diff --git a/spaces/101-5/gpt4free/g4f/.v1/gpt4free/README.md b/spaces/101-5/gpt4free/g4f/.v1/gpt4free/README.md
deleted file mode 100644
index 73e7fa09f1502c9a79f5324cabb51128cad13fbc..0000000000000000000000000000000000000000
--- a/spaces/101-5/gpt4free/g4f/.v1/gpt4free/README.md
+++ /dev/null
@@ -1,110 +0,0 @@
-# gpt4free package
-
-### What is it?
-
-gpt4free is a python package that provides some language model api's
-
-### Main Features
-
-- It's free to use
-- Easy access
-
-### Installation:
-
-```bash
-pip install gpt4free
-```
-
-#### Usage:
-
-```python
-import gpt4free
-from gpt4free import Provider, quora, forefront
-
-# usage You
-response = gpt4free.Completion.create(Provider.You, prompt='Write a poem on Lionel Messi')
-print(response)
-
-# usage Poe
-token = quora.Account.create(logging=False)
-response = gpt4free.Completion.create(Provider.Poe, prompt='Write a poem on Lionel Messi', token=token, model='ChatGPT')
-print(response)
-
-# usage forefront
-token = forefront.Account.create(logging=False)
-response = gpt4free.Completion.create(
- Provider.ForeFront, prompt='Write a poem on Lionel Messi', model='gpt-4', token=token
-)
-print(response)
-print(f'END')
-
-# usage theb
-response = gpt4free.Completion.create(Provider.Theb, prompt='Write a poem on Lionel Messi')
-print(response)
-
-
-```
-
-### Invocation Arguments
-
-`gpt4free.Completion.create()` method has two required arguments
-
-1. Provider: This is an enum representing different provider
-2. prompt: This is the user input
-
-#### Keyword Arguments
-
-Some of the keyword arguments are optional, while others are required.
-
-- You:
- - `safe_search`: boolean - default value is `False`
- - `include_links`: boolean - default value is `False`
- - `detailed`: boolean - default value is `False`
-- Quora:
- - `token`: str - this needs to be provided by the user
- - `model`: str - default value is `gpt-4`.
-
- (Available models: `['Sage', 'GPT-4', 'Claude+', 'Claude-instant', 'ChatGPT', 'Dragonfly', 'NeevaAI']`)
-- ForeFront:
- - `token`: str - this need to be provided by the user
-
-- Theb:
- (no keyword arguments required)
-
-#### Token generation of quora
-```python
-from gpt4free import quora
-
-token = quora.Account.create(logging=False)
-```
-
-### Token generation of ForeFront
-```python
-from gpt4free import forefront
-
-token = forefront.Account.create(logging=False)
-```
-
-## Copyright:
-
-This program is licensed under the [GNU GPL v3](https://www.gnu.org/licenses/gpl-3.0.txt)
-
-### Copyright Notice:
-
-```
-xtekky/gpt4free: multiple reverse engineered language-model api's to decentralise the ai industry.
-Copyright (C) 2023 xtekky
-
-This program is free software: you can redistribute it and/or modify
-it under the terms of the GNU General Public License as published by
-the Free Software Foundation, either version 3 of the License, or
-(at your option) any later version.
-
-This program is distributed in the hope that it will be useful,
-but WITHOUT ANY WARRANTY; without even the implied warranty of
-MERCHANTABILITY or FITNESS FOR A PARTICULAR PURPOSE. See the
-GNU General Public License for more details.
-
-You should have received a copy of the GNU General Public License
-along with this program. If not, see .
-```
diff --git a/spaces/101-5/gpt4free/testing/binghuan/testing.py b/spaces/101-5/gpt4free/testing/binghuan/testing.py
deleted file mode 100644
index 2db0b427a0aa6b78a5ffae2f3b0204325b022232..0000000000000000000000000000000000000000
--- a/spaces/101-5/gpt4free/testing/binghuan/testing.py
+++ /dev/null
@@ -1,31 +0,0 @@
-from BingHuan import ChatCompletion
-
-# Test 1
-response = ChatCompletion.create(model="gpt-3.5-turbo",
- provider="BingHuan",
- stream=False,
- messages=[{'role': 'user', 'content': 'who are you?'}])
-
-print(response)
-
-# Test 2
-# this prompt will return emoji in end of response
-response = ChatCompletion.create(model="gpt-3.5-turbo",
- provider="BingHuan",
- stream=False,
- messages=[{'role': 'user', 'content': 'what you can do?'}])
-
-print(response)
-
-
-# Test 3
-response = ChatCompletion.create(model="gpt-4",
- provider="BingHuan",
- stream=False,
- messages=[
- {'role': 'user', 'content': 'now your name is Bob'},
- {'role': 'assistant', 'content': 'Hello Im Bob, you asistant'},
- {'role': 'user', 'content': 'what your name again?'},
- ])
-
-print(response)
\ No newline at end of file
diff --git a/spaces/1gistliPinn/ChatGPT4/Examples/Adobe After Effects Cc 2014 Crack Amtlib.dll.md b/spaces/1gistliPinn/ChatGPT4/Examples/Adobe After Effects Cc 2014 Crack Amtlib.dll.md
deleted file mode 100644
index 0213bde1355d998c3a25b284191f9366716da902..0000000000000000000000000000000000000000
--- a/spaces/1gistliPinn/ChatGPT4/Examples/Adobe After Effects Cc 2014 Crack Amtlib.dll.md
+++ /dev/null
@@ -1,38 +0,0 @@
-
adobe after effects cc 2014 crack amtlib.dll DOWNLOAD — https://imgfil.com/2uy1W2
-
-not found
-
-I'm running Adobe After Effects CC 2014 on a windows 7 system 64bit.
-
-I'm trying to add a CS6 project to this installation. It is running fine but everytime I try to add a css file or stylesheet it fails saying amtlib.dll was not found. I am running the 64bit OS. I've looked through other threads here and I've tried to:
-
-Add the libraries to the Adobe directory located in C:\Program Files\Adobe\Adobe After Effects CC 2014
-
-Create a symbolic link pointing to C:\Program Files\Adobe\Adobe After Effects CC 2014\amtlib.dll
-
-Restart computer
-
-Nothing seems to work. Any thoughts? Any further help is appreciated. Thank you.
-
-A:
-
-In my case Adobe added the dll in the wrong folder. Where it was pointing to is the Adobe Shared\amtlib.dll, if you delete this folder and open the installation folder and make the symbolic link again, it will work.
-
-Pages
-
-Thursday, May 14, 2012
-
-Thursday Thirteen - Next chapter!
-
-And that is the end of this story. It's been a good ride, but I think it's time for me to move on to other projects. But, what projects?
-
-Next story is going to be written by my buddy Gary Marti. Gary lives about thirty-five miles away from me in a little city in Texas named Oasis. He and I went to school together (seven years) and have been friends since. His wife, Kari, and I have been friends as well.
-
-While I've known Gary for many years, I'm really looking forward to sharing a great friendship with him. Gary and I have been discussing a story and I'm excited that he's going to write it for me. I'm even more excited that I can write along side Gary and we'll take turns with each chapter. Gary has been taking his time in working on the chapter, so he doesn't have any chapters in writing yet.
-
-I'm not telling you anything about this story except for the fact that it will involve a sports team and a man that will determine the fate of the team. And, just as important, he will determine the fate of the man.
-
-Right now, I'm thinking of some of my writing projects and decided that I'm going to write a short story about 4fefd39f24
-
-
-
diff --git a/spaces/1line/AutoGPT/autogpt/agent/agent_manager.py b/spaces/1line/AutoGPT/autogpt/agent/agent_manager.py
deleted file mode 100644
index 898767a485e50b5e62625a7883edf1b30d5fddf9..0000000000000000000000000000000000000000
--- a/spaces/1line/AutoGPT/autogpt/agent/agent_manager.py
+++ /dev/null
@@ -1,103 +0,0 @@
-"""Agent manager for managing GPT agents"""
-from __future__ import annotations
-
-from typing import Union
-
-from autogpt.config.config import Singleton
-from autogpt.llm_utils import create_chat_completion
-
-
-class AgentManager(metaclass=Singleton):
- """Agent manager for managing GPT agents"""
-
- def __init__(self):
- self.next_key = 0
- self.agents = {} # key, (task, full_message_history, model)
-
- # Create new GPT agent
- # TODO: Centralise use of create_chat_completion() to globally enforce token limit
-
- def create_agent(self, task: str, prompt: str, model: str) -> tuple[int, str]:
- """Create a new agent and return its key
-
- Args:
- task: The task to perform
- prompt: The prompt to use
- model: The model to use
-
- Returns:
- The key of the new agent
- """
- messages = [
- {"role": "user", "content": prompt},
- ]
-
- # Start GPT instance
- agent_reply = create_chat_completion(
- model=model,
- messages=messages,
- )
-
- # Update full message history
- messages.append({"role": "assistant", "content": agent_reply})
-
- key = self.next_key
- # This is done instead of len(agents) to make keys unique even if agents
- # are deleted
- self.next_key += 1
-
- self.agents[key] = (task, messages, model)
-
- return key, agent_reply
-
- def message_agent(self, key: str | int, message: str) -> str:
- """Send a message to an agent and return its response
-
- Args:
- key: The key of the agent to message
- message: The message to send to the agent
-
- Returns:
- The agent's response
- """
- task, messages, model = self.agents[int(key)]
-
- # Add user message to message history before sending to agent
- messages.append({"role": "user", "content": message})
-
- # Start GPT instance
- agent_reply = create_chat_completion(
- model=model,
- messages=messages,
- )
-
- # Update full message history
- messages.append({"role": "assistant", "content": agent_reply})
-
- return agent_reply
-
- def list_agents(self) -> list[tuple[str | int, str]]:
- """Return a list of all agents
-
- Returns:
- A list of tuples of the form (key, task)
- """
-
- # Return a list of agent keys and their tasks
- return [(key, task) for key, (task, _, _) in self.agents.items()]
-
- def delete_agent(self, key: Union[str, int]) -> bool:
- """Delete an agent from the agent manager
-
- Args:
- key: The key of the agent to delete
-
- Returns:
- True if successful, False otherwise
- """
-
- try:
- del self.agents[int(key)]
- return True
- except KeyError:
- return False
diff --git a/spaces/1pelhydcardo/ChatGPT-prompt-generator/assets/COD Warzone Vondel Map High Stakes Event and More - Download Today.md b/spaces/1pelhydcardo/ChatGPT-prompt-generator/assets/COD Warzone Vondel Map High Stakes Event and More - Download Today.md
deleted file mode 100644
index 3d6f653e7661b119fdcb1d1ff77b3a5a19022f14..0000000000000000000000000000000000000000
--- a/spaces/1pelhydcardo/ChatGPT-prompt-generator/assets/COD Warzone Vondel Map High Stakes Event and More - Download Today.md
+++ /dev/null
@@ -1,117 +0,0 @@
-
-How to Download and Play COD Warzone: A Complete Guide
-If you are looking for a thrilling and action-packed battle royale game, you might want to check out COD Warzone. This free-to-play game is set in the Modern Warfare universe and offers a variety of modes, features, and challenges to keep you entertained. In this guide, we will show you how to download and play COD Warzone on PC, PS4, and Xbox One, as well as give you some tips and tricks to help you win.
-cod warzone download Download File ✔ https://urlin.us/2uT1zl
- What is COD Warzone?
-A free-to-play battle royale game set in the Modern Warfare universe
-COD Warzone is a spin-off of the popular Call of Duty franchise, developed by Infinity Ward and Raven Software. It was released in March 2020 as a standalone game that does not require any previous Call of Duty titles to play. It is also cross-platform, meaning that you can play with your friends regardless of what device they are using.
-COD Warzone is set in Verdansk, a fictional city inspired by real-world locations in Eastern Europe. The game features over 300 points of interest, multiple named zones, and distinct landmarks to explore. The map is constantly evolving with new updates, events, and seasons that introduce new content and changes.
- The main features and modes of COD Warzone
-Battle Royale: Survive against up to 150 players in a shrinking map
-The core mode of COD Warzone is Battle Royale, where you can play solo or in teams of two, three, or four. Your goal is to be the last one standing out of up to 150 players who parachute into the map. You have to scavenge for weapons, equipment, cash, and contracts that give you objectives and rewards. You also have to avoid the gas that closes in on the map over time, forcing you to move to safer zones.
-One of the unique features of COD Warzone's Battle Royale is the Gulag. When you die for the first time in a match, you are sent to the Gulag, where you have a chance to fight another fallen player in a 1v1 match. The winner gets to redeploy back into the game, while the loser is eliminated. You can also be revived by your teammates or buy back your teammates at Buy Stations if they have enough cash.
- Plunder: Collect cash and loot in a race to reach $1 million
-If you prefer a more casual and less stressful mode, you can try Plunder. In this mode, you can play in teams of two, three, or four, and your goal is to collect as much cash as possible by looting, completing contracts, killing enemies, or depositing at helipads or balloons. The first team to reach $1 million triggers overtime
where the cash values are doubled and the team with the most cash at the end wins. You can respawn unlimited times in this mode, but you lose some of your cash when you die. You can also loot cash from other players or steal their deposits.
- Strongholds: Raid AI-protected buildings for high-tier loot and rewards
-A new mode that was added in Season 6 of COD Warzone is Strongholds. In this mode, you can play in teams of two, three, or four, and your goal is to raid buildings that are guarded by AI enemies. These buildings contain high-tier loot, such as legendary weapons, killstreaks, and armor satchels. You also get rewards for clearing each floor and reaching the rooftop, where you can find a helicopter that will take you to the next stronghold.
-cod warzone download size
-cod warzone download pc free
-cod warzone download ps4
-cod warzone download xbox one
-cod warzone download time
-cod warzone download error
-cod warzone download stuck
-cod warzone download slow
-cod warzone download season 4
-cod warzone download not working
-cod warzone download update
-cod warzone download link
-cod warzone download requirements
-cod warzone download mac
-cod warzone download steam
-cod warzone download speed
-cod warzone download without modern warfare
-cod warzone download problem
-cod warzone download vondel map
-cod warzone download free to play
-cod warzone download latest version
-cod warzone download offline
-cod warzone download for android
-cod warzone download for windows 10
-cod warzone download for laptop
-cod warzone download from activision website[^1^]
-cod warzone download from playstation store[^2^]
-cod warzone download from xbox store[^2^]
-cod warzone download from battle.net[^2^]
-cod warzone download from steam[^2^]
-cod warzone download high stakes event[^1^]
-cod warzone download tactical amphibious vehicle[^1^]
-cod warzone download ricochet anti-cheat[^1^]
-cod warzone download blackcell sector[^1^]
-cod warzone download tips and tricks[^3^]
-cod warzone download best settings[^3^]
-cod warzone download best weapons[^3^]
-cod warzone download best loadouts[^3^]
-cod warzone download best operators[^3^]
-cod warzone download best perks[^3^]
-However, you are not alone in this mode. Other teams can also enter the same stronghold and compete with you for the loot and rewards. You can also encounter other teams on your way to the next stronghold or at the extraction point. You have to balance between speed and stealth, as well as teamwork and strategy, to survive and win this mode.
- Black Sites: Explore mysterious locations for secrets and surprises
-Another new feature that was added in Season 6 of COD Warzone is Black Sites. These are hidden locations that are scattered around the map and can only be accessed by finding and activating red access cards. These cards can be found by looting crates, completing contracts, or killing enemies. Once you activate a card, you can enter a black site and explore its secrets and surprises.
-Black sites contain rare loot, such as specialist tokens, juggernaut suits, advanced UAVs, and self-revive kits. They also have clues and hints about the lore and story of COD Warzone, as well as Easter eggs and puzzles that can unlock rewards or trigger events. Some black sites are more dangerous than others, as they may have traps, alarms, or enemies waiting for you. You also have to watch out for other players who may follow you or ambush you at the black sites.
- How to download COD Warzone on PC, PS4, and Xbox One
-PC: Download the Battle.net launcher and install the game
-If you want to play COD Warzone on PC, you need to download the Battle.net launcher from the official website of Blizzard Entertainment. This is a free platform that allows you to access and play games developed by Blizzard or its partners, such as COD Warzone. Once you download and install the launcher, you need to create an account or log in with an existing one.
-After that, you can find COD Warzone in the Games tab of the launcher. You can click on it and then click on Install to start downloading the game. The game size is about 100 GB, so make sure you have enough space and a stable internet connection. You can also adjust the download settings and preferences in the launcher.
- The system requirements for PC
-Before you download COD Warzone on PC, you should check if your system meets the minimum or recommended requirements for the game. Here are the system requirements according to the official website of COD Warzone:
- | Minimum | Recommended | | --- | --- | | OS: Windows 7 64-Bit (SP1) or Windows 10 64-Bit | OS: Windows 10 64 Bit (latest update) | | CPU: Intel Core i3-4340 or AMD FX-6300 | CPU: Intel Core i5-2500K or AMD Ryzen R5 1600X | | RAM: 8 GB | RAM: 12 GB | | GPU: NVIDIA GeForce GTX 670 / NVIDIA GeForce GTX 1650 or AMD Radeon HD 7950 | GPU: NVIDIA GeForce GTX 970 / NVIDIA GeForce GTX 1660 or AMD Radeon R9 390 / AMD Radeon RX 580 | | HDD: 100 GB | HDD: 100 GB | | DirectX: Version 12 | DirectX: Version 12 | PS4: Download the game from the PlayStation Store
-If you want to play COD Warzone on PS4, you need to download the game from the PlayStation Store. You can access the store from your PS4 console or from a web browser on your PC or mobile device. You need to have a PlayStation Network account to access the store and download the game.
-Once you find COD Warzone in the store, you can click on Download to start downloading the game. The game size is about 100 GB, so make sure you have enough space and a stable internet connection. You can also check the download progress and status in your Notifications menu on your PS4 console.
-
- As mentioned, you need to have at least 100 GB of free space on your PS4 console to download and install COD Warzone. You can check your available space in the Settings menu of your console. You can also delete or move some files or games to free up some space if needed.
-Another thing you need to play COD Warzone on PS4 is an online subscription. You need to have a PlayStation Plus membership to play online multiplayer games on PS4. This is a paid service that gives you access to online gaming, free monthly games, exclusive discounts, and more. You can buy a PlayStation Plus membership from the PlayStation Store or from a retailer. You can choose from different plans, such as monthly, quarterly, or yearly.
- Xbox One: Download the game from the Microsoft Store
-If you want to play COD Warzone on Xbox One, you need to download the game from the Microsoft Store. You can access the store from your Xbox One console or from a web browser on your PC or mobile device. You need to have a Microsoft account to access the store and download the game.
-Once you find COD Warzone in the store, you can click on Get to start downloading the game. The game size is about 100 GB, so make sure you have enough space and a stable internet connection. You can also check the download progress and status in your Queue menu on your Xbox One console.
- The storage space and online subscription required for Xbox One
-As mentioned, you need to have at least 100 GB of free space on your Xbox One console to download and install COD Warzone. You can check your available space in the Settings menu of your console. You can also delete or move some files or games to free up some space if needed.
-Another thing you need to play COD Warzone on Xbox One is an online subscription. You need to have an Xbox Live Gold membership to play online multiplayer games on Xbox One. This is a paid service that gives you access to online gaming, free monthly games, exclusive discounts, and more. You can buy an Xbox Live Gold membership from the Microsoft Store or from a retailer. You can choose from different plans, such as monthly, quarterly, or yearly.
- How to play COD Warzone: Tips and tricks for beginners
-Prioritize getting your loadout and armor satchel
-One of the most important things to do in COD Warzone is to get your loadout and armor satchel as soon as possible. Your loadout is a custom set of weapons, perks, and equipment that you can create in the main menu of the game. You can access your loadout in a match by buying a loadout drop at a Buy Station for $10,000 or by finding one that drops randomly on the map.
-Your loadout allows you to use your preferred weapons and perks that suit your playstyle and strategy. For example, you can use a sniper rifle and a ghost perk if you want to be stealthy and snipe enemies from afar, or you can use a shotgun and an overkill perk if you want to rush enemies and deal high damage up close.
