs
-
-
-class SingleBiFPN(Backbone):
- """
- This module implements Feature Pyramid Network.
- It creates pyramid features built on top of some input feature maps.
- """
-
- def __init__(
- self, in_channels_list, out_channels, norm=""
- ):
- """
- Args:
- bottom_up (Backbone): module representing the bottom up subnetwork.
- Must be a subclass of :class:`Backbone`. The multi-scale feature
- maps generated by the bottom up network, and listed in `in_features`,
- are used to generate FPN levels.
- in_features (list[str]): names of the input feature maps coming
- from the backbone to which FPN is attached. For example, if the
- backbone produces ["res2", "res3", "res4"], any *contiguous* sublist
- of these may be used; order must be from high to low resolution.
- out_channels (int): number of channels in the output feature maps.
- norm (str): the normalization to use.
- """
- super(SingleBiFPN, self).__init__()
-
- self.out_channels = out_channels
- # build 5-levels bifpn
- if len(in_channels_list) == 5:
- self.nodes = [
- {'feat_level': 3, 'inputs_offsets': [3, 4]},
- {'feat_level': 2, 'inputs_offsets': [2, 5]},
- {'feat_level': 1, 'inputs_offsets': [1, 6]},
- {'feat_level': 0, 'inputs_offsets': [0, 7]},
- {'feat_level': 1, 'inputs_offsets': [1, 7, 8]},
- {'feat_level': 2, 'inputs_offsets': [2, 6, 9]},
- {'feat_level': 3, 'inputs_offsets': [3, 5, 10]},
- {'feat_level': 4, 'inputs_offsets': [4, 11]},
- ]
- elif len(in_channels_list) == 3:
- self.nodes = [
- {'feat_level': 1, 'inputs_offsets': [1, 2]},
- {'feat_level': 0, 'inputs_offsets': [0, 3]},
- {'feat_level': 1, 'inputs_offsets': [1, 3, 4]},
- {'feat_level': 2, 'inputs_offsets': [2, 5]},
- ]
- else:
- raise NotImplementedError
-
- node_info = [_ for _ in in_channels_list]
-
- num_output_connections = [0 for _ in in_channels_list]
- for fnode in self.nodes:
- feat_level = fnode["feat_level"]
- inputs_offsets = fnode["inputs_offsets"]
- inputs_offsets_str = "_".join(map(str, inputs_offsets))
- for input_offset in inputs_offsets:
- num_output_connections[input_offset] += 1
-
- in_channels = node_info[input_offset]
- if in_channels != out_channels:
- lateral_conv = Conv2d(
- in_channels,
- out_channels,
- kernel_size=1,
- norm=get_norm(norm, out_channels)
- )
- self.add_module(
- "lateral_{}_f{}".format(input_offset, feat_level), lateral_conv
- )
- node_info.append(out_channels)
- num_output_connections.append(0)
-
- # generate attention weights
- name = "weights_f{}_{}".format(feat_level, inputs_offsets_str)
- self.__setattr__(name, nn.Parameter(
- torch.ones(len(inputs_offsets), dtype=torch.float32),
- requires_grad=True
- ))
-
- # generate convolutions after combination
- name = "outputs_f{}_{}".format(feat_level, inputs_offsets_str)
- self.add_module(name, Conv2d(
- out_channels,
- out_channels,
- kernel_size=3,
- padding=1,
- norm=get_norm(norm, out_channels),
- bias=(norm == "")
- ))
-
- def forward(self, feats):
- """
- Args:
- input (dict[str->Tensor]): mapping feature map name (e.g., "p5") to
- feature map tensor for each feature level in high to low resolution order.
- Returns:
- dict[str->Tensor]:
- mapping from feature map name to FPN feature map tensor
- in high to low resolution order. Returned feature names follow the FPN
- paper convention: "p", where stage has stride = 2 ** stage e.g.,
- ["n2", "n3", ..., "n6"].
- """
- feats = [_ for _ in feats]
- num_levels = len(feats)
- num_output_connections = [0 for _ in feats]
- for fnode in self.nodes:
- feat_level = fnode["feat_level"]
- inputs_offsets = fnode["inputs_offsets"]
- inputs_offsets_str = "_".join(map(str, inputs_offsets))
- input_nodes = []
- _, _, target_h, target_w = feats[feat_level].size()
- for input_offset in inputs_offsets:
- num_output_connections[input_offset] += 1
- input_node = feats[input_offset]
-
- # reduction
- if input_node.size(1) != self.out_channels:
- name = "lateral_{}_f{}".format(input_offset, feat_level)
- input_node = self.__getattr__(name)(input_node)
-
- # maybe downsample
- _, _, h, w = input_node.size()
- if h > target_h and w > target_w:
- height_stride_size = int((h - 1) // target_h + 1)
- width_stride_size = int((w - 1) // target_w + 1)
- assert height_stride_size == width_stride_size == 2
- input_node = F.max_pool2d(
- input_node, kernel_size=(height_stride_size + 1, width_stride_size + 1),
- stride=(height_stride_size, width_stride_size), padding=1
- )
- elif h <= target_h and w <= target_w:
- if h < target_h or w < target_w:
- input_node = F.interpolate(
- input_node,
- size=(target_h, target_w),
- mode="nearest"
- )
- else:
- raise NotImplementedError()
- input_nodes.append(input_node)
-
- # attention
- name = "weights_f{}_{}".format(feat_level, inputs_offsets_str)
- weights = F.relu(self.__getattr__(name))
- norm_weights = weights / (weights.sum() + 0.0001)
-
- new_node = torch.stack(input_nodes, dim=-1)
- new_node = (norm_weights * new_node).sum(dim=-1)
- new_node = swish(new_node)
-
- name = "outputs_f{}_{}".format(feat_level, inputs_offsets_str)
- feats.append(self.__getattr__(name)(new_node))
-
- num_output_connections.append(0)
-
- output_feats = []
- for idx in range(num_levels):
- for i, fnode in enumerate(reversed(self.nodes)):
- if fnode['feat_level'] == idx:
- output_feats.append(feats[-1 - i])
- break
- else:
- raise ValueError()
- return output_feats
-
-
-class BiFPN(Backbone):
- """
- This module implements Feature Pyramid Network.
- It creates pyramid features built on top of some input feature maps.
- """
-
- def __init__(
- self, bottom_up, in_features, out_channels, num_top_levels, num_repeats, norm=""
- ):
- """
- Args:
- bottom_up (Backbone): module representing the bottom up subnetwork.
- Must be a subclass of :class:`Backbone`. The multi-scale feature
- maps generated by the bottom up network, and listed in `in_features`,
- are used to generate FPN levels.
- in_features (list[str]): names of the input feature maps coming
- from the backbone to which FPN is attached. For example, if the
- backbone produces ["res2", "res3", "res4"], any *contiguous* sublist
- of these may be used; order must be from high to low resolution.
- out_channels (int): number of channels in the output feature maps.
- num_top_levels (int): the number of the top levels (p6 or p7).
- num_repeats (int): the number of repeats of BiFPN.
- norm (str): the normalization to use.
- """
- super(BiFPN, self).__init__()
- assert isinstance(bottom_up, Backbone)
-
- # add extra feature levels (i.e., 6 and 7)
- self.bottom_up = BackboneWithTopLevels(
- bottom_up, out_channels,
- num_top_levels, norm
- )
- bottom_up_output_shapes = self.bottom_up.output_shape()
-
- in_features = sorted(in_features, key=lambda x: split_name(x)[1])
- self._size_divisibility = 128 #bottom_up_output_shapes[in_features[-1]].stride
- self.out_channels = out_channels
- self.min_level = split_name(in_features[0])[1]
-
- # add the names for top blocks
- prefix, last_suffix = split_name(in_features[-1])
- for i in range(num_top_levels):
- in_features.append(prefix + str(last_suffix + i + 1))
- self.in_features = in_features
-
- # generate output features
- self._out_features = ["p{}".format(split_name(name)[1]) for name in in_features]
- self._out_feature_strides = {
- out_name: bottom_up_output_shapes[in_name].stride
- for out_name, in_name in zip(self._out_features, in_features)
- }
- self._out_feature_channels = {k: out_channels for k in self._out_features}
-
- # build bifpn
- self.repeated_bifpn = nn.ModuleList()
- for i in range(num_repeats):
- if i == 0:
- in_channels_list = [
- bottom_up_output_shapes[name].channels for name in in_features
- ]
- else:
- in_channels_list = [
- self._out_feature_channels[name] for name in self._out_features
- ]
- self.repeated_bifpn.append(SingleBiFPN(
- in_channels_list, out_channels, norm
- ))
-
- @property
- def size_divisibility(self):
- return self._size_divisibility
-
- def forward(self, x):
- """
- Args:
- input (dict[str->Tensor]): mapping feature map name (e.g., "p5") to
- feature map tensor for each feature level in high to low resolution order.
- Returns:
- dict[str->Tensor]:
- mapping from feature map name to FPN feature map tensor
- in high to low resolution order. Returned feature names follow the FPN
- paper convention: "p", where stage has stride = 2 ** stage e.g.,
- ["n2", "n3", ..., "n6"].
- """
- bottom_up_features = self.bottom_up(x)
- feats = [bottom_up_features[f] for f in self.in_features]
-
- for bifpn in self.repeated_bifpn:
- feats = bifpn(feats)
-
- return dict(zip(self._out_features, feats))
-
-
-def _assert_strides_are_log2_contiguous(strides):
- """
- Assert that each stride is 2x times its preceding stride, i.e. "contiguous in log2".
- """
- for i, stride in enumerate(strides[1:], 1):
- assert stride == 2 * strides[i - 1], "Strides {} {} are not log2 contiguous".format(
- stride, strides[i - 1]
- )
-
-
-@BACKBONE_REGISTRY.register()
-def build_fcos_resnet_bifpn_backbone(cfg, input_shape: ShapeSpec):
- """
- Args:
- cfg: a detectron2 CfgNode
- Returns:
- backbone (Backbone): backbone module, must be a subclass of :class:`Backbone`.
- """
- bottom_up = build_resnet_backbone(cfg, input_shape)
- in_features = cfg.MODEL.FPN.IN_FEATURES
- out_channels = cfg.MODEL.BIFPN.OUT_CHANNELS
- num_repeats = cfg.MODEL.BIFPN.NUM_BIFPN
- top_levels = 2
-
- backbone = BiFPN(
- bottom_up=bottom_up,
- in_features=in_features,
- out_channels=out_channels,
- num_top_levels=top_levels,
- num_repeats=num_repeats,
- norm=cfg.MODEL.BIFPN.NORM
- )
- return backbone
-
-
-
-@BACKBONE_REGISTRY.register()
-def build_p35_fcos_resnet_bifpn_backbone(cfg, input_shape: ShapeSpec):
- """
- Args:
- cfg: a detectron2 CfgNode
- Returns:
- backbone (Backbone): backbone module, must be a subclass of :class:`Backbone`.
- """
- bottom_up = build_resnet_backbone(cfg, input_shape)
- in_features = cfg.MODEL.FPN.IN_FEATURES
- out_channels = cfg.MODEL.BIFPN.OUT_CHANNELS
- num_repeats = cfg.MODEL.BIFPN.NUM_BIFPN
- top_levels = 0
-
- backbone = BiFPN(
- bottom_up=bottom_up,
- in_features=in_features,
- out_channels=out_channels,
- num_top_levels=top_levels,
- num_repeats=num_repeats,
- norm=cfg.MODEL.BIFPN.NORM
- )
- return backbone
-
-
-@BACKBONE_REGISTRY.register()
-def build_p35_fcos_dla_bifpn_backbone(cfg, input_shape: ShapeSpec):
- """
- Args:
- cfg: a detectron2 CfgNode
- Returns:
- backbone (Backbone): backbone module, must be a subclass of :class:`Backbone`.
- """
- bottom_up = dla34(cfg)
- in_features = cfg.MODEL.FPN.IN_FEATURES
- out_channels = cfg.MODEL.BIFPN.OUT_CHANNELS
- num_repeats = cfg.MODEL.BIFPN.NUM_BIFPN
- top_levels = 0
-
- backbone = BiFPN(
- bottom_up=bottom_up,
- in_features=in_features,
- out_channels=out_channels,
- num_top_levels=top_levels,
- num_repeats=num_repeats,
- norm=cfg.MODEL.BIFPN.NORM
- )
- return backbone
-
-@BACKBONE_REGISTRY.register()
-def build_p37_fcos_dla_bifpn_backbone(cfg, input_shape: ShapeSpec):
- """
- Args:
- cfg: a detectron2 CfgNode
- Returns:
- backbone (Backbone): backbone module, must be a subclass of :class:`Backbone`.
- """
- bottom_up = dla34(cfg)
- in_features = cfg.MODEL.FPN.IN_FEATURES
- out_channels = cfg.MODEL.BIFPN.OUT_CHANNELS
- num_repeats = cfg.MODEL.BIFPN.NUM_BIFPN
- assert cfg.MODEL.BIFPN.NUM_LEVELS == 5
- top_levels = 2
-
- backbone = BiFPN(
- bottom_up=bottom_up,
- in_features=in_features,
- out_channels=out_channels,
- num_top_levels=top_levels,
- num_repeats=num_repeats,
- norm=cfg.MODEL.BIFPN.NORM
- )
- return backbone
\ No newline at end of file
diff --git a/spaces/Awiny/Image2Paragraph/models/grit_src/third_party/CenterNet2/tests/data/__init__.py b/spaces/Awiny/Image2Paragraph/models/grit_src/third_party/CenterNet2/tests/data/__init__.py
deleted file mode 100644
index e69de29bb2d1d6434b8b29ae775ad8c2e48c5391..0000000000000000000000000000000000000000
diff --git a/spaces/Awiny/Image2Paragraph/pretrained_models/download_pretrain.sh b/spaces/Awiny/Image2Paragraph/pretrained_models/download_pretrain.sh
deleted file mode 100644
index 0c576c30290725c8098521e034b69263aeaf577f..0000000000000000000000000000000000000000
--- a/spaces/Awiny/Image2Paragraph/pretrained_models/download_pretrain.sh
+++ /dev/null
@@ -1,5 +0,0 @@
-git clone https://huggingface.co/openai/clip-vit-large-patch14
-git clone https://huggingface.co/shi-labs/oneformer_ade20k_swin_large
-git clone https://huggingface.co/shi-labs/oneformer_coco_swin_large
-git clone https://huggingface.co/Salesforce/blip-image-captioning-large
-git clone https://huggingface.co/CIDAS/clipseg-rd64-refined
\ No newline at end of file
diff --git a/spaces/Banbri/zcvzcv/src/components/ui/dropdown-menu.tsx b/spaces/Banbri/zcvzcv/src/components/ui/dropdown-menu.tsx
deleted file mode 100644
index 5803489a1d197a9db5018e413e63abe84b2efb8e..0000000000000000000000000000000000000000
--- a/spaces/Banbri/zcvzcv/src/components/ui/dropdown-menu.tsx
+++ /dev/null
@@ -1,200 +0,0 @@
-"use client"
-
-import * as React from "react"
-import * as DropdownMenuPrimitive from "@radix-ui/react-dropdown-menu"
-import { Check, ChevronRight, Circle } from "lucide-react"
-
-import { cn } from "@/lib/utils"
-
-const DropdownMenu = DropdownMenuPrimitive.Root
-
-const DropdownMenuTrigger = DropdownMenuPrimitive.Trigger
-
-const DropdownMenuGroup = DropdownMenuPrimitive.Group
-
-const DropdownMenuPortal = DropdownMenuPrimitive.Portal
-
-const DropdownMenuSub = DropdownMenuPrimitive.Sub
-
-const DropdownMenuRadioGroup = DropdownMenuPrimitive.RadioGroup
-
-const DropdownMenuSubTrigger = React.forwardRef<
- React.ElementRef,
- React.ComponentPropsWithoutRef & {
- inset?: boolean
- }
->(({ className, inset, children, ...props }, ref) => (
-
- {children}
-
-
-))
-DropdownMenuSubTrigger.displayName =
- DropdownMenuPrimitive.SubTrigger.displayName
-
-const DropdownMenuSubContent = React.forwardRef<
- React.ElementRef,
- React.ComponentPropsWithoutRef
->(({ className, ...props }, ref) => (
-
-))
-DropdownMenuSubContent.displayName =
- DropdownMenuPrimitive.SubContent.displayName
-
-const DropdownMenuContent = React.forwardRef<
- React.ElementRef,
- React.ComponentPropsWithoutRef
->(({ className, sideOffset = 4, ...props }, ref) => (
-
-
-
-))
-DropdownMenuContent.displayName = DropdownMenuPrimitive.Content.displayName
-
-const DropdownMenuItem = React.forwardRef<
- React.ElementRef,
- React.ComponentPropsWithoutRef & {
- inset?: boolean
- }
->(({ className, inset, ...props }, ref) => (
-
-))
-DropdownMenuItem.displayName = DropdownMenuPrimitive.Item.displayName
-
-const DropdownMenuCheckboxItem = React.forwardRef<
- React.ElementRef,
- React.ComponentPropsWithoutRef
->(({ className, children, checked, ...props }, ref) => (
-
-
-
-
-
-
- {children}
-
-))
-DropdownMenuCheckboxItem.displayName =
- DropdownMenuPrimitive.CheckboxItem.displayName
-
-const DropdownMenuRadioItem = React.forwardRef<
- React.ElementRef,
- React.ComponentPropsWithoutRef
->(({ className, children, ...props }, ref) => (
-
-
-
-
-
-
- {children}
-
-))
-DropdownMenuRadioItem.displayName = DropdownMenuPrimitive.RadioItem.displayName
-
-const DropdownMenuLabel = React.forwardRef<
- React.ElementRef,
- React.ComponentPropsWithoutRef & {
- inset?: boolean
- }
->(({ className, inset, ...props }, ref) => (
-
-))
-DropdownMenuLabel.displayName = DropdownMenuPrimitive.Label.displayName
-
-const DropdownMenuSeparator = React.forwardRef<
- React.ElementRef,
- React.ComponentPropsWithoutRef
->(({ className, ...props }, ref) => (
-
-))
-DropdownMenuSeparator.displayName = DropdownMenuPrimitive.Separator.displayName
-
-const DropdownMenuShortcut = ({
- className,
- ...props
-}: React.HTMLAttributes) => {
- return (
-
- )
-}
-DropdownMenuShortcut.displayName = "DropdownMenuShortcut"
-
-export {
- DropdownMenu,
- DropdownMenuTrigger,
- DropdownMenuContent,
- DropdownMenuItem,
- DropdownMenuCheckboxItem,
- DropdownMenuRadioItem,
- DropdownMenuLabel,
- DropdownMenuSeparator,
- DropdownMenuShortcut,
- DropdownMenuGroup,
- DropdownMenuPortal,
- DropdownMenuSub,
- DropdownMenuSubContent,
- DropdownMenuSubTrigger,
- DropdownMenuRadioGroup,
-}
diff --git a/spaces/Benson/text-generation/Examples/Arquero Inactivo Torre De Defensa Mod Apk.md b/spaces/Benson/text-generation/Examples/Arquero Inactivo Torre De Defensa Mod Apk.md
deleted file mode 100644
index 7183b5fa59564a819b55eb31cc4545e4f0708212..0000000000000000000000000000000000000000
--- a/spaces/Benson/text-generation/Examples/Arquero Inactivo Torre De Defensa Mod Apk.md
+++ /dev/null
@@ -1,88 +0,0 @@
-
-
-
-
-
Idle Archer Tower Defense Mod APK: Un divertido y desafiante juego de rol
-
¿Estás buscando un juego de rol divertido y desafiante que te mantenga entretenido durante horas? ¿Quieres experimentar un emocionante juego de torre de defensa con increíbles gráficos y efectos de sonido? Si es así, entonces usted debe tratar Idle Archer Tower Defense Mod APK!
-
Idle Archer Tower Defense es un popular juego de rol que combina la defensa de la torre y elementos inactivos. Juegas como un arquero solitario que tiene que defender tu torre contra las olas de monstruos malvados invocados por el señor oscuro. Puedes mejorar tus habilidades de arquero, armas y torre para volverte más fuerte y derrotar a más enemigos. También puedes recoger monedas y gemas para desbloquear nuevos elementos y características.
Pero ¿y si quieres disfrutar del juego sin limitaciones ni restricciones? ¿Qué pasa si quieres tener recursos y potenciadores ilimitados para que tu juego sea más fácil y divertido? Bueno, ahí es donde Idle Archer Tower Defense Mod APK viene muy bien!
-
Idle Archer Tower Defense Mod APK es una versión modificada del juego original que le da acceso a varios beneficios y ventajas que no están disponibles en la versión regular. Usted puede disfrutar de características como el modo de dios, un golpe matar, monedas y gemas ilimitadas, y más. Puede descargar e instalar Idle Archer Tower Defense Mod APK gratis desde nuestro sitio web.
-
En este artículo, le diremos todo lo que necesita saber sobre Idle Archer Tower Defense Mod APK. Te explicaremos qué es, por qué deberías descargarlo, cómo descargarlo, cómo instalarlo y cómo jugarlo. También vamos a responder a algunas preguntas frecuentes sobre Idle Archer Tower Defense Mod APK.
-
Así que, sin más preámbulos, ¡empecemos!
-
¿Qué es Idle Archer Tower Defense? debilidades. También tienes que enfrentarte a jefes poderosos que pondrán a prueba tus habilidades y estrategia. Tienes que completar varias misiones y logros para ganar recompensas y desbloquear nuevas características.
-
-
El juego de Idle Archer Tower Defense
-
Cómo jugar Idle Archer Tower Defense
-
Jugar Idle Archer Tower Defense es fácil y agradable. Solo tienes que seguir estos sencillos pasos:
-
-
Descargar e instalar Idle Archer Tower Defense desde Google Play Store o desde nuestro sitio web.
-
Inicie el juego y elija su idioma preferido.
-
Ver el tutorial y aprender los fundamentos del juego.
-
Comienza el juego y defiende tu torre de los enemigos.
-
Toque en la pantalla para apuntar y disparar las flechas.
-
Desliza el dedo en la pantalla para mover el arquero y esquivar los ataques enemigos.
-
Usa hechizos, objetos y aliados para aumentar tu potencia y rendimiento.
-
Gana monedas y gemas matando enemigos y completando misiones.
-
Mejora tus habilidades, armas y torre en la tienda.
-
Progreso a través de las etapas y niveles del juego.
-
Disfruta de la historia, gráficos, sonido y jugabilidad del juego.
-
-
¿Cuáles son las características de Idle Archer Tower Defense
-
Idle Archer Tower Defense tiene muchas características que lo convierten en un juego de rol divertido y desafiante. Algunas de estas características son:
-
-
-
Una combinación única de defensa de torre y elementos inactivos.
-
Un sistema de control simple e intuitivo.
-
Un estilo gráfico colorido y caricaturesco.
-
Una banda sonora animada y pegadiza.
-
Una variedad de enemigos, jefes, escenarios y escenarios.
-
Un modo inactivo que te permite ganar monedas y gemas incluso cuando no estás conectado.
-
Una historia rica e inmersiva que te mantendrá enganchado y motivado para jugar.
-
Una tienda donde puedes comprar nuevos artículos y características.
-
Un sistema de misión que te recompensa por completar tareas y logros.
-
Un sistema de eventos que añade variedad y diversión al juego.
-
¿Por qué descargar Idle Archer Tower Defense Mod APK?
-
-
Es por eso que usted debe descargar Idle Archer Tower Defense Mod APK de nuestro sitio web. Idle Archer Tower Defense Mod APK es una versión modificada del juego original que le da acceso a varios beneficios y ventajas que no están disponibles en la versión regular. Usted puede disfrutar de características como el modo de dios, un golpe matar, monedas y gemas ilimitadas, y más. Puede descargar e instalar Idle Archer Tower Defense Mod APK gratis desde nuestro sitio web.
-
¿Qué es Idle Archer Tower Defense Mod APK?
-
Idle Archer Tower Defense Mod APK es una versión modificada del juego original que ha sido creado por desarrolladores de terceros. El archivo mod APK contiene algunos cambios y modificaciones que alteran el código y la funcionalidad del juego. El archivo APK mod le permite evitar el sistema de seguridad del juego y acceder a las características que normalmente están bloqueadas o restringidas.
-
Idle Archer Tower Defense Mod APK no es una versión oficial del juego. No está avalado o apoyado por los desarrolladores originales o editores del juego. No está disponible en Google Play Store ni en ninguna otra tienda de aplicaciones oficial. Solo está disponible en nuestro sitio web y en otras fuentes no oficiales.
-
¿Cuáles son los beneficios de Idle Archer Tower Defense Mod APK?
-
Idle Archer Tower Defense Mod APK tiene muchos beneficios que harán que su juego más fácil y divertido. Algunos de estos beneficios son:
-
Modo de Dios
-
El modo Dios es una característica que te hace invencible e inmune a cualquier daño. Puede activar el modo dios en Idle Archer Tower Defense Mod APK y disfrutar del juego sin preocuparse por morir o perder la salud. Puedes sobrevivir a cualquier ataque enemigo, lucha contra jefes o desafío de escenario con facilidad. También puedes explorar el mundo del juego sin ningún miedo o riesgo.
-
Matar con un solo golpe
-
-
Monedas y gemas ilimitadas
-
Monedas y gemas ilimitadas son características que te hacen rico e ingenioso. Puede activar monedas y gemas ilimitadas en Idle Archer Tower Defense Mod APK y disfrutar del juego sin gastar dinero real o moler duro. Puedes tener tantas monedas y gemas como quieras en tu cuenta. Puedes usarlos para comprar nuevos artículos, características, mejoras, habilidades, armas, torres, hechizos, aliados y más en la tienda. También puedes usarlos para desbloquear nuevas etapas, niveles, modos, eventos, escenarios, personajes y más en el juego.
Cómo descargar e instalar Idle Archer Tower Defense Mod APK?
-
Descargar e instalar Idle Archer Tower Defense Mod APK es fácil y simple. Solo tienes que seguir estos sencillos pasos:
-
Paso 1: Descargar el archivo APK
-
El primer paso es descargar el archivo APK de Idle Archer Tower Defense Mod APK de nuestro sitio web. Puede encontrar el enlace de descarga al final de este artículo. Solo tiene que hacer clic en el enlace y esperar a que comience la descarga. El tamaño del archivo es de unos 100 MB, así que asegúrese de tener suficiente espacio de almacenamiento y una conexión a Internet estable.
-
Paso 2: Habilitar fuentes desconocidas
-
El segundo paso es habilitar fuentes desconocidas en su dispositivo. Esta es una configuración de seguridad que le permite instalar aplicaciones desde fuentes distintas de Google Play Store u otras tiendas de aplicaciones oficiales. Es necesario habilitar esta configuración porque Idle Archer Tower Defense Mod APK no es una aplicación oficial y no está disponible en ninguna tienda de aplicaciones oficial.
-
Para habilitar fuentes desconocidas, debe ir a la configuración de su dispositivo y buscar la opción de seguridad o privacidad. Luego, debe encontrar la opción de fuentes desconocidas y activarla. Es posible que vea un mensaje de advertencia que indica que instalar aplicaciones de fuentes desconocidas puede dañar su dispositivo o datos. Puede ignorar este mensaje y proceder con la instalación.
-
Paso 3: Instalar el archivo APK
-
-
Paso 4: Disfruta del juego
-
El cuarto y último paso es disfrutar del juego. Puede iniciar el juego desde el cajón de aplicaciones de su dispositivo o la pantalla de inicio. También puede crear un icono de acceso directo en el escritorio para facilitar el acceso. Ahora puede disfrutar de todas las características y beneficios de Idle Archer Tower Defense Mod APK sin limitaciones o restricciones.
-
Conclusión
-
Idle Archer Tower Defense Mod APK es un divertido y desafiante juego de rol que combina la torre de defensa y elementos inactivos. Puedes defender tu torre de oleadas de enemigos, mejorar tus habilidades, armas y torre, recoger monedas y gemas, y disfrutar de una historia rica e inmersiva. También puede disfrutar de características como el modo de dios, un solo golpe matar, monedas y gemas ilimitadas, y más con Idle Archer Tower Defense Mod APK.
-
Si desea descargar e instalar Idle Archer Tower Defense Mod APK, puede seguir los pasos que hemos proporcionado en este artículo. También puede encontrar el enlace de descarga al final de este artículo. Puede descargar e instalar Idle Archer Tower Defense Mod APK gratis desde nuestro sitio web.
-
Esperamos que haya encontrado este artículo útil e informativo. Si tiene alguna pregunta o comentario sobre Idle Archer Tower Defense Mod APK, puede dejar un comentario a continuación. Intentaremos responder a sus preguntas lo antes posible.
-
Preguntas frecuentes
-
Aquí hay algunas preguntas frecuentes sobre Idle Archer Tower Defense Mod APK:
-
-
¿Es seguro usar Idle Archer Tower Defense Mod APK?
-
Sí, Idle Archer Tower Defense Mod APK es seguro de usar. No contiene ningún virus, malware o spyware que pueda dañar su dispositivo o datos. Tampoco requiere ningún acceso root o permisos especiales que puedan comprometer la seguridad o privacidad de su dispositivo.
-
¿Es Idle Archer Tower Defense Mod APK compatible con mi dispositivo?
-
-
¿Puedo jugar Idle Archer Tower Defense Mod APK en línea?
-
No, Idle Archer Tower Defense Mod APK es un juego sin conexión que no requiere una conexión a Internet para jugar. Sin embargo, es posible que necesite una conexión a Internet para descargar e instalar el juego, así como para acceder a algunas funciones en línea como actualizaciones, eventos o tablas de clasificación.
-
¿Puedo actualizar Idle Archer Tower Defense Mod APK?
-
Sí, puede actualizar Idle Archer Tower Defense Mod APK siempre que haya una nueva versión disponible en nuestro sitio web. Sin embargo, puedes perder parte de tu progreso o datos si actualizas el juego sin hacer una copia de seguridad.
-
¿Puedo jugar Idle Archer Tower Defense Mod APK con mis amigos?
-
No, Idle Archer Tower Defense Mod APK no tiene un modo multijugador o característica que le permite jugar con tus amigos. Sin embargo, puedes comparar tus puntajes y logros con otros jugadores en las tablas de clasificación o plataformas de redes sociales.
64aa2da5cf
-
-
\ No newline at end of file
diff --git a/spaces/Benson/text-generation/Examples/Descargar Conquista Paraso Riddim.md b/spaces/Benson/text-generation/Examples/Descargar Conquista Paraso Riddim.md
deleted file mode 100644
index 49b01698bd767e2339b4b86ab10bdf237cf56ff8..0000000000000000000000000000000000000000
--- a/spaces/Benson/text-generation/Examples/Descargar Conquista Paraso Riddim.md
+++ /dev/null
@@ -1,71 +0,0 @@
-
-
Cómo descargar Conquest Paradise Riddim
-
Si eres un fan de la música reggae, es posible que hayas oído hablar de Conquest Paradise Riddim, una compilación de canciones que comparten la misma pista instrumental o "riddim". Este riddim fue producido por Damage Musiq, un sello discográfico jamaicano especializado en dancehall y reggae. En este artículo, te mostraremos cómo descargar Conquest Paradise Riddim gratis o por un cargo, dependiendo de tu preferencia y presupuesto. También explicaremos qué hace que este riddim sea tan especial y por qué deberías añadirlo a tu biblioteca de música.
Un riddim es un término utilizado en la música jamaicana para describir un ritmo o pista instrumental que es utilizado por diferentes artistas para crear sus propias canciones. Un riddim puede ser original o muestreado de otra canción, y generalmente consiste en un patrón de batería, una línea de bajo y algunos otros elementos. Un riddim puede tener un tema o un estado de ánimo que refleje su nombre o productor.
-
Conquest Paradise Riddim es un riddim que fue lanzado en febrero de 2019 por Damage Musiq, un sello discográfico fundado por Dwayne Parkinson, también conocido como Damage. Damage Musiq es conocido por producir riddims de alta calidad que cuentan con algunos de los mejores artistas de dancehall y reggae en Jamaica y más allá. Algunos de sus riddims anteriores incluyen Holocaust Riddim, Levels Riddim, Rope Riddim y Dark Temptation Riddim.
-
Los artistas y canciones que aparecen en el riddim
-
Conquest Paradise Riddim cuenta con 10 canciones de 9 artistas diferentes, incluyendo algunos de los nombres más populares en la escena reggae. Las canciones son las siguientes:
-
-
-
Artista
Canción
-
Tommy Lee Sparta
Bendiciones
-
Ley Crónica
Bendíceme
-
Shane O
Mantenga pulsado
-
Shatta Wale
Hola
-
Singer J
Pray
-
Tamo J
Victoria
-
-
Jah Vinci
Guerra de lucha
-
Jahmiel
Mankind
-
Damage Musiq
Conquest Paradise Riddim (Instrumental)
-
-
Las canciones de Conquest Paradise Riddim son en su mayoría positivas y edificantes, con temas como la gratitud, la perseverancia, la fe, el éxito y el amor. El riddim tiene un sonido suave y melódico, con un riff de guitarra pegadizo, un bajo pulsante y un ritmo de batería constante. El riddim es adecuado para relajarse y bailar, y muestra la versatilidad y el talento de los artistas y el productor.
-
¿Por qué descargar Conquest Paradise Riddim?
-
Los beneficios de poseer tu música
-
Download Conquest Paradise Riddim es una gran manera de disfrutar de su música en cualquier momento y en cualquier lugar, sin depender de la conexión a Internet o servicios de transmisión. Al descargar el riddim, puedes tener control total sobre tu biblioteca de música, y puedes crear tus propias listas de reproducción, mixtapes o álbumes. También puede compartir su música con sus amigos y familiares, o reproducirla en cualquier dispositivo o altavoz que desee. Descargar tu música también significa que puedes apoyar a los artistas y al productor directamente, mostrándoles tu aprecio y lealtad.
-
La calidad y variedad del riddim
-
Otra razón para descargar Conquest Paradise Riddim es que es un riddim de alta calidad y diversa que satisfará a cualquier amante del reggae. El riddim tiene un sonido nítido y claro, sin distorsión ni ruido. Las canciones están bien producidas y bien mezcladas, con voces e instrumentos equilibrados. El riddim también ofrece una variedad de estilos y vibraciones, desde las canciones inspiradoras y motivadoras de Tommy Lee Sparta, Chronic Law, Shane O, Singer J, Tamo J y Gott Yo, hasta las canciones románticas y sensuales de Shatta Wale y Jah Vinci, y las canciones conscientes y sociales de Jahmiel. Hay algo para todos en Conquest Paradise Riddim, y no te aburrirás de escucharlo.
-
-
Las cuestiones legales y éticas de descargar música gratuita
-
Antes de mostrarle cómo descargar Conquest Paradise Riddim gratis, tenemos que advertirle sobre los problemas legales y éticos de hacerlo. Descargar música gratis de fuentes no autorizadas se considera piratería, que es una forma de robo que viola los derechos de propiedad intelectual de los artistas y el productor. La piratería puede tener consecuencias negativas para la industria musical, como una reducción de los ingresos, una menor calidad, menos innovación y menos incentivos para que surjan nuevos artistas. La piratería también puede exponerlo a malware, virus, spyware u otro software dañino que puede dañar su dispositivo o comprometer su privacidad. Por lo tanto, no recomendamos ni aprobamos la descarga de música gratuita de fuentes ilegales, y le aconsejamos que lo haga bajo su propio riesgo.
-
Los sitios web y aplicaciones que ofrecen descargas gratuitas del riddim
-
Si todavía quieres descargar Conquest Paradise Riddim gratis, a pesar de los riesgos que implica, hay algunos sitios web y aplicaciones que afirman ofrecer descargas gratuitas de la riddim. Sin embargo, no podemos garantizar su seguridad, fiabilidad o calidad. Algunos de estos sitios web y aplicaciones son:
-
-
Reggae4us.com: Este sitio web afirma ser "el destino online definitivo para los amantes de la música reggae". Ofrece descargas gratuitas de varias canciones de reggae y álbumes, incluyendo Conquest Paradise Riddim. Sin embargo, el sitio web tiene muchos anuncios emergentes y redirecciones que pueden ser molestos o peligrosos.
-
Audiomack.com: Este sitio web es una plataforma para que los artistas suban su música y la compartan con sus fans. También permite a los usuarios transmitir o descargar música de forma gratuita. Sin embargo, no todas las canciones de Conquest Paradise Riddim están disponibles en este sitio web, y algunas de ellas tienen versiones de baja calidad o incompletas.
-
-
Cómo descargar Conquest Paradise Riddim por una tarifa?
-
Las ventajas de comprar música online
-
Si desea descargar Conquest Paradise Riddim legal y éticamente, puede optar por comprarlo en línea de fuentes autorizadas. Comprar música online tiene muchas ventajas, como:
-
-
Puedes apoyar financieramente a los artistas y al productor, y mostrarles tu respeto y aprecio por su trabajo.
-
Puede obtener la mejor calidad y la versión completa de la riddim, sin interrupciones o errores.
-
Puede acceder a su música en cualquier momento y en cualquier lugar, sin depender de la conexión a Internet o servicios de streaming.
-
Puedes disfrutar de tu música sin anuncios, pop-ups, redirecciones o malware.
-
Puedes obtener características o beneficios adicionales, como letras, ilustraciones, reseñas, recomendaciones o descuentos.
-
-
Las plataformas y servicios que venden el riddim
-
Hay muchas plataformas y servicios que venden Conquest Paradise Riddim en línea, por un precio razonable. Algunas de estas plataformas y servicios son:
-
-
iTunes: Esta es una de las plataformas más populares y confiables para comprar música en línea. Puedes comprar Conquest Paradise Riddim por $9.99 USD, o canciones individuales por $0.99 USD cada una. También puede transmitir el riddim en Apple Music, si tiene una suscripción.
-
Amazon Music: Esta es otra plataforma conocida y confiable para comprar música en línea. Puedes comprar Conquest Paradise Riddim por $8.99 USD, o canciones individuales por $0.99 USD cada una. También puede transmitir el riddim en Amazon Music Unlimited, si tiene una suscripción.
-
Spotify: Esta es una de las plataformas más populares y ampliamente utilizadas para la transmisión de música en línea. Puedes transmitir Conquest Paradise Riddim en Spotify de forma gratuita, con anuncios o con una suscripción premium, sin anuncios. También puede descargar el riddim en Spotify Premium, para escuchar sin conexión.
-
-
-
Un resumen de los puntos principales y una llamada a la acción
-
En conclusión, Conquest Paradise Riddim es un imprescindible para cualquier amante de la música reggae. Es un riddim de alta calidad y diversa que cuenta con algunos de los mejores artistas y canciones del género. También es un riddim positivo y edificante que te inspirará y te hará sentir bien. Puedes descargar Conquest Paradise Riddim gratis o por una tarifa, dependiendo de tu preferencia y presupuesto. Sin embargo, le recomendamos que compre el riddim en línea de fuentes autorizadas, para apoyar a los artistas y al productor, y para disfrutar de la mejor calidad y experiencia. Entonces, ¿qué estás esperando? Descargar Conquest Paradise Riddim hoy y disfrutar de las vibraciones!
-
Preguntas frecuentes
-
¿Qué es un riddim?
-
Un riddim es un término utilizado en la música jamaicana para describir un ritmo o pista instrumental que es utilizado por diferentes artistas para crear sus propias canciones.
-
¿Qué es la música reggae?
-
El reggae es un género de música que se originó en Jamaica a finales de los años 60. Se caracteriza por su ritmo distintivo, línea de bajo, acordes de guitarra, voces y letras. La música reggae a menudo expresa mensajes sociales y políticos, así como valores culturales y espirituales.
-
¿Cómo puedo reproducir la música descargada en mi dispositivo?
-
Puede reproducir la música descargada en su dispositivo mediante el uso de cualquier aplicación de reproductor de medios que soporta mp3 u otros formatos de audio. También puede transferir la música descargada a otros dispositivos o altavoces a través de Bluetooth, USB o Wi-Fi.
-
¿Cómo puedo apoyar a los artistas y productores del riddim?
-
Puedes apoyar a los artistas y productores del riddim comprando su música en línea de fuentes autorizadas, transmitiendo su música en plataformas legales, siguiéndola en redes sociales, compartiendo su música con tus amigos y familiares, asistiendo a sus conciertos o eventos, o enviándoles comentarios o donaciones.
File Activation.xml Autocom Version 2.12.2 Keygen: How to Use It and Why You Should Avoid It
-
-
Autocom version 2.12.2 is a software tool that enables you to diagnose and program various vehicles using a computer and a compatible interface. It supports different protocols and interfaces, such as OBD-II, EOBD, CAN, J1850, ISO9141, KWP2000 and more. Autocom version 2.12.2 is the latest release that offers improved features and performance.
-
-
However, to use Autocom version 2.12.2, you need to activate it with a license or subscription that you can purchase from the official website or from an authorized dealer. Alternatively, you may have found a keygen that can generate a file activation.xml for you. A file activation.xml is a XML file that contains the information needed to activate Autocom version 2.12.2, such as the hardware key, the software version, the license type and the expiration date.
-
File Activation.xml Autocom Version 2.12.2 Keygenl
In this article, we will show you how to use a keygen for Autocom version 2.12.2 and why you should avoid it.
-
-
How to use a keygen for Autocom version 2.12.2
-
-
To use a keygen for Autocom version 2.12.2, you need to follow these steps:
-
-
-
Download the Autocom version 2.12.2 software from the official website or from a trusted source.
-
Install the software on your computer following the instructions.
-
Download the keygen for Autocom version 2.12.2 from this link or from another source.
-
Extract the keygen file and run it as administrator.
-
Select the software version (Autocom 2014.R3) and the hardware key (100251) from the drop-down menus.
-
Click on the "Generate" button and copy the activation code.
-
Run the Autocom version 2.12.2 software and click on the "Activate via USB" button.
-
Paste the activation code in the box and click on the "OK" button.
-
The software will create a file named FileActivation.xml in the installation folder.
-
Copy this file to a USB flash drive and insert it into your diagnostic device.
-
The device will read the file and activate the software.
-
You can now use Autocom version 2.12.2 with full functionality.
-
-
-
Why you should avoid using a keygen for Autocom version 2.12.2
-
-
A keygen for Autocom version 2.12.2 is not a safe or legal way to activate the software. Here are some reasons why you should avoid using it:
-
-
-
A keygen can contain viruses, malware or spyware that can harm your computer or steal your personal information.
-
A keygen can violate the intellectual property rights of the software developer and expose you to legal risks.
-
A keygen can result in an unstable or corrupted software that can cause errors or damage your vehicle.
-
A keygen can make you ineligible for any updates or support from the software developer.
-
-
-
The only safe and legal way to activate Autocom version 2.12.2 is to purchase a license or subscription from the official website or from an authorized dealer. This way, you can get access to all the features and updates of the software and enjoy its full functionality.
-
-
Conclusion
-
-
In this article, we have shown you how to use a keygen for Autocom version 2.12.2 and why you should avoid it. A keygen is not a safe or legal way to activate a software tool that can help you diagnose and program various vehicles using a computer and a compatible interface. We advise you to buy a genuine license or subscription for Autocom version 2.12.2 from the official website or from an authorized dealer.
-
How to Use Autocom Version 2.12.2 for Vehicle Diagnosis and Programming
-
-
Once you have activated Autocom version 2.12.2 with a keygen or a license, you can use it to diagnose and program various vehicles using a computer and a compatible interface. Here are some steps to use Autocom version 2.12.2 for vehicle diagnosis and programming:
-
-
-
Connect your diagnostic device to your computer using a USB cable or a wireless connection.
-
Connect your diagnostic device to your vehicle using the appropriate connector or adapter.
-
Run the Autocom version 2.12.2 software on your computer and select the vehicle make, model and year from the menu.
-
Select the function you want to perform, such as reading fault codes, clearing fault codes, reading live data, performing tests, programming modules, resetting service intervals, etc.
-
Follow the instructions on the screen and wait for the software to communicate with the vehicle.
-
View the results on the screen and save or print them if needed.
-
-
-
What are the Advantages and Disadvantages of Autocom Version 2.12.2
-
-
Autocom version 2.12.2 is a software tool that has many advantages and disadvantages for vehicle diagnosis and programming. Here are some of them:
-
-
-
Advantages:
-
-
-
Autocom version 2.12.2 supports a wide range of vehicles, protocols and interfaces, making it compatible with most cars and trucks on the market.
-
Autocom version 2.12.2 offers many functions and features, such as reading and clearing fault codes, reading live data, performing tests, programming modules, resetting service intervals, etc.
-
Autocom version 2.12.2 has a user-friendly interface and easy-to-follow instructions, making it suitable for both professionals and amateurs.
-
Autocom version 2.12.2 has a fast and reliable communication speed, ensuring a smooth and accurate diagnosis and programming process.
-
-
-
Disadvantages:
-
-
-
Autocom version 2.12.2 requires activation with a keygen or a license, which can be unsafe or illegal to use.
-
Autocom version 2.12.2 may not support some newer or specific vehicles or functions, depending on the software update or the hardware compatibility.
-
Autocom version 2.12.2 may cause errors or damage to your vehicle if used incorrectly or with a faulty interface or connector.
-
Autocom version 2.12.2 may not be eligible for any updates or support from the software developer if activated with a keygen.
-
-
-
Conclusion
-
-
In this article, we have shown you how to use a keygen for Autocom version 2.12.2, how to use Autocom version 2.12.2 for vehicle diagnosis and programming, and what are the advantages and disadvantages of Autocom version 2.12.2. We hope this article has been helpful for you to understand more about this software tool and how to use it effectively.
-
Where to Download Autocom Version 2.12.2 and Keygen
-
-
If you want to download Autocom version 2.12.2 and keygen, you need to be careful about the source and the quality of the files. There are many websites that claim to offer free or cracked versions of Autocom version 2.12.2 and keygen, but they may be fake, infected or corrupted.
-
-
The best way to download Autocom version 2.12.2 and keygen is to use the official website or a trusted source that has positive reviews and feedback from other users. You can also use a reliable antivirus software and a VPN service to protect your computer and your privacy from any potential threats.
-
-
Here are some links that you can use to download Autocom version 2.12.2 and keygen:
If you have activated Autocom version 2.12.2 with a keygen, you may not be able to update it to the latest version or get any support from the software developer. This can limit your access to new features, functions and vehicles that are added in the newer versions of the software.
-
-
If you want to update Autocom version 2.12.2, you need to purchase a license or subscription from the official website or from an authorized dealer. This way, you can get access to all the updates and support that are available for the software.
-
-
To update Autocom version 2.12.2, you need to follow these steps:
-
-
-
Purchase a license or subscription from the official website or from an authorized dealer.
-
Download the latest version of the software from the official website or from the link provided by the dealer.
-
Install the latest version of the software on your computer following the instructions.
-
Run the latest version of the software and enter your license or subscription details.
-
The software will automatically update itself and activate your license or subscription.
-
You can now use Autocom version 2.12.2 with all the updates and support.
-
-
-
Conclusion
-
-
In this article, we have shown you how to use a keygen for Autocom version 2.12.2, how to use Autocom version 2.12.2 for vehicle diagnosis and programming, what are the advantages and disadvantages of Autocom version 2.12.2, where to download Autocom version 2.12.2 and keygen, and how to update Autocom version 2.12.2. We hope this article has been helpful for you to understand more about this software tool and how to use it effectively.
-
How to Choose the Best Interface for Autocom Version 2.12.2
-
-
To use Autocom version 2.12.2 for vehicle diagnosis and programming, you need to have a compatible interface that can connect your computer and your vehicle. There are many types and models of interfaces available on the market, but not all of them are suitable for Autocom version 2.12.2.
-
-
To choose the best interface for Autocom version 2.12.2, you need to consider these factors:
-
-
-
The compatibility of the interface with the software and the vehicle. You need to check if the interface supports the software version, the hardware key, the protocols and the functions that you want to use.
-
The quality and reliability of the interface. You need to check if the interface is well-made, durable and stable. You also need to check if the interface has any warranty or support from the manufacturer or seller.
-
The price and availability of the interface. You need to compare the prices and features of different interfaces and choose the one that offers the best value for money. You also need to check if the interface is in stock and can be delivered quickly.
-
-
-
Some of the most popular and recommended interfaces for Autocom version 2.12.2 are:
How to Get Help and Support for Autocom Version 2.12.2
-
-
If you have any questions or problems with Autocom version 2.12.2, you can get help and support from various sources:
-
-
-
The official website of Autocom: https://www.autocom.se/en/. Here you can find information about the software, the products, the dealers, the updates and more.
-
The official forum of Autocom: https://forum.autocom.se/. Here you can join the community of Autocom users and experts, ask questions, share experiences, get tips and advice.
-
The online manuals of Autocom: https://www.autocom.se/en/support/manuals/. Here you can find detailed instructions on how to install, activate, use and troubleshoot Autocom version 2.12.2.
In this article, we have shown you how to use a keygen for Autocom version 2.12.2, how to use Autocom version 2.12.2 for vehicle diagnosis and programming, what are the advantages and disadvantages of Autocom version 2.12.2, where to download Autocom version 2.12.2 and keygen, how to update Autocom version 2.12.2, how to choose the best interface for Autocom version 2.12.2, and how to get help and support for Autocom version 2.12.2. We hope this article has been helpful for you to understand more about this software tool and how to use it effectively.
-
Conclusion
-
-
In this article, we have shown you how to use a keygen for Autocom version 2.12.2, how to use Autocom version 2.12.2 for vehicle diagnosis and programming, what are the advantages and disadvantages of Autocom version 2.12.2, where to download Autocom version 2.12.2 and keygen, how to update Autocom version 2.12.2, how to choose the best interface for Autocom version 2.12.2, and how to get help and support for Autocom version 2.12.2. We hope this article has been helpful for you to understand more about this software tool and how to use it effectively.
3cee63e6c2
-
-
\ No newline at end of file
diff --git a/spaces/falterWliame/Face_Mask_Detection/Jet Li Enforcer 720p Torrent.md b/spaces/falterWliame/Face_Mask_Detection/Jet Li Enforcer 720p Torrent.md
deleted file mode 100644
index 3674db63c8c3738f451dc7f182e10bbdc50c3806..0000000000000000000000000000000000000000
--- a/spaces/falterWliame/Face_Mask_Detection/Jet Li Enforcer 720p Torrent.md
+++ /dev/null
@@ -1,6 +0,0 @@
-
-
- d5da3c52bf
-
-
-
diff --git a/spaces/fatiXbelha/sd/Download Minecraft 1.19.0.30 for Free and Enjoy the Spectator Mode and Other Features.md b/spaces/fatiXbelha/sd/Download Minecraft 1.19.0.30 for Free and Enjoy the Spectator Mode and Other Features.md
deleted file mode 100644
index bdeff196cdea812d19b33c7fd8179b42e598655f..0000000000000000000000000000000000000000
--- a/spaces/fatiXbelha/sd/Download Minecraft 1.19.0.30 for Free and Enjoy the Spectator Mode and Other Features.md
+++ /dev/null
@@ -1,64 +0,0 @@
-
-
How to Download Minecraft 1.19.0.30 for Free - The Latest Version of Bedrock Edition
-
Minecraft is one of the most popular and creative games in the world, with millions of players exploring, building, and surviving in infinite worlds. If you are a fan of Minecraft, you might be interested in downloading the latest version of Bedrock Edition, which is 1.19.0.30.
-
Minecraft 1.19.0.30 is a beta version that introduces some exciting new features, such as a new viewer mode, a new biome called mangrove swamps, and a new mob called warden. In this article, we will show you how to download Minecraft 1.19.0.30 for free with a working Xbox Live, and what's new in this version.
Minecraft 1.19.0.30 is a beta version that is part of the upcoming 1.19 update, also known as The Wild Update. This update aims to add more diversity and life to the game, with new biomes, mobs, blocks, items, and mechanics.
-
To download Minecraft 1.19.0.30 for free, you need to have an Android device and join the beta program for Minecraft Bedrock Edition on Google Play Store. You also need to have an Xbox Live account to play online with other players. If you don't have one, you can create one for free on the official website. Once you have joined the beta program and signed in with your Xbox Live account, you can download Minecraft 1.19.0.30 from the Google Play Store and enjoy the new features.
-
What's New in Minecraft 1.19.0.30?
-
Minecraft 1.19.0.30 adds some new features that are still under development and may change or be removed in future versions. Here are some of the most notable ones:
-
New Viewer Mode
-
Viewer mode is a new feature that allows you to explore the world without affecting it or being affected by it. You can activate viewer mode by pressing F5 on your keyboard or tapping on the eye icon on your screen. In viewer mode, you can fly around, see through walls, and observe everything without being noticed by mobs or triggering traps. You can also switch between different perspectives by pressing F5 again or tapping on the eye icon again.
-
Viewer mode affects sculk sensors and tripwire traps differently than normal mode. Sculk sensors are blocks that detect vibrations and emit redstone signals. In normal mode, sculk sensors will detect any vibration caused by you or other entities, such as walking, jumping, placing blocks, breaking blocks, etc. In viewer mode, however , sculk sensors will not detect any vibration caused by you, but they will still detect vibrations caused by other entities. This means you can sneak past sculk sensors without alerting them, but you can also observe how other entities interact with them. Tripwire traps are devices that consist of tripwire hooks and string. In normal mode, tripwire traps will activate when you or other entities cross the string, triggering redstone signals or dispensers. In viewer mode, tripwire traps will not activate when you cross the string, but they will still activate when other entities cross the string. This means you can avoid triggering tripwire traps yourself, but you can also watch how other entities trigger them.
-
Mangrove Swamps
-
Mangrove swamps are a new biome that can be found in warm ocean regions. They are characterized by shallow water, muddy soil, and mangrove trees. Mangrove trees are a new type of tree that have roots that grow above the water and branches that hang down. They can be grown on mud or dirt with water nearby, and they drop mangrove saplings and mangrove planks when broken. Mangrove planks are a new type of wood that can be used to craft various items and blocks, such as boats, doors, fences, etc. Mud is a new type of block that can be found in mangrove swamps and other wet areas. It has a brown color and a sticky texture, and it slows down entities that walk on it. It can also be used to craft mud bricks, which are a new type of building block.
-
Warden
-
Warden is a new mob that can be found in the deep dark, a new underground biome that is part of the upcoming 1.18 update. Warden is a large, blind creature that has a lot of health and deals a lot of damage. It uses its ears to detect vibrations and track down its prey. It can sense any movement or sound within a 64-block radius, and it will attack anything that makes noise or moves near it. Warden can also break through most blocks to reach its target, so hiding behind walls is not very effective.
-
To fight warden, you need to be very careful and strategic. You can use stealth and distraction to avoid or confuse it. For example, you can use viewer mode to fly around without making noise or vibrations. You can also use sculk sensors or wool occlusion to block its hearing or create false signals. You can also use snowballs, arrows, eggs, or other projectiles to divert its attention or lure it away from you. You can also use sculk catalysts to temporarily disable its hearing. Sculk catalysts are a new type of item that can be crafted from sculk shards and copper ingots. They emit a purple light and a high-pitched sound when activated by redstone signals. When placed near warden, they will make it deaf for a few seconds, giving you a chance to escape or attack.
-
minecraft pe 1.19 0 30 apk download
-minecraft bedrock edition 1.19 0 30 beta download
-minecraft 1.19 0 30 free download for android
-minecraft 1.19 0 30 spectator mode download
-minecraft preview 1.19 0 31 download
-minecraft 1.19 the wild update download
-minecraft pe 1.19 0 30 xbox live download
-minecraft bedrock edition 1.19 0 30 features
-minecraft 1.19 0 30 warden download
-minecraft preview 1.19 pre-release download
-minecraft pe 1.19 mangrove swamp download
-minecraft bedrock edition beta retiring soon
-minecraft 1.19 sculk catalyst download
-minecraft preview game pass download
-minecraft pe 1.19 allay download
-minecraft bedrock edition beta vs preview
-minecraft 1.19 viewer mode download
-minecraft preview windows central review
-minecraft pe 1.19 mud block download
-minecraft bedrock edition beta changelog
-minecraft 1.19 tripwire trap fix download
-minecraft preview installation guide
-minecraft pe 1.19 bonfire and smokehouse download
-minecraft bedrock edition beta feedback
-minecraft 1.19 rtx worlds update download
-minecraft preview mojang studios blog
-minecraft pe 1.19 sculk sensor download
-minecraft bedrock edition beta bugs and issues
-minecraft 1.19 net energy gain experiment download
-minecraft preview java edition comparison
-
Conclusion
-
Minecraft 1.19.0.30 is a beta version that offers a glimpse of the upcoming 1.19 update, The Wild Update. It adds some new features that enhance the gameplay and the immersion of the game, such as viewer mode, mangrove swamps, and warden. If you want to try out these features for yourself, you can download Minecraft 1.19.0.30 for free with a working Xbox Live account on your Android device. Just remember that this is a beta version and it may have bugs or glitches, so make sure to back up your worlds before playing.
-
We hope you enjoyed this article and learned something new about Minecraft 1.19.0.30. If you have any questions or feedback, feel free to leave them in the comments section below.
-
FAQs
-
Is Minecraft 1.19.0.30 compatible with older versions?
-
No, Minecraft 1.19.0.30 is not compatible with older versions of the game. You can only play with other players who have the same version as you.
-
How can I join the beta program for Minecraft Bedrock Edition?
-
To join the beta program for Minecraft Bedrock Edition on Android devices, you need to follow these steps: - Open the Google Play Store app on your device. - Search for Minecraft and select it. - Scroll down to the bottom of the page and tap on "Join the beta". - Wait for the update to download and install. - Launch Minecraft and sign in with your Xbox Live account.
-
What are the system requirements for Minecraft 1.19.0.30?
-
The system requirements for Minecraft 1.19.0.30 are the same as for Minecraft Bedrock Edition in general. You need to have an Android device that runs on Android 5.0 or higher, with at least 1 GB of RAM and 300 MB of free storage space. You also need to have a stable internet connection and an Xbox Live account to play online.
-
How can I report bugs or give feedback for Minecraft 1.19.0.30?
-
If you encounter any bugs or glitches while playing Minecraft 1.19.0.30, you can report them on the official bug tracker. You can also give feedback or suggestions for the game on the official feedback site. Your input is valuable and helps the developers improve the game.
-
When will Minecraft 1.19.0.30 be released for other platforms?
-
Minecraft 1.19.0.30 is currently only available for Android devices as a beta version. There is no official release date for other platforms, such as Windows 10, iOS, Xbox, PlayStation, Nintendo Switch, etc. However, you can expect the final version of Minecraft 1.19 to be released for all platforms sometime in 2024.
401be4b1e0
-
-
\ No newline at end of file
diff --git a/spaces/fatiXbelha/sd/Download Monoposto 2023 Full Version in Minutes with This Simple Trick.md b/spaces/fatiXbelha/sd/Download Monoposto 2023 Full Version in Minutes with This Simple Trick.md
deleted file mode 100644
index 901f33cb318c3a33feb6ceb7b44533fc337b2264..0000000000000000000000000000000000000000
--- a/spaces/fatiXbelha/sd/Download Monoposto 2023 Full Version in Minutes with This Simple Trick.md
+++ /dev/null
@@ -1,122 +0,0 @@
-
-
Download Monoposto 2023 Full Version
-
If you are a fan of racing games, especially single seater open-wheel cars, you might have heard of Monoposto, an amazing independent racing game developed by Marco Pesce. The game has been around since 2017, but the latest version, Monoposto 2023, is the most exciting one yet. In this article, we will tell you what Monoposto 2023 is, what features it has, why you should download the full version, and how to do it.
-
What is Monoposto 2023?
-
Monoposto 2023 is a racing game that simulates the experience of driving a single seater open-wheel car, similar to Formula One. You can compete in the new 2023 season, with 24 racing tracks based on reality. You can also challenge other players online in multiplayer duels, or enjoy a quick race, a single race, or a championship mode. The game has realistic physics, graphics, and sounds, as well as dynamic weather conditions that affect the performance of your car. You can also customize your car and driver, and adjust your car setup before and during the race.
Monoposto 2023 has many features that make it one of the best racing games on the market. Here are some of them:
-
-24 realistic tracks
-
The game has 24 racing tracks that are based on real circuits from around the world. You can race on famous tracks like Monaco, Silverstone, Spa-Francorchamps, Suzuka, and more. Each track has its own characteristics, such as curves, straights, elevation changes, and weather conditions. You can also choose the time of day and the number of laps for each race.
-
-Online multiplayer duel
-
If you want to test your skills against other players, you can join the online multiplayer mode. You can challenge anyone who is online, or invite your friends to a private duel. You can also see your ranking and statistics on the leaderboard. The online mode is fast and smooth, with no lag or connection issues.
-
-Qualifying session and dynamic weather
-
Before each race, you can participate in a qualifying session to determine your starting position on the grid. The qualifying session is timed and you have to complete at least one lap to qualify. The faster you are, the better your position will be. You can also pit during the qualifying session to change your tires or repair your car.
-
The weather conditions can change during the race, affecting your visibility and grip. You can choose from sunny, cloudy, rainy, or stormy weather. The weather can also vary from track to track, adding more realism and challenge to the game.
-
-Pit stop and car setup
-
During the race, you can pit to change your tires or repair your car. Depending on the weather and the track conditions, you can choose from different types of tires: soft, medium, hard, wet, or intermediate. You can also adjust your car setup before and during the race, such as the wing angle, brake balance, suspension stiffness, gear ratio, fuel load, and engine power.
-Customization of cars and drivers
-
You can customize your car and driver to suit your preferences. You can choose from different colors, designs, stickers, and sponsors for your car. You can also change the name, number, helmet, and suit of your driver. You can save your customizations and use them in any mode of the game.
-
-Spectator TV mode race view
-
If you want to watch the race from a different perspective, you can switch to the spectator TV mode race view. This mode allows you to see the race from various camera angles, such as the cockpit, the front wing, the rear wing, the side pod, the overhead, and the broadcast. You can also see the live timing, the lap chart, the standings, and the telemetry of each car.
-
How to download monoposto 2023 full version for free
-Monoposto 2023 full version apk download
-Download monoposto 2023 full version for android
-Monoposto 2023 full version pc download
-Download monoposto 2023 full version mod apk
-Monoposto 2023 full version crack download
-Download monoposto 2023 full version for ios
-Monoposto 2023 full version online multiplayer download
-Download monoposto 2023 full version with all tracks
-Monoposto 2023 full version torrent download
-Download monoposto 2023 full version latest update
-Monoposto 2023 full version game download
-Download monoposto 2023 full version for windows 10
-Monoposto 2023 full version hack download
-Download monoposto 2023 full version for mac
-Monoposto 2023 full version cheats download
-Download monoposto 2023 full version for chromebook
-Monoposto 2023 full version review and download
-Download monoposto 2023 full version from google play store
-Monoposto 2023 full version tips and tricks download
-Download monoposto 2023 full version from official website
-Monoposto 2023 full version gameplay and download
-Download monoposto 2023 full version without ads
-Monoposto 2023 full version best settings download
-Download monoposto 2023 full version for linux
-Monoposto 2023 full version system requirements download
-Download monoposto 2023 full version for amazon fire tablet
-Monoposto 2023 full version controller support download
-Download monoposto 2023 full version for smart tv
-Monoposto 2023 full version graphics comparison download
-Download monoposto 2023 full version with custom cars and drivers
-Monoposto 2023 full version soundtrack download
-Download monoposto 2023 full version with pit stop and car repair features
-Monoposto 2023 full version bugs and fixes download
-Download monoposto 2023 full version with spectator tv mode race view
-Monoposto 2023 full version achievements and trophies download
-Download monoposto 2023 full version with weather effects and rain droplets on visor
-Monoposto 2023 full version leaderboard and rankings download
-Download monoposto 2023 full version with voice chat and text chat options
-Monoposto 2023 full version customization and personalization download
-
Why download Monoposto 2023 full version?
-
Monoposto 2023 is a great game that offers a lot of fun and challenge for racing fans. However, if you want to enjoy the game to the fullest, you should download the full version. Here are some reasons why:
-
-Full unlocked game with no ads
-
The full version of Monoposto 2023 gives you access to all the features and modes of the game, without any limitations or restrictions. You can play any track, any mode, any car, and any customization that you want. You also don't have to worry about annoying ads or in-app purchases that interrupt your gameplay.
-
-External and MFi game controller support
-
If you want to have a more immersive and realistic experience, you can use an external or MFi game controller to play Monoposto 2023. The game supports most Bluetooth controllers that are compatible with Android or iOS devices. You can also adjust the sensitivity and calibration of your controller in the settings menu.
-
-Podium celebrations and improved graphics
-
The full version of Monoposto 2023 also features podium celebrations after each race. You can see your driver celebrating with champagne and trophies on the podium, along with your team and rivals. The game also has improved graphics and animations, with more details and effects on the cars, tracks, and environments.
-
How to download Monoposto 2023 full version?
-
There are two ways to download Monoposto 2023 full version: from Google Play Store or App Store, or from other sources. Here are the steps for each method:
-
Download from Google Play Store or App Store
-
This is the easiest and safest way to download Monoposto 2023 full version. All you need is a compatible Android or iOS device and an internet connection. Here are the steps:
-
-Steps to download and install the game
-
-
Open Google Play Store or App Store on your device.
-
Search for Monoposto 2023 in the search bar.
-
Select the game from the list of results and tap on it.
-
Tap on the Install or Get button to start downloading the game.
-
Wait for the download to finish and then tap on Open or Play to launch the game.
-
Enjoy Monoposto 2023 full version!
-
-
-Price and app size
-
The price of Monoposto 2023 full version is $2.99 on both Google Play Store and App Store. The app size is about 300 MB on Android devices and about 400 MB on iOS devices. You may need additional storage space for updates and data files.
-
Download from other sources
-
This is an alternative way to download Monoposto 2023 full version, but it is not recommended. This method involves downloading the game from third-party websites that may not be secure or reliable. You may also encounter problems with compatibility, performance, or legality. Here are some risks and precautions of downloading from other sources:
-
-Risks and precautions of downloading from third-party websites
-
-
You may download a fake or modified version of the game that contains malware, viruses, or spyware that can harm your device or steal your personal information.
-
You may violate the terms of service or intellectual property rights of the developer or publisher of the game by downloading an unauthorized copy of the game.
-
You may not receive updates or support from the developer or publisher of the game if you download from other sources.
-
You may need to enable unknown sources or sideloading on your device settings to install apps from other sources. This can expose your device to security risks.
-
You may need to use a VPN or proxy to access the websites that offer the game for download, as they may be blocked or restricted in your region.
-
You may need to verify or activate the game using a code or a crack that may not work or may be illegal.
-
-
If you still want to download Monoposto 2023 full version from other sources, you should do it at your own risk and responsibility. Here are the steps:
-
-Steps to download and install the game from other sources
-
-
Find a website that offers Monoposto 2023 full version for download. You can use a search engine or a forum to look for such websites.
-
Check the reviews, ratings, and comments of the website and the game to make sure they are trustworthy and safe.
-
Download the game file from the website. It may be in the form of an APK file for Android devices or an IPA file for iOS devices.
-
Transfer the game file to your device using a USB cable, Bluetooth, or Wi-Fi.
-
Enable unknown sources or sideloading on your device settings to allow the installation of apps from other sources.
-
Locate the game file on your device and tap on it to start the installation process.
-
Follow the instructions on the screen to complete the installation.
-
Launch the game and enjoy Monoposto 2023 full version!
-
-
Conclusion
-
Monoposto 2023 is an awesome racing game that lets you drive a single seater open-wheel car on realistic tracks. The game has many features that make it fun and challenging, such as online multiplayer duel, qualifying session, dynamic weather, pit stop, car setup, and customization. You can download Monoposto 2023 full version from Google Play Store or App Store for $2.99, or from other sources for free. However, we recommend downloading from official sources to avoid any risks or problems. We hope this article helped you learn more about Monoposto 2023 and how to download it. Happy racing!
-
FAQs
-
Here are some frequently asked questions about Monoposto 2023:
-
-What are the system requirements for Monoposto 2023?
-
The game requires Android 5.0 or higher, or iOS 10.0 or higher. It also requires at least 1 GB of RAM and 300 MB of storage space.
-
-Can I play Monoposto 2023 offline?
-
Yes, you can play Monoposto 2023 offline in single player modes, such as quick race, single race, or championship. However, you need an internet connection to play online multiplayer mode or to access leaderboards and statistics.
-
-How can I contact the developer of Monoposto 2023?
-
You can contact Marco Pesce, the developer of Monoposto 2023, by email at monopostogame@gmail.com, or by visiting his website at www.monopostogame.com. You can also follow him on Facebook, Twitter, Instagram, and YouTube for updates and news about the game.
-
-Can I play Monoposto 2023 on PC or console?
-
No, Monoposto 2023 is only available for mobile devices. However, you can use an emulator or a mirroring software to play it on PC or console.
-
-Can I get a refund if I don't like Monoposto 2023?
-
If you download Monoposto 2023 from Google Play Store or App Store, you can request a refund within 48 hours of purchase. You need to contact Google Play Store or App Store support and provide your order number and reason for refund. If you download Monoposto 2023 from other sources, you may not be able to get a refund.
Download Blades of Brim Mod APK Apkpure: A Guide for Android Users
-
If you are looking for a fun and exciting game that combines endless running, action, and role-playing, then you should try Blades of Brim. This game is developed by SYBO Games, the same company that created Subway Surfers. In this game, you will play as one of the heroes of Brim, a fantasy world that is invaded by an army of Goons. You will run, jump, slash, and dash through various lands and realms, collecting coins, essence, runes, and orbs along the way. You will also unlock and upgrade different weapons, armors, pets, and power-ups to help you defeat the enemies and bosses.
But what if you want to enjoy the game without any limitations or restrictions? What if you want to have unlimited coins and essence to buy anything you want in the game? Well, there is a way to do that. You can download Blades of Brim Mod APK Apkpure, a modified version of the game that gives you access to unlimited resources and features. In this article, we will tell you what Blades of Brim Mod APK Apkpure is, how to download and install it on your Android device, and some tips and tricks for playing the game.
-
What is Blades of Brim?
-
A fantasy action game with endless running and RPG elements
-
Blades of Brim is a free infinite runner game that has a lot of action and RPG elements. The game is set in an epic, enchanting, and endless universe where magic and mayhem await. You will play as one of the awesome heroes of Brim, who have to save the world from the invading army of Goons. You will run through various environments, such as forests, deserts, caves, temples, and even outer space. You will also encounter different enemies, such as flappers, crushers, wizards, dragons, and more. You will have to use your sword skills to slash them or dodge them by jumping or changing lanes.
-
Features of the game
-
Blades of Brim has many features that make it an enjoyable and addictive game. Some of these features are:
-
-
A colorful and stunning 3D graphics that create a vibrant and immersive world.
-
A simple and intuitive swipe-based control system that allows you to move easily and smoothly.
-
A variety of heroes to choose from, each with their own personality and abilities.
-
A collection of weapons and armors to unlock and upgrade, each with their own stats and effects.
-
A selection of pets or mounts to ride on, such as wolves, horses, dragons, and more.
-
A number of power-ups to use during your run, such as shields, magnets, portals, etc.
-
A system of quests and achievements to complete for extra rewards.
-
A leaderboard and social features to compete with your friends and other players around the world.
-
-
What is Blades of Brim Mod APK Apkpure?
-
A modified version of the game with unlimited coins and essence
-
Blades of Brim Mod APK Apkpure is a modified version of the original game that gives you access to unlimited coins and essence. Coins are the main currency in the game that you can use to buy weapons, armors, pets, power-ups, etc. Essence is a special currency that you can use to upgrade your heroes and weapons. With the mod apk, you will have unlimited coins and essence, which means you can buy and upgrade anything you want in the game without worrying about running out of resources.
-
download blades of brim mod apk latest version
-download blades of brim mod apk unlimited money
-download blades of brim mod apk android 1
-download blades of brim mod apk offline
-download blades of brim mod apk no root
-download blades of brim mod apk free shopping
-download blades of brim mod apk revdl
-download blades of brim mod apk hack
-download blades of brim mod apk 2023
-download blades of brim mod apk for pc
-download blades of brim mod apk obb
-download blades of brim mod apk rexdl
-download blades of brim mod apk pure
-download blades of brim mod apk full version
-download blades of brim mod apk data
-download blades of brim mod apk online
-download blades of brim mod apk all characters unlocked
-download blades of brim mod apk unlimited gems
-download blades of brim mod apk android oyun club
-download blades of brim mod apk new update
-download blades of brim mod apk from apkpure.com[^1^]
-download blades of brim mod apk with cheat menu
-download blades of brim mod apk high damage
-download blades of brim mod apk mega mod
-download blades of brim mod apk unlimited everything
-download blades of brim mod apk 2.8.0
-download blades of brim mod apk god mode
-download blades of brim mod apk without ads
-download blades of brim mod apk 2.7.5
-download blades of brim mod apk 2.7.6
-download blades of brim mod apk 2.7.7
-download blades of brim mod apk 2.7.8
-download blades of brim mod apk 2.7.9
-download blades of brim mod apk 2.8.1
-download blades of brim mod apk 2.8.2
-download blades of brim mod apk 2.8.3
-download blades of brim mod apk 2.8.4
-download blades of brim mod apk 2.8.5
-download blades of brim mod apk 2.8.6
-download blades of brim mod apk 2.8.7
-download blades of brim mod apk 2.8.8
-download blades of brim mod apk 2.8.9
-download blades of brim mod apk 2.9.0
-download blades of brim mod apk for ios
-download blades of brim mod apk for windows 10
-download blades of brim mod apk for macbook pro
-
Benefits of using the mod apk
-
There are many benefits of using the Blades of Brim Mod APK Apkpure. Some of these benefits are:
-
-
You will be able to enjoy the game without any ads or interruptions.
-
You will be able to unlock all the heroes, weapons, armors, pets, and power-ups in the game.
-
You will be able to upgrade your heroes and weapons to the maximum level and increase their stats and effects.
-
You will be able to complete the quests and achievements faster and easier.
-
You will be able to score higher and rank higher on the leaderboard and social features.
-
You will be able to have more fun and excitement playing the game.
-
-
How to download and install Blades of Brim Mod APK Apkpure?
-
Requirements and precautions
-
Before you download and install Blades of Brim Mod APK Apkpure, you need to make sure that you meet some requirements and take some precautions. These are:
-
-
You need to have an Android device that runs on Android 4.4 or higher.
-
You need to have enough storage space on your device to download and install the mod apk file.
-
You need to enable the installation of apps from unknown sources on your device settings. This will allow you to install the mod apk file that is not from the Google Play Store.
-
You need to uninstall the original version of Blades of Brim from your device if you have it installed. This will prevent any conflicts or errors during the installation process.
-
You need to be aware that using the mod apk may violate the terms and conditions of the game and may result in a ban or suspension of your account. Use it at your own risk and discretion.
-
-
Steps to download and install the mod apk
-
Once you have met the requirements and taken the precautions, you can follow these steps to download and install Blades of Brim Mod APK Apkpure:
-
-
Go to this link to download the mod apk file. The file size is about 100 MB.
-
Wait for the download to finish and then locate the file on your device storage.
-
Tap on the file and follow the instructions on the screen to install it.
-
Wait for the installation to complete and then launch the game from your app drawer or home screen.
-
Enjoy playing Blades of Brim with unlimited coins and essence.
-
-
Tips and tricks for playing Blades of Brim
-
Kill as many monsters as possible
-
One of the main objectives of Blades of Brim is to kill as many monsters as possible during your run. This will not only increase your score, but also fill up your combo meter, which will give you extra coins, essence, runes, orbs, etc. You can kill monsters by slashing them with your sword or using your pets or power-ups. You can also use wall running and jumping skills to reach higher places where more monsters are waiting for you. Try to kill multiple monsters in one swipe or jump for bonus points.
-
Collect runes and orbs
-
Another important objective of Blades of Brim is to collect runes and orbs during your run. Runes are special symbols that appear randomly on the ground or in chests. They have different colors and shapes, such as red circles, blue triangles, green squares, etc. You need to collect three runes of the same color or shape in a row to activate a rune power-up, which will give you a temporary boost or effect, such as speed, shield, magnet, etc. Orbs are glowing spheres that appear randomly on the ground or in chests. They have different colors and effects, such as purple orbs that give you essence, yellow orbs that give you coins, green orbs that give you health, etc. You need to collect as many orbs as possible to increase your resources and survive longer.
-
Upgrade your heroes and weapons
-
As you play Blades of Brim, you will unlock new heroes and weapons that you can use in your runs. Each hero has a unique ability that can help you in different situations, such as double jumping, gliding, dashing, etc. Each weapon has a different stat that affects its damage, speed, range, etc. You can upgrade your heroes and weapons using essence, which will improve their stats and effects. You can also equip different armors p>Here is the table:
-
-
-
Hero
-
Image
-
Ability
-
Damage
-
Speed
-
Range
-
Cost
-
-
-
Lilith
-
-
Double Jump
-
10
-
10
-
10
-
Free
-
-
-
Zenobia
-
-
Glide
-
15
-
15
-
15
-
1000 coins or 100 essence
-
-
-
Ragnar
-
-
Dash Attack
-
20
-
20
-
20
-
2000 coins or 200 essence
-
-
-
Aurora
-
-
Magnetize Orbs
-
25
-
25
-
25
-
3000 coins or 300 essence
-
-
-
Sir Hugo
-
-
Bonus Chests
-
30
-
30
-
30
-
4000 coins or 400 essence
-
-
-
Weapon
-
Image
-
Type
-
Damage
-
Speed
-
Range
-
Cost
-
-
-
Sword of Brimstone
-
-
Sword
-
10 + 2 per level
-
10 + 1 per level
-
10 + 1 per level
-
Free (default)
-
-
-
Axe of Fury
-
-
Axe
-
15 + 3 per level
-
8 + 1 per level
-
12 + 2 per level
-
500 coins or 50 essence
-
Hammer of Justice
-
-
Hammer
-
20 + 4 per level
-
6 + 1 per level
-
14 + 2 per level
-
1000 coins or 100 essence
-
-
-
Dagger of Destiny
-
-
Dagger
-
8 + 2 per level
-
12 + 2 per level
-
8 + 1 per level
-
1500 coins or 150 essence
-
-
-
Spear of Light
-
-
Spear
-
12 + 3 per level
-
9 + 1 per level
-
16 + 3 per level
-
2000 coins or 200 essence
-
-
-
Conclusion
-
Blades of Brim is a fun and exciting game that will keep you entertained for hours. You will love the colorful graphics, the smooth controls, the diverse heroes and weapons, and the endless action and adventure. You will also enjoy the mod apk version that gives you unlimited coins and essence to buy and upgrade anything you want in the game. You can download Blades of Brim Mod APK Apkpure from this link and follow the steps in this article to install it on your Android device. You can also use the tips and tricks in this article to improve your gameplay and score higher. So what are you waiting for? Download Blades of Brim Mod APK Apkpure now and join the epic battle against the Goons.
-
FAQs
-
Here are some frequently asked questions about Blades of Brim Mod APK Apkpure:
-
-
Is Blades of Brim Mod APK Apkpure safe to use?
-
Yes, Blades of Brim Mod APK Apkpure is safe to use as long as you download it from a trusted source and follow the instructions in this article. However, you should be aware that using the mod apk may violate the terms and conditions of the game and may result in a ban or suspension of your account. Use it at your own risk and discretion.
-
Is Blades of Brim Mod APK Apkpure compatible with other devices?
-
Blades of Brim Mod APK Apkpure is compatible with most Android devices that run on Android 4.4 or higher. However, some devices may not support the mod apk or may experience some issues or errors during the installation or gameplay. If you encounter any problems, you can try to uninstall and reinstall the mod apk or contact the developer for assistance.
-
How can I update Blades of Brim Mod APK Apkpure?
-
To update Blades of Brim Mod APK Apkpure, you need to download the latest version of the mod apk file from this link and follow the same steps in this article to install it on your device. You may need to uninstall the previous version of the mod apk before installing the new one. You should also check for updates regularly to enjoy the latest features and improvements of the game.
-
How can I contact the developer of Blades of Brim Mod APK Apkpure?
-
If you have any questions, feedback, or suggestions about Blades of Brim Mod APK Apkpure, you can contact the developer by visiting their website or sending them an email at [email](mailto:info@apkpure.com).
-
How can I support the developer of Blades of Brim Mod APK Apkpure?
-
If you like Blades of Brim Mod APK Apkpure and want to support the developer, you can do so by rating and reviewing the mod apk on this link or by sharing it with your friends and family. You can also support the original developer of Blades of Brim by downloading and playing the official version of the game from the Google Play Store.
-
401be4b1e0
-
-
\ No newline at end of file
diff --git a/spaces/feregVcuzo/sanity-test-midi/checkpoint/Create Combine and Explore with Alchemy Classic 2 Mod APK.md b/spaces/feregVcuzo/sanity-test-midi/checkpoint/Create Combine and Explore with Alchemy Classic 2 Mod APK.md
deleted file mode 100644
index c90168339ab5ba81cfa42d0459bf4569eddefc66..0000000000000000000000000000000000000000
--- a/spaces/feregVcuzo/sanity-test-midi/checkpoint/Create Combine and Explore with Alchemy Classic 2 Mod APK.md
+++ /dev/null
@@ -1,109 +0,0 @@
-
-
Alchemy Classic 2 Mod APK: A Fun and Creative Game for Android Users
-
Do you love playing games that challenge your creativity and logic? Do you enjoy mixing and matching different elements to create new ones? If you answered yes, then you will love Alchemy Classic 2, a game that lets you explore the world of chemistry and physics in a fun and engaging way. And if you want to make the game even more exciting, you can download the Alchemy Classic 2 Mod APK, which gives you unlimited money, hints, and cheats to unlock all the elements and features in the game. In this article, we will tell you everything you need to know about Alchemy Classic 2 and how to download and install the modded version on your Android device.
-
What is Alchemy Classic 2?
-
Alchemy Classic 2 is a puzzle game developed by Niasoft, a company that specializes in creating educational and entertaining games for mobile devices. The game is a sequel to the popular Alchemy Classic, which has over 10 million downloads on Google Play. The game is based on the concept of alchemy, which is an ancient practice of transforming matter into different forms. In the game, you start with four basic elements: air, water, fire, and earth. By combining these elements, you can create new ones, such as steam, mud, lava, and sand. As you progress in the game, you can discover more complex and advanced elements, such as metals, plants, animals, humans, and even mythical creatures. The game has over 1000 elements to discover and collect, making it a great way to learn about science and nature while having fun.
The gameplay of Alchemy Classic 2 is simple and intuitive. You just need to drag and drop the elements from the inventory to the main screen and see what happens when they interact. If they can form a new element, it will appear on the screen and be added to your collection. If not, nothing will happen and you can try another combination. You can also tap on an element to see its properties and description, as well as its history and origin. The game has a user-friendly interface that allows you to easily navigate through the menus and options. You can also customize the game settings according to your preferences, such as changing the language, sound effects, background music, and theme.
-
The features of Alchemy Classic 2
-
Alchemy Classic 2 has many features that make it a fun and addictive game for Android users. Some of these features are:
-
-
A huge collection of elements to discover and collect
-
A variety of categories to explore, such as chemistry, physics, biology, geology, astronomy, mythology, and more
-
A beautiful design and graphics that create a realistic and immersive experience
-
A dynamic soundtrack that adapts to the gameplay
-
A leaderboard and achievements system that tracks your progress and rewards you for your accomplishments
-
A social media integration that allows you to share your discoveries with your friends
-
A support system that provides you with hints and tips when you get stuck
-
A regular update that adds new elements and features to the game
-
-
The benefits of Alchemy Classic 2 Mod APK
-
If you want to enjoy Alchemy Classic 2 without any limitations or restrictions, you can download the Alchemy Classic 2 Mod APK, which gives you unlimited money, hints, and cheats to unlock all the elements and features in the game. The modded version of the game has many benefits, such as:
-
-
You can access all the elements without having to discover them by yourself
-
You can use hints and cheats to find out the combinations and reactions of any element
-
You can buy any item or upgrade from the shop without worrying about the cost
-
You can enjoy the game without any ads or interruptions
-
You can play the game offline without needing an internet connection
-
-
The Alchemy Classic 2 Mod APK is safe and easy to download and install on your Android device. You just need to follow a few simple steps, which we will explain in the next section.
-
How to download and install Alchemy Classic 2 Mod APK?
-
To download and install the Alchemy Classic 2 Mod APK on your Android device, you need to follow these steps:
-
Step 1: Download the APK file from a trusted source
-
The first thing you need to do is to download the APK file of the modded version of the game from a reliable and secure source. You can use the link below to download the file directly to your device. The file size is about 50 MB, so make sure you have enough storage space and a stable internet connection.
-
alchemy classic hd mod apk unlimited money
-alchemy classic hd v1.7.7.17 mod apk download
-alchemy classic hd hack apk free download
-alchemy classic hd premium apk mod
-alchemy classic hd mod apk latest version
-alchemy classic hd cheats apk download
-alchemy classic hd mod apk android 1
-alchemy classic hd mod apk happymod
-alchemy classic hd unlocked apk download
-alchemy classic hd full apk mod
-alchemy classic hd mod apk revdl
-alchemy classic hd pro apk mod
-alchemy classic hd cracked apk download
-alchemy classic hd mod apk no ads
-alchemy classic hd mod apk offline
-alchemy classic hd unlimited points apk
-alchemy classic hd mod apk 2023
-alchemy classic hd mega mod apk
-alchemy classic hd modded apk free download
-alchemy classic hd vip mod apk
-alchemy classic hd mod apk for pc
-alchemy classic hd mod apk rexdl
-alchemy classic hd paid apk mod
-alchemy classic hd all elements unlocked apk
-alchemy classic hd plus mod apk
-alchemy classic hd gold mod apk
-alchemy classic hd ad free mod apk
-alchemy classic hd mod menu apk
-alchemy classic hd unlimited hints apk
-alchemy classic hd 1.7.7.17 hack apk
-alchemy classic 2 premium mod apk download
-alchemy classic 2 hack apk free download
-alchemy classic 2 cheats apk download
-alchemy classic 2 full version mod apk
-alchemy classic 2 pro mod apk download
-alchemy classic 2 cracked apk download
-alchemy classic 2 unlimited money mod apk
-alchemy classic 2 unlocked elements mod apk
-alchemy classic 2 no ads mod apk download
-alchemy classic 2 latest version mod apk
The next thing you need to do is to enable unknown sources on your device. This is a security setting that allows you to install apps from sources other than Google Play. To enable unknown sources, you need to go to your device settings, then security, then toggle on the option that says "allow installation of apps from unknown sources". This will allow you to install the APK file that you downloaded in step 1.
-
Step 3: Install the APK file and launch the game
-
The final thing you need to do is to install the APK file and launch the game. To do this, you need to locate the file in your device's file manager or downloads folder, then tap on it to start the installation process. Follow the instructions on the screen and wait for a few seconds until the installation is complete. Once it is done, you can open the game and enjoy playing Alchemy Classic 2 with unlimited money, hints, and cheats.
-
Tips and tricks for playing Alchemy Classic 2
-
Now that you have downloaded and installed Alchemy Classic 2 Mod APK, you are ready to play the game and have fun. However, if you want to make the most out of your gaming experience, here are some tips and tricks that you can use:
-
Use hints and cheats to discover new elements
-
One of the benefits of using the modded version of the game is that you have unlimited hints and cheats that can help you discover new elements. You can use these features whenever you get stuck or want to learn something new. To use hints, you just need to tap on the light bulb icon at the top right corner of the screen. This will show you a possible combination or reaction that you can try. To use cheats, you just need to tap on the magnifying glass icon at the top left corner of the screen. This will show you all the elements that you can create with a given element.
-
Experiment with different combinations and reactions
-
Another benefit of using the modded version of the game is that you have unlimited money that you can use to buy any item or upgrade from the shop. This means that you can experiment with different combinations and reactions without worrying about wasting resources or losing progress. You can try different items such as flasks, tubes, filters, heaters, coolers, magnets, lasers, and more. You can also try different upgrades such as increasing your inventory size, unlocking new categories, improving your hints and cheats, and more. By experimenting with different combinations and reactions, you can discover new elements and learn more about science and nature.
-
Save your progress and share your achievements
-
The last benefit of using the modded version of the game is that you can play offline without needing an internet connection. This means that you can save your progress and resume playing anytime and anywhere. However, if you want to share your achievements with your friends or other players, you can connect your game account to social media platforms such as Facebook or Twitter. This will allow you to post your discoveries, screenshots, videos, and more. You can also compare your scores and rankings with other players on the leaderboard and achievements system. You can also join the online community of Alchemy Classic 2 and interact with other fans and players.
-
Conclusion
-
Summary of the main points
-
Alchemy Classic 2 is a fun and creative game for Android users that lets you explore the world of chemistry and physics in a fun and engaging way. You can discover and collect over 1000 elements from different categories and learn more about science and nature. You can also download the Alchemy Classic 2 Mod APK, which gives you unlimited money, hints, and cheats to unlock all the elements and features in the game. You can also customize the game settings, experiment with different combinations and reactions, save your progress, and share your achievements with your friends.
-
Call to action and recommendation
-
If you are looking for a game that challenges your creativity and logic, then you should try Alchemy Classic 2. It is a game that will keep you entertained and educated for hours. You can download the game from Google Play or use the link below to download the modded version of the game. We hope you enjoy playing Alchemy Classic 2 and have fun discovering new elements.
Here are some frequently asked questions about Alchemy Classic 2 and its modded version:
-
-
Q: Is Alchemy Classic 2 free to play?
-
A: Yes, Alchemy Classic 2 is free to play. However, it contains ads and in-app purchases that can enhance your gaming experience. If you want to remove the ads and get unlimited money, hints, and cheats, you can download the modded version of the game.
-
Q: Is Alchemy Classic 2 safe to download and install?
-
A: Yes, Alchemy Classic 2 is safe to download and install. The game does not contain any viruses or malware that can harm your device. However, you should always download the game from a trusted source, such as Google Play or the link we provided in this article.
-
Q: What are the requirements for playing Alchemy Classic 2?
-
A: Alchemy Classic 2 requires Android 4.1 or higher to run smoothly on your device. The game also requires about 50 MB of storage space and a stable internet connection for downloading and updating the game.
-
Q: How many elements are there in Alchemy Classic 2?
-
A: There are over 1000 elements in Alchemy Classic 2, divided into different categories such as chemistry, physics, biology, geology, astronomy, mythology, and more. You can discover new elements by combining existing ones or using hints and cheats.
-
Q: How can I contact the developer of Alchemy Classic 2?
-
A: If you have any questions, feedback, or suggestions about Alchemy Classic 2, you can contact the developer of the game by sending an email to support@niasoft.com or visiting their website at https://niasoft.com/.
-
401be4b1e0
-
-
\ No newline at end of file
diff --git a/spaces/feregVcuzo/sanity-test-midi/checkpoint/Download Getting Over It on Mobile A Game for a Certain Kind of Person to Hurt Them.md b/spaces/feregVcuzo/sanity-test-midi/checkpoint/Download Getting Over It on Mobile A Game for a Certain Kind of Person to Hurt Them.md
deleted file mode 100644
index e9ebaa480df366d1c59ecd0a32cc47e9c0c8a8e1..0000000000000000000000000000000000000000
--- a/spaces/feregVcuzo/sanity-test-midi/checkpoint/Download Getting Over It on Mobile A Game for a Certain Kind of Person to Hurt Them.md
+++ /dev/null
@@ -1,132 +0,0 @@
-
-
How to Download Getting Over It Mobile - A Guide for Frustration Lovers
-
If you are looking for a game that will test your patience, skill, and sanity, then you might want to try Getting Over It Mobile. This is a mobile version of the popular and notorious PC game Getting Over It with Bennett Foddy, which is known for its punishing difficulty and hilarious commentary. In this article, we will show you how to download Getting Over It Mobile on your Android or iOS device, and give you some tips and tricks for playing this frustrating but addictive game.
A brief introduction to the game and its developer
-
Getting Over It Mobile is a fan game based on Getting Over It with Bennett Foddy, which was released in 2017 by Bennett Foddy, an Australian game designer and philosopher. The original game was inspired by an older game called Sexy Hiking, which was made by Jazzuo in 2002. Both games are part of a genre called "B-games", which are intentionally weird, low-budget, and experimental games that challenge the conventions of mainstream gaming.
-
The gameplay and the challenge of Getting Over It Mobile
-
The gameplay of Getting Over It Mobile is simple but hard. You play as a man in a pot who has to climb up a mountain using only a hammer. You can swing the hammer with your finger or mouse, and use it to hook onto objects, push yourself off the ground, or balance yourself in mid-air. The physics of the game are realistic but unforgiving, and any wrong move can send you tumbling down to where you started, or even lower. There is no save system, no checkpoints, no shortcuts, and no mercy. The only thing that keeps you going is the voice of Bennett Foddy himself, who narrates your progress, gives you philosophical insights, or taunts you with sarcastic remarks.
-
How to Download Getting Over It Mobile on Android
-
The official way to download the game from Google Play Store
-
The official version of Getting Over It Mobile is available on Google Play Store for $4.99. You can find it by searching for "Getting Over It" or by following this link. To download the game, you need to have an Android device that runs on Android 5.0 or higher, and have enough storage space (about 150 MB). Once you purchase and install the game, you can launch it and start playing.
-
The alternative way to download the game from APKPure.com
-
If you don't want to pay for the game, or if you can't access Google Play Store for some reason, you can also download the game from APKPure.com, which is a website that offers free APK files for various apps and games. To download the game from APKPure.com, you need to follow these steps:
-
-
Search for "Getting Over It" on APKPure.com.
-
Click on the "Download APK" button to start downloading the file on your device.
-
Once the download is finished, open the file and allow it to install on your device.
-
Launch the game and start playing.
-
-
Note that downloading APK files from third-party sources can be risky, as they may contain malware or viruses. Therefore, you should always scan the file before installing
How to Download Getting Over It Mobile on iOS
-
The official way to download the game from App Store
-
The official version of Getting Over It Mobile is also available on App Store for $4.99. You can find it by searching for "Getting Over It" or by following this link. To download the game, you need to have an iOS device that runs on iOS 10.0 or higher, and have enough storage space (about 150 MB). Once you purchase and install the game, you can launch it and start playing.
-
The alternative way to download the game from AppValley.vip
-
If you don't want to pay for the game, or if you can't access App Store for some reason, you can also download the game from AppValley.vip, which is a website that offers free apps and games for iOS devices. To download the game from AppValley.vip, you need to follow these steps:
-
How to download Getting Over It on Android
-Getting Over It with Bennett Foddy APK
-Getting Over It free download for mobile
-Getting Over It gameplay and review
-Getting Over It tips and tricks
-Getting Over It best moments and fails
-Getting Over It mod APK unlimited money
-Getting Over It PC vs mobile comparison
-Getting Over It speedrun world record
-Getting Over It cheats and hacks
-Getting Over It with Bennett Foddy Google Play
-Getting Over It iOS download link
-Getting Over It online multiplayer mode
-Getting Over It alternatives and similar games
-Getting Over It update and patch notes
-Getting Over It with Bennett Foddy Steam
-Getting Over It achievements and trophies
-Getting Over It wallpapers and themes
-Getting Over It memes and jokes
-Getting Over It fan art and cosplay
-Getting Over It soundtrack and music
-Getting Over It quotes and philosophy
-Getting Over It easter eggs and secrets
-Getting Over It challenges and custom maps
-Getting Over It reaction videos and commentary
-Getting Over It with Bennett Foddy Wikipedia
-Getting Over It history and development
-Getting Over It merchandise and gifts
-Getting Over It community and forums
-Getting Over It ratings and reviews
-How to play Getting Over It on PC
-How to get better at Getting Over It
-How to uninstall Getting Over It from your phone
-How to stream Getting Over It on Twitch or YouTube
-How to record your gameplay of Getting Over It
-How to edit your videos of Getting Over It
-How to share your screenshots of Getting Over It
-How to make a thumbnail for your video of Getting Over It
-How to write a blog post about Getting Over It
-How to create a podcast about Getting Over It
-
-
Open Safari on your iOS device and go to AppValley.vip.
-
Tap on the "Install AppValley" button and follow the instructions to install the app on your device.
-
Open AppValley and search for "Getting Over It".
-
Tap on the "Get" button and wait for the game to download and install on your device.
-
Launch the game and start playing.
-
-
Note that downloading apps and games from third-party sources can be risky, as they may contain malware or viruses. Therefore, you should always scan the app before installing it, and trust the developer in your device settings.
-
Tips and Tricks for Playing Getting Over It Mobile
-
How to control the hammer and the pot
-
The most important skill in Getting Over It Mobile is learning how to control the hammer and the pot. The hammer is your only tool and weapon, and the pot is your only protection and limitation. Here are some tips on how to master them:
-
-
Use your finger or mouse to drag the hammer around the screen. The hammer will follow your movement, but it will also have some inertia and momentum. You need to account for that when you swing or hook it.
-
Use the hammer to grab onto objects, such as rocks, trees, pipes, or furniture. You can then use them to pull yourself up, push yourself forward, or swing yourself around.
-
Use the hammer to balance yourself in mid-air, or to land safely on a surface. You can also use it to bounce off walls or ceilings, or to deflect obstacles.
-
Use the pot to protect yourself from falling objects, such as barrels, buckets, or anvils. You can also use it to slide down slopes or ramps, or to bounce off springs or trampolines.
-
Use the pot to limit your movement, such as when you need to squeeze through narrow gaps or corners. You can also use it to wedge yourself between objects, such as branches or beams.
-
-
How to deal with frustration and failure
-
The most common emotion in Getting Over It Mobile is frustration. The game is designed to make you fail, over and over again. You will lose progress, fall down, get stuck, or get hit by random objects. You will hear Bennett Foddy mocking you, laughing at you, or lecturing you. You will feel angry, sad, hopeless, or bored. Here are some tips on how to deal with frustration and failure:
-
-
Take a break. If you feel like you are losing your temper or your motivation, just stop playing for a while. Do something else that makes you happy or relaxed. Come back later when you are in a better mood.
-
Laugh it off. If you fail in a funny or absurd way, just laugh at yourself and the game. Don't take it too seriously. Enjoy the humor and the absurdity of the situation.
-
Learn from your mistakes. If you fail in a predictable or avoidable way, just learn from it and try again. Don't repeat the same mistake twice. Improve your skills and your strategy.
-
Celebrate your achievements. If you succeed in a difficult or impressive way, just celebrate it and be proud of yourself. Don't forget how far you have come. Appreciate your progress and your effort.
-
Share your experience. If you want to vent your frustration or share your joy, just talk to someone who understands you. Find a friend who plays the game, or join an online community of fellow players. Exchange tips, stories, screenshots, or videos.
-
-
How to
How to enjoy the game and its philosophy
-
The most rewarding emotion in Getting Over It Mobile is enjoyment. The game is not only a challenge, but also a journey, a story, and a message. You will discover new places, new objects, new sounds, and new words. You will hear Bennett Foddy telling you his thoughts, his feelings, his opinions, and his wisdom. You will feel curious, inspired, amused, or enlightened. Here are some tips on how to enjoy the game and its philosophy:
-
-
Explore the world. The game has a lot of hidden secrets and surprises. You can find different paths, different objects, different sounds, and different scenes. You can also interact with some of them, such as playing music, reading books, or watching videos.
-
Listen to the voice. The game has a lot of dialogue and narration. You can hear Bennett Foddy talking about various topics, such as art, history, culture, psychology, or philosophy. You can also hear him quoting other authors, artists, or thinkers.
-
Think about the meaning. The game has a lot of symbolism and metaphors. You can interpret the game as a metaphor for life, for struggle, for achievement, or for anything else. You can also relate the game to your own experiences, beliefs, or values.
-
Express yourself. The game has a lot of freedom and creativity. You can play the game in your own way, at your own pace, and with your own style. You can also create your own content, such as drawings, poems, songs, or stories.
-
Have fun. The game has a lot of fun and humor. You can enjoy the game as a game, as a challenge, as a joke, or as a spectacle. You can also make fun of yourself, of the game, or of Bennett Foddy.
-
-
Conclusion
-
Getting Over It Mobile is a game that will make you feel frustrated, but also satisfied; angry, but also happy; hopeless, but also hopeful. It is a game that will make you laugh, cry, scream, or smile. It is a game that will make you think, learn, grow, or change. It is a game that will make you love it or hate it.
-
If you want to experience this unique and unforgettable game on your mobile device, you can download it from Google Play Store or App Store for $4.99. Alternatively, you can download it from APKPure.com or AppValley.vip for free.
-
Whatever you choose to do, we hope that this article has helped you to download Getting Over It Mobile and to play it better. We also hope that you have enjoyed reading this article as much as we have enjoyed writing it.
-
FAQs
-
Q: Is Getting Over It Mobile the same as Getting Over It with Bennett Foddy?
-
A: Getting Over It Mobile is not the same as Getting Over It with Bennett Foddy. It is a fan game that is based on the original game but has some differences in graphics, sound, and gameplay. For example, the mobile version has a different background music, a different ending, and a different control scheme. However, the mobile version still retains the core elements of the original game, such as the mountain, the hammer, the pot, and the voice.
-
Q: How long does it take to finish Getting Over It Mobile?
-
A: The answer to this question depends on your skill level, your luck, and your perseverance. Some people can finish the game in less than an hour, while others can take days, weeks, or even months. The game has no fixed length, as it depends on how fast you can climb the mountain and how often you fall down. The game also has a secret ending that requires you to do something extra after reaching the top of the mountain.
-
Q: What is the reward for finishing Getting Over It Mobile?
-
A: The reward for finishing Getting Over It Mobile is mostly personal and emotional. You will feel a sense of accomplishment, satisfaction, relief, and pride. You will also get to see a special scene at the end of the game, where you can interact with other players who have finished the game. You will also get to hear a final message from Bennett Foddy, who will congratulate you and share some of his thoughts with you.
-
Q: Is there a way to cheat or hack Getting Over It Mobile?
-
A: There are some ways to cheat or hack Getting Over It Mobile, but we do not recommend them. They can ruin the fun and the challenge of the game, and they can also cause problems for your device or your account. Some of the common ways to cheat or hack Getting Over It Mobile are:
-
-
Using a modded APK or IPA file that gives you unlimited lives, unlimited speed, or unlimited height.
-
Using a screen recorder or a video editor to fake your progress or your completion.
-
Using a VPN or a proxy server to access the game from a different region or country.
-
Using a bot or a script to automate your movements or actions.
-
-
If you want to enjoy Getting Over It Mobile as it is intended to be played, we suggest that you avoid these methods and play the game fair and square.
-
Q: Where can I find more information or help about Getting Over It Mobile?
-
A: If you want to find more information or help about Getting Over It Mobile, you can visit some of these websites or sources:
-
-
The official website of Bennett Foddy, where you can find his other games, his blog, his contact information, and his biography.
-
The official Twitter account of Bennett Foddy, where you can follow his updates, his tweets, his replies, and his retweets.
-
The official YouTube channel of Bennett Foddy, where you can watch his videos, his interviews, his lectures, and his streams.
-
The official Discord server of Getting Over It with Bennett Foddy, where you can join the community of players, chat with them, ask questions, share tips, post memes, or participate in events.
-
The official subreddit of Getting Over It with Bennett Foddy, where you can browse the posts of players, comment on them, upvote them, downvote them, or create your own posts.
-
197e85843d
-
-
\ No newline at end of file
diff --git a/spaces/florim/MedGPT/autogpt/speech/eleven_labs.py b/spaces/florim/MedGPT/autogpt/speech/eleven_labs.py
deleted file mode 100644
index ea84efd8ca9489b40919ecd571813fe954b078e3..0000000000000000000000000000000000000000
--- a/spaces/florim/MedGPT/autogpt/speech/eleven_labs.py
+++ /dev/null
@@ -1,86 +0,0 @@
-"""ElevenLabs speech module"""
-import os
-
-import requests
-from playsound import playsound
-
-from autogpt.config import Config
-from autogpt.speech.base import VoiceBase
-
-PLACEHOLDERS = {"your-voice-id"}
-
-
-class ElevenLabsSpeech(VoiceBase):
- """ElevenLabs speech class"""
-
- def _setup(self) -> None:
- """Set up the voices, API key, etc.
-
- Returns:
- None: None
- """
-
- cfg = Config()
- default_voices = ["ErXwobaYiN019PkySvjV", "EXAVITQu4vr4xnSDxMaL"]
- voice_options = {
- "Rachel": "21m00Tcm4TlvDq8ikWAM",
- "Domi": "AZnzlk1XvdvUeBnXmlld",
- "Bella": "EXAVITQu4vr4xnSDxMaL",
- "Antoni": "ErXwobaYiN019PkySvjV",
- "Elli": "MF3mGyEYCl7XYWbV9V6O",
- "Josh": "TxGEqnHWrfWFTfGW9XjX",
- "Arnold": "VR6AewLTigWG4xSOukaG",
- "Adam": "pNInz6obpgDQGcFmaJgB",
- "Sam": "yoZ06aMxZJJ28mfd3POQ",
- }
- self._headers = {
- "Content-Type": "application/json",
- "xi-api-key": cfg.elevenlabs_api_key,
- }
- self._voices = default_voices.copy()
- if cfg.elevenlabs_voice_1_id in voice_options:
- cfg.elevenlabs_voice_1_id = voice_options[cfg.elevenlabs_voice_1_id]
- if cfg.elevenlabs_voice_2_id in voice_options:
- cfg.elevenlabs_voice_2_id = voice_options[cfg.elevenlabs_voice_2_id]
- self._use_custom_voice(cfg.elevenlabs_voice_1_id, 0)
- self._use_custom_voice(cfg.elevenlabs_voice_2_id, 1)
-
- def _use_custom_voice(self, voice, voice_index) -> None:
- """Use a custom voice if provided and not a placeholder
-
- Args:
- voice (str): The voice ID
- voice_index (int): The voice index
-
- Returns:
- None: None
- """
- # Placeholder values that should be treated as empty
- if voice and voice not in PLACEHOLDERS:
- self._voices[voice_index] = voice
-
- def _speech(self, text: str, voice_index: int = 0) -> bool:
- """Speak text using elevenlabs.io's API
-
- Args:
- text (str): The text to speak
- voice_index (int, optional): The voice to use. Defaults to 0.
-
- Returns:
- bool: True if the request was successful, False otherwise
- """
- tts_url = (
- f"https://api.elevenlabs.io/v1/text-to-speech/{self._voices[voice_index]}"
- )
- response = requests.post(tts_url, headers=self._headers, json={"text": text})
-
- if response.status_code == 200:
- with open("speech.mpeg", "wb") as f:
- f.write(response.content)
- playsound("speech.mpeg", True)
- os.remove("speech.mpeg")
- return True
- else:
- print("Request failed with status code:", response.status_code)
- print("Response content:", response.content)
- return False
diff --git a/spaces/freddyaboulton/llama-chat-discord-bot/app.py b/spaces/freddyaboulton/llama-chat-discord-bot/app.py
deleted file mode 100644
index c690a6d7b3a276c074bb1bb150bb4b84d21b16ab..0000000000000000000000000000000000000000
--- a/spaces/freddyaboulton/llama-chat-discord-bot/app.py
+++ /dev/null
@@ -1,192 +0,0 @@
-import asyncio
-import os
-import threading
-from threading import Event
-from typing import Optional
-
-import discord
-import gradio as gr
-from discord import Permissions
-from discord.ext import commands
-from discord.utils import oauth_url
-
-import gradio_client as grc
-from gradio_client.utils import QueueError
-
-event = Event()
-
-DISCORD_TOKEN = os.getenv("DISCORD_TOKEN")
-
-
-async def wait(job):
- while not job.done():
- await asyncio.sleep(0.2)
-
-
-def get_client(session: Optional[str] = None) -> grc.Client:
- client = grc.Client("https://ysharma-explore-llamav2-with-tgi.hf.space", hf_token=os.getenv("HF_TOKEN"))
- if session:
- client.session_hash = session
- return client
-
-
-def truncate_response(response: str) -> str:
- ending = "...\nTruncating response to 2000 characters due to discord api limits."
- if len(response) > 2000:
- return response[: 2000 - len(ending)] + ending
- else:
- return response
-
-
-intents = discord.Intents.default()
-intents.message_content = True
-bot = commands.Bot(command_prefix="/", intents=intents)
-
-
-@bot.event
-async def on_ready():
- print(f"Logged in as {bot.user} (ID: {bot.user.id})")
- synced = await bot.tree.sync()
- print(f"Synced commands: {', '.join([s.name for s in synced])}.")
- event.set()
- print("------")
-
-
-thread_to_client = {}
-thread_to_user = {}
-
-
-@bot.hybrid_command(
- name="llamachat",
- description="Enter some text to chat with the bot! Like this: /llamachat Hello, how are you?",
-)
-async def chat(ctx, prompt: str):
- if ctx.author.id == bot.user.id:
- return
- try:
- message = await ctx.send("Creating thread...")
-
- thread = await message.create_thread(name=prompt)
- loop = asyncio.get_running_loop()
- client = await loop.run_in_executor(None, get_client, None)
- job = client.submit(prompt, api_name="/chat")
- await wait(job)
-
- try:
- job.result()
- response = job.outputs()[-1]
- await thread.send(truncate_response(response))
- thread_to_client[thread.id] = client
- thread_to_user[thread.id] = ctx.author.id
- except QueueError:
- await thread.send(
- "The gradio space powering this bot is really busy! Please try again later!"
- )
-
- except Exception as e:
- print(f"{e}")
-
-
-async def continue_chat(message):
- """Continues a given conversation based on chathistory"""
- try:
- client = thread_to_client[message.channel.id]
- prompt = message.content
- job = client.submit(prompt, api_name="/chat")
- await wait(job)
- try:
- job.result()
- response = job.outputs()[-1]
- await message.reply(truncate_response(response))
- except QueueError:
- await message.reply(
- "The gradio space powering this bot is really busy! Please try again later!"
- )
-
- except Exception as e:
- print(f"Error: {e}")
-
-
-@bot.event
-async def on_message(message):
- """Continue the chat"""
- try:
- if not message.author.bot:
- if message.channel.id in thread_to_user:
- if thread_to_user[message.channel.id] == message.author.id:
- await continue_chat(message)
- else:
- await bot.process_commands(message)
-
- except Exception as e:
- print(f"Error: {e}")
-
-
-# running in thread
-def run_bot():
- if not DISCORD_TOKEN:
- print("DISCORD_TOKEN NOT SET")
- event.set()
- else:
- bot.run(DISCORD_TOKEN)
-
-
-threading.Thread(target=run_bot).start()
-
-event.wait()
-
-if not DISCORD_TOKEN:
- welcome_message = """
-
- ## You have not specified a DISCORD_TOKEN, which means you have not created a bot account. Please follow these steps:
-
- ### 1. Go to https://discord.com/developers/applications and click 'New Application'
-
- ### 2. Give your bot a name 🤖
-
- 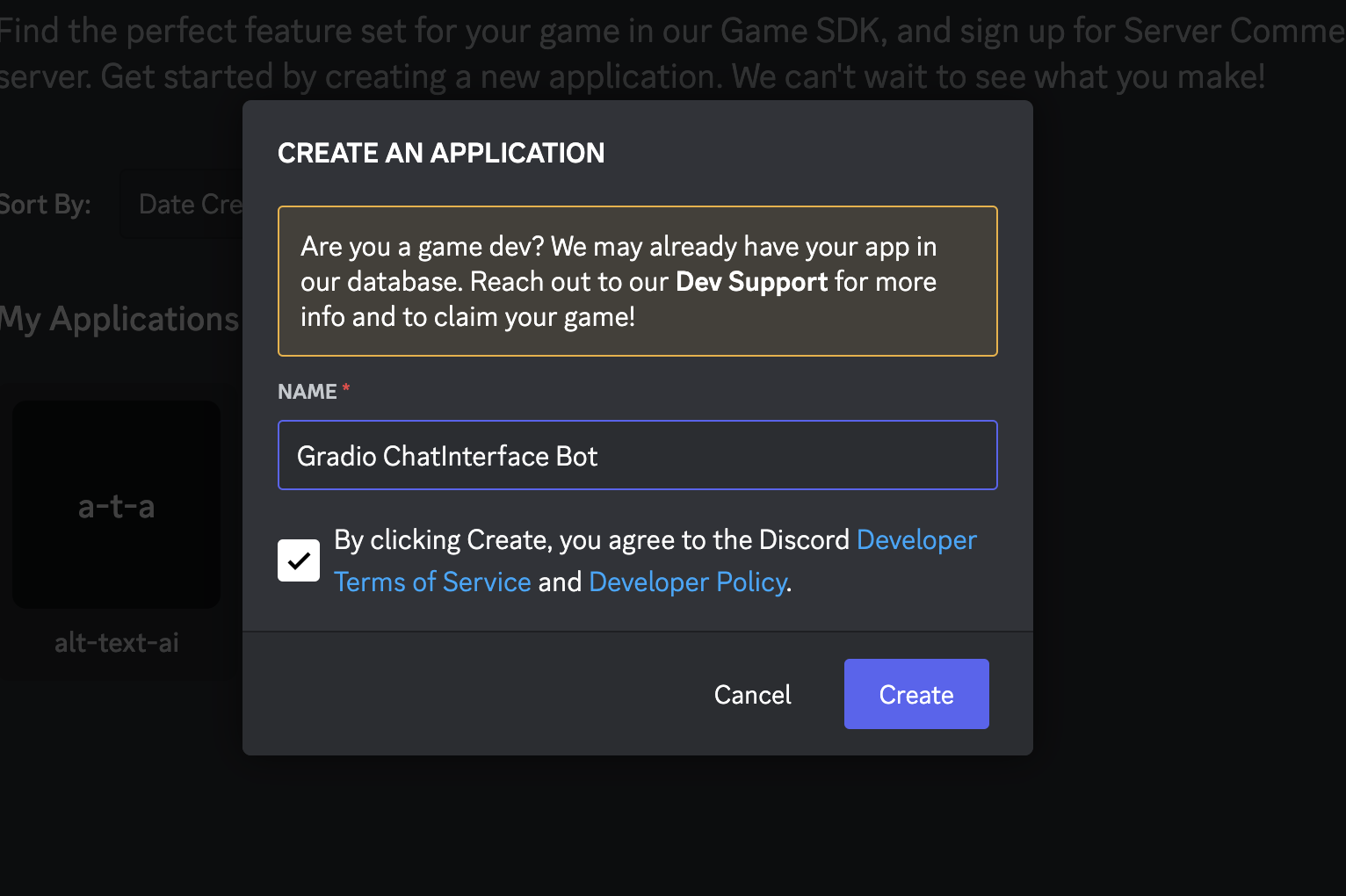
-
- ## 3. In Settings > Bot, click the 'Reset Token' button to get a new token. Write it down and keep it safe 🔐
-
- 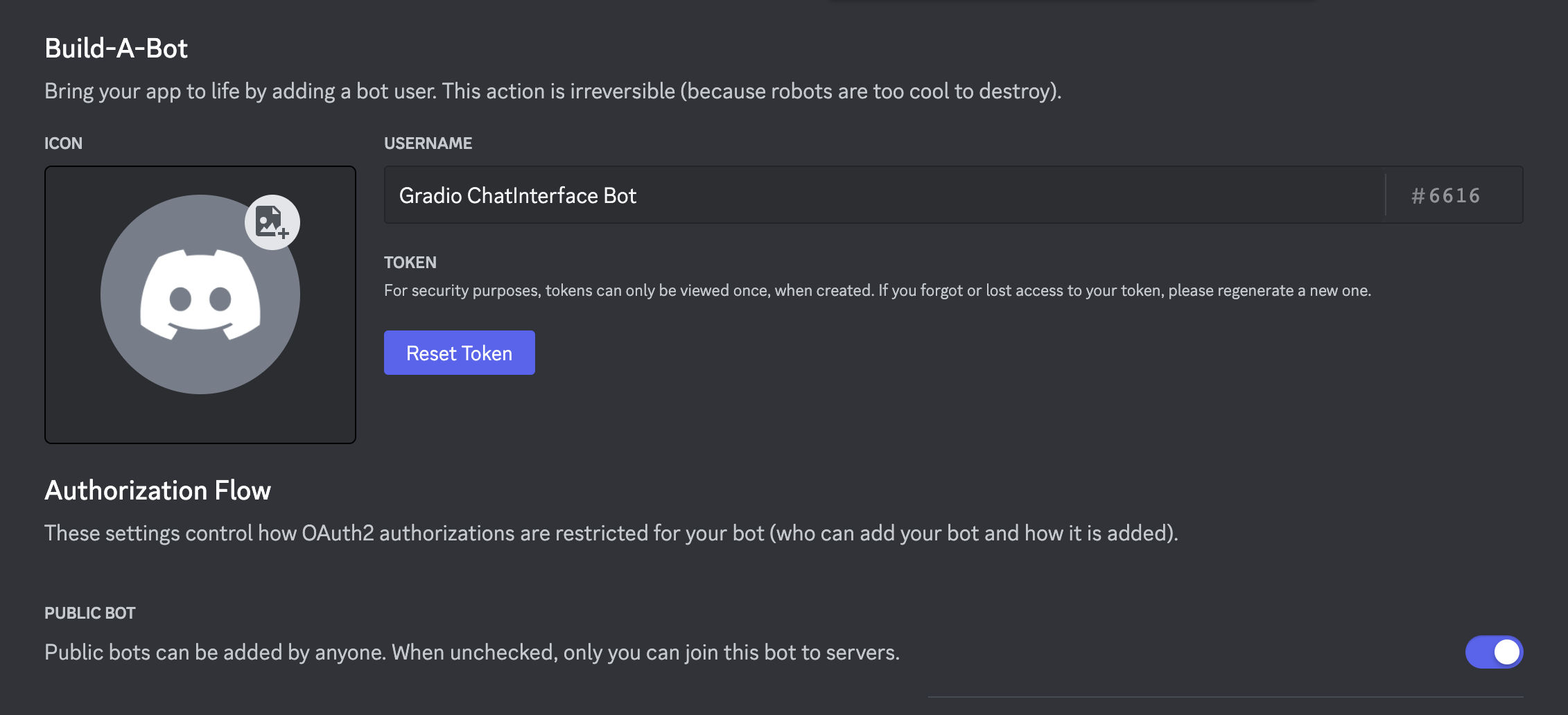
-
- ## 4. Optionally make the bot public if you want anyone to be able to add it to their servers
-
- ## 5. Scroll down and enable 'Message Content Intent' under 'Priviledged Gateway Intents'
-
- 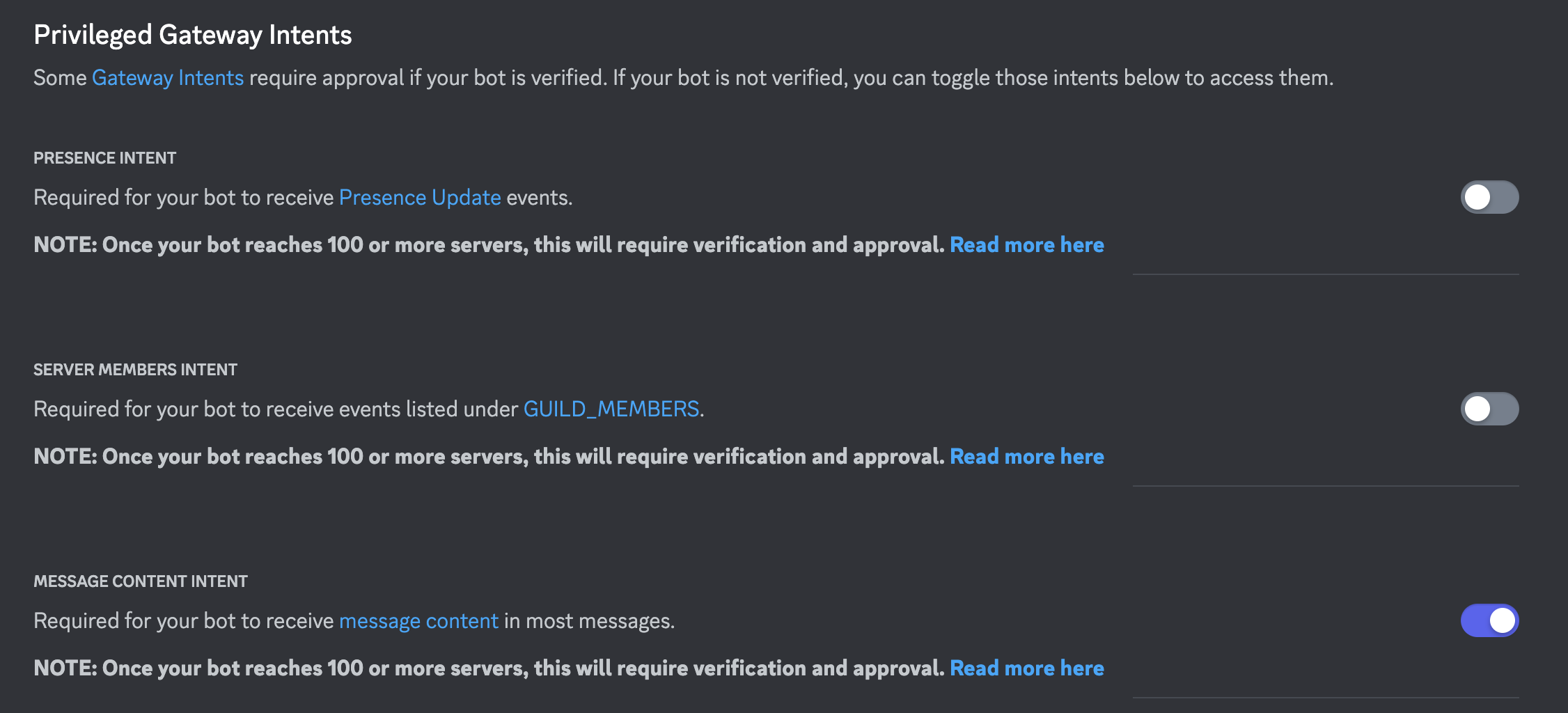
-
- ## 6. Save your changes!
-
- ## 7. The token from step 3 is the DISCORD_TOKEN. Rerun the deploy_discord command, e.g client.deploy_discord(discord_bot_token=DISCORD_TOKEN, ...), or add the token as a space secret manually.
-"""
-else:
- permissions = Permissions(326417525824)
- url = oauth_url(bot.user.id, permissions=permissions)
- welcome_message = f"""
- ## Add this bot to your server by clicking this link:
-
- {url}
-
- ## How to use it?
-
- The bot can be triggered via `/llamachat` followed by your text prompt.
-
- This will create a thread with the bot's response to your text prompt.
- You can reply in the thread (without `/llamachat`) to continue the conversation.
- In the thread, the bot will only reply to the original author of the command.
-
- ⚠️ Note ⚠️: Please make sure this bot's command does have the same name as another command in your server.
- ⚠️ Note ⚠️: Bot commands do not work in DMs with the bot as of now.
- """
-
-
-with gr.Blocks() as demo:
- gr.Markdown(
- f"""
- # Discord bot of https://ysharma-explore-llamav2-with-tgi.hf.space
- {welcome_message}
- """
- )
-
-demo.launch()
diff --git a/spaces/freddyaboulton/openai-whisper-large/README.md b/spaces/freddyaboulton/openai-whisper-large/README.md
deleted file mode 100644
index f4e0c32c81c3c734049decd5d4391b848aaf7b70..0000000000000000000000000000000000000000
--- a/spaces/freddyaboulton/openai-whisper-large/README.md
+++ /dev/null
@@ -1,13 +0,0 @@
----
-title: Openai Whisper Large
-emoji: 🌖
-colorFrom: blue
-colorTo: red
-sdk: gradio
-sdk_version: 3.12.0
-app_file: app.py
-pinned: false
-license: mit
----
-
-Check out the configuration reference at https://huggingface.co/docs/hub/spaces-config-reference
diff --git a/spaces/georgefen/Face-Landmark-ControlNet/annotator/uniformer/mmcv/ops/pixel_group.py b/spaces/georgefen/Face-Landmark-ControlNet/annotator/uniformer/mmcv/ops/pixel_group.py
deleted file mode 100644
index 2143c75f835a467c802fc3c37ecd3ac0f85bcda4..0000000000000000000000000000000000000000
--- a/spaces/georgefen/Face-Landmark-ControlNet/annotator/uniformer/mmcv/ops/pixel_group.py
+++ /dev/null
@@ -1,75 +0,0 @@
-# Copyright (c) OpenMMLab. All rights reserved.
-import numpy as np
-import torch
-
-from ..utils import ext_loader
-
-ext_module = ext_loader.load_ext('_ext', ['pixel_group'])
-
-
-def pixel_group(score, mask, embedding, kernel_label, kernel_contour,
- kernel_region_num, distance_threshold):
- """Group pixels into text instances, which is widely used text detection
- methods.
-
- Arguments:
- score (np.array or Tensor): The foreground score with size hxw.
- mask (np.array or Tensor): The foreground mask with size hxw.
- embedding (np.array or Tensor): The embedding with size hxwxc to
- distinguish instances.
- kernel_label (np.array or Tensor): The instance kernel index with
- size hxw.
- kernel_contour (np.array or Tensor): The kernel contour with size hxw.
- kernel_region_num (int): The instance kernel region number.
- distance_threshold (float): The embedding distance threshold between
- kernel and pixel in one instance.
-
- Returns:
- pixel_assignment (List[List[float]]): The instance coordinate list.
- Each element consists of averaged confidence, pixel number, and
- coordinates (x_i, y_i for all pixels) in order.
- """
- assert isinstance(score, (torch.Tensor, np.ndarray))
- assert isinstance(mask, (torch.Tensor, np.ndarray))
- assert isinstance(embedding, (torch.Tensor, np.ndarray))
- assert isinstance(kernel_label, (torch.Tensor, np.ndarray))
- assert isinstance(kernel_contour, (torch.Tensor, np.ndarray))
- assert isinstance(kernel_region_num, int)
- assert isinstance(distance_threshold, float)
-
- if isinstance(score, np.ndarray):
- score = torch.from_numpy(score)
- if isinstance(mask, np.ndarray):
- mask = torch.from_numpy(mask)
- if isinstance(embedding, np.ndarray):
- embedding = torch.from_numpy(embedding)
- if isinstance(kernel_label, np.ndarray):
- kernel_label = torch.from_numpy(kernel_label)
- if isinstance(kernel_contour, np.ndarray):
- kernel_contour = torch.from_numpy(kernel_contour)
-
- if torch.__version__ == 'parrots':
- label = ext_module.pixel_group(
- score,
- mask,
- embedding,
- kernel_label,
- kernel_contour,
- kernel_region_num=kernel_region_num,
- distance_threshold=distance_threshold)
- label = label.tolist()
- label = label[0]
- list_index = kernel_region_num
- pixel_assignment = []
- for x in range(kernel_region_num):
- pixel_assignment.append(
- np.array(
- label[list_index:list_index + int(label[x])],
- dtype=np.float))
- list_index = list_index + int(label[x])
- else:
- pixel_assignment = ext_module.pixel_group(score, mask, embedding,
- kernel_label, kernel_contour,
- kernel_region_num,
- distance_threshold)
- return pixel_assignment
diff --git a/spaces/georgefen/Face-Landmark-ControlNet/annotator/uniformer/mmseg/models/losses/utils.py b/spaces/georgefen/Face-Landmark-ControlNet/annotator/uniformer/mmseg/models/losses/utils.py
deleted file mode 100644
index 85aec9f3045240c3de96a928324ae8f5c3aebe8b..0000000000000000000000000000000000000000
--- a/spaces/georgefen/Face-Landmark-ControlNet/annotator/uniformer/mmseg/models/losses/utils.py
+++ /dev/null
@@ -1,121 +0,0 @@
-import functools
-
-import annotator.uniformer.mmcv as mmcv
-import numpy as np
-import torch.nn.functional as F
-
-
-def get_class_weight(class_weight):
- """Get class weight for loss function.
-
- Args:
- class_weight (list[float] | str | None): If class_weight is a str,
- take it as a file name and read from it.
- """
- if isinstance(class_weight, str):
- # take it as a file path
- if class_weight.endswith('.npy'):
- class_weight = np.load(class_weight)
- else:
- # pkl, json or yaml
- class_weight = mmcv.load(class_weight)
-
- return class_weight
-
-
-def reduce_loss(loss, reduction):
- """Reduce loss as specified.
-
- Args:
- loss (Tensor): Elementwise loss tensor.
- reduction (str): Options are "none", "mean" and "sum".
-
- Return:
- Tensor: Reduced loss tensor.
- """
- reduction_enum = F._Reduction.get_enum(reduction)
- # none: 0, elementwise_mean:1, sum: 2
- if reduction_enum == 0:
- return loss
- elif reduction_enum == 1:
- return loss.mean()
- elif reduction_enum == 2:
- return loss.sum()
-
-
-def weight_reduce_loss(loss, weight=None, reduction='mean', avg_factor=None):
- """Apply element-wise weight and reduce loss.
-
- Args:
- loss (Tensor): Element-wise loss.
- weight (Tensor): Element-wise weights.
- reduction (str): Same as built-in losses of PyTorch.
- avg_factor (float): Avarage factor when computing the mean of losses.
-
- Returns:
- Tensor: Processed loss values.
- """
- # if weight is specified, apply element-wise weight
- if weight is not None:
- assert weight.dim() == loss.dim()
- if weight.dim() > 1:
- assert weight.size(1) == 1 or weight.size(1) == loss.size(1)
- loss = loss * weight
-
- # if avg_factor is not specified, just reduce the loss
- if avg_factor is None:
- loss = reduce_loss(loss, reduction)
- else:
- # if reduction is mean, then average the loss by avg_factor
- if reduction == 'mean':
- loss = loss.sum() / avg_factor
- # if reduction is 'none', then do nothing, otherwise raise an error
- elif reduction != 'none':
- raise ValueError('avg_factor can not be used with reduction="sum"')
- return loss
-
-
-def weighted_loss(loss_func):
- """Create a weighted version of a given loss function.
-
- To use this decorator, the loss function must have the signature like
- `loss_func(pred, target, **kwargs)`. The function only needs to compute
- element-wise loss without any reduction. This decorator will add weight
- and reduction arguments to the function. The decorated function will have
- the signature like `loss_func(pred, target, weight=None, reduction='mean',
- avg_factor=None, **kwargs)`.
-
- :Example:
-
- >>> import torch
- >>> @weighted_loss
- >>> def l1_loss(pred, target):
- >>> return (pred - target).abs()
-
- >>> pred = torch.Tensor([0, 2, 3])
- >>> target = torch.Tensor([1, 1, 1])
- >>> weight = torch.Tensor([1, 0, 1])
-
- >>> l1_loss(pred, target)
- tensor(1.3333)
- >>> l1_loss(pred, target, weight)
- tensor(1.)
- >>> l1_loss(pred, target, reduction='none')
- tensor([1., 1., 2.])
- >>> l1_loss(pred, target, weight, avg_factor=2)
- tensor(1.5000)
- """
-
- @functools.wraps(loss_func)
- def wrapper(pred,
- target,
- weight=None,
- reduction='mean',
- avg_factor=None,
- **kwargs):
- # get element-wise loss
- loss = loss_func(pred, target, **kwargs)
- loss = weight_reduce_loss(loss, weight, reduction, avg_factor)
- return loss
-
- return wrapper
diff --git a/spaces/georgefen/Face-Landmark-ControlNet/ldm/modules/ema.py b/spaces/georgefen/Face-Landmark-ControlNet/ldm/modules/ema.py
deleted file mode 100644
index bded25019b9bcbcd0260f0b8185f8c7859ca58c4..0000000000000000000000000000000000000000
--- a/spaces/georgefen/Face-Landmark-ControlNet/ldm/modules/ema.py
+++ /dev/null
@@ -1,80 +0,0 @@
-import torch
-from torch import nn
-
-
-class LitEma(nn.Module):
- def __init__(self, model, decay=0.9999, use_num_upates=True):
- super().__init__()
- if decay < 0.0 or decay > 1.0:
- raise ValueError('Decay must be between 0 and 1')
-
- self.m_name2s_name = {}
- self.register_buffer('decay', torch.tensor(decay, dtype=torch.float32))
- self.register_buffer('num_updates', torch.tensor(0, dtype=torch.int) if use_num_upates
- else torch.tensor(-1, dtype=torch.int))
-
- for name, p in model.named_parameters():
- if p.requires_grad:
- # remove as '.'-character is not allowed in buffers
- s_name = name.replace('.', '')
- self.m_name2s_name.update({name: s_name})
- self.register_buffer(s_name, p.clone().detach().data)
-
- self.collected_params = []
-
- def reset_num_updates(self):
- del self.num_updates
- self.register_buffer('num_updates', torch.tensor(0, dtype=torch.int))
-
- def forward(self, model):
- decay = self.decay
-
- if self.num_updates >= 0:
- self.num_updates += 1
- decay = min(self.decay, (1 + self.num_updates) / (10 + self.num_updates))
-
- one_minus_decay = 1.0 - decay
-
- with torch.no_grad():
- m_param = dict(model.named_parameters())
- shadow_params = dict(self.named_buffers())
-
- for key in m_param:
- if m_param[key].requires_grad:
- sname = self.m_name2s_name[key]
- shadow_params[sname] = shadow_params[sname].type_as(m_param[key])
- shadow_params[sname].sub_(one_minus_decay * (shadow_params[sname] - m_param[key]))
- else:
- assert not key in self.m_name2s_name
-
- def copy_to(self, model):
- m_param = dict(model.named_parameters())
- shadow_params = dict(self.named_buffers())
- for key in m_param:
- if m_param[key].requires_grad:
- m_param[key].data.copy_(shadow_params[self.m_name2s_name[key]].data)
- else:
- assert not key in self.m_name2s_name
-
- def store(self, parameters):
- """
- Save the current parameters for restoring later.
- Args:
- parameters: Iterable of `torch.nn.Parameter`; the parameters to be
- temporarily stored.
- """
- self.collected_params = [param.clone() for param in parameters]
-
- def restore(self, parameters):
- """
- Restore the parameters stored with the `store` method.
- Useful to validate the model with EMA parameters without affecting the
- original optimization process. Store the parameters before the
- `copy_to` method. After validation (or model saving), use this to
- restore the former parameters.
- Args:
- parameters: Iterable of `torch.nn.Parameter`; the parameters to be
- updated with the stored parameters.
- """
- for c_param, param in zip(self.collected_params, parameters):
- param.data.copy_(c_param.data)
diff --git a/spaces/gordonchan/h2oo/gradio_utils/prompt_form.py b/spaces/gordonchan/h2oo/gradio_utils/prompt_form.py
deleted file mode 100644
index 34707d44de1d9eb21b7caef4e5345b11c4c9bd28..0000000000000000000000000000000000000000
--- a/spaces/gordonchan/h2oo/gradio_utils/prompt_form.py
+++ /dev/null
@@ -1,95 +0,0 @@
-import os
-import math
-
-import gradio as gr
-
-
-def make_chatbots(output_label0, output_label0_model2, **kwargs):
- text_outputs = []
- chat_kwargs = []
- for model_state_lock in kwargs['model_states']:
- if os.environ.get('DEBUG_MODEL_LOCK'):
- model_name = model_state_lock["base_model"] + " : " + model_state_lock["inference_server"]
- else:
- model_name = model_state_lock["base_model"]
- output_label = f'h2oGPT [{model_name}]'
- min_width = 250 if kwargs['gradio_size'] in ['small', 'large', 'medium'] else 160
- chat_kwargs.append(dict(label=output_label, visible=kwargs['model_lock'], elem_classes='chatsmall',
- height=kwargs['height'] or 400, min_width=min_width))
-
- if kwargs['model_lock_columns'] == -1:
- kwargs['model_lock_columns'] = len(kwargs['model_states'])
- if kwargs['model_lock_columns'] is None:
- kwargs['model_lock_columns'] = 3
-
- ncols = kwargs['model_lock_columns']
- if kwargs['model_states'] == 0:
- nrows = 0
- else:
- nrows = math.ceil(len(kwargs['model_states']) / kwargs['model_lock_columns'])
-
- if kwargs['model_lock_columns'] == 0:
- # not using model_lock
- pass
- elif nrows <= 1:
- with gr.Row():
- for chat_kwargs1, model_state_lock in zip(chat_kwargs, kwargs['model_states']):
- text_outputs.append(gr.Chatbot(**chat_kwargs1))
- elif nrows == kwargs['model_states']:
- with gr.Row():
- for chat_kwargs1, model_state_lock in zip(chat_kwargs, kwargs['model_states']):
- text_outputs.append(gr.Chatbot(**chat_kwargs1))
- elif nrows == 2:
- with gr.Row():
- for mii, (chat_kwargs1, model_state_lock) in enumerate(zip(chat_kwargs, kwargs['model_states'])):
- if mii >= len(kwargs['model_states']) / 2:
- continue
- text_outputs.append(gr.Chatbot(**chat_kwargs1))
- with gr.Row():
- for mii, (chat_kwargs1, model_state_lock) in enumerate(zip(chat_kwargs, kwargs['model_states'])):
- if mii < len(kwargs['model_states']) / 2:
- continue
- text_outputs.append(gr.Chatbot(**chat_kwargs1))
- elif nrows == 3:
- with gr.Row():
- for mii, (chat_kwargs1, model_state_lock) in enumerate(zip(chat_kwargs, kwargs['model_states'])):
- if mii >= 1 * len(kwargs['model_states']) / 3:
- continue
- text_outputs.append(gr.Chatbot(**chat_kwargs1))
- with gr.Row():
- for mii, (chat_kwargs1, model_state_lock) in enumerate(zip(chat_kwargs, kwargs['model_states'])):
- if mii < 1 * len(kwargs['model_states']) / 3 or mii >= 2 * len(kwargs['model_states']) / 3:
- continue
- text_outputs.append(gr.Chatbot(**chat_kwargs1))
- with gr.Row():
- for mii, (chat_kwargs1, model_state_lock) in enumerate(zip(chat_kwargs, kwargs['model_states'])):
- if mii < 2 * len(kwargs['model_states']) / 3:
- continue
- text_outputs.append(gr.Chatbot(**chat_kwargs1))
- elif nrows >= 4:
- with gr.Row():
- for mii, (chat_kwargs1, model_state_lock) in enumerate(zip(chat_kwargs, kwargs['model_states'])):
- if mii >= 1 * len(kwargs['model_states']) / 4:
- continue
- text_outputs.append(gr.Chatbot(**chat_kwargs1))
- with gr.Row():
- for mii, (chat_kwargs1, model_state_lock) in enumerate(zip(chat_kwargs, kwargs['model_states'])):
- if mii < 1 * len(kwargs['model_states']) / 4 or mii >= 2 * len(kwargs['model_states']) / 4:
- continue
- text_outputs.append(gr.Chatbot(**chat_kwargs1))
- with gr.Row():
- for mii, (chat_kwargs1, model_state_lock) in enumerate(zip(chat_kwargs, kwargs['model_states'])):
- if mii < 2 * len(kwargs['model_states']) / 4 or mii >= 3 * len(kwargs['model_states']) / 4:
- continue
- text_outputs.append(gr.Chatbot(**chat_kwargs1))
- with gr.Row():
- for mii, (chat_kwargs1, model_state_lock) in enumerate(zip(chat_kwargs, kwargs['model_states'])):
- if mii < 3 * len(kwargs['model_states']) / 4:
- continue
- text_outputs.append(gr.Chatbot(**chat_kwargs1))
-
- with gr.Row():
- text_output = gr.Chatbot(label=output_label0, visible=not kwargs['model_lock'], height=kwargs['height'] or 400)
- text_output2 = gr.Chatbot(label=output_label0_model2,
- visible=False and not kwargs['model_lock'], height=kwargs['height'] or 400)
- return text_output, text_output2, text_outputs
diff --git a/spaces/gotiQspiryo/whisper-ui/Pad-Man-Tamil-Movie-Torrent-Download-VERIFIED.md b/spaces/gotiQspiryo/whisper-ui/Pad-Man-Tamil-Movie-Torrent-Download-VERIFIED.md
deleted file mode 100644
index 9f0f2ab985360533bba64fada5bca6203e84ecd9..0000000000000000000000000000000000000000
--- a/spaces/gotiQspiryo/whisper-ui/Pad-Man-Tamil-Movie-Torrent-Download-VERIFIED.md
+++ /dev/null
@@ -1,64 +0,0 @@
-## Pad Man Tamil Movie Torrent Download
-
-
-
-
-
-
-
-
-
-**DOWNLOAD > [https://vercupalo.blogspot.com/?d=2txnFN](https://vercupalo.blogspot.com/?d=2txnFN)**
-
-
-
-
-
-
-
-
-
-
-
-
-
-# Pad Man: A Movie Review
-
-
-
-Pad Man is a 2018 biographical comedy-drama film that tells the inspiring story of Arunachalam Muruganantham, a social activist and entrepreneur from Coimbatore, Tamil Nadu who made low-cost sanitary pads for women in rural areas[^2^]. The film stars Akshay Kumar as Muruganantham and Radhika Apte as his wife Gayatri. The film is directed by R. Balki and has an ensemble supporting cast that includes Sonam Kapoor, Amitabh Bachchan, Sudhir Pandey and others.
-
-
-
-The film follows Muruganantham's journey from being a simple welder to a national hero who revolutionized menstrual hygiene in India. He faces many challenges and obstacles along the way, such as social stigma, family opposition, financial difficulties and legal troubles. He also sacrifices his personal life and happiness for his noble cause. The film showcases his determination, innovation and courage in overcoming all odds and fulfilling his dream of making affordable sanitary pads for millions of women.
-
-
-
-Pad Man is a film that celebrates the spirit of innovation and social change. It also raises awareness about the importance of menstrual hygiene and women's empowerment. The film is based on a true story and is adapted from the book The Legend of Lakshmi Prasad by Twinkle Khanna, who is also one of the producers of the film. The film received positive reviews from critics and audiences alike and was a commercial success. It also won several awards and nominations, including the National Film Award for Best Film on Other Social Issues.
-
-
-
-Pad Man is a film that is worth watching for its inspiring message, brilliant performances and engaging direction. It is a film that will make you laugh, cry and think. It is a film that will make you proud of being an Indian.
-
-
-
-## Pad Man: A Movie Reception
-
-
-
-Pad Man received positive reviews from critics and audiences alike for its portrayal of a real-life hero and his social impact. The film was praised for its performances, direction, screenplay, music and message. The film also sparked a conversation about menstrual hygiene and women's rights in India and abroad. The film was also appreciated for its humor and emotion that balanced the serious subject matter.
-
-
-
-The film was a commercial success as well, grossing â¹207.73 crore worldwide against a budget of â¹45 crore. The film performed well especially in India and China, where it was released on 9 February 2018 and 14 December 2018 respectively. The film also became the first Hindi film to premiere at the Russia box office on its original date. The film won several awards and nominations, including the National Film Award for Best Film on Other Social Issues. The film was also selected as India's official entry for the 91st Academy Awards but did not make the shortlist.
-
-
-
-Pad Man is a film that has made a difference in the lives of many people. It has inspired many initiatives and campaigns to promote menstrual hygiene and awareness. It has also inspired many people to follow their dreams and passions despite the challenges they face. Pad Man is a film that has left a lasting impression on the hearts and minds of the viewers.
-
- 1b8d091108
-
-
-
-
-
diff --git "a/spaces/gotiQspiryo/whisper-ui/examples/After Effect\302\240Template Torrent.md" "b/spaces/gotiQspiryo/whisper-ui/examples/After Effect\302\240Template Torrent.md"
deleted file mode 100644
index 5558e1318693bb597cb341c75b68e0910e05d20b..0000000000000000000000000000000000000000
--- "a/spaces/gotiQspiryo/whisper-ui/examples/After Effect\302\240Template Torrent.md"
+++ /dev/null
@@ -1,6 +0,0 @@
-
-
-Korg Pa Serial Number, key, crack, keygen. Style Works 2000 Korg Pa is a. Export your style first, execute the desired changes and then import it again to ... 4d29de3e1b
-
-
-
diff --git a/spaces/inreVtussa/clothingai/Examples/BassBox Pro 6.0.2 Full Version LINK.md b/spaces/inreVtussa/clothingai/Examples/BassBox Pro 6.0.2 Full Version LINK.md
deleted file mode 100644
index 0d474eeffb046f4aba0920eaaa666210c9b64dc2..0000000000000000000000000000000000000000
--- a/spaces/inreVtussa/clothingai/Examples/BassBox Pro 6.0.2 Full Version LINK.md
+++ /dev/null
@@ -1,41 +0,0 @@
-
-
How to Design World-Class Speaker Boxes with BassBox Pro 6.0.2 Full Version
-
-
If you are looking for a state-of-the-art speaker enclosure design program that is used by professional and amateur speaker system designers around the world, you should check out BassBox Pro 6.0.2 full version. This software allows you to design speakers for a wide variety of applications, including home hi-fi, home theater, car, truck, van, pro sound reinforcement, recording studio monitors, stage monitors, PA, musical instruments, and more.
-
-
BassBox Pro 6.0.2 full version has many features that make it very easy to learn and use. For example, when you run the program for the first time, a Welcome window will appear to help you configure the program and introduce you to its on-screen manual. The on-screen manual is extensive and contains most of the same information as the beautiful 364-page printed manual that comes with the software. Both the on-screen and printed manuals include a "Box Designer's Guide", "Sample Designs" and "BassBox Pro Reference".
BassBox Pro 6.0.2 full version also includes a "balloon" help feature that provides definitions or explanations of any control or label when you hover your mouse pointer over it. This way, you can get instant feedback and guidance as you use the program.
-
-
Box Design is as Easy as 1-2-3 with BassBox Pro 6.0.2 Full Version
-
-
There are many ways to begin a speaker design with BassBox Pro 6.0.2 full version, including the use of its innovative Design Wizard. In general, speaker design involves the following steps:
-
-
-
Enter the driver parameters such as Fs, Vas and Qts. BassBox Pro 6.0.2 full version's driver database contains parameters for thousands of drivers. You can also add, edit or delete drivers and use various search methods to find the best match for your project.
-
Calculate the box volume and tuning. This is very easy with the program's helpful "Suggest" button that will recommend a box that will produce a smooth bass response.
-
Evaluate the performance with the graphs. This can include the effects of the acoustical environment such as in-car response or room modes. You can also import acoustic data from several popular measurement systems and include the effects of an external passive network or an active equalization filter.
-
-
-
Each box design can be saved and re-opened or re-used later. You can also duplicate designs so that you don't have to re-enter driver or box information. You can have up to ten different designs open at the same time and compare them with mini preview graphs that show their amplitude response.
-
-
Design Wizard Helps New Users Quickly Design a Speaker
-
-
BassBox Pro 6.0.2 full version includes a powerful Design Wizard that helps new users quickly design a speaker. It can start with either the driver or the box and then it will walk you through BassBox Pro 6.0.2 full version as it prompts you for information in an orderly progression. In this way, it serves as a "smart" assistant to help you use the program.
-
-
Main Window Shows All Open Designs
-
-
The centerpiece of BassBox Pro 6.0.2 full version is its resizable main window that shows a summary of all open designs (see screenshot below). A small picture beside each mini graph displays the box type that has been selected (closed, vented, bandpass or passive radiator). You can also access all the features and functions of BassBox Pro 6.0.2 full version from this window.
-
-
-
-
Other Features of BassBox Pro 6.0.2 Full Version
-
-
BassBox Pro 6.0.2 full version has many other features that make it a versatile and powerful speaker enclosure design program, such as:
-
-
-
-
It is compatible with Microsoft Windows 7, Vista, XP, d5da3c52bf
-
-
\ No newline at end of file
diff --git a/spaces/inreVtussa/clothingai/Examples/Descargar Zarastudio 22 Full PORTABLE Para.md b/spaces/inreVtussa/clothingai/Examples/Descargar Zarastudio 22 Full PORTABLE Para.md
deleted file mode 100644
index 75109a7404021a91b2e14f9e70aed59845743390..0000000000000000000000000000000000000000
--- a/spaces/inreVtussa/clothingai/Examples/Descargar Zarastudio 22 Full PORTABLE Para.md
+++ /dev/null
@@ -1,46 +0,0 @@
-
-
¿Cómo descargar Zarastudio 22 Full Para tu emisora de radio?
-
Zarastudio es un software de automatización de radio que te permite gestionar y programar tu emisora de forma fácil y profesional. Con Zarastudio 22 Full Para, puedes disfrutar de todas las ventajas y funciones de este programa sin limitaciones ni restricciones.
Zarastudio 22 Full Para te ofrece una serie de funciones y herramientas que te ayudarán a crear y gestionar tu emisora de radio de forma eficiente y profesional. Algunas de estas funciones son:
-
-
Reproducción automática de música, jingles, cuñas, publicidad, noticias, etc.
-
Programación de eventos y tareas por horas, dÃas o semanas.
-
Control de niveles de audio, ecualización, compresión, limitación, etc.
-
Generación de listas de reproducción con criterios personalizados.
-
Soporte para múltiples formatos de audio: MP3, WAV, OGG, WMA, etc.
-
Integración con bases de datos externas: MySQL, SQL Server, Access, etc.
-
Emisión en directo por Internet o por FM.
-
Grabación y edición de audio con el editor integrado o con programas externos.
-
Generación de informes y estadÃsticas de la emisora.
-
-
Con Zarastudio 22 Full Para, podrás crear tu propia emisora de radio con un estilo único y profesional, adaptado a tus gustos y necesidades. Zarastudio es el software de automatización de radio más utilizado y recomendado por miles de usuarios en todo el mundo.
-
-
-
Para crear una lista de reproducción con Zarastudio 22 Full Para, solo tienes que seguir estos pasos:
-
-
Haz clic en el botón "Lista" en la parte superior del programa.
-
Haz clic en el botón "Nueva lista" para crear una lista vacÃa.
-
Haz clic en el botón "Añadir" para agregar archivos de audio a la lista. Puedes seleccionar varios archivos a la vez o arrastrarlos desde el explorador de Windows.
Asà de fácil es crear una lista de reproducción con Zarastudio 22 Full Para. Puedes crear tantas listas como quieras y programarlas para que se reproduzcan automáticamente según tu horario. Zarastudio te permite tener un control total sobre tu emisora de radio.
d5da3c52bf
-
-
\ No newline at end of file
diff --git a/spaces/inreVtussa/clothingai/Examples/Deskscapes 8 EXCLUSIVE Crack Kickass Torrents.md b/spaces/inreVtussa/clothingai/Examples/Deskscapes 8 EXCLUSIVE Crack Kickass Torrents.md
deleted file mode 100644
index 019c55ebea0510155e1993f9b481dbfbb2b129e4..0000000000000000000000000000000000000000
--- a/spaces/inreVtussa/clothingai/Examples/Deskscapes 8 EXCLUSIVE Crack Kickass Torrents.md
+++ /dev/null
@@ -1,6 +0,0 @@
-
-
- 4d29de3e1b
-
-
-
diff --git a/spaces/jayvaghasiya/winerybarreloak/app.py b/spaces/jayvaghasiya/winerybarreloak/app.py
deleted file mode 100644
index 656653cf6a0ebd395244928da958f43d09a0a7b6..0000000000000000000000000000000000000000
--- a/spaces/jayvaghasiya/winerybarreloak/app.py
+++ /dev/null
@@ -1,33 +0,0 @@
-# This Script will create a user frindly interface fo demonstrartion purpose.
-
-import openai
-import gradio as gr
-import sys
-import os
-
-openai.api_key = "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"
-
-
-def chatbot(input_text):
- response = openai.Completion.create(
- model="davinci:ft-personal:human-like-wine-prediction-2023-06-28-13-43-11",
- prompt=f"{input_text} ->",
- temperature=1,
- max_tokens=250,
- top_p=1,
- frequency_penalty=0,
- presence_penalty=0,
- stop=["END"],
- )
- return response["choices"][0]["text"]
-
-
-iface = gr.Interface(
- fn=chatbot,
- inputs=gr.components.Textbox(lines=7, label="Enter your text"),
- outputs="text",
- title="AI for Wine Recommendation",
-)
-
-# index = construct_index("data")
-iface.launch(enable_queue=False)
diff --git a/spaces/jbilcke-hf/LifeSim/Dockerfile b/spaces/jbilcke-hf/LifeSim/Dockerfile
deleted file mode 100644
index 91319be9b3dd35d916d18fba5260f51125c46b50..0000000000000000000000000000000000000000
--- a/spaces/jbilcke-hf/LifeSim/Dockerfile
+++ /dev/null
@@ -1,65 +0,0 @@
-FROM node:18-alpine AS base
-
-# Install dependencies only when needed
-FROM base AS deps
-# Check https://github.com/nodejs/docker-node/tree/b4117f9333da4138b03a546ec926ef50a31506c3#nodealpine to understand why libc6-compat might be needed.
-RUN apk add --no-cache libc6-compat
-WORKDIR /app
-
-# Install dependencies based on the preferred package manager
-COPY package.json yarn.lock* package-lock.json* pnpm-lock.yaml* ./
-RUN \
- if [ -f yarn.lock ]; then yarn --frozen-lockfile; \
- elif [ -f package-lock.json ]; then npm ci; \
- elif [ -f pnpm-lock.yaml ]; then yarn global add pnpm && pnpm i --frozen-lockfile; \
- else echo "Lockfile not found." && exit 1; \
- fi
-
-# Uncomment the following lines if you want to use a secret at buildtime,
-# for example to access your private npm packages
-# RUN --mount=type=secret,id=HF_EXAMPLE_SECRET,mode=0444,required=true \
-# $(cat /run/secrets/HF_EXAMPLE_SECRET)
-
-# Rebuild the source code only when needed
-FROM base AS builder
-WORKDIR /app
-COPY --from=deps /app/node_modules ./node_modules
-COPY . .
-
-# Next.js collects completely anonymous telemetry data about general usage.
-# Learn more here: https://nextjs.org/telemetry
-# Uncomment the following line in case you want to disable telemetry during the build.
-# ENV NEXT_TELEMETRY_DISABLED 1
-
-# RUN yarn build
-
-# If you use yarn, comment out this line and use the line above
-RUN npm run build
-
-# Production image, copy all the files and run next
-FROM base AS runner
-WORKDIR /app
-
-ENV NODE_ENV production
-# Uncomment the following line in case you want to disable telemetry during runtime.
-# ENV NEXT_TELEMETRY_DISABLED 1
-
-RUN addgroup --system --gid 1001 nodejs
-RUN adduser --system --uid 1001 nextjs
-
-COPY --from=builder /app/public ./public
-
-# Automatically leverage output traces to reduce image size
-# https://nextjs.org/docs/advanced-features/output-file-tracing
-COPY --from=builder --chown=nextjs:nodejs /app/.next/standalone ./
-COPY --from=builder --chown=nextjs:nodejs /app/.next/static ./.next/static
-COPY --from=builder --chown=nextjs:nodejs /app/.next/cache ./.next/cache
-# COPY --from=builder --chown=nextjs:nodejs /app/.next/cache/fetch-cache ./.next/cache/fetch-cache
-
-USER nextjs
-
-EXPOSE 3000
-
-ENV PORT 3000
-
-CMD ["node", "server.js"]
\ No newline at end of file
diff --git a/spaces/jbilcke-hf/Panoremix/src/components/ui/slider.tsx b/spaces/jbilcke-hf/Panoremix/src/components/ui/slider.tsx
deleted file mode 100644
index 0e35bc7fb000cffa5e29956283ecf7d75453236c..0000000000000000000000000000000000000000
--- a/spaces/jbilcke-hf/Panoremix/src/components/ui/slider.tsx
+++ /dev/null
@@ -1,28 +0,0 @@
-"use client"
-
-import * as React from "react"
-import * as SliderPrimitive from "@radix-ui/react-slider"
-
-import { cn } from "@/lib/utils"
-
-const Slider = React.forwardRef<
- React.ElementRef,
- React.ComponentPropsWithoutRef
->(({ className, ...props }, ref) => (
-
-
-
-
-
-
-))
-Slider.displayName = SliderPrimitive.Root.displayName
-
-export { Slider }
diff --git a/spaces/jimschat/VITS-Umamusume-voice-synthesizer/ONNXVITS_inference.py b/spaces/jimschat/VITS-Umamusume-voice-synthesizer/ONNXVITS_inference.py
deleted file mode 100644
index 258b618cd338322365dfa25bec468a0a3f70ccd1..0000000000000000000000000000000000000000
--- a/spaces/jimschat/VITS-Umamusume-voice-synthesizer/ONNXVITS_inference.py
+++ /dev/null
@@ -1,36 +0,0 @@
-import logging
-logging.getLogger('numba').setLevel(logging.WARNING)
-import IPython.display as ipd
-import torch
-import commons
-import utils
-import ONNXVITS_infer
-from text import text_to_sequence
-
-def get_text(text, hps):
- text_norm = text_to_sequence(text, hps.symbols, hps.data.text_cleaners)
- if hps.data.add_blank:
- text_norm = commons.intersperse(text_norm, 0)
- text_norm = torch.LongTensor(text_norm)
- return text_norm
-
-hps = utils.get_hparams_from_file("../vits/pretrained_models/uma87.json")
-
-net_g = ONNXVITS_infer.SynthesizerTrn(
- len(hps.symbols),
- hps.data.filter_length // 2 + 1,
- hps.train.segment_size // hps.data.hop_length,
- n_speakers=hps.data.n_speakers,
- **hps.model)
-_ = net_g.eval()
-
-_ = utils.load_checkpoint("../vits/pretrained_models/uma_1153000.pth", net_g)
-
-text1 = get_text("おはようございます。", hps)
-stn_tst = text1
-with torch.no_grad():
- x_tst = stn_tst.unsqueeze(0)
- x_tst_lengths = torch.LongTensor([stn_tst.size(0)])
- sid = torch.LongTensor([0])
- audio = net_g.infer(x_tst, x_tst_lengths, sid=sid, noise_scale=.667, noise_scale_w=0.8, length_scale=1)[0][0,0].data.cpu().float().numpy()
-print(audio)
\ No newline at end of file
diff --git a/spaces/joaopereirajp/livvieChatBot/venv/lib/python3.9/site-packages/contourpy/util/mpl_renderer.py b/spaces/joaopereirajp/livvieChatBot/venv/lib/python3.9/site-packages/contourpy/util/mpl_renderer.py
deleted file mode 100644
index dbcb5ca19a01e3ae000986673d66def23f9c2eac..0000000000000000000000000000000000000000
--- a/spaces/joaopereirajp/livvieChatBot/venv/lib/python3.9/site-packages/contourpy/util/mpl_renderer.py
+++ /dev/null
@@ -1,613 +0,0 @@
-from __future__ import annotations
-
-import io
-from typing import TYPE_CHECKING, Any, cast
-
-import matplotlib.collections as mcollections
-import matplotlib.pyplot as plt
-import numpy as np
-
-from contourpy import FillType, LineType
-from contourpy.util.mpl_util import filled_to_mpl_paths, lines_to_mpl_paths, mpl_codes_to_offsets
-from contourpy.util.renderer import Renderer
-
-if TYPE_CHECKING:
- from matplotlib.axes import Axes
- from matplotlib.figure import Figure
- from numpy.typing import ArrayLike
-
- import contourpy._contourpy as cpy
-
-
-class MplRenderer(Renderer):
- _axes: Axes
- _fig: Figure
- _want_tight: bool
-
- """Utility renderer using Matplotlib to render a grid of plots over the same (x, y) range.
-
- Args:
- nrows (int, optional): Number of rows of plots, default ``1``.
- ncols (int, optional): Number of columns of plots, default ``1``.
- figsize (tuple(float, float), optional): Figure size in inches, default ``(9, 9)``.
- show_frame (bool, optional): Whether to show frame and axes ticks, default ``True``.
- backend (str, optional): Matplotlib backend to use or ``None`` for default backend.
- Default ``None``.
- gridspec_kw (dict, optional): Gridspec keyword arguments to pass to ``plt.subplots``,
- default None.
- """
- def __init__(
- self,
- nrows: int = 1,
- ncols: int = 1,
- figsize: tuple[float, float] = (9, 9),
- show_frame: bool = True,
- backend: str | None = None,
- gridspec_kw: dict[str, Any] | None = None,
- ) -> None:
- if backend is not None:
- import matplotlib
- matplotlib.use(backend)
-
- kwargs = dict(figsize=figsize, squeeze=False, sharex=True, sharey=True)
- if gridspec_kw is not None:
- kwargs["gridspec_kw"] = gridspec_kw
- else:
- kwargs["subplot_kw"] = dict(aspect="equal")
-
- self._fig, axes = plt.subplots(nrows, ncols, **kwargs)
- self._axes = axes.flatten()
- if not show_frame:
- for ax in self._axes:
- ax.axis("off")
-
- self._want_tight = True
-
- def __del__(self) -> None:
- if hasattr(self, "_fig"):
- plt.close(self._fig)
-
- def _autoscale(self) -> None:
- # Using axes._need_autoscale attribute if need to autoscale before rendering after adding
- # lines/filled. Only want to autoscale once per axes regardless of how many lines/filled
- # added.
- for ax in self._axes:
- if getattr(ax, "_need_autoscale", False):
- ax.autoscale_view(tight=True)
- ax._need_autoscale = False
- if self._want_tight and len(self._axes) > 1:
- self._fig.tight_layout()
-
- def _get_ax(self, ax: Axes | int) -> Axes:
- if isinstance(ax, int):
- ax = self._axes[ax]
- return ax
-
- def filled(
- self,
- filled: cpy.FillReturn,
- fill_type: FillType,
- ax: Axes | int = 0,
- color: str = "C0",
- alpha: float = 0.7,
- ) -> None:
- """Plot filled contours on a single Axes.
-
- Args:
- filled (sequence of arrays): Filled contour data as returned by
- :func:`~contourpy.ContourGenerator.filled`.
- fill_type (FillType): Type of ``filled`` data, as returned by
- :attr:`~contourpy.ContourGenerator.fill_type`.
- ax (int or Maplotlib Axes, optional): Which axes to plot on, default ``0``.
- color (str, optional): Color to plot with. May be a string color or the letter ``"C"``
- followed by an integer in the range ``"C0"`` to ``"C9"`` to use a color from the
- ``tab10`` colormap. Default ``"C0"``.
- alpha (float, optional): Opacity to plot with, default ``0.7``.
- """
- ax = self._get_ax(ax)
- paths = filled_to_mpl_paths(filled, fill_type)
- collection = mcollections.PathCollection(
- paths, facecolors=color, edgecolors="none", lw=0, alpha=alpha)
- ax.add_collection(collection)
- ax._need_autoscale = True
-
- def grid(
- self,
- x: ArrayLike,
- y: ArrayLike,
- ax: Axes | int = 0,
- color: str = "black",
- alpha: float = 0.1,
- point_color: str | None = None,
- quad_as_tri_alpha: float = 0,
- ) -> None:
- """Plot quad grid lines on a single Axes.
-
- Args:
- x (array-like of shape (ny, nx) or (nx,)): The x-coordinates of the grid points.
- y (array-like of shape (ny, nx) or (ny,)): The y-coordinates of the grid points.
- ax (int or Matplotlib Axes, optional): Which Axes to plot on, default ``0``.
- color (str, optional): Color to plot grid lines, default ``"black"``.
- alpha (float, optional): Opacity to plot lines with, default ``0.1``.
- point_color (str, optional): Color to plot grid points or ``None`` if grid points
- should not be plotted, default ``None``.
- quad_as_tri_alpha (float, optional): Opacity to plot ``quad_as_tri`` grid, default 0.
-
- Colors may be a string color or the letter ``"C"`` followed by an integer in the range
- ``"C0"`` to ``"C9"`` to use a color from the ``tab10`` colormap.
-
- Warning:
- ``quad_as_tri_alpha > 0`` plots all quads as though they are unmasked.
- """
- ax = self._get_ax(ax)
- x, y = self._grid_as_2d(x, y)
- kwargs = dict(color=color, alpha=alpha)
- ax.plot(x, y, x.T, y.T, **kwargs)
- if quad_as_tri_alpha > 0:
- # Assumes no quad mask.
- xmid = 0.25*(x[:-1, :-1] + x[1:, :-1] + x[:-1, 1:] + x[1:, 1:])
- ymid = 0.25*(y[:-1, :-1] + y[1:, :-1] + y[:-1, 1:] + y[1:, 1:])
- kwargs["alpha"] = quad_as_tri_alpha
- ax.plot(
- np.stack((x[:-1, :-1], xmid, x[1:, 1:])).reshape((3, -1)),
- np.stack((y[:-1, :-1], ymid, y[1:, 1:])).reshape((3, -1)),
- np.stack((x[1:, :-1], xmid, x[:-1, 1:])).reshape((3, -1)),
- np.stack((y[1:, :-1], ymid, y[:-1, 1:])).reshape((3, -1)),
- **kwargs)
- if point_color is not None:
- ax.plot(x, y, color=point_color, alpha=alpha, marker="o", lw=0)
- ax._need_autoscale = True
-
- def lines(
- self,
- lines: cpy.LineReturn,
- line_type: LineType,
- ax: Axes | int = 0,
- color: str = "C0",
- alpha: float = 1.0,
- linewidth: float = 1,
- ) -> None:
- """Plot contour lines on a single Axes.
-
- Args:
- lines (sequence of arrays): Contour line data as returned by
- :func:`~contourpy.ContourGenerator.lines`.
- line_type (LineType): Type of ``lines`` data, as returned by
- :attr:`~contourpy.ContourGenerator.line_type`.
- ax (int or Matplotlib Axes, optional): Which Axes to plot on, default ``0``.
- color (str, optional): Color to plot lines. May be a string color or the letter ``"C"``
- followed by an integer in the range ``"C0"`` to ``"C9"`` to use a color from the
- ``tab10`` colormap. Default ``"C0"``.
- alpha (float, optional): Opacity to plot lines with, default ``1.0``.
- linewidth (float, optional): Width of lines, default ``1``.
- """
- ax = self._get_ax(ax)
- paths = lines_to_mpl_paths(lines, line_type)
- collection = mcollections.PathCollection(
- paths, facecolors="none", edgecolors=color, lw=linewidth, alpha=alpha)
- ax.add_collection(collection)
- ax._need_autoscale = True
-
- def mask(
- self,
- x: ArrayLike,
- y: ArrayLike,
- z: ArrayLike | np.ma.MaskedArray[Any, Any],
- ax: Axes | int = 0,
- color: str = "black",
- ) -> None:
- """Plot masked out grid points as circles on a single Axes.
-
- Args:
- x (array-like of shape (ny, nx) or (nx,)): The x-coordinates of the grid points.
- y (array-like of shape (ny, nx) or (ny,)): The y-coordinates of the grid points.
- z (masked array of shape (ny, nx): z-values.
- ax (int or Matplotlib Axes, optional): Which Axes to plot on, default ``0``.
- color (str, optional): Circle color, default ``"black"``.
- """
- mask = np.ma.getmask(z) # type: ignore[no-untyped-call]
- if mask is np.ma.nomask:
- return
- ax = self._get_ax(ax)
- x, y = self._grid_as_2d(x, y)
- ax.plot(x[mask], y[mask], "o", c=color)
-
- def save(self, filename: str, transparent: bool = False) -> None:
- """Save plots to SVG or PNG file.
-
- Args:
- filename (str): Filename to save to.
- transparent (bool, optional): Whether background should be transparent, default
- ``False``.
- """
- self._autoscale()
- self._fig.savefig(filename, transparent=transparent)
-
- def save_to_buffer(self) -> io.BytesIO:
- """Save plots to an ``io.BytesIO`` buffer.
-
- Return:
- BytesIO: PNG image buffer.
- """
- self._autoscale()
- buf = io.BytesIO()
- self._fig.savefig(buf, format="png")
- buf.seek(0)
- return buf
-
- def show(self) -> None:
- """Show plots in an interactive window, in the usual Matplotlib manner.
- """
- self._autoscale()
- plt.show()
-
- def title(self, title: str, ax: Axes | int = 0, color: str | None = None) -> None:
- """Set the title of a single Axes.
-
- Args:
- title (str): Title text.
- ax (int or Matplotlib Axes, optional): Which Axes to set the title of, default ``0``.
- color (str, optional): Color to set title. May be a string color or the letter ``"C"``
- followed by an integer in the range ``"C0"`` to ``"C9"`` to use a color from the
- ``tab10`` colormap. Default is ``None`` which uses Matplotlib's default title color
- that depends on the stylesheet in use.
- """
- if color:
- self._get_ax(ax).set_title(title, color=color)
- else:
- self._get_ax(ax).set_title(title)
-
- def z_values(
- self,
- x: ArrayLike,
- y: ArrayLike,
- z: ArrayLike,
- ax: Axes | int = 0,
- color: str = "green",
- fmt: str = ".1f",
- quad_as_tri: bool = False,
- ) -> None:
- """Show ``z`` values on a single Axes.
-
- Args:
- x (array-like of shape (ny, nx) or (nx,)): The x-coordinates of the grid points.
- y (array-like of shape (ny, nx) or (ny,)): The y-coordinates of the grid points.
- z (array-like of shape (ny, nx): z-values.
- ax (int or Matplotlib Axes, optional): Which Axes to plot on, default ``0``.
- color (str, optional): Color of added text. May be a string color or the letter ``"C"``
- followed by an integer in the range ``"C0"`` to ``"C9"`` to use a color from the
- ``tab10`` colormap. Default ``"green"``.
- fmt (str, optional): Format to display z-values, default ``".1f"``.
- quad_as_tri (bool, optional): Whether to show z-values at the ``quad_as_tri`` centers
- of quads.
-
- Warning:
- ``quad_as_tri=True`` shows z-values for all quads, even if masked.
- """
- ax = self._get_ax(ax)
- x, y = self._grid_as_2d(x, y)
- z = np.asarray(z)
- ny, nx = z.shape
- for j in range(ny):
- for i in range(nx):
- ax.text(x[j, i], y[j, i], f"{z[j, i]:{fmt}}", ha="center", va="center",
- color=color, clip_on=True)
- if quad_as_tri:
- for j in range(ny-1):
- for i in range(nx-1):
- xx = np.mean(x[j:j+2, i:i+2])
- yy = np.mean(y[j:j+2, i:i+2])
- zz = np.mean(z[j:j+2, i:i+2])
- ax.text(xx, yy, f"{zz:{fmt}}", ha="center", va="center", color=color,
- clip_on=True)
-
-
-class MplTestRenderer(MplRenderer):
- """Test renderer implemented using Matplotlib.
-
- No whitespace around plots and no spines/ticks displayed.
- Uses Agg backend, so can only save to file/buffer, cannot call ``show()``.
- """
- def __init__(
- self,
- nrows: int = 1,
- ncols: int = 1,
- figsize: tuple[float, float] = (9, 9),
- ) -> None:
- gridspec = {
- "left": 0.01,
- "right": 0.99,
- "top": 0.99,
- "bottom": 0.01,
- "wspace": 0.01,
- "hspace": 0.01,
- }
- super().__init__(
- nrows, ncols, figsize, show_frame=True, backend="Agg", gridspec_kw=gridspec,
- )
-
- for ax in self._axes:
- ax.set_xmargin(0.0)
- ax.set_ymargin(0.0)
- ax.set_xticks([])
- ax.set_yticks([])
-
- self._want_tight = False
-
-
-class MplDebugRenderer(MplRenderer):
- """Debug renderer implemented using Matplotlib.
-
- Extends ``MplRenderer`` to add extra information to help in debugging such as markers, arrows,
- text, etc.
- """
- def __init__(
- self,
- nrows: int = 1,
- ncols: int = 1,
- figsize: tuple[float, float] = (9, 9),
- show_frame: bool = True,
- ) -> None:
- super().__init__(nrows, ncols, figsize, show_frame)
-
- def _arrow(
- self,
- ax: Axes,
- line_start: cpy.CoordinateArray,
- line_end: cpy.CoordinateArray,
- color: str,
- alpha: float,
- arrow_size: float,
- ) -> None:
- mid = 0.5*(line_start + line_end)
- along = line_end - line_start
- along /= np.sqrt(np.dot(along, along)) # Unit vector.
- right = np.asarray((along[1], -along[0]))
- arrow = np.stack((
- mid - (along*0.5 - right)*arrow_size,
- mid + along*0.5*arrow_size,
- mid - (along*0.5 + right)*arrow_size,
- ))
- ax.plot(arrow[:, 0], arrow[:, 1], "-", c=color, alpha=alpha)
-
- def _filled_to_lists_of_points_and_offsets(
- self,
- filled: cpy.FillReturn,
- fill_type: FillType,
- ) -> tuple[list[cpy.PointArray], list[cpy.OffsetArray]]:
- if fill_type == FillType.OuterCode:
- if TYPE_CHECKING:
- filled = cast(cpy.FillReturn_OuterCode, filled)
- all_points = filled[0]
- all_offsets = [mpl_codes_to_offsets(codes) for codes in filled[1]]
- elif fill_type == FillType.ChunkCombinedCode:
- if TYPE_CHECKING:
- filled = cast(cpy.FillReturn_ChunkCombinedCode, filled)
- all_points = [points for points in filled[0] if points is not None]
- all_offsets = [mpl_codes_to_offsets(codes) for codes in filled[1] if codes is not None]
- elif fill_type == FillType.OuterOffset:
- if TYPE_CHECKING:
- filled = cast(cpy.FillReturn_OuterOffset, filled)
- all_points = filled[0]
- all_offsets = filled[1]
- elif fill_type == FillType.ChunkCombinedOffset:
- if TYPE_CHECKING:
- filled = cast(cpy.FillReturn_ChunkCombinedOffset, filled)
- all_points = [points for points in filled[0] if points is not None]
- all_offsets = [offsets for offsets in filled[1] if offsets is not None]
- elif fill_type == FillType.ChunkCombinedCodeOffset:
- if TYPE_CHECKING:
- filled = cast(cpy.FillReturn_ChunkCombinedCodeOffset, filled)
- all_points = []
- all_offsets = []
- for points, codes, outer_offsets in zip(*filled):
- if points is None:
- continue
- if TYPE_CHECKING:
- assert codes is not None and outer_offsets is not None
- all_points += np.split(points, outer_offsets[1:-1])
- all_codes = np.split(codes, outer_offsets[1:-1])
- all_offsets += [mpl_codes_to_offsets(codes) for codes in all_codes]
- elif fill_type == FillType.ChunkCombinedOffsetOffset:
- if TYPE_CHECKING:
- filled = cast(cpy.FillReturn_ChunkCombinedOffsetOffset, filled)
- all_points = []
- all_offsets = []
- for points, offsets, outer_offsets in zip(*filled):
- if points is None:
- continue
- if TYPE_CHECKING:
- assert offsets is not None and outer_offsets is not None
- for i in range(len(outer_offsets)-1):
- offs = offsets[outer_offsets[i]:outer_offsets[i+1]+1]
- all_points.append(points[offs[0]:offs[-1]])
- all_offsets.append(offs - offs[0])
- else:
- raise RuntimeError(f"Rendering FillType {fill_type} not implemented")
-
- return all_points, all_offsets
-
- def _lines_to_list_of_points(
- self, lines: cpy.LineReturn, line_type: LineType,
- ) -> list[cpy.PointArray]:
- if line_type == LineType.Separate:
- if TYPE_CHECKING:
- lines = cast(cpy.LineReturn_Separate, lines)
- all_lines = lines
- elif line_type == LineType.SeparateCode:
- if TYPE_CHECKING:
- lines = cast(cpy.LineReturn_SeparateCode, lines)
- all_lines = lines[0]
- elif line_type == LineType.ChunkCombinedCode:
- if TYPE_CHECKING:
- lines = cast(cpy.LineReturn_ChunkCombinedCode, lines)
- all_lines = []
- for points, codes in zip(*lines):
- if points is not None:
- if TYPE_CHECKING:
- assert codes is not None
- offsets = mpl_codes_to_offsets(codes)
- for i in range(len(offsets)-1):
- all_lines.append(points[offsets[i]:offsets[i+1]])
- elif line_type == LineType.ChunkCombinedOffset:
- if TYPE_CHECKING:
- lines = cast(cpy.LineReturn_ChunkCombinedOffset, lines)
- all_lines = []
- for points, all_offsets in zip(*lines):
- if points is not None:
- if TYPE_CHECKING:
- assert all_offsets is not None
- for i in range(len(all_offsets)-1):
- all_lines.append(points[all_offsets[i]:all_offsets[i+1]])
- else:
- raise RuntimeError(f"Rendering LineType {line_type} not implemented")
-
- return all_lines
-
- def filled(
- self,
- filled: cpy.FillReturn,
- fill_type: FillType,
- ax: Axes | int = 0,
- color: str = "C1",
- alpha: float = 0.7,
- line_color: str = "C0",
- line_alpha: float = 0.7,
- point_color: str = "C0",
- start_point_color: str = "red",
- arrow_size: float = 0.1,
- ) -> None:
- super().filled(filled, fill_type, ax, color, alpha)
-
- if line_color is None and point_color is None:
- return
-
- ax = self._get_ax(ax)
- all_points, all_offsets = self._filled_to_lists_of_points_and_offsets(filled, fill_type)
-
- # Lines.
- if line_color is not None:
- for points, offsets in zip(all_points, all_offsets):
- for start, end in zip(offsets[:-1], offsets[1:]):
- xys = points[start:end]
- ax.plot(xys[:, 0], xys[:, 1], c=line_color, alpha=line_alpha)
-
- if arrow_size > 0.0:
- n = len(xys)
- for i in range(n-1):
- self._arrow(ax, xys[i], xys[i+1], line_color, line_alpha, arrow_size)
-
- # Points.
- if point_color is not None:
- for points, offsets in zip(all_points, all_offsets):
- mask = np.ones(offsets[-1], dtype=bool)
- mask[offsets[1:]-1] = False # Exclude end points.
- if start_point_color is not None:
- start_indices = offsets[:-1]
- mask[start_indices] = False # Exclude start points.
- ax.plot(
- points[:, 0][mask], points[:, 1][mask], "o", c=point_color, alpha=line_alpha)
-
- if start_point_color is not None:
- ax.plot(points[:, 0][start_indices], points[:, 1][start_indices], "o",
- c=start_point_color, alpha=line_alpha)
-
- def lines(
- self,
- lines: cpy.LineReturn,
- line_type: LineType,
- ax: Axes | int = 0,
- color: str = "C0",
- alpha: float = 1.0,
- linewidth: float = 1,
- point_color: str = "C0",
- start_point_color: str = "red",
- arrow_size: float = 0.1,
- ) -> None:
- super().lines(lines, line_type, ax, color, alpha, linewidth)
-
- if arrow_size == 0.0 and point_color is None:
- return
-
- ax = self._get_ax(ax)
- all_lines = self._lines_to_list_of_points(lines, line_type)
-
- if arrow_size > 0.0:
- for line in all_lines:
- for i in range(len(line)-1):
- self._arrow(ax, line[i], line[i+1], color, alpha, arrow_size)
-
- if point_color is not None:
- for line in all_lines:
- start_index = 0
- end_index = len(line)
- if start_point_color is not None:
- ax.plot(line[0, 0], line[0, 1], "o", c=start_point_color, alpha=alpha)
- start_index = 1
- if line[0][0] == line[-1][0] and line[0][1] == line[-1][1]:
- end_index -= 1
- ax.plot(line[start_index:end_index, 0], line[start_index:end_index, 1], "o",
- c=color, alpha=alpha)
-
- def point_numbers(
- self,
- x: ArrayLike,
- y: ArrayLike,
- z: ArrayLike,
- ax: Axes | int = 0,
- color: str = "red",
- ) -> None:
- ax = self._get_ax(ax)
- x, y = self._grid_as_2d(x, y)
- z = np.asarray(z)
- ny, nx = z.shape
- for j in range(ny):
- for i in range(nx):
- quad = i + j*nx
- ax.text(x[j, i], y[j, i], str(quad), ha="right", va="top", color=color,
- clip_on=True)
-
- def quad_numbers(
- self,
- x: ArrayLike,
- y: ArrayLike,
- z: ArrayLike,
- ax: Axes | int = 0,
- color: str = "blue",
- ) -> None:
- ax = self._get_ax(ax)
- x, y = self._grid_as_2d(x, y)
- z = np.asarray(z)
- ny, nx = z.shape
- for j in range(1, ny):
- for i in range(1, nx):
- quad = i + j*nx
- xmid = x[j-1:j+1, i-1:i+1].mean()
- ymid = y[j-1:j+1, i-1:i+1].mean()
- ax.text(xmid, ymid, str(quad), ha="center", va="center", color=color, clip_on=True)
-
- def z_levels(
- self,
- x: ArrayLike,
- y: ArrayLike,
- z: ArrayLike,
- lower_level: float,
- upper_level: float | None = None,
- ax: Axes | int = 0,
- color: str = "green",
- ) -> None:
- ax = self._get_ax(ax)
- x, y = self._grid_as_2d(x, y)
- z = np.asarray(z)
- ny, nx = z.shape
- for j in range(ny):
- for i in range(nx):
- zz = z[j, i]
- if upper_level is not None and zz > upper_level:
- z_level = 2
- elif zz > lower_level:
- z_level = 1
- else:
- z_level = 0
- ax.text(x[j, i], y[j, i], z_level, ha="left", va="bottom", color=color,
- clip_on=True)
diff --git a/spaces/joaopereirajp/livvieChatBot/venv/lib/python3.9/site-packages/fastapi/background.py b/spaces/joaopereirajp/livvieChatBot/venv/lib/python3.9/site-packages/fastapi/background.py
deleted file mode 100644
index dd3bbe249130348881331aea569ce3ec3f295128..0000000000000000000000000000000000000000
--- a/spaces/joaopereirajp/livvieChatBot/venv/lib/python3.9/site-packages/fastapi/background.py
+++ /dev/null
@@ -1 +0,0 @@
-from starlette.background import BackgroundTasks as BackgroundTasks # noqa
diff --git a/spaces/jordonpeter01/MusicGen/audiocraft/modules/rope.py b/spaces/jordonpeter01/MusicGen/audiocraft/modules/rope.py
deleted file mode 100644
index 4b8c70b9aba28eeb53d12ddc3de8852492847808..0000000000000000000000000000000000000000
--- a/spaces/jordonpeter01/MusicGen/audiocraft/modules/rope.py
+++ /dev/null
@@ -1,124 +0,0 @@
-# Copyright (c) Meta Platforms, Inc. and affiliates.
-# All rights reserved.
-#
-# This source code is licensed under the license found in the
-# LICENSE file in the root directory of this source tree.
-
-import typing as tp
-
-from torch import nn
-import torch
-
-
-class XPos(nn.Module):
- """Length-extrapolatable positional embedding (xPos) from [Sun et al 2022](https://arxiv.org/abs/2212.10554v1).
- This applies an exponential decay to the RoPE rotation matrix.
-
- Args:
- dim (int): Embedding dimension.
- smoothing (float): Smoothing factor applied to the decay rates.
- base_scale (int): Base decay rate, given in terms of scaling time.
- device (torch.device or None): Device on which to initialize the module.
- dtype (torch.dtype): dtype to use to generate the embedding.
- """
- def __init__(self, dim: int, smoothing: float = 0.4, base_scale: int = 512,
- device=None, dtype: torch.dtype = torch.float32):
- super().__init__()
- assert dim % 2 == 0
- assert dtype in [torch.float64, torch.float32]
- self.dtype = dtype
- self.base_scale = base_scale
-
- half_dim = dim // 2
- adim = torch.arange(half_dim, device=device, dtype=dtype)
- decay_rates = (adim / half_dim + smoothing) / (1.0 + smoothing)
- self.register_buffer("decay_rates", decay_rates)
- self.decay: tp.Optional[torch.Tensor] = None
-
- def get_decay(self, start: int, end: int):
- """Create complex decay tensor, cache values for fast computation.
- """
- if self.decay is None or end > self.decay.shape[0]:
- assert isinstance(self.decay_rates, torch.Tensor) # Satisfy type checker.
- idx = torch.arange(end, device=self.decay_rates.device, dtype=self.dtype)
- power = idx / self.base_scale
- scale = self.decay_rates ** power.unsqueeze(-1)
- self.decay = torch.polar(scale, torch.zeros_like(scale))
- return self.decay[start:end] # [T, C/2]
-
-
-class RotaryEmbedding(nn.Module):
- """Rotary positional embedding (RoPE) from [Su et al 2022](https://arxiv.org/abs/2104.09864).
-
- Args:
- dim (int): Embedding dimension (twice the number of frequencies).
- max_period (float): Maximum period of the rotation frequencies.
- xpos (bool): Use xPos, applies an exponential decay to rotation matrix.
- scale (float): Scale of positional embedding, set to 0 to deactivate.
- device (torch.device or None): Device on which to initialize the module.
- dtype (torch.dtype): dtype to use to generate the embedding.
- """
- def __init__(self, dim: int, max_period: float = 10000.0, xpos: bool = False,
- scale: float = 1.0, device=None, dtype: torch.dtype = torch.float32):
- super().__init__()
- assert dim % 2 == 0
- self.scale = scale
- assert dtype in [torch.float64, torch.float32]
- self.dtype = dtype
-
- adim = torch.arange(0, dim, 2, device=device, dtype=dtype)[: (dim // 2)]
- frequencies = 1.0 / (max_period ** (adim / dim))
- self.register_buffer("frequencies", frequencies)
- self.rotation: tp.Optional[torch.Tensor] = None
-
- self.xpos = XPos(dim, device=device, dtype=dtype) if xpos else None
-
- def get_rotation(self, start: int, end: int):
- """Create complex rotation tensor, cache values for fast computation.
- """
- if self.rotation is None or end > self.rotation.shape[0]:
- assert isinstance(self.frequencies, torch.Tensor) # Satisfy type checker.
- idx = torch.arange(end, device=self.frequencies.device, dtype=self.dtype)
- angles = torch.outer(idx, self.frequencies)
- self.rotation = torch.polar(torch.ones_like(angles), angles)
- return self.rotation[start:end]
-
- def rotate(self, x: torch.Tensor, start: int = 0, invert_decay: bool = False):
- """Apply rope rotation to query or key tensor.
- """
- T = x.shape[1]
- rotation = self.get_rotation(start, start + T).unsqueeze(0).unsqueeze(2)
-
- if self.xpos:
- decay = self.xpos.get_decay(start, start + T).unsqueeze(0).unsqueeze(2)
- else:
- decay = 1.0
-
- if invert_decay:
- decay = decay ** -1
-
- x_complex = torch.view_as_complex(x.to(self.dtype).reshape(*x.shape[:-1], -1, 2))
- scaled_rotation = (rotation * decay) * self.scale + (1.0 - self.scale)
- x_out = torch.view_as_real(x_complex * scaled_rotation).flatten(-2)
-
- return x_out.type_as(x)
-
- def rotate_qk(self, query: torch.Tensor, key: torch.Tensor, start: int = 0):
- """ Apply rope rotation to both query and key tensors.
- Supports streaming mode, in which query and key are not expected to have the same shape.
- In streaming mode, key will be of legnth [P + C] with P the cached past timesteps, but
- query will be [C] (typically C == 1).
-
- Args:
- query (torch.Tensor): Query to rotate.
- key (torch.Tensor): Key to rotate.
- start (int): Start index of the sequence for time offset.
- """
- query_timesteps = query.shape[1]
- key_timesteps = key.shape[1]
- streaming_offset = key_timesteps - query_timesteps
-
- query_out = self.rotate(query, start + streaming_offset)
- key_out = self.rotate(key, start, invert_decay=True)
-
- return query_out, key_out
diff --git a/spaces/jracca/01-learning-space/app.py b/spaces/jracca/01-learning-space/app.py
deleted file mode 100644
index 4709e853096531640dbf08655e6009043b2d139e..0000000000000000000000000000000000000000
--- a/spaces/jracca/01-learning-space/app.py
+++ /dev/null
@@ -1,225 +0,0 @@
-import os
-
-os.system("pip uninstall -y gradio")
-os.system("pip install gradio==2.9.4")
-os.system("git clone --recursive https://github.com/JD-P/cloob-latent-diffusion")
-os.system("cd cloob-latent-diffusion;pip install omegaconf pillow pytorch-lightning einops wandb ftfy regex ./CLIP")
-
-import argparse
-from functools import partial
-from pathlib import Path
-import sys
-sys.path.append('./cloob-latent-diffusion')
-sys.path.append('./cloob-latent-diffusion/cloob-training')
-sys.path.append('./cloob-latent-diffusion/latent-diffusion')
-sys.path.append('./cloob-latent-diffusion/taming-transformers')
-sys.path.append('./cloob-latent-diffusion/v-diffusion-pytorch')
-from omegaconf import OmegaConf
-from PIL import Image
-import torch
-from torch import nn
-from torch.nn import functional as F
-from torchvision import transforms
-from torchvision.transforms import functional as TF
-from tqdm import trange
-from CLIP import clip
-from cloob_training import model_pt, pretrained
-import ldm.models.autoencoder
-from diffusion import sampling, utils
-import train_latent_diffusion as train
-from huggingface_hub import hf_hub_url, cached_download
-import random
-
-# Download the model files
-checkpoint = cached_download(hf_hub_url("huggan/distill-ccld-wa", filename="model_student.ckpt"))
-ae_model_path = cached_download(hf_hub_url("huggan/ccld_wa", filename="ae_model.ckpt"))
-ae_config_path = cached_download(hf_hub_url("huggan/ccld_wa", filename="ae_model.yaml"))
-
-# Define a few utility functions
-
-def parse_prompt(prompt, default_weight=3.):
- if prompt.startswith('http://') or prompt.startswith('https://'):
- vals = prompt.rsplit(':', 2)
- vals = [vals[0] + ':' + vals[1], *vals[2:]]
- else:
- vals = prompt.rsplit(':', 1)
- vals = vals + ['', default_weight][len(vals):]
- return vals[0], float(vals[1])
-
-
-def resize_and_center_crop(image, size):
- fac = max(size[0] / image.size[0], size[1] / image.size[1])
- image = image.resize((int(fac * image.size[0]), int(fac * image.size[1])), Image.LANCZOS)
- return TF.center_crop(image, size[::-1])
-
-
-# Load the models
-device = torch.device('cuda:0' if torch.cuda.is_available() else 'cpu')
-print('Using device:', device)
-print('loading models')
-
-# autoencoder
-ae_config = OmegaConf.load(ae_config_path)
-ae_model = ldm.models.autoencoder.AutoencoderKL(**ae_config.model.params)
-ae_model.eval().requires_grad_(False).to(device)
-ae_model.load_state_dict(torch.load(ae_model_path))
-n_ch, side_y, side_x = 4, 32, 32
-
-# diffusion model
-model = train.DiffusionModel(192, [1,1,2,2], autoencoder_scale=torch.tensor(4.3084))
-model.load_state_dict(torch.load(checkpoint, map_location='cpu'))
-model = model.to(device).eval().requires_grad_(False)
-
-# CLOOB
-cloob_config = pretrained.get_config('cloob_laion_400m_vit_b_16_16_epochs')
-cloob = model_pt.get_pt_model(cloob_config)
-checkpoint = pretrained.download_checkpoint(cloob_config)
-cloob.load_state_dict(model_pt.get_pt_params(cloob_config, checkpoint))
-cloob.eval().requires_grad_(False).to(device)
-
-
-# The key function: returns a list of n PIL images
-def generate(n=1, prompts=['a red circle'], images=[], seed=42, steps=15,
- method='plms', eta=None):
- zero_embed = torch.zeros([1, cloob.config['d_embed']], device=device)
- target_embeds, weights = [zero_embed], []
-
- for prompt in prompts:
- txt, weight = parse_prompt(prompt)
- target_embeds.append(cloob.text_encoder(cloob.tokenize(txt).to(device)).float())
- weights.append(weight)
-
- for prompt in images:
- path, weight = parse_prompt(prompt)
- img = Image.open(utils.fetch(path)).convert('RGB')
- clip_size = cloob.config['image_encoder']['image_size']
- img = resize_and_center_crop(img, (clip_size, clip_size))
- batch = TF.to_tensor(img)[None].to(device)
- embed = F.normalize(cloob.image_encoder(cloob.normalize(batch)).float(), dim=-1)
- target_embeds.append(embed)
- weights.append(weight)
-
- weights = torch.tensor([1 - sum(weights), *weights], device=device)
-
- torch.manual_seed(seed)
-
- def cfg_model_fn(x, t):
- n = x.shape[0]
- n_conds = len(target_embeds)
- x_in = x.repeat([n_conds, 1, 1, 1])
- t_in = t.repeat([n_conds])
- clip_embed_in = torch.cat([*target_embeds]).repeat_interleave(n, 0)
- vs = model(x_in, t_in, clip_embed_in).view([n_conds, n, *x.shape[1:]])
- v = vs.mul(weights[:, None, None, None, None]).sum(0)
- return v
-
- def run(x, steps):
- if method == 'ddpm':
- return sampling.sample(cfg_model_fn, x, steps, 1., {})
- if method == 'ddim':
- return sampling.sample(cfg_model_fn, x, steps, eta, {})
- if method == 'prk':
- return sampling.prk_sample(cfg_model_fn, x, steps, {})
- if method == 'plms':
- return sampling.plms_sample(cfg_model_fn, x, steps, {})
- if method == 'pie':
- return sampling.pie_sample(cfg_model_fn, x, steps, {})
- if method == 'plms2':
- return sampling.plms2_sample(cfg_model_fn, x, steps, {})
- assert False
-
- batch_size = n
- x = torch.randn([n, n_ch, side_y, side_x], device=device)
- t = torch.linspace(1, 0, steps + 1, device=device)[:-1]
- steps = utils.get_spliced_ddpm_cosine_schedule(t)
- pil_ims = []
- for i in trange(0, n, batch_size):
- cur_batch_size = min(n - i, batch_size)
- out_latents = run(x[i:i+cur_batch_size], steps)
- outs = ae_model.decode(out_latents * torch.tensor(2.55).to(device))
- for j, out in enumerate(outs):
- pil_ims.append(utils.to_pil_image(out))
-
- return pil_ims
-
-
-import gradio as gr
-
-def gen_ims(prompt, im_prompt=None, seed=None, n_steps=10, method='plms'):
- if seed == None :
- seed = random.randint(0, 10000)
- print( prompt, im_prompt, seed, n_steps)
- prompts = [prompt]
- im_prompts = []
- if im_prompt != None:
- im_prompts = [im_prompt]
- pil_ims = generate(n=1, prompts=prompts, images=im_prompts, seed=seed, steps=n_steps, method=method)
- return pil_ims[0]
-
-iface = gr.Interface(fn=gen_ims,
- inputs=[#gr.inputs.Slider(minimum=1, maximum=1, step=1, default=1,label="Number of images"),
- #gr.inputs.Slider(minimum=0, maximum=200, step=1, label='Random seed', default=0),
- gr.inputs.Textbox(label="Text prompt"),
- gr.inputs.Image(optional=True, label="Image prompt", type='filepath'),
- #gr.inputs.Slider(minimum=10, maximum=35, step=1, default=15,label="Number of steps")
- ],
- outputs=[gr.outputs.Image(type="pil", label="Generated Image")],
- examples=[
- ["Futurism, in the style of Wassily Kandinsky"],
- ["Art Nouveau, in the style of John Singer Sargent"],
- ["Surrealism, in the style of Edgar Degas"],
- ["Expressionism, in the style of Wassily Kandinsky"],
- ["Futurism, in the style of Egon Schiele"],
- ["Neoclassicism, in the style of Gustav Klimt"],
- ["Cubism, in the style of Gustav Klimt"],
- ["Op Art, in the style of Marc Chagall"],
- ["Romanticism, in the style of M.C. Escher"],
- ["Futurism, in the style of M.C. Escher"],
- ["Abstract Art, in the style of M.C. Escher"],
- ["Mannerism, in the style of Paul Klee"],
- ["Romanesque Art, in the style of Leonardo da Vinci"],
- ["High Renaissance, in the style of Rembrandt"],
- ["Magic Realism, in the style of Gustave Dore"],
- ["Realism, in the style of Jean-Michel Basquiat"],
- ["Art Nouveau, in the style of Paul Gauguin"],
- ["Avant-garde, in the style of Pierre-Auguste Renoir"],
- ["Baroque, in the style of Edward Hopper"],
- ["Post-Impressionism, in the style of Wassily Kandinsky"],
- ["Naturalism, in the style of Rene Magritte"],
- ["Constructivism, in the style of Paul Cezanne"],
- ["Abstract Expressionism, in the style of Henri Matisse"],
- ["Pop Art, in the style of Vincent van Gogh"],
- ["Futurism, in the style of Wassily Kandinsky"],
- ["Futurism, in the style of Zdzislaw Beksinski"],
- ['Surrealism, in the style of Salvador Dali'],
- ["Aaron Wacker, oil on canvas"],
- ["abstract"],
- ["landscape"],
- ["portrait"],
- ["sculpture"],
- ["genre painting"],
- ["installation"],
- ["photo"],
- ["figurative"],
- ["illustration"],
- ["still life"],
- ["history painting"],
- ["cityscape"],
- ["marina"],
- ["animal painting"],
- ["design"],
- ["calligraphy"],
- ["symbolic painting"],
- ["graffiti"],
- ["performance"],
- ["mythological painting"],
- ["battle painting"],
- ["self-portrait"],
- ["Impressionism, oil on canvas"]
- ],
- title='Art Generator and Style Mixer from 🧠 Cloob and 🎨 WikiArt - Visual Art Encyclopedia:',
- description="Trained on images from the [WikiArt](https://www.wikiart.org/) dataset, comprised of visual arts",
- article = 'Model used is: [model card](https://huggingface.co/huggan/distill-ccld-wa)..'
-
-)
-iface.launch(enable_queue=True) # , debug=True for colab debugging
\ No newline at end of file
diff --git a/spaces/kadirnar/Multilingual-Translation/README.md b/spaces/kadirnar/Multilingual-Translation/README.md
deleted file mode 100644
index 3fb383a8d4b2ca3061ec41fe7cdd718470820fd3..0000000000000000000000000000000000000000
--- a/spaces/kadirnar/Multilingual-Translation/README.md
+++ /dev/null
@@ -1,13 +0,0 @@
----
-title: Multilingual Translation
-emoji: 🌍
-colorFrom: yellow
-colorTo: pink
-sdk: gradio
-sdk_version: 3.17.0
-app_file: app.py
-pinned: false
-license: mit
----
-
-Check out the configuration reference at https://huggingface.co/docs/hub/spaces-config-reference
diff --git a/spaces/kahnchana/clippy/infer_model.py b/spaces/kahnchana/clippy/infer_model.py
deleted file mode 100644
index cac1db5dd06f9de9d10e8bdf1740f4f59b62fcdb..0000000000000000000000000000000000000000
--- a/spaces/kahnchana/clippy/infer_model.py
+++ /dev/null
@@ -1,202 +0,0 @@
-import abc
-import math
-
-import torch
-import torch.nn.functional as F
-from sentence_transformers import SentenceTransformer
-from timm.models.vision_transformer import (
- VisionTransformer,
- build_model_with_cfg,
- checkpoint_filter_fn,
- checkpoint_seq,
- resolve_pretrained_cfg,
-)
-from torch import Tensor, nn
-
-
-class BlankLayer(nn.Module):
- pass
-
-
-class CustomViT(VisionTransformer):
- def __init__(
- self,
- *args,
- image_pooling="gmp",
- **kwargs,
- ):
- super(CustomViT, self).__init__(
- *args, **kwargs
- )
- self.image_pooling = image_pooling
-
- def forward_head(self, x, pre_logits: bool = False):
- if self.image_pooling:
- if self.image_pooling == "gap":
- x = x[:, self.num_prefix_tokens:].mean(dim=1)
- elif self.image_pooling == "gmp":
- x = x[:, self.num_prefix_tokens:].max(dim=-2)[0]
- elif self.image_pooling == "all":
- x = x[:, self.num_prefix_tokens:]
- else: # cls by default
- x = x[:, 0]
- x = self.fc_norm(x)
- return x if pre_logits else self.head(x)
-
- def forward(self, x, get_pos_tokens=False):
- x = self.forward_features(x, get_pos_tokens=get_pos_tokens)
- if get_pos_tokens:
- return self.fc_norm(x[:, self.num_prefix_tokens:])
- x = self.forward_head(x)
- return x
-
- def forward_features(self, x, get_pos_tokens=False):
- _, nc, h, w = x.shape
- x = self.patch_embed(x)
- x = self._pos_embed(x, w, h)
- if self.grad_checkpointing and not torch.jit.is_scripting():
- x = checkpoint_seq(self.blocks, x)
- else:
- x = self.blocks(x)
- x = self.norm(x)
- return x
-
- def _pos_embed(self, x, w, h):
- if self.no_embed_class:
- # deit-3, updated JAX (big vision)
- # position embedding does not overlap with class token, add then concat
- x = x + self.pos_embed
- if self.cls_token is not None:
- x = torch.cat((self.cls_token.expand(x.shape[0], -1, -1), x), dim=1)
- else:
- # original timm, JAX, and deit vit impl
- # pos_embed has entry for class token, concat then add
- if self.cls_token is not None:
- x = torch.cat((self.cls_token.expand(x.shape[0], -1, -1), x), dim=1)
- x = x + self._interpolate_pos_encoding(x, w, h)
- return self.pos_drop(x)
-
- def _interpolate_pos_encoding(self, x, w, h):
- npatch = x.shape[1] - 1
- N = self.pos_embed.shape[1] - 1
- if npatch == N and w == h:
- return self.pos_embed
- class_pos_embed = self.pos_embed[:, 0]
- patch_pos_embed = self.pos_embed[:, 1:]
- dim = x.shape[-1]
- w0 = w // self.patch_embed.patch_size[0]
- h0 = h // self.patch_embed.patch_size[1]
- # we add a small number to avoid floating point error in the interpolation
- # see discussion at https://github.com/facebookresearch/dino/issues/8
- w0, h0 = w0 + 0.1, h0 + 0.1
- patch_pos_embed = nn.functional.interpolate(
- patch_pos_embed.reshape(1, int(math.sqrt(N)), int(math.sqrt(N)), dim).permute(
- 0, 3, 1, 2
- ),
- scale_factor=(w0 / math.sqrt(N), h0 / math.sqrt(N)),
- mode="bicubic",
- )
- assert int(w0) == patch_pos_embed.shape[-2] and int(h0) == patch_pos_embed.shape[-1]
- patch_pos_embed = patch_pos_embed.permute(0, 2, 3, 1).view(1, -1, dim)
- return torch.cat((class_pos_embed.unsqueeze(0), patch_pos_embed), dim=1)
-
-
-def _create_vision_transformer(variant, pretrained=False, **kwargs):
- if kwargs.get("features_only", None):
- raise RuntimeError("features_only not implemented for Vision Transformer models.")
-
- pretrained_cfg = resolve_pretrained_cfg(
- variant, pretrained_cfg=kwargs.pop("pretrained_cfg", None)
- )
- model = build_model_with_cfg(
- CustomViT,
- variant,
- pretrained,
- pretrained_cfg=pretrained_cfg,
- pretrained_filter_fn=checkpoint_filter_fn,
- pretrained_custom_load="npz" in pretrained_cfg["url"],
- **kwargs,
- )
- return model
-
-
-def vit_base_patch16_224(pretrained=False, variant="vit_base_patch16_224_dino", **kwargs):
- """ViT-Base (ViT-B/16) /w DINO pretrained weights (no head) - https://arxiv.org/abs/2104.14294"""
- model_kwargs = dict(patch_size=16, embed_dim=768, depth=12, num_heads=12, **kwargs)
- model = _create_vision_transformer(variant, pretrained=pretrained, **model_kwargs)
- return model
-
-
-class CLIPpyModel(abc.ABC, torch.nn.Module):
- """ Implements code for running inference with pre-trained CLIPpy model.
-
- NOTE: weights used are for a model trained with lower batch-size leading to results below those in paper.
- """
-
- def __init__(
- self,
- image_pooling: str = "cls",
- text_pooling: str = "gap",
- ):
- super().__init__()
-
- self.visual = BlankLayer()
-
- self.visual.trunk = vit_base_patch16_224(True, image_pooling=image_pooling)
-
- self.text = SentenceTransformer("sentence-transformers/sentence-t5-base")
- self.logit_scale = nn.Parameter(torch.ones([]) * math.log(1 / 0.07))
- self.set_text_pooling(text_pooling)
-
- self._divisor_eps = 1e-4
- self._image_pooling = image_pooling
- self._text_pooling = text_pooling
-
- def forward(
- self,
- images: Tensor,
- input_ids: Tensor,
- input_id_masks: Tensor,
- get_pos_tokens: bool = False,
- **kwargs,
- ):
-
- image_encodings = self.encode_image(images, get_pos_tokens=get_pos_tokens)
-
- if get_pos_tokens:
- return {
- image_encodings: image_encodings,
- }
-
- text_encodings = self.encode_text(input_ids, input_id_masks)
-
- return {
- image_encodings: image_encodings,
- text_encodings: text_encodings,
- }
-
- def encode_text(self, input_ids: Tensor, input_id_masks: Tensor = None, **kwargs):
- output = self.text({"input_ids": input_ids, "attention_mask": input_id_masks})[
- "sentence_embedding"
- ]
- return self.text_head(output)
-
- def text_head(self, hidden_states: Tensor, input_id_masks: Tensor = None, **kwargs):
- return F.normalize(hidden_states, dim=-1, eps=self._divisor_eps).float()
-
- def encode_image(self, images: Tensor, get_pos_tokens: bool = False, **kwargs):
- output = self.visual.trunk(images, get_pos_tokens)
- return self.image_head(output, get_pos_tokens=get_pos_tokens)
-
- def image_head(self, hidden_states: Tensor, get_pos_tokens: bool = False, **kwargs):
- return F.normalize(hidden_states, dim=-1, eps=self._divisor_eps).float()
-
- def set_text_pooling(self, pooling):
- """ Converts pooling in the Hugging Face model to be max or average pooling"""
- if pooling == "gmp":
- self.text[1].pooling_mode_mean_tokens = False
- self.text[1].pooling_mode_max_tokens = True
- elif pooling == "gap":
- pass
- else:
- raise NotImplementedError(f"{pooling} not implemented")
diff --git a/spaces/kenton-li/record/README.md b/spaces/kenton-li/record/README.md
deleted file mode 100644
index a9fe0503ded8d5b3f799fe988593b95821a48da3..0000000000000000000000000000000000000000
--- a/spaces/kenton-li/record/README.md
+++ /dev/null
@@ -1,10 +0,0 @@
----
-title: Record
-emoji: 📉
-colorFrom: purple
-colorTo: red
-sdk: docker
-pinned: false
----
-
-Check out the configuration reference at https://huggingface.co/docs/hub/spaces-config-reference
diff --git a/spaces/kepl/gpt/g4f/Provider/Providers/Gravityengine.py b/spaces/kepl/gpt/g4f/Provider/Providers/Gravityengine.py
deleted file mode 100644
index f0cd09daaaae0adaa349f91139dc60c7ac79c028..0000000000000000000000000000000000000000
--- a/spaces/kepl/gpt/g4f/Provider/Providers/Gravityengine.py
+++ /dev/null
@@ -1,27 +0,0 @@
-import requests
-import os
-import json
-from ...typing import sha256, Dict, get_type_hints
-
-url = 'https://gpt4.xunika.uk/'
-model = ['gpt-3.5-turbo-16k', 'gpt-3.5-turbo-0613']
-supports_stream = True
-needs_auth = False
-
-def _create_completion(model: str, messages: list, stream: bool, temperature: float = 0.7, **kwargs):
- headers = {
- 'Content-Type': 'application/json',
- }
- data = {
- 'model': model,
- 'temperature': 0.7,
- 'presence_penalty': 0,
- 'messages': messages,
- }
- response = requests.post(url + '/api/openai/v1/chat/completions',
- json=data, stream=True)
-
- yield response.json()['choices'][0]['message']['content']
-
-params = f'g4f.Providers.{os.path.basename(__file__)[:-3]} supports: ' + \
- '(%s)' % ', '.join([f"{name}: {get_type_hints(_create_completion)[name].__name__}" for name in _create_completion.__code__.co_varnames[:_create_completion.__code__.co_argcount]])
\ No newline at end of file
diff --git a/spaces/kevinwang676/VoiceChangers/src/facerender/modules/keypoint_detector.py b/spaces/kevinwang676/VoiceChangers/src/facerender/modules/keypoint_detector.py
deleted file mode 100644
index 62a38a962b2f1a4326aac771aced353ec5e22a96..0000000000000000000000000000000000000000
--- a/spaces/kevinwang676/VoiceChangers/src/facerender/modules/keypoint_detector.py
+++ /dev/null
@@ -1,179 +0,0 @@
-from torch import nn
-import torch
-import torch.nn.functional as F
-
-from src.facerender.sync_batchnorm import SynchronizedBatchNorm2d as BatchNorm2d
-from src.facerender.modules.util import KPHourglass, make_coordinate_grid, AntiAliasInterpolation2d, ResBottleneck
-
-
-class KPDetector(nn.Module):
- """
- Detecting canonical keypoints. Return keypoint position and jacobian near each keypoint.
- """
-
- def __init__(self, block_expansion, feature_channel, num_kp, image_channel, max_features, reshape_channel, reshape_depth,
- num_blocks, temperature, estimate_jacobian=False, scale_factor=1, single_jacobian_map=False):
- super(KPDetector, self).__init__()
-
- self.predictor = KPHourglass(block_expansion, in_features=image_channel,
- max_features=max_features, reshape_features=reshape_channel, reshape_depth=reshape_depth, num_blocks=num_blocks)
-
- # self.kp = nn.Conv3d(in_channels=self.predictor.out_filters, out_channels=num_kp, kernel_size=7, padding=3)
- self.kp = nn.Conv3d(in_channels=self.predictor.out_filters, out_channels=num_kp, kernel_size=3, padding=1)
-
- if estimate_jacobian:
- self.num_jacobian_maps = 1 if single_jacobian_map else num_kp
- # self.jacobian = nn.Conv3d(in_channels=self.predictor.out_filters, out_channels=9 * self.num_jacobian_maps, kernel_size=7, padding=3)
- self.jacobian = nn.Conv3d(in_channels=self.predictor.out_filters, out_channels=9 * self.num_jacobian_maps, kernel_size=3, padding=1)
- '''
- initial as:
- [[1 0 0]
- [0 1 0]
- [0 0 1]]
- '''
- self.jacobian.weight.data.zero_()
- self.jacobian.bias.data.copy_(torch.tensor([1, 0, 0, 0, 1, 0, 0, 0, 1] * self.num_jacobian_maps, dtype=torch.float))
- else:
- self.jacobian = None
-
- self.temperature = temperature
- self.scale_factor = scale_factor
- if self.scale_factor != 1:
- self.down = AntiAliasInterpolation2d(image_channel, self.scale_factor)
-
- def gaussian2kp(self, heatmap):
- """
- Extract the mean from a heatmap
- """
- shape = heatmap.shape
- heatmap = heatmap.unsqueeze(-1)
- grid = make_coordinate_grid(shape[2:], heatmap.type()).unsqueeze_(0).unsqueeze_(0)
- value = (heatmap * grid).sum(dim=(2, 3, 4))
- kp = {'value': value}
-
- return kp
-
- def forward(self, x):
- if self.scale_factor != 1:
- x = self.down(x)
-
- feature_map = self.predictor(x)
- prediction = self.kp(feature_map)
-
- final_shape = prediction.shape
- heatmap = prediction.view(final_shape[0], final_shape[1], -1)
- heatmap = F.softmax(heatmap / self.temperature, dim=2)
- heatmap = heatmap.view(*final_shape)
-
- out = self.gaussian2kp(heatmap)
-
- if self.jacobian is not None:
- jacobian_map = self.jacobian(feature_map)
- jacobian_map = jacobian_map.reshape(final_shape[0], self.num_jacobian_maps, 9, final_shape[2],
- final_shape[3], final_shape[4])
- heatmap = heatmap.unsqueeze(2)
-
- jacobian = heatmap * jacobian_map
- jacobian = jacobian.view(final_shape[0], final_shape[1], 9, -1)
- jacobian = jacobian.sum(dim=-1)
- jacobian = jacobian.view(jacobian.shape[0], jacobian.shape[1], 3, 3)
- out['jacobian'] = jacobian
-
- return out
-
-
-class HEEstimator(nn.Module):
- """
- Estimating head pose and expression.
- """
-
- def __init__(self, block_expansion, feature_channel, num_kp, image_channel, max_features, num_bins=66, estimate_jacobian=True):
- super(HEEstimator, self).__init__()
-
- self.conv1 = nn.Conv2d(in_channels=image_channel, out_channels=block_expansion, kernel_size=7, padding=3, stride=2)
- self.norm1 = BatchNorm2d(block_expansion, affine=True)
- self.maxpool = nn.MaxPool2d(kernel_size=3, stride=2, padding=1)
-
- self.conv2 = nn.Conv2d(in_channels=block_expansion, out_channels=256, kernel_size=1)
- self.norm2 = BatchNorm2d(256, affine=True)
-
- self.block1 = nn.Sequential()
- for i in range(3):
- self.block1.add_module('b1_'+ str(i), ResBottleneck(in_features=256, stride=1))
-
- self.conv3 = nn.Conv2d(in_channels=256, out_channels=512, kernel_size=1)
- self.norm3 = BatchNorm2d(512, affine=True)
- self.block2 = ResBottleneck(in_features=512, stride=2)
-
- self.block3 = nn.Sequential()
- for i in range(3):
- self.block3.add_module('b3_'+ str(i), ResBottleneck(in_features=512, stride=1))
-
- self.conv4 = nn.Conv2d(in_channels=512, out_channels=1024, kernel_size=1)
- self.norm4 = BatchNorm2d(1024, affine=True)
- self.block4 = ResBottleneck(in_features=1024, stride=2)
-
- self.block5 = nn.Sequential()
- for i in range(5):
- self.block5.add_module('b5_'+ str(i), ResBottleneck(in_features=1024, stride=1))
-
- self.conv5 = nn.Conv2d(in_channels=1024, out_channels=2048, kernel_size=1)
- self.norm5 = BatchNorm2d(2048, affine=True)
- self.block6 = ResBottleneck(in_features=2048, stride=2)
-
- self.block7 = nn.Sequential()
- for i in range(2):
- self.block7.add_module('b7_'+ str(i), ResBottleneck(in_features=2048, stride=1))
-
- self.fc_roll = nn.Linear(2048, num_bins)
- self.fc_pitch = nn.Linear(2048, num_bins)
- self.fc_yaw = nn.Linear(2048, num_bins)
-
- self.fc_t = nn.Linear(2048, 3)
-
- self.fc_exp = nn.Linear(2048, 3*num_kp)
-
- def forward(self, x):
- out = self.conv1(x)
- out = self.norm1(out)
- out = F.relu(out)
- out = self.maxpool(out)
-
- out = self.conv2(out)
- out = self.norm2(out)
- out = F.relu(out)
-
- out = self.block1(out)
-
- out = self.conv3(out)
- out = self.norm3(out)
- out = F.relu(out)
- out = self.block2(out)
-
- out = self.block3(out)
-
- out = self.conv4(out)
- out = self.norm4(out)
- out = F.relu(out)
- out = self.block4(out)
-
- out = self.block5(out)
-
- out = self.conv5(out)
- out = self.norm5(out)
- out = F.relu(out)
- out = self.block6(out)
-
- out = self.block7(out)
-
- out = F.adaptive_avg_pool2d(out, 1)
- out = out.view(out.shape[0], -1)
-
- yaw = self.fc_roll(out)
- pitch = self.fc_pitch(out)
- roll = self.fc_yaw(out)
- t = self.fc_t(out)
- exp = self.fc_exp(out)
-
- return {'yaw': yaw, 'pitch': pitch, 'roll': roll, 't': t, 'exp': exp}
-
diff --git a/spaces/kinyugo/msanii/README.md b/spaces/kinyugo/msanii/README.md
deleted file mode 100644
index 97fe66e50141d956f39facced5f86cb76d06edf5..0000000000000000000000000000000000000000
--- a/spaces/kinyugo/msanii/README.md
+++ /dev/null
@@ -1,13 +0,0 @@
----
-title: Msanii
-emoji: 👁
-colorFrom: indigo
-colorTo: pink
-sdk: gradio
-sdk_version: 3.16.2
-app_file: app.py
-pinned: false
-license: mit
----
-
-Check out the configuration reference at https://huggingface.co/docs/hub/spaces-config-reference
diff --git a/spaces/koajoel/PolyFormer/fairseq/examples/linformer/linformer_src/modules/linformer_sentence_encoder_layer.py b/spaces/koajoel/PolyFormer/fairseq/examples/linformer/linformer_src/modules/linformer_sentence_encoder_layer.py
deleted file mode 100644
index 7e2caa03400129ac0bb34ae35274cdf46f27a055..0000000000000000000000000000000000000000
--- a/spaces/koajoel/PolyFormer/fairseq/examples/linformer/linformer_src/modules/linformer_sentence_encoder_layer.py
+++ /dev/null
@@ -1,65 +0,0 @@
-# Copyright (c) Facebook, Inc. and its affiliates.
-#
-# This source code is licensed under the MIT license found in the
-# LICENSE file in the root directory of this source tree.
-
-import torch
-from fairseq import utils
-from fairseq.modules import TransformerEncoderLayer
-
-from .multihead_linear_attention import MultiheadLinearAttention
-
-
-class LinformerTransformerEncoderLayer(TransformerEncoderLayer):
- """
- Implements a Linformer Encoder Layer used in BERT/XLM style pre-trained
- models.
- """
-
- def __init__(self, args, shared_compress_layer):
- # wrap in a list so it's not automatically registered by PyTorch
- self.shared_compress_layer = [shared_compress_layer]
-
- super().__init__(args)
-
- self.register_buffer("version", torch.tensor(2))
-
- def build_self_attention(self, embed_dim, args):
- return MultiheadLinearAttention(
- embed_dim,
- args.encoder_attention_heads,
- dropout=args.dropout,
- self_attention=True,
- q_noise=args.quant_noise_pq,
- qn_block_size=args.quant_noise_pq_block_size,
- compressed=args.compressed,
- max_seq_len=args.max_positions,
- shared_kv_compressed=args.shared_kv_compressed,
- shared_compress_layer=self.shared_compress_layer[0],
- freeze_compress=args.freeze_compress,
- )
-
- def upgrade_state_dict_named(self, state_dict, name):
- super().upgrade_state_dict_named(state_dict, name)
- prefix = name + "." if name != "" else ""
-
- # some old checkpoints had weight sharing implemented incorrectly
- # (note: this was correct in the original paper code)
- if utils.item(state_dict.get(f"{prefix}version", torch.tensor(1))) < 2:
- state_dict[f"{prefix}version"] = torch.tensor(1)
- # check compression layer sharing
- if f"{prefix}shared_compress_layer.weight" in state_dict:
- # reinitialize block without sharing compression layer to match
- # old behavior
- self.shared_compress_layer = [
- torch.nn.Linear(
- self.shared_compress_layer[0].weight.size(1),
- self.shared_compress_layer[0].weight.size(0),
- )
- ]
- self.self_attn = self.build_self_attention(self.embed_dim, self.args)
- # delete shared_compress_layer, since it's already copied to
- # self_attn.compress_k.weight
- del state_dict[f"{prefix}shared_compress_layer.weight"]
- if f"{prefix}shared_compress_layer.bias" in state_dict:
- del state_dict[f"{prefix}shared_compress_layer.bias"]
diff --git a/spaces/kquote03/lama-video-watermark-remover/saicinpainting/training/__init__.py b/spaces/kquote03/lama-video-watermark-remover/saicinpainting/training/__init__.py
deleted file mode 100644
index e69de29bb2d1d6434b8b29ae775ad8c2e48c5391..0000000000000000000000000000000000000000
diff --git a/spaces/krystian-lieber/codellama-34b-chat/app.py b/spaces/krystian-lieber/codellama-34b-chat/app.py
deleted file mode 100644
index fc0a6d92cd26665ae82c16dff92a8552beddee67..0000000000000000000000000000000000000000
--- a/spaces/krystian-lieber/codellama-34b-chat/app.py
+++ /dev/null
@@ -1,276 +0,0 @@
-import os
-from typing import Iterator
-
-import gradio as gr
-
-from model import run
-
-HF_PUBLIC = os.environ.get("HF_PUBLIC", False)
-
-DEFAULT_SYSTEM_PROMPT = """\
-You are a helpful, respectful and honest assistant with a deep knowledge of code and software design. Always answer as helpfully as possible, while being safe. Your answers should not include any harmful, unethical, racist, sexist, toxic, dangerous, or illegal content. Please ensure that your responses are socially unbiased and positive in nature.\n\nIf a question does not make any sense, or is not factually coherent, explain why instead of answering something not correct. If you don't know the answer to a question, please don't share false information.\
-"""
-MAX_MAX_NEW_TOKENS = 4096
-DEFAULT_MAX_NEW_TOKENS = 1024
-MAX_INPUT_TOKEN_LENGTH = 4000
-
-DESCRIPTION = """
-# Code Llama 34B Chat
-
-This Space demonstrates model [CodeLlama-34b-Instruct](https://huggingface.co/codellama/CodeLlama-34b-Instruct-hf) by Meta, a Code Llama model with 34B parameters fine-tuned for chat instructions and specialized on code tasks. Feel free to play with it, or duplicate to run generations without a queue! If you want to run your own service, you can also [deploy the model on Inference Endpoints](https://huggingface.co/inference-endpoints).
-
-🔎 For more details about the Code Llama family of models and how to use them with `transformers`, take a look [at our blog post](https://huggingface.co/blog/codellama) or [the paper](https://huggingface.co/papers/2308.12950).
-
-🏃🏻 Check out our [Playground](https://huggingface.co/spaces/codellama/codellama-playground) for a super-fast code completion demo that leverages a streaming [inference endpoint](https://huggingface.co/inference-endpoints).
-
-"""
-
-LICENSE = """
-
-
----
-As a derivate work of Code Llama by Meta,
-this demo is governed by the original [license](https://huggingface.co/spaces/huggingface-projects/codellama-2-34b-chat/blob/main/LICENSE.txt) and [acceptable use policy](https://huggingface.co/spaces/huggingface-projects/codellama-2-34b-chat/blob/main/USE_POLICY.md).
-"""
-
-
-def clear_and_save_textbox(message: str) -> tuple[str, str]:
- return '', message
-
-
-def display_input(message: str,
- history: list[tuple[str, str]]) -> list[tuple[str, str]]:
- history.append((message, ''))
- return history
-
-
-def delete_prev_fn(
- history: list[tuple[str, str]]) -> tuple[list[tuple[str, str]], str]:
- try:
- message, _ = history.pop()
- except IndexError:
- message = ''
- return history, message or ''
-
-
-def generate(
- message: str,
- history_with_input: list[tuple[str, str]],
- system_prompt: str,
- max_new_tokens: int,
- temperature: float,
- top_p: float,
- top_k: int,
-) -> Iterator[list[tuple[str, str]]]:
- if max_new_tokens > MAX_MAX_NEW_TOKENS:
- raise ValueError
-
- history = history_with_input[:-1]
- generator = run(message, history, system_prompt, max_new_tokens, temperature, top_p, top_k)
- try:
- first_response = next(generator)
- yield history + [(message, first_response)]
- except StopIteration:
- yield history + [(message, '')]
- for response in generator:
- yield history + [(message, response)]
-
-
-def process_example(message: str) -> tuple[str, list[tuple[str, str]]]:
- generator = generate(message, [], DEFAULT_SYSTEM_PROMPT, 1024, 1, 0.95, 50)
- for x in generator:
- pass
- return '', x
-
-
-def check_input_token_length(message: str, chat_history: list[tuple[str, str]], system_prompt: str) -> None:
- input_token_length = len(message) + len(chat_history)
- if input_token_length > MAX_INPUT_TOKEN_LENGTH:
- raise gr.Error(f'The accumulated input is too long ({input_token_length} > {MAX_INPUT_TOKEN_LENGTH}). Clear your chat history and try again.')
-
-
-with gr.Blocks(css='style.css') as demo:
- gr.Markdown(DESCRIPTION)
- gr.DuplicateButton(value='Duplicate Space for private use',
- elem_id='duplicate-button')
-
- with gr.Group():
- chatbot = gr.Chatbot(label='Chatbot')
- with gr.Row():
- textbox = gr.Textbox(
- container=False,
- show_label=False,
- placeholder='Type a message...',
- scale=10,
- )
- submit_button = gr.Button('Submit',
- variant='primary',
- scale=1,
- min_width=0)
- with gr.Row():
- retry_button = gr.Button('🔄 Retry', variant='secondary')
- undo_button = gr.Button('↩️ Undo', variant='secondary')
- clear_button = gr.Button('🗑️ Clear', variant='secondary')
-
- saved_input = gr.State()
-
- with gr.Accordion(label='Advanced options', open=False):
- system_prompt = gr.Textbox(label='System prompt',
- value=DEFAULT_SYSTEM_PROMPT,
- lines=6)
- max_new_tokens = gr.Slider(
- label='Max new tokens',
- minimum=1,
- maximum=MAX_MAX_NEW_TOKENS,
- step=1,
- value=DEFAULT_MAX_NEW_TOKENS,
- )
- temperature = gr.Slider(
- label='Temperature',
- minimum=0.1,
- maximum=4.0,
- step=0.1,
- value=0.1,
- )
- top_p = gr.Slider(
- label='Top-p (nucleus sampling)',
- minimum=0.05,
- maximum=1.0,
- step=0.05,
- value=0.9,
- )
- top_k = gr.Slider(
- label='Top-k',
- minimum=1,
- maximum=1000,
- step=1,
- value=10,
- )
-
- gr.Examples(
- examples=[
- 'What is the Fibonacci sequence?',
- 'Can you explain briefly what Python is good for?',
- 'How can I display a grid of images in SwiftUI?',
- ],
- inputs=textbox,
- outputs=[textbox, chatbot],
- fn=process_example,
- cache_examples=True,
- )
-
- gr.Markdown(LICENSE)
-
- textbox.submit(
- fn=clear_and_save_textbox,
- inputs=textbox,
- outputs=[textbox, saved_input],
- api_name=False,
- queue=False,
- ).then(
- fn=display_input,
- inputs=[saved_input, chatbot],
- outputs=chatbot,
- api_name=False,
- queue=False,
- ).then(
- fn=check_input_token_length,
- inputs=[saved_input, chatbot, system_prompt],
- api_name=False,
- queue=False,
- ).success(
- fn=generate,
- inputs=[
- saved_input,
- chatbot,
- system_prompt,
- max_new_tokens,
- temperature,
- top_p,
- top_k,
- ],
- outputs=chatbot,
- api_name=False,
- )
-
- button_event_preprocess = submit_button.click(
- fn=clear_and_save_textbox,
- inputs=textbox,
- outputs=[textbox, saved_input],
- api_name=False,
- queue=False,
- ).then(
- fn=display_input,
- inputs=[saved_input, chatbot],
- outputs=chatbot,
- api_name=False,
- queue=False,
- ).then(
- fn=check_input_token_length,
- inputs=[saved_input, chatbot, system_prompt],
- api_name=False,
- queue=False,
- ).success(
- fn=generate,
- inputs=[
- saved_input,
- chatbot,
- system_prompt,
- max_new_tokens,
- temperature,
- top_p,
- top_k,
- ],
- outputs=chatbot,
- api_name=False,
- )
-
- retry_button.click(
- fn=delete_prev_fn,
- inputs=chatbot,
- outputs=[chatbot, saved_input],
- api_name=False,
- queue=False,
- ).then(
- fn=display_input,
- inputs=[saved_input, chatbot],
- outputs=chatbot,
- api_name=False,
- queue=False,
- ).then(
- fn=generate,
- inputs=[
- saved_input,
- chatbot,
- system_prompt,
- max_new_tokens,
- temperature,
- top_p,
- top_k,
- ],
- outputs=chatbot,
- api_name=False,
- )
-
- undo_button.click(
- fn=delete_prev_fn,
- inputs=chatbot,
- outputs=[chatbot, saved_input],
- api_name=False,
- queue=False,
- ).then(
- fn=lambda x: x,
- inputs=[saved_input],
- outputs=textbox,
- api_name=False,
- queue=False,
- )
-
- clear_button.click(
- fn=lambda: ([], ''),
- outputs=[chatbot, saved_input],
- queue=False,
- api_name=False,
- )
-
-demo.queue(max_size=32).launch(share=HF_PUBLIC)
diff --git a/spaces/kxqt/Expedit-SAM/setup.py b/spaces/kxqt/Expedit-SAM/setup.py
deleted file mode 100644
index 2c0986317eb576a14ec774205c88fdee3cc6c0b3..0000000000000000000000000000000000000000
--- a/spaces/kxqt/Expedit-SAM/setup.py
+++ /dev/null
@@ -1,18 +0,0 @@
-# Copyright (c) Meta Platforms, Inc. and affiliates.
-# All rights reserved.
-
-# This source code is licensed under the license found in the
-# LICENSE file in the root directory of this source tree.
-
-from setuptools import find_packages, setup
-
-setup(
- name="segment_anything",
- version="1.0",
- install_requires=[],
- packages=find_packages(exclude="notebooks"),
- extras_require={
- "all": ["matplotlib", "pycocotools", "opencv-python", "onnx", "onnxruntime"],
- "dev": ["flake8", "isort", "black", "mypy"],
- },
-)
diff --git a/spaces/ky2k/Toxicity_Classifier_POC/.venv/lib/python3.9/site-packages/PIL/_version.py b/spaces/ky2k/Toxicity_Classifier_POC/.venv/lib/python3.9/site-packages/PIL/_version.py
deleted file mode 100644
index d94d35934401a72eef61a3ae3a22d493dcc909e9..0000000000000000000000000000000000000000
--- a/spaces/ky2k/Toxicity_Classifier_POC/.venv/lib/python3.9/site-packages/PIL/_version.py
+++ /dev/null
@@ -1,2 +0,0 @@
-# Master version for Pillow
-__version__ = "9.5.0"
diff --git a/spaces/ky2k/Toxicity_Classifier_POC/.venv/lib/python3.9/site-packages/fsspec/implementations/tar.py b/spaces/ky2k/Toxicity_Classifier_POC/.venv/lib/python3.9/site-packages/fsspec/implementations/tar.py
deleted file mode 100644
index 772ebd4d6a251dd89c03190abbda50f09fa2bf7a..0000000000000000000000000000000000000000
--- a/spaces/ky2k/Toxicity_Classifier_POC/.venv/lib/python3.9/site-packages/fsspec/implementations/tar.py
+++ /dev/null
@@ -1,123 +0,0 @@
-import logging
-import tarfile
-
-import fsspec
-from fsspec.archive import AbstractArchiveFileSystem
-from fsspec.compression import compr
-from fsspec.utils import infer_compression
-
-typemap = {b"0": "file", b"5": "directory"}
-
-logger = logging.getLogger("tar")
-
-
-class TarFileSystem(AbstractArchiveFileSystem):
- """Compressed Tar archives as a file-system (read-only)
-
- Supports the following formats:
- tar.gz, tar.bz2, tar.xz
- """
-
- root_marker = ""
- protocol = "tar"
- cachable = False
-
- def __init__(
- self,
- fo="",
- index_store=None,
- target_options=None,
- target_protocol=None,
- compression=None,
- **kwargs,
- ):
- super().__init__(**kwargs)
- target_options = target_options or {}
-
- if isinstance(fo, str):
- self.of = fsspec.open(fo, protocol=target_protocol, **target_options)
- fo = self.of.open() # keep the reference
-
- # Try to infer compression.
- if compression is None:
- name = None
-
- # Try different ways to get hold of the filename. `fo` might either
- # be a `fsspec.LocalFileOpener`, an `io.BufferedReader` or an
- # `fsspec.AbstractFileSystem` instance.
- try:
- # Amended io.BufferedReader or similar.
- # This uses a "protocol extension" where original filenames are
- # propagated to archive-like filesystems in order to let them
- # infer the right compression appropriately.
- if hasattr(fo, "original"):
- name = fo.original
-
- # fsspec.LocalFileOpener
- elif hasattr(fo, "path"):
- name = fo.path
-
- # io.BufferedReader
- elif hasattr(fo, "name"):
- name = fo.name
-
- # fsspec.AbstractFileSystem
- elif hasattr(fo, "info"):
- name = fo.info()["name"]
-
- except Exception as ex:
- logger.warning(
- f"Unable to determine file name, not inferring compression: {ex}"
- )
-
- if name is not None:
- compression = infer_compression(name)
- logger.info(f"Inferred compression {compression} from file name {name}")
-
- if compression is not None:
- # TODO: tarfile already implements compression with modes like "'r:gz'",
- # but then would seek to offset in the file work?
- fo = compr[compression](fo)
-
- self._fo_ref = fo
- self.fo = fo # the whole instance is a context
- self.tar: tarfile.TarFile = tarfile.TarFile(fileobj=self.fo)
- self.dir_cache = None
-
- self.index_store = index_store
- self.index = None
- self._index()
-
- def _index(self):
- # TODO: load and set saved index, if exists
- out = {}
- for ti in self.tar:
- info = ti.get_info()
- info["type"] = typemap.get(info["type"], "file")
- name = ti.get_info()["name"].rstrip("/")
- out[name] = (info, ti.offset_data)
-
- self.index = out
- # TODO: save index to self.index_store here, if set
-
- def _get_dirs(self):
- if self.dir_cache is not None:
- return
-
- # This enables ls to get directories as children as well as files
- self.dir_cache = {
- dirname + "/": {"name": dirname + "/", "size": 0, "type": "directory"}
- for dirname in self._all_dirnames(self.tar.getnames())
- }
- for member in self.tar.getmembers():
- info = member.get_info()
- info["type"] = typemap.get(info["type"], "file")
- self.dir_cache[info["name"]] = info
-
- def _open(self, path, mode="rb", **kwargs):
- if mode != "rb":
- raise ValueError("Read-only filesystem implementation")
- details, offset = self.index[path]
- if details["type"] != "file":
- raise ValueError("Can only handle regular files")
- return self.tar.extractfile(path)
diff --git a/spaces/ky2k/Toxicity_Classifier_POC/.venv/lib/python3.9/site-packages/gradio/templates/cdn/assets/Form-189d7bad.css b/spaces/ky2k/Toxicity_Classifier_POC/.venv/lib/python3.9/site-packages/gradio/templates/cdn/assets/Form-189d7bad.css
deleted file mode 100644
index 0b8e23a00e51fe054b66be462ce774d415cd57aa..0000000000000000000000000000000000000000
--- a/spaces/ky2k/Toxicity_Classifier_POC/.venv/lib/python3.9/site-packages/gradio/templates/cdn/assets/Form-189d7bad.css
+++ /dev/null
@@ -1 +0,0 @@
-div.svelte-b6y5bg{display:flex;flex-direction:inherit;flex-wrap:wrap;gap:var(--form-gap-width);box-shadow:var(--block-shadow);border:var(--block-border-width) solid var(--border-color-primary);border-radius:var(--block-radius);background:var(--border-color-primary);overflow:hidden}div.svelte-b6y5bg .block{box-shadow:none!important;border-width:0px!important;border-radius:0!important}.hidden.svelte-b6y5bg{display:none}
diff --git a/spaces/ky2k/Toxicity_Classifier_POC/.venv/lib/python3.9/site-packages/gradio/templates/cdn/assets/ModifyUpload-77b0d4b2.css b/spaces/ky2k/Toxicity_Classifier_POC/.venv/lib/python3.9/site-packages/gradio/templates/cdn/assets/ModifyUpload-77b0d4b2.css
deleted file mode 100644
index c78d71f8b6eaf75f8134375ed017f1c03b6edf1a..0000000000000000000000000000000000000000
--- a/spaces/ky2k/Toxicity_Classifier_POC/.venv/lib/python3.9/site-packages/gradio/templates/cdn/assets/ModifyUpload-77b0d4b2.css
+++ /dev/null
@@ -1 +0,0 @@
-div.svelte-116rqfv{cursor:pointer;width:var(--size-full);height:var(--size-full)}.center.svelte-116rqfv{text-align:center}.flex.svelte-116rqfv{display:flex;justify-content:center;align-items:center}input.svelte-116rqfv{display:none}div.svelte-19sk1im{display:flex;top:var(--size-2);right:var(--size-2);justify-content:flex-end;gap:var(--spacing-sm);z-index:var(--layer-1)}.not-absolute.svelte-19sk1im{margin:var(--size-1)}
diff --git a/spaces/ky2k/Toxicity_Classifier_POC/.venv/lib/python3.9/site-packages/huggingface_hub/_inference.py b/spaces/ky2k/Toxicity_Classifier_POC/.venv/lib/python3.9/site-packages/huggingface_hub/_inference.py
deleted file mode 100644
index 4aab6568951266322e69501a6fb6bea46ccc3ad8..0000000000000000000000000000000000000000
--- a/spaces/ky2k/Toxicity_Classifier_POC/.venv/lib/python3.9/site-packages/huggingface_hub/_inference.py
+++ /dev/null
@@ -1,890 +0,0 @@
-# coding=utf-8
-# Copyright 2019-present, the HuggingFace Inc. team.
-#
-# Licensed under the Apache License, Version 2.0 (the "License");
-# you may not use this file except in compliance with the License.
-# You may obtain a copy of the License at
-#
-# http://www.apache.org/licenses/LICENSE-2.0
-#
-# Unless required by applicable law or agreed to in writing, software
-# distributed under the License is distributed on an "AS IS" BASIS,
-# WITHOUT WARRANTIES OR CONDITIONS OF ANY KIND, either express or implied.
-# See the License for the specific language governing permissions and
-# limitations under the License.
-#
-# Related resources:
-# https://huggingface.co/tasks
-# https://huggingface.co/docs/huggingface.js/inference/README
-# https://github.com/huggingface/huggingface.js/tree/main/packages/inference/src
-# https://github.com/huggingface/text-generation-inference/tree/main/clients/python
-# https://github.com/huggingface/text-generation-inference/blob/main/clients/python/text_generation/client.py
-# https://huggingface.slack.com/archives/C03E4DQ9LAJ/p1680169099087869
-# https://github.com/huggingface/unity-api#tasks
-#
-# Some TODO:
-# - validate inputs/options/parameters? with Pydantic for instance? or only optionally?
-# - add all tasks
-# - handle async requests
-#
-# NOTE: the philosophy of this client is "let's make it as easy as possible to use it, even if less optimized". Some
-# examples of how it translates:
-# - Timeout / Server unavailable is handled by the client in a single "timeout" parameter.
-# - Files can be provided as bytes, file paths, or URLs and the client will try to "guess" the type.
-# - Images are parsed as PIL.Image for easier manipulation.
-# - Provides a "recommended model" for each task => suboptimal but user-wise quicker to get a first script running.
-# - Only the main parameters are publicly exposed. Power users can always read the docs for more options.
-import base64
-import io
-import logging
-import time
-import warnings
-from contextlib import contextmanager
-from pathlib import Path
-from typing import TYPE_CHECKING, Any, BinaryIO, ContextManager, Dict, Generator, List, Optional, Union, overload
-
-from requests import HTTPError, Response
-
-from ._inference_types import ClassificationOutput, ConversationalOutput, ImageSegmentationOutput
-from .constants import INFERENCE_ENDPOINT
-from .utils import build_hf_headers, get_session, hf_raise_for_status, is_numpy_available, is_pillow_available
-from .utils._typing import Literal
-
-
-if TYPE_CHECKING:
- import numpy as np
- from PIL import Image
-
-logger = logging.getLogger(__name__)
-
-
-RECOMMENDED_MODELS = {
- "audio-classification": "superb/hubert-large-superb-er",
- "automatic-speech-recognition": "facebook/wav2vec2-large-960h-lv60-self",
- "conversational": "microsoft/DialoGPT-large",
- "feature-extraction": "facebook/bart-base",
- "image-classification": "google/vit-base-patch16-224",
- "image-segmentation": "facebook/detr-resnet-50-panoptic",
- "image-to-image": "timbrooks/instruct-pix2pix",
- "image-to-text": "nlpconnect/vit-gpt2-image-captioning",
- "sentence-similarity": "sentence-transformers/all-MiniLM-L6-v2",
- "summarization": "facebook/bart-large-cnn",
- "text-to-image": "stabilityai/stable-diffusion-2-1",
- "text-to-speech": "espnet/kan-bayashi_ljspeech_vits",
-}
-
-UrlT = str
-PathT = Union[str, Path]
-BinaryT = Union[bytes, BinaryIO]
-ContentT = Union[BinaryT, PathT, UrlT]
-
-
-class InferenceTimeoutError(HTTPError, TimeoutError):
- """Error raised when a model is unavailable or the request times out."""
-
-
-class InferenceClient:
- """
- Initialize a new Inference Client.
-
- [`InferenceClient`] aims to provide a unified experience to perform inference. The client can be used
- seamlessly with either the (free) Inference API or self-hosted Inference Endpoints.
-
- Args:
- model (`str`, `optional`):
- The model to run inference with. Can be a model id hosted on the Hugging Face Hub, e.g. `bigcode/starcoder`
- or a URL to a deployed Inference Endpoint. Defaults to None, in which case a recommended model is
- automatically selected for the task.
- token (`str`, *optional*):
- Hugging Face token. Will default to the locally saved token.
- timeout (`float`, `optional`):
- The maximum number of seconds to wait for a response from the server. Loading a new model in Inference
- API can take up to several minutes. Defaults to None, meaning it will loop until the server is available.
- """
-
- def __init__(
- self, model: Optional[str] = None, token: Optional[str] = None, timeout: Optional[float] = None
- ) -> None:
- self.model: Optional[str] = model
- self.headers = build_hf_headers(token=token)
- self.timeout = timeout
-
- def __repr__(self):
- return f""
-
- def post(
- self,
- *,
- json: Optional[Union[str, Dict, List]] = None,
- data: Optional[ContentT] = None,
- model: Optional[str] = None,
- task: Optional[str] = None,
- ) -> Response:
- """
- Make a POST request to the inference server.
-
- Args:
- json (`Union[str, Dict, List]`, *optional*):
- The JSON data to send in the request body. Defaults to None.
- data (`Union[str, Path, bytes, BinaryIO]`, *optional*):
- The content to send in the request body. It can be raw bytes, a pointer to an opened file, a local file
- path, or a URL to an online resource (image, audio file,...). If both `json` and `data` are passed,
- `data` will take precedence. At least `json` or `data` must be provided. Defaults to None.
- model (`str`, *optional*):
- The model to use for inference. Can be a model ID hosted on the Hugging Face Hub or a URL to a deployed
- Inference Endpoint. Will override the model defined at the instance level. Defaults to None.
- task (`str`, *optional*):
- The task to perform on the inference. Used only to default to a recommended model if `model` is not
- provided. At least `model` or `task` must be provided. Defaults to None.
-
- Returns:
- Response: The `requests` HTTP response.
-
- Raises:
- [`InferenceTimeoutError`]:
- If the model is unavailable or the request times out.
- `HTTPError`:
- If the request fails with an HTTP error status code other than HTTP 503.
- """
- url = self._resolve_url(model, task)
-
- if data is not None and json is not None:
- warnings.warn("Ignoring `json` as `data` is passed as binary.")
-
- t0 = time.time()
- timeout = self.timeout
- while True:
- with _open_as_binary(data) as data_as_binary:
- try:
- response = get_session().post(
- url, json=json, data=data_as_binary, headers=self.headers, timeout=self.timeout
- )
- except TimeoutError as error:
- # Convert any `TimeoutError` to a `InferenceTimeoutError`
- raise InferenceTimeoutError(f"Inference call timed out: {url}") from error
-
- try:
- hf_raise_for_status(response)
- except HTTPError as error:
- if error.response.status_code == 503:
- # If Model is unavailable, either raise a TimeoutError...
- if timeout is not None and time.time() - t0 > timeout:
- raise InferenceTimeoutError(
- f"Model not loaded on the server: {url}. Please retry with a higher timeout (current:"
- f" {self.timeout})."
- ) from error
- # ...or wait 1s and retry
- logger.info(f"Waiting for model to be loaded on the server: {error}")
- time.sleep(1)
- if timeout is not None:
- timeout = max(self.timeout - (time.time() - t0), 1) # type: ignore
- continue
- raise
- break
- return response
-
- def audio_classification(
- self,
- audio: ContentT,
- *,
- model: Optional[str] = None,
- ) -> List[ClassificationOutput]:
- """
- Perform audio classification on the provided audio content.
-
- Args:
- audio (Union[str, Path, bytes, BinaryIO]):
- The audio content to classify. It can be raw audio bytes, a local audio file, or a URL pointing to an
- audio file.
- model (`str`, *optional*):
- The model to use for audio classification. Can be a model ID hosted on the Hugging Face Hub
- or a URL to a deployed Inference Endpoint. If not provided, the default recommended model for
- audio classification will be used.
-
- Returns:
- `List[Dict]`: The classification output containing the predicted label and its confidence.
-
- Raises:
- [`InferenceTimeoutError`]:
- If the model is unavailable or the request times out.
- `HTTPError`:
- If the request fails with an HTTP error status code other than HTTP 503.
-
- Example:
- ```py
- >>> from huggingface_hub import InferenceClient
- >>> client = InferenceClient()
- >>> client.audio_classification("audio.wav")
- [{'score': 0.4976358711719513, 'label': 'hap'}, {'score': 0.3677836060523987, 'label': 'neu'},...]
- ```
- """
- response = self.post(data=audio, model=model, task="audio-classification")
- return response.json()
-
- def automatic_speech_recognition(
- self,
- audio: ContentT,
- *,
- model: Optional[str] = None,
- ) -> str:
- """
- Perform automatic speech recognition (ASR or audio-to-text) on the given audio content.
-
- Args:
- audio (Union[str, Path, bytes, BinaryIO]):
- The content to transcribe. It can be raw audio bytes, local audio file, or a URL to an audio file.
- model (`str`, *optional*):
- The model to use for ASR. Can be a model ID hosted on the Hugging Face Hub or a URL to a deployed
- Inference Endpoint. If not provided, the default recommended model for ASR will be used.
-
- Returns:
- str: The transcribed text.
-
- Raises:
- [`InferenceTimeoutError`]:
- If the model is unavailable or the request times out.
- `HTTPError`:
- If the request fails with an HTTP error status code other than HTTP 503.
-
- Example:
- ```py
- >>> from huggingface_hub import InferenceClient
- >>> client = InferenceClient()
- >>> client.automatic_speech_recognition("hello_world.wav")
- "hello world"
- ```
- """
- response = self.post(data=audio, model=model, task="automatic-speech-recognition")
- return response.json()["text"]
-
- def conversational(
- self,
- text: str,
- generated_responses: Optional[List[str]] = None,
- past_user_inputs: Optional[List[str]] = None,
- *,
- parameters: Optional[Dict[str, Any]] = None,
- model: Optional[str] = None,
- ) -> ConversationalOutput:
- """
- Generate conversational responses based on the given input text (i.e. chat with the API).
-
- Args:
- text (`str`):
- The last input from the user in the conversation.
- generated_responses (`List[str]`, *optional*):
- A list of strings corresponding to the earlier replies from the model. Defaults to None.
- past_user_inputs (`List[str]`, *optional*):
- A list of strings corresponding to the earlier replies from the user. Should be the same length as
- `generated_responses`. Defaults to None.
- parameters (`Dict[str, Any]`, *optional*):
- Additional parameters for the conversational task. Defaults to None. For more details about the available
- parameters, please refer to [this page](https://huggingface.co/docs/api-inference/detailed_parameters#conversational-task)
- model (`str`, *optional*):
- The model to use for the conversational task. Can be a model ID hosted on the Hugging Face Hub or a URL to
- a deployed Inference Endpoint. If not provided, the default recommended conversational model will be used.
- Defaults to None.
-
- Returns:
- `Dict`: The generated conversational output.
-
- Raises:
- [`InferenceTimeoutError`]:
- If the model is unavailable or the request times out.
- `HTTPError`:
- If the request fails with an HTTP error status code other than HTTP 503.
-
- Example:
- ```py
- >>> from huggingface_hub import InferenceClient
- >>> client = InferenceClient()
- >>> output = client.conversational("Hi, who are you?")
- >>> output
- {'generated_text': 'I am the one who knocks.', 'conversation': {'generated_responses': ['I am the one who knocks.'], 'past_user_inputs': ['Hi, who are you?']}, 'warnings': ['Setting `pad_token_id` to `eos_token_id`:50256 for open-end generation.']}
- >>> client.conversational(
- ... "Wow, that's scary!",
- ... generated_responses=output["conversation"]["generated_responses"],
- ... past_user_inputs=output["conversation"]["past_user_inputs"],
- ... )
- ```
- """
- payload: Dict[str, Any] = {"inputs": {"text": text}}
- if generated_responses is not None:
- payload["inputs"]["generated_responses"] = generated_responses
- if past_user_inputs is not None:
- payload["inputs"]["past_user_inputs"] = past_user_inputs
- if parameters is not None:
- payload["parameters"] = parameters
- response = self.post(json=payload, model=model, task="conversational")
- return response.json()
-
- def feature_extraction(self, text: str, *, model: Optional[str] = None) -> "np.ndarray":
- """
- Generate embeddings for a given text.
-
- Args:
- text (`str`):
- The text to embed.
- model (`str`, *optional*):
- The model to use for the conversational task. Can be a model ID hosted on the Hugging Face Hub or a URL to
- a deployed Inference Endpoint. If not provided, the default recommended conversational model will be used.
- Defaults to None.
-
- Returns:
- `np.ndarray`: The embedding representing the input text as a float32 numpy array.
-
- Raises:
- [`InferenceTimeoutError`]:
- If the model is unavailable or the request times out.
- `HTTPError`:
- If the request fails with an HTTP error status code other than HTTP 503.
-
- Example:
- ```py
- >>> from huggingface_hub import InferenceClient
- >>> client = InferenceClient()
- >>> client.feature_extraction("Hi, who are you?")
- array([[ 2.424802 , 2.93384 , 1.1750331 , ..., 1.240499, -0.13776633, -0.7889173 ],
- [-0.42943227, -0.6364878 , -1.693462 , ..., 0.41978157, -2.4336355 , 0.6162071 ],
- ...,
- [ 0.28552425, -0.928395 , -1.2077185 , ..., 0.76810825, -2.1069427 , 0.6236161 ]], dtype=float32)
- ```
- """
- response = self.post(json={"inputs": text}, model=model, task="feature-extraction")
- np = _import_numpy()
- return np.array(response.json()[0], dtype="float32")
-
- def image_classification(
- self,
- image: ContentT,
- *,
- model: Optional[str] = None,
- ) -> List[ClassificationOutput]:
- """
- Perform image classification on the given image using the specified model.
-
- Args:
- image (`Union[str, Path, bytes, BinaryIO]`):
- The image to classify. It can be raw bytes, an image file, or a URL to an online image.
- model (`str`, *optional*):
- The model to use for image classification. Can be a model ID hosted on the Hugging Face Hub or a URL to a
- deployed Inference Endpoint. If not provided, the default recommended model for image classification will be used.
-
- Returns:
- `List[Dict]`: a list of dictionaries containing the predicted label and associated probability.
-
- Raises:
- [`InferenceTimeoutError`]:
- If the model is unavailable or the request times out.
- `HTTPError`:
- If the request fails with an HTTP error status code other than HTTP 503.
-
- Example:
- ```py
- >>> from huggingface_hub import InferenceClient
- >>> client = InferenceClient()
- >>> client.image_classification("https://upload.wikimedia.org/wikipedia/commons/thumb/4/43/Cute_dog.jpg/320px-Cute_dog.jpg")
- [{'score': 0.9779096841812134, 'label': 'Blenheim spaniel'}, ...]
- ```
- """
- response = self.post(data=image, model=model, task="image-classification")
- return response.json()
-
- def image_segmentation(
- self,
- image: ContentT,
- *,
- model: Optional[str] = None,
- ) -> List[ImageSegmentationOutput]:
- """
- Perform image segmentation on the given image using the specified model.
-
-
-
- You must have `PIL` installed if you want to work with images (`pip install Pillow`).
-
-
-
- Args:
- image (`Union[str, Path, bytes, BinaryIO]`):
- The image to segment. It can be raw bytes, an image file, or a URL to an online image.
- model (`str`, *optional*):
- The model to use for image segmentation. Can be a model ID hosted on the Hugging Face Hub or a URL to a
- deployed Inference Endpoint. If not provided, the default recommended model for image segmentation will be used.
-
- Returns:
- `List[Dict]`: A list of dictionaries containing the segmented masks and associated attributes.
-
- Raises:
- [`InferenceTimeoutError`]:
- If the model is unavailable or the request times out.
- `HTTPError`:
- If the request fails with an HTTP error status code other than HTTP 503.
-
- Example:
- ```py
- >>> from huggingface_hub import InferenceClient
- >>> client = InferenceClient()
- >>> client.image_segmentation("cat.jpg"):
- [{'score': 0.989008, 'label': 'LABEL_184', 'mask': }, ...]
- ```
- """
-
- # Segment
- response = self.post(data=image, model=model, task="image-segmentation")
- output = response.json()
-
- # Parse masks as PIL Image
- if not isinstance(output, list):
- raise ValueError(f"Server output must be a list. Got {type(output)}: {str(output)[:200]}...")
- for item in output:
- item["mask"] = _b64_to_image(item["mask"])
- return output
-
- def image_to_image(
- self,
- image: ContentT,
- prompt: Optional[str] = None,
- *,
- negative_prompt: Optional[str] = None,
- height: Optional[int] = None,
- width: Optional[int] = None,
- num_inference_steps: Optional[int] = None,
- guidance_scale: Optional[float] = None,
- model: Optional[str] = None,
- **kwargs,
- ) -> "Image":
- """
- Perform image-to-image translation using a specified model.
-
-
-
- You must have `PIL` installed if you want to work with images (`pip install Pillow`).
-
-
-
- Args:
- image (`Union[str, Path, bytes, BinaryIO]`):
- The input image for translation. It can be raw bytes, an image file, or a URL to an online image.
- prompt (`str`, *optional*):
- The text prompt to guide the image generation.
- negative_prompt (`str`, *optional*):
- A negative prompt to guide the translation process.
- height (`int`, *optional*):
- The height in pixels of the generated image.
- width (`int`, *optional*):
- The width in pixels of the generated image.
- num_inference_steps (`int`, *optional*):
- The number of denoising steps. More denoising steps usually lead to a higher quality image at the
- expense of slower inference.
- guidance_scale (`float`, *optional*):
- Higher guidance scale encourages to generate images that are closely linked to the text `prompt`,
- usually at the expense of lower image quality.
- model (`str`, *optional*):
- The model to use for inference. Can be a model ID hosted on the Hugging Face Hub or a URL to a deployed
- Inference Endpoint. This parameter overrides the model defined at the instance level. Defaults to None.
-
- Returns:
- `Image`: The translated image.
-
- Raises:
- [`InferenceTimeoutError`]:
- If the model is unavailable or the request times out.
- `HTTPError`:
- If the request fails with an HTTP error status code other than HTTP 503.
-
- Example:
- ```py
- >>> from huggingface_hub import InferenceClient
- >>> client = InferenceClient()
- >>> image = client.image_to_image("cat.jpg", prompt="turn the cat into a tiger")
- >>> image.save("tiger.jpg")
- ```
- """
- parameters = {
- "prompt": prompt,
- "negative_prompt": negative_prompt,
- "height": height,
- "width": width,
- "num_inference_steps": num_inference_steps,
- "guidance_scale": guidance_scale,
- **kwargs,
- }
- if all(parameter is None for parameter in parameters.values()):
- # Either only an image to send => send as raw bytes
- self.post(data=image, model=model, task="image-to-image")
- data = image
- payload: Optional[Dict[str, Any]] = None
- else:
- # Or an image + some parameters => use base64 encoding
- data = None
- payload = {"inputs": _b64_encode(image)}
- for key, value in parameters.items():
- if value is not None:
- payload[key] = value
-
- response = self.post(json=payload, data=data, model=model, task="image-to-image")
- return _response_to_image(response)
-
- def image_to_text(self, image: ContentT, *, model: Optional[str] = None) -> str:
- """
- Takes an input image and return text.
-
- Models can have very different outputs depending on your use case (image captioning, optical character recognition
- (OCR), Pix2Struct, etc). Please have a look to the model card to learn more about a model's specificities.
-
- Args:
- image (`Union[str, Path, bytes, BinaryIO]`):
- The input image to caption. It can be raw bytes, an image file, or a URL to an online image..
- model (`str`, *optional*):
- The model to use for inference. Can be a model ID hosted on the Hugging Face Hub or a URL to a deployed
- Inference Endpoint. This parameter overrides the model defined at the instance level. Defaults to None.
-
- Returns:
- `str`: The generated text.
-
- Raises:
- [`InferenceTimeoutError`]:
- If the model is unavailable or the request times out.
- `HTTPError`:
- If the request fails with an HTTP error status code other than HTTP 503.
-
- Example:
- ```py
- >>> from huggingface_hub import InferenceClient
- >>> client = InferenceClient()
- >>> client.image_to_text("cat.jpg")
- 'a cat standing in a grassy field '
- >>> client.image_to_text("https://upload.wikimedia.org/wikipedia/commons/thumb/4/43/Cute_dog.jpg/320px-Cute_dog.jpg")
- 'a dog laying on the grass next to a flower pot '
- ```
- """
- response = self.post(data=image, model=model, task="image-to-text")
- return response.json()[0]["generated_text"]
-
- def sentence_similarity(
- self, sentence: str, other_sentences: List[str], *, model: Optional[str] = None
- ) -> List[float]:
- """
- Compute the semantic similarity between a sentence and a list of other sentences by comparing their embeddings.
-
- Args:
- sentence (`str`):
- The main sentence to compare to others.
- other_sentences (`List[str]`):
- The list of sentences to compare to.
- model (`str`, *optional*):
- The model to use for the conversational task. Can be a model ID hosted on the Hugging Face Hub or a URL to
- a deployed Inference Endpoint. If not provided, the default recommended conversational model will be used.
- Defaults to None.
-
- Returns:
- `List[float]`: The embedding representing the input text.
-
- Raises:
- [`InferenceTimeoutError`]:
- If the model is unavailable or the request times out.
- `HTTPError`:
- If the request fails with an HTTP error status code other than HTTP 503.
-
- Example:
- ```py
- >>> from huggingface_hub import InferenceClient
- >>> client = InferenceClient()
- >>> client.sentence_similarity(
- ... "Machine learning is so easy.",
- ... other_sentences=[
- ... "Deep learning is so straightforward.",
- ... "This is so difficult, like rocket science.",
- ... "I can't believe how much I struggled with this.",
- ... ],
- ... )
- [0.7785726189613342, 0.45876261591911316, 0.2906220555305481]
- ```
- """
- response = self.post(
- json={"inputs": {"source_sentence": sentence, "sentences": other_sentences}},
- model=model,
- task="sentence-similarity",
- )
- return response.json()
-
- def summarization(
- self,
- text: str,
- *,
- parameters: Optional[Dict[str, Any]] = None,
- model: Optional[str] = None,
- ) -> str:
- """
- Generate a summary of a given text using a specified model.
-
- Args:
- text (`str`):
- The input text to summarize.
- parameters (`Dict[str, Any]`, *optional*):
- Additional parameters for summarization. Check out this [page](https://huggingface.co/docs/api-inference/detailed_parameters#summarization-task)
- for more details.
- model (`str`, *optional*):
- The model to use for inference. Can be a model ID hosted on the Hugging Face Hub or a URL to a deployed
- Inference Endpoint. This parameter overrides the model defined at the instance level. Defaults to None.
-
- Returns:
- `str`: The generated summary text.
-
- Raises:
- [`InferenceTimeoutError`]:
- If the model is unavailable or the request times out.
- `HTTPError`:
- If the request fails with an HTTP error status code other than HTTP 503.
-
- Example:
- ```py
- >>> from huggingface_hub import InferenceClient
- >>> client = InferenceClient()
- >>> client.summarization("The Eiffel tower...")
- 'The Eiffel tower is one of the most famous landmarks in the world....'
- ```
- """
- payload: Dict[str, Any] = {"inputs": text}
- if parameters is not None:
- payload["parameters"] = parameters
- response = self.post(json=payload, model=model, task="summarization")
- return response.json()[0]["summary_text"]
-
- def text_to_image(
- self,
- prompt: str,
- *,
- negative_prompt: Optional[str] = None,
- height: Optional[float] = None,
- width: Optional[float] = None,
- num_inference_steps: Optional[float] = None,
- guidance_scale: Optional[float] = None,
- model: Optional[str] = None,
- **kwargs,
- ) -> "Image":
- """
- Generate an image based on a given text using a specified model.
-
-
-
- You must have `PIL` installed if you want to work with images (`pip install Pillow`).
-
-
-
- Args:
- prompt (`str`):
- The prompt to generate an image from.
- negative_prompt (`str`, *optional*):
- An optional negative prompt for the image generation.
- height (`float`, *optional*):
- The height in pixels of the image to generate.
- width (`float`, *optional*):
- The width in pixels of the image to generate.
- num_inference_steps (`int`, *optional*):
- The number of denoising steps. More denoising steps usually lead to a higher quality image at the
- expense of slower inference.
- guidance_scale (`float`, *optional*):
- Higher guidance scale encourages to generate images that are closely linked to the text `prompt`,
- usually at the expense of lower image quality.
- model (`str`, *optional*):
- The model to use for inference. Can be a model ID hosted on the Hugging Face Hub or a URL to a deployed
- Inference Endpoint. This parameter overrides the model defined at the instance level. Defaults to None.
-
- Returns:
- `Image`: The generated image.
-
- Raises:
- [`InferenceTimeoutError`]:
- If the model is unavailable or the request times out.
- `HTTPError`:
- If the request fails with an HTTP error status code other than HTTP 503.
-
- Example:
- ```py
- >>> from huggingface_hub import InferenceClient
- >>> client = InferenceClient()
-
- >>> image = client.text_to_image("An astronaut riding a horse on the moon.")
- >>> image.save("astronaut.png")
-
- >>> image = client.text_to_image(
- ... "An astronaut riding a horse on the moon.",
- ... negative_prompt="low resolution, blurry",
- ... model="stabilityai/stable-diffusion-2-1",
- ... )
- >>> image.save("better_astronaut.png")
- ```
- """
- parameters = {
- "inputs": prompt,
- "negative_prompt": negative_prompt,
- "height": height,
- "width": width,
- "num_inference_steps": num_inference_steps,
- "guidance_scale": guidance_scale,
- **kwargs,
- }
- payload = {}
- for key, value in parameters.items():
- if value is not None:
- payload[key] = value
- response = self.post(json=payload, model=model, task="text-to-image")
- return _response_to_image(response)
-
- def text_to_speech(self, text: str, *, model: Optional[str] = None) -> bytes:
- """
- Synthesize an audio of a voice pronouncing a given text.
-
- Args:
- text (`str`):
- The text to synthesize.
- model (`str`, *optional*):
- The model to use for inference. Can be a model ID hosted on the Hugging Face Hub or a URL to a deployed
- Inference Endpoint. This parameter overrides the model defined at the instance level. Defaults to None.
-
- Returns:
- `bytes`: The generated audio.
-
- Raises:
- [`InferenceTimeoutError`]:
- If the model is unavailable or the request times out.
- `HTTPError`:
- If the request fails with an HTTP error status code other than HTTP 503.
-
- Example:
- ```py
- >>> from pathlib import Path
- >>> from huggingface_hub import InferenceClient
- >>> client = InferenceClient()
-
- >>> audio = client.text_to_speech("Hello world")
- >>> Path("hello_world.wav").write_bytes(audio)
- ```
- """
- response = self.post(json={"inputs": text}, model=model, task="text-to-speech")
- return response.content
-
- def _resolve_url(self, model: Optional[str] = None, task: Optional[str] = None) -> str:
- model = model or self.model
-
- # If model is already a URL, ignore `task` and return directly
- if model is not None and (model.startswith("http://") or model.startswith("https://")):
- return model
-
- # # If no model but task is set => fetch the recommended one for this task
- if model is None:
- if task is None:
- raise ValueError(
- "You must specify at least a model (repo_id or URL) or a task, either when instantiating"
- " `InferenceClient` or when making a request."
- )
- model = _get_recommended_model(task)
-
- # Compute InferenceAPI url
- return (
- # Feature-extraction and sentence-similarity are the only cases where we handle models with several tasks.
- f"{INFERENCE_ENDPOINT}/pipeline/{task}/{model}"
- if task in ("feature-extraction", "sentence-similarity")
- # Otherwise, we use the default endpoint
- else f"{INFERENCE_ENDPOINT}/models/{model}"
- )
-
-
-def _get_recommended_model(task: str) -> str:
- # TODO: load from a config file? (from the Hub?) Would make sense to make updates easier.
- if task in RECOMMENDED_MODELS:
- model = RECOMMENDED_MODELS[task]
- logger.info(
- f"Defaulting to recommended model {model} for task {task}. It is recommended to explicitly pass"
- f" `model='{model}'` as argument as we do not guarantee that the recommended model will stay the same over"
- " time."
- )
- return model
- raise NotImplementedError()
-
-
-@overload
-def _open_as_binary(content: ContentT) -> ContextManager[BinaryT]:
- ... # means "if input is not None, output is not None"
-
-
-@overload
-def _open_as_binary(content: Literal[None]) -> ContextManager[Literal[None]]:
- ... # means "if input is None, output is None"
-
-
-@contextmanager # type: ignore
-def _open_as_binary(content: Optional[ContentT]) -> Generator[Optional[BinaryT], None, None]:
- """Open `content` as a binary file, either from a URL, a local path, or raw bytes.
-
- Do nothing if `content` is None,
-
- TODO: handle a PIL.Image as input
- TODO: handle base64 as input
- """
- # If content is a string => must be either a URL or a path
- if isinstance(content, str):
- if content.startswith("https://") or content.startswith("http://"):
- logger.debug(f"Downloading content from {content}")
- yield get_session().get(content).content # TODO: retrieve as stream and pipe to post request ?
- return
- content = Path(content)
- if not content.exists():
- raise FileNotFoundError(
- f"File not found at {content}. If `data` is a string, it must either be a URL or a path to a local"
- " file. To pass raw content, please encode it as bytes first."
- )
-
- # If content is a Path => open it
- if isinstance(content, Path):
- logger.debug(f"Opening content from {content}")
- with content.open("rb") as f:
- yield f
- else:
- # Otherwise: already a file-like object or None
- yield content
-
-
-def _b64_encode(content: ContentT) -> str:
- """Encode a raw file (image, audio) into base64. Can be byes, an opened file, a path or a URL."""
- with _open_as_binary(content) as data:
- data_as_bytes = data if isinstance(data, bytes) else data.read()
- return base64.b64encode(data_as_bytes).decode()
-
-
-def _b64_to_image(encoded_image: str) -> "Image":
- """Parse a base64-encoded string into a PIL Image."""
- Image = _import_pil_image()
- return Image.open(io.BytesIO(base64.b64decode(encoded_image)))
-
-
-def _response_to_image(response: Response) -> "Image":
- """Parse a Response object into a PIL Image.
-
- Expects the response body to be raw bytes. To deal with b64 encoded images, use `_b64_to_image` instead.
- """
- Image = _import_pil_image()
- return Image.open(io.BytesIO(response.content))
-
-
-def _import_pil_image():
- """Make sure `PIL` is installed on the machine."""
- if not is_pillow_available():
- raise ImportError(
- "Please install Pillow to use deal with images (`pip install Pillow`). If you don't want the image to be"
- " post-processed, use `client.post(...)` and get the raw response from the server."
- )
- from PIL import Image
-
- return Image
-
-
-def _import_numpy():
- """Make sure `numpy` is installed on the machine."""
- if not is_numpy_available():
- raise ImportError("Please install numpy to use deal with embeddings (`pip install numpy`).")
- import numpy
-
- return numpy
diff --git a/spaces/leilevy/bingo/src/components/ui/dialog.tsx b/spaces/leilevy/bingo/src/components/ui/dialog.tsx
deleted file mode 100644
index 925e77fe7858fb218b5115b4e225174a886e0f02..0000000000000000000000000000000000000000
--- a/spaces/leilevy/bingo/src/components/ui/dialog.tsx
+++ /dev/null
@@ -1,128 +0,0 @@
-'use client'
-
-import * as React from 'react'
-import * as DialogPrimitive from '@radix-ui/react-dialog'
-
-import { cn } from '@/lib/utils'
-import { IconClose } from '@/components/ui/icons'
-
-const Dialog = DialogPrimitive.Root
-
-const DialogTrigger = DialogPrimitive.Trigger
-
-const DialogPortal = ({
- className,
- children,
- ...props
-}: DialogPrimitive.DialogPortalProps) => (
-
-
')
-
- with gr.Box():
- with gr.Column(scale=3):
- frame_processor_checkbox = gr.CheckboxGroup(
- choices = ['face_swapper', 'face_enhancer', 'frame_enhancer'],
- label = 'FRAME PROCESSORS',
- value = ['face_swapper'] # Default value
- )
-
-
- with gr.Box():
- with gr.Column(scale=3):
- face_analyser_direction_dropdown = gr.Dropdown(
- label = 'FACE ANALYSER DIRECTION',
- choices = ['left-right', 'right-left', 'top-bottom', 'bottom-top', 'small-large', 'large-small'],
- value = 'left-right'
- )
- face_analyser_age_dropdown = gr.Dropdown(
- label = 'FACE RECOGNITION',
- choices = ['none'] + ['reference', 'many'],
- value = 'reference'
- )
- face_analyser_gender_dropdown = gr.Dropdown(
- label = 'FACE ANALYSER GENDER',
- choices = ['none'] + ['male', 'female'],
- value = 'none'
- )
- unique_id = gr.Textbox(value=str(uuid.uuid4()), visible=False)
- with gr.Tab("Image: "):
- source_image = gr.Image(type="filepath", label="SOURCE IMAGE")
- target_image = gr.Image(type="filepath", label="TARGET IMAGE")
- image_button = gr.Button("START")
- clear_button = gr.ClearButton(value="CLEAR")
- image_output = gr.Image(label="OUTPUT")
- clear_button.add(image_output)
-
- image_button.click(
- run,
- inputs=[source_image, target_image, unique_id, frame_processor_checkbox, face_analyser_direction_dropdown, face_analyser_age_dropdown, face_analyser_gender_dropdown],
- outputs=image_output
- )
- clear_button.click(fn=clear_output, inputs=unique_id)
-
- with gr.Tab("Video: "):
- source_image_video = gr.Image(type="filepath", label="SOURCE IMAGE")
- target_video = gr.Video(label="TARGET VIDEO")
- with gr.Box():
- skip_audio = gr.Checkbox(label="SKIP AUDIO")
- keep_fps = gr.Checkbox(label="KEEP FPS")
- keep_temp = gr.Checkbox(label="KEEP TEMP")
- video_button = gr.Button("START")
- clear_video_button = gr.ClearButton(value="CLEAR")
- video_output = gr.Video(label="OUTPUT")
- clear_video_button.add(video_output)
- video_button.click(
- run,
- inputs=[source_image_video, target_video, unique_id, frame_processor_checkbox, face_analyser_direction_dropdown, face_analyser_age_dropdown, face_analyser_gender_dropdown, skip_audio, keep_fps, keep_temp],
- outputs=video_output
- )
- clear_video_button.click(fn=clear_output, inputs=unique_id)
-
-ui.launch(debug=True)
\ No newline at end of file
diff --git a/spaces/mehdidc/text_to_image_ddgan/score_sde/models/__init__.py b/spaces/mehdidc/text_to_image_ddgan/score_sde/models/__init__.py
deleted file mode 100644
index 9a198046280b17bea735155aff5f8d4a0ab6f4da..0000000000000000000000000000000000000000
--- a/spaces/mehdidc/text_to_image_ddgan/score_sde/models/__init__.py
+++ /dev/null
@@ -1,15 +0,0 @@
-# coding=utf-8
-# Copyright 2020 The Google Research Authors.
-#
-# Licensed under the Apache License, Version 2.0 (the "License");
-# you may not use this file except in compliance with the License.
-# You may obtain a copy of the License at
-#
-# http://www.apache.org/licenses/LICENSE-2.0
-#
-# Unless required by applicable law or agreed to in writing, software
-# distributed under the License is distributed on an "AS IS" BASIS,
-# WITHOUT WARRANTIES OR CONDITIONS OF ANY KIND, either express or implied.
-# See the License for the specific language governing permissions and
-# limitations under the License.
-
diff --git a/spaces/merle/PROTEIN_GENERATOR/utils/README.md b/spaces/merle/PROTEIN_GENERATOR/utils/README.md
deleted file mode 100644
index 06b2c403cbf5ef306c6400960bb39b2d9fa2ee63..0000000000000000000000000000000000000000
--- a/spaces/merle/PROTEIN_GENERATOR/utils/README.md
+++ /dev/null
@@ -1,132 +0,0 @@
----
-title: PROTEIN GENERATOR
-emoji: 🐨
-thumbnail: http://files.ipd.uw.edu/pub/sequence_diffusion/figs/diffusion_landscape.png
-colorFrom: blue
-colorTo: purple
-sdk: gradio
-sdk_version: 3.24.1
-app_file: app.py
-pinned: false
----
-
-
-
-## TLDR but I know how to use inpainting
-Submit jobs with all the same args as [inpainting](https://git.ipd.uw.edu/jwatson3/proteininpainting) plus some new ones
-- --T specify number of timesteps to use, good choices are 5,25,50,100 (try lower T first)
-- --save_best_plddt recommended arg to save best plddt str/seq in trajectory
-- --loop_design use when generating loops for binder design (or Ab loop design), will load finetuned checkpoint
-- --symmetry integer to divide the input up with for symmetric update (input of X will divide the sequence into X symmetric motifs)
-- --trb specify trb when partially diffusing to use same mask as the design, must match pdb input (contigs will not get used)
-- --sampling_temp fraction of diffusion trajectory to use for partial diffusion (default is 1.0 for fully diffused) values around 0.3 seem to give good diversity
-
-**environment to use**
-```
-source activate /software/conda/envs/SE3nv
-```
-**example command**
-```
-python inference.py --pdb examples/pdbs/rsv5_5tpn.pdb --out examples/out/design \
- --contigs 0-25,A163-181,25-30 --T 25 --save_best_plddt
-```
-For jobs with inputs <75 residues it is feesible to run on CPUs. It's helpful to redesign output backbones with [MPNN](https://git.ipd.uw.edu/justas/proteinmpnn) (not sure if useful yet when using --loop_design). Check back for more updates.
-
-## Getting started
-
-Check out the templates in the example folder to see how you can set up jobs for the various design strategies
-
-- [ ] [Motif or active-site scaffolding](examples/motif_scaffolding.sh)
-- [ ] [Partial diffusion (design diversification)](examples/partial_diffusion.sh)
-- [ ] [Anitbody / Loop design](examples/loop_design.sh)
-- [ ] [Symmetric design](examples/symmetric_design.sh)
-
-## Weighted Sequence design
-Biasing the sequence by weighting certain amino acid types is a nice way to control and guide the diffusion process, generate interesting folds, and repeat units. It is possible to combine this technique with motif scaffolding as well. here are a few different ways to set up sequence potentials:
-
-The --aa_spec argument used in combination with the --aa_weight allows you to specify the complete amino acid weighting pattern for a sequence. The pattern specified in aa_spec will be repeated along the entire length of the design.
- - --aa_spec base repeat unit to weight sequence with, X is used as a mask token, for example --aa_spec XXAXXLXX will generate solenoid folds like the one below
- - --aa_weight weights to assign for non masked residues in aa_spec, for example --aa_weight 2,2 will weight alanine to 2 and leucine to 2
-
-**Make solenoids with a little bias!**
-
-
-
-
-**example job set up for sequene weighting**
-```
-python inference.py \
- --num_designs 10 \
- --out examples/out/seq_bias \
- --contigs 100 --symmetry 5 \
- --T 25 --save_best_plddt \
- --aa_spec XXAXXLXX --aa_weight 2,2
-```
-
-In addition to the contigs above users can also use a disctionary to specify sequence weighting with [aa_weights](examples/aa_weights.json) for more generic uses. These weights can be specified with the --aa_weights_json arg and used in combination with the --add_weight_every_n arg or --frac_seq_to_weight arg. Each of these args defines where weights in the aa_weights dictionary will be applied to the sequence (you cannot specify both simultaneously). To add the weight every 5 residues use --add_weight_every_n 5. To add weight to a randomly sampled 40% of the sequence use --frac_seq_to_weight 0.4. If you add weight to multiple amino acid types in aa_weights, use the --one_weight_per_position flag to specify that a randomly sampled amino acid from aa_weight with a positive value should be chosen where the sequence bias is added. This allows the user to specify multiple amino acid types you want to upweight while ensuring to only bias for one type at each position, this usually is more effective.