-Your armor satchel is an item that allows you to carry up to eight armor plates instead of five. Armor plates are essential for surviving in COD Warzone, as they give you extra health and protection from enemy fire. You can find armor plates by looting crates, enemies, or Buy Stations. You can also find armor satchels by looting legendary crates, enemies, or Buy Stations.
- Communicate and use the ping system with your teammates
-Another important thing to do in COD Warzone is to communicate and use the ping system with your teammates. Communication is key for teamwork and coordination in any multiplayer game, especially in a battle royale game where you have to work together to survive and win. You can communicate with your teammates by using voice chat or text chat in the game.
-The ping system is a feature that allows you to mark locations, enemies, items, or other points of interest on the map or on your screen for your teammates to see. You can use the ping system by pressing the D-pad on your controller or the left alt key on your keyboard. You can also use different types of pings by holding down the ping button and selecting an option from the wheel menu.
-The ping system is very useful for sharing information and giving commands without using voice chat or text chat. For example, you can ping an enemy location to warn your teammates of danger, ping a loot crate to tell your teammates where to find items, ping a Buy Station to suggest buying something, or ping a location to tell your teammates where to go or regroup.
- Keep an eye on the map and the circle movements
-A third important thing to do in COD Warzone is to keep an eye on the map and the circle movements. The map is your best friend in a battle royale game, as it shows you where you are, where your teammates are, where your enemies are, where the loot is, where the contracts are, where the Buy Stations are, and more. You can access the map by pressing the touchpad on your controller or the M key on your keyboard.
-The circle movements are the mechanism that forces you and your enemies to move closer together as the match progresses. The circle is a safe zone that shrinks over time, and anyone who is outside of it will take damage from the gas. The circle movements are shown on the map as white and yellow lines, and you can also see a timer that tells you when the next circle will start moving.
-You should always be aware of where the circle is and where it is going, as well as plan your route and position accordingly. You don't want to be caught in the gas or in a bad spot when the circle closes in. You also want to avoid being in the open or in a crowded area where you can be easily spotted or ambushed by enemies.
- Visit strongholds and black sites for better loot and challenges
-A fourth important thing to do in COD Warzone is to visit strongholds and black sites for better loot and challenges. As we mentioned earlier, these are new features that were added in Season 6 of COD Warzone, and they offer a lot of benefits and risks for players who dare to explore them.
-Strongholds are buildings that are guarded by AI enemies, and they contain high-tier loot and rewards. You can find strongholds by looking for red icons on the map or on your screen. You can enter a stronghold by finding a keypad and entering a code that you can get from crates, contracts, or enemies. You can then clear each floor of the stronghold and reach the rooftop, where you can find a helicopter that will take you to the next stronghold.
-Black sites are hidden locations that can only be accessed by finding and activating red access cards. These cards can be found by looting crates, contracts, or enemies. You can then use a card to open a door or an elevator that will take you to a black site. Black sites contain rare loot, clues, Easter eggs, puzzles, and events.
-Both strongholds and black sites are great places to find better loot and challenges, but they also come with risks. You have to fight against AI enemies or other players who may enter the same location. You also have to manage your time and resources, as you may miss out on other opportunities or get caught by the circle if you spend too much time in these locations.
- Play to your strengths and use cover wisely
-A fifth important thing to do in COD Warzone is to play to your strengths and use cover wisely. COD Warzone is a game that rewards skill, strategy, and creativity, but it also punishes mistakes, carelessness, and recklessness. You have to know your strengths and weaknesses as a player, as well as your weapons and equipment.
-You should play to your strengths and use weapons and equipment that suit your playstyle and strategy. For example, if you are good at sniping, you should use a sniper rifle and a scope that allow you to hit long-range shots. If you are good at rushing, you should use a shotgun or an SMG that allow you to deal high damage up close.
-You should also use cover wisely and avoid exposing yourself unnecessarily. Cover is anything that can protect you from enemy fire, such as walls, buildings, rocks, trees, vehicles, etc. You should always move from cover to cover and avoid running in the open or standing still for too long. You should also use different types of cover depending on the situation. For example, if you are being sniped from afar, you should use hard cover that blocks bullets completely. If you are being rushed by enemies nearby , you should use soft cover that allows you to peek and shoot quickly.
- Conclusion
-COD Warzone is a fun and exciting battle royale game that offers a lot of variety, content, and challenges for players of all skill levels. Whether you want to play solo or with your friends, you can enjoy the different modes, features, and events that COD Warzone has to offer. You can also customize your loadout, explore the map, and discover secrets and surprises along the way.
-To play COD Warzone, you need to download the game from the appropriate store depending on your device. You also need to have enough space and a stable internet connection. You may also need to have an online subscription if you are playing on PS4 or Xbox One. You can then start playing the game and follow the tips and tricks we have shared in this guide to help you win.
-We hope you found this guide helpful and informative. If you have any questions or feedback, please let us know in the comments below. Thank you for reading and happy gaming!
- FAQs
-Q: How much does COD Warzone cost?
-A: COD Warzone is a free-to-play game that does not require any previous Call of Duty titles to play. However, you may need to pay for an online subscription if you are playing on PS4 or Xbox One.
- Q: How often does COD Warzone update?
-A: COD Warzone updates regularly with new seasons, events, and patches that introduce new content and changes. Each season lasts for about two months and has its own theme, story, and rewards. Each event lasts for a limited time and has its own objectives, challenges, and rewards. Each patch fixes bugs, balances gameplay, and improves performance.
- Q: How many players can play COD Warzone?
-A: COD Warzone supports up to 150 players in a match, depending on the mode and settings. You can play solo or in teams of two, three, or four.
- Q: How do I get better at COD Warzone?
-A: The best way to get better at COD Warzone is to practice and learn from your mistakes. You can also watch tutorials, guides, and streams from other players who are more experienced or skilled than you. You can also try different weapons, perks, and strategies to find what works best for you.
- Q: Is COD Warzone cross-platform?
-A: Yes, COD Warzone is cross-platform, meaning that you can play with your friends regardless of what device they are using. You can also enable or disable cross-play in the settings menu of the game.
197e85843d
-
-
\ No newline at end of file
diff --git a/spaces/1phancelerku/anime-remove-background/Download Ludo Nasa Now and Enjoy the Best Mobile Game of the Year.md b/spaces/1phancelerku/anime-remove-background/Download Ludo Nasa Now and Enjoy the Best Mobile Game of the Year.md
deleted file mode 100644
index aab6644f717b072560f728c31b5480d7c3843624..0000000000000000000000000000000000000000
--- a/spaces/1phancelerku/anime-remove-background/Download Ludo Nasa Now and Enjoy the Best Mobile Game of the Year.md
+++ /dev/null
@@ -1,132 +0,0 @@
-
-Ludo Nasa Download: A Guide to the Most Popular Game of the Year
-Ludo nasa is a free-to-play mobile game application that has taken the world by storm. It is a modern version of the classic board game ludo, which is derived from the ancient Indian game of Pachisi. Ludo nasa offers a variety of features and themes that make it more fun and engaging than ever. Whether you want to play with your family and friends, or challenge players from around the world, ludo nasa has something for everyone. In this article, we will tell you everything you need to know about ludo nasa download, including its history, features, and benefits.
- History of ludo game
-Ludo game has a long and rich history that dates back to the 6th century CE in India. It is believed that the game was created by the Indian maharajas, who played it on a board made of cloth or slate, using seeds, shells, or dice as tokens. The original version of the game was called Chaupar, and it was also described in the Indian epic Mahabharata, where it was used as a tool for gambling and deception. The game was later modified by the Mughal emperors, such as Akbar, who played it with real people as tokens on a life-sized board. The game was also known as Pachisi, which means twenty-five in Hindi, referring to the highest score possible in the game.
-ludo nasa download Download --->>> https://jinyurl.com/2uNRSm
-The game spread to other countries and regions through trade and colonization, and acquired different names and variations. For example, in Spain, it was called Parcheesi; in China, it was called Chatush pada; and in Africa, it was called Ludu. The game reached England in the 19th century, where it was patented as Ludo by Alfred Collier in 1896. Ludo means "I play" in Latin, and it became a popular board game for children and adults alike. Ludo also inspired other games, such as Uckers, which was played by the Royal Navy.
- Features of ludo game
-Ludo game is a simple yet strategic board game that can be played by two to four players. The objective of the game is to move four tokens of the same color from the starting point to the finishing point on the board, according to the rolls of a single die. The first player to do so wins the game. However, there are some challenges and twists along the way, such as:
-
-If a player rolls a six, they get another turn to roll the die.
-If a player lands on a square occupied by an opponent's token, they can capture that token and send it back to the starting point.
-If a player lands on a square occupied by their own token, they can form a block that cannot be captured by opponents.
-If a player reaches the square below their home column, they can move their tokens up the column to the finishing point.
-
-Ludo game can be played in different modes and themes, depending on the preference of the players. Some of the common modes and themes are:
-
-vs Computer: This mode allows players to play offline against the computer AI.
-Local Mode: This mode allows players to play offline with their family and friends on the same device.
-Online Multiplayer: This mode allows players to play online with other players from around the world.
-Private Multiplayer: This mode allows players to play online with their Facebook friends or other invited players in private rooms.
-Nature Theme: This theme continues the theme of the board game with natural elements, such as trees, flowers, and animals.
-Egypt Theme: This theme adds a touch of ancient history and mythology to the board game, with pyramids, sphinxes, and pharaohs.
-Disco Theme: This theme brings some fun and excitement to the board game, with colorful lights, music, and dance moves.
-NASA Theme: This theme takes the board game to outer space, with planets, stars, and rockets.
-
-Ludo game also has some social benefits that make it more enjoyable and rewarding for the players. Some of these benefits are:
-
-It improves the cognitive skills and logical thinking of the players, as they have to plan their moves and strategies.
-It enhances the communication and teamwork skills of the players, as they have to interact and cooperate with each other.
-It reduces stress and boredom, as it provides a fun and relaxing way to pass the time.
-It strengthens the bonds and relationships of the players, as it creates a sense of camaraderie and competition.
-
- Ludo nasa download
-Ludo nasa is one of the most popular and downloaded versions of ludo game in the market. It has over 100 million downloads on Google Play Store and over 10 million downloads on App Store. It is compatible with Android and iOS devices, as well as Windows PC and Mac. To download and play ludo nasa on your device, you can follow these simple steps:
- For Android devices
-
-Go to Google Play Store and search for ludo nasa.
-Select the app from the list and tap on Install.
-Wait for the app to download and install on your device.
-Open the app and enjoy playing ludo nasa with your friends or online players.
-
- For iOS devices
-
-Go to App Store and search for ludo nasa.
-Select the app from the list and tap on Get.
-Enter your Apple ID password or use Touch ID or Face ID to confirm.
-Wait for the app to download and install on your device.
-Open the app and enjoy playing ludo nasa with your friends or online players.
-
- For Windows PC or Mac
-
-Go to https://ludonasa.com/ and click on Download for PC or Download for Mac.
-Select the version that matches your operating system and click on Download Now.
-Wait for the file to download on your computer.
-Open the file and follow the instructions to install ludo nasa on your computer.
-Launch ludo nasa from your desktop or start menu and enjoy playing ludo nasa with your friends or online players.
-
- Conclusion
-Ludo nasa is a fun and exciting game that you can play anytime, anywhere, with anyone. It is based on the classic board game ludo, which has a long and rich history in India and other countries. Ludo nasa offers a variety of features and themes that make it more appealing and engaging than ever. It also has some social benefits that improve your cognitive, communication, and emotional skills. If you are looking for a game that can entertain you, challenge you, and connect you with others, then you should definitely try ludo nasa download. Here are some tips and tricks that can help you win more games:
-ludo nasa game download
-ludo nasa app download
-ludo nasa apk download
-ludo nasa online game download
-ludo nasa play and win money download
-ludo nasa free download
-ludo nasa download for pc
-ludo nasa download for android
-ludo nasa download for ios
-ludo nasa download link
-ludo nasa latest version download
-ludo nasa mod apk download
-ludo nasa hack apk download
-ludo nasa unlimited money download
-ludo nasa real money game download
-ludo nasa best ludo game download
-ludo nasa india gaming awards 2023 winner download
-ludo nasa quick mode game download
-ludo nasa entertainment game download
-ludo nasa board game download
-how to download ludo nasa game
-where to download ludo nasa game
-why to download ludo nasa game
-what is ludo nasa game download
-when to download ludo nasa game
-ludonasa.com game download
-ludonasa.com app download
-ludonasa.com apk download
-ludonasa.com online game download
-ludonasa.com play and win money download
-ludonasa.com free download
-ludonasa.com download for pc
-ludonasa.com download for android
-ludonasa.com download for ios
-ludonasa.com download link
-ludonasa.com latest version download
-ludonasa.com mod apk download
-ludonasa.com hack apk download
-ludonasa.com unlimited money download
-ludonasa.com real money game download
-ludonasa.com best ludo game download
-ludonasa.com india gaming awards 2023 winner download
-ludonasa.com quick mode game download
-ludonasa.com entertainment game download
-ludonasa.com board game download
-how to play ludo nasa after downloading it
-how to win money on ludo nasa after downloading it
-how to invite friends on ludo nasa after downloading it
-how to update ludo nasa after downloading it
-how to contact support on ludo nasa after downloading it
-
-Always try to roll a six at the beginning of the game, so that you can move your tokens out of the starting point faster.
-Avoid landing on squares that are occupied by your opponents' tokens, as they can capture them and send them back to the starting point.
-Use blocks to protect your tokens from being captured by your opponents. You can form a block by landing two or more tokens of the same color on the same square.
-Be careful when moving your tokens up the home column, as they can only move according to the exact number rolled on the die. If you roll a higher number than needed, you will have to skip your turn.
-Use different themes to spice up your game experience. Each theme has its own music, sound effects, graphics, and animations that can make your game more enjoyable.
-
- We hope that this article has given you some useful information about ludo nasa download. If you have any questions or feedback about ludo nasa, feel free to share them with us in the comments section below. Thank you for reading our article. We hope that you have learned something new and interesting about ludo nasa download. Before we end, we would like to answer some of the frequently asked questions that you might have about ludo nasa. Here are the top five FAQs that we have selected for you:
FAQs
-
-What is the difference between ludo nasa and ludo king?
-Ludo nasa and ludo king are both popular versions of ludo game, but they have some differences in terms of features and themes. Ludo nasa has more themes than ludo king, such as nature, Egypt, disco, and NASA. Ludo nasa also has more modes than ludo king, such as vs computer, local mode, online multiplayer, and private multiplayer. Ludo nasa also has a better user interface and graphics than ludo king.
-How can I play ludo nasa with voice chat?
-Ludo nasa has a voice chat feature that allows you to communicate with your friends or online players while playing the game. To use this feature, you need to enable the microphone permission on your device and join a private room with your friends or online players. Then, you can tap on the microphone icon on the top right corner of the screen to start or stop the voice chat.
-How can I earn coins and gems in ludo nasa?
-Coins and gems are the in-game currencies that you can use to buy different themes and items in ludo nasa. You can earn coins and gems by playing and winning games, completing daily tasks, watching ads, spinning the wheel, or inviting your friends to play the game. You can also buy coins and gems with real money if you want to.
-How can I update ludo nasa to the latest version?
-Ludo nasa is constantly updated with new features and improvements to enhance your gaming experience. To update ludo nasa to the latest version, you need to go to Google Play Store or App Store and check if there is any update available for the app. If there is, you can tap on Update and wait for the app to download and install on your device.
-How can I contact the customer support of ludo nasa?
-If you have any issues or queries about ludo nasa, you can contact the customer support of ludo nasa by sending an email to ludonasa@gmail.com or by filling out the feedback form on their website https://ludonasa.com/. They will try to respond to your message as soon as possible.
- 197e85843d
-
-
\ No newline at end of file
diff --git a/spaces/1phancelerku/anime-remove-background/Download QS Ar-Rahman - The Surah that Will Make You Cry.md b/spaces/1phancelerku/anime-remove-background/Download QS Ar-Rahman - The Surah that Will Make You Cry.md
deleted file mode 100644
index 16416a8580589e88c6d2eeee5e136adfb6f79118..0000000000000000000000000000000000000000
--- a/spaces/1phancelerku/anime-remove-background/Download QS Ar-Rahman - The Surah that Will Make You Cry.md
+++ /dev/null
@@ -1,174 +0,0 @@
-
-Download QS Ar Rahman: How to Listen to the Beautiful Surah Online
-QS Ar Rahman is one of the most beautiful and powerful surahs in the Quran. It is also known as "The Beneficent" or "The Most Merciful" because it begins with the name of Allah, the Most Compassionate. In this article, we will explore what QS Ar Rahman is, why it is important, and how you can download it in different formats and languages. We will also share some tips on how to benefit from listening to or reading this surah.
- What is QS Ar Rahman and Why is it Important?
-QS Ar Rahman is the 55th surah in the Quran, consisting of 78 verses. It was revealed in Medina, after the migration of the Prophet Muhammad (peace be upon him) and his companions from Mecca. It is one of the surahs that begins with one of the names of Allah, which is a rare feature in the Quran. It is also one of the surahs that has a refrain or chorus, which is repeated 31 times throughout the surah: "Maka, nikmat Tuhanmu manakah yang kamu dustakan (wahai jin dan manusia)?" This means "Then which of the favors of your Lord will you deny (O jinn and mankind)?"
-download qs ar rahman Download Zip › https://jinyurl.com/2uNIib
- QS Ar Rahman is important because it reminds us of the countless blessings and favors that Allah has bestowed upon us, both in this world and the hereafter. It also invites us to reflect on the signs of Allah's power and wisdom in His creation, such as the sun, the moon, the stars, the plants, the animals, the seas, and the human beings. It also warns us of the consequences of denying or rejecting Allah's favors, such as the punishment of hellfire or the deprivation of paradise. It also encourages us to be grateful, humble, and obedient to Allah, who is the Most Merciful and the Most Generous.
- The Meaning and Benefits of QS Ar Rahman
-The meaning of QS Ar Rahman is derived from its first verse, which states: "Ar-Rahman (The Most Compassionate)". This is one of the names of Allah, which describes His attribute of being infinitely kind, loving, caring, and forgiving to His creation. He is also Ar-Raheem (The Most Merciful), which means He bestows His mercy upon those who believe in Him and do good deeds. He is also Al-Wadud (The Most Loving), which means He loves those who love Him and follow His guidance.
- The benefits of QS Ar Rahman are many, as it contains verses that praise Allah's greatness, glorify His majesty, describe His favors, warn against His wrath, promise His reward, and invite to His worship. Some of the benefits are:
-
-It increases one's faith and gratitude towards Allah.
-It protects one from evil influences and temptations.
-It brings peace and tranquility to one's heart and mind.
-It purifies one's soul and cleanses one It increases one's knowledge and understanding of the Quran.
-It enhances one's love and admiration for Allah and His creation.
-
- The Occasion and Context of Revelation of QS Ar Rahman
-The occasion and context of revelation of QS Ar Rahman are related to the events that took place in Medina, after the migration of the Prophet Muhammad (peace be upon him) and his companions from Mecca. The surah was revealed to address the challenges and opportunities that the Muslim community faced in their new environment, such as:
-
-The interaction and coexistence with the Jews, Christians, and polytheists of Medina.
-The establishment and consolidation of the Islamic state and society.
-The expansion and propagation of Islam to other regions and peoples.
-The defense and security of the Muslim community from external threats and enemies.
-
- The surah was also revealed to highlight the contrast between the mercy and justice of Allah, and the ingratitude and rebellion of some of His creation, especially the jinn and mankind. The surah was also revealed to show the beauty and harmony of Allah's creation, and the signs and proofs of His oneness and lordship.