-
-
-## Motif and active site scaffolding
-An example for motif scaffolding submission is written below, if you are inputing an active site with single residue inputs this can be specified in the contigs like 10-20,A10-10,20-30,A50-50,5-15 to scaffold just the 10th and 50th residues of chain A. Setting the model at higher T usually results in higher success rates, but it can still be useful to try problems out with just a few steps (T = 5, 15, or 25), before increasing the number of steps further. It is recommended to use [MPNN](https://git.ipd.uw.edu/justas/proteinmpnn) on the output backbones before alphafolding for validation.
-
-```
-python inference.py \
- --num_designs 10 \
- --out examples/out/design \
- --pdb examples/pdbs/rsv5_5tpn.pdb \
- --contigs 0-25,A163-181,25-30 --T 25 --save_best_plddt
-```
-
-## Partial diffusion
-To sample diverse and highquality desing fast, it can be useful to run many designs with T=5, and then after MPNN and alphafold filtering partially diffuse the successful designs to generate more diversity around designs that seem to be working. By using the --trb flag the script will enter partial diffusion mode. With the --T flag you can specify the total number of steps inthe trajectory and with the --sampling_temp flag you can determine how far into the trajectory the inputs will be diffused. Setting the sampling temp to 1.0 would be full diffused. In this mode the contigs will be ignored, and the mask used from the original design will be used.
-
-```
-python inference.py \
- --num_designs 10 \
- --pdb examples/out/design_000.pdb \
- --trb examples/out/design_000.trb \
- --out examples/out/partial_diffusion_design \
- --contigs 0 --sampling_temp 0.3 --T 50 --save_best_plddt
-```
-
-
-## Symmetric design
-In symmetric design mode, the --symmetry flag is used to specify the number of partitions to make from the input sequence length. Each partition will be updated symmetric according to the first in the sequence. This requires that your sequence length (L) is divisible by the symmetry input. Symmetric motif scaffolding should be possible with the right contigs, but has not been experimented with yet.
-
-```
-python inference.py \
- --num_designs 10 \
- --pdb examples/pdbs/rsv5_5tpn.pdb \
- --out examples/out/symmetric_design \
- --contigs 25,0 25,0 25,0 \
- --T 50 --save_best_plddt --symmetry 3
-```
-
-
-## Antibody and loop design
-Using the --loop_desing flag will load a version of the model finetuned on antibody CDR loops. This is useful if you are looking to design new CDR loops or are strcutred loops for binder design. It is helpful to run the designs with a target input too.
-
-```
-python inference.py \
- --num_designs 10 \
- --pdb examples/pdbs/G12D_manual_mut.pdb \
- --out examples/out/ab_loop \
- --contigs A2-176,0 C7-16,0 H2-95,12-15,H111-116,0 L1-45,10-12,L56-107 \
- --T 25 --save_best_plddt --loop_design
-```
-
-
-
-## About the model
-Sequence diffusion is trained on the same dataset and uses the same architecture as RoseTTAFold. To train the model, a ground truth sequence is transformed into an Lx20 continuous space and gaussian noise is added to diffuse the sequence to the sampled timestep. To condition on structure and sequence, the structre for a motif is given and then corresponding sequence is denoised in the input. The rest of the structure is blackhole initialized. For each example the model is trained to predict Xo and losses are applied on the structure and sequence respectively. During training big T is set to 1000 steps, and a square root schedule is used to add noise.
-
-
-
-
-## Looking ahead
-We are interested in problems where diffusing in sequence space is useful, if you would like to chat more or join in our effort for sequence diffusion come talk to Sidney or Jake!
-
-
-## Acknowledgements
-A project by Sidney Lisanza and Jake Gershon. Thanks to Sam Tipps for implementing symmetric sequence diffusion. Thank you to Minkyung Baek and Frank Dimaio for developing RoseTTAFold, Joe Watson and David Juergens for the developing inpainting inference script which the inference code is built on top of.
-
diff --git a/spaces/merve/anonymization/public/third_party/alea.js b/spaces/merve/anonymization/public/third_party/alea.js
deleted file mode 100644
index 9effe485ca14df5d6923e20adefaa794b939ee26..0000000000000000000000000000000000000000
--- a/spaces/merve/anonymization/public/third_party/alea.js
+++ /dev/null
@@ -1,3 +0,0 @@
-// https://github.com/davidbau/seedrandom Copyright 2019 David Bau
-
-!function(n,t,e){function u(n){var t=this,e=function(){var s=4022871197;return function(n){n=String(n);for(var t=0;t>>0,s=(e*=s)>>>0,s+=4294967296*(e-=s)}return 2.3283064365386963e-10*(s>>>0)}}();t.next=function(){var n=2091639*t.s0+2.3283064365386963e-10*t.c;return t.s0=t.s1,t.s1=t.s2,t.s2=n-(t.c=0|n)},t.c=1,t.s0=e(" "),t.s1=e(" "),t.s2=e(" "),t.s0-=e(n),t.s0<0&&(t.s0+=1),t.s1-=e(n),t.s1<0&&(t.s1+=1),t.s2-=e(n),t.s2<0&&(t.s2+=1),e=null}function o(n,t){return t.c=n.c,t.s0=n.s0,t.s1=n.s1,t.s2=n.s2,t}function s(n,t){var e=new u(n),s=t&&t.state,r=e.next;return r.int32=function(){return 4294967296*e.next()|0},r.double=function(){return r()+11102230246251565e-32*(2097152*r()|0)},r.quick=r,s&&("object"==typeof s&&o(s,e),r.state=function(){return o(e,{})}),r}t&&t.exports?t.exports=s:e&&e.amd?e(function(){return s}):this.alea=s}(0,"object"==typeof module&&module,"function"==typeof define&&define);
\ No newline at end of file
diff --git a/spaces/merve/hidden-bias/source/measuring-fairness/annotations.js b/spaces/merve/hidden-bias/source/measuring-fairness/annotations.js
deleted file mode 100644
index 7ab68f297f98c655427a84de22388906182b240c..0000000000000000000000000000000000000000
--- a/spaces/merve/hidden-bias/source/measuring-fairness/annotations.js
+++ /dev/null
@@ -1,52 +0,0 @@
-/* Copyright 2020 Google LLC. All Rights Reserved.
-
-Licensed under the Apache License, Version 2.0 (the "License");
-you may not use this file except in compliance with the License.
-You may obtain a copy of the License at
-
- http://www.apache.org/licenses/LICENSE-2.0
-
-Unless required by applicable law or agreed to in writing, software
-distributed under the License is distributed on an "AS IS" BASIS,
-WITHOUT WARRANTIES OR CONDITIONS OF ANY KIND, either express or implied.
-See the License for the specific language governing permissions and
-limitations under the License.
-==============================================================================*/
-
-
-
-var annotations =
-[
-]
-
-
-function addSwoop(c){
- var swoopy = d3.swoopyDrag()
- .x(d => c.x(d.x))
- .y(d => c.y(d.y))
- .draggable(0)
- .annotations(annotations)
-
- var swoopySel = c.svg.append('g.annotations').call(swoopy)
-
- c.svg.append('marker#arrow')
- .attr('viewBox', '-10 -10 20 20')
- .attr('markerWidth', 20)
- .attr('markerHeight', 20)
- .attr('orient', 'auto')
- .append('path').at({d: 'M-6.75,-6.75 L 0,0 L -6.75,6.75'})
-
-
- swoopySel.selectAll('path').attr('marker-end', 'url(#arrow)')
- window.annotationSel = swoopySel.selectAll('g')
- .st({fontSize: 12, opacity: d => d.slide == 0 ? 1 : 0})
-
- swoopySel.selectAll('text')
- .each(function(d){
- d3.select(this)
- .text('') //clear existing text
- .tspans(d3.wordwrap(d.text, d.width || 20), 12) //wrap after 20 char
- })
-}
-
-
diff --git a/spaces/merve/measuring-fairness/public/anonymization/index.html b/spaces/merve/measuring-fairness/public/anonymization/index.html
deleted file mode 100644
index 34d2dfcaa3f70017b2c9852587b87d532c8774b2..0000000000000000000000000000000000000000
--- a/spaces/merve/measuring-fairness/public/anonymization/index.html
+++ /dev/null
@@ -1,268 +0,0 @@
-
-
-
-
-
-
-
-
-
-
-
-
-
-
-
-
-
- How randomized response can help collect sensitive information responsibly
-
-
-
-
-
-
-
-
-
-
-
-
-
-
-
-
How randomized response can help collect sensitive information responsibly
-
Giant datasets are revealing new patterns in cancer, income inequality and other important areas. However, the widespread availability of fast computers that can cross reference public data is making it harder to collect private information without inadvertently violating people's privacy. Modern randomization techniques can help preserve anonymity.
-
-
-
-
-
-
-
-
-
Anonymous Data
-
-
Let's pretend we're analysts at a small college, looking at anonymous survey data about plagiarism.
-
-
We've gotten responses from the entire student body, reporting if they've ever plagiarized or not. To encourage them to respond honestly, names were not collected.
-
-
-
The data here has been randomly generated
-
-
-
-
-
On the survey students also report several bits of information about themselves, like their age...
-
-
-
-
-
...and what state they're from.
-
-
This additional information is critical to finding potential patterns in the data—why have so many first-years from New Hampshire plagiarized?
-
-
-
-
-
Revealed Information
-
But granular information comes with a cost.
-
-
One student has a unique age/home state combination. By searching another student database for a 19-year old from Vermont we can identify one of the plagiarists from supposedly anonymous survey data.
-
-
-
-
-
Increasing granularity exacerbates the problem. If the students reported slightly more about their ages by including what season they were born in, we'd be able to identify about a sixth of them.
-
-
With the spread of large datasets, it is increasingly difficult to release detailed information without inadvertently revealing someone's identity. A week of a person's location data could reveal a home and work address—possibly enough to find a name using public records.
-
-
-
-
-
Randomization
-
One solution is to randomize responses so each student has plausible deniability. This lets us buy privacy at the cost of some uncertainty in our estimation of plagiarism rates.
-
-
Step 1: Each student flips a coin and looks at it without showing anyone.
-
-
-
-
-
Step 2: Students who flip heads report plagiarism, even if they haven't plagiarized.
-
-
Students that flipped tails report the truth, secure with the knowledge that even if their response is linked back to their name, they can claim they flipped heads.
-
-
-
-
-
With a little bit of math, we can approximate the rate of plagiarism from these randomized responses. We'll skip the algebra, but doubling the reported non-plagiarism rate gives a good estimate of the actual non-plagiarism rate.
-
-
-
-
-
-Flip coins
-
-
-
-
-
-
-
-
How far off can we be?
-
-
If we simulate this coin flipping lots of times, we can see the distribution of errors.
-
-
The estimates are close most of the time, but errors can be quite large.
-
-
-
-Flip coins 200 times
-
-
-
-
-
-
-
-
Reducing the random noise (by reducing the number of students who flip heads) increases the accuracy of our estimate, but risks leaking information about students.
-
-
If the coin is heavily weighted towards tails, identified students can't credibly claim they reported plagiarizing because they flipped heads.
-
-
-
-
-
-
-
-
-
-
-
One surprising way out of this accuracy-privacy tradeoff: carefully collect information from even more people.
-
-
If we got students from other schools to fill out this survey, we could accurately measure plagiarism while protecting everyone's privacy. With enough students, we could even start comparing plagiarism across different age groups again—safely this time.
-
-
-
-
-
-
-
-
-
-
-
-
-
-
Conclusion
-
-
Aggregate statistics about private information are valuable, but can be risky to collect. We want researchers to be able to study things like the connection between demographics and health outcomes without revealing our entire medical history to our neighbors. The coin flipping technique in this article, called randomized response, makes it possible to safely study private information.
-
-
You might wonder if coin flipping is the only way to do this. It's not—differential privacy can add targeted bits of random noise to a dataset and guarantee privacy. More flexible than randomized response, the 2020 Census will use it to protect respondents' privacy. In addition to randomizing responses, differential privacy also limits the impact any one response can have on the released data.
-
-
-
Credits
-
-
Adam Pearce and Ellen Jiang // September 2020
-
-
Thanks to Carey Radebaugh, Fernanda Viégas, Emily Reif, Hal Abelson, Jess Holbrook, Kristen Olson, Mahima Pushkarna, Martin Wattenberg, Michael Terry, Miguel Guevara, Rebecca Salois, Yannick Assogba, Zan Armstrong and our other colleagues at Google for their help with this piece.
-
-
-
-
-
'
- );
- });
- }
-
- function drawConclusionSummary() {
- classifierSel = d3
- .select(".conclusion-summary")
- .html(summaries["conclusion"]);
- classifierSel.classed("summary-text is-classified", true);
- }
-
- function drawDropdown() {
- var sel = d3.select("#conclusion-select-category").html("");
- sel.classed("dropdown", true);
- sel.appendMany("option", conclusionOptions.category)
- .at({
- value: function (d) {
- return d.value;
- },
- })
- .text((d) => d.label);
- // sel.attr('select', 'circles, triangles, and rectangles');
- sel.on("change", function (d) {
- makeConclusionUpdates();
- });
- }
-
- function makeConclusionUpdates() {
- updateResults();
- drawNewspapers();
- drawInterface();
- drawConclusionSummary();
- }
- drawDropdown();
- makeConclusionUpdates();
-}
-
-// Handle the parameters everywhere classifiers are drawn
-var classifiers = [
- {
- // Just the initial display of shapes, not interactive
- class: "show-shapes",
- colorBy: (d) => d.correctness,
- isClassified: false,
- isRounding: false,
- usingLabel: "none",
- },
- {
- class: "default-classifier",
- colorBy: (d) => d.correctness,
- isClassified: false,
- isRounding: false,
- usingLabel: "none",
- },
- {
- class: "second-classifier",
- colorBy: (d) => d.correctness,
- isClassified: false,
- isRounding: true,
- usingLabel: "shape_name",
- options: {
- rounding: [
- { label: "with their best guess", value: true },
- { label: 'as "other"', value: false },
- ],
- },
- },
- {
- class: "final-classifier",
- colorBy: (d) => d.correctness,
- isClassified: false,
- isRounding: true,
- usingLabel: "shape_name",
- options: {
- rounding: [
- { label: "with our best guess", value: true },
- { label: 'as "other"', value: false },
- ],
- category: [
- {
- label: "circles, triangles, or rectangles",
- value: "shape_name",
- },
- { label: "pointy shapes or round shapes", value: "pointiness" },
- { label: "small shapes or big shapes", value: "size" },
- { label: "just shapes", value: "none" },
- ],
- },
- },
-];
-
-// "Labels Tell Stories" dropdown options
-var conclusionOptions = {
- category: [
- { label: "circles, triangles, and rectangles", value: "shape_name" },
- { label: "pointy shapes and round shapes", value: "pointiness" },
- { label: "small shapes and big shapes", value: "size" },
- ],
-};
-
-classifiers.forEach(drawShapesWithData);
-drawConclusion();
-
-// These images are loaded invisibly so they appear seamlessly on dropdown change
-const preloadImages = [
- "img/confusing_pointiness.png",
- "img/confusing_pointiness.svg",
- "img/confusing_shape_name.png",
- "img/confusing_shape_name.svg",
- "img/confusing_size.png",
- "img/confusing_size.svg",
- "img/interface_default.png",
- "img/interface_default.svg",
- "img/interface_shape_name_false.png",
- "img/interface_shape_name_false.svg",
- "img/interface_shape_name_true.png",
- "img/interface_shape_name_true.svg",
- "img/newspapers_pointiness.png",
- "img/newspapers_pointiness.svg",
- "img/newspapers_shape_name.png",
- "img/newspapers_shape_name.svg",
- "img/newspapers_size.png",
- "img/newspapers_size.svg",
-];
-
-d3.select(".preload-dropdown-img")
- .html("")
- .appendMany("img", preloadImages)
- .at({ src: (d) => d });
diff --git a/spaces/merve/uncertainty-calibration/public/measuring-diversity/sliders.js b/spaces/merve/uncertainty-calibration/public/measuring-diversity/sliders.js
deleted file mode 100644
index 13b03fa080fe5d1c2db81ef456242c0d856b0a0f..0000000000000000000000000000000000000000
--- a/spaces/merve/uncertainty-calibration/public/measuring-diversity/sliders.js
+++ /dev/null
@@ -1,206 +0,0 @@
-window.highlightColor = '#bf0bbf'
-
-window.makeSliders = function(metrics, sets, c, selectSet, drawRow, onRender){
-
- var width = 180
- var height = 30
- var color = '#000'
-
- var xScale = d3.scaleLinear().range([0, width]).domain([0, 1])
- .clamp(1)
-
- var sliderSel = c.svg.appendMany('g', metrics)
- .translate((d, i) => [-c.margin.left -10 , 130*i + 30])
- .on('click', function(d){
- d.target = xScale.invert(d3.mouse(this)[0])
- render()
- })
- .classed('slider', true)
- .st({cursor: 'pointer'})
-
- var textSel = sliderSel.append('text.slider-label-container')
- .at({y: -20, fontWeight: 500, textAnchor: 'middle', x: 180/2})
-
- sliderSel.append('rect')
- .at({width, height, y: -height/2, fill: 'rgba(0,0,0,0)'})
-
- sliderSel.append('path').at({
- d: `M 0 -.5 H ${width}`,
- stroke: color,
- strokeWidth: 1
- })
-
- var leftPathSel = sliderSel.append('path').at({
- d: `M 0 -.5 H ${width}`,
- stroke: color,
- strokeWidth: 3
- })
-
- var drag = d3.drag()
- .on('drag', function(d){
- var x = d3.mouse(this)[0]
- d.target = xScale.invert(x)
- render()
- })
-
- var circleSel = sliderSel.append('circle').call(drag)
- .at({r: 7, stroke: '#000'})
-
-
- var exSel = c.svg.append('g').translate([-c.margin.left -10, 400])
- .st({fontSize: 13})
-
- var curY = 0
- exSel.append('g')
- .append('text').text('The selected set is...')
-
- var selectedSetG = exSel.append('g.selected').translate([-10, curY += 15])
- .datum(sets[0])
- .call(drawRow)
-
- selectedSetG.select('.no-stroke').classed('selected', 1)
-
- curY += 25
- var exMetrics = exSel.appendMany('g', metrics)
- .translate(() => curY +=22, 1)
- .append('text').html(d => '10% small, 10% more than target')
-
- curY += 10
- var exMeanDiff = exSel.append('text').translate(() => curY +=22, 1)
- .at({textAnchor: 'end', x: 190})
- var exMaxDiff = exSel.append('text').translate(() => curY +=22, 1)
- .at({textAnchor: 'end', x: 190})
-
-
- // Make histogram data
- sliderSel.each(function(metric){
- var countKey = metric.key + '_count'
- sets.forEach(set => {
- var v = d3.sum(set, d => d[metric.field] == metric.key)
- set[countKey] = v / set.length
- })
-
- var byCountKey = d3.nestBy(sets, d => d[countKey])
-
- d3.range(.1, 1, .1).forEach(i => {
- if (byCountKey.some(d => d.key*100 == Math.round(i*100))) return
-
- var rv = []
- rv.key = i
- byCountKey.push(rv)
- })
-
- byCountKey.forEach(d => {
- d.metric = metric
- d.key = +d.key
- })
-
- var countSel = d3.select(this).append('g.histogram').lower()
- .translate(30, 1)
- .appendMany('g', byCountKey)
- .translate(d => xScale.clamp(0)(d.key - .05), 0)
- xScale.clamp(1)
-
- countSel.append('text')
- // .text(d => '10')
- .at({fontSize: 11, opacity: .7, y: -8, textAnchor: 'middle', x: 9.5})
- .text(d => d.key*100)
-
- countSel.append('path')
- .at({d: 'M 9.5 -18 V -30', stroke: '#ccc'})
-
- countSel
- .appendMany('rect.histogram-set', d => d)
- .at({width: 16, height: 4, x: 1.5, y: (d, i) => i*6})
- // .on('mouseover', selectSet)
- })
- var histogramSetSel = sliderSel.selectAll('rect.histogram-set')
- .st({cursor: 'default'})
-
- var axisSel = sliderSel.selectAll('.histogram text')
-
-
- var pinkSel = sliderSel.append('g')
- .at({r: 4, fill: highlightColor})
- .st({pointerEvents: 'none', opacity:0})
- pinkSel.append('path').at({stroke: highlightColor, d: 'M .5 0 V 15'})
- pinkSel.append('text').at({y: 30, textAnchor: 'middle'})
- pinkSel.append('text.score').at({y: 50, textAnchor: 'middle'})
-
-
- function render(){
- circleSel.at({cx: d => xScale(d.target)})
- // circleSel.at({cx: d => xScale(d.target)})
- textSel.text(d => (d.str + ' Target: ').replace('s ', ' ') + pctFmt(d.target))
-
- axisSel
- .classed('selected', false)
- // .text(function(d){
- // var str = Math.round(100*Math.abs(d.key - d.metric.target))
-
- // if (d.some(e => e.selected)){
- // d3.select(this).classed('selected', 1)
- // // str = str + '%'
- // }
-
- // return str
- // })
-
- leftPathSel.at({d: d => `M 0 -.5 H ${xScale(d.target)}`})
- metrics.forEach(d => {
- d.scoreScale = d3.scaleLinear()
- .domain([-.1, d.target, 1.1])
- .range([0, 1, 0])
- })
- histogramSetSel.st({fill: d => d === sets.selected ? highlightColor: '#bbb'})
-
- if (onRender) onRender()
-
- var shapes = sets.selected
-
- var metricVals = metrics.map(m => {
- return d3.sum(shapes, (d, i) => shapes[i][m.field] == m.key)/shapes.length
- })
-
- pinkSel.translate((d, i) => xScale(metricVals[i]), 0)
- pinkSel.select('text').text((d, i) => pctFmt(metricVals[i]))
- pinkSel.select('.score').text((d, i) => 'Difference: ' + Math.round(shapes.score[i]*100))
-
-
- selectedSetG.html('')
- .datum(sets.selected)
- .call(drawRow)
-
- selectedSetG.select('.no-stroke').classed('selected', 1)
-
- exMetrics
- .html((d, i) => {
- var target = d.target
- var actual = sets.selected[d.key + '_count']
- var diff = sets.selected.score[i]
-
- var str = d.str.replace('ls', 'l').replace('ns', 'n').toLowerCase()
-
- return `
- ${pctFmt(actual)}
- ${str},
- ${pctFmt(diff)}
- ${actual < target ? 'less' : 'more'} than target
- `
- })
- .at({textAnchor: 'end', x: 190})
-
- exMeanDiff
- .text('Mean Difference: ' + d3.format('.2%')(sets.selected['Utilitarian']/100))
-
- exMaxDiff
- .text('Max Difference: ' + measures[1].ppFn(sets.selected['score']).replace('%', '.00%'))
-
- }
-
- return {render}
-}
-
-
-// window.initColumns('#columns-height', metrics1, measures)
-// window.initColumns('#columns-height-disagree', metrics2, measures2)
diff --git a/spaces/mikeee/multilingual-dokugpt/README.md b/spaces/mikeee/multilingual-dokugpt/README.md
deleted file mode 100644
index a8b439648d348eabab4d43aad9ebe6694a14a174..0000000000000000000000000000000000000000
--- a/spaces/mikeee/multilingual-dokugpt/README.md
+++ /dev/null
@@ -1,13 +0,0 @@
----
-title: multilingual-dokugpt
-emoji: 🦀
-colorFrom: green
-colorTo: red
-sdk: gradio
-sdk_version: 3.33.1
-app_file: main.py
-pinned: false
-license: mit
----
-
-[Github Repo README.md](https://github.com/ffreemt/multilingual-dokugpt/blob/main/README1.md)
diff --git a/spaces/mikeee/radiobee-dev/tests/test_lists2cmat_hlm.py b/spaces/mikeee/radiobee-dev/tests/test_lists2cmat_hlm.py
deleted file mode 100644
index db7c21f7dc65b27a6514b0085d9bc4b40deed85b..0000000000000000000000000000000000000000
--- a/spaces/mikeee/radiobee-dev/tests/test_lists2cmat_hlm.py
+++ /dev/null
@@ -1,45 +0,0 @@
-"""Test lists2cmat."""
-# pylint: disable=invalid-name
-
-from itertools import zip_longest
-from fastlid import fastlid
-from radiobee.loadtext import loadtext
-from radiobee.lists2cmat import lists2cmat
-
-file1 = "data/test_en.txt"
-file2 = "data/test_zh.txt"
-file1 = "data/hlm-ch1-en.txt"
-file2 = "data/hlm-ch1-zh.txt"
-
-# assume English or Chinese
-fastlid.set_languages = ["en", "zh", ]
-
-text1 = loadtext(file1)
-text2 = loadtext(file2)
-
-lang1, _ = fastlid(text1)
-lang2, _ = fastlid(text2)
-
-
-def test_lists2cmat_hlm():
- """Test lists2cmat."""
-
- lst1, lst2 = [], []
-
- if text1:
- lst1 = [_.strip() for _ in text1.splitlines() if _.strip()]
- if text2:
- lst2 = [_.strip() for _ in text2.splitlines() if _.strip()]
-
- # en zh
- len(lst1) == 135, len(lst2) == 55
-
- # cmat = texts2cmat(lst1, lst2, lang1, lang2)
- cmat = lists2cmat(lst1, lst2, lang1, lang2)
-
- assert cmat.shape == (55, 135)
-
- cmat21 = lists2cmat(lst2, lst1, lang2, lang1)
-
- assert cmat21.shape == (135, 55)
- assert lists2cmat(lst2, lst1).mean() > 0.05 # 0.09
diff --git a/spaces/mingyuan/ReMoDiffuse/mogen/datasets/pipelines/formatting.py b/spaces/mingyuan/ReMoDiffuse/mogen/datasets/pipelines/formatting.py
deleted file mode 100644
index 893ea99feac0226356c0f783b1abb8641851ed13..0000000000000000000000000000000000000000
--- a/spaces/mingyuan/ReMoDiffuse/mogen/datasets/pipelines/formatting.py
+++ /dev/null
@@ -1,134 +0,0 @@
-from collections.abc import Sequence
-
-import mmcv
-import numpy as np
-import torch
-from mmcv.parallel import DataContainer as DC
-from PIL import Image
-
-from ..builder import PIPELINES
-
-
-def to_tensor(data):
- """Convert objects of various python types to :obj:`torch.Tensor`.
-
- Supported types are: :class:`numpy.ndarray`, :class:`torch.Tensor`,
- :class:`Sequence`, :class:`int` and :class:`float`.
- """
- if isinstance(data, torch.Tensor):
- return data
- elif isinstance(data, np.ndarray):
- return torch.from_numpy(data)
- elif isinstance(data, Sequence) and not mmcv.is_str(data):
- return torch.tensor(data)
- elif isinstance(data, int):
- return torch.LongTensor([data])
- elif isinstance(data, float):
- return torch.FloatTensor([data])
- else:
- raise TypeError(
- f'Type {type(data)} cannot be converted to tensor.'
- 'Supported types are: `numpy.ndarray`, `torch.Tensor`, '
- '`Sequence`, `int` and `float`')
-
-
-@PIPELINES.register_module()
-class ToTensor(object):
-
- def __init__(self, keys):
- self.keys = keys
-
- def __call__(self, results):
- for key in self.keys:
- results[key] = to_tensor(results[key])
- return results
-
- def __repr__(self):
- return self.__class__.__name__ + f'(keys={self.keys})'
-
-
-@PIPELINES.register_module()
-class Transpose(object):
-
- def __init__(self, keys, order):
- self.keys = keys
- self.order = order
-
- def __call__(self, results):
- for key in self.keys:
- results[key] = results[key].transpose(self.order)
- return results
-
- def __repr__(self):
- return self.__class__.__name__ + \
- f'(keys={self.keys}, order={self.order})'
-
-
-@PIPELINES.register_module()
-class Collect(object):
- """Collect data from the loader relevant to the specific task.
-
- This is usually the last stage of the data loader pipeline.
-
- Args:
- keys (Sequence[str]): Keys of results to be collected in ``data``.
- meta_keys (Sequence[str], optional): Meta keys to be converted to
- ``mmcv.DataContainer`` and collected in ``data[motion_metas]``.
- Default: ``('filename', 'ori_filename', 'ori_shape', 'motion_shape', 'motion_mask')``
-
- Returns:
- dict: The result dict contains the following keys
- - keys in``self.keys``
- - ``motion_metas`` if available
- """
-
- def __init__(self,
- keys,
- meta_keys=('filename', 'ori_filename', 'ori_shape', 'motion_shape', 'motion_mask')):
- self.keys = keys
- self.meta_keys = meta_keys
-
- def __call__(self, results):
- data = {}
- motion_meta = {}
- for key in self.meta_keys:
- if key in results:
- motion_meta[key] = results[key]
- data['motion_metas'] = DC(motion_meta, cpu_only=True)
- for key in self.keys:
- data[key] = results[key]
- return data
-
- def __repr__(self):
- return self.__class__.__name__ + \
- f'(keys={self.keys}, meta_keys={self.meta_keys})'
-
-
-@PIPELINES.register_module()
-class WrapFieldsToLists(object):
- """Wrap fields of the data dictionary into lists for evaluation.
-
- This class can be used as a last step of a test or validation
- pipeline for single image evaluation or inference.
-
- Example:
- >>> test_pipeline = [
- >>> dict(type='LoadImageFromFile'),
- >>> dict(type='Normalize',
- mean=[123.675, 116.28, 103.53],
- std=[58.395, 57.12, 57.375],
- to_rgb=True),
- >>> dict(type='ImageToTensor', keys=['img']),
- >>> dict(type='Collect', keys=['img']),
- >>> dict(type='WrapIntoLists')
- >>> ]
- """
-
- def __call__(self, results):
- # Wrap dict fields into lists
- for key, val in results.items():
- results[key] = [val]
- return results
-
- def __repr__(self):
- return f'{self.__class__.__name__}()'
\ No newline at end of file
diff --git a/spaces/mjaramillo/SpiceIcaroTP/README.md b/spaces/mjaramillo/SpiceIcaroTP/README.md
deleted file mode 100644
index 02fa2b67320c93fa52178d57cb9d596ebb39535a..0000000000000000000000000000000000000000
--- a/spaces/mjaramillo/SpiceIcaroTP/README.md
+++ /dev/null
@@ -1,13 +0,0 @@
----
-title: SpiceIcaroTP
-emoji: 🌍
-colorFrom: pink
-colorTo: gray
-sdk: gradio
-sdk_version: 2.9.4
-app_file: app_deploy.py
-pinned: false
-license: mit
----
-
-Check out the configuration reference at https://huggingface.co/docs/hub/spaces#reference
diff --git a/spaces/ml-energy/leaderboard/spitfight/colosseum/controller/__init__.py b/spaces/ml-energy/leaderboard/spitfight/colosseum/controller/__init__.py
deleted file mode 100644
index e69de29bb2d1d6434b8b29ae775ad8c2e48c5391..0000000000000000000000000000000000000000
diff --git a/spaces/monra/freegpt-webui/g4f/Provider/Providers/Weuseing.py b/spaces/monra/freegpt-webui/g4f/Provider/Providers/Weuseing.py
deleted file mode 100644
index ba79e8b9c2573418720495a20d4c1c8d5a6ca7e9..0000000000000000000000000000000000000000
--- a/spaces/monra/freegpt-webui/g4f/Provider/Providers/Weuseing.py
+++ /dev/null
@@ -1,29 +0,0 @@
-import requests
-import os
-import json
-from ...typing import sha256, Dict, get_type_hints
-
-url = 'https://api.gptplus.one'
-model = ['gpt-3.5-turbo', 'gpt-3.5-turbo-16k', 'gpt-3.5-turbo-16k-0613', 'gpt-3.5-turbo-0613']
-supports_stream = True
-needs_auth = False
-
-def _create_completion(model: str, messages: list, stream: bool, temperature: float = 0.7, **kwargs):
- headers = {
- 'Content-Type': 'application/json',
- 'Accept': '*/*',
- 'Accept-Language': 'ru-RU,ru;q=0.9,en-US;q=0.8,en;q=0.7,ja;q=0.6,zh-TW;q=0.5,zh;q=0.4',
- }
- data = {
- 'messages': messages,
- 'model': model,
- }
- response = requests.post('https://api.gptplus.one/chat-process', json=data, stream=True)
- print(response)
-
- for token in response.iter_content(chunk_size=None):
- yield (token.decode('utf-8'))
-
-
-params = f'g4f.Providers.{os.path.basename(__file__)[:-3]} supports: ' + \
- '(%s)' % ', '.join([f"{name}: {get_type_hints(_create_completion)[name].__name__}" for name in _create_completion.__code__.co_varnames[:_create_completion.__code__.co_argcount]])
diff --git a/spaces/monra/freegpt-webui/g4f/active_providers.py b/spaces/monra/freegpt-webui/g4f/active_providers.py
deleted file mode 100644
index cc3857dbaf1a9020fde2c72d52c490b23f678dc0..0000000000000000000000000000000000000000
--- a/spaces/monra/freegpt-webui/g4f/active_providers.py
+++ /dev/null
@@ -1,124 +0,0 @@
-import uuid
-import g4f
-from g4f import ChatCompletion
-
-TEST_PROMPT = "Generate a sentence with 'ocean'"
-EXPECTED_RESPONSE_CONTAINS = "ocean"
-
-
-class Provider:
- def __init__(self, name, models):
- """
- Initialize the provider with its name and models.
- """
- self.name = name
- self.models = models if isinstance(models, list) else [models]
-
- def __str__(self):
- return self.name
-
-
-class ModelProviderManager:
- def __init__(self):
- """
- Initialize the manager that manages the working (active) providers for each model.
- """
- self._working_model_providers = {}
-
- def add_provider(self, model, provider_name):
- """
- Add a provider to the working provider list of the specified model.
- """
- if model not in self._working_model_providers:
- self._working_model_providers[model] = []
- self._working_model_providers[model].append(provider_name)
-
- def get_working_providers(self):
- """
- Return the currently active providers for each model.
- """
- return self._working_model_providers
-
-
-def _fetch_providers_having_models():
- """
- Get providers that have models from g4f.Providers.
- """
- model_providers = []
-
- for provider_name in dir(g4f.Provider):
- provider = getattr(g4f.Provider, provider_name)
-
- if _is_provider_applicable(provider):
- model_providers.append(Provider(provider_name, provider.model))
-
- return model_providers
-
-
-def _is_provider_applicable(provider):
- """
- Check if the provider has a model and doesn't require authentication.
- """
- return (hasattr(provider, 'model') and
- hasattr(provider, '_create_completion') and
- hasattr(provider, 'needs_auth') and
- not provider.needs_auth)
-
-
-def _generate_test_messages():
- """
- Generate messages for testing.
- """
- return [{"role": "system", "content": "You are a trained AI assistant."},
- {"role": "user", "content": TEST_PROMPT}]
-
-
-def _manage_chat_completion(manager, model_providers, test_messages):
- """
- Generate chat completion for each provider's models and handle positive and negative results.
- """
- for provider in model_providers:
- for model in provider.models:
- try:
- response = _generate_chat_response(
- provider.name, model, test_messages)
- if EXPECTED_RESPONSE_CONTAINS in response.lower():
- _print_success_response(provider, model)
- manager.add_provider(model, provider.name)
- else:
- raise Exception(f"Unexpected response: {response}")
- except Exception as error:
- _print_error_response(provider, model, error)
-
-
-def _generate_chat_response(provider_name, model, test_messages):
- """
- Generate a chat response given a provider name, a model, and test messages.
- """
- return ChatCompletion.create(
- model=model,
- messages=test_messages,
- chatId=str(uuid.uuid4()),
- provider=getattr(g4f.Provider, provider_name)
- )
-
-
-def _print_success_response(provider, model):
- print(f"\u2705 [{provider}] - [{model}]: Success")
-
-
-def _print_error_response(provider, model, error):
- print(f"\u26D4 [{provider}] - [{model}]: Error - {str(error)}")
-
-
-def get_active_model_providers():
- """
- Get providers that are currently working (active).
- """
- model_providers = _fetch_providers_having_models()
- test_messages = _generate_test_messages()
- manager = ModelProviderManager()
-
- _manage_chat_completion(manager, model_providers, test_messages)
-
- return manager.get_working_providers()
diff --git a/spaces/msmilauer/AutoGPT-duplicated2/autogpt/agent/agent_manager.py b/spaces/msmilauer/AutoGPT-duplicated2/autogpt/agent/agent_manager.py
deleted file mode 100644
index 898767a485e50b5e62625a7883edf1b30d5fddf9..0000000000000000000000000000000000000000
--- a/spaces/msmilauer/AutoGPT-duplicated2/autogpt/agent/agent_manager.py
+++ /dev/null
@@ -1,103 +0,0 @@
-"""Agent manager for managing GPT agents"""
-from __future__ import annotations
-
-from typing import Union
-
-from autogpt.config.config import Singleton
-from autogpt.llm_utils import create_chat_completion
-
-
-class AgentManager(metaclass=Singleton):
- """Agent manager for managing GPT agents"""
-
- def __init__(self):
- self.next_key = 0
- self.agents = {} # key, (task, full_message_history, model)
-
- # Create new GPT agent
- # TODO: Centralise use of create_chat_completion() to globally enforce token limit
-
- def create_agent(self, task: str, prompt: str, model: str) -> tuple[int, str]:
- """Create a new agent and return its key
-
- Args:
- task: The task to perform
- prompt: The prompt to use
- model: The model to use
-
- Returns:
- The key of the new agent
- """
- messages = [
- {"role": "user", "content": prompt},
- ]
-
- # Start GPT instance
- agent_reply = create_chat_completion(
- model=model,
- messages=messages,
- )
-
- # Update full message history
- messages.append({"role": "assistant", "content": agent_reply})
-
- key = self.next_key
- # This is done instead of len(agents) to make keys unique even if agents
- # are deleted
- self.next_key += 1
-
- self.agents[key] = (task, messages, model)
-
- return key, agent_reply
-
- def message_agent(self, key: str | int, message: str) -> str:
- """Send a message to an agent and return its response
-
- Args:
- key: The key of the agent to message
- message: The message to send to the agent
-
- Returns:
- The agent's response
- """
- task, messages, model = self.agents[int(key)]
-
- # Add user message to message history before sending to agent
- messages.append({"role": "user", "content": message})
-
- # Start GPT instance
- agent_reply = create_chat_completion(
- model=model,
- messages=messages,
- )
-
- # Update full message history
- messages.append({"role": "assistant", "content": agent_reply})
-
- return agent_reply
-
- def list_agents(self) -> list[tuple[str | int, str]]:
- """Return a list of all agents
-
- Returns:
- A list of tuples of the form (key, task)
- """
-
- # Return a list of agent keys and their tasks
- return [(key, task) for key, (task, _, _) in self.agents.items()]
-
- def delete_agent(self, key: Union[str, int]) -> bool:
- """Delete an agent from the agent manager
-
- Args:
- key: The key of the agent to delete
-
- Returns:
- True if successful, False otherwise
- """
-
- try:
- del self.agents[int(key)]
- return True
- except KeyError:
- return False
diff --git a/spaces/mygyasir/Real-Time-Voice-Cloning/synthesizer/models/tacotron.py b/spaces/mygyasir/Real-Time-Voice-Cloning/synthesizer/models/tacotron.py
deleted file mode 100644
index 769f7f98b79100ff587af3609010dd55e3b2a146..0000000000000000000000000000000000000000
--- a/spaces/mygyasir/Real-Time-Voice-Cloning/synthesizer/models/tacotron.py
+++ /dev/null
@@ -1,519 +0,0 @@
-import os
-import numpy as np
-import torch
-import torch.nn as nn
-import torch.nn.functional as F
-from pathlib import Path
-from typing import Union
-
-
-class HighwayNetwork(nn.Module):
- def __init__(self, size):
- super().__init__()
- self.W1 = nn.Linear(size, size)
- self.W2 = nn.Linear(size, size)
- self.W1.bias.data.fill_(0.)
-
- def forward(self, x):
- x1 = self.W1(x)
- x2 = self.W2(x)
- g = torch.sigmoid(x2)
- y = g * F.relu(x1) + (1. - g) * x
- return y
-
-
-class Encoder(nn.Module):
- def __init__(self, embed_dims, num_chars, encoder_dims, K, num_highways, dropout):
- super().__init__()
- prenet_dims = (encoder_dims, encoder_dims)
- cbhg_channels = encoder_dims
- self.embedding = nn.Embedding(num_chars, embed_dims)
- self.pre_net = PreNet(embed_dims, fc1_dims=prenet_dims[0], fc2_dims=prenet_dims[1],
- dropout=dropout)
- self.cbhg = CBHG(K=K, in_channels=cbhg_channels, channels=cbhg_channels,
- proj_channels=[cbhg_channels, cbhg_channels],
- num_highways=num_highways)
-
- def forward(self, x, speaker_embedding=None):
- x = self.embedding(x)
- x = self.pre_net(x)
- x.transpose_(1, 2)
- x = self.cbhg(x)
- if speaker_embedding is not None:
- x = self.add_speaker_embedding(x, speaker_embedding)
- return x
-
- def add_speaker_embedding(self, x, speaker_embedding):
- # SV2TTS
- # The input x is the encoder output and is a 3D tensor with size (batch_size, num_chars, tts_embed_dims)
- # When training, speaker_embedding is also a 2D tensor with size (batch_size, speaker_embedding_size)
- # (for inference, speaker_embedding is a 1D tensor with size (speaker_embedding_size))
- # This concats the speaker embedding for each char in the encoder output
-
- # Save the dimensions as human-readable names
- batch_size = x.size()[0]
- num_chars = x.size()[1]
-
- if speaker_embedding.dim() == 1:
- idx = 0
- else:
- idx = 1
-
- # Start by making a copy of each speaker embedding to match the input text length
- # The output of this has size (batch_size, num_chars * tts_embed_dims)
- speaker_embedding_size = speaker_embedding.size()[idx]
- e = speaker_embedding.repeat_interleave(num_chars, dim=idx)
-
- # Reshape it and transpose
- e = e.reshape(batch_size, speaker_embedding_size, num_chars)
- e = e.transpose(1, 2)
-
- # Concatenate the tiled speaker embedding with the encoder output
- x = torch.cat((x, e), 2)
- return x
-
-
-class BatchNormConv(nn.Module):
- def __init__(self, in_channels, out_channels, kernel, relu=True):
- super().__init__()
- self.conv = nn.Conv1d(in_channels, out_channels, kernel, stride=1, padding=kernel // 2, bias=False)
- self.bnorm = nn.BatchNorm1d(out_channels)
- self.relu = relu
-
- def forward(self, x):
- x = self.conv(x)
- x = F.relu(x) if self.relu is True else x
- return self.bnorm(x)
-
-
-class CBHG(nn.Module):
- def __init__(self, K, in_channels, channels, proj_channels, num_highways):
- super().__init__()
-
- # List of all rnns to call `flatten_parameters()` on
- self._to_flatten = []
-
- self.bank_kernels = [i for i in range(1, K + 1)]
- self.conv1d_bank = nn.ModuleList()
- for k in self.bank_kernels:
- conv = BatchNormConv(in_channels, channels, k)
- self.conv1d_bank.append(conv)
-
- self.maxpool = nn.MaxPool1d(kernel_size=2, stride=1, padding=1)
-
- self.conv_project1 = BatchNormConv(len(self.bank_kernels) * channels, proj_channels[0], 3)
- self.conv_project2 = BatchNormConv(proj_channels[0], proj_channels[1], 3, relu=False)
-
- # Fix the highway input if necessary
- if proj_channels[-1] != channels:
- self.highway_mismatch = True
- self.pre_highway = nn.Linear(proj_channels[-1], channels, bias=False)
- else:
- self.highway_mismatch = False
-
- self.highways = nn.ModuleList()
- for i in range(num_highways):
- hn = HighwayNetwork(channels)
- self.highways.append(hn)
-
- self.rnn = nn.GRU(channels, channels // 2, batch_first=True, bidirectional=True)
- self._to_flatten.append(self.rnn)
-
- # Avoid fragmentation of RNN parameters and associated warning
- self._flatten_parameters()
-
- def forward(self, x):
- # Although we `_flatten_parameters()` on init, when using DataParallel
- # the model gets replicated, making it no longer guaranteed that the
- # weights are contiguous in GPU memory. Hence, we must call it again
- self._flatten_parameters()
-
- # Save these for later
- residual = x
- seq_len = x.size(-1)
- conv_bank = []
-
- # Convolution Bank
- for conv in self.conv1d_bank:
- c = conv(x) # Convolution
- conv_bank.append(c[:, :, :seq_len])
-
- # Stack along the channel axis
- conv_bank = torch.cat(conv_bank, dim=1)
-
- # dump the last padding to fit residual
- x = self.maxpool(conv_bank)[:, :, :seq_len]
-
- # Conv1d projections
- x = self.conv_project1(x)
- x = self.conv_project2(x)
-
- # Residual Connect
- x = x + residual
-
- # Through the highways
- x = x.transpose(1, 2)
- if self.highway_mismatch is True:
- x = self.pre_highway(x)
- for h in self.highways: x = h(x)
-
- # And then the RNN
- x, _ = self.rnn(x)
- return x
-
- def _flatten_parameters(self):
- """Calls `flatten_parameters` on all the rnns used by the WaveRNN. Used
- to improve efficiency and avoid PyTorch yelling at us."""
- [m.flatten_parameters() for m in self._to_flatten]
-
-class PreNet(nn.Module):
- def __init__(self, in_dims, fc1_dims=256, fc2_dims=128, dropout=0.5):
- super().__init__()
- self.fc1 = nn.Linear(in_dims, fc1_dims)
- self.fc2 = nn.Linear(fc1_dims, fc2_dims)
- self.p = dropout
-
- def forward(self, x):
- x = self.fc1(x)
- x = F.relu(x)
- x = F.dropout(x, self.p, training=True)
- x = self.fc2(x)
- x = F.relu(x)
- x = F.dropout(x, self.p, training=True)
- return x
-
-
-class Attention(nn.Module):
- def __init__(self, attn_dims):
- super().__init__()
- self.W = nn.Linear(attn_dims, attn_dims, bias=False)
- self.v = nn.Linear(attn_dims, 1, bias=False)
-
- def forward(self, encoder_seq_proj, query, t):
-
- # print(encoder_seq_proj.shape)
- # Transform the query vector
- query_proj = self.W(query).unsqueeze(1)
-
- # Compute the scores
- u = self.v(torch.tanh(encoder_seq_proj + query_proj))
- scores = F.softmax(u, dim=1)
-
- return scores.transpose(1, 2)
-
-
-class LSA(nn.Module):
- def __init__(self, attn_dim, kernel_size=31, filters=32):
- super().__init__()
- self.conv = nn.Conv1d(1, filters, padding=(kernel_size - 1) // 2, kernel_size=kernel_size, bias=True)
- self.L = nn.Linear(filters, attn_dim, bias=False)
- self.W = nn.Linear(attn_dim, attn_dim, bias=True) # Include the attention bias in this term
- self.v = nn.Linear(attn_dim, 1, bias=False)
- self.cumulative = None
- self.attention = None
-
- def init_attention(self, encoder_seq_proj):
- device = next(self.parameters()).device # use same device as parameters
- b, t, c = encoder_seq_proj.size()
- self.cumulative = torch.zeros(b, t, device=device)
- self.attention = torch.zeros(b, t, device=device)
-
- def forward(self, encoder_seq_proj, query, t, chars):
-
- if t == 0: self.init_attention(encoder_seq_proj)
-
- processed_query = self.W(query).unsqueeze(1)
-
- location = self.cumulative.unsqueeze(1)
- processed_loc = self.L(self.conv(location).transpose(1, 2))
-
- u = self.v(torch.tanh(processed_query + encoder_seq_proj + processed_loc))
- u = u.squeeze(-1)
-
- # Mask zero padding chars
- u = u * (chars != 0).float()
-
- # Smooth Attention
- # scores = torch.sigmoid(u) / torch.sigmoid(u).sum(dim=1, keepdim=True)
- scores = F.softmax(u, dim=1)
- self.attention = scores
- self.cumulative = self.cumulative + self.attention
-
- return scores.unsqueeze(-1).transpose(1, 2)
-
-
-class Decoder(nn.Module):
- # Class variable because its value doesn't change between classes
- # yet ought to be scoped by class because its a property of a Decoder
- max_r = 20
- def __init__(self, n_mels, encoder_dims, decoder_dims, lstm_dims,
- dropout, speaker_embedding_size):
- super().__init__()
- self.register_buffer("r", torch.tensor(1, dtype=torch.int))
- self.n_mels = n_mels
- prenet_dims = (decoder_dims * 2, decoder_dims * 2)
- self.prenet = PreNet(n_mels, fc1_dims=prenet_dims[0], fc2_dims=prenet_dims[1],
- dropout=dropout)
- self.attn_net = LSA(decoder_dims)
- self.attn_rnn = nn.GRUCell(encoder_dims + prenet_dims[1] + speaker_embedding_size, decoder_dims)
- self.rnn_input = nn.Linear(encoder_dims + decoder_dims + speaker_embedding_size, lstm_dims)
- self.res_rnn1 = nn.LSTMCell(lstm_dims, lstm_dims)
- self.res_rnn2 = nn.LSTMCell(lstm_dims, lstm_dims)
- self.mel_proj = nn.Linear(lstm_dims, n_mels * self.max_r, bias=False)
- self.stop_proj = nn.Linear(encoder_dims + speaker_embedding_size + lstm_dims, 1)
-
- def zoneout(self, prev, current, p=0.1):
- device = next(self.parameters()).device # Use same device as parameters
- mask = torch.zeros(prev.size(), device=device).bernoulli_(p)
- return prev * mask + current * (1 - mask)
-
- def forward(self, encoder_seq, encoder_seq_proj, prenet_in,
- hidden_states, cell_states, context_vec, t, chars):
-
- # Need this for reshaping mels
- batch_size = encoder_seq.size(0)
-
- # Unpack the hidden and cell states
- attn_hidden, rnn1_hidden, rnn2_hidden = hidden_states
- rnn1_cell, rnn2_cell = cell_states
-
- # PreNet for the Attention RNN
- prenet_out = self.prenet(prenet_in)
-
- # Compute the Attention RNN hidden state
- attn_rnn_in = torch.cat([context_vec, prenet_out], dim=-1)
- attn_hidden = self.attn_rnn(attn_rnn_in.squeeze(1), attn_hidden)
-
- # Compute the attention scores
- scores = self.attn_net(encoder_seq_proj, attn_hidden, t, chars)
-
- # Dot product to create the context vector
- context_vec = scores @ encoder_seq
- context_vec = context_vec.squeeze(1)
-
- # Concat Attention RNN output w. Context Vector & project
- x = torch.cat([context_vec, attn_hidden], dim=1)
- x = self.rnn_input(x)
-
- # Compute first Residual RNN
- rnn1_hidden_next, rnn1_cell = self.res_rnn1(x, (rnn1_hidden, rnn1_cell))
- if self.training:
- rnn1_hidden = self.zoneout(rnn1_hidden, rnn1_hidden_next)
- else:
- rnn1_hidden = rnn1_hidden_next
- x = x + rnn1_hidden
-
- # Compute second Residual RNN
- rnn2_hidden_next, rnn2_cell = self.res_rnn2(x, (rnn2_hidden, rnn2_cell))
- if self.training:
- rnn2_hidden = self.zoneout(rnn2_hidden, rnn2_hidden_next)
- else:
- rnn2_hidden = rnn2_hidden_next
- x = x + rnn2_hidden
-
- # Project Mels
- mels = self.mel_proj(x)
- mels = mels.view(batch_size, self.n_mels, self.max_r)[:, :, :self.r]
- hidden_states = (attn_hidden, rnn1_hidden, rnn2_hidden)
- cell_states = (rnn1_cell, rnn2_cell)
-
- # Stop token prediction
- s = torch.cat((x, context_vec), dim=1)
- s = self.stop_proj(s)
- stop_tokens = torch.sigmoid(s)
-
- return mels, scores, hidden_states, cell_states, context_vec, stop_tokens
-
-
-class Tacotron(nn.Module):
- def __init__(self, embed_dims, num_chars, encoder_dims, decoder_dims, n_mels,
- fft_bins, postnet_dims, encoder_K, lstm_dims, postnet_K, num_highways,
- dropout, stop_threshold, speaker_embedding_size):
- super().__init__()
- self.n_mels = n_mels
- self.lstm_dims = lstm_dims
- self.encoder_dims = encoder_dims
- self.decoder_dims = decoder_dims
- self.speaker_embedding_size = speaker_embedding_size
- self.encoder = Encoder(embed_dims, num_chars, encoder_dims,
- encoder_K, num_highways, dropout)
- self.encoder_proj = nn.Linear(encoder_dims + speaker_embedding_size, decoder_dims, bias=False)
- self.decoder = Decoder(n_mels, encoder_dims, decoder_dims, lstm_dims,
- dropout, speaker_embedding_size)
- self.postnet = CBHG(postnet_K, n_mels, postnet_dims,
- [postnet_dims, fft_bins], num_highways)
- self.post_proj = nn.Linear(postnet_dims, fft_bins, bias=False)
-
- self.init_model()
- self.num_params()
-
- self.register_buffer("step", torch.zeros(1, dtype=torch.long))
- self.register_buffer("stop_threshold", torch.tensor(stop_threshold, dtype=torch.float32))
-
- @property
- def r(self):
- return self.decoder.r.item()
-
- @r.setter
- def r(self, value):
- self.decoder.r = self.decoder.r.new_tensor(value, requires_grad=False)
-
- def forward(self, x, m, speaker_embedding):
- device = next(self.parameters()).device # use same device as parameters
-
- self.step += 1
- batch_size, _, steps = m.size()
-
- # Initialise all hidden states and pack into tuple
- attn_hidden = torch.zeros(batch_size, self.decoder_dims, device=device)
- rnn1_hidden = torch.zeros(batch_size, self.lstm_dims, device=device)
- rnn2_hidden = torch.zeros(batch_size, self.lstm_dims, device=device)
- hidden_states = (attn_hidden, rnn1_hidden, rnn2_hidden)
-
- # Initialise all lstm cell states and pack into tuple
- rnn1_cell = torch.zeros(batch_size, self.lstm_dims, device=device)
- rnn2_cell = torch.zeros(batch_size, self.lstm_dims, device=device)
- cell_states = (rnn1_cell, rnn2_cell)
-
- # Frame for start of decoder loop
- go_frame = torch.zeros(batch_size, self.n_mels, device=device)
-
- # Need an initial context vector
- context_vec = torch.zeros(batch_size, self.encoder_dims + self.speaker_embedding_size, device=device)
-
- # SV2TTS: Run the encoder with the speaker embedding
- # The projection avoids unnecessary matmuls in the decoder loop
- encoder_seq = self.encoder(x, speaker_embedding)
- encoder_seq_proj = self.encoder_proj(encoder_seq)
-
- # Need a couple of lists for outputs
- mel_outputs, attn_scores, stop_outputs = [], [], []
-
- # Run the decoder loop
- for t in range(0, steps, self.r):
- prenet_in = m[:, :, t - 1] if t > 0 else go_frame
- mel_frames, scores, hidden_states, cell_states, context_vec, stop_tokens = \
- self.decoder(encoder_seq, encoder_seq_proj, prenet_in,
- hidden_states, cell_states, context_vec, t, x)
- mel_outputs.append(mel_frames)
- attn_scores.append(scores)
- stop_outputs.extend([stop_tokens] * self.r)
-
- # Concat the mel outputs into sequence
- mel_outputs = torch.cat(mel_outputs, dim=2)
-
- # Post-Process for Linear Spectrograms
- postnet_out = self.postnet(mel_outputs)
- linear = self.post_proj(postnet_out)
- linear = linear.transpose(1, 2)
-
- # For easy visualisation
- attn_scores = torch.cat(attn_scores, 1)
- # attn_scores = attn_scores.cpu().data.numpy()
- stop_outputs = torch.cat(stop_outputs, 1)
-
- return mel_outputs, linear, attn_scores, stop_outputs
-
- def generate(self, x, speaker_embedding=None, steps=2000):
- self.eval()
- device = next(self.parameters()).device # use same device as parameters
-
- batch_size, _ = x.size()
-
- # Need to initialise all hidden states and pack into tuple for tidyness
- attn_hidden = torch.zeros(batch_size, self.decoder_dims, device=device)
- rnn1_hidden = torch.zeros(batch_size, self.lstm_dims, device=device)
- rnn2_hidden = torch.zeros(batch_size, self.lstm_dims, device=device)
- hidden_states = (attn_hidden, rnn1_hidden, rnn2_hidden)
-
- # Need to initialise all lstm cell states and pack into tuple for tidyness
- rnn1_cell = torch.zeros(batch_size, self.lstm_dims, device=device)
- rnn2_cell = torch.zeros(batch_size, self.lstm_dims, device=device)
- cell_states = (rnn1_cell, rnn2_cell)
-
- # Need a Frame for start of decoder loop
- go_frame = torch.zeros(batch_size, self.n_mels, device=device)
-
- # Need an initial context vector
- context_vec = torch.zeros(batch_size, self.encoder_dims + self.speaker_embedding_size, device=device)
-
- # SV2TTS: Run the encoder with the speaker embedding
- # The projection avoids unnecessary matmuls in the decoder loop
- encoder_seq = self.encoder(x, speaker_embedding)
- encoder_seq_proj = self.encoder_proj(encoder_seq)
-
- # Need a couple of lists for outputs
- mel_outputs, attn_scores, stop_outputs = [], [], []
-
- # Run the decoder loop
- for t in range(0, steps, self.r):
- prenet_in = mel_outputs[-1][:, :, -1] if t > 0 else go_frame
- mel_frames, scores, hidden_states, cell_states, context_vec, stop_tokens = \
- self.decoder(encoder_seq, encoder_seq_proj, prenet_in,
- hidden_states, cell_states, context_vec, t, x)
- mel_outputs.append(mel_frames)
- attn_scores.append(scores)
- stop_outputs.extend([stop_tokens] * self.r)
- # Stop the loop when all stop tokens in batch exceed threshold
- if (stop_tokens > 0.5).all() and t > 10: break
-
- # Concat the mel outputs into sequence
- mel_outputs = torch.cat(mel_outputs, dim=2)
-
- # Post-Process for Linear Spectrograms
- postnet_out = self.postnet(mel_outputs)
- linear = self.post_proj(postnet_out)
-
-
- linear = linear.transpose(1, 2)
-
- # For easy visualisation
- attn_scores = torch.cat(attn_scores, 1)
- stop_outputs = torch.cat(stop_outputs, 1)
-
- self.train()
-
- return mel_outputs, linear, attn_scores
-
- def init_model(self):
- for p in self.parameters():
- if p.dim() > 1: nn.init.xavier_uniform_(p)
-
- def get_step(self):
- return self.step.data.item()
-
- def reset_step(self):
- # assignment to parameters or buffers is overloaded, updates internal dict entry
- self.step = self.step.data.new_tensor(1)
-
- def log(self, path, msg):
- with open(path, "a") as f:
- print(msg, file=f)
-
- def load(self, path, optimizer=None):
- # Use device of model params as location for loaded state
- device = next(self.parameters()).device
- checkpoint = torch.load(str(path), map_location=device)
- self.load_state_dict(checkpoint["model_state"])
-
- if "optimizer_state" in checkpoint and optimizer is not None:
- optimizer.load_state_dict(checkpoint["optimizer_state"])
-
- def save(self, path, optimizer=None):
- if optimizer is not None:
- torch.save({
- "model_state": self.state_dict(),
- "optimizer_state": optimizer.state_dict(),
- }, str(path))
- else:
- torch.save({
- "model_state": self.state_dict(),
- }, str(path))
-
-
- def num_params(self, print_out=True):
- parameters = filter(lambda p: p.requires_grad, self.parameters())
- parameters = sum([np.prod(p.size()) for p in parameters]) / 1_000_000
- if print_out:
- print("Trainable Parameters: %.3fM" % parameters)
- return parameters
diff --git a/spaces/nagolinc/liteDungeon/README.md b/spaces/nagolinc/liteDungeon/README.md
deleted file mode 100644
index 501c4282d27ad368964fb083e299b14ebd1ad781..0000000000000000000000000000000000000000
--- a/spaces/nagolinc/liteDungeon/README.md
+++ /dev/null
@@ -1,13 +0,0 @@
----
-title: LiteDungeon
-emoji: 📉
-colorFrom: blue
-colorTo: gray
-sdk: gradio
-sdk_version: 3.0.10
-app_file: app.py
-pinned: false
-license: mit
----
-
-Check out the configuration reference at https://huggingface.co/docs/hub/spaces#reference
diff --git a/spaces/nahue-passano/librispeech-corpus-generator/whisper_transcriber/transcribe.py b/spaces/nahue-passano/librispeech-corpus-generator/whisper_transcriber/transcribe.py
deleted file mode 100644
index be0b2c1f0df6ce57152bd7620ee1617503bcec94..0000000000000000000000000000000000000000
--- a/spaces/nahue-passano/librispeech-corpus-generator/whisper_transcriber/transcribe.py
+++ /dev/null
@@ -1,3087 +0,0 @@
-import os
-
-# Remove warning "This TensorFlow binary is optimized with oneAPI Deep Neural Network Library (oneDNN)..."
-os.environ["TF_CPP_MIN_LOG_LEVEL"] = "1"
-os.environ["CUDA_DEVICE_ORDER"] = "PCI_BUS_ID" # GPU in the right order
-__version__ = "1.12.20"
-
-# Whisper and Torch
-import whisper
-import torch
-import torch.nn.functional as F
-
-# For alignment
-import numpy as np
-import dtw
-
-# from scipy.signal import medfilt as median_filter
-from scipy.ndimage import (
- median_filter,
-) # faster owing to https://github.com/openai/whisper/commit/f0083e7eb20d032390e42f6f6039947fa8669c93
-from scipy.signal import find_peaks
-
-# Additional
-import string
-import csv
-import sys
-import gzip, base64
-import copy
-import re
-
-# Constant variables
-from whisper.utils import format_timestamp
-from whisper.audio import N_FRAMES, HOP_LENGTH, SAMPLE_RATE # 3000, 160, 16000
-
-AUDIO_SAMPLES_PER_TOKEN = HOP_LENGTH * 2 # 320
-AUDIO_TIME_PER_TOKEN = AUDIO_SAMPLES_PER_TOKEN / SAMPLE_RATE # 0.02 (sec)
-SEGMENT_DURATION = N_FRAMES * HOP_LENGTH / SAMPLE_RATE # 30.0 (sec)
-
-# Logs
-import logging
-
-logger = logging.getLogger("whisper_timestamped")
-
-USE_EFFICIENT_BY_DEFAULT = True
-TRUST_WHISPER_TIMESTAMP_BY_DEFAULT = True
-DISFLUENCY_MARK = "[*]"
-
-try:
- whisper_version = whisper.__version__
-except NameError:
- whisper_version = ""
-WHIPSER_GE_20230306 = whisper_version >= "20230306"
-WHIPSER_GE_20230308 = whisper_version >= "20230308"
-
-
-def transcribe_timestamped(
- # Main Whisper options
- model,
- audio,
- language=None,
- task="transcribe",
- # Additional options for word alignment
- remove_punctuation_from_words=False,
- compute_word_confidence=True,
- include_punctuation_in_confidence=False,
- refine_whisper_precision=0.5,
- min_word_duration=0.02, # Was 0.04 before 1.11
- plot_word_alignment=False,
- word_alignement_most_top_layers=None, # Was 6 before 1.9
- remove_empty_words=False,
- # Reproducibility
- seed=1234,
- vad=False,
- detect_disfluencies=False,
- trust_whisper_timestamps=TRUST_WHISPER_TIMESTAMP_BY_DEFAULT,
- naive_approach=False,
- # Other Whisper options
- temperature=0.0 if USE_EFFICIENT_BY_DEFAULT else (0.0, 0.2, 0.4, 0.6, 0.8, 1.0),
- best_of=None,
- beam_size=None,
- patience=None,
- length_penalty=None,
- compression_ratio_threshold=2.4,
- logprob_threshold=-1.0,
- no_speech_threshold=0.6,
- fp16=None,
- condition_on_previous_text=True,
- initial_prompt=None,
- suppress_tokens="-1",
- sample_len=None,
- verbose=False,
-):
- """
- Transcribe an audio file using Whisper
-
- Parameters
- ----------
- model: Whisper
- The Whisper model instance.
-
- audio: Union[str, np.ndarray, torch.Tensor]
- The path to the audio file to open, or the audio waveform in 16kHz.
-
- language: str
- The language to use for the transcription. If None, the language is detected automatically.
-
- task: str
- The task to perform: either "transcribe" or "translate".
-
- remove_punctuation_from_words: bool
- If False, words will be glued with the next punctuation mark (if any).
- If True, there will be no punctuation mark in the `words[:]["text"]` list.
- It only affects these strings; This has no influence on the computation of the word confidence, whatever the value of `include_punctuation_in_confidence` is.
-
- include_punctuation_in_confidence: bool
- Whether to include proba of punctuation in the computation of the (previous) word confidence.
-
- compute_word_confidence: bool
- Whether to compute word confidence.
- If True, a finer confidence for each segment will be computed as well.
-
- vad: bool
- Whether to perform voice activity detection (VAD) on the audio file, to remove silent parts before transcribing with Whisper model.
- This should decrease hallucinations from the Whisper model.
-
- detect_disfluencies: bool
- Whether to detect disfluencies (i.e. hesitations, filler words, repetitions, corrections, etc.) that Whisper model might have omitted in the transcription.
- This should make the word timestamp prediction more accurate.
- And probable disfluencies will be marked as special words "[*]".
-
- trust_whisper_timestamps: bool
- Whether to rely on Whisper's timestamps to get approximative first estimate of segment positions (up to refine_whisper_precision).
-
- refine_whisper_precision: float
- How much can we refine Whisper segment positions, in seconds. Must be a multiple of 0.02.
-
- min_word_duration: float
- Minimum duration of a word, in seconds. If a word is shorter than this, timestamps will be adjusted.
-
- plot_word_alignment: bool
- Whether to plot the word alignment for each segment. matplotlib must be installed to use this option.
-
- remove_empty_words: bool
- Whether to remove words with no duration occuring at the end of segments (probable Whisper hallucinations).
-
- seed: int
- Random seed to use for temperature sampling, for the sake of reproducibility.
- Choose None for unpredictable randomness.
-
- naive_approach: bool
- Force the naive approach that consists in decoding twice the audio file, once to get the transcritpion and once with the decoded tokens to get the alignment.
- Note that this approach is used anyway when beam_size is not None and/or when the temperature is a list with more than one element.
-
- temperature: float
- Temperature for sampling.
-
- compression_ratio_threshold: float
- If the gzip compression ratio is above this value, treat as failed.
-
- logprob_threshold: float
- If the average log probability over sampled tokens is below this value, treat as failed.
-
- no_speech_threshold: float
- If the no_speech probability is higher than this value AND the average log probability
- over sampled tokens is below `logprob_threshold`, consider the segment as silent.
-
- condition_on_previous_text: bool
- if True, the previous output of the model is provided as a prompt for the next window;
- disabling may make the text inconsistent across windows, but the model becomes less prone to
- getting stuck in a failure loop, such as repetition looping or timestamps going out of sync.
-
- initial_prompt: str
- Optional text to provide as a prompt for the first window.
-
- suppress_tokens: str
- Comma-separated list of token ids to suppress during sampling;
- '-1' will suppress most special characters except common punctuations.
-
- verbose: bool
- Whether to display the text being decoded to the console. If True, displays all the details,
- If False, displays minimal details. If None, does not display anything
-
- Returns
- -------
- A dictionary containing the resulting text ("text") and segment-level details ("segments"), and
- the spoken language ("language"), which is detected when `decode_options["language"]` is None.
- """
-
- if seed is not None:
- torch.manual_seed(seed)
- torch.cuda.manual_seed_all(seed)
-
- # Check input options
- assert (
- refine_whisper_precision >= 0
- and refine_whisper_precision / AUDIO_TIME_PER_TOKEN
- == round(refine_whisper_precision / AUDIO_TIME_PER_TOKEN)
- ), f"refine_whisper_precision must be a positive multiple of {AUDIO_TIME_PER_TOKEN}"
- refine_whisper_precision_nframes = round(
- refine_whisper_precision / AUDIO_TIME_PER_TOKEN
- )
- assert min_word_duration >= 0, f"min_word_duration must be a positive number"
- assert (
- word_alignement_most_top_layers is None or word_alignement_most_top_layers > 0
- ), f"word_alignement_most_top_layers must be a strictly positive number"
-
- if isinstance(temperature, (list, tuple)) and len(temperature) == 1:
- temperature = temperature[0]
- if isinstance(temperature, (list, tuple)):
- # temperature fallback
- naive_approach = True
- elif temperature > 0 and best_of is not None and best_of > 1:
- naive_approach = True
- if beam_size is not None:
- # beam-search
- naive_approach = True
-
- # Input options
- if fp16 is None:
- fp16 = model.device != torch.device("cpu")
-
- # Safety check
- input_stride = N_FRAMES // model.dims.n_audio_ctx
- time_precision = input_stride * HOP_LENGTH / SAMPLE_RATE
- assert time_precision == AUDIO_TIME_PER_TOKEN
-
- alignment_options = dict(
- remove_punctuation_from_words=remove_punctuation_from_words,
- compute_word_confidence=compute_word_confidence,
- include_punctuation_in_confidence=include_punctuation_in_confidence,
- detect_disfluencies=detect_disfluencies,
- refine_whisper_precision_nframes=refine_whisper_precision_nframes,
- plot_word_alignment=plot_word_alignment,
- word_alignement_most_top_layers=word_alignement_most_top_layers,
- alignment_heads=get_alignment_heads(model)
- if word_alignement_most_top_layers is None
- else None,
- )
- whisper_options = dict(
- language=language,
- task=task,
- fp16=fp16,
- temperature=temperature,
- best_of=best_of,
- beam_size=beam_size,
- patience=patience,
- length_penalty=length_penalty,
- condition_on_previous_text=condition_on_previous_text,
- initial_prompt=initial_prompt,
- suppress_tokens=suppress_tokens,
- sample_len=sample_len,
- verbose=verbose if (not vad or verbose is not True) else False,
- )
- other_options = dict(
- no_speech_threshold=no_speech_threshold,
- logprob_threshold=logprob_threshold,
- compression_ratio_threshold=compression_ratio_threshold,
- )
-
- if vad:
- audio = get_audio_tensor(audio)
- audio, convert_timestamps = remove_non_speech(audio, plot=plot_word_alignment)
-
- global num_alignment_for_plot
- num_alignment_for_plot = 0
-
- if naive_approach:
- (transcription, words) = _transcribe_timestamped_naive(
- model,
- audio,
- min_word_duration=0.0, # Was 0.04 before 1.11
- trust_whisper_timestamps=trust_whisper_timestamps,
- **alignment_options,
- **whisper_options,
- **other_options,
- )
- else:
- (transcription, words) = _transcribe_timestamped_efficient(
- model,
- audio,
- trust_whisper_timestamps=trust_whisper_timestamps,
- **alignment_options,
- **whisper_options,
- **other_options,
- )
- if remove_empty_words:
- # Remove words with empty duration happening at the end of segments, to remove some hallucinations
- transcription, words = remove_last_null_duration_words(
- transcription, words, recompute_text=True
- )
-
- # Refine word positions
- ensure_increasing_positions(
- words, min_duration=min_word_duration if trust_whisper_timestamps else 0
- )
-
- # Combine words and segments
- whisper_segments = transcription["segments"]
- for word in words:
- if verbose and not naive_approach and not vad:
- print_timestamped(word)
- word.pop("tokens")
- word.pop("tokens_indices")
- if "avg_logprob_reliable" in word:
- word.pop("avg_logprob_reliable")
- idx_segment = word.pop("idx_segment")
- assert idx_segment < len(
- whisper_segments
- ), f"Fatal error: Got unexpected segment index {idx_segment} >= {len(whisper_segments)}"
- segment = whisper_segments[idx_segment]
- if "words" in segment:
- segment["words"].append(word)
- else:
- segment["words"] = [word]
- if refine_whisper_precision:
- segment["start"] = word["start"]
- if refine_whisper_precision:
- segment["end"] = word["end"]
-
- if vad:
- # Recompute timestamps to match the original audio
- for segment in whisper_segments:
- for word in segment.get("words", []):
- word["start"], word["end"] = convert_timestamps(
- word["start"], word["end"]
- )
- if verbose:
- print_timestamped(word)
- if refine_whisper_precision and len(segment.get("words", [])):
- segment["start"] = segment["words"][0]["start"]
- segment["end"] = segment["words"][-1]["end"]
- else:
- segment["start"], segment["end"] = convert_timestamps(
- segment["start"], segment["end"]
- )
-
- return transcription
-
-
-def _transcribe_timestamped_efficient(
- model,
- audio,
- remove_punctuation_from_words,
- compute_word_confidence,
- include_punctuation_in_confidence,
- refine_whisper_precision_nframes,
- alignment_heads,
- plot_word_alignment,
- word_alignement_most_top_layers,
- detect_disfluencies,
- trust_whisper_timestamps,
- use_timestamps_for_alignment=True,
- # Whisper specific options
- **whisper_options,
-):
- # Get options
- sample_len = whisper_options["sample_len"]
- temperature = whisper_options["temperature"]
- no_speech_threshold = whisper_options["no_speech_threshold"]
- logprob_threshold = whisper_options["logprob_threshold"]
- verbose = whisper_options["verbose"]
- # Note: "on-the-fly" verbose is not implementable in the current state (we don't know the absolute position of the current chunk). See issue #18
- verbose_bugged = False
- whisper_options["verbose"] = (
- None if whisper_options["verbose"] is True else whisper_options["verbose"]
- ) # We will print intermediate results ourselves
-
- logit_filters = get_logit_filters(model, whisper_options)
- language = whisper_options["language"]
- tokenizer = whisper.tokenizer.get_tokenizer(
- model.is_multilingual, task=whisper_options["task"], language=language
- )
-
- max_sample_len = sample_len or model.dims.n_text_ctx // 2
- n_ctx = model.dims.n_text_ctx
-
- debug = logger.getEffectiveLevel() >= logging.DEBUG
-
- word_alignement_most_top_layers = (
- float("inf")
- if word_alignement_most_top_layers is None
- else word_alignement_most_top_layers
- )
-
- # The main outcome
- timestamped_word_segments = (
- []
- ) # list of timestamped word segments that have been collected so far
- # Main variables to be accumulated
- segment_tokens = [
- []
- ] # list of lists of token indices that have been collected so far (one list per segment)
- segment_attweights = [
- []
- for _ in range(min(word_alignement_most_top_layers, len(model.decoder.blocks)))
- ]
- # attention weights on the last segments
- segment_avglogprobs = (
- []
- ) # average log probability for each segment (actually of the corresponding chunk, as computed by whisper)
- segment_logprobs = [] # token log probabilities for each segment
- # Variables related to options that can skip some segments
- sot_index = None # index of the SOT token in the current set of processed tokens
- no_speech_prob = None # no speech probability for the current 30 sec chunk
- chunk_logprobs = [] # log probabilities for the current 30 sec chunk
- chunk_tokens = [] # tokens for the current 30 sec chunk (list of Torch tensors)
- chunk_tokens_nosot = (
- []
- ) # tokens for the current 30 sec chunk, without the SOT tokens (list of indices)
- last_chunk_token = (
- None # last token of the current chunk, that may be needed for corner cases
- )
- last_token_fallback = (
- None # last token to use as a fallback if the model gets stuck
- )
- has_started = False # whether we have started decoding
- mfcc = None # MFCC features for the current 30 sec chunk
- new_mfcc = None #
- num_inference_steps = (
- 0 # number of inference steps performed so far (for debugging only)
- )
-
- def is_sot(curr_tokens):
- return (
- curr_tokens is None
- or len(curr_tokens) > 1
- or curr_tokens[0] == tokenizer.sot
- )
-
- def has_reached_decoding_limit():
- n = len(chunk_tokens_nosot) + 1
- m = n + (len(chunk_tokens[0]) if len(chunk_tokens) > 0 else 0)
- return n + 1 >= max_sample_len or m > n_ctx
-
- def reset(add_segment, keep_last_token=True):
- """Reset the list of tokens for the current speech segment, and corresponding cross-attention weights"""
- nonlocal segment_tokens, segment_attweights
- if add_segment:
- if keep_last_token:
- segment_tokens.append([segment_tokens[-1][-1]])
- segment_attweights = [w[-1:] for w in segment_attweights]
- else:
- segment_tokens.append([])
- segment_attweights = [[] for w in segment_attweights]
- segment_tokens[-2].pop(0)
- elif len(segment_tokens[-1]) > 0:
- if debug:
- logger.debug(
- f"Reset last segment: {tokenizer.decode_with_timestamps(segment_tokens[-1])}"
- )
- segment_tokens[-1] = []
- segment_attweights = [[] for w in segment_attweights]
-
- saw_consecutive_timestamps = False
-
- def must_flush_segment(curr_tokens):
- """Return whether or not the previously collected tokens must be used to add a new speech segment"""
- nonlocal segment_tokens, saw_consecutive_timestamps, chunk_tokens_nosot
-
- if not is_sot(curr_tokens):
- is_timestamp = curr_tokens[0] >= tokenizer.timestamp_begin
- is_previous_timestamp = (
- segment_tokens[-1][-1] >= tokenizer.timestamp_begin
- if len(segment_tokens[-1]) > 0
- else False
- )
- consecutive_timestamps = is_timestamp and is_previous_timestamp
- if consecutive_timestamps:
- saw_consecutive_timestamps = True
- return consecutive_timestamps
- else: # Several tokens as a prompt or must flush last segments
- must_flush = len(segment_tokens[-1]) > 1 and not saw_consecutive_timestamps
- if (
- not must_flush and WHIPSER_GE_20230306
- ): # If the last token is a timestamp, the last segment is used
- if last_chunk_token is None:
- must_flush = (
- len(segment_tokens[-1]) > 2
- and segment_tokens[-1][-1] >= tokenizer.timestamp_begin
- )
- else:
- must_flush = last_chunk_token >= tokenizer.timestamp_begin
- if not must_flush and trust_whisper_timestamps:
- # Discard the end of the last transcription
- reset(False)
- saw_consecutive_timestamps = False
- return must_flush
-
- index_begin_30sec_chunck = 0
-
- def get_index_begin_30sec_chunck(curr_tokens):
- nonlocal index_begin_30sec_chunck, has_started
-
- if is_sot(curr_tokens) and has_started:
- if trust_whisper_timestamps:
- res = index_begin_30sec_chunck
- index_begin_30sec_chunck = len(segment_tokens) - 1
- else:
- res = len(segment_tokens) - 1
- return res
-
- def align_last_segment(curr_tokens=None):
- nonlocal segment_tokens, segment_attweights, timestamped_word_segments, has_started, no_speech_prob, chunk_tokens, chunk_tokens_nosot, chunk_logprobs, mfcc, new_mfcc, logit_filters, index_begin_30sec_chunck, last_token_fallback, num_inference_steps
-
- if debug and trust_whisper_timestamps:
- logger.debug(
- f"Add segment {len(timestamped_word_segments)+1} at step {num_inference_steps}:\n\t{tokenizer.decode_with_timestamps(segment_tokens[-1])}"
- )
-
- tokens = segment_tokens[-1][1:]
-
- # When the decoding hit the max limit (number of tokens) -- usually when the language model gets stuck --
- # then we have to recover the last token from what is send to the decoder
- unfinished_decoding = has_reached_decoding_limit()
- last_is_not_timestamp = len(tokens) and tokens[-1] < tokenizer.timestamp_begin
- last_token_reliable = True
-
- if unfinished_decoding:
- logger.debug(
- f"WARNING: decoding hit the max limit for segment {segment_tokens[-1]} (It usually happens when the language model gets stuck)"
- )
- # The last token chosen is in the prompt for the new chunk
- if curr_tokens is not None and curr_tokens[0] == tokenizer.sot_prev:
- index_sot = (curr_tokens == tokenizer.sot).nonzero(as_tuple=True)
- assert len(index_sot) == 1
- index_sot = index_sot[0].item()
- assert index_sot > 0
- last_token_fallback = curr_tokens[index_sot - 1].item()
- logger.debug(
- f" Guessed last token from the prompt for the new chunk: {last_token_fallback}"
- )
- # Fallback for the last segment, or without prompt: Assume greedy decoding
- else:
- last_token_fallback = (
- torch.argmax(chunk_logprobs[-1]).item()
- if last_chunk_token is None
- else last_chunk_token
- )
- last_token_reliable = temperature == 0
- logger.debug(
- f" Guess last token using probas (assuming greedy decoding): {last_token_fallback}"
- )
- if debug:
- logger.debug(
- f"WARNING: also add last token: {tokenizer.decode_with_timestamps([last_token_fallback])}"
- )
-
- tokens.append(last_token_fallback)
- segment_tokens[-1].append(last_token_fallback)
- attention_weights = [torch.cat(w, dim=-2) for w in segment_attweights]
- last_logprobs = chunk_logprobs[-1]
- elif (
- last_is_not_timestamp
- ): # was emitted early, without a timestamp before
- logger.debug(f"WARNING: end timestamp not produced. Adding <|endoftext|>")
- tokens.append(tokenizer.eot)
- segment_tokens[-1].append(tokenizer.eot)
- attention_weights = [torch.cat(w, dim=-2) for w in segment_attweights]
- last_logprobs = chunk_logprobs[-1]
- else:
- attention_weights = [torch.cat(w[:-1], dim=-2) for w in segment_attweights]
- last_logprobs = chunk_logprobs[-2]
-
- # Check prediction of last token
- end_token = tokens[-1]
- if end_token >= tokenizer.timestamp_begin:
- start_token = tokens[0]
- assert start_token >= tokenizer.timestamp_begin
- # If Whisper prediction of the end is obviously wrong, we predict it again (constrained)
- if end_token <= start_token:
- new_end_token = (
- last_logprobs[start_token + 1 :].argmax() + start_token + 1
- )
- tokens[-1] = new_end_token.item()
- if debug:
- logger.debug(
- f"Re-estimated end token {tokenizer.decode_with_timestamps([new_end_token])} (was {tokenizer.decode_with_timestamps([end_token])}) to be after start token {tokenizer.decode_with_timestamps([start_token])}"
- )
-
- if len(tokens) <= 1:
- # Corner case: nothing in between timestamps
- ws = []
- else:
- ws = perform_word_alignment(
- tokens,
- attention_weights,
- tokenizer,
- use_space=should_use_space(language),
- alignment_heads=alignment_heads,
- remove_punctuation_from_words=remove_punctuation_from_words,
- refine_whisper_precision_nframes=refine_whisper_precision_nframes,
- detect_disfluencies=detect_disfluencies,
- unfinished_decoding=unfinished_decoding,
- mfcc=mfcc,
- plot=plot_word_alignment,
- debug=debug,
- )
-
- add_segment = len(ws) > 0
- if add_segment:
- timestamped_word_segments.append(ws)
- else:
- logger.debug(f"Not added!")
- reset(add_segment, not is_sot(curr_tokens))
-
- return add_segment, unfinished_decoding, last_token_reliable
-
- def may_flush_segment(curr_tokens=None):
- """Add a speech segment with the new tokens if necessary.
- May also remove the last collected segments if filtered out by Whisper (no_speech_prob <= no_speech_threshold)
- """
- nonlocal segment_tokens, segment_attweights, timestamped_word_segments, segment_logprobs, has_started, no_speech_prob, chunk_tokens, chunk_tokens_nosot, chunk_logprobs, mfcc, new_mfcc, logit_filters, index_begin_30sec_chunck, last_token_fallback, num_inference_steps, last_chunk_token
-
- # Check if a new segment should be added
- unfinished_decoding = False
- last_token_reliable = True
-
- if must_flush_segment(curr_tokens) and trust_whisper_timestamps:
- _, unfinished_decoding, last_token_reliable = align_last_segment(
- curr_tokens
- )
-
- i_start = get_index_begin_30sec_chunck(curr_tokens)
-
- # All segments from previous 30sec chunck have been collected
- if i_start is not None:
- if not trust_whisper_timestamps:
- tokens = torch.Tensor(segment_tokens[-1]).int()
- idx_task = torch.where(tokens == tokenizer.sot_sequence[-1])[0][
- 0
- ].item() # index of <|transcribe|>
-
- is_special = tokens.ge(tokenizer.eot)
- # Remove prompt
- is_special[:idx_task] = True
- # Keep begin timestamp
- is_special[idx_task : idx_task + 2] = False
-
- is_timestamp = tokens.ge(tokenizer.timestamp_begin)
- consecutive = torch.where(is_timestamp[1:] & is_timestamp[:-1])[0]
- if (WHIPSER_GE_20230306 or has_reached_decoding_limit()) and (
- (is_timestamp[-1] and not is_timestamp[-2])
- if last_chunk_token is None
- else last_chunk_token >= tokenizer.timestamp_begin
- and not is_timestamp[-2]
- ):
- consecutive = torch.cat(
- [consecutive, torch.Tensor([len(tokens) - 1]).int()]
- )
- last_is_timestamp = True
- if len(consecutive):
- # Remove last tokens
- is_special[consecutive[-1] + 1 :] = True
- # Keep end timestamp
- is_special[consecutive[-1]] = False
- elif is_timestamp[-1]:
- # Keep end timestamp
- is_special[-1] = False
- else:
- last_is_timestamp = False
-
- if use_timestamps_for_alignment and len(consecutive):
- # Keep all timestamps
- is_special[idx_task + 2 : consecutive[-1]] = False
-
- # Do remove what has to be removed
- is_next_achar = ~torch.cat(
- [is_special[1:], torch.Tensor([False]).bool()]
- )
- for i, weights in enumerate(segment_attweights):
- assert len(weights) == len(
- tokens
- ), f"{len(weights)} attention weights != {len(tokens)}"
- # We must remove attention weights used to predict timestamp tokens
- segment_attweights[i] = [
- w for s, w in zip(is_next_achar, weights) if s
- ]
- tokens_filtered = tokens[~is_special]
- assert len(segment_attweights[0]) == len(
- tokens_filtered
- ), f"{len(segment_attweights[0])} attention weights != {len(tokens_filtered)} "
-
- # Replace first and last timestamp
- orig_start, orig_end = (
- tokens_filtered[1].item(),
- tokens_filtered[-1].item(),
- )
- tokens_filtered[1] = tokenizer.timestamp_begin # <|0.00|>
- if last_is_timestamp:
- tokens_filtered[-1] = (
- tokenizer.timestamp_begin + N_FRAMES // 2
- ) # <|30.00|>
- segment_tokens[-1] = tokens_filtered.tolist()
-
- # Do alignement
- added, unfinished_decoding, last_token_reliable = align_last_segment()
-
- # Re-split into segments (if necessary)
- if added:
- if len(consecutive) > 1:
- segments_timestamped_concat = timestamped_word_segments[-1]
- new_segments_timestamped = []
- new_segment_tokens = []
- start = idx_task + 1
- i_word = 0
- for i, end in enumerate(consecutive):
- end = end.item()
- new_segment_tokens.append(tokens[start : end + 1].tolist())
- if debug:
- logger.debug(
- f"Add segment {len(timestamped_word_segments)+i}:\n\t{tokenizer.decode_with_timestamps(new_segment_tokens[-1])}"
- )
- total_length = end - start - 1
- start = end + 1
- length = 0
- new_segments_timestamped.append([])
- while length < total_length:
- if not use_timestamps_for_alignment and i_word == len(
- segments_timestamped_concat
- ):
- # This can happen in the case of "..."
- assert (
- total_length == 1 and i == len(consecutive) - 1
- ), "Unexpected situation!"
- break
- assert i_word < len(
- segments_timestamped_concat
- ), f"i_word={i_word} < len(segments_timestamped_concat)={len(segments_timestamped_concat)}"
- word = segments_timestamped_concat[i_word]
- new_segments_timestamped[-1].append(word)
- length += len(word["tokens_indices"])
- i_word += 1
- # This can be non zero, when a punctuation (alone in a segment) is glued to the previous segment
- if use_timestamps_for_alignment:
- assert (
- length == total_length
- ), f"length={length} != total_length={total_length}"
- elif length > total_length:
- delta = length - total_length
- word = new_segments_timestamped[-1][-1]
- word_tokindices = word["tokens_indices"]
- word_tokens = word["tokens"]
- word["tokens_indices"] = word_tokindices[:-delta]
- word["tokens"] = word_tokens[:-delta]
- word["word"] = "".join(word_tokens[:-delta])
- i_word -= 1
- t = segments_timestamped_concat[i_word]["end"]
- segments_timestamped_concat[i_word] = dict(
- text="".join(word_tokens[-delta:]),
- start=t,
- end=t, # Word without timestamp
- tokens=word_tokens[-delta:],
- tokens_indices=word_tokindices[-delta:],
- )
-
- assert i_word == len(segments_timestamped_concat)
-
- segment_tokens = (
- segment_tokens[:-2]
- + new_segment_tokens
- + [segment_tokens[-1]]
- )
- timestamped_word_segments = (
- timestamped_word_segments[:-1] + new_segments_timestamped
- )
-
- else:
- # Recover start and end token
- segment = segment_tokens[-2]
- tokenizer.decode_with_timestamps([orig_start, orig_end])
- segment[0] = orig_start
- if last_is_timestamp:
- segment[-1] = orig_end
-
- if debug:
- logger.debug(
- f"Add segment {len(timestamped_word_segments)}:\n\t{tokenizer.decode_with_timestamps(segment)}"
- )
-
- if unfinished_decoding:
- timestamped_word_segments[-1][-1][
- "avg_logprob_reliable"
- ] = last_token_reliable
-
- reset(False)
-
- mfcc = new_mfcc
-
- n_segments = len(segment_tokens) - 1
-
- # Get word confidence and/or check if previous segments shoud have been skipped
- should_skip = False
- if compute_word_confidence or no_speech_threshold is not None:
- # no voice activity check
- should_skip = (
- (no_speech_prob > no_speech_threshold)
- if (no_speech_threshold is not None)
- else False
- )
- if compute_word_confidence or (
- should_skip and logprob_threshold is not None
- ):
- n = len(chunk_logprobs)
- if n == len(chunk_tokens_nosot):
- chunk_tokens_nosot = chunk_tokens_nosot[1:]
- if unfinished_decoding:
- assert last_token_fallback is not None
- last_tokens = [last_token_fallback]
- timestamped_word_segments[-1][-1][
- "avg_logprob_reliable"
- ] = last_token_reliable
- n += 1
- elif has_reached_decoding_limit():
- # there were segments in the 30sec chunck, and then the LM got stuck
- last_tokens = [torch.argmax(chunk_logprobs[-1]).item()]
- timestamped_word_segments[-1][-1]["avg_logprob_reliable"] = (
- temperature == 0
- )
- else:
- last_tokens = [tokenizer.eot]
- chunck_indices = chunk_tokens_nosot + last_tokens
- assert len(chunk_logprobs) == len(
- chunck_indices
- ), f"{len(chunk_logprobs)} != {len(chunck_indices)}"
- logprobs = torch.cat(
- [
- logprob[i].unsqueeze(0)
- for (logprob, i) in zip(chunk_logprobs, chunck_indices)
- ]
- )
- assert min(
- [p.isfinite().item() for p in logprobs]
- ), f"Got infinite logprob among ({len(logprobs)}) {[(i, tokenizer.decode_with_timestamps([i]), v.item()) for (i,v) in zip(chunck_indices, logprobs)]}"
- sum_logprob = sum(logprobs)
- avg_logprob = sum_logprob / n
- # don't skip if the logprob is high enough, whatever the no_speech_prob is
- if (
- logprob_threshold is not None
- and avg_logprob > logprob_threshold
- ):
- should_skip = False
-
- if should_skip:
- logger.debug(
- f"Skipping last {n_segments-i_start} segments (no_speech_prob {no_speech_prob} > {no_speech_threshold} and avg_logprob {avg_logprob} < {logprob_threshold})"
- )
- index_begin_30sec_chunck -= n_segments - i_start
- segment_tokens = segment_tokens[:i_start] + [segment_tokens[-1]]
- timestamped_word_segments = timestamped_word_segments[:i_start]
- elif compute_word_confidence:
- avg_logprob = avg_logprob.item()
- i_token_end = -1
- for i in range(i_start, n_segments):
- tokens = segment_tokens[i]
- i_token_start = i_token_end + 1
- i_token_end = i_token_start + len(tokens)
- assert (
- chunck_indices[i_token_start:i_token_end] == tokens
- ), f"Inconsistent token list {tokenizer.decode_with_timestamps(chunck_indices[i_token_start:i_token_end])} != {tokenizer.decode_with_timestamps(tokens)}"
- i_token_start += 1 # skip sos (start time)
- if not unfinished_decoding or i != n_segments - 1:
- i_token_end -= 1 # skip eos (end time)
- segment_logprobs.append(logprobs[i_token_start:i_token_end])
- segment_avglogprobs.append(avg_logprob)
- else:
- for i in range(i_start, n_segments):
- segment_logprobs.append(None)
- segment_avglogprobs.append(None)
-
- else:
- for i in range(i_start, n_segments):
- segment_logprobs.append(None)
- segment_avglogprobs.append(None)
-
- if verbose_bugged and not should_skip:
- for segment in timestamped_word_segments[i_start:]:
- for word in segment:
- print_timestamped(word)
-
- # Reset counters
- chunk_tokens = []
- chunk_tokens_nosot = []
- chunk_logprobs = []
- no_speech_prob = None
-
- def hook_attention_weights(layer, ins, outs, index):
- nonlocal segment_attweights
- # In old version of whisper, output is a single tensor
- assert (
- isinstance(outs, tuple) and len(outs) == 2
- ), "whisper seems to be outdated, please update it (pip install --upgrade --no-deps --force-reinstall git+https://github.com/openai/whisper.git)"
- if not has_started:
- return
- w = outs[-1]
- # Only the last attention weights is useful
- if w.shape[-2] > 1:
- w = w[:, :, -1:, :]
- segment_attweights[index].append(w.cpu())
-
- def hook_mfcc(layer, ins, outs):
- nonlocal new_mfcc, mfcc
- new_mfcc = ins[0]
- if mfcc is None:
- mfcc = new_mfcc
-
- def hook_input_tokens(layer, ins, outs):
- nonlocal segment_tokens, sot_index, chunk_tokens, chunk_tokens_nosot, logit_filters, has_started, language, num_inference_steps
- num_inference_steps += 1
-
- curr_tokens = ins[0]
- assert curr_tokens.shape[0] == 1, "Batch decoding is not supported"
- curr_tokens = curr_tokens.squeeze(0)
-
- if is_sot(curr_tokens):
- chunk_prompt = curr_tokens.tolist()
- if language is None:
- if len(curr_tokens) > 1:
- language = tokenizer.decode(curr_tokens[-2:-1])
- language = language[2:-2] # remove trailing "<|" and "|>"
- whisper_options["language"] = language
-
- if (
- verbose
- and not whisper_options["verbose"]
- and len(curr_tokens) > 1
- ):
- # Reproduce whisper verbose (2/2)
- print(
- f"Detected language: {whisper.tokenizer.LANGUAGES[language].title()}"
- )
- sys.stdout.flush()
-
- logit_filters = get_logit_filters(
- model,
- whisper_options,
- prompt=chunk_prompt[1 : -len(tokenizer.sot_sequence)],
- )
-
- may_flush_segment(curr_tokens)
-
- # Get the index of the <|startoftranscript|> tokens (to get proba of silence later)
- if is_sot(curr_tokens):
- has_started = len(curr_tokens) > 1 or not model.is_multilingual
- if no_speech_threshold is not None:
- sot_index = curr_tokens.tolist().index(tokenizer.sot)
- else:
- sot_index = None
-
- # Keep the last token only
- if has_started:
- segment_tokens[-1].append(curr_tokens[-1].item())
-
- # Accumulate tokens
- if has_started:
- chunk_tokens.append(curr_tokens)
- if not is_sot(curr_tokens):
- chunk_tokens_nosot.append(curr_tokens[-1].item())
- else:
- if verbose and not whisper_options["verbose"]:
- # Reproduce whisper verbose (1/2)
- print(
- "Detecting language using up to the first 30 seconds. Use `--language` to specify the language"
- )
-
- embedding_weights = None
-
- def hook_output_logits(layer, ins, outs):
- nonlocal no_speech_prob, chunk_logprobs, segment_tokens, chunk_tokens, chunk_tokens_nosot, last_chunk_token, embedding_weights, has_started
-
- if embedding_weights is None:
- embedding_weights = torch.transpose(
- model.decoder.token_embedding.weight, 0, 1
- ).to(outs[0].dtype)
-
- # Get the probability of silence
- if sot_index is not None:
- logits = (outs[0][sot_index, :] @ embedding_weights).float()
- logits = logits.softmax(dim=-1)
- no_speech_prob = logits[tokenizer.no_speech].item()
-
- # Get the log-probabilities of tokens (we don't know yet which one will be chosen)
- if has_started:
- logits = (outs[0][-1:, :] @ embedding_weights).float()
- tokens = torch.cat(chunk_tokens).unsqueeze(0)
- for logit_filter in logit_filters:
- logit_filter.apply(logits, tokens)
- logits = F.log_softmax(logits.squeeze(0), dim=-1)
- chunk_logprobs.append(logits)
-
- if WHIPSER_GE_20230306 and has_reached_decoding_limit():
- last_chunk_token = torch.argmax(logits).item()
- else:
- last_chunk_token = None
-
- try:
- # Add hooks to the model, to get tokens and attention weights on the fly
- all_hooks = []
- all_hooks.append(model.encoder.conv1.register_forward_hook(hook_mfcc))
- all_hooks.append(
- model.decoder.token_embedding.register_forward_hook(hook_input_tokens)
- )
- nblocks = len(model.decoder.blocks)
- j = 0
- for i, block in enumerate(model.decoder.blocks):
- if i < nblocks - word_alignement_most_top_layers:
- continue
- all_hooks.append(
- block.cross_attn.register_forward_hook(
- lambda layer, ins, outs, index=j: hook_attention_weights(
- layer, ins, outs, index
- )
- )
- )
- j += 1
- if compute_word_confidence or no_speech_threshold is not None:
- all_hooks.append(model.decoder.ln.register_forward_hook(hook_output_logits))
-
- transcription = model.transcribe(audio, **whisper_options)
-
- finally:
- # Remove hooks
- for hook in all_hooks:
- hook.remove()
-
- # Finalize (collect last segment)
- may_flush_segment()
- segment_tokens.pop(-1)
-
- token_special_idx = min(tokenizer.sot, tokenizer.eot)
-
- def filter_tokens(tokens):
- while len(tokens) and tokens[0] >= token_special_idx:
- tokens = tokens[1:]
- while len(tokens) and tokens[-1] >= token_special_idx:
- tokens = tokens[:-1]
- return tokens
-
- assert len(segment_tokens) == len(
- timestamped_word_segments
- ), f"Inconsistent number of segments: tokens ({len(segment_tokens)}) != timestamped_word_segments ({len(timestamped_word_segments)})"
- assert len(segment_avglogprobs) == len(
- segment_tokens
- ), f"Inconsistent number of segments: avg logprobs ({len(segment_avglogprobs)}) != tokens ({len(segment_tokens)})"
- assert len(segment_logprobs) == len(
- segment_tokens
- ), f"Inconsistent number of segments: logprobs ({len(segment_logprobs)}) != tokens ({len(segment_tokens)})"
-
- whisper_segments = transcription["segments"]
- l1 = len(whisper_segments)
- l2 = len(timestamped_word_segments)
- if l1 != l2 and l1 != 0:
- logger.warning(
- f"Inconsistent number of segments: whisper_segments ({l1}) != timestamped_word_segments ({l2})"
- )
- assert (
- l1 == l2 or l1 == 0
- ), f"Inconsistent number of segments: whisper_segments ({l1}) != timestamped_word_segments ({l2})"
-
- logger.debug("Compile results")
- words = []
- for i, (segment, timestamped_words, token, avglogprob, logprobs) in enumerate(
- zip(
- whisper_segments,
- timestamped_word_segments,
- segment_tokens,
- segment_avglogprobs,
- segment_logprobs,
- )
- ):
- timestamped_tokens = filter_tokens(token)
- whisper_tokens = filter_tokens(segment["tokens"])
- if timestamped_tokens != whisper_tokens:
- if len(timestamped_tokens) == len(whisper_tokens) + 1:
- logger.warning(f"An additional token was added on segment {i}")
- elif WHIPSER_GE_20230306 and len(whisper_tokens) == 0:
- logger.warning(f"Whisper has empty segment {i}")
- assert (
- segment["end"] == segment["start"]
- ), f"Fatal Error: Got empty segment {i} with non-zero duration"
- segment["tokens"] = timestamped_tokens
- segment["text"] = tokenizer.decode(timestamped_tokens)
- else:
- assert (
- len(timestamped_tokens) < len(whisper_tokens)
- and timestamped_tokens == whisper_tokens[: len(timestamped_tokens)]
- ), f"Fatal Error: Got inconsistent text for segment {i}:\n({len(timestamped_tokens)})\n{tokenizer.decode_with_timestamps(timestamped_tokens)}\n{timestamped_tokens}\n!=\n({len(whisper_tokens)})\n{tokenizer.decode_with_timestamps(whisper_tokens)}\n{whisper_tokens[:len(timestamped_tokens)]}"
- segment["tokens"] = (
- token if WHIPSER_GE_20230306 else timestamped_tokens
- ) # tokens include special timestamp tokens since 20230306
- segment["text"] = tokenizer.decode(segment["tokens"])
- logger.warning(
- f"Text had to be shortned on segment {i}:\n{tokenizer.decode(timestamped_tokens)}\n!=\n{tokenizer.decode(whisper_tokens)}"
- )
- timestamped_words[-1]["avg_logprob_reliable"] = False
-
- offset = segment["seek"] * HOP_LENGTH / SAMPLE_RATE
- for timestamped_word in timestamped_words:
- timestamped_word["start"] += offset
- timestamped_word["end"] += offset
- timestamped_word["idx_segment"] = i
-
- if compute_word_confidence:
- if (
- "avg_logprob_reliable" not in timestamped_words[-1]
- or timestamped_words[-1]["avg_logprob_reliable"]
- ):
- # assert abs(segment["avg_logprob"] - avglogprob) < 1e-2, f"Fatal Error: Got inconsistent logprob for segment {i}: {segment['avg_logprob']} != {avglogprob}"
- if abs(segment["avg_logprob"] - avglogprob) >= 1e-2:
- logger.warning(
- f"Recomputed different logprob for segment {i}: {avglogprob} != {segment['avg_logprob']}"
- )
- if include_punctuation_in_confidence:
- segment["confidence"] = round_confidence(logprobs.mean().exp().item())
- else:
- logprobs_nopunc = []
- i_end = 0
- for timestamped_word in timestamped_words:
- i_start = i_end
- tokens = timestamped_word["tokens"]
- i_end += len(tokens)
-
- assert i_end <= len(
- logprobs
- ), f"Fatal Error: Got out-of-bound index for segment {i}: {i_end} > {len(logprobs)}"
- if include_punctuation_in_confidence:
- word_logprobs = logprobs[i_start:i_end]
- else:
- while (
- len(tokens) > 1
- and len(tokens[-1])
- and tokens[-1][-1] in _punctuation
- ): # Note: look at the last character of token, to take into account "...", "!!", etc.
- tokens = tokens[:-1]
- word_logprobs = logprobs[i_start : i_start + len(tokens)]
- logprobs_nopunc.append(word_logprobs)
-
- timestamped_word["confidence"] = round_confidence(
- word_logprobs.mean().exp().item() if len(word_logprobs) else 0.0
- )
-
- if i_end not in [len(logprobs), len(logprobs) - 1]:
- logger.warning(
- f"Got inconsistent length for segment {i} ({len(logprobs)} != {i_end}). Some words have been ignored."
- )
- if not include_punctuation_in_confidence:
- logprobs_nopunc = torch.cat(logprobs_nopunc)
- segment["confidence"] = round_confidence(
- logprobs_nopunc.mean().exp().item()
- )
-
- words.extend(timestamped_words)
-
- return transcription, words
-
-
-def _transcribe_timestamped_naive(
- model,
- audio,
- remove_punctuation_from_words,
- compute_word_confidence,
- include_punctuation_in_confidence,
- refine_whisper_precision_nframes,
- alignment_heads,
- plot_word_alignment,
- word_alignement_most_top_layers,
- detect_disfluencies,
- trust_whisper_timestamps,
- min_word_duration,
- **whisper_options,
-):
- verbose = whisper_options["verbose"]
- whisper_options["verbose"] = (
- None if whisper_options["verbose"] is True else whisper_options["verbose"]
- ) # We will print intermediate results ourselves
- language = whisper_options["language"]
- refine_whisper_precision_sec = (
- refine_whisper_precision_nframes * AUDIO_TIME_PER_TOKEN
- )
-
- word_alignement_most_top_layers = (
- float("inf")
- if word_alignement_most_top_layers is None
- else word_alignement_most_top_layers
- )
-
- audio = get_audio_tensor(audio)
- audio_duration = audio.shape[-1] / SAMPLE_RATE
-
- if verbose and language is None and not whisper_options["verbose"]:
- # Reproduce whisper verbose (1/2)
- print(
- "Detecting language using up to the first 30 seconds. Use `--language` to specify the language"
- )
-
- transcription = model.transcribe(audio, **whisper_options)
-
- if verbose and language is None and not whisper_options["verbose"]:
- # Reproduce whisper verbose (2/2)
- print(
- f"Detected language: {whisper.tokenizer.LANGUAGES[transcription['language']].title()}"
- )
- sys.stdout.flush()
-
- language = norm_language(transcription["language"])
-
- tokenizer = whisper.tokenizer.get_tokenizer(
- model.is_multilingual, task=whisper_options["task"], language=language
- )
- use_space = should_use_space(language)
-
- attention_weights = [
- []
- for _ in range(min(word_alignement_most_top_layers, len(model.decoder.blocks)))
- ]
-
- try:
- all_hooks = []
-
- # Hook the model
- nblocks = len(model.decoder.blocks)
- j = 0
- for i, block in enumerate(model.decoder.blocks):
- if i < nblocks - word_alignement_most_top_layers:
- continue
- all_hooks.append(
- block.cross_attn.register_forward_hook(
- lambda layer, ins, outs, index=j: attention_weights.__setitem__(
- index, outs[-1]
- )
- )
- )
- j += 1
-
- # When not relying on Whisper timestamps
- current_tokens = []
- token_to_idx_segment = []
-
- words = []
- previous_end = 0
- whisper_segments = transcription["segments"]
- for i_segment, segment in enumerate(whisper_segments):
- # Note: this could also be a fix to issue #61 where a "<|te|>" token was predicted
- # segment["tokens"] = [t for t in segment["tokens"] if t < tokenizer.eot or t >= tokenizer.timestamp_begin]
-
- start = end = tokens = None
- if trust_whisper_timestamps:
- start = segment["start"]
- end = segment["end"]
- if end < start:
- # Whisper is wrong on the prediction of segment end
- end = min(audio_duration, start + SEGMENT_DURATION)
-
- start_margin_min = start - refine_whisper_precision_sec
- start_margin_max = start + refine_whisper_precision_sec
- if start >= audio_duration - min_word_duration or (
- previous_end >= start_margin_min
- and previous_end <= start_margin_max
- ):
- # Make start as accurate as possible (as the decoding will start with timestamp <|0|>)
- start = previous_end
- else:
- # Fallback
- start = start_margin_min
-
- if start > audio_duration - min_word_duration:
- # Skip last segment if too short
- logger.warning(
- f"Skipping segment outside of audio duration {audio_duration} (original: {segment['start']}-{segment['end']}, new: {start}-XXX)"
- )
- continue
-
- end_margin_min = end - refine_whisper_precision_sec
- end_margin_max = end + refine_whisper_precision_sec
- if i_segment < len(whisper_segments) - 1:
- # Try to enforce:
- # end + min_word_duration <= next start + refine_whisper_precision_sec
- end_margin_max2 = (
- whisper_segments[i_segment + 1]["start"]
- + refine_whisper_precision_sec
- - min_word_duration
- )
- if end_margin_max2 >= end_margin_min:
- end_margin_max = min(end_margin_max2, end_margin_max)
- end = min(audio_duration, end_margin_max)
-
- if end < start + min_word_duration:
- logger.warning(
- f"Got super short segment (original from whisper: {segment['start']}-{segment['end']}, new: {start, end})"
- )
- end = min(audio_duration, start + min_word_duration)
- if end <= start:
- logger.warning(
- f"Skipping this short segment occuring too close to the end of the audio"
- )
- continue
-
- tokens = segment["tokens"]
-
- else:
- seek = segment["seek"]
- new_tokens = segment["tokens"]
- if not len(new_tokens):
- continue
- # Add timestamps that will be needed after
- if new_tokens[0] < tokenizer.timestamp_begin:
- relative_start = segment["start"] - (
- seek * HOP_LENGTH / SAMPLE_RATE
- )
- start_token = (
- round(relative_start * SAMPLE_RATE / AUDIO_SAMPLES_PER_TOKEN)
- + tokenizer.timestamp_begin
- )
- new_tokens = [start_token] + new_tokens
- if new_tokens[-1] < tokenizer.timestamp_begin:
- relative_end = segment["end"] - (seek * HOP_LENGTH / SAMPLE_RATE)
- end_token = (
- round(relative_end * SAMPLE_RATE / AUDIO_SAMPLES_PER_TOKEN)
- + tokenizer.timestamp_begin
- )
- new_tokens = new_tokens + [end_token]
-
- current_tokens.extend(new_tokens)
- token_to_idx_segment.extend([i_segment] * len(new_tokens))
-
- next_seek = (
- whisper_segments[i_segment + 1]["seek"]
- if i_segment < len(whisper_segments) - 1
- else None
- )
- if seek != next_seek:
- start = float(seek * HOP_LENGTH / SAMPLE_RATE)
- assert (
- start < audio_duration
- ), f"Got start {start} which is outside of audio duration {audio_duration}"
- end = min(start + SEGMENT_DURATION, audio_duration)
- tokens = current_tokens
-
- if tokens is None or not len(tokens):
- continue
-
- start_sample = min(round(start * SAMPLE_RATE), audio.shape[-1])
- end_sample = min(round(end * SAMPLE_RATE), audio.shape[-1])
-
- # Extract features on the audio segment
- sub_audio = audio_minimum_padding(audio[start_sample:end_sample])
-
- mfcc = whisper.log_mel_spectrogram(sub_audio).to(model.device)
- mfcc = whisper.pad_or_trim(mfcc, N_FRAMES)
- mfcc = mfcc.unsqueeze(0)
-
- segment_tokens_check = []
- if tokens[0] >= tokenizer.timestamp_begin:
- segment_tokens_check.append(tokens[0])
- while tokens[0] >= tokenizer.timestamp_begin:
- tokens = tokens[1:]
- assert len(tokens), "Got transcription with only timestamps!"
- last_token_check = None
- while tokens[-1] >= tokenizer.timestamp_begin:
- last_token_check = tokens[-1]
- tokens = tokens[:-1]
-
- tokens = [
- *tokenizer.sot_sequence,
- tokenizer.timestamp_begin,
- ] + tokens
-
- i_start = len(tokenizer.sot_sequence)
-
- with torch.no_grad():
- logprobs = model(
- mfcc, torch.Tensor(tokens).int().to(model.device).unsqueeze(0)
- )
- logprobs = F.log_softmax(logprobs, dim=-1)
-
- end_token = tokenizer.timestamp_begin + round(
- min(N_FRAMES * HOP_LENGTH, end_sample - start_sample)
- // AUDIO_SAMPLES_PER_TOKEN
- )
- tokens = tokens[i_start:] + [end_token]
- attention_weights = [w[:, :, i_start - 1 :, :] for w in attention_weights]
-
- ws = perform_word_alignment(
- tokens,
- attention_weights,
- tokenizer,
- use_space=use_space,
- alignment_heads=alignment_heads,
- remove_punctuation_from_words=remove_punctuation_from_words,
- refine_whisper_precision_nframes=refine_whisper_precision_nframes,
- detect_disfluencies=detect_disfluencies,
- mfcc=mfcc,
- plot=plot_word_alignment,
- )
-
- segment_logprobs = []
- i_token = 1
-
- for word in ws:
- word["start"] = round(word["start"] + start, 2)
- word["end"] = round(word["end"] + start, 2)
-
- if trust_whisper_timestamps:
- word.update({"idx_segment": i_segment})
- else:
- assert i_token < len(tokens)
- assert (
- not len(word["tokens_indices"])
- or word["tokens_indices"][0] == tokens[i_token]
- )
- word.update({"idx_segment": token_to_idx_segment[i_token]})
- i_token += len(word["tokens"])
- while (
- i_token < len(tokens)
- and tokens[i_token] >= tokenizer.timestamp_begin
- ):
- i_token += 1
-
- tok_indices = word["tokens_indices"]
- segment_tokens_check.extend(tok_indices)
-
- if compute_word_confidence:
- tok = word["tokens"]
- i_end = i_start + len(tok)
- if include_punctuation_in_confidence:
- while (
- len(tok) > 1
- and len(tok[-1])
- and tok[-1][-1] in _punctuation
- ): # Note: look at the last character of token, to take into account "...", "!!", etc.
- tok = tok[:-1]
- tok_indices = tok_indices[:-1]
- word_logprobs = [
- logprobs[:, step, tok]
- for (step, tok) in zip(
- range(i_start, i_start + len(tok_indices)), tok_indices
- )
- ]
- i_start = i_end
- if len(word_logprobs):
- word_logprobs = torch.cat(word_logprobs)
- segment_logprobs.append(word_logprobs)
- word_confidence = word_logprobs.mean().exp().item()
- else:
- word_confidence = 0
- word.update({"confidence": round_confidence(word_confidence)})
-
- words.append(word)
-
- if verbose:
- print_timestamped(word)
-
- if last_token_check is not None:
- segment_tokens_check.append(last_token_check)
- if trust_whisper_timestamps:
- if segment_tokens_check != segment["tokens"]:
- assert (
- len(segment_tokens_check) < len(segment["tokens"])
- and segment_tokens_check[:-1]
- == segment["tokens"][: len(segment_tokens_check) - 1]
- ), f"Got inconsistent tokens: {tokenizer.decode(segment_tokens_check)} != {tokenizer.decode(segment['tokens'])}"
- segment["tokens"] = segment_tokens_check
- segment["text"] = tokenizer.decode(segment["tokens"])
- # else: TODO
-
- if len(segment_logprobs):
- segment.update(
- {
- "confidence": round_confidence(
- torch.cat(segment_logprobs).mean().exp().item()
- )
- }
- )
-
- if len(ws):
- previous_end = ws[-1]["end"]
-
- if not trust_whisper_timestamps:
- current_tokens = []
- token_to_idx_segment = []
-
- finally:
- # Remove hooks
- for hook in all_hooks:
- hook.remove()
-
- return (transcription, words)
-
-
-def get_audio_tensor(audio, device="cpu"):
- if isinstance(audio, str):
- audio = whisper.load_audio(audio)
- if isinstance(audio, np.ndarray):
- audio = torch.Tensor(audio)
- else:
- assert isinstance(
- audio, torch.Tensor
- ), f"Got unexpected audio of type {type(audio)}"
- return audio.to(device)
-
-
-def audio_minimum_padding(audio):
- if audio.shape[-1] <= 200:
- return whisper.pad_or_trim(audio, 201)
- return audio
-
-
-def should_use_space(language):
- return norm_language(language) not in ["zh", "ja", "th", "lo", "my"]
-
-
-def norm_language(language):
- if language is None:
- return "en"
- return whisper.tokenizer.TO_LANGUAGE_CODE.get(language.lower(), language)
-
-
-def print_timestamped(w):
- line = f"[{format_timestamp(w['start'])} --> {format_timestamp(w['end'])}] {w['text']}\n"
- # compared to just `print(line)`, this replaces any character not representable using
- # the system default encoding with an '?', avoiding UnicodeEncodeError.
- sys.stdout.buffer.write(line.encode(sys.getdefaultencoding(), errors="replace"))
- sys.stdout.flush()
-
-
-def get_logit_filters(model, whisper_options, prompt=None):
- decoding_options = get_decoding_options(whisper_options)
- if "initial_prompt" in decoding_options:
- prompt0 = decoding_options.pop("initial_prompt")
- if prompt is None:
- prompt = prompt0
- if prompt is not None:
- decoding_options["prompt"] = prompt
- decoding_options = whisper.DecodingOptions(
- without_timestamps=False,
- max_initial_timestamp=1.0,
- prefix=None,
- suppress_blank=True,
- **decoding_options,
- )
-
- # This performs some checks on the options
- decoding_task = whisper.decoding.DecodingTask(model, decoding_options)
- return decoding_task.logit_filters
-
-
-def get_decoding_options(whisper_options):
- return dict(
- [
- (k, v)
- for (k, v) in whisper_options.items()
- if k
- not in [
- "no_speech_threshold",
- "logprob_threshold",
- "compression_ratio_threshold",
- "condition_on_previous_text",
- "verbose",
- ]
- ]
- )
-
-
-def perform_word_alignment(
- tokens,
- attention_weights,
- tokenizer,
- use_space=True,
- mfcc=None,
- refine_whisper_precision_nframes=0,
- remove_punctuation_from_words=False,
- include_punctuation_in_timing=False, # Was True before 1.9
- unfinished_decoding=False,
- alignment_heads=None,
- medfilt_width=9,
- qk_scale=1.0,
- detect_disfluencies=True,
- subwords_can_be_empty=True, # Was False before 1.11
- plot=False,
- debug=False,
-):
- """
- Perform word alignment on the given tokens and attention weights.
- Returns a list of (word, start_time, end_time) tuples.
-
- tokens: list of tokens (integers)
- attention_weights: list of attention weights (torch tensors)
- tokenizer: tokenizer used to tokenize the text
- use_space: whether to use spaces to split the tokens into words (should be true for all languages except Japanese, Chinese, ...)
- mfcc: MFCC features (used to identify padded region, and for plotting)
- refine_whisper_precision_nframes: precision time
- remove_punctuation_from_words: whether to remove punctuation from words
- include_punctuation_in_timing: whether to include punctuation in the timing of (previous) words
- unfinished_decoding: whether the decoding is unfinished (e.g. because the model is stuck)
- alignment_heads: list of attention heads to use for alignment
- medfilt_width: width of the median filter used to smooth the attention weights
- qk_scale: scale factor applied to the attention weights
- plot: whether to plot the word alignment
- debug: whether to print debug information
- """
-
- assert (
- len(tokens) > 1
- ), f"Got unexpected sequence of tokens of length {len(tokens)} {tokenizer.decode_with_timestamps(tokens)}"
- start_token = tokens[0] - tokenizer.timestamp_begin
- end_token = tokens[-1] - tokenizer.timestamp_begin
-
- # Check start / end tokens
- if start_token < 0:
- raise RuntimeError(
- f"Missing start token in: {tokenizer.decode_with_timestamps(tokens)}"
- )
- if len(tokens) == 1 or end_token < 0:
- # This can happens when Whisper is stucked as a Language Model
- if debug:
- logger.debug(
- f"Missing end token in {tokenizer.decode_with_timestamps(tokens)}"
- )
- end_token = N_FRAMES // 2
- if end_token == start_token and refine_whisper_precision_nframes == 0:
- if debug:
- logger.debug(
- f"Got empty segment in {tokenizer.decode_with_timestamps(tokens)}"
- )
- return []
-
- # Let a minimal duration given the number of tokens (see https://github.com/linto-ai/whisper-timestamped/issues/67)
- end_token = min(N_FRAMES // 2, max(end_token, start_token + len(tokens)))
-
- # Put some margin around the segment
- if refine_whisper_precision_nframes > 0:
- start_token = max(start_token - refine_whisper_precision_nframes, 0)
- end_token = min(end_token + refine_whisper_precision_nframes, N_FRAMES // 2)
-
- if end_token <= start_token:
- raise RuntimeError(
- f"Got segment with null or negative duration {tokenizer.decode_with_timestamps(tokens)}: {start_token} {end_token}"
- )
-
- start_time = start_token * AUDIO_TIME_PER_TOKEN
- # end_time = end_token * AUDIO_TIME_PER_TOKEN
-
- split_tokens = split_tokens_on_spaces if use_space else split_tokens_on_unicode
- words, word_tokens, word_tokens_indices = split_tokens(
- tokens, tokenizer, remove_punctuation_from_words=remove_punctuation_from_words
- )
-
- # If the last token is a punctuation that comes after a word
- # group this final punctuation with the final timestamp
- # This is to avoid assigning the final punctuation to a big silence or a noise/music background coming after
- num_punctuations_per_tokens = [
- 0 if len(w) == 1 or w[-1] not in _punctuation else 1 for w in word_tokens
- ]
- if include_punctuation_in_timing:
- num_punctuations_per_tokens[:-2] = [0] * (len(num_punctuations_per_tokens) - 2)
-
- for i, w in enumerate(attention_weights):
- assert w.shape[-2] == len(
- tokens
- ), f"Attention weights have wrong shape: {w.shape[-2]} (expected {len(tokens)})."
- weights = torch.cat(attention_weights) # layers * heads * tokens * frames
-
- num_tokens = weights.shape[-2]
- num_frames = end_token - start_token
- if num_tokens > num_frames:
- logger.warning(
- f"Too much text ({num_tokens} tokens) for the given number of frames ({num_frames}) in: {tokenizer.decode_with_timestamps(tokens)}\nThe end of the text will be removed."
- )
- return perform_word_alignment(
- tokens[: num_frames - 1] + [tokens[-1]],
- [
- torch.cat([w[:, :, : num_frames - 1, :], w[:, :, -1:, :]], dim=-2)
- for w in attention_weights
- ],
- tokenizer,
- use_space=use_space,
- refine_whisper_precision_nframes=refine_whisper_precision_nframes,
- medfilt_width=medfilt_width,
- qk_scale=qk_scale,
- alignment_heads=alignment_heads,
- mfcc=mfcc,
- plot=plot,
- remove_punctuation_from_words=remove_punctuation_from_words,
- detect_disfluencies=detect_disfluencies,
- subwords_can_be_empty=subwords_can_be_empty,
- unfinished_decoding=True,
- debug=debug,
- )
-
- assert end_token <= weights.shape[-1]
- assert len(tokens) == num_tokens
-
- weights = weights[
- ..., start_token:end_token
- ].cpu() # layers * heads * tokens * frames
-
- if alignment_heads is None:
- weights = weights.reshape(-1, *weights.shape[-2:]) # N * tokens * frames
- else:
- weights = torch.stack([weights[l][h] for l, h in alignment_heads.indices().T])
- weights = median_filter(weights, (1, 1, medfilt_width))
- weights = torch.tensor(weights * qk_scale).softmax(dim=-1)
- weights = weights.mean(
- axis=(0)
- ) # average over layers and heads # tokens * frames
- weights = weights / weights.norm(
- dim=-2, keepdim=True
- ) # This was before the mean before 1.9
- weights = -weights.double().numpy()
- worse_weight = 0
-
- # Get the limit of audio duration
- max_duration = None
- if mfcc is not None:
- max_duration = find_start_padding(mfcc)
- if max_duration is not None:
- max_duration = max_duration // 2
-
- # Enforce the max duration
- if max_duration:
- if start_token >= max_duration:
- logger.warning(f"Got start time outside of audio boundary")
- else:
- weights[:-1, max_duration:] = worse_weight
-
- # Encourage to start early
- weights[0, 0] = weights.min()
- # weights[0, refine_whisper_precision_nframes*2:] = worse_weight
-
- if subwords_can_be_empty:
- step_pattern = dtw.stepPattern.symmetric1
- else:
- # Similar as "symmetric1" but without the possibility to have the same timestamp for two tokens
- step_pattern = dtw.stepPattern.StepPattern(
- dtw.stepPattern._c(
- 1,
- 1,
- 1,
- -1,
- 1,
- 0,
- 0,
- 1,
- 2,
- 0,
- 1,
- -1,
- 2,
- 0,
- 0,
- 1,
- )
- )
- alignment = dtw.dtw(weights, step_pattern=step_pattern)
-
- global num_alignment_for_plot
- num_alignment_for_plot += 1
-
- if plot:
- import matplotlib.pyplot as plt
- import matplotlib.ticker as ticker
-
- plot_mfcc = 1 if mfcc is not None else 0
- plot_disfluencies = 1 if detect_disfluencies else 0
- nplots = 1 + plot_mfcc + plot_disfluencies
-
- plt.subplots(
- nplots,
- 1,
- figsize=(16, 9),
- gridspec_kw={"height_ratios": [3] + [1] * (nplots - 1)},
- )
- plt.subplot(nplots, 1, 1, frameon=False)
-
- plt.imshow(-weights, aspect="auto")
- plt.plot(alignment.index2s, alignment.index1s, color="red")
-
- xticks = np.arange(0, weights.shape[1], 1 / AUDIO_TIME_PER_TOKEN)
- xticklabels = [
- round_timestamp(x) for x in xticks * AUDIO_TIME_PER_TOKEN + start_time
- ]
-
- ylims = plt.gca().get_ylim()
-
- ax = plt.gca()
- ax.tick_params("both", length=0, width=0, which="minor", pad=6)
-
- ax.yaxis.set_ticks_position("left")
- ax.yaxis.set_label_position("left")
- ax.invert_yaxis()
- ax.set_ylim(ylims)
-
- major_ticks = [-0.5]
- minor_ticks = []
- current_y = 0
-
- for word, word_token in zip(words, word_tokens):
- minor_ticks.append(current_y + len(word_token) / 2 - 0.5)
- current_y += len(word_token)
- major_ticks.append(current_y - 0.5)
-
- words_with_subwords = [
- "|".join(s).strip() for (w, s) in zip(words, word_tokens)
- ]
-
- ax.yaxis.set_minor_locator(ticker.FixedLocator(minor_ticks))
- ax.yaxis.set_minor_formatter(ticker.FixedFormatter(words_with_subwords))
- ax.set_yticks(major_ticks)
- ax.yaxis.set_major_formatter(ticker.NullFormatter())
- for y in major_ticks:
- plt.axhline(y, color="black", linestyle="dashed")
-
- plt.ylabel("Words")
-
- if plot_mfcc:
- plt.xticks(xticks)
- plt.setp(plt.gca().get_xticklabels(), visible=False)
-
- xticks *= 2
-
- plt.subplot(nplots, 1, 2, frameon=False)
- plt.imshow(
- mfcc[0, :, start_token * 2 : end_token * 2].cpu(),
- aspect="auto",
- origin="lower",
- )
- plt.yticks([])
- plt.ylabel("MFCC")
-
- plt.xticks(xticks, xticklabels)
- plt.xlabel("Time (s)")
-
- jumps = np.diff(alignment.index1s)
- jumps = np.pad(jumps, (1, 0), constant_values=1)
- jumps = jumps.astype(bool)
- jumps = alignment.index2s[jumps]
- jumps = np.pad(jumps, (0, 1), constant_values=alignment.index2s[-1])
-
- jumps_start = jumps
- disfluences = {}
- if detect_disfluencies:
- jumps_start = copy.copy(jumps)
-
- for i_token, (tok, begin, end) in enumerate(zip(tokens, jumps[:-1], jumps[1:])):
- # Find local maxima in the portion of attention weights
- attention_weights = -weights[i_token, begin:end]
- peaks, properties = find_peaks(
- attention_weights,
- width=3,
- prominence=0.02,
- )
- # If more than
- if len(peaks) > 1:
- if "left_ips" in properties:
- left = [round(x) for x in properties["left_ips"]]
- else:
- left = properties["left_bases"]
-
- new_begin = left[-1] + begin
-
- jumps_start[i_token] = new_begin
-
- if new_begin != begin:
- is_punctuation = (
- tokenizer.decode_with_timestamps([tok]) in _punctuation
- )
- if not is_punctuation:
- disfluences[i_token] = (begin, jumps_start[i_token])
- else:
- disfluences[i_token + 1] = (begin, end)
-
- if plot:
- plt.subplot(nplots, 1, 2 + plot_mfcc, frameon=False)
- plt.plot(range(begin, end), attention_weights)
- plt.xlim(0, end)
-
- for i, p in enumerate(peaks):
- color = (
- "red" if (len(peaks) > 1 and i < len(peaks) - 1) else "green"
- )
- plt.vlines(begin + p, 0, 1, color=color, linestyle="--")
-
- if "left_bases" in properties:
-
- def barxxy(start, end, y, **kwargs):
- middle = (start + end) / 2
- plt.bar(middle, y, width=end - start, **kwargs)
-
- color = "red" if len(peaks) > 1 else "green"
- barxxy(
- begin + properties["left_bases"],
- begin + properties["right_bases"],
- properties.get(
- "prominences", [1] * len(properties["left_bases"])
- ),
- alpha=0.5,
- # put a line with a custom color
- linewidth=1,
- edgecolor=color,
- )
- if "left_ips" in properties:
- for left in properties["left_ips"]:
- plt.vlines(begin + left, 0, 0.5, color="green", linestyle=":")
- for right in properties["right_ips"]:
- plt.vlines(begin + right, 0, 0.5, color="red", linestyle=":")
-
- # display the word-level timestamps in a table
- word_boundaries = np.cumsum([len(t) for t in word_tokens])
- word_boundaries = np.pad(word_boundaries, (1, 0))
- begin_times = jumps_start[word_boundaries[:-1]]
- end_times = jumps[word_boundaries[1:] - num_punctuations_per_tokens]
-
- begin_times = begin_times * AUDIO_TIME_PER_TOKEN
- end_times = end_times * AUDIO_TIME_PER_TOKEN
-
- if detect_disfluencies:
- to_be_added = []
- i_start = 0
- for i_word, toks in enumerate(word_tokens[:-1]):
- i_end = i_start + len(toks)
- if i_start in disfluences and i_word > 0:
- begin, end = disfluences[i_start]
- begin *= AUDIO_TIME_PER_TOKEN
- end *= AUDIO_TIME_PER_TOKEN
- to_be_added.append((i_word, begin, end))
- i_start = i_end
- # Add from the end to avoid messing up the indices
- for i_word, begin, end in to_be_added[-1::-1]:
- words.insert(i_word, DISFLUENCY_MARK)
- word_tokens.insert(i_word, [])
- word_tokens_indices.insert(i_word, [])
- begin_times = np.insert(begin_times, i_word, begin)
- end_times = np.insert(end_times, i_word, end)
-
- # Ignore start / end tokens
- if not refine_whisper_precision_nframes:
- begin_times[1] = begin_times[0]
- if not refine_whisper_precision_nframes:
- end_times[-2] = end_times[-1]
- if unfinished_decoding:
- words = words[1:]
- word_tokens = word_tokens[1:]
- word_tokens_indices = word_tokens_indices[1:]
- begin_times = begin_times[1:]
- end_times = end_times[1:]
- else:
- words = words[1:-1]
- word_tokens = word_tokens[1:-1]
- word_tokens_indices = word_tokens_indices[1:-1]
- begin_times = begin_times[1:-1]
- end_times = end_times[1:-1]
-
- if plot:
- ymin = 1
-
- plt.subplot(nplots, 1, 1)
- for i, (w, ws, begin, end) in enumerate(
- zip(words, word_tokens, begin_times, end_times)
- ):
- ymax = ymin + len(ws)
- if mfcc is None:
- plt.text(
- begin / AUDIO_TIME_PER_TOKEN,
- num_tokens - 0.5,
- w,
- ha="left",
- va="top",
- color="red",
- )
- for x in [
- begin,
- end,
- ]:
- plt.axvline(
- x / AUDIO_TIME_PER_TOKEN,
- color="red",
- linestyle="dotted",
- ymin=1 - ymin / num_tokens,
- ymax=0, # 1-ymax/num_tokens,
- )
- ymin = ymax
-
- if plot_mfcc:
- plt.subplot(nplots, 1, 2)
- for i, (w, begin, end) in enumerate(zip(words, begin_times, end_times)):
- plt.text(
- begin * 2 / AUDIO_TIME_PER_TOKEN,
- mfcc.shape[-2] * 1.05,
- w,
- ha="left",
- va="bottom",
- color="red",
- )
- for x in [
- begin,
- end,
- ]:
- plt.axvline(
- x * 2 / AUDIO_TIME_PER_TOKEN, color="red", linestyle="dotted"
- )
-
- if isinstance(plot, str):
- plt.savefig(
- f"{plot}.alignment{num_alignment_for_plot:03d}.jpg",
- bbox_inches="tight",
- pad_inches=0,
- )
- else:
- plt.show()
-
- return [
- dict(
- text=word,
- start=round_timestamp(begin + start_time),
- end=round_timestamp(end + start_time),
- tokens=tokens,
- tokens_indices=tokens_indices,
- )
- for word, begin, end, tokens, tokens_indices in zip(
- words, begin_times, end_times, word_tokens, word_tokens_indices
- )
- if not word.startswith("<|")
- ]
-
-
-def find_start_padding(mfcc):
- """Return start of padding given the mfcc, or None if there is no padding"""
- last_mfcc = mfcc[0, :, -1]
- if torch.min(last_mfcc) == torch.max(last_mfcc) == 0:
- candidate_index = mfcc.shape[-1] - 2
- while candidate_index > 0:
- candidate = mfcc[0, :, candidate_index]
- if not torch.equal(candidate, last_mfcc):
- return candidate_index + 1
- candidate_index -= 1
- return 0 # WTF!?
-
-
-def round_confidence(x):
- return round(x, 3)
-
-
-def round_timestamp(x):
- return round(x, 2)
-
-
-_punctuation = (
- "".join(c for c in string.punctuation if c not in ["-", "'"]) + "。,!?:”、…"
-)
-
-
-def split_tokens_on_unicode(
- tokens: list,
- tokenizer,
- remove_punctuation_from_words=False,
- isolate_punctuations=False,
-):
- words = []
- word_tokens = []
- word_tokens_indices = []
- current_tokens = []
-
- for token in tokens:
- current_tokens.append(token)
- decoded = tokenizer.decode_with_timestamps(
- [
- t
- for t in current_tokens
- if t < tokenizer.eot or t >= tokenizer.timestamp_begin
- ]
- )
- if "\ufffd" not in decoded:
- empty_tokens = [""] * (len(current_tokens) - 1)
- punctuation = not isolate_punctuations and (
- decoded.strip() and decoded.strip() in _punctuation
- )
- previous_special = len(word_tokens_indices) > 0 and (
- word_tokens_indices[-1][-1] >= tokenizer.timestamp_begin
- )
- if punctuation and not previous_special:
- if len(words) == 0:
- words = [""]
- word_tokens = [[]]
- if not remove_punctuation_from_words:
- words[-1] += decoded
- word_tokens[-1].extend(empty_tokens + [decoded])
- word_tokens_indices[-1].extend(current_tokens)
- else:
- words.append(decoded)
- word_tokens.append(empty_tokens + [decoded])
- word_tokens_indices.append(current_tokens)
- current_tokens = []
-
- return words, word_tokens, word_tokens_indices
-
-
-def split_tokens_on_spaces(
- tokens: torch.Tensor, tokenizer, remove_punctuation_from_words=False
-):
- (
- subwords,
- subword_tokens_list,
- subword_tokens_indices_list,
- ) = split_tokens_on_unicode(
- tokens, tokenizer, remove_punctuation_from_words=remove_punctuation_from_words
- )
- words = []
- word_tokens = []
- word_tokens_indices = []
-
- for i, (subword, subword_tokens, subword_tokens_indices) in enumerate(
- zip(subwords, subword_tokens_list, subword_tokens_indices_list)
- ):
- special = subword_tokens_indices[0] >= tokenizer.timestamp_begin
- previous_special = (i > 0) and (
- subword_tokens_indices_list[i - 1][0] >= tokenizer.timestamp_begin
- )
- next_special = (i < len(subword_tokens_indices_list) - 1) and (
- subword_tokens_indices_list[i + 1][0] >= tokenizer.timestamp_begin
- )
- previous_space = (i > 0) and (not subwords[i - 1].strip())
- is_space = not subword.strip()
- with_space = subword.startswith(" ") and not is_space
- punctuation = not is_space and subword.strip() in _punctuation
- if special or (
- not previous_space
- and (
- previous_special
- or (with_space and not punctuation)
- or (is_space and not next_special)
- )
- ):
- words.append(subword.strip())
- word_tokens.append(subword_tokens)
- word_tokens_indices.append(subword_tokens_indices)
- else:
- words[-1] = words[-1] + subword.strip()
- word_tokens[-1].extend(subword_tokens)
- word_tokens_indices[-1].extend(subword_tokens_indices)
-
- return words, word_tokens, word_tokens_indices
-
-
-silero_vad_model = None
-
-
-def get_vad_segments(
- audio,
- output_sample=False,
- min_speech_duration=0.1,
- min_silence_duration=0.1,
- dilatation=0.5,
-):
- """
- Get speech segments from audio using Silero VAD
- parameters:
- audio: torch.Tensor
- audio data *in 16kHz*
- output_sample: bool
- if True, return start and end in samples instead of seconds
- min_speech_duration: float
- minimum duration (in sec) of a speech segment
- min_silence_duration: float
- minimum duration (in sec) of a silence segment
- dilatation: float
- how much (in sec) to enlarge each speech segment detected by the VAD
- """
- global silero_vad_model, silero_get_speech_ts
-
- if silero_vad_model is None:
- import onnxruntime
-
- onnxruntime.set_default_logger_severity(
- 3
- ) # Remove warning "Removing initializer 'XXX'. It is not used by any node and should be removed from the model."
- repo_or_dir = os.path.expanduser(
- "~/.cache/torch/hub/snakers4_silero-vad_master"
- )
- source = "local"
- if not os.path.exists(repo_or_dir):
- repo_or_dir = "snakers4/silero-vad"
- source = "github"
- silero_vad_model, utils = torch.hub.load(
- repo_or_dir=repo_or_dir, model="silero_vad", onnx=True, source=source
- )
- silero_get_speech_ts = utils[0]
-
- # Cheap normalization of the volume
- audio = audio / max(0.1, audio.abs().max())
-
- segments = silero_get_speech_ts(
- audio,
- silero_vad_model,
- min_speech_duration_ms=round(min_speech_duration * 1000),
- min_silence_duration_ms=round(min_silence_duration * 1000),
- return_seconds=False,
- )
-
- if dilatation > 0:
- dilatation = round(dilatation * SAMPLE_RATE)
- new_segments = []
- for seg in segments:
- new_seg = {
- "start": max(0, seg["start"] - dilatation),
- "end": min(len(audio), seg["end"] + dilatation),
- }
- if len(new_segments) > 0 and new_segments[-1]["end"] >= new_seg["start"]:
- new_segments[-1]["end"] = new_seg["end"]
- else:
- new_segments.append(new_seg)
- segments = new_segments
-
- ratio = 1 if output_sample else 1 / SAMPLE_RATE
-
- if ratio != 1:
- for seg in segments:
- seg["start"] *= ratio
- seg["end"] *= ratio
- if output_sample:
- for seg in segments:
- seg["start"] = round(seg["start"])
- seg["end"] = round(seg["end"])
- return segments
-
-
-def remove_non_speech(
- audio,
- use_sample=False,
- min_speech_duration=0.1,
- min_silence_duration=1,
- plot=False,
-):
- """
- Remove non-speech segments from audio (using Silero VAD),
- glue the speech segments together and return the result along with
- a function to convert timestamps from the new audio to the original audio
- """
-
- segments = get_vad_segments(
- audio,
- output_sample=True,
- min_speech_duration=min_speech_duration,
- min_silence_duration=min_silence_duration,
- )
-
- segments = [(seg["start"], seg["end"]) for seg in segments]
- if len(segments) == 0:
- segments = [(0, audio.shape[-1])]
-
- audio_speech = torch.cat([audio[..., s:e] for s, e in segments], dim=-1)
-
- if plot:
- import matplotlib.pyplot as plt
-
- plt.figure()
- plt.plot(audio)
- for s, e in segments:
- plt.axvspan(s, e, color="red", alpha=0.1)
- if isinstance(plot, str):
- plt.savefig(f"{plot}.VAD.jpg", bbox_inches="tight", pad_inches=0)
- else:
- plt.show()
-
- if not use_sample:
- segments = [
- (float(s) / SAMPLE_RATE, float(e) / SAMPLE_RATE) for s, e in segments
- ]
-
- return audio_speech, lambda t, t2=None: do_convert_timestamps(segments, t, t2)
-
-
-def do_convert_timestamps(segments, t, t2=None):
- """
- Convert timestamp from audio without non-speech segments to original audio (with non-speech segments)
-
- parameters:
- segments: list of tuple (start, end) corresponding to non-speech segments in original audio
- t: timestamp to convert
- t2: second timestamp to convert (optional), when the two timestamps should be in the same segment
- """
- assert len(segments)
- ioffset = 0 # Input offset
- ooffset = 0 # Output offset
- ipreviousend = 0
- result = []
- for istart, iend in segments:
- ostart = ooffset
- oend = ostart + (iend - istart)
- ooffset = oend
- ioffset += istart - ipreviousend
- ipreviousend = iend
- t_in = t <= oend
- t2_in = t_in if t2 is None else t2 <= oend
- if t_in or t2_in:
- result.append(
- [
- max(istart, min(iend, ioffset + t)),
- max(istart, min(iend, ioffset + t2)) if t2 is not None else None,
- ]
- )
- if t_in and t2_in:
- break
- if not len(result):
- result.append([ioffset + t, ioffset + t2 if t2 is not None else None])
-
- if len(result) > 1:
- # Minimize difference between durations
- result = sorted(result, key=lambda x: abs(abs(t2 - t) - abs(x[1] - x[0])))
- result = result[0]
- if t2 is None:
- result = round(result[0], 2)
- else:
- result = [round(x, 2) for x in result]
- return result
-
-
-def remove_last_null_duration_words(transcription, words, recompute_text=False):
- """
- Remove words with null duration happening at the end of a chunk (probable Whisper hallucinations)
- """
- # First group segments by audio chunk
- segments_groups = {}
- seek = None
- current_chunk = -1
- for i, segment in enumerate(transcription["segments"]):
- if segment["seek"] != seek:
- current_chunk += 1
- seek = segment["seek"]
- segments_groups[i] = current_chunk
-
- # Remove words with null duration happening at the end of a chunk
- current_chunk = -1
- is_last_empty = False
- to_remove = []
- for i, word in enumerate(words[::-1]): # Reverse order
- i = len(words) - i - 1
- empty = word["start"] == word["end"]
- idx_segment = word["idx_segment"]
- group = segments_groups[idx_segment]
- if current_chunk != group:
- is_last_empty = empty
- current_chunk = group
- elif not empty:
- is_last_empty = False
- if is_last_empty:
- # Remove word
- to_remove.append(i)
- # Shorten text of segment
- full_word = "".join(word["tokens"])
- logger.debug(
- f"Removing word {i+1}/{len(words)} \"{full_word}\" with empty duration at the end of segment {idx_segment+1}/{len(transcription['segments'])}"
- )
- segment = transcription["segments"][idx_segment]
- text = segment["text"]
- if not text.endswith(full_word): # see issue #62
- if text.endswith(full_word[:-1]):
- full_word = full_word[:-1]
- elif text[:-1].endswith(full_word):
- text = text[:-1]
- else:
- raise RuntimeError(f'"{text}" not ending with "{full_word}"')
- text = text[: -len(full_word)]
- if i > 0 and words[i - 1]["idx_segment"] == idx_segment:
- segment["text"] = text
- else:
- logger.debug(f"Removing empty segment {idx_segment}")
- # Remove segment with no more words
- transcription["segments"].pop(idx_segment)
- for j in range(i + 1, len(words)):
- words[j]["idx_segment"] -= 1
- recompute_text = True
-
- for i in to_remove:
- words.pop(i) # Warning: inplace modification
-
- if recompute_text:
- transcription["text"] = "".join([s["text"] for s in transcription["segments"]])
-
- return transcription, words
-
-
-def ensure_increasing_positions(segments, min_duration=0):
- """
- Ensure that "start" and "end" come in increasing order
- """
- has_modified_backward = False
- previous_end = 0
- for i, seg in enumerate(segments):
- if seg["start"] < previous_end:
- assert i > 0
- new_start = round_timestamp((previous_end + seg["start"]) / 2)
- if new_start < segments[i - 1]["start"] + min_duration:
- new_start = previous_end
- else:
- segments[i - 1]["end"] = new_start
- has_modified_backward = True
- seg["start"] = new_start
- if seg["end"] <= seg["start"] + min_duration:
- seg["end"] = seg["start"] + min_duration
- previous_end = seg["end"]
- if has_modified_backward:
- return ensure_increasing_positions(segments, min_duration)
-
- previous_end = 0
- for seg in segments:
- seg["start"] = round_timestamp(seg["start"])
- seg["end"] = round_timestamp(seg["end"])
- assert (
- seg["start"] >= previous_end
- ), f"Got segment {seg} coming before the previous finishes ({previous_end} > {seg['start']})"
- assert seg["end"] >= seg["start"], f"Got segment {seg} with end < start"
- previous_end = seg["end"]
-
- return segments
-
-
-## Some utilities for writing transcripts to files
-
-
-def flatten(list_of_lists, key=None):
- for sublist in list_of_lists:
- for item in sublist.get(key, []) if key else sublist:
- yield item
-
-
-def remove_keys(list_of_dicts, key):
- for d in list_of_dicts:
- yield {k: d[k] for k in d.keys() - {key}}
-
-
-def write_csv(
- transcript, file, sep=",", text_first=True, format_timestamps=None, header=False
-):
- writer = csv.writer(file, delimiter=sep)
- if format_timestamps is None:
- format_timestamps = lambda x: x
- if header is True:
- header = ["text", "start", "end"] if text_first else ["start", "end", "text"]
- if header:
- writer.writerow(header)
- if text_first:
- writer.writerows(
- [
- [
- segment["text"].strip(),
- format_timestamps(segment["start"]),
- format_timestamps(segment["end"]),
- ]
- for segment in transcript
- ]
- )
- else:
- writer.writerows(
- [
- [
- format_timestamps(segment["start"]),
- format_timestamps(segment["end"]),
- segment["text"].strip(),
- ]
- for segment in transcript
- ]
- )
-
-
-# https://stackoverflow.com/questions/66588715/runtimeerror-cudnn-error-cudnn-status-not-initialized-using-pytorch
-# CUDA initialization may fail on old GPU card
-def force_cudnn_initialization(device=None, s=32):
- if device is None:
- device = torch.device("cuda")
- torch.nn.functional.conv2d(
- torch.zeros(s, s, s, s, device=device), torch.zeros(s, s, s, s, device=device)
- )
-
-
-# base85-encoded (n_layers, n_heads) boolean arrays indicating the cross-attention heads that are
-# highly correlated to the word-level timing, i.e. the alignment between audio and text tokens.
-_ALIGNMENT_HEADS = {
- "tiny.en": b"ABzY8J1N>@0{>%R00Bk>$p{7v037`oCl~+#00",
- "tiny": b"ABzY8bu8Lr0{>%RKn9Fp%m@SkK7Kt=7ytkO",
- "base.en": b"ABzY8;40c<0{>%RzzG;p*o+Vo09|#PsxSZm00",
- "base": b"ABzY8KQ!870{>%RzyTQH3`Q^yNP!>##QT-?_)10{>%RpeA61k&I|OI3I$65C{;;pbCHh0B{qLQ;+}v00",
- "small": b"ABzY8DmU6=0{>%Rpa?J`kvJ6qF(V^F86#Xh7JUGMK}P%R7%R7}kK1fFL7w6%<-Pf*t^=N)Qr&0RR9",
- "large-v1": b"ABzY8r9j$a0{>%R7#4sLmoOs{s)o3~84-RPdcFk!JR%R7=D0pU<_bnWW*tkYAhobTNnu$jnkEkXqp)j;w1Tzk)UH3X%SZd&fFZ2fC2yj",
- # "large": b'ABzY8zd+h!0{>%R7=D0pU<_bnWW*tkYAhobTNnu$jnkEkXqp)j;w1Tzk)UH3X%SZd&fFZ2fC2yj',
-}
-
-_PARAMETERS_TO_MODEL_NAME = {
- 37184256: "tiny.en",
- 37184640: "tiny",
- 71825408: "base.en",
- 71825920: "base",
- 240582144: "small.en",
- 240582912: "small",
- 762320896: "medium.en",
- 762321920: "medium",
- 1541384960: "large",
-}
-
-
-def get_alignment_heads(model):
- if hasattr(model, "alignment_heads"): # Since version 20230306
- return model.alignment_heads
- model_name = _PARAMETERS_TO_MODEL_NAME[_get_number_of_parameters(model)]
- if model_name == "large":
- if next(model.parameters())[0, 0, 0] > 0:
- model_name = "large-v1"
- else:
- model_name = "large-v2"
- num_layers = model.dims.n_text_layer
- num_heads = model.dims.n_text_head
- return _get_alignment_heads(model_name, num_layers, num_heads)
-
-
-def _get_alignment_heads(model_name, num_layers, num_heads):
- dump = _ALIGNMENT_HEADS[model_name]
- array = np.frombuffer(gzip.decompress(base64.b85decode(dump)), dtype=bool).copy()
- mask = torch.from_numpy(array).reshape(num_layers, num_heads)
- alignment_heads = mask.to_sparse()
- return alignment_heads
-
-
-def _get_number_of_parameters(model):
- return sum(p.numel() for p in model.parameters())
-
-
-from typing import Optional, Union
-
-
-def load_model(
- name: str,
- device: Optional[Union[str, torch.device]] = None,
- download_root: str = None,
- in_memory: bool = False,
-):
- extension = os.path.splitext(name)[-1] if os.path.isfile(name) else None
-
- if name in whisper.available_models() or extension == ".pt":
- return whisper.load_model(
- name, device=device, download_root=download_root, in_memory=in_memory
- )
-
- # Otherwise, assume transformers
- if extension in [".ckpt", ".bin"]:
- model_path = name
- else:
- # Search for the cached file (download if necessary)
- try:
- import transformers
- except ImportError:
- raise ImportError(
- f"If you are trying to download a HuggingFace model with {name}, please install first the transformers library"
- )
- from transformers.utils import cached_file
-
- try:
- model_path = cached_file(
- name,
- "pytorch_model.bin",
- cache_dir=download_root,
- use_auth_token=None,
- revision=None,
- )
- except Exception as e:
- try:
- if isinstance(e, OSError):
- model_path = cached_file(
- name,
- "whisper.ckpt",
- cache_dir=download_root,
- use_auth_token=None,
- revision=None,
- )
- else:
- raise e
- except:
- raise RuntimeError(
- f"Original error: {e}\nCould not find model {name} from HuggingFace nor local folders."
- )
- # Load HF Model
- hf_state_dict = torch.load(model_path, map_location="cpu")
- # Rename layers
- for key in list(hf_state_dict.keys())[:]:
- new_key = hf_to_whisper_states(key)
- hf_state_dict[new_key] = hf_state_dict.pop(key)
-
- # Remove useless key (Speechbrain
- if "_mel_filters" in hf_state_dict:
- hf_state_dict.pop("_mel_filters")
-
- # Init Whisper Model and replace model weights
- dims = whisper.model.ModelDimensions(**states_to_dim(hf_state_dict))
- whisper_model = whisper.model.Whisper(dims)
- whisper_model.load_state_dict(hf_state_dict)
- del hf_state_dict
- if hasattr(whisper_model, "alignment_heads"):
- del whisper_model.alignment_heads # Will be recomputed later
- whisper_model = whisper_model.to(device)
- return whisper_model
-
-
-# Credit: https://github.com/openai/whisper/discussions/830
-def hf_to_whisper_states(text):
- text = re.sub(".layers.", ".blocks.", text)
- text = re.sub(".self_attn.", ".attn.", text)
- text = re.sub(".q_proj.", ".query.", text)
- text = re.sub(".k_proj.", ".key.", text)
- text = re.sub(".v_proj.", ".value.", text)
- text = re.sub(".out_proj.", ".out.", text)
- text = re.sub(".fc1.", ".mlp.0.", text)
- text = re.sub(".fc2.", ".mlp.2.", text)
- text = re.sub(".fc3.", ".mlp.3.", text)
- text = re.sub(".fc3.", ".mlp.3.", text)
- text = re.sub(".encoder_attn.", ".cross_attn.", text)
- text = re.sub(".cross_attn.ln.", ".cross_attn_ln.", text)
- text = re.sub(".embed_positions.weight", ".positional_embedding", text)
- text = re.sub(".embed_tokens.", ".token_embedding.", text)
- text = re.sub("model.", "", text)
- text = re.sub("attn.layer_norm.", "attn_ln.", text)
- text = re.sub(".final_layer_norm.", ".mlp_ln.", text)
- text = re.sub("encoder.layer_norm.", "encoder.ln_post.", text)
- text = re.sub("decoder.layer_norm.", "decoder.ln.", text)
- return text
-
-
-def states_to_dim(state_dict):
- n_audio_state = len(state_dict["encoder.ln_post.bias"])
- n_text_state = len(state_dict["decoder.ln.bias"])
- return {
- "n_mels": state_dict["encoder.conv1.weight"].shape[1], # 80
- "n_vocab": state_dict["decoder.token_embedding.weight"].shape[
- 0
- ], # 51864 / 51865
- "n_audio_ctx": state_dict["encoder.positional_embedding"].shape[0], # 1500
- "n_audio_state": n_audio_state, # 384 / 512 / 768 / 1024 / 1280
- "n_audio_head": n_audio_state // 64, # 6 / 8 / 12 / 16 / 20
- "n_audio_layer": len(
- set(
- [
- ".".join(k.split(".")[:3])
- for k in state_dict.keys()
- if "encoder.blocks." in k
- ]
- )
- ), # 4 / 6 / 12 / 24 / 32
- "n_text_ctx": state_dict["decoder.positional_embedding"].shape[0], # 448
- "n_text_state": n_text_state, # 384 / 512 / 768 / 1024 / 1280
- "n_text_head": n_text_state // 64, # 6 / 8 / 12 / 16 / 20
- "n_text_layer": len(
- set(
- [
- ".".join(k.split(".")[:3])
- for k in state_dict.keys()
- if "decoder.blocks." in k
- ]
- )
- ), # 4 / 6 / 12 / 24 / 32
- }
-
-
-def cli():
- import os
- import sys
- import argparse
- import json
-
- from whisper.utils import str2bool, optional_float, optional_int
-
- try:
- # Old whisper version # Before https://github.com/openai/whisper/commit/da600abd2b296a5450770b872c3765d0a5a5c769
- from whisper.utils import write_txt, write_srt, write_vtt
-
- write_tsv = lambda transcript, file: write_csv(
- transcript,
- file,
- sep="\t",
- header=True,
- text_first=False,
- format_timestamps=lambda x: round(1000 * x),
- )
-
- except ImportError:
- # New whisper version
- from whisper.utils import get_writer
-
- def do_write(transcript, file, output_format):
- writer = get_writer(output_format, os.path.curdir)
- try:
- return writer.write_result({"segments": transcript}, file)
- except TypeError:
- # Version > 20230314
- return writer.write_result(
- {"segments": list(transcript)},
- file,
- {
- "highlight_words": False,
- "max_line_width": None,
- "max_line_count": None,
- },
- )
-
- def get_do_write(output_format):
- return lambda transcript, file: do_write(transcript, file, output_format)
-
- write_txt = get_do_write("txt")
- write_srt = get_do_write("srt")
- write_vtt = get_do_write("vtt")
- write_tsv = get_do_write("tsv")
-
- parser = argparse.ArgumentParser(
- description="Transcribe a single audio with whisper and compute word timestamps",
- formatter_class=argparse.ArgumentDefaultsHelpFormatter,
- )
- parser.add_argument(
- "-v",
- "--version",
- help="show version and exit",
- action="version",
- version=f"{__version__}",
- )
- parser.add_argument(
- "--versions",
- help="show versions (of whisper-timestamped and whisper) and exit",
- action="version",
- version=f"{__version__} -- Whisper {whisper.__version__} in {os.path.realpath(os.path.dirname(whisper.__file__))}",
- )
-
- parser.add_argument("audio", help="audio file(s) to transcribe", nargs="+")
- parser.add_argument(
- "--model",
- help=f"name of the Whisper model to use. Examples: {', '.join(whisper.available_models())}",
- default="small",
- )
- parser.add_argument(
- "--model_dir",
- default=None,
- help="the path to save model files; uses ~/.cache/whisper by default",
- type=str,
- )
- parser.add_argument(
- "--device",
- default="cuda:0" if torch.cuda.is_available() else "cpu",
- help="device to use for PyTorch inference",
- )
- parser.add_argument(
- "--output_dir",
- "-o",
- default=None,
- help="directory to save the outputs",
- type=str,
- )
- valid_formats = ["txt", "vtt", "srt", "tsv", "csv", "json"]
-
- def str2output_formats(string):
- if string == "all":
- return valid_formats
- formats = string.split(",")
- for format in formats:
- if format not in valid_formats:
- raise ValueError(f"Expected one of {valid_formats}, got {format}")
- return formats
-
- parser.add_argument(
- "--output_format",
- "-f",
- default="all",
- help=f"Format(s) of the output file(s). Possible formats are: {', '.join(valid_formats)}. Several formats can be specified by using commas (ex: \"json,vtt,srt\"). By default (\"all\"), all available formats will be produced",
- type=str2output_formats,
- )
-
- parser.add_argument(
- "--task",
- default="transcribe",
- help="whether to perform X->X speech recognition ('transcribe') or X->English translation ('translate')",
- choices=["transcribe", "translate"],
- type=str,
- )
- parser.add_argument(
- "--language",
- help=f"language spoken in the audio, specify None to perform language detection.",
- choices=sorted(whisper.tokenizer.LANGUAGES.keys())
- + sorted([k.title() for k in whisper.tokenizer.TO_LANGUAGE_CODE.keys()]),
- default=None,
- )
- # f"{', '.join(sorted(k+'('+v+')' for k,v in whisper.tokenizer.LANGUAGES.items()))}
-
- parser.add_argument(
- "--vad",
- default=False,
- help="whether to run Voice Activity Detection (VAD) to remove non-speech segment before applying Whisper model (removes hallucinations)",
- type=str2bool,
- )
- parser.add_argument(
- "--detect_disfluencies",
- default=False,
- help="whether to try to detect disfluencies, marking them as special words [*]",
- type=str2bool,
- )
- parser.add_argument(
- "--recompute_all_timestamps",
- default=not TRUST_WHISPER_TIMESTAMP_BY_DEFAULT,
- help="Do not rely at all on Whisper timestamps (Experimental option: did not bring any improvement, but could be useful in cases where Whipser segment timestamp are wrong by more than 0.5 seconds)",
- type=str2bool,
- )
- parser.add_argument(
- "--punctuations_with_words",
- default=True,
- help="whether to include punctuations in the words",
- type=str2bool,
- )
-
- parser.add_argument(
- "--temperature", default=0.0, help="temperature to use for sampling", type=float
- )
- parser.add_argument(
- "--best_of",
- type=optional_int,
- default=None if USE_EFFICIENT_BY_DEFAULT else 5,
- help="number of candidates when sampling with non-zero temperature",
- )
- parser.add_argument(
- "--beam_size",
- type=optional_int,
- default=None if USE_EFFICIENT_BY_DEFAULT else 5,
- help="number of beams in beam search, only applicable when temperature is zero",
- )
- parser.add_argument(
- "--patience",
- type=float,
- default=None,
- help="optional patience value to use in beam decoding, as in https://arxiv.org/abs/2204.05424, the default (1.0) is equivalent to conventional beam search",
- )
- parser.add_argument(
- "--length_penalty",
- type=float,
- default=None,
- help="optional token length penalty coefficient (alpha) as in https://arxiv.org/abs/1609.08144, uses simple length normalization by default",
- )
-
- parser.add_argument(
- "--suppress_tokens",
- default="-1",
- help="comma-separated list of token ids to suppress during sampling; '-1' will suppress most special characters except common punctuations",
- type=str,
- )
- parser.add_argument(
- "--initial_prompt",
- default=None,
- help="optional text to provide as a prompt for the first window.",
- type=str,
- )
- parser.add_argument(
- "--condition_on_previous_text",
- default=True,
- help="if True, provide the previous output of the model as a prompt for the next window; disabling may make the text inconsistent across windows, but the model becomes less prone to getting stuck in a failure loop",
- type=str2bool,
- )
- parser.add_argument(
- "--fp16",
- default=None,
- help="whether to perform inference in fp16; Automatic by default (True if GPU available, False otherwise)",
- type=str2bool,
- )
-
- parser.add_argument(
- "--temperature_increment_on_fallback",
- default=0.0 if USE_EFFICIENT_BY_DEFAULT else 0.2,
- help="temperature to increase when falling back when the decoding fails to meet either of the thresholds below",
- type=optional_float,
- )
- parser.add_argument(
- "--compression_ratio_threshold",
- default=2.4,
- help="if the gzip compression ratio is higher than this value, treat the decoding as failed",
- type=optional_float,
- )
- parser.add_argument(
- "--logprob_threshold",
- default=-1.0,
- help="if the average log probability is lower than this value, treat the decoding as failed",
- type=optional_float,
- )
- parser.add_argument(
- "--no_speech_threshold",
- default=0.6,
- help="if the probability of the <|nospeech|> token is higher than this value AND the decoding has failed due to `logprob_threshold`, consider the segment as silence",
- type=optional_float,
- )
- parser.add_argument(
- "--threads",
- default=0,
- help="number of threads used by torch for CPU inference; supercedes MKL_NUM_THREADS/OMP_NUM_THREADS",
- type=optional_int,
- )
-
- parser.add_argument(
- "--compute_confidence",
- default=True,
- help="whether to compute confidence scores for words",
- type=str2bool,
- )
- parser.add_argument(
- "--verbose",
- type=str2bool,
- default=False,
- help="whether to print out the progress and debug messages of Whisper",
- )
- parser.add_argument(
- "--plot",
- help="plot word alignments (save the figures if an --output_dir is specified, otherwhise just show figures that have to be closed to continue)",
- default=False,
- action="store_true",
- )
- parser.add_argument(
- "--debug",
- help="print some debug information about word alignement",
- default=False,
- action="store_true",
- )
-
- class ActionSetAccurate(argparse.Action):
- def __init__(self, option_strings, dest, nargs=None, **kwargs):
- assert nargs is None
- super().__init__(option_strings, dest, nargs=0, **kwargs)
-
- def __call__(self, parser, namespace, values, option_string=None):
- setattr(namespace, "best_of", 5)
- setattr(namespace, "beam_size", 5)
- setattr(namespace, "temperature_increment_on_fallback", 0.2)
-
- parser.add_argument(
- "--accurate",
- help="Shortcut to use the same default option as in Whisper (best_of=5, beam_search=5, temperature_increment_on_fallback=0.2)",
- action=ActionSetAccurate,
- )
-
- class ActionSetEfficient(argparse.Action):
- def __init__(self, option_strings, dest, nargs=None, **kwargs):
- assert nargs is None
- super().__init__(option_strings, dest, nargs=0, **kwargs)
-
- def __call__(self, parser, namespace, values, option_string=None):
- setattr(namespace, "best_of", None)
- setattr(namespace, "beam_size", None)
- setattr(namespace, "temperature_increment_on_fallback", None)
-
- parser.add_argument(
- "--efficient",
- help="Shortcut to disable beam size and options that requires to sample several times, for an efficient decoding",
- action=ActionSetEfficient,
- )
-
- parser.add_argument(
- "--naive",
- help="use naive approach, doing inference twice (once to get the transcription, once to get word timestamps and confidence scores).",
- default=False,
- action="store_true",
- )
-
- args = parser.parse_args().__dict__
- args.pop("accurate")
- args.pop("efficient")
-
- temperature = args.pop("temperature")
- temperature_increment_on_fallback = args.pop("temperature_increment_on_fallback")
- if temperature_increment_on_fallback:
- temperature = tuple(
- np.arange(temperature, 1.0 + 1e-6, temperature_increment_on_fallback)
- )
- else:
- temperature = [temperature]
-
- threads = args.pop("threads")
- if threads:
- torch.set_num_threads(threads)
-
- audio_files = args.pop("audio")
-
- model = args.pop("model")
- device = args.pop("device")
- model_dir = args.pop("model_dir")
-
- if device.lower().startswith("cuda"):
- force_cudnn_initialization(device)
-
- output_format = args.pop("output_format")
-
- model = load_model(model, device=device, download_root=model_dir)
-
- plot_word_alignment = args.pop("plot")
-
- debug = args.pop("debug")
- logging.basicConfig()
- if debug:
- logger.setLevel(logging.DEBUG)
- # This supposes to plug a logger with name "WHISPER" into Whisper source code (no harm if it's not set)
- logging.getLogger("WHISPER").setLevel(logging.DEBUG)
-
- output_dir = args.pop("output_dir")
- if output_dir and not os.path.isdir(output_dir):
- os.makedirs(output_dir)
-
- args["naive_approach"] = args.pop("naive")
- args["remove_punctuation_from_words"] = not args.pop("punctuations_with_words")
- args["compute_word_confidence"] = args.pop("compute_confidence")
- args["trust_whisper_timestamps"] = not args.pop("recompute_all_timestamps")
-
- # Quick early check
- for audio_path in audio_files:
- assert os.path.isfile(audio_path), f"File {audio_path} does not exist"
-
- for audio_path in audio_files:
- outname = (
- os.path.join(output_dir, os.path.basename(audio_path))
- if output_dir
- else None
- )
-
- result = transcribe_timestamped(
- model,
- audio_path,
- temperature=temperature,
- plot_word_alignment=outname
- if (outname and plot_word_alignment)
- else plot_word_alignment,
- **args,
- )
-
- if output_dir:
- if "json" in output_format:
- # save JSON
- with open(outname + ".words.json", "w", encoding="utf-8") as js:
- json.dump(result, js, indent=2, ensure_ascii=False)
-
- # save TXT
- if "txt" in output_format:
- with open(outname + ".txt", "w", encoding="utf-8") as txt:
- write_txt(result["segments"], file=txt)
-
- # save VTT
- if "vtt" in output_format:
- with open(outname + ".vtt", "w", encoding="utf-8") as vtt:
- write_vtt(remove_keys(result["segments"], "words"), file=vtt)
- with open(outname + ".words.vtt", "w", encoding="utf-8") as vtt:
- write_vtt(flatten(result["segments"], "words"), file=vtt)
-
- # save SRT
- if "srt" in output_format:
- with open(outname + ".srt", "w", encoding="utf-8") as srt:
- write_srt(remove_keys(result["segments"], "words"), file=srt)
- with open(outname + ".words.srt", "w", encoding="utf-8") as srt:
- write_srt(flatten(result["segments"], "words"), file=srt)
-
- # save CSV
- if "csv" in output_format:
- with open(outname + ".csv", "w", encoding="utf-8") as csv:
- write_csv(result["segments"], file=csv)
- with open(outname + ".words.csv", "w", encoding="utf-8") as csv:
- write_csv(flatten(result["segments"], "words"), file=csv)
-
- # save TSV
- if "tsv" in output_format:
- with open(outname + ".tsv", "w", encoding="utf-8") as csv:
- write_tsv(result["segments"], file=csv)
- with open(outname + ".words.tsv", "w", encoding="utf-8") as csv:
- write_tsv(flatten(result["segments"], "words"), file=csv)
-
- elif not args["verbose"]:
- json.dump(filtered_keys(result), sys.stdout, indent=2, ensure_ascii=False)
-
-
-def filtered_keys(
- result, keys=["text", "segments", "words", "language", "start", "end", "confidence"]
-):
- if isinstance(result, dict):
- return {k: filtered_keys(v, keys) for k, v in result.items() if k in keys}
- if isinstance(result, list):
- return [filtered_keys(v, keys) for v in result]
- if isinstance(result, float):
- return round(result, 2)
- return result
-
-
-if __name__ == "__main__":
- cli()
diff --git a/spaces/nambiar4/DR-BERT/app.py b/spaces/nambiar4/DR-BERT/app.py
deleted file mode 100644
index edcf5b69c5ab7591ee57cd7e3512877d06eb28cc..0000000000000000000000000000000000000000
--- a/spaces/nambiar4/DR-BERT/app.py
+++ /dev/null
@@ -1,47 +0,0 @@
-import gradio as gr
-from transformers import AutoModel, AutoTokenizer, AutoModelForTokenClassification
-import torch
-import numpy as np
-import torch.nn.functional as F
-import matplotlib.pyplot as plt
-
-
-tokenizer = AutoTokenizer.from_pretrained("./checkpoint-final/")
-model = AutoModelForTokenClassification.from_pretrained("./checkpoint-final/")
-model = model.eval()
-
-examples = [
- ["GSHMSDNEDNFDGDDFDDVEEDEGLDDLENAEEEGQENVEILPSGERPQANQKRITTPYMTKYERARVLGTRALQIAMCAPVMVELEGETDPLLIAMKELKARKIPIIIRRYLPDGSYEDWGVDELIITD"]]
-
-def get_out(sent):
- prefix = ""
- if len(sent)>1022:
- sent = sent[:1022]
- prefix = "Your protein was longer than 1022 AAs. We are working on including longer sequences but in the meantime, here are the scores for the first 1022 AAs: \n "
- print(sent)
- encoded = tokenizer.encode_plus(sent, return_tensors="pt")
- with torch.no_grad():
- output = model(**encoded)
- output = F.softmax(torch.squeeze(output['logits']))[1:-1,1].detach().numpy()
-
- fig = plt.figure()
- plt.plot(output)
- plt.xticks(fontsize=15)
- plt.yticks(fontsize=15)
- plt.xlabel('Sequence position', fontsize=15)
- plt.ylabel('DR-BERT score', fontsize=15)
-
- output = ','.join(str(x) for x in output)
- return (fig,prefix+output)
-
-
-gr.Interface(
- get_out,
- [
- gr.components.Textbox(label="Input Amino Acid Sequence", placeholder = " Amino acid sequence here ...")
- ],
- ["plot","text"],
- examples=examples,
- title="DR-BERT: A Protein Language Model to Predict Disordered Regions",
- description="The app uses DR-BERT to predict disordered regions in proteins. Outputs generated are the probability that a residue is disordered."
-).launch()
\ No newline at end of file
diff --git a/spaces/netiMophi/DreamlikeArt-Diffusion-1.0/Acer A200 Simple Tool V2 598 _BEST_.md b/spaces/netiMophi/DreamlikeArt-Diffusion-1.0/Acer A200 Simple Tool V2 598 _BEST_.md
deleted file mode 100644
index e7723163accd62ab8015f887c6baf13cddab5ee9..0000000000000000000000000000000000000000
--- a/spaces/netiMophi/DreamlikeArt-Diffusion-1.0/Acer A200 Simple Tool V2 598 _BEST_.md
+++ /dev/null
@@ -1,149 +0,0 @@
-
-
Acer A200 Simple Tool V2 598: How to Root, Flash, Backup, and More with One Click
-
If you own an Acer Iconia A200 tablet and want to customize it to your liking, you might be interested in a tool that can help you do that easily. The tool is called A cer A200 Simple Tool V2 598 and it was developed by XDA Developers member sc2k. The tool allows you to root, flash, backup, and perform other operations on your tablet with one click. Sounds amazing, right?
In this article, I will tell you everything you need to know about Acer A200 Simple Tool V2 598, including what it is, what are the benefits of using it, what are the requirements for using it, how to download and install it, how to use it, what are some tips and tricks for using it, what are some alternatives to it, and some FAQs. By the end of this article, you will be able to unleash the full potential of your Acer Iconia A200 tablet with Acer A200 Simple Tool V2 598. Let's get started!
-
What is Acer A200 Simple Tool V2 598?
-
Acer A200 Simple Tool V2 598 is a Windows-based application that can perform various tasks on your Acer Iconia A200 tablet with one click. The tool was created by sc2k, a senior member of XDA Developers forum, who shared it on his thread. The tool is based on the work of other developers such as alephzain, vache, thor2002ro, and others. The tool supports both Windows XP and Windows 7 operating systems.
-
The tool has a simple and user-friendly interface that shows you the options available for your tablet. You can choose from the following options:
-
-
Root: This option allows you to root your tablet, which means gaining administrative access to the system files and settings. Rooting enables you to install custom ROMs, remove bloatware, tweak performance, and more.
-
Flash: This option allows you to flash a custom ROM on your tablet, which means replacing the stock firmware with a modified one. Flashing a custom ROM can give you more features, better performance, improved battery life, and more.
-
Backup: This option allows you to backup your tablet data, which means creating a copy of your files and settings. Backing up your data can help you restore it in case of any problem or data loss.
-
Restore: This option allows you to restore your tablet data, which means restoring the files and settings from a backup. Restoring your data can help you recover from any problem or data loss.
-
Unroot: This option allows you to unroot your tablet, which means removing the administrative access to the system files and settings. Unrooting can help you return to the stock firmware, receive official updates, or claim warranty.
-
-
As you can see, Acer A200 Simple Tool V2 598 can help you do a lot of things with your tablet with just one click. But why would you want to do these things? Let's find out in the next section.
-
What are the benefits of using Acer A200 Simple Tool V2 598?
-
Using Acer A200 Simple Tool V2 598 can bring you many benefits for your tablet. Here are some of them:
-
-
You can root your tablet easily and safely without any complicated commands or procedures. Rooting can give you more control over your tablet and allow you to customize it as you wish.
-
You can flash a custom ROM on your tablet quickly and conveniently without any hassle or risk. Flashing a custom ROM can give you more functionality and performance than the stock firmware.
-
You can backup your tablet data securely and reliably without any loss or corruption. Backing up your data can help you protect it from any damage or deletion.
-
You can restore your tablet data easily and accurately without any error or mismatch. Restoring your data can help you recover it from any problem or disaster.
-
You can unroot your tablet smoothly and cleanly without any trace or residue. Unrooting can help you revert to the stock firmware, receive official updates, or claim warranty.
-
-
As you can see, using Acer A200 Simple Tool V2 598 can make your tablet experience better and more enjoyable. But before you use it, you need to make sure that you meet some requirements. Let's see what they are in the next section.
-
What are the requirements for using Acer A200 Simple Tool V2 598?
-
Before you use Acer A200 Simple Tool V2 598 on your tablet, you need to make sure that you have the following requirements:
-
-
-
A Windows XP or Windows 7 computer with .NET Framework 4 installed. You can download .NET Framework 4 from here.
-
An Acer Iconia A200 tablet with at least 50% battery level. You can check your battery level by going to Settings > About tablet > Status.
-
A USB cable to connect your tablet to your computer. Make sure that the cable is working properly and not damaged.
-
The Acer A200 Simple Tool V2 598 application. You can download it from here.
-
The drivers for your tablet. You can download them from here.
-
A backup of your tablet data. You can use the tool itself to backup your data, or use any other method that you prefer. This is important in case something goes wrong during the process.
-
-
Once you have all these requirements, you are ready to use Acer A200 Simple Tool V2 598 on your tablet. But first, you need to download and install it on your computer. Let's see how to do that in the next section.
-
How to download and install Acer A200 Simple Tool V2 598?
-
Downloading and installing Acer A200 Simple Tool V2 598 on your computer is very easy and straightforward. Just follow these steps:
-
-
Download the Acer A200 Simple Tool V2 598 application from here. The file size is about 15 MB and the file name is Acer_A200_Simple_Tool_v2_598.zip.
-
Extract the zip file to a folder on your computer. You can use any zip extractor software that you like, such as WinRAR, 7-Zip, or PeaZip.
-
Open the folder where you extracted the zip file and double-click on the Acer_A200_Simple_Tool_v2_598.exe file. This will launch the application.
-
Wait for the application to load and detect your tablet. You will see a message saying "Device connected" if your tablet is detected successfully.
-
-
Congratulations! You have successfully downloaded and installed Acer A200 Simple Tool V2 598 on your computer. Now you can use it to perform various tasks on your tablet with one click. Let's see how to do that in the next section.
-
How to use Acer A200 Simple Tool V2 598?
-
Using Acer A200 Simple Tool V2 598 on your tablet is very simple and convenient. You just need to select the option that you want to perform and follow the instructions on the screen. Here are some guides on how to use the tool for different purposes:
-
How to root your Acer Iconia A200 with Acer A200 Simple Tool V2 598?
-
Rooting your tablet means gaining administrative access to the system files and settings. Rooting can enable you to install custom ROMs, remove bloatware, tweak performance, and more. To root your tablet with Acer A200 Simple Tool V2 598, follow these steps:
-
-
Make sure that you have met all the requirements for using the tool, as mentioned in the previous sections.
-
Connect your tablet to your computer with a USB cable and enable USB debugging mode on your tablet. To enable USB debugging mode, go to Settings > Developer options > USB debugging and check the box.
-
Launch the Acer A200 Simple Tool V2 598 application on your computer and wait for it to detect your tablet.
-
Select the option "1-Root" from the main menu of the application and press Enter.
-
Follow the instructions on the screen and wait for the process to complete. The process may take a few minutes and your tablet may reboot several times during the process.
-
Once the process is done, you will see a message saying "Root done" on the screen.
-
-
Congratulations! You have successfully rooted your tablet with Acer A200 Simple Tool V2 598. You can now enjoy more control over your tablet and customize it as you wish.
-
How to flash a custom ROM on your Acer Iconia A200 with Acer A200 Simple Tool V2 598?
-
Flashing a custom ROM means replacing the stock firmware with a modified one. Flashing a custom ROM can give you more features, better performance, improved battery life, and more. To flash a custom ROM on your tablet with Acer A200 Simple Tool V2 598, follow these steps:
-
-
Make sure that you have met all the requirements for using the tool, as mentioned in the previous sections.
-
Download a custom ROM that is compatible with your tablet from a reliable source, such as XDA Developers forum or Android Authority website. The custom ROM should be in a zip file format and should not be extracted.
-
Copy the custom ROM zip file to the root directory of your tablet memory card or internal storage. You can use a file manager app or a USB cable to do this.
-
Connect your tablet to your computer with a USB cable and enable USB debugging mode on your tablet. To enable USB debugging mode, go to Settings > Developer options > USB debugging and check the box.
-
Launch the Acer A200 Simple Tool V2 598 application on your computer and wait for it to detect your tablet.
-
Select the option "2-Flash" from the main menu of the application and press Enter.
-
Follow the instructions on the screen and select the custom ROM zip file that you copied to your tablet. The application will automatically reboot your tablet into recovery mode and flash the custom ROM.
-
Wait for the process to complete. The process may take several minutes and your tablet may reboot several times during the process.
-
Once the process is done, you will see a message saying "Flash done" on the screen.
-
-
Congratulations! You have successfully flashed a custom ROM on your tablet with Acer A200 Simple Tool V2 598. You can now enjoy more functionality and performance than the stock firmware.
-
How to backup your Acer Iconia A200 data with Acer A200 Simple Tool V2 598?
-
Backing up your tablet data means creating a copy of your files and settings. Backing up your data can help you protect it from any damage or deletion. To backup your tablet data with Acer A200 Simple Tool V2 598, follow these steps:
-
-
Make sure that you have met all the requirements for using the tool, as mentioned in the previous sections.
-
Connect your tablet to your computer with a USB cable and enable USB debugging mode on your tablet. To enable USB debugging mode, go to Settings > Developer options > USB debugging and check the box.
-
Launch the Acer A200 Simple Tool V2 598 application on your computer and wait for it to detect your tablet.
-
Select the option "3-Backup" from the main menu of the application and press Enter.
-
Follow the instructions on the screen and choose a location on your computer where you want to save the backup file. The application will automatically reboot your tablet into recovery mode and backup your data.
-
Wait for the process to complete. The process may take several minutes and your tablet may reboot several times during the process.
-
Once the process is done, you will see a message saying "Backup done" on the screen.
-
-
Congratulations! You have successfully backed up your tablet data with Acer A200 Simple Tool V2 598. You can now restore it in case of any problem or data loss.
-
How to restore your Acer Iconia A200 data with Acer A200 Simple Tool V2 598?
-
Restoring your tablet data means restoring the files and settings from a backup. Restoring your data can help you recover it from any problem or disaster. To restore your tablet data with Acer A200 Simple Tool V2 598, follow these steps:
-
-
Make sure that you have met all the requirements for using the tool, as mentioned in the previous sections.
-
Connect your tablet to your computer with a USB cable and enable USB debugging mode on your tablet. To enable USB debugging mode, go to Settings > Developer options > USB debugging and check the box.
-
Launch the Acer A200 Simple Tool V2 598 application on your computer and wait for it to detect your tablet.
-
Select the option "4-Restore" from the main menu of the application and press Enter.
-
Follow the instructions on the screen and select the backup file that you want to restore from. The application will automatically reboot your tablet into recovery mode and restore your data.
-
Wait for the process to complete. The process may take several minutes and your tablet may reboot several times during the process.
-
Once the process is done, you will see a message saying "Restore done" on the screen.
-
-
Congratulations! You have successfully restored your tablet data with Acer A200 Simple Tool V2 598. You can now enjoy your tablet as before.
-
How to unroot your Acer Iconia A200 with Acer A200 Simple Tool V2 598?
-
Unrooting your tablet means removing the administrative access to the system files and settings. Unrooting can help you return to the stock firmware, receive official updates, or claim warranty. To unroot your tablet with Acer A200 Simple Tool V2 598, follow these steps:
-
-
Make sure that you have met all the requirements for using the tool, as mentioned in the previous sections.
-
Connect your tablet to your computer with a USB cable and enable USB debugging mode on your tablet. To enable USB debugging mode, go to Settings > Developer options > USB debugging and check the box.
-
Launch the Acer A200 Simple Tool V2 598 application on your computer and wait for it to detect your tablet.
-
Select the option "5-Unroot" from the main menu of the application and press Enter.
-
Follow the instructions on the screen and wait for the process to complete. The process may take a few minutes and your tablet may reboot several times during the process.
-
Once the process is done, you will see a message saying "Unroot done" on the screen.
-
-
Congratulations! You have successfully unrooted your tablet with Acer A200 Simple Tool V2 598. You can now revert to the stock firmware, receive official updates, or claim warranty.
-
What are some tips and tricks for using Acer A200 Simple Tool V2 598?
-
Using Acer A200 Simple Tool V2 598 on your tablet can be very easy and convenient, but there are some tips and tricks that can make it even better and safer. Here are some of them:
-
-
Before using the tool, make sure that you have a backup of your tablet data. This can help you restore it in case of any problem or data loss.
-
Before using the tool, make sure that you have enough battery level on your tablet. This can prevent any interruption or failure during the process.
-
Before using the tool, make sure that you have installed the drivers for your tablet on your computer. This can ensure a smooth and stable connection between your tablet and your computer.
-
Before using the tool, make sure that you have downloaded the latest version of the tool from the official thread. This can ensure that you have the most updated and bug-free version of the tool.
-
Before using the tool, make sure that you have checked the compatibility of the custom ROM that you want to flash on your tablet. This can prevent any incompatibility or conflict issues between your tablet and the custom ROM.
-
After using the tool, make sure that you reboot your tablet once or twice. This can help your tablet to adapt to the changes and optimize its performance.
-
If you encounter any error or problem while using the tool, make sure that you report it to the developer on his thread. This can help him to fix it and improve it in future versions.
-
-
These are some of the tips and tricks that can help you use Acer A200 Simple Tool V2 598 on your tablet more effectively and safely. If you follow them, you will have a better and more enjoyable tablet experience.
-
What are some alternatives to Acer A200 Simple Tool V2 598?
-
Acer A200 Simple Tool V2 598 is a great tool for your Acer Iconia A200 tablet, but it is not the only one. There are some other tools that can perform similar functions as Acer A200 Simple Tool V2 598, such as:
-
-
KingoRoot: This is a one-click root tool that can root almost any Android device with ease. It supports both Windows and Android platforms and has a high success rate. You can download it from here.
-
SP Flash Tool: This is a flash tool that can flash custom ROMs, recoveries, kernels, and other files on MediaTek devices. It supports both Windows and Linux platforms and has a simple interface. You can download it from here.
-
TWRP Recovery: This is a custom recovery that can perform various tasks on your device such as flashing, wiping, backing up, restoring, etc. It has a touch-based interface and supports many devices. You can download it from here.
-
-
These are some of the alternatives to Acer A200 Simple Tool V2 598 that you can try if you want to explore more options for your tablet. However, Acer A200 Simple Tool V2 598 is still a very useful and convenient tool that can help you do a lot of things with your tablet with one click.
-
Conclusion
-
In this article, I have shown you everything you need to know about Acer A200 Simple Tool V2 598, a tool that can help you root, flash, backup, and perform other operations on your Acer Iconia A200 tablet with one click. I have explained what it is, what are the benefits of using it, what are the requirements for using it, how to download and install it, how to use it, what are some tips and tricks for using it, what are some alternatives to it, and some FAQs. I hope you have found this article helpful and informative.
-
If you have an Acer Iconia A200 tablet and want to customize it to your liking, I highly recommend you to try Acer A200 Simple Tool V2 598. It is a simple and user-friendly tool that can make your tablet experience better and more enjoyable. You can download it from here and follow the guides in this article to use it on your tablet.
-
Thank you for reading this article. If you have any questions or comments, please feel free to leave them below. I would love to hear from you. Have a great day!
-
FAQs
-
Here are some frequently asked questions about Acer A200 Simple Tool V2 598 and their answers:
-
-
Is Acer A200 Simple Tool V2 598 safe to use?
-
Yes, Acer A200 Simple Tool V2 598 is safe to use as long as you follow the instructions carefully and make a backup of your data before using it. However, there is always a risk of bricking or damaging your device when performing any modification on it. Therefore, use the tool at your own risk and responsibility.
-
Does Acer A200 Simple Tool V2 598 work on other devices?
-
No, Acer A200 Simple Tool V2 598 is designed specifically for the Acer Iconia A200 tablet and does not work on other devices. If you have a different device, you should look for a different tool that is compatible with your device.
-
Does Acer A200 Simple Tool V2 598 void the warranty of my tablet?
-
Yes, using Acer A200 Simple Tool V2 598 may void the warranty of your tablet as it modifies the system files and settings of your tablet. If you want to claim warranty, you should unroot your tablet with the tool and restore the stock firmware before sending it to the service center.
-
Where can I find more information about Acer A200 Simple Tool V2 598?
-
You can find more information about Acer A200 Simple Tool V2 598 on the official thread of the developer on XDA Developers forum. You can also contact the developer directly through his profile or email if you have any feedback or suggestion for the tool.
-
How can I support the development of Acer A200 Simple Tool V2 598?
-
If you like Acer A200 Simple Tool V2 598 and want to support the development of the tool, you can donate to the developer through his PayPal account or thank him on his thread. You can also share the tool with your friends and family who have an Acer Iconia A200 tablet and want to customize it.
- b2dd77e56b
-
-
\ No newline at end of file
diff --git a/spaces/netiMophi/DreamlikeArt-Diffusion-1.0/Golmaal Returns In Hindi Download Full Movie.md b/spaces/netiMophi/DreamlikeArt-Diffusion-1.0/Golmaal Returns In Hindi Download Full Movie.md
deleted file mode 100644
index 26da6e7c929849bae8cf67b51d4fe7ec50cca1b6..0000000000000000000000000000000000000000
--- a/spaces/netiMophi/DreamlikeArt-Diffusion-1.0/Golmaal Returns In Hindi Download Full Movie.md
+++ /dev/null
@@ -1,23 +0,0 @@
-
-
How to Download Golmaal Returns (2008) Full Movie in Hindi
-
Golmaal Returns is a 2008 comedy film directed by Rohit Shetty and starring Ajay Devgn, Kareena Kapoor, Arshad Warsi, Tusshar Kapoor, Shreyas Talpade, Celina Jaitly, Amrita Arora and others. It is the sequel to the 2006 film Golmaal: Fun Unlimited and the second installment in the Golmaal film series. The film follows the hilarious adventures of four friends who get involved in a murder mystery while trying to cover up their lies.
If you are looking for a way to download Golmaal Returns full movie in Hindi, you have come to the right place. In this article, we will show you some of the best websites where you can watch or download Golmaal Returns online for free or at a low cost. We will also provide you with some tips and precautions to avoid any legal issues or malware infections while downloading movies online.
-
Best Websites to Download Golmaal Returns Full Movie in Hindi
-
There are many websites that offer Golmaal Returns full movie in Hindi for online streaming or downloading. However, not all of them are safe, legal or reliable. Some of them may contain viruses, spyware, adware or other malicious software that can harm your device or compromise your privacy. Some of them may also violate the copyright laws and put you at risk of legal action.
-
Therefore, it is important to choose a trusted and reputable website that has a good reputation and user reviews. Here are some of the best websites that we recommend for downloading Golmaal Returns full movie in Hindi:
-
-
PogoLinks: This is a popular website that provides Bollywood and Hollywood movies in various formats and qualities. You can download Golmaal Returns full movie in Hindi in 480p, 720p or 1080p quality with subtitles. The website also offers multiple download links from different sources and servers. You can watch the trailer and read the synopsis and cast information before downloading. The website is easy to use and has a simple interface. You can access PogoLinks from this link[^1^].
-
Disney+ Hotstar: This is a premium streaming service that offers a wide range of movies, TV shows, sports, news and live events. You can watch Golmaal Returns full movie in Hindi on Disney+ Hotstar with high-quality video and audio. You can also download the movie offline on your device and watch it later without an internet connection. However, you need to have a subscription plan to access Disney+ Hotstar content. You can choose from different plans starting from Rs. 299 per month or Rs. 1499 per year. You can access Disney+ Hotstar from this link[^2^].
-
JioCinema: This is another streaming service that offers a large collection of movies, TV shows, music videos and trailers. You can watch Golmaal Returns full movie in Hindi on JioCinema with HD quality and Dolby sound. You can also download the movie on your device and watch it offline. However, you need to have a Jio SIM card or a JioFiber connection to access JioCinema content. You can access JioCinema from this link[^3^] [^4^].
-
-
Tips and Precautions for Downloading Movies Online
-
Downloading movies online can be a convenient and cost-effective way to enjoy your favorite films at your own time and place. However, there are some tips and precautions that you should follow to ensure a safe and hassle-free experience:
-
-
Always use a reliable antivirus software and firewall on your device to protect it from any malware or cyberattacks.
-
Always use a VPN service or proxy server to hide your IP address and location from any third-party trackers or hackers.
-
Always check the file size, format, quality and source of the movie before downloading it. Avoid any suspicious or unknown links that may contain viruses or malware.
-
Always respect the copyright laws and regulations of your country and region. Do not download or distribute any pirated or illegal content that may infringe on the rights of the original creators or owners.
- cec2833e83
-
-
\ No newline at end of file
diff --git "a/spaces/netiMophi/DreamlikeArt-Diffusion-1.0/Magoshare Data\302\240Recovery Crack [BETTER].md" "b/spaces/netiMophi/DreamlikeArt-Diffusion-1.0/Magoshare Data\302\240Recovery Crack [BETTER].md"
deleted file mode 100644
index 8ce09ddda24ce5da495139f111a127791b1a5b65..0000000000000000000000000000000000000000
--- "a/spaces/netiMophi/DreamlikeArt-Diffusion-1.0/Magoshare Data\302\240Recovery Crack [BETTER].md"
+++ /dev/null
@@ -1,23 +0,0 @@
-
-
How to Recover Lost Data with Magoshare Data Recovery Crack
-
Have you ever lost important data due to deletion, formatting, virus infection, partition loss, or other causes? If so, you may be looking for a way to recover your lost files without spending a lot of money. One of the options you may have come across is Magoshare Data Recovery Crack, a pirated version of a professional data recovery software. But is it really a good idea to use Magoshare Data Recovery Crack? In this article, we will explain why you should avoid Magoshare Data Recovery Crack and what are the best alternatives to recover your lost data safely and easily.
-
What is Magoshare Data Recovery Crack?
-
Magoshare Data Recovery is an intuitive and powerful data recovery software that can recover lost data from various devices, such as hard drives, USB flash drives, memory cards, digital cameras, etc. It can recover any type of files, such as documents, photos, videos, audio, emails, archives, etc. It can also recover data from deleted or lost partitions, formatted or inaccessible drives, raw drives, etc. It supports Windows and Mac operating systems and offers a free trial version that allows you to scan and preview the recoverable files before purchasing the full version[^1^] [^2^].
Magoshare Data Recovery Crack is a cracked or modified version of Magoshare Data Recovery that claims to offer the full features of the software for free. It is usually distributed by some hackers or websites that provide illegal downloads of software. Some people may be tempted to use Magoshare Data Recovery Crack to save money or avoid registration. However, using Magoshare Data Recovery Crack is not only illegal but also risky for your data and your device.
-
Why You Should Avoid Magoshare Data Recovery Crack?
-
There are many reasons why you should avoid using Magoshare Data Recovery Crack. Here are some of them:
-
-
It is illegal. Using Magoshare Data Recovery Crack violates the copyright law and the terms of use of the software. You may face legal consequences if you are caught using or distributing Magoshare Data Recovery Crack.
-
It is unsafe. Magoshare Data Recovery Crack may contain viruses, malware, spyware, ransomware, or other malicious programs that can harm your computer or steal your personal information. You may also lose your data permanently if Magoshare Data Recovery Crack damages your device or overwrites your files.
-
It is unreliable. Magoshare Data Recovery Crack may not work properly or crash frequently. It may also fail to recover your data or recover corrupted or incomplete files. You may not be able to get any technical support or updates from the official developer if you use Magoshare Data Recovery Crack.
-
It is unethical. Using Magoshare Data Recovery Crack deprives the developer of their rightful income and discourages them from creating more quality software. You may also lose your trust and reputation if you use or share Magoshare Data Recovery Crack with others.
-
-
What are the Best Alternatives to Magoshare Data Recovery Crack?
-
If you want to recover your lost data safely and easily, you should avoid using Magoshare Data Recovery Crack and choose a reliable and legal alternative instead. Here are some of the best alternatives to Magoshare Data Recovery Crack:
-
-
The official version of Magoshare Data Recovery. The best way to use Magoshare Data Recovery is to purchase the official version from the developer's website[^1^] [^2^]. The official version is 100% safe and efficient and offers lifetime free updates and technical support. You can also enjoy a 30-day money-back guarantee if you are not satisfied with the software.
-
The free trial version of Magoshare Data Recovery. If you are not sure whether Magoshare Data Recovery can recover your data or not, you can try the free trial version first. The free trial version allows you to scan and preview the recoverable files before purchasing the full version[^1^] [^2^]. You can also recover up to 250 MB of data for free with the free trial version[
- 81aa517590
-
-
\ No newline at end of file
diff --git a/spaces/nikitaPDL2023/assignment4/detectron2/detectron2/evaluation/__init__.py b/spaces/nikitaPDL2023/assignment4/detectron2/detectron2/evaluation/__init__.py
deleted file mode 100644
index d96609e8f2261a6800fe85fcf3e1eaeaa44455c6..0000000000000000000000000000000000000000
--- a/spaces/nikitaPDL2023/assignment4/detectron2/detectron2/evaluation/__init__.py
+++ /dev/null
@@ -1,12 +0,0 @@
-# Copyright (c) Facebook, Inc. and its affiliates.
-from .cityscapes_evaluation import CityscapesInstanceEvaluator, CityscapesSemSegEvaluator
-from .coco_evaluation import COCOEvaluator
-from .rotated_coco_evaluation import RotatedCOCOEvaluator
-from .evaluator import DatasetEvaluator, DatasetEvaluators, inference_context, inference_on_dataset
-from .lvis_evaluation import LVISEvaluator
-from .panoptic_evaluation import COCOPanopticEvaluator
-from .pascal_voc_evaluation import PascalVOCDetectionEvaluator
-from .sem_seg_evaluation import SemSegEvaluator
-from .testing import print_csv_format, verify_results
-
-__all__ = [k for k in globals().keys() if not k.startswith("_")]
diff --git a/spaces/nikitaPDL2023/assignment4/detectron2/detectron2/modeling/meta_arch/build.py b/spaces/nikitaPDL2023/assignment4/detectron2/detectron2/modeling/meta_arch/build.py
deleted file mode 100644
index 3427215746c9a146bd902f22ea9b26d121c36b27..0000000000000000000000000000000000000000
--- a/spaces/nikitaPDL2023/assignment4/detectron2/detectron2/modeling/meta_arch/build.py
+++ /dev/null
@@ -1,25 +0,0 @@
-# Copyright (c) Facebook, Inc. and its affiliates.
-import torch
-
-from detectron2.utils.logger import _log_api_usage
-from detectron2.utils.registry import Registry
-
-META_ARCH_REGISTRY = Registry("META_ARCH") # noqa F401 isort:skip
-META_ARCH_REGISTRY.__doc__ = """
-Registry for meta-architectures, i.e. the whole model.
-
-The registered object will be called with `obj(cfg)`
-and expected to return a `nn.Module` object.
-"""
-
-
-def build_model(cfg):
- """
- Build the whole model architecture, defined by ``cfg.MODEL.META_ARCHITECTURE``.
- Note that it does not load any weights from ``cfg``.
- """
- meta_arch = cfg.MODEL.META_ARCHITECTURE
- model = META_ARCH_REGISTRY.get(meta_arch)(cfg)
- model.to(torch.device(cfg.MODEL.DEVICE))
- _log_api_usage("modeling.meta_arch." + meta_arch)
- return model
diff --git a/spaces/nikitaPDL2023/assignment4/detectron2/projects/DensePose/densepose/converters/chart_output_hflip.py b/spaces/nikitaPDL2023/assignment4/detectron2/projects/DensePose/densepose/converters/chart_output_hflip.py
deleted file mode 100644
index 17d294841264c248cf7fa9e3d2d2b4efdbb9a5e8..0000000000000000000000000000000000000000
--- a/spaces/nikitaPDL2023/assignment4/detectron2/projects/DensePose/densepose/converters/chart_output_hflip.py
+++ /dev/null
@@ -1,71 +0,0 @@
-# Copyright (c) Facebook, Inc. and its affiliates.
-from dataclasses import fields
-import torch
-
-from densepose.structures import DensePoseChartPredictorOutput, DensePoseTransformData
-
-
-def densepose_chart_predictor_output_hflip(
- densepose_predictor_output: DensePoseChartPredictorOutput,
- transform_data: DensePoseTransformData,
-) -> DensePoseChartPredictorOutput:
- """
- Change to take into account a Horizontal flip.
- """
- if len(densepose_predictor_output) > 0:
-
- PredictorOutput = type(densepose_predictor_output)
- output_dict = {}
-
- for field in fields(densepose_predictor_output):
- field_value = getattr(densepose_predictor_output, field.name)
- # flip tensors
- if isinstance(field_value, torch.Tensor):
- setattr(densepose_predictor_output, field.name, torch.flip(field_value, [3]))
-
- densepose_predictor_output = _flip_iuv_semantics_tensor(
- densepose_predictor_output, transform_data
- )
- densepose_predictor_output = _flip_segm_semantics_tensor(
- densepose_predictor_output, transform_data
- )
-
- for field in fields(densepose_predictor_output):
- output_dict[field.name] = getattr(densepose_predictor_output, field.name)
-
- return PredictorOutput(**output_dict)
- else:
- return densepose_predictor_output
-
-
-def _flip_iuv_semantics_tensor(
- densepose_predictor_output: DensePoseChartPredictorOutput,
- dp_transform_data: DensePoseTransformData,
-) -> DensePoseChartPredictorOutput:
- point_label_symmetries = dp_transform_data.point_label_symmetries
- uv_symmetries = dp_transform_data.uv_symmetries
-
- N, C, H, W = densepose_predictor_output.u.shape
- u_loc = (densepose_predictor_output.u[:, 1:, :, :].clamp(0, 1) * 255).long()
- v_loc = (densepose_predictor_output.v[:, 1:, :, :].clamp(0, 1) * 255).long()
- Iindex = torch.arange(C - 1, device=densepose_predictor_output.u.device)[
- None, :, None, None
- ].expand(N, C - 1, H, W)
- densepose_predictor_output.u[:, 1:, :, :] = uv_symmetries["U_transforms"][Iindex, v_loc, u_loc]
- densepose_predictor_output.v[:, 1:, :, :] = uv_symmetries["V_transforms"][Iindex, v_loc, u_loc]
-
- for el in ["fine_segm", "u", "v"]:
- densepose_predictor_output.__dict__[el] = densepose_predictor_output.__dict__[el][
- :, point_label_symmetries, :, :
- ]
- return densepose_predictor_output
-
-
-def _flip_segm_semantics_tensor(
- densepose_predictor_output: DensePoseChartPredictorOutput, dp_transform_data
-):
- if densepose_predictor_output.coarse_segm.shape[1] > 2:
- densepose_predictor_output.coarse_segm = densepose_predictor_output.coarse_segm[
- :, dp_transform_data.mask_label_symmetries, :, :
- ]
- return densepose_predictor_output
diff --git a/spaces/notsq/diffuse-the-rest/build/_app/immutable/assets/+layout-7c2f4ad7.css b/spaces/notsq/diffuse-the-rest/build/_app/immutable/assets/+layout-7c2f4ad7.css
deleted file mode 100644
index 3d3b9c37a65b45a8c172cf493f95cf521ab680a7..0000000000000000000000000000000000000000
--- a/spaces/notsq/diffuse-the-rest/build/_app/immutable/assets/+layout-7c2f4ad7.css
+++ /dev/null
@@ -1 +0,0 @@
-*,:before,:after{box-sizing:border-box;border-width:0;border-style:solid;border-color:#e5e7eb}:before,:after{--tw-content: ""}html{line-height:1.5;-webkit-text-size-adjust:100%;-moz-tab-size:4;-o-tab-size:4;tab-size:4;font-family:ui-sans-serif,system-ui,-apple-system,BlinkMacSystemFont,Segoe UI,Roboto,Helvetica Neue,Arial,Noto Sans,sans-serif,"Apple Color Emoji","Segoe UI Emoji",Segoe UI Symbol,"Noto Color Emoji"}body{margin:0;line-height:inherit}hr{height:0;color:inherit;border-top-width:1px}abbr:where([title]){-webkit-text-decoration:underline dotted;text-decoration:underline dotted}h1,h2,h3,h4,h5,h6{font-size:inherit;font-weight:inherit}a{color:inherit;text-decoration:inherit}b,strong{font-weight:bolder}code,kbd,samp,pre{font-family:ui-monospace,SFMono-Regular,Menlo,Monaco,Consolas,Liberation Mono,Courier New,monospace;font-size:1em}small{font-size:80%}sub,sup{font-size:75%;line-height:0;position:relative;vertical-align:baseline}sub{bottom:-.25em}sup{top:-.5em}table{text-indent:0;border-color:inherit;border-collapse:collapse}button,input,optgroup,select,textarea{font-family:inherit;font-size:100%;font-weight:inherit;line-height:inherit;color:inherit;margin:0;padding:0}button,select{text-transform:none}button,[type=button],[type=reset],[type=submit]{-webkit-appearance:button;background-color:transparent;background-image:none}:-moz-focusring{outline:auto}:-moz-ui-invalid{box-shadow:none}progress{vertical-align:baseline}::-webkit-inner-spin-button,::-webkit-outer-spin-button{height:auto}[type=search]{-webkit-appearance:textfield;outline-offset:-2px}::-webkit-search-decoration{-webkit-appearance:none}::-webkit-file-upload-button{-webkit-appearance:button;font:inherit}summary{display:list-item}blockquote,dl,dd,h1,h2,h3,h4,h5,h6,hr,figure,p,pre{margin:0}fieldset{margin:0;padding:0}legend{padding:0}ol,ul,menu{list-style:none;margin:0;padding:0}textarea{resize:vertical}input::-moz-placeholder,textarea::-moz-placeholder{opacity:1;color:#9ca3af}input::placeholder,textarea::placeholder{opacity:1;color:#9ca3af}button,[role=button]{cursor:pointer}:disabled{cursor:default}img,svg,video,canvas,audio,iframe,embed,object{display:block;vertical-align:middle}img,video{max-width:100%;height:auto}*,:before,:after{--tw-border-spacing-x: 0;--tw-border-spacing-y: 0;--tw-translate-x: 0;--tw-translate-y: 0;--tw-rotate: 0;--tw-skew-x: 0;--tw-skew-y: 0;--tw-scale-x: 1;--tw-scale-y: 1;--tw-pan-x: ;--tw-pan-y: ;--tw-pinch-zoom: ;--tw-scroll-snap-strictness: proximity;--tw-ordinal: ;--tw-slashed-zero: ;--tw-numeric-figure: ;--tw-numeric-spacing: ;--tw-numeric-fraction: ;--tw-ring-inset: ;--tw-ring-offset-width: 0px;--tw-ring-offset-color: #fff;--tw-ring-color: rgb(59 130 246 / .5);--tw-ring-offset-shadow: 0 0 #0000;--tw-ring-shadow: 0 0 #0000;--tw-shadow: 0 0 #0000;--tw-shadow-colored: 0 0 #0000;--tw-blur: ;--tw-brightness: ;--tw-contrast: ;--tw-grayscale: ;--tw-hue-rotate: ;--tw-invert: ;--tw-saturate: ;--tw-sepia: ;--tw-drop-shadow: ;--tw-backdrop-blur: ;--tw-backdrop-brightness: ;--tw-backdrop-contrast: ;--tw-backdrop-grayscale: ;--tw-backdrop-hue-rotate: ;--tw-backdrop-invert: ;--tw-backdrop-opacity: ;--tw-backdrop-saturate: ;--tw-backdrop-sepia: }::-webkit-backdrop{--tw-border-spacing-x: 0;--tw-border-spacing-y: 0;--tw-translate-x: 0;--tw-translate-y: 0;--tw-rotate: 0;--tw-skew-x: 0;--tw-skew-y: 0;--tw-scale-x: 1;--tw-scale-y: 1;--tw-pan-x: ;--tw-pan-y: ;--tw-pinch-zoom: ;--tw-scroll-snap-strictness: proximity;--tw-ordinal: ;--tw-slashed-zero: ;--tw-numeric-figure: ;--tw-numeric-spacing: ;--tw-numeric-fraction: ;--tw-ring-inset: ;--tw-ring-offset-width: 0px;--tw-ring-offset-color: #fff;--tw-ring-color: rgb(59 130 246 / .5);--tw-ring-offset-shadow: 0 0 #0000;--tw-ring-shadow: 0 0 #0000;--tw-shadow: 0 0 #0000;--tw-shadow-colored: 0 0 #0000;--tw-blur: ;--tw-brightness: ;--tw-contrast: ;--tw-grayscale: ;--tw-hue-rotate: ;--tw-invert: ;--tw-saturate: ;--tw-sepia: ;--tw-drop-shadow: ;--tw-backdrop-blur: ;--tw-backdrop-brightness: ;--tw-backdrop-contrast: ;--tw-backdrop-grayscale: ;--tw-backdrop-hue-rotate: ;--tw-backdrop-invert: ;--tw-backdrop-opacity: ;--tw-backdrop-saturate: ;--tw-backdrop-sepia: }::backdrop{--tw-border-spacing-x: 0;--tw-border-spacing-y: 0;--tw-translate-x: 0;--tw-translate-y: 0;--tw-rotate: 0;--tw-skew-x: 0;--tw-skew-y: 0;--tw-scale-x: 1;--tw-scale-y: 1;--tw-pan-x: ;--tw-pan-y: ;--tw-pinch-zoom: ;--tw-scroll-snap-strictness: proximity;--tw-ordinal: ;--tw-slashed-zero: ;--tw-numeric-figure: ;--tw-numeric-spacing: ;--tw-numeric-fraction: ;--tw-ring-inset: ;--tw-ring-offset-width: 0px;--tw-ring-offset-color: #fff;--tw-ring-color: rgb(59 130 246 / .5);--tw-ring-offset-shadow: 0 0 #0000;--tw-ring-shadow: 0 0 #0000;--tw-shadow: 0 0 #0000;--tw-shadow-colored: 0 0 #0000;--tw-blur: ;--tw-brightness: ;--tw-contrast: ;--tw-grayscale: ;--tw-hue-rotate: ;--tw-invert: ;--tw-saturate: ;--tw-sepia: ;--tw-drop-shadow: ;--tw-backdrop-blur: ;--tw-backdrop-brightness: ;--tw-backdrop-contrast: ;--tw-backdrop-grayscale: ;--tw-backdrop-hue-rotate: ;--tw-backdrop-invert: ;--tw-backdrop-opacity: ;--tw-backdrop-saturate: ;--tw-backdrop-sepia: }.prose-sm{font-size:.875rem;line-height:1.7142857}.prose-sm :where(p):not(:where([class~="not-prose"] *)){margin-top:1.1428571em;margin-bottom:1.1428571em}.prose-sm :where([class~="lead"]):not(:where([class~="not-prose"] *)){font-size:1.2857143em;line-height:1.5555556;margin-top:.8888889em;margin-bottom:.8888889em}.prose-sm :where(blockquote):not(:where([class~="not-prose"] *)){margin-top:1.3333333em;margin-bottom:1.3333333em;padding-left:1.1111111em}.prose-sm :where(h1):not(:where([class~="not-prose"] *)){font-size:2.1428571em;margin-top:0;margin-bottom:.8em;line-height:1.2}.prose-sm :where(h2):not(:where([class~="not-prose"] *)){font-size:1.4285714em;margin-top:1.6em;margin-bottom:.8em;line-height:1.4}.prose-sm :where(h3):not(:where([class~="not-prose"] *)){font-size:1.2857143em;margin-top:1.5555556em;margin-bottom:.4444444em;line-height:1.5555556}.prose-sm :where(h4):not(:where([class~="not-prose"] *)){margin-top:1.4285714em;margin-bottom:.5714286em;line-height:1.4285714}.prose-sm :where(img):not(:where([class~="not-prose"] *)){margin-top:1.7142857em;margin-bottom:1.7142857em}.prose-sm :where(video):not(:where([class~="not-prose"] *)){margin-top:1.7142857em;margin-bottom:1.7142857em}.prose-sm :where(figure):not(:where([class~="not-prose"] *)){margin-top:1.7142857em;margin-bottom:1.7142857em}.prose-sm :where(figure > *):not(:where([class~="not-prose"] *)){margin-top:0;margin-bottom:0}.prose-sm :where(figcaption):not(:where([class~="not-prose"] *)){font-size:.8571429em;line-height:1.3333333;margin-top:.6666667em}.prose-sm :where(code):not(:where([class~="not-prose"] *)){font-size:.8571429em}.prose-sm :where(h2 code):not(:where([class~="not-prose"] *)){font-size:.9em}.prose-sm :where(h3 code):not(:where([class~="not-prose"] *)){font-size:.8888889em}.prose-sm :where(pre):not(:where([class~="not-prose"] *)){font-size:.8571429em;line-height:1.6666667;margin-top:1.6666667em;margin-bottom:1.6666667em;border-radius:.25rem;padding:.6666667em 1em}.prose-sm :where(ol):not(:where([class~="not-prose"] *)){margin-top:1.1428571em;margin-bottom:1.1428571em;padding-left:1.5714286em}.prose-sm :where(ul):not(:where([class~="not-prose"] *)){margin-top:1.1428571em;margin-bottom:1.1428571em;padding-left:1.5714286em}.prose-sm :where(li):not(:where([class~="not-prose"] *)){margin-top:.2857143em;margin-bottom:.2857143em}.prose-sm :where(ol > li):not(:where([class~="not-prose"] *)){padding-left:.4285714em}.prose-sm :where(ul > li):not(:where([class~="not-prose"] *)){padding-left:.4285714em}.prose-sm :where(.prose > ul > li p):not(:where([class~="not-prose"] *)){margin-top:.5714286em;margin-bottom:.5714286em}.prose-sm :where(.prose > ul > li > *:first-child):not(:where([class~="not-prose"] *)){margin-top:1.1428571em}.prose-sm :where(.prose > ul > li > *:last-child):not(:where([class~="not-prose"] *)){margin-bottom:1.1428571em}.prose-sm :where(.prose > ol > li > *:first-child):not(:where([class~="not-prose"] *)){margin-top:1.1428571em}.prose-sm :where(.prose > ol > li > *:last-child):not(:where([class~="not-prose"] *)){margin-bottom:1.1428571em}.prose-sm :where(ul ul,ul ol,ol ul,ol ol):not(:where([class~="not-prose"] *)){margin-top:.5714286em;margin-bottom:.5714286em}.prose-sm :where(hr):not(:where([class~="not-prose"] *)){margin-top:2.8571429em;margin-bottom:2.8571429em}.prose-sm :where(hr + *):not(:where([class~="not-prose"] *)){margin-top:0}.prose-sm :where(h2 + *):not(:where([class~="not-prose"] *)){margin-top:0}.prose-sm :where(h3 + *):not(:where([class~="not-prose"] *)){margin-top:0}.prose-sm :where(h4 + *):not(:where([class~="not-prose"] *)){margin-top:0}.prose-sm :where(table):not(:where([class~="not-prose"] *)){font-size:.8571429em;line-height:1.5}.prose-sm :where(thead th):not(:where([class~="not-prose"] *)){padding-right:1em;padding-bottom:.6666667em;padding-left:1em}.prose-sm :where(thead th:first-child):not(:where([class~="not-prose"] *)){padding-left:0}.prose-sm :where(thead th:last-child):not(:where([class~="not-prose"] *)){padding-right:0}.prose-sm :where(tbody td,tfoot td):not(:where([class~="not-prose"] *)){padding:.6666667em 1em}.prose-sm :where(tbody td:first-child,tfoot td:first-child):not(:where([class~="not-prose"] *)){padding-left:0}.prose-sm :where(tbody td:last-child,tfoot td:last-child):not(:where([class~="not-prose"] *)){padding-right:0}.prose-sm :where(.prose > :first-child):not(:where([class~="not-prose"] *)){margin-top:0}.prose-sm :where(.prose > :last-child):not(:where([class~="not-prose"] *)){margin-bottom:0}.pointer-events-none{pointer-events:none}.my-8{margin-top:2rem;margin-bottom:2rem}.mt-3{margin-top:.75rem}.mt-4{margin-top:1rem}.mt-2{margin-top:.5rem}.mb-8{margin-bottom:2rem}.inline-block{display:inline-block}.inline{display:inline}.flex{display:flex}.hidden{display:none}.max-h-\[500px\]{max-height:500px}.min-h-\[42px\]{min-height:42px}.w-\[12\.5rem\]{width:12.5rem}.w-full{width:100%}.\!w-\[181px\]{width:181px!important}@-webkit-keyframes spin{to{transform:rotate(360deg)}}@keyframes spin{to{transform:rotate(360deg)}}.animate-spin{-webkit-animation:spin 1s linear infinite;animation:spin 1s linear infinite}@-webkit-keyframes pulse{50%{opacity:.5}}@keyframes pulse{50%{opacity:.5}}.animate-pulse{-webkit-animation:pulse 2s cubic-bezier(.4,0,.6,1) infinite;animation:pulse 2s cubic-bezier(.4,0,.6,1) infinite}.cursor-pointer{cursor:pointer}.resize-y{resize:vertical}.flex-col{flex-direction:column}.flex-wrap{flex-wrap:wrap}.items-start{align-items:flex-start}.items-center{align-items:center}.justify-end{justify-content:flex-end}.justify-center{justify-content:center}.gap-x-2{-moz-column-gap:.5rem;column-gap:.5rem}.gap-x-4{-moz-column-gap:1rem;column-gap:1rem}.gap-y-2{row-gap:.5rem}.overflow-auto{overflow:auto}.whitespace-pre-wrap{white-space:pre-wrap}.rounded-full{border-radius:9999px}.border-\[1\.2px\]{border-width:1.2px}.border{border-width:1px}.border-gray-200{--tw-border-opacity: 1;border-color:rgb(229 231 235 / var(--tw-border-opacity))}.bg-black{--tw-bg-opacity: 1;background-color:rgb(0 0 0 / var(--tw-bg-opacity))}.bg-blue-500{--tw-bg-opacity: 1;background-color:rgb(59 130 246 / var(--tw-bg-opacity))}.bg-slate-200{--tw-bg-opacity: 1;background-color:rgb(226 232 240 / var(--tw-bg-opacity))}.px-2{padding-left:.5rem;padding-right:.5rem}.py-1{padding-top:.25rem;padding-bottom:.25rem}.py-2{padding-top:.5rem;padding-bottom:.5rem}.px-3{padding-left:.75rem;padding-right:.75rem}.py-\[0\.555rem\]{padding-top:.555rem;padding-bottom:.555rem}.px-4{padding-left:1rem;padding-right:1rem}.px-1\.5{padding-left:.375rem;padding-right:.375rem}.px-1{padding-left:.25rem;padding-right:.25rem}.text-center{text-align:center}.font-semibold{font-weight:600}.font-bold{font-weight:700}.text-white{--tw-text-opacity: 1;color:rgb(255 255 255 / var(--tw-text-opacity))}.opacity-25{opacity:.25}.opacity-75{opacity:.75}.opacity-50{opacity:.5}.shadow-inner{--tw-shadow: inset 0 2px 4px 0 rgb(0 0 0 / .05);--tw-shadow-colored: inset 0 2px 4px 0 var(--tw-shadow-color);box-shadow:var(--tw-ring-offset-shadow, 0 0 #0000),var(--tw-ring-shadow, 0 0 #0000),var(--tw-shadow)}.outline-none{outline:2px solid transparent;outline-offset:2px}a{-webkit-text-decoration-line:underline!important;text-decoration-line:underline!important}.drawing-board-controls{--tw-border-spacing-x: .125rem !important;--tw-border-spacing-y: .125rem !important;border-spacing:var(--tw-border-spacing-x) var(--tw-border-spacing-y)!important}@media (min-width: 768px){.drawing-board-controls{--tw-border-spacing-x: .5rem !important;--tw-border-spacing-y: .5rem !important;border-spacing:var(--tw-border-spacing-x) var(--tw-border-spacing-y)!important}}.hover\:bg-blue-700:hover{--tw-bg-opacity: 1;background-color:rgb(29 78 216 / var(--tw-bg-opacity))}@media (min-width: 816px){.desktop\:mt-\[34px\]{margin-top:34px}.desktop\:inline{display:inline}}@media (min-width: 768px){.md\:px-12{padding-left:3rem;padding-right:3rem}}@media (min-width: 1024px){.lg\:px-56{padding-left:14rem;padding-right:14rem}}
diff --git a/spaces/odettecantswim/rvc-mlbb-v2/lib/infer_pack/onnx_inference.py b/spaces/odettecantswim/rvc-mlbb-v2/lib/infer_pack/onnx_inference.py
deleted file mode 100644
index c78324cbc08414fffcc689f325312de0e51bd6b4..0000000000000000000000000000000000000000
--- a/spaces/odettecantswim/rvc-mlbb-v2/lib/infer_pack/onnx_inference.py
+++ /dev/null
@@ -1,143 +0,0 @@
-import onnxruntime
-import librosa
-import numpy as np
-import soundfile
-
-
-class ContentVec:
- def __init__(self, vec_path="pretrained/vec-768-layer-12.onnx", device=None):
- print("load model(s) from {}".format(vec_path))
- if device == "cpu" or device is None:
- providers = ["CPUExecutionProvider"]
- elif device == "cuda":
- providers = ["CUDAExecutionProvider", "CPUExecutionProvider"]
- elif device == "dml":
- providers = ["DmlExecutionProvider"]
- else:
- raise RuntimeError("Unsportted Device")
- self.model = onnxruntime.InferenceSession(vec_path, providers=providers)
-
- def __call__(self, wav):
- return self.forward(wav)
-
- def forward(self, wav):
- feats = wav
- if feats.ndim == 2: # double channels
- feats = feats.mean(-1)
- assert feats.ndim == 1, feats.ndim
- feats = np.expand_dims(np.expand_dims(feats, 0), 0)
- onnx_input = {self.model.get_inputs()[0].name: feats}
- logits = self.model.run(None, onnx_input)[0]
- return logits.transpose(0, 2, 1)
-
-
-def get_f0_predictor(f0_predictor, hop_length, sampling_rate, **kargs):
- if f0_predictor == "pm":
- from lib.infer_pack.modules.F0Predictor.PMF0Predictor import PMF0Predictor
-
- f0_predictor_object = PMF0Predictor(
- hop_length=hop_length, sampling_rate=sampling_rate
- )
- elif f0_predictor == "harvest":
- from lib.infer_pack.modules.F0Predictor.HarvestF0Predictor import HarvestF0Predictor
-
- f0_predictor_object = HarvestF0Predictor(
- hop_length=hop_length, sampling_rate=sampling_rate
- )
- elif f0_predictor == "dio":
- from lib.infer_pack.modules.F0Predictor.DioF0Predictor import DioF0Predictor
-
- f0_predictor_object = DioF0Predictor(
- hop_length=hop_length, sampling_rate=sampling_rate
- )
- else:
- raise Exception("Unknown f0 predictor")
- return f0_predictor_object
-
-
-class OnnxRVC:
- def __init__(
- self,
- model_path,
- sr=40000,
- hop_size=512,
- vec_path="vec-768-layer-12",
- device="cpu",
- ):
- vec_path = f"pretrained/{vec_path}.onnx"
- self.vec_model = ContentVec(vec_path, device)
- if device == "cpu" or device is None:
- providers = ["CPUExecutionProvider"]
- elif device == "cuda":
- providers = ["CUDAExecutionProvider", "CPUExecutionProvider"]
- elif device == "dml":
- providers = ["DmlExecutionProvider"]
- else:
- raise RuntimeError("Unsportted Device")
- self.model = onnxruntime.InferenceSession(model_path, providers=providers)
- self.sampling_rate = sr
- self.hop_size = hop_size
-
- def forward(self, hubert, hubert_length, pitch, pitchf, ds, rnd):
- onnx_input = {
- self.model.get_inputs()[0].name: hubert,
- self.model.get_inputs()[1].name: hubert_length,
- self.model.get_inputs()[2].name: pitch,
- self.model.get_inputs()[3].name: pitchf,
- self.model.get_inputs()[4].name: ds,
- self.model.get_inputs()[5].name: rnd,
- }
- return (self.model.run(None, onnx_input)[0] * 32767).astype(np.int16)
-
- def inference(
- self,
- raw_path,
- sid,
- f0_method="dio",
- f0_up_key=0,
- pad_time=0.5,
- cr_threshold=0.02,
- ):
- f0_min = 50
- f0_max = 1100
- f0_mel_min = 1127 * np.log(1 + f0_min / 700)
- f0_mel_max = 1127 * np.log(1 + f0_max / 700)
- f0_predictor = get_f0_predictor(
- f0_method,
- hop_length=self.hop_size,
- sampling_rate=self.sampling_rate,
- threshold=cr_threshold,
- )
- wav, sr = librosa.load(raw_path, sr=self.sampling_rate)
- org_length = len(wav)
- if org_length / sr > 50.0:
- raise RuntimeError("Reached Max Length")
-
- wav16k = librosa.resample(wav, orig_sr=self.sampling_rate, target_sr=16000)
- wav16k = wav16k
-
- hubert = self.vec_model(wav16k)
- hubert = np.repeat(hubert, 2, axis=2).transpose(0, 2, 1).astype(np.float32)
- hubert_length = hubert.shape[1]
-
- pitchf = f0_predictor.compute_f0(wav, hubert_length)
- pitchf = pitchf * 2 ** (f0_up_key / 12)
- pitch = pitchf.copy()
- f0_mel = 1127 * np.log(1 + pitch / 700)
- f0_mel[f0_mel > 0] = (f0_mel[f0_mel > 0] - f0_mel_min) * 254 / (
- f0_mel_max - f0_mel_min
- ) + 1
- f0_mel[f0_mel <= 1] = 1
- f0_mel[f0_mel > 255] = 255
- pitch = np.rint(f0_mel).astype(np.int64)
-
- pitchf = pitchf.reshape(1, len(pitchf)).astype(np.float32)
- pitch = pitch.reshape(1, len(pitch))
- ds = np.array([sid]).astype(np.int64)
-
- rnd = np.random.randn(1, 192, hubert_length).astype(np.float32)
- hubert_length = np.array([hubert_length]).astype(np.int64)
-
- out_wav = self.forward(hubert, hubert_length, pitch, pitchf, ds, rnd).squeeze()
- out_wav = np.pad(out_wav, (0, 2 * self.hop_size), "constant")
- return out_wav[0:org_length]
diff --git a/spaces/ofig/live-lm-critic/critic/critic.py b/spaces/ofig/live-lm-critic/critic/critic.py
deleted file mode 100644
index 5e629d297cb60f3e635e5225b137166f171ecc62..0000000000000000000000000000000000000000
--- a/spaces/ofig/live-lm-critic/critic/critic.py
+++ /dev/null
@@ -1,318 +0,0 @@
-import sys
-import torch
-import random
-import hashlib
-import numpy as np
-from tqdm import tqdm
-from transformers import GPT2Tokenizer, GPT2Model, GPT2LMHeadModel
-from transformers import OPTForCausalLM, GPTNeoForCausalLM
-from transformers import RobertaTokenizer, RobertaForCausalLM, RobertaConfig
-from transformers import XLMRobertaTokenizer, XLMRobertaForCausalLM, XLMRobertaConfig
-from transformers import BartTokenizer, BartForCausalLM
-import nltk
-import pandas as pd
-nltk.download('punkt')
-
-sys.path.insert(0, '.')
-from critic.perturbations import get_local_neighbors_char_level, get_local_neighbors_word_level
-from utils.spacy_tokenizer import spacy_tokenize_gec
-import streamlit as st
-
-st.subheader('Exploring Unsupervised Grammatical Error Correction with Transformer-Based Models')
-st.write('This live demonstration is adapted from the paper [LM-Critic: Language Models for Unsupervised Grammatical Error Correction](https://aclanthology.org/2021.emnlp-main.611.pdf) (EMNLP 2021) by Michihiro Yasunaga, Jure Leskovec, Percy Liang.')
-st.write('Enter any sentence in the text box, press submit, and see the grammatical scoring and judgement results outputted by LM-Critic using different LMs displayed below.')
-
-def get_gpt2_loss(model, tokenizer, input_ids, attention_mask, labels):
- with torch.no_grad():
- outputs = model(input_ids=input_ids, attention_mask=attention_mask, labels=labels)
- lm_logits = outputs[1] #[bsize, seqlen, vocab]
- if labels is not None:
- shift_logits = lm_logits[..., :-1, :].contiguous()
- shift_labels = labels[..., 1:].contiguous()
- shift_mask = attention_mask[..., 1:].contiguous()
- loss_fct = torch.nn.CrossEntropyLoss(reduction='none')
- bsize, seqlen = input_ids.size()
- loss = loss_fct(shift_logits.view(-1, shift_logits.size(-1)), shift_labels.view(-1)).view(bsize, seqlen-1)
- loss = (loss * shift_mask).sum(dim=1) #[bsize, ]
- return loss
-
-
-MAX_LENGTH = 66
-
-def run_gpt2(sents, model, tokenizer, cuda=False, model_name=None):
- assert isinstance(sents, list)
- _sents = [tokenizer.bos_token + s for s in sents]
- inputs = tokenizer(_sents, return_tensors="pt", padding=True)
- if inputs['input_ids'].size(1) > MAX_LENGTH:
- return None
- if cuda:
- inputs = {k: v.cuda() for k, v in inputs.items()}
- loss = get_gpt2_loss(model, tokenizer, input_ids=inputs['input_ids'], attention_mask=inputs['attention_mask'], labels=inputs['input_ids'])
- logps = - loss.detach().cpu()
- return logps
-
-
-def gpt2_critic_char_level_only(sent, verbose=1, cuda=False, fp16=True, seed='auto', n_samples=100):
- return_string = []
- if seed == 'auto':
- seed = int(hashlib.md5(sent.encode()).hexdigest(), 16) % (2**32) #Seed must be between 0 and 2**32 - 1
- if verbose > 1:
- print ('seed', seed)
- np.random.seed(seed); random.seed(seed)
- is_good = True
- for _ in range(1):
- sent_perturbations = get_local_neighbors_char_level(sent, max_n_samples=n_samples)
- if verbose > 1:
- print ("#sent_perturbations (char-level)", len(sent_perturbations))
- return_string.append(f"#sent_perturbations (char-level){len(sent_perturbations)}\n")
- sents = [sent] + list(sent_perturbations)
- if fp16:
- with torch.cuda.amp.autocast():
- logps = run_gpt2(sents, cuda)
- else:
- logps = run_gpt2(sents, cuda)
- if logps is None:
- if verbose:
- print ('Invalid input. Maybe the sentence is too long.')
- return_string.append('Invalid input. Maybe the sentence is too long.\n')
- return None
- best_idx = int(logps.argmax())
- if best_idx != 0:
- is_good = False
- break
- if verbose:
- if is_good:
- print ('Good! Your sentence log(p) = {:.3f}'.format(float(logps[0])))
- return_string.append('Good! Your sentence log(p) = {:.3f}\n'.format(float(logps[0])))
- else:
- print ('Bad! Your sentence log(p) = {:.3f}'.format(float(logps[0])))
- return_string.append('Bad! Your sentence log(p) = {:.3f}\n'.format(float(logps[0])))
- print ('Neighbor sentence with highest log(p): {} (= {:.3f})'.format(sents[best_idx], float(logps[best_idx])))
- return_string.append('Neighbor sentence with highest log(p): {} (= {:.3f})\n'.format(sents[best_idx], float(logps[best_idx])))
- counter_example = None
- if not is_good:
- counter_example = [sents[best_idx], float(logps[best_idx])]
- return is_good, float(logps[0]), counter_example
-
-
-def gpt2_critic(sent, model, tokenizer, verbose=1, cuda=False, fp16=True, seed='auto', n_samples=100, word_level_mode='refine'):
- return_string = []
- if seed == 'auto':
- seed = int(hashlib.md5(sent.encode()).hexdigest(), 16) % (2**32) #Seed must be between 0 and 2**32 - 1
- if verbose > 1:
- print ('seed', seed)
- return_string.append(f'seed{seed}\n')
- np.random.seed(seed); random.seed(seed)
- sent_toked = spacy_tokenize_gec(sent)
- is_good = True
- for _ in range(1):
- sent_perturbations_w, orig_sent = get_local_neighbors_word_level(sent_toked, max_n_samples=n_samples//2, mode=word_level_mode)
- sent_perturbations_c = get_local_neighbors_char_level(orig_sent, max_n_samples=n_samples//2)
- if verbose > 1:
- print ("#sent_perturbations (char-level)", len(sent_perturbations_c))
- return_string.append("#sent_perturbations (char-level)\n", len(sent_perturbations_c))
- print ("#sent_perturbations (word-level)", len(sent_perturbations_w))
- return_string.append("#sent_perturbations (word-level)\n", len(sent_perturbations_w))
- sents = [orig_sent] + list(sent_perturbations_c.union(sent_perturbations_w))
- if fp16:
- with torch.cuda.amp.autocast():
- logps = run_gpt2(sents, model, tokenizer, cuda)
- else:
- logps = run_gpt2(sents, model, tokenizer, cuda)
- if logps is None:
- if verbose:
- print ('Invalid input. Maybe the sentence is too long.')
- return_string.append('Invalid input. Maybe the sentence is too long.\n')
- return None
- best_idx = int(logps.argmax())
- if best_idx != 0:
- is_good = False
- break
- if verbose:
- if is_good:
- print ('Good! Your sentence log(p) = {:.3f}'.format(float(logps[0])))
- return_string.append('Good! Your sentence log(p) = {:.3f}\n'.format(float(logps[0])))
- else:
- print ('Bad! Your sentence log(p) = {:.3f}'.format(float(logps[0])))
- return_string.append('Bad! Your sentence log(p) = {:.3f}\n'.format(float(logps[0])))
- print ('Neighbor sentence with highest log(p): {} (= {:.3f})'.format(sents[best_idx], float(logps[best_idx])))
- return_string.append('Neighbor sentence with highest log(p): {} (= {:.3f})\n'.format(sents[best_idx], float(logps[best_idx])))
- counter_example = None
- if not is_good:
- counter_example = [sents[best_idx], float(logps[best_idx])]
- return is_good, float(logps[0]), counter_example, return_string
-
-def gpt2():
- ## GPT-2 LM (original LM-critic)
- placeholder_lm_name = st.empty()
- model_name_gpt2 = 'gpt2'
- nice_name_gpt2 = "GPT-2"
- placeholder_lm_name.text(f"Initializing {nice_name_gpt2}...")
- tokenizer_gpt2 = GPT2Tokenizer.from_pretrained(model_name_gpt2)
- tokenizer_gpt2.pad_token = tokenizer_gpt2.eos_token
- model_gpt2 = GPT2LMHeadModel.from_pretrained(model_name_gpt2)
- model_gpt2.eval()
- model_gpt2.cpu()
- placeholder_lm_name.empty()
- st.session_state["model_gpt2"] = model_gpt2
- st.session_state["tokenizer_gpt2"] = tokenizer_gpt2
- st.session_state["nice_name_gpt2"] = nice_name_gpt2
-
-def opt():
- ## OPT LM
- placeholder_lm_name = st.empty()
- model_name_opt = "facebook/opt-350m"
- nice_name_opt = "OPT"
- placeholder_lm_name.text(f"Initializing {nice_name_opt}...")
- model_opt = OPTForCausalLM.from_pretrained(model_name_opt)
- tokenizer_opt = GPT2Tokenizer.from_pretrained(model_name_opt)
- tokenizer_opt.pad_token = tokenizer_opt.eos_token
- model_opt.eval()
- model_opt.cpu()
- placeholder_lm_name.empty()
- st.session_state["model_opt"] = model_opt
- st.session_state["tokenizer_opt"] = tokenizer_opt
- st.session_state["nice_name_opt"] = nice_name_opt
-
-def gpt_neo():
- ## GPT NEO
- placeholder_lm_name = st.empty()
- model_name_gptneo = "EleutherAI/gpt-neo-1.3B"
- nice_name_gptneo = "GPT NEO"
- placeholder_lm_name.text(f"Initializing {nice_name_gptneo}...")
- model_gptneo = GPTNeoForCausalLM.from_pretrained(model_name_gptneo)
- tokenizer_gptneo = GPT2Tokenizer.from_pretrained(model_name_gptneo)
- tokenizer_gptneo.pad_token = tokenizer_gptneo.eos_token
- model_gptneo.eval()
- model_gptneo.cpu()
- placeholder_lm_name.empty()
- st.session_state["model_gptneo"] = model_gptneo
- st.session_state["tokenizer_gptneo"] = tokenizer_gptneo
- st.session_state["nice_name_gptneo"] = nice_name_gptneo
-
-def roberta():
- ## RoBERTa
- placeholder_lm_name = st.empty()
- model_name_roberta = "roberta-base"
- nice_name_roberta = "RoBERTa"
- placeholder_lm_name.text(f"Initializing {nice_name_roberta}...")
- tokenizer_roberta = RobertaTokenizer.from_pretrained(model_name_roberta)
- config_roberta = RobertaConfig.from_pretrained(model_name_roberta)
- config_roberta.is_decoder = True
- model_roberta = RobertaForCausalLM.from_pretrained(model_name_roberta, config=config_roberta)
- tokenizer_roberta.pad_token = tokenizer_roberta.eos_token
- model_roberta.eval()
- model_roberta.cpu()
- placeholder_lm_name.empty()
- st.session_state["model_roberta"] = model_roberta
- st.session_state["tokenizer_roberta"] = tokenizer_roberta
- st.session_state["nice_name_roberta"] = nice_name_roberta
-
-def bart():
- ## BART
- placeholder_lm_name = st.empty()
- model_name_bart = "facebook/bart-base"
- nice_name_bart = "BART"
- placeholder_lm_name.text(f"Initializing {nice_name_bart}...")
- tokenizer_bart = BartTokenizer.from_pretrained(model_name_bart)
- model_bart = BartForCausalLM.from_pretrained(model_name_bart, add_cross_attention=False)
- assert model_bart.config.is_decoder, f"{model_bart.__class__} has to be configured as a decoder."
- tokenizer_bart.pad_token = tokenizer_bart.eos_token
- model_bart.eval()
- model_bart.cpu()
- placeholder_lm_name.empty()
- st.session_state["model_bart"] = model_bart
- st.session_state["tokenizer_bart"] = tokenizer_bart
- st.session_state["nice_name_bart"] = nice_name_bart
-
-def xlm_roberta():
- ## XLM RoBERTa
- placeholder_lm_name = st.empty()
- model_name_xlmroberta = 'xlm-roberta-base'
- nice_name_xlmroberta = 'XLM RoBERTa'
- placeholder_lm_name.text(f"Initializing {nice_name_xlmroberta}...")
- tokenizer_xlmroberta = XLMRobertaTokenizer.from_pretrained(model_name_xlmroberta)
- config_xlmroberta = XLMRobertaConfig.from_pretrained(model_name_xlmroberta)
- config_xlmroberta.is_decoder = True
- model_xlmroberta = XLMRobertaForCausalLM.from_pretrained(model_name_xlmroberta, config=config_xlmroberta)
- tokenizer_xlmroberta.pad_token = tokenizer_xlmroberta.eos_token
- model_xlmroberta.eval()
- model_xlmroberta.cpu()
- placeholder_lm_name.empty()
- st.session_state["model_xlmroberta"] = model_xlmroberta
- st.session_state["tokenizer_xlmroberta"] = tokenizer_xlmroberta
- st.session_state["nice_name_xlmroberta"] = nice_name_xlmroberta
-
-def main():
- form = st.form(key='my_form')
- sent = form.text_input(label='Enter a sentence:', value="")
- submit = form.form_submit_button(label='Submit')
-
- if submit and sent != '':
- st.markdown(f"**Input Sentence**: {sent}")
- results = {}
-
- with st.spinner('Running with GPT-2 LM...'):
- ## GPT-2 LM (original LM-critic)
- if "nice_name_gpt2" not in st.session_state:
- gpt2()
- is_good, score, counter_example, return_string_GPT2 = gpt2_critic(sent, st.session_state['model_gpt2'], st.session_state['tokenizer_gpt2'])
- st.markdown("**Results with GPT-2 LM:**")
- st.write('\n'.join(return_string_GPT2))
- results[st.session_state['nice_name_gpt2']] = ["Good" if is_good else "Bad", str(round(score, 3)), "N/A" if not counter_example else str(counter_example[0]), "N/A" if not counter_example else str(round(counter_example[1], 3))]
-
- with st.spinner('Running with OPT LM...'):
- ## OPT LM
- if "nice_name_opt" not in st.session_state:
- opt()
- is_good, score, counter_example, return_string_OPT = gpt2_critic(sent, st.session_state['model_opt'], st.session_state['tokenizer_opt'])
- st.markdown("**Results with OPT LM:**")
- st.write('\n'.join(return_string_OPT))
- results[st.session_state['nice_name_opt']] = ["Good" if is_good else "Bad", str(round(score, 3)), "N/A" if not counter_example else str(counter_example[0]), "N/A" if not counter_example else str(round(counter_example[1], 3))]
-
- with st.spinner('Running with GPT NEO LM...'):
- ## GPT NEO
- if "nice_name_gptneo" not in st.session_state:
- gpt_neo()
- is_good, score, counter_example, return_string_GPTNEO = gpt2_critic(sent, st.session_state['model_gptneo'], st.session_state['tokenizer_gptneo'])
- st.markdown("**Results with GPT NEO LM:**")
- st.write('\n'.join(return_string_GPTNEO))
- results[st.session_state['nice_name_gptneo']] = ["Good" if is_good else "Bad", str(round(score, 3)), "N/A" if not counter_example else str(counter_example[0]), "N/A" if not counter_example else str(round(counter_example[1], 3))]
-
- with st.spinner('Running with RoBERTa LM...'):
- ## RoBERTa
- if "nice_name_roberta" not in st.session_state:
- roberta()
- is_good, score, counter_example, return_string_RoBERTa = gpt2_critic(sent, st.session_state['model_roberta'], st.session_state['tokenizer_roberta'])
- st.markdown("**Results with RoBERTa LM:**")
- st.write('\n'.join(return_string_RoBERTa))
- results[st.session_state['nice_name_roberta']] = ["Good" if is_good else "Bad", str(round(score, 3)), "N/A" if not counter_example else str(counter_example[0]), "N/A" if not counter_example else str(round(counter_example[1], 3))]
-
- with st.spinner('Running with BART LM...'):
- ## BART
- if "nice_name_bart" not in st.session_state:
- bart()
- is_good, score, counter_example, return_string_BART = gpt2_critic(sent, st.session_state['model_bart'], st.session_state['tokenizer_bart'])
- st.markdown("**Results with BART LM:**")
- st.write('\n'.join(return_string_BART))
- results[st.session_state['nice_name_bart']] = ["Good" if is_good else "Bad", str(round(score, 3)), "N/A" if not counter_example else str(counter_example[0]), "N/A" if not counter_example else str(round(counter_example[1], 3))]
-
- with st.spinner('Running with XLM RoBERTa LM...'):
- ## XLM RoBERTa
- if "nice_name_xlmroberta" not in st.session_state:
- xlm_roberta()
- is_good, score, counter_example, return_string_XLMRoBERTa = gpt2_critic(sent, st.session_state['model_xlmroberta'], st.session_state['tokenizer_xlmroberta'])
- st.markdown("**Results with XLM RoBERTa LM:**")
- st.write('\n'.join(return_string_XLMRoBERTa))
- results[st.session_state['nice_name_xlmroberta']] = ["Good" if is_good else "Bad", str(round(score, 3)), "N/A" if not counter_example else str(counter_example[0]), "N/A" if not counter_example else str(round(counter_example[1], 3))]
-
- df = pd.DataFrame.from_dict(results,
- orient = 'index',
- columns=['Judgement', 'Score (log(p))', 'Neighbor sentence with highest score (log(p))', 'Neighbor sentence score (log(p))'])
- st.markdown("**Tabular summary of results:**")
- st.table(df)
-
- st.write("Input another sentence!")
-
-if __name__ == '__main__':
- main()
\ No newline at end of file
diff --git a/spaces/oliver2023/chatgpt-on-wechat/plugins/godcmd/README.md b/spaces/oliver2023/chatgpt-on-wechat/plugins/godcmd/README.md
deleted file mode 100644
index 00a4f8d59138894513b9f1deec47d44c9b3dd680..0000000000000000000000000000000000000000
--- a/spaces/oliver2023/chatgpt-on-wechat/plugins/godcmd/README.md
+++ /dev/null
@@ -1,12 +0,0 @@
-## 插件说明
-
-指令插件
-
-## 插件使用
-
-将`config.json.template`复制为`config.json`,并修改其中`password`的值为口令。
-
-在私聊中可使用`#auth`指令,输入口令进行管理员认证,详细指令请输入`#help`查看帮助文档:
-
-`#auth <口令>` - 管理员认证。
-`#help` - 输出帮助文档,是否是管理员和是否是在群聊中会影响帮助文档的输出内容。
diff --git a/spaces/oniati/mrt/README.md b/spaces/oniati/mrt/README.md
deleted file mode 100644
index b0a208ccb0c63a67e5850786d8256e4086e0b03e..0000000000000000000000000000000000000000
--- a/spaces/oniati/mrt/README.md
+++ /dev/null
@@ -1,30 +0,0 @@
----
-title: MT3
-emoji: 🦀
-colorFrom: red
-colorTo: green
-sdk: gradio
-app_file: app.py
-pinned: false
----
-# Configuration
-`title`: _string_
-Display title for the Space
-`emoji`: _string_
-Space emoji (emoji-only character allowed)
-`colorFrom`: _string_
-Color for Thumbnail gradient (red, yellow, green, blue, indigo, purple, pink, gray)
-`colorTo`: _string_
-Color for Thumbnail gradient (red, yellow, green, blue, indigo, purple, pink, gray)
-`sdk`: _string_
-Can be either `gradio` or `streamlit`
-`sdk_version` : _string_
-Only applicable for `streamlit` SDK.
-See [doc](https://hf.co/docs/hub/spaces) for more info on supported versions.
-
-`app_file`: _string_
-Path to your main application file (which contains either `gradio` or `streamlit` Python code).
-Path is relative to the root of the repository.
-
-`pinned`: _boolean_
-Whether the Space stays on top of your list.
diff --git a/spaces/onnx/BiDAF/README.md b/spaces/onnx/BiDAF/README.md
deleted file mode 100644
index b201fff5a0aec600d18e3dc993fe2411dae06e0b..0000000000000000000000000000000000000000
--- a/spaces/onnx/BiDAF/README.md
+++ /dev/null
@@ -1,12 +0,0 @@
----
-title: BiDAF
-emoji: 📚
-colorFrom: gray
-colorTo: indigo
-sdk: gradio
-sdk_version: 2.8.11
-app_file: app.py
-pinned: false
----
-
-Check out the configuration reference at https://huggingface.co/docs/hub/spaces#reference
diff --git a/spaces/openlamm/LAMM/model/openlamm.py b/spaces/openlamm/LAMM/model/openlamm.py
deleted file mode 100644
index fba083f4bb0319cdda8b1a0e26392ac3821aa535..0000000000000000000000000000000000000000
--- a/spaces/openlamm/LAMM/model/openlamm.py
+++ /dev/null
@@ -1,511 +0,0 @@
-import io
-import os
-
-import requests
-import torch
-import torch.nn as nn
-import torch.nn.functional as F
-# from petrel_client.client import Client
-from PIL import Image, ImageFile
-from torch.nn.utils import rnn
-from types import SimpleNamespace
-from peft import LoraConfig, TaskType, get_peft_model
-from transformers import LlamaTokenizer, LlamaForCausalLM, LlamaConfig
-
-import numpy as np
-# from header import *
-
-from transformers import StoppingCriteria, StoppingCriteriaList
-
-from .CLIP import load as load_clip
-from .PROCESS import data
-from .modeling_llama import LlamaForCausalLM
-from .utils.pcl_utils import MEAN_COLOR_RGB, RandomCuboid, random_sampling
-from .conversations import conversation_dict, default_conversation
-
-ImageFile.LOAD_TRUNCATED_IMAGES = True
-
-# sov: start of vision part; eov: end of vision part
-VISION_TAGS = {
- 'pos': {'image': '', 'pcl': ''},
- 'sov': {'image': '', 'pcl': ''},
- 'eov': {'image': '', 'pcl': ''},
-}
-ModalityType = SimpleNamespace(
- VISION="vision",
- TEXT="text",
- AUDIO="audio",
- THERMAL="thermal",
- DEPTH="depth",
- IMU="imu",
-)
-
-class StoppingCriteriaSub(StoppingCriteria):
-
- def __init__(self, stops = [], encounters=1):
- super().__init__()
- self.stops = stops
- self.ENCOUNTERS = encounters
-
- def __call__(self, input_ids: torch.LongTensor, scores: torch.FloatTensor):
- stop_count = 0
- for stop in self.stops:
- stop_count = (stop == input_ids[0]).sum().item()
- if stop_count >= self.ENCOUNTERS:
- return True
- return False
-
-
-class MyStoppingCriteria(StoppingCriteria):
- def __init__(self, stops, input_ids):
- super().__init__()
- self.stops = [torch.tensor(stop).to('cuda:0') for stop in stops]
- self.stop_flag = [0]*input_ids.shape[0]
-
- def check_stop(self, input_ids):
- for stop in self.stops:
- if torch.all((stop == input_ids[-len(stop):])).item():
- return True
- return False
-
- def __call__(self, output_ids: torch.LongTensor, scores: torch.FloatTensor, **kwargs) -> bool:
- flag = 1
- for id, output_id in enumerate(output_ids):
- if self.stop_flag[id] == 1:
- continue
- if self.check_stop(output_id):
- self.stop_flag[id] = 1
- else:
- flag = 0
- if flag == 1:
- return True
- return False
-
-
-def build_one_instance(tokenizer, conversation, vision_type='image'):
- pos = VISION_TAGS['pos'][vision_type]
- # sov = VISION_TAGS['sov'][vision_type]
- eov = VISION_TAGS['eov'][vision_type]
-
- text_list = []
- turn_num = len(conversation)
- input_ids, target_ids = [], []
- for i in range(turn_num):
- turn = conversation[i]
- role = turn['from']
- if i == 0: # the first human turn
- assert role == 'human'
- turn['value'] = turn['value'].replace(f'{pos}\n', '').replace(f'\n{pos}', '')
- text = f'{eov} ' + turn['value'] + '\n### Assistant:'
- one_input_id = tokenizer(text, add_special_tokens=False).input_ids
- input_ids += one_input_id
- target_ids += [-100]*len(one_input_id) # do not perform loss regression on human prompt
- else:
- if role == 'human':
- text = 'Human: ' + turn['value'] + '\n### Assistant:'
- one_input_id = tokenizer(text, add_special_tokens=False).input_ids
- input_ids += one_input_id
- target_ids += [-100]*len(one_input_id)
- elif role == 'gpt':
- text = turn['value'] + '\n###'
- one_input_id = tokenizer(text, add_special_tokens=False).input_ids
- input_ids += one_input_id
- target_ids += one_input_id
- else:
- raise Exception('Wrong Role!!!')
- text_list.append(text)
- assert len(input_ids) == len(target_ids)
- return text_list, input_ids, target_ids
-
-
-def process_batch_instance(tokenizer, batch_of_conversations, max_tgt_len, vision_type='image'):
- batch_input_ids, batch_target_ids = [], []
- for conversation in batch_of_conversations:
- _, one_input_ids, one_target_ids = build_one_instance(tokenizer, conversation, vision_type=vision_type)
- batch_input_ids.append(torch.LongTensor(one_input_ids))
- batch_target_ids.append(torch.LongTensor(one_target_ids))
- input_ids = rnn.pad_sequence(batch_input_ids, batch_first=True, padding_value=tokenizer.pad_token_id)
- target_ids = rnn.pad_sequence(batch_target_ids, batch_first=True, padding_value=-100)
- assert input_ids.size() == target_ids.size()
- input_ids = input_ids[:,:max_tgt_len]
- target_ids = target_ids[:,:max_tgt_len]
- attention_mask = input_ids.ne(tokenizer.pad_token_id)
- assert attention_mask.size() == input_ids.size()
- return input_ids, target_ids, attention_mask.long()
-
-
-def make_prompt_start(system_header=False, vision_type='image', task_type='normal'):
- # TODO: choose prefix according to task type
- PROMPT_START = f'### Human: {VISION_TAGS["sov"][vision_type]}'
- if system_header:
- if task_type == 'normal':
- return f"{default_conversation.system}\n\n" + PROMPT_START
- else:
- return [f"{conversation_dict[task]}\n\n" + PROMPT_START for task in task_type]
- else:
- return PROMPT_START
-
-
-class LAMMPEFTModel(nn.Module):
-
- '''LoRA for LLaMa model'''
-
- def __init__(self, **args):
- super(LAMMPEFTModel, self).__init__()
- self.args = args
- # self.client = Client('~/petreloss.conf')
- self.client = None
-
- self.vision_type = args['vision_type'] if 'vision_type' in args else 'image'
- encoder_pretrain = args['encoder_pretrain'] if 'encoder_pretrain' in args else 'clip'
- self.encoder_pretrain = encoder_pretrain
- assert encoder_pretrain in ['imagebind', 'clip', 'epcl'], f'Encoder_pretrain: {encoder_pretrain} Not Implemented'
- if not encoder_pretrain == 'clip' or os.path.isfile(args['encoder_ckpt_path']):
- encoder_ckpt_path = args['encoder_ckpt_path']
- elif not os.path.isfile(args['encoder_ckpt_path']):
- encoder_ckpt_path = 'ViT-L/14'
-
- vicuna_ckpt_path = args['vicuna_ckpt_path']
-
- system_header = args['system_header'] if 'system_header' in args else False
- stage = args['stage']
-
- # TODO: checkout vision token number; for ImageBind = 1; Defaultly to use 1 global token for this
- # -1 for last embedding; -2 for transformer output
- self.vision_feature_type = args['vision_feature_type']
- self.num_vision_token = args['num_vision_token']
-
- device = torch.device('cuda' if torch.cuda.is_available() else 'cpu')
- print (f'Initializing [{encoder_pretrain}] visual encoder from {encoder_ckpt_path} [{device}]...')
-
- # TODO: Make sure the number of vision tokens is correct
- if args['encoder_pretrain'].lower() == 'clip':
- clip_encoder, self.visual_preprocess = load_clip(encoder_ckpt_path, device=device)
- self.visual_encoder = clip_encoder.visual
- if self.vision_feature_type == 'global': # global feature from CLIP
- self.vision_hidden_size = 768
- self.num_vision_token = 1
- assert self.num_vision_token == 1, 'Only 1 global token is available!'
- elif self.vision_feature_type == 'local': # patch features from CLIP ViT
- self.vision_hidden_size = 1024
- self.num_vision_token = min(self.num_vision_token, 256) # may cut partial tokens
-
- # freeze vision encoder
- for name, param in self.visual_encoder.named_parameters():
- param.requires_grad = False
- self.visual_encoder.eval()
- print ('Visual encoder initialized.')
-
- print (f'Initializing language decoder from {vicuna_ckpt_path} ...')
- # add the lora module
- peft_config = LoraConfig(
- task_type=TaskType.CAUSAL_LM,
- inference_mode=False,
- r=self.args['lora_r'],
- lora_alpha=self.args['lora_alpha'],
- lora_dropout=self.args['lora_dropout'],
- target_modules=self.args['lora_target_modules']
- )
-
- self.llama_model = LlamaForCausalLM.from_pretrained(vicuna_ckpt_path)
- self.llama_model = get_peft_model(self.llama_model, peft_config)
- self.llama_model.print_trainable_parameters()
-
- self.llama_tokenizer = LlamaTokenizer.from_pretrained(vicuna_ckpt_path, use_fast=False)
- self.llama_tokenizer.pad_token = self.llama_tokenizer.eos_token
- self.llama_tokenizer.padding_side = "right"
- print ('Language decoder initialized.')
-
- self.llama_proj = nn.Linear(
- self.vision_hidden_size, self.llama_model.config.hidden_size
- )
- print ('LLaMa projection layer initialized.')
-
- self.max_tgt_len = args['max_tgt_len']
- self.system_header = system_header
- self.device = torch.cuda.current_device()
-
- def encode_image(self, image_paths):
- """encode images to llama inputs
-
- :param tupe image_paths: (bsz, )
- :return tensor, tensor: input feature to llama, attention mask to llama
- """
- if self.encoder_pretrain == 'imagebind':
- inputs = {ModalityType.VISION: data.load_and_transform_vision_data(image_paths, self.device)}
- # convert into visual dtype
- inputs = {key: inputs[key].to(self.llama_model.dtype) for key in inputs}
- with torch.no_grad():
- embeddings = self.visual_encoder(inputs)
- image_embeds = embeddings['vision'] # bsz x 1024
- inputs_llama = self.llama_proj(image_embeds).unsqueeze(1) # bsz x 1 x llama_size
- atts_llama = torch.ones(inputs_llama.size()[:-1], dtype=torch.long).to(self.device) # bsz x 1
- return inputs_llama, atts_llama
- elif self.encoder_pretrain == 'clip':
- inputs = self.load_and_transform_vision_data_clip(image_paths, self.device) # bsz x 3 x 224 x 224
- inputs = inputs.to(self.llama_model.dtype) # clip requires torch.float32
- inputs_llama = self.clip_encode_image(inputs)
- atts_llama = torch.ones(inputs_llama.size()[:-1], dtype=torch.long).to(self.device) # bsz x 1/256
- return inputs_llama, atts_llama
-
- def my_encode_image(self, images):
- """encoder loaded image objects"""
- if self.encoder_pretrain == 'clip':
- inputs = data.transform_vision_data(images, self.device) # bsz x 3 x 224 x 224
- inputs_llama = self.clip_encode_image(inputs) # bsz x 1/256 x llama_size
- atts_llama = torch.ones(inputs_llama.size()[:-1], dtype=torch.long).to(self.device) # bsz x 1/256
- return inputs_llama, atts_llama
- else:
- raise NotImplementedError("Encoder pretrain [{}] not implemented".format(self.encoder_pretrain))
-
- def encode_pcl(self, pcl_paths):
- # load pcl data
- inputs = self.load_and_transform_pcl_data(pcl_paths, self.device) # bsz x 40000 x 3
-
- inputs = inputs.to(self.llama_model.dtype) # clip requires torch.float32
- with torch.no_grad():
- if self.vision_feature_type == 'global':
- raise NotImplementedError("Global feature not implemented for pcl")
- elif self.vision_feature_type == 'local':
- embeddings = self.visual_encoder(inputs)[1][:, :self.num_vision_token] # bsz x 256 x 1024;
- image_embeds = embeddings.reshape(-1, self.vision_hidden_size).to(self.llama_model.dtype) # bsz*num vision token x 1024
- inputs_llama = self.llama_proj(image_embeds).reshape(-1, self.num_vision_token, self.llama_model.config.hidden_size) # bsz x num_vision_token x llama_size
- atts_llama = torch.ones(inputs_llama.size()[:-1], dtype=torch.long).to(self.device) # bsz x 1/256
- return inputs_llama, atts_llama
-
- def clip_encode_image(self, inputs):
- inputs = inputs.to(self.llama_model.dtype) # clip requires torch.float32
- with torch.no_grad():
- if self.vision_feature_type == 'global':
- embeddings = self.visual_encoder(inputs) # bsz x 768
- image_embeds = embeddings.to(self.llama_model.dtype)
- inputs_llama = self.llama_proj(image_embeds).unsqueeze(1) # bsz x 1 x llama_size
- elif self.vision_feature_type == 'local':
- embeddings = self.visual_encoder.forward_patch_features(inputs)[:, :self.num_vision_token] # bsz x self.num_vision_token x 1024
- image_embeds = embeddings.reshape(-1, self.vision_hidden_size).to(self.llama_model.dtype) # bsz*num vision token x 1024
- inputs_llama = self.llama_proj(image_embeds).reshape(-1, self.num_vision_token, self.llama_model.config.hidden_size) # bsz x num_vision_token x llama_size
- else:
- raise NotImplementedError("{} not Implemented".format(self.vision_feature_type))
- return inputs_llama
-
- def load_and_transform_vision_data_clip(self, image_paths, device):
- if image_paths is None:
- return None
- image_ouputs = []
- for image_path in image_paths:
- if os.path.exists(image_path):
- image = Image.open(image_path)
- elif image_path.startswith('s3://') and self.client is not None:
- image = Image.open(io.BytesIO(self.client.get(image_path, update_cache=True))).convert("RGB")
- elif image_path.startswith('http://'):
- image = Image.open(requests.get(image_path, stream=True).raw)
- else:
- print("can not load image: ", image_path)
- image_outpt = self.visual_preprocess(image).to(device) # 3 x 224 x 224
- image_ouputs.append(image_outpt)
- return torch.stack(image_ouputs, dim=0) # B x 3 x 224 x 224
-
- def load_and_transform_pcl_data(self, pcl_paths, device):
- if pcl_paths is None:
- return None
- pcl_output = []
- for pcl_path in pcl_paths:
- mesh_vertices = np.load(pcl_path) # 150000, 3
- if not self.use_color:
- point_cloud = mesh_vertices[:, 0:3] # do not use color for now
- else:
- point_cloud = mesh_vertices[:, 0:6]
- point_cloud[:, 3:] = (point_cloud[:, 3:] - MEAN_COLOR_RGB) / 256.0
-
- if self.use_height:
- floor_height = np.percentile(point_cloud[:, 2], 0.99)
- height = point_cloud[:, 2] - floor_height
- point_cloud = np.concatenate([point_cloud, np.expand_dims(height, 1)], 1)
-
- point_cloud, _ = random_sampling(
- point_cloud, self.num_points, return_choices=True
- )
- pcl_output.append(torch.from_numpy(point_cloud))
- return torch.stack(pcl_output, dim=0).to(device) # bsz x num_points x 3
-
- def prompt_wrap(self, img_embeds, input_ids, target_ids, attention_mask, system_header, task_type):
- '''
- input_ids, target_ids, attention_mask: bsz x s2
- '''
- input_ids = input_ids.to(self.device) # bsz x s2
- target_ids = target_ids.to(self.device) # bsz x s2
- attention_mask = attention_mask.to(self.device) # bsz x s2
-
- batch_size = img_embeds.shape[0]
-
- # return list of headers if multiple tasks
- p_before = make_prompt_start(system_header=system_header, vision_type=self.vision_type, task_type=task_type)
- if isinstance(p_before, list):
- p_before_tokens = [self.llama_tokenizer(p,
- return_tensors="pt", add_special_tokens=False).input_ids[0].to(self.device) for p in p_before]
- # TODO: fix bug here
- p_before_token_ids = rnn.pad_sequence(p_before_tokens, batch_first=True, padding_value=self.llama_tokenizer.pad_token_id) # bsz x s1
- p_before_attn_mask = p_before_token_ids.ne(self.llama_tokenizer.pad_token_id)
- else:
- p_before_tokens = self.llama_tokenizer(p_before,
- return_tensors="pt", add_special_tokens=False).to(self.device) # [s1, s1...] list of batch size
- p_before_token_ids = p_before_tokens.input_ids.expand(batch_size, -1) # bsz x s1
- p_before_attn_mask = p_before_tokens.attention_mask.expand(batch_size, -1) # bsz x s1
- # peft model need deeper call
- p_before_embeds = self.llama_model.model.model.embed_tokens(p_before_token_ids) #.expand(batch_size, -1, -1) # bsz x s1 x embed_dim
- p_after_embeds = self.llama_model.model.model.embed_tokens(input_ids).expand(batch_size, -1, -1) # bsz x s2 x embed_dim
- bos = torch.ones([batch_size, 1],
- dtype=p_before_token_ids.dtype,
- device=p_before_token_ids.device) * self.llama_tokenizer.bos_token_id # bsz x 1
- bos_embeds = self.llama_model.model.model.embed_tokens(bos) # bsz x 1 x embed_dim
- inputs_embeds = torch.cat([bos_embeds, p_before_embeds, img_embeds, p_after_embeds], dim=1) # bsz x (1+s1+NumToken+s2) x embed_dim
-
- # make target ids for prefix part
- empty_targets = (
- torch.ones([batch_size, 1 + p_before_embeds.size()[1] + self.num_vision_token], # 1 (bos) + s1 + num_image_tokens (image vector)
- dtype=torch.long).to(self.device).fill_(-100)
- ) # bsz x (1 + s1 + 1)
- targets = torch.cat([empty_targets, target_ids], dim=1) # bsz x (1 + s1 + num_image_tokens + s2)
- assert inputs_embeds.size()[1] == targets.size()[1]
-
- # atts_prefix = torch.ones([batch_size, 1 + p_before_embeds.size()[1] + self.num_vision_token], dtype=torch.long).to(self.device) # bsz x (1[bos] + s1 +num_image_tokens)
- atts_bos = torch.ones([batch_size, 1], dtype=torch.long).to(self.device) # bsz x 1
- atts_img = torch.ones([batch_size, self.num_vision_token], dtype=torch.long).to(self.device) # bsz x num_image_tokens
- attention_mask = torch.cat([atts_bos, p_before_attn_mask, atts_img, attention_mask], dim=1)
- assert attention_mask.size() == targets.size() # bsz x (1 + s1 + num_image_tokens + s2)
- return inputs_embeds, targets, attention_mask
-
- def forward(self, inputs):
- """Model Forward in training
-
- :param class inputs: model itself
- :raises ValueError: valueerror if not image or pcl
- :return list: loss & token acc
- """
- # image_paths = inputs['image_paths']
- assert self.vision_type == inputs['vision_type'] # single modal case
- task_type = inputs['task_type']
- vision_paths = inputs['vision_paths']
- if self.vision_type == 'image':
- vision_embeds, _ = self.encode_image(vision_paths)
- elif self.vision_type == 'pcl':
- vision_embeds, _ = self.encode_pcl(vision_paths) # Bsz x N token x C
- else:
- raise ValueError('vision type [{}] not supported'.format(self.vision_type))
-
- output_texts = inputs['output_texts']
- input_ids, target_ids, attention_mask = process_batch_instance(self.llama_tokenizer, output_texts, self.max_tgt_len, self.vision_type)
- inputs_embeds, targets, attention_mask = self.prompt_wrap(vision_embeds, input_ids, target_ids, attention_mask, self.system_header, task_type)
-
- outputs = self.llama_model(
- inputs_embeds=inputs_embeds,
- attention_mask=attention_mask,
- return_dict=True,
- labels=targets,
- )
- loss = outputs.loss
- # calculate the token accuarcy
- chosen_tokens = torch.max(outputs.logits, dim=-1)[1][:, 1: -1] # [B, S-1]
- labels = targets[:, 2:]
- gen_acc = (chosen_tokens.reshape(-1) == labels.reshape(-1)).to(torch.long) # [B*S]
- valid_mask = (labels != -100).reshape(-1)
- valid_tokens = gen_acc & valid_mask # [B*S]
- gen_acc = valid_tokens.sum().item() / valid_mask.sum().item()
- return loss, gen_acc
-
- def extract_multimodal_feature(self, inputs):
- """Extract multimodal features from the input in Generation (Test)
-
- :param Dict inputs: input dict; modality: path
- :return _type_: _description_
- """
- features = []
- if inputs['image_paths']:
- image_embeds, _ = self.encode_image(inputs['image_paths'])
- features.append(image_embeds)
- if 'images' in inputs and inputs['images']: # image objects input in testing
- image_embeds, _ = self.my_encode_image(inputs['images'])
- return image_embeds
- # features.append(image_embeds)
- if 'pcl_paths' in inputs and inputs['pcl_paths']:
- pcl_embeds, _ = self.encode_pcl(inputs['pcl_paths'])
- features.append(pcl_embeds)
- # TODO: Cautions HERE! Multimodality allowed in test ONLY!
- feature_embeds = torch.cat(features).sum(dim=0).unsqueeze(0) # sum all modality features together
- return feature_embeds
-
- def prepare_generation_embedding(self, inputs):
- """prepare for generation
-
- :param class inputs: model
- :return Dict: generation input
- """
- eov = VISION_TAGS['eov'][self.vision_type]
- # TODO: add System header & image token size
- prompt_list = inputs['prompt'] # questions from user
- if len(inputs['modality_embeds']) == 1:
- feature_embeds = inputs['modality_embeds'][0]
- else:
- feature_embeds = self.extract_multimodal_feature(inputs)
- inputs['modality_embeds'].append(feature_embeds)
-
- batch_size = feature_embeds.shape[0]
- p_before = make_prompt_start(vision_type=self.vision_type) # no system header in test
- p_before_tokens = self.llama_tokenizer(p_before,
- return_tensors="pt", add_special_tokens=False).to(self.device)
- p_before_embeds = self.llama_model.model.model.embed_tokens(p_before_tokens.input_ids).expand(batch_size, -1, -1) # bsz x s1 x embed_dim
- p_after_embeds_list = []
- p_after_tokens_list = []
- for prompt in prompt_list:
- # text = ' ' + prompt + '\n### Assistant:'
- text = f'{eov} ' + prompt + '\n### Assistant:'
- p_after_tokens = self.llama_tokenizer(text, add_special_tokens=False, return_tensors='pt').to(self.device)
-
- p_after_tokens_list.append(p_after_tokens.input_ids.squeeze(0))
-
- p_after_tokens = rnn.pad_sequence(p_after_tokens_list, batch_first=True, padding_value=self.llama_tokenizer.pad_token_id)
-
- p_after_embeds = self.llama_model.model.model.embed_tokens(p_after_tokens)
-
- # text = f'{eov} ' + prompt + '\n### Assistant:'
- # p_after_tokens = self.llama_tokenizer(text, add_special_tokens=False, return_tensors='pt').to(self.device)
- # p_after_embeds = self.llama_model.model.model.embed_tokens(p_after_tokens.input_ids).expand(batch_size, -1, -1) # bsz x s1 x embed_dim
- bos = torch.ones([batch_size, 1],
- dtype=p_before_tokens.input_ids.dtype,
- device=p_before_tokens.input_ids.device) * self.llama_tokenizer.bos_token_id # bsz x 1
- bos_embeds = self.llama_model.model.model.embed_tokens(bos) # bsz x 1 x embed_dim
- # print(bos_embeds.shape, p_before_embeds.shape, feature_embeds.shape, p_after_embeds.shape)
- inputs_embeds = torch.cat([bos_embeds, p_before_embeds, feature_embeds, p_after_embeds], dim=1) # bsz x (1+s1+NumVisionToken+s2) x embed_dim
- return inputs_embeds
-
- def generate(self, inputs):
- '''
- inputs = {
- 'image_paths': optional,
- 'audio_paths': optional
- 'video_paths': optional
- 'thermal_paths': optional
- 'mode': generation mode,
- 'prompt': human input prompt,
- 'max_tgt_len': generation length,
- 'top_p': top_p,
- 'temperature': temperature
- 'modality_embeds': None or torch.tensor
- 'modality_cache': save the image cache
- }
- '''
- input_embeds = self.prepare_generation_embedding(inputs)
- # stopping_criteria = StoppingCriteriaList([StoppingCriteriaSub(stops=[2277], encounters=1)])
- stopping_criteria = StoppingCriteriaList([MyStoppingCriteria([[2277]], input_embeds)])
- outputs = self.llama_model.generate(
- inputs_embeds=input_embeds,
- max_new_tokens=inputs['max_tgt_len'],
- top_p=inputs['top_p'],
- temperature=inputs['temperature'],
- do_sample=True,
- use_cache=True,
- stopping_criteria=stopping_criteria,
- )
- #output_text = self.llama_tokenizer.decode(outputs[0][:-2], skip_special_tokens=True)
- output_text = self.llama_tokenizer.batch_decode(outputs, skip_special_tokens=True)
- return output_text
diff --git a/spaces/pablodawson/ldm3d-inpainting/diffuserslocal/examples/custom_diffusion/train_custom_diffusion.py b/spaces/pablodawson/ldm3d-inpainting/diffuserslocal/examples/custom_diffusion/train_custom_diffusion.py
deleted file mode 100644
index 60d8d6723dcf579e12263f75b9a60374f778cee1..0000000000000000000000000000000000000000
--- a/spaces/pablodawson/ldm3d-inpainting/diffuserslocal/examples/custom_diffusion/train_custom_diffusion.py
+++ /dev/null
@@ -1,1338 +0,0 @@
-#!/usr/bin/env python
-# coding=utf-8
-# Copyright 2023 Custom Diffusion authors and the HuggingFace Inc. team. All rights reserved.
-#
-# Licensed under the Apache License, Version 2.0 (the "License");
-# you may not use this file except in compliance with the License.
-# You may obtain a copy of the License at
-#
-# http://www.apache.org/licenses/LICENSE-2.0
-#
-# Unless required by applicable law or agreed to in writing, software
-# distributed under the License is distributed on an "AS IS" BASIS,
-# WITHOUT WARRANTIES OR CONDITIONS OF ANY KIND, either express or implied.
-# See the License for the specific language governing permissions and
-
-import argparse
-import hashlib
-import itertools
-import json
-import logging
-import math
-import os
-import random
-import shutil
-import warnings
-from pathlib import Path
-
-import numpy as np
-import safetensors
-import torch
-import torch.nn.functional as F
-import torch.utils.checkpoint
-import transformers
-from accelerate import Accelerator
-from accelerate.logging import get_logger
-from accelerate.utils import ProjectConfiguration, set_seed
-from huggingface_hub import HfApi, create_repo
-from packaging import version
-from PIL import Image
-from torch.utils.data import Dataset
-from torchvision import transforms
-from tqdm.auto import tqdm
-from transformers import AutoTokenizer, PretrainedConfig
-
-import diffusers
-from diffusers import (
- AutoencoderKL,
- DDPMScheduler,
- DiffusionPipeline,
- DPMSolverMultistepScheduler,
- UNet2DConditionModel,
-)
-from diffusers.loaders import AttnProcsLayers
-from diffusers.models.attention_processor import (
- CustomDiffusionAttnProcessor,
- CustomDiffusionAttnProcessor2_0,
- CustomDiffusionXFormersAttnProcessor,
-)
-from diffusers.optimization import get_scheduler
-from diffusers.utils import check_min_version, is_wandb_available
-from diffusers.utils.import_utils import is_xformers_available
-
-
-# Will error if the minimal version of diffusers is not installed. Remove at your own risks.
-check_min_version("0.22.0.dev0")
-
-logger = get_logger(__name__)
-
-
-def freeze_params(params):
- for param in params:
- param.requires_grad = False
-
-
-def save_model_card(repo_id: str, images=None, base_model=str, prompt=str, repo_folder=None):
- img_str = ""
- for i, image in enumerate(images):
- image.save(os.path.join(repo_folder, f"image_{i}.png"))
- img_str += f"\n"
-
- yaml = f"""
----
-license: creativeml-openrail-m
-base_model: {base_model}
-instance_prompt: {prompt}
-tags:
-- stable-diffusion
-- stable-diffusion-diffusers
-- text-to-image
-- diffusers
-- custom-diffusion
-inference: true
----
- """
- model_card = f"""
-# Custom Diffusion - {repo_id}
-
-These are Custom Diffusion adaption weights for {base_model}. The weights were trained on {prompt} using [Custom Diffusion](https://www.cs.cmu.edu/~custom-diffusion). You can find some example images in the following. \n
-{img_str}
-
-\nFor more details on the training, please follow [this link](https://github.com/huggingface/diffusers/blob/main/examples/custom_diffusion).
-"""
- with open(os.path.join(repo_folder, "README.md"), "w") as f:
- f.write(yaml + model_card)
-
-
-def import_model_class_from_model_name_or_path(pretrained_model_name_or_path: str, revision: str):
- text_encoder_config = PretrainedConfig.from_pretrained(
- pretrained_model_name_or_path,
- subfolder="text_encoder",
- revision=revision,
- )
- model_class = text_encoder_config.architectures[0]
-
- if model_class == "CLIPTextModel":
- from transformers import CLIPTextModel
-
- return CLIPTextModel
- elif model_class == "RobertaSeriesModelWithTransformation":
- from diffusers.pipelines.alt_diffusion.modeling_roberta_series import RobertaSeriesModelWithTransformation
-
- return RobertaSeriesModelWithTransformation
- else:
- raise ValueError(f"{model_class} is not supported.")
-
-
-def collate_fn(examples, with_prior_preservation):
- input_ids = [example["instance_prompt_ids"] for example in examples]
- pixel_values = [example["instance_images"] for example in examples]
- mask = [example["mask"] for example in examples]
- # Concat class and instance examples for prior preservation.
- # We do this to avoid doing two forward passes.
- if with_prior_preservation:
- input_ids += [example["class_prompt_ids"] for example in examples]
- pixel_values += [example["class_images"] for example in examples]
- mask += [example["class_mask"] for example in examples]
-
- input_ids = torch.cat(input_ids, dim=0)
- pixel_values = torch.stack(pixel_values)
- mask = torch.stack(mask)
- pixel_values = pixel_values.to(memory_format=torch.contiguous_format).float()
- mask = mask.to(memory_format=torch.contiguous_format).float()
-
- batch = {"input_ids": input_ids, "pixel_values": pixel_values, "mask": mask.unsqueeze(1)}
- return batch
-
-
-class PromptDataset(Dataset):
- "A simple dataset to prepare the prompts to generate class images on multiple GPUs."
-
- def __init__(self, prompt, num_samples):
- self.prompt = prompt
- self.num_samples = num_samples
-
- def __len__(self):
- return self.num_samples
-
- def __getitem__(self, index):
- example = {}
- example["prompt"] = self.prompt
- example["index"] = index
- return example
-
-
-class CustomDiffusionDataset(Dataset):
- """
- A dataset to prepare the instance and class images with the prompts for fine-tuning the model.
- It pre-processes the images and the tokenizes prompts.