- How to Download QS Ar Rahman in Different Formats and Languages
-If you want to download QS Ar Rahman in different formats and languages, you have many options available online. You can choose from various websites and apps that offer Quran recitations, translations, tafsirs, and other resources. Here are some of the best sources that you can use:
-Download qs ar rahman full mp3
-Download qs ar rahman 1-78 ayat
-Download qs ar rahman latin dan terjemahan
-Download qs ar rahman muzammil hasballah
-Download qs ar rahman mishary rashid alafasy
-Download qs ar rahman muhammad taha al junayd
-Download qs ar rahman maghfirah m hussein
-Download qs ar rahman hanan attaki
-Download qs ar rahman abdul basit abdus samad
-Download qs ar rahman saad al ghamdi
-Download qs ar rahman hani ar rifai
-Download qs ar rahman syekh sudais
-Download qs ar rahman nasser al qatami
-Download qs ar rahman ahmad saud
-Download qs ar rahman yusuf mansur
-Download qs ar rahman muhammad thaha dewasa
-Download qs ar rahman fatih seferagic
-Download qs ar rahman wafiq azizah
-Download qs ar rahman yusuf kalo
-Download qs ar rahman imam masjidil haram
-Download qs ar rahman muammar za
-Download qs ar rahman muhammad toha al junaid dewasa
-Download qs ar rahman salim bahanan
-Download qs ar rahman idris abkar
-Download qs ar rahman maher al muaiqly
-Download qs ar rahman ahmad al ajmi
-Download qs ar rahman abdurrahman as sudais
-Download qs ar rahman syaikh ali jaber
-Download qs ar rahman syekh ali hudaify
-Download qs ar rahman syekh shuraim
-Download qs ar rahman syekh mahmud khalil al husary
-Download qs ar rahman syekh abdullah awad al juhani
-Download qs ar rahman syekh abdullah basfar
-Download qs ar rahman syekh abdul aziz al ahmad
-Download qs ar rahman syekh abdul muhsin al qasim
-Download qs ar rahman syekh abdul wadud haneef
-Download qs ar rahman syekh abu bakr ash shatri
-Download qs ar rahman syekh adil al kalbani
-Download qs ar rahman syekh ahmad bin ali al ajmy
-Download qs ar rahman syekh akram al alaqimy
-Download qs ar rahman syekh ali abdurrahman al hudzaify
-Download qs ar rahman syekh bandar baleela
-Download qs ar rahman syekh fawaz al kaabi
-Download qs ar rahman syekh faysal noman
-Download qs ar rahman syekh ibrahim al akhdar
-Download qs ar rahman syekh ibrahim al jibreen
-Download qs ar rahman syekh ibrahim as sudaisi an nabawy
- Download QS Ar Rahman in MP3 and Audio Formats
-If you want to download QS Ar Rahman in MP3 and audio formats, you can use the following websites:
- Quran.com: The Best Source for High Quality Quran Recitations
-Quran.com is one of the most popular and reliable websites for Quran recitations. It offers high quality audio files by various reciters from different countries and styles. You can listen to or download Surah Ar Rahman by any reciter of your choice, such as Abdul Basit, Mishary Rashid, Saad Al Ghamdi, Maher Al Mueaqly, etc. You can also choose from different translations in English, Urdu, Indonesian, French, Spanish, etc. You can also read the Arabic text along with the audio, or view the word by word translation and transliteration. You can access Quran.com from any device, such as your computer, smartphone, or tablet.
- To download Surah Ar Rahman from Quran.com, you can follow these steps:
-
-Go to [Quran.com] and search for Surah Ar Rahman in the search bar.
-Select the reciter and translation of your choice from the drop-down menus.
-Click on the play button to listen to the surah online, or click on the download button to save it on your device.
-You can also click on the settings icon to adjust the speed, repeat mode, night mode, etc.
-
- QuranicAudio.com: Stream or Download Quran Audio by Various Reciters
-QuranicAudio.com is another great website for Quran audio. It offers a large collection of Quran recitations by various reciters from different countries and styles. You can stream or download Surah Ar Rahman by any reciter of your choice, such as Abdullah Basfar, Abdur Rahman As Sudais, Abu Bakr Al Shatri, Ahmed Al Ajmi, etc. You can also choose from different translations in English, Urdu, Indonesian, French, Spanish, etc. You can also read the Arabic text along with the audio.
- To download Surah Ar Rahman from QuranicAudio.com, you can follow these steps:
-
-Go to [QuranicAudio.com] and search for Surah Ar Rahman in the search bar.
-Select the reciter and translation of your choice from the drop-down menus.
-Click on the play button to listen to the surah online, or right-click on the download button and select "Save link as" to save it on your device.
-You can also click on the settings icon to adjust the speed, repeat mode, night mode, etc.
-
- QuranCentral.com: Listen to Surah Ar Rahman by Different Qaris and Translations
-QuranCentral.com is another excellent website for Quran audio. It offers a wide range of Quran recitations by different qaris (reciters) from different countries and styles. You can listen to or download Surah Ar Rahman by any qari of your choice, such as Abdul Rahman Al Sudais, Muhammad Siddiq Al Minshawi, Muhammad Jibreel, Nasser Al Qatami, etc. You can also choose from different translations in English, Urdu, Indonesian, French, Spanish, etc. You can also read the Arabic text along with the audio, or view the word by word translation and transliteration. You can access QuranCentral.com from any device, such as your computer, smartphone, or tablet.
- To download Surah Ar Rahman from QuranCentral.com, you can follow these steps:
-
-Go to [QuranCentral.com] and search for Surah Ar Rahman in the search bar.
-Select the qari and translation of your choice from the drop-down menus.
-Click on the play button to listen to the surah online, or click on the download button to save it on your device.
-You can also click on the settings icon to adjust the speed, repeat mode, night mode, etc.
-
- Download QS Ar Rahman in PDF and Text Formats
-If you want to download QS Ar Rahman in PDF and text formats, you can use the following websites:
- LiteQuran.net: Read Surah Ar Rahman in Arabic, Latin, and Indonesian
-LiteQuran.net is a simple and easy-to-use website for reading Quran online. It offers Surah Ar Rahman in Arabic, Latin (transliteration), and Indonesian (translation). You can also listen to the audio recitation by various reciters. You can also view the tajweed rules and color codes for each verse. You can access LiteQuran.net from any device, such as your computer, smartphone, or tablet.
- To download Surah Ar Rahman from LiteQuran.net, you can follow these steps:
-
-Go to [LiteQuran.net] and search for Surah Ar Rahman in the search bar.
-Select the language and reciter of your choice from the drop-down menus.
-Click on the play button to listen to the surah online, or click on the PDF icon to download it on your device.
-You can also click on the settings icon to adjust the font size, color theme, night mode, etc.
-
- QuranBest.com: Read Surah Ar Rahman in Arabic and English with Tafsir
-QuranBest.com is a comprehensive and interactive website for reading Quran online. It offers Surah Ar Rahman in Arabic and English (translation) with tafsir (explanation) by various scholars and sources. You can also listen to the audio recitation by various reciters. You can also view the word by word translation and transliteration for each verse. You can also access other features such as bookmarks, notes, highlights, etc. You can access QuranBest.com from any device, such as your computer, smartphone, or tablet.
- To download Surah Ar Rahman from QuranBest.com, you can follow these steps:
-
-Go to [QuranBest.com] and search for Surah Ar Rahman in the search bar.
-Select the language, reciter, and tafsir of your choice from the drop-down menus.
-Click on the play button to listen to the surah online, or click on the PDF icon to download it on your device.
-You can also click on the settings icon to adjust the font size, color theme, night mode, etc.
-
- TafsirWeb.com: Read Surah Ar Rahman in Arabic and Indonesian with Tafsir
-TafsirWeb.com is a dedicated website for reading Quran tafsir online. It offers Surah Ar Rahman in Arabic and Indonesian (translation) with tafsir (explanation) by various scholars and sources. You can also listen to the audio recitation by various reciters. You can also view the word by word translation and transliteration for each verse. You can also access other features such as bookmarks, notes, highlights, etc. You can access TafsirWeb.com from any device, such as your computer, smartphone, or tablet.
- To download Surah Ar Rahman from TafsirWeb.com, you can follow these steps:
-
-Go to [TafsirWeb.com] and search for Surah Ar Rahman in the search bar.
-Select the language, reciter and tafsir of your choice from the drop-down menus.
-Click on the play button to listen to the surah online, or click on the PDF icon to download it on your device.
-You can also click on the settings icon to adjust the font size, color theme, night mode, etc.
-
- How to Benefit from Listening to or Reading QS Ar Rahman
-Listening to or reading QS Ar Rahman is not enough to benefit from its blessings and lessons. We also need to understand its meaning, reflect on its message, and apply its teachings in our daily life. Here are some tips on how to do that:
- Tips for Reciting or Listening to QS Ar Rahman with Focus and Reflection
-Reciting or listening to QS Ar Rahman with focus and reflection means paying attention to the words and their meanings, and thinking about their implications and relevance for us. Here are some tips on how to do that:
-
-Choose a suitable time and place where you can recite or listen to QS Ar Rahman without distractions or interruptions.
-Prepare yourself mentally and spiritually by making wudu (ablution), seeking refuge from Satan, and asking Allah for guidance and understanding.
-Recite or listen to QS Ar Rahman with a clear and melodious voice, following the rules of tajweed (proper pronunciation) and tartil (moderate speed).
-Pause at the end of each verse or section, and repeat the refrain "Maka, nikmat Tuhanmu manakah yang kamu dustakan (wahai jin dan manusia)?" This means "Then which of the favors of your Lord will you deny (O jinn and mankind)?" Try to answer this question in your mind or heart, and acknowledge Allah's favors upon you.
-Contemplate on the signs of Allah's power and wisdom in His creation, such as the sun, the moon, the stars, the plants, the animals, the seas, and the human beings. Think about how they reflect Allah's mercy and generosity towards us.
-Reflect on the consequences of denying or rejecting Allah's favors, such as the punishment of hellfire or the deprivation of paradise. Think about how you can avoid them by being grateful, humble, and obedient to Allah.
-Remember the promises of Allah's reward for those who believe in Him and do good deeds, such as the gardens of paradise or the companionship of the righteous. Think about how you can attain them by following Allah's guidance and commands.
-
- Tips for Applying the Lessons of QS Ar Rahman in Daily Life
-Applying the lessons of QS Ar Rahman in daily life means living according to its teachings and values, and implementing its wisdom and advice in our actions and interactions. Here are some tips on how to do that:
-
-Be grateful for Allah's favors and blessings upon you, and express your gratitude by praising Him, thanking Him, and worshipping Him.
-Be humble before Allah and His creation, and avoid arrogance, pride, and self-conceit. Recognize your limitations and weaknesses, and seek Allah's help and forgiveness.
-Be obedient to Allah and His messenger (peace be upon him), and follow their commands and prohibitions. Avoid sins, innovations, and deviations from the straight path.
-Be generous with Allah's favors and blessings upon you, and share them with others. Give charity, help the needy, support the cause of Islam, and spread goodness.
-Be respectful of Allah's creation, and treat them with kindness, justice, and compassion. Do not harm them, abuse them, or waste them. Appreciate their diversity and beauty.
-Be hopeful of Allah's mercy and forgiveness, and do not despair or give up. Repent from your sins, seek His pardon, and trust in His plan.
-
- Conclusion
-QS Ar Rahman is a beautiful and powerful surah that reminds us of Allah's mercy and favors, and invites us to reflect on His signs and proofs. It also warns us of the consequences of denying or rejecting His favors, and encourages us to be grateful, humble, and obedient to Him. We can benefit from this surah by downloading it in different formats and languages, and by reciting or listening to it with focus and reflection. We can also apply its lessons in our daily life by living according to its teachings and values. We ask Allah to make us among those who recite, listen, understand, and act upon QS Ar Rahman. Ameen.
- FAQs
-Here are some frequently asked questions about QS Ar Rahman:
-
-What is the main theme of QS Ar Rahman?
-The main theme of QS Ar Rahman is the mercy and favors of Allah, and the response of His creation to them.
-How many times is the refrain "Then which of the favors of your Lord will you deny (O jinn and mankind)?" repeated in QS Ar Rahman?
-The refrain is repeated 31 times throughout the surah.
-What are some of the favors of Allah that are mentioned in QS Ar Rahman?
-Some of the favors of Allah that are mentioned in QS Ar Rahman are: the Quran, the creation of man and jinn, the sun and the moon, the stars and the trees, the sky and the earth, the seas and the rivers, the fruits and the grains, the pearls and the corals, the gardens and the springs, etc.
-What are some of the consequences of denying or rejecting Allah's favors that are mentioned in QS Ar Rahman?
-Some of the consequences of denying or rejecting Allah's favors that are mentioned in QS Ar Rahman are: the punishment of hellfire, the scorching wind and boiling water, the chains and iron collars, etc.
-What are some of the rewards for those who believe in Allah and do good deeds that are mentioned in QS Ar Rahman?
-Some of the rewards for those who believe in Allah and do good deeds that are mentioned in QS Ar Rahman are: the gardens of paradise, the companionship of pure spouses, the honor and dignity from Allah, etc.
- 197e85843d
-
-
\ No newline at end of file
diff --git a/spaces/2ndelement/voicevox/voicevox_engine/dev/synthesis_engine/__init__.py b/spaces/2ndelement/voicevox/voicevox_engine/dev/synthesis_engine/__init__.py
deleted file mode 100644
index e7b2ac5b17594c2a9a137e23a72210209f2cbd4b..0000000000000000000000000000000000000000
--- a/spaces/2ndelement/voicevox/voicevox_engine/dev/synthesis_engine/__init__.py
+++ /dev/null
@@ -1,3 +0,0 @@
-from .mock import MockSynthesisEngine
-
-__all__ = ["MockSynthesisEngine"]
diff --git a/spaces/4Taps/SadTalker/src/utils/preprocess.py b/spaces/4Taps/SadTalker/src/utils/preprocess.py
deleted file mode 100644
index 4e3dad8d4a49080a3300f672965a11a8a2054fa2..0000000000000000000000000000000000000000
--- a/spaces/4Taps/SadTalker/src/utils/preprocess.py
+++ /dev/null
@@ -1,152 +0,0 @@
-import numpy as np
-import cv2, os, sys, torch
-from tqdm import tqdm
-from PIL import Image
-
-# 3dmm extraction
-from src.face3d.util.preprocess import align_img
-from src.face3d.util.load_mats import load_lm3d
-from src.face3d.models import networks
-from src.face3d.extract_kp_videos import KeypointExtractor
-
-from scipy.io import loadmat, savemat
-from src.utils.croper import Croper
-
-import warnings
-warnings.filterwarnings("ignore")
-
-def split_coeff(coeffs):
- """
- Return:
- coeffs_dict -- a dict of torch.tensors
-
- Parameters:
- coeffs -- torch.tensor, size (B, 256)
- """
- id_coeffs = coeffs[:, :80]
- exp_coeffs = coeffs[:, 80: 144]
- tex_coeffs = coeffs[:, 144: 224]
- angles = coeffs[:, 224: 227]
- gammas = coeffs[:, 227: 254]
- translations = coeffs[:, 254:]
- return {
- 'id': id_coeffs,
- 'exp': exp_coeffs,
- 'tex': tex_coeffs,
- 'angle': angles,
- 'gamma': gammas,
- 'trans': translations
- }
-
-
-class CropAndExtract():
- def __init__(self, path_of_lm_croper, path_of_net_recon_model, dir_of_BFM_fitting, device):
-
- self.croper = Croper(path_of_lm_croper)
- self.kp_extractor = KeypointExtractor(device)
- self.net_recon = networks.define_net_recon(net_recon='resnet50', use_last_fc=False, init_path='').to(device)
- checkpoint = torch.load(path_of_net_recon_model, map_location=torch.device(device))
- self.net_recon.load_state_dict(checkpoint['net_recon'])
- self.net_recon.eval()
- self.lm3d_std = load_lm3d(dir_of_BFM_fitting)
- self.device = device
-
- def generate(self, input_path, save_dir, crop_or_resize='crop'):
-
- pic_size = 256
- pic_name = os.path.splitext(os.path.split(input_path)[-1])[0]
-
- landmarks_path = os.path.join(save_dir, pic_name+'_landmarks.txt')
- coeff_path = os.path.join(save_dir, pic_name+'.mat')
- png_path = os.path.join(save_dir, pic_name+'.png')
-
- #load input
- if not os.path.isfile(input_path):
- raise ValueError('input_path must be a valid path to video/image file')
- elif input_path.split('.')[1] in ['jpg', 'png', 'jpeg']:
- # loader for first frame
- full_frames = [cv2.imread(input_path)]
- fps = 25
- else:
- # loader for videos
- video_stream = cv2.VideoCapture(input_path)
- fps = video_stream.get(cv2.CAP_PROP_FPS)
- full_frames = []
- while 1:
- still_reading, frame = video_stream.read()
- if not still_reading:
- video_stream.release()
- break
- full_frames.append(frame)
- break
- x_full_frames = [cv2.cvtColor(full_frames[0], cv2.COLOR_BGR2RGB) ]
-
- if crop_or_resize.lower() == 'crop': # default crop
- x_full_frames, crop, quad = self.croper.crop(x_full_frames, xsize=pic_size)
- clx, cly, crx, cry = crop
- lx, ly, rx, ry = quad
- lx, ly, rx, ry = int(lx), int(ly), int(rx), int(ry)
- oy1, oy2, ox1, ox2 = cly+ly, cly+ry, clx+lx, clx+rx
- original_size = (ox2 - ox1, oy2 - oy1)
- else:
- oy1, oy2, ox1, ox2 = 0, x_full_frames[0].shape[0], 0, x_full_frames[0].shape[1]
- original_size = (ox2 - ox1, oy2 - oy1)
-
- frames_pil = [Image.fromarray(cv2.resize(frame,(pic_size, pic_size))) for frame in x_full_frames]
- if len(frames_pil) == 0:
- print('No face is detected in the input file')
- return None, None
-
- # save crop info
- for frame in frames_pil:
- cv2.imwrite(png_path, cv2.cvtColor(np.array(frame), cv2.COLOR_RGB2BGR))
-
- # 2. get the landmark according to the detected face.
- if not os.path.isfile(landmarks_path):
- lm = self.kp_extractor.extract_keypoint(frames_pil, landmarks_path)
- else:
- print(' Using saved landmarks.')
- lm = np.loadtxt(landmarks_path).astype(np.float32)
- lm = lm.reshape([len(x_full_frames), -1, 2])
-
- if not os.path.isfile(coeff_path):
- # load 3dmm paramter generator from Deep3DFaceRecon_pytorch
- video_coeffs, full_coeffs = [], []
- for idx in tqdm(range(len(frames_pil)), desc='3DMM Extraction In Video:'):
- frame = frames_pil[idx]
- W,H = frame.size
- lm1 = lm[idx].reshape([-1, 2])
-
- if np.mean(lm1) == -1:
- lm1 = (self.lm3d_std[:, :2]+1)/2.