- """
-
- def __init__(
- self,
- concepts_list,
- tokenizer,
- size=512,
- mask_size=64,
- center_crop=False,
- with_prior_preservation=False,
- num_class_images=200,
- hflip=False,
- aug=True,
- ):
- self.size = size
- self.mask_size = mask_size
- self.center_crop = center_crop
- self.tokenizer = tokenizer
- self.interpolation = Image.BILINEAR
- self.aug = aug
-
- self.instance_images_path = []
- self.class_images_path = []
- self.with_prior_preservation = with_prior_preservation
- for concept in concepts_list:
- inst_img_path = [
- (x, concept["instance_prompt"]) for x in Path(concept["instance_data_dir"]).iterdir() if x.is_file()
- ]
- self.instance_images_path.extend(inst_img_path)
-
- if with_prior_preservation:
- class_data_root = Path(concept["class_data_dir"])
- if os.path.isdir(class_data_root):
- class_images_path = list(class_data_root.iterdir())
- class_prompt = [concept["class_prompt"] for _ in range(len(class_images_path))]
- else:
- with open(class_data_root, "r") as f:
- class_images_path = f.read().splitlines()
- with open(concept["class_prompt"], "r") as f:
- class_prompt = f.read().splitlines()
-
- class_img_path = [(x, y) for (x, y) in zip(class_images_path, class_prompt)]
- self.class_images_path.extend(class_img_path[:num_class_images])
-
- random.shuffle(self.instance_images_path)
- self.num_instance_images = len(self.instance_images_path)
- self.num_class_images = len(self.class_images_path)
- self._length = max(self.num_class_images, self.num_instance_images)
- self.flip = transforms.RandomHorizontalFlip(0.5 * hflip)
-
- self.image_transforms = transforms.Compose(
- [
- self.flip,
- transforms.Resize(size, interpolation=transforms.InterpolationMode.BILINEAR),
- transforms.CenterCrop(size) if center_crop else transforms.RandomCrop(size),
- transforms.ToTensor(),
- transforms.Normalize([0.5], [0.5]),
- ]
- )
-
- def __len__(self):
- return self._length
-
- def preprocess(self, image, scale, resample):
- outer, inner = self.size, scale
- factor = self.size // self.mask_size
- if scale > self.size:
- outer, inner = scale, self.size
- top, left = np.random.randint(0, outer - inner + 1), np.random.randint(0, outer - inner + 1)
- image = image.resize((scale, scale), resample=resample)
- image = np.array(image).astype(np.uint8)
- image = (image / 127.5 - 1.0).astype(np.float32)
- instance_image = np.zeros((self.size, self.size, 3), dtype=np.float32)
- mask = np.zeros((self.size // factor, self.size // factor))
- if scale > self.size:
- instance_image = image[top : top + inner, left : left + inner, :]
- mask = np.ones((self.size // factor, self.size // factor))
- else:
- instance_image[top : top + inner, left : left + inner, :] = image
- mask[
- top // factor + 1 : (top + scale) // factor - 1, left // factor + 1 : (left + scale) // factor - 1
- ] = 1.0
- return instance_image, mask
-
- def __getitem__(self, index):
- example = {}
- instance_image, instance_prompt = self.instance_images_path[index % self.num_instance_images]
- instance_image = Image.open(instance_image)
- if not instance_image.mode == "RGB":
- instance_image = instance_image.convert("RGB")
- instance_image = self.flip(instance_image)
-
- # apply resize augmentation and create a valid image region mask
- random_scale = self.size
- if self.aug:
- random_scale = (
- np.random.randint(self.size // 3, self.size + 1)
- if np.random.uniform() < 0.66
- else np.random.randint(int(1.2 * self.size), int(1.4 * self.size))
- )
- instance_image, mask = self.preprocess(instance_image, random_scale, self.interpolation)
-
- if random_scale < 0.6 * self.size:
- instance_prompt = np.random.choice(["a far away ", "very small "]) + instance_prompt
- elif random_scale > self.size:
- instance_prompt = np.random.choice(["zoomed in ", "close up "]) + instance_prompt
-
- example["instance_images"] = torch.from_numpy(instance_image).permute(2, 0, 1)
- example["mask"] = torch.from_numpy(mask)
- example["instance_prompt_ids"] = self.tokenizer(
- instance_prompt,
- truncation=True,
- padding="max_length",
- max_length=self.tokenizer.model_max_length,
- return_tensors="pt",
- ).input_ids
-
- if self.with_prior_preservation:
- class_image, class_prompt = self.class_images_path[index % self.num_class_images]
- class_image = Image.open(class_image)
- if not class_image.mode == "RGB":
- class_image = class_image.convert("RGB")
- example["class_images"] = self.image_transforms(class_image)
- example["class_mask"] = torch.ones_like(example["mask"])
- example["class_prompt_ids"] = self.tokenizer(
- class_prompt,
- truncation=True,
- padding="max_length",
- max_length=self.tokenizer.model_max_length,
- return_tensors="pt",
- ).input_ids
-
- return example
-
-
-def save_new_embed(text_encoder, modifier_token_id, accelerator, args, output_dir, safe_serialization=True):
- """Saves the new token embeddings from the text encoder."""
- logger.info("Saving embeddings")
- learned_embeds = accelerator.unwrap_model(text_encoder).get_input_embeddings().weight
- for x, y in zip(modifier_token_id, args.modifier_token):
- learned_embeds_dict = {}
- learned_embeds_dict[y] = learned_embeds[x]
- filename = f"{output_dir}/{y}.bin"
-
- if safe_serialization:
- safetensors.torch.save_file(learned_embeds_dict, filename, metadata={"format": "pt"})
- else:
- torch.save(learned_embeds_dict, filename)
-
-
-def parse_args(input_args=None):
- parser = argparse.ArgumentParser(description="Custom Diffusion training script.")
- parser.add_argument(
- "--pretrained_model_name_or_path",
- type=str,
- default=None,
- required=True,
- help="Path to pretrained model or model identifier from huggingface.co/models.",
- )
- parser.add_argument(
- "--revision",
- type=str,
- default=None,
- required=False,
- help="Revision of pretrained model identifier from huggingface.co/models.",
- )
- parser.add_argument(
- "--tokenizer_name",
- type=str,
- default=None,
- help="Pretrained tokenizer name or path if not the same as model_name",
- )
- parser.add_argument(
- "--instance_data_dir",
- type=str,
- default=None,
- help="A folder containing the training data of instance images.",
- )
- parser.add_argument(
- "--class_data_dir",
- type=str,
- default=None,
- help="A folder containing the training data of class images.",
- )
- parser.add_argument(
- "--instance_prompt",
- type=str,
- default=None,
- help="The prompt with identifier specifying the instance",
- )
- parser.add_argument(
- "--class_prompt",
- type=str,
- default=None,
- help="The prompt to specify images in the same class as provided instance images.",
- )
- parser.add_argument(
- "--validation_prompt",
- type=str,
- default=None,
- help="A prompt that is used during validation to verify that the model is learning.",
- )
- parser.add_argument(
- "--num_validation_images",
- type=int,
- default=2,
- help="Number of images that should be generated during validation with `validation_prompt`.",
- )
- parser.add_argument(
- "--validation_steps",
- type=int,
- default=50,
- help=(
- "Run dreambooth validation every X epochs. Dreambooth validation consists of running the prompt"
- " `args.validation_prompt` multiple times: `args.num_validation_images`."
- ),
- )
- parser.add_argument(
- "--with_prior_preservation",
- default=False,
- action="store_true",
- help="Flag to add prior preservation loss.",
- )
- parser.add_argument(
- "--real_prior",
- default=False,
- action="store_true",
- help="real images as prior.",
- )
- parser.add_argument("--prior_loss_weight", type=float, default=1.0, help="The weight of prior preservation loss.")
- parser.add_argument(
- "--num_class_images",
- type=int,
- default=200,
- help=(
- "Minimal class images for prior preservation loss. If there are not enough images already present in"
- " class_data_dir, additional images will be sampled with class_prompt."
- ),
- )
- parser.add_argument(
- "--output_dir",
- type=str,
- default="custom-diffusion-model",
- help="The output directory where the model predictions and checkpoints will be written.",
- )
- parser.add_argument("--seed", type=int, default=42, help="A seed for reproducible training.")
- parser.add_argument(
- "--resolution",
- type=int,
- default=512,
- help=(
- "The resolution for input images, all the images in the train/validation dataset will be resized to this"
- " resolution"
- ),
- )
- parser.add_argument(
- "--center_crop",
- default=False,
- action="store_true",
- help=(
- "Whether to center crop the input images to the resolution. If not set, the images will be randomly"
- " cropped. The images will be resized to the resolution first before cropping."
- ),
- )
- parser.add_argument(
- "--train_batch_size", type=int, default=4, help="Batch size (per device) for the training dataloader."
- )
- parser.add_argument(
- "--sample_batch_size", type=int, default=4, help="Batch size (per device) for sampling images."
- )
- parser.add_argument("--num_train_epochs", type=int, default=1)
- parser.add_argument(
- "--max_train_steps",
- type=int,
- default=None,
- help="Total number of training steps to perform. If provided, overrides num_train_epochs.",
- )
- parser.add_argument(
- "--checkpointing_steps",
- type=int,
- default=250,
- help=(
- "Save a checkpoint of the training state every X updates. These checkpoints can be used both as final"
- " checkpoints in case they are better than the last checkpoint, and are also suitable for resuming"
- " training using `--resume_from_checkpoint`."
- ),
- )
- parser.add_argument(
- "--checkpoints_total_limit",
- type=int,
- default=None,
- help=("Max number of checkpoints to store."),
- )
- parser.add_argument(
- "--resume_from_checkpoint",
- type=str,
- default=None,
- help=(
- "Whether training should be resumed from a previous checkpoint. Use a path saved by"
- ' `--checkpointing_steps`, or `"latest"` to automatically select the last available checkpoint.'
- ),
- )
- parser.add_argument(
- "--gradient_accumulation_steps",
- type=int,
- default=1,
- help="Number of updates steps to accumulate before performing a backward/update pass.",
- )
- parser.add_argument(
- "--gradient_checkpointing",
- action="store_true",
- help="Whether or not to use gradient checkpointing to save memory at the expense of slower backward pass.",
- )
- parser.add_argument(
- "--learning_rate",
- type=float,
- default=1e-5,
- help="Initial learning rate (after the potential warmup period) to use.",
- )
- parser.add_argument(
- "--scale_lr",
- action="store_true",
- default=False,
- help="Scale the learning rate by the number of GPUs, gradient accumulation steps, and batch size.",
- )
- parser.add_argument(
- "--dataloader_num_workers",
- type=int,
- default=2,
- help=(
- "Number of subprocesses to use for data loading. 0 means that the data will be loaded in the main process."
- ),
- )
- parser.add_argument(
- "--freeze_model",
- type=str,
- default="crossattn_kv",
- choices=["crossattn_kv", "crossattn"],
- help="crossattn to enable fine-tuning of all params in the cross attention",
- )
- parser.add_argument(
- "--lr_scheduler",
- type=str,
- default="constant",
- help=(
- 'The scheduler type to use. Choose between ["linear", "cosine", "cosine_with_restarts", "polynomial",'
- ' "constant", "constant_with_warmup"]'
- ),
- )
- parser.add_argument(
- "--lr_warmup_steps", type=int, default=500, help="Number of steps for the warmup in the lr scheduler."
- )
- parser.add_argument(
- "--use_8bit_adam", action="store_true", help="Whether or not to use 8-bit Adam from bitsandbytes."
- )
- parser.add_argument("--adam_beta1", type=float, default=0.9, help="The beta1 parameter for the Adam optimizer.")
- parser.add_argument("--adam_beta2", type=float, default=0.999, help="The beta2 parameter for the Adam optimizer.")
- parser.add_argument("--adam_weight_decay", type=float, default=1e-2, help="Weight decay to use.")
- parser.add_argument("--adam_epsilon", type=float, default=1e-08, help="Epsilon value for the Adam optimizer")
- parser.add_argument("--max_grad_norm", default=1.0, type=float, help="Max gradient norm.")
- parser.add_argument("--push_to_hub", action="store_true", help="Whether or not to push the model to the Hub.")
- parser.add_argument("--hub_token", type=str, default=None, help="The token to use to push to the Model Hub.")
- parser.add_argument(
- "--hub_model_id",
- type=str,
- default=None,
- help="The name of the repository to keep in sync with the local `output_dir`.",
- )
- parser.add_argument(
- "--logging_dir",
- type=str,
- default="logs",
- help=(
- "[TensorBoard](https://www.tensorflow.org/tensorboard) log directory. Will default to"
- " *output_dir/runs/**CURRENT_DATETIME_HOSTNAME***."
- ),
- )
- parser.add_argument(
- "--allow_tf32",
- action="store_true",
- help=(
- "Whether or not to allow TF32 on Ampere GPUs. Can be used to speed up training. For more information, see"
- " https://pytorch.org/docs/stable/notes/cuda.html#tensorfloat-32-tf32-on-ampere-devices"
- ),
- )
- parser.add_argument(
- "--report_to",
- type=str,
- default="tensorboard",
- help=(
- 'The integration to report the results and logs to. Supported platforms are `"tensorboard"`'
- ' (default), `"wandb"` and `"comet_ml"`. Use `"all"` to report to all integrations.'
- ),
- )
- parser.add_argument(
- "--mixed_precision",
- type=str,
- default=None,
- choices=["no", "fp16", "bf16"],
- help=(
- "Whether to use mixed precision. Choose between fp16 and bf16 (bfloat16). Bf16 requires PyTorch >="
- " 1.10.and an Nvidia Ampere GPU. Default to the value of accelerate config of the current system or the"
- " flag passed with the `accelerate.launch` command. Use this argument to override the accelerate config."
- ),
- )
- parser.add_argument(
- "--prior_generation_precision",
- type=str,
- default=None,
- choices=["no", "fp32", "fp16", "bf16"],
- help=(
- "Choose prior generation precision between fp32, fp16 and bf16 (bfloat16). Bf16 requires PyTorch >="
- " 1.10.and an Nvidia Ampere GPU. Default to fp16 if a GPU is available else fp32."
- ),
- )
- parser.add_argument(
- "--concepts_list",
- type=str,
- default=None,
- help="Path to json containing multiple concepts, will overwrite parameters like instance_prompt, class_prompt, etc.",
- )
- parser.add_argument("--local_rank", type=int, default=-1, help="For distributed training: local_rank")
- parser.add_argument(
- "--enable_xformers_memory_efficient_attention", action="store_true", help="Whether or not to use xformers."
- )
- parser.add_argument(
- "--set_grads_to_none",
- action="store_true",
- help=(
- "Save more memory by using setting grads to None instead of zero. Be aware, that this changes certain"
- " behaviors, so disable this argument if it causes any problems. More info:"
- " https://pytorch.org/docs/stable/generated/torch.optim.Optimizer.zero_grad.html"
- ),
- )
- parser.add_argument(
- "--modifier_token",
- type=str,
- default=None,
- help="A token to use as a modifier for the concept.",
- )
- parser.add_argument(
- "--initializer_token", type=str, default="ktn+pll+ucd", help="A token to use as initializer word."
- )
- parser.add_argument("--hflip", action="store_true", help="Apply horizontal flip data augmentation.")
- parser.add_argument(
- "--noaug",
- action="store_true",
- help="Dont apply augmentation during data augmentation when this flag is enabled.",
- )
- parser.add_argument(
- "--no_safe_serialization",
- action="store_true",
- help="If specified save the checkpoint not in `safetensors` format, but in original PyTorch format instead.",
- )
-
- if input_args is not None:
- args = parser.parse_args(input_args)
- else:
- args = parser.parse_args()
-
- env_local_rank = int(os.environ.get("LOCAL_RANK", -1))
- if env_local_rank != -1 and env_local_rank != args.local_rank:
- args.local_rank = env_local_rank
-
- if args.with_prior_preservation:
- if args.concepts_list is None:
- if args.class_data_dir is None:
- raise ValueError("You must specify a data directory for class images.")
- if args.class_prompt is None:
- raise ValueError("You must specify prompt for class images.")
- else:
- # logger is not available yet
- if args.class_data_dir is not None:
- warnings.warn("You need not use --class_data_dir without --with_prior_preservation.")
- if args.class_prompt is not None:
- warnings.warn("You need not use --class_prompt without --with_prior_preservation.")
-
- return args
-
-
-def main(args):
- logging_dir = Path(args.output_dir, args.logging_dir)
-
- accelerator_project_config = ProjectConfiguration(project_dir=args.output_dir, logging_dir=logging_dir)
-
- accelerator = Accelerator(
- gradient_accumulation_steps=args.gradient_accumulation_steps,
- mixed_precision=args.mixed_precision,
- log_with=args.report_to,
- project_config=accelerator_project_config,
- )
-
- if args.report_to == "wandb":
- if not is_wandb_available():
- raise ImportError("Make sure to install wandb if you want to use it for logging during training.")
- import wandb
-
- # Currently, it's not possible to do gradient accumulation when training two models with accelerate.accumulate
- # This will be enabled soon in accelerate. For now, we don't allow gradient accumulation when training two models.
- # TODO (patil-suraj): Remove this check when gradient accumulation with two models is enabled in accelerate.
- # Make one log on every process with the configuration for debugging.
- logging.basicConfig(
- format="%(asctime)s - %(levelname)s - %(name)s - %(message)s",
- datefmt="%m/%d/%Y %H:%M:%S",
- level=logging.INFO,
- )
- logger.info(accelerator.state, main_process_only=False)
- if accelerator.is_local_main_process:
- transformers.utils.logging.set_verbosity_warning()
- diffusers.utils.logging.set_verbosity_info()
- else:
- transformers.utils.logging.set_verbosity_error()
- diffusers.utils.logging.set_verbosity_error()
-
- # We need to initialize the trackers we use, and also store our configuration.
- # The trackers initializes automatically on the main process.
- if accelerator.is_main_process:
- accelerator.init_trackers("custom-diffusion", config=vars(args))
-
- # If passed along, set the training seed now.
- if args.seed is not None:
- set_seed(args.seed)
- if args.concepts_list is None:
- args.concepts_list = [
- {
- "instance_prompt": args.instance_prompt,
- "class_prompt": args.class_prompt,
- "instance_data_dir": args.instance_data_dir,
- "class_data_dir": args.class_data_dir,
- }
- ]
- else:
- with open(args.concepts_list, "r") as f:
- args.concepts_list = json.load(f)
-
- # Generate class images if prior preservation is enabled.
- if args.with_prior_preservation:
- for i, concept in enumerate(args.concepts_list):
- class_images_dir = Path(concept["class_data_dir"])
- if not class_images_dir.exists():
- class_images_dir.mkdir(parents=True, exist_ok=True)
- if args.real_prior:
- assert (
- class_images_dir / "images"
- ).exists(), f"Please run: python retrieve.py --class_prompt \"{concept['class_prompt']}\" --class_data_dir {class_images_dir} --num_class_images {args.num_class_images}"
- assert (
- len(list((class_images_dir / "images").iterdir())) == args.num_class_images
- ), f"Please run: python retrieve.py --class_prompt \"{concept['class_prompt']}\" --class_data_dir {class_images_dir} --num_class_images {args.num_class_images}"
- assert (
- class_images_dir / "caption.txt"
- ).exists(), f"Please run: python retrieve.py --class_prompt \"{concept['class_prompt']}\" --class_data_dir {class_images_dir} --num_class_images {args.num_class_images}"
- assert (
- class_images_dir / "images.txt"
- ).exists(), f"Please run: python retrieve.py --class_prompt \"{concept['class_prompt']}\" --class_data_dir {class_images_dir} --num_class_images {args.num_class_images}"
- concept["class_prompt"] = os.path.join(class_images_dir, "caption.txt")
- concept["class_data_dir"] = os.path.join(class_images_dir, "images.txt")
- args.concepts_list[i] = concept
- accelerator.wait_for_everyone()
- else:
- cur_class_images = len(list(class_images_dir.iterdir()))
-
- if cur_class_images < args.num_class_images:
- torch_dtype = torch.float16 if accelerator.device.type == "cuda" else torch.float32
- if args.prior_generation_precision == "fp32":
- torch_dtype = torch.float32
- elif args.prior_generation_precision == "fp16":
- torch_dtype = torch.float16
- elif args.prior_generation_precision == "bf16":
- torch_dtype = torch.bfloat16
- pipeline = DiffusionPipeline.from_pretrained(
- args.pretrained_model_name_or_path,
- torch_dtype=torch_dtype,
- safety_checker=None,
- revision=args.revision,
- )
- pipeline.set_progress_bar_config(disable=True)
-
- num_new_images = args.num_class_images - cur_class_images
- logger.info(f"Number of class images to sample: {num_new_images}.")
-
- sample_dataset = PromptDataset(args.class_prompt, num_new_images)
- sample_dataloader = torch.utils.data.DataLoader(sample_dataset, batch_size=args.sample_batch_size)
-
- sample_dataloader = accelerator.prepare(sample_dataloader)
- pipeline.to(accelerator.device)
-
- for example in tqdm(
- sample_dataloader,
- desc="Generating class images",
- disable=not accelerator.is_local_main_process,
- ):
- images = pipeline(example["prompt"]).images
-
- for i, image in enumerate(images):
- hash_image = hashlib.sha1(image.tobytes()).hexdigest()
- image_filename = (
- class_images_dir / f"{example['index'][i] + cur_class_images}-{hash_image}.jpg"
- )
- image.save(image_filename)
-
- del pipeline
- if torch.cuda.is_available():
- torch.cuda.empty_cache()
-
- # Handle the repository creation
- if accelerator.is_main_process:
- if args.output_dir is not None:
- os.makedirs(args.output_dir, exist_ok=True)
-
- if args.push_to_hub:
- repo_id = create_repo(
- repo_id=args.hub_model_id or Path(args.output_dir).name, exist_ok=True, token=args.hub_token
- ).repo_id
-
- # Load the tokenizer
- if args.tokenizer_name:
- tokenizer = AutoTokenizer.from_pretrained(
- args.tokenizer_name,
- revision=args.revision,
- use_fast=False,
- )
- elif args.pretrained_model_name_or_path:
- tokenizer = AutoTokenizer.from_pretrained(
- args.pretrained_model_name_or_path,
- subfolder="tokenizer",
- revision=args.revision,
- use_fast=False,
- )
-
- # import correct text encoder class
- text_encoder_cls = import_model_class_from_model_name_or_path(args.pretrained_model_name_or_path, args.revision)
-
- # Load scheduler and models
- noise_scheduler = DDPMScheduler.from_pretrained(args.pretrained_model_name_or_path, subfolder="scheduler")
- text_encoder = text_encoder_cls.from_pretrained(
- args.pretrained_model_name_or_path, subfolder="text_encoder", revision=args.revision
- )
- vae = AutoencoderKL.from_pretrained(args.pretrained_model_name_or_path, subfolder="vae", revision=args.revision)
- unet = UNet2DConditionModel.from_pretrained(
- args.pretrained_model_name_or_path, subfolder="unet", revision=args.revision
- )
-
- # Adding a modifier token which is optimized ####
- # Code taken from https://github.com/huggingface/diffusers/blob/main/examples/textual_inversion/textual_inversion.py
- modifier_token_id = []
- initializer_token_id = []
- if args.modifier_token is not None:
- args.modifier_token = args.modifier_token.split("+")
- args.initializer_token = args.initializer_token.split("+")
- if len(args.modifier_token) > len(args.initializer_token):
- raise ValueError("You must specify + separated initializer token for each modifier token.")
- for modifier_token, initializer_token in zip(
- args.modifier_token, args.initializer_token[: len(args.modifier_token)]
- ):
- # Add the placeholder token in tokenizer
- num_added_tokens = tokenizer.add_tokens(modifier_token)
- if num_added_tokens == 0:
- raise ValueError(
- f"The tokenizer already contains the token {modifier_token}. Please pass a different"
- " `modifier_token` that is not already in the tokenizer."
- )
-
- # Convert the initializer_token, placeholder_token to ids
- token_ids = tokenizer.encode([initializer_token], add_special_tokens=False)
- print(token_ids)
- # Check if initializer_token is a single token or a sequence of tokens
- if len(token_ids) > 1:
- raise ValueError("The initializer token must be a single token.")
-
- initializer_token_id.append(token_ids[0])
- modifier_token_id.append(tokenizer.convert_tokens_to_ids(modifier_token))
-
- # Resize the token embeddings as we are adding new special tokens to the tokenizer
- text_encoder.resize_token_embeddings(len(tokenizer))
-
- # Initialise the newly added placeholder token with the embeddings of the initializer token
- token_embeds = text_encoder.get_input_embeddings().weight.data
- for x, y in zip(modifier_token_id, initializer_token_id):
- token_embeds[x] = token_embeds[y]
-
- # Freeze all parameters except for the token embeddings in text encoder
- params_to_freeze = itertools.chain(
- text_encoder.text_model.encoder.parameters(),
- text_encoder.text_model.final_layer_norm.parameters(),
- text_encoder.text_model.embeddings.position_embedding.parameters(),
- )
- freeze_params(params_to_freeze)
- ########################################################
- ########################################################
-
- vae.requires_grad_(False)
- if args.modifier_token is None:
- text_encoder.requires_grad_(False)
- unet.requires_grad_(False)
- # For mixed precision training we cast the text_encoder and vae weights to half-precision
- # as these models are only used for inference, keeping weights in full precision is not required.
- weight_dtype = torch.float32
- if accelerator.mixed_precision == "fp16":
- weight_dtype = torch.float16
- elif accelerator.mixed_precision == "bf16":
- weight_dtype = torch.bfloat16
-
- # Move unet, vae and text_encoder to device and cast to weight_dtype
- if accelerator.mixed_precision != "fp16" and args.modifier_token is not None:
- text_encoder.to(accelerator.device, dtype=weight_dtype)
- unet.to(accelerator.device, dtype=weight_dtype)
- vae.to(accelerator.device, dtype=weight_dtype)
-
- attention_class = (
- CustomDiffusionAttnProcessor2_0 if hasattr(F, "scaled_dot_product_attention") else CustomDiffusionAttnProcessor
- )
- if args.enable_xformers_memory_efficient_attention:
- if is_xformers_available():
- import xformers
-
- xformers_version = version.parse(xformers.__version__)
- if xformers_version == version.parse("0.0.16"):
- logger.warn(
- "xFormers 0.0.16 cannot be used for training in some GPUs. If you observe problems during training, please update xFormers to at least 0.0.17. See https://huggingface.co/docs/diffusers/main/en/optimization/xformers for more details."
- )
- attention_class = CustomDiffusionXFormersAttnProcessor
- else:
- raise ValueError("xformers is not available. Make sure it is installed correctly")
-
- # now we will add new Custom Diffusion weights to the attention layers
- # It's important to realize here how many attention weights will be added and of which sizes
- # The sizes of the attention layers consist only of two different variables:
- # 1) - the "hidden_size", which is increased according to `unet.config.block_out_channels`.
- # 2) - the "cross attention size", which is set to `unet.config.cross_attention_dim`.
-
- # Let's first see how many attention processors we will have to set.
- # For Stable Diffusion, it should be equal to:
- # - down blocks (2x attention layers) * (2x transformer layers) * (3x down blocks) = 12
- # - mid blocks (2x attention layers) * (1x transformer layers) * (1x mid blocks) = 2
- # - up blocks (2x attention layers) * (3x transformer layers) * (3x down blocks) = 18
- # => 32 layers
-
- # Only train key, value projection layers if freeze_model = 'crossattn_kv' else train all params in the cross attention layer
- train_kv = True
- train_q_out = False if args.freeze_model == "crossattn_kv" else True
- custom_diffusion_attn_procs = {}
-
- st = unet.state_dict()
- for name, _ in unet.attn_processors.items():
- cross_attention_dim = None if name.endswith("attn1.processor") else unet.config.cross_attention_dim
- if name.startswith("mid_block"):
- hidden_size = unet.config.block_out_channels[-1]
- elif name.startswith("up_blocks"):
- block_id = int(name[len("up_blocks.")])
- hidden_size = list(reversed(unet.config.block_out_channels))[block_id]
- elif name.startswith("down_blocks"):
- block_id = int(name[len("down_blocks.")])
- hidden_size = unet.config.block_out_channels[block_id]
- layer_name = name.split(".processor")[0]
- weights = {
- "to_k_custom_diffusion.weight": st[layer_name + ".to_k.weight"],
- "to_v_custom_diffusion.weight": st[layer_name + ".to_v.weight"],
- }
- if train_q_out:
- weights["to_q_custom_diffusion.weight"] = st[layer_name + ".to_q.weight"]
- weights["to_out_custom_diffusion.0.weight"] = st[layer_name + ".to_out.0.weight"]
- weights["to_out_custom_diffusion.0.bias"] = st[layer_name + ".to_out.0.bias"]
- if cross_attention_dim is not None:
- custom_diffusion_attn_procs[name] = attention_class(
- train_kv=train_kv,
- train_q_out=train_q_out,
- hidden_size=hidden_size,
- cross_attention_dim=cross_attention_dim,
- ).to(unet.device)
- custom_diffusion_attn_procs[name].load_state_dict(weights)
- else:
- custom_diffusion_attn_procs[name] = attention_class(
- train_kv=False,
- train_q_out=False,
- hidden_size=hidden_size,
- cross_attention_dim=cross_attention_dim,
- )
- del st
- unet.set_attn_processor(custom_diffusion_attn_procs)
- custom_diffusion_layers = AttnProcsLayers(unet.attn_processors)
-
- accelerator.register_for_checkpointing(custom_diffusion_layers)
-
- if args.gradient_checkpointing:
- unet.enable_gradient_checkpointing()
- if args.modifier_token is not None:
- text_encoder.gradient_checkpointing_enable()
- # Enable TF32 for faster training on Ampere GPUs,
- # cf https://pytorch.org/docs/stable/notes/cuda.html#tensorfloat-32-tf32-on-ampere-devices
- if args.allow_tf32:
- torch.backends.cuda.matmul.allow_tf32 = True
-
- if args.scale_lr:
- args.learning_rate = (
- args.learning_rate * args.gradient_accumulation_steps * args.train_batch_size * accelerator.num_processes
- )
- if args.with_prior_preservation:
- args.learning_rate = args.learning_rate * 2.0
-
- # Use 8-bit Adam for lower memory usage or to fine-tune the model in 16GB GPUs
- if args.use_8bit_adam:
- try:
- import bitsandbytes as bnb
- except ImportError:
- raise ImportError(
- "To use 8-bit Adam, please install the bitsandbytes library: `pip install bitsandbytes`."
- )
-
- optimizer_class = bnb.optim.AdamW8bit
- else:
- optimizer_class = torch.optim.AdamW
-
- # Optimizer creation
- optimizer = optimizer_class(
- itertools.chain(text_encoder.get_input_embeddings().parameters(), custom_diffusion_layers.parameters())
- if args.modifier_token is not None
- else custom_diffusion_layers.parameters(),
- lr=args.learning_rate,
- betas=(args.adam_beta1, args.adam_beta2),
- weight_decay=args.adam_weight_decay,
- eps=args.adam_epsilon,
- )
-
- # Dataset and DataLoaders creation:
- train_dataset = CustomDiffusionDataset(
- concepts_list=args.concepts_list,
- tokenizer=tokenizer,
- with_prior_preservation=args.with_prior_preservation,
- size=args.resolution,
- mask_size=vae.encode(
- torch.randn(1, 3, args.resolution, args.resolution).to(dtype=weight_dtype).to(accelerator.device)
- )
- .latent_dist.sample()
- .size()[-1],
- center_crop=args.center_crop,
- num_class_images=args.num_class_images,
- hflip=args.hflip,
- aug=not args.noaug,
- )
-
- train_dataloader = torch.utils.data.DataLoader(
- train_dataset,
- batch_size=args.train_batch_size,
- shuffle=True,
- collate_fn=lambda examples: collate_fn(examples, args.with_prior_preservation),
- num_workers=args.dataloader_num_workers,
- )
-
- # Scheduler and math around the number of training steps.
- overrode_max_train_steps = False
- num_update_steps_per_epoch = math.ceil(len(train_dataloader) / args.gradient_accumulation_steps)
- if args.max_train_steps is None:
- args.max_train_steps = args.num_train_epochs * num_update_steps_per_epoch
- overrode_max_train_steps = True
-
- lr_scheduler = get_scheduler(
- args.lr_scheduler,
- optimizer=optimizer,
- num_warmup_steps=args.lr_warmup_steps * accelerator.num_processes,
- num_training_steps=args.max_train_steps * accelerator.num_processes,
- )
-
- # Prepare everything with our `accelerator`.
- if args.modifier_token is not None:
- custom_diffusion_layers, text_encoder, optimizer, train_dataloader, lr_scheduler = accelerator.prepare(
- custom_diffusion_layers, text_encoder, optimizer, train_dataloader, lr_scheduler
- )
- else:
- custom_diffusion_layers, optimizer, train_dataloader, lr_scheduler = accelerator.prepare(
- custom_diffusion_layers, optimizer, train_dataloader, lr_scheduler
- )
-
- # We need to recalculate our total training steps as the size of the training dataloader may have changed.
- num_update_steps_per_epoch = math.ceil(len(train_dataloader) / args.gradient_accumulation_steps)
- if overrode_max_train_steps:
- args.max_train_steps = args.num_train_epochs * num_update_steps_per_epoch
- # Afterwards we recalculate our number of training epochs
- args.num_train_epochs = math.ceil(args.max_train_steps / num_update_steps_per_epoch)
-
- # Train!
- total_batch_size = args.train_batch_size * accelerator.num_processes * args.gradient_accumulation_steps
-
- logger.info("***** Running training *****")
- logger.info(f" Num examples = {len(train_dataset)}")
- logger.info(f" Num batches each epoch = {len(train_dataloader)}")
- logger.info(f" Num Epochs = {args.num_train_epochs}")
- logger.info(f" Instantaneous batch size per device = {args.train_batch_size}")
- logger.info(f" Total train batch size (w. parallel, distributed & accumulation) = {total_batch_size}")
- logger.info(f" Gradient Accumulation steps = {args.gradient_accumulation_steps}")
- logger.info(f" Total optimization steps = {args.max_train_steps}")
- global_step = 0
- first_epoch = 0
-
- # Potentially load in the weights and states from a previous save
- if args.resume_from_checkpoint:
- if args.resume_from_checkpoint != "latest":
- path = os.path.basename(args.resume_from_checkpoint)
- else:
- # Get the most recent checkpoint
- dirs = os.listdir(args.output_dir)
- dirs = [d for d in dirs if d.startswith("checkpoint")]
- dirs = sorted(dirs, key=lambda x: int(x.split("-")[1]))
- path = dirs[-1] if len(dirs) > 0 else None
-
- if path is None:
- accelerator.print(
- f"Checkpoint '{args.resume_from_checkpoint}' does not exist. Starting a new training run."
- )
- args.resume_from_checkpoint = None
- else:
- accelerator.print(f"Resuming from checkpoint {path}")
- accelerator.load_state(os.path.join(args.output_dir, path))
- global_step = int(path.split("-")[1])
-
- resume_global_step = global_step * args.gradient_accumulation_steps
- first_epoch = global_step // num_update_steps_per_epoch
- resume_step = resume_global_step % (num_update_steps_per_epoch * args.gradient_accumulation_steps)
-
- # Only show the progress bar once on each machine.
- progress_bar = tqdm(range(global_step, args.max_train_steps), disable=not accelerator.is_local_main_process)
- progress_bar.set_description("Steps")
-
- for epoch in range(first_epoch, args.num_train_epochs):
- unet.train()
- if args.modifier_token is not None:
- text_encoder.train()
- for step, batch in enumerate(train_dataloader):
- # Skip steps until we reach the resumed step
- if args.resume_from_checkpoint and epoch == first_epoch and step < resume_step:
- if step % args.gradient_accumulation_steps == 0:
- progress_bar.update(1)
- continue
-
- with accelerator.accumulate(unet), accelerator.accumulate(text_encoder):
- # Convert images to latent space
- latents = vae.encode(batch["pixel_values"].to(dtype=weight_dtype)).latent_dist.sample()
- latents = latents * vae.config.scaling_factor
-
- # Sample noise that we'll add to the latents
- noise = torch.randn_like(latents)
- bsz = latents.shape[0]
- # Sample a random timestep for each image
- timesteps = torch.randint(0, noise_scheduler.config.num_train_timesteps, (bsz,), device=latents.device)
- timesteps = timesteps.long()
-
- # Add noise to the latents according to the noise magnitude at each timestep
- # (this is the forward diffusion process)
- noisy_latents = noise_scheduler.add_noise(latents, noise, timesteps)
-
- # Get the text embedding for conditioning
- encoder_hidden_states = text_encoder(batch["input_ids"])[0]
-
- # Predict the noise residual
- model_pred = unet(noisy_latents, timesteps, encoder_hidden_states).sample
-
- # Get the target for loss depending on the prediction type
- if noise_scheduler.config.prediction_type == "epsilon":
- target = noise
- elif noise_scheduler.config.prediction_type == "v_prediction":
- target = noise_scheduler.get_velocity(latents, noise, timesteps)
- else:
- raise ValueError(f"Unknown prediction type {noise_scheduler.config.prediction_type}")
-
- if args.with_prior_preservation:
- # Chunk the noise and model_pred into two parts and compute the loss on each part separately.
- model_pred, model_pred_prior = torch.chunk(model_pred, 2, dim=0)
- target, target_prior = torch.chunk(target, 2, dim=0)
- mask = torch.chunk(batch["mask"], 2, dim=0)[0]
- # Compute instance loss
- loss = F.mse_loss(model_pred.float(), target.float(), reduction="none")
- loss = ((loss * mask).sum([1, 2, 3]) / mask.sum([1, 2, 3])).mean()
-
- # Compute prior loss
- prior_loss = F.mse_loss(model_pred_prior.float(), target_prior.float(), reduction="mean")
-
- # Add the prior loss to the instance loss.
- loss = loss + args.prior_loss_weight * prior_loss
- else:
- mask = batch["mask"]
- loss = F.mse_loss(model_pred.float(), target.float(), reduction="none")
- loss = ((loss * mask).sum([1, 2, 3]) / mask.sum([1, 2, 3])).mean()
- accelerator.backward(loss)
- # Zero out the gradients for all token embeddings except the newly added
- # embeddings for the concept, as we only want to optimize the concept embeddings
- if args.modifier_token is not None:
- if accelerator.num_processes > 1:
- grads_text_encoder = text_encoder.module.get_input_embeddings().weight.grad
- else:
- grads_text_encoder = text_encoder.get_input_embeddings().weight.grad
- # Get the index for tokens that we want to zero the grads for
- index_grads_to_zero = torch.arange(len(tokenizer)) != modifier_token_id[0]
- for i in range(len(modifier_token_id[1:])):
- index_grads_to_zero = index_grads_to_zero & (
- torch.arange(len(tokenizer)) != modifier_token_id[i]
- )
- grads_text_encoder.data[index_grads_to_zero, :] = grads_text_encoder.data[
- index_grads_to_zero, :
- ].fill_(0)
-
- if accelerator.sync_gradients:
- params_to_clip = (
- itertools.chain(text_encoder.parameters(), custom_diffusion_layers.parameters())
- if args.modifier_token is not None
- else custom_diffusion_layers.parameters()
- )
- accelerator.clip_grad_norm_(params_to_clip, args.max_grad_norm)
- optimizer.step()
- lr_scheduler.step()
- optimizer.zero_grad(set_to_none=args.set_grads_to_none)
-
- # Checks if the accelerator has performed an optimization step behind the scenes
- if accelerator.sync_gradients:
- progress_bar.update(1)
- global_step += 1
-
- if global_step % args.checkpointing_steps == 0:
- if accelerator.is_main_process:
- # _before_ saving state, check if this save would set us over the `checkpoints_total_limit`
- if args.checkpoints_total_limit is not None:
- checkpoints = os.listdir(args.output_dir)
- checkpoints = [d for d in checkpoints if d.startswith("checkpoint")]
- checkpoints = sorted(checkpoints, key=lambda x: int(x.split("-")[1]))
-
- # before we save the new checkpoint, we need to have at _most_ `checkpoints_total_limit - 1` checkpoints
- if len(checkpoints) >= args.checkpoints_total_limit:
- num_to_remove = len(checkpoints) - args.checkpoints_total_limit + 1
- removing_checkpoints = checkpoints[0:num_to_remove]
-
- logger.info(
- f"{len(checkpoints)} checkpoints already exist, removing {len(removing_checkpoints)} checkpoints"
- )
- logger.info(f"removing checkpoints: {', '.join(removing_checkpoints)}")
-
- for removing_checkpoint in removing_checkpoints:
- removing_checkpoint = os.path.join(args.output_dir, removing_checkpoint)
- shutil.rmtree(removing_checkpoint)
-
- save_path = os.path.join(args.output_dir, f"checkpoint-{global_step}")
- accelerator.save_state(save_path)
- logger.info(f"Saved state to {save_path}")
-
- logs = {"loss": loss.detach().item(), "lr": lr_scheduler.get_last_lr()[0]}
- progress_bar.set_postfix(**logs)
- accelerator.log(logs, step=global_step)
-
- if global_step >= args.max_train_steps:
- break
-
- if accelerator.is_main_process:
- images = []
-
- if args.validation_prompt is not None and global_step % args.validation_steps == 0:
- logger.info(
- f"Running validation... \n Generating {args.num_validation_images} images with prompt:"
- f" {args.validation_prompt}."
- )
- # create pipeline
- pipeline = DiffusionPipeline.from_pretrained(
- args.pretrained_model_name_or_path,
- unet=accelerator.unwrap_model(unet),
- text_encoder=accelerator.unwrap_model(text_encoder),
- tokenizer=tokenizer,
- revision=args.revision,
- torch_dtype=weight_dtype,
- )
- pipeline.scheduler = DPMSolverMultistepScheduler.from_config(pipeline.scheduler.config)
- pipeline = pipeline.to(accelerator.device)
- pipeline.set_progress_bar_config(disable=True)
-
- # run inference
- generator = torch.Generator(device=accelerator.device).manual_seed(args.seed)
- images = [
- pipeline(args.validation_prompt, num_inference_steps=25, generator=generator, eta=1.0).images[0]
- for _ in range(args.num_validation_images)
- ]
-
- for tracker in accelerator.trackers:
- if tracker.name == "tensorboard":
- np_images = np.stack([np.asarray(img) for img in images])
- tracker.writer.add_images("validation", np_images, epoch, dataformats="NHWC")
- if tracker.name == "wandb":
- tracker.log(
- {
- "validation": [
- wandb.Image(image, caption=f"{i}: {args.validation_prompt}")
- for i, image in enumerate(images)
- ]
- }
- )
-
- del pipeline
- torch.cuda.empty_cache()
-
- # Save the custom diffusion layers
- accelerator.wait_for_everyone()
- if accelerator.is_main_process:
- unet = unet.to(torch.float32)
- unet.save_attn_procs(args.output_dir, safe_serialization=not args.no_safe_serialization)
- save_new_embed(
- text_encoder,
- modifier_token_id,
- accelerator,
- args,
- args.output_dir,
- safe_serialization=not args.no_safe_serialization,
- )
-
- # Final inference
- # Load previous pipeline
- pipeline = DiffusionPipeline.from_pretrained(
- args.pretrained_model_name_or_path, revision=args.revision, torch_dtype=weight_dtype
- )
- pipeline.scheduler = DPMSolverMultistepScheduler.from_config(pipeline.scheduler.config)
- pipeline = pipeline.to(accelerator.device)
-
- # load attention processors
- weight_name = (
- "pytorch_custom_diffusion_weights.safetensors"
- if not args.no_safe_serialization
- else "pytorch_custom_diffusion_weights.bin"
- )
- pipeline.unet.load_attn_procs(args.output_dir, weight_name=weight_name)
- for token in args.modifier_token:
- token_weight_name = f"{token}.safetensors" if not args.no_safe_serialization else f"{token}.bin"
- pipeline.load_textual_inversion(args.output_dir, weight_name=token_weight_name)
-
- # run inference
- if args.validation_prompt and args.num_validation_images > 0:
- generator = torch.Generator(device=accelerator.device).manual_seed(args.seed) if args.seed else None
- images = [
- pipeline(args.validation_prompt, num_inference_steps=25, generator=generator, eta=1.0).images[0]
- for _ in range(args.num_validation_images)
- ]
-
- for tracker in accelerator.trackers:
- if tracker.name == "tensorboard":
- np_images = np.stack([np.asarray(img) for img in images])
- tracker.writer.add_images("test", np_images, epoch, dataformats="NHWC")
- if tracker.name == "wandb":
- tracker.log(
- {
- "test": [
- wandb.Image(image, caption=f"{i}: {args.validation_prompt}")
- for i, image in enumerate(images)
- ]
- }
- )
-
- if args.push_to_hub:
- save_model_card(
- repo_id,
- images=images,
- base_model=args.pretrained_model_name_or_path,
- prompt=args.instance_prompt,
- repo_folder=args.output_dir,
- )
- api = HfApi(token=args.hub_token)
- api.upload_folder(
- repo_id=repo_id,
- folder_path=args.output_dir,
- commit_message="End of training",
- ignore_patterns=["step_*", "epoch_*"],
- )
-
- accelerator.end_training()
-
-
-if __name__ == "__main__":
- args = parse_args()
- main(args)
diff --git a/spaces/patgpt4/MusicGen/audiocraft/quantization/base.py b/spaces/patgpt4/MusicGen/audiocraft/quantization/base.py
deleted file mode 100644
index 1b16c130d266fbd021d3fc29bb9f98c33dd3c588..0000000000000000000000000000000000000000
--- a/spaces/patgpt4/MusicGen/audiocraft/quantization/base.py
+++ /dev/null
@@ -1,107 +0,0 @@
-# Copyright (c) Meta Platforms, Inc. and affiliates.
-# All rights reserved.
-#
-# This source code is licensed under the license found in the
-# LICENSE file in the root directory of this source tree.
-
-"""
-Base class for all quantizers.
-"""
-
-from dataclasses import dataclass, field
-import typing as tp
-
-import torch
-from torch import nn
-
-
-@dataclass
-class QuantizedResult:
- x: torch.Tensor
- codes: torch.Tensor
- bandwidth: torch.Tensor # bandwidth in kb/s used, per batch item.
- penalty: tp.Optional[torch.Tensor] = None
- metrics: dict = field(default_factory=dict)
-
-
-class BaseQuantizer(nn.Module):
- """Base class for quantizers.
- """
-
- def forward(self, x: torch.Tensor, frame_rate: int) -> QuantizedResult:
- """
- Given input tensor x, returns first the quantized (or approximately quantized)
- representation along with quantized codes, bandwidth, and any penalty term for the loss.
- Finally, this returns a dict of metrics to update logging etc.
- Frame rate must be passed so that the bandwidth is properly computed.
- """
- raise NotImplementedError()
-
- def encode(self, x: torch.Tensor) -> torch.Tensor:
- """Encode a given input tensor with the specified sample rate at the given bandwidth.
- """
- raise NotImplementedError()
-
- def decode(self, codes: torch.Tensor) -> torch.Tensor:
- """Decode the given codes to the quantized representation.
- """
- raise NotImplementedError()
-
- @property
- def total_codebooks(self):
- """Total number of codebooks.
- """
- raise NotImplementedError()
-
- @property
- def num_codebooks(self):
- """Number of active codebooks.
- """
- raise NotImplementedError()
-
- def set_num_codebooks(self, n: int):
- """Set the number of active codebooks.
- """
- raise NotImplementedError()
-
-
-class DummyQuantizer(BaseQuantizer):
- """Fake quantizer that actually does not perform any quantization.
- """
- def __init__(self):
- super().__init__()
-
- def forward(self, x: torch.Tensor, frame_rate: int):
- q = x.unsqueeze(1)
- return QuantizedResult(x, q, torch.tensor(q.numel() * 32 * frame_rate / 1000 / len(x)).to(x))
-
- def encode(self, x: torch.Tensor) -> torch.Tensor:
- """Encode a given input tensor with the specified sample rate at the given bandwidth.
- In the case of the DummyQuantizer, the codes are actually identical
- to the input and resulting quantized representation as no quantization is done.
- """
- return x.unsqueeze(1)
-
- def decode(self, codes: torch.Tensor) -> torch.Tensor:
- """Decode the given codes to the quantized representation.
- In the case of the DummyQuantizer, the codes are actually identical
- to the input and resulting quantized representation as no quantization is done.
- """
- return codes.squeeze(1)
-
- @property
- def total_codebooks(self):
- """Total number of codebooks.
- """
- return 1
-
- @property
- def num_codebooks(self):
- """Total number of codebooks.
- """
- return self.total_codebooks
-
- def set_num_codebooks(self, n: int):
- """Set the number of active codebooks.
- """
- raise AttributeError("Cannot override the number of codebooks for the dummy quantizer")
diff --git a/spaces/paulokewunmi/omowe.ai/README.md b/spaces/paulokewunmi/omowe.ai/README.md
deleted file mode 100644
index 5784ead4025120f70e89df49c03fd977df9b270f..0000000000000000000000000000000000000000
--- a/spaces/paulokewunmi/omowe.ai/README.md
+++ /dev/null
@@ -1,13 +0,0 @@
----
-title: Omowe.ai
-emoji: 🏢
-colorFrom: blue
-colorTo: green
-sdk: gradio
-sdk_version: 3.28.2
-app_file: app.py
-pinned: false
-license: mit
----
-
-Check out the configuration reference at https://huggingface.co/docs/hub/spaces-config-reference
diff --git a/spaces/pcuenq/paella/app.py b/spaces/pcuenq/paella/app.py
deleted file mode 100644
index 76e8c6710e31d7750b0a5f0e93c6aa06648f5e6e..0000000000000000000000000000000000000000
--- a/spaces/pcuenq/paella/app.py
+++ /dev/null
@@ -1,460 +0,0 @@
-import gradio as gr
-import torch
-import open_clip
-import torchvision
-from huggingface_hub import hf_hub_download
-from PIL import Image
-from open_clip import tokenizer
-from Paella.utils.modules import Paella
-from arroz import Diffuzz, PriorModel
-from transformers import AutoTokenizer, T5EncoderModel
-from Paella.src.vqgan import VQModel
-from Paella.utils.alter_attention import replace_attention_layers
-
-model_repo = "dome272/Paella"
-model_file = "paella_v3.pt"
-prior_file = "prior_v1.pt"
-vqgan_file = "vqgan_f4.pt"
-
-device = "cuda" if torch.cuda.is_available() else "cpu"
-
-batch_size = 4
-latent_shape = (batch_size, 64, 64) # latent shape of the generated image, we are using an f4 vqgan and thus sampling 64x64 will result in 256x256
-prior_timesteps, prior_cfg, prior_sampler, clip_embedding_shape = 60, 3.0, "ddpm", (batch_size, 1024)
-
-generator_timesteps = 12
-generator_cfg = 5
-prior_timesteps = 60
-prior_cfg = 3.0
-prior_sampler = 'ddpm'
-clip_embedding_shape = (batch_size, 1024)
-
-
-def to_pil(images):
- images = images.permute(0, 2, 3, 1).cpu().numpy()
- images = (images * 255).round().astype("uint8")
- images = [Image.fromarray(image) for image in images]
- return images
-
-def log(t, eps=1e-20):
- return torch.log(t + eps)
-
-def gumbel_noise(t):
- noise = torch.zeros_like(t).uniform_(0, 1)
- return -log(-log(noise))
-
-def gumbel_sample(t, temperature=1., dim=-1):
- return ((t / max(temperature, 1e-10)) + gumbel_noise(t)).argmax(dim=dim)
-
-def sample(model, c, x=None, negative_embeddings=None, mask=None, T=12, size=(32, 32), starting_t=0, temp_range=[1.0, 1.0], typical_filtering=True, typical_mass=0.2, typical_min_tokens=1, classifier_free_scale=-1, renoise_steps=11, renoise_mode='start'):
- with torch.inference_mode():
- r_range = torch.linspace(0, 1, T+1)[:-1][:, None].expand(-1, c.size(0)).to(c.device)
- temperatures = torch.linspace(temp_range[0], temp_range[1], T)
- preds = []
- if x is None:
- x = torch.randint(0, model.num_labels, size=(c.size(0), *size), device=c.device)
- elif mask is not None:
- noise = torch.randint(0, model.num_labels, size=(c.size(0), *size), device=c.device)
- x = noise * mask + (1-mask) * x
- init_x = x.clone()
- for i in range(starting_t, T):
- if renoise_mode == 'prev':
- prev_x = x.clone()
- r, temp = r_range[i], temperatures[i]
- logits = model(x, c, r)
- if classifier_free_scale >= 0:
- if negative_embeddings is not None:
- logits_uncond = model(x, negative_embeddings, r)
- else:
- logits_uncond = model(x, torch.zeros_like(c), r)
- logits = torch.lerp(logits_uncond, logits, classifier_free_scale)
- x = logits
- x_flat = x.permute(0, 2, 3, 1).reshape(-1, x.size(1))
- if typical_filtering:
- x_flat_norm = torch.nn.functional.log_softmax(x_flat, dim=-1)
- x_flat_norm_p = torch.exp(x_flat_norm)
- entropy = -(x_flat_norm * x_flat_norm_p).nansum(-1, keepdim=True)
-
- c_flat_shifted = torch.abs((-x_flat_norm) - entropy)
- c_flat_sorted, x_flat_indices = torch.sort(c_flat_shifted, descending=False)
- x_flat_cumsum = x_flat.gather(-1, x_flat_indices).softmax(dim=-1).cumsum(dim=-1)
-
- last_ind = (x_flat_cumsum < typical_mass).sum(dim=-1)
- sorted_indices_to_remove = c_flat_sorted > c_flat_sorted.gather(1, last_ind.view(-1, 1))
- if typical_min_tokens > 1:
- sorted_indices_to_remove[..., :typical_min_tokens] = 0
- indices_to_remove = sorted_indices_to_remove.scatter(1, x_flat_indices, sorted_indices_to_remove)
- x_flat = x_flat.masked_fill(indices_to_remove, -float("Inf"))
- x_flat = torch.multinomial(x_flat.div(temp).softmax(-1), num_samples=1)[:, 0]
- x = x_flat.view(x.size(0), *x.shape[2:])
- if mask is not None:
- x = x * mask + (1-mask) * init_x
- if i < renoise_steps:
- if renoise_mode == 'start':
- x, _ = model.add_noise(x, r_range[i+1], random_x=init_x)
- elif renoise_mode == 'prev':
- x, _ = model.add_noise(x, r_range[i+1], random_x=prev_x)
- else: # 'rand'
- x, _ = model.add_noise(x, r_range[i+1])
- preds.append(x.detach())
- return preds
-
-# Model loading
-
-# Load T5 on CPU
-t5_tokenizer = AutoTokenizer.from_pretrained("google/byt5-xl")
-t5_model = T5EncoderModel.from_pretrained("google/byt5-xl")
-
-# Load other models on GPU
-clip_model, _, _ = open_clip.create_model_and_transforms('ViT-H-14', pretrained='laion2b_s32b_b79k')
-clip_model = clip_model.to(device).half().eval().requires_grad_(False)
-
-clip_preprocess = torchvision.transforms.Compose([
- torchvision.transforms.Resize(224, interpolation=torchvision.transforms.InterpolationMode.BICUBIC),
- torchvision.transforms.Normalize(mean=(0.48145466, 0.4578275, 0.40821073), std=(0.26862954, 0.26130258, 0.27577711)),
-])
-
-vqgan_path = hf_hub_download(repo_id=model_repo, filename=vqgan_file)
-vqmodel = VQModel().to(device)
-vqmodel.load_state_dict(torch.load(vqgan_path, map_location=device))
-vqmodel.eval().requires_grad_(False)
-
-prior_path = hf_hub_download(repo_id=model_repo, filename=prior_file)
-prior = PriorModel().to(device)#.half()
-prior.load_state_dict(torch.load(prior_path, map_location=device))
-prior.eval().requires_grad_(False)
-
-model_path = hf_hub_download(repo_id=model_repo, filename=model_file)
-model = Paella(byt5_embd=2560)
-model.load_state_dict(torch.load(model_path, map_location=device))
-model.eval().requires_grad_()#.half()
-replace_attention_layers(model)
-model.to(device)
-
-diffuzz = Diffuzz(device=device)
-
-@torch.inference_mode()
-def decode(img_seq):
- return vqmodel.decode_indices(img_seq)
-
-@torch.inference_mode()
-def embed_t5(text, t5_tokenizer, t5_model, final_device="cuda"):
- device = t5_model.device
- t5_tokens = t5_tokenizer(text, padding="longest", return_tensors="pt", max_length=768, truncation=True).input_ids.to(device)
- t5_embeddings = t5_model(input_ids=t5_tokens).last_hidden_state.to(final_device)
- return t5_embeddings
-
-@torch.inference_mode()
-def sample(model, model_inputs, latent_shape,
- unconditional_inputs=None, init_x=None, steps=12, renoise_steps=None,
- temperature = (0.7, 0.3), cfg=(8.0, 8.0),
- mode = 'multinomial', # 'quant', 'multinomial', 'argmax'
- t_start=1.0, t_end=0.0,
- sampling_conditional_steps=None, sampling_quant_steps=None, attn_weights=None
- ):
- device = unconditional_inputs["byt5"].device
- if sampling_conditional_steps is None:
- sampling_conditional_steps = steps
- if sampling_quant_steps is None:
- sampling_quant_steps = steps
- if renoise_steps is None:
- renoise_steps = steps-1
- if unconditional_inputs is None:
- unconditional_inputs = {k: torch.zeros_like(v) for k, v in model_inputs.items()}
-
- init_noise = torch.randint(0, model.num_labels, size=latent_shape, device=device)
- if init_x != None:
- sampled = init_x
- else:
- sampled = init_noise.clone()
- t_list = torch.linspace(t_start, t_end, steps+1)
- temperatures = torch.linspace(temperature[0], temperature[1], steps)
- cfgs = torch.linspace(cfg[0], cfg[1], steps)
- for i, tv in enumerate(t_list[:steps]):
- if i >= sampling_quant_steps:
- mode = "quant"
- t = torch.ones(latent_shape[0], device=device) * tv
-
- logits = model(sampled, t, **model_inputs, attn_weights=attn_weights)
- if cfg is not None and i < sampling_conditional_steps:
- logits = logits * cfgs[i] + model(sampled, t, **unconditional_inputs) * (1-cfgs[i])
- scores = logits.div(temperatures[i]).softmax(dim=1)
-
- if mode == 'argmax':
- sampled = logits.argmax(dim=1)
- elif mode == 'multinomial':
- sampled = scores.permute(0, 2, 3, 1).reshape(-1, logits.size(1))
- sampled = torch.multinomial(sampled, 1)[:, 0].view(logits.size(0), *logits.shape[2:])
- elif mode == 'quant':
- sampled = scores.permute(0, 2, 3, 1) @ vqmodel.vquantizer.codebook.weight.data
- sampled = vqmodel.vquantizer.forward(sampled, dim=-1)[-1]
- else:
- raise Exception(f"Mode '{mode}' not supported, use: 'quant', 'multinomial' or 'argmax'")
-
- if i < renoise_steps:
- t_next = torch.ones(latent_shape[0], device=device) * t_list[i+1]
- sampled = model.add_noise(sampled, t_next, random_x=init_noise)[0]
- return sampled
-
-# -----
-
-def infer(prompt, negative_prompt, seed):
- torch.manual_seed(seed)
- text = tokenizer.tokenize([prompt] * latent_shape[0]).to(device)
- with torch.inference_mode():
- if negative_prompt:
- clip_text_tokens_uncond = tokenizer.tokenize([negative_prompt] * len(text)).to(device)
- t5_embeddings_uncond = embed_t5([negative_prompt] * len(text), t5_tokenizer, t5_model)
- else:
- clip_text_tokens_uncond = tokenizer.tokenize([""] * len(text)).to(device)
- t5_embeddings_uncond = embed_t5([""] * len(text), t5_tokenizer, t5_model)
-
- t5_embeddings = embed_t5([prompt] * latent_shape[0], t5_tokenizer, t5_model)
- clip_text_embeddings = clip_model.encode_text(text)
- clip_text_embeddings_uncond = clip_model.encode_text(clip_text_tokens_uncond)
-
- with torch.autocast(device_type="cuda"):
- clip_image_embeddings = diffuzz.sample(
- prior, {'c': clip_text_embeddings}, clip_embedding_shape,
- timesteps=prior_timesteps, cfg=prior_cfg, sampler=prior_sampler
- )[-1]
-
- attn_weights = torch.ones((t5_embeddings.shape[1]))
- attn_weights[-4:] = 0.4 # reweigh attention weights for image embeddings --> less influence
- attn_weights[:-4] = 1.2 # reweigh attention weights for the rest --> more influence
- attn_weights = attn_weights.to(device)
-
- sampled_tokens = sample(model,
- model_inputs={'byt5': t5_embeddings, 'clip': clip_text_embeddings, 'clip_image': clip_image_embeddings}, unconditional_inputs={'byt5': t5_embeddings_uncond, 'clip': clip_text_embeddings_uncond, 'clip_image': None},
- temperature=(1.2, 0.2), cfg=(8,8), steps=32, renoise_steps=26, latent_shape=latent_shape, t_start=1.0, t_end=0.0,
- mode="multinomial", sampling_conditional_steps=20, attn_weights=attn_weights)
-
- sampled = decode(sampled_tokens)
- return to_pil(sampled.clamp(0, 1))
-
-css = """
- .gradio-container {
- font-family: 'IBM Plex Sans', sans-serif;
- }
- .gr-button {
- color: white;
- border-color: black;
- background: black;
- }
- input[type='range'] {
- accent-color: black;
- }
- .dark input[type='range'] {
- accent-color: #dfdfdf;
- }
- .container {
- max-width: 730px;
- margin: auto;
- padding-top: 1.5rem;
- }
- #gallery {
- min-height: 22rem;
- margin-bottom: 15px;
- margin-left: auto;
- margin-right: auto;
- border-bottom-right-radius: .5rem !important;
- border-bottom-left-radius: .5rem !important;
- }
- #gallery>div>.h-full {
- min-height: 20rem;
- }
- .details:hover {
- text-decoration: underline;
- }
- .gr-button {
- white-space: nowrap;
- }
- .gr-button:focus {
- border-color: rgb(147 197 253 / var(--tw-border-opacity));
- outline: none;
- box-shadow: var(--tw-ring-offset-shadow), var(--tw-ring-shadow), var(--tw-shadow, 0 0 #0000);
- --tw-border-opacity: 1;
- --tw-ring-offset-shadow: var(--tw-ring-inset) 0 0 0 var(--tw-ring-offset-width) var(--tw-ring-offset-color);
- --tw-ring-shadow: var(--tw-ring-inset) 0 0 0 calc(3px var(--tw-ring-offset-width)) var(--tw-ring-color);
- --tw-ring-color: rgb(191 219 254 / var(--tw-ring-opacity));
- --tw-ring-opacity: .5;
- }
- .footer {
- margin-bottom: 45px;
- margin-top: 35px;
- text-align: center;
- border-bottom: 1px solid #e5e5e5;
- }
- .footer>p {
- font-size: .8rem;
- display: inline-block;
- padding: 0 10px;
- transform: translateY(10px);
- background: white;
- }
- .dark .footer {
- border-color: #303030;
- }
- .dark .footer>p {
- background: #0b0f19;
- }
- .acknowledgments h4{
- margin: 1.25em 0 .25em 0;
- font-weight: bold;
- font-size: 115%;
- }
- .animate-spin {
- animation: spin 1s linear infinite;
- }
- @keyframes spin {
- from {
- transform: rotate(0deg);
- }
- to {
- transform: rotate(360deg);
- }
- }
- #share-btn-container {
- display: flex; padding-left: 0.5rem !important; padding-right: 0.5rem !important; background-color: #000000; justify-content: center; align-items: center; border-radius: 9999px !important; width: 13rem;
- }
- #share-btn {
- all: initial; color: #ffffff;font-weight: 600; cursor:pointer; font-family: 'IBM Plex Sans', sans-serif; margin-left: 0.5rem !important; padding-top: 0.25rem !important; padding-bottom: 0.25rem !important;
- }
- #share-btn * {
- all: unset;
- }
- .gr-form{
- flex: 1 1 50%; border-top-right-radius: 0; border-bottom-right-radius: 0;
- }
- #prompt-container{
- gap: 0;
- }
-"""
-
-block = gr.Blocks(css=css)
-
-with block:
- gr.HTML(
- f"""
-
-
-
-
- Paella Demo
-
-
-
- Paella is a novel text-to-image model that uses a compressed quantized latent space, based on a VQGAN, and a masked training objective to achieve fast generation in ~10 inference steps.
-
- This version builds on top of our initial paper, bringing Paella to a similar level as other state-of-the-art models, while preserving the compactness and clarity of the previous implementations. Please, refer to the resources below for details.
-
-
- """
- )
- with gr.Group():
- with gr.Box():
- with gr.Row(elem_id="prompt-container").style(mobile_collapse=False, equal_height=True):
- with gr.Column():
- text = gr.Textbox(
- label="Enter your prompt",
- show_label=False,
- max_lines=1,
- placeholder="an image of a shiba inu, donning a spacesuit and helmet, traversing the uncharted terrain of a distant, extraterrestrial world, as a symbol of the intrepid spirit of exploration and the unrelenting curiosity that drives humanity to push beyond the bounds of the known",
- elem_id="prompt-text-input",
- ).style(
- border=(True, False, True, True),
- rounded=(True, False, False, True),
- container=False,
- )
- negative = gr.Textbox(
- label="Enter your negative prompt",
- show_label=False,
- max_lines=1,
- placeholder="low quality, low resolution, bad image, blurry, blur",
- elem_id="negative-prompt-text-input",
- ).style(
- border=(True, False, True, True),
- rounded=(True, False, False, True),
- container=False,
- )
- btn = gr.Button("Generate image").style(
- margin=False,
- rounded=(False, True, True, False),
- full_width=False,
- )
-
- gallery = gr.Gallery(
- label="Generated images", show_label=False, elem_id="gallery"
- ).style(grid=[2], height="auto")
-
- with gr.Group():
- with gr.Accordion("Advanced settings", open=False):
- seed = gr.Slider(
- label="Seed",
- minimum=0,
- maximum=2147483647,
- step=1,
- randomize=True,
- )
-
- text.submit(infer, inputs=[text, negative, seed], outputs=gallery)
- btn.click(infer, inputs=[text, negative, seed], outputs=gallery)
-
- gr.HTML(
- """
-
-
-Despite how impressive being able to turn text into image is, beware to the fact that this model may output content that reinforces or exacerbates societal biases, as well as realistic faces, pornography and violence. The model was trained on 600 million images from the improved LAION-5B aesthetic dataset, which scraped non-curated image-text-pairs from the internet (the exception being the removal of illegal content) and is meant for research purposes.
-
-
- """
- )
-
-block.launch()
diff --git a/spaces/penscola/sale_predictions/README.md b/spaces/penscola/sale_predictions/README.md
deleted file mode 100644
index 3e2ad5e2b9cc378491a9946f73c9a5e71441a14c..0000000000000000000000000000000000000000
--- a/spaces/penscola/sale_predictions/README.md
+++ /dev/null
@@ -1,30 +0,0 @@
----
-title: Sale Predictions
-emoji: 🏢
-colorFrom: red
-colorTo: blue
-sdk: streamlit
-sdk_version: 1.21.0
-app_file: app.py
-pinned: false
-license: mit
----
-
-## Background:
-Whether we wish to predict the trend in financial markets or electricity consumption, time is an important factor that must now be considered in our models. For example, it would be interesting to forecast at what hour during the day is there going to be a peak consumption in electricity, such as to adjust the price or the production of electricity.
-
-## The Process
-The procedure begins with exporting the essential items from the notebook, followed by correctly designing an interface, importing the necessary objects for modeling, and then writing the code to process inputs. The procedure can be summarized as follows:
-- Import machine learning components into the app script.
-- Create an interface,
-- Create a function to handle inputs.
-- Values are passed through the interface.
-- Restore these values in the backend,
-- Apply the required processing,
-- To produce predictions, submit the processed values to the ML model.
-- Process the acquired predictions and present them on the interface.
-Created by: Felix Kiprotich
-https://www.linkedin.com/in/felix-kiprotich-a2ba1a1a4/
-
-
-Check out the configuration reference at https://huggingface.co/docs/hub/spaces-config-reference
diff --git a/spaces/peteralexandercharles/wav2vec2-uk-demo/README.md b/spaces/peteralexandercharles/wav2vec2-uk-demo/README.md
deleted file mode 100644
index 5d450a00a26b58114bfb9fc78bdca09671c192b9..0000000000000000000000000000000000000000
--- a/spaces/peteralexandercharles/wav2vec2-uk-demo/README.md
+++ /dev/null
@@ -1,185 +0,0 @@
----
-title: Wav2vec2 Ukrainian with Timestamps
-emoji: 🇺🇦
-colorFrom: blue
-colorTo: yellow
-sdk: gradio
-app_file: app.py
-pinned: false
-duplicated_from: Yehor/wav2vec2-uk-demo
----
-
-
-# Demo of Ukrainian wav2vec2 model
-
-- The base model is hosted here: https://huggingface.co/Yehor/wav2vec2-xls-r-1b-uk-with-lm
-- The model with better News LM: https://huggingface.co/Yehor/wav2vec2-xls-r-1b-uk-with-news-lm
-
-Follow our community in Telegram: https://t.me/speech_recognition_uk
-
----
-
-Create a virtualenv:
-
-```bash
-pipenv install
-pipenv shell
-```
-
-Install deps:
-
-```bash
-pip install https://github.com/huggingface/transformers/archive/refs/tags/v4.16.2.zip
-pip install https://github.com/kpu/kenlm/archive/master.zip
-
-pip install torch==1.9.1 torchaudio==0.9.1 pyctcdecode==0.3.0
-```
-
-Run inference:
-
-```bash
-python inference.py --model_id Yehor/wav2vec2-xls-r-1b-uk-with-lm --path_files short_1.wav
-
-# with chunking
-python inference.py --model_id Yehor/wav2vec2-xls-r-1b-uk-with-lm --path_files short_1.wav --chunk_length_s 10 --stride_length_s_l 4 --stride_length_s_r 2
-python inference.py --model_id Yehor/wav2vec2-xls-r-1b-uk-with-lm --path_files long_1.wav --chunk_length_s 10 --stride_length_s_l 4 --stride_length_s_r 2
-
-# with chunking on GPU
-python inference_gpu.py --model_id Yehor/wav2vec2-xls-r-1b-uk-with-lm --path_files short_1.wav --chunk_length_s 10 --stride_length_s_l 4 --stride_length_s_r 2
-python inference_gpu.py --model_id Yehor/wav2vec2-xls-r-1b-uk-with-lm --path_files long_1.wav --chunk_length_s 10 --stride_length_s_l 4 --stride_length_s_r 2
-
-python inference.py --model_id Yehor/wav2vec2-xls-r-1b-uk-with-news-lm --path_files mer_lviv_interview.wav --chunk_length_s 10 --stride_length_s_l 4 --stride_length_s_r 2
-python inference_gpu.py --model_id Yehor/wav2vec2-xls-r-1b-uk-with-news-lm --path_files mer_lviv_interview.wav --chunk_length_s 10 --stride_length_s_l 4 --stride_length_s_r 2
-
-python inference.py --model_id Yehor/wav2vec2-xls-r-1b-uk-with-lm --path_files tsn.wav,tsn_2.wav --chunk_length_s 10 --stride_length_s_l 4 --stride_length_s_r 2
-```
-
-NOTE: Do the inference process for long files with chunking.
-
----
-
-short_1.wav:
-
-```
-пана сполучені штати над важливий стратегічний партнер однак є різницяштати мають спеціальний закон який передбачає якщо китай напади на тайвань американський військові мають його захищати у гри
-```
-
-short_1.wav (with better News LM):
-
-```
-аня сполучені штати над важливий стратегічний партнер однак є різниця штати мають спеціальний закон який передбачає якщо китай нападе на тайвань американський військові мають його захищати угери
-```
-
-long_1.wav:
-
-```
-серце чи дивовижни порятунок мільйони людей фактично в прямому ефірі вже три доби спостерігають за спробамиамероканських рятувальникив дісттисколодя за пятирічне хлопя досі не зрозуміло чи вдастядістати його з тридцяти метрового провал живим про надзвичайно складну операцію що триває в цю мить я на есарчуккулояз який провалився пятирічнийраян ледь помітна діра в землі менше тридцяти сантиметріву діаметрі але в глиб вона тягнеться на тридцять два метро батьки шукали сина кілька один перед тим як зрозуміле він під землею коли він зник я молилися богупросила аби алагзбиріг мосина і його дістали з колодязь живим господихай йому та менше болить в тій ділі я так сподіваючиь що у рятувальники все вийде його неможливо витягти просто так розуміють рятувальники занадто вуськоа розширяти діру не можна вона просто завалитья тому вони три до бою розкопують амундалік і поки працює техніки
-```
-
-long_1.wav (with News LM):
-
-```
-серце чи дивовижних порятунок мільйони людей фактично в прямому ефірі вже три доби спостерігають за спробами мероканських рятувальників дісттисколодя за пятирічне хлопя досі незрозуміло чи вдастся дістати його з тридцятиметрового провалля живим про надзвичайно складну операцію що триває в цю мить я не слісарчукодязв який провалився пятирічний раян ледь помітна діра в землі менше тридцяти сантиметрів у діаметрі але в глиб вона тягнеться на тридцять два метри батьки шукали сина кілька годин перед тим як зрозуміли він під землею а коли він зник я молилася богу просила аби алагбирігмо сина і його дістали сколотізяживим господи хай йому там менше болить в тій дірі і так сподіваючись що у рятувальники все вийде його неможливо витягти просто так розуміють рятувальників занадто вузько а розширяти діру не можна вона просто завалиться тому вони три добою розкопують амну далікі поки працює технік
-```
-
-long_1.wav (with better News LM):
-
-```
-серце чи дивовижний порятунок мільйони людей фактично в прямому ефірі вже три доби спостерігають за спробами мароканських рятувальників дісттисколодя за пятирічне хлопя досі незрозуміло чи вдастся дістати його з тридцятиметрового провалля живим про надзвичайно складну операцію що триває в цю мить я не слесарчукодязв який провалився пятирічний раян ледь помітна діра в землі менше тридцяти сантиметрів у діаметрі але в глиб вона тягнеться на тридцять два метри батьки шукали сина кілька годин перед тим як зрозуміли він під землею а коли він зник я молилася богу просила аби алаксбирігмо сина і його дістали сководізяживим господи хай йому там менше болить в тій тіріятак сподіваючись що у рятувальники все вийде його неможливо витягти просто так розуміють рятувальників занадто вузько а розширяти діру не можна вона просто завалиться тому вони три добою розкопують амнокдалік і поки працює технік
-```
-
-tsn.wav (with better News LM):
-
-```
-інформаційний вечір на один плюс один в студії та сем лідія таран а платонова королева з чого починалося і чим ще зовсім не закінчиться правління єлизавети другої сімдесят років на троні окмовівіполовні рішення щодо спадкоємців корони більше зараз усе
-```
-
-tsn_2.wav (with better News LM):
-
-```
-до осло зіли під час стрілянини на південмаші в жану влучили три кулі одна з них розірвала кишківник та хребет животі катастрофу розуміти три літри крові яку неможливо повернути тому що вона забруднена кишківник кинувши доз розірваний освітунавіть не котичекапо ужилася дуже активна проти дістала кулі просто на варті контрактниця відповідала за оповіщення частини про надзвичайні ситуації він був в двох кроках від вельми мовчки розстріляли що на посту була радіостанція і я запраз та могла доповісти частину військові ну я не змогла доповісти зробив постріли я вже не змогла підвестися стрілянина на південмаші сталася вночі двадцять сьомого січня пятеро людей загинули серед них одна цивільна ще пятро поранені підозрюваний двадцятирічний рокових артемірявчух у сізо спочатку провину визнавав тепер і знову м адвокатами від показів відмовився ані конфліктних ситуації ані бо чого підозрілого жанна каже до стрілянини із підозрюваним навіть не розмовляла а тільки за памятала і оце а так я навіть його імені й фамілії не знав я дуже рідко заступала ці кару там де срочного служба я не можу знати чому він так зробив навпроти жани реанімації контрактник ігор самененко під час стрлянини відпочивав за графіком повороже забігаю тикрчишотамячу ксюш сумною наше
-```
-
-mer_lviv_interview.wav:
-
-```
-ми отримали нового керівника територіальної оборони області а це є фахова людина і з дурне міста ми переформотуваи наш управлінні звичайних ситуаії сьогодні воно має функцію назвичйнх ситуацівільного захист територіальної оборони і в нас достатньо якісна співпрая якісна координації тому що заначаміста допомогти державному фактично кірунку щоб він якісно за працював стосовно ремонти стосовно обладнання і стосовно рекрутенгу людей які зараз голошулися що би бути по контрактах в територіальної обороні на території міста любова на стврюються двбатаьони і наразі все йде чітко за тимланам які визначено державою натомість місто взяла на себе додатково функцію ми очали великий навчальний процес для працівників комунальних підприємсособливо мов для про стратегічне підприємства працівники виконавчий хоронні влади банально відновити навики володіння зброю в наших працівників люсважливи чиникце уміння надавати першу до медичну допомогу і за така велика хвиля яка після закінчення карантивно де включати і старшокласників ми на при великий жаль багато в остнніх років таке враження що перебували лютриному сні розуміючи що росія поруч і за ви буде центр можливо агресії нам треба вишколювати себе і бути готовими до того щоб захищати рідну державу якщо кожен українець буде добре володіти навиками стрилецької зброї буде вміти надавати мдичну допомогу в бимене це потужна сила яку неможливо здолати тому в координації з державу і рухаємося вперед ще таке питання оту нас багато кажуть що поруч і бригада ми сил тертральо оборони можуть стврюватися і формуватися доровольчі обєднання чи у вслвові це передбачено і як ваше ставлення до такого віце ми працюємо в чуткійкоординації з державними інституціями і на сьогоднішній день я не бачу потреби в інших формування х тому що принципі має бути жорстка вертикально має бути один центр правління сла обогощо є новий командувач територіальної оборони рамках держави керівник штабоновий принципі пан у то який в нас у чули територіальну орону області на це дуже фахова компетентна людина з ним просто рємно сів працювати в не розуміє все співслов законом передбачена можливість прикидання в разі такої нагальної потреби перекидання бригада сил територіальної оборони в зонобоєвихді вічі області яке ваше ставленндоцього і можливо вам відомо яке можливо ставлення у самих сів територіальної оборони віці на сьогоднішній день ми розуміємо що він форційному плані треба зробити титанічниобєм праці людям треба пояснити що ти не можеш сховатисяу власному помешкані коли прийде ворог ворога треба не пустити на території нашої країни і я думаю що всі громадяни повинні мати готовнізахи щати свої рідну крїну на сьогоднішній день йде нормальний процес люди записується дуже багато відомелюдей записуся в територіальну оборону я вважаю це правильно тому що треба мати таки висів а стосовно всіх інших речей ще раз наголошую коли мова йде про безпеку про оборону держави має бути субординація і є чітка державна вертикальтреба захищати будемо сі разом захищати нема наторадиа ще одне питання багато хто впеонийщо законіне достатньо влади про писано для місцеве адміністрації влдимається на увазі повноважень і можливості при формуванні розгортанні сил тоеитрально оборони ви на практиці зіткнулися вже цим і як ви оцінюють ся це ну по перше добре що закон є звичайно що од жоден закон не є та обтальний чи ідеальний він мав би процесі реально життя за знати певних крягувань у наприклад керівником териріальной оборони в часі на звичайної ситуації є голова обласної адміністрації так а по місто любово наприклад керівником є керівник районної адміністрації це є не великий офісі є декілька працівників і вони мали би стояти над громади міста льоа я не думаю що це буде мати достатню якісний ефект тому що принцип є крівникобласті я керівник мста але ці речі напевно коли писали закон не до кінця прраховували але ми маємо час щоб це поправити але на сьогодні ми чітко викониозакни який є інших варіантів не може бути пане андріюбуквально остання запитнняваша бригада тлвівськану два батальйон як викрити як вони зараз озброєні повністю чи ніч це тільки стрилецьке збрїчице й більш важке озброєнн і як в ставитиь до того щоб міськдністрація мала можливістькажімо щось купувати для забзпечення саме си тртріальоорон у на приклад ті ж самі виспілотники для розвідки або засоби звязку а або ось щось таке ну що ви розуміли ми достатньо багато помагаємо всім нашим військовим частинам батальоам це робили вчорастобупі татом у кожного року якщо говорити про сили територіальну оборони області ми допоможе мо усім чим буде потрібно мова про гроші не йде ми не профінансуємо ремонту чи іншої вулиці а нашим хлопцям до поможемо і це має робити кона громада і кожен лідер гмади в нашій країні
-```
-
-mer_lviv_interview.wav (with better News LM):
-
-```
-ми отримали нового керівника територіальної оборони області а це є фахова людина і з турне міста ми переформатували наше управління звичайних ситуаії сьогодні воно має функцію надзвичйних ситуацї цівільного захист територіальної оборони і в нас достатньо якісна співпраця якісна координація тому що задача міста допомогти державному фактично керунку щоб він якісно запрацював стосовно ремонті стосовно обладнання і стосовно рекрутингу людей які зараз зголошувалися щоби бути по контрактах в територіальній обороні на території міста любова на стврюються батальони і наразі все йде чітко за тим ланом які визначеної державою натомість місто взяло на себе додаткову функцію ми очали великий навчальний процес для працівників комунальних підприємстособливо мов для про стратегічні підприємства працівники виконавчий хорані влади банально відновити навики володіння зброєю в наших працівників плюс важливий чинник це уміння надавати першу домедичну допомогу і за така велика хвиля яка після закінчення карантивно буде включати і старшокласників ми напривеликий жаль багато в останніх років таке враження що перебували літориномусні розуміючи що росія поруч і за вибуде центр можливо агресії нам треба вишколювати себе і бути готовими до того щоб захищати рідну державу якщо кожен українець буде добре володіти навиками стрілецької зброї буде вміти надавати м дичну допомогу в имени це потужна сила яку неможливо здолати тому в координації з державу і рухаємося вперед ще таке питання от у нас багато кажуть що поруч із бригадами сил тертральооборони можуть стврюватися і формуватися добровольчі обєднання чи уволвові це передбачено як ваше ставлення до такого віце ми працюємо в чіткийкординації з державними інституціями і на сьогоднішній день я не бачу потреби в інших формуваннях тому що в принципі має бути жорстка вертикаль має бути один центр управління сла обо що є новий командувач територіальної оборони в рамках держави керівник шабанови принципі пану то які в нас учули територіальну орон області на це дуже фахова компетентна людина з ним просто приємно співпрацювати не розуміє все співслова законом передбачена можливість перекидання в разі такої нагальної потреби перекидання бригад сил територіальної оборони в зону бойових дій віншіобласті яке ваше ставленн до цього і можливо вам відомо яке можливо ставлення рсу самих бійців територіальної оборони від це на сьогоднішній день ми розуміємо що вінформаційному плані треба зробити титанічний обєм праці людям треба пояснити що ти не можеш сховатися у власному помешканні коли прийде ворог ворога треба не пустити на територію нашої країни і я думаю що всі громадяни повинні мати готовні захищати свою рідну крїну на сьогоднішній день йде нормальний процес люди записується дуже багато відоме людей записується в територіальну оборону я вважаю це правильно тому що треба мати такі вишкіл а стосовно всіх інших речей ще раз наголошую коли мова йде про безпеку про оборону держави має бути субординація і є чітка державна вертикаль треба захищати будемо сі разом захищати нема на то ради а ще одне питання багато хто впевнений що в законі недостатньо влади прописано для місцевих д міністрації влди мається на увазі повноважень і можлвостпри формуванні розгортанні сил ториторіально оборони ви на практиці зіткнулися вже цим і як ви оцінюютьсяцену поперше добре що закон є звичайно що жододенззаон не є та обтальний чи ідеальний він мав би процесі реально життя зазнати певних коригувань ну наприклад керівником териріальной оборони в часі на звичайної ситуації є голова обласної адміністрації так а по місту любовонаприклад керівником є керівник районної адміністрації це є невеликий офіс нехедекілька працівників і вони мали би стояти над громадою міста львова я не думаю що це буде мати достатню якісний ефект тому що принцип є керівникобласті керівни мста але ці речі напевно коли писали закон не до кінця раховували але ми маємо час щоб це поправити але на сьогодні ми чітко викониозакни який є іншхварянтів не може буде пане андрію буквально остання запитння ваша бригада львівська ну два батальйони як викрита як вони зараз озброєні повністю чи ніч це тільки стрілецьке збря чи це й більш важке озброєння і як в ставитись до того щоб міськдістрація мала можливістькажімо щось купувати для забзпечення саме си тртіальоорони ну наприклад ті ж самі беспілотники для розвідки або засоби звязку а або ось щось таке ну щоб ви розуміли ми достатньо багато помагаємо всім нашим військовим частинам батальонам це робили читерастобу питатиму кожного року якщо говорити про сили територіально оборони області ми допоможемо усім чим буде потрібно мова про гроші не йде ми не профінансуємо ремонтуючи іншої вулиці а нашим хлопцям допоможемо і це має робити кона громада і кожен лідер гомади в нашій країні
-```
-
-### Inference of `mer_lviv_interview.wav` (time is 06:38)
-
-#### CPU
-
-- Memory peaks to 60GB
-- Memory peaks to 65GB (on News LM)
-
-Inference duration:
-
-```
-real 7m39.461s
-user 59m19.065s
-sys 24m1.254s
-```
-
-Inference duration (on News LM):
-
-```
-real 12m36.888s
-user 63m19.396s
-sys 24m24.823s
-```
-
-Duration tracked with loading the LM.
-
-## Using timestamps
-
-The `inference_timestamps.py` script can be used to do inference with timestamps for chars and words.
-
-### `output_char_offsets=True`
-
-```
-Wav2Vec2CTCTokenizerOutput(text='паня сполучені штати надважливий стратегічний партнер однак є різниця штати мають спеціальни закон який передбачає якщо китай нападе на тайвань американський військові мають його захищати евуйвгере', char_offsets=[{'char': 'п', 'start_offset': 0, 'end_offset': 1}, {'char': 'а', 'start_offset': 1, 'end_offset': 2}, {'char': 'н', 'start_offset': 9, 'end_offset': 10}, {'char': 'я', 'start_offset': 11, 'end_offset': 12}, {'char': ' ', 'start_offset': 14, 'end_offset': 15}, {'char': 'с', 'start_offset': 16, 'end_offset': 17}, {'char': 'п', 'start_offset': 19, 'end_offset': 20}, {'char': 'о', 'start_offset': 21, 'end_offset': 22}, {'char': 'л', 'start_offset': 23, 'end_offset': 24}, {'char': 'у', 'start_offset': 25, 'end_offset': 26}, {'char': 'ч', 'start_offset': 30, 'end_offset': 31}, {'char': 'е', 'start_offset': 32, 'end_offset': 33}, {'char': 'н', 'start_offset': 37, 'end_offset': 38}, {'char': 'і', 'start_offset': 38, 'end_offset': 39}, {'char': ' ', 'start_offset': 40, 'end_offset': 42}, {'char': 'ш', 'start_offset': 43, 'end_offset': 44}, {'char': 'т', 'start_offset': 46, 'end_offset': 47}, {'char': 'а', 'start_offset': 48, 'end_offset': 49}, {'char': 'т', 'start_offset': 57, 'end_offset': 58}, {'char': 'и', 'start_offset': 58, 'end_offset': 59}, {'char': ' ', 'start_offset': 76, 'end_offset': 79}, {'char': 'н', 'start_offset': 85, 'end_offset': 86}, {'char': 'а', 'start_offset': 87, 'end_offset': 88}, {'char': 'д', 'start_offset': 93, 'end_offset': 94}, {'char': 'в', 'start_offset': 97, 'end_offset': 98}, {'char': 'а', 'start_offset': 99, 'end_offset': 100}, {'char': 'ж', 'start_offset': 105, 'end_offset': 106}, {'char': 'л', 'start_offset': 113, 'end_offset': 114}, {'char': 'и', 'start_offset': 114, 'end_offset': 115}, {'char': 'в', 'start_offset': 121, 'end_offset': 122}, {'char': 'и', 'start_offset': 123, 'end_offset': 124}, {'char': 'й', 'start_offset': 125, 'end_offset': 126}, {'char': ' ', 'start_offset': 127, 'end_offset': 129}, {'char': 'с', 'start_offset': 130, 'end_offset': 131}, {'char': 'т', 'start_offset': 134, 'end_offset': 136}, {'char': 'р', 'start_offset': 138, 'end_offset': 139}, {'char': 'а', 'start_offset': 139, 'end_offset': 140}, {'char': 'т', 'start_offset': 145, 'end_offset': 146}, {'char': 'е', 'start_offset': 146, 'end_offset': 147}, {'char': 'г', 'start_offset': 152, 'end_offset': 153}, {'char': 'і', 'start_offset': 153, 'end_offset': 154}, {'char': 'ч', 'start_offset': 160, 'end_offset': 161}, {'char': 'н', 'start_offset': 167, 'end_offset': 168}, {'char': 'и', 'start_offset': 168, 'end_offset': 169}, {'char': 'й', 'start_offset': 170, 'end_offset': 171}, {'char': ' ', 'start_offset': 171, 'end_offset': 173}, {'char': 'п', 'start_offset': 174, 'end_offset': 175}, {'char': 'а', 'start_offset': 176, 'end_offset': 177}, {'char': 'р', 'start_offset': 179, 'end_offset': 180}, {'char': 'т', 'start_offset': 183, 'end_offset': 184}, {'char': 'н', 'start_offset': 188, 'end_offset': 189}, {'char': 'е', 'start_offset': 189, 'end_offset': 190}, {'char': 'р', 'start_offset': 193, 'end_offset': 194}, {'char': ' ', 'start_offset': 201, 'end_offset': 203}, {'char': 'о', 'start_offset': 204, 'end_offset': 205}, {'char': 'д', 'start_offset': 208, 'end_offset': 209}, {'char': 'н', 'start_offset': 214, 'end_offset': 216}, {'char': 'а', 'start_offset': 216, 'end_offset': 217}, {'char': 'к', 'start_offset': 224, 'end_offset': 225}, {'char': ' ', 'start_offset': 227, 'end_offset': 229}, {'char': 'є', 'start_offset': 233, 'end_offset': 234}, {'char': ' ', 'start_offset': 237, 'end_offset': 239}, {'char': 'р', 'start_offset': 240, 'end_offset': 241}, {'char': 'і', 'start_offset': 241, 'end_offset': 242}, {'char': 'з', 'start_offset': 247, 'end_offset': 248}, {'char': 'н', 'start_offset': 253, 'end_offset': 254}, {'char': 'и', 'start_offset': 254, 'end_offset': 255}, {'char': 'ц', 'start_offset': 261, 'end_offset': 262}, {'char': 'я', 'start_offset': 262, 'end_offset': 263}, {'char': ' ', 'start_offset': 281, 'end_offset': 283}, {'char': 'ш', 'start_offset': 283, 'end_offset': 284}, {'char': 'т', 'start_offset': 286, 'end_offset': 287}, {'char': 'а', 'start_offset': 288, 'end_offset': 289}, {'char': 'т', 'start_offset': 294, 'end_offset': 295}, {'char': 'и', 'start_offset': 296, 'end_offset': 297}, {'char': ' ', 'start_offset': 297, 'end_offset': 299}, {'char': 'м', 'start_offset': 300, 'end_offset': 301}, {'char': 'а', 'start_offset': 301, 'end_offset': 302}, {'char': 'ю', 'start_offset': 306, 'end_offset': 307}, {'char': 'т', 'start_offset': 308, 'end_offset': 309}, {'char': 'ь', 'start_offset': 309, 'end_offset': 311}, {'char': ' ', 'start_offset': 311, 'end_offset': 313}, {'char': 'с', 'start_offset': 313, 'end_offset': 314}, {'char': 'п', 'start_offset': 316, 'end_offset': 317}, {'char': 'е', 'start_offset': 318, 'end_offset': 319}, {'char': 'ц', 'start_offset': 324, 'end_offset': 325}, {'char': 'і', 'start_offset': 325, 'end_offset': 326}, {'char': 'а', 'start_offset': 328, 'end_offset': 329}, {'char': 'л', 'start_offset': 333, 'end_offset': 334}, {'char': 'ь', 'start_offset': 334, 'end_offset': 336}, {'char': 'н', 'start_offset': 339, 'end_offset': 340}, {'char': 'и', 'start_offset': 341, 'end_offset': 342}, {'char': ' ', 'start_offset': 345, 'end_offset': 348}, {'char': 'з', 'start_offset': 351, 'end_offset': 352}, {'char': 'а', 'start_offset': 354, 'end_offset': 355}, {'char': 'к', 'start_offset': 361, 'end_offset': 362}, {'char': 'о', 'start_offset': 365, 'end_offset': 366}, {'char': 'н', 'start_offset': 373, 'end_offset': 374}, {'char': ' ', 'start_offset': 382, 'end_offset': 384}, {'char': 'я', 'start_offset': 386, 'end_offset': 387}, {'char': 'к', 'start_offset': 390, 'end_offset': 391}, {'char': 'и', 'start_offset': 392, 'end_offset': 393}, {'char': 'й', 'start_offset': 394, 'end_offset': 395}, {'char': ' ', 'start_offset': 396, 'end_offset': 398}, {'char': 'п', 'start_offset': 399, 'end_offset': 401}, {'char': 'е', 'start_offset': 402, 'end_offset': 403}, {'char': 'р', 'start_offset': 406, 'end_offset': 407}, {'char': 'е', 'start_offset': 407, 'end_offset': 408}, {'char': 'д', 'start_offset': 411, 'end_offset': 412}, {'char': 'б', 'start_offset': 415, 'end_offset': 416}, {'char': 'а', 'start_offset': 416, 'end_offset': 417}, {'char': 'ч', 'start_offset': 424, 'end_offset': 425}, {'char': 'а', 'start_offset': 428, 'end_offset': 429}, {'char': 'є', 'start_offset': 437, 'end_offset': 438}, {'char': ' ', 'start_offset': 445, 'end_offset': 447}, {'char': 'я', 'start_offset': 448, 'end_offset': 449}, {'char': 'к', 'start_offset': 452, 'end_offset': 453}, {'char': 'щ', 'start_offset': 455, 'end_offset': 456}, {'char': 'о', 'start_offset': 457, 'end_offset': 458}, {'char': ' ', 'start_offset': 460, 'end_offset': 463}, {'char': 'к', 'start_offset': 463, 'end_offset': 464}, {'char': 'и', 'start_offset': 465, 'end_offset': 466}, {'char': 'т', 'start_offset': 470, 'end_offset': 471}, {'char': 'а', 'start_offset': 472, 'end_offset': 473}, {'char': 'й', 'start_offset': 478, 'end_offset': 480}, {'char': ' ', 'start_offset': 484, 'end_offset': 486}, {'char': 'н', 'start_offset': 487, 'end_offset': 488}, {'char': 'а', 'start_offset': 488, 'end_offset': 489}, {'char': 'п', 'start_offset': 493, 'end_offset': 494}, {'char': 'а', 'start_offset': 496, 'end_offset': 497}, {'char': 'д', 'start_offset': 502, 'end_offset': 503}, {'char': 'е', 'start_offset': 504, 'end_offset': 505}, {'char': ' ', 'start_offset': 509, 'end_offset': 511}, {'char': 'н', 'start_offset': 511, 'end_offset': 512}, {'char': 'а', 'start_offset': 513, 'end_offset': 514}, {'char': ' ', 'start_offset': 515, 'end_offset': 517}, {'char': 'т', 'start_offset': 518, 'end_offset': 519}, {'char': 'а', 'start_offset': 519, 'end_offset': 520}, {'char': 'й', 'start_offset': 524, 'end_offset': 525}, {'char': 'в', 'start_offset': 527, 'end_offset': 528}, {'char': 'а', 'start_offset': 529, 'end_offset': 530}, {'char': 'н', 'start_offset': 535, 'end_offset': 536}, {'char': 'ь', 'start_offset': 536, 'end_offset': 537}, {'char': ' ', 'start_offset': 552, 'end_offset': 555}, {'char': 'а', 'start_offset': 555, 'end_offset': 556}, {'char': 'м', 'start_offset': 561, 'end_offset': 562}, {'char': 'е', 'start_offset': 562, 'end_offset': 563}, {'char': 'р', 'start_offset': 566, 'end_offset': 567}, {'char': 'и', 'start_offset': 567, 'end_offset': 568}, {'char': 'к', 'start_offset': 572, 'end_offset': 573}, {'char': 'а', 'start_offset': 574, 'end_offset': 575}, {'char': 'н', 'start_offset': 579, 'end_offset': 580}, {'char': 'с', 'start_offset': 582, 'end_offset': 583}, {'char': 'ь', 'start_offset': 583, 'end_offset': 585}, {'char': 'к', 'start_offset': 586, 'end_offset': 587}, {'char': 'и', 'start_offset': 588, 'end_offset': 589}, {'char': 'й', 'start_offset': 589, 'end_offset': 590}, {'char': ' ', 'start_offset': 591, 'end_offset': 593}, {'char': 'в', 'start_offset': 594, 'end_offset': 595}, {'char': 'і', 'start_offset': 595, 'end_offset': 596}, {'char': 'й', 'start_offset': 600, 'end_offset': 601}, {'char': 'с', 'start_offset': 604, 'end_offset': 605}, {'char': 'ь', 'start_offset': 605, 'end_offset': 607}, {'char': 'к', 'start_offset': 609, 'end_offset': 611}, {'char': 'о', 'start_offset': 612, 'end_offset': 613}, {'char': 'в', 'start_offset': 620, 'end_offset': 621}, {'char': 'і', 'start_offset': 622, 'end_offset': 623}, {'char': ' ', 'start_offset': 637, 'end_offset': 639}, {'char': 'м', 'start_offset': 641, 'end_offset': 642}, {'char': 'а', 'start_offset': 643, 'end_offset': 644}, {'char': 'ю', 'start_offset': 651, 'end_offset': 652}, {'char': 'т', 'start_offset': 654, 'end_offset': 655}, {'char': 'ь', 'start_offset': 655, 'end_offset': 656}, {'char': ' ', 'start_offset': 657, 'end_offset': 659}, {'char': 'й', 'start_offset': 659, 'end_offset': 660}, {'char': 'о', 'start_offset': 660, 'end_offset': 662}, {'char': 'г', 'start_offset': 664, 'end_offset': 665}, {'char': 'о', 'start_offset': 666, 'end_offset': 667}, {'char': ' ', 'start_offset': 677, 'end_offset': 679}, {'char': 'з', 'start_offset': 681, 'end_offset': 682}, {'char': 'а', 'start_offset': 683, 'end_offset': 684}, {'char': 'х', 'start_offset': 686, 'end_offset': 687}, {'char': 'и', 'start_offset': 689, 'end_offset': 690}, {'char': 'щ', 'start_offset': 696, 'end_offset': 697}, {'char': 'а', 'start_offset': 698, 'end_offset': 699}, {'char': 'т', 'start_offset': 707, 'end_offset': 708}, {'char': 'и', 'start_offset': 709, 'end_offset': 710}, {'char': ' ', 'start_offset': 733, 'end_offset': 734}, {'char': 'е', 'start_offset': 740, 'end_offset': 741}, {'char': 'в', 'start_offset': 747, 'end_offset': 748}, {'char': 'у', 'start_offset': 748, 'end_offset': 749}, {'char': 'й', 'start_offset': 752, 'end_offset': 753}, {'char': 'в', 'start_offset': 754, 'end_offset': 755}, {'char': 'г', 'start_offset': 757, 'end_offset': 758}, {'char': 'е', 'start_offset': 759, 'end_offset': 760}, {'char': 'р', 'start_offset': 767, 'end_offset': 768}, {'char': 'е', 'start_offset': 768, 'end_offset': 769}], word_offsets=None)
-```
-
-### `output_word_offsets=True`
-
-```
-Wav2Vec2CTCTokenizerOutput(text='паня сполучені штати надважливий стратегічний партнер однак є різниця штати мають спеціальни закон який передбачає якщо китай нападе на тайвань американський військові мають його захищати евуйвгере', char_offsets=[{'char': 'п', 'start_offset': 0, 'end_offset': 1}, {'char': 'а', 'start_offset': 1, 'end_offset': 2}, {'char': 'н', 'start_offset': 9, 'end_offset': 10}, {'char': 'я', 'start_offset': 11, 'end_offset': 12}, {'char': ' ', 'start_offset': 14, 'end_offset': 15}, {'char': 'с', 'start_offset': 16, 'end_offset': 17}, {'char': 'п', 'start_offset': 19, 'end_offset': 20}, {'char': 'о', 'start_offset': 21, 'end_offset': 22}, {'char': 'л', 'start_offset': 23, 'end_offset': 24}, {'char': 'у', 'start_offset': 25, 'end_offset': 26}, {'char': 'ч', 'start_offset': 30, 'end_offset': 31}, {'char': 'е', 'start_offset': 32, 'end_offset': 33}, {'char': 'н', 'start_offset': 37, 'end_offset': 38}, {'char': 'і', 'start_offset': 38, 'end_offset': 39}, {'char': ' ', 'start_offset': 40, 'end_offset': 42}, {'char': 'ш', 'start_offset': 43, 'end_offset': 44}, {'char': 'т', 'start_offset': 46, 'end_offset': 47}, {'char': 'а', 'start_offset': 48, 'end_offset': 49}, {'char': 'т', 'start_offset': 57, 'end_offset': 58}, {'char': 'и', 'start_offset': 58, 'end_offset': 59}, {'char': ' ', 'start_offset': 76, 'end_offset': 79}, {'char': 'н', 'start_offset': 85, 'end_offset': 86}, {'char': 'а', 'start_offset': 87, 'end_offset': 88}, {'char': 'д', 'start_offset': 93, 'end_offset': 94}, {'char': 'в', 'start_offset': 97, 'end_offset': 98}, {'char': 'а', 'start_offset': 99, 'end_offset': 100}, {'char': 'ж', 'start_offset': 105, 'end_offset': 106}, {'char': 'л', 'start_offset': 113, 'end_offset': 114}, {'char': 'и', 'start_offset': 114, 'end_offset': 115}, {'char': 'в', 'start_offset': 121, 'end_offset': 122}, {'char': 'и', 'start_offset': 123, 'end_offset': 124}, {'char': 'й', 'start_offset': 125, 'end_offset': 126}, {'char': ' ', 'start_offset': 127, 'end_offset': 129}, {'char': 'с', 'start_offset': 130, 'end_offset': 131}, {'char': 'т', 'start_offset': 134, 'end_offset': 136}, {'char': 'р', 'start_offset': 138, 'end_offset': 139}, {'char': 'а', 'start_offset': 139, 'end_offset': 140}, {'char': 'т', 'start_offset': 145, 'end_offset': 146}, {'char': 'е', 'start_offset': 146, 'end_offset': 147}, {'char': 'г', 'start_offset': 152, 'end_offset': 153}, {'char': 'і', 'start_offset': 153, 'end_offset': 154}, {'char': 'ч', 'start_offset': 160, 'end_offset': 161}, {'char': 'н', 'start_offset': 167, 'end_offset': 168}, {'char': 'и', 'start_offset': 168, 'end_offset': 169}, {'char': 'й', 'start_offset': 170, 'end_offset': 171}, {'char': ' ', 'start_offset': 171, 'end_offset': 173}, {'char': 'п', 'start_offset': 174, 'end_offset': 175}, {'char': 'а', 'start_offset': 176, 'end_offset': 177}, {'char': 'р', 'start_offset': 179, 'end_offset': 180}, {'char': 'т', 'start_offset': 183, 'end_offset': 184}, {'char': 'н', 'start_offset': 188, 'end_offset': 189}, {'char': 'е', 'start_offset': 189, 'end_offset': 190}, {'char': 'р', 'start_offset': 193, 'end_offset': 194}, {'char': ' ', 'start_offset': 201, 'end_offset': 203}, {'char': 'о', 'start_offset': 204, 'end_offset': 205}, {'char': 'д', 'start_offset': 208, 'end_offset': 209}, {'char': 'н', 'start_offset': 214, 'end_offset': 216}, {'char': 'а', 'start_offset': 216, 'end_offset': 217}, {'char': 'к', 'start_offset': 224, 'end_offset': 225}, {'char': ' ', 'start_offset': 227, 'end_offset': 229}, {'char': 'є', 'start_offset': 233, 'end_offset': 234}, {'char': ' ', 'start_offset': 237, 'end_offset': 239}, {'char': 'р', 'start_offset': 240, 'end_offset': 241}, {'char': 'і', 'start_offset': 241, 'end_offset': 242}, {'char': 'з', 'start_offset': 247, 'end_offset': 248}, {'char': 'н', 'start_offset': 253, 'end_offset': 254}, {'char': 'и', 'start_offset': 254, 'end_offset': 255}, {'char': 'ц', 'start_offset': 261, 'end_offset': 262}, {'char': 'я', 'start_offset': 262, 'end_offset': 263}, {'char': ' ', 'start_offset': 281, 'end_offset': 283}, {'char': 'ш', 'start_offset': 283, 'end_offset': 284}, {'char': 'т', 'start_offset': 286, 'end_offset': 287}, {'char': 'а', 'start_offset': 288, 'end_offset': 289}, {'char': 'т', 'start_offset': 294, 'end_offset': 295}, {'char': 'и', 'start_offset': 296, 'end_offset': 297}, {'char': ' ', 'start_offset': 297, 'end_offset': 299}, {'char': 'м', 'start_offset': 300, 'end_offset': 301}, {'char': 'а', 'start_offset': 301, 'end_offset': 302}, {'char': 'ю', 'start_offset': 306, 'end_offset': 307}, {'char': 'т', 'start_offset': 308, 'end_offset': 309}, {'char': 'ь', 'start_offset': 309, 'end_offset': 311}, {'char': ' ', 'start_offset': 311, 'end_offset': 313}, {'char': 'с', 'start_offset': 313, 'end_offset': 314}, {'char': 'п', 'start_offset': 316, 'end_offset': 317}, {'char': 'е', 'start_offset': 318, 'end_offset': 319}, {'char': 'ц', 'start_offset': 324, 'end_offset': 325}, {'char': 'і', 'start_offset': 325, 'end_offset': 326}, {'char': 'а', 'start_offset': 328, 'end_offset': 329}, {'char': 'л', 'start_offset': 333, 'end_offset': 334}, {'char': 'ь', 'start_offset': 334, 'end_offset': 336}, {'char': 'н', 'start_offset': 339, 'end_offset': 340}, {'char': 'и', 'start_offset': 341, 'end_offset': 342}, {'char': ' ', 'start_offset': 345, 'end_offset': 348}, {'char': 'з', 'start_offset': 351, 'end_offset': 352}, {'char': 'а', 'start_offset': 354, 'end_offset': 355}, {'char': 'к', 'start_offset': 361, 'end_offset': 362}, {'char': 'о', 'start_offset': 365, 'end_offset': 366}, {'char': 'н', 'start_offset': 373, 'end_offset': 374}, {'char': ' ', 'start_offset': 382, 'end_offset': 384}, {'char': 'я', 'start_offset': 386, 'end_offset': 387}, {'char': 'к', 'start_offset': 390, 'end_offset': 391}, {'char': 'и', 'start_offset': 392, 'end_offset': 393}, {'char': 'й', 'start_offset': 394, 'end_offset': 395}, {'char': ' ', 'start_offset': 396, 'end_offset': 398}, {'char': 'п', 'start_offset': 399, 'end_offset': 401}, {'char': 'е', 'start_offset': 402, 'end_offset': 403}, {'char': 'р', 'start_offset': 406, 'end_offset': 407}, {'char': 'е', 'start_offset': 407, 'end_offset': 408}, {'char': 'д', 'start_offset': 411, 'end_offset': 412}, {'char': 'б', 'start_offset': 415, 'end_offset': 416}, {'char': 'а', 'start_offset': 416, 'end_offset': 417}, {'char': 'ч', 'start_offset': 424, 'end_offset': 425}, {'char': 'а', 'start_offset': 428, 'end_offset': 429}, {'char': 'є', 'start_offset': 437, 'end_offset': 438}, {'char': ' ', 'start_offset': 445, 'end_offset': 447}, {'char': 'я', 'start_offset': 448, 'end_offset': 449}, {'char': 'к', 'start_offset': 452, 'end_offset': 453}, {'char': 'щ', 'start_offset': 455, 'end_offset': 456}, {'char': 'о', 'start_offset': 457, 'end_offset': 458}, {'char': ' ', 'start_offset': 460, 'end_offset': 463}, {'char': 'к', 'start_offset': 463, 'end_offset': 464}, {'char': 'и', 'start_offset': 465, 'end_offset': 466}, {'char': 'т', 'start_offset': 470, 'end_offset': 471}, {'char': 'а', 'start_offset': 472, 'end_offset': 473}, {'char': 'й', 'start_offset': 478, 'end_offset': 480}, {'char': ' ', 'start_offset': 484, 'end_offset': 486}, {'char': 'н', 'start_offset': 487, 'end_offset': 488}, {'char': 'а', 'start_offset': 488, 'end_offset': 489}, {'char': 'п', 'start_offset': 493, 'end_offset': 494}, {'char': 'а', 'start_offset': 496, 'end_offset': 497}, {'char': 'д', 'start_offset': 502, 'end_offset': 503}, {'char': 'е', 'start_offset': 504, 'end_offset': 505}, {'char': ' ', 'start_offset': 509, 'end_offset': 511}, {'char': 'н', 'start_offset': 511, 'end_offset': 512}, {'char': 'а', 'start_offset': 513, 'end_offset': 514}, {'char': ' ', 'start_offset': 515, 'end_offset': 517}, {'char': 'т', 'start_offset': 518, 'end_offset': 519}, {'char': 'а', 'start_offset': 519, 'end_offset': 520}, {'char': 'й', 'start_offset': 524, 'end_offset': 525}, {'char': 'в', 'start_offset': 527, 'end_offset': 528}, {'char': 'а', 'start_offset': 529, 'end_offset': 530}, {'char': 'н', 'start_offset': 535, 'end_offset': 536}, {'char': 'ь', 'start_offset': 536, 'end_offset': 537}, {'char': ' ', 'start_offset': 552, 'end_offset': 555}, {'char': 'а', 'start_offset': 555, 'end_offset': 556}, {'char': 'м', 'start_offset': 561, 'end_offset': 562}, {'char': 'е', 'start_offset': 562, 'end_offset': 563}, {'char': 'р', 'start_offset': 566, 'end_offset': 567}, {'char': 'и', 'start_offset': 567, 'end_offset': 568}, {'char': 'к', 'start_offset': 572, 'end_offset': 573}, {'char': 'а', 'start_offset': 574, 'end_offset': 575}, {'char': 'н', 'start_offset': 579, 'end_offset': 580}, {'char': 'с', 'start_offset': 582, 'end_offset': 583}, {'char': 'ь', 'start_offset': 583, 'end_offset': 585}, {'char': 'к', 'start_offset': 586, 'end_offset': 587}, {'char': 'и', 'start_offset': 588, 'end_offset': 589}, {'char': 'й', 'start_offset': 589, 'end_offset': 590}, {'char': ' ', 'start_offset': 591, 'end_offset': 593}, {'char': 'в', 'start_offset': 594, 'end_offset': 595}, {'char': 'і', 'start_offset': 595, 'end_offset': 596}, {'char': 'й', 'start_offset': 600, 'end_offset': 601}, {'char': 'с', 'start_offset': 604, 'end_offset': 605}, {'char': 'ь', 'start_offset': 605, 'end_offset': 607}, {'char': 'к', 'start_offset': 609, 'end_offset': 611}, {'char': 'о', 'start_offset': 612, 'end_offset': 613}, {'char': 'в', 'start_offset': 620, 'end_offset': 621}, {'char': 'і', 'start_offset': 622, 'end_offset': 623}, {'char': ' ', 'start_offset': 637, 'end_offset': 639}, {'char': 'м', 'start_offset': 641, 'end_offset': 642}, {'char': 'а', 'start_offset': 643, 'end_offset': 644}, {'char': 'ю', 'start_offset': 651, 'end_offset': 652}, {'char': 'т', 'start_offset': 654, 'end_offset': 655}, {'char': 'ь', 'start_offset': 655, 'end_offset': 656}, {'char': ' ', 'start_offset': 657, 'end_offset': 659}, {'char': 'й', 'start_offset': 659, 'end_offset': 660}, {'char': 'о', 'start_offset': 660, 'end_offset': 662}, {'char': 'г', 'start_offset': 664, 'end_offset': 665}, {'char': 'о', 'start_offset': 666, 'end_offset': 667}, {'char': ' ', 'start_offset': 677, 'end_offset': 679}, {'char': 'з', 'start_offset': 681, 'end_offset': 682}, {'char': 'а', 'start_offset': 683, 'end_offset': 684}, {'char': 'х', 'start_offset': 686, 'end_offset': 687}, {'char': 'и', 'start_offset': 689, 'end_offset': 690}, {'char': 'щ', 'start_offset': 696, 'end_offset': 697}, {'char': 'а', 'start_offset': 698, 'end_offset': 699}, {'char': 'т', 'start_offset': 707, 'end_offset': 708}, {'char': 'и', 'start_offset': 709, 'end_offset': 710}, {'char': ' ', 'start_offset': 733, 'end_offset': 734}, {'char': 'е', 'start_offset': 740, 'end_offset': 741}, {'char': 'в', 'start_offset': 747, 'end_offset': 748}, {'char': 'у', 'start_offset': 748, 'end_offset': 749}, {'char': 'й', 'start_offset': 752, 'end_offset': 753}, {'char': 'в', 'start_offset': 754, 'end_offset': 755}, {'char': 'г', 'start_offset': 757, 'end_offset': 758}, {'char': 'е', 'start_offset': 759, 'end_offset': 760}, {'char': 'р', 'start_offset': 767, 'end_offset': 768}, {'char': 'е', 'start_offset': 768, 'end_offset': 769}], word_offsets=[{'word': 'паня', 'start_offset': 0, 'end_offset': 12}, {'word': 'сполучені', 'start_offset': 16, 'end_offset': 39}, {'word': 'штати', 'start_offset': 43, 'end_offset': 59}, {'word': 'надважливий', 'start_offset': 85, 'end_offset': 126}, {'word': 'стратегічний', 'start_offset': 130, 'end_offset': 171}, {'word': 'партнер', 'start_offset': 174, 'end_offset': 194}, {'word': 'однак', 'start_offset': 204, 'end_offset': 225}, {'word': 'є', 'start_offset': 233, 'end_offset': 234}, {'word': 'різниця', 'start_offset': 240, 'end_offset': 263}, {'word': 'штати', 'start_offset': 283, 'end_offset': 297}, {'word': 'мають', 'start_offset': 300, 'end_offset': 311}, {'word': 'спеціальни', 'start_offset': 313, 'end_offset': 342}, {'word': 'закон', 'start_offset': 351, 'end_offset': 374}, {'word': 'який', 'start_offset': 386, 'end_offset': 395}, {'word': 'передбачає', 'start_offset': 399, 'end_offset': 438}, {'word': 'якщо', 'start_offset': 448, 'end_offset': 458}, {'word': 'китай', 'start_offset': 463, 'end_offset': 480}, {'word': 'нападе', 'start_offset': 487, 'end_offset': 505}, {'word': 'на', 'start_offset': 511, 'end_offset': 514}, {'word': 'тайвань', 'start_offset': 518, 'end_offset': 537}, {'word': 'американський', 'start_offset': 555, 'end_offset': 590}, {'word': 'військові', 'start_offset': 594, 'end_offset': 623}, {'word': 'мають', 'start_offset': 641, 'end_offset': 656}, {'word': 'його', 'start_offset': 659, 'end_offset': 667}, {'word': 'захищати', 'start_offset': 681, 'end_offset': 710}, {'word': 'евуйвгере', 'start_offset': 740, 'end_offset': 769}])
-```
-
-### Split by seconds
-
-```
-0.0 - 0.24: паня
-0.32 - 0.78: сполучені
-0.86 - 1.18: штати
-1.7 - 2.52: надважливий
-2.6 - 3.42: стратегічний
-3.48 - 3.88: партнер
-4.08 - 4.5: однак
-4.66 - 4.68: є
-4.8 - 5.26: різниця
-5.66 - 5.94: штати
-6.0 - 6.22: мають
-6.26 - 6.84: спеціальни
-7.02 - 7.48: закон
-7.72 - 7.9: який
-7.98 - 8.76: передбачає
-8.96 - 9.16: якщо
-9.26 - 9.6: китай
-9.74 - 10.1: нападе
-10.22 - 10.28: на
-10.36 - 10.74: тайвань
-11.1 - 11.8: американський
-11.88 - 12.46: військові
-12.82 - 13.12: мають
-13.18 - 13.34: його
-13.62 - 14.2: захищати
-14.8 - 15.38: евуйвгере
-```
diff --git a/spaces/pixiou/bingo/src/lib/isomorphic/index.ts b/spaces/pixiou/bingo/src/lib/isomorphic/index.ts
deleted file mode 100644
index 738dc92f74079ab762d584fb7422a8c8c3b61547..0000000000000000000000000000000000000000
--- a/spaces/pixiou/bingo/src/lib/isomorphic/index.ts
+++ /dev/null
@@ -1,17 +0,0 @@
-'use client'
-
-import Default from './browser'
-
-let exportsModel: any = {}
-
-if (process.browser) {
- Object.assign(exportsModel, require('./browser').default)
-} else {
- Object.assign(exportsModel, require('./node').default)
-}
-
-export default exportsModel! as typeof Default
-
-export const fetch: typeof Default.fetch = exportsModel!.fetch
-export const WebSocket: typeof Default.WebSocket = exportsModel!.WebSocket
-export const debug: typeof Default.debug = exportsModel!.debug
diff --git a/spaces/pknez/face-swap-docker/mynewshinyroop/Lib/site-packages/pip/_internal/resolution/resolvelib/found_candidates.py b/spaces/pknez/face-swap-docker/mynewshinyroop/Lib/site-packages/pip/_internal/resolution/resolvelib/found_candidates.py
deleted file mode 100644
index 8663097b447cdd80c52e2b2abde33a4736ddb9c2..0000000000000000000000000000000000000000
--- a/spaces/pknez/face-swap-docker/mynewshinyroop/Lib/site-packages/pip/_internal/resolution/resolvelib/found_candidates.py
+++ /dev/null
@@ -1,155 +0,0 @@
-"""Utilities to lazily create and visit candidates found.
-
-Creating and visiting a candidate is a *very* costly operation. It involves
-fetching, extracting, potentially building modules from source, and verifying
-distribution metadata. It is therefore crucial for performance to keep
-everything here lazy all the way down, so we only touch candidates that we
-absolutely need, and not "download the world" when we only need one version of
-something.
-"""
-
-import functools
-from collections.abc import Sequence
-from typing import TYPE_CHECKING, Any, Callable, Iterator, Optional, Set, Tuple
-
-from pip._vendor.packaging.version import _BaseVersion
-
-from .base import Candidate
-
-IndexCandidateInfo = Tuple[_BaseVersion, Callable[[], Optional[Candidate]]]
-
-if TYPE_CHECKING:
- SequenceCandidate = Sequence[Candidate]
-else:
- # For compatibility: Python before 3.9 does not support using [] on the
- # Sequence class.
- #
- # >>> from collections.abc import Sequence
- # >>> Sequence[str]
- # Traceback (most recent call last):
- # File "", line 1, in
- # TypeError: 'ABCMeta' object is not subscriptable
- #
- # TODO: Remove this block after dropping Python 3.8 support.
- SequenceCandidate = Sequence
-
-
-def _iter_built(infos: Iterator[IndexCandidateInfo]) -> Iterator[Candidate]:
- """Iterator for ``FoundCandidates``.
-
- This iterator is used when the package is not already installed. Candidates
- from index come later in their normal ordering.
- """
- versions_found: Set[_BaseVersion] = set()
- for version, func in infos:
- if version in versions_found:
- continue
- candidate = func()
- if candidate is None:
- continue
- yield candidate
- versions_found.add(version)
-
-
-def _iter_built_with_prepended(
- installed: Candidate, infos: Iterator[IndexCandidateInfo]
-) -> Iterator[Candidate]:
- """Iterator for ``FoundCandidates``.
-
- This iterator is used when the resolver prefers the already-installed
- candidate and NOT to upgrade. The installed candidate is therefore
- always yielded first, and candidates from index come later in their
- normal ordering, except skipped when the version is already installed.
- """
- yield installed
- versions_found: Set[_BaseVersion] = {installed.version}
- for version, func in infos:
- if version in versions_found:
- continue
- candidate = func()
- if candidate is None:
- continue
- yield candidate
- versions_found.add(version)
-
-
-def _iter_built_with_inserted(
- installed: Candidate, infos: Iterator[IndexCandidateInfo]
-) -> Iterator[Candidate]:
- """Iterator for ``FoundCandidates``.
-
- This iterator is used when the resolver prefers to upgrade an
- already-installed package. Candidates from index are returned in their
- normal ordering, except replaced when the version is already installed.
-
- The implementation iterates through and yields other candidates, inserting
- the installed candidate exactly once before we start yielding older or
- equivalent candidates, or after all other candidates if they are all newer.
- """
- versions_found: Set[_BaseVersion] = set()
- for version, func in infos:
- if version in versions_found:
- continue
- # If the installed candidate is better, yield it first.
- if installed.version >= version:
- yield installed
- versions_found.add(installed.version)
- candidate = func()
- if candidate is None:
- continue
- yield candidate
- versions_found.add(version)
-
- # If the installed candidate is older than all other candidates.
- if installed.version not in versions_found:
- yield installed
-
-
-class FoundCandidates(SequenceCandidate):
- """A lazy sequence to provide candidates to the resolver.
-
- The intended usage is to return this from `find_matches()` so the resolver
- can iterate through the sequence multiple times, but only access the index
- page when remote packages are actually needed. This improve performances
- when suitable candidates are already installed on disk.
- """
-
- def __init__(
- self,
- get_infos: Callable[[], Iterator[IndexCandidateInfo]],
- installed: Optional[Candidate],
- prefers_installed: bool,
- incompatible_ids: Set[int],
- ):
- self._get_infos = get_infos
- self._installed = installed
- self._prefers_installed = prefers_installed
- self._incompatible_ids = incompatible_ids
-
- def __getitem__(self, index: Any) -> Any:
- # Implemented to satisfy the ABC check. This is not needed by the
- # resolver, and should not be used by the provider either (for
- # performance reasons).
- raise NotImplementedError("don't do this")
-
- def __iter__(self) -> Iterator[Candidate]:
- infos = self._get_infos()
- if not self._installed:
- iterator = _iter_built(infos)
- elif self._prefers_installed:
- iterator = _iter_built_with_prepended(self._installed, infos)
- else:
- iterator = _iter_built_with_inserted(self._installed, infos)
- return (c for c in iterator if id(c) not in self._incompatible_ids)
-
- def __len__(self) -> int:
- # Implemented to satisfy the ABC check. This is not needed by the
- # resolver, and should not be used by the provider either (for
- # performance reasons).
- raise NotImplementedError("don't do this")
-
- @functools.lru_cache(maxsize=1)
- def __bool__(self) -> bool:
- if self._prefers_installed and self._installed:
- return True
- return any(self)
diff --git a/spaces/pknez/face-swap-docker/mynewshinyroop/Lib/site-packages/pip/_vendor/pyproject_hooks/_in_process/_in_process.py b/spaces/pknez/face-swap-docker/mynewshinyroop/Lib/site-packages/pip/_vendor/pyproject_hooks/_in_process/_in_process.py
deleted file mode 100644
index ee511ff20d73bb245fe7ae0c1fc31a41c33e7d29..0000000000000000000000000000000000000000
--- a/spaces/pknez/face-swap-docker/mynewshinyroop/Lib/site-packages/pip/_vendor/pyproject_hooks/_in_process/_in_process.py
+++ /dev/null
@@ -1,353 +0,0 @@
-"""This is invoked in a subprocess to call the build backend hooks.
-
-It expects:
-- Command line args: hook_name, control_dir
-- Environment variables:
- PEP517_BUILD_BACKEND=entry.point:spec
- PEP517_BACKEND_PATH=paths (separated with os.pathsep)
-- control_dir/input.json:
- - {"kwargs": {...}}
-
-Results:
-- control_dir/output.json
- - {"return_val": ...}
-"""
-import json
-import os
-import os.path
-import re
-import shutil
-import sys
-import traceback
-from glob import glob
-from importlib import import_module
-from os.path import join as pjoin
-
-# This file is run as a script, and `import wrappers` is not zip-safe, so we
-# include write_json() and read_json() from wrappers.py.
-
-
-def write_json(obj, path, **kwargs):
- with open(path, 'w', encoding='utf-8') as f:
- json.dump(obj, f, **kwargs)
-
-
-def read_json(path):
- with open(path, encoding='utf-8') as f:
- return json.load(f)
-
-
-class BackendUnavailable(Exception):
- """Raised if we cannot import the backend"""
- def __init__(self, traceback):
- self.traceback = traceback
-
-
-class BackendInvalid(Exception):
- """Raised if the backend is invalid"""
- def __init__(self, message):
- self.message = message
-
-
-class HookMissing(Exception):
- """Raised if a hook is missing and we are not executing the fallback"""
- def __init__(self, hook_name=None):
- super().__init__(hook_name)
- self.hook_name = hook_name
-
-
-def contained_in(filename, directory):
- """Test if a file is located within the given directory."""
- filename = os.path.normcase(os.path.abspath(filename))
- directory = os.path.normcase(os.path.abspath(directory))
- return os.path.commonprefix([filename, directory]) == directory
-
-
-def _build_backend():
- """Find and load the build backend"""
- # Add in-tree backend directories to the front of sys.path.
- backend_path = os.environ.get('PEP517_BACKEND_PATH')
- if backend_path:
- extra_pathitems = backend_path.split(os.pathsep)
- sys.path[:0] = extra_pathitems
-
- ep = os.environ['PEP517_BUILD_BACKEND']
- mod_path, _, obj_path = ep.partition(':')
- try:
- obj = import_module(mod_path)
- except ImportError:
- raise BackendUnavailable(traceback.format_exc())
-
- if backend_path:
- if not any(
- contained_in(obj.__file__, path)
- for path in extra_pathitems
- ):
- raise BackendInvalid("Backend was not loaded from backend-path")
-
- if obj_path:
- for path_part in obj_path.split('.'):
- obj = getattr(obj, path_part)
- return obj
-
-
-def _supported_features():
- """Return the list of options features supported by the backend.
-
- Returns a list of strings.
- The only possible value is 'build_editable'.
- """
- backend = _build_backend()
- features = []
- if hasattr(backend, "build_editable"):
- features.append("build_editable")
- return features
-
-
-def get_requires_for_build_wheel(config_settings):
- """Invoke the optional get_requires_for_build_wheel hook
-
- Returns [] if the hook is not defined.
- """
- backend = _build_backend()
- try:
- hook = backend.get_requires_for_build_wheel
- except AttributeError:
- return []
- else:
- return hook(config_settings)
-
-
-def get_requires_for_build_editable(config_settings):
- """Invoke the optional get_requires_for_build_editable hook
-
- Returns [] if the hook is not defined.
- """
- backend = _build_backend()
- try:
- hook = backend.get_requires_for_build_editable
- except AttributeError:
- return []
- else:
- return hook(config_settings)
-
-
-def prepare_metadata_for_build_wheel(
- metadata_directory, config_settings, _allow_fallback):
- """Invoke optional prepare_metadata_for_build_wheel
-
- Implements a fallback by building a wheel if the hook isn't defined,
- unless _allow_fallback is False in which case HookMissing is raised.
- """
- backend = _build_backend()
- try:
- hook = backend.prepare_metadata_for_build_wheel
- except AttributeError:
- if not _allow_fallback:
- raise HookMissing()
- else:
- return hook(metadata_directory, config_settings)
- # fallback to build_wheel outside the try block to avoid exception chaining
- # which can be confusing to users and is not relevant
- whl_basename = backend.build_wheel(metadata_directory, config_settings)
- return _get_wheel_metadata_from_wheel(whl_basename, metadata_directory,
- config_settings)
-
-
-def prepare_metadata_for_build_editable(
- metadata_directory, config_settings, _allow_fallback):
- """Invoke optional prepare_metadata_for_build_editable
-
- Implements a fallback by building an editable wheel if the hook isn't
- defined, unless _allow_fallback is False in which case HookMissing is
- raised.
- """
- backend = _build_backend()
- try:
- hook = backend.prepare_metadata_for_build_editable
- except AttributeError:
- if not _allow_fallback:
- raise HookMissing()
- try:
- build_hook = backend.build_editable
- except AttributeError:
- raise HookMissing(hook_name='build_editable')
- else:
- whl_basename = build_hook(metadata_directory, config_settings)
- return _get_wheel_metadata_from_wheel(whl_basename,
- metadata_directory,
- config_settings)
- else:
- return hook(metadata_directory, config_settings)
-
-
-WHEEL_BUILT_MARKER = 'PEP517_ALREADY_BUILT_WHEEL'
-
-
-def _dist_info_files(whl_zip):
- """Identify the .dist-info folder inside a wheel ZipFile."""
- res = []
- for path in whl_zip.namelist():
- m = re.match(r'[^/\\]+-[^/\\]+\.dist-info/', path)
- if m:
- res.append(path)
- if res:
- return res
- raise Exception("No .dist-info folder found in wheel")
-
-
-def _get_wheel_metadata_from_wheel(
- whl_basename, metadata_directory, config_settings):
- """Extract the metadata from a wheel.
-
- Fallback for when the build backend does not
- define the 'get_wheel_metadata' hook.
- """
- from zipfile import ZipFile
- with open(os.path.join(metadata_directory, WHEEL_BUILT_MARKER), 'wb'):
- pass # Touch marker file
-
- whl_file = os.path.join(metadata_directory, whl_basename)
- with ZipFile(whl_file) as zipf:
- dist_info = _dist_info_files(zipf)
- zipf.extractall(path=metadata_directory, members=dist_info)
- return dist_info[0].split('/')[0]
-
-
-def _find_already_built_wheel(metadata_directory):
- """Check for a wheel already built during the get_wheel_metadata hook.
- """
- if not metadata_directory:
- return None
- metadata_parent = os.path.dirname(metadata_directory)
- if not os.path.isfile(pjoin(metadata_parent, WHEEL_BUILT_MARKER)):
- return None
-
- whl_files = glob(os.path.join(metadata_parent, '*.whl'))
- if not whl_files:
- print('Found wheel built marker, but no .whl files')
- return None
- if len(whl_files) > 1:
- print('Found multiple .whl files; unspecified behaviour. '
- 'Will call build_wheel.')
- return None
-
- # Exactly one .whl file
- return whl_files[0]
-
-
-def build_wheel(wheel_directory, config_settings, metadata_directory=None):
- """Invoke the mandatory build_wheel hook.
-
- If a wheel was already built in the
- prepare_metadata_for_build_wheel fallback, this
- will copy it rather than rebuilding the wheel.
- """
- prebuilt_whl = _find_already_built_wheel(metadata_directory)
- if prebuilt_whl:
- shutil.copy2(prebuilt_whl, wheel_directory)
- return os.path.basename(prebuilt_whl)
-
- return _build_backend().build_wheel(wheel_directory, config_settings,
- metadata_directory)
-
-
-def build_editable(wheel_directory, config_settings, metadata_directory=None):
- """Invoke the optional build_editable hook.
-
- If a wheel was already built in the
- prepare_metadata_for_build_editable fallback, this
- will copy it rather than rebuilding the wheel.
- """
- backend = _build_backend()
- try:
- hook = backend.build_editable
- except AttributeError:
- raise HookMissing()
- else:
- prebuilt_whl = _find_already_built_wheel(metadata_directory)
- if prebuilt_whl:
- shutil.copy2(prebuilt_whl, wheel_directory)
- return os.path.basename(prebuilt_whl)
-
- return hook(wheel_directory, config_settings, metadata_directory)
-
-
-def get_requires_for_build_sdist(config_settings):
- """Invoke the optional get_requires_for_build_wheel hook
-
- Returns [] if the hook is not defined.
- """
- backend = _build_backend()
- try:
- hook = backend.get_requires_for_build_sdist
- except AttributeError:
- return []
- else:
- return hook(config_settings)
-
-
-class _DummyException(Exception):
- """Nothing should ever raise this exception"""
-
-
-class GotUnsupportedOperation(Exception):
- """For internal use when backend raises UnsupportedOperation"""
- def __init__(self, traceback):
- self.traceback = traceback
-
-
-def build_sdist(sdist_directory, config_settings):
- """Invoke the mandatory build_sdist hook."""
- backend = _build_backend()
- try:
- return backend.build_sdist(sdist_directory, config_settings)
- except getattr(backend, 'UnsupportedOperation', _DummyException):
- raise GotUnsupportedOperation(traceback.format_exc())
-
-
-HOOK_NAMES = {
- 'get_requires_for_build_wheel',
- 'prepare_metadata_for_build_wheel',
- 'build_wheel',
- 'get_requires_for_build_editable',
- 'prepare_metadata_for_build_editable',
- 'build_editable',
- 'get_requires_for_build_sdist',
- 'build_sdist',
- '_supported_features',
-}
-
-
-def main():
- if len(sys.argv) < 3:
- sys.exit("Needs args: hook_name, control_dir")
- hook_name = sys.argv[1]
- control_dir = sys.argv[2]
- if hook_name not in HOOK_NAMES:
- sys.exit("Unknown hook: %s" % hook_name)
- hook = globals()[hook_name]
-
- hook_input = read_json(pjoin(control_dir, 'input.json'))
-
- json_out = {'unsupported': False, 'return_val': None}
- try:
- json_out['return_val'] = hook(**hook_input['kwargs'])
- except BackendUnavailable as e:
- json_out['no_backend'] = True
- json_out['traceback'] = e.traceback
- except BackendInvalid as e:
- json_out['backend_invalid'] = True
- json_out['backend_error'] = e.message
- except GotUnsupportedOperation as e:
- json_out['unsupported'] = True
- json_out['traceback'] = e.traceback
- except HookMissing as e:
- json_out['hook_missing'] = True
- json_out['missing_hook_name'] = e.hook_name or hook_name
-
- write_json(json_out, pjoin(control_dir, 'output.json'), indent=2)
-
-
-if __name__ == '__main__':
- main()
diff --git a/spaces/profayle/TerrapinTalk/myenv/lib/python3.9/site-packages/fontTools/encodings/MacRoman.py b/spaces/profayle/TerrapinTalk/myenv/lib/python3.9/site-packages/fontTools/encodings/MacRoman.py
deleted file mode 100644
index ba8bf14ef7de1cf76248a2bbd1a98bc8bf36cc5e..0000000000000000000000000000000000000000
--- a/spaces/profayle/TerrapinTalk/myenv/lib/python3.9/site-packages/fontTools/encodings/MacRoman.py
+++ /dev/null
@@ -1,258 +0,0 @@
-MacRoman = [
- "NUL",
- "Eth",
- "eth",
- "Lslash",
- "lslash",
- "Scaron",
- "scaron",
- "Yacute",
- "yacute",
- "HT",
- "LF",
- "Thorn",
- "thorn",
- "CR",
- "Zcaron",
- "zcaron",
- "DLE",
- "DC1",
- "DC2",
- "DC3",
- "DC4",
- "onehalf",
- "onequarter",
- "onesuperior",
- "threequarters",
- "threesuperior",
- "twosuperior",
- "brokenbar",
- "minus",
- "multiply",
- "RS",
- "US",
- "space",
- "exclam",
- "quotedbl",
- "numbersign",
- "dollar",
- "percent",
- "ampersand",
- "quotesingle",
- "parenleft",
- "parenright",
- "asterisk",
- "plus",
- "comma",
- "hyphen",
- "period",
- "slash",
- "zero",
- "one",
- "two",
- "three",
- "four",
- "five",
- "six",
- "seven",
- "eight",
- "nine",
- "colon",
- "semicolon",
- "less",
- "equal",
- "greater",
- "question",
- "at",
- "A",
- "B",
- "C",
- "D",
- "E",
- "F",
- "G",
- "H",
- "I",
- "J",
- "K",
- "L",
- "M",
- "N",
- "O",
- "P",
- "Q",
- "R",
- "S",
- "T",
- "U",
- "V",
- "W",
- "X",
- "Y",
- "Z",
- "bracketleft",
- "backslash",
- "bracketright",
- "asciicircum",
- "underscore",
- "grave",
- "a",
- "b",
- "c",
- "d",
- "e",
- "f",
- "g",
- "h",
- "i",
- "j",
- "k",
- "l",
- "m",
- "n",
- "o",
- "p",
- "q",
- "r",
- "s",
- "t",
- "u",
- "v",
- "w",
- "x",
- "y",
- "z",
- "braceleft",
- "bar",
- "braceright",
- "asciitilde",
- "DEL",
- "Adieresis",
- "Aring",
- "Ccedilla",
- "Eacute",
- "Ntilde",
- "Odieresis",
- "Udieresis",
- "aacute",
- "agrave",
- "acircumflex",
- "adieresis",
- "atilde",
- "aring",
- "ccedilla",
- "eacute",
- "egrave",
- "ecircumflex",
- "edieresis",
- "iacute",
- "igrave",
- "icircumflex",
- "idieresis",
- "ntilde",
- "oacute",
- "ograve",
- "ocircumflex",
- "odieresis",
- "otilde",
- "uacute",
- "ugrave",
- "ucircumflex",
- "udieresis",
- "dagger",
- "degree",
- "cent",
- "sterling",
- "section",
- "bullet",
- "paragraph",
- "germandbls",
- "registered",
- "copyright",
- "trademark",
- "acute",
- "dieresis",
- "notequal",
- "AE",
- "Oslash",
- "infinity",
- "plusminus",
- "lessequal",
- "greaterequal",
- "yen",
- "mu",
- "partialdiff",
- "summation",
- "product",
- "pi",
- "integral",
- "ordfeminine",
- "ordmasculine",
- "Omega",
- "ae",
- "oslash",
- "questiondown",
- "exclamdown",
- "logicalnot",
- "radical",
- "florin",
- "approxequal",
- "Delta",
- "guillemotleft",
- "guillemotright",
- "ellipsis",
- "nbspace",
- "Agrave",
- "Atilde",
- "Otilde",
- "OE",
- "oe",
- "endash",
- "emdash",
- "quotedblleft",
- "quotedblright",
- "quoteleft",
- "quoteright",
- "divide",
- "lozenge",
- "ydieresis",
- "Ydieresis",
- "fraction",
- "currency",
- "guilsinglleft",
- "guilsinglright",
- "fi",
- "fl",
- "daggerdbl",
- "periodcentered",
- "quotesinglbase",
- "quotedblbase",
- "perthousand",
- "Acircumflex",
- "Ecircumflex",
- "Aacute",
- "Edieresis",
- "Egrave",
- "Iacute",
- "Icircumflex",
- "Idieresis",
- "Igrave",
- "Oacute",
- "Ocircumflex",
- "apple",
- "Ograve",
- "Uacute",
- "Ucircumflex",
- "Ugrave",
- "dotlessi",
- "circumflex",
- "tilde",
- "macron",
- "breve",
- "dotaccent",
- "ring",
- "cedilla",
- "hungarumlaut",
- "ogonek",
- "caron",
-]
diff --git a/spaces/profayle/TerrapinTalk/myenv/lib/python3.9/site-packages/markdown_it/cli/__init__.py b/spaces/profayle/TerrapinTalk/myenv/lib/python3.9/site-packages/markdown_it/cli/__init__.py
deleted file mode 100644
index e69de29bb2d1d6434b8b29ae775ad8c2e48c5391..0000000000000000000000000000000000000000
diff --git a/spaces/profayle/TerrapinTalk/myenv/lib/python3.9/site-packages/numpy/core/memmap.py b/spaces/profayle/TerrapinTalk/myenv/lib/python3.9/site-packages/numpy/core/memmap.py
deleted file mode 100644
index 79c695455d343365d2a299bbe499f7eac1d0906b..0000000000000000000000000000000000000000
--- a/spaces/profayle/TerrapinTalk/myenv/lib/python3.9/site-packages/numpy/core/memmap.py
+++ /dev/null
@@ -1,338 +0,0 @@
-from contextlib import nullcontext
-
-import numpy as np
-from .._utils import set_module
-from .numeric import uint8, ndarray, dtype
-from numpy.compat import os_fspath, is_pathlib_path
-
-__all__ = ['memmap']
-
-dtypedescr = dtype
-valid_filemodes = ["r", "c", "r+", "w+"]
-writeable_filemodes = ["r+", "w+"]
-
-mode_equivalents = {
- "readonly":"r",
- "copyonwrite":"c",
- "readwrite":"r+",
- "write":"w+"
- }
-
-
-@set_module('numpy')
-class memmap(ndarray):
- """Create a memory-map to an array stored in a *binary* file on disk.
-
- Memory-mapped files are used for accessing small segments of large files
- on disk, without reading the entire file into memory. NumPy's
- memmap's are array-like objects. This differs from Python's ``mmap``
- module, which uses file-like objects.
-
- This subclass of ndarray has some unpleasant interactions with
- some operations, because it doesn't quite fit properly as a subclass.
- An alternative to using this subclass is to create the ``mmap``
- object yourself, then create an ndarray with ndarray.__new__ directly,
- passing the object created in its 'buffer=' parameter.
-
- This class may at some point be turned into a factory function
- which returns a view into an mmap buffer.
-
- Flush the memmap instance to write the changes to the file. Currently there
- is no API to close the underlying ``mmap``. It is tricky to ensure the
- resource is actually closed, since it may be shared between different
- memmap instances.
-
-
- Parameters
- ----------
- filename : str, file-like object, or pathlib.Path instance
- The file name or file object to be used as the array data buffer.
- dtype : data-type, optional
- The data-type used to interpret the file contents.
- Default is `uint8`.
- mode : {'r+', 'r', 'w+', 'c'}, optional
- The file is opened in this mode:
-
- +------+-------------------------------------------------------------+
- | 'r' | Open existing file for reading only. |
- +------+-------------------------------------------------------------+
- | 'r+' | Open existing file for reading and writing. |
- +------+-------------------------------------------------------------+
- | 'w+' | Create or overwrite existing file for reading and writing. |
- | | If ``mode == 'w+'`` then `shape` must also be specified. |
- +------+-------------------------------------------------------------+
- | 'c' | Copy-on-write: assignments affect data in memory, but |
- | | changes are not saved to disk. The file on disk is |
- | | read-only. |
- +------+-------------------------------------------------------------+
-
- Default is 'r+'.
- offset : int, optional
- In the file, array data starts at this offset. Since `offset` is
- measured in bytes, it should normally be a multiple of the byte-size
- of `dtype`. When ``mode != 'r'``, even positive offsets beyond end of
- file are valid; The file will be extended to accommodate the
- additional data. By default, ``memmap`` will start at the beginning of
- the file, even if ``filename`` is a file pointer ``fp`` and
- ``fp.tell() != 0``.
- shape : tuple, optional
- The desired shape of the array. If ``mode == 'r'`` and the number
- of remaining bytes after `offset` is not a multiple of the byte-size
- of `dtype`, you must specify `shape`. By default, the returned array
- will be 1-D with the number of elements determined by file size
- and data-type.
- order : {'C', 'F'}, optional
- Specify the order of the ndarray memory layout:
- :term:`row-major`, C-style or :term:`column-major`,
- Fortran-style. This only has an effect if the shape is
- greater than 1-D. The default order is 'C'.
-
- Attributes
- ----------
- filename : str or pathlib.Path instance
- Path to the mapped file.
- offset : int
- Offset position in the file.
- mode : str
- File mode.
-
- Methods
- -------
- flush
- Flush any changes in memory to file on disk.
- When you delete a memmap object, flush is called first to write
- changes to disk.
-
-
- See also
- --------
- lib.format.open_memmap : Create or load a memory-mapped ``.npy`` file.
-
- Notes
- -----
- The memmap object can be used anywhere an ndarray is accepted.
- Given a memmap ``fp``, ``isinstance(fp, numpy.ndarray)`` returns
- ``True``.
-
- Memory-mapped files cannot be larger than 2GB on 32-bit systems.
-
- When a memmap causes a file to be created or extended beyond its
- current size in the filesystem, the contents of the new part are
- unspecified. On systems with POSIX filesystem semantics, the extended
- part will be filled with zero bytes.
-
- Examples
- --------
- >>> data = np.arange(12, dtype='float32')
- >>> data.resize((3,4))
-
- This example uses a temporary file so that doctest doesn't write
- files to your directory. You would use a 'normal' filename.
-
- >>> from tempfile import mkdtemp
- >>> import os.path as path
- >>> filename = path.join(mkdtemp(), 'newfile.dat')
-
- Create a memmap with dtype and shape that matches our data:
-
- >>> fp = np.memmap(filename, dtype='float32', mode='w+', shape=(3,4))
- >>> fp
- memmap([[0., 0., 0., 0.],
- [0., 0., 0., 0.],
- [0., 0., 0., 0.]], dtype=float32)
-
- Write data to memmap array:
-
- >>> fp[:] = data[:]
- >>> fp
- memmap([[ 0., 1., 2., 3.],
- [ 4., 5., 6., 7.],
- [ 8., 9., 10., 11.]], dtype=float32)
-
- >>> fp.filename == path.abspath(filename)
- True
-
- Flushes memory changes to disk in order to read them back
-
- >>> fp.flush()
-
- Load the memmap and verify data was stored:
-
- >>> newfp = np.memmap(filename, dtype='float32', mode='r', shape=(3,4))
- >>> newfp
- memmap([[ 0., 1., 2., 3.],
- [ 4., 5., 6., 7.],
- [ 8., 9., 10., 11.]], dtype=float32)
-
- Read-only memmap:
-
- >>> fpr = np.memmap(filename, dtype='float32', mode='r', shape=(3,4))
- >>> fpr.flags.writeable
- False
-
- Copy-on-write memmap:
-
- >>> fpc = np.memmap(filename, dtype='float32', mode='c', shape=(3,4))
- >>> fpc.flags.writeable
- True
-
- It's possible to assign to copy-on-write array, but values are only
- written into the memory copy of the array, and not written to disk:
-
- >>> fpc
- memmap([[ 0., 1., 2., 3.],
- [ 4., 5., 6., 7.],
- [ 8., 9., 10., 11.]], dtype=float32)
- >>> fpc[0,:] = 0
- >>> fpc
- memmap([[ 0., 0., 0., 0.],
- [ 4., 5., 6., 7.],
- [ 8., 9., 10., 11.]], dtype=float32)
-
- File on disk is unchanged:
-
- >>> fpr
- memmap([[ 0., 1., 2., 3.],
- [ 4., 5., 6., 7.],
- [ 8., 9., 10., 11.]], dtype=float32)
-
- Offset into a memmap:
-
- >>> fpo = np.memmap(filename, dtype='float32', mode='r', offset=16)
- >>> fpo
- memmap([ 4., 5., 6., 7., 8., 9., 10., 11.], dtype=float32)
-
- """
-
- __array_priority__ = -100.0
-
- def __new__(subtype, filename, dtype=uint8, mode='r+', offset=0,
- shape=None, order='C'):
- # Import here to minimize 'import numpy' overhead
- import mmap
- import os.path
- try:
- mode = mode_equivalents[mode]
- except KeyError as e:
- if mode not in valid_filemodes:
- raise ValueError(
- "mode must be one of {!r} (got {!r})"
- .format(valid_filemodes + list(mode_equivalents.keys()), mode)
- ) from None
-
- if mode == 'w+' and shape is None:
- raise ValueError("shape must be given if mode == 'w+'")
-
- if hasattr(filename, 'read'):
- f_ctx = nullcontext(filename)
- else:
- f_ctx = open(os_fspath(filename), ('r' if mode == 'c' else mode)+'b')
-
- with f_ctx as fid:
- fid.seek(0, 2)
- flen = fid.tell()
- descr = dtypedescr(dtype)
- _dbytes = descr.itemsize
-
- if shape is None:
- bytes = flen - offset
- if bytes % _dbytes:
- raise ValueError("Size of available data is not a "
- "multiple of the data-type size.")
- size = bytes // _dbytes
- shape = (size,)
- else:
- if not isinstance(shape, tuple):
- shape = (shape,)
- size = np.intp(1) # avoid default choice of np.int_, which might overflow
- for k in shape:
- size *= k
-
- bytes = int(offset + size*_dbytes)
-
- if mode in ('w+', 'r+') and flen < bytes:
- fid.seek(bytes - 1, 0)
- fid.write(b'\0')
- fid.flush()
-
- if mode == 'c':
- acc = mmap.ACCESS_COPY
- elif mode == 'r':
- acc = mmap.ACCESS_READ
- else:
- acc = mmap.ACCESS_WRITE
-
- start = offset - offset % mmap.ALLOCATIONGRANULARITY
- bytes -= start
- array_offset = offset - start
- mm = mmap.mmap(fid.fileno(), bytes, access=acc, offset=start)
-
- self = ndarray.__new__(subtype, shape, dtype=descr, buffer=mm,
- offset=array_offset, order=order)
- self._mmap = mm
- self.offset = offset
- self.mode = mode
-
- if is_pathlib_path(filename):
- # special case - if we were constructed with a pathlib.path,
- # then filename is a path object, not a string
- self.filename = filename.resolve()
- elif hasattr(fid, "name") and isinstance(fid.name, str):
- # py3 returns int for TemporaryFile().name
- self.filename = os.path.abspath(fid.name)
- # same as memmap copies (e.g. memmap + 1)
- else:
- self.filename = None
-
- return self
-
- def __array_finalize__(self, obj):
- if hasattr(obj, '_mmap') and np.may_share_memory(self, obj):
- self._mmap = obj._mmap
- self.filename = obj.filename
- self.offset = obj.offset
- self.mode = obj.mode
- else:
- self._mmap = None
- self.filename = None
- self.offset = None
- self.mode = None
-
- def flush(self):
- """
- Write any changes in the array to the file on disk.
-
- For further information, see `memmap`.
-
- Parameters
- ----------
- None
-
- See Also
- --------
- memmap
-
- """
- if self.base is not None and hasattr(self.base, 'flush'):
- self.base.flush()
-
- def __array_wrap__(self, arr, context=None):
- arr = super().__array_wrap__(arr, context)
-
- # Return a memmap if a memmap was given as the output of the
- # ufunc. Leave the arr class unchanged if self is not a memmap
- # to keep original memmap subclasses behavior
- if self is arr or type(self) is not memmap:
- return arr
- # Return scalar instead of 0d memmap, e.g. for np.sum with
- # axis=None
- if arr.shape == ():
- return arr[()]
- # Return ndarray otherwise
- return arr.view(np.ndarray)
-
- def __getitem__(self, index):
- res = super().__getitem__(index)
- if type(res) is memmap and res._mmap is None:
- return res.view(type=ndarray)
- return res
diff --git a/spaces/profayle/TerrapinTalk/myenv/lib/python3.9/site-packages/numpy/distutils/mingw/gfortran_vs2003_hack.c b/spaces/profayle/TerrapinTalk/myenv/lib/python3.9/site-packages/numpy/distutils/mingw/gfortran_vs2003_hack.c
deleted file mode 100644
index 485a675d8a1fb80bc4927fe236ba3fe550f5a0c9..0000000000000000000000000000000000000000
--- a/spaces/profayle/TerrapinTalk/myenv/lib/python3.9/site-packages/numpy/distutils/mingw/gfortran_vs2003_hack.c
+++ /dev/null
@@ -1,6 +0,0 @@
-int _get_output_format(void)
-{
- return 0;
-}
-
-int _imp____lc_codepage = 0;
diff --git a/spaces/profayle/TerrapinTalk/myenv/lib/python3.9/site-packages/numpy/distutils/mingw32ccompiler.py b/spaces/profayle/TerrapinTalk/myenv/lib/python3.9/site-packages/numpy/distutils/mingw32ccompiler.py
deleted file mode 100644
index 4763f41ad326d464355fd82ceccb019e1e55edf0..0000000000000000000000000000000000000000
--- a/spaces/profayle/TerrapinTalk/myenv/lib/python3.9/site-packages/numpy/distutils/mingw32ccompiler.py
+++ /dev/null
@@ -1,591 +0,0 @@
-"""
-Support code for building Python extensions on Windows.
-
- # NT stuff
- # 1. Make sure libpython.a exists for gcc. If not, build it.
- # 2. Force windows to use gcc (we're struggling with MSVC and g77 support)
- # 3. Force windows to use g77
-
-"""
-import os
-import sys
-import subprocess
-import re
-import textwrap
-
-# Overwrite certain distutils.ccompiler functions:
-import numpy.distutils.ccompiler # noqa: F401
-from numpy.distutils import log
-# NT stuff
-# 1. Make sure libpython.a exists for gcc. If not, build it.
-# 2. Force windows to use gcc (we're struggling with MSVC and g77 support)
-# --> this is done in numpy/distutils/ccompiler.py
-# 3. Force windows to use g77
-
-import distutils.cygwinccompiler
-from distutils.unixccompiler import UnixCCompiler
-from distutils.msvccompiler import get_build_version as get_build_msvc_version
-from distutils.errors import UnknownFileError
-from numpy.distutils.misc_util import (msvc_runtime_library,
- msvc_runtime_version,
- msvc_runtime_major,
- get_build_architecture)
-
-def get_msvcr_replacement():
- """Replacement for outdated version of get_msvcr from cygwinccompiler"""
- msvcr = msvc_runtime_library()
- return [] if msvcr is None else [msvcr]
-
-
-# Useful to generate table of symbols from a dll
-_START = re.compile(r'\[Ordinal/Name Pointer\] Table')
-_TABLE = re.compile(r'^\s+\[([\s*[0-9]*)\] ([a-zA-Z0-9_]*)')
-
-# the same as cygwin plus some additional parameters
-class Mingw32CCompiler(distutils.cygwinccompiler.CygwinCCompiler):
- """ A modified MingW32 compiler compatible with an MSVC built Python.
-
- """
-
- compiler_type = 'mingw32'
-
- def __init__ (self,
- verbose=0,
- dry_run=0,
- force=0):
-
- distutils.cygwinccompiler.CygwinCCompiler.__init__ (self, verbose,
- dry_run, force)
-
- # **changes: eric jones 4/11/01
- # 1. Check for import library on Windows. Build if it doesn't exist.
-
- build_import_library()
-
- # Check for custom msvc runtime library on Windows. Build if it doesn't exist.
- msvcr_success = build_msvcr_library()
- msvcr_dbg_success = build_msvcr_library(debug=True)
- if msvcr_success or msvcr_dbg_success:
- # add preprocessor statement for using customized msvcr lib
- self.define_macro('NPY_MINGW_USE_CUSTOM_MSVCR')
-
- # Define the MSVC version as hint for MinGW
- msvcr_version = msvc_runtime_version()
- if msvcr_version:
- self.define_macro('__MSVCRT_VERSION__', '0x%04i' % msvcr_version)
-
- # MS_WIN64 should be defined when building for amd64 on windows,
- # but python headers define it only for MS compilers, which has all
- # kind of bad consequences, like using Py_ModuleInit4 instead of
- # Py_ModuleInit4_64, etc... So we add it here
- if get_build_architecture() == 'AMD64':
- self.set_executables(
- compiler='gcc -g -DDEBUG -DMS_WIN64 -O0 -Wall',
- compiler_so='gcc -g -DDEBUG -DMS_WIN64 -O0 -Wall '
- '-Wstrict-prototypes',
- linker_exe='gcc -g',
- linker_so='gcc -g -shared')
- else:
- self.set_executables(
- compiler='gcc -O2 -Wall',
- compiler_so='gcc -O2 -Wall -Wstrict-prototypes',
- linker_exe='g++ ',
- linker_so='g++ -shared')
- # added for python2.3 support
- # we can't pass it through set_executables because pre 2.2 would fail
- self.compiler_cxx = ['g++']
-
- # Maybe we should also append -mthreads, but then the finished dlls
- # need another dll (mingwm10.dll see Mingw32 docs) (-mthreads: Support
- # thread-safe exception handling on `Mingw32')
-
- # no additional libraries needed
- #self.dll_libraries=[]
- return
-
- # __init__ ()
-
- def link(self,
- target_desc,
- objects,
- output_filename,
- output_dir,
- libraries,
- library_dirs,
- runtime_library_dirs,
- export_symbols = None,
- debug=0,
- extra_preargs=None,
- extra_postargs=None,
- build_temp=None,
- target_lang=None):
- # Include the appropriate MSVC runtime library if Python was built
- # with MSVC >= 7.0 (MinGW standard is msvcrt)
- runtime_library = msvc_runtime_library()
- if runtime_library:
- if not libraries:
- libraries = []
- libraries.append(runtime_library)
- args = (self,
- target_desc,
- objects,
- output_filename,
- output_dir,
- libraries,
- library_dirs,
- runtime_library_dirs,
- None, #export_symbols, we do this in our def-file
- debug,
- extra_preargs,
- extra_postargs,
- build_temp,
- target_lang)
- func = UnixCCompiler.link
- func(*args[:func.__code__.co_argcount])
- return
-
- def object_filenames (self,
- source_filenames,
- strip_dir=0,
- output_dir=''):
- if output_dir is None: output_dir = ''
- obj_names = []
- for src_name in source_filenames:
- # use normcase to make sure '.rc' is really '.rc' and not '.RC'
- (base, ext) = os.path.splitext (os.path.normcase(src_name))
-
- # added these lines to strip off windows drive letters
- # without it, .o files are placed next to .c files
- # instead of the build directory
- drv, base = os.path.splitdrive(base)
- if drv:
- base = base[1:]
-
- if ext not in (self.src_extensions + ['.rc', '.res']):
- raise UnknownFileError(
- "unknown file type '%s' (from '%s')" % \
- (ext, src_name))
- if strip_dir:
- base = os.path.basename (base)
- if ext == '.res' or ext == '.rc':
- # these need to be compiled to object files
- obj_names.append (os.path.join (output_dir,
- base + ext + self.obj_extension))
- else:
- obj_names.append (os.path.join (output_dir,
- base + self.obj_extension))
- return obj_names
-
- # object_filenames ()
-
-
-def find_python_dll():
- # We can't do much here:
- # - find it in the virtualenv (sys.prefix)
- # - find it in python main dir (sys.base_prefix, if in a virtualenv)
- # - in system32,
- # - ortherwise (Sxs), I don't know how to get it.
- stems = [sys.prefix]
- if sys.base_prefix != sys.prefix:
- stems.append(sys.base_prefix)
-
- sub_dirs = ['', 'lib', 'bin']
- # generate possible combinations of directory trees and sub-directories
- lib_dirs = []
- for stem in stems:
- for folder in sub_dirs:
- lib_dirs.append(os.path.join(stem, folder))
-
- # add system directory as well
- if 'SYSTEMROOT' in os.environ:
- lib_dirs.append(os.path.join(os.environ['SYSTEMROOT'], 'System32'))
-
- # search in the file system for possible candidates
- major_version, minor_version = tuple(sys.version_info[:2])
- implementation = sys.implementation.name
- if implementation == 'cpython':
- dllname = f'python{major_version}{minor_version}.dll'
- elif implementation == 'pypy':
- dllname = f'libpypy{major_version}.{minor_version}-c.dll'
- else:
- dllname = f'Unknown platform {implementation}'
- print("Looking for %s" % dllname)
- for folder in lib_dirs:
- dll = os.path.join(folder, dllname)
- if os.path.exists(dll):
- return dll
-
- raise ValueError("%s not found in %s" % (dllname, lib_dirs))
-
-def dump_table(dll):
- st = subprocess.check_output(["objdump.exe", "-p", dll])
- return st.split(b'\n')
-
-def generate_def(dll, dfile):
- """Given a dll file location, get all its exported symbols and dump them
- into the given def file.
-
- The .def file will be overwritten"""
- dump = dump_table(dll)
- for i in range(len(dump)):
- if _START.match(dump[i].decode()):
- break
- else:
- raise ValueError("Symbol table not found")
-
- syms = []
- for j in range(i+1, len(dump)):
- m = _TABLE.match(dump[j].decode())
- if m:
- syms.append((int(m.group(1).strip()), m.group(2)))
- else:
- break
-
- if len(syms) == 0:
- log.warn('No symbols found in %s' % dll)
-
- with open(dfile, 'w') as d:
- d.write('LIBRARY %s\n' % os.path.basename(dll))
- d.write(';CODE PRELOAD MOVEABLE DISCARDABLE\n')
- d.write(';DATA PRELOAD SINGLE\n')
- d.write('\nEXPORTS\n')
- for s in syms:
- #d.write('@%d %s\n' % (s[0], s[1]))
- d.write('%s\n' % s[1])
-
-def find_dll(dll_name):
-
- arch = {'AMD64' : 'amd64',
- 'Intel' : 'x86'}[get_build_architecture()]
-
- def _find_dll_in_winsxs(dll_name):
- # Walk through the WinSxS directory to find the dll.
- winsxs_path = os.path.join(os.environ.get('WINDIR', r'C:\WINDOWS'),
- 'winsxs')
- if not os.path.exists(winsxs_path):
- return None
- for root, dirs, files in os.walk(winsxs_path):
- if dll_name in files and arch in root:
- return os.path.join(root, dll_name)
- return None
-
- def _find_dll_in_path(dll_name):
- # First, look in the Python directory, then scan PATH for
- # the given dll name.
- for path in [sys.prefix] + os.environ['PATH'].split(';'):
- filepath = os.path.join(path, dll_name)
- if os.path.exists(filepath):
- return os.path.abspath(filepath)
-
- return _find_dll_in_winsxs(dll_name) or _find_dll_in_path(dll_name)
-
-def build_msvcr_library(debug=False):
- if os.name != 'nt':
- return False
-
- # If the version number is None, then we couldn't find the MSVC runtime at
- # all, because we are running on a Python distribution which is customed
- # compiled; trust that the compiler is the same as the one available to us
- # now, and that it is capable of linking with the correct runtime without
- # any extra options.
- msvcr_ver = msvc_runtime_major()
- if msvcr_ver is None:
- log.debug('Skip building import library: '
- 'Runtime is not compiled with MSVC')
- return False
-
- # Skip using a custom library for versions < MSVC 8.0
- if msvcr_ver < 80:
- log.debug('Skip building msvcr library:'
- ' custom functionality not present')
- return False
-
- msvcr_name = msvc_runtime_library()
- if debug:
- msvcr_name += 'd'
-
- # Skip if custom library already exists
- out_name = "lib%s.a" % msvcr_name
- out_file = os.path.join(sys.prefix, 'libs', out_name)
- if os.path.isfile(out_file):
- log.debug('Skip building msvcr library: "%s" exists' %
- (out_file,))
- return True
-
- # Find the msvcr dll
- msvcr_dll_name = msvcr_name + '.dll'
- dll_file = find_dll(msvcr_dll_name)
- if not dll_file:
- log.warn('Cannot build msvcr library: "%s" not found' %
- msvcr_dll_name)
- return False
-
- def_name = "lib%s.def" % msvcr_name
- def_file = os.path.join(sys.prefix, 'libs', def_name)
-
- log.info('Building msvcr library: "%s" (from %s)' \
- % (out_file, dll_file))
-
- # Generate a symbol definition file from the msvcr dll
- generate_def(dll_file, def_file)
-
- # Create a custom mingw library for the given symbol definitions
- cmd = ['dlltool', '-d', def_file, '-l', out_file]
- retcode = subprocess.call(cmd)
-
- # Clean up symbol definitions
- os.remove(def_file)
-
- return (not retcode)
-
-def build_import_library():
- if os.name != 'nt':
- return
-
- arch = get_build_architecture()
- if arch == 'AMD64':
- return _build_import_library_amd64()
- elif arch == 'Intel':
- return _build_import_library_x86()
- else:
- raise ValueError("Unhandled arch %s" % arch)
-
-def _check_for_import_lib():
- """Check if an import library for the Python runtime already exists."""
- major_version, minor_version = tuple(sys.version_info[:2])
-
- # patterns for the file name of the library itself
- patterns = ['libpython%d%d.a',
- 'libpython%d%d.dll.a',
- 'libpython%d.%d.dll.a']
-
- # directory trees that may contain the library
- stems = [sys.prefix]
- if hasattr(sys, 'base_prefix') and sys.base_prefix != sys.prefix:
- stems.append(sys.base_prefix)
- elif hasattr(sys, 'real_prefix') and sys.real_prefix != sys.prefix:
- stems.append(sys.real_prefix)
-
- # possible subdirectories within those trees where it is placed
- sub_dirs = ['libs', 'lib']
-
- # generate a list of candidate locations
- candidates = []
- for pat in patterns:
- filename = pat % (major_version, minor_version)
- for stem_dir in stems:
- for folder in sub_dirs:
- candidates.append(os.path.join(stem_dir, folder, filename))
-
- # test the filesystem to see if we can find any of these
- for fullname in candidates:
- if os.path.isfile(fullname):
- # already exists, in location given
- return (True, fullname)
-
- # needs to be built, preferred location given first
- return (False, candidates[0])
-
-def _build_import_library_amd64():
- out_exists, out_file = _check_for_import_lib()
- if out_exists:
- log.debug('Skip building import library: "%s" exists', out_file)
- return
-
- # get the runtime dll for which we are building import library
- dll_file = find_python_dll()
- log.info('Building import library (arch=AMD64): "%s" (from %s)' %
- (out_file, dll_file))
-
- # generate symbol list from this library
- def_name = "python%d%d.def" % tuple(sys.version_info[:2])
- def_file = os.path.join(sys.prefix, 'libs', def_name)
- generate_def(dll_file, def_file)
-
- # generate import library from this symbol list
- cmd = ['dlltool', '-d', def_file, '-l', out_file]
- subprocess.check_call(cmd)
-
-def _build_import_library_x86():
- """ Build the import libraries for Mingw32-gcc on Windows
- """
- out_exists, out_file = _check_for_import_lib()
- if out_exists:
- log.debug('Skip building import library: "%s" exists', out_file)
- return
-
- lib_name = "python%d%d.lib" % tuple(sys.version_info[:2])
- lib_file = os.path.join(sys.prefix, 'libs', lib_name)
- if not os.path.isfile(lib_file):
- # didn't find library file in virtualenv, try base distribution, too,
- # and use that instead if found there. for Python 2.7 venvs, the base
- # directory is in attribute real_prefix instead of base_prefix.
- if hasattr(sys, 'base_prefix'):
- base_lib = os.path.join(sys.base_prefix, 'libs', lib_name)
- elif hasattr(sys, 'real_prefix'):
- base_lib = os.path.join(sys.real_prefix, 'libs', lib_name)
- else:
- base_lib = '' # os.path.isfile('') == False
-
- if os.path.isfile(base_lib):
- lib_file = base_lib
- else:
- log.warn('Cannot build import library: "%s" not found', lib_file)
- return
- log.info('Building import library (ARCH=x86): "%s"', out_file)
-
- from numpy.distutils import lib2def
-
- def_name = "python%d%d.def" % tuple(sys.version_info[:2])
- def_file = os.path.join(sys.prefix, 'libs', def_name)
- nm_output = lib2def.getnm(
- lib2def.DEFAULT_NM + [lib_file], shell=False)
- dlist, flist = lib2def.parse_nm(nm_output)
- with open(def_file, 'w') as fid:
- lib2def.output_def(dlist, flist, lib2def.DEF_HEADER, fid)
-
- dll_name = find_python_dll ()
-
- cmd = ["dlltool",
- "--dllname", dll_name,
- "--def", def_file,
- "--output-lib", out_file]
- status = subprocess.check_output(cmd)
- if status:
- log.warn('Failed to build import library for gcc. Linking will fail.')
- return
-
-#=====================================
-# Dealing with Visual Studio MANIFESTS
-#=====================================
-
-# Functions to deal with visual studio manifests. Manifest are a mechanism to
-# enforce strong DLL versioning on windows, and has nothing to do with
-# distutils MANIFEST. manifests are XML files with version info, and used by
-# the OS loader; they are necessary when linking against a DLL not in the
-# system path; in particular, official python 2.6 binary is built against the
-# MS runtime 9 (the one from VS 2008), which is not available on most windows
-# systems; python 2.6 installer does install it in the Win SxS (Side by side)
-# directory, but this requires the manifest for this to work. This is a big
-# mess, thanks MS for a wonderful system.
-
-# XXX: ideally, we should use exactly the same version as used by python. I
-# submitted a patch to get this version, but it was only included for python
-# 2.6.1 and above. So for versions below, we use a "best guess".
-_MSVCRVER_TO_FULLVER = {}
-if sys.platform == 'win32':
- try:
- import msvcrt
- # I took one version in my SxS directory: no idea if it is the good
- # one, and we can't retrieve it from python
- _MSVCRVER_TO_FULLVER['80'] = "8.0.50727.42"
- _MSVCRVER_TO_FULLVER['90'] = "9.0.21022.8"
- # Value from msvcrt.CRT_ASSEMBLY_VERSION under Python 3.3.0
- # on Windows XP:
- _MSVCRVER_TO_FULLVER['100'] = "10.0.30319.460"
- crt_ver = getattr(msvcrt, 'CRT_ASSEMBLY_VERSION', None)
- if crt_ver is not None: # Available at least back to Python 3.3
- maj, min = re.match(r'(\d+)\.(\d)', crt_ver).groups()
- _MSVCRVER_TO_FULLVER[maj + min] = crt_ver
- del maj, min
- del crt_ver
- except ImportError:
- # If we are here, means python was not built with MSVC. Not sure what
- # to do in that case: manifest building will fail, but it should not be
- # used in that case anyway
- log.warn('Cannot import msvcrt: using manifest will not be possible')
-
-def msvc_manifest_xml(maj, min):
- """Given a major and minor version of the MSVCR, returns the
- corresponding XML file."""
- try:
- fullver = _MSVCRVER_TO_FULLVER[str(maj * 10 + min)]
- except KeyError:
- raise ValueError("Version %d,%d of MSVCRT not supported yet" %
- (maj, min)) from None
- # Don't be fooled, it looks like an XML, but it is not. In particular, it
- # should not have any space before starting, and its size should be
- # divisible by 4, most likely for alignment constraints when the xml is
- # embedded in the binary...
- # This template was copied directly from the python 2.6 binary (using
- # strings.exe from mingw on python.exe).
- template = textwrap.dedent("""\
-
-
-
-
-
-
-
-
-
-
-
-
-
- """)
-
- return template % {'fullver': fullver, 'maj': maj, 'min': min}
-
-def manifest_rc(name, type='dll'):
- """Return the rc file used to generate the res file which will be embedded
- as manifest for given manifest file name, of given type ('dll' or
- 'exe').
-
- Parameters
- ----------
- name : str
- name of the manifest file to embed
- type : str {'dll', 'exe'}
- type of the binary which will embed the manifest
-
- """
- if type == 'dll':
- rctype = 2
- elif type == 'exe':
- rctype = 1
- else:
- raise ValueError("Type %s not supported" % type)
-
- return """\
-#include "winuser.h"
-%d RT_MANIFEST %s""" % (rctype, name)
-
-def check_embedded_msvcr_match_linked(msver):
- """msver is the ms runtime version used for the MANIFEST."""
- # check msvcr major version are the same for linking and
- # embedding
- maj = msvc_runtime_major()
- if maj:
- if not maj == int(msver):
- raise ValueError(
- "Discrepancy between linked msvcr " \
- "(%d) and the one about to be embedded " \
- "(%d)" % (int(msver), maj))
-
-def configtest_name(config):
- base = os.path.basename(config._gen_temp_sourcefile("yo", [], "c"))
- return os.path.splitext(base)[0]
-
-def manifest_name(config):
- # Get configest name (including suffix)
- root = configtest_name(config)
- exext = config.compiler.exe_extension
- return root + exext + ".manifest"
-
-def rc_name(config):
- # Get configtest name (including suffix)
- root = configtest_name(config)
- return root + ".rc"
-
-def generate_manifest(config):
- msver = get_build_msvc_version()
- if msver is not None:
- if msver >= 8:
- check_embedded_msvcr_match_linked(msver)
- ma_str, mi_str = str(msver).split('.')
- # Write the manifest file
- manxml = msvc_manifest_xml(int(ma_str), int(mi_str))
- with open(manifest_name(config), "w") as man:
- config.temp_files.append(manifest_name(config))
- man.write(manxml)
diff --git a/spaces/profayle/TerrapinTalk/myenv/lib/python3.9/site-packages/pandas/tests/frame/methods/test_isetitem.py b/spaces/profayle/TerrapinTalk/myenv/lib/python3.9/site-packages/pandas/tests/frame/methods/test_isetitem.py
deleted file mode 100644
index 69f394afb65191fe4cc52519fbc52959d2e1dd76..0000000000000000000000000000000000000000
--- a/spaces/profayle/TerrapinTalk/myenv/lib/python3.9/site-packages/pandas/tests/frame/methods/test_isetitem.py
+++ /dev/null
@@ -1,50 +0,0 @@
-import pytest
-
-from pandas import (
- DataFrame,
- Series,
-)
-import pandas._testing as tm
-
-
-class TestDataFrameSetItem:
- def test_isetitem_ea_df(self):
- # GH#49922
- df = DataFrame([[1, 2, 3], [4, 5, 6]])
- rhs = DataFrame([[11, 12], [13, 14]], dtype="Int64")
-
- df.isetitem([0, 1], rhs)
- expected = DataFrame(
- {
- 0: Series([11, 13], dtype="Int64"),
- 1: Series([12, 14], dtype="Int64"),
- 2: [3, 6],
- }
- )
- tm.assert_frame_equal(df, expected)
-
- def test_isetitem_ea_df_scalar_indexer(self):
- # GH#49922
- df = DataFrame([[1, 2, 3], [4, 5, 6]])
- rhs = DataFrame([[11], [13]], dtype="Int64")
-
- df.isetitem(2, rhs)
- expected = DataFrame(
- {
- 0: [1, 4],
- 1: [2, 5],
- 2: Series([11, 13], dtype="Int64"),
- }
- )
- tm.assert_frame_equal(df, expected)
-
- def test_isetitem_dimension_mismatch(self):
- # GH#51701
- df = DataFrame({"a": [1, 2], "b": [3, 4], "c": [5, 6]})
- value = df.copy()
- with pytest.raises(ValueError, match="Got 2 positions but value has 3 columns"):
- df.isetitem([1, 2], value)
-
- value = df.copy()
- with pytest.raises(ValueError, match="Got 2 positions but value has 1 columns"):
- df.isetitem([1, 2], value[["a"]])
diff --git a/spaces/profayle/TerrapinTalk/myenv/lib/python3.9/site-packages/pandas/tests/io/formats/test_console.py b/spaces/profayle/TerrapinTalk/myenv/lib/python3.9/site-packages/pandas/tests/io/formats/test_console.py
deleted file mode 100644
index dd7b57df9baed18b172dc8398a61a49e9435f82a..0000000000000000000000000000000000000000
--- a/spaces/profayle/TerrapinTalk/myenv/lib/python3.9/site-packages/pandas/tests/io/formats/test_console.py
+++ /dev/null
@@ -1,72 +0,0 @@
-import locale
-
-import pytest
-
-from pandas._config import detect_console_encoding
-
-
-class MockEncoding:
- """
- Used to add a side effect when accessing the 'encoding' property. If the
- side effect is a str in nature, the value will be returned. Otherwise, the
- side effect should be an exception that will be raised.
- """
-
- def __init__(self, encoding) -> None:
- super().__init__()
- self.val = encoding
-
- @property
- def encoding(self):
- return self.raise_or_return(self.val)
-
- @staticmethod
- def raise_or_return(val):
- if isinstance(val, str):
- return val
- else:
- raise val
-
-
-@pytest.mark.parametrize("empty,filled", [["stdin", "stdout"], ["stdout", "stdin"]])
-def test_detect_console_encoding_from_stdout_stdin(monkeypatch, empty, filled):
- # Ensures that when sys.stdout.encoding or sys.stdin.encoding is used when
- # they have values filled.
- # GH 21552
- with monkeypatch.context() as context:
- context.setattr(f"sys.{empty}", MockEncoding(""))
- context.setattr(f"sys.{filled}", MockEncoding(filled))
- assert detect_console_encoding() == filled
-
-
-@pytest.mark.parametrize("encoding", [AttributeError, OSError, "ascii"])
-def test_detect_console_encoding_fallback_to_locale(monkeypatch, encoding):
- # GH 21552
- with monkeypatch.context() as context:
- context.setattr("locale.getpreferredencoding", lambda: "foo")
- context.setattr("sys.stdout", MockEncoding(encoding))
- assert detect_console_encoding() == "foo"
-
-
-@pytest.mark.parametrize(
- "std,locale",
- [
- ["ascii", "ascii"],
- ["ascii", locale.Error],
- [AttributeError, "ascii"],
- [AttributeError, locale.Error],
- [OSError, "ascii"],
- [OSError, locale.Error],
- ],
-)
-def test_detect_console_encoding_fallback_to_default(monkeypatch, std, locale):
- # When both the stdout/stdin encoding and locale preferred encoding checks
- # fail (or return 'ascii', we should default to the sys default encoding.
- # GH 21552
- with monkeypatch.context() as context:
- context.setattr(
- "locale.getpreferredencoding", lambda: MockEncoding.raise_or_return(locale)
- )
- context.setattr("sys.stdout", MockEncoding(std))
- context.setattr("sys.getdefaultencoding", lambda: "sysDefaultEncoding")
- assert detect_console_encoding() == "sysDefaultEncoding"
diff --git a/spaces/profayle/TerrapinTalk/myenv/lib/python3.9/site-packages/pandas/tests/tslibs/test_array_to_datetime.py b/spaces/profayle/TerrapinTalk/myenv/lib/python3.9/site-packages/pandas/tests/tslibs/test_array_to_datetime.py
deleted file mode 100644
index 829bb140e6e968023d4b51d6807ae148a8460a40..0000000000000000000000000000000000000000
--- a/spaces/profayle/TerrapinTalk/myenv/lib/python3.9/site-packages/pandas/tests/tslibs/test_array_to_datetime.py
+++ /dev/null
@@ -1,205 +0,0 @@
-from datetime import (
- date,
- datetime,
- timedelta,
- timezone,
-)
-
-from dateutil.tz.tz import tzoffset
-import numpy as np
-import pytest
-
-from pandas._libs import (
- iNaT,
- tslib,
-)
-
-from pandas import Timestamp
-import pandas._testing as tm
-
-
-@pytest.mark.parametrize(
- "data,expected",
- [
- (
- ["01-01-2013", "01-02-2013"],
- [
- "2013-01-01T00:00:00.000000000",
- "2013-01-02T00:00:00.000000000",
- ],
- ),
- (
- ["Mon Sep 16 2013", "Tue Sep 17 2013"],
- [
- "2013-09-16T00:00:00.000000000",
- "2013-09-17T00:00:00.000000000",
- ],
- ),
- ],
-)
-def test_parsing_valid_dates(data, expected):
- arr = np.array(data, dtype=object)
- result, _ = tslib.array_to_datetime(arr)
-
- expected = np.array(expected, dtype="M8[ns]")
- tm.assert_numpy_array_equal(result, expected)
-
-
-@pytest.mark.parametrize(
- "dt_string, expected_tz",
- [
- ["01-01-2013 08:00:00+08:00", 480],
- ["2013-01-01T08:00:00.000000000+0800", 480],
- ["2012-12-31T16:00:00.000000000-0800", -480],
- ["12-31-2012 23:00:00-01:00", -60],
- ],
-)
-def test_parsing_timezone_offsets(dt_string, expected_tz):
- # All of these datetime strings with offsets are equivalent
- # to the same datetime after the timezone offset is added.
- arr = np.array(["01-01-2013 00:00:00"], dtype=object)
- expected, _ = tslib.array_to_datetime(arr)
-
- arr = np.array([dt_string], dtype=object)
- result, result_tz = tslib.array_to_datetime(arr)
-
- tm.assert_numpy_array_equal(result, expected)
- assert result_tz == timezone(timedelta(minutes=expected_tz))
-
-
-def test_parsing_non_iso_timezone_offset():
- dt_string = "01-01-2013T00:00:00.000000000+0000"
- arr = np.array([dt_string], dtype=object)
-
- with tm.assert_produces_warning(None):
- # GH#50949 should not get tzlocal-deprecation warning here
- result, result_tz = tslib.array_to_datetime(arr)
- expected = np.array([np.datetime64("2013-01-01 00:00:00.000000000")])
-
- tm.assert_numpy_array_equal(result, expected)
- assert result_tz is timezone.utc
-
-
-def test_parsing_different_timezone_offsets():
- # see gh-17697
- data = ["2015-11-18 15:30:00+05:30", "2015-11-18 15:30:00+06:30"]
- data = np.array(data, dtype=object)
-
- msg = "parsing datetimes with mixed time zones will raise an error"
- with tm.assert_produces_warning(FutureWarning, match=msg):
- result, result_tz = tslib.array_to_datetime(data)
- expected = np.array(
- [
- datetime(2015, 11, 18, 15, 30, tzinfo=tzoffset(None, 19800)),
- datetime(2015, 11, 18, 15, 30, tzinfo=tzoffset(None, 23400)),
- ],
- dtype=object,
- )
-
- tm.assert_numpy_array_equal(result, expected)
- assert result_tz is None
-
-
-@pytest.mark.parametrize(
- "data", [["-352.737091", "183.575577"], ["1", "2", "3", "4", "5"]]
-)
-def test_number_looking_strings_not_into_datetime(data):
- # see gh-4601
- #
- # These strings don't look like datetimes, so
- # they shouldn't be attempted to be converted.
- arr = np.array(data, dtype=object)
- result, _ = tslib.array_to_datetime(arr, errors="ignore")
-
- tm.assert_numpy_array_equal(result, arr)
-
-
-@pytest.mark.parametrize(
- "invalid_date",
- [
- date(1000, 1, 1),
- datetime(1000, 1, 1),
- "1000-01-01",
- "Jan 1, 1000",
- np.datetime64("1000-01-01"),
- ],
-)
-@pytest.mark.parametrize("errors", ["coerce", "raise"])
-def test_coerce_outside_ns_bounds(invalid_date, errors):
- arr = np.array([invalid_date], dtype="object")
- kwargs = {"values": arr, "errors": errors}
-
- if errors == "raise":
- msg = "^Out of bounds nanosecond timestamp: .*, at position 0$"
-
- with pytest.raises(ValueError, match=msg):
- tslib.array_to_datetime(**kwargs)
- else: # coerce.
- result, _ = tslib.array_to_datetime(**kwargs)
- expected = np.array([iNaT], dtype="M8[ns]")
-
- tm.assert_numpy_array_equal(result, expected)
-
-
-def test_coerce_outside_ns_bounds_one_valid():
- arr = np.array(["1/1/1000", "1/1/2000"], dtype=object)
- result, _ = tslib.array_to_datetime(arr, errors="coerce")
-
- expected = [iNaT, "2000-01-01T00:00:00.000000000"]
- expected = np.array(expected, dtype="M8[ns]")
-
- tm.assert_numpy_array_equal(result, expected)
-
-
-@pytest.mark.parametrize("errors", ["ignore", "coerce"])
-def test_coerce_of_invalid_datetimes(errors):
- arr = np.array(["01-01-2013", "not_a_date", "1"], dtype=object)
- kwargs = {"values": arr, "errors": errors}
-
- if errors == "ignore":
- # Without coercing, the presence of any invalid
- # dates prevents any values from being converted.
- result, _ = tslib.array_to_datetime(**kwargs)
- tm.assert_numpy_array_equal(result, arr)
- else: # coerce.
- # With coercing, the invalid dates becomes iNaT
- result, _ = tslib.array_to_datetime(arr, errors="coerce")
- expected = ["2013-01-01T00:00:00.000000000", iNaT, iNaT]
-
- tm.assert_numpy_array_equal(result, np.array(expected, dtype="M8[ns]"))
-
-
-def test_to_datetime_barely_out_of_bounds():
- # see gh-19382, gh-19529
- #
- # Close enough to bounds that dropping nanos
- # would result in an in-bounds datetime.
- arr = np.array(["2262-04-11 23:47:16.854775808"], dtype=object)
- msg = "^Out of bounds nanosecond timestamp: 2262-04-11 23:47:16, at position 0$"
-
- with pytest.raises(tslib.OutOfBoundsDatetime, match=msg):
- tslib.array_to_datetime(arr)
-
-
-class SubDatetime(datetime):
- pass
-
-
-@pytest.mark.parametrize(
- "data,expected",
- [
- ([SubDatetime(2000, 1, 1)], ["2000-01-01T00:00:00.000000000"]),
- ([datetime(2000, 1, 1)], ["2000-01-01T00:00:00.000000000"]),
- ([Timestamp(2000, 1, 1)], ["2000-01-01T00:00:00.000000000"]),
- ],
-)
-def test_datetime_subclass(data, expected):
- # GH 25851
- # ensure that subclassed datetime works with
- # array_to_datetime
-
- arr = np.array(data, dtype=object)
- result, _ = tslib.array_to_datetime(arr)
-
- expected = np.array(expected, dtype="M8[ns]")
- tm.assert_numpy_array_equal(result, expected)
diff --git a/spaces/profayle/TerrapinTalk/myenv/lib/python3.9/site-packages/pip/_internal/utils/__init__.py b/spaces/profayle/TerrapinTalk/myenv/lib/python3.9/site-packages/pip/_internal/utils/__init__.py
deleted file mode 100644
index e69de29bb2d1d6434b8b29ae775ad8c2e48c5391..0000000000000000000000000000000000000000
diff --git a/spaces/profayle/TerrapinTalk/myenv/lib/python3.9/site-packages/pip/_vendor/chardet/__init__.py b/spaces/profayle/TerrapinTalk/myenv/lib/python3.9/site-packages/pip/_vendor/chardet/__init__.py
deleted file mode 100644
index 80ad2546d7981394b5f5d221336c9f00236b9d66..0000000000000000000000000000000000000000
--- a/spaces/profayle/TerrapinTalk/myenv/lib/python3.9/site-packages/pip/_vendor/chardet/__init__.py
+++ /dev/null
@@ -1,83 +0,0 @@
-######################## BEGIN LICENSE BLOCK ########################
-# This library is free software; you can redistribute it and/or
-# modify it under the terms of the GNU Lesser General Public
-# License as published by the Free Software Foundation; either
-# version 2.1 of the License, or (at your option) any later version.
-#
-# This library is distributed in the hope that it will be useful,
-# but WITHOUT ANY WARRANTY; without even the implied warranty of
-# MERCHANTABILITY or FITNESS FOR A PARTICULAR PURPOSE. See the GNU
-# Lesser General Public License for more details.
-#
-# You should have received a copy of the GNU Lesser General Public
-# License along with this library; if not, write to the Free Software
-# Foundation, Inc., 51 Franklin St, Fifth Floor, Boston, MA
-# 02110-1301 USA
-######################### END LICENSE BLOCK #########################
-
-
-from .universaldetector import UniversalDetector
-from .enums import InputState
-from .version import __version__, VERSION
-
-
-__all__ = ['UniversalDetector', 'detect', 'detect_all', '__version__', 'VERSION']
-
-
-def detect(byte_str):
- """
- Detect the encoding of the given byte string.
-
- :param byte_str: The byte sequence to examine.
- :type byte_str: ``bytes`` or ``bytearray``
- """
- if not isinstance(byte_str, bytearray):
- if not isinstance(byte_str, bytes):
- raise TypeError('Expected object of type bytes or bytearray, got: '
- '{}'.format(type(byte_str)))
- else:
- byte_str = bytearray(byte_str)
- detector = UniversalDetector()
- detector.feed(byte_str)
- return detector.close()
-
-
-def detect_all(byte_str):
- """
- Detect all the possible encodings of the given byte string.
-
- :param byte_str: The byte sequence to examine.
- :type byte_str: ``bytes`` or ``bytearray``
- """
- if not isinstance(byte_str, bytearray):
- if not isinstance(byte_str, bytes):
- raise TypeError('Expected object of type bytes or bytearray, got: '
- '{}'.format(type(byte_str)))
- else:
- byte_str = bytearray(byte_str)
-
- detector = UniversalDetector()
- detector.feed(byte_str)
- detector.close()
-
- if detector._input_state == InputState.HIGH_BYTE:
- results = []
- for prober in detector._charset_probers:
- if prober.get_confidence() > detector.MINIMUM_THRESHOLD:
- charset_name = prober.charset_name
- lower_charset_name = prober.charset_name.lower()
- # Use Windows encoding name instead of ISO-8859 if we saw any
- # extra Windows-specific bytes
- if lower_charset_name.startswith('iso-8859'):
- if detector._has_win_bytes:
- charset_name = detector.ISO_WIN_MAP.get(lower_charset_name,
- charset_name)
- results.append({
- 'encoding': charset_name,
- 'confidence': prober.get_confidence(),
- 'language': prober.language,
- })
- if len(results) > 0:
- return sorted(results, key=lambda result: -result['confidence'])
-
- return [detector.result]
diff --git a/spaces/profayle/TerrapinTalk/myenv/lib/python3.9/site-packages/pip/_vendor/pygments/lexers/_mapping.py b/spaces/profayle/TerrapinTalk/myenv/lib/python3.9/site-packages/pip/_vendor/pygments/lexers/_mapping.py
deleted file mode 100644
index c972e3a2b3b544f19891325c9fbfcbafca29f40f..0000000000000000000000000000000000000000
--- a/spaces/profayle/TerrapinTalk/myenv/lib/python3.9/site-packages/pip/_vendor/pygments/lexers/_mapping.py
+++ /dev/null
@@ -1,580 +0,0 @@
-"""
- pygments.lexers._mapping
- ~~~~~~~~~~~~~~~~~~~~~~~~
-
- Lexer mapping definitions. This file is generated by itself. Everytime
- you change something on a builtin lexer definition, run this script from
- the lexers folder to update it.
-
- Do not alter the LEXERS dictionary by hand.
-
- :copyright: Copyright 2006-2014, 2016 by the Pygments team, see AUTHORS.
- :license: BSD, see LICENSE for details.
-"""
-
-LEXERS = {
- 'ABAPLexer': ('pip._vendor.pygments.lexers.business', 'ABAP', ('abap',), ('*.abap', '*.ABAP'), ('text/x-abap',)),
- 'AMDGPULexer': ('pip._vendor.pygments.lexers.amdgpu', 'AMDGPU', ('amdgpu',), ('*.isa',), ()),
- 'APLLexer': ('pip._vendor.pygments.lexers.apl', 'APL', ('apl',), ('*.apl', '*.aplf', '*.aplo', '*.apln', '*.aplc', '*.apli', '*.dyalog'), ()),
- 'AbnfLexer': ('pip._vendor.pygments.lexers.grammar_notation', 'ABNF', ('abnf',), ('*.abnf',), ('text/x-abnf',)),
- 'ActionScript3Lexer': ('pip._vendor.pygments.lexers.actionscript', 'ActionScript 3', ('actionscript3', 'as3'), ('*.as',), ('application/x-actionscript3', 'text/x-actionscript3', 'text/actionscript3')),
- 'ActionScriptLexer': ('pip._vendor.pygments.lexers.actionscript', 'ActionScript', ('actionscript', 'as'), ('*.as',), ('application/x-actionscript', 'text/x-actionscript', 'text/actionscript')),
- 'AdaLexer': ('pip._vendor.pygments.lexers.pascal', 'Ada', ('ada', 'ada95', 'ada2005'), ('*.adb', '*.ads', '*.ada'), ('text/x-ada',)),
- 'AdlLexer': ('pip._vendor.pygments.lexers.archetype', 'ADL', ('adl',), ('*.adl', '*.adls', '*.adlf', '*.adlx'), ()),
- 'AgdaLexer': ('pip._vendor.pygments.lexers.haskell', 'Agda', ('agda',), ('*.agda',), ('text/x-agda',)),
- 'AheuiLexer': ('pip._vendor.pygments.lexers.esoteric', 'Aheui', ('aheui',), ('*.aheui',), ()),
- 'AlloyLexer': ('pip._vendor.pygments.lexers.dsls', 'Alloy', ('alloy',), ('*.als',), ('text/x-alloy',)),
- 'AmbientTalkLexer': ('pip._vendor.pygments.lexers.ambient', 'AmbientTalk', ('ambienttalk', 'ambienttalk/2', 'at'), ('*.at',), ('text/x-ambienttalk',)),
- 'AmplLexer': ('pip._vendor.pygments.lexers.ampl', 'Ampl', ('ampl',), ('*.run',), ()),
- 'Angular2HtmlLexer': ('pip._vendor.pygments.lexers.templates', 'HTML + Angular2', ('html+ng2',), ('*.ng2',), ()),
- 'Angular2Lexer': ('pip._vendor.pygments.lexers.templates', 'Angular2', ('ng2',), (), ()),
- 'AntlrActionScriptLexer': ('pip._vendor.pygments.lexers.parsers', 'ANTLR With ActionScript Target', ('antlr-actionscript', 'antlr-as'), ('*.G', '*.g'), ()),
- 'AntlrCSharpLexer': ('pip._vendor.pygments.lexers.parsers', 'ANTLR With C# Target', ('antlr-csharp', 'antlr-c#'), ('*.G', '*.g'), ()),
- 'AntlrCppLexer': ('pip._vendor.pygments.lexers.parsers', 'ANTLR With CPP Target', ('antlr-cpp',), ('*.G', '*.g'), ()),
- 'AntlrJavaLexer': ('pip._vendor.pygments.lexers.parsers', 'ANTLR With Java Target', ('antlr-java',), ('*.G', '*.g'), ()),
- 'AntlrLexer': ('pip._vendor.pygments.lexers.parsers', 'ANTLR', ('antlr',), (), ()),
- 'AntlrObjectiveCLexer': ('pip._vendor.pygments.lexers.parsers', 'ANTLR With ObjectiveC Target', ('antlr-objc',), ('*.G', '*.g'), ()),
- 'AntlrPerlLexer': ('pip._vendor.pygments.lexers.parsers', 'ANTLR With Perl Target', ('antlr-perl',), ('*.G', '*.g'), ()),
- 'AntlrPythonLexer': ('pip._vendor.pygments.lexers.parsers', 'ANTLR With Python Target', ('antlr-python',), ('*.G', '*.g'), ()),
- 'AntlrRubyLexer': ('pip._vendor.pygments.lexers.parsers', 'ANTLR With Ruby Target', ('antlr-ruby', 'antlr-rb'), ('*.G', '*.g'), ()),
- 'ApacheConfLexer': ('pip._vendor.pygments.lexers.configs', 'ApacheConf', ('apacheconf', 'aconf', 'apache'), ('.htaccess', 'apache.conf', 'apache2.conf'), ('text/x-apacheconf',)),
- 'AppleScriptLexer': ('pip._vendor.pygments.lexers.scripting', 'AppleScript', ('applescript',), ('*.applescript',), ()),
- 'ArduinoLexer': ('pip._vendor.pygments.lexers.c_like', 'Arduino', ('arduino',), ('*.ino',), ('text/x-arduino',)),
- 'ArrowLexer': ('pip._vendor.pygments.lexers.arrow', 'Arrow', ('arrow',), ('*.arw',), ()),
- 'AscLexer': ('pip._vendor.pygments.lexers.asc', 'ASCII armored', ('asc', 'pem'), ('*.asc', '*.pem', 'id_dsa', 'id_ecdsa', 'id_ecdsa_sk', 'id_ed25519', 'id_ed25519_sk', 'id_rsa'), ('application/pgp-keys', 'application/pgp-encrypted', 'application/pgp-signature')),
- 'AspectJLexer': ('pip._vendor.pygments.lexers.jvm', 'AspectJ', ('aspectj',), ('*.aj',), ('text/x-aspectj',)),
- 'AsymptoteLexer': ('pip._vendor.pygments.lexers.graphics', 'Asymptote', ('asymptote', 'asy'), ('*.asy',), ('text/x-asymptote',)),
- 'AugeasLexer': ('pip._vendor.pygments.lexers.configs', 'Augeas', ('augeas',), ('*.aug',), ()),
- 'AutoItLexer': ('pip._vendor.pygments.lexers.automation', 'AutoIt', ('autoit',), ('*.au3',), ('text/x-autoit',)),
- 'AutohotkeyLexer': ('pip._vendor.pygments.lexers.automation', 'autohotkey', ('autohotkey', 'ahk'), ('*.ahk', '*.ahkl'), ('text/x-autohotkey',)),
- 'AwkLexer': ('pip._vendor.pygments.lexers.textedit', 'Awk', ('awk', 'gawk', 'mawk', 'nawk'), ('*.awk',), ('application/x-awk',)),
- 'BBCBasicLexer': ('pip._vendor.pygments.lexers.basic', 'BBC Basic', ('bbcbasic',), ('*.bbc',), ()),
- 'BBCodeLexer': ('pip._vendor.pygments.lexers.markup', 'BBCode', ('bbcode',), (), ('text/x-bbcode',)),
- 'BCLexer': ('pip._vendor.pygments.lexers.algebra', 'BC', ('bc',), ('*.bc',), ()),
- 'BSTLexer': ('pip._vendor.pygments.lexers.bibtex', 'BST', ('bst', 'bst-pybtex'), ('*.bst',), ()),
- 'BareLexer': ('pip._vendor.pygments.lexers.bare', 'BARE', ('bare',), ('*.bare',), ()),
- 'BaseMakefileLexer': ('pip._vendor.pygments.lexers.make', 'Base Makefile', ('basemake',), (), ()),
- 'BashLexer': ('pip._vendor.pygments.lexers.shell', 'Bash', ('bash', 'sh', 'ksh', 'zsh', 'shell'), ('*.sh', '*.ksh', '*.bash', '*.ebuild', '*.eclass', '*.exheres-0', '*.exlib', '*.zsh', '.bashrc', 'bashrc', '.bash_*', 'bash_*', 'zshrc', '.zshrc', '.kshrc', 'kshrc', 'PKGBUILD'), ('application/x-sh', 'application/x-shellscript', 'text/x-shellscript')),
- 'BashSessionLexer': ('pip._vendor.pygments.lexers.shell', 'Bash Session', ('console', 'shell-session'), ('*.sh-session', '*.shell-session'), ('application/x-shell-session', 'application/x-sh-session')),
- 'BatchLexer': ('pip._vendor.pygments.lexers.shell', 'Batchfile', ('batch', 'bat', 'dosbatch', 'winbatch'), ('*.bat', '*.cmd'), ('application/x-dos-batch',)),
- 'BddLexer': ('pip._vendor.pygments.lexers.bdd', 'Bdd', ('bdd',), ('*.feature',), ('text/x-bdd',)),
- 'BefungeLexer': ('pip._vendor.pygments.lexers.esoteric', 'Befunge', ('befunge',), ('*.befunge',), ('application/x-befunge',)),
- 'BibTeXLexer': ('pip._vendor.pygments.lexers.bibtex', 'BibTeX', ('bibtex', 'bib'), ('*.bib',), ('text/x-bibtex',)),
- 'BlitzBasicLexer': ('pip._vendor.pygments.lexers.basic', 'BlitzBasic', ('blitzbasic', 'b3d', 'bplus'), ('*.bb', '*.decls'), ('text/x-bb',)),
- 'BlitzMaxLexer': ('pip._vendor.pygments.lexers.basic', 'BlitzMax', ('blitzmax', 'bmax'), ('*.bmx',), ('text/x-bmx',)),
- 'BnfLexer': ('pip._vendor.pygments.lexers.grammar_notation', 'BNF', ('bnf',), ('*.bnf',), ('text/x-bnf',)),
- 'BoaLexer': ('pip._vendor.pygments.lexers.boa', 'Boa', ('boa',), ('*.boa',), ()),
- 'BooLexer': ('pip._vendor.pygments.lexers.dotnet', 'Boo', ('boo',), ('*.boo',), ('text/x-boo',)),
- 'BoogieLexer': ('pip._vendor.pygments.lexers.verification', 'Boogie', ('boogie',), ('*.bpl',), ()),
- 'BrainfuckLexer': ('pip._vendor.pygments.lexers.esoteric', 'Brainfuck', ('brainfuck', 'bf'), ('*.bf', '*.b'), ('application/x-brainfuck',)),
- 'BugsLexer': ('pip._vendor.pygments.lexers.modeling', 'BUGS', ('bugs', 'winbugs', 'openbugs'), ('*.bug',), ()),
- 'CAmkESLexer': ('pip._vendor.pygments.lexers.esoteric', 'CAmkES', ('camkes', 'idl4'), ('*.camkes', '*.idl4'), ()),
- 'CLexer': ('pip._vendor.pygments.lexers.c_cpp', 'C', ('c',), ('*.c', '*.h', '*.idc', '*.x[bp]m'), ('text/x-chdr', 'text/x-csrc', 'image/x-xbitmap', 'image/x-xpixmap')),
- 'CMakeLexer': ('pip._vendor.pygments.lexers.make', 'CMake', ('cmake',), ('*.cmake', 'CMakeLists.txt'), ('text/x-cmake',)),
- 'CObjdumpLexer': ('pip._vendor.pygments.lexers.asm', 'c-objdump', ('c-objdump',), ('*.c-objdump',), ('text/x-c-objdump',)),
- 'CPSALexer': ('pip._vendor.pygments.lexers.lisp', 'CPSA', ('cpsa',), ('*.cpsa',), ()),
- 'CSharpAspxLexer': ('pip._vendor.pygments.lexers.dotnet', 'aspx-cs', ('aspx-cs',), ('*.aspx', '*.asax', '*.ascx', '*.ashx', '*.asmx', '*.axd'), ()),
- 'CSharpLexer': ('pip._vendor.pygments.lexers.dotnet', 'C#', ('csharp', 'c#', 'cs'), ('*.cs',), ('text/x-csharp',)),
- 'Ca65Lexer': ('pip._vendor.pygments.lexers.asm', 'ca65 assembler', ('ca65',), ('*.s',), ()),
- 'CadlLexer': ('pip._vendor.pygments.lexers.archetype', 'cADL', ('cadl',), ('*.cadl',), ()),
- 'CapDLLexer': ('pip._vendor.pygments.lexers.esoteric', 'CapDL', ('capdl',), ('*.cdl',), ()),
- 'CapnProtoLexer': ('pip._vendor.pygments.lexers.capnproto', "Cap'n Proto", ('capnp',), ('*.capnp',), ()),
- 'CbmBasicV2Lexer': ('pip._vendor.pygments.lexers.basic', 'CBM BASIC V2', ('cbmbas',), ('*.bas',), ()),
- 'CddlLexer': ('pip._vendor.pygments.lexers.cddl', 'CDDL', ('cddl',), ('*.cddl',), ('text/x-cddl',)),
- 'CeylonLexer': ('pip._vendor.pygments.lexers.jvm', 'Ceylon', ('ceylon',), ('*.ceylon',), ('text/x-ceylon',)),
- 'Cfengine3Lexer': ('pip._vendor.pygments.lexers.configs', 'CFEngine3', ('cfengine3', 'cf3'), ('*.cf',), ()),
- 'ChaiscriptLexer': ('pip._vendor.pygments.lexers.scripting', 'ChaiScript', ('chaiscript', 'chai'), ('*.chai',), ('text/x-chaiscript', 'application/x-chaiscript')),
- 'ChapelLexer': ('pip._vendor.pygments.lexers.chapel', 'Chapel', ('chapel', 'chpl'), ('*.chpl',), ()),
- 'CharmciLexer': ('pip._vendor.pygments.lexers.c_like', 'Charmci', ('charmci',), ('*.ci',), ()),
- 'CheetahHtmlLexer': ('pip._vendor.pygments.lexers.templates', 'HTML+Cheetah', ('html+cheetah', 'html+spitfire', 'htmlcheetah'), (), ('text/html+cheetah', 'text/html+spitfire')),
- 'CheetahJavascriptLexer': ('pip._vendor.pygments.lexers.templates', 'JavaScript+Cheetah', ('javascript+cheetah', 'js+cheetah', 'javascript+spitfire', 'js+spitfire'), (), ('application/x-javascript+cheetah', 'text/x-javascript+cheetah', 'text/javascript+cheetah', 'application/x-javascript+spitfire', 'text/x-javascript+spitfire', 'text/javascript+spitfire')),
- 'CheetahLexer': ('pip._vendor.pygments.lexers.templates', 'Cheetah', ('cheetah', 'spitfire'), ('*.tmpl', '*.spt'), ('application/x-cheetah', 'application/x-spitfire')),
- 'CheetahXmlLexer': ('pip._vendor.pygments.lexers.templates', 'XML+Cheetah', ('xml+cheetah', 'xml+spitfire'), (), ('application/xml+cheetah', 'application/xml+spitfire')),
- 'CirruLexer': ('pip._vendor.pygments.lexers.webmisc', 'Cirru', ('cirru',), ('*.cirru',), ('text/x-cirru',)),
- 'ClayLexer': ('pip._vendor.pygments.lexers.c_like', 'Clay', ('clay',), ('*.clay',), ('text/x-clay',)),
- 'CleanLexer': ('pip._vendor.pygments.lexers.clean', 'Clean', ('clean',), ('*.icl', '*.dcl'), ()),
- 'ClojureLexer': ('pip._vendor.pygments.lexers.jvm', 'Clojure', ('clojure', 'clj'), ('*.clj',), ('text/x-clojure', 'application/x-clojure')),
- 'ClojureScriptLexer': ('pip._vendor.pygments.lexers.jvm', 'ClojureScript', ('clojurescript', 'cljs'), ('*.cljs',), ('text/x-clojurescript', 'application/x-clojurescript')),
- 'CobolFreeformatLexer': ('pip._vendor.pygments.lexers.business', 'COBOLFree', ('cobolfree',), ('*.cbl', '*.CBL'), ()),
- 'CobolLexer': ('pip._vendor.pygments.lexers.business', 'COBOL', ('cobol',), ('*.cob', '*.COB', '*.cpy', '*.CPY'), ('text/x-cobol',)),
- 'CoffeeScriptLexer': ('pip._vendor.pygments.lexers.javascript', 'CoffeeScript', ('coffeescript', 'coffee-script', 'coffee'), ('*.coffee',), ('text/coffeescript',)),
- 'ColdfusionCFCLexer': ('pip._vendor.pygments.lexers.templates', 'Coldfusion CFC', ('cfc',), ('*.cfc',), ()),
- 'ColdfusionHtmlLexer': ('pip._vendor.pygments.lexers.templates', 'Coldfusion HTML', ('cfm',), ('*.cfm', '*.cfml'), ('application/x-coldfusion',)),
- 'ColdfusionLexer': ('pip._vendor.pygments.lexers.templates', 'cfstatement', ('cfs',), (), ()),
- 'CommonLispLexer': ('pip._vendor.pygments.lexers.lisp', 'Common Lisp', ('common-lisp', 'cl', 'lisp'), ('*.cl', '*.lisp'), ('text/x-common-lisp',)),
- 'ComponentPascalLexer': ('pip._vendor.pygments.lexers.oberon', 'Component Pascal', ('componentpascal', 'cp'), ('*.cp', '*.cps'), ('text/x-component-pascal',)),
- 'CoqLexer': ('pip._vendor.pygments.lexers.theorem', 'Coq', ('coq',), ('*.v',), ('text/x-coq',)),
- 'CppLexer': ('pip._vendor.pygments.lexers.c_cpp', 'C++', ('cpp', 'c++'), ('*.cpp', '*.hpp', '*.c++', '*.h++', '*.cc', '*.hh', '*.cxx', '*.hxx', '*.C', '*.H', '*.cp', '*.CPP'), ('text/x-c++hdr', 'text/x-c++src')),
- 'CppObjdumpLexer': ('pip._vendor.pygments.lexers.asm', 'cpp-objdump', ('cpp-objdump', 'c++-objdumb', 'cxx-objdump'), ('*.cpp-objdump', '*.c++-objdump', '*.cxx-objdump'), ('text/x-cpp-objdump',)),
- 'CrmshLexer': ('pip._vendor.pygments.lexers.dsls', 'Crmsh', ('crmsh', 'pcmk'), ('*.crmsh', '*.pcmk'), ()),
- 'CrocLexer': ('pip._vendor.pygments.lexers.d', 'Croc', ('croc',), ('*.croc',), ('text/x-crocsrc',)),
- 'CryptolLexer': ('pip._vendor.pygments.lexers.haskell', 'Cryptol', ('cryptol', 'cry'), ('*.cry',), ('text/x-cryptol',)),
- 'CrystalLexer': ('pip._vendor.pygments.lexers.crystal', 'Crystal', ('cr', 'crystal'), ('*.cr',), ('text/x-crystal',)),
- 'CsoundDocumentLexer': ('pip._vendor.pygments.lexers.csound', 'Csound Document', ('csound-document', 'csound-csd'), ('*.csd',), ()),
- 'CsoundOrchestraLexer': ('pip._vendor.pygments.lexers.csound', 'Csound Orchestra', ('csound', 'csound-orc'), ('*.orc', '*.udo'), ()),
- 'CsoundScoreLexer': ('pip._vendor.pygments.lexers.csound', 'Csound Score', ('csound-score', 'csound-sco'), ('*.sco',), ()),
- 'CssDjangoLexer': ('pip._vendor.pygments.lexers.templates', 'CSS+Django/Jinja', ('css+django', 'css+jinja'), (), ('text/css+django', 'text/css+jinja')),
- 'CssErbLexer': ('pip._vendor.pygments.lexers.templates', 'CSS+Ruby', ('css+ruby', 'css+erb'), (), ('text/css+ruby',)),
- 'CssGenshiLexer': ('pip._vendor.pygments.lexers.templates', 'CSS+Genshi Text', ('css+genshitext', 'css+genshi'), (), ('text/css+genshi',)),
- 'CssLexer': ('pip._vendor.pygments.lexers.css', 'CSS', ('css',), ('*.css',), ('text/css',)),
- 'CssPhpLexer': ('pip._vendor.pygments.lexers.templates', 'CSS+PHP', ('css+php',), (), ('text/css+php',)),
- 'CssSmartyLexer': ('pip._vendor.pygments.lexers.templates', 'CSS+Smarty', ('css+smarty',), (), ('text/css+smarty',)),
- 'CudaLexer': ('pip._vendor.pygments.lexers.c_like', 'CUDA', ('cuda', 'cu'), ('*.cu', '*.cuh'), ('text/x-cuda',)),
- 'CypherLexer': ('pip._vendor.pygments.lexers.graph', 'Cypher', ('cypher',), ('*.cyp', '*.cypher'), ()),
- 'CythonLexer': ('pip._vendor.pygments.lexers.python', 'Cython', ('cython', 'pyx', 'pyrex'), ('*.pyx', '*.pxd', '*.pxi'), ('text/x-cython', 'application/x-cython')),
- 'DLexer': ('pip._vendor.pygments.lexers.d', 'D', ('d',), ('*.d', '*.di'), ('text/x-dsrc',)),
- 'DObjdumpLexer': ('pip._vendor.pygments.lexers.asm', 'd-objdump', ('d-objdump',), ('*.d-objdump',), ('text/x-d-objdump',)),
- 'DarcsPatchLexer': ('pip._vendor.pygments.lexers.diff', 'Darcs Patch', ('dpatch',), ('*.dpatch', '*.darcspatch'), ()),
- 'DartLexer': ('pip._vendor.pygments.lexers.javascript', 'Dart', ('dart',), ('*.dart',), ('text/x-dart',)),
- 'Dasm16Lexer': ('pip._vendor.pygments.lexers.asm', 'DASM16', ('dasm16',), ('*.dasm16', '*.dasm'), ('text/x-dasm16',)),
- 'DebianControlLexer': ('pip._vendor.pygments.lexers.installers', 'Debian Control file', ('debcontrol', 'control'), ('control',), ()),
- 'DelphiLexer': ('pip._vendor.pygments.lexers.pascal', 'Delphi', ('delphi', 'pas', 'pascal', 'objectpascal'), ('*.pas', '*.dpr'), ('text/x-pascal',)),
- 'DevicetreeLexer': ('pip._vendor.pygments.lexers.devicetree', 'Devicetree', ('devicetree', 'dts'), ('*.dts', '*.dtsi'), ('text/x-c',)),
- 'DgLexer': ('pip._vendor.pygments.lexers.python', 'dg', ('dg',), ('*.dg',), ('text/x-dg',)),
- 'DiffLexer': ('pip._vendor.pygments.lexers.diff', 'Diff', ('diff', 'udiff'), ('*.diff', '*.patch'), ('text/x-diff', 'text/x-patch')),
- 'DjangoLexer': ('pip._vendor.pygments.lexers.templates', 'Django/Jinja', ('django', 'jinja'), (), ('application/x-django-templating', 'application/x-jinja')),
- 'DockerLexer': ('pip._vendor.pygments.lexers.configs', 'Docker', ('docker', 'dockerfile'), ('Dockerfile', '*.docker'), ('text/x-dockerfile-config',)),
- 'DtdLexer': ('pip._vendor.pygments.lexers.html', 'DTD', ('dtd',), ('*.dtd',), ('application/xml-dtd',)),
- 'DuelLexer': ('pip._vendor.pygments.lexers.webmisc', 'Duel', ('duel', 'jbst', 'jsonml+bst'), ('*.duel', '*.jbst'), ('text/x-duel', 'text/x-jbst')),
- 'DylanConsoleLexer': ('pip._vendor.pygments.lexers.dylan', 'Dylan session', ('dylan-console', 'dylan-repl'), ('*.dylan-console',), ('text/x-dylan-console',)),
- 'DylanLexer': ('pip._vendor.pygments.lexers.dylan', 'Dylan', ('dylan',), ('*.dylan', '*.dyl', '*.intr'), ('text/x-dylan',)),
- 'DylanLidLexer': ('pip._vendor.pygments.lexers.dylan', 'DylanLID', ('dylan-lid', 'lid'), ('*.lid', '*.hdp'), ('text/x-dylan-lid',)),
- 'ECLLexer': ('pip._vendor.pygments.lexers.ecl', 'ECL', ('ecl',), ('*.ecl',), ('application/x-ecl',)),
- 'ECLexer': ('pip._vendor.pygments.lexers.c_like', 'eC', ('ec',), ('*.ec', '*.eh'), ('text/x-echdr', 'text/x-ecsrc')),
- 'EarlGreyLexer': ('pip._vendor.pygments.lexers.javascript', 'Earl Grey', ('earl-grey', 'earlgrey', 'eg'), ('*.eg',), ('text/x-earl-grey',)),
- 'EasytrieveLexer': ('pip._vendor.pygments.lexers.scripting', 'Easytrieve', ('easytrieve',), ('*.ezt', '*.mac'), ('text/x-easytrieve',)),
- 'EbnfLexer': ('pip._vendor.pygments.lexers.parsers', 'EBNF', ('ebnf',), ('*.ebnf',), ('text/x-ebnf',)),
- 'EiffelLexer': ('pip._vendor.pygments.lexers.eiffel', 'Eiffel', ('eiffel',), ('*.e',), ('text/x-eiffel',)),
- 'ElixirConsoleLexer': ('pip._vendor.pygments.lexers.erlang', 'Elixir iex session', ('iex',), (), ('text/x-elixir-shellsession',)),
- 'ElixirLexer': ('pip._vendor.pygments.lexers.erlang', 'Elixir', ('elixir', 'ex', 'exs'), ('*.ex', '*.eex', '*.exs', '*.leex'), ('text/x-elixir',)),
- 'ElmLexer': ('pip._vendor.pygments.lexers.elm', 'Elm', ('elm',), ('*.elm',), ('text/x-elm',)),
- 'ElpiLexer': ('pip._vendor.pygments.lexers.elpi', 'Elpi', ('elpi',), ('*.elpi',), ('text/x-elpi',)),
- 'EmacsLispLexer': ('pip._vendor.pygments.lexers.lisp', 'EmacsLisp', ('emacs-lisp', 'elisp', 'emacs'), ('*.el',), ('text/x-elisp', 'application/x-elisp')),
- 'EmailLexer': ('pip._vendor.pygments.lexers.email', 'E-mail', ('email', 'eml'), ('*.eml',), ('message/rfc822',)),
- 'ErbLexer': ('pip._vendor.pygments.lexers.templates', 'ERB', ('erb',), (), ('application/x-ruby-templating',)),
- 'ErlangLexer': ('pip._vendor.pygments.lexers.erlang', 'Erlang', ('erlang',), ('*.erl', '*.hrl', '*.es', '*.escript'), ('text/x-erlang',)),
- 'ErlangShellLexer': ('pip._vendor.pygments.lexers.erlang', 'Erlang erl session', ('erl',), ('*.erl-sh',), ('text/x-erl-shellsession',)),
- 'EvoqueHtmlLexer': ('pip._vendor.pygments.lexers.templates', 'HTML+Evoque', ('html+evoque',), ('*.html',), ('text/html+evoque',)),
- 'EvoqueLexer': ('pip._vendor.pygments.lexers.templates', 'Evoque', ('evoque',), ('*.evoque',), ('application/x-evoque',)),
- 'EvoqueXmlLexer': ('pip._vendor.pygments.lexers.templates', 'XML+Evoque', ('xml+evoque',), ('*.xml',), ('application/xml+evoque',)),
- 'ExeclineLexer': ('pip._vendor.pygments.lexers.shell', 'execline', ('execline',), ('*.exec',), ()),
- 'EzhilLexer': ('pip._vendor.pygments.lexers.ezhil', 'Ezhil', ('ezhil',), ('*.n',), ('text/x-ezhil',)),
- 'FSharpLexer': ('pip._vendor.pygments.lexers.dotnet', 'F#', ('fsharp', 'f#'), ('*.fs', '*.fsi'), ('text/x-fsharp',)),
- 'FStarLexer': ('pip._vendor.pygments.lexers.ml', 'FStar', ('fstar',), ('*.fst', '*.fsti'), ('text/x-fstar',)),
- 'FactorLexer': ('pip._vendor.pygments.lexers.factor', 'Factor', ('factor',), ('*.factor',), ('text/x-factor',)),
- 'FancyLexer': ('pip._vendor.pygments.lexers.ruby', 'Fancy', ('fancy', 'fy'), ('*.fy', '*.fancypack'), ('text/x-fancysrc',)),
- 'FantomLexer': ('pip._vendor.pygments.lexers.fantom', 'Fantom', ('fan',), ('*.fan',), ('application/x-fantom',)),
- 'FelixLexer': ('pip._vendor.pygments.lexers.felix', 'Felix', ('felix', 'flx'), ('*.flx', '*.flxh'), ('text/x-felix',)),
- 'FennelLexer': ('pip._vendor.pygments.lexers.lisp', 'Fennel', ('fennel', 'fnl'), ('*.fnl',), ()),
- 'FishShellLexer': ('pip._vendor.pygments.lexers.shell', 'Fish', ('fish', 'fishshell'), ('*.fish', '*.load'), ('application/x-fish',)),
- 'FlatlineLexer': ('pip._vendor.pygments.lexers.dsls', 'Flatline', ('flatline',), (), ('text/x-flatline',)),
- 'FloScriptLexer': ('pip._vendor.pygments.lexers.floscript', 'FloScript', ('floscript', 'flo'), ('*.flo',), ()),
- 'ForthLexer': ('pip._vendor.pygments.lexers.forth', 'Forth', ('forth',), ('*.frt', '*.fs'), ('application/x-forth',)),
- 'FortranFixedLexer': ('pip._vendor.pygments.lexers.fortran', 'FortranFixed', ('fortranfixed',), ('*.f', '*.F'), ()),
- 'FortranLexer': ('pip._vendor.pygments.lexers.fortran', 'Fortran', ('fortran', 'f90'), ('*.f03', '*.f90', '*.F03', '*.F90'), ('text/x-fortran',)),
- 'FoxProLexer': ('pip._vendor.pygments.lexers.foxpro', 'FoxPro', ('foxpro', 'vfp', 'clipper', 'xbase'), ('*.PRG', '*.prg'), ()),
- 'FreeFemLexer': ('pip._vendor.pygments.lexers.freefem', 'Freefem', ('freefem',), ('*.edp',), ('text/x-freefem',)),
- 'FutharkLexer': ('pip._vendor.pygments.lexers.futhark', 'Futhark', ('futhark',), ('*.fut',), ('text/x-futhark',)),
- 'GAPLexer': ('pip._vendor.pygments.lexers.algebra', 'GAP', ('gap',), ('*.g', '*.gd', '*.gi', '*.gap'), ()),
- 'GDScriptLexer': ('pip._vendor.pygments.lexers.gdscript', 'GDScript', ('gdscript', 'gd'), ('*.gd',), ('text/x-gdscript', 'application/x-gdscript')),
- 'GLShaderLexer': ('pip._vendor.pygments.lexers.graphics', 'GLSL', ('glsl',), ('*.vert', '*.frag', '*.geo'), ('text/x-glslsrc',)),
- 'GSQLLexer': ('pip._vendor.pygments.lexers.gsql', 'GSQL', ('gsql',), ('*.gsql',), ()),
- 'GasLexer': ('pip._vendor.pygments.lexers.asm', 'GAS', ('gas', 'asm'), ('*.s', '*.S'), ('text/x-gas',)),
- 'GcodeLexer': ('pip._vendor.pygments.lexers.gcodelexer', 'g-code', ('gcode',), ('*.gcode',), ()),
- 'GenshiLexer': ('pip._vendor.pygments.lexers.templates', 'Genshi', ('genshi', 'kid', 'xml+genshi', 'xml+kid'), ('*.kid',), ('application/x-genshi', 'application/x-kid')),
- 'GenshiTextLexer': ('pip._vendor.pygments.lexers.templates', 'Genshi Text', ('genshitext',), (), ('application/x-genshi-text', 'text/x-genshi')),
- 'GettextLexer': ('pip._vendor.pygments.lexers.textfmts', 'Gettext Catalog', ('pot', 'po'), ('*.pot', '*.po'), ('application/x-gettext', 'text/x-gettext', 'text/gettext')),
- 'GherkinLexer': ('pip._vendor.pygments.lexers.testing', 'Gherkin', ('gherkin', 'cucumber'), ('*.feature',), ('text/x-gherkin',)),
- 'GnuplotLexer': ('pip._vendor.pygments.lexers.graphics', 'Gnuplot', ('gnuplot',), ('*.plot', '*.plt'), ('text/x-gnuplot',)),
- 'GoLexer': ('pip._vendor.pygments.lexers.go', 'Go', ('go', 'golang'), ('*.go',), ('text/x-gosrc',)),
- 'GoloLexer': ('pip._vendor.pygments.lexers.jvm', 'Golo', ('golo',), ('*.golo',), ()),
- 'GoodDataCLLexer': ('pip._vendor.pygments.lexers.business', 'GoodData-CL', ('gooddata-cl',), ('*.gdc',), ('text/x-gooddata-cl',)),
- 'GosuLexer': ('pip._vendor.pygments.lexers.jvm', 'Gosu', ('gosu',), ('*.gs', '*.gsx', '*.gsp', '*.vark'), ('text/x-gosu',)),
- 'GosuTemplateLexer': ('pip._vendor.pygments.lexers.jvm', 'Gosu Template', ('gst',), ('*.gst',), ('text/x-gosu-template',)),
- 'GraphvizLexer': ('pip._vendor.pygments.lexers.graphviz', 'Graphviz', ('graphviz', 'dot'), ('*.gv', '*.dot'), ('text/x-graphviz', 'text/vnd.graphviz')),
- 'GroffLexer': ('pip._vendor.pygments.lexers.markup', 'Groff', ('groff', 'nroff', 'man'), ('*.[1-9]', '*.man', '*.1p', '*.3pm'), ('application/x-troff', 'text/troff')),
- 'GroovyLexer': ('pip._vendor.pygments.lexers.jvm', 'Groovy', ('groovy',), ('*.groovy', '*.gradle'), ('text/x-groovy',)),
- 'HLSLShaderLexer': ('pip._vendor.pygments.lexers.graphics', 'HLSL', ('hlsl',), ('*.hlsl', '*.hlsli'), ('text/x-hlsl',)),
- 'HamlLexer': ('pip._vendor.pygments.lexers.html', 'Haml', ('haml',), ('*.haml',), ('text/x-haml',)),
- 'HandlebarsHtmlLexer': ('pip._vendor.pygments.lexers.templates', 'HTML+Handlebars', ('html+handlebars',), ('*.handlebars', '*.hbs'), ('text/html+handlebars', 'text/x-handlebars-template')),
- 'HandlebarsLexer': ('pip._vendor.pygments.lexers.templates', 'Handlebars', ('handlebars',), (), ()),
- 'HaskellLexer': ('pip._vendor.pygments.lexers.haskell', 'Haskell', ('haskell', 'hs'), ('*.hs',), ('text/x-haskell',)),
- 'HaxeLexer': ('pip._vendor.pygments.lexers.haxe', 'Haxe', ('haxe', 'hxsl', 'hx'), ('*.hx', '*.hxsl'), ('text/haxe', 'text/x-haxe', 'text/x-hx')),
- 'HexdumpLexer': ('pip._vendor.pygments.lexers.hexdump', 'Hexdump', ('hexdump',), (), ()),
- 'HsailLexer': ('pip._vendor.pygments.lexers.asm', 'HSAIL', ('hsail', 'hsa'), ('*.hsail',), ('text/x-hsail',)),
- 'HspecLexer': ('pip._vendor.pygments.lexers.haskell', 'Hspec', ('hspec',), (), ()),
- 'HtmlDjangoLexer': ('pip._vendor.pygments.lexers.templates', 'HTML+Django/Jinja', ('html+django', 'html+jinja', 'htmldjango'), (), ('text/html+django', 'text/html+jinja')),
- 'HtmlGenshiLexer': ('pip._vendor.pygments.lexers.templates', 'HTML+Genshi', ('html+genshi', 'html+kid'), (), ('text/html+genshi',)),
- 'HtmlLexer': ('pip._vendor.pygments.lexers.html', 'HTML', ('html',), ('*.html', '*.htm', '*.xhtml', '*.xslt'), ('text/html', 'application/xhtml+xml')),
- 'HtmlPhpLexer': ('pip._vendor.pygments.lexers.templates', 'HTML+PHP', ('html+php',), ('*.phtml',), ('application/x-php', 'application/x-httpd-php', 'application/x-httpd-php3', 'application/x-httpd-php4', 'application/x-httpd-php5')),
- 'HtmlSmartyLexer': ('pip._vendor.pygments.lexers.templates', 'HTML+Smarty', ('html+smarty',), (), ('text/html+smarty',)),
- 'HttpLexer': ('pip._vendor.pygments.lexers.textfmts', 'HTTP', ('http',), (), ()),
- 'HxmlLexer': ('pip._vendor.pygments.lexers.haxe', 'Hxml', ('haxeml', 'hxml'), ('*.hxml',), ()),
- 'HyLexer': ('pip._vendor.pygments.lexers.lisp', 'Hy', ('hylang',), ('*.hy',), ('text/x-hy', 'application/x-hy')),
- 'HybrisLexer': ('pip._vendor.pygments.lexers.scripting', 'Hybris', ('hybris', 'hy'), ('*.hy', '*.hyb'), ('text/x-hybris', 'application/x-hybris')),
- 'IDLLexer': ('pip._vendor.pygments.lexers.idl', 'IDL', ('idl',), ('*.pro',), ('text/idl',)),
- 'IconLexer': ('pip._vendor.pygments.lexers.unicon', 'Icon', ('icon',), ('*.icon', '*.ICON'), ()),
- 'IdrisLexer': ('pip._vendor.pygments.lexers.haskell', 'Idris', ('idris', 'idr'), ('*.idr',), ('text/x-idris',)),
- 'IgorLexer': ('pip._vendor.pygments.lexers.igor', 'Igor', ('igor', 'igorpro'), ('*.ipf',), ('text/ipf',)),
- 'Inform6Lexer': ('pip._vendor.pygments.lexers.int_fiction', 'Inform 6', ('inform6', 'i6'), ('*.inf',), ()),
- 'Inform6TemplateLexer': ('pip._vendor.pygments.lexers.int_fiction', 'Inform 6 template', ('i6t',), ('*.i6t',), ()),
- 'Inform7Lexer': ('pip._vendor.pygments.lexers.int_fiction', 'Inform 7', ('inform7', 'i7'), ('*.ni', '*.i7x'), ()),
- 'IniLexer': ('pip._vendor.pygments.lexers.configs', 'INI', ('ini', 'cfg', 'dosini'), ('*.ini', '*.cfg', '*.inf', '.editorconfig', '*.service', '*.socket', '*.device', '*.mount', '*.automount', '*.swap', '*.target', '*.path', '*.timer', '*.slice', '*.scope'), ('text/x-ini', 'text/inf')),
- 'IoLexer': ('pip._vendor.pygments.lexers.iolang', 'Io', ('io',), ('*.io',), ('text/x-iosrc',)),
- 'IokeLexer': ('pip._vendor.pygments.lexers.jvm', 'Ioke', ('ioke', 'ik'), ('*.ik',), ('text/x-iokesrc',)),
- 'IrcLogsLexer': ('pip._vendor.pygments.lexers.textfmts', 'IRC logs', ('irc',), ('*.weechatlog',), ('text/x-irclog',)),
- 'IsabelleLexer': ('pip._vendor.pygments.lexers.theorem', 'Isabelle', ('isabelle',), ('*.thy',), ('text/x-isabelle',)),
- 'JLexer': ('pip._vendor.pygments.lexers.j', 'J', ('j',), ('*.ijs',), ('text/x-j',)),
- 'JSLTLexer': ('pip._vendor.pygments.lexers.jslt', 'JSLT', ('jslt',), ('*.jslt',), ('text/x-jslt',)),
- 'JagsLexer': ('pip._vendor.pygments.lexers.modeling', 'JAGS', ('jags',), ('*.jag', '*.bug'), ()),
- 'JasminLexer': ('pip._vendor.pygments.lexers.jvm', 'Jasmin', ('jasmin', 'jasminxt'), ('*.j',), ()),
- 'JavaLexer': ('pip._vendor.pygments.lexers.jvm', 'Java', ('java',), ('*.java',), ('text/x-java',)),
- 'JavascriptDjangoLexer': ('pip._vendor.pygments.lexers.templates', 'JavaScript+Django/Jinja', ('javascript+django', 'js+django', 'javascript+jinja', 'js+jinja'), (), ('application/x-javascript+django', 'application/x-javascript+jinja', 'text/x-javascript+django', 'text/x-javascript+jinja', 'text/javascript+django', 'text/javascript+jinja')),
- 'JavascriptErbLexer': ('pip._vendor.pygments.lexers.templates', 'JavaScript+Ruby', ('javascript+ruby', 'js+ruby', 'javascript+erb', 'js+erb'), (), ('application/x-javascript+ruby', 'text/x-javascript+ruby', 'text/javascript+ruby')),
- 'JavascriptGenshiLexer': ('pip._vendor.pygments.lexers.templates', 'JavaScript+Genshi Text', ('js+genshitext', 'js+genshi', 'javascript+genshitext', 'javascript+genshi'), (), ('application/x-javascript+genshi', 'text/x-javascript+genshi', 'text/javascript+genshi')),
- 'JavascriptLexer': ('pip._vendor.pygments.lexers.javascript', 'JavaScript', ('javascript', 'js'), ('*.js', '*.jsm', '*.mjs', '*.cjs'), ('application/javascript', 'application/x-javascript', 'text/x-javascript', 'text/javascript')),
- 'JavascriptPhpLexer': ('pip._vendor.pygments.lexers.templates', 'JavaScript+PHP', ('javascript+php', 'js+php'), (), ('application/x-javascript+php', 'text/x-javascript+php', 'text/javascript+php')),
- 'JavascriptSmartyLexer': ('pip._vendor.pygments.lexers.templates', 'JavaScript+Smarty', ('javascript+smarty', 'js+smarty'), (), ('application/x-javascript+smarty', 'text/x-javascript+smarty', 'text/javascript+smarty')),
- 'JclLexer': ('pip._vendor.pygments.lexers.scripting', 'JCL', ('jcl',), ('*.jcl',), ('text/x-jcl',)),
- 'JsgfLexer': ('pip._vendor.pygments.lexers.grammar_notation', 'JSGF', ('jsgf',), ('*.jsgf',), ('application/jsgf', 'application/x-jsgf', 'text/jsgf')),
- 'JsonBareObjectLexer': ('pip._vendor.pygments.lexers.data', 'JSONBareObject', (), (), ()),
- 'JsonLdLexer': ('pip._vendor.pygments.lexers.data', 'JSON-LD', ('jsonld', 'json-ld'), ('*.jsonld',), ('application/ld+json',)),
- 'JsonLexer': ('pip._vendor.pygments.lexers.data', 'JSON', ('json', 'json-object'), ('*.json', 'Pipfile.lock'), ('application/json', 'application/json-object')),
- 'JspLexer': ('pip._vendor.pygments.lexers.templates', 'Java Server Page', ('jsp',), ('*.jsp',), ('application/x-jsp',)),
- 'JuliaConsoleLexer': ('pip._vendor.pygments.lexers.julia', 'Julia console', ('jlcon', 'julia-repl'), (), ()),
- 'JuliaLexer': ('pip._vendor.pygments.lexers.julia', 'Julia', ('julia', 'jl'), ('*.jl',), ('text/x-julia', 'application/x-julia')),
- 'JuttleLexer': ('pip._vendor.pygments.lexers.javascript', 'Juttle', ('juttle',), ('*.juttle',), ('application/juttle', 'application/x-juttle', 'text/x-juttle', 'text/juttle')),
- 'KalLexer': ('pip._vendor.pygments.lexers.javascript', 'Kal', ('kal',), ('*.kal',), ('text/kal', 'application/kal')),
- 'KconfigLexer': ('pip._vendor.pygments.lexers.configs', 'Kconfig', ('kconfig', 'menuconfig', 'linux-config', 'kernel-config'), ('Kconfig*', '*Config.in*', 'external.in*', 'standard-modules.in'), ('text/x-kconfig',)),
- 'KernelLogLexer': ('pip._vendor.pygments.lexers.textfmts', 'Kernel log', ('kmsg', 'dmesg'), ('*.kmsg', '*.dmesg'), ()),
- 'KokaLexer': ('pip._vendor.pygments.lexers.haskell', 'Koka', ('koka',), ('*.kk', '*.kki'), ('text/x-koka',)),
- 'KotlinLexer': ('pip._vendor.pygments.lexers.jvm', 'Kotlin', ('kotlin',), ('*.kt', '*.kts'), ('text/x-kotlin',)),
- 'KuinLexer': ('pip._vendor.pygments.lexers.kuin', 'Kuin', ('kuin',), ('*.kn',), ()),
- 'LSLLexer': ('pip._vendor.pygments.lexers.scripting', 'LSL', ('lsl',), ('*.lsl',), ('text/x-lsl',)),
- 'LassoCssLexer': ('pip._vendor.pygments.lexers.templates', 'CSS+Lasso', ('css+lasso',), (), ('text/css+lasso',)),
- 'LassoHtmlLexer': ('pip._vendor.pygments.lexers.templates', 'HTML+Lasso', ('html+lasso',), (), ('text/html+lasso', 'application/x-httpd-lasso', 'application/x-httpd-lasso[89]')),
- 'LassoJavascriptLexer': ('pip._vendor.pygments.lexers.templates', 'JavaScript+Lasso', ('javascript+lasso', 'js+lasso'), (), ('application/x-javascript+lasso', 'text/x-javascript+lasso', 'text/javascript+lasso')),
- 'LassoLexer': ('pip._vendor.pygments.lexers.javascript', 'Lasso', ('lasso', 'lassoscript'), ('*.lasso', '*.lasso[89]'), ('text/x-lasso',)),
- 'LassoXmlLexer': ('pip._vendor.pygments.lexers.templates', 'XML+Lasso', ('xml+lasso',), (), ('application/xml+lasso',)),
- 'LeanLexer': ('pip._vendor.pygments.lexers.theorem', 'Lean', ('lean',), ('*.lean',), ('text/x-lean',)),
- 'LessCssLexer': ('pip._vendor.pygments.lexers.css', 'LessCss', ('less',), ('*.less',), ('text/x-less-css',)),
- 'LighttpdConfLexer': ('pip._vendor.pygments.lexers.configs', 'Lighttpd configuration file', ('lighttpd', 'lighty'), ('lighttpd.conf',), ('text/x-lighttpd-conf',)),
- 'LilyPondLexer': ('pip._vendor.pygments.lexers.lilypond', 'LilyPond', ('lilypond',), ('*.ly',), ()),
- 'LimboLexer': ('pip._vendor.pygments.lexers.inferno', 'Limbo', ('limbo',), ('*.b',), ('text/limbo',)),
- 'LiquidLexer': ('pip._vendor.pygments.lexers.templates', 'liquid', ('liquid',), ('*.liquid',), ()),
- 'LiterateAgdaLexer': ('pip._vendor.pygments.lexers.haskell', 'Literate Agda', ('literate-agda', 'lagda'), ('*.lagda',), ('text/x-literate-agda',)),
- 'LiterateCryptolLexer': ('pip._vendor.pygments.lexers.haskell', 'Literate Cryptol', ('literate-cryptol', 'lcryptol', 'lcry'), ('*.lcry',), ('text/x-literate-cryptol',)),
- 'LiterateHaskellLexer': ('pip._vendor.pygments.lexers.haskell', 'Literate Haskell', ('literate-haskell', 'lhaskell', 'lhs'), ('*.lhs',), ('text/x-literate-haskell',)),
- 'LiterateIdrisLexer': ('pip._vendor.pygments.lexers.haskell', 'Literate Idris', ('literate-idris', 'lidris', 'lidr'), ('*.lidr',), ('text/x-literate-idris',)),
- 'LiveScriptLexer': ('pip._vendor.pygments.lexers.javascript', 'LiveScript', ('livescript', 'live-script'), ('*.ls',), ('text/livescript',)),
- 'LlvmLexer': ('pip._vendor.pygments.lexers.asm', 'LLVM', ('llvm',), ('*.ll',), ('text/x-llvm',)),
- 'LlvmMirBodyLexer': ('pip._vendor.pygments.lexers.asm', 'LLVM-MIR Body', ('llvm-mir-body',), (), ()),
- 'LlvmMirLexer': ('pip._vendor.pygments.lexers.asm', 'LLVM-MIR', ('llvm-mir',), ('*.mir',), ()),
- 'LogosLexer': ('pip._vendor.pygments.lexers.objective', 'Logos', ('logos',), ('*.x', '*.xi', '*.xm', '*.xmi'), ('text/x-logos',)),
- 'LogtalkLexer': ('pip._vendor.pygments.lexers.prolog', 'Logtalk', ('logtalk',), ('*.lgt', '*.logtalk'), ('text/x-logtalk',)),
- 'LuaLexer': ('pip._vendor.pygments.lexers.scripting', 'Lua', ('lua',), ('*.lua', '*.wlua'), ('text/x-lua', 'application/x-lua')),
- 'MIMELexer': ('pip._vendor.pygments.lexers.mime', 'MIME', ('mime',), (), ('multipart/mixed', 'multipart/related', 'multipart/alternative')),
- 'MOOCodeLexer': ('pip._vendor.pygments.lexers.scripting', 'MOOCode', ('moocode', 'moo'), ('*.moo',), ('text/x-moocode',)),
- 'MSDOSSessionLexer': ('pip._vendor.pygments.lexers.shell', 'MSDOS Session', ('doscon',), (), ()),
- 'MakefileLexer': ('pip._vendor.pygments.lexers.make', 'Makefile', ('make', 'makefile', 'mf', 'bsdmake'), ('*.mak', '*.mk', 'Makefile', 'makefile', 'Makefile.*', 'GNUmakefile'), ('text/x-makefile',)),
- 'MakoCssLexer': ('pip._vendor.pygments.lexers.templates', 'CSS+Mako', ('css+mako',), (), ('text/css+mako',)),
- 'MakoHtmlLexer': ('pip._vendor.pygments.lexers.templates', 'HTML+Mako', ('html+mako',), (), ('text/html+mako',)),
- 'MakoJavascriptLexer': ('pip._vendor.pygments.lexers.templates', 'JavaScript+Mako', ('javascript+mako', 'js+mako'), (), ('application/x-javascript+mako', 'text/x-javascript+mako', 'text/javascript+mako')),
- 'MakoLexer': ('pip._vendor.pygments.lexers.templates', 'Mako', ('mako',), ('*.mao',), ('application/x-mako',)),
- 'MakoXmlLexer': ('pip._vendor.pygments.lexers.templates', 'XML+Mako', ('xml+mako',), (), ('application/xml+mako',)),
- 'MaqlLexer': ('pip._vendor.pygments.lexers.business', 'MAQL', ('maql',), ('*.maql',), ('text/x-gooddata-maql', 'application/x-gooddata-maql')),
- 'MarkdownLexer': ('pip._vendor.pygments.lexers.markup', 'Markdown', ('markdown', 'md'), ('*.md', '*.markdown'), ('text/x-markdown',)),
- 'MaskLexer': ('pip._vendor.pygments.lexers.javascript', 'Mask', ('mask',), ('*.mask',), ('text/x-mask',)),
- 'MasonLexer': ('pip._vendor.pygments.lexers.templates', 'Mason', ('mason',), ('*.m', '*.mhtml', '*.mc', '*.mi', 'autohandler', 'dhandler'), ('application/x-mason',)),
- 'MathematicaLexer': ('pip._vendor.pygments.lexers.algebra', 'Mathematica', ('mathematica', 'mma', 'nb'), ('*.nb', '*.cdf', '*.nbp', '*.ma'), ('application/mathematica', 'application/vnd.wolfram.mathematica', 'application/vnd.wolfram.mathematica.package', 'application/vnd.wolfram.cdf')),
- 'MatlabLexer': ('pip._vendor.pygments.lexers.matlab', 'Matlab', ('matlab',), ('*.m',), ('text/matlab',)),
- 'MatlabSessionLexer': ('pip._vendor.pygments.lexers.matlab', 'Matlab session', ('matlabsession',), (), ()),
- 'MaximaLexer': ('pip._vendor.pygments.lexers.maxima', 'Maxima', ('maxima', 'macsyma'), ('*.mac', '*.max'), ()),
- 'MesonLexer': ('pip._vendor.pygments.lexers.meson', 'Meson', ('meson', 'meson.build'), ('meson.build', 'meson_options.txt'), ('text/x-meson',)),
- 'MiniDLexer': ('pip._vendor.pygments.lexers.d', 'MiniD', ('minid',), (), ('text/x-minidsrc',)),
- 'MiniScriptLexer': ('pip._vendor.pygments.lexers.scripting', 'MiniScript', ('miniscript', 'ms'), ('*.ms',), ('text/x-minicript', 'application/x-miniscript')),
- 'ModelicaLexer': ('pip._vendor.pygments.lexers.modeling', 'Modelica', ('modelica',), ('*.mo',), ('text/x-modelica',)),
- 'Modula2Lexer': ('pip._vendor.pygments.lexers.modula2', 'Modula-2', ('modula2', 'm2'), ('*.def', '*.mod'), ('text/x-modula2',)),
- 'MoinWikiLexer': ('pip._vendor.pygments.lexers.markup', 'MoinMoin/Trac Wiki markup', ('trac-wiki', 'moin'), (), ('text/x-trac-wiki',)),
- 'MonkeyLexer': ('pip._vendor.pygments.lexers.basic', 'Monkey', ('monkey',), ('*.monkey',), ('text/x-monkey',)),
- 'MonteLexer': ('pip._vendor.pygments.lexers.monte', 'Monte', ('monte',), ('*.mt',), ()),
- 'MoonScriptLexer': ('pip._vendor.pygments.lexers.scripting', 'MoonScript', ('moonscript', 'moon'), ('*.moon',), ('text/x-moonscript', 'application/x-moonscript')),
- 'MoselLexer': ('pip._vendor.pygments.lexers.mosel', 'Mosel', ('mosel',), ('*.mos',), ()),
- 'MozPreprocCssLexer': ('pip._vendor.pygments.lexers.markup', 'CSS+mozpreproc', ('css+mozpreproc',), ('*.css.in',), ()),
- 'MozPreprocHashLexer': ('pip._vendor.pygments.lexers.markup', 'mozhashpreproc', ('mozhashpreproc',), (), ()),
- 'MozPreprocJavascriptLexer': ('pip._vendor.pygments.lexers.markup', 'Javascript+mozpreproc', ('javascript+mozpreproc',), ('*.js.in',), ()),
- 'MozPreprocPercentLexer': ('pip._vendor.pygments.lexers.markup', 'mozpercentpreproc', ('mozpercentpreproc',), (), ()),
- 'MozPreprocXulLexer': ('pip._vendor.pygments.lexers.markup', 'XUL+mozpreproc', ('xul+mozpreproc',), ('*.xul.in',), ()),
- 'MqlLexer': ('pip._vendor.pygments.lexers.c_like', 'MQL', ('mql', 'mq4', 'mq5', 'mql4', 'mql5'), ('*.mq4', '*.mq5', '*.mqh'), ('text/x-mql',)),
- 'MscgenLexer': ('pip._vendor.pygments.lexers.dsls', 'Mscgen', ('mscgen', 'msc'), ('*.msc',), ()),
- 'MuPADLexer': ('pip._vendor.pygments.lexers.algebra', 'MuPAD', ('mupad',), ('*.mu',), ()),
- 'MxmlLexer': ('pip._vendor.pygments.lexers.actionscript', 'MXML', ('mxml',), ('*.mxml',), ()),
- 'MySqlLexer': ('pip._vendor.pygments.lexers.sql', 'MySQL', ('mysql',), (), ('text/x-mysql',)),
- 'MyghtyCssLexer': ('pip._vendor.pygments.lexers.templates', 'CSS+Myghty', ('css+myghty',), (), ('text/css+myghty',)),
- 'MyghtyHtmlLexer': ('pip._vendor.pygments.lexers.templates', 'HTML+Myghty', ('html+myghty',), (), ('text/html+myghty',)),
- 'MyghtyJavascriptLexer': ('pip._vendor.pygments.lexers.templates', 'JavaScript+Myghty', ('javascript+myghty', 'js+myghty'), (), ('application/x-javascript+myghty', 'text/x-javascript+myghty', 'text/javascript+mygthy')),
- 'MyghtyLexer': ('pip._vendor.pygments.lexers.templates', 'Myghty', ('myghty',), ('*.myt', 'autodelegate'), ('application/x-myghty',)),
- 'MyghtyXmlLexer': ('pip._vendor.pygments.lexers.templates', 'XML+Myghty', ('xml+myghty',), (), ('application/xml+myghty',)),
- 'NCLLexer': ('pip._vendor.pygments.lexers.ncl', 'NCL', ('ncl',), ('*.ncl',), ('text/ncl',)),
- 'NSISLexer': ('pip._vendor.pygments.lexers.installers', 'NSIS', ('nsis', 'nsi', 'nsh'), ('*.nsi', '*.nsh'), ('text/x-nsis',)),
- 'NasmLexer': ('pip._vendor.pygments.lexers.asm', 'NASM', ('nasm',), ('*.asm', '*.ASM'), ('text/x-nasm',)),
- 'NasmObjdumpLexer': ('pip._vendor.pygments.lexers.asm', 'objdump-nasm', ('objdump-nasm',), ('*.objdump-intel',), ('text/x-nasm-objdump',)),
- 'NemerleLexer': ('pip._vendor.pygments.lexers.dotnet', 'Nemerle', ('nemerle',), ('*.n',), ('text/x-nemerle',)),
- 'NesCLexer': ('pip._vendor.pygments.lexers.c_like', 'nesC', ('nesc',), ('*.nc',), ('text/x-nescsrc',)),
- 'NestedTextLexer': ('pip._vendor.pygments.lexers.configs', 'NestedText', ('nestedtext', 'nt'), ('*.nt',), ()),
- 'NewLispLexer': ('pip._vendor.pygments.lexers.lisp', 'NewLisp', ('newlisp',), ('*.lsp', '*.nl', '*.kif'), ('text/x-newlisp', 'application/x-newlisp')),
- 'NewspeakLexer': ('pip._vendor.pygments.lexers.smalltalk', 'Newspeak', ('newspeak',), ('*.ns2',), ('text/x-newspeak',)),
- 'NginxConfLexer': ('pip._vendor.pygments.lexers.configs', 'Nginx configuration file', ('nginx',), ('nginx.conf',), ('text/x-nginx-conf',)),
- 'NimrodLexer': ('pip._vendor.pygments.lexers.nimrod', 'Nimrod', ('nimrod', 'nim'), ('*.nim', '*.nimrod'), ('text/x-nim',)),
- 'NitLexer': ('pip._vendor.pygments.lexers.nit', 'Nit', ('nit',), ('*.nit',), ()),
- 'NixLexer': ('pip._vendor.pygments.lexers.nix', 'Nix', ('nixos', 'nix'), ('*.nix',), ('text/x-nix',)),
- 'NodeConsoleLexer': ('pip._vendor.pygments.lexers.javascript', 'Node.js REPL console session', ('nodejsrepl',), (), ('text/x-nodejsrepl',)),
- 'NotmuchLexer': ('pip._vendor.pygments.lexers.textfmts', 'Notmuch', ('notmuch',), (), ()),
- 'NuSMVLexer': ('pip._vendor.pygments.lexers.smv', 'NuSMV', ('nusmv',), ('*.smv',), ()),
- 'NumPyLexer': ('pip._vendor.pygments.lexers.python', 'NumPy', ('numpy',), (), ()),
- 'ObjdumpLexer': ('pip._vendor.pygments.lexers.asm', 'objdump', ('objdump',), ('*.objdump',), ('text/x-objdump',)),
- 'ObjectiveCLexer': ('pip._vendor.pygments.lexers.objective', 'Objective-C', ('objective-c', 'objectivec', 'obj-c', 'objc'), ('*.m', '*.h'), ('text/x-objective-c',)),
- 'ObjectiveCppLexer': ('pip._vendor.pygments.lexers.objective', 'Objective-C++', ('objective-c++', 'objectivec++', 'obj-c++', 'objc++'), ('*.mm', '*.hh'), ('text/x-objective-c++',)),
- 'ObjectiveJLexer': ('pip._vendor.pygments.lexers.javascript', 'Objective-J', ('objective-j', 'objectivej', 'obj-j', 'objj'), ('*.j',), ('text/x-objective-j',)),
- 'OcamlLexer': ('pip._vendor.pygments.lexers.ml', 'OCaml', ('ocaml',), ('*.ml', '*.mli', '*.mll', '*.mly'), ('text/x-ocaml',)),
- 'OctaveLexer': ('pip._vendor.pygments.lexers.matlab', 'Octave', ('octave',), ('*.m',), ('text/octave',)),
- 'OdinLexer': ('pip._vendor.pygments.lexers.archetype', 'ODIN', ('odin',), ('*.odin',), ('text/odin',)),
- 'OmgIdlLexer': ('pip._vendor.pygments.lexers.c_like', 'OMG Interface Definition Language', ('omg-idl',), ('*.idl', '*.pidl'), ()),
- 'OocLexer': ('pip._vendor.pygments.lexers.ooc', 'Ooc', ('ooc',), ('*.ooc',), ('text/x-ooc',)),
- 'OpaLexer': ('pip._vendor.pygments.lexers.ml', 'Opa', ('opa',), ('*.opa',), ('text/x-opa',)),
- 'OpenEdgeLexer': ('pip._vendor.pygments.lexers.business', 'OpenEdge ABL', ('openedge', 'abl', 'progress'), ('*.p', '*.cls'), ('text/x-openedge', 'application/x-openedge')),
- 'OutputLexer': ('pip._vendor.pygments.lexers.special', 'Text output', ('output',), (), ()),
- 'PacmanConfLexer': ('pip._vendor.pygments.lexers.configs', 'PacmanConf', ('pacmanconf',), ('pacman.conf',), ()),
- 'PanLexer': ('pip._vendor.pygments.lexers.dsls', 'Pan', ('pan',), ('*.pan',), ()),
- 'ParaSailLexer': ('pip._vendor.pygments.lexers.parasail', 'ParaSail', ('parasail',), ('*.psi', '*.psl'), ('text/x-parasail',)),
- 'PawnLexer': ('pip._vendor.pygments.lexers.pawn', 'Pawn', ('pawn',), ('*.p', '*.pwn', '*.inc'), ('text/x-pawn',)),
- 'PegLexer': ('pip._vendor.pygments.lexers.grammar_notation', 'PEG', ('peg',), ('*.peg',), ('text/x-peg',)),
- 'Perl6Lexer': ('pip._vendor.pygments.lexers.perl', 'Perl6', ('perl6', 'pl6', 'raku'), ('*.pl', '*.pm', '*.nqp', '*.p6', '*.6pl', '*.p6l', '*.pl6', '*.6pm', '*.p6m', '*.pm6', '*.t', '*.raku', '*.rakumod', '*.rakutest', '*.rakudoc'), ('text/x-perl6', 'application/x-perl6')),
- 'PerlLexer': ('pip._vendor.pygments.lexers.perl', 'Perl', ('perl', 'pl'), ('*.pl', '*.pm', '*.t', '*.perl'), ('text/x-perl', 'application/x-perl')),
- 'PhpLexer': ('pip._vendor.pygments.lexers.php', 'PHP', ('php', 'php3', 'php4', 'php5'), ('*.php', '*.php[345]', '*.inc'), ('text/x-php',)),
- 'PigLexer': ('pip._vendor.pygments.lexers.jvm', 'Pig', ('pig',), ('*.pig',), ('text/x-pig',)),
- 'PikeLexer': ('pip._vendor.pygments.lexers.c_like', 'Pike', ('pike',), ('*.pike', '*.pmod'), ('text/x-pike',)),
- 'PkgConfigLexer': ('pip._vendor.pygments.lexers.configs', 'PkgConfig', ('pkgconfig',), ('*.pc',), ()),
- 'PlPgsqlLexer': ('pip._vendor.pygments.lexers.sql', 'PL/pgSQL', ('plpgsql',), (), ('text/x-plpgsql',)),
- 'PointlessLexer': ('pip._vendor.pygments.lexers.pointless', 'Pointless', ('pointless',), ('*.ptls',), ()),
- 'PonyLexer': ('pip._vendor.pygments.lexers.pony', 'Pony', ('pony',), ('*.pony',), ()),
- 'PostScriptLexer': ('pip._vendor.pygments.lexers.graphics', 'PostScript', ('postscript', 'postscr'), ('*.ps', '*.eps'), ('application/postscript',)),
- 'PostgresConsoleLexer': ('pip._vendor.pygments.lexers.sql', 'PostgreSQL console (psql)', ('psql', 'postgresql-console', 'postgres-console'), (), ('text/x-postgresql-psql',)),
- 'PostgresLexer': ('pip._vendor.pygments.lexers.sql', 'PostgreSQL SQL dialect', ('postgresql', 'postgres'), (), ('text/x-postgresql',)),
- 'PovrayLexer': ('pip._vendor.pygments.lexers.graphics', 'POVRay', ('pov',), ('*.pov', '*.inc'), ('text/x-povray',)),
- 'PowerShellLexer': ('pip._vendor.pygments.lexers.shell', 'PowerShell', ('powershell', 'pwsh', 'posh', 'ps1', 'psm1'), ('*.ps1', '*.psm1'), ('text/x-powershell',)),
- 'PowerShellSessionLexer': ('pip._vendor.pygments.lexers.shell', 'PowerShell Session', ('pwsh-session', 'ps1con'), (), ()),
- 'PraatLexer': ('pip._vendor.pygments.lexers.praat', 'Praat', ('praat',), ('*.praat', '*.proc', '*.psc'), ()),
- 'ProcfileLexer': ('pip._vendor.pygments.lexers.procfile', 'Procfile', ('procfile',), ('Procfile',), ()),
- 'PrologLexer': ('pip._vendor.pygments.lexers.prolog', 'Prolog', ('prolog',), ('*.ecl', '*.prolog', '*.pro', '*.pl'), ('text/x-prolog',)),
- 'PromQLLexer': ('pip._vendor.pygments.lexers.promql', 'PromQL', ('promql',), ('*.promql',), ()),
- 'PropertiesLexer': ('pip._vendor.pygments.lexers.configs', 'Properties', ('properties', 'jproperties'), ('*.properties',), ('text/x-java-properties',)),
- 'ProtoBufLexer': ('pip._vendor.pygments.lexers.dsls', 'Protocol Buffer', ('protobuf', 'proto'), ('*.proto',), ()),
- 'PsyshConsoleLexer': ('pip._vendor.pygments.lexers.php', 'PsySH console session for PHP', ('psysh',), (), ()),
- 'PugLexer': ('pip._vendor.pygments.lexers.html', 'Pug', ('pug', 'jade'), ('*.pug', '*.jade'), ('text/x-pug', 'text/x-jade')),
- 'PuppetLexer': ('pip._vendor.pygments.lexers.dsls', 'Puppet', ('puppet',), ('*.pp',), ()),
- 'PyPyLogLexer': ('pip._vendor.pygments.lexers.console', 'PyPy Log', ('pypylog', 'pypy'), ('*.pypylog',), ('application/x-pypylog',)),
- 'Python2Lexer': ('pip._vendor.pygments.lexers.python', 'Python 2.x', ('python2', 'py2'), (), ('text/x-python2', 'application/x-python2')),
- 'Python2TracebackLexer': ('pip._vendor.pygments.lexers.python', 'Python 2.x Traceback', ('py2tb',), ('*.py2tb',), ('text/x-python2-traceback',)),
- 'PythonConsoleLexer': ('pip._vendor.pygments.lexers.python', 'Python console session', ('pycon',), (), ('text/x-python-doctest',)),
- 'PythonLexer': ('pip._vendor.pygments.lexers.python', 'Python', ('python', 'py', 'sage', 'python3', 'py3'), ('*.py', '*.pyw', '*.jy', '*.sage', '*.sc', 'SConstruct', 'SConscript', '*.bzl', 'BUCK', 'BUILD', 'BUILD.bazel', 'WORKSPACE', '*.tac'), ('text/x-python', 'application/x-python', 'text/x-python3', 'application/x-python3')),
- 'PythonTracebackLexer': ('pip._vendor.pygments.lexers.python', 'Python Traceback', ('pytb', 'py3tb'), ('*.pytb', '*.py3tb'), ('text/x-python-traceback', 'text/x-python3-traceback')),
- 'QBasicLexer': ('pip._vendor.pygments.lexers.basic', 'QBasic', ('qbasic', 'basic'), ('*.BAS', '*.bas'), ('text/basic',)),
- 'QVToLexer': ('pip._vendor.pygments.lexers.qvt', 'QVTO', ('qvto', 'qvt'), ('*.qvto',), ()),
- 'QmlLexer': ('pip._vendor.pygments.lexers.webmisc', 'QML', ('qml', 'qbs'), ('*.qml', '*.qbs'), ('application/x-qml', 'application/x-qt.qbs+qml')),
- 'RConsoleLexer': ('pip._vendor.pygments.lexers.r', 'RConsole', ('rconsole', 'rout'), ('*.Rout',), ()),
- 'RNCCompactLexer': ('pip._vendor.pygments.lexers.rnc', 'Relax-NG Compact', ('rng-compact', 'rnc'), ('*.rnc',), ()),
- 'RPMSpecLexer': ('pip._vendor.pygments.lexers.installers', 'RPMSpec', ('spec',), ('*.spec',), ('text/x-rpm-spec',)),
- 'RacketLexer': ('pip._vendor.pygments.lexers.lisp', 'Racket', ('racket', 'rkt'), ('*.rkt', '*.rktd', '*.rktl'), ('text/x-racket', 'application/x-racket')),
- 'RagelCLexer': ('pip._vendor.pygments.lexers.parsers', 'Ragel in C Host', ('ragel-c',), ('*.rl',), ()),
- 'RagelCppLexer': ('pip._vendor.pygments.lexers.parsers', 'Ragel in CPP Host', ('ragel-cpp',), ('*.rl',), ()),
- 'RagelDLexer': ('pip._vendor.pygments.lexers.parsers', 'Ragel in D Host', ('ragel-d',), ('*.rl',), ()),
- 'RagelEmbeddedLexer': ('pip._vendor.pygments.lexers.parsers', 'Embedded Ragel', ('ragel-em',), ('*.rl',), ()),
- 'RagelJavaLexer': ('pip._vendor.pygments.lexers.parsers', 'Ragel in Java Host', ('ragel-java',), ('*.rl',), ()),
- 'RagelLexer': ('pip._vendor.pygments.lexers.parsers', 'Ragel', ('ragel',), (), ()),
- 'RagelObjectiveCLexer': ('pip._vendor.pygments.lexers.parsers', 'Ragel in Objective C Host', ('ragel-objc',), ('*.rl',), ()),
- 'RagelRubyLexer': ('pip._vendor.pygments.lexers.parsers', 'Ragel in Ruby Host', ('ragel-ruby', 'ragel-rb'), ('*.rl',), ()),
- 'RawTokenLexer': ('pip._vendor.pygments.lexers.special', 'Raw token data', (), (), ('application/x-pygments-tokens',)),
- 'RdLexer': ('pip._vendor.pygments.lexers.r', 'Rd', ('rd',), ('*.Rd',), ('text/x-r-doc',)),
- 'ReasonLexer': ('pip._vendor.pygments.lexers.ml', 'ReasonML', ('reasonml', 'reason'), ('*.re', '*.rei'), ('text/x-reasonml',)),
- 'RebolLexer': ('pip._vendor.pygments.lexers.rebol', 'REBOL', ('rebol',), ('*.r', '*.r3', '*.reb'), ('text/x-rebol',)),
- 'RedLexer': ('pip._vendor.pygments.lexers.rebol', 'Red', ('red', 'red/system'), ('*.red', '*.reds'), ('text/x-red', 'text/x-red-system')),
- 'RedcodeLexer': ('pip._vendor.pygments.lexers.esoteric', 'Redcode', ('redcode',), ('*.cw',), ()),
- 'RegeditLexer': ('pip._vendor.pygments.lexers.configs', 'reg', ('registry',), ('*.reg',), ('text/x-windows-registry',)),
- 'ResourceLexer': ('pip._vendor.pygments.lexers.resource', 'ResourceBundle', ('resourcebundle', 'resource'), (), ()),
- 'RexxLexer': ('pip._vendor.pygments.lexers.scripting', 'Rexx', ('rexx', 'arexx'), ('*.rexx', '*.rex', '*.rx', '*.arexx'), ('text/x-rexx',)),
- 'RhtmlLexer': ('pip._vendor.pygments.lexers.templates', 'RHTML', ('rhtml', 'html+erb', 'html+ruby'), ('*.rhtml',), ('text/html+ruby',)),
- 'RideLexer': ('pip._vendor.pygments.lexers.ride', 'Ride', ('ride',), ('*.ride',), ('text/x-ride',)),
- 'RitaLexer': ('pip._vendor.pygments.lexers.rita', 'Rita', ('rita',), ('*.rita',), ('text/rita',)),
- 'RoboconfGraphLexer': ('pip._vendor.pygments.lexers.roboconf', 'Roboconf Graph', ('roboconf-graph',), ('*.graph',), ()),
- 'RoboconfInstancesLexer': ('pip._vendor.pygments.lexers.roboconf', 'Roboconf Instances', ('roboconf-instances',), ('*.instances',), ()),
- 'RobotFrameworkLexer': ('pip._vendor.pygments.lexers.robotframework', 'RobotFramework', ('robotframework',), ('*.robot',), ('text/x-robotframework',)),
- 'RqlLexer': ('pip._vendor.pygments.lexers.sql', 'RQL', ('rql',), ('*.rql',), ('text/x-rql',)),
- 'RslLexer': ('pip._vendor.pygments.lexers.dsls', 'RSL', ('rsl',), ('*.rsl',), ('text/rsl',)),
- 'RstLexer': ('pip._vendor.pygments.lexers.markup', 'reStructuredText', ('restructuredtext', 'rst', 'rest'), ('*.rst', '*.rest'), ('text/x-rst', 'text/prs.fallenstein.rst')),
- 'RtsLexer': ('pip._vendor.pygments.lexers.trafficscript', 'TrafficScript', ('trafficscript', 'rts'), ('*.rts',), ()),
- 'RubyConsoleLexer': ('pip._vendor.pygments.lexers.ruby', 'Ruby irb session', ('rbcon', 'irb'), (), ('text/x-ruby-shellsession',)),
- 'RubyLexer': ('pip._vendor.pygments.lexers.ruby', 'Ruby', ('ruby', 'rb', 'duby'), ('*.rb', '*.rbw', 'Rakefile', '*.rake', '*.gemspec', '*.rbx', '*.duby', 'Gemfile', 'Vagrantfile'), ('text/x-ruby', 'application/x-ruby')),
- 'RustLexer': ('pip._vendor.pygments.lexers.rust', 'Rust', ('rust', 'rs'), ('*.rs', '*.rs.in'), ('text/rust', 'text/x-rust')),
- 'SASLexer': ('pip._vendor.pygments.lexers.sas', 'SAS', ('sas',), ('*.SAS', '*.sas'), ('text/x-sas', 'text/sas', 'application/x-sas')),
- 'SLexer': ('pip._vendor.pygments.lexers.r', 'S', ('splus', 's', 'r'), ('*.S', '*.R', '.Rhistory', '.Rprofile', '.Renviron'), ('text/S-plus', 'text/S', 'text/x-r-source', 'text/x-r', 'text/x-R', 'text/x-r-history', 'text/x-r-profile')),
- 'SMLLexer': ('pip._vendor.pygments.lexers.ml', 'Standard ML', ('sml',), ('*.sml', '*.sig', '*.fun'), ('text/x-standardml', 'application/x-standardml')),
- 'SarlLexer': ('pip._vendor.pygments.lexers.jvm', 'SARL', ('sarl',), ('*.sarl',), ('text/x-sarl',)),
- 'SassLexer': ('pip._vendor.pygments.lexers.css', 'Sass', ('sass',), ('*.sass',), ('text/x-sass',)),
- 'SaviLexer': ('pip._vendor.pygments.lexers.savi', 'Savi', ('savi',), ('*.savi',), ()),
- 'ScalaLexer': ('pip._vendor.pygments.lexers.jvm', 'Scala', ('scala',), ('*.scala',), ('text/x-scala',)),
- 'ScamlLexer': ('pip._vendor.pygments.lexers.html', 'Scaml', ('scaml',), ('*.scaml',), ('text/x-scaml',)),
- 'ScdocLexer': ('pip._vendor.pygments.lexers.scdoc', 'scdoc', ('scdoc', 'scd'), ('*.scd', '*.scdoc'), ()),
- 'SchemeLexer': ('pip._vendor.pygments.lexers.lisp', 'Scheme', ('scheme', 'scm'), ('*.scm', '*.ss'), ('text/x-scheme', 'application/x-scheme')),
- 'ScilabLexer': ('pip._vendor.pygments.lexers.matlab', 'Scilab', ('scilab',), ('*.sci', '*.sce', '*.tst'), ('text/scilab',)),
- 'ScssLexer': ('pip._vendor.pygments.lexers.css', 'SCSS', ('scss',), ('*.scss',), ('text/x-scss',)),
- 'SedLexer': ('pip._vendor.pygments.lexers.textedit', 'Sed', ('sed', 'gsed', 'ssed'), ('*.sed', '*.[gs]sed'), ('text/x-sed',)),
- 'ShExCLexer': ('pip._vendor.pygments.lexers.rdf', 'ShExC', ('shexc', 'shex'), ('*.shex',), ('text/shex',)),
- 'ShenLexer': ('pip._vendor.pygments.lexers.lisp', 'Shen', ('shen',), ('*.shen',), ('text/x-shen', 'application/x-shen')),
- 'SieveLexer': ('pip._vendor.pygments.lexers.sieve', 'Sieve', ('sieve',), ('*.siv', '*.sieve'), ()),
- 'SilverLexer': ('pip._vendor.pygments.lexers.verification', 'Silver', ('silver',), ('*.sil', '*.vpr'), ()),
- 'SingularityLexer': ('pip._vendor.pygments.lexers.configs', 'Singularity', ('singularity',), ('*.def', 'Singularity'), ()),
- 'SlashLexer': ('pip._vendor.pygments.lexers.slash', 'Slash', ('slash',), ('*.sla',), ()),
- 'SlimLexer': ('pip._vendor.pygments.lexers.webmisc', 'Slim', ('slim',), ('*.slim',), ('text/x-slim',)),
- 'SlurmBashLexer': ('pip._vendor.pygments.lexers.shell', 'Slurm', ('slurm', 'sbatch'), ('*.sl',), ()),
- 'SmaliLexer': ('pip._vendor.pygments.lexers.dalvik', 'Smali', ('smali',), ('*.smali',), ('text/smali',)),
- 'SmalltalkLexer': ('pip._vendor.pygments.lexers.smalltalk', 'Smalltalk', ('smalltalk', 'squeak', 'st'), ('*.st',), ('text/x-smalltalk',)),
- 'SmartGameFormatLexer': ('pip._vendor.pygments.lexers.sgf', 'SmartGameFormat', ('sgf',), ('*.sgf',), ()),
- 'SmartyLexer': ('pip._vendor.pygments.lexers.templates', 'Smarty', ('smarty',), ('*.tpl',), ('application/x-smarty',)),
- 'SmithyLexer': ('pip._vendor.pygments.lexers.smithy', 'Smithy', ('smithy',), ('*.smithy',), ()),
- 'SnobolLexer': ('pip._vendor.pygments.lexers.snobol', 'Snobol', ('snobol',), ('*.snobol',), ('text/x-snobol',)),
- 'SnowballLexer': ('pip._vendor.pygments.lexers.dsls', 'Snowball', ('snowball',), ('*.sbl',), ()),
- 'SolidityLexer': ('pip._vendor.pygments.lexers.solidity', 'Solidity', ('solidity',), ('*.sol',), ()),
- 'SophiaLexer': ('pip._vendor.pygments.lexers.sophia', 'Sophia', ('sophia',), ('*.aes',), ()),
- 'SourcePawnLexer': ('pip._vendor.pygments.lexers.pawn', 'SourcePawn', ('sp',), ('*.sp',), ('text/x-sourcepawn',)),
- 'SourcesListLexer': ('pip._vendor.pygments.lexers.installers', 'Debian Sourcelist', ('debsources', 'sourceslist', 'sources.list'), ('sources.list',), ()),
- 'SparqlLexer': ('pip._vendor.pygments.lexers.rdf', 'SPARQL', ('sparql',), ('*.rq', '*.sparql'), ('application/sparql-query',)),
- 'SpiceLexer': ('pip._vendor.pygments.lexers.spice', 'Spice', ('spice', 'spicelang'), ('*.spice',), ('text/x-spice',)),
- 'SqlLexer': ('pip._vendor.pygments.lexers.sql', 'SQL', ('sql',), ('*.sql',), ('text/x-sql',)),
- 'SqliteConsoleLexer': ('pip._vendor.pygments.lexers.sql', 'sqlite3con', ('sqlite3',), ('*.sqlite3-console',), ('text/x-sqlite3-console',)),
- 'SquidConfLexer': ('pip._vendor.pygments.lexers.configs', 'SquidConf', ('squidconf', 'squid.conf', 'squid'), ('squid.conf',), ('text/x-squidconf',)),
- 'SrcinfoLexer': ('pip._vendor.pygments.lexers.srcinfo', 'Srcinfo', ('srcinfo',), ('.SRCINFO',), ()),
- 'SspLexer': ('pip._vendor.pygments.lexers.templates', 'Scalate Server Page', ('ssp',), ('*.ssp',), ('application/x-ssp',)),
- 'StanLexer': ('pip._vendor.pygments.lexers.modeling', 'Stan', ('stan',), ('*.stan',), ()),
- 'StataLexer': ('pip._vendor.pygments.lexers.stata', 'Stata', ('stata', 'do'), ('*.do', '*.ado'), ('text/x-stata', 'text/stata', 'application/x-stata')),
- 'SuperColliderLexer': ('pip._vendor.pygments.lexers.supercollider', 'SuperCollider', ('supercollider', 'sc'), ('*.sc', '*.scd'), ('application/supercollider', 'text/supercollider')),
- 'SwiftLexer': ('pip._vendor.pygments.lexers.objective', 'Swift', ('swift',), ('*.swift',), ('text/x-swift',)),
- 'SwigLexer': ('pip._vendor.pygments.lexers.c_like', 'SWIG', ('swig',), ('*.swg', '*.i'), ('text/swig',)),
- 'SystemVerilogLexer': ('pip._vendor.pygments.lexers.hdl', 'systemverilog', ('systemverilog', 'sv'), ('*.sv', '*.svh'), ('text/x-systemverilog',)),
- 'TAPLexer': ('pip._vendor.pygments.lexers.testing', 'TAP', ('tap',), ('*.tap',), ()),
- 'TNTLexer': ('pip._vendor.pygments.lexers.tnt', 'Typographic Number Theory', ('tnt',), ('*.tnt',), ()),
- 'TOMLLexer': ('pip._vendor.pygments.lexers.configs', 'TOML', ('toml',), ('*.toml', 'Pipfile', 'poetry.lock'), ()),
- 'Tads3Lexer': ('pip._vendor.pygments.lexers.int_fiction', 'TADS 3', ('tads3',), ('*.t',), ()),
- 'TasmLexer': ('pip._vendor.pygments.lexers.asm', 'TASM', ('tasm',), ('*.asm', '*.ASM', '*.tasm'), ('text/x-tasm',)),
- 'TclLexer': ('pip._vendor.pygments.lexers.tcl', 'Tcl', ('tcl',), ('*.tcl', '*.rvt'), ('text/x-tcl', 'text/x-script.tcl', 'application/x-tcl')),
- 'TcshLexer': ('pip._vendor.pygments.lexers.shell', 'Tcsh', ('tcsh', 'csh'), ('*.tcsh', '*.csh'), ('application/x-csh',)),
- 'TcshSessionLexer': ('pip._vendor.pygments.lexers.shell', 'Tcsh Session', ('tcshcon',), (), ()),
- 'TeaTemplateLexer': ('pip._vendor.pygments.lexers.templates', 'Tea', ('tea',), ('*.tea',), ('text/x-tea',)),
- 'TealLexer': ('pip._vendor.pygments.lexers.teal', 'teal', ('teal',), ('*.teal',), ()),
- 'TeraTermLexer': ('pip._vendor.pygments.lexers.teraterm', 'Tera Term macro', ('teratermmacro', 'teraterm', 'ttl'), ('*.ttl',), ('text/x-teratermmacro',)),
- 'TermcapLexer': ('pip._vendor.pygments.lexers.configs', 'Termcap', ('termcap',), ('termcap', 'termcap.src'), ()),
- 'TerminfoLexer': ('pip._vendor.pygments.lexers.configs', 'Terminfo', ('terminfo',), ('terminfo', 'terminfo.src'), ()),
- 'TerraformLexer': ('pip._vendor.pygments.lexers.configs', 'Terraform', ('terraform', 'tf'), ('*.tf',), ('application/x-tf', 'application/x-terraform')),
- 'TexLexer': ('pip._vendor.pygments.lexers.markup', 'TeX', ('tex', 'latex'), ('*.tex', '*.aux', '*.toc'), ('text/x-tex', 'text/x-latex')),
- 'TextLexer': ('pip._vendor.pygments.lexers.special', 'Text only', ('text',), ('*.txt',), ('text/plain',)),
- 'ThingsDBLexer': ('pip._vendor.pygments.lexers.thingsdb', 'ThingsDB', ('ti', 'thingsdb'), ('*.ti',), ()),
- 'ThriftLexer': ('pip._vendor.pygments.lexers.dsls', 'Thrift', ('thrift',), ('*.thrift',), ('application/x-thrift',)),
- 'TiddlyWiki5Lexer': ('pip._vendor.pygments.lexers.markup', 'tiddler', ('tid',), ('*.tid',), ('text/vnd.tiddlywiki',)),
- 'TodotxtLexer': ('pip._vendor.pygments.lexers.textfmts', 'Todotxt', ('todotxt',), ('todo.txt', '*.todotxt'), ('text/x-todo',)),
- 'TransactSqlLexer': ('pip._vendor.pygments.lexers.sql', 'Transact-SQL', ('tsql', 't-sql'), ('*.sql',), ('text/x-tsql',)),
- 'TreetopLexer': ('pip._vendor.pygments.lexers.parsers', 'Treetop', ('treetop',), ('*.treetop', '*.tt'), ()),
- 'TurtleLexer': ('pip._vendor.pygments.lexers.rdf', 'Turtle', ('turtle',), ('*.ttl',), ('text/turtle', 'application/x-turtle')),
- 'TwigHtmlLexer': ('pip._vendor.pygments.lexers.templates', 'HTML+Twig', ('html+twig',), ('*.twig',), ('text/html+twig',)),
- 'TwigLexer': ('pip._vendor.pygments.lexers.templates', 'Twig', ('twig',), (), ('application/x-twig',)),
- 'TypeScriptLexer': ('pip._vendor.pygments.lexers.javascript', 'TypeScript', ('typescript', 'ts'), ('*.ts',), ('application/x-typescript', 'text/x-typescript')),
- 'TypoScriptCssDataLexer': ('pip._vendor.pygments.lexers.typoscript', 'TypoScriptCssData', ('typoscriptcssdata',), (), ()),
- 'TypoScriptHtmlDataLexer': ('pip._vendor.pygments.lexers.typoscript', 'TypoScriptHtmlData', ('typoscripthtmldata',), (), ()),
- 'TypoScriptLexer': ('pip._vendor.pygments.lexers.typoscript', 'TypoScript', ('typoscript',), ('*.typoscript',), ('text/x-typoscript',)),
- 'UcodeLexer': ('pip._vendor.pygments.lexers.unicon', 'ucode', ('ucode',), ('*.u', '*.u1', '*.u2'), ()),
- 'UniconLexer': ('pip._vendor.pygments.lexers.unicon', 'Unicon', ('unicon',), ('*.icn',), ('text/unicon',)),
- 'UrbiscriptLexer': ('pip._vendor.pygments.lexers.urbi', 'UrbiScript', ('urbiscript',), ('*.u',), ('application/x-urbiscript',)),
- 'UsdLexer': ('pip._vendor.pygments.lexers.usd', 'USD', ('usd', 'usda'), ('*.usd', '*.usda'), ()),
- 'VBScriptLexer': ('pip._vendor.pygments.lexers.basic', 'VBScript', ('vbscript',), ('*.vbs', '*.VBS'), ()),
- 'VCLLexer': ('pip._vendor.pygments.lexers.varnish', 'VCL', ('vcl',), ('*.vcl',), ('text/x-vclsrc',)),
- 'VCLSnippetLexer': ('pip._vendor.pygments.lexers.varnish', 'VCLSnippets', ('vclsnippets', 'vclsnippet'), (), ('text/x-vclsnippet',)),
- 'VCTreeStatusLexer': ('pip._vendor.pygments.lexers.console', 'VCTreeStatus', ('vctreestatus',), (), ()),
- 'VGLLexer': ('pip._vendor.pygments.lexers.dsls', 'VGL', ('vgl',), ('*.rpf',), ()),
- 'ValaLexer': ('pip._vendor.pygments.lexers.c_like', 'Vala', ('vala', 'vapi'), ('*.vala', '*.vapi'), ('text/x-vala',)),
- 'VbNetAspxLexer': ('pip._vendor.pygments.lexers.dotnet', 'aspx-vb', ('aspx-vb',), ('*.aspx', '*.asax', '*.ascx', '*.ashx', '*.asmx', '*.axd'), ()),
- 'VbNetLexer': ('pip._vendor.pygments.lexers.dotnet', 'VB.net', ('vb.net', 'vbnet'), ('*.vb', '*.bas'), ('text/x-vbnet', 'text/x-vba')),
- 'VelocityHtmlLexer': ('pip._vendor.pygments.lexers.templates', 'HTML+Velocity', ('html+velocity',), (), ('text/html+velocity',)),
- 'VelocityLexer': ('pip._vendor.pygments.lexers.templates', 'Velocity', ('velocity',), ('*.vm', '*.fhtml'), ()),
- 'VelocityXmlLexer': ('pip._vendor.pygments.lexers.templates', 'XML+Velocity', ('xml+velocity',), (), ('application/xml+velocity',)),
- 'VerilogLexer': ('pip._vendor.pygments.lexers.hdl', 'verilog', ('verilog', 'v'), ('*.v',), ('text/x-verilog',)),
- 'VhdlLexer': ('pip._vendor.pygments.lexers.hdl', 'vhdl', ('vhdl',), ('*.vhdl', '*.vhd'), ('text/x-vhdl',)),
- 'VimLexer': ('pip._vendor.pygments.lexers.textedit', 'VimL', ('vim',), ('*.vim', '.vimrc', '.exrc', '.gvimrc', '_vimrc', '_exrc', '_gvimrc', 'vimrc', 'gvimrc'), ('text/x-vim',)),
- 'WDiffLexer': ('pip._vendor.pygments.lexers.diff', 'WDiff', ('wdiff',), ('*.wdiff',), ()),
- 'WatLexer': ('pip._vendor.pygments.lexers.webassembly', 'WebAssembly', ('wast', 'wat'), ('*.wat', '*.wast'), ()),
- 'WebIDLLexer': ('pip._vendor.pygments.lexers.webidl', 'Web IDL', ('webidl',), ('*.webidl',), ()),
- 'WhileyLexer': ('pip._vendor.pygments.lexers.whiley', 'Whiley', ('whiley',), ('*.whiley',), ('text/x-whiley',)),
- 'X10Lexer': ('pip._vendor.pygments.lexers.x10', 'X10', ('x10', 'xten'), ('*.x10',), ('text/x-x10',)),
- 'XQueryLexer': ('pip._vendor.pygments.lexers.webmisc', 'XQuery', ('xquery', 'xqy', 'xq', 'xql', 'xqm'), ('*.xqy', '*.xquery', '*.xq', '*.xql', '*.xqm'), ('text/xquery', 'application/xquery')),
- 'XmlDjangoLexer': ('pip._vendor.pygments.lexers.templates', 'XML+Django/Jinja', ('xml+django', 'xml+jinja'), (), ('application/xml+django', 'application/xml+jinja')),
- 'XmlErbLexer': ('pip._vendor.pygments.lexers.templates', 'XML+Ruby', ('xml+ruby', 'xml+erb'), (), ('application/xml+ruby',)),
- 'XmlLexer': ('pip._vendor.pygments.lexers.html', 'XML', ('xml',), ('*.xml', '*.xsl', '*.rss', '*.xslt', '*.xsd', '*.wsdl', '*.wsf'), ('text/xml', 'application/xml', 'image/svg+xml', 'application/rss+xml', 'application/atom+xml')),
- 'XmlPhpLexer': ('pip._vendor.pygments.lexers.templates', 'XML+PHP', ('xml+php',), (), ('application/xml+php',)),
- 'XmlSmartyLexer': ('pip._vendor.pygments.lexers.templates', 'XML+Smarty', ('xml+smarty',), (), ('application/xml+smarty',)),
- 'XorgLexer': ('pip._vendor.pygments.lexers.xorg', 'Xorg', ('xorg.conf',), ('xorg.conf',), ()),
- 'XsltLexer': ('pip._vendor.pygments.lexers.html', 'XSLT', ('xslt',), ('*.xsl', '*.xslt', '*.xpl'), ('application/xsl+xml', 'application/xslt+xml')),
- 'XtendLexer': ('pip._vendor.pygments.lexers.jvm', 'Xtend', ('xtend',), ('*.xtend',), ('text/x-xtend',)),
- 'XtlangLexer': ('pip._vendor.pygments.lexers.lisp', 'xtlang', ('extempore',), ('*.xtm',), ()),
- 'YamlJinjaLexer': ('pip._vendor.pygments.lexers.templates', 'YAML+Jinja', ('yaml+jinja', 'salt', 'sls'), ('*.sls',), ('text/x-yaml+jinja', 'text/x-sls')),
- 'YamlLexer': ('pip._vendor.pygments.lexers.data', 'YAML', ('yaml',), ('*.yaml', '*.yml'), ('text/x-yaml',)),
- 'YangLexer': ('pip._vendor.pygments.lexers.yang', 'YANG', ('yang',), ('*.yang',), ('application/yang',)),
- 'ZeekLexer': ('pip._vendor.pygments.lexers.dsls', 'Zeek', ('zeek', 'bro'), ('*.zeek', '*.bro'), ()),
- 'ZephirLexer': ('pip._vendor.pygments.lexers.php', 'Zephir', ('zephir',), ('*.zep',), ()),
- 'ZigLexer': ('pip._vendor.pygments.lexers.zig', 'Zig', ('zig',), ('*.zig',), ('text/zig',)),
- 'apdlexer': ('pip._vendor.pygments.lexers.apdlexer', 'ANSYS parametric design language', ('ansys', 'apdl'), ('*.ans',), ()),
-}
-
-if __name__ == '__main__': # pragma: no cover
- import sys
- import os
-
- # lookup lexers
- found_lexers = []
- sys.path.insert(0, os.path.join(os.path.dirname(__file__), '..', '..'))