- lm1 = np.concatenate(
- [lm1[:, :1]*W, lm1[:, 1:2]*H], 1
- )
- else:
- lm1[:, -1] = H - 1 - lm1[:, -1]
-
- trans_params, im1, lm1, _ = align_img(frame, lm1, self.lm3d_std)
-
- trans_params = np.array([float(item) for item in np.hsplit(trans_params, 5)]).astype(np.float32)
- im_t = torch.tensor(np.array(im1)/255., dtype=torch.float32).permute(2, 0, 1).to(self.device).unsqueeze(0)
-
- with torch.no_grad():
- full_coeff = self.net_recon(im_t)
- coeffs = split_coeff(full_coeff)
-
- pred_coeff = {key:coeffs[key].cpu().numpy() for key in coeffs}
-
- pred_coeff = np.concatenate([
- pred_coeff['exp'],
- pred_coeff['angle'],
- pred_coeff['trans'],
- trans_params[2:][None],
- ], 1)
- video_coeffs.append(pred_coeff)
- full_coeffs.append(full_coeff.cpu().numpy())
-
- semantic_npy = np.array(video_coeffs)[:,0]
-
- savemat(coeff_path, {'coeff_3dmm': semantic_npy, 'full_3dmm': np.array(full_coeffs)[0]})
-
- return coeff_path, png_path, original_size
\ No newline at end of file
diff --git a/spaces/801artistry/RVC801/rvc_for_realtime.py b/spaces/801artistry/RVC801/rvc_for_realtime.py
deleted file mode 100644
index 55070f668c385ba0a9ba50989b282448cd75e59b..0000000000000000000000000000000000000000
--- a/spaces/801artistry/RVC801/rvc_for_realtime.py
+++ /dev/null
@@ -1,297 +0,0 @@
-import faiss, torch, traceback, parselmouth, numpy as np, torchcrepe, torch.nn as nn, pyworld
-from fairseq import checkpoint_utils
-from lib.infer_pack.models import (
- SynthesizerTrnMs256NSFsid,
- SynthesizerTrnMs256NSFsid_nono,
- SynthesizerTrnMs768NSFsid,
- SynthesizerTrnMs768NSFsid_nono,
-)
-import os, sys
-from time import time as ttime
-import torch.nn.functional as F
-import scipy.signal as signal
-
-now_dir = os.getcwd()
-sys.path.append(now_dir)
-from configs.config import Config
-from multiprocessing import Manager as M
-
-mm = M()
-config = Config()
-
-
-class RVC:
- def __init__(
- self, key, pth_path, index_path, index_rate, n_cpu, inp_q, opt_q, device
- ) -> None:
- """
- 初始化
- """
- try:
- global config
- self.inp_q = inp_q
- self.opt_q = opt_q
- self.device = device
- self.f0_up_key = key
- self.time_step = 160 / 16000 * 1000
- self.f0_min = 50
- self.f0_max = 1100
- self.f0_mel_min = 1127 * np.log(1 + self.f0_min / 700)
- self.f0_mel_max = 1127 * np.log(1 + self.f0_max / 700)
- self.sr = 16000
- self.window = 160
- self.n_cpu = n_cpu
- if index_rate != 0:
- self.index = faiss.read_index(index_path)
- self.big_npy = self.index.reconstruct_n(0, self.index.ntotal)
- print("index search enabled")
- self.index_rate = index_rate
- models, _, _ = checkpoint_utils.load_model_ensemble_and_task(
- ["hubert_base.pt"],
- suffix="",
- )
- hubert_model = models[0]
- hubert_model = hubert_model.to(config.device)
- if config.is_half:
- hubert_model = hubert_model.half()
- else:
- hubert_model = hubert_model.float()
- hubert_model.eval()
- self.model = hubert_model
- cpt = torch.load(pth_path, map_location="cpu")
- self.tgt_sr = cpt["config"][-1]
- cpt["config"][-3] = cpt["weight"]["emb_g.weight"].shape[0]
- self.if_f0 = cpt.get("f0", 1)
- self.version = cpt.get("version", "v1")
- if self.version == "v1":
- if self.if_f0 == 1:
- self.net_g = SynthesizerTrnMs256NSFsid(
- *cpt["config"], is_half=config.is_half
- )
- else:
- self.net_g = SynthesizerTrnMs256NSFsid_nono(*cpt["config"])
- elif self.version == "v2":
- if self.if_f0 == 1:
- self.net_g = SynthesizerTrnMs768NSFsid(
- *cpt["config"], is_half=config.is_half
- )
- else:
- self.net_g = SynthesizerTrnMs768NSFsid_nono(*cpt["config"])
- del self.net_g.enc_q
- print(self.net_g.load_state_dict(cpt["weight"], strict=False))
- self.net_g.eval().to(device)
- if config.is_half:
- self.net_g = self.net_g.half()
- else:
- self.net_g = self.net_g.float()
- self.is_half = config.is_half
- except:
- print(traceback.format_exc())
-
- def get_f0_post(self, f0):
- f0_min = self.f0_min
- f0_max = self.f0_max
- f0_mel_min = 1127 * np.log(1 + f0_min / 700)
- f0_mel_max = 1127 * np.log(1 + f0_max / 700)
- f0bak = f0.copy()
- f0_mel = 1127 * np.log(1 + f0 / 700)
- f0_mel[f0_mel > 0] = (f0_mel[f0_mel > 0] - f0_mel_min) * 254 / (
- f0_mel_max - f0_mel_min
- ) + 1
- f0_mel[f0_mel <= 1] = 1
- f0_mel[f0_mel > 255] = 255
- f0_coarse = np.rint(f0_mel).astype(np.int_)
- return f0_coarse, f0bak
-
- def get_f0(self, x, f0_up_key, n_cpu, method="harvest"):
- n_cpu = int(n_cpu)
- if method == "crepe":
- return self.get_f0_crepe(x, f0_up_key)
- if method == "rmvpe":
- return self.get_f0_rmvpe(x, f0_up_key)
- if method == "pm":
- p_len = x.shape[0] // 160
- f0 = (
- parselmouth.Sound(x, 16000)
- .to_pitch_ac(
- time_step=0.01,
- voicing_threshold=0.6,
- pitch_floor=50,
- pitch_ceiling=1100,
- )
- .selected_array["frequency"]
- )
-
- pad_size = (p_len - len(f0) + 1) // 2
- if pad_size > 0 or p_len - len(f0) - pad_size > 0:
- print(pad_size, p_len - len(f0) - pad_size)
- f0 = np.pad(
- f0, [[pad_size, p_len - len(f0) - pad_size]], mode="constant"
- )
-
- f0 *= pow(2, f0_up_key / 12)
- return self.get_f0_post(f0)
- if n_cpu == 1:
- f0, t = pyworld.harvest(
- x.astype(np.double),
- fs=16000,
- f0_ceil=1100,
- f0_floor=50,
- frame_period=10,
- )
- f0 = signal.medfilt(f0, 3)
- f0 *= pow(2, f0_up_key / 12)
- return self.get_f0_post(f0)
- f0bak = np.zeros(x.shape[0] // 160, dtype=np.float64)
- length = len(x)
- part_length = int(length / n_cpu / 160) * 160
- ts = ttime()
- res_f0 = mm.dict()
- for idx in range(n_cpu):
- tail = part_length * (idx + 1) + 320
- if idx == 0:
- self.inp_q.put((idx, x[:tail], res_f0, n_cpu, ts))
- else:
- self.inp_q.put(
- (idx, x[part_length * idx - 320 : tail], res_f0, n_cpu, ts)
- )
- while 1:
- res_ts = self.opt_q.get()
- if res_ts == ts:
- break
- f0s = [i[1] for i in sorted(res_f0.items(), key=lambda x: x[0])]
- for idx, f0 in enumerate(f0s):
- if idx == 0:
- f0 = f0[:-3]
- elif idx != n_cpu - 1:
- f0 = f0[2:-3]
- else:
- f0 = f0[2:-1]
- f0bak[
- part_length * idx // 160 : part_length * idx // 160 + f0.shape[0]
- ] = f0
- f0bak = signal.medfilt(f0bak, 3)
- f0bak *= pow(2, f0_up_key / 12)
- return self.get_f0_post(f0bak)
-
- def get_f0_crepe(self, x, f0_up_key):
- audio = torch.tensor(np.copy(x))[None].float()
- f0, pd = torchcrepe.predict(
- audio,
- self.sr,
- 160,
- self.f0_min,
- self.f0_max,
- "full",
- batch_size=512,
- device=self.device,
- return_periodicity=True,
- )
- pd = torchcrepe.filter.median(pd, 3)
- f0 = torchcrepe.filter.mean(f0, 3)
- f0[pd < 0.1] = 0
- f0 = f0[0].cpu().numpy()
- f0 *= pow(2, f0_up_key / 12)
- return self.get_f0_post(f0)
-
- def get_f0_rmvpe(self, x, f0_up_key):
- if hasattr(self, "model_rmvpe") == False:
- from infer.lib.rmvpe import RMVPE
-
- print("loading rmvpe model")
- self.model_rmvpe = RMVPE(
- "rmvpe.pt", is_half=self.is_half, device=self.device
- )
- # self.model_rmvpe = RMVPE("aug2_58000_half.pt", is_half=self.is_half, device=self.device)
- f0 = self.model_rmvpe.infer_from_audio(x, thred=0.03)
- f0 *= pow(2, f0_up_key / 12)
- return self.get_f0_post(f0)
-
- def infer(
- self,
- feats: torch.Tensor,
- indata: np.ndarray,
- rate1,
- rate2,
- cache_pitch,
- cache_pitchf,
- f0method,
- ) -> np.ndarray:
- feats = feats.view(1, -1)
- if config.is_half:
- feats = feats.half()
- else:
- feats = feats.float()
- feats = feats.to(self.device)
- t1 = ttime()
- with torch.no_grad():
- padding_mask = torch.BoolTensor(feats.shape).to(self.device).fill_(False)
- inputs = {
- "source": feats,
- "padding_mask": padding_mask,
- "output_layer": 9 if self.version == "v1" else 12,
- }
- logits = self.model.extract_features(**inputs)
- feats = (
- self.model.final_proj(logits[0]) if self.version == "v1" else logits[0]
- )
- t2 = ttime()
- try:
- if hasattr(self, "index") and self.index_rate != 0:
- leng_replace_head = int(rate1 * feats[0].shape[0])
- npy = feats[0][-leng_replace_head:].cpu().numpy().astype("float32")
- score, ix = self.index.search(npy, k=8)
- weight = np.square(1 / score)
- weight /= weight.sum(axis=1, keepdims=True)
- npy = np.sum(self.big_npy[ix] * np.expand_dims(weight, axis=2), axis=1)
- if config.is_half:
- npy = npy.astype("float16")
- feats[0][-leng_replace_head:] = (
- torch.from_numpy(npy).unsqueeze(0).to(self.device) * self.index_rate
- + (1 - self.index_rate) * feats[0][-leng_replace_head:]
- )
- else:
- print("index search FAIL or disabled")
- except:
- traceback.print_exc()
- print("index search FAIL")
- feats = F.interpolate(feats.permute(0, 2, 1), scale_factor=2).permute(0, 2, 1)
- t3 = ttime()
- if self.if_f0 == 1:
- pitch, pitchf = self.get_f0(indata, self.f0_up_key, self.n_cpu, f0method)
- cache_pitch[:] = np.append(cache_pitch[pitch[:-1].shape[0] :], pitch[:-1])
- cache_pitchf[:] = np.append(
- cache_pitchf[pitchf[:-1].shape[0] :], pitchf[:-1]
- )
- p_len = min(feats.shape[1], 13000, cache_pitch.shape[0])
- else:
- cache_pitch, cache_pitchf = None, None
- p_len = min(feats.shape[1], 13000)
- t4 = ttime()
- feats = feats[:, :p_len, :]
- if self.if_f0 == 1:
- cache_pitch = cache_pitch[:p_len]
- cache_pitchf = cache_pitchf[:p_len]
- cache_pitch = torch.LongTensor(cache_pitch).unsqueeze(0).to(self.device)
- cache_pitchf = torch.FloatTensor(cache_pitchf).unsqueeze(0).to(self.device)
- p_len = torch.LongTensor([p_len]).to(self.device)
- ii = 0 # sid
- sid = torch.LongTensor([ii]).to(self.device)
- with torch.no_grad():
- if self.if_f0 == 1:
- infered_audio = (
- self.net_g.infer(
- feats, p_len, cache_pitch, cache_pitchf, sid, rate2
- )[0][0, 0]
- .data.cpu()
- .float()
- )
- else:
- infered_audio = (
- self.net_g.infer(feats, p_len, sid, rate2)[0][0, 0]
- .data.cpu()
- .float()
- )
- t5 = ttime()
- print("time->fea-index-f0-model:", t2 - t1, t3 - t2, t4 - t3, t5 - t4)
- return infered_audio
diff --git a/spaces/AIFILMS/StyleGANEX/app.py b/spaces/AIFILMS/StyleGANEX/app.py
deleted file mode 100644
index 022debd0728451b2b850833b5e6640ade823e428..0000000000000000000000000000000000000000
--- a/spaces/AIFILMS/StyleGANEX/app.py
+++ /dev/null
@@ -1,124 +0,0 @@
-from __future__ import annotations
-
-import argparse
-import pathlib
-import torch
-import gradio as gr
-
-import os
-
-from webUI.app_task import *
-from webUI.styleganex_model import Model
-
-def parse_args() -> argparse.Namespace:
- parser = argparse.ArgumentParser()
- parser.add_argument('--device', type=str, default='cpu')
- parser.add_argument('--theme', type=str)
- parser.add_argument('--share', action='store_true')
- parser.add_argument('--port', type=int)
- parser.add_argument('--disable-queue',
- dest='enable_queue',
- action='store_false')
- return parser.parse_args()
-
-is_shared_ui = True if "AIFILMS/StyleGANEX" in os.environ['SPACE_ID'] else False
-
-DESCRIPTION = '''
-
-
- Face Manipulation with StyleGANEX
-
-
For faster inference without waiting in queue, you may duplicate the space and upgrade to GPU in settings.
-
-
-
-
-'''
-ARTICLE = r"""
-If StyleGANEX is helpful, please help to ⭐ the Github Repo . Thanks!
-[](https://github.com/williamyang1991/StyleGANEX)
----
-📝 **Citation**
-If our work is useful for your research, please consider citing:
-```bibtex
-@article{yang2023styleganex,
- title = {StyleGANEX: StyleGAN-Based Manipulation Beyond Cropped Aligned Faces},
- author = {Yang, Shuai and Jiang, Liming and Liu, Ziwei and and Loy, Chen Change},
- journal = {arXiv preprint arXiv:2303.06146},
- year={2023},
-}
-```
-📋 **License**
-This project is licensed under S-Lab License 1.0 .
-Redistribution and use for non-commercial purposes should follow this license.
-
-📧 **Contact**
-If you have any questions, please feel free to reach me out at williamyang@pku.edu.cn .
-"""
-
-FOOTER = ''
-
-def main():
- args = parse_args()
- args.device = 'cuda' if torch.cuda.is_available() else 'cpu'
- print('*** Now using %s.'%(args.device))
- model = Model(device=args.device)
-
-
- torch.hub.download_url_to_file('https://raw.githubusercontent.com/williamyang1991/StyleGANEX/main/data/234_sketch.jpg',
- '234_sketch.jpg')
- torch.hub.download_url_to_file('https://github.com/williamyang1991/StyleGANEX/raw/main/output/ILip77SbmOE_inversion.pt',
- 'ILip77SbmOE_inversion.pt')
- torch.hub.download_url_to_file('https://raw.githubusercontent.com/williamyang1991/StyleGANEX/main/data/ILip77SbmOE.png',
- 'ILip77SbmOE.png')
- torch.hub.download_url_to_file('https://raw.githubusercontent.com/williamyang1991/StyleGANEX/main/data/ILip77SbmOE_mask.png',
- 'ILip77SbmOE_mask.png')
- torch.hub.download_url_to_file('https://raw.githubusercontent.com/williamyang1991/StyleGANEX/main/data/pexels-daniel-xavier-1239291.jpg',
- 'pexels-daniel-xavier-1239291.jpg')
- torch.hub.download_url_to_file('https://github.com/williamyang1991/StyleGANEX/raw/main/data/529_2.mp4',
- '529_2.mp4')
- torch.hub.download_url_to_file('https://github.com/williamyang1991/StyleGANEX/raw/main/data/684.mp4',
- '684.mp4')
- torch.hub.download_url_to_file('https://github.com/williamyang1991/StyleGANEX/raw/main/data/pexels-anthony-shkraba-production-8136210.mp4',
- 'pexels-anthony-shkraba-production-8136210.mp4')
-
-
- with gr.Blocks(css='style.css') as demo:
- if(is_shared_ui):
- with gr.Box():
- top_description = gr.HTML(f'''
-
-
Attention - This Space doesn't work in this shared UI
-
For it to work, you can access the original or duplicate this Space and run it on your own profile using a GPU.
-
- ''')
- gr.Markdown(DESCRIPTION)
- with gr.Tabs():
- with gr.TabItem('Inversion for Editing'):
- create_demo_inversion(model.process_inversion, allow_optimization=False)
- with gr.TabItem('Image Face Toonify'):
- create_demo_toonify(model.process_toonify)
- with gr.TabItem('Video Face Toonify'):
- create_demo_vtoonify(model.process_vtoonify, max_frame_num=12)
- with gr.TabItem('Image Face Editing'):
- create_demo_editing(model.process_editing)
- with gr.TabItem('Video Face Editing'):
- create_demo_vediting(model.process_vediting, max_frame_num=12)
- with gr.TabItem('Sketch2Face'):
- create_demo_s2f(model.process_s2f)
- with gr.TabItem('Mask2Face'):
- create_demo_m2f(model.process_m2f)
- with gr.TabItem('SR'):
- create_demo_sr(model.process_sr)
- gr.Markdown(ARTICLE)
- gr.Markdown(FOOTER)
-
- demo.launch(
- enable_queue=args.enable_queue,
- server_port=args.port,
- share=args.share,
- )
-
-if __name__ == '__main__':
- main()
-
diff --git a/spaces/AIFILMS/StyleGANEX/configs/__init__.py b/spaces/AIFILMS/StyleGANEX/configs/__init__.py
deleted file mode 100644
index e69de29bb2d1d6434b8b29ae775ad8c2e48c5391..0000000000000000000000000000000000000000
diff --git a/spaces/AIKey/ai_date/style.css b/spaces/AIKey/ai_date/style.css
deleted file mode 100644
index 114adf441e9032febb46bc056b2a8bb651075f0d..0000000000000000000000000000000000000000
--- a/spaces/AIKey/ai_date/style.css
+++ /dev/null
@@ -1,28 +0,0 @@
-body {
- padding: 2rem;
- font-family: -apple-system, BlinkMacSystemFont, "Arial", sans-serif;
-}
-
-h1 {
- font-size: 16px;
- margin-top: 0;
-}
-
-p {
- color: rgb(107, 114, 128);
- font-size: 15px;
- margin-bottom: 10px;
- margin-top: 5px;
-}
-
-.card {
- max-width: 620px;
- margin: 0 auto;
- padding: 16px;
- border: 1px solid lightgray;
- border-radius: 16px;
-}
-
-.card p:last-child {
- margin-bottom: 0;
-}
diff --git a/spaces/AIZero2HeroBootcamp/AnimatedGifGallery/app.py b/spaces/AIZero2HeroBootcamp/AnimatedGifGallery/app.py
deleted file mode 100644
index ab7cb9583171b765412463f9c8d16b14f2a25d59..0000000000000000000000000000000000000000
--- a/spaces/AIZero2HeroBootcamp/AnimatedGifGallery/app.py
+++ /dev/null
@@ -1,52 +0,0 @@
-import streamlit as st
-import os
-import random
-
-def get_gifs(directory):
- return [f for f in os.listdir(directory) if f.endswith('.gif')]
-
-def showAnimatedGif(gif):
- import streamlit as st
- import base64
- #st.markdown("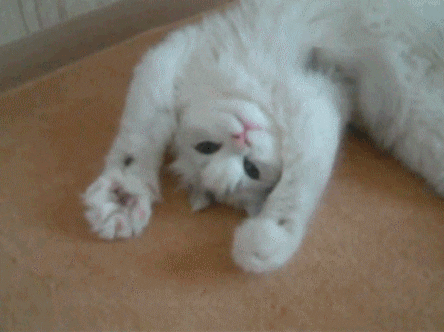")
- st.write('Loading: ' + gif)
- file_ = open(gif, "rb")
- contents = file_.read()
- data_url = base64.b64encode(contents).decode("utf-8")
- file_.close()
- st.write(data_url)
-
- st.markdown(
- f' ',
- unsafe_allow_html=True,
- )
-
-def main():
- st.title('Animated GIFs in Streamlit')
-
- directory = './gifs' # Replace with your directory of GIFs
- gif_files = get_gifs(directory)
-
- num_rows = len(gif_files) // 3
- if len(gif_files) % 3:
- num_rows += 1
-
- cols = [st.columns(3) for _ in range(num_rows)]
-
- for i in range(num_rows):
- for j in range(3):
- idx = i*3 + j
- if idx < len(gif_files):
- #showAnimatedGif(os.path.join(directory, gif_files[idx]))
- cols[i][j].image(os.path.join(directory, gif_files[idx]), width=200)
-
- if st.button('Randomize'):
- random.shuffle(gif_files)
- for i in range(num_rows):
- for j in range(3):
- idx = i*3 + j
- if idx < len(gif_files):
- cols[i][j].image(os.path.join(directory, gif_files[idx]), width=200)
-
-if __name__ == "__main__":
- main()
diff --git a/spaces/ARTeLab/ARTeLab-SummIT/README.md b/spaces/ARTeLab/ARTeLab-SummIT/README.md
deleted file mode 100644
index bb93ae203d08bbe8ce08d83803038a4933b63148..0000000000000000000000000000000000000000
--- a/spaces/ARTeLab/ARTeLab-SummIT/README.md
+++ /dev/null
@@ -1,30 +0,0 @@
----
-title: ARTeLab SummIT
-emoji: 📰
-colorFrom: indigo
-colorTo: green
-sdk: streamlit
-app_file: app.py
-pinned: false
----
-# Configuration
-`title`: _string_
-Display title for the Space
-`emoji`: _string_
-Space emoji (emoji-only character allowed)
-`colorFrom`: _string_
-Color for Thumbnail gradient (red, yellow, green, blue, indigo, purple, pink, gray)
-`colorTo`: _string_
-Color for Thumbnail gradient (red, yellow, green, blue, indigo, purple, pink, gray)
-`sdk`: _string_
-Can be either `gradio` or `streamlit`
-`sdk_version` : _string_
-Only applicable for `streamlit` SDK.