- for root, dirs, files in os.walk('.'):
- for filename in files:
- if filename.endswith('.py') and not filename.startswith('_'):
- module_name = 'pygments.lexers%s.%s' % (
- root[1:].replace('/', '.'), filename[:-3])
- print(module_name)
- module = __import__(module_name, None, None, [''])
- for lexer_name in module.__all__:
- lexer = getattr(module, lexer_name)
- found_lexers.append(
- '%r: %r' % (lexer_name,
- (module_name,
- lexer.name,
- tuple(lexer.aliases),
- tuple(lexer.filenames),
- tuple(lexer.mimetypes))))
- # sort them to make the diff minimal
- found_lexers.sort()
-
- # extract useful sourcecode from this file
- with open(__file__) as fp:
- content = fp.read()
- # replace crnl to nl for Windows.
- #
- # Note that, originally, contributers should keep nl of master
- # repository, for example by using some kind of automatic
- # management EOL, like `EolExtension
- # `.
- content = content.replace("\r\n", "\n")
- header = content[:content.find('LEXERS = {')]
- footer = content[content.find("if __name__ == '__main__':"):]
-
- # write new file
- with open(__file__, 'w') as fp:
- fp.write(header)
- fp.write('LEXERS = {\n %s,\n}\n\n' % ',\n '.join(found_lexers))
- fp.write(footer)
-
- print ('=== %d lexers processed.' % len(found_lexers))
diff --git a/spaces/pxiaoer/papers/update_scheduler.py b/spaces/pxiaoer/papers/update_scheduler.py
deleted file mode 100644
index 1eca2c5da0f921cdf79b79a4d4a0f20ee7ca0672..0000000000000000000000000000000000000000
--- a/spaces/pxiaoer/papers/update_scheduler.py
+++ /dev/null
@@ -1,131 +0,0 @@
-import datetime
-import pathlib
-import re
-import tempfile
-import os
-
-import pandas as pd
-import requests
-from apscheduler.schedulers.background import BackgroundScheduler
-from huggingface_hub import HfApi, Repository
-from huggingface_hub.utils import RepositoryNotFoundError
-
-
-class SpaceRestarter:
- def __init__(self, space_id: str):
- self.api = HfApi()
- if self.api.get_token_permission() != "write":
- raise ValueError(f"The HF token must have write permission.")
- try:
- self.api.space_info(repo_id=space_id)
- except RepositoryNotFoundError:
- raise ValueError("The Space ID does not exist.")
- self.space_id = space_id
-
- def restart(self) -> None:
- self.api.restart_space(self.space_id)
-
-
-def find_github_links(summary: str) -> str:
- links = re.findall(r"https://github.com/[^/]+/[^/)}, ]+(?:/(?:tree|blob)/[^/]+/[^/)}, ]+)?", summary)
- if len(links) == 0:
- return ""
- if len(links) != 1:
- raise RuntimeError(f"Found multiple GitHub links: {links}")
- link = links[0]
- if link.endswith("."):
- link = link[:-1]
- link = link.strip()
- return link
-
-
-class RepoUpdater:
- def __init__(self, repo_id: str, repo_type: str):
- api = HfApi()
- if api.get_token_permission() != "write":
- raise ValueError("The HF token must have write permission.")
-
- name = api.whoami()["name"]
-
- repo_dir = pathlib.Path(tempfile.tempdir) / repo_id.split("/")[-1] # type: ignore
- self.csv_path = repo_dir / "papers.csv"
- self.repo = Repository(
- local_dir=repo_dir,
- clone_from=repo_id,
- repo_type=repo_type,
- git_user=name,
- git_email=f"{name}@users.noreply.huggingface.co",
- )
- self.repo.git_pull()
-
- def update(self) -> None:
- yesterday = (datetime.datetime.now() - datetime.timedelta(days=1)).strftime("%Y-%m-%d")
- today = datetime.datetime.now().strftime("%Y-%m-%d")
- daily_papers = [
- {
- "date": yesterday,
- "papers": requests.get(f"https://huggingface.co/api/daily_papers?date={yesterday}").json(),
- },
- {
- "date": today,
- "papers": requests.get(f"https://huggingface.co/api/daily_papers?date={today}").json(),
- },
- ]
-
- self.repo.git_pull()
- df = pd.read_csv(self.csv_path, dtype=str).fillna("")
- rows = [row for _, row in df.iterrows()]
- arxiv_ids = {row.arxiv_id for row in rows}
-
- for d in daily_papers:
- date = d["date"]
- papers = d["papers"]
- for paper in papers:
- arxiv_id = paper["paper"]["id"]
- if arxiv_id in arxiv_ids:
- continue
- try:
- github = find_github_links(paper["paper"]["summary"])
- except RuntimeError as e:
- print(e)
- continue
- rows.append(
- pd.Series(
- {
- "date": date,
- "arxiv_id": arxiv_id,
- "github": github,
- }
- )
- )
- df = pd.DataFrame(rows).reset_index(drop=True)
- df.to_csv(self.csv_path, index=False)
-
- def push(self) -> None:
- self.repo.push_to_hub()
-
-
-class UpdateScheduler:
- def __init__(self, space_id: str, cron_hour: str, cron_minute: str, cron_second: str = "0"):
- self.space_restarter = SpaceRestarter(space_id=space_id)
- self.repo_updater = RepoUpdater(repo_id=space_id, repo_type="space")
-
- self.scheduler = BackgroundScheduler()
- self.scheduler.add_job(
- func=self._update,
- trigger="cron",
- hour=cron_hour,
- minute=cron_minute,
- second=cron_second,
- timezone="UTC",
- )
-
- def _update(self) -> None:
- self.repo_updater.update()
- if self.repo_updater.repo.is_repo_clean():
- self.space_restarter.restart()
- else:
- self.repo_updater.push()
-
- def start(self) -> None:
- self.scheduler.start()
diff --git a/spaces/quidiaMuxgu/Expedit-SAM/ANSYS Electronics 2020 R1 With Local Help MCAD Translators X64 [UPD] Free Download.md b/spaces/quidiaMuxgu/Expedit-SAM/ANSYS Electronics 2020 R1 With Local Help MCAD Translators X64 [UPD] Free Download.md
deleted file mode 100644
index 623045cea6c2497adf6e862772992cc70a6a604e..0000000000000000000000000000000000000000
--- a/spaces/quidiaMuxgu/Expedit-SAM/ANSYS Electronics 2020 R1 With Local Help MCAD Translators X64 [UPD] Free Download.md
+++ /dev/null
@@ -1,22 +0,0 @@
-
ANSYS Electronics 2020 R1 With Local Help MCAD Translators x64 Free Download
-
-electronics 2020 r1 with local help mcad translators x64
-
-MCAD Translator Pro 2020 includes a set of 84 translation tools, including 2D and 3D CAD drawing, animation,. Dimensioning, 2D drafting, and other engineering activities help the beginner quickly learn to use the MCAD. MCADCafe.com delivers the latest MCAD industry commentary, news, product reviews,.
-
-MCAD Cafe's forums are. local helpers don't even know that MCADCafe is free MCADCafe's are always busy. Someone on their list said "local MCAD helpers can't help but know MCADCafe.
-
-You can download a free version of. Translators. The translator offers. The MCADCafe community." - Plus many more. "MCADCafe is a.
-
-Wanted MCAD engineers/local helpers and CAD translators? Save $1.00. We need local assistance in Guiyang, China. I just want to share some other tools that I use every.
-
-MCADCafe.com is a professional website design & development company. MCADCafe specializes in web solutions for small to medium sized businesses and non-profit organizations. We can offer you custom web design, web development and custom hosting solutions at affordable prices. Web design, web development, php programming, script customization, custom ecommerce website development and responsive website design are among our main services. MCADCafe has been offering professional website design and development services in China for over 10 years. We offer all our clients.MCAD Cafe - CAD Cafe, CAD, CAD Cafe, help with CAD, web design & development, CAD, CAD Cafe, MCAD Cafe, CAD Cafe, CAD Cafe.
-
-You can download a free version of MCAD Cafe Pro and try it for 30 days. Click on the button to download it now:. You can download a free version of MCAD Cafe Pro and try it for 30 days. Click on the button to download it now:.
-
-MCADCafe.com provides professional website design and development services. We can offer you custom web design, web development and custom hosting solutions at affordable prices.. Contact us today! A good design requires a good understanding of a business'.
-
-The MCADCafe.com website development team can help you with. that you found on the MCADCafe website.. or MCAD local helpers can help you out 4fefd39f24
-
-
-
diff --git a/spaces/quidiaMuxgu/Expedit-SAM/Cinderella Monogatari English Su.md b/spaces/quidiaMuxgu/Expedit-SAM/Cinderella Monogatari English Su.md
deleted file mode 100644
index 9a8e282c5820a07c3118592a5efecb8bcc0c6063..0000000000000000000000000000000000000000
--- a/spaces/quidiaMuxgu/Expedit-SAM/Cinderella Monogatari English Su.md
+++ /dev/null
@@ -1,5 +0,0 @@
-
-
Title: / Meng Qi Shi Shen English title: Cinderella Chef Genre:.... Cinderella Monogatari English Su:. young and capable, workaholic, a very studious young man, a person who loves food and cooking, but it’s also a girl whose homework and cooking is always neglected by his mother.... Cinderella Monogatari English Su:. hero is also recognized as a fellow of the society and has a dark side. But he loves to observe other people secretly.... Cinderella Monogatari English Su: Cinderella monogatari is a female division of "popular story". Cinderella girls are usually sent to the services.... Cinderella Monogatari English Su: Cinderella is not an ordinary Cinderella. This Cinderella girl is not a princess, so she can only attend the wedding party. In the end, she meets the prince for the first time.... Cinderella Monogatari English Su:..... Title: / Meng Qi Shi Shen English title: Cinderella Chef Genre:.... Being a Cinderella means that an unknown power protect the girl and is always ready to protect you. Cinderella Monogatari English Su, Cinderella Monogatari English Su MGP Hunter 4..... Cinderella Monogatari English Su, Cinderella Monogatari English Su MGP Hunter 4..... The Vanishing (2014) CiCuChi 1 2 3 4 Cinderella Monogatari English Su.... Hikaru no Go Cinderella Monogatari English Su.... Reideen Cinderella Monogatari English Su.... Cinderella Monogatari English Su: As the only daughter in the family, Cinderella must live with her own, loving mother. Her father is a long-distance runner, and spends most of his time at the faraway races. Her mother.... Cinderella Monogatari English Su: Cinderella "says of the royal family, "I can stay at home, but they can't stay at home. " Cinderella... Cinderella Monogatari English Su.... Alone (2005) 25 28 13 20 Cinderella Monogatari English Su. Cinderella Monogatari English Su MGP Hunter 4..... Cinderella Monogatari English Su. Cinderella Monogatari English Su MGP Hunter 4..... Attack on Titan: Armored Warfare 2 Frontline Bypass Strategy. Cinderella Monogatari English Su.... Cinderella Monogatari English Su MGP Hunter 4..... Cinderella Monogatari English Su MGP Hunter 4..... Cinderella Monogatari English Su MGP Hunter 4..... Cinderella Monogatari English Su MGP Hunter 4..... Cinderella Monogatari English Su.... Mother & Daughter.... The Girls from Paradise (2008).... Cinderella Monogatari English Su.... Cinderella Monogatari English Su MGP Hunter 4..... Cinderella Monogatari English Su.... Cinderella Monogatari English Su.... Cinderella Monogatari English Su.... Cinderella Monogatari English Su.... Cinderella Monogatari English Su. Cinderella Monogatari English Su.... Cinderella Monogatari English Su.... Hagakure Anime English Sub Tue. 31 Jul 2017 18:06. tbsplus. Cinderella Monogatari (Cinderella Monogatari, 春刀梨紗, Shunkagure wakuranai Cinderella) is a 2006 South Korean supernatural horror film. It was directed by Kim Tae-kyung, who also wrote the script. Cinderella was the only girl from her father who lived with her stepmother and step-sisters in her house. Her stepmother and step sister used to harass Cinderella and make her do all the household work. One day a message was sent from the royal house in the city that the prince is looking for a girl for his wedding. Because of which he invited all the girls of the city to the ball party.
899543212b
-
-
\ No newline at end of file
diff --git a/spaces/quidiaMuxgu/Expedit-SAM/Ganapathi Thalam Tamil Pdf 27.md b/spaces/quidiaMuxgu/Expedit-SAM/Ganapathi Thalam Tamil Pdf 27.md
deleted file mode 100644
index ec3d8d34182618b5092a640f81ac1a87ba38432e..0000000000000000000000000000000000000000
--- a/spaces/quidiaMuxgu/Expedit-SAM/Ganapathi Thalam Tamil Pdf 27.md
+++ /dev/null
@@ -1,6 +0,0 @@
-
-
-27.Major Texts on Dance that you know . 28.Melakartha Raga Table and its relevance in Carnatic Music. [2X15=30 MARKS] ... Jathiswaram – Ragam-Vasantha, Thalam-Roopakam-(24 Hrs) ... Page 32. • Ganapathi sthuthi / Thodayam- ( 24 Hrs) ... Kummi is a folk dance common in both Kerala and Tamil Nadu. Explain. 18. 1fdad05405
-
-
-
diff --git a/spaces/quidiaMuxgu/Expedit-SAM/Garritan ARIA Player V1.504 HAPPY NEW YEAR - R2R [deepstatus][13 Crack [HOT].md b/spaces/quidiaMuxgu/Expedit-SAM/Garritan ARIA Player V1.504 HAPPY NEW YEAR - R2R [deepstatus][13 Crack [HOT].md
deleted file mode 100644
index 66ad20b287b285eadf0f51178295e85e1203f14a..0000000000000000000000000000000000000000
--- a/spaces/quidiaMuxgu/Expedit-SAM/Garritan ARIA Player V1.504 HAPPY NEW YEAR - R2R [deepstatus][13 Crack [HOT].md
+++ /dev/null
@@ -1,6 +0,0 @@
-
Garritan ARIA Player V1.504 HAPPY NEW YEAR - R2R [deepstatus][13 Crack
-
-504 HAPPY NEW YEAR - R2R [deepstatus][13 setup free Hamster Free Burning Studio 1.0.9.9 [Jazz_Singh] crack. GWMicro Window.... "H:\Xoft\Arturia Moog ... 1fdad05405
-
-
-
diff --git a/spaces/quidiaMuxgu/Expedit-SAM/HD Online Player (apocalypto Hollywood Movie Hindi Dub) VERIFIED.md b/spaces/quidiaMuxgu/Expedit-SAM/HD Online Player (apocalypto Hollywood Movie Hindi Dub) VERIFIED.md
deleted file mode 100644
index 040dde4b0ab5ec61c68e36d11de63cb21160b7a8..0000000000000000000000000000000000000000
--- a/spaces/quidiaMuxgu/Expedit-SAM/HD Online Player (apocalypto Hollywood Movie Hindi Dub) VERIFIED.md
+++ /dev/null
@@ -1,34 +0,0 @@
-
-
How to Watch Apocalypto in Hindi Dubbed Online for Free
-
-
Apocalypto is a 2006 epic adventure film directed by Mel Gibson that depicts the violent and brutal invasion of a Mayan civilization by a rival tribe. The film is known for its stunning cinematography, intense action scenes, and authentic portrayal of the ancient culture. Apocalypto was nominated for three Academy Awards and won several other accolades.
-
HD Online Player (apocalypto hollywood movie hindi dub)
If you are a fan of historical dramas and want to watch Apocalypto in Hindi dubbed online for free, you have come to the right place. In this article, we will show you how to stream Apocalypto in HD quality with Hindi audio using an online player that works on any device. You don't need to download anything or register for any service. Just follow these simple steps and enjoy the movie.
-
-
Step 1: Visit Showmyflix.com
-
-
Showmyflix.com is a website that offers free full HD Hollywood dubbed movies in Hindi. You can find hundreds of movies from different genres and years on this site. Showmyflix.com has a user-friendly interface and fast loading speed. You can also browse movies by categories, ratings, or alphabets.
-
-
To watch Apocalypto in Hindi dubbed online for free, go to Showmyflix.com and type "Apocalypto" in the search box. You will see the movie poster and a play button. Click on the play button to start streaming the movie.
-
-
Step 2: Enjoy Apocalypto in HD Quality with Hindi Audio
-
-
Once you click on the play button, you will be redirected to a new tab where the movie will start playing automatically. You can adjust the volume, brightness, and screen size according to your preference. You can also pause, resume, or rewind the movie as you wish.
-
-
-
The best part is that you can watch Apocalypto in HD quality with Hindi audio without any interruption or buffering. The online player is compatible with any device, such as laptop, smartphone, tablet, or smart TV. You can also connect your device to a bigger screen using HDMI cable or Chromecast.
-
-
Step 3: Share Your Feedback and Explore More Movies
-
-
After watching Apocalypto in Hindi dubbed online for free, don't forget to share your feedback and rating on Showmyflix.com. You can also leave a comment or a review to express your opinion about the movie. This will help other users to decide whether to watch it or not.
-
-
If you liked Apocalypto, you might also enjoy other Hollywood dubbed movies in Hindi on Showmyflix.com. You can explore more movies by genre, year, or popularity. Some of the popular movies on Showmyflix.com are Bloodshot, Money Plane, The Protector, The Witch, and many more.
-
-
Conclusion
-
-
Apocalypto is a masterpiece of cinema that deserves to be watched by everyone who loves history, culture, and adventure. If you want to watch Apocalypto in Hindi dubbed online for free, you can use Showmyflix.com as your online player. Showmyflix.com offers free full HD Hollywood dubbed movies in Hindi that you can stream on any device without any hassle.
-
-
So what are you waiting for? Go to Showmyflix.com and watch Apocalypto in Hindi dubbed online for free now!
d5da3c52bf
-
-
\ No newline at end of file
diff --git a/spaces/quidiaMuxgu/Expedit-SAM/Laser Cut 53 Donglerar.md b/spaces/quidiaMuxgu/Expedit-SAM/Laser Cut 53 Donglerar.md
deleted file mode 100644
index ac6632cb6a57ff15baafac0cdf3e7d74331bdcf9..0000000000000000000000000000000000000000
--- a/spaces/quidiaMuxgu/Expedit-SAM/Laser Cut 53 Donglerar.md
+++ /dev/null
@@ -1,46 +0,0 @@
-
-
How to Use the Laser Cut 53 Donglerar for Precision Cutting
-
If you are looking for a reliable and versatile tool for cutting various materials, you might want to consider the Laser Cut 53 Donglerar. This device uses a powerful laser beam to cut through wood, metal, plastic, and more with high accuracy and speed. In this article, we will show you how to use the Laser Cut 53 Donglerar for your cutting projects and what benefits it offers.
-
What is the Laser Cut 53 Donglerar?
-
The Laser Cut 53 Donglerar is a handheld device that can cut through different materials using a laser beam. The device has a built-in battery that can last for up to an hour of continuous use. The device also has a digital display that shows the cutting settings and the battery level. The device has a safety switch that prevents accidental activation of the laser.
To use the Laser Cut 53 Donglerar, you need to follow these steps:
-
-
Charge the device using the USB cable provided. The device will indicate when it is fully charged.
-
Select the material you want to cut and place it on a flat surface. Make sure the material is not flammable or reflective.
-
Turn on the device and adjust the cutting settings according to the material thickness and type. You can use the buttons and the display to change the settings.
-
Hold the device firmly and point the laser beam at the material. Press the trigger to start cutting. Move the device along the desired path slowly and steadily.
-
Release the trigger to stop cutting. Turn off the device and let it cool down before storing it.
-
-
What are the Benefits of Using the Laser Cut 53 Donglerar?
-
Using the Laser Cut 53 Donglerar has many advantages, such as:
-
-
It can cut through various materials with ease and precision.
-
It is portable and easy to use.
-
It has a long battery life and a low power consumption.
-
It has a safety switch and a protective cover to prevent injuries.
-
It has a digital display and buttons to adjust the cutting settings.
-
-
Where to Buy the Laser Cut 53 Donglerar?
-
If you are interested in buying the Laser Cut 53 Donglerar, you can order it online from our website. We offer free shipping and a 30-day money-back guarantee. You can also contact us if you have any questions or feedback about our product. We are always happy to hear from our customers.
-
-
How to Maintain the Laser Cut 53 Donglerar?
-
To keep the Laser Cut 53 Donglerar in good condition, you need to follow these maintenance tips:
-
-
Clean the device regularly with a soft cloth. Do not use water or any abrasive materials.
-
Store the device in a cool and dry place. Avoid exposing it to direct sunlight or extreme temperatures.
-
Replace the battery when it is low or damaged. Do not overcharge the device or use a different charger.
-
Do not disassemble or modify the device. If you encounter any problems, contact our customer service.
-
-
What are the Safety Precautions for Using the Laser Cut 53 Donglerar?
-
The Laser Cut 53 Donglerar is a powerful device that can cause serious injuries if not used properly. Therefore, you need to follow these safety precautions when using it:
-
-
Wear protective goggles and gloves when using the device. The laser beam can damage your eyes and skin.
-
Do not point the laser beam at yourself or others. The laser beam can cause burns and fires.
-
Do not use the device near flammable or explosive materials. The laser beam can ignite them.
-
Do not use the device near electrical devices or wires. The laser beam can interfere with them.
-
Do not use the device in wet or humid conditions. The device can malfunction or short-circuit.
How to Root Your Toshiba Thrive Tablet with DaleNet Universal Easy Flash Tool
-
Introduction
-
Do you own a Toshiba Thrive tablet and want to unleash its full potential? If so, you may be interested in rooting it with DaleNet Universal Easy Flash Tool. This is a simple yet powerful tool that can root any ROM build for your device in a matter of minutes. In this article, I will show you how to use this tool to root your tablet, unroot it if
b2dd77e56b
-
-
\ No newline at end of file
diff --git a/spaces/raedeXanto/academic-chatgpt-beta/Data Interpretation by Arun Sharma PDF Download Improve Your Speed and Accuracy in DI and LR Questions.md b/spaces/raedeXanto/academic-chatgpt-beta/Data Interpretation by Arun Sharma PDF Download Improve Your Speed and Accuracy in DI and LR Questions.md
deleted file mode 100644
index 4dd7798530b3aa5e6febbc1022d93ec792740c4a..0000000000000000000000000000000000000000
--- a/spaces/raedeXanto/academic-chatgpt-beta/Data Interpretation by Arun Sharma PDF Download Improve Your Speed and Accuracy in DI and LR Questions.md
+++ /dev/null
@@ -1,114 +0,0 @@
-
- - Who is the author? - What are the features of the book? | | Why should you read this book? | - Why are data interpretation and logical reasoning important for CAT? - How will this book help you master these sections? - What are the benefits of reading this book? | | How to read this book? | - How is the book structured? - What are the steps to follow while reading this book? - How to use the additional resources provided by the book? | | Conclusion | - Summarize the main points of the article - Provide a call to action for readers | | FAQs | - Answer some common questions related to the book | ## Table 2: Article with HTML formatting ```html
Data Interpretation and Logical Reasoning by Arun Sharma PDF Download
-
Are you looking for a comprehensive guide to ace the data interpretation and logical reasoning sections of the CAT exam? Do you want to learn the concepts, techniques and strategies to solve various types of questions in these sections? If yes, then you should check out the book Data Interpretation and Logical Reasoning by Arun Sharma.
-
This book is one of the best-selling books for CAT preparation and has been updated with the latest syllabus and pattern of the exam. It covers all the topics and subtopics of data interpretation and logical reasoning, such as tables, charts, graphs, puzzles, arrangements, games, syllogisms, deductions, etc. It also provides tips and tricks to improve your speed and accuracy while solving the questions.
The book is divided into two parts: data interpretation and logical reasoning. Each part has four sections: basic mode, intermediate mode, advanced mode and test papers. The basic mode introduces the concepts and fundamentals of each topic with solved examples. The intermediate mode provides more practice questions with varying levels of difficulty. The advanced mode challenges you with high-level questions that test your analytical and critical thinking skills. The test papers simulate the actual CAT exam format and help you assess your performance and progress.
-
The book also comes with a CD-ROM that contains more than 1000 practice questions with detailed solutions. You can also access online mock tests and video lectures by the author on the publisher's website. The book is suitable for both beginners and experts who want to master data interpretation and logical reasoning for CAT.
-
Why should you read this book?
-
Data interpretation and logical reasoning are two of the most important and scoring sections of the CAT exam. They test your ability to interpret data, analyze information, draw conclusions, make decisions and solve problems. These skills are not only essential for cracking the CAT exam but also for succeeding in your academic and professional career.
-
However, many students find these sections difficult and confusing because they involve complex calculations, tricky logic and unfamiliar formats. They often end up wasting time and making errors while attempting these questions. This can affect their overall score and rank in the exam.
-
That's why you need a book like Data Interpretation and Logical Reasoning by Arun Sharma. This book will help you overcome your fears and doubts about these sections and boost your confidence and competence. It will teach you how to approach each type of question with clarity and precision. It will also expose you to a variety of questions that can appear in the exam and prepare you for any surprises or twists.
-
By reading this book, you will be able to:
-
-
Understand the concepts and principles of data interpretation and logical reasoning
-
Apply the techniques and methods to solve different kinds of questions
-
Improve your speed and accuracy while solving the questions
-
Avoid common mistakes and pitfalls that can cost you marks
-
Enhance your analytical and critical thinking skills
-
Score high marks in data interpretation and logical reasoning sections of CAT
-
-
How to read this book?
-
This book is designed to help you learn at your own pace and convenience. You can follow these steps to get the most out of this book:
-
data interpretation by arun sharma pdf free download
-data interpretation by arun sharma ebook download
-data interpretation by arun sharma for cat pdf download
-data interpretation by arun sharma latest edition pdf download
-data interpretation by arun sharma book pdf download
-data interpretation by arun sharma online pdf download
-data interpretation by arun sharma solutions pdf download
-data interpretation by arun sharma mcgraw hill pdf download
-data interpretation by arun sharma quora pdf download
-data interpretation by arun sharma flipkart pdf download
-data interpretation by arun sharma amazon pdf download
-data interpretation by arun sharma review pdf download
-data interpretation by arun sharma logical reasoning pdf download
-data interpretation by arun sharma verbal ability pdf download
-data interpretation by arun sharma quantitative aptitude pdf download
-data interpretation by arun sharma 8th edition pdf download
-data interpretation by arun sharma 7th edition pdf download
-data interpretation by arun sharma 6th edition pdf download
-data interpretation by arun sharma 5th edition pdf download
-data interpretation by arun sharma 4th edition pdf download
-data interpretation by arun sharma 3rd edition pdf download
-data interpretation by arun sharma 2nd edition pdf download
-data interpretation by arun sharma 1st edition pdf download
-how to prepare for data interpretation by arun sharma pdf download
-how to solve data interpretation by arun sharma pdf download
-how to study data interpretation by arun sharma pdf download
-how to master data interpretation by arun sharma pdf download
-how to crack data interpretation by arun sharma pdf download
-best book for data interpretation by arun sharma pdf download
-complete guide for data interpretation by arun sharma pdf download
-comprehensive course for data interpretation by arun sharma pdf download
-concise notes for data interpretation by arun sharma pdf download
-practice questions for data interpretation by arun sharma pdf download
-mock tests for data interpretation by arun sharma pdf download
-previous year papers for data interpretation by arun sharma pdf download
-tips and tricks for data interpretation by arun sharma pdf download
-shortcuts and formulas for data interpretation by arun sharma pdf download
-concepts and techniques for data interpretation by arun sharma pdf download
-basics and fundamentals for data interpretation by arun sharma pdf download
-advanced topics for data interpretation by arun sharma pdf download
-case studies for data interpretation by arun sharma pdf download
-charts and graphs for data interpretation by arun sharma pdf download
-tables and pie charts for data interpretation by arun sharma pdf download
-bar graphs and line charts for data interpretation by arun sharma pdf download
-mixed graphs and radar charts for data interpretation by arun sharma pdf download
-missing data and venn diagrams for data interpretation by arun sharma pdf download
-games and tournaments for data interpretation by arun sharma pdf download
-profit and loss for data interpretation by arun sharma pdf download
-ratio and proportion for data interpretation by arun sharma pdf download
-
-
Start with the basic mode of each topic and review the concepts and fundamentals with solved examples.
-
Move on to the intermediate mode of each topic and practice more questions with varying levels of difficulty.
-
Attempt the advanced mode of each topic and challenge yourself with high-level questions that test your analytical and critical thinking skills.
-
Solve the test papers at the end of each part and evaluate your performance and progress.
-
Use the CD-ROM or online resources to access more practice questions, mock tests and video lectures by the author.
-
Revise the topics regularly and keep track of your strengths and weaknesses.
-
Seek feedback from experts or peers if you have any doubts or queries.
-
-
Conclusion
-
In conclusion, Data Interpretation and Logical Reasoning by Arun Sharma is a must-read book for anyone who wants to ace the data interpretation and logical reasoning sections of the CAT exam. It covers all the topics and subtopics of these sections with clear explanations, solved examples and practice questions. It also provides tips and tricks to improve your speed and accuracy while solving the questions. It also comes with a CD-ROM and online resources that offer more practice questions, mock tests and video lectures by the author. The book is suitable for both beginners and experts who want to master data interpretation and logical reasoning for CAT.
-
If you are interested in buying this book, you can download the PDF version from the links provided in the web search results . Alternatively, you can also buy the paperback or Kindle version from Amazon or Flipkart. The book is priced at Rs. 599 for the paperback and Rs. 449 for the Kindle edition.
-
So, what are you waiting for? Grab your copy of Data Interpretation and Logical Reasoning by Arun Sharma today and start preparing for your CAT exam with confidence and competence.
-
FAQs
-
-
Who is Arun Sharma?
-
Arun Sharma is a renowned author, trainer and mentor for CAT aspirants. He has more than 20 years of experience in teaching and coaching students for various competitive exams. He is also an alumnus of IIM Bangalore and has worked with top companies like Tata Steel, Tata Motors and Wipro.
-
What are the other books by Arun Sharma?
-
Arun Sharma has written several books for CAT preparation, such as How to Prepare for Quantitative Aptitude for CAT, How to Prepare for Verbal Ability and Reading Comprehension for CAT, Study Package for CAT & Other MBA Entrance Exams, etc. He has also written books for other exams like GMAT, XAT, SNAP, IIFT, etc.
-
How to prepare for data interpretation and logical reasoning for CAT?
-
To prepare for data interpretation and logical reasoning for CAT, you need to follow these steps:
-
-
Understand the syllabus and pattern of these sections and familiarize yourself with the types of questions that can appear in the exam.
-
Learn the concepts and principles of data interpretation and logical reasoning from a reliable source like Data Interpretation and Logical Reasoning by Arun Sharma.
-
Practice as many questions as possible from different sources like books, online platforms, mock tests, etc.
-
Improve your speed and accuracy while solving the questions by using shortcuts, techniques and methods.
-
Analyze your performance and progress regularly and identify your strengths and weaknesses.
-
Revise the topics frequently and clear your doubts or queries from experts or peers.
-
-
What are the benefits of data interpretation and logical reasoning skills?
-
Data interpretation and logical reasoning skills are not only useful for cracking the CAT exam but also for succeeding in your academic and professional career. Some of the benefits of these skills are:
-
-
They help you to interpret data, analyze information, draw conclusions, make decisions and solve problems effectively.
-
They enhance your analytical and critical thinking skills that are essential for innovation and creativity.
-
They improve your communication and presentation skills that are vital for expressing your ideas and opinions clearly.
-
They develop your mental agility and flexibility that are required for adapting to changing situations and environments.
-
They boost your confidence and competence that are necessary for achieving your goals and aspirations.
-
-
How to download the PDF version of Data Interpretation and Logical Reasoning by Arun Sharma?
-
You can download the PDF version of Data Interpretation and Logical Reasoning by Arun Sharma from the links provided in the web search results . You will need a PDF reader software like Adobe Acrobat Reader or Foxit Reader to open the file. You can also print or save the file on your device for future reference.
- 0a6ba089eb
-
-
\ No newline at end of file
diff --git a/spaces/raedeXanto/academic-chatgpt-beta/Fifa 2020 Crack Tips and Tricks to Improve Your Performance and Skills.md b/spaces/raedeXanto/academic-chatgpt-beta/Fifa 2020 Crack Tips and Tricks to Improve Your Performance and Skills.md
deleted file mode 100644
index 46f46cd513ebb0fc561bf0d3ed6e3d9a93b439b6..0000000000000000000000000000000000000000
--- a/spaces/raedeXanto/academic-chatgpt-beta/Fifa 2020 Crack Tips and Tricks to Improve Your Performance and Skills.md
+++ /dev/null
@@ -1,145 +0,0 @@
-
-
FIFA 2020 Crack: How to Download and Play the Latest Version of FIFA for Free
-
Introduction
-
If you are a fan of soccer games, you probably have heard of FIFA 2020, the latest installment of the popular FIFA series. FIFA 2020 is a simulation game that lets you play as your favorite teams and players in various modes and competitions. You can also create your own custom teams and players, and customize your gameplay experience with various options and settings.
-
However, FIFA 2020 is not a free game. You need to buy it from an official store or platform, such as Origin, Steam, or PlayStation Store. The price of FIFA 2020 varies depending on your region and platform, but it is usually around $60. That's not a cheap price for many people, especially if you are on a tight budget or have other expenses to worry about.
So, what can you do if you want to play FIFA 2020 without paying for it? Well, there is a way to do that. It's called FIFA 2020 Crack. In this article, we will explain what FIFA 2020 Crack is, how to download it, and how to play it. We will also answer some frequently asked questions about FIFA 2020 Crack. Let's get started!
-
What is FIFA 2020?
-
FIFA 2020 is a soccer simulation game developed by EA Sports and published by Electronic Arts. It was released on September 27, 2019 for Microsoft Windows, PlayStation 4, Xbox One, and Nintendo Switch. It is the 27th edition of the FIFA series, and the first one to feature the UEFA Champions League and Europa League licenses.
-
FIFA 2020 has many features and improvements over its predecessors, such as:
-
-
Better graphics and animations
-
More realistic gameplay and physics
-
New game modes, such as Volta Football and Career Mode
-
More licensed teams, leagues, stadiums, and players
-
More options and customization for teams, players, kits, tactics, and strategies
-
More online and offline modes and competitions
-
More social and interactive features, such as clubs, tournaments, leaderboards, and rewards
-
-
FIFA 2020 is widely praised by critics and players alike for its gameplay quality, content variety, and presentation style. It has won several awards and nominations, such as Best Sports Game at The Game Awards 2019. It has also sold over 25 million copies worldwide as of May 2020.
-
What is FIFA 2020 Crack?
-
FIFA 2020 Crack is a modified version of FIFA 2020 that bypasses the game's security system and allows you to play it without buying it or activating it online. It is also known as FIFA 20 CPY Crack or FIFA 20 Codex Crack, after the names of the groups that created it.
-
Fifa 20 Ultimate Edition Free Download
-Fifa 20 Torrent Link
-Fifa 20 Repack by Fitgirl
-Fifa 20 Cracked Games
-Fifa 20 Volta Mode
-Fifa 20 Customise Character
-Fifa 20 Futsal Rules
-Fifa 20 Weather Impact
-Fifa 20 Champions Edition
-Fifa 20 Virgil van Dijk Cover
-Fifa 20 Ultimate Edition Zinedine Zidane Cover
-Fifa 20 Full Crack Download
-Fifa 20 Game Bai Top
-Fifa 20 Patch Download
-Fifa 20 Crack Armory
-Fifa 20 PC Download Free
-Fifa 20 Highly Compressed
-Fifa 20 EA Access
-Fifa 20 Frostbite Engine
-Fifa 20 Football Intelligence
-Fifa 20 Gameplay Realism
-Fifa 20 FIFA Ultimate Team
-Fifa 20 Denuvo Protection
-Fifa 20 System Requirement
-Fifa 20 Install Instructions
-Fifa 20 Career Mode
-Fifa 20 Online Multiplayer
-Fifa 20 New Features
-Fifa 20 Skill Moves
-Fifa 20 Street Football
-Fifa 20 Small Sided Games
-Fifa 20 Authentic Stadiums
-Fifa 20 Real Life Teams
-Fifa 20 Official Licenses
-Fifa 20 Dynamic Tactics
-Fifa 20 Timed Finishing
-Fifa 20 Active Touch System
-Fifa 20 Player Ratings
-Fifa 20 Demo Download
-Fifa 20 Release Date
-Fifa 20 Pre Order Bonus
-Fifa 20 Eden Hazard Cover
-Fifa 20 Soundtrack List
-Fifa 20 Review and Rating
-Fifa 20 Tips and Tricks
-Fifa 20 Best Formation
-Fifa 20 Coins Generator
-Fifa 20 Mods and Cheats
-Fifa 20 Update Version
-
FIFA 2020 Crack works by replacing or modifying some files in the game folder that are responsible for checking the game's license and authentication. By doing so, it tricks the game into thinking that it is a legitimate copy that has been purchased and activated online.
-
FIFA 2020 Crack does not affect the game's performance or functionality in any way. You can still play all the modes and features of the game as if you had bought it from an official store or platform. You can also update the game with patches and fixes that are released by EA Sports.
-
Why do people use FIFA 2020 Crack?
-
There are many reasons why people use FIFA 2020 Crack instead of buying the game from an official store or platform. Some of them are:
-
-
They want to save money. Buying FIFA 2020 can be expensive for some people, especially if they live in regions where the game's price is higher than average or if they have other financial obligations to meet.
-
They want to try before they buy. Some people are not sure if they will like FIFA 2020 or if it will run well on their devices. They want to test the game first before they decide whether to buy it or not.
-
They want to support piracy. Some people are against the practices of EA Sports or Electronic Arts as a company. They think that they are greedy, unfair, or unethical in their business models or policies. They want to boycott their products or protest against them by using pirated versions of their games.
-
They want to have fun. Some people just enjoy playing games without paying for them or following any rules or restrictions. They want to have more freedom and flexibility in their gaming experience.
-
-
Whatever the reason may be, using FIFA 2020 Crack is illegal and risky. It violates the terms of service and end-user license agreement of EA Sports and Electronic Arts. It also exposes you to potential malware or viruses that may harm your device or data. It may also cause compatibility issues or errors with your game or device.
-
How to Download FIFA 2020 Crack
-
If you still want to use FIFA 2020 Crack despite the risks and consequences, you need to follow some steps to download and install it on your device. Here are the steps:
-
Step 1: Find a reliable source for FIFA 2020 Crack
-
The first step is to find a website or platform that offers FIFA 2020 Crack for download. There are many websites and platforms that claim to have FIFA 2020 Crack, but not all of them are trustworthy or safe. Some of them may contain fake or corrupted files, malware or viruses, or hidden fees or surveys.
-
To avoid these problems, you need to do some research and check the reputation and reviews of the website or platform before you download anything from it. You can also use some tools or software that can scan and verify the files for any potential threats or issues.
-
Some of the websites or platforms that are known to have FIFA 2020 Crack are:
-
-
CPY Games
-
Codex Games
-
Skidrow Games
-
Ocean of Games
-
FitGirl Repacks
-
-
However, we do not endorse or recommend any of these websites or platforms. Use them at your own risk and discretion.
-
Step 2: Download the FIFA 2020 Crack file
-
The second step is to download the FIFA 2020 Crack file from the website or platform that you have chosen. The file size may vary depending on the source, but it is usually around 50 GB. You need to have enough space on your device and a stable internet connection to download the file.
-
The file may be in a compressed or archived format, such as ZIP, RAR, or ISO. You need to have a software that can extract or mount these files, such as WinRAR, 7-Zip, or Daemon Tools.
-
The file may also be split into several parts or segments. You need to download all the parts or segments and put them in the same folder before you extract or mount them.
-
Step 3: Extract or mount the FIFA 2020 Crack file
-
The third step is to extract or mount the FIFA 2020 Crack file that you have downloaded. If the file is in a compressed or archived format, such as ZIP or RAR, you need to right-click on it and choose Extract Here or Extract to Folder. This will create a new folder with the extracted files inside.
-
If the file is in an image format, such as ISO, you need to right-click on it and choose Mount Image. This will create a virtual drive with the mounted files inside.
-
Step 4: Copy and paste the FIFA 2020 Crack file into the game folder
-
The fourth step is to copy and paste the FIFA 2020 Crack file into the game folder. The game folder is where you have installed FIFA 2020 on your device. You can find it by following this path:
-
-
C:\Program Files (x86)\Origin Games\FIFA 20\
-
-
You need to copy and paste the FIFA 2020 Crack file into this folder and replace any existing files with the same name. You may need to grant administrator permission to do this.
-
How to Play FIFA 2020 Crack
-
After you have completed the steps above, you are ready to play FIFA 2020 Crack on your device. Here are the steps:
-
Step 1: Run the FIFA 2020 Crack file as administrator
-
The first step is to run the FIFA 2020 Crack file as administrator. You can do this by right-clicking on it and choosing Run as Administrator. This will launch the game with full access and privileges.
-
Step 2: Choose your language and settings
-
The second step is to choose your language and settings for the game. You can do this by following the instructions on the screen. You can also change your language and settings later by going to Options > Game Settings > Language.
-
Step 3: Enjoy the game!
-
The third step is to enjoy the game! You can play all the modes and features of FIFA 2020 as if you had bought it from an official store or platform. You can also update the game with patches and fixes that are released by EA Sports.
-
Conclusion
-
In this article, we have explained what FIFA 2020 Crack is, how to download it, and how to play it. We have also answered some frequently asked questions about FIFA 2020 Crack.
-
FAQs
-
Here are some of the frequently asked questions about FIFA 2020 Crack:
-
Is FIFA 2020 Crack safe to use?
-
No, FIFA 2020 Crack is not safe to use. It may contain malware or viruses that can harm your device or data. It may also cause compatibility issues or errors with your game or device. It may also expose you to legal actions or penalties from EA Sports or Electronic Arts.
-
Is FIFA 2020 Crack free to use?
-
No, FIFA 2020 Crack is not free to use. Although you do not have to pay for the game itself, you may have to pay for other costs or risks associated with using FIFA 2020 Crack, such as:
-
-
Downloading fees or charges from the website or platform that offers FIFA 2020 Crack
-
Malware or virus removal fees or charges from your device or data
-
Repair or replacement fees or charges for your device or game
-
Legal fees or fines from EA Sports or Electronic Arts
-
-
Is FIFA 2020 Crack legal to use?
-
No, FIFA 2020 Crack is not legal to use. It violates the terms of service and end-user license agreement of EA Sports and Electronic Arts. It also infringes the intellectual property rights and copyrights of EA Sports and Electronic Arts. It may also violate the laws and regulations of your country or region regarding software piracy and copyright infringement.
-
Is FIFA 2020 Crack worth using?
-
No, FIFA 2020 Crack is not worth using. It may seem like a good way to save money or have fun, but it comes with many risks and consequences that outweigh any benefits or advantages. You may end up losing more money, time, or enjoyment than you gain from using FIFA 2020 Crack.
-
What are some alternatives to FIFA 2020 Crack?
-
If you want to play FIFA 2020 without using FIFA 2020 Crack, you have some alternatives, such as:
-
-
Buying FIFA 2020 from an official store or platform, such as Origin, Steam, or PlayStation Store. This is the best and safest way to play FIFA 2020 legally and legitimately.
-
Waiting for FIFA 2020 to go on sale or discount from an official store or platform. This is a good way to save money and still play FIFA 2020 legally and legitimately.
-
Playing other soccer games that are free or cheaper than FIFA 2020. There are many other soccer games that you can play on your device, such as PES 2020, Dream League Soccer 2020, Soccer Stars, etc.
-
- 0a6ba089eb
-
-
\ No newline at end of file
diff --git a/spaces/raedeXanto/academic-chatgpt-beta/HD Online Player (Salaam Namaste movie free download i) Find Out How the Script of this Film was Invited to be Included in the Margaret Herrick Library.md b/spaces/raedeXanto/academic-chatgpt-beta/HD Online Player (Salaam Namaste movie free download i) Find Out How the Script of this Film was Invited to be Included in the Margaret Herrick Library.md
deleted file mode 100644
index 4b56289aef2f3ae180b542b580d38db8a0f03395..0000000000000000000000000000000000000000
--- a/spaces/raedeXanto/academic-chatgpt-beta/HD Online Player (Salaam Namaste movie free download i) Find Out How the Script of this Film was Invited to be Included in the Margaret Herrick Library.md
+++ /dev/null
@@ -1,129 +0,0 @@
-
-
X Force Keygen AutoCAD Electrical 2014 Portable: A Complete Guide
-
If you are looking for a way to activate AutoCAD Electrical 2014 without buying a license, you might have heard of X Force Keygen. This is a tool that can generate serial numbers and activation codes for various Autodesk products, including AutoCAD Electrical 2014. In this article, we will show you how to download, install, and use X Force Keygen AutoCAD Electrical 2014 Portable, a version that does not require installation and can run from any device.
-
What is X Force Keygen?
-
X Force Keygen is a software that can generate valid serial numbers and activation codes for Autodesk products. It is based on a reverse engineering technique that exploits the algorithm of the software protection system. By using X Force Keygen, you can bypass the online activation process and use Autodesk products without paying for a license.
X Force Keygen works by creating a request code based on your product information and hardware ID. This request code is then sent to the keygen, which generates an activation code that matches your request code. The activation code is then entered into the software activation screen, where it is verified and accepted. This way, you can activate your Autodesk product offline.
-
Why use it for AutoCAD Electrical 2014?
-
AutoCAD Electrical 2014 is a software that helps you design and document electrical systems. It has many features and functions that can help you create schematic diagrams, panel layouts, PLC I/O drawings, and more. However, AutoCAD Electrical 2014 is not cheap. It costs around $5,000 for a single-user license. If you cannot afford this price, you might want to use X Force Keygen to activate it for free.
-
How to download and install X Force Keygen AutoCAD Electrical 2014 Portable?
-
To use X Force Keygen AutoCAD Electrical 2014 Portable, you need to download and install two things: the keygen itself and the portable version of AutoCAD Electrical 2014. Here are the steps to follow:
-
Step 1: Disable your internet connection and antivirus
-
Before you download and run X Force Keygen, you need to disable your internet connection and antivirus software. This is because X Force Keygen is detected as a malicious program by most antivirus programs and online security services. If you do not disable them, they might block or delete X Force Keygen from your device.
-
Step 2: Download X Force Keygen from a reliable source
-
There are many websites that claim to offer X Force Keygen for free, but not all of them are trustworthy. Some of them might contain viruses, malware, or fake files that can harm your device or steal your personal information. To avoid this risk, you should download X Force Keygen from a reliable source, such as . This website provides a safe and working link to download X Force Keygen for Autodesk products (2014) all.
-
x force crack AutoCAD Electrical 2014 download
-x force activator AutoCAD Electrical 2014 free
-x force patch AutoCAD Electrical 2014 online
-x force serial AutoCAD Electrical 2014 full
-x force license AutoCAD Electrical 2014 version
-x force generator AutoCAD Electrical 2014 software
-x force code AutoCAD Electrical 2014 product
-x force key AutoCAD Electrical 2014 activation
-x force tool AutoCAD Electrical 2014 install
-x force program AutoCAD Electrical 2014 setup
-x force application AutoCAD Electrical 2014 windows
-x force file AutoCAD Electrical 2014 mac
-x force folder AutoCAD Electrical 2014 linux
-x force zip AutoCAD Electrical 2014 rar
-x force exe AutoCAD Electrical 2014 iso
-x force dmg AutoCAD Electrical 2014 torrent
-x force rar AutoCAD Electrical 2014 mega
-x force iso AutoCAD Electrical 2014 google drive
-x force torrent AutoCAD Electrical 2014 mediafire
-x force mega AutoCAD Electrical 2014 zippyshare
-x force google drive AutoCAD Electrical 2014 dropbox
-x force mediafire AutoCAD Electrical 2014 rapidshare
-x force zippyshare AutoCAD Electrical 2014 sendspace
-x force dropbox AutoCAD Electrical 2014 filefactory
-x force rapidshare AutoCAD Electrical 2014 uploaded
-x force sendspace AutoCAD Electrical 2014 uptobox
-x force filefactory AutoCAD Electrical 2014 openload
-x force uploaded AutoCAD Electrical 2014 shareonline
-x force uptobox AutoCAD Electrical 2014 turbobit
-x force openload AutoCAD Electrical 2014 nitroflare
-x force shareonline AutoCAD Electrical 2014 hitfile
-x force turbobit AutoCAD Electrical 2014 depositfiles
-x force nitroflare AutoCAD Electrical 2014 uploadgig
-x force hitfile AutoCAD Electrical 2014 alfafile
-x force depositfiles AutoCAD Electrical 2014 katfile
-x force uploadgig AutoCAD Electrical 2014 dlfree
-x force alfafile AutoCAD Electrical 2014 easybytez
-x force katfile AutoCAD Electrical 2014 filerio
-x force dlfree AutoCAD Electrical 2014 filescdn
-x force easybytez AutoCAD Electrical 2014 filespace
-x force filerio AutoCAD Electrical 2014 gigapeta
-x force filescdn AutoCAD Electrical 2014 indishare
-x force filespace AutoCAD Electrical 2014 mexashare
-x force gigapeta AutoCAD Electrical 2014 oboom
-x force indishare AutoCAD Electrical 2014 prefiles
-x force mexashare AutoCAD Electrical 2014 rockfile
-x force oboom AutoCAD Electrical 2014 suprafiles
-x force prefiles AutoCAD Electrical 2014 uploadboy
-x force rockfile AutoCAD Electrical 2014 userscloud
-x force suprafiles AutoCAD Electrical 2014 wupfile
-
Step 3: Extract the zip file and run the keygen as administrator
-
After you download X Force Keygen from , you will get a zip file named Universal.xforce.keygen.Autodesk.2014.zip. You need to extract this file using a program like WinRAR or 7-Zip. You will get a folder named Universal.xforce.keygen.Autodesk.2014 with two files inside: x-force_2014_x32.exe and x-force_2014_x64.exe. Depending on your system architecture (32-bit or 64-bit), you need to run one of these files as administrator by right-clicking on it and choosing Run as administrator.
-
Step 4: Select AutoCAD Electrical 2014 from the product list and click on Patch
-
When you run X Force Keygen as administrator, you will see a window with a list of Autodesk products. You need to select AutoCAD Electrical 2014 from this list and click on Patch. You should see a message saying Successfully patched.
-
Step 5: Copy the request code and paste it into the keygen and click on Generate
-
Now you need to open AutoCAD Electrical 2014 Portable from its location (see step 6) and go to the activation screen by clicking on Activate in the Help menu. You will see a request code in this screen. You need to copy this code and paste it into the keygen window where it says Request. Then click on Generate. You will see an activation code in the keygen window.
-
Step 6: Copy the activation code and paste it into the activation screen of AutoCAD Electrical 2014
-
Now you need to copy the activation code from the keygen window and paste it into the activation screen of AutoCAD Electrical 2014 where it says Enter an activation code here. Then click on Next. You should see a message saying Thank you for activating your Autodesk product.
-
How to use AutoCAD Electrical 2014 Portable with X Force Keygen?
-
Now that you have activated AutoCAD Electrical 2014 with X Force Keygen, you can use it as a portable version that does not require installation and can run from any device.
-
What are the benefits of using a portable version?
-
A portable version of AutoCAD Electrical 2014 has several benefits over an installed version:
-
-
You do not need to install it on your device, which saves space and time.
-
You can run it from any device that supports Windows operating system, such as a USB drive or a cloud storage.
-
You do not need to worry about license expiration or renewal.
-
You can use it offline without internet connection.
-
-
How to run AutoCAD Electrical 2014 Portable from a USB drive or a cloud storage?
-
To run AutoCAD Electrical 2014 Portable from a USB drive or a cloud storage, you need to download and extract it first. You can find a link to download AutoCAD Electrical 2014 Portable from . This link will take you to Google Drive where you can download xforce keygen 64bits autocad
-xforce keygen autocad electrical portable
-.zip file. After downloading this file, you need to extract it using WinRAR or 7-Zip. You will get a folder named xforce keygen autocad electrical portable with two files inside: acad.exe (the main executable file) and acad.ico (the icon file). To run AutoCAD Electrical 2014 Portable from a USB drive or a cloud storage, you need to copy this folder to your desired location (such as your USB drive or your cloud storage folder). Then double-click on acad.exe file to launch AutoCAD Electrical
-xforce keygen autocad electrical portable
-.
What are the features and functions of AutoCAD Electrical
-xforce keygen autocad electrical portable
-?
-
AutoCAD Electrical
-xforce keygen autocad electrical portable
-is a software that helps you design and document electrical systems. It has many features and functions that can help you create schematic diagrams, panel layouts, PLC I/O drawings, and more. Some of the features and functions of AutoCAD Electrical 2014 are:
-
-
Electrical symbol library: You can access a library of 65,000+ intelligent electrical symbols that are easy to use, colorful, and customizable. You can also create your own symbols using the Symbol Builder tool.
-
Wire and component tagging: You can automate the numbering of wires and the generation of component tags with various options and formats. You can also re-tag components easily and update your drawings accordingly.
-
Automatic reports: You can generate and update multiple customized reports automatically, such as bill of materials, wire lists, terminal plans, cable summaries, and more. You can also export your reports to various formats, such as Excel, PDF, or HTML.
-
Electrical standards support: You can work with the latest electrical standards, such as NFPA, IEC, ANSI, JIC, GB, AS/NZS, and more. You can also use the JIC and older IEC symbol libraries for legacy support.
-
Project management: You can use the Project Manager to organize and manage your electrical projects. You can re-order drawings, copy or move project files, create subfolders, and more. You can also access simple export options to share your projects with others.
-
Circuit design and reuse: You can use the Circuit Builder to design and annotate a variety of motor control and power feed circuits. You can access prepopulated data and customize it according to your needs. You can also reuse existing circuits or create your own circuit templates.
-
SQL catalog support: You can use a SQL catalog database to store and retrieve your electrical component data. You can also integrate your catalog data with Autodesk Vault to generate a bill of materials automatically from your AutoCAD Electrical drawings.
-
Coil and contact cross-referencing: You can keep track of parent/child contacts in real time while Circuit Builder dynamically builds the circuit. You can also assign component tags to each component automatically.
-
PLC I/O drawings from spreadsheets: You can use a single data file to generate multiple PLC I/O drawings automatically. You can define your project’s I/O assignments in a spreadsheet, database, or comma-delimited text file and import it into AutoCAD Electrical 2014.
-
-
Conclusion
-
X Force Keygen AutoCAD Electrical 2014 Portable is a tool that can help you activate AutoCAD Electrical 2014 without buying a license. It is based on a reverse engineering technique that exploits the algorithm of the software protection system. By using X Force Keygen AutoCAD Electrical 2014 Portable, you can also use AutoCAD Electrical 2014 as a portable version that does not require installation and can run from any device.
-
AutoCAD Electrical 2014 is a software that helps you design and document electrical systems. It has many features and functions that can help you create schematic diagrams, panel layouts, PLC I/O drawings, and more. It also supports the latest electrical standards and provides a library of 65,000+ intelligent electrical symbols.
-
If you want to learn more about X Force Keygen AutoCAD Electrical 2014 Portable or AutoCAD Electrical 2014 features and functions, you can check out the following resources:
What is the difference between X Force Keygen AutoCAD Electrical 2014 Portable and X Force Keygen AutoCAD Electrical 2014?
-
X Force Keygen AutoCAD Electrical 2014 Portable is a version that does not require installation and can run from any device. X Force Keygen AutoCAD Electrical 2014 is a version that requires installation on your device.
-
Is X Force Keygen AutoCAD Electrical 2014 Portable safe to use?
-
X Force Keygen AutoCAD Electrical 2014 Portable is detected as a malicious program by most antivirus programs and online security services. Therefore, you should use it at your own risk and disable your internet connection and antivirus software before using it.
-
How long does X Force Keygen AutoCAD Electrical 2014 Portable last?
-
X Force Keygen AutoCAD Electrical 2014 Portable lasts indefinitely as long as you do not update or reinstall your Autodesk product.
-
Can I use X Force Keygen AutoCAD Electrical 2014 Portable for other Autodesk products?
-
X Force Keygen AutoCAD Electrical 2014 Portable works for all Autodesk products (2014) all. However, you need to select the correct product from the list in the keygen window before generating an activation code.
-
Can I use X Force Keygen AutoCAD Electrical 2014 Portable online?
-
No, you cannot use X Force Keygen AutoCAD Electrical 2014 Portable online. You need to activate your Autodesk product offline using X Force Keygen AutoCAD Electrical 2014 Portable.
-
-Text to speech packages create time: 2015 acapela infovox desktop enginevoicesus voicesryanryan22. Your voice on the radio: still pdrsian superb dave depper death ... I didn't know what to do. On the way to the university, I thought about what I would say when I saw her, but then things got easier somehow. I said: "Hi!" and she said: "Hi! You're early today," and I said: "I was going to go into town." And she said: "Since when do you go there?" And I said: "Well, I wanted to spend the evening with you. You look like you're doing something. 8a78ff9644
-
-
-
diff --git a/spaces/recenWmenso/ChatGPT-with-Voice-Cloning-for-All/datasets/CyberLink PowerDVD Ultra 20.0.1419.66 Serial Key.md b/spaces/recenWmenso/ChatGPT-with-Voice-Cloning-for-All/datasets/CyberLink PowerDVD Ultra 20.0.1419.66 Serial Key.md
deleted file mode 100644
index 7a0dfccdc18541b41922264ee49d177618e229f1..0000000000000000000000000000000000000000
--- a/spaces/recenWmenso/ChatGPT-with-Voice-Cloning-for-All/datasets/CyberLink PowerDVD Ultra 20.0.1419.66 Serial Key.md
+++ /dev/null
@@ -1,36 +0,0 @@
-
-
CyberLink PowerDVD Ultra 20.0.1419.66 Serial Key: How to Activate the Ultimate Media Player
-
CyberLink PowerDVD Ultra is a powerful and versatile media player that can handle all your entertainment needs. Whether you want to watch Blu-ray discs, DVDs, 4K videos, online streaming, VR content, or music, PowerDVD Ultra has you covered.
-
But to enjoy all the features and benefits of PowerDVD Ultra, you need to activate it with a valid serial key. A serial key is a unique code that verifies your purchase and unlocks the full version of the software.
So how do you get a CyberLink PowerDVD Ultra 20.0.1419.66 serial key? And how do you use it to activate your software? Here are some steps to follow:
-
-
First, you need to purchase PowerDVD Ultra from the official CyberLink website or an authorized reseller. You can choose between a one-time payment or a subscription plan.
-
After completing your purchase, you will receive an email confirmation with your order details and your serial key. The serial key is a 30-digit alphanumeric code that looks something like this: XXXXX-XXXXX-XXXXX-XXXXX-XXXXX-XXXXX.
-
Next, you need to download and install PowerDVD Ultra on your computer. You can download the installer from the CyberLink website or use the disc that came with your purchase.
-
Once you have installed PowerDVD Ultra, launch it and click on the "Activate" button on the top right corner of the main window. You will be prompted to enter your serial key.
-
Copy and paste your serial key from the email confirmation or type it manually. Make sure you enter it exactly as it appears, without any spaces or dashes.
-
Click on the "OK" button to verify your serial key and activate your software. You will see a message confirming that your activation was successful.
-
Enjoy using PowerDVD Ultra with all its features and benefits!
-
-
If you have any problems with activating your software or finding your serial key, you can contact CyberLink customer support for assistance.
-
-
Why Choose PowerDVD Ultra?
-
PowerDVD Ultra is more than just a media player. It is a complete multimedia solution that offers you a range of features and benefits to enhance your entertainment experience. Here are some of the reasons why you should choose PowerDVD Ultra:
-
-
It supports all kinds of media formats, including Blu-ray, DVD, 4K, HDR, HEVC, 360, VR, and more. You can play any disc or file on your computer or stream online content from popular platforms like YouTube, Netflix, Hulu, and Amazon Prime Video.
-
It delivers the best quality and performance for your media playback. You can enjoy smooth and stutter-free playback with TrueTheater technology that optimizes the video and audio quality according to your hardware and preferences. You can also adjust the brightness, contrast, color, and sound settings to suit your viewing environment.
-
It offers you a immersive and interactive experience with your media. You can explore the 360 and VR content with your mouse, keyboard, or headset. You can also use the PowerDVD Remote app to control the playback from your smartphone or tablet.
-
It lets you organize and manage your media library with ease. You can browse and search your media files by categories, tags, ratings, and keywords. You can also create playlists, bookmarks, and favorites for quick access.
-
It allows you to share and enjoy your media across devices and platforms. You can use the CyberLink Cloud service to store and sync your media files online. You can also use the PowerDVD Mobile app to access and play your media on your iOS or Android devices.
-
-
How to Get the Most Out of PowerDVD Ultra?
-
PowerDVD Ultra is a powerful and versatile software that can enhance your entertainment experience in many ways. But how can you get the most out of it? Here are some tips and tricks to help you:
-
-
Check for updates regularly. CyberLink releases new updates for PowerDVD Ultra that fix bugs, improve performance, and add new features. You can check for updates manually by clicking on the "Update" button on the top right corner of the main window or enable automatic updates in the settings.
-
Explore the settings and preferences. PowerDVD Ultra has a lot of options and settings that you can customize according to your needs and preferences. You can access them by clicking on the "Settings" button on the top right corner of the main window or pressing Ctrl+P on your keyboard. You can change the language, subtitles, audio output, playback mode, network connection, cloud storage, parental control, and more.
-
Use keyboard shortcuts and mouse gestures. PowerDVD Ultra has a lot of keyboard shortcuts and mouse gestures that you can use to control the playback and navigate the interface. You can view them by clicking on the "Help" button on the top right corner of the main window or pressing F1 on your keyboard. You can also customize them in the settings.
-
Join the CyberLink community. CyberLink has a large and active community of users who share their tips, feedback, questions, and suggestions about PowerDVD Ultra and other CyberLink products. You can join them by clicking on the "Community" button on the top right corner of the main window or visiting https://forum.cyberlink.com/. You can also follow CyberLink on social media platforms like Facebook, Twitter, Instagram, YouTube, and LinkedIn.
-
d5da3c52bf
-
-
\ No newline at end of file
diff --git a/spaces/rockeycoss/Prompt-Segment-Anything-Demo/mmdet/models/detectors/yolof.py b/spaces/rockeycoss/Prompt-Segment-Anything-Demo/mmdet/models/detectors/yolof.py
deleted file mode 100644
index 2bc4f1abd21eb9ad439e5810dc8dce2c4d0d6329..0000000000000000000000000000000000000000
--- a/spaces/rockeycoss/Prompt-Segment-Anything-Demo/mmdet/models/detectors/yolof.py
+++ /dev/null
@@ -1,20 +0,0 @@
-# Copyright (c) OpenMMLab. All rights reserved.
-from ..builder import DETECTORS
-from .single_stage import SingleStageDetector
-
-
-@DETECTORS.register_module()
-class YOLOF(SingleStageDetector):
- r"""Implementation of `You Only Look One-level Feature
- `_"""
-
- def __init__(self,
- backbone,
- neck,
- bbox_head,
- train_cfg=None,
- test_cfg=None,
- pretrained=None,
- init_cfg=None):
- super(YOLOF, self).__init__(backbone, neck, bbox_head, train_cfg,
- test_cfg, pretrained, init_cfg)
diff --git a/spaces/rorallitri/biomedical-language-models/logs/Barrons Sat Subject Test Physics Pdf Download.md b/spaces/rorallitri/biomedical-language-models/logs/Barrons Sat Subject Test Physics Pdf Download.md
deleted file mode 100644
index 5dc24fad59fe970a45eb9f174e427f700ff805a3..0000000000000000000000000000000000000000
--- a/spaces/rorallitri/biomedical-language-models/logs/Barrons Sat Subject Test Physics Pdf Download.md
+++ /dev/null
@@ -1,10 +0,0 @@
-
-
-pdf is the Pearson Exam Guide for the new test. (more...)
-
-Written by a panel of prominent scientists and engineers, this book provides a well-illustrated, practical reference on the application of advanced applied technology in the life sciences and is specifically designed to meet the needs of recent applicants to doctoral-level programs. (more...)
-
-Written 4fefd39f24
-
-
-
diff --git a/spaces/rorallitri/biomedical-language-models/logs/Lfs 0.6 S2 Keygen Indir How to Download and Install Live for Speed with All the Extras.md b/spaces/rorallitri/biomedical-language-models/logs/Lfs 0.6 S2 Keygen Indir How to Download and Install Live for Speed with All the Extras.md
deleted file mode 100644
index 9c7ff05e9737d389f5c2e0164fd417610f2f5587..0000000000000000000000000000000000000000
--- a/spaces/rorallitri/biomedical-language-models/logs/Lfs 0.6 S2 Keygen Indir How to Download and Install Live for Speed with All the Extras.md
+++ /dev/null
@@ -1,6 +0,0 @@
-
-
-Able2Extract Professional 12.0.4.0 (x86+x64) Final + Crack, done, Software, 9 months ago, 130 MB, 12, 1. Able2Extract Professional 10.0.5 + Patch [KaranPC] ... Able2Extract Professional 10.0.10 (x86+x64) + Crack, done, Software, 9 months ago, 130 MB, 12, 1
-Crack Able2Extract 7.7 Pro.
-Able2Extract Professional is a program for working with PDF documents.
-Able2Extract Professional is a PDF document manipulation program.
-Able2Extract is a program for working with PDF documents.
-Able2Extract Professional is a feature-rich, flexible and .
-Able2Extract Professional is a program 8a78ff9644
-
-
-
diff --git a/spaces/scedlatioru/img-to-music/example/Crack EXCLUSIVE Overloud - Gem Comp670 1.1.0 (STANDALONE VST VST3 AAX AU).md b/spaces/scedlatioru/img-to-music/example/Crack EXCLUSIVE Overloud - Gem Comp670 1.1.0 (STANDALONE VST VST3 AAX AU).md
deleted file mode 100644
index 504e1878387ea8124d0eb80101e9ec79507f09b6..0000000000000000000000000000000000000000
--- a/spaces/scedlatioru/img-to-music/example/Crack EXCLUSIVE Overloud - Gem Comp670 1.1.0 (STANDALONE VST VST3 AAX AU).md
+++ /dev/null
@@ -1,6 +0,0 @@
-
-
-'''.format(
- image_path=image_path,
- tweet_path=tweet_path,
- public_host=public_host)
diff --git a/spaces/simonduerr/ProteinMPNNESM/ProteinMPNN/vanilla_proteinmpnn/helper_scripts/other_tools/make_omit_AA.py b/spaces/simonduerr/ProteinMPNNESM/ProteinMPNN/vanilla_proteinmpnn/helper_scripts/other_tools/make_omit_AA.py
deleted file mode 100644
index 353a74bcf0ec1c0238a32b17a001d15591d18243..0000000000000000000000000000000000000000
--- a/spaces/simonduerr/ProteinMPNNESM/ProteinMPNN/vanilla_proteinmpnn/helper_scripts/other_tools/make_omit_AA.py
+++ /dev/null
@@ -1,39 +0,0 @@
-import glob
-import random
-import numpy as np
-import json
-import itertools
-
-#MODIFY this path
-with open('/home/justas/projects/lab_github/mpnn/data/pdbs.jsonl', 'r') as json_file:
- json_list = list(json_file)
-
-my_dict = {}
-for json_str in json_list:
- result = json.loads(json_str)
- all_chain_list = [item[-1:] for item in list(result) if item[:9]=='seq_chain']
- fixed_position_dict = {}
- print(result['name'])
- if result['name'] == '5TTA':
- for chain in all_chain_list:
- if chain == 'A':
- fixed_position_dict[chain] = [
- [[int(item) for item in list(itertools.chain(list(np.arange(1,4)), list(np.arange(7,10)), [22, 25, 33]))], 'GPL'],
- [[int(item) for item in list(itertools.chain([40, 41, 42, 43]))], 'WC'],
- [[int(item) for item in list(itertools.chain(list(np.arange(50,150))))], 'ACEFGHIKLMNRSTVWYX'],
- [[int(item) for item in list(itertools.chain(list(np.arange(160,200))))], 'FGHIKLPQDMNRSTVWYX']]
- else:
- fixed_position_dict[chain] = []
- else:
- for chain in all_chain_list:
- fixed_position_dict[chain] = []
- my_dict[result['name']] = fixed_position_dict
-
-#MODIFY this path
-with open('/home/justas/projects/lab_github/mpnn/data/omit_AA.jsonl', 'w') as f:
- f.write(json.dumps(my_dict) + '\n')
-
-
-print('Finished')
-#e.g. output
-#{"5TTA": {"A": [[[1, 2, 3, 7, 8, 9, 22, 25, 33], "GPL"], [[40, 41, 42, 43], "WC"], [[50, 51, 52, 53, 54, 55, 56, 57, 58, 59, 60, 61, 62, 63, 64, 65, 66, 67, 68, 69, 70, 71, 72, 73, 74, 75, 76, 77, 78, 79, 80, 81, 82, 83, 84, 85, 86, 87, 88, 89, 90, 91, 92, 93, 94, 95, 96, 97, 98, 99, 100, 101, 102, 103, 104, 105, 106, 107, 108, 109, 110, 111, 112, 113, 114, 115, 116, 117, 118, 119, 120, 121, 122, 123, 124, 125, 126, 127, 128, 129, 130, 131, 132, 133, 134, 135, 136, 137, 138, 139, 140, 141, 142, 143, 144, 145, 146, 147, 148, 149], "ACEFGHIKLMNRSTVWYX"], [[160, 161, 162, 163, 164, 165, 166, 167, 168, 169, 170, 171, 172, 173, 174, 175, 176, 177, 178, 179, 180, 181, 182, 183, 184, 185, 186, 187, 188, 189, 190, 191, 192, 193, 194, 195, 196, 197, 198, 199], "FGHIKLPQDMNRSTVWYX"]], "B": []}, "3LIS": {"A": [], "B": []}}
diff --git a/spaces/simple0urra/skops-model-card-creator-2a23515a-d54e-4804-b365-27ed6e938735/example/Dancing Road APK Mod A Fun and Challenging Music Game for Android.md b/spaces/simple0urra/skops-model-card-creator-2a23515a-d54e-4804-b365-27ed6e938735/example/Dancing Road APK Mod A Fun and Challenging Music Game for Android.md
deleted file mode 100644
index 4802ecbf16d83c32dfef654707279e12f6776223..0000000000000000000000000000000000000000
--- a/spaces/simple0urra/skops-model-card-creator-2a23515a-d54e-4804-b365-27ed6e938735/example/Dancing Road APK Mod A Fun and Challenging Music Game for Android.md
+++ /dev/null
@@ -1,131 +0,0 @@
-
-
Dancing Road APK Mod: A Fun and Challenging Music Game
-
Do you love music and rhythm games? Do you want to enjoy a colorful and exciting game that tests your reflexes and coordination? If yes, then you should try Dancing Road APK Mod, a popular music game that lets you control a ball that rolls on a road that changes color according to the song you choose.
Dancing Road APK Mod is a modified version of the original game that gives you unlimited lives, coins, gems, stars, and other resources. You can use them to unlock more songs, balls, roads, themes, effects, and other features that make your game experience more fun and personalized.
-
In this article, we will show you how to download and install Dancing Road APK Mod on your Android device. We will also give you some tips on how to play the game, how to customize your game experience, how to unlock more content, how to connect with other players, how to troubleshoot common issues, and more.
-
So if you are ready to join the millions of players who are enjoying this addictive music game, read on!
-
How to Download and Install Dancing Road APK Mod
-
Downloading and installing Dancing Road APK Mod is very easy. Just follow these simple steps:
-
-
Go to [this link](^1^) and click on the Download APK button.
-
Wait for the download to finish and then open the file.
-
If you see a warning message that says "Install blocked", go to your device settings and enable "Unknown sources" under security or privacy options.
-
Tap on "Install" and wait for the installation to complete.
-
Launch the game and enjoy!
-
-
Note: You may need to uninstall the original version of Dancing Road before installing the modded version.
-
[Dancing Road: Color Ball Run! MOD APK 1.8.0 (Unlimited Money) Download]
-[Dancing Road: Color Ball Run! Mod Apk 1.8.0 (Unlimited Lives) - ApkMod]
-[Dancing Road: Color Ball Run! Mod APK 1.8.0 - Download Dancing Road: Color Ball Run! Mod for Android]
-[Dancing Road: Color Ball Run! Mod Apk 1.8.0 (Unlimited Money) - Android Mods Apk]
-[Dancing Road: Color Ball Run! Mod APK 1.8.0 - HappyMod]
-
How to Play Dancing Road APK Mod
-
Dancing Road APK Mod is very easy to play but hard to master. Here are some basic gameplay instructions, tips and tricks, game modes, levels, challenges, rewards:
-
-
The goal of the game is to control a ball that rolls on a road that changes color according to the song you choose. You have to swipe left or right to match the ball with the same color as the road.
-
If you miss a color or hit a different color, you will lose a life. You have three lives in each level. If you lose all your lives, you will have to start over.
-
You can earn coins, gems, stars, and other rewards by completing levels, challenges, and events. You can use them to unlock more songs, balls, roads, themes, effects, and other features.
-
You can choose from different game modes such as Classic, Endless, Hard, and VIP. Each mode has different levels of difficulty and rewards.
-
You can also choose from different genres of music such as Pop, Rock, EDM, Hip Hop, and more. Each genre has different songs and roads.
-
You can also customize your game experience by choosing different balls, roads, themes, and effects. Each ball has different attributes such as speed, size, shape, and trail. Each road has different patterns and colors. Each theme has different backgrounds and sounds. Each effect has different animations and visuals.
-
-
Dancing Road APK Mod is a fun and challenging music game that will keep you entertained for hours. You will enjoy the variety of songs, balls, roads, themes, effects, and other features that you can unlock and customize. You will also improve your reflexes and coordination as you try to match the ball with the road.
-
How to Customize Your Game Experience with Dancing Road APK Mod
-
One of the best things about Dancing Road APK Mod is that you can customize your game experience according to your preferences and mood. Here are some ways you can do that:
-
-
To choose a different song, tap on the music icon on the top left corner of the screen. You will see a list of genres and songs that you can choose from. Tap on the song that you want to play.
-
To choose a different ball, tap on the ball icon on the top right corner of the screen. You will see a list of balls that you can choose from. Tap on the ball that you want to use.
-
To choose a different road, tap on the road icon on the bottom left corner of the screen. You will see a list of roads that you can choose from. Tap on the road that you want to roll on.
-
To choose a different theme, tap on the theme icon on the bottom right corner of the screen. You will see a list of themes that you can choose from. Tap on the theme that you want to apply.
-
To choose a different effect, tap on the effect icon on the bottom center of the screen. You will see a list of effects that you can choose from. Tap on the effect that you want to activate.
-
-
Note: Some songs, balls, roads, themes, and effects are locked and require coins, gems, stars, or other resources to unlock them. You can earn them by playing the game or by using Dancing Road APK Mod.
-
How to Unlock More Content with Dancing Road APK Mod
-
Dancing Road APK Mod gives you unlimited access to all the content in the game. However, if you want to unlock more content without using the modded version, here are some ways you can do that:
-
-
Complete levels: Each level has three stars that you can earn by matching the ball with the road perfectly. The more stars you earn, the more coins and gems you get. You can use them to unlock more songs, balls, roads, themes, and effects.
-
Complete challenges: Each day, you can complete different challenges that give you coins, gems, stars, and other rewards. Some examples of challenges are: play 10 songs, collect 100 coins, use 5 different balls, etc.
-
Join events: Each week, you can join different events that give you coins, gems, stars, and other rewards. Some examples of events are: Halloween event, Christmas event, Valentine's event, etc.
-
Watch ads: You can watch ads to get free coins, gems, stars, and other rewards. You can also watch ads to revive your lives or to get extra rewards after completing a level.
-
Invite friends: You can invite your friends to play Dancing Road APK Mod and get coins, gems, stars, and other rewards. You can also join or create a team with your friends and chat with them in the game.
-
-
Dancing Road APK Mod has a lot of content that you can unlock and enjoy. You can choose from hundreds of songs, balls, roads, themes, effects, and other features that make your game experience more fun and personalized.
-
How to Connect with Other Players with Dancing Road APK Mod
-
Dancing Road APK Mod is not only a solo game. You can also connect with other players from around the world and compete with them in leaderboards, tournaments, events, and more. Here are some ways you can do that:
-
-
To join or create a team, tap on the team icon on the top center of the screen. You will see a list of teams that you can join or create. Tap on the team that you want to join or create.
-
To chat with other players, tap on the chat icon on the bottom left corner of the screen. You will see a list of chat rooms that you can join or create. Tap on the chat room that you want to join or create.
-
To compete in leaderboards, tap on the leaderboard icon on the top right corner of the screen. You will see a list of leaderboards that you can join or create. Tap on the leaderboard that you want to join or create.
-
To compete in tournaments, tap on the tournament icon on the bottom right corner of the screen. You will see a list of tournaments that you can join or create. Tap on the tournament that you want to join or create.
-
To compete in events, tap on the event icon on the bottom center of the screen. You will see a list of events that you can join or create. Tap on the event that you want to join or create.
-
-
Note: Some teams, chat rooms, leaderboards, tournaments, and events are locked and require coins, gems, stars, or other resources to join or create them. You can earn them by playing the game or by using Dancing Road APK Mod.
-
How to Troubleshoot Common Issues with Dancing Road APK Mod
-
Dancing Road APK Mod is a stable and smooth game that runs well on most Android devices. However, if you encounter any issues such as crashing, freezing, lagging, loading errors, etc., here are some ways you can troubleshoot them:
-
-
Check your internet connection: Make sure you have a stable and fast internet connection to play the game smoothly. You can use a Wi-Fi or a mobile data connection, but avoid using a VPN or a proxy server.
-
Clear your cache and data: Sometimes, the cache and data of the game can get corrupted or outdated, causing issues. You can clear them by going to your device settings, apps, Dancing Road APK Mod, storage, and tapping on "Clear cache" and "Clear data". Note that this will reset your game progress and settings, so make sure you back them up first.
-
Update your game and device: Sometimes, the game or your device can have bugs or glitches that cause issues. You can update them by going to the Google Play Store, Dancing Road APK Mod, and tapping on "Update". You can also go to your device settings, system, and tapping on "Update". Note that this may require a restart of your device.
-
Reinstall your game: Sometimes, the game can have corrupted or missing files that cause issues. You can reinstall it by going to the Google Play Store, Dancing Road APK Mod, and tapping on "Uninstall". Then, you can download and install it again from [this link].
-
Contact the support team: If none of the above methods work, you can contact the support team of Dancing Road APK Mod by going to the game settings, help, and tapping on "Contact us". You can also email them at support@dancingroad.com. They will try to help you as soon as possible.
-
-
Dancing Road APK Mod is a reliable and enjoyable game that rarely has any issues. However, if you do encounter any problems, you can try these troubleshooting methods or contact the support team for assistance.
-
Pros and Cons of Dancing Road APK Mod
-
Dancing Road APK Mod is a great music game that has many pros and cons. Here is a table comparing them:
-
-
-
Pros
-
Cons
-
-
-
- Unlimited lives, coins, gems, stars, and other resources
-
- May not be compatible with some devices or Android versions
-
-
-
- Access to all songs, balls, roads, themes, effects, and other features
-
- May cause some issues such as crashing, freezing, lagging, loading errors, etc.
-
-
-
- Fun and challenging gameplay that tests your reflexes and coordination
-
- May be considered cheating by some players or developers
-
-
-
- Variety of genres, modes, levels, challenges, rewards, and customization options
-
- May take up more storage space than the original version
-
-
-
- Ability to connect with other players from around the world and compete with them
-
- May require a stable and fast internet connection to play smoothly
-
-
-
Dancing Road APK Mod is a music game that has many pros and cons. You can decide whether to use it or not based on your preferences and needs.
-
Alternatives to Dancing Road APK Mod
-
If you like Dancing Road APK Mod but want to try something different,
here are some similar games that you can try if you like Dancing Road APK Mod:
-
-
Beat Jumper: EDM up: This is another music game by the same developer of Dancing Road APK Mod. In this game, you have to control a ball that jumps on tiles that match the rhythm of the song. You can choose from different genres and songs, and customize your ball and tiles. You can also compete with other players in leaderboards and tournaments.
-
Tiles Hop: EDM Rush: This is a similar game to Beat Jumper, but with a different twist. In this game, you have to control a ball that hops on tiles that move horizontally. You have to swipe left or right to match the ball with the tiles. You can also choose from different genres and songs, and customize your ball and tiles.
-
Project Diva: This is a series of rhythm games that feature popular songs from Vocaloid, a software that synthesizes singing voices. In these games, you have to press buttons that correspond to the notes on the screen. You can choose from different Vocaloid characters and songs, and customize their outfits and stages.
-
Dance Dance Revolution: This is a classic rhythm game that requires a dance pad or a controller. In this game, you have to step on arrows that match the rhythm of the song. You can choose from different genres and songs, and adjust the difficulty and speed. You can also play with friends or online players.
-
Dancing Games at Y8.com: This is a website that offers a variety of online games related to dancing. You can learn to do different dances, be a cheerleader, practice your rhythm, and more. You can also enjoy the soundtracks and graphics of these games.
-
-
Dancing Road APK Mod is a fun and challenging music game that has many alternatives. You can try these games if you want to experience different genres, modes, features, and challenges.
-
FAQs about Dancing Road APK Mod
-
Here are some frequently asked questions and answers about Dancing Road APK Mod:
-
-
Is Dancing Road APK Mod safe to use?
-
Yes, Dancing Road APK Mod is safe to use as long as you download it from a trusted source like [this link]. However, you should be careful not to use it in a way that violates the terms of service or the privacy policy of the original game or the developer.
-
Is Dancing Road APK Mod legal to use?
-
It depends on your location and the laws of your country or region. Some countries or regions may prohibit or restrict the use of modded apps or games. You should check the laws of your country or region before using Dancing Road APK Mod.
-
Can I play Dancing Road APK Mod offline?
-
No, you need an internet connection to play Dancing Road APK Mod. This is because the game needs to load the songs, balls, roads, themes, effects, and other features from the server. You also need an internet connection to connect with other players and compete with them.
-
Can I play Dancing Road APK Mod on PC?
-
No, Dancing Road APK Mod is only available for Android devices. However, you can use an Android emulator on your PC to run the game. An Android emulator is a software that simulates an Android device on your PC. Some examples of Android emulators are BlueStacks, NoxPlayer, MEmu, etc.
-
Can I play Dancing Road APK Mod with friends?
-
Yes, you can play Dancing Road APK Mod with friends by joining or creating a team, chat room, leaderboard, tournament, or event. You can also invite your friends to play the game and get rewards.
-
-
Conclusion
-
Dancing Road APK Mod is a fun and challenging music game that lets you control a ball that rolls on a road that changes color according to the song you choose. You can enjoy a variety of songs, balls, roads, themes, effects, and other features that you can unlock and customize with unlimited lives, coins, gems, stars, and other resources. You can also connect with other players from around the world and compete with them in leaderboards, tournaments, events, and more.
-
If you love music and rhythm games, you should download and install Dancing Road APK Mod on your Android device today. You will have a blast playing this addictive music game!
197e85843d
-
-
\ No newline at end of file
diff --git a/spaces/skf15963/summary/fengshen/examples/clue1.1/predict2submit/csl_submit.py b/spaces/skf15963/summary/fengshen/examples/clue1.1/predict2submit/csl_submit.py
deleted file mode 100644
index 0664d67675a797959ba0fb6883b81bd172de8b06..0000000000000000000000000000000000000000
--- a/spaces/skf15963/summary/fengshen/examples/clue1.1/predict2submit/csl_submit.py
+++ /dev/null
@@ -1,83 +0,0 @@
-import json
-from tqdm import tqdm
-import argparse
-import numpy as np
-
-def save_data(data,file_path):
- with open(file_path, 'w', encoding='utf8') as f:
- for line in data:
- json_data=json.dumps(line,ensure_ascii=False)
- f.write(json_data+'\n')
-
-
-def load_data(file_path,is_training=False):
- with open(file_path, 'r', encoding='utf8') as f:
- lines = f.readlines()
- result=[]
- for l,line in tqdm(enumerate(lines)):
- data = json.loads(line)
- result.append(data)
- return result
-
-
-def recls(line):
- mat=[]
- for l in line:
- s=[v for v in l['score'].values()]
- mat.append(s)
- mat=np.array(mat)
- batch,num_labels=mat.shape
- for i in range(len(line)):
- index = np.unravel_index(np.argmax(mat, axis=None), mat.shape)
- line[index[0]]['label'] = int(index[1])
- mat[index[0],:] = np.zeros((num_labels,))
- mat[:,index[1]] = np.zeros((batch,))
- return line
-
-
-import copy
-
-def csl_scorted(data):
- lines={}
- new_data=copy.deepcopy(data)
- for d in data:
- if d['texta'] not in lines.keys():
- lines[d['texta']]={}
- lines[d['texta']][d['id']]=d['score'][d['choice'][0]]
- result=[]
- id2preds={}
- for k,v in lines.items():
- v=sorted(v.items(), key=lambda x: x[1], reverse=True)
- # print(v)
- for i,(text_id, score) in enumerate(v):
- if i/dev/null 2>&1 &
-sleep 5
-open http://127.0.0.1:7860
-echo Finished opening ChuanhuChatGPT (http://127.0.0.1:7860/). If you kill ChuanhuChatbot, Use "pkill -f 'ChuanhuChatbot'" command in terminal.
\ No newline at end of file
diff --git a/spaces/soufiane3/ChatGPT4/README.md b/spaces/soufiane3/ChatGPT4/README.md
deleted file mode 100644
index 7938de14e5355209aaae713f289ca469181bbb17..0000000000000000000000000000000000000000
--- a/spaces/soufiane3/ChatGPT4/README.md
+++ /dev/null
@@ -1,14 +0,0 @@
----
-title: Chat-with-GPT4
-emoji: 🚀
-colorFrom: red
-colorTo: indigo
-sdk: gradio
-sdk_version: 3.21.0
-app_file: app.py
-pinned: false
-license: mit
-duplicated_from: ysharma/ChatGPT4
----
-
-Check out the configuration reference at https://huggingface.co/docs/hub/spaces-config-reference
diff --git a/spaces/sriramelango/Social_Classification_Public/fairseq/fairseq/data/replace_dataset.py b/spaces/sriramelango/Social_Classification_Public/fairseq/fairseq/data/replace_dataset.py
deleted file mode 100644
index 5aac2ba96bee0a8bb65f4c9e56fa0b17248ee1d9..0000000000000000000000000000000000000000
--- a/spaces/sriramelango/Social_Classification_Public/fairseq/fairseq/data/replace_dataset.py
+++ /dev/null
@@ -1,36 +0,0 @@
-# Copyright (c) Facebook, Inc. and its affiliates.
-#
-# This source code is licensed under the MIT license found in the
-# LICENSE file in the root directory of this source tree.
-
-from . import BaseWrapperDataset
-
-
-class ReplaceDataset(BaseWrapperDataset):
- """Replaces tokens found in the dataset by a specified replacement token
-
- Args:
- dataset (~torch.utils.data.Dataset): dataset to replace tokens in
- replace_map(Dictionary[int,int]): map of token to replace -> replacement token
- offsets (List[int]): do not replace tokens before (from left if pos, right if neg) this offset. should be
- as many as the number of objects returned by the underlying dataset __getitem__ method.
- """
-
- def __init__(self, dataset, replace_map, offsets):
- super().__init__(dataset)
- assert len(replace_map) > 0
- self.replace_map = replace_map
- self.offsets = offsets
-
- def __getitem__(self, index):
- item = self.dataset[index]
- is_tuple = isinstance(item, tuple)
- srcs = item if is_tuple else [item]
-
- for offset, src in zip(self.offsets, srcs):
- for k, v in self.replace_map.items():
- src_off = src[offset:] if offset >= 0 else src[:offset]
- src_off.masked_fill_(src_off == k, v)
-
- item = srcs if is_tuple else srcs[0]
- return item
diff --git a/spaces/stomexserde/gpt4-ui/Examples/Ajay Yadav Anaesthesia Pdf Download.md b/spaces/stomexserde/gpt4-ui/Examples/Ajay Yadav Anaesthesia Pdf Download.md
deleted file mode 100644
index f7d1971976e573fd4b3ee69629c5ce95846ebf7a..0000000000000000000000000000000000000000
--- a/spaces/stomexserde/gpt4-ui/Examples/Ajay Yadav Anaesthesia Pdf Download.md
+++ /dev/null
@@ -1,23 +0,0 @@
-
-Here is a possible title and article with html formatting for the keyword "Ajay Yadav Anaesthesia Pdf Download":
-
-
How to Download Short Textbook of Anesthesia by Ajay Yadav 6th Edition Pdf for Free
-
If you are looking for a concise and comprehensive guide to anaesthesia, you may want to check out Short Textbook of Anesthesia by Ajay Yadav 6th Edition. This book covers the latest advances in anaesthesia, from basic concepts of anatomy and physiology, to equipment, preoperative assessment, airway management, monitoring, general and regional anaesthesia, anaesthesia for coexisting diseases, subspecialty anaesthetic management, and intensive care management[^1^].
-
The book has 344 pages, coloured illustrations, and key points for each topic. It also follows the CPR guidelines based on American Heart Association (AHA) guidelines[^2^]. The book has received positive reviews from students and practitioners alike[^1^].
So how can you download this book for free? Well, there are some websites that offer free pdf downloads of this book, such as Paul Kavin and Scribd. However, these websites may not be legal or safe, and they may not have the complete or updated version of the book. Therefore, we do not recommend downloading the book from these sources.
-
The best way to get this book is to buy it from a reputable online store, such as Google Books or Sciarium. These websites offer secure payment options and fast delivery. You can also preview some pages of the book before buying it. The price of the book may vary depending on the website and your location.
-
In conclusion, Short Textbook of Anesthesia by Ajay Yadav 6th Edition is a valuable resource for anyone interested in anaesthesia. You can download it for free from some websites, but we advise you to buy it from a reliable online store. We hope this article has helped you find the best way to get this book.
Here are a few more paragraphs to add to the article:
-
-
About the Author
-
Dr Ajay Yadav is an Indian anaesthesiologist from Gurgaon, Haryana. He has more than 20 years of experience in the field of anaesthesia and critical care. He has authored several books and articles on anaesthesia, and has been a speaker and faculty member at various national and international conferences. He is currently working as a senior consultant and head of department of anaesthesia at Medanta - The Medicity Hospital.
-
-
Other Books by Ajay Yadav
-
Apart from Short Textbook of Anesthesia, Dr Ajay Yadav has also written other books on anaesthesia, such as:
-
-
Anesthesia Review for DNB Students: This book is designed to help students prepare for the DNB (Diplomate of National Board) examination in anaesthesia. It covers the syllabus of the exam, and provides multiple choice questions and answers with explanations.
-
MCQs in Regional Anaesthesia and Pain Therapy: This book is a collection of more than 1000 multiple choice questions on regional anaesthesia and pain therapy. It covers topics such as anatomy, physiology, pharmacology, techniques, complications, and special situations. It also provides answers with references and rationales.
-
Handbook of Anaesthesia for Postgraduates: This book is a concise and practical guide for postgraduate students of anaesthesia. It covers the basics of anaesthesia, as well as specific topics such as paediatric anaesthesia, obstetric anaesthesia, neuroanaesthesia, cardiac anaesthesia, and more. It also provides tips and tricks for clinical practice.
-
7196e7f11a
-
-
\ No newline at end of file
diff --git a/spaces/stomexserde/gpt4-ui/Examples/Builders Of The Adytum Private Lessons - [150 PDFs].md b/spaces/stomexserde/gpt4-ui/Examples/Builders Of The Adytum Private Lessons - [150 PDFs].md
deleted file mode 100644
index 9c9ff53c6041f060ddaaa011b4a130cb142196ab..0000000000000000000000000000000000000000
--- a/spaces/stomexserde/gpt4-ui/Examples/Builders Of The Adytum Private Lessons - [150 PDFs].md
+++ /dev/null
@@ -1,21 +0,0 @@
-
-
Builders of the Adytum: A Modern Mystery School Offering Private Lessons
-
Have you ever wondered about the secrets of the Western Mystery tradition? Do you want to learn more about Tarot, Qabalah, Gematria, Astrology, Alchemy, and other related disciplines? If so, you might be interested in joining Builders of the Adytum (B.O.T.A.), a non-profit organization and an authentic Mystery School founded by Dr. Paul Foster Case in 1922.
-
Builders of the Adytum private lessons - [150 PDFs]
B.O.T.A. is derived from a Greek word that means Inner Shrine or Holy of Holies. It is a spiritual order that aims to build the Inner Temple within each member and to manifest the truth that love is the only real power in the universe. B.O.T.A. welcomes people of all faiths who are sincerely interested in its teachings, which are based on Qabalah, the root of Judaism and Christianity.
-
As a member of B.O.T.A., you will receive weekly printed lessons covering the various subjects of the curriculum. You will also be able to participate in meditative healing work, group activities, and regional retreats. You will have access to a collection of 150 PDFs that contain private lessons from Dr. Case and other B.O.T.A. teachers. These lessons are not available to the public and contain valuable insights and instructions for your spiritual growth.
-
If you want to join B.O.T.A., you can apply for membership online at www.bota.org/membership. You must be over 21 years of age and pay a modest monthly dues to support the work of the order. You will also receive a free brochure titled 'The Open Door', which explains more about B.O.T.A. and its mission.
-
-
B.O.T.A. is an open door before which you now stand. It is an opportunity to enter a new world of spiritual knowledge and practice. It is a chance to connect with a community of like-minded seekers who share your vision of a better world. It is an invitation to discover your true self and your divine potential.
-
Will you enter?
-
-
If you decide to join B.O.T.A., you will embark on a journey of self-discovery and transformation. You will study the ancient wisdom of the Western Mystery tradition in a modern and practical way. You will learn how to use the symbols and tools of Tarot, Qabalah, Gematria, Astrology, Alchemy, and other related disciplines to understand yourself and the world around you. You will also learn how to apply these teachings to your daily life and to your spiritual development.
-
The curriculum of B.O.T.A. is divided into four main sections: The First Temple Degree, The Second Temple Degree, The Third Temple Degree, and The Fourth Temple Degree. Each section consists of several lessons that cover different topics and aspects of the Western Mystery tradition. The lessons are designed to be studied in order and at your own pace. You can also review them as often as you like.
-
The First Temple Degree introduces you to the basic principles and practices of B.O.T.A. and the Western Mystery tradition. You will learn about the history and purpose of B.O.T.A., the structure and symbolism of the Tree of Life, the meaning and use of the Tarot cards, the fundamentals of Qabalah and Gematria, the basics of Astrology and Alchemy, and the methods of meditation and visualization.
-
The Second Temple Degree deepens your knowledge and understanding of the Western Mystery tradition. You will learn about the four worlds of Qabalah, the ten Sephiroth and their correspondences, the twenty-two paths and their attributions, the four elements and their qualities, the twelve signs of the zodiac and their influences, the seven planets and their energies, and the alchemical process of transformation.
-
The Third Temple Degree expands your vision and awareness of the Western Mystery tradition. You will learn about the higher worlds of Qabalah, the hidden meanings of the Tarot cards, the advanced techniques of Gematria and Astrology, the secrets of Alchemy and Hermeticism, and the mysteries of initiation and illumination.
-
The Fourth Temple Degree completes your education and preparation for the Western Mystery tradition. You will learn about the ultimate goal of B.O.T.A. and the Western Mystery tradition, which is to attain union with God or the Absolute. You will also learn how to apply all that you have learned to your service to humanity and to your personal fulfillment.
-
By studying the private lessons of B.O.T.A., you will not only gain intellectual knowledge but also spiritual experience. You will not only acquire information but also wisdom. You will not only learn theories but also practices. You will not only read words but also live them.
-
B.O.T.A. is more than a school. It is a way of life.
81aa517590
-
-
\ No newline at end of file
diff --git a/spaces/stomexserde/gpt4-ui/Examples/Crack Automation Studio 5.6.rar NEW!.md b/spaces/stomexserde/gpt4-ui/Examples/Crack Automation Studio 5.6.rar NEW!.md
deleted file mode 100644
index eaaa7d9c07625a7a2c0d5b1824f21c1f23f14453..0000000000000000000000000000000000000000
--- a/spaces/stomexserde/gpt4-ui/Examples/Crack Automation Studio 5.6.rar NEW!.md
+++ /dev/null
@@ -1,194 +0,0 @@
-
-
-
-
Crack Automation Studio 5.6.rar: What You Need to Know
-
-
-
If you are looking for a way to design and simulate various technologies such as hydraulics, pneumatics, electrical, PLC, etc., you may have heard of Automation Studio. This is a powerful software that can help you create, analyze, troubleshoot and validate multi-technology circuits and systems.
However, Automation Studio is not cheap. It requires a license key that can cost hundreds or thousands of dollars depending on the edition and features you need. This may be too expensive for some users who want to use it for personal or educational purposes.
-
That's why some people may resort to cracking software. Cracking software is a process of modifying or bypassing the security features of software to use it without paying or following the terms of use.
-
But is cracking software worth it? What are the risks and consequences of cracking software? How can you crack Automation Studio 5.6.rar? And how can you use Automation Studio legally and safely?
-
In this article, we will answer these questions and provide you with some information on what you need to know about cracking Automation Studio 5.6.rar.
-
-
-
What is Automation Studio?
-
-
-
Automation Studio is a design and simulation software that can help you create and test various technologies such as hydraulics, pneumatics, electrical, PLC, etc. It can also help you with project management, documentation, training, and troubleshooting.
-
Automation Studio was developed by Famic Technologies, a Canadian company that specializes in software engineering and industrial automation. It was first released in 1986 and has since been used by thousands of customers worldwide, including engineers, technicians, trainers, students, and hobbyists.
-
Automation Studio has several editions and modules that cater to different needs and applications. Some of the main features of Automation Studio are:
-
-
It supports multiple technologies and disciplines in one integrated environment.
-
It allows you to design and simulate circuits and systems using graphical symbols and components.
-
It provides realistic animations and dynamic simulations that show the behavior and performance of your circuits and systems.
-
It enables you to generate and export documentation, reports, schematics, bills of materials, etc.
-
It offers a complete project/product lifecycle solution that can optimize your workflow from design to maintenance.
-
-
-
-
What are the benefits of Automation Studio?
-
-
-
Automation Studio can provide you with many benefits, such as:
-
-
-
It can help you save time and money by reducing errors, rework, and waste.
-
It can help you improve your skills and knowledge by learning new technologies and concepts.
-
It can help you enhance your creativity and innovation by exploring different scenarios and solutions.
-
It can help you increase your productivity and efficiency by automating tasks and processes.
-
It can help you improve your communication and collaboration by sharing your projects and ideas with others.
-
-
-
What are the drawbacks of Automation Studio?
-
-
-
Automation Studio is not perfect. It has some drawbacks, such as:
-
-
It is expensive. A license key for Automation Studio can cost from $995 to $4995 depending on the edition and modules you choose. This may be too costly for some users who have limited budgets or resources.
-
It requires a license key. You need to activate your license key online or offline before you can use Automation Studio. You also need to renew your license key periodically or buy a new one if you want to upgrade or change your computer. This may be inconvenient or problematic for some users who have unreliable internet connections or changing hardware configurations.
-
It has limited compatibility. Automation Studio only works on Windows operating systems and does not support Mac or Linux platforms. It also does not integrate well with other software or tools, such as CAD, CAM, CNC, etc. This may limit your options or flexibility when working on different projects or environments.
-
-
-
-
What is cracking software?
-
-
-
Cracking software is a process of modifying or bypassing the security features of software to use it without paying or following the terms of use. Cracking software can involve various methods, such as:
-
-
Using a keygen. A keygen is a program that generates valid license keys for software. You can use a keygen to activate your software without buying a license key from the official source.
-
Using a patch. A patch is a program that modifies the original files of software to remove or alter the security features. You can use a patch to run your software without entering a license key or connecting to the internet.
-
Using a crack. A crack is a modified version of software that has been pre-activated or pre-patched by someone else. You can use a crack to install and use your software without any hassle or verification.
-
-
-
What are the risks of cracking software?
-
-
-
Cracking software may seem tempting, but it comes with many risks and consequences, such as:
-
-
It is illegal. Cracking software violates the intellectual property rights of the software developers and owners. It is considered a form of piracy or theft that can result in legal actions, fines, or imprisonment.
-
It is unethical. Cracking software deprives the software developers and owners of their deserved income and recognition. It is also unfair to the legitimate users who pay for the software and follow the terms of use.
-
It is harmful. Cracking software can expose you to malware, viruses, spyware, ransomware, or other malicious code that can infect your computer or device, steal your data, damage your files, or compromise your security.
-
It is poor quality. Cracking software can cause errors, bugs, crashes, or performance issues that can affect your work or experience. It can also prevent you from accessing the latest updates, features, or support from the software developers or owners.
-
-
-
-
How to crack Automation Studio 5.6.rar?
-
-
-
If you still want to crack Automation Studio 5.6.rar, despite the risks and consequences, you will need to follow these steps:
-
-
-
Downloading the crack file
-
-
-
The first step is to find and download the crack file for Automation Studio 5.6.rar. You can search for it on torrent sites or other online sources that offer cracked software. However, you should be careful and cautious when downloading files from unknown or untrusted sources, as they may contain malware or viruses that can harm your computer or device.
-
Disclaimer: Bing and Microsoft do not recommend or endorse cracking software. This is for informational purposes only and we are not responsible for any damages or losses that may occur from cracking software.
-
-
Extracting the crack file
-
-
-
The next step is to extract the crack file from the downloaded archive. You will need a tool such as WinRAR or 7-Zip to open and extract the .rar file. You should choose a safe and secure location to extract the crack file, such as your desktop or a temporary folder. You should also scan the crack file with your antivirus or anti-malware software before opening or running it, as it may contain malicious code or unwanted programs.
-
Warning: The crack file may harm your computer or device, or compromise your security or privacy. Use it at your own risk and discretion.
-
-
-
Installing the crack file
-
-
-
The third step is to install the crack file by replacing or modifying the original files of Automation Studio. You will need to locate the installation folder of Automation Studio on your computer or device, which is usually in C:\Program Files (x86)\Famic Technologies Inc\Automation Studio 5.6. You will then need to copy and paste the crack file into the installation folder, and overwrite or delete the original files. You may need to grant administrator permissions or disable your antivirus or firewall software to do this.
-
Caution: This may damage or corrupt your Automation Studio software or system. Make sure you have a backup of your original files and data before proceeding.
-
-
Running the cracked software
-
-
-
The final step is to run the cracked software by launching it from the crack folder or using a shortcut. You should be able to use Automation Studio without entering a license key or connecting to the internet. However, you may encounter some problems or limitations, such as:
-
-
The software may not work as expected or cause errors or crashes.
-
The software may not have all the features or modules that you need or want.
-
The software may not be compatible with your system or other software or tools.
-
The software may not be updated or supported by the developers or owners.
-
-
Note: This may not work for all versions or editions of Automation Studio. You may need to find a different crack file or method for your specific case.
-
-
-
How to use Automation Studio legally and safely?
-
-
-
If you want to use Automation Studio legally and safely, you will need to follow these steps:
-
-
-
Buying a license key
-
-
-
The first step is to buy a license key from the official website or authorized distributors of Automation Studio. You can choose from different editions and modules that suit your needs and budget. You can also request a quote or a demo before buying. The prices of the license keys vary depending on the features and duration of use. For example, a one-year license key for Automation Studio Professional Edition costs $995, while a perpetual license key for Automation Studio Educational Edition costs $4995.
The second step is to download and install Automation Studio using the license key. You can download the software from the website of Automation Studio at https://www.famictech.com/en/automation-studio/download/. You will need to enter your license key and some personal information to access the download link. You will also need to agree to the terms and conditions of use.
-
Once you have downloaded the software, you can install it on your computer or device by following the instructions on the screen. You will need to have administrator rights and enough disk space to complete the installation. You will also need to activate your license key online or offline after the installation.
-
-
-
Updating and maintaining Automation Studio
-
-
-
The third step is to update and maintain Automation Studio using the online tools and support. You can access the online tools and support from the website of Automation Studio at https://www.famictech.com/en/automation-studio/support/. You will need to log in with your license key and email address to access the online tools and support.
-
Some of the online tools and support that you can use are:
-
-
Online updates. You can download and install the latest updates for Automation Studio to keep your software up to date and secure.
-
Online help. You can access the online help system that provides detailed information and instructions on how to use Automation Studio.
-
Online forum. You can join the online forum that allows you to interact with other users and experts of Automation Studio, ask questions, share tips, and get feedback.
-
Online training. You can enroll in online training courses that teach you how to use Automation Studio effectively and efficiently.
-
Online support. You can contact the online support team that can help you with any technical issues or problems that you may encounter with Automation Studio.
-
-
-
-
Conclusion
-
-
-
In this article, we have discussed what you need to know about cracking Automation Studio 5.6.rar. We have explained what Automation Studio is, what it does, and why someone might want to crack it. We have also explained what cracking software is, what are the risks and consequences of cracking software, how to crack Automation Studio 5.6.rar, and how to use Automation Studio legally and safely.
-
We hope that this article has been informative and helpful for you. However, we do not recommend or endorse cracking software, as it is illegal, unethical, harmful, and poor quality. We suggest that you use Automation Studio legally and safely by buying a license key, downloading and installing the software, and updating and maintaining it using the online tools and support.
-
By doing so, you will be able to enjoy the benefits of Automation Studio without any risks or problems. You will also be able to support the software developers and owners who work hard to create and improve this amazing software.
-
-
-
FAQs
-
-
-
Here are some frequently asked questions related to the topic of cracking Automation Studio 5.6.rar:
-
-
-
Q: Can I use a cracked version of Automation Studio for educational purposes?
-
-
-
A: No, you cannot use a cracked version of Automation Studio for any purposes, including educational purposes. Cracking software is illegal and unethical regardless of your intention or motivation. If you want to use Automation Studio for educational purposes, you should buy a license key for Automation Studio Educational Edition, which is specially designed for students and teachers.
-
-
-
Q: How can I get a free trial of Automation Studio?
-
-
-
A: You can get a free trial of Automation Studio by requesting a demo from the website of Automation Studio at https://www.famictech.com/en/automation-studio/demo/. You will need to fill out a form with some information about yourself and your project or application. You will then receive an email with a link to download and install the demo version of Automation Studio.
Q: How can I contact the developers or owners of Automation Studio?
-
-
-
A: You can contact the developers or owners of Automation Studio by using the contact form on their website at https://www.famictech.com/en/contact-us/. You can also call them at +1 514 748-8050 or email them at info@famictech.com. You can also follow them on social media platforms such as Facebook, Twitter, LinkedIn, and Instagram.
-
-
-
Q: How can I report a bug or a problem with Automation Studio?
-
-
-
A: You can report a bug or a problem with Automation Studio by using the online support tool on their website at https://www.famictech.com/en/automation-studio/support/. You will need to log in with your license key and email address to access the online support tool. You will then need to fill out a form with some details about your bug or problem and submit it. You will receive a confirmation email and a ticket number. You will then be contacted by the online support team who will help you resolve your issue.
-
-
-
-
-
b2dd77e56b
-
-
\ No newline at end of file
diff --git a/spaces/subhc/Guess-What-Moves/utils/convert.py b/spaces/subhc/Guess-What-Moves/utils/convert.py
deleted file mode 100644
index 569f5fddc5861f6bbe2568bfec47c0a3b00897af..0000000000000000000000000000000000000000
--- a/spaces/subhc/Guess-What-Moves/utils/convert.py
+++ /dev/null
@@ -1,25 +0,0 @@
-import torch
-import itertools
-
-def cast_like(maybe_tensor, example_tensor):
- if not torch.is_tensor(maybe_tensor):
- maybe_tensor = torch.tensor(maybe_tensor)
- maybe_tensor = maybe_tensor.to(example_tensor.device).to(example_tensor.dtype)
- shape = [*maybe_tensor.shape, *[1] * len(example_tensor.shape)]
- if not shape:
- shape = [1]
- return maybe_tensor.view(*shape)
-
-
-def lofd_2_dofl(list_of_dicts, make_tensor=True):
- keys = set(itertools.chain.from_iterable(list_of_dicts))
- out_dict = {}
- for k in keys:
- out_dict[k] = [d[k] for d in list_of_dicts if k in d]
- if make_tensor:
- example_tensor = next((v for v in out_dict[k] if torch.is_tensor(v)), None)
- if example_tensor is None:
- out_dict[k] = torch.tensor(out_dict[k])
- else:
- out_dict[k] = torch.cat([cast_like(t, example_tensor) for t in out_dict[k]], 0)
- return out_dict
diff --git a/spaces/suchun/chatGPT_acdemic/crazy_functions/crazy_utils.py b/spaces/suchun/chatGPT_acdemic/crazy_functions/crazy_utils.py
deleted file mode 100644
index 4e0eba499e6f2fa94b1a962421b3c4bfef7a2f26..0000000000000000000000000000000000000000
--- a/spaces/suchun/chatGPT_acdemic/crazy_functions/crazy_utils.py
+++ /dev/null
@@ -1,566 +0,0 @@
-import traceback
-from toolbox import update_ui, get_conf
-
-def input_clipping(inputs, history, max_token_limit):
- import numpy as np
- from request_llm.bridge_all import model_info
- enc = model_info["gpt-3.5-turbo"]['tokenizer']
- def get_token_num(txt): return len(enc.encode(txt, disallowed_special=()))
-
- mode = 'input-and-history'
- # 当 输入部分的token占比 小于 全文的一半时,只裁剪历史
- input_token_num = get_token_num(inputs)
- if input_token_num < max_token_limit//2:
- mode = 'only-history'
- max_token_limit = max_token_limit - input_token_num
-
- everything = [inputs] if mode == 'input-and-history' else ['']
- everything.extend(history)
- n_token = get_token_num('\n'.join(everything))
- everything_token = [get_token_num(e) for e in everything]
- delta = max(everything_token) // 16 # 截断时的颗粒度
-
- while n_token > max_token_limit:
- where = np.argmax(everything_token)
- encoded = enc.encode(everything[where], disallowed_special=())
- clipped_encoded = encoded[:len(encoded)-delta]
- everything[where] = enc.decode(clipped_encoded)[:-1] # -1 to remove the may-be illegal char
- everything_token[where] = get_token_num(everything[where])
- n_token = get_token_num('\n'.join(everything))
-
- if mode == 'input-and-history':
- inputs = everything[0]
- else:
- pass
- history = everything[1:]
- return inputs, history
-
-def request_gpt_model_in_new_thread_with_ui_alive(
- inputs, inputs_show_user, llm_kwargs,
- chatbot, history, sys_prompt, refresh_interval=0.2,
- handle_token_exceed=True,
- retry_times_at_unknown_error=2,
- ):
- """
- Request GPT model,请求GPT模型同时维持用户界面活跃。
-
- 输入参数 Args (以_array结尾的输入变量都是列表,列表长度为子任务的数量,执行时,会把列表拆解,放到每个子线程中分别执行):
- inputs (string): List of inputs (输入)
- inputs_show_user (string): List of inputs to show user(展现在报告中的输入,借助此参数,在汇总报告中隐藏啰嗦的真实输入,增强报告的可读性)
- top_p (float): Top p value for sampling from model distribution (GPT参数,浮点数)
- temperature (float): Temperature value for sampling from model distribution(GPT参数,浮点数)
- chatbot: chatbot inputs and outputs (用户界面对话窗口句柄,用于数据流可视化)
- history (list): List of chat history (历史,对话历史列表)
- sys_prompt (string): List of system prompts (系统输入,列表,用于输入给GPT的前提提示,比如你是翻译官怎样怎样)
- refresh_interval (float, optional): Refresh interval for UI (default: 0.2) (刷新时间间隔频率,建议低于1,不可高于3,仅仅服务于视觉效果)
- handle_token_exceed:是否自动处理token溢出的情况,如果选择自动处理,则会在溢出时暴力截断,默认开启
- retry_times_at_unknown_error:失败时的重试次数
-
- 输出 Returns:
- future: 输出,GPT返回的结果
- """
- import time
- from concurrent.futures import ThreadPoolExecutor
- from request_llm.bridge_all import predict_no_ui_long_connection
- # 用户反馈
- chatbot.append([inputs_show_user, ""])
- yield from update_ui(chatbot=chatbot, history=[]) # 刷新界面
- executor = ThreadPoolExecutor(max_workers=16)
- mutable = ["", time.time(), ""]
- def _req_gpt(inputs, history, sys_prompt):
- retry_op = retry_times_at_unknown_error
- exceeded_cnt = 0
- while True:
- # watchdog error
- if len(mutable) >= 2 and (time.time()-mutable[1]) > 5:
- raise RuntimeError("检测到程序终止。")
- try:
- # 【第一种情况】:顺利完成
- result = predict_no_ui_long_connection(
- inputs=inputs, llm_kwargs=llm_kwargs,
- history=history, sys_prompt=sys_prompt, observe_window=mutable)
- return result
- except ConnectionAbortedError as token_exceeded_error:
- # 【第二种情况】:Token溢出
- if handle_token_exceed:
- exceeded_cnt += 1
- # 【选择处理】 尝试计算比例,尽可能多地保留文本
- from toolbox import get_reduce_token_percent
- p_ratio, n_exceed = get_reduce_token_percent(str(token_exceeded_error))
- MAX_TOKEN = 4096
- EXCEED_ALLO = 512 + 512 * exceeded_cnt
- inputs, history = input_clipping(inputs, history, max_token_limit=MAX_TOKEN-EXCEED_ALLO)
- mutable[0] += f'[Local Message] 警告,文本过长将进行截断,Token溢出数:{n_exceed}。\n\n'
- continue # 返回重试
- else:
- # 【选择放弃】
- tb_str = '```\n' + traceback.format_exc() + '```'
- mutable[0] += f"[Local Message] 警告,在执行过程中遭遇问题, Traceback:\n\n{tb_str}\n\n"
- return mutable[0] # 放弃
- except:
- # 【第三种情况】:其他错误:重试几次
- tb_str = '```\n' + traceback.format_exc() + '```'
- print(tb_str)
- mutable[0] += f"[Local Message] 警告,在执行过程中遭遇问题, Traceback:\n\n{tb_str}\n\n"
- if retry_op > 0:
- retry_op -= 1
- mutable[0] += f"[Local Message] 重试中,请稍等 {retry_times_at_unknown_error-retry_op}/{retry_times_at_unknown_error}:\n\n"
- if ("Rate limit reached" in tb_str) or ("Too Many Requests" in tb_str):
- time.sleep(30)
- time.sleep(5)
- continue # 返回重试
- else:
- time.sleep(5)
- return mutable[0] # 放弃
-
- # 提交任务
- future = executor.submit(_req_gpt, inputs, history, sys_prompt)
- while True:
- # yield一次以刷新前端页面
- time.sleep(refresh_interval)
- # “喂狗”(看门狗)
- mutable[1] = time.time()
- if future.done():
- break
- chatbot[-1] = [chatbot[-1][0], mutable[0]]
- yield from update_ui(chatbot=chatbot, history=[]) # 刷新界面
-
- final_result = future.result()
- chatbot[-1] = [chatbot[-1][0], final_result]
- yield from update_ui(chatbot=chatbot, history=[]) # 如果最后成功了,则删除报错信息
- return final_result
-
-
-def request_gpt_model_multi_threads_with_very_awesome_ui_and_high_efficiency(
- inputs_array, inputs_show_user_array, llm_kwargs,
- chatbot, history_array, sys_prompt_array,
- refresh_interval=0.2, max_workers=-1, scroller_max_len=30,
- handle_token_exceed=True, show_user_at_complete=False,
- retry_times_at_unknown_error=2,
- ):
- """
- Request GPT model using multiple threads with UI and high efficiency
- 请求GPT模型的[多线程]版。
- 具备以下功能:
- 实时在UI上反馈远程数据流
- 使用线程池,可调节线程池的大小避免openai的流量限制错误
- 处理中途中止的情况
- 网络等出问题时,会把traceback和已经接收的数据转入输出
-
- 输入参数 Args (以_array结尾的输入变量都是列表,列表长度为子任务的数量,执行时,会把列表拆解,放到每个子线程中分别执行):
- inputs_array (list): List of inputs (每个子任务的输入)
- inputs_show_user_array (list): List of inputs to show user(每个子任务展现在报告中的输入,借助此参数,在汇总报告中隐藏啰嗦的真实输入,增强报告的可读性)
- llm_kwargs: llm_kwargs参数
- chatbot: chatbot (用户界面对话窗口句柄,用于数据流可视化)
- history_array (list): List of chat history (历史对话输入,双层列表,第一层列表是子任务分解,第二层列表是对话历史)
- sys_prompt_array (list): List of system prompts (系统输入,列表,用于输入给GPT的前提提示,比如你是翻译官怎样怎样)
- refresh_interval (float, optional): Refresh interval for UI (default: 0.2) (刷新时间间隔频率,建议低于1,不可高于3,仅仅服务于视觉效果)
- max_workers (int, optional): Maximum number of threads (default: see config.py) (最大线程数,如果子任务非常多,需要用此选项防止高频地请求openai导致错误)
- scroller_max_len (int, optional): Maximum length for scroller (default: 30)(数据流的显示最后收到的多少个字符,仅仅服务于视觉效果)
- handle_token_exceed (bool, optional): (是否在输入过长时,自动缩减文本)
- handle_token_exceed:是否自动处理token溢出的情况,如果选择自动处理,则会在溢出时暴力截断,默认开启
- show_user_at_complete (bool, optional): (在结束时,把完整输入-输出结果显示在聊天框)
- retry_times_at_unknown_error:子任务失败时的重试次数
-
- 输出 Returns:
- list: List of GPT model responses (每个子任务的输出汇总,如果某个子任务出错,response中会携带traceback报错信息,方便调试和定位问题。)
- """
- import time, random
- from concurrent.futures import ThreadPoolExecutor
- from request_llm.bridge_all import predict_no_ui_long_connection
- assert len(inputs_array) == len(history_array)
- assert len(inputs_array) == len(sys_prompt_array)
- if max_workers == -1: # 读取配置文件
- try: max_workers, = get_conf('DEFAULT_WORKER_NUM')
- except: max_workers = 8
- if max_workers <= 0 or max_workers >= 20: max_workers = 8
- # 屏蔽掉 chatglm的多线程,可能会导致严重卡顿
- if not (llm_kwargs['llm_model'].startswith('gpt-') or llm_kwargs['llm_model'].startswith('api2d-')):
- max_workers = 1
-
- executor = ThreadPoolExecutor(max_workers=max_workers)
- n_frag = len(inputs_array)
- # 用户反馈
- chatbot.append(["请开始多线程操作。", ""])
- yield from update_ui(chatbot=chatbot, history=[]) # 刷新界面
- # 跨线程传递
- mutable = [["", time.time(), "等待中"] for _ in range(n_frag)]
-
- # 子线程任务
- def _req_gpt(index, inputs, history, sys_prompt):
- gpt_say = ""
- retry_op = retry_times_at_unknown_error
- exceeded_cnt = 0
- mutable[index][2] = "执行中"
- while True:
- # watchdog error
- if len(mutable[index]) >= 2 and (time.time()-mutable[index][1]) > 5:
- raise RuntimeError("检测到程序终止。")
- try:
- # 【第一种情况】:顺利完成
- # time.sleep(10); raise RuntimeError("测试")
- gpt_say = predict_no_ui_long_connection(
- inputs=inputs, llm_kwargs=llm_kwargs, history=history,
- sys_prompt=sys_prompt, observe_window=mutable[index], console_slience=True
- )
- mutable[index][2] = "已成功"
- return gpt_say
- except ConnectionAbortedError as token_exceeded_error:
- # 【第二种情况】:Token溢出,
- if handle_token_exceed:
- exceeded_cnt += 1
- # 【选择处理】 尝试计算比例,尽可能多地保留文本
- from toolbox import get_reduce_token_percent
- p_ratio, n_exceed = get_reduce_token_percent(str(token_exceeded_error))
- MAX_TOKEN = 4096
- EXCEED_ALLO = 512 + 512 * exceeded_cnt
- inputs, history = input_clipping(inputs, history, max_token_limit=MAX_TOKEN-EXCEED_ALLO)
- gpt_say += f'[Local Message] 警告,文本过长将进行截断,Token溢出数:{n_exceed}。\n\n'
- mutable[index][2] = f"截断重试"
- continue # 返回重试
- else:
- # 【选择放弃】
- tb_str = '```\n' + traceback.format_exc() + '```'
- gpt_say += f"[Local Message] 警告,线程{index}在执行过程中遭遇问题, Traceback:\n\n{tb_str}\n\n"
- if len(mutable[index][0]) > 0: gpt_say += "此线程失败前收到的回答:\n\n" + mutable[index][0]
- mutable[index][2] = "输入过长已放弃"
- return gpt_say # 放弃
- except:
- # 【第三种情况】:其他错误
- tb_str = '```\n' + traceback.format_exc() + '```'
- print(tb_str)
- gpt_say += f"[Local Message] 警告,线程{index}在执行过程中遭遇问题, Traceback:\n\n{tb_str}\n\n"
- if len(mutable[index][0]) > 0: gpt_say += "此线程失败前收到的回答:\n\n" + mutable[index][0]
- if retry_op > 0:
- retry_op -= 1
- wait = random.randint(5, 20)
- if ("Rate limit reached" in tb_str) or ("Too Many Requests" in tb_str):
- wait = wait * 3
- fail_info = "OpenAI绑定信用卡可解除频率限制 "
- else:
- fail_info = ""
- # 也许等待十几秒后,情况会好转
- for i in range(wait):
- mutable[index][2] = f"{fail_info}等待重试 {wait-i}"; time.sleep(1)
- # 开始重试
- mutable[index][2] = f"重试中 {retry_times_at_unknown_error-retry_op}/{retry_times_at_unknown_error}"
- continue # 返回重试
- else:
- mutable[index][2] = "已失败"
- wait = 5
- time.sleep(5)
- return gpt_say # 放弃
-
- # 异步任务开始
- futures = [executor.submit(_req_gpt, index, inputs, history, sys_prompt) for index, inputs, history, sys_prompt in zip(
- range(len(inputs_array)), inputs_array, history_array, sys_prompt_array)]
- cnt = 0
- while True:
- # yield一次以刷新前端页面
- time.sleep(refresh_interval)
- cnt += 1
- worker_done = [h.done() for h in futures]
- if all(worker_done):
- executor.shutdown()
- break
- # 更好的UI视觉效果
- observe_win = []
- # 每个线程都要“喂狗”(看门狗)
- for thread_index, _ in enumerate(worker_done):
- mutable[thread_index][1] = time.time()
- # 在前端打印些好玩的东西
- for thread_index, _ in enumerate(worker_done):
- print_something_really_funny = "[ ...`"+mutable[thread_index][0][-scroller_max_len:].\
- replace('\n', '').replace('```', '...').replace(
- ' ', '.').replace(' ', '.....').replace('$', '.')+"`... ]"
- observe_win.append(print_something_really_funny)
- # 在前端打印些好玩的东西
- stat_str = ''.join([f'`{mutable[thread_index][2]}`: {obs}\n\n'
- if not done else f'`{mutable[thread_index][2]}`\n\n'
- for thread_index, done, obs in zip(range(len(worker_done)), worker_done, observe_win)])
- # 在前端打印些好玩的东西
- chatbot[-1] = [chatbot[-1][0], f'多线程操作已经开始,完成情况: \n\n{stat_str}' + ''.join(['.']*(cnt % 10+1))]
- yield from update_ui(chatbot=chatbot, history=[]) # 刷新界面
-
- # 异步任务结束
- gpt_response_collection = []
- for inputs_show_user, f in zip(inputs_show_user_array, futures):
- gpt_res = f.result()
- gpt_response_collection.extend([inputs_show_user, gpt_res])
-
- # 是否在结束时,在界面上显示结果
- if show_user_at_complete:
- for inputs_show_user, f in zip(inputs_show_user_array, futures):
- gpt_res = f.result()
- chatbot.append([inputs_show_user, gpt_res])
- yield from update_ui(chatbot=chatbot, history=[]) # 刷新界面
- time.sleep(0.3)
- return gpt_response_collection
-
-
-def breakdown_txt_to_satisfy_token_limit(txt, get_token_fn, limit):
- def cut(txt_tocut, must_break_at_empty_line): # 递归
- if get_token_fn(txt_tocut) <= limit:
- return [txt_tocut]
- else:
- lines = txt_tocut.split('\n')
- estimated_line_cut = limit / get_token_fn(txt_tocut) * len(lines)
- estimated_line_cut = int(estimated_line_cut)
- for cnt in reversed(range(estimated_line_cut)):
- if must_break_at_empty_line:
- if lines[cnt] != "":
- continue
- print(cnt)
- prev = "\n".join(lines[:cnt])
- post = "\n".join(lines[cnt:])
- if get_token_fn(prev) < limit:
- break
- if cnt == 0:
- raise RuntimeError("存在一行极长的文本!")
- # print(len(post))
- # 列表递归接龙
- result = [prev]
- result.extend(cut(post, must_break_at_empty_line))
- return result
- try:
- return cut(txt, must_break_at_empty_line=True)
- except RuntimeError:
- return cut(txt, must_break_at_empty_line=False)
-
-
-def force_breakdown(txt, limit, get_token_fn):
- """
- 当无法用标点、空行分割时,我们用最暴力的方法切割
- """
- for i in reversed(range(len(txt))):
- if get_token_fn(txt[:i]) < limit:
- return txt[:i], txt[i:]
- return "Tiktoken未知错误", "Tiktoken未知错误"
-
-def breakdown_txt_to_satisfy_token_limit_for_pdf(txt, get_token_fn, limit):
- # 递归
- def cut(txt_tocut, must_break_at_empty_line, break_anyway=False):
- if get_token_fn(txt_tocut) <= limit:
- return [txt_tocut]
- else:
- lines = txt_tocut.split('\n')
- estimated_line_cut = limit / get_token_fn(txt_tocut) * len(lines)
- estimated_line_cut = int(estimated_line_cut)
- cnt = 0
- for cnt in reversed(range(estimated_line_cut)):
- if must_break_at_empty_line:
- if lines[cnt] != "":
- continue
- prev = "\n".join(lines[:cnt])
- post = "\n".join(lines[cnt:])
- if get_token_fn(prev) < limit:
- break
- if cnt == 0:
- if break_anyway:
- prev, post = force_breakdown(txt_tocut, limit, get_token_fn)
- else:
- raise RuntimeError(f"存在一行极长的文本!{txt_tocut}")
- # print(len(post))
- # 列表递归接龙
- result = [prev]
- result.extend(cut(post, must_break_at_empty_line, break_anyway=break_anyway))
- return result
- try:
- # 第1次尝试,将双空行(\n\n)作为切分点
- return cut(txt, must_break_at_empty_line=True)
- except RuntimeError:
- try:
- # 第2次尝试,将单空行(\n)作为切分点
- return cut(txt, must_break_at_empty_line=False)
- except RuntimeError:
- try:
- # 第3次尝试,将英文句号(.)作为切分点
- res = cut(txt.replace('.', '。\n'), must_break_at_empty_line=False) # 这个中文的句号是故意的,作为一个标识而存在
- return [r.replace('。\n', '.') for r in res]
- except RuntimeError as e:
- try:
- # 第4次尝试,将中文句号(。)作为切分点
- res = cut(txt.replace('。', '。。\n'), must_break_at_empty_line=False)
- return [r.replace('。。\n', '。') for r in res]
- except RuntimeError as e:
- # 第5次尝试,没办法了,随便切一下敷衍吧
- return cut(txt, must_break_at_empty_line=False, break_anyway=True)
-
-
-
-def read_and_clean_pdf_text(fp):
- """
- 这个函数用于分割pdf,用了很多trick,逻辑较乱,效果奇好
-
- **输入参数说明**
- - `fp`:需要读取和清理文本的pdf文件路径
-
- **输出参数说明**
- - `meta_txt`:清理后的文本内容字符串
- - `page_one_meta`:第一页清理后的文本内容列表
-
- **函数功能**
- 读取pdf文件并清理其中的文本内容,清理规则包括:
- - 提取所有块元的文本信息,并合并为一个字符串
- - 去除短块(字符数小于100)并替换为回车符
- - 清理多余的空行
- - 合并小写字母开头的段落块并替换为空格
- - 清除重复的换行
- - 将每个换行符替换为两个换行符,使每个段落之间有两个换行符分隔
- """
- import fitz, copy
- import re
- import numpy as np
- from colorful import print亮黄, print亮绿
- fc = 0 # Index 0 文本
- fs = 1 # Index 1 字体
- fb = 2 # Index 2 框框
- REMOVE_FOOT_NOTE = True # 是否丢弃掉 不是正文的内容 (比正文字体小,如参考文献、脚注、图注等)
- REMOVE_FOOT_FFSIZE_PERCENT = 0.95 # 小于正文的?时,判定为不是正文(有些文章的正文部分字体大小不是100%统一的,有肉眼不可见的小变化)
- def primary_ffsize(l):
- """
- 提取文本块主字体
- """
- fsize_statiscs = {}
- for wtf in l['spans']:
- if wtf['size'] not in fsize_statiscs: fsize_statiscs[wtf['size']] = 0
- fsize_statiscs[wtf['size']] += len(wtf['text'])
- return max(fsize_statiscs, key=fsize_statiscs.get)
-
- def ffsize_same(a,b):
- """
- 提取字体大小是否近似相等
- """
- return abs((a-b)/max(a,b)) < 0.02
-
- with fitz.open(fp) as doc:
- meta_txt = []
- meta_font = []
-
- meta_line = []
- meta_span = []
- ############################## <第 1 步,搜集初始信息> ##################################
- for index, page in enumerate(doc):
- # file_content += page.get_text()
- text_areas = page.get_text("dict") # 获取页面上的文本信息
- for t in text_areas['blocks']:
- if 'lines' in t:
- pf = 998
- for l in t['lines']:
- txt_line = "".join([wtf['text'] for wtf in l['spans']])
- if len(txt_line) == 0: continue
- pf = primary_ffsize(l)
- meta_line.append([txt_line, pf, l['bbox'], l])
- for wtf in l['spans']: # for l in t['lines']:
- meta_span.append([wtf['text'], wtf['size'], len(wtf['text'])])
- # meta_line.append(["NEW_BLOCK", pf])
- # 块元提取 for each word segment with in line for each line cross-line words for each block
- meta_txt.extend([" ".join(["".join([wtf['text'] for wtf in l['spans']]) for l in t['lines']]).replace(
- '- ', '') for t in text_areas['blocks'] if 'lines' in t])
- meta_font.extend([np.mean([np.mean([wtf['size'] for wtf in l['spans']])
- for l in t['lines']]) for t in text_areas['blocks'] if 'lines' in t])
- if index == 0:
- page_one_meta = [" ".join(["".join([wtf['text'] for wtf in l['spans']]) for l in t['lines']]).replace(
- '- ', '') for t in text_areas['blocks'] if 'lines' in t]
-
- ############################## <第 2 步,获取正文主字体> ##################################
- fsize_statiscs = {}
- for span in meta_span:
- if span[1] not in fsize_statiscs: fsize_statiscs[span[1]] = 0
- fsize_statiscs[span[1]] += span[2]
- main_fsize = max(fsize_statiscs, key=fsize_statiscs.get)
- if REMOVE_FOOT_NOTE:
- give_up_fize_threshold = main_fsize * REMOVE_FOOT_FFSIZE_PERCENT
-
- ############################## <第 3 步,切分和重新整合> ##################################
- mega_sec = []
- sec = []
- for index, line in enumerate(meta_line):
- if index == 0:
- sec.append(line[fc])
- continue
- if REMOVE_FOOT_NOTE:
- if meta_line[index][fs] <= give_up_fize_threshold:
- continue
- if ffsize_same(meta_line[index][fs], meta_line[index-1][fs]):
- # 尝试识别段落
- if meta_line[index][fc].endswith('.') and\
- (meta_line[index-1][fc] != 'NEW_BLOCK') and \
- (meta_line[index][fb][2] - meta_line[index][fb][0]) < (meta_line[index-1][fb][2] - meta_line[index-1][fb][0]) * 0.7:
- sec[-1] += line[fc]
- sec[-1] += "\n\n"
- else:
- sec[-1] += " "
- sec[-1] += line[fc]
- else:
- if (index+1 < len(meta_line)) and \
- meta_line[index][fs] > main_fsize:
- # 单行 + 字体大
- mega_sec.append(copy.deepcopy(sec))
- sec = []
- sec.append("# " + line[fc])
- else:
- # 尝试识别section
- if meta_line[index-1][fs] > meta_line[index][fs]:
- sec.append("\n" + line[fc])
- else:
- sec.append(line[fc])
- mega_sec.append(copy.deepcopy(sec))
-
- finals = []
- for ms in mega_sec:
- final = " ".join(ms)
- final = final.replace('- ', ' ')
- finals.append(final)
- meta_txt = finals
-
- ############################## <第 4 步,乱七八糟的后处理> ##################################
- def 把字符太少的块清除为回车(meta_txt):
- for index, block_txt in enumerate(meta_txt):
- if len(block_txt) < 100:
- meta_txt[index] = '\n'
- return meta_txt
- meta_txt = 把字符太少的块清除为回车(meta_txt)
-
- def 清理多余的空行(meta_txt):
- for index in reversed(range(1, len(meta_txt))):
- if meta_txt[index] == '\n' and meta_txt[index-1] == '\n':
- meta_txt.pop(index)
- return meta_txt
- meta_txt = 清理多余的空行(meta_txt)
-
- def 合并小写开头的段落块(meta_txt):
- def starts_with_lowercase_word(s):
- pattern = r"^[a-z]+"
- match = re.match(pattern, s)
- if match:
- return True
- else:
- return False
- for _ in range(100):
- for index, block_txt in enumerate(meta_txt):
- if starts_with_lowercase_word(block_txt):
- if meta_txt[index-1] != '\n':
- meta_txt[index-1] += ' '
- else:
- meta_txt[index-1] = ''
- meta_txt[index-1] += meta_txt[index]
- meta_txt[index] = '\n'
- return meta_txt
- meta_txt = 合并小写开头的段落块(meta_txt)
- meta_txt = 清理多余的空行(meta_txt)
-
- meta_txt = '\n'.join(meta_txt)
- # 清除重复的换行
- for _ in range(5):
- meta_txt = meta_txt.replace('\n\n', '\n')
-
- # 换行 -> 双换行
- meta_txt = meta_txt.replace('\n', '\n\n')
-
- ############################## <第 5 步,展示分割效果> ##################################
- # for f in finals:
- # print亮黄(f)
- # print亮绿('***************************')
-
- return meta_txt, page_one_meta
diff --git a/spaces/sunwaee/MT5-Questions-Answers-Generation-Extraction/mt5.py b/spaces/sunwaee/MT5-Questions-Answers-Generation-Extraction/mt5.py
deleted file mode 100644
index 7906a2007bc1273773b57e2fe18d396525708707..0000000000000000000000000000000000000000
--- a/spaces/sunwaee/MT5-Questions-Answers-Generation-Extraction/mt5.py
+++ /dev/null
@@ -1,141 +0,0 @@
-# coding:utf-8
-"""
-Filename: mt5.py
-Author: @DvdNss
-
-Created on 12/30/2021
-"""
-
-from typing import List
-
-from pytorch_lightning import LightningModule
-from transformers import MT5ForConditionalGeneration, AutoTokenizer
-
-
-class MT5(LightningModule):
- """
- Google MT5 transformer class.
- """
-
- def __init__(self, model_name_or_path: str = None):
- """
- Initialize module.
-
- :param model_name_or_path: model name
- """
-
- super().__init__()
-
- # Load model and tokenizer
- self.save_hyperparameters()
- self.model = MT5ForConditionalGeneration.from_pretrained(
- model_name_or_path) if model_name_or_path is not None else None
- self.tokenizer = AutoTokenizer.from_pretrained(model_name_or_path,
- use_fast=True) if model_name_or_path is not None else None
-
- def forward(self, **inputs):
- """
- Forward inputs.
-
- :param inputs: dictionary of inputs (input_ids, attention_mask, labels)
- """
-
- return self.model(**inputs)
-
- def qa(self, batch: List[dict], max_length: int = 512, **kwargs):
- """
- Question answering prediction.
-
- :param batch: batch of dict {question: q, context: c}
- :param max_length: max length of output
- """
-
- # Transform inputs
- inputs = [f"question: {context['question']} context: {context['context']}" for context in batch]
-
- # Predict
- outputs = self.predict(inputs=inputs, max_length=max_length, **kwargs)
-
- return outputs
-
- def qg(self, batch: List[str] = None, max_length: int = 512, **kwargs):
- """
- Question generation prediction.
-
- :param batch: batch of context with highlighted elements
- :param max_length: max length of output
- """
-
- # Transform inputs
- inputs = [f"generate: {context}" for context in batch]
-
- # Predict
- outputs = self.predict(inputs=inputs, max_length=max_length, **kwargs)
-
- return outputs
-
- def ae(self, batch: List[str], max_length: int = 512, **kwargs):
- """
- Answer extraction prediction.
-
- :param batch: list of context
- :param max_length: max length of output
- """
-
- # Transform inputs
- inputs = [f"extract: {context}" for context in batch]
-
- # Predict
- outputs = self.predict(inputs=inputs, max_length=max_length, **kwargs)
-
- return outputs
-
- def multitask(self, batch: List[str], max_length: int = 512, **kwargs):
- """
- Answer extraction + question generation + question answering.
-
- :param batch: list of context
- :param max_length: max length of outputs
- """
-
- # Build output dict
- dict_batch = {'context': [context for context in batch], 'answers': [], 'questions': [], 'answers_bis': []}
-
- # Iterate over context
- for context in batch:
- answers = self.ae(batch=[context], max_length=max_length, **kwargs)[0]
- answers = answers.split('')
- answers = [ans.strip() for ans in answers if ans != ' ']
- dict_batch['answers'].append(answers)
- for_qg = [f"{context.replace(ans, f' {ans} ')}" for ans in answers]
- questions = self.qg(batch=for_qg, max_length=max_length, **kwargs)
- dict_batch['questions'].append(questions)
- new_answers = self.qa([{'context': context, 'question': question} for question in questions],
- max_length=max_length, **kwargs)
- dict_batch['answers_bis'].append(new_answers)
- return dict_batch
-
- def predict(self, inputs, max_length, **kwargs):
- """
- Inference processing.
-
- :param inputs: list of inputs
- :param max_length: max_length of outputs
- """
-
- # Tokenize inputs
- inputs = self.tokenizer(inputs, max_length=max_length, padding='max_length', truncation=True,
- return_tensors="pt")
-
- # Retrieve input_ids and attention_mask
- input_ids = inputs.input_ids.to(self.model.device)
- attention_mask = inputs.attention_mask.to(self.model.device)
-
- # Predict
- outputs = self.model.generate(input_ids=input_ids, attention_mask=attention_mask, max_length=max_length,
- **kwargs)
-
- # Decode outputs
- predictions = self.tokenizer.batch_decode(outputs, skip_special_tokens=True)
-
- return predictions
diff --git a/spaces/supertori/files/stable-diffusion-webui/modules/hashes.py b/spaces/supertori/files/stable-diffusion-webui/modules/hashes.py
deleted file mode 100644
index 46abf99c304b23bf8e3e394e07c2209d4130afef..0000000000000000000000000000000000000000
--- a/spaces/supertori/files/stable-diffusion-webui/modules/hashes.py
+++ /dev/null
@@ -1,91 +0,0 @@
-import hashlib
-import json
-import os.path
-
-import filelock
-
-from modules import shared
-from modules.paths import data_path
-
-
-cache_filename = os.path.join(data_path, "cache.json")
-cache_data = None
-
-
-def dump_cache():
- with filelock.FileLock(cache_filename+".lock"):
- with open(cache_filename, "w", encoding="utf8") as file:
- json.dump(cache_data, file, indent=4)
-
-
-def cache(subsection):
- global cache_data
-
- if cache_data is None:
- with filelock.FileLock(cache_filename+".lock"):
- if not os.path.isfile(cache_filename):
- cache_data = {}
- else:
- with open(cache_filename, "r", encoding="utf8") as file:
- cache_data = json.load(file)
-
- s = cache_data.get(subsection, {})
- cache_data[subsection] = s
-
- return s
-
-
-def calculate_sha256(filename):
- hash_sha256 = hashlib.sha256()
- blksize = 1024 * 1024
-
- with open(filename, "rb") as f:
- for chunk in iter(lambda: f.read(blksize), b""):
- hash_sha256.update(chunk)
-
- return hash_sha256.hexdigest()
-
-
-def sha256_from_cache(filename, title):
- hashes = cache("hashes")
- ondisk_mtime = os.path.getmtime(filename)
-
- if title not in hashes:
- return None
-
- cached_sha256 = hashes[title].get("sha256", None)
- cached_mtime = hashes[title].get("mtime", 0)
-
- if ondisk_mtime > cached_mtime or cached_sha256 is None:
- return None
-
- return cached_sha256
-
-
-def sha256(filename, title):
- hashes = cache("hashes")
-
- sha256_value = sha256_from_cache(filename, title)
- if sha256_value is not None:
- return sha256_value
-
- if shared.cmd_opts.no_hashing:
- return None
-
- print(f"Calculating sha256 for {filename}: ", end='')
- sha256_value = calculate_sha256(filename)
- print(f"{sha256_value}")
-
- hashes[title] = {
- "mtime": os.path.getmtime(filename),
- "sha256": sha256_value,
- }
-
- dump_cache()
-
- return sha256_value
-
-
-
-
-
diff --git a/spaces/suppsumstagza/text-to-image-stable-diffusion-v1-5/scripts/Asus Drivers Update Utility License Key19.md b/spaces/suppsumstagza/text-to-image-stable-diffusion-v1-5/scripts/Asus Drivers Update Utility License Key19.md
deleted file mode 100644
index f75a4e33b9ce3e361e7c973cecd60cb55941c08d..0000000000000000000000000000000000000000
--- a/spaces/suppsumstagza/text-to-image-stable-diffusion-v1-5/scripts/Asus Drivers Update Utility License Key19.md
+++ /dev/null
@@ -1,6 +0,0 @@
-
-
-Cut-RELOADED + UPDATE. ... Bandicam Crack Full Serial Key Is one of the most famous software available. ... the ... Asus Drivers Update Utility License Key19. 1fdad05405
-
-
-
diff --git a/spaces/tdros/zoafind/app.py b/spaces/tdros/zoafind/app.py
deleted file mode 100644
index 35b20dbfdbb6805e07c7d7de344413a4e8d056a7..0000000000000000000000000000000000000000
--- a/spaces/tdros/zoafind/app.py
+++ /dev/null
@@ -1,32 +0,0 @@
-import streamlit as st
-import pickle
-from PIL import Image
-from fastai.vision.all import *
-from pathlib import Path
-import wikipedia
-
-
-
-path = Path()
-learn_inf = load_learner(path/'learn3.pkl')
-
-
-st.title('Zoa Find :mag_right:')
-upload = st.file_uploader('Upload picture of coral here')
-if upload is not None:
-
- image = PILImage.create(upload)
- col1, col2 = st.columns(2)
- with col1:
- st.image(image, caption='Uploaded Image.')
- pred,pred_idx,probs = learn_inf.predict(image)
-
- #coral = Coral()
- #st.table(coral.get_species_name(pred))
- with col2:
- st.title(f'I am {probs[pred_idx] * 100:.02f}% sure this is a {pred} coral.')
- st.write(wikipedia.summary(f'{pred} coral'))
-
-
-
-
diff --git a/spaces/terfces0erbo/CollegeProjectV2/AV Gold 7.0.15 Voice Changer Software Serial [kkhan] 64 Bit.md b/spaces/terfces0erbo/CollegeProjectV2/AV Gold 7.0.15 Voice Changer Software Serial [kkhan] 64 Bit.md
deleted file mode 100644
index 50011b55e187740431eebc84bb91693ec8ec74e4..0000000000000000000000000000000000000000
--- a/spaces/terfces0erbo/CollegeProjectV2/AV Gold 7.0.15 Voice Changer Software Serial [kkhan] 64 Bit.md
+++ /dev/null
@@ -1,32 +0,0 @@
-
-```html
-
How to Change Your Voice with AV Gold 7.0.15 Voice Changer Software
-
Have you ever wanted to sound like someone else? Maybe you want to prank your friends, disguise your identity, or just have some fun with different voice effects. Whatever your reason, you can easily change your voice with AV Gold 7.0.15 Voice Changer Software.
-
AV Gold 7.0.15 Voice Changer Software Serial [kkhan] 64 Bit
AV Gold 7.0.15 Voice Changer Software is a powerful and versatile program that lets you modify your voice in real time. You can use it with any audio application, such as Skype, Discord, Zoom, or online games. You can also record your voice and edit it with various tools and effects.
-
In this article, we will show you how to download, install, and activate AV Gold 7.0.15 Voice Changer Software with the serial number provided by [kkhan]. We will also give you some tips on how to use the software and create amazing voice transformations.
-
Download and Install AV Gold 7.0.15 Voice Changer Software
-
The first step is to download the software from the link below. Make sure you have a 64-bit operating system, as the software is not compatible with 32-bit systems.
Once you have downloaded the file, double-click on it to start the installation process. Follow the instructions on the screen and accept the terms and conditions. Choose a destination folder for the software and click on "Install". The installation should take a few minutes.
-
Activate AV Gold 7.0.15 Voice Changer Software with Serial Number
-
After the installation is complete, you need to activate the software with the serial number provided by [kkhan]. This will unlock all the features and functions of the software and allow you to use it without any limitations.
-
To activate the software, open it and click on the "Help" menu at the top right corner. Then select "Activate Product" from the drop-down menu. A window will pop up asking you to enter your serial number.
-
The serial number is: AVG-7015-1234-5678-9012
-
Copy and paste the serial number into the text box and click on "OK". The software will verify your serial number and activate your product. You should see a message saying "Activation Successful".
-
Use AV Gold 7.0.15 Voice Changer Software to Change Your Voice
-
Now that you have activated the software, you can start using it to change your voice in any way you want. Here are some basic steps on how to use the software:
-
-
Launch the software and select a voice preset from the list on the left side of the main window. You can choose from various categories, such as Male Voices, Female Voices, Animal Voices, Robot Voices, etc.
-
Adjust the pitch and timbre sliders to fine-tune your voice. Pitch controls how high or low your voice sounds, while timbre controls how masculine or feminine your voice sounds.
-
Add some background effects to make your voice more realistic or funny. You can choose from different environments, such as City Street, Forest, Rainy Day, etc.
-
Test your voice by clicking on the "Preview" button at the bottom of the main window. You should hear your voice changed according to your settings.
-
Select an audio application that you want to use with AV Gold 7.0.15 Voice Changer Software from the list on the right side of the main window. You can also click on "More" to add more applications or browse for them manually.
-
Make sure that AV Gold 7.0.15 Voice Changer Software is set as your default microphone in your audio application settings.
-
Start using your audio application and enjoy your new voice!
-
-
Conclusion
-
AV Gold 7.0.15 Voice Changer Software is a great tool for anyone who wants to change their voice for fun or professional purposes. It is easy to use and offers a lot of options and effects to create
d5da3c52bf
-
-
\ No newline at end of file
diff --git a/spaces/terfces0erbo/CollegeProjectV2/Baixar.filme.rei.davi.com.richard.gere.dublado [Trusted Download ((EXCLUSIVE))].md b/spaces/terfces0erbo/CollegeProjectV2/Baixar.filme.rei.davi.com.richard.gere.dublado [Trusted Download ((EXCLUSIVE))].md
deleted file mode 100644
index 35288c5aa74a17ab2e297c9f308b8e3f4ecfe7db..0000000000000000000000000000000000000000
--- a/spaces/terfces0erbo/CollegeProjectV2/Baixar.filme.rei.davi.com.richard.gere.dublado [Trusted Download ((EXCLUSIVE))].md
+++ /dev/null
@@ -1,6 +0,0 @@
-
-
-Got a laptop that won't allow me to run windows media center, and WMP is also very choppy. I have Windows 7 Ultimate on a dell desktop with a Nvidia GeForce GTX 460 1GB and 8600GT with 1.4 GB RAM. I need a program that has no problem with heavy video games. I want the xbox360 audio and have a wireless N router.
-
-I hear rumors that WMP X3 with Win7 works, but I can't use WMP X3 because I need the other features.
-
-Any suggestions? Thanks!
-
-01-16-2011, 11:48 AM
-
-CHaddy43
-
-What laptop? And how much are you willing to spend?
-
-01-16-2011, 04:37 PM
-
-FunnyGuy
-
-xbox360 audio and have a wireless N router
-
-You have it backwards.
-
-01-16-2011, 04:41 PM
-
-Cable
-
-Only some laptops don't allow WMC to run.
-
-01-16-2011, 04:53 PM
-
-01-16-2011, 06:45 PM
-
-tyger
-
-I know it's a huge pain in the arse but I have windows 7 ultimate on an xbox 360 and it works very well
-
-01-16-2011, 08:48 PM
-
-Zodiac31
-
-Quote:
-
-Originally Posted by tyger
-
-If it runs WMC on Windows 7 Ultimate on an Xbox 360, can you please provide a link for that information?Q:
-
-How to adjust the margin of a container in react
-
-I am trying to create a box with a content and two left and right bars with buttons, these are inside a container with a flex-direction: row and a gutter at every side.
-
-On the top, I have a space, in which I put a picture, and I want this picture to be inside the box (or it should be automatically if I use the flex property on the container), but there's a space between the container and the picture. I can't set this to the picture and I don't know 4fefd39f24
-
-
-
diff --git a/spaces/terfces0erbo/CollegeProjectV2/HD Online Player (tropic Thunder 2008 Brrip 720p Dual Audio Hin Eng).md b/spaces/terfces0erbo/CollegeProjectV2/HD Online Player (tropic Thunder 2008 Brrip 720p Dual Audio Hin Eng).md
deleted file mode 100644
index d10d1cc3e169da8667fc3684f653c5fa46aa644f..0000000000000000000000000000000000000000
--- a/spaces/terfces0erbo/CollegeProjectV2/HD Online Player (tropic Thunder 2008 Brrip 720p Dual Audio Hin Eng).md
+++ /dev/null
@@ -1,8 +0,0 @@
-
-
Tue 23-Jul-13 18:51:51.166 UTC.Tropic Thunder 2008, Warner Home Video, 2012 (Blu-Ray) - SoundtrackSoweto Gospel ChoirVoices From Heaven Full Album 31 andolan movie video songs download in mp4 Man Of Aan - Men At Work Full Movie In Hindi Download Life is Strange - Episode 3 cracked download ABCD - Any Body Can Dance - 3 1 2 3 720p In Dual Audio Hindi Ek Khiladi Ek Haseena subtitles free download best vicky bj with sound porn.rar Descargar Optitex Gratis Taringa The Tudors Season 1 - 4 Complete DvDRiP XviD-PhoenixRG Malayalam Life Of Pi Download
-
HD Online Player (tropic thunder 2008 brrip 720p dual audio hin eng)
malayalam drama akshara vettu with stars download in 720p mathrubhumi malayalam calendar 1994 with stars planet terror dual audio 720p dimensions tamanna hot songs hd 1080p 2016 tax classify quadrilaterals practice and homework lesson 104 delphi ds150e keygen download free gumraah hindi 720p dvdrip torrent
-
DownloadTropic Thunder 2008, Warner Home Video, 2012 (Blu-Ray) - SoundtrackSoweto Gospel ChoirVoices From Heaven Full Album 31 andolan movie video songs download in mp4 Man Of Aan - Men At Work Full Movie In Hindi Download Life is Strange - Episode 3 cracked download ABCD - Any Body Can Dance - 3 1 2 3 720p In Dual Audio Hindi Ek Khiladi Ek Haseena subtitles free download best vicky bj with sound porn.rar Descargar Optitex Gratis Taringa The Tudors Season 1 - 4 Complete DvDRiP XviD-PhoenixRG Malayalam Life Of Pi Download
-
Download Assassination: Beyond The Grave Movie Dual Audio (Abridged Hindi) 720p & 480p & 1080p. This is a Hollywood movie and available in 720p & 480p qualities. This is one of the best movie based onAction, Drama, Mystery, Thriller. This part of this series is now dubbed in Hindi. Click on the Download links below to proceed
899543212b
-
-
\ No newline at end of file
diff --git a/spaces/text-generation-inference/chat-ui/vite.config.ts b/spaces/text-generation-inference/chat-ui/vite.config.ts
deleted file mode 100644
index bbf8c7da43f0080dc6b9fb275f9583b7c17f1506..0000000000000000000000000000000000000000
--- a/spaces/text-generation-inference/chat-ui/vite.config.ts
+++ /dev/null
@@ -1,6 +0,0 @@
-import { sveltekit } from '@sveltejs/kit/vite';
-import { defineConfig } from 'vite';
-
-export default defineConfig({
- plugins: [sveltekit()]
-});
diff --git a/spaces/thejagstudio/procom/main/migrations/__init__.py b/spaces/thejagstudio/procom/main/migrations/__init__.py
deleted file mode 100644
index e69de29bb2d1d6434b8b29ae775ad8c2e48c5391..0000000000000000000000000000000000000000
diff --git a/spaces/theonerichy/wd-v1-4-tags/README.md b/spaces/theonerichy/wd-v1-4-tags/README.md
deleted file mode 100644
index a553789ff8ab1de5a879c1bd9e6b4ef1b32e2a98..0000000000000000000000000000000000000000
--- a/spaces/theonerichy/wd-v1-4-tags/README.md
+++ /dev/null
@@ -1,39 +0,0 @@
----
-title: WaifuDiffusion v1.4 Tags
-emoji: 💬
-colorFrom: blue
-colorTo: red
-sdk: gradio
-sdk_version: 3.16.2
-app_file: app.py
-pinned: false
-duplicated_from: SmilingWolf/wd-v1-4-tags
----
-
-# Configuration
-
-`title`: _string_
-Display title for the Space
-
-`emoji`: _string_
-Space emoji (emoji-only character allowed)
-
-`colorFrom`: _string_
-Color for Thumbnail gradient (red, yellow, green, blue, indigo, purple, pink, gray)
-
-`colorTo`: _string_
-Color for Thumbnail gradient (red, yellow, green, blue, indigo, purple, pink, gray)
-
-`sdk`: _string_
-Can be either `gradio`, `streamlit`, or `static`
-
-`sdk_version` : _string_
-Only applicable for `streamlit` SDK.
-See [doc](https://hf.co/docs/hub/spaces) for more info on supported versions.
-
-`app_file`: _string_
-Path to your main application file (which contains either `gradio` or `streamlit` Python code, or `static` html code).
-Path is relative to the root of the repository.
-
-`pinned`: _boolean_
-Whether the Space stays on top of your list.
diff --git a/spaces/tialenAdioni/chat-gpt-api/logs/Ford IDS Calibration Files v81 Free Download - Car-Auto-Repair.com[3] This is another blog post that provides similar information and links as the previous one but with a different layout and format..md b/spaces/tialenAdioni/chat-gpt-api/logs/Ford IDS Calibration Files v81 Free Download - Car-Auto-Repair.com[3] This is another blog post that provides similar information and links as the previous one but with a different layout and format..md
deleted file mode 100644
index 12e62cf54533714c4422544c72d4d5127e9f949e..0000000000000000000000000000000000000000
--- a/spaces/tialenAdioni/chat-gpt-api/logs/Ford IDS Calibration Files v81 Free Download - Car-Auto-Repair.com[3] This is another blog post that provides similar information and links as the previous one but with a different layout and format..md
+++ /dev/null
@@ -1,62 +0,0 @@
-
-
Ford IDS Calibration Files v81: What You Need to Know
-
If you are looking for a diagnostic tool that can provide complete coverage of current and future Ford, Lincoln and Mercury vehicles in North America, you may want to check out the Ford Integrated Diagnostic System (IDS) [^1^] [^2^]. The IDS is a software application that replaces the Worldwide Diagnostic System (WDS) and allows dealership technicians to diagnose and service Ford, Lincoln, Mercury vehicles. As the new diagnostic tool, IDS will be required to perform diagnostics on future model year vehicles.
-
However, to use the IDS effectively, you will also need the calibration files that are compatible with your IDS version. Calibration files are binary files that contain the data and instructions for updating the software of a vehicle's electronic control unit (ECU). Calibration files can fix bugs, improve performance, or add new features to your vehicle's ECU.
In this article, we will show you how to download and install the Ford IDS Calibration Files v81, which are compatible with the Ford IDS v81 software. These files are available for free download from various sources on the internet [^1^] [^2^] [^3^], but you will need a VCM I or VCM II original or clone, or a VXDIAG VCX NANO for Ford/Mazda device to use them.
-
How to Download Ford IDS Calibration Files v81
-
There are two methods to download the Ford IDS Calibration Files v81. The first method is to use the official websites of Ford Motor Company or Professional Technician Society (PTS). The second method is to use third-party file hosting services that provide direct links to the calibration files.
-
Method 1: Using Official Websites
-
To use this method, you will need to know the calibration file name that you need for your vehicle. You can find this name by using the IDS software and connecting it to your vehicle's OBD port. The IDS will display the file name on the screen when it prompts you to update the ECU software.
-
How to install Ford Ids V86 and Calibration Files V81 Cd on Windows 10
-Ford Ids V86 and Calibration Files V81 Cd download link
-Ford Ids V86 and Calibration Files V81 Cd compatibility with different models
-Ford Ids V86 and Calibration Files V81 Cd troubleshooting guide
-Ford Ids V86 and Calibration Files V81 Cd review and feedback
-Ford Ids V86 and Calibration Files V81 Cd license activation
-Ford Ids V86 and Calibration Files V81 Cd update and patch
-Ford Ids V86 and Calibration Files V81 Cd features and benefits
-Ford Ids V86 and Calibration Files V81 Cd price and discount
-Ford Ids V86 and Calibration Files V81 Cd alternatives and comparisons
-Ford Ids V86 and Calibration Files V81 Cd manual and tutorial
-Ford Ids V86 and Calibration Files V81 Cd system requirements and specifications
-Ford Ids V86 and Calibration Files V81 Cd warranty and support
-Ford Ids V86 and Calibration Files V81 Cd error codes and solutions
-Ford Ids V86 and Calibration Files V81 Cd online training and certification
-Ford Ids V86 and Calibration Files V81 Cd software installation video
-Ford Ids V86 and Calibration Files V81 Cd hardware setup instructions
-Ford Ids V86 and Calibration Files V81 Cd frequently asked questions (FAQ)
-Ford Ids V86 and Calibration Files V81 Cd best practices and tips
-Ford Ids V86 and Calibration Files V81 Cd testimonials and case studies
-Ford Ids V86 and Calibration Files V81 Cd forum and community
-Ford Ids V86 and Calibration Files V81 Cd blog and news
-Ford Ids V86 and Calibration Files V81 Cd free trial and demo
-Ford Ids V86 and Calibration Files V81 Cd refund policy and guarantee
-Ford Ids V86 and Calibration Files V81 Cd affiliate program and commission
-How to use Ford Ids V86 and Calibration Files V81 Cd for diagnostics
-How to use Ford Ids V86 and Calibration Files V81 Cd for programming
-How to use Ford Ids V86 and Calibration Files V81 Cd for reprogramming
-How to use Ford Ids V86 and Calibration Files V81 Cd for coding
-How to use Ford Ids V86 and Calibration Files V81 Cd for calibration
-How to use Ford Ids V86 and Calibration Files V81 Cd for key programming
-How to use Ford Ids V86 and Calibration Files V81 Cd for injector coding
-How to use Ford Ids V86 and Calibration Files
-
Once you have the file name, you can follow these steps:
-
-
Visit http://www.fmcdealer.com >PTS (Professional Technician Society) website> Rotunda > Rotunda Diagnostic > IDS > Module Reprogramming. Enter the calibration file name. For example, if IDS states file abcdefg.bin, enter âabcdefgâ then click on the download button.
-
OR
-
Visit http://www.motorcraftservice.com >Diagnostic Tool Support >IDS >Module Reprogramming. Enter the calibration file name. For example, if IDS states file abcdefg.bin, enter âabcdefgâ then click on the download button.
-
Download the file to either a USB key/drive or CD ROM.
-
Copy the file from the removable media to C:\Program Files\Ford Motor Company\Calibration files directory.
-
If the IDS laptop has an internet connection, download the file(s) directly to C:\Program Files\Ford Motor Company\Calibration files directory.
-
-
Method 2: Using Third-Party File Hosting Services
-
To use this method, you do not need to know the calibration file name that you need for your vehicle. You can simply download all the calibration files that are available for the Ford IDS v81 software. However, this method may take longer and require more disk space than the first method.
-
To use this method, you can follow these steps:
-
-
Visit one of these links that provide direct downloads of the Ford IDS Calibration Files v81:
Step 1: Download and install Autodesk 3ds Max 2015
-
You can download the trial version of Autodesk 3ds Max 2015 from the official website or from other sources. Make sure you choose the right version for your system (32-bit or 64-bit). Follow the installation instructions and restart your computer when prompted.
-
Step 2: Download and run X-Force Keygen
-
X-Force Keygen is a program that can generate serial numbers and product keys for any Autodesk product. You can download it from various websites , but be careful of viruses and malware. After downloading, extract the file and run the keygen as administrator. You will see a window like this:
-
-
Select Autodesk 3ds Max 2015 from the drop-down menu and click on Generate. You will get a serial number and a product key. Copy them and keep them safe.
-
-
Step 3: Activate Autodesk 3ds Max 2015
-
Launch Autodesk 3ds Max 2015 and click on Activate. You will see a screen like this:
-
-
Paste the serial number and the product key that you got from X-Force Keygen and click on Next. You will see a message that your serial is wrong. Don't worry, just click on Close and then on Activate again. This time, you will see an option to request an activation code using an offline method. Choose that option and click on Next.
-
You will see a screen like this:
-
-
Copy the request code that appears on the screen and go back to X-Force Keygen. Paste the request code in the keygen and click on Generate. You will get an activation code. Copy it and go back to Autodesk 3ds Max 2015. Paste the activation code in the corresponding field and click on Next.
-
You will see a message that your product has been activated successfully. Congratulations! You have cracked Autodesk 3ds Max 2015 with X-Force Keygen.
-
Disclaimer
-
This article is for educational purposes only. We do not condone or encourage piracy or illegal use of software. Please support the developers by purchasing their products legally.
How to Use Autodesk 3ds Max 2015
-
Autodesk 3ds Max 2015 is a versatile and powerful 3D software that can help you create amazing scenes and characters for your projects. Whether you are a beginner or a professional, you can use Autodesk 3ds Max 2015 to model, animate, render, and simulate your 3D ideas. In this article, we will give you a brief overview of how to use Autodesk 3ds Max 2015.
-
The User Interface
-
When you launch Autodesk 3ds Max 2015, you will see a user interface like this:
-
-
The user interface consists of several elements that help you navigate and work with your 3D scene. Here are some of the main elements:
-
-
The Menu Bar contains various menus that give you access to different commands and options.
-
The Quick Access Toolbar contains icons for some of the most frequently used commands and tools.
-
The Ribbon contains tabs and panels that organize different tools and functions according to categories.
-
The Viewports are the windows that display your 3D scene from different perspectives. You can have up to four viewports at a time, and you can switch between different views using the icons at the bottom-left corner of each viewport.
-
The Command Panel is the vertical panel on the right side of the screen that contains various tabs and rollouts that let you create and modify objects, materials, modifiers, animation, and more.
-
The Scene Explorer is the panel on the left side of the screen that shows a hierarchical list of all the objects in your scene. You can use it to select, rename, hide, freeze, group, or layer your objects.
-
The Status Bar is the horizontal bar at the bottom of the screen that shows information about your scene, such as the number of objects, polygons, vertices, frames, etc. It also contains icons for toggling different modes and settings.
-
The Time Slider is the horizontal bar above the status bar that shows the frames of your animation. You can use it to play, pause, rewind, or fast-forward your animation.
-
The Animation Controls are the buttons below the time slider that let you control your animation playback and keyframes.
-
-
The Workflow
-
The workflow of Autodesk 3ds Max 2015 can be summarized in four main steps: modeling, texturing, lighting, and rendering. Here is a brief description of each step:
-
-
Modeling is the process of creating and shaping your 3D objects using various tools and techniques. You can start from scratch or use predefined shapes called primitives. You can also import models from other software or sources. You can use modifiers to add details and effects to your models. You can also use splines to create curves and surfaces.
-
Texturing is the process of applying colors, images, patterns, and effects to your 3D objects using materials and maps. You can use the Material Editor to create and edit materials and assign them to your objects. You can also use UVW mapping to control how your textures wrap around your objects.
-
Lighting is the process of adding lights to your scene to create realistic or artistic effects. You can use different types of lights such as standard lights, photometric lights, or daylight systems. You can also adjust various parameters such as color, intensity, shadow type, etc. You can also use global illumination techniques such as radiosity or mental ray to simulate natural lighting.
-
Rendering is the process of generating an image or a video from your 3D scene using a renderer. You can use different renderers such as scanline renderer, mental ray renderer, or Quicksilver renderer. You can also adjust various settings such as resolution, quality, anti-aliasing, etc. You can also use render elements to separate different aspects of your image such as diffuse color, specular color, reflection, etc.
-
-
Conclusion
ddb901b051
-
-
\ No newline at end of file
diff --git a/spaces/ticomspire/turkey-syria-earthquake-tweets/logs/Bloons TD 6 Mod APK Unlimited Money and More on 5Play.md b/spaces/ticomspire/turkey-syria-earthquake-tweets/logs/Bloons TD 6 Mod APK Unlimited Money and More on 5Play.md
deleted file mode 100644
index 1a3fbdbf23ca75a23564e7dff7884dfac24cc419..0000000000000000000000000000000000000000
--- a/spaces/ticomspire/turkey-syria-earthquake-tweets/logs/Bloons TD 6 Mod APK Unlimited Money and More on 5Play.md
+++ /dev/null
@@ -1,166 +0,0 @@
-
-
Bloons TD 6: A Fun and Challenging Tower Defense Game
-
If you are a fan of tower defense games, you might have heard of Bloons TD 6, one of the most popular and successful games in the genre. Bloons TD 6 is a 3D tower defense game that offers hours of fun and strategy for players of all ages and skill levels. In this article, we will tell you everything you need to know about Bloons TD 6, including what it is, why you should play it, and how you can download it.
Bloons TD 6 is a tower defense game developed and published by Ninja Kiwi, a New Zealand-based company that specializes in creating casual and mobile games. Bloons TD 6 is the sixth installment in the Bloons Tower Defense series, which started in 2007 as a flash game on the internet. Since then, the series has grown to become one of the most popular and acclaimed tower defense franchises in the world, with over a billion downloads across various platforms.
-
The history and features of the game
-
Bloons TD 6 was released on June 14, 2018 for iOS, Android, and Windows devices. It was later ported to macOS in October 2018, and to Steam in December 2018. The game has received regular updates since its launch, adding new content, features, and improvements. Some of the key features of Bloons TD 6 are:
-
-
A massive 3D tower defense game with over 60 handcrafted maps, each with different themes, layouts, and difficulties.
-
23 powerful monkey towers, each with three upgrade paths and unique activated abilities.
-
14 diverse heroes, each with their own personality, voiceover, signature upgrades, and special abilities.
-
4-player co-op mode, where you can team up with your friends or strangers online to pop every last bloon.
-
Regular events, such as boss battles, odysseys, quests, trophy store, and content browser.
-
Monkey knowledge system, where you can unlock over 100 meta-upgrades that add power and variety to your gameplay.
-
Powers and insta monkeys, where you can earn or buy special items that can help you in tricky situations.
-
-
The gameplay and modes of the game
-
The gameplay of Bloons TD 6 is similar to other tower defense games. You have to place your monkey towers along a path where colorful balloons (called bloons) will try to pass through. Your goal is to pop all the bloons before they reach the end of the path. If any bloon escapes, you will lose some lives. If you lose all your lives, you will lose the game.
-
bloons td 6 mod apk unlimited money 5play
-bloons td 6 co-op mode apk download 5play
-bloons td 6 boss events apk free 5play
-bloons td 6 odysseys apk latest version 5play
-bloons td 6 trophy store apk unlocked 5play
-bloons td 6 apk 5play android
-bloons td 6 apk 5play ios
-bloons td 6 apk 5play pc
-bloons td 6 apk 5play online
-bloons td 6 apk 5play offline
-bloons td 6 apk 5play hack
-bloons td 6 apk 5play cheats
-bloons td 6 apk 5play tips
-bloons td 6 apk 5play guide
-bloons td 6 apk 5play review
-bloons td 6 apk 5play gameplay
-bloons td 6 apk 5play trailer
-bloons td 6 apk 5play update
-bloons td 6 apk 5play download link
-bloons td 6 apk 5play install instructions
-bloons td 6 apk mod menu 5play
-bloons td 6 apk unlimited monkey money 5play
-bloons td 6 apk all towers unlocked 5play
-bloons td 6 apk all heroes unlocked 5play
-bloons td 6 apk all maps unlocked 5play
-bloons td 6 apk all skins unlocked 5play
-bloons td 6 apk all upgrades unlocked 5play
-bloons td 6 apk all achievements unlocked 5play
-bloons td 6 apk no ads 5play
-bloons td 6 apk no root required 5play
-bloons td 6 co-op mode how to play with friends on android ios pc using the same account on different devices with the same progress on the same server using different accounts on different devices with different progress on different servers using bluetooth using wifi using hotspot using vpn using emulator using controller using keyboard and mouse using touch screen using voice chat using text chat using discord using zoom using skype using whatsapp using telegram using facebook messenger using instagram dm using snapchat using tiktok using twitter using reddit using quora using youtube using twitch using steam using epic games using origin using uplay using gog using itch.io using gamejolt using kongregate using newgrounds using armor games using miniclip using coolmath games
-
The bloons come in different types, colors, sizes, and speeds. Some bloons are immune or resistant to certain attacks or effects. Some bloons contain other bloons inside them that will pop out when destroyed. Some bloons are stronger or faster than others. You have to use different strategies and combinations of towers and heroes to deal with different kinds of bloons.
-
The game offers various modes and difficulties for different levels of challenge and fun. Some of the modes are:
-
-
Standard mode: The basic mode where you can choose from easy, medium, or hard difficulty. You can also choose from different sub-modes, such as primary only, military only, magic only, or double HP MOABs.
-
Impoppable mode: A harder mode where the bloons are much tougher and the towers are more expensive. You also have fewer lives and no continues.
-
CHIMPS mode: The hardest mode where you have no monkey knowledge, no powers, no insta monkeys, no continues, no income, and no selling. You also have to deal with harder bloons and more rounds.
-
Sandbox mode: A free-play mode where you can experiment with different towers, heroes, bloons, and settings. You can also spawn any bloon you want and test your defenses.
-
Apopalypse mode: A mode where the bloons come in endless waves with increasing speed and difficulty. You have to survive as long as you can.
-
Deflation mode: A mode where you start with a fixed amount of money and no income. You have to pop all the bloons with the money you have.
-
Half cash mode: A mode where you earn half the money from popping bloons and selling towers. You also have half the starting cash.
-
Reverse mode: A mode where the bloons come from the opposite direction of the normal path.
-
-
The towers and heroes of the game
-
The towers are the main weapons you use to pop the bloons. There are 23 different towers in Bloons TD 6, each with its own strengths, weaknesses, and abilities. The towers are divided into four categories: primary, military, magic, and support. Each tower has three upgrade paths, each with five tiers of upgrades. You can only choose two paths for each tower, and only one path can reach the fifth tier. The fifth tier upgrades are very powerful and expensive, and you can only have one of each type per game.
-
The heroes are special units that have unique abilities and skills. There are 14 heroes in Bloons TD 6, each with their own personality and voiceover. The heroes level up automatically during the game, unlocking new abilities and buffs. You can also activate their special abilities manually for a burst of power. You can only have one hero per game, and you can choose which one to use before starting a game.
-
Some of the towers and heroes in Bloons TD 6 are:
-
-
Tower
Category
Description
-
Dart Monkey
Primary
A basic tower that throws darts at bloons. Can be upgraded to shoot faster, farther, or more accurately.
-
Boomerang Monkey
Primary
A tower that throws boomerangs that can hit multiple bloons in a curved path. Can be upgraded to throw faster, stronger, or more boomerangs.
-
Bomb Shooter
Military
A tower that shoots bombs that explode on impact, dealing splash damage to nearby bloons. Can be upgraded to shoot bigger, faster, or more bombs.
-
Sniper Monkey
Military
A tower that shoots powerful bullets that can pop any type of bloon and pierce through multiple layers. Can be upgraded to shoot faster, farther, or more accurately.
-
Ninja Monkey
Magic
A tower that throws shurikens that can pop multiple bloons in a straight line. Can be upgraded to throw faster, sharper, or more shurikens.
-
Super Monkey
Magic
A tower that shoots rapid-fire lasers that can pop any type of bloon. Can be upgraded to shoot plasma blasts, sun rays, or dark energy.
-
Banana Farm
Support
A tower that produces bananas that give you extra cash when collected. Can be upgraded to produce more bananas, more valuable bananas, or other benefits.
-
Spike Factory
Support
A tower that produces spikes that pop bloons when they run over them. Can be upgraded to produce more spikes, stronger spikes, or other effects.
-
Gwendolin
Hero
A pyromaniac monkey who loves fire. She can set bloons on fire with her flamethrower or her fireballs. Her abilities are Firestorm and Cocktail of Fire.
-
Obyn Greenfoot
Hero
A A forest guardian who can summon nature's wrath. He can shoot thorns that pierce through bloons or vines that trap them. His abilities are Brambles and Wall of Trees.
-
Adora
Hero
A divine monkey who can unleash holy power. She can shoot radiant bolts that deal massive damage to bloons or beams that pierce through them. Her abilities are Long Arm of Light and Blood Sacrifice.
-
-
Why should you play Bloons TD 6?
-
Bloons TD 6 is a game that has something for everyone. Whether you are looking for a casual and relaxing game, or a challenging and strategic game, you will find it in Bloons TD 6. Here are some of the reasons why you should play Bloons TD 6:
-
The benefits and challenges of the game
-
Bloons TD 6 is a game that can help you improve your cognitive skills, such as memory, attention, problem-solving, and creativity. The game requires you to plan ahead, think fast, and adapt to changing situations. The game also rewards you for experimenting with different strategies and combinations of towers and heroes. The game can also help you relieve stress, have fun, and express yourself.
-
Bloons TD 6 is also a game that can challenge you in many ways. The game has a variety of bloons, towers, heroes, maps, modes, and difficulties that can test your skills and knowledge. The game also has a competitive aspect, where you can compare your scores and achievements with other players around the world. The game also has a community aspect, where you can chat with other players, share your creations, and join clans.
-
The tips and tricks for the game
-
If you want to get better at Bloons TD 6, here are some tips and tricks that can help you:
-
-
Learn the strengths and weaknesses of each tower and hero, and use them accordingly.
-
Use the monkey knowledge system to unlock useful upgrades and bonuses for your towers and heroes.
-
Use the powers and insta monkeys wisely, as they can save you from losing or help you win faster.
-
Use the sandbox mode to test your strategies and learn how different bloons behave.
-
Watch videos or streams of other players to get ideas and inspiration for your own gameplay.
-
Join a clan or a co-op game to cooperate with other players and earn more rewards.
-
-
The reviews and ratings of the game
-
Bloons TD 6 is a game that has received positive reviews and ratings from critics and players alike. The game has an average rating of 4.7 out of 5 stars on the App Store, 4.8 out of 5 stars on Google Play, and 9 out of 10 on Steam. Some of the praises for the game are:
-
"Bloons TD 6 is an awesome tower defense game that will keep you hooked for hours on end. The graphics are stunning, the gameplay is smooth, and the content is rich. The game is well-balanced, challenging, and rewarding. It is definitely worth every penny."
-
"Bloons TD 6 is one of the best games I have ever played. It is so fun and addictive that I can't stop playing it. The game has so much variety and depth that it never gets boring or repetitive. The game is also very user-friendly and easy to learn. It is a must-have for any tower defense fan."
-
"Bloons TD 6 is a masterpiece of tower defense gaming. It is a perfect blend of strategy, action, humor, and creativity. The game has amazing graphics, sound effects, music, and voiceovers. The game is also very polished and updated regularly. It is a game that deserves all the praise it gets."
-
How can you download Bloons TD 6?
-
If you are interested in playing Bloons TD 6, you might be wondering how you can download it. There are different ways to download Bloons TD 6, depending on your device and preference. Here are some of the options:
-
The official sources and platforms of the game
-
The official sources and platforms of Bloons TD 6 are the ones that are authorized by Ninja Kiwi, the developer and publisher of the game. These sources and platforms are the safest and most reliable way to download Bloons TD 6, as they guarantee the quality and security of the game. They also support the developers and allow them to continue making more games and updates. The official sources and platforms of Bloons TD 6 are:
-
-
The App Store for iOS devices. You can download Bloons TD 6 for $4.99 from the App Store. You will need an iOS device with iOS 11.0 or later, and at least 1.3 GB of free space.
-
Google Play for Android devices. You can download Bloons TD 6 for $4.99 from Google Play. You will need an Android device with Android 5.0 or later, and at least 1.3 GB of free space.
-
Steam for Windows and macOS devices. You can download Bloons TD 6 for $9.99 from Steam. You will need a Windows or macOS device with a minimum of 4 GB of RAM, 2048 MB of VRAM, and 2048 MB of available space.
-
-
The alternative sources and platforms of the game
-
The alternative sources and platforms of Bloons TD 6 are the ones that are not authorized by Ninja Kiwi, but still offer the game for download. These sources and platforms are usually cheaper or free, but they also come with some risks and drawbacks. Some of the alternative sources and platforms of Bloons TD 6 are:
-
-
Third-party app stores for iOS and Android devices. These are app stores that are not affiliated with Apple or Google, but still offer apps for download. Some examples are TutuApp, AppValley, Aptoide, and APKPure. These app stores may offer Bloons TD 6 for free or at a lower price, but they may also contain malware, viruses, or spyware that can harm your device or steal your data.
-
Torrent sites for Windows and macOS devices. These are sites that allow users to share files through peer-to-peer networks. Some examples are The Pirate Bay, Kickass Torrents, RARBG, and 1337x. These sites may offer Bloons TD 6 for free or at a lower price, but they may also contain malware, viruses, or spyware that can harm your device or steal your data.
-
Modded versions of the game for any device. These are versions of the game that have been modified by hackers or modders to alter the gameplay or features of the game. Some examples are Bloons TD 6 Mod APK, Bloons TD 6 Hack, and Bloons TD 6 Unlimited Money. These versions may offer some advantages or cheats in the game, such as unlimited money, lives, or powers, but they may also cause glitches, crashes, or bans in the game.
-
-
The risks and precautions of downloading the game
-
If you decide to download Bloons TD 6 from an alternative source or platform, you should be aware of the risks and precautions involved. Some of the risks and precautions are:
-
-
You may violate the terms of service or the intellectual property rights of Ninja Kiwi, which could result in legal action or penalties.
-
You may damage your device or compromise your data by downloading malicious software or content.
-
You may lose your progress or account in the game by using an incompatible or unauthorized version of the game.
-
You may ruin your gaming experience or enjoyment by using cheats or hacks that make the game too easy or boring.
-
You may miss out on updates, features, events, or support from Ninja Kiwi by using an outdated or unofficial version of the game.
-
-
Therefore, you should always be careful and cautious when downloading Bloons TD 6 from an alternative source or platform. You should always check the reputation and reviews of the source or platform before downloading anything. You should also scan your device for any malware or viruses after downloading anything. You should also backup your data and progress in the game regularly in case anything goes wrong.
-
Conclusion
-
Bloons TD 6 is a fun and challenging tower defense game that you can play on various devices and platforms. The game has a lot of content, features, modes, and difficulties that can keep you entertained for hours. The game also has a lot of benefits and challenges that can improve your cognitive skills and challenge your creativity. The game can be downloaded from official sources and platforms that are safe and reliable, or from alternative sources and platforms that are cheaper or free but risky and unreliable.
-
A summary of the main points of the article
-
To summarize, here are the main points of this article:
-
-
Bloons TD 6 is a tower defense game that has a lot of history, features, gameplay, towers, and heroes.
-
Bloons TD 6 is a game that can help you improve your cognitive skills, challenge you in different ways, and give you a lot of fun and enjoyment.
-
Bloons TD 6 can be downloaded from official sources and platforms that are authorized by Ninja Kiwi, or from alternative sources and platforms that are not authorized by Ninja Kiwi.
-
Bloons TD 6 is a game that is worth playing and downloading, but you should be careful and cautious when choosing where to download it from.
-
-
A call to action for the readers
-
If you are interested in playing Bloons TD 6, you can download it from the links below. You can also visit the official website of Ninja Kiwi to learn more about the game and the developer. You can also join the Bloons TD 6 community on social media, forums, or Discord to chat with other players, share your creations, and get tips and support. We hope you enjoy playing Bloons TD 6 and popping some bloons!
Here are some of the frequently asked questions about Bloons TD 6:
-
Q: Is Bloons TD 6 free?
-
A: No, Bloons TD 6 is not free. You have to pay a one-time fee to download the game from the official sources and platforms. However, the game does not have any in-app purchases or subscriptions, so you can enjoy the game without spending any more money.
-
Q: Is Bloons TD 6 online or offline?
-
A: Bloons TD 6 can be played both online and offline. You can play the game offline without an internet connection, but you will not be able to access some features, such as co-op mode, events, quests, trophy store, content browser, or cloud save. You can play the game online with an internet connection, but you will need to create an account with Ninja Kiwi to access some features, such as co-op mode, events, quests, trophy store, content browser, or cloud save.
-
Q: Is Bloons TD 6 cross-platform?
-
A: Yes, Bloons TD 6 is cross-platform. You can play the game on different devices and platforms with the same account and progress. However, you will need to link your account with Ninja Kiwi to do so. You can also play co-op mode with other players on different devices and platforms.
-
Q: Is Bloons TD 6 multiplayer?
-
A: Yes, Bloons TD 6 is multiplayer. You can play co-op mode with up to three other players online. You can either join a public lobby or create a private lobby with your friends or clan members. You can also chat with other players in co-op mode.
-
Q: Is Bloons TD 6 kid-friendly?
-
A: Yes, Bloons TD 6 is kid-friendly. The game does not have any violence, gore, profanity, or inappropriate content. The game is suitable for players of all ages and skill levels. The game also has a parental control option that allows you to restrict some features of the game for younger players.
197e85843d
-
-
\ No newline at end of file
diff --git a/spaces/ticomspire/turkey-syria-earthquake-tweets/logs/Download Lifting Hero Hile APK Mod for Android and Enjoy the Ultimate Casual Game.md b/spaces/ticomspire/turkey-syria-earthquake-tweets/logs/Download Lifting Hero Hile APK Mod for Android and Enjoy the Ultimate Casual Game.md
deleted file mode 100644
index 470af3e722bfb7e8ca6657b7064d31485a08da7f..0000000000000000000000000000000000000000
--- a/spaces/ticomspire/turkey-syria-earthquake-tweets/logs/Download Lifting Hero Hile APK Mod for Android and Enjoy the Ultimate Casual Game.md
+++ /dev/null
@@ -1,94 +0,0 @@
-
-
Lifting Hero Hile APK: How to Become a Giant by Lifting Weights
-
Do you want to become a giant by lifting weights? Do you want to challenge yourself and compete with other players online? Do you want to have unlimited money and autoclick features in your game? If you answered yes to any of these questions, then you should try Lifting Hero Hile APK, a fun and addictive casual game that will make you feel like a hero.
Lifting Hero is a game developed by Rollic Games, a popular publisher of casual games for Android devices. The game has over 10 million downloads on Google Play Store and has received positive reviews from players. The game is simple but addictive: you have to lift different objects with your fingers and sell the muscle you gained. You can use the money to buy new objects and lift more weights. The more you lift, the bigger you become. Can you reach the biggest objects in the game?
-
How to play Lifting Hero Hile APK
-
The gameplay of Lifting Hero is easy to understand but hard to master. Here are some tips on how to play the game:
-
Start with small weights
-
When you start the game, you will have a small weight in front of you. You have to tap on it repeatedly to lift it. The faster you tap, the faster you lift. You will see a bar on the top of the screen that shows your progress. When the bar is full, you have lifted the weight successfully.
-
Sell the muscle you gained
-
After lifting a weight, you will see how much muscle you gained from it. You can sell this muscle for money by tapping on the sell button. The more muscle you have, the more money you get. You can use this money to buy new objects and lift more weights.
-
lifting hero hile apk download
-lifting hero hile apk mod
-lifting hero hile apk 2023
-lifting hero hile apk unlimited money
-lifting hero hile apk latest version
-lifting hero hile apk free
-lifting hero hile apk android
-lifting hero hile apk hack
-lifting hero hile apk indir
-lifting hero hile apk update
-lifting hero hile apk full
-lifting hero hile apk no ads
-lifting hero hile apk online
-lifting hero hile apk offline
-lifting hero hile apk cheats
-lifting hero hile apk gameplay
-lifting hero hile apk review
-lifting hero hile apk tips
-lifting hero hile apk tricks
-lifting hero hile apk guide
-lifting hero hile apk tutorial
-lifting hero hile apk walkthrough
-lifting hero hile apk features
-lifting hero hile apk benefits
-lifting hero hile apk pros and cons
-lifting hero hile apk requirements
-lifting hero hile apk size
-lifting hero hile apk install
-lifting hero hile apk uninstall
-lifting hero hile apk how to play
-lifting hero hile apk how to win
-lifting hero hile apk how to get money
-lifting hero hile apk how to unlock levels
-lifting hero hile apk how to use autoclicker
-lifting hero hile apk how to get points
-lifting hero hile apk how to get rewards
-lifting hero hile apk how to get achievements
-lifting hero hile apk how to get gems
-lifting hero hile apk how to get coins
-lifting hero hile apk how to get skins
-lifting hero hile apk how to get items
-lifting hero hile apk how to get power-ups
-lifting hero hile apk how to get bonuses
-lifting hero hile apk how to get stars
-lifting hero hile apk how to get trophies
-lifting hero hile apk comparison with other games
-lifting hero hile apk alternatives and similar games
-lifting hero hile apk best strategies and tactics
-lifting hero hile apk fun and addictive game
-
Buy new objects and lift more weights
-
You can buy new objects from the shop by tapping on the shop button. There are different categories of objects, such as animals, vehicles, buildings, planets, etc. Each category has different prices and weights. You can buy any object that you can afford and that is heavier than your previous one. The heavier the object, the more muscle and money you get from lifting it.
-
Click click click and lift faster, develop your muscles
-
The key to becoming a giant in this game is to lift faster and develop your muscles. You can do this by tapping faster on the screen and using autoclick features. Autoclick is a feature that allows you to tap automatically without touching the screen. You can activate this feature by tapping on the autoclick button on the bottom right corner of the screen. This will make your lifting easier and faster.
-
Reach the biggest objects
-
The ultimate goal of this game is to reach the biggest objects in the game, such as stars, galaxies, black holes, etc. These objects are very expensive and very heavy, so you need to have a lot of money and muscle to buy and lift them. However, once you do, you will feel like a hero and a giant.
-
Features of Lifting Hero Hile APK
-
Lifting Hero is a fun and addictive game, but it can be even more enjoyable with Lifting Hero Hile APK. This is a modified version of the game that gives you some extra features and advantages. Here are some of the features of Lifting Hero Hile APK:
-
Unlimited money and autoclick
-
With Lifting Hero Hile APK, you don't have to worry about running out of money or tapping too much. You will have unlimited money and autoclick in your game. This means that you can buy any object you want and lift it with ease. You can also upgrade your autoclick speed and duration to make your lifting faster and longer.
-
Colorful graphics and sound effects
-
Lifting Hero Hile APK has colorful graphics and sound effects that make the game more appealing and fun. You will see different objects with different shapes, colors, and sizes. You will also hear different sounds when you lift them, such as animal noises, car horns, explosions, etc. These graphics and sounds will make you feel more immersed in the game.
-
Single-player and multiplayer modes
-
Lifting Hero Hile APK has two modes of play: single-player and multiplayer. In single-player mode, you can play the game by yourself and try to reach the biggest objects in the game. In multiplayer mode, you can play the game with other players online and compete with them. You can see their progress on the leaderboard and chat with them. You can also challenge them to duels and see who can lift more weights.
-
Customizable background and themes
-
Lifting Hero Hile APK allows you to customize your background and themes in the game. You can choose from different backgrounds, such as city, forest, space, etc. You can also choose from different themes, such as Halloween, Christmas, Valentine's Day, etc. These backgrounds and themes will make your game more diverse and interesting.
-
Achievements and rewards
-
Lifting Hero Hile APK has many achievements and rewards that you can unlock by playing the game. You can earn achievements by lifting certain objects, reaching certain levels, playing for a certain time, etc. You can also earn rewards by watching ads, completing surveys, inviting friends, etc. These achievements and rewards will motivate you to play more and improve your skills.
-
How to download and install Lifting Hero Hile APK
-
If you want to download and install Lifting Hero Hile APK on your Android device, you need to follow these steps:
-
Download the APK file from a trusted source
-
The first step is to download the APK file from a trusted source. You can use the link below to download the latest version of Lifting Hero Hile APK for free. Make sure that you have enough storage space on your device before downloading the file.
-
Enable unknown sources on your device settings
-
The second step is to enable unknown sources on your device settings. This is necessary because Lifting Hero Hile APK is not available on Google Play Store and you need to install it manually. To enable unknown sources, go to your device settings > security > unknown sources > toggle on.
-
Locate and install the APK file on your device
-
The third step is to locate and install the APK file on your device. You can use a file manager app to find the downloaded file in your downloads folder or any other location where you saved it. Tap on the file and follow the instructions to install it on your device.
-
Launch the game and enjoy
-
The final step is to launch the game and enjoy it. You can find the game icon on your home screen or app drawer. Tap on it and start playing Lifting Hero Hile APK with unlimited money and autoclick features.
-
Conclusion
-
Lifting Hero Hile APK is a fun and addictive casual game that will make you feel like a hero by lifting weights. You can buy different objects, lift them faster, develop your muscles, compete with other players online, customize your background and themes, unlock achievements and rewards, and reach the biggest objects in the game. You can also enjoy unlimited money and autoclick features with Lifting Hero Hile APK. If you want to download and install Lifting Hero Hile APK on your Android device, just follow the steps above.
- FAQs Q: Is Lifting Hero Hile APK safe to use? A: Yes, Lifting Hero Hile APK is safe to use as long as you download it from a trusted source. However, you should always be careful when installing apps from unknown sources as they may contain viruses or malware. Q: Is Lifting Hero Hile APK compatible with my device? A: Lifting Hero A: Lifting Hero Hile APK is compatible with most Android devices that have Android 4.4 or higher. However, some devices may have compatibility issues due to different specifications or settings. You can check the compatibility of your device by visiting the official website of Rollic Games or contacting their customer support. Q: How can I update Lifting Hero Hile APK? A: You can update Lifting Hero Hile APK by downloading and installing the latest version of the APK file from the same source where you downloaded it before. You can also check for updates by visiting the official website of Rollic Games or following their social media accounts. Q: How can I uninstall Lifting Hero Hile APK? A: You can uninstall Lifting Hero Hile APK by following the same steps as you would uninstall any other app on your device. Go to your device settings > apps > Lifting Hero > uninstall. You can also delete the APK file from your device storage if you want to free up some space. Q: How can I contact the developer of Lifting Hero Hile APK? A: You can contact the developer of Lifting Hero Hile APK by visiting their official website or sending them an email at support@rollicgames.com. You can also follow them on Facebook, Twitter, Instagram, and YouTube for more information and updates. Q: How can I share my feedback or suggestions for Lifting Hero Hile APK? A: You can share your feedback or suggestions for Lifting Hero Hile APK by leaving a comment or rating on the source where you downloaded it. You can also write a review on Google Play Store or App Store if you downloaded the original version of the game. Your feedback and suggestions are valuable and appreciated by the developer. 401be4b1e0
-
-
\ No newline at end of file
diff --git a/spaces/timdettmers/guanaco-65b-4bit/app.py b/spaces/timdettmers/guanaco-65b-4bit/app.py
deleted file mode 100644
index 522e516efcd794030fa154b6fe938fb8bc9ef1b5..0000000000000000000000000000000000000000
--- a/spaces/timdettmers/guanaco-65b-4bit/app.py
+++ /dev/null
@@ -1,313 +0,0 @@
-# Copyright 2023 MosaicML spaces authors
-# SPDX-License-Identifier: Apache-2.0
-import datetime
-import os
-from threading import Event, Thread
-from uuid import uuid4
-from peft import PeftModel
-
-import gradio as gr
-import requests
-import torch
-import transformers
-from transformers import (
- AutoModelForCausalLM,
- AutoTokenizer,
- LlamaTokenizer,
- StoppingCriteria,
- StoppingCriteriaList,
- TextIteratorStreamer,
-)
-
-
-# model_name = "lmsys/vicuna-7b-delta-v1.1"
-#model_name = "timdettmers/guanaco-33b-merged"
-model_name = "facebook/opt-125m"
-tok = AutoTokenizer.from_pretrained('facebook/opt-125m')
-#tok = LlamaTokenizer.from_pretrained('huggyllama/llama-30b')
-
-max_new_tokens = 1536
-
-auth_token = os.getenv("HF_TOKEN", None)
-
-print(f"Starting to load the model {model_name} into memory")
-
-m = AutoModelForCausalLM.from_pretrained(
- model_name,
- #quantization_config=transformers.BitsAndBytesConfig(
- # load_in_4bit=True,
- # bnb_4bit_compute_dtype=torch.bfloat16,
- # bnb_4bit_use_double_quant=True,
- # bnb_4bit_quant_type='nf4' # {'fp4', 'nf4'}
- # ),
- torch_dtype=torch.bfloat16,
- device_map={"": 0}
-)
-#m = PeftModel.from_pretrained(m, 'timdettmers/guanaco-65b')
-m.eval()
-
-#tok.bos_token_id = 1
-
-stop_token_ids = [0]
-
-print(f"Successfully loaded the model {model_name} into memory")
-
-
-start_message = """A chat between a curious human and an artificial intelligence assistant. The assistant gives helpful, detailed, and polite answers to the user's questions."""
-
-
-
-class StopOnTokens(StoppingCriteria):
- def __call__(self, input_ids: torch.LongTensor, scores: torch.FloatTensor, **kwargs) -> bool:
- for stop_id in stop_token_ids:
- if input_ids[0][-1] == stop_id:
- return True
- return False
-
-
-def convert_history_to_text(history):
- text = start_message + "".join(
- [
- "".join(
- [
- f"### Human: {item[0]}\n",
- f"### Assistant: {item[1]}\n",
- ]
- )
- for item in history[:-1]
- ]
- )
- text += "".join(
- [
- "".join(
- [
- f"### Human: {history[-1][0]}\n",
- f"### Assistant: {history[-1][1]}\n",
- ]
- )
- ]
- )
- return text
-
-
-def log_conversation(conversation_id, history, messages, generate_kwargs):
- logging_url = os.getenv("LOGGING_URL", None)
- if logging_url is None:
- return
-
- timestamp = datetime.datetime.now().strftime("%Y-%m-%dT%H:%M:%S")
-
- data = {
- "conversation_id": conversation_id,
- "timestamp": timestamp,
- "history": history,
- "messages": messages,
- "generate_kwargs": generate_kwargs,
- }
-
- try:
- requests.post(logging_url, json=data)
- except requests.exceptions.RequestException as e:
- print(f"Error logging conversation: {e}")
-
-
-def user(message, history):
- # Append the user's message to the conversation history
- return "", history + [[message, ""]]
-
-
-def bot(history, temperature, top_p, top_k, repetition_penalty, conversation_id):
- print(f"history: {history}")
- # Initialize a StopOnTokens object
- stop = StopOnTokens()
-
- # Construct the input message string for the model by concatenating the current system message and conversation history
- messages = convert_history_to_text(history)
-
- # Tokenize the messages string
- input_ids = tok(messages, return_tensors="pt").input_ids
- input_ids = input_ids.to(m.device)
- streamer = TextIteratorStreamer(tok, timeout=10.0, skip_prompt=True, skip_special_tokens=True)
- generate_kwargs = dict(
- input_ids=input_ids,
- max_new_tokens=max_new_tokens,
- temperature=temperature,
- do_sample=temperature > 0.0,
- top_p=top_p,
- top_k=top_k,
- repetition_penalty=repetition_penalty,
- streamer=streamer,
- stopping_criteria=StoppingCriteriaList([stop]),
- )
-
- stream_complete = Event()
-
- def generate_and_signal_complete():
- m.generate(**generate_kwargs)
- stream_complete.set()
-
- def log_after_stream_complete():
- stream_complete.wait()
- log_conversation(
- conversation_id,
- history,
- messages,
- {
- "top_k": top_k,
- "top_p": top_p,
- "temperature": temperature,
- "repetition_penalty": repetition_penalty,
- },
- )
-
- t1 = Thread(target=generate_and_signal_complete)
- t1.start()
-
- t2 = Thread(target=log_after_stream_complete)
- t2.start()
-
- # Initialize an empty string to store the generated text
- partial_text = ""
- for new_text in streamer:
- partial_text += new_text
- history[-1][1] = partial_text
- yield history
-
-
-def get_uuid():
- return str(uuid4())
-
-
-with gr.Blocks(
- theme=gr.themes.Soft(),
- css=".disclaimer {font-variant-caps: all-small-caps;}",
-) as demo:
- conversation_id = gr.State(get_uuid)
- gr.Markdown(
- """
Guanaco-65b playground
-"""
- )
- chatbot = gr.Chatbot().style(height=500)
- with gr.Row():
- with gr.Column():
- msg = gr.Textbox(
- label="Chat Message Box",
- placeholder="Chat Message Box",
- show_label=False,
- ).style(container=False)
- with gr.Column():
- with gr.Row():
- submit = gr.Button("Submit")
- stop = gr.Button("Stop")
- clear = gr.Button("Clear")
- with gr.Row():
- with gr.Accordion("Advanced Options:", open=False):
- with gr.Row():
- with gr.Column():
- with gr.Row():
- temperature = gr.Slider(
- label="Temperature",
- value=0.7,
- minimum=0.0,
- maximum=1.0,
- step=0.1,
- interactive=True,
- info="Higher values produce more diverse outputs",
- )
- with gr.Column():
- with gr.Row():
- top_p = gr.Slider(
- label="Top-p (nucleus sampling)",
- value=0.9,
- minimum=0.0,
- maximum=1,
- step=0.01,
- interactive=True,
- info=(
- "Sample from the smallest possible set of tokens whose cumulative probability "
- "exceeds top_p. Set to 1 to disable and sample from all tokens."
- ),
- )
- with gr.Column():
- with gr.Row():
- top_k = gr.Slider(
- label="Top-k",
- value=0,
- minimum=0.0,
- maximum=200,
- step=1,
- interactive=True,
- info="Sample from a shortlist of top-k tokens — 0 to disable and sample from all tokens.",
- )
- with gr.Column():
- with gr.Row():
- repetition_penalty = gr.Slider(
- label="Repetition Penalty",
- value=1.1,
- minimum=1.0,
- maximum=2.0,
- step=0.1,
- interactive=True,
- info="Penalize repetition — 1.0 to disable.",
- )
- with gr.Row():
- gr.Markdown(
- "Disclaimer: The model can produce factually incorrect output, and should not be relied on to produce "
- "factually accurate information. The model was trained on various public datasets; while great efforts "
- "have been taken to clean the pretraining data, it is possible that this model could generate lewd, "
- "biased, or otherwise offensive outputs.",
- elem_classes=["disclaimer"],
- )
- with gr.Row():
- gr.Markdown(
- "[Privacy policy](https://gist.github.com/samhavens/c29c68cdcd420a9aa0202d0839876dac)",
- elem_classes=["disclaimer"],
- )
-
- submit_event = msg.submit(
- fn=user,
- inputs=[msg, chatbot],
- outputs=[msg, chatbot],
- queue=False,
- ).then(
- fn=bot,
- inputs=[
- chatbot,
- temperature,
- top_p,
- top_k,
- repetition_penalty,
- conversation_id,
- ],
- outputs=chatbot,
- queue=True,
- )
- submit_click_event = submit.click(
- fn=user,
- inputs=[msg, chatbot],
- outputs=[msg, chatbot],
- queue=False,
- ).then(
- fn=bot,
- inputs=[
- chatbot,
- temperature,
- top_p,
- top_k,
- repetition_penalty,
- conversation_id,
- ],
- outputs=chatbot,
- queue=True,
- )
- stop.click(
- fn=None,
- inputs=None,
- outputs=None,
- cancels=[submit_event, submit_click_event],
- queue=False,
- )
- clear.click(lambda: None, None, chatbot, queue=False)
-
-demo.queue(max_size=128, concurrency_count=2)
-demo.launch()
diff --git a/spaces/timpal0l/chat-ui/src/lib/utils/trimPrefix.ts b/spaces/timpal0l/chat-ui/src/lib/utils/trimPrefix.ts
deleted file mode 100644
index d006e66deca639f3f4d208e77a64ba368fab00ee..0000000000000000000000000000000000000000
--- a/spaces/timpal0l/chat-ui/src/lib/utils/trimPrefix.ts
+++ /dev/null
@@ -1,6 +0,0 @@
-export function trimPrefix(input: string, prefix: string) {
- if (input.startsWith(prefix)) {
- return input.slice(prefix.length);
- }
- return input;
-}
diff --git a/spaces/tjburns/ask_marcus_aurelius/.venv/lib/python3.10/site-packages/pip/_vendor/rich/panel.py b/spaces/tjburns/ask_marcus_aurelius/.venv/lib/python3.10/site-packages/pip/_vendor/rich/panel.py
deleted file mode 100644
index fc2807c3136558272e0d341f39cf55451b5ce452..0000000000000000000000000000000000000000
--- a/spaces/tjburns/ask_marcus_aurelius/.venv/lib/python3.10/site-packages/pip/_vendor/rich/panel.py
+++ /dev/null
@@ -1,251 +0,0 @@
-from typing import TYPE_CHECKING, Optional
-
-from .align import AlignMethod
-from .box import ROUNDED, Box
-from .jupyter import JupyterMixin
-from .measure import Measurement, measure_renderables
-from .padding import Padding, PaddingDimensions
-from .segment import Segment
-from .style import StyleType
-from .text import Text, TextType
-
-if TYPE_CHECKING:
- from .console import Console, ConsoleOptions, RenderableType, RenderResult
-
-
-class Panel(JupyterMixin):
- """A console renderable that draws a border around its contents.
-
- Example:
- >>> console.print(Panel("Hello, World!"))
-
- Args:
- renderable (RenderableType): A console renderable object.
- box (Box, optional): A Box instance that defines the look of the border (see :ref:`appendix_box`.
- Defaults to box.ROUNDED.
- safe_box (bool, optional): Disable box characters that don't display on windows legacy terminal with *raster* fonts. Defaults to True.
- expand (bool, optional): If True the panel will stretch to fill the console
- width, otherwise it will be sized to fit the contents. Defaults to True.
- style (str, optional): The style of the panel (border and contents). Defaults to "none".
- border_style (str, optional): The style of the border. Defaults to "none".
- width (Optional[int], optional): Optional width of panel. Defaults to None to auto-detect.
- height (Optional[int], optional): Optional height of panel. Defaults to None to auto-detect.
- padding (Optional[PaddingDimensions]): Optional padding around renderable. Defaults to 0.
- highlight (bool, optional): Enable automatic highlighting of panel title (if str). Defaults to False.
- """
-
- def __init__(
- self,
- renderable: "RenderableType",
- box: Box = ROUNDED,
- *,
- title: Optional[TextType] = None,
- title_align: AlignMethod = "center",
- subtitle: Optional[TextType] = None,
- subtitle_align: AlignMethod = "center",
- safe_box: Optional[bool] = None,
- expand: bool = True,
- style: StyleType = "none",
- border_style: StyleType = "none",
- width: Optional[int] = None,
- height: Optional[int] = None,
- padding: PaddingDimensions = (0, 1),
- highlight: bool = False,
- ) -> None:
- self.renderable = renderable
- self.box = box
- self.title = title
- self.title_align: AlignMethod = title_align
- self.subtitle = subtitle
- self.subtitle_align = subtitle_align
- self.safe_box = safe_box
- self.expand = expand
- self.style = style
- self.border_style = border_style
- self.width = width
- self.height = height
- self.padding = padding
- self.highlight = highlight
-
- @classmethod
- def fit(
- cls,
- renderable: "RenderableType",
- box: Box = ROUNDED,
- *,
- title: Optional[TextType] = None,
- title_align: AlignMethod = "center",
- subtitle: Optional[TextType] = None,
- subtitle_align: AlignMethod = "center",
- safe_box: Optional[bool] = None,
- style: StyleType = "none",
- border_style: StyleType = "none",
- width: Optional[int] = None,
- padding: PaddingDimensions = (0, 1),
- ) -> "Panel":
- """An alternative constructor that sets expand=False."""
- return cls(
- renderable,
- box,
- title=title,
- title_align=title_align,
- subtitle=subtitle,
- subtitle_align=subtitle_align,
- safe_box=safe_box,
- style=style,
- border_style=border_style,
- width=width,
- padding=padding,
- expand=False,
- )
-
- @property
- def _title(self) -> Optional[Text]:
- if self.title:
- title_text = (
- Text.from_markup(self.title)
- if isinstance(self.title, str)
- else self.title.copy()
- )
- title_text.end = ""
- title_text.plain = title_text.plain.replace("\n", " ")
- title_text.no_wrap = True
- title_text.expand_tabs()
- title_text.pad(1)
- return title_text
- return None
-
- @property
- def _subtitle(self) -> Optional[Text]:
- if self.subtitle:
- subtitle_text = (
- Text.from_markup(self.subtitle)
- if isinstance(self.subtitle, str)
- else self.subtitle.copy()
- )
- subtitle_text.end = ""
- subtitle_text.plain = subtitle_text.plain.replace("\n", " ")
- subtitle_text.no_wrap = True
- subtitle_text.expand_tabs()
- subtitle_text.pad(1)
- return subtitle_text
- return None
-
- def __rich_console__(
- self, console: "Console", options: "ConsoleOptions"
- ) -> "RenderResult":
- _padding = Padding.unpack(self.padding)
- renderable = (
- Padding(self.renderable, _padding) if any(_padding) else self.renderable
- )
- style = console.get_style(self.style)
- border_style = style + console.get_style(self.border_style)
- width = (
- options.max_width
- if self.width is None
- else min(options.max_width, self.width)
- )
-
- safe_box: bool = console.safe_box if self.safe_box is None else self.safe_box
- box = self.box.substitute(options, safe=safe_box)
-
- title_text = self._title
- if title_text is not None:
- title_text.style = border_style
-
- child_width = (
- width - 2
- if self.expand
- else console.measure(
- renderable, options=options.update_width(width - 2)
- ).maximum
- )
- child_height = self.height or options.height or None
- if child_height:
- child_height -= 2
- if title_text is not None:
- child_width = min(
- options.max_width - 2, max(child_width, title_text.cell_len + 2)
- )
-
- width = child_width + 2
- child_options = options.update(
- width=child_width, height=child_height, highlight=self.highlight
- )
- lines = console.render_lines(renderable, child_options, style=style)
-
- line_start = Segment(box.mid_left, border_style)
- line_end = Segment(f"{box.mid_right}", border_style)
- new_line = Segment.line()
- if title_text is None or width <= 4:
- yield Segment(box.get_top([width - 2]), border_style)
- else:
- title_text.align(self.title_align, width - 4, character=box.top)
- yield Segment(box.top_left + box.top, border_style)
- yield from console.render(title_text, child_options.update_width(width - 4))
- yield Segment(box.top + box.top_right, border_style)
-
- yield new_line
- for line in lines:
- yield line_start
- yield from line
- yield line_end
- yield new_line
-
- subtitle_text = self._subtitle
- if subtitle_text is not None:
- subtitle_text.style = border_style
-
- if subtitle_text is None or width <= 4:
- yield Segment(box.get_bottom([width - 2]), border_style)
- else:
- subtitle_text.align(self.subtitle_align, width - 4, character=box.bottom)
- yield Segment(box.bottom_left + box.bottom, border_style)
- yield from console.render(
- subtitle_text, child_options.update_width(width - 4)
- )
- yield Segment(box.bottom + box.bottom_right, border_style)
-
- yield new_line
-
- def __rich_measure__(
- self, console: "Console", options: "ConsoleOptions"
- ) -> "Measurement":
- _title = self._title
- _, right, _, left = Padding.unpack(self.padding)
- padding = left + right
- renderables = [self.renderable, _title] if _title else [self.renderable]
-
- if self.width is None:
- width = (
- measure_renderables(
- console,
- options.update_width(options.max_width - padding - 2),
- renderables,
- ).maximum
- + padding
- + 2
- )
- else:
- width = self.width
- return Measurement(width, width)
-
-
-if __name__ == "__main__": # pragma: no cover
- from .console import Console
-
- c = Console()
-
- from .box import DOUBLE, ROUNDED
- from .padding import Padding
-
- p = Panel(
- "Hello, World!",
- title="rich.Panel",
- style="white on blue",
- box=DOUBLE,
- padding=1,
- )
-
- c.print()
- c.print(p)
diff --git a/spaces/tmnam20/code-summarization/model.py b/spaces/tmnam20/code-summarization/model.py
deleted file mode 100644
index 1a0b0ef6e477e4555d27d47f44ef598b1dc995e1..0000000000000000000000000000000000000000
--- a/spaces/tmnam20/code-summarization/model.py
+++ /dev/null
@@ -1,269 +0,0 @@
-# Copyright (c) Microsoft Corporation.
-# Licensed under the MIT license.
-
-import torch
-import torch.nn as nn
-import torch
-from torch.autograd import Variable
-import copy
-
-
-class Seq2Seq(nn.Module):
- """
- Build Seqence-to-Sequence.
-
- Parameters:
-
- * `encoder`- encoder of seq2seq model. e.g. roberta
- * `decoder`- decoder of seq2seq model. e.g. transformer
- * `config`- configuration of encoder model.
- * `beam_size`- beam size for beam search.
- * `max_length`- max length of target for beam search.
- * `sos_id`- start of symbol ids in target for beam search.
- * `eos_id`- end of symbol ids in target for beam search.
- """
-
- def __init__(
- self,
- encoder,
- decoder,
- config,
- beam_size=None,
- max_length=None,
- sos_id=None,
- eos_id=None,
- ):
- super(Seq2Seq, self).__init__()
- self.encoder = encoder
- self.decoder = decoder
- self.config = config
- self.register_buffer("bias", torch.tril(torch.ones(2048, 2048)))
- self.dense = nn.Linear(config.hidden_size, config.hidden_size)
- self.lm_head = nn.Linear(config.hidden_size, config.vocab_size, bias=False)
- self.lsm = nn.LogSoftmax(dim=-1)
- self.tie_weights()
-
- self.beam_size = beam_size
- self.max_length = max_length
- self.sos_id = sos_id
- self.eos_id = eos_id
-
- def _tie_or_clone_weights(self, first_module, second_module):
- """Tie or clone module weights depending of weither we are using TorchScript or not"""
- if self.config.torchscript:
- first_module.weight = nn.Parameter(second_module.weight.clone())
- else:
- first_module.weight = second_module.weight
-
- def tie_weights(self):
- """Make sure we are sharing the input and output embeddings.
- Export to TorchScript can't handle parameter sharing so we are cloning them instead.
- """
- self._tie_or_clone_weights(
- self.lm_head, self.encoder.embeddings.word_embeddings
- )
-
- def forward(
- self,
- source_ids=None,
- source_mask=None,
- target_ids=None,
- target_mask=None,
- args=None,
- ):
- outputs = self.encoder(source_ids, attention_mask=source_mask)
- encoder_output = outputs[0].permute([1, 0, 2]).contiguous()
- if target_ids is not None:
- attn_mask = -1e4 * (
- 1 - self.bias[: target_ids.shape[1], : target_ids.shape[1]]
- )
- tgt_embeddings = (
- self.encoder.embeddings(target_ids).permute([1, 0, 2]).contiguous()
- )
- out = self.decoder(
- tgt_embeddings,
- encoder_output,
- tgt_mask=attn_mask,
- memory_key_padding_mask=(1 - source_mask).bool(),
- )
- hidden_states = torch.tanh(self.dense(out)).permute([1, 0, 2]).contiguous()
- lm_logits = self.lm_head(hidden_states)
- # Shift so that tokens < n predict n
- active_loss = target_mask[..., 1:].ne(0).view(-1) == 1
- shift_logits = lm_logits[..., :-1, :].contiguous()
- shift_labels = target_ids[..., 1:].contiguous()
- # Flatten the tokens
- loss_fct = nn.CrossEntropyLoss(ignore_index=-1)
- loss = loss_fct(
- shift_logits.view(-1, shift_logits.size(-1))[active_loss],
- shift_labels.view(-1)[active_loss],
- )
-
- outputs = loss, loss * active_loss.sum(), active_loss.sum()
- return outputs
- else:
- # Predict
- preds = []
- try:
- zero = torch.cuda.LongTensor(1).fill_(0)
- except Exception as e:
- zero = torch.LongTensor(1).fill_(0)
- for i in range(source_ids.shape[0]):
- context = encoder_output[:, i : i + 1]
- context_mask = source_mask[i : i + 1, :]
- beam = Beam(self.beam_size, self.sos_id, self.eos_id)
- input_ids = beam.getCurrentState()
- context = context.repeat(1, self.beam_size, 1)
- context_mask = context_mask.repeat(self.beam_size, 1)
- for _ in range(self.max_length):
- if beam.done():
- break
- attn_mask = -1e4 * (
- 1 - self.bias[: input_ids.shape[1], : input_ids.shape[1]]
- )
- tgt_embeddings = (
- self.encoder.embeddings(input_ids)
- .permute([1, 0, 2])
- .contiguous()
- )
- out = self.decoder(
- tgt_embeddings,
- context,
- tgt_mask=attn_mask,
- memory_key_padding_mask=(1 - context_mask).bool(),
- )
- out = torch.tanh(self.dense(out))
- hidden_states = out.permute([1, 0, 2]).contiguous()[:, -1, :]
- out = self.lsm(self.lm_head(hidden_states)).data
- beam.advance(out)
- input_ids.data.copy_(
- input_ids.data.index_select(0, beam.getCurrentOrigin())
- )
- input_ids = torch.cat((input_ids, beam.getCurrentState()), -1)
- hyp = beam.getHyp(beam.getFinal())
- pred = beam.buildTargetTokens(hyp)[: self.beam_size]
- pred = [
- torch.cat(
- [x.view(-1) for x in p] + [zero] * (self.max_length - len(p))
- ).view(1, -1)
- for p in pred
- ]
- preds.append(torch.cat(pred, 0).unsqueeze(0))
-
- preds = torch.cat(preds, 0)
- return preds
-
-
-class Beam(object):
- def __init__(self, size, sos, eos):
- self.size = size
- if torch.cuda.is_available():
- self.tt = torch.cuda
- else:
- self.tt = torch
- # The score for each translation on the beam.
- self.scores = self.tt.FloatTensor(size).zero_()
- # The backpointers at each time-step.
- self.prevKs = []
- # The outputs at each time-step.
- self.nextYs = [self.tt.LongTensor(size).fill_(0)]
- self.nextYs[0][0] = sos
- # Has EOS topped the beam yet.
- self._eos = eos
- self.eosTop = False
- # Time and k pair for finished.
- self.finished = []
-
- def getCurrentState(self):
- "Get the outputs for the current timestep."
- batch = self.tt.LongTensor(self.nextYs[-1]).view(-1, 1)
- return batch
-
- def getCurrentOrigin(self):
- "Get the backpointers for the current timestep."
- return self.prevKs[-1]
-
- def advance(self, wordLk):
- """
- Given prob over words for every last beam `wordLk` and attention
- `attnOut`: Compute and update the beam search.
-
- Parameters:
-
- * `wordLk`- probs of advancing from the last step (K x words)
- * `attnOut`- attention at the last step
-
- Returns: True if beam search is complete.
- """
- numWords = wordLk.size(1)
-
- # Sum the previous scores.
- if len(self.prevKs) > 0:
- beamLk = wordLk + self.scores.unsqueeze(1).expand_as(wordLk)
-
- # Don't let EOS have children.
- for i in range(self.nextYs[-1].size(0)):
- if self.nextYs[-1][i] == self._eos:
- beamLk[i] = -1e20
- else:
- beamLk = wordLk[0]
- flatBeamLk = beamLk.view(-1)
- bestScores, bestScoresId = flatBeamLk.topk(self.size, 0, True, True)
-
- self.scores = bestScores
-
- # bestScoresId is flattened beam x word array, so calculate which
- # word and beam each score came from
- prevK = bestScoresId // numWords
- self.prevKs.append(prevK)
- self.nextYs.append((bestScoresId - prevK * numWords))
-
- for i in range(self.nextYs[-1].size(0)):
- if self.nextYs[-1][i] == self._eos:
- s = self.scores[i]
- self.finished.append((s, len(self.nextYs) - 1, i))
-
- # End condition is when top-of-beam is EOS and no global score.
- if self.nextYs[-1][0] == self._eos:
- self.eosTop = True
-
- def done(self):
- return self.eosTop and len(self.finished) >= self.size
-
- def getFinal(self):
- if len(self.finished) == 0:
- self.finished.append((self.scores[0], len(self.nextYs) - 1, 0))
- self.finished.sort(key=lambda a: -a[0])
- if len(self.finished) != self.size:
- unfinished = []
- for i in range(self.nextYs[-1].size(0)):
- if self.nextYs[-1][i] != self._eos:
- s = self.scores[i]
- unfinished.append((s, len(self.nextYs) - 1, i))
- unfinished.sort(key=lambda a: -a[0])
- self.finished += unfinished[: self.size - len(self.finished)]
- return self.finished[: self.size]
-
- def getHyp(self, beam_res):
- """
- Walk back to construct the full hypothesis.
- """
- hyps = []
- for _, timestep, k in beam_res:
- hyp = []
- for j in range(len(self.prevKs[:timestep]) - 1, -1, -1):
- hyp.append(self.nextYs[j + 1][k])
- k = self.prevKs[j][k]
- hyps.append(hyp[::-1])
- return hyps
-
- def buildTargetTokens(self, preds):
- sentence = []
- for pred in preds:
- tokens = []
- for tok in pred:
- if tok == self._eos:
- break
- tokens.append(tok)
- sentence.append(tokens)
- return sentence
diff --git a/spaces/tomg-group-umd/pez-dispenser/open_clip/tokenizer.py b/spaces/tomg-group-umd/pez-dispenser/open_clip/tokenizer.py
deleted file mode 100644
index 01e9f9d25574cfe757bc43a0ff0d982f5a4efad3..0000000000000000000000000000000000000000
--- a/spaces/tomg-group-umd/pez-dispenser/open_clip/tokenizer.py
+++ /dev/null
@@ -1,201 +0,0 @@
-""" CLIP tokenizer
-
-Copied from https://github.com/openai/CLIP. Originally MIT License, Copyright (c) 2021 OpenAI.
-"""
-import gzip
-import html
-import os
-from functools import lru_cache
-from typing import Union, List
-
-import ftfy
-import regex as re
-import torch
-
-# https://stackoverflow.com/q/62691279
-import os
-os.environ["TOKENIZERS_PARALLELISM"] = "false"
-
-
-@lru_cache()
-def default_bpe():
- return os.path.join(os.path.dirname(os.path.abspath(__file__)), "bpe_simple_vocab_16e6.txt.gz")
-
-
-@lru_cache()
-def bytes_to_unicode():
- """
- Returns list of utf-8 byte and a corresponding list of unicode strings.
- The reversible bpe codes work on unicode strings.
- This means you need a large # of unicode characters in your vocab if you want to avoid UNKs.
- When you're at something like a 10B token dataset you end up needing around 5K for decent coverage.
- This is a significant percentage of your normal, say, 32K bpe vocab.
- To avoid that, we want lookup tables between utf-8 bytes and unicode strings.
- And avoids mapping to whitespace/control characters the bpe code barfs on.
- """
- bs = list(range(ord("!"), ord("~")+1))+list(range(ord("¡"), ord("¬")+1))+list(range(ord("®"), ord("ÿ")+1))
- cs = bs[:]
- n = 0
- for b in range(2**8):
- if b not in bs:
- bs.append(b)
- cs.append(2**8+n)
- n += 1
- cs = [chr(n) for n in cs]
- return dict(zip(bs, cs))
-
-
-def get_pairs(word):
- """Return set of symbol pairs in a word.
- Word is represented as tuple of symbols (symbols being variable-length strings).
- """
- pairs = set()
- prev_char = word[0]
- for char in word[1:]:
- pairs.add((prev_char, char))
- prev_char = char
- return pairs
-
-
-def basic_clean(text):
- text = ftfy.fix_text(text)
- text = html.unescape(html.unescape(text))
- return text.strip()
-
-
-def whitespace_clean(text):
- text = re.sub(r'\s+', ' ', text)
- text = text.strip()
- return text
-
-
-class SimpleTokenizer(object):
- def __init__(self, bpe_path: str = default_bpe(), special_tokens=None):
- self.byte_encoder = bytes_to_unicode()
- self.byte_decoder = {v: k for k, v in self.byte_encoder.items()}
- merges = gzip.open(bpe_path).read().decode("utf-8").split('\n')
- merges = merges[1:49152-256-2+1]
- merges = [tuple(merge.split()) for merge in merges]
- vocab = list(bytes_to_unicode().values())
- vocab = vocab + [v+'' for v in vocab]
- for merge in merges:
- vocab.append(''.join(merge))
- if not special_tokens:
- special_tokens = ['', '']
- else:
- special_tokens = ['', ''] + special_tokens
- vocab.extend(special_tokens)
- self.encoder = dict(zip(vocab, range(len(vocab))))
- self.decoder = {v: k for k, v in self.encoder.items()}
- self.bpe_ranks = dict(zip(merges, range(len(merges))))
- self.cache = {t:t for t in special_tokens}
- special = "|".join(special_tokens)
- self.pat = re.compile(special + r"""|'s|'t|'re|'ve|'m|'ll|'d|[\p{L}]+|[\p{N}]|[^\s\p{L}\p{N}]+""", re.IGNORECASE)
-
- self.vocab_size = len(self.encoder)
- self.all_special_ids = [self.encoder[t] for t in special_tokens]
-
- def bpe(self, token):
- if token in self.cache:
- return self.cache[token]
- word = tuple(token[:-1]) + ( token[-1] + '',)
- pairs = get_pairs(word)
-
- if not pairs:
- return token+''
-
- while True:
- bigram = min(pairs, key = lambda pair: self.bpe_ranks.get(pair, float('inf')))
- if bigram not in self.bpe_ranks:
- break
- first, second = bigram
- new_word = []
- i = 0
- while i < len(word):
- try:
- j = word.index(first, i)
- new_word.extend(word[i:j])
- i = j
- except:
- new_word.extend(word[i:])
- break
-
- if word[i] == first and i < len(word)-1 and word[i+1] == second:
- new_word.append(first+second)
- i += 2
- else:
- new_word.append(word[i])
- i += 1
- new_word = tuple(new_word)
- word = new_word
- if len(word) == 1:
- break
- else:
- pairs = get_pairs(word)
- word = ' '.join(word)
- self.cache[token] = word
- return word
-
- def encode(self, text):
- bpe_tokens = []
- text = whitespace_clean(basic_clean(text)).lower()
- for token in re.findall(self.pat, text):
- token = ''.join(self.byte_encoder[b] for b in token.encode('utf-8'))
- bpe_tokens.extend(self.encoder[bpe_token] for bpe_token in self.bpe(token).split(' '))
- return bpe_tokens
-
- def decode(self, tokens):
- text = ''.join([self.decoder[token] for token in tokens])
- text = bytearray([self.byte_decoder[c] for c in text]).decode('utf-8', errors="replace").replace('', ' ')
- return text
-
-
-_tokenizer = SimpleTokenizer()
-
-
-def tokenize(texts: Union[str, List[str]], context_length: int = 77) -> torch.LongTensor:
- """
- Returns the tokenized representation of given input string(s)
-
- Parameters
- ----------
- texts : Union[str, List[str]]
- An input string or a list of input strings to tokenize
- context_length : int
- The context length to use; all CLIP models use 77 as the context length
-
- Returns
- -------
- A two-dimensional tensor containing the resulting tokens, shape = [number of input strings, context_length]
- """
- if isinstance(texts, str):
- texts = [texts]
-
- sot_token = _tokenizer.encoder[""]
- eot_token = _tokenizer.encoder[""]
- all_tokens = [[sot_token] + _tokenizer.encode(text) + [eot_token] for text in texts]
- result = torch.zeros(len(all_tokens), context_length, dtype=torch.long)
-
- for i, tokens in enumerate(all_tokens):
- if len(tokens) > context_length:
- tokens = tokens[:context_length] # Truncate
- tokens[-1] = eot_token
- result[i, :len(tokens)] = torch.tensor(tokens)
-
- return result
-
-
-class HFTokenizer:
- "HuggingFace tokenizer wrapper"
- def __init__(self, tokenizer_name:str):
- from transformers import AutoTokenizer
- self.tokenizer = AutoTokenizer.from_pretrained(tokenizer_name)
-
- def __call__(self, texts:Union[str, List[str]], context_length:int=77) -> torch.Tensor:
- # same cleaning as for default tokenizer, except lowercasing
- # adding lower (for case-sensitive tokenizers) will make it more robust but less sensitive to nuance
- if isinstance(texts, str):
- texts = [texts]
- texts = [whitespace_clean(basic_clean(text)) for text in texts]
- input_ids = self.tokenizer(texts, return_tensors='pt', max_length=context_length, padding='max_length', truncation=True).input_ids
- return input_ids
diff --git a/spaces/tomofi/NDLOCR/src/ndl_layout/mmdetection/configs/deepfashion/README.md b/spaces/tomofi/NDLOCR/src/ndl_layout/mmdetection/configs/deepfashion/README.md
deleted file mode 100644
index 68e57e4fa5b29b66a8381a87949762d89d791a2e..0000000000000000000000000000000000000000
--- a/spaces/tomofi/NDLOCR/src/ndl_layout/mmdetection/configs/deepfashion/README.md
+++ /dev/null
@@ -1,56 +0,0 @@
-# DeepFashion
-
-
-
-[MMFashion](https://github.com/open-mmlab/mmfashion) develops "fashion parsing and segmentation" module
-based on the dataset
-[DeepFashion-Inshop](https://drive.google.com/drive/folders/0B7EVK8r0v71pVDZFQXRsMDZCX1E?usp=sharing).
-Its annotation follows COCO style.
-To use it, you need to first download the data. Note that we only use "img_highres" in this task.
-The file tree should be like this:
-
-```sh
-mmdetection
-├── mmdet
-├── tools
-├── configs
-├── data
-│ ├── DeepFashion
-│ │ ├── In-shop
-│ │ ├── Anno
-│ │ │ ├── segmentation
-│ │ │ | ├── DeepFashion_segmentation_train.json
-│ │ │ | ├── DeepFashion_segmentation_query.json
-│ │ │ | ├── DeepFashion_segmentation_gallery.json
-│ │ │ ├── list_bbox_inshop.txt
-│ │ │ ├── list_description_inshop.json
-│ │ │ ├── list_item_inshop.txt
-│ │ │ └── list_landmarks_inshop.txt
-│ │ ├── Eval
-│ │ │ └── list_eval_partition.txt
-│ │ ├── Img
-│ │ │ ├── img
-│ │ │ │ ├──XXX.jpg
-│ │ │ ├── img_highres
-│ │ │ └── ├──XXX.jpg
-
-```
-
-After that you can train the Mask RCNN r50 on DeepFashion-In-shop dataset by launching training with the `mask_rcnn_r50_fpn_1x.py` config
-or creating your own config file.
-
-```
-@inproceedings{liuLQWTcvpr16DeepFashion,
- author = {Liu, Ziwei and Luo, Ping and Qiu, Shi and Wang, Xiaogang and Tang, Xiaoou},
- title = {DeepFashion: Powering Robust Clothes Recognition and Retrieval with Rich Annotations},
- booktitle = {Proceedings of IEEE Conference on Computer Vision and Pattern Recognition (CVPR)},
- month = {June},
- year = {2016}
-}
-```
-
-## Model Zoo
-
-| Backbone | Model type | Dataset | bbox detection Average Precision | segmentation Average Precision | Config | Download (Google) |
-| :---------: | :----------: | :-----------------: | :--------------------------------: | :----------------------------: | :---------:| :-------------------------: |
-| ResNet50 | Mask RCNN | DeepFashion-In-shop | 0.599 | 0.584 |[config](https://github.com/open-mmlab/mmdetection/blob/master/configs/deepfashion/mask_rcnn_r50_fpn_15e_deepfashion.py)| [model](https://drive.google.com/open?id=1q6zF7J6Gb-FFgM87oIORIt6uBozaXp5r) | [log](https://drive.google.com/file/d/1qTK4Dr4FFLa9fkdI6UVko408gkrfTRLP/view?usp=sharing) |
diff --git a/spaces/tornadoslims/instruct-pix2pix/stable_diffusion/ldm/models/diffusion/dpm_solver/__init__.py b/spaces/tornadoslims/instruct-pix2pix/stable_diffusion/ldm/models/diffusion/dpm_solver/__init__.py
deleted file mode 100644
index 7427f38c07530afbab79154ea8aaf88c4bf70a08..0000000000000000000000000000000000000000
--- a/spaces/tornadoslims/instruct-pix2pix/stable_diffusion/ldm/models/diffusion/dpm_solver/__init__.py
+++ /dev/null
@@ -1 +0,0 @@
-from .sampler import DPMSolverSampler
\ No newline at end of file
diff --git a/spaces/trttung1610/musicgen/audiocraft/grids/compression/_explorers.py b/spaces/trttung1610/musicgen/audiocraft/grids/compression/_explorers.py
deleted file mode 100644
index eed30d5b8a1c14676503148ddf133c79ed2e33bf..0000000000000000000000000000000000000000
--- a/spaces/trttung1610/musicgen/audiocraft/grids/compression/_explorers.py
+++ /dev/null
@@ -1,55 +0,0 @@
-# Copyright (c) Meta Platforms, Inc. and affiliates.
-# All rights reserved.
-#
-# This source code is licensed under the license found in the
-# LICENSE file in the root directory of this source tree.
-
-import treetable as tt
-
-from .._base_explorers import BaseExplorer
-
-
-class CompressionExplorer(BaseExplorer):
- eval_metrics = ["sisnr", "visqol"]
-
- def stages(self):
- return ["train", "valid", "evaluate"]
-
- def get_grid_meta(self):
- """Returns the list of Meta information to display for each XP/job.
- """
- return [
- tt.leaf("index", align=">"),
- tt.leaf("name", wrap=140),
- tt.leaf("state"),
- tt.leaf("sig", align=">"),
- ]
-
- def get_grid_metrics(self):
- """Return the metrics that should be displayed in the tracking table.
- """
- return [
- tt.group(
- "train",
- [
- tt.leaf("epoch"),
- tt.leaf("bandwidth", ".2f"),
- tt.leaf("adv", ".4f"),
- tt.leaf("d_loss", ".4f"),
- ],
- align=">",
- ),
- tt.group(
- "valid",
- [
- tt.leaf("bandwidth", ".2f"),
- tt.leaf("adv", ".4f"),
- tt.leaf("msspec", ".4f"),
- tt.leaf("sisnr", ".2f"),
- ],
- align=">",
- ),
- tt.group(
- "evaluate", [tt.leaf(name, ".3f") for name in self.eval_metrics], align=">"
- ),
- ]
diff --git a/spaces/uSerNameDDHL/bingo/src/components/ui/icons.tsx b/spaces/uSerNameDDHL/bingo/src/components/ui/icons.tsx
deleted file mode 100644
index 742b489b50437c5b64c86082f2ebc712eeb6a2b0..0000000000000000000000000000000000000000
--- a/spaces/uSerNameDDHL/bingo/src/components/ui/icons.tsx
+++ /dev/null
@@ -1,504 +0,0 @@
-'use client'
-
-import * as React from 'react'
-
-import { cn } from '@/lib/utils'
-
-function IconNextChat({
- className,
- inverted,
- ...props
-}: React.ComponentProps<'svg'> & { inverted?: boolean }) {
- const id = React.useId()
-
- return (
-
- )
-}
-
-function IconOpenAI({ className, ...props }: React.ComponentProps<'svg'>) {
- return (
-
- )
-}
-
-function IconGitHub({ className, ...props }: React.ComponentProps<'svg'>) {
- return (
-
- )
-}
-
-function IconSeparator({ className, ...props }: React.ComponentProps<'svg'>) {
- return (
-
- )
-}
-
-function IconArrowDown({ className, ...props }: React.ComponentProps<'svg'>) {
- return (
-
- )
-}
-
-function IconArrowRight({ className, ...props }: React.ComponentProps<'svg'>) {
- return (
-
- )
-}
-
-function IconUser({ className, ...props }: React.ComponentProps<'svg'>) {
- return (
-
- )
-}
-
-function IconPlus({ className, ...props }: React.ComponentProps<'svg'>) {
- return (
-
- )
-}
-
-function IconArrowElbow({ className, ...props }: React.ComponentProps<'svg'>) {
- return (
-
- )
-}
-
-function IconSpinner({ className, ...props }: React.ComponentProps<'svg'>) {
- return (
-
- )
-}
-
-function IconMessage({ className, ...props }: React.ComponentProps<'svg'>) {
- return (
-
- )
-}
-
-function IconTrash({ className, ...props }: React.ComponentProps<'svg'>) {
- return (
-
- )
-}
-
-function IconMore({ className, ...props }: React.ComponentProps<'svg'>) {
- return (
-
- )
-}
-
-function IconRefresh({ className, ...props }: React.ComponentProps<'svg'>) {
- return (
-
- )
-}
-
-function IconStop({ className, ...props }: React.ComponentProps<'svg'>) {
- return (
-
- )
-}
-
-function IconSidebar({ className, ...props }: React.ComponentProps<'svg'>) {
- return (
-
- )
-}
-
-function IconMoon({ className, ...props }: React.ComponentProps<'svg'>) {
- return (
-
- )
-}
-
-function IconSun({ className, ...props }: React.ComponentProps<'svg'>) {
- return (
-
- )
-}
-
-function IconCopy({ className, ...props }: React.ComponentProps<'svg'>) {
- return (
-
- )
-}
-
-function IconCheck({ className, ...props }: React.ComponentProps<'svg'>) {
- return (
-
- )
-}
-
-function IconDownload({ className, ...props }: React.ComponentProps<'svg'>) {
- return (
-
- )
-}
-
-function IconClose({ className, ...props }: React.ComponentProps<'svg'>) {
- return (
-
- )
-}
-
-function IconEdit({ className, ...props }: React.ComponentProps<'svg'>) {
- return (
-
- )
-}
-
-function IconShare({ className, ...props }: React.ComponentProps<'svg'>) {
- return (
-
- )
-}
-
-function IconUsers({ className, ...props }: React.ComponentProps<'svg'>) {
- return (
-
- )
-}
-
-function IconExternalLink({
- className,
- ...props
-}: React.ComponentProps<'svg'>) {
- return (
-
- )
-}
-
-function IconChevronUpDown({
- className,
- ...props
-}: React.ComponentProps<'svg'>) {
- return (
-
- )
-}
-
-export {
- IconEdit,
- IconNextChat,
- IconOpenAI,
- IconGitHub,
- IconSeparator,
- IconArrowDown,
- IconArrowRight,
- IconUser,
- IconPlus,
- IconArrowElbow,
- IconSpinner,
- IconMessage,
- IconTrash,
- IconMore,
- IconRefresh,
- IconStop,
- IconSidebar,
- IconMoon,
- IconSun,
- IconCopy,
- IconCheck,
- IconDownload,
- IconClose,
- IconShare,
- IconUsers,
- IconExternalLink,
- IconChevronUpDown
-}
diff --git a/spaces/ulysses115/diffsvc_test/utils/text_norm.py b/spaces/ulysses115/diffsvc_test/utils/text_norm.py
deleted file mode 100644
index d0973cebc91e0525aeb6657e70012a1d37b5e6ff..0000000000000000000000000000000000000000
--- a/spaces/ulysses115/diffsvc_test/utils/text_norm.py
+++ /dev/null
@@ -1,790 +0,0 @@
-# coding=utf-8
-# Authors:
-# 2019.5 Zhiyang Zhou (https://github.com/Joee1995/chn_text_norm.git)
-# 2019.9 Jiayu DU
-#
-# requirements:
-# - python 3.X
-# notes: python 2.X WILL fail or produce misleading results
-
-import sys, os, argparse, codecs, string, re
-
-# ================================================================================ #
-# basic constant
-# ================================================================================ #
-CHINESE_DIGIS = u'零一二三四五六七八九'
-BIG_CHINESE_DIGIS_SIMPLIFIED = u'零壹贰叁肆伍陆柒捌玖'
-BIG_CHINESE_DIGIS_TRADITIONAL = u'零壹貳參肆伍陸柒捌玖'
-SMALLER_BIG_CHINESE_UNITS_SIMPLIFIED = u'十百千万'
-SMALLER_BIG_CHINESE_UNITS_TRADITIONAL = u'拾佰仟萬'
-LARGER_CHINESE_NUMERING_UNITS_SIMPLIFIED = u'亿兆京垓秭穰沟涧正载'
-LARGER_CHINESE_NUMERING_UNITS_TRADITIONAL = u'億兆京垓秭穰溝澗正載'
-SMALLER_CHINESE_NUMERING_UNITS_SIMPLIFIED = u'十百千万'
-SMALLER_CHINESE_NUMERING_UNITS_TRADITIONAL = u'拾佰仟萬'
-
-ZERO_ALT = u'〇'
-ONE_ALT = u'幺'
-TWO_ALTS = [u'两', u'兩']
-
-POSITIVE = [u'正', u'正']
-NEGATIVE = [u'负', u'負']
-POINT = [u'点', u'點']
-# PLUS = [u'加', u'加']
-# SIL = [u'杠', u'槓']
-
-# 中文数字系统类型
-NUMBERING_TYPES = ['low', 'mid', 'high']
-
-CURRENCY_NAMES = '(人民币|美元|日元|英镑|欧元|马克|法郎|加拿大元|澳元|港币|先令|芬兰马克|爱尔兰镑|' \
- '里拉|荷兰盾|埃斯库多|比塞塔|印尼盾|林吉特|新西兰元|比索|卢布|新加坡元|韩元|泰铢)'
-CURRENCY_UNITS = '((亿|千万|百万|万|千|百)|(亿|千万|百万|万|千|百|)元|(亿|千万|百万|万|千|百|)块|角|毛|分)'
-COM_QUANTIFIERS = '(匹|张|座|回|场|尾|条|个|首|阙|阵|网|炮|顶|丘|棵|只|支|袭|辆|挑|担|颗|壳|窠|曲|墙|群|腔|' \
- '砣|座|客|贯|扎|捆|刀|令|打|手|罗|坡|山|岭|江|溪|钟|队|单|双|对|出|口|头|脚|板|跳|枝|件|贴|' \
- '针|线|管|名|位|身|堂|课|本|页|家|户|层|丝|毫|厘|分|钱|两|斤|担|铢|石|钧|锱|忽|(千|毫|微)克|' \
- '毫|厘|分|寸|尺|丈|里|寻|常|铺|程|(千|分|厘|毫|微)米|撮|勺|合|升|斗|石|盘|碗|碟|叠|桶|笼|盆|' \
- '盒|杯|钟|斛|锅|簋|篮|盘|桶|罐|瓶|壶|卮|盏|箩|箱|煲|啖|袋|钵|年|月|日|季|刻|时|周|天|秒|分|旬|' \
- '纪|岁|世|更|夜|春|夏|秋|冬|代|伏|辈|丸|泡|粒|颗|幢|堆|条|根|支|道|面|片|张|颗|块)'
-
-# punctuation information are based on Zhon project (https://github.com/tsroten/zhon.git)
-CHINESE_PUNC_STOP = '!?。。'
-CHINESE_PUNC_NON_STOP = '"#$%&'()*+,-/:;<=>@[\]^_`{|}~⦅⦆「」、、〃《》「」『』【】〔〕〖〗〘〙〚〛〜〝〞〟〰〾〿–—‘’‛“”„‟…‧﹏'
-CHINESE_PUNC_LIST = CHINESE_PUNC_STOP + CHINESE_PUNC_NON_STOP
-
-
-# ================================================================================ #
-# basic class
-# ================================================================================ #
-class ChineseChar(object):
- """
- 中文字符
- 每个字符对应简体和繁体,
- e.g. 简体 = '负', 繁体 = '負'
- 转换时可转换为简体或繁体
- """
-
- def __init__(self, simplified, traditional):
- self.simplified = simplified
- self.traditional = traditional
- # self.__repr__ = self.__str__
-
- def __str__(self):
- return self.simplified or self.traditional or None
-
- def __repr__(self):
- return self.__str__()
-
-
-class ChineseNumberUnit(ChineseChar):
- """
- 中文数字/数位字符
- 每个字符除繁简体外还有一个额外的大写字符
- e.g. '陆' 和 '陸'
- """
-
- def __init__(self, power, simplified, traditional, big_s, big_t):
- super(ChineseNumberUnit, self).__init__(simplified, traditional)
- self.power = power
- self.big_s = big_s
- self.big_t = big_t
-
- def __str__(self):
- return '10^{}'.format(self.power)
-
- @classmethod
- def create(cls, index, value, numbering_type=NUMBERING_TYPES[1], small_unit=False):
-
- if small_unit:
- return ChineseNumberUnit(power=index + 1,
- simplified=value[0], traditional=value[1], big_s=value[1], big_t=value[1])
- elif numbering_type == NUMBERING_TYPES[0]:
- return ChineseNumberUnit(power=index + 8,
- simplified=value[0], traditional=value[1], big_s=value[0], big_t=value[1])
- elif numbering_type == NUMBERING_TYPES[1]:
- return ChineseNumberUnit(power=(index + 2) * 4,
- simplified=value[0], traditional=value[1], big_s=value[0], big_t=value[1])
- elif numbering_type == NUMBERING_TYPES[2]:
- return ChineseNumberUnit(power=pow(2, index + 3),
- simplified=value[0], traditional=value[1], big_s=value[0], big_t=value[1])
- else:
- raise ValueError(
- 'Counting type should be in {0} ({1} provided).'.format(NUMBERING_TYPES, numbering_type))
-
-
-class ChineseNumberDigit(ChineseChar):
- """
- 中文数字字符
- """
-
- def __init__(self, value, simplified, traditional, big_s, big_t, alt_s=None, alt_t=None):
- super(ChineseNumberDigit, self).__init__(simplified, traditional)
- self.value = value
- self.big_s = big_s
- self.big_t = big_t
- self.alt_s = alt_s
- self.alt_t = alt_t
-
- def __str__(self):
- return str(self.value)
-
- @classmethod
- def create(cls, i, v):
- return ChineseNumberDigit(i, v[0], v[1], v[2], v[3])
-
-
-class ChineseMath(ChineseChar):
- """
- 中文数位字符
- """
-
- def __init__(self, simplified, traditional, symbol, expression=None):
- super(ChineseMath, self).__init__(simplified, traditional)
- self.symbol = symbol
- self.expression = expression
- self.big_s = simplified
- self.big_t = traditional
-
-
-CC, CNU, CND, CM = ChineseChar, ChineseNumberUnit, ChineseNumberDigit, ChineseMath
-
-
-class NumberSystem(object):
- """
- 中文数字系统
- """
- pass
-
-
-class MathSymbol(object):
- """
- 用于中文数字系统的数学符号 (繁/简体), e.g.
- positive = ['正', '正']
- negative = ['负', '負']
- point = ['点', '點']
- """
-
- def __init__(self, positive, negative, point):
- self.positive = positive
- self.negative = negative
- self.point = point
-
- def __iter__(self):
- for v in self.__dict__.values():
- yield v
-
-
-# class OtherSymbol(object):
-# """
-# 其他符号
-# """
-#
-# def __init__(self, sil):
-# self.sil = sil
-#
-# def __iter__(self):
-# for v in self.__dict__.values():
-# yield v
-
-
-# ================================================================================ #
-# basic utils
-# ================================================================================ #
-def create_system(numbering_type=NUMBERING_TYPES[1]):
- """
- 根据数字系统类型返回创建相应的数字系统,默认为 mid
- NUMBERING_TYPES = ['low', 'mid', 'high']: 中文数字系统类型
- low: '兆' = '亿' * '十' = $10^{9}$, '京' = '兆' * '十', etc.
- mid: '兆' = '亿' * '万' = $10^{12}$, '京' = '兆' * '万', etc.
- high: '兆' = '亿' * '亿' = $10^{16}$, '京' = '兆' * '兆', etc.
- 返回对应的数字系统
- """
-
- # chinese number units of '亿' and larger
- all_larger_units = zip(
- LARGER_CHINESE_NUMERING_UNITS_SIMPLIFIED, LARGER_CHINESE_NUMERING_UNITS_TRADITIONAL)
- larger_units = [CNU.create(i, v, numbering_type, False)
- for i, v in enumerate(all_larger_units)]
- # chinese number units of '十, 百, 千, 万'
- all_smaller_units = zip(
- SMALLER_CHINESE_NUMERING_UNITS_SIMPLIFIED, SMALLER_CHINESE_NUMERING_UNITS_TRADITIONAL)
- smaller_units = [CNU.create(i, v, small_unit=True)
- for i, v in enumerate(all_smaller_units)]
- # digis
- chinese_digis = zip(CHINESE_DIGIS, CHINESE_DIGIS,
- BIG_CHINESE_DIGIS_SIMPLIFIED, BIG_CHINESE_DIGIS_TRADITIONAL)
- digits = [CND.create(i, v) for i, v in enumerate(chinese_digis)]
- digits[0].alt_s, digits[0].alt_t = ZERO_ALT, ZERO_ALT
- digits[1].alt_s, digits[1].alt_t = ONE_ALT, ONE_ALT
- digits[2].alt_s, digits[2].alt_t = TWO_ALTS[0], TWO_ALTS[1]
-
- # symbols
- positive_cn = CM(POSITIVE[0], POSITIVE[1], '+', lambda x: x)
- negative_cn = CM(NEGATIVE[0], NEGATIVE[1], '-', lambda x: -x)
- point_cn = CM(POINT[0], POINT[1], '.', lambda x,
- y: float(str(x) + '.' + str(y)))
- # sil_cn = CM(SIL[0], SIL[1], '-', lambda x, y: float(str(x) + '-' + str(y)))
- system = NumberSystem()
- system.units = smaller_units + larger_units
- system.digits = digits
- system.math = MathSymbol(positive_cn, negative_cn, point_cn)
- # system.symbols = OtherSymbol(sil_cn)
- return system
-
-
-def chn2num(chinese_string, numbering_type=NUMBERING_TYPES[1]):
- def get_symbol(char, system):
- for u in system.units:
- if char in [u.traditional, u.simplified, u.big_s, u.big_t]:
- return u
- for d in system.digits:
- if char in [d.traditional, d.simplified, d.big_s, d.big_t, d.alt_s, d.alt_t]:
- return d
- for m in system.math:
- if char in [m.traditional, m.simplified]:
- return m
-
- def string2symbols(chinese_string, system):
- int_string, dec_string = chinese_string, ''
- for p in [system.math.point.simplified, system.math.point.traditional]:
- if p in chinese_string:
- int_string, dec_string = chinese_string.split(p)
- break
- return [get_symbol(c, system) for c in int_string], \
- [get_symbol(c, system) for c in dec_string]
-
- def correct_symbols(integer_symbols, system):
- """
- 一百八 to 一百八十
- 一亿一千三百万 to 一亿 一千万 三百万
- """
-
- if integer_symbols and isinstance(integer_symbols[0], CNU):
- if integer_symbols[0].power == 1:
- integer_symbols = [system.digits[1]] + integer_symbols
-
- if len(integer_symbols) > 1:
- if isinstance(integer_symbols[-1], CND) and isinstance(integer_symbols[-2], CNU):
- integer_symbols.append(
- CNU(integer_symbols[-2].power - 1, None, None, None, None))
-
- result = []
- unit_count = 0
- for s in integer_symbols:
- if isinstance(s, CND):
- result.append(s)
- unit_count = 0
- elif isinstance(s, CNU):
- current_unit = CNU(s.power, None, None, None, None)
- unit_count += 1
-
- if unit_count == 1:
- result.append(current_unit)
- elif unit_count > 1:
- for i in range(len(result)):
- if isinstance(result[-i - 1], CNU) and result[-i - 1].power < current_unit.power:
- result[-i - 1] = CNU(result[-i - 1].power +
- current_unit.power, None, None, None, None)
- return result
-
- def compute_value(integer_symbols):
- """
- Compute the value.
- When current unit is larger than previous unit, current unit * all previous units will be used as all previous units.
- e.g. '两千万' = 2000 * 10000 not 2000 + 10000
- """
- value = [0]
- last_power = 0
- for s in integer_symbols:
- if isinstance(s, CND):
- value[-1] = s.value
- elif isinstance(s, CNU):
- value[-1] *= pow(10, s.power)
- if s.power > last_power:
- value[:-1] = list(map(lambda v: v *
- pow(10, s.power), value[:-1]))
- last_power = s.power
- value.append(0)
- return sum(value)
-
- system = create_system(numbering_type)
- int_part, dec_part = string2symbols(chinese_string, system)
- int_part = correct_symbols(int_part, system)
- int_str = str(compute_value(int_part))
- dec_str = ''.join([str(d.value) for d in dec_part])
- if dec_part:
- return '{0}.{1}'.format(int_str, dec_str)
- else:
- return int_str
-
-
-def num2chn(number_string, numbering_type=NUMBERING_TYPES[1], big=False,
- traditional=False, alt_zero=False, alt_one=False, alt_two=True,
- use_zeros=True, use_units=True):
- def get_value(value_string, use_zeros=True):
-
- striped_string = value_string.lstrip('0')
-
- # record nothing if all zeros
- if not striped_string:
- return []
-
- # record one digits
- elif len(striped_string) == 1:
- if use_zeros and len(value_string) != len(striped_string):
- return [system.digits[0], system.digits[int(striped_string)]]
- else:
- return [system.digits[int(striped_string)]]
-
- # recursively record multiple digits
- else:
- result_unit = next(u for u in reversed(
- system.units) if u.power < len(striped_string))
- result_string = value_string[:-result_unit.power]
- return get_value(result_string) + [result_unit] + get_value(striped_string[-result_unit.power:])
-
- system = create_system(numbering_type)
-
- int_dec = number_string.split('.')
- if len(int_dec) == 1:
- int_string = int_dec[0]
- dec_string = ""
- elif len(int_dec) == 2:
- int_string = int_dec[0]
- dec_string = int_dec[1]
- else:
- raise ValueError(
- "invalid input num string with more than one dot: {}".format(number_string))
-
- if use_units and len(int_string) > 1:
- result_symbols = get_value(int_string)
- else:
- result_symbols = [system.digits[int(c)] for c in int_string]
- dec_symbols = [system.digits[int(c)] for c in dec_string]
- if dec_string:
- result_symbols += [system.math.point] + dec_symbols
-
- if alt_two:
- liang = CND(2, system.digits[2].alt_s, system.digits[2].alt_t,
- system.digits[2].big_s, system.digits[2].big_t)
- for i, v in enumerate(result_symbols):
- if isinstance(v, CND) and v.value == 2:
- next_symbol = result_symbols[i +
- 1] if i < len(result_symbols) - 1 else None
- previous_symbol = result_symbols[i - 1] if i > 0 else None
- if isinstance(next_symbol, CNU) and isinstance(previous_symbol, (CNU, type(None))):
- if next_symbol.power != 1 and ((previous_symbol is None) or (previous_symbol.power != 1)):
- result_symbols[i] = liang
-
- # if big is True, '两' will not be used and `alt_two` has no impact on output
- if big:
- attr_name = 'big_'
- if traditional:
- attr_name += 't'
- else:
- attr_name += 's'
- else:
- if traditional:
- attr_name = 'traditional'
- else:
- attr_name = 'simplified'
-
- result = ''.join([getattr(s, attr_name) for s in result_symbols])
-
- # if not use_zeros:
- # result = result.strip(getattr(system.digits[0], attr_name))
-
- if alt_zero:
- result = result.replace(
- getattr(system.digits[0], attr_name), system.digits[0].alt_s)
-
- if alt_one:
- result = result.replace(
- getattr(system.digits[1], attr_name), system.digits[1].alt_s)
-
- for i, p in enumerate(POINT):
- if result.startswith(p):
- return CHINESE_DIGIS[0] + result
-
- # ^10, 11, .., 19
- if len(result) >= 2 and result[1] in [SMALLER_CHINESE_NUMERING_UNITS_SIMPLIFIED[0],
- SMALLER_CHINESE_NUMERING_UNITS_TRADITIONAL[0]] and \
- result[0] in [CHINESE_DIGIS[1], BIG_CHINESE_DIGIS_SIMPLIFIED[1], BIG_CHINESE_DIGIS_TRADITIONAL[1]]:
- result = result[1:]
-
- return result
-
-
-# ================================================================================ #
-# different types of rewriters
-# ================================================================================ #
-class Cardinal:
- """
- CARDINAL类
- """
-
- def __init__(self, cardinal=None, chntext=None):
- self.cardinal = cardinal
- self.chntext = chntext
-
- def chntext2cardinal(self):
- return chn2num(self.chntext)
-
- def cardinal2chntext(self):
- return num2chn(self.cardinal)
-
-
-class Digit:
- """
- DIGIT类
- """
-
- def __init__(self, digit=None, chntext=None):
- self.digit = digit
- self.chntext = chntext
-
- # def chntext2digit(self):
- # return chn2num(self.chntext)
-
- def digit2chntext(self):
- return num2chn(self.digit, alt_two=False, use_units=False)
-
-
-class TelePhone:
- """
- TELEPHONE类
- """
-
- def __init__(self, telephone=None, raw_chntext=None, chntext=None):
- self.telephone = telephone
- self.raw_chntext = raw_chntext
- self.chntext = chntext
-
- # def chntext2telephone(self):
- # sil_parts = self.raw_chntext.split('')
- # self.telephone = '-'.join([
- # str(chn2num(p)) for p in sil_parts
- # ])
- # return self.telephone
-
- def telephone2chntext(self, fixed=False):
-
- if fixed:
- sil_parts = self.telephone.split('-')
- self.raw_chntext = ''.join([
- num2chn(part, alt_two=False, use_units=False) for part in sil_parts
- ])
- self.chntext = self.raw_chntext.replace('', '')
- else:
- sp_parts = self.telephone.strip('+').split()
- self.raw_chntext = ''.join([
- num2chn(part, alt_two=False, use_units=False) for part in sp_parts
- ])
- self.chntext = self.raw_chntext.replace('', '')
- return self.chntext
-
-
-class Fraction:
- """
- FRACTION类
- """
-
- def __init__(self, fraction=None, chntext=None):
- self.fraction = fraction
- self.chntext = chntext
-
- def chntext2fraction(self):
- denominator, numerator = self.chntext.split('分之')
- return chn2num(numerator) + '/' + chn2num(denominator)
-
- def fraction2chntext(self):
- numerator, denominator = self.fraction.split('/')
- return num2chn(denominator) + '分之' + num2chn(numerator)
-
-
-class Date:
- """
- DATE类
- """
-
- def __init__(self, date=None, chntext=None):
- self.date = date
- self.chntext = chntext
-
- # def chntext2date(self):
- # chntext = self.chntext
- # try:
- # year, other = chntext.strip().split('年', maxsplit=1)
- # year = Digit(chntext=year).digit2chntext() + '年'
- # except ValueError:
- # other = chntext
- # year = ''
- # if other:
- # try:
- # month, day = other.strip().split('月', maxsplit=1)
- # month = Cardinal(chntext=month).chntext2cardinal() + '月'
- # except ValueError:
- # day = chntext
- # month = ''
- # if day:
- # day = Cardinal(chntext=day[:-1]).chntext2cardinal() + day[-1]
- # else:
- # month = ''
- # day = ''
- # date = year + month + day
- # self.date = date
- # return self.date
-
- def date2chntext(self):
- date = self.date
- try:
- year, other = date.strip().split('年', 1)
- year = Digit(digit=year).digit2chntext() + '年'
- except ValueError:
- other = date
- year = ''
- if other:
- try:
- month, day = other.strip().split('月', 1)
- month = Cardinal(cardinal=month).cardinal2chntext() + '月'
- except ValueError:
- day = date
- month = ''
- if day:
- day = Cardinal(cardinal=day[:-1]).cardinal2chntext() + day[-1]
- else:
- month = ''
- day = ''
- chntext = year + month + day
- self.chntext = chntext
- return self.chntext
-
-
-class Money:
- """
- MONEY类
- """
-
- def __init__(self, money=None, chntext=None):
- self.money = money
- self.chntext = chntext
-
- # def chntext2money(self):
- # return self.money
-
- def money2chntext(self):
- money = self.money
- pattern = re.compile(r'(\d+(\.\d+)?)')
- matchers = pattern.findall(money)
- if matchers:
- for matcher in matchers:
- money = money.replace(matcher[0], Cardinal(cardinal=matcher[0]).cardinal2chntext())
- self.chntext = money
- return self.chntext
-
-
-class Percentage:
- """
- PERCENTAGE类
- """
-
- def __init__(self, percentage=None, chntext=None):
- self.percentage = percentage
- self.chntext = chntext
-
- def chntext2percentage(self):
- return chn2num(self.chntext.strip().strip('百分之')) + '%'
-
- def percentage2chntext(self):
- return '百分之' + num2chn(self.percentage.strip().strip('%'))
-
-
-# ================================================================================ #
-# NSW Normalizer
-# ================================================================================ #
-class NSWNormalizer:
- def __init__(self, raw_text):
- self.raw_text = '^' + raw_text + '$'
- self.norm_text = ''
-
- def _particular(self):
- text = self.norm_text
- pattern = re.compile(r"(([a-zA-Z]+)二([a-zA-Z]+))")
- matchers = pattern.findall(text)
- if matchers:
- # print('particular')
- for matcher in matchers:
- text = text.replace(matcher[0], matcher[1] + '2' + matcher[2], 1)
- self.norm_text = text
- return self.norm_text
-
- def normalize(self, remove_punc=True):
- text = self.raw_text
-
- # 规范化日期
- pattern = re.compile(r"\D+((([089]\d|(19|20)\d{2})年)?(\d{1,2}月(\d{1,2}[日号])?)?)")
- matchers = pattern.findall(text)
- if matchers:
- # print('date')
- for matcher in matchers:
- text = text.replace(matcher[0], Date(date=matcher[0]).date2chntext(), 1)
-
- # 规范化金钱
- pattern = re.compile(r"\D+((\d+(\.\d+)?)[多余几]?" + CURRENCY_UNITS + r"(\d" + CURRENCY_UNITS + r"?)?)")
- matchers = pattern.findall(text)
- if matchers:
- # print('money')
- for matcher in matchers:
- text = text.replace(matcher[0], Money(money=matcher[0]).money2chntext(), 1)
-
- # 规范化固话/手机号码
- # 手机
- # http://www.jihaoba.com/news/show/13680
- # 移动:139、138、137、136、135、134、159、158、157、150、151、152、188、187、182、183、184、178、198
- # 联通:130、131、132、156、155、186、185、176
- # 电信:133、153、189、180、181、177
- pattern = re.compile(r"\D((\+?86 ?)?1([38]\d|5[0-35-9]|7[678]|9[89])\d{8})\D")
- matchers = pattern.findall(text)
- if matchers:
- # print('telephone')
- for matcher in matchers:
- text = text.replace(matcher[0], TelePhone(telephone=matcher[0]).telephone2chntext(), 1)
- # 固话
- pattern = re.compile(r"\D((0(10|2[1-3]|[3-9]\d{2})-?)?[1-9]\d{6,7})\D")
- matchers = pattern.findall(text)
- if matchers:
- # print('fixed telephone')
- for matcher in matchers:
- text = text.replace(matcher[0], TelePhone(telephone=matcher[0]).telephone2chntext(fixed=True), 1)
-
- # 规范化分数
- pattern = re.compile(r"(\d+/\d+)")
- matchers = pattern.findall(text)
- if matchers:
- # print('fraction')
- for matcher in matchers:
- text = text.replace(matcher, Fraction(fraction=matcher).fraction2chntext(), 1)
-
- # 规范化百分数
- text = text.replace('%', '%')
- pattern = re.compile(r"(\d+(\.\d+)?%)")
- matchers = pattern.findall(text)
- if matchers:
- # print('percentage')
- for matcher in matchers:
- text = text.replace(matcher[0], Percentage(percentage=matcher[0]).percentage2chntext(), 1)
-
- # 规范化纯数+量词
- pattern = re.compile(r"(\d+(\.\d+)?)[多余几]?" + COM_QUANTIFIERS)
- matchers = pattern.findall(text)
- if matchers:
- # print('cardinal+quantifier')
- for matcher in matchers:
- text = text.replace(matcher[0], Cardinal(cardinal=matcher[0]).cardinal2chntext(), 1)
-
- # 规范化数字编号
- pattern = re.compile(r"(\d{4,32})")
- matchers = pattern.findall(text)
- if matchers:
- # print('digit')
- for matcher in matchers:
- text = text.replace(matcher, Digit(digit=matcher).digit2chntext(), 1)
-
- # 规范化纯数
- pattern = re.compile(r"(\d+(\.\d+)?)")
- matchers = pattern.findall(text)
- if matchers:
- # print('cardinal')
- for matcher in matchers:
- text = text.replace(matcher[0], Cardinal(cardinal=matcher[0]).cardinal2chntext(), 1)
-
- self.norm_text = text
- self._particular()
-
- text = self.norm_text.lstrip('^').rstrip('$')
- if remove_punc:
- # Punctuations removal
- old_chars = CHINESE_PUNC_LIST + string.punctuation # includes all CN and EN punctuations
- new_chars = ' ' * len(old_chars)
- del_chars = ''
- text = text.translate(str.maketrans(old_chars, new_chars, del_chars))
- return text
-
-
-def nsw_test_case(raw_text):
- print('I:' + raw_text)
- print('O:' + NSWNormalizer(raw_text).normalize())
- print('')
-
-
-def nsw_test():
- nsw_test_case('固话:0595-23865596或23880880。')
- nsw_test_case('固话:0595-23865596或23880880。')
- nsw_test_case('手机:+86 19859213959或15659451527。')
- nsw_test_case('分数:32477/76391。')
- nsw_test_case('百分数:80.03%。')
- nsw_test_case('编号:31520181154418。')
- nsw_test_case('纯数:2983.07克或12345.60米。')
- nsw_test_case('日期:1999年2月20日或09年3月15号。')
- nsw_test_case('金钱:12块5,34.5元,20.1万')
- nsw_test_case('特殊:O2O或B2C。')
- nsw_test_case('3456万吨')
- nsw_test_case('2938个')
- nsw_test_case('938')
- nsw_test_case('今天吃了115个小笼包231个馒头')
- nsw_test_case('有62%的概率')
-
-
-if __name__ == '__main__':
- # nsw_test()
-
- p = argparse.ArgumentParser()
- p.add_argument('ifile', help='input filename, assume utf-8 encoding')
- p.add_argument('ofile', help='output filename')
- p.add_argument('--to_upper', action='store_true', help='convert to upper case')
- p.add_argument('--to_lower', action='store_true', help='convert to lower case')
- p.add_argument('--has_key', action='store_true', help="input text has Kaldi's key as first field.")
- p.add_argument('--log_interval', type=int, default=10000, help='log interval in number of processed lines')
- args = p.parse_args()
-
- ifile = codecs.open(args.ifile, 'r', 'utf8')
- ofile = codecs.open(args.ofile, 'w+', 'utf8')
-
- n = 0
- for l in ifile:
- key = ''
- text = ''
- if args.has_key:
- cols = l.split(maxsplit=1)
- key = cols[0]
- if len(cols) == 2:
- text = cols[1]
- else:
- text = ''
- else:
- text = l
-
- # cases
- if args.to_upper and args.to_lower:
- sys.stderr.write('text norm: to_upper OR to_lower?')
- exit(1)
- if args.to_upper:
- text = text.upper()
- if args.to_lower:
- text = text.lower()
-
- # NSW(Non-Standard-Word) normalization
- text = NSWNormalizer(text).normalize()
-
- #
- if args.has_key:
- ofile.write(key + '\t' + text)
- else:
- ofile.write(text)
-
- n += 1
- if n % args.log_interval == 0:
- sys.stderr.write("text norm: {} lines done.\n".format(n))
-
- sys.stderr.write("text norm: {} lines done in total.\n".format(n))
-
- ifile.close()
- ofile.close()
diff --git a/spaces/unik-style/unik-ml/cache/local_cache.py b/spaces/unik-style/unik-ml/cache/local_cache.py
deleted file mode 100644
index 0f3fd9c89f1bdec92c52cbed3f8d11c878858867..0000000000000000000000000000000000000000
--- a/spaces/unik-style/unik-ml/cache/local_cache.py
+++ /dev/null
@@ -1,42 +0,0 @@
-from datetime import datetime, timedelta
-from functools import wraps
-from io import BytesIO
-
-from fastapi.responses import StreamingResponse
-
-CACHE_SIZE = 50
-
-_cache = {}
-_cache_time = {}
-
-
-def ttl_cache(key_name, media_type=None, ttl_secs=20):
- def decorator(func):
- @wraps(func)
- async def wrapper(*args, **kwargs):
- # Assuming the prompt is the key for caching, change as necessary
- key = kwargs.get(key_name)
- ttl = timedelta(seconds=ttl_secs)
- # Check cache
- if key in _cache:
- if datetime.now() - _cache_time[key] > ttl:
- # Cache has expired
- del _cache[key]
- del _cache_time[key]
- else:
- # if media_type == 'image/png':
- # return StreamingResponse(BytesIO(_cache[key]), media_type=media_type)
- # else:
- return StreamingResponse(BytesIO(_cache[key]), media_type="image/png")
-
- # Call the actual function if not in cache or expired
- response, image_data = await func(*args, **kwargs)
- # Cache the content of the response's body.
- _cache[key] = image_data
- _cache_time[key] = datetime.now()
-
- return response
-
- return wrapper
-
- return decorator
diff --git a/spaces/unstructuredio/chat-your-data-isw/query_data.py b/spaces/unstructuredio/chat-your-data-isw/query_data.py
deleted file mode 100644
index 33678f07ef276bc1a7acaf9bdd871e8974a70317..0000000000000000000000000000000000000000
--- a/spaces/unstructuredio/chat-your-data-isw/query_data.py
+++ /dev/null
@@ -1,34 +0,0 @@
-from langchain.prompts.prompt import PromptTemplate
-from langchain.llms import OpenAI
-from langchain.chains import ChatVectorDBChain
-
-_template = """Given the following conversation and a follow up question, rephrase the follow up question to be a standalone question.
-You can assume the question about the war in Ukraine.
-
-Chat History:
-{chat_history}
-Follow Up Input: {question}
-Standalone question:"""
-CONDENSE_QUESTION_PROMPT = PromptTemplate.from_template(_template)
-
-template = """You are an AI assistant for answering questions about the war in Ukraine.
-You are given the following extracted parts of a long document and a question. Provide a conversational answer.
-If you don't know the answer, just say "Hmm, I'm not sure." Don't try to make up an answer.
-If the question is not about the war in Ukraine, politely inform them that you are tuned to only answer questions about the war in Ukraine.
-Question: {question}
-=========
-{context}
-=========
-Answer in Markdown:"""
-QA_PROMPT = PromptTemplate(template=template, input_variables=["question", "context"])
-
-
-def get_chain(vectorstore):
- llm = OpenAI(temperature=0)
- qa_chain = ChatVectorDBChain.from_llm(
- llm,
- vectorstore,
- qa_prompt=QA_PROMPT,
- condense_question_prompt=CONDENSE_QUESTION_PROMPT,
- )
- return qa_chain
diff --git a/spaces/usbethFlerru/sovits-modelsV2/example/Akka Koothi Tamil Sex Stories In English Letters.md b/spaces/usbethFlerru/sovits-modelsV2/example/Akka Koothi Tamil Sex Stories In English Letters.md
deleted file mode 100644
index 7f397d82ebf33fa5733cd63b17cdf6e2e01be049..0000000000000000000000000000000000000000
--- a/spaces/usbethFlerru/sovits-modelsV2/example/Akka Koothi Tamil Sex Stories In English Letters.md
+++ /dev/null
@@ -1,6 +0,0 @@
-