-See [doc](https://hf.co/docs/hub/spaces) for more info on supported versions.
-
-`app_file`: _string_
-Path to your main application file (which contains either `gradio` or `streamlit` Python code).
-Path is relative to the root of the repository.
-
-`pinned`: _boolean_
-Whether the Space stays on top of your list.
\ No newline at end of file
diff --git a/spaces/AbandonedMuse/UnlimitedMusicGen/audiocraft/modules/transformer.py b/spaces/AbandonedMuse/UnlimitedMusicGen/audiocraft/modules/transformer.py
deleted file mode 100644
index e69cca829d774d0b8b36c0de9b7924373da81b43..0000000000000000000000000000000000000000
--- a/spaces/AbandonedMuse/UnlimitedMusicGen/audiocraft/modules/transformer.py
+++ /dev/null
@@ -1,747 +0,0 @@
-# Copyright (c) Meta Platforms, Inc. and affiliates.
-# All rights reserved.
-#
-# This source code is licensed under the license found in the
-# LICENSE file in the root directory of this source tree.
-
-"""
-Transformer model, with streaming support, xformer attention support
-and easy causal attention with a potentially finite receptive field.
-
-See `StreamingTransformer` for more information.
-
-Unlike regular PyTorch Transformer, we make the hard choice that batches are first.
-"""
-
-import typing as tp
-
-from einops import rearrange
-import torch
-import torch.nn as nn
-from torch.nn import functional as F
-from torch.utils.checkpoint import checkpoint as torch_checkpoint
-from xformers import ops
-
-from .rope import RotaryEmbedding
-from .streaming import StreamingModule
-
-_efficient_attention_backend: str = 'torch'
-
-
-def set_efficient_attention_backend(backend: str = 'torch'):
- # Using torch by default, it seems a bit faster on older P100 GPUs (~20% faster).
- global _efficient_attention_backend
- assert _efficient_attention_backend in ['xformers', 'torch']
- _efficient_attention_backend = backend
-
-
-def _get_attention_time_dimension() -> int:
- if _efficient_attention_backend == 'torch':
- return 2
- else:
- return 1
-
-
-def _is_profiled() -> bool:
- # Return true if we are currently running with a xformers profiler activated.
- try:
- from xformers.profiler import profiler
- except ImportError:
- return False
- return profiler._Profiler._CURRENT_PROFILER is not None
-
-
-def create_norm_fn(norm_type: str, dim: int, **kwargs) -> nn.Module:
- """Create normalization module for transformer encoder layer.
-
- Args:
- norm_type (str): Normalization method.
- dim (int): Dimension of the normalized layer.
- **kwargs (dict): Additional parameters for normalization layer.
- Returns:
- nn.Module: Normalization module.
- """
- if norm_type == 'layer_norm':
- return nn.LayerNorm(dim, eps=1e-5, **kwargs)
- else:
- raise ValueError(f"Unknown norm type: {norm_type}")
-
-
-def create_sin_embedding(positions: torch.Tensor, dim: int, max_period: float = 10000,
- dtype: torch.dtype = torch.float32) -> torch.Tensor:
- """Create sinusoidal positional embedding, with shape `[B, T, C]`.
-
- Args:
- positions (torch.Tensor): LongTensor of positions.
- dim (int): Dimension of the embedding.
- max_period (float): Maximum period of the cosine/sine functions.
- dtype (torch.dtype or str): dtype to use to generate the embedding.
- Returns:
- torch.Tensor: Sinusoidal positional embedding.
- """
- # We aim for BTC format
- assert dim % 2 == 0
- half_dim = dim // 2
- positions = positions.to(dtype)
- adim = torch.arange(half_dim, device=positions.device, dtype=dtype).view(1, 1, -1)
- max_period_tensor = torch.full([], max_period, device=positions.device, dtype=dtype) # avoid sync point
- phase = positions / (max_period_tensor ** (adim / (half_dim - 1)))
- return torch.cat([torch.cos(phase), torch.sin(phase)], dim=-1)
-
-
-def expand_repeated_kv(x: torch.Tensor, n_rep: int) -> torch.Tensor:
- """torch.repeat_interleave(x, dim=2, repeats=n_rep) from xlformers"""
- if n_rep == 1:
- return x
- if _efficient_attention_backend == 'torch':
- bs, n_kv_heads, slen, head_dim = x.shape
- return (
- x[:, :, None, :, :]
- .expand(bs, n_kv_heads, n_rep, slen, head_dim)
- .reshape(bs, n_kv_heads * n_rep, slen, head_dim)
- )
- else:
- bs, slen, n_kv_heads, head_dim = x.shape
- return (
- x[:, :, :, None, :]
- .expand(bs, slen, n_kv_heads, n_rep, head_dim)
- .reshape(bs, slen, n_kv_heads * n_rep, head_dim)
- )
-
-
-class LayerScale(nn.Module):
- """Layer scale from [Touvron et al 2021] (https://arxiv.org/pdf/2103.17239.pdf).
- This rescales diagonaly the residual outputs close to 0, with a learnt scale.
-
- Args:
- channels (int): Number of channels.
- init (float): Initial scale.
- channel_last (bool): If True, expect `[*, C]` shaped tensors, otherwise, `[*, C, T]`.
- device (torch.device or None): Device on which to initialize the module.
- dtype (torch.dtype or None): dtype to use to initialize the module.
- """
- def __init__(self, channels: int, init: float = 1e-4, channel_last: bool = True,
- device=None, dtype=None):
- super().__init__()
- self.channel_last = channel_last
- self.scale = nn.Parameter(
- torch.full((channels,), init,
- requires_grad=True, device=device, dtype=dtype))
-
- def forward(self, x: torch.Tensor):
- if self.channel_last:
- return self.scale * x
- else:
- return self.scale[:, None] * x
-
-
-class StreamingMultiheadAttention(StreamingModule):
- """Similar to `nn.MultiheadAttention` but with support for streaming, causal evaluation.
-
- Args:
- embed_dim (int): Dimension to project to.
- num_heads (int): Number of heads.
- dropout (float): Dropout level.
- bias (bool): Use bias in projections.
- causal (bool): Causal mask applied automatically.
- past_context (int or None): Receptive field for the causal mask, infinite if None.
- custom (bool): Use custom MHA implementation, for testing / benchmarking.
- memory_efficient (bool): Use xformers based memory efficient attention.
- attention_as_float32 (bool): Perform the attention as float32
- (especially important with memory_efficient as autocast won't do this automatically).
- rope (`RotaryEmbedding` or None): Rope embedding to use.
- cross_attention: Should be true when used as a cross attention.
- All keys and values must be available at once, streaming is only for the queries.
- Cannot be used with `causal` or `rope` (as it wouldn't make sens to
- intepret the time steps in the keys relative to those in the queries).
- safe_streaming (bool): Bug fix, will go away with xformers update.
- qk_layer_norm (bool): Layer normalization applied to queries and keys before dot product.
- kv_repeat (int): If > 1, will repeat keys and queries multiple times (need to divide num_heads).
- This will lead to faster decoding time on A100 or other GPUs with tensorcore.
- device (torch.device or None): Sevice on which to initialize.
- dtype (torch.dtype or None): dtype to use.
- """
- def __init__(self, embed_dim: int, num_heads: int, dropout: float = 0.0, bias: bool = True,
- causal: bool = False, past_context: tp.Optional[int] = None, custom: bool = False,
- memory_efficient: bool = False, attention_as_float32: bool = False,
- rope: tp.Optional[RotaryEmbedding] = None, cross_attention: bool = False,
- safe_streaming: bool = True, qk_layer_norm: bool = False, kv_repeat: int = 1,
- device=None, dtype=None):
- super().__init__()
- factory_kwargs = {'device': device, 'dtype': dtype}
- if past_context is not None:
- assert causal
-
- self.embed_dim = embed_dim
- self.causal = causal
- self.past_context = past_context
- self.memory_efficient = memory_efficient
- self.attention_as_float32 = attention_as_float32
- self.rope = rope
- self.cross_attention = cross_attention
- self.safe_streaming = safe_streaming
- self.num_heads = num_heads
- self.dropout = dropout
- self.kv_repeat = kv_repeat
- if cross_attention:
- assert not causal, "Causal cannot work with cross attention."
- assert rope is None, "Rope cannot work with cross attention."
-
- if memory_efficient:
- _verify_xformers_memory_efficient_compat()
-
- self.custom = _is_custom(custom, memory_efficient)
- if self.custom:
- out_dim = embed_dim
- assert num_heads % kv_repeat == 0
- assert not cross_attention or kv_repeat == 1
- num_kv = num_heads // kv_repeat
- kv_dim = (embed_dim // num_heads) * num_kv
- out_dim += 2 * kv_dim
- in_proj = nn.Linear(embed_dim, out_dim, bias=bias, **factory_kwargs)
- # We try to follow the default PyTorch MHA convention, to easily compare results.
- self.in_proj_weight = in_proj.weight
- self.in_proj_bias = in_proj.bias
- if bias:
- self.in_proj_bias.data.zero_() # Following Pytorch convention
- self.out_proj = nn.Linear(embed_dim, embed_dim, bias=bias, **factory_kwargs)
- if bias:
- self.out_proj.bias.data.zero_()
- else:
- assert not qk_layer_norm
- assert kv_repeat == 1
- self.mha = nn.MultiheadAttention(
- embed_dim, num_heads, dropout=dropout, bias=bias, batch_first=True,
- **factory_kwargs)
- self.qk_layer_norm = qk_layer_norm
- if qk_layer_norm:
- assert self.custom
- assert kv_repeat == 1
- ln_dim = embed_dim
- self.q_layer_norm = nn.LayerNorm(ln_dim)
- self.k_layer_norm = nn.LayerNorm(ln_dim)
-
- def _load_from_state_dict(self, state_dict, prefix, *args, **kwargs):
- if not self.custom:
- # Support compat with regular MHA
- keys = [n for n, _ in self.mha.named_parameters()]
- for key in keys:
- if prefix + key in state_dict:
- state_dict[prefix + "mha." + key] = state_dict.pop(prefix + key)
- super()._load_from_state_dict(state_dict, prefix, *args, **kwargs)
-
- def _get_mask(self, current_steps: int, device: torch.device, dtype: torch.dtype):
- # Return a causal mask, accounting for potentially stored past keys/values
- # We actually return a bias for the attention score, as this has the same
- # convention both in the builtin MHA in Pytorch, and Xformers functions.
- time_dim = _get_attention_time_dimension()
- if self.memory_efficient:
- from xformers.ops import LowerTriangularMask
- if current_steps == 1:
- # If we only have one step, then we do not need a mask.
- return None
- elif 'past_keys' in self._streaming_state:
- raise RuntimeError('Not supported at the moment')
- else:
- # Then we can safely use a lower triangular mask
- return LowerTriangularMask()
- if self._streaming_state:
- past_keys = self._streaming_state['past_keys']
- past_steps = past_keys.shape[time_dim]
- else:
- past_steps = 0
-
- queries_pos = torch.arange(
- past_steps, current_steps + past_steps, device=device).view(-1, 1)
- keys_pos = torch.arange(past_steps + current_steps, device=device).view(1, -1)
- delta = queries_pos - keys_pos
- valid = delta >= 0
- if self.past_context is not None:
- valid &= (delta <= self.past_context)
- return torch.where(
- valid,
- torch.zeros([], device=device, dtype=dtype),
- torch.full([], float('-inf'), device=device, dtype=dtype))
-
- def _complete_kv(self, k, v):
- time_dim = _get_attention_time_dimension()
- if self.cross_attention:
- # With cross attention we assume all keys and values
- # are already available, and streaming is with respect
- # to the queries only.
- return k, v
- # Complete the key/value pair using the streaming state.
- if self._streaming_state:
- pk = self._streaming_state['past_keys']
- nk = torch.cat([pk, k], dim=time_dim)
- if v is k:
- nv = nk
- else:
- pv = self._streaming_state['past_values']
- nv = torch.cat([pv, v], dim=time_dim)
- else:
- nk = k
- nv = v
-
- assert nk.shape[time_dim] == nv.shape[time_dim]
- offset = 0
- if self.past_context is not None:
- offset = max(0, nk.shape[time_dim] - self.past_context)
- if self._is_streaming:
- self._streaming_state['past_keys'] = nk[:, offset:]
- if v is not k:
- self._streaming_state['past_values'] = nv[:, offset:]
- if 'offset' in self._streaming_state:
- self._streaming_state['offset'] += offset
- else:
- self._streaming_state['offset'] = torch.tensor(0)
- return nk, nv
-
- def _apply_rope(self, query: torch.Tensor, key: torch.Tensor):
- # TODO: fix and verify layout.
- assert _efficient_attention_backend == 'xformers', 'Rope not supported with torch attn.'
- # Apply rope embeddings to query and key tensors.
- assert self.rope is not None
- if 'past_keys' in self._streaming_state:
- past_keys_offset = self._streaming_state['past_keys'].shape[1]
- else:
- past_keys_offset = 0
- if 'offset' in self._streaming_state:
- past_context_offset = int(self._streaming_state['offset'].item())
- else:
- past_context_offset = 0
- streaming_offset = past_context_offset + past_keys_offset
- return self.rope.rotate_qk(query, key, start=streaming_offset)
-
- def forward(self, query: torch.Tensor, key: torch.Tensor, value: torch.Tensor,
- key_padding_mask=None, need_weights=False, attn_mask=None,
- average_attn_weights=True, is_causal=False):
- assert attn_mask is None
- assert not is_causal, ("new param added in torch 2.0.1 not supported, "
- "use the causal args in the constructor.")
-
- time_dim = _get_attention_time_dimension()
- if time_dim == 2:
- layout = "b h t d"
- else:
- layout = "b t h d"
- dtype = query.dtype
- if self._is_streaming:
- assert self.causal or self.cross_attention, \
- "Streaming only available for causal or cross attention"
-
- if self.causal:
- # At the moment we specialize only for the self-attention case.
- assert query.shape[1] == key.shape[1], "Causal only for same length query / key / value"
- assert value.shape[1] == key.shape[1], "Causal only for same length query / key / value"
- attn_mask = self._get_mask(query.shape[1], query.device, query.dtype)
-
- if self.custom:
- # custom implementation
- assert need_weights is False
- assert key_padding_mask is None
- if self.cross_attention:
- # Different queries, keys, values, we have to spit manually the weights
- # before applying the linear.
- dim = self.in_proj_weight.shape[0] // 3
- if self.in_proj_bias is None:
- bias_q, bias_k, bias_v = None, None, None
- else:
- bias_q = self.in_proj_bias[:dim]
- bias_k = self.in_proj_bias[dim: 2 * dim]
- bias_v = self.in_proj_bias[2 * dim:]
- q = nn.functional.linear(query, self.in_proj_weight[:dim], bias_q)
- # todo: when streaming, we could actually save k, v and check the shape actually match.
- k = nn.functional.linear(key, self.in_proj_weight[dim: 2 * dim], bias_k)
- v = nn.functional.linear(value, self.in_proj_weight[2 * dim:], bias_v)
- if self.qk_layer_norm is True:
- q = self.q_layer_norm(q)
- k = self.k_layer_norm(k)
- q, k, v = [rearrange(x, f"b t (h d) -> {layout}", h=self.num_heads) for x in [q, k, v]]
- else:
- if not _is_profiled():
- # profiling breaks that propertysomehow.
- assert query is key, "specialized implementation"
- assert value is key, "specialized implementation"
- projected = nn.functional.linear(query, self.in_proj_weight, self.in_proj_bias)
- if self.kv_repeat == 1:
- if time_dim == 2:
- bound_layout = "b h p t d"
- else:
- bound_layout = "b t p h d"
- packed = rearrange(projected, f"b t (p h d) -> {bound_layout}", p=3, h=self.num_heads)
- q, k, v = ops.unbind(packed, dim=2)
- else:
- embed_dim = self.embed_dim
- per_head_dim = (embed_dim // self.num_heads)
- kv_heads = self.num_heads // self.kv_repeat
- q = projected[:, :, :embed_dim]
- start = embed_dim
- end = start + per_head_dim * kv_heads
- k = projected[:, :, start: end]
- v = projected[:, :, end:]
- q = rearrange(q, f"b t (h d) -> {layout}", h=self.num_heads)
- k = rearrange(k, f"b t (h d) -> {layout}", h=kv_heads)
- v = rearrange(v, f"b t (h d) -> {layout}", h=kv_heads)
-
- if self.qk_layer_norm is True:
- assert self.kv_repeat == 1
- q, k = [rearrange(x, f"{layout} -> b t (h d)") for x in [q, k]]
- q = self.q_layer_norm(q)
- k = self.k_layer_norm(k)
- q, k = [rearrange(x, f"b t (h d) -> {layout}", h=self.num_heads) for x in [q, k]]
- if self.rope:
- q, k = self._apply_rope(q, k)
- k, v = self._complete_kv(k, v)
- if self.kv_repeat > 1:
- k = expand_repeated_kv(k, self.kv_repeat)
- v = expand_repeated_kv(v, self.kv_repeat)
- if self.attention_as_float32:
- q, k, v = [x.float() for x in [q, k, v]]
- if self.memory_efficient:
- p = self.dropout if self.training else 0
- if _efficient_attention_backend == 'torch':
- x = torch.nn.functional.scaled_dot_product_attention(
- q, k, v, is_causal=attn_mask is not None, dropout_p=p)
- else:
- x = ops.memory_efficient_attention(q, k, v, attn_mask, p=p)
- else:
- # We include the dot product as float32, for consistency
- # with the other implementations that include that step
- # as part of the attention. Note that when using `autocast`,
- # the einsums would be done as bfloat16, but the softmax
- # would be done as bfloat16, so `attention_as_float32` will
- # extend a bit the range of operations done in float32,
- # although this should make no difference.
- q = q / q.shape[-1] ** 0.5
- key_layout = layout.replace('t', 'k')
- query_layout = layout
- if self._is_streaming and self.safe_streaming and q.device.type == 'cuda':
- with torch.autocast(device_type=q.device.type, dtype=torch.float32):
- pre_w = torch.einsum(f"{query_layout},{key_layout}-> b h t k", q, k)
- else:
- pre_w = torch.einsum(f"{query_layout},{key_layout}-> b h t k", q, k)
- if attn_mask is not None:
- pre_w = pre_w + attn_mask
- w = torch.softmax(pre_w, dim=-1)
- w = F.dropout(w, self.dropout, training=self.training).to(v)
- # Key and value have the same format.
- x = torch.einsum(f"b h t k, {key_layout} -> {layout}", w, v)
- x = x.to(dtype)
- x = rearrange(x, f"{layout} -> b t (h d)", h=self.num_heads)
- x = self.out_proj(x)
- else:
- key, value = self._complete_kv(key, value)
- if self.attention_as_float32:
- query, key, value = [x.float() for x in [query, key, value]]
- x, _ = self.mha(
- query, key, value, key_padding_mask,
- need_weights, attn_mask, average_attn_weights)
- x = x.to(dtype)
-
- return x, None
-
-
-class StreamingTransformerLayer(nn.TransformerEncoderLayer):
- """TransformerLayer with Streaming / Causal support.
- This also integrates cross_attention, when passing `cross_attention=True`,
- rather than having two separate classes like in PyTorch.
-
- Args:
- d_model (int): Dimension of the data.
- num_heads (int): Number of heads.
- dim_feedforward (int): Intermediate dimension of FF module.
- dropout (float): Dropout both for MHA and FF.
- bias_ff (bool): Use bias for FF.
- bias_attn (bool): Use bias for MHA.
- causal (bool): Causal mask applied automatically.
- past_context (int or None): Receptive field for the causal mask, infinite if None.
- custom (bool): Use custom MHA implementation, for testing / benchmarking.
- memory_efficient (bool): Use xformers based memory efficient attention.
- attention_as_float32 (bool): Perform the attention as float32
- (especially important with memory_efficient as autocast won't do this automatically).
- qk_layer_norm (bool): Layer normalization applied to queries and keys before dot product in attention.
- qk_layer_norm_cross (bool): Same for the cross attention.
- cross_attention (bool): If True, expect to get secondary input for cross-attention.
- Cross attention will use the default MHA, as it typically won't require
- special treatment.
- layer_scale (float or None): If not None, LayerScale will be used with
- the given value as initial scale.
- rope (`RotaryEmbedding` or None): Rope embedding to use.
- attention_dropout (float or None): If not None, separate the value of the dimension dropout
- in FFN and of the attention dropout.
- kv_repeat (int): If > 1, will repeat keys and queries multiple times (need to divide num_heads).
- This will lead to faster decoding time on A100 or other GPUs with tensorcore.
- device (torch.device or None): Device on which to initialize.
- dtype (torch.dtype or None): dtype to use.
- **kwargs: See `nn.TransformerEncoderLayer`.
- """
- def __init__(self, d_model: int, num_heads: int, dim_feedforward: int = 2048, dropout: float = 0.1,
- bias_ff: bool = True, bias_attn: bool = True, causal: bool = False,
- past_context: tp.Optional[int] = None, custom: bool = False,
- memory_efficient: bool = False, attention_as_float32: bool = False,
- qk_layer_norm: bool = False, qk_layer_norm_cross: bool = False,
- cross_attention: bool = False, layer_scale: tp.Optional[float] = None,
- rope: tp.Optional[RotaryEmbedding] = None, attention_dropout: tp.Optional[float] = None,
- kv_repeat: int = 1, norm: str = 'layer_norm', device=None, dtype=None, **kwargs):
- super().__init__(d_model, num_heads, dim_feedforward, dropout,
- device=device, dtype=dtype, batch_first=True, **kwargs)
- factory_kwargs = {'device': device, 'dtype': dtype}
- # Redefine self_attn to our streaming multi-head attention
- attn_kwargs: tp.Dict[str, tp.Any] = {
- 'embed_dim': d_model,
- 'num_heads': num_heads,
- 'dropout': dropout if attention_dropout is None else attention_dropout,
- 'bias': bias_attn,
- 'custom': custom,
- 'memory_efficient': memory_efficient,
- 'attention_as_float32': attention_as_float32,
- }
- self.self_attn: StreamingMultiheadAttention = StreamingMultiheadAttention(
- causal=causal, past_context=past_context, rope=rope, qk_layer_norm=qk_layer_norm,
- kv_repeat=kv_repeat, **attn_kwargs, **factory_kwargs) # type: ignore
- # Redefine feedforward layers to expose bias parameter
- self.linear1 = nn.Linear(d_model, dim_feedforward, bias=bias_ff, **factory_kwargs)
- self.linear2 = nn.Linear(dim_feedforward, d_model, bias=bias_ff, **factory_kwargs)
-
- self.layer_scale_1: nn.Module
- self.layer_scale_2: nn.Module
- if layer_scale is None:
- self.layer_scale_1 = nn.Identity()
- self.layer_scale_2 = nn.Identity()
- else:
- self.layer_scale_1 = LayerScale(d_model, layer_scale, **factory_kwargs)
- self.layer_scale_2 = LayerScale(d_model, layer_scale, **factory_kwargs)
-
- self.cross_attention: tp.Optional[nn.Module] = None
- if cross_attention:
- self.cross_attention = StreamingMultiheadAttention(
- cross_attention=True, qk_layer_norm=qk_layer_norm_cross,
- **attn_kwargs, **factory_kwargs)
- # Norm and dropout
- self.dropout_cross = nn.Dropout(dropout)
- # eps value matching that used in PyTorch reference implementation.
- self.norm_cross = nn.LayerNorm(d_model, eps=1e-5, **factory_kwargs)
- self.layer_scale_cross: nn.Module
- if layer_scale is None:
- self.layer_scale_cross = nn.Identity()
- else:
- self.layer_scale_cross = LayerScale(d_model, layer_scale, **factory_kwargs)
- self.norm1 = create_norm_fn(norm, d_model, **factory_kwargs) # type: ignore
- self.norm2 = create_norm_fn(norm, d_model, **factory_kwargs) # type: ignore
-
- def _cross_attention_block(self, src: torch.Tensor,
- cross_attention_src: torch.Tensor) -> torch.Tensor:
- assert self.cross_attention is not None
- # queries are from src, keys and values from cross_attention_src.
- x = self.cross_attention(
- src, cross_attention_src, cross_attention_src, need_weights=False)[0]
- return self.dropout_cross(x) # type: ignore
-
- def forward(self, src: torch.Tensor, src_mask: tp.Optional[torch.Tensor] = None, # type: ignore
- src_key_padding_mask: tp.Optional[torch.Tensor] = None,
- cross_attention_src: tp.Optional[torch.Tensor] = None):
- if self.cross_attention is None:
- assert cross_attention_src is None
- else:
- assert cross_attention_src is not None
- x = src
- if self.norm_first:
- x = x + self.layer_scale_1(
- self._sa_block(self.norm1(x), src_mask, src_key_padding_mask))
- if cross_attention_src is not None:
- x = x + self.layer_scale_cross(
- self._cross_attention_block(
- self.norm_cross(x), cross_attention_src))
- x = x + self.layer_scale_2(self._ff_block(self.norm2(x)))
- else:
- x = self.norm1(x + self.layer_scale_1(
- self._sa_block(x, src_mask, src_key_padding_mask)))
- if cross_attention_src is not None:
- x = self.norm_cross(
- x + self.layer_scale_cross(
- self._cross_attention_block(src, cross_attention_src)))
- x = self.norm2(x + self.layer_scale_2(self._ff_block(x)))
- return x
-
-
-class StreamingTransformer(StreamingModule):
- """Transformer with Streaming / Causal support.
-
- Args:
- d_model (int): Dimension of the data.
- num_heads (int): Number of heads.
- dim_feedforward (int): Intermediate dimension of FF module.
- dropout (float): Dropout both for MHA and FF.
- bias_ff (bool): Use bias for FF.
- bias_attn (bool): Use bias for MHA.
- causal (bool): Causal mask applied automatically.
- past_context (int or None): Receptive field for the causal mask, infinite if None.
- custom (bool): Use custom MHA implementation, for testing / benchmarking.
- memory_efficient (bool): Use xformers based memory efficient attention.
- attention_as_float32 (bool): Perform the attention as float32
- (especially important with memory_efficient as autocast won't do this automatically).
- cross_attention (bool): If True, expect to get secondary input for cross-attention.
- layer_scale (float or None): If not None, LayerScale will be used
- with the given value as initial scale.
- positional_embedding (str): Positional embedding strategy (sin, rope, or sin_rope).
- max_period (float): Maximum period of the time embedding.
- positional_scale (float): Scale of positional embedding, set to 0 to deactivate.
- xpos (bool): Apply xpos exponential decay to positional embedding (rope only).
- lr (float or None): learning rate override through the `make_optim_group` API.
- weight_decay (float or None): Weight_decay override through the `make_optim_group` API.
- layer_class: (subclass of `StreamingTransformerLayer): class to use
- to initialize the layers, allowing further customization outside of Audiocraft.
- checkpointing (str): Checkpointing strategy to reduce memory usage.
- No checkpointing if set to 'none'. Per layer checkpointing using PyTorch
- if set to 'torch' (entire layer checkpointed, i.e. linears are evaluated twice,
- minimal memory usage, but maximal runtime). Finally, `xformers_default` provide
- a policy for opting-out some operations of the checkpointing like
- linear layers and attention, providing a middle ground between speed and memory.
- device (torch.device or None): Device on which to initialize.
- dtype (torch.dtype or None): dtype to use.
- **kwargs: See `nn.TransformerEncoderLayer`.
- """
- def __init__(self, d_model: int, num_heads: int, num_layers: int, dim_feedforward: int = 2048,
- dropout: float = 0.1, bias_ff: bool = True, bias_attn: bool = True,
- causal: bool = False, past_context: tp.Optional[int] = None,
- custom: bool = False, memory_efficient: bool = False, attention_as_float32: bool = False,
- cross_attention: bool = False, layer_scale: tp.Optional[float] = None,
- positional_embedding: str = 'sin', max_period: float = 10_000, positional_scale: float = 1.,
- xpos: bool = False, lr: tp.Optional[float] = None, weight_decay: tp.Optional[float] = None,
- layer_class: tp.Type[StreamingTransformerLayer] = StreamingTransformerLayer,
- checkpointing: str = 'none', device=None, dtype=None, **kwargs):
- super().__init__()
- assert d_model % num_heads == 0
-
- self.positional_embedding = positional_embedding
- self.max_period = max_period
- self.positional_scale = positional_scale
- self.weight_decay = weight_decay
- self.lr = lr
-
- assert positional_embedding in ['sin', 'rope', 'sin_rope']
- self.rope: tp.Optional[RotaryEmbedding] = None
- if self.positional_embedding in ['rope', 'sin_rope']:
- assert _is_custom(custom, memory_efficient)
- self.rope = RotaryEmbedding(d_model // num_heads, max_period=max_period,
- xpos=xpos, scale=positional_scale, device=device)
-
- self.checkpointing = checkpointing
-
- assert checkpointing in ['none', 'torch', 'xformers_default', 'xformers_mm']
- if self.checkpointing.startswith('xformers'):
- _verify_xformers_internal_compat()
-
- self.layers = nn.ModuleList()
- for idx in range(num_layers):
- self.layers.append(
- layer_class(
- d_model=d_model, num_heads=num_heads, dim_feedforward=dim_feedforward,
- dropout=dropout, bias_ff=bias_ff, bias_attn=bias_attn,
- causal=causal, past_context=past_context, custom=custom,
- memory_efficient=memory_efficient, attention_as_float32=attention_as_float32,
- cross_attention=cross_attention, layer_scale=layer_scale, rope=self.rope,
- device=device, dtype=dtype, **kwargs))
-
- if self.checkpointing != 'none':
- for layer in self.layers:
- # see audiocraft/optim/fsdp.py, magic signal to indicate this requires fixing the
- # backward hook inside of FSDP...
- layer._magma_checkpointed = True # type: ignore
- assert layer.layer_drop == 0., "Need further checking" # type: ignore
-
- def _apply_layer(self, layer, *args, **kwargs):
- method = self.checkpointing
- if method == 'none':
- return layer(*args, **kwargs)
- elif method == 'torch':
- return torch_checkpoint(layer, *args, use_reentrant=False, **kwargs)
- elif method.startswith('xformers'):
- from xformers.checkpoint_fairinternal import checkpoint, _get_default_policy
- if method == 'xformers_default':
- # those operations will be saved, and not recomputed.
- # According to Francisco we can get smarter policies but this is a good start.
- allow_list = [
- "xformers.efficient_attention_forward_cutlass.default",
- "xformers_flash.flash_fwd.default",
- "aten.addmm.default",
- "aten.mm.default",
- ]
- elif method == 'xformers_mm':
- # those operations will be saved, and not recomputed.
- # According to Francisco we can get smarter policies but this is a good start.
- allow_list = [
- "aten.addmm.default",
- "aten.mm.default",
- ]
- else:
- raise ValueError(f"xformers checkpointing xformers policy {method} is not known.")
- policy_fn = _get_default_policy(allow_list)
- return checkpoint(layer, *args, policy_fn=policy_fn, **kwargs)
- else:
- raise ValueError(f"Checkpointing method {method} is unknown.")
-
- def forward(self, x: torch.Tensor, *args, **kwargs):
- B, T, C = x.shape
-
- if 'offsets' in self._streaming_state:
- offsets = self._streaming_state['offsets']
- else:
- offsets = torch.zeros(B, dtype=torch.long, device=x.device)
-
- if self.positional_embedding in ['sin', 'sin_rope']:
- positions = torch.arange(T, device=x.device).view(1, -1, 1)
- positions = positions + offsets.view(-1, 1, 1)
- pos_emb = create_sin_embedding(positions, C, max_period=self.max_period, dtype=x.dtype)
- x = x + self.positional_scale * pos_emb
-
- for layer in self.layers:
- x = self._apply_layer(layer, x, *args, **kwargs)
-
- if self._is_streaming:
- self._streaming_state['offsets'] = offsets + T
-
- return x
-
- def make_optim_group(self):
- group = {"params": list(self.parameters())}
- if self.lr is not None:
- group["lr"] = self.lr
- if self.weight_decay is not None:
- group["weight_decay"] = self.weight_decay
- return group
-
-
-# special attention attention related function
-
-def _verify_xformers_memory_efficient_compat():
- try:
- from xformers.ops import memory_efficient_attention, LowerTriangularMask # noqa
- except ImportError:
- raise ImportError(
- "xformers is not installed. Please install it and try again.\n"
- "To install on AWS and Azure, run \n"
- "FORCE_CUDA=1 TORCH_CUDA_ARCH_LIST='8.0'\\\n"
- "pip install -U git+https://git@github.com/fairinternal/xformers.git#egg=xformers\n"
- "To install on FAIR Cluster, run \n"
- "FORCE_CUDA=1 TORCH_CUDA_ARCH_LIST='6.0;7.0'\\\n"
- "pip install -U git+https://git@github.com/fairinternal/xformers.git#egg=xformers\n")
-
-
-def _verify_xformers_internal_compat():
- try:
- from xformers.checkpoint_fairinternal import checkpoint, _get_default_policy # noqa
- except ImportError:
- raise ImportError(
- "Francisco's fairinternal xformers is not installed. Please install it and try again.\n"
- "To install on AWS and Azure, run \n"
- "FORCE_CUDA=1 TORCH_CUDA_ARCH_LIST='8.0'\\\n"
- "pip install -U git+https://git@github.com/fairinternal/xformers.git#egg=xformers\n"
- "To install on FAIR Cluster, run \n"
- "FORCE_CUDA=1 TORCH_CUDA_ARCH_LIST='6.0;7.0'\\\n"
- "pip install -U git+https://git@github.com/fairinternal/xformers.git#egg=xformers\n")
-
-
-def _is_custom(custom: bool, memory_efficient: bool):
- return custom or memory_efficient
diff --git a/spaces/AchyuthGamer/OpenGPT-Chat-UI/src/routes/login/+page.server.ts b/spaces/AchyuthGamer/OpenGPT-Chat-UI/src/routes/login/+page.server.ts
deleted file mode 100644
index 28692b5304687ce69551c5015d71a4419069415a..0000000000000000000000000000000000000000
--- a/spaces/AchyuthGamer/OpenGPT-Chat-UI/src/routes/login/+page.server.ts
+++ /dev/null
@@ -1,16 +0,0 @@
-import { redirect } from "@sveltejs/kit";
-import { getOIDCAuthorizationUrl } from "$lib/server/auth";
-import { base } from "$app/paths";
-
-export const actions = {
- default: async function ({ url, locals, request }) {
- // TODO: Handle errors if provider is not responding
- const referer = request.headers.get("referer");
- const authorizationUrl = await getOIDCAuthorizationUrl(
- { redirectURI: `${(referer ? new URL(referer) : url).origin}${base}/login/callback` },
- { sessionId: locals.sessionId }
- );
-
- throw redirect(303, authorizationUrl);
- },
-};
diff --git a/spaces/AchyuthGamer/OpenGPT/g4f/Provider/Providers/helpers/phind.py b/spaces/AchyuthGamer/OpenGPT/g4f/Provider/Providers/helpers/phind.py
deleted file mode 100644
index 70525d51d849c43bd1cf29c7f9b18f22bff1e982..0000000000000000000000000000000000000000
--- a/spaces/AchyuthGamer/OpenGPT/g4f/Provider/Providers/helpers/phind.py
+++ /dev/null
@@ -1,69 +0,0 @@
-import sys
-import json
-import datetime
-import urllib.parse
-
-from curl_cffi import requests
-
-config = json.loads(sys.argv[1])
-prompt = config['messages'][-1]['content']
-
-skill = 'expert' if config['model'] == 'gpt-4' else 'intermediate'
-
-json_data = json.dumps({
- 'question': prompt,
- 'options': {
- 'skill': skill,
- 'date': datetime.datetime.now().strftime('%d/%m/%Y'),
- 'language': 'en',
- 'detailed': True,
- 'creative': True,
- 'customLinks': []}}, separators=(',', ':'))
-
-headers = {
- 'Content-Type': 'application/json',
- 'Pragma': 'no-cache',
- 'Accept': '*/*',
- 'Sec-Fetch-Site': 'same-origin',
- 'Accept-Language': 'en-GB,en;q=0.9',
- 'Cache-Control': 'no-cache',
- 'Sec-Fetch-Mode': 'cors',
- 'Content-Length': str(len(json_data)),
- 'Origin': 'https://www.phind.com',
- 'User-Agent': 'Mozilla/5.0 (Macintosh; Intel Mac OS X 10_15_7) AppleWebKit/605.1.15 (KHTML, like Gecko) Version/16.4 Safari/605.1.15',
- 'Referer': f'https://www.phind.com/search?q={urllib.parse.quote(prompt)}&source=searchbox',
- 'Connection': 'keep-alive',
- 'Host': 'www.phind.com',
- 'Sec-Fetch-Dest': 'empty'
-}
-
-
-def output(chunk):
- try:
- if b'PHIND_METADATA' in chunk:
- return
-
- if chunk == b'data: \r\ndata: \r\ndata: \r\n\r\n':
- chunk = b'data: \n\r\n\r\n'
-
- chunk = chunk.decode()
-
- chunk = chunk.replace('data: \r\n\r\ndata: ', 'data: \n')
- chunk = chunk.replace('\r\ndata: \r\ndata: \r\n\r\n', '\n\r\n\r\n')
- chunk = chunk.replace('data: ', '').replace('\r\n\r\n', '')
-
- print(chunk, flush=True, end = '')
-
- except json.decoder.JSONDecodeError:
- pass
-
-while True:
- try:
- response = requests.post('https://www.phind.com/api/infer/answer',
- headers=headers, data=json_data, content_callback=output, timeout=999999, impersonate='safari15_5')
-
- exit(0)
-
- except Exception as e:
- print('an error occured, retrying... |', e, flush=True)
- continue
\ No newline at end of file
diff --git a/spaces/AgentVerse/agentVerse/ui/src/phaser3-rex-plugins/templates/ui/basesizer/utils/ClearChildren.js b/spaces/AgentVerse/agentVerse/ui/src/phaser3-rex-plugins/templates/ui/basesizer/utils/ClearChildren.js
deleted file mode 100644
index 6a527b2be5b32c08db23656af96d16ed637bd0a5..0000000000000000000000000000000000000000
--- a/spaces/AgentVerse/agentVerse/ui/src/phaser3-rex-plugins/templates/ui/basesizer/utils/ClearChildren.js
+++ /dev/null
@@ -1,29 +0,0 @@
-import Container from '../../container/Container.js';
-
-const ContainerClear = Container.prototype.clear;
-
-var ClearChildren = function (destroyChild) {
- if (this.backgroundChildren) {
- this.backgroundChildren.length = 0;
- }
-
- var fireRemoveEvent = !destroyChild && this.sizerEventsEnable;
- var children;
- if (fireRemoveEvent) {
- children = this.getChildren([]);
- }
-
- ContainerClear.call(this, destroyChild);
-
- if (fireRemoveEvent) {
- var gameObject;
- for (var i = 0, cnt = children.length; i < cnt; i++) {
- gameObject = children[i];
- gameObject.emit('sizer.remove', gameObject, this);
- this.emit('remove', gameObject, this);
- }
- }
- return this;
-}
-
-export default ClearChildren;
\ No newline at end of file
diff --git a/spaces/AgentVerse/agentVerse/ui/src/phaser3-rex-plugins/templates/ui/slider/Slider.d.ts b/spaces/AgentVerse/agentVerse/ui/src/phaser3-rex-plugins/templates/ui/slider/Slider.d.ts
deleted file mode 100644
index f94fe29277b5b7f94748fa5a55ab54e0914b1b20..0000000000000000000000000000000000000000
--- a/spaces/AgentVerse/agentVerse/ui/src/phaser3-rex-plugins/templates/ui/slider/Slider.d.ts
+++ /dev/null
@@ -1,58 +0,0 @@
-// import * as Phaser from 'phaser';
-import Sizer from '../sizer/Sizer';
-import RoundRecrangle from '../../../plugins/roundrectangle';
-
-
-export default Slider;
-
-declare namespace Slider {
-
- type InputTypes = 0 | 1 | -1 | 'drag' | 'pan' | 'click' | 'none';
-
- interface IConfig extends Sizer.IConfig {
- reverseAxis?: boolean,
- background?: Phaser.GameObjects.GameObject | RoundRecrangle.IConfig,
- track?: Phaser.GameObjects.GameObject | RoundRecrangle.IConfig,
- indicator?: Phaser.GameObjects.GameObject | RoundRecrangle.IConfig,
- thumb?: Phaser.GameObjects.GameObject | RoundRecrangle.IConfig,
-
- input?: InputTypes,
-
- gap?: number,
-
- value?: number,
- min?: number, max?: number,
-
- easeValue?: {
- duration?: number,
- ease?: string
- },
-
- valuechangeCallback: (newValue: number, oldValue: number, slider: Slider) => void,
-
- enable?: boolean,
- }
-}
-
-declare class Slider extends Sizer {
- constructor(
- scene: Phaser.Scene,
- config?: Slider.IConfig
- );
-
- value: number;
- getValue(min?: number, max?: number): number;
- setValue(value?: number, min?: number, max?: number): this;
- addValue(inc?: number, min?: number, max?: number): this;
-
- easeValueTo(value?: number, min?: number, max?: number): this;
- stopEaseValue(): this;
- setEaseValueDuration(duration: number): this;
- setEaseValueFunction(ease: string): this;
-
- setGap(gap?: number, min?: number, max?: number): this;
- gap: number;
-
- setEnable(enable?: boolean): this;
- enable: boolean;
-}
\ No newline at end of file
diff --git a/spaces/Akmyradov/TurkmenTTSweSTT/uroman/lib/NLP/utilities.pm b/spaces/Akmyradov/TurkmenTTSweSTT/uroman/lib/NLP/utilities.pm
deleted file mode 100644
index 7be117449190533d826bd63b9266c1434d00408f..0000000000000000000000000000000000000000
--- a/spaces/Akmyradov/TurkmenTTSweSTT/uroman/lib/NLP/utilities.pm
+++ /dev/null
@@ -1,3652 +0,0 @@
-################################################################
-# #
-# utilities #
-# #
-################################################################
-
-package NLP::utilities;
-
-use File::Spec;
-use Time::HiRes qw(time);
-use Time::Local;
-use NLP::English;
-use NLP::UTF8;
-
-$utf8 = NLP::UTF8;
-$englishPM = NLP::English;
-
-%empty_ht = ();
-
-use constant DEBUGGING => 0;
-
-sub member {
- local($this,$elem,@array) = @_;
-
- my $a;
- if (defined($elem)) {
- foreach $a (@array) {
- if (defined($a)) {
- return 1 if $elem eq $a;
- } else {
- $DB::single = 1; # debugger breakpoint
- print STDERR "\nWarning: Undefined variable utilities::member::a\n";
- }
- }
- } else {
- $DB::single = 1; # debugger breakpoint
- print STDERR "\nWarning: Undefined variable utilities::member::elem\n";
- }
- return 0;
-}
-
-sub dual_member {
- local($this,$elem1,$elem2,*array1,*array2) = @_;
- # returns 1 if there exists a position $n
- # such that $elem1 occurs at position $n in @array1
- # and $elem2 occurs at same position $n in @array2
-
- return 0 unless defined($elem1) && defined($elem2);
- my $last_index = ($#array1 < $#array2) ? $#array1 : $#array2; #min
- my $a;
- my $b;
- foreach $i ((0 .. $last_index)) {
- return 1 if defined($a = $array1[$i]) && defined($b = $array2[$i]) && ($a eq $elem1) && ($b eq $elem2);
- }
- return 0;
-}
-
-sub sorted_list_equal {
- local($this,*list1,*list2) = @_;
-
- return 0 unless $#list1 == $#list2;
- foreach $i ((0 .. $#list1)) {
- return 0 unless $list1[$i] eq $list2[$i];
- }
- return 1;
-}
-
-sub trim {
- local($this, $s) = @_;
-
- $s =~ s/^\s*//;
- $s =~ s/\s*$//;
- $s =~ s/\s+/ /g;
- return $s;
-}
-
-sub trim2 {
- local($this, $s) = @_;
-
- $s =~ s/^\s*//;
- $s =~ s/\s*$//;
- return $s;
-}
-
-sub trim_left {
- local($this, $s) = @_;
- $s =~ s/^\s*//;
- return $s;
-}
-
-sub cap_member {
- local($this,$elem,@array) = @_;
-
- my $a;
- my $lc_elem = lc $elem;
- foreach $a (@array) {
- return $a if $lc_elem eq lc $a;
- }
- return "";
-}
-
-sub remove_elem {
- local($this,$elem,@array) = @_;
-
- return @array unless $this->member($elem, @array);
- @rm_list = ();
- foreach $a (@array) {
- push(@rm_list, $a) unless $elem eq $a;
- }
- return @rm_list;
-}
-
-sub intersect_p {
- local($this,*list1,*list2) = @_;
-
- foreach $elem1 (@list1) {
- if (defined($elem1)) {
- foreach $elem2 (@list2) {
- if (defined($elem2)) {
- return 1 if $elem1 eq $elem2;
- } else {
- $DB::single = 1; # debugger breakpoint
- print STDERR "\nWarning: Undefined variable utilities::intersect_p::elem2\n";
- }
- }
- } else {
- $DB::single = 1; # debugger breakpoint
- print STDERR "\nWarning: Undefined variable utilities::intersect_p::elem1\n";
- }
- }
- return 0;
-}
-
-sub intersect_expl_p {
- local($this,*list1,@list2) = @_;
-
- foreach $elem1 (@list1) {
- foreach $elem2 (@list2) {
- return 1 if $elem1 eq $elem2;
- }
- }
- return 0;
-}
-
-sub intersection {
- local($this,*list1,*list2) = @_;
-
- @intersection_list = ();
- foreach $elem1 (@list1) {
- foreach $elem2 (@list2) {
- push(@intersection_list, $elem1) if ($elem1 eq $elem2) && ! $this->member($elem1, @intersection_list);
- }
- }
- return @intersection_list;
-}
-
-sub cap_intersect_p {
- local($this,*list1,*list2) = @_;
-
- foreach $elem1 (@list1) {
- $lc_elem1 = lc $elem1;
- foreach $elem2 (@list2) {
- return 1 if $lc_elem1 eq lc $elem2;
- }
- }
- return 0;
-}
-
-sub subset_p {
- local($this,*list1,*list2) = @_;
-
- foreach $elem1 (@list1) {
- return 0 unless $this->member($elem1, @list2);
- }
- return 1;
-}
-
-sub cap_subset_p {
- local($this,*list1,*list2) = @_;
-
- foreach $elem1 (@list1) {
- return 0 unless $this->cap_member($elem1, @list2);
- }
- return 1;
-}
-
-sub unique {
- local($this, @list) = @_;
-
- my %seen = ();
- @uniq = ();
- foreach $item (@list) {
- push(@uniq, $item) unless $seen{$item}++;
- }
- return @uniq;
-}
-
-sub position {
- local($this,$elem,@array) = @_;
- $i = 0;
- foreach $a (@array) {
- return $i if $elem eq $a;
- $i++;
- }
- return -1;
-}
-
-sub positions {
- local($this,$elem,@array) = @_;
- $i = 0;
- @positions_in_list = ();
- foreach $a (@array) {
- push(@positions_in_list, $i) if $elem eq $a;
- $i++;
- }
- return @positions_in_list;
-}
-
-sub last_position {
- local($this,$elem,@array) = @_;
-
- $result = -1;
- $i = 0;
- foreach $a (@array) {
- $result = $i if $elem eq $a;
- $i++;
- }
- return $result;
-}
-
-sub rand_n_digit_number {
- local($this,$n) = @_;
-
- return 0 unless $n =~ /^[1-9]\d*$/;
- $ten_power_n = 10 ** ($n - 1);
- return int(rand(9 * $ten_power_n)) + $ten_power_n;
-}
-
-# Consider File::Temp
-sub new_tmp_filename {
- local($this,$filename) = @_;
-
- $loop_limit = 1000;
- ($dir,$simple_filename) = ($filename =~ /^(.+)\/([^\/]+)$/);
- $simple_filename = $filename unless defined($simple_filename);
- $new_filename = "$dir/tmp-" . $this->rand_n_digit_number(8) . "-$simple_filename";
- while ((-e $new_filename) && ($loop_limit-- >= 0)) {
- $new_filename = "$dir/tmp-" . $this->rand_n_digit_number(8) . "-$simple_filename";
- }
- return $new_filename;
-}
-
-# support sorting order: "8", "8.0", "8.5", "8.5.1.", "8.10", "10", "10-12"
-
-sub compare_complex_numeric {
- local($this,$a,$b) = @_;
-
- (my $a_num,my $a_rest) = ($a =~ /^(\d+)\D*(.*)$/);
- (my $b_num,my $b_rest) = ($b =~ /^(\d+)\D*(.*)$/);
-
- if (defined($a_rest) && defined($b_rest)) {
- return ($a_num <=> $b_num)
- || $this->compare_complex_numeric($a_rest,$b_rest);
- } else {
- return $a cmp $b;
- }
-}
-
-# support sorting order: "lesson8-ps-v1.9.xml", "Lesson 10_ps-v_1.11.xml"
-# approach: segment strings into alphabetic and numerical sections and compare pairwise
-
-sub compare_mixed_alpha_numeric {
- local($this,$a,$b) = @_;
-
- ($a_alpha,$a_num,$a_rest) = ($a =~ /^(\D*)(\d[-\d\.]*)(.*)$/);
- ($b_alpha,$b_num,$b_rest) = ($b =~ /^(\D*)(\d[-\d\.]*)(.*)$/);
-
- ($a_alpha) = ($a =~ /^(\D*)/) unless defined $a_alpha;
- ($b_alpha) = ($b =~ /^(\D*)/) unless defined $b_alpha;
-
- # ignore non-alphabetic characters in alpha sections
- $a_alpha =~ s/\W|_//g;
- $b_alpha =~ s/\W|_//g;
-
- if ($alpha_cmp = lc $a_alpha cmp lc $b_alpha) {
- return $alpha_cmp;
- } elsif (defined($a_rest) && defined($b_rest)) {
- return $this->compare_complex_numeric($a_num,$b_num)
- || $this->compare_mixed_alpha_numeric ($a_rest,$b_rest);
- } else {
- return (defined($a_num) <=> defined($b_num)) || ($a cmp $b);
- }
-}
-
-# @sorted_lessons = sort { NLP::utilities->compare_mixed_alpha_numeric($a,$b) } @lessons;
-
-sub html_guarded_p {
- local($this,$string) = @_;
-
- return 0 if $string =~ /[<>"]/;
- $string .= " ";
- @segs = split('&',$string);
- shift @segs;
- foreach $seg (@segs) {
- next if $seg =~ /^[a-z]{2,6};/i;
- # next if $seg =~ /^amp;/;
- # next if $seg =~ /^quot;/;
- # next if $seg =~ /^nbsp;/;
- # next if $seg =~ /^gt;/;
- # next if $seg =~ /^lt;/;
- next if $seg =~ /^#(\d+);/;
- next if $seg =~ /^#x([0-9a-fA-F]+);/;
- return 0;
- }
- return 1;
-}
-
-sub guard_tooltip_text {
- local($this,$string) = @_;
-
- $string =~ s/\xCB\x88/'/g;
- return $string;
-}
-
-sub guard_html {
- local($this,$string,$control_string) = @_;
-
- return "" unless defined($string);
- my $guarded_string;
- $control_string = "" unless defined($control_string);
- return $string if ($string =~ /&/)
- && (! ($control_string =~ /\bstrict\b/))
- && $this->html_guarded_p($string);
- $guarded_string = $string;
- $guarded_string =~ s/&/&/g;
- if ($control_string =~ /slash quote/) {
- $guarded_string =~ s/"/\\"/g;
- } elsif ($control_string =~ /keep quote/) {
- } else {
- $guarded_string =~ s/\"/"/g;
- }
- if ($control_string =~ /escape-slash/) {
- $guarded_string =~ s/\//&x2F;/g;
- }
- $guarded_string =~ s/>/>/g;
- $guarded_string =~ s/</g;
- return $guarded_string;
-}
-
-sub unguard_html {
- local($this,$string) = @_;
-
- return undef unless defined($string);
- $string=~ s[&(\S*?);]{
- local $_ = $1;
- /^amp$/i ? "&" :
- /^quot$/i ? '"' :
- /^apos$/i ? "'" :
- /^gt$/i ? ">" :
- /^lt$/i ? "<" :
- /^x2F$/i ? "/" :
- /^nbsp$/i ? "\xC2\xA0" :
- /^#(\d+)$/ ? $this->chr($1) :
- /^#x([0-9a-f]+)$/i ? $this->chr(hex($1)) :
- $_
- }gex;
- return $string;
-}
-
-sub unguard_html_r {
- local($this,$string) = @_;
-
- return undef unless defined($string);
-
- $string =~ s/&/&/g;
- $string =~ s/"/'/g;
- $string =~ s/<//g;
-
- ($d) = ($string =~ /(\d+);/);
- while (defined($d)) {
- $c = $this->chr($d);
- $string =~ s/$d;/$c/g;
- ($d) = ($string =~ /(\d+);/);
- }
- ($x) = ($string =~ /([0-9a-f]+);/i);
- while (defined($x)) {
- $c = $this->chr(hex($x));
- $string =~ s/$x;/$c/g;
- ($x) = ($string =~ /([0-9a-f]+);/i);
- }
- $string0 = $string;
- ($x) = ($string =~ /(?:https?|www|\.com)\S*\%([0-9a-f]{2,2})/i);
- while (defined($x)) {
- $c = $this->chr("%" . hex($x));
- $string =~ s/\%$x/$c/g;
- ($x) = ($string =~ /(?:https?|www|\.com)\S*\%([0-9a-f]{2,2})/i);
- }
- return $string;
-}
-
-sub unguard_html_l {
- local($caller,$string) = @_;
-
- return undef unless defined($string);
-
- my $pre;
- my $core;
- my $post;
- my $repl;
- my $s = $string;
- if (($pre,$core,$post) = ($s =~ /^(.*)&(amp|quot|lt|gt|#\d+|#x[0-9a-f]+);(.*)$/i)) {
- $repl = "?";
- $repl = "&" if $core =~ /^amp$/i;
- $repl = "'" if $core =~ /^quot$/i;
- $repl = "<" if $core =~ /^lt$/i;
- $repl = ">" if $core =~ /^gt$/i;
- if ($core =~ /^#\d+$/i) {
- $core2 = substr($core,1);
- $repl = $caller->chr($core2);
- }
- $repl = $caller->chr(hex(substr($core,2))) if $core =~ /^#x[0-9a-f]+$/i;
- $s = $pre . $repl . $post;
- }
- return $s;
-}
-
-sub guard_html_quote {
- local($caller,$string) = @_;
-
- $string =~ s/"/"/g;
- return $string;
-}
-
-sub unguard_html_quote {
- local($caller,$string) = @_;
-
- $string =~ s/"/"/g;
- return $string;
-}
-
-sub uri_encode {
- local($caller,$string) = @_;
-
- $string =~ s/([^^A-Za-z0-9\-_.!~*()'])/ sprintf "%%%02x", ord $1 /eg;
- return $string;
-}
-
-sub uri_decode {
- local($caller,$string) = @_;
-
- $string =~ s/%([0-9A-Fa-f]{2})/chr(hex($1))/eg;
- return $string;
-}
-
-sub remove_xml_tags {
- local($caller,$string) = @_;
-
- $string =~ s/<\/?[a-zA-Z][-_:a-zA-Z0-9]*(\s+[a-zA-Z][-_:a-zA-Z0-9]*=\"[^"]*\")*\s*\/?>//g;
- return $string;
-}
-
-sub remove_any_tokenization_at_signs_around_xml_tags {
- local($caller,$string) = @_;
-
- $string =~ s/(?:\@ \@)?(<[^<>]+>)(?:\@ \@)?/$1/g;
- $string =~ s/\@?(<[^<>]+>)\@?/$1/g;
- return $string;
-}
-
-sub remove_xml_tags_and_any_bordering_at_signs {
- # at-signs from tokenization
- local($caller,$string) = @_;
-
- $string =~ s/\@?<\/?[a-zA-Z][-_:a-zA-Z0-9]*(\s+[a-zA-Z][-_:a-zA-Z0-9]*=\"[^"]*\")*\s*\/?>\@?//g;
- return $string;
-}
-
-sub chr {
- local($caller,$i) = @_;
-
- return undef unless $i =~ /^\%?\d+$/;
- if ($i =~ /^%/) {
- $i =~ s/^\%//;
- return chr($i) if $i < 128;
- return "\x80" | chr($i - 128) if $i < 256;
- } else {
- return chr($i) if $i < 128;
- return ("\xC0" | chr(($i / 64) % 32))
- . ("\x80" | chr($i % 64)) if $i < 2048;
- return ("\xE0" | chr(int($i / 4096) % 16))
- . ("\x80" | chr(int($i / 64) % 64))
- . ("\x80" | chr($i % 64)) if $i < 65536;
- return ("\xF0" | chr(int($i / 262144) % 8))
- . ("\x80" | chr(int($i / 4096) % 64))
- . ("\x80" | chr(int($i / 64) % 64))
- . ("\x80" | chr($i % 64)) if $i < 2097152;
- }
- return "?";
-}
-
-sub guard_cgi {
- local($caller, $string) = @_;
-
- $guarded_string = $string;
- if ($string =~ /[\x80-\xFF]/) {
- $guarded_string = "";
- while ($string ne "") {
- $char = substr($string, 0, 1);
- $string = substr($string, 1);
- if ($char =~ /^[\\ ;\#\&\:\=\"\'\+\?\x00-\x1F\x80-\xFF]$/) {
- $hex = sprintf("%2.2x",ord($char));
- $guarded_string .= uc "%$hex";
- } else {
- $guarded_string .= $char;
- }
- }
- } else {
- $guarded_string = $string;
- $guarded_string =~ s/%/%25/g;
- $guarded_string =~ s/\n/%5Cn/g;
- $guarded_string =~ s/\t/%5Ct/g;
- $guarded_string =~ s/ /%20/g;
- $guarded_string =~ s/"/%22/g;
- $guarded_string =~ s/#/%23/g;
- $guarded_string =~ s/&/%26/g;
- $guarded_string =~ s/'/%27/g;
- $guarded_string =~ s/\+/%2B/g;
- $guarded_string =~ s/\//%2F/g;
- $guarded_string =~ s/:/%3A/g;
- $guarded_string =~ s/;/%3B/g;
- $guarded_string =~ s/%3C/g;
- $guarded_string =~ s/=/%3D/g;
- $guarded_string =~ s/>/%3E/g;
- $guarded_string =~ s/\?/%3F/g;
- }
- return $guarded_string;
-}
-
-sub repair_cgi_guard {
- local($caller,$string) = @_;
- # undo second cgi-guard, e.g. "Jo%25C3%25ABlle_Aubron" -> "Jo%C3%ABlle_Aubron"
-
- $string =~ s/(%)25([CD][0-9A-F]%)25([89AB][0-9A-F])/$1$2$3/g;
- $string =~ s/(%)25(E[0-9A-F]%)25([89AB][0-9A-F]%)25([89AB][0-9A-F])/$1$2$3$4/g;
- return $string;
-}
-
-sub unguard_cgi {
- local($caller,$string) = @_;
-
- $unguarded_string = $string;
- $unguarded_string =~ s/%5Cn/\n/g;
- $unguarded_string =~ s/%5Ct/\t/g;
- $unguarded_string =~ s/%20/ /g;
- $unguarded_string =~ s/%23/#/g;
- $unguarded_string =~ s/%26/&/g;
- $unguarded_string =~ s/%2B/+/g;
- $unguarded_string =~ s/%2C/,/g;
- $unguarded_string =~ s/%3A/:/g;
- $unguarded_string =~ s/%3D/=/g;
- $unguarded_string =~ s/%3F/?/g;
- $unguarded_string =~ s/%C3%A9/\xC3\xA9/g;
-
- # more general
- ($code) = ($unguarded_string =~ /%([0-9A-F]{2,2})/);
- while (defined($code)) {
- $percent_code = "%" . $code;
- $hex_code = sprintf("%c", hex($code));
- $unguarded_string =~ s/$percent_code/$hex_code/g;
- ($code) = ($unguarded_string =~ /%([0-9A-F]{2,2})/);
- }
-
- return $unguarded_string;
-}
-
-sub regex_guard {
- local($caller,$string) = @_;
-
- $guarded_string = $string;
- $guarded_string =~ s/([\\\/\^\|\(\)\{\}\$\@\*\+\?\.\[\]])/\\$1/g
- if $guarded_string =~ /[\\\/\^\|\(\)\{\}\$\@\*\+\?\.\[\]]/;
-
- return $guarded_string;
-}
-
-sub g_regex_spec_tok_p {
- local($this,$string) = @_;
-
- # specials: ( ) (?: ) [ ]
- return ($string =~ /^(\(\?:|[()\[\]])$/);
-}
-
-sub regex_guard_norm {
- local($this,$string) = @_;
-
- return $string unless $string =~ /[\[\]\\()$@?+]/;
- my $rest = $string;
- my @stack = ("");
- while ($rest ne "") {
- # specials: ( ) (?: ) [ ] ? +
- if (($pre, $special, $post) = ($rest =~ /^((?:\\.|[^\[\]()?+])*)(\(\?:|[\[\]()?+])(.*)$/)) {
- # print STDERR "Special: $pre *$special* $post\n";
- unless ($pre eq "") {
- push(@stack, $pre);
- while (($#stack >= 1) && (! $this->g_regex_spec_tok_p($stack[$#stack-1]))
- && (! $this->g_regex_spec_tok_p($stack[$#stack]))) {
- $s1 = pop @stack;
- $s2 = pop @stack;
- push(@stack, "$s2$s1");
- }
- }
- if ($special =~ /^[?+]$/) {
- push(@stack, "\\") if ($stack[$#stack] eq "")
- || ($this->g_regex_spec_tok_p($stack[$#stack]) && ($stack[$#stack] ne "["));
- push(@stack, $special);
- } elsif ($special eq "]") {
- if (($#stack >= 1) && ($stack[$#stack-1] eq "[") && ! $this->g_regex_spec_tok_p($stack[$#stack])) {
- $char_expression = pop @stack;
- pop @stack;
- push(@stack, "[$char_expression]");
- } else {
- push(@stack, $special);
- }
- } elsif (($special =~ /^[()]/) && (($stack[$#stack] eq "[")
- || (($#stack >= 1)
- && ($stack[$#stack-1] eq "[")
- && ! $this->g_regex_spec_tok_p($stack[$#stack])))) {
- push(@stack, "\\$special");
- } elsif ($special eq ")") {
- if (($#stack >= 1) && ($stack[$#stack-1] =~ /^\((\?:)?$/) && ! $this->g_regex_spec_tok_p($stack[$#stack])) {
- $alt_expression = pop @stack;
- $open_para = pop @stack;
- if ($open_para eq "(") {
- push(@stack, "(?:$alt_expression)");
- } else {
- push(@stack, "$open_para$alt_expression)");
- }
- } else {
- push(@stack, $special);
- }
- } else {
- push(@stack, $special);
- }
- while (($#stack >= 1) && (! $this->g_regex_spec_tok_p($stack[$#stack-1]))
- && (! $this->g_regex_spec_tok_p($stack[$#stack]))) {
- $s1 = pop @stack;
- $s2 = pop @stack;
- push(@stack, "$s2$s1");
- }
- $rest = $post;
- } else {
- push(@stack, $rest);
- $rest = "";
- }
- }
- # print STDERR "Stack: " . join(";", @stack) . "\n";
- foreach $i ((0 .. $#stack)) {
- $stack_elem = $stack[$i];
- if ($stack_elem =~ /^[()\[\]]$/) {
- $stack[$i] = "\\" . $stack[$i];
- }
- }
- return join("", @stack);
-}
-
-sub string_guard {
- local($caller,$string) = @_;
-
- return "" unless defined($string);
- $guarded_string = $string;
- $guarded_string =~ s/([\\"])/\\$1/g
- if $guarded_string =~ /[\\"]/;
-
- return $guarded_string;
-}
-
-sub json_string_guard {
- local($caller,$string) = @_;
-
- return "" unless defined($string);
- $guarded_string = $string;
- $guarded_string =~ s/([\\"])/\\$1/g
- if $guarded_string =~ /[\\"]/;
- $guarded_string =~ s/\r*\n/\\n/g
- if $guarded_string =~ /\n/;
-
- return $guarded_string;
-}
-
-sub json_string_unguard {
- local($caller,$string) = @_;
-
- return "" unless defined($string);
- $string =~ s/\\n/\n/g
- if $string =~ /\\n/;
- return $string;
-}
-
-sub guard_javascript_arg {
- local($caller,$string) = @_;
-
- return "" unless defined($string);
- $guarded_string = $string;
- $guarded_string =~ s/\\/\\\\/g;
- $guarded_string =~ s/'/\\'/g;
- return $guarded_string;
-}
-
-sub guard_substitution_right_hand_side {
- # "$1x" => "$1 . \"x\""
- local($caller,$string) = @_;
-
- my $result = "";
- ($pre,$var,$post) = ($string =~ /^([^\$]*)(\$\d)(.*)$/);
- while (defined($var)) {
- $result .= " . " if $result;
- $result .= "\"$pre\" . " unless $pre eq "";
- $result .= $var;
- $string = $post;
- ($pre,$var,$post) = ($string =~ /^([^\$]*)(\$\d)(.*)$/);
- }
- $result .= " . \"$string\"" if $string;
- return $result;
-}
-
-sub string_starts_with_substring {
- local($caller,$string,$substring) = @_;
-
- $guarded_substring = $caller->regex_guard($substring);
- return $string =~ /^$guarded_substring/;
-}
-
-sub one_string_starts_with_the_other {
- local($caller,$s1,$s2) = @_;
-
- return ($s1 eq $s2)
- || $caller->string_starts_with_substring($s1,$s2)
- || $caller->string_starts_with_substring($s2,$s1);
-}
-
-sub string_ends_in_substring {
- local($caller,$string,$substring) = @_;
-
- $guarded_substring = $caller->regex_guard($substring);
- return $string =~ /$guarded_substring$/;
-}
-
-sub string_equal_ignore_leading_multiple_or_trailing_blanks {
- local($caller,$string1,$string2) = @_;
-
- return 1 if $string1 eq $string2;
- $string1 =~ s/\s+/ /;
- $string2 =~ s/\s+/ /;
- $string1 =~ s/^\s+//;
- $string2 =~ s/^\s+//;
- $string1 =~ s/\s+$//;
- $string2 =~ s/\s+$//;
-
- return $string1 eq $string2;
-}
-
-sub strip_substring_from_start_of_string {
- local($caller,$string,$substring,$error_code) = @_;
-
- $error_code = "ERROR" unless defined($error_code);
- my $reg_surf = $caller->regex_guard($substring);
- if ($string =~ /^$guarded_substring/) {
- $string =~ s/^$reg_surf//;
- return $string;
- } else {
- return $error_code;
- }
-}
-
-sub strip_substring_from_end_of_string {
- local($caller,$string,$substring,$error_code) = @_;
-
- $error_code = "ERROR" unless defined($error_code);
- my $reg_surf = $caller->regex_guard($substring);
- if ($string =~ /$reg_surf$/) {
- $string =~ s/$reg_surf$//;
- return $string;
- } else {
- return $error_code;
- }
-}
-
-# to be deprecated
-sub lang_code {
- local($caller,$language) = @_;
-
- $langPM = NLP::Language->new();
- return $langPM->lang_code($language);
-}
-
-sub full_language {
- local($caller,$lang_code) = @_;
-
- return "Arabic" if $lang_code eq "ar";
- return "Chinese" if $lang_code eq "zh";
- return "Czech" if $lang_code eq "cs";
- return "Danish" if $lang_code eq "da";
- return "Dutch" if $lang_code eq "nl";
- return "English" if $lang_code eq "en";
- return "Finnish" if $lang_code eq "fi";
- return "French" if $lang_code eq "fr";
- return "German" if $lang_code eq "de";
- return "Greek" if $lang_code eq "el";
- return "Hebrew" if $lang_code eq "he";
- return "Hindi" if $lang_code eq "hi";
- return "Hungarian" if $lang_code eq "hu";
- return "Icelandic" if $lang_code eq "is";
- return "Indonesian" if $lang_code eq "id";
- return "Italian" if $lang_code eq "it";
- return "Japanese" if $lang_code eq "ja";
- return "Kinyarwanda" if $lang_code eq "rw";
- return "Korean" if $lang_code eq "ko";
- return "Latin" if $lang_code eq "la";
- return "Malagasy" if $lang_code eq "mg";
- return "Norwegian" if $lang_code eq "no";
- return "Pashto" if $lang_code eq "ps";
- return "Persian" if $lang_code eq "fa";
- return "Polish" if $lang_code eq "pl";
- return "Portuguese" if $lang_code eq "pt";
- return "Romanian" if $lang_code eq "ro";
- return "Russian" if $lang_code eq "ru";
- return "Spanish" if $lang_code eq "es";
- return "Swedish" if $lang_code eq "sv";
- return "Turkish" if $lang_code eq "tr";
- return "Urdu" if $lang_code eq "ur";
- return "";
-}
-
-# to be deprecated
-sub short_lang_name {
- local($caller,$lang_code) = @_;
-
- $langPM = NLP::Language->new();
- return $langPM->shortname($lang_code);
-}
-
-sub ml_dir {
- local($caller,$language,$type) = @_;
-
- $type = "MSB" unless defined($type);
- $lang_code = $langPM->lang_code($language);
- return $caller->ml_dir($lang_code, "lex") . "/corpora" if $type eq "corpora";
- return "" unless defined($rc);
- $ml_home = $rc->ml_home_dir();
- return File::Spec->catfile($ml_home, "arabic")
- if ($lang_code eq "ar-iq") && ! $caller->member(lc $type,"lex","onto","dict");
- $langPM = NLP::Language->new();
- $lexdir = $langPM->lexdir($lang_code);
- return $lexdir if defined($lexdir);
- return "";
-}
-
-sub language_lex_filename {
- local($caller,$language,$type) = @_;
-
- $langPM = NLP::Language->new();
- if (($lang_code = $langPM->lang_code($language))
- && ($ml_dir = $caller->ml_dir($lang_code,$type))
- && ($norm_language = $caller->short_lang_name($lang_code))) {
- return "$ml_dir/$norm_language-lex" if ($type eq "lex");
- return "$ml_dir/onto" if ($type eq "onto");
- return "$ml_dir/$norm_language-english-dict" if ($type eq "dict") && !($lang_code eq "en");
- return "";
- } else {
- return "";
- }
-}
-
-# filename_without_path is obsolete - replace with
-# use File::Basename;
-# basename($filename)
-sub filename_without_path {
- local($caller,$filename) = @_;
-
- $filename =~ s/^.*\/([^\/]+)$/$1/;
- return $filename;
-}
-
-sub option_string {
- local($caller,$input_name,$default,*values,*labels) = @_;
-
- my $s = "";
- for $i (0 .. $#values) {
- my $value = $values[$i];
- my $label = $labels[$i];
- my $selected_clause = ($default eq $value) ? "selected" : "";
- $s .= "$label ";
- }
- $s .= " ";
- return $s;
-}
-
-sub pes_subseq_surf {
- local($this,$start,$length,$langCode,@pes) = @_;
-
- my $surf = "";
- if ($start+$length-1 <= $#pes) {
- foreach $i ($start .. $start + $length - 1) {
- my $pe = $pes[$i];
- $surf .= $pe->get("surf","");
- $surf .= " " if $langCode =~ /^(ar|en|fr)$/;
- }
- }
- $surf =~ s/\s+$//;
- return $surf;
-}
-
-sub copyList {
- local($this,@list) = @_;
-
- @copy_list = ();
- foreach $elem (@list) {
- push(@copy_list,$elem);
- }
- return @copy_list;
-}
-
-sub list_with_same_elem {
- local($this,$size,$elem) = @_;
-
- @list = ();
- foreach $i (0 .. $size-1) {
- push(@list,$elem);
- }
- return @list;
-}
-
-sub count_occurrences {
- local($this,$s,$substring) = @_;
-
- $occ = 0;
- $new = $s;
- $guarded_substring = $this->regex_guard($substring);
- $new =~ s/$guarded_substring//;
- while ($new ne $s) {
- $occ++;
- $s = $new;
- $new =~ s/$guarded_substring//;
- }
- return $occ;
-}
-
-sub position_of_nth_occurrence {
- local($this,$s,$substring,$occ) = @_;
-
- return -1 unless $occ > 0;
- my $pos = 0;
- while (($pos = index($s, $substring, $pos)) >= 0) {
- return $pos if $occ == 1;
- $occ--;
- $pos = $pos + length($substring);
- }
- return -1;
-}
-
-sub has_diff_elements_p {
- local($this,@array) = @_;
-
- return 0 if $#array < 1;
- $elem = $array[0];
-
- foreach $a (@array) {
- return 1 if $elem ne $a;
- }
- return 0;
-}
-
-sub init_log {
- local($this,$logfile, $control) = @_;
-
- $control = "" unless defined($control);
- if ((DEBUGGING || ($control =~ /debug/i)) && $logfile) {
- system("rm -f $logfile");
- system("date > $logfile; chmod 777 $logfile");
- }
-}
-
-sub time_stamp_log {
- local($this,$logfile, $control) = @_;
-
- $control = "" unless defined($control);
- if ((DEBUGGING || ($control =~ /debug/i)) && $logfile) {
- system("date >> $logfile; chmod 777 $logfile");
- }
-}
-
-sub log {
- local($this,$message,$logfile,$control) = @_;
-
- $control = "" unless defined($control);
- if ((DEBUGGING || ($control =~ /debug/i)) && $logfile) {
- $this->init_log($logfile, $control) unless -w $logfile;
- if ($control =~ /timestamp/i) {
- $this->time_stamp_log($logfile, $control);
- }
- $guarded_message = $message;
- $guarded_message =~ s/"/\\"/g;
- system("echo \"$guarded_message\" >> $logfile");
- }
-}
-
-sub month_name_to_month_number {
- local($this,$month_name) = @_;
-
- $month_name_init = lc substr($month_name,0,3);
- return $this->position($month_name_init, "jan", "feb", "mar", "apr", "may", "jun", "jul", "aug", "sep", "oct", "nov", "dec") + 1;
-}
-
-my @short_month_names = ("Jan.","Febr.","March","April","May","June","July","Aug.","Sept.","Oct.","Nov.","Dec.");
-my @full_month_names = ("January","February","March","April","May","June","July","August","September","October","November","December");
-
-sub month_number_to_month_name {
- local($this,$month_number, $control) = @_;
-
- $month_number =~ s/^0//;
- if ($month_number =~ /^([1-9]|1[0-2])$/) {
- return ($control && ($control =~ /short/i))
- ? $short_month_names[$month_number-1]
- : $full_month_names[$month_number-1];
- } else {
- return "";
- }
-}
-
-sub leap_year {
- local($this,$year) = @_;
-
- return 0 if $year % 4 != 0;
- return 1 if $year % 400 == 0;
- return 0 if $year % 100 == 0;
- return 1;
-}
-
-sub datetime {
- local($this,$format,$time_in_secs, $command) = @_;
-
- $command = "" unless defined($command);
- $time_in_secs = time unless defined($time_in_secs) && $time_in_secs;
- @time_vector = ($command =~ /\b(gm|utc)\b/i) ? gmtime($time_in_secs) : localtime($time_in_secs);
- ($sec,$min,$hour,$mday,$mon,$year,$wday,$yday,$isdst)=@time_vector;
- $thisyear = $year + 1900;
- $thismon=(Jan,Feb,Mar,Apr,May,Jun,Jul,Aug,Sep,Oct,Nov,Dec)[$mon];
- $thismon2=("Jan.","Febr.","March","April","May","June","July","Aug.","Sept.","Oct.","Nov.","Dec.")[$mon];
- $thismonth = $mon + 1;
- $thisday=(Sun,Mon,Tue,Wed,Thu,Fri,Sat)[$wday];
- $milliseconds = int(($time_in_secs - int($time_in_secs)) * 1000);
- $date="$thisday $thismon $mday, $thisyear";
- $sdate="$thismon $mday, $thisyear";
- $dashedDate = sprintf("%04d-%02d-%02d",$thisyear,$thismonth,$mday);
- $slashedDate = sprintf("%02d/%02d/%04d",$mday,$thismonth,$thisyear);
- $time=sprintf("%02d:%02d:%02d",$hour,$min,$sec);
- $shorttime=sprintf("%d:%02d",$hour,$min);
- $shortdatetime = "$thismon2 $mday, $shorttime";
-
- if ($date =~ /undefined/) {
- return "";
- } elsif ($format eq "date at time") {
- return "$date at $time";
- } elsif ($format eq "date") {
- return "$date";
- } elsif ($format eq "sdate") {
- return "$sdate";
- } elsif ($format eq "ddate") {
- return "$dashedDate";
- } elsif ($format eq "time") {
- return "$time";
- } elsif ($format eq "dateTtime+ms") {
- return $dashedDate . "T" . $time . "." . $milliseconds;
- } elsif ($format eq "dateTtime") {
- return $dashedDate . "T" . $time;
- } elsif ($format eq "yyyymmdd") {
- return sprintf("%04d%02d%02d",$thisyear,$thismonth,$mday);
- } elsif ($format eq "short date at time") {
- return $shortdatetime;
- } else {
- return "$date at $time";
- }
-}
-
-sub datetime_of_last_file_modification {
- local($this,$format,$filename) = @_;
-
- return $this->datetime($format,(stat($filename))[9]);
-}
-
-sub add_1sec {
- local($this,$datetime) = @_;
-
- if (($year,$month,$day,$hour,$minute,$second) = ($datetime =~ /^(\d\d\d\d)-(\d\d)-(\d\d)T(\d\d):(\d\d):(\d\d)$/)) {
- $second++;
- if ($second >= 60) { $second -= 60; $minute++; }
- if ($minute >= 60) { $minute -= 60; $hour++; }
- if ($hour >= 24) { $hour -= 24; $day++; }
- if ($month =~ /^(01|03|05|07|08|10|12)$/) {
- if ($day > 31) { $day -= 31; $month++; }
- } elsif ($month =~ /^(04|06|09|11)$/) {
- if ($day > 30) { $day -= 30; $month++; }
- } elsif (($month eq "02") && $this->leap_year($year)) {
- if ($day > 29) { $day -= 29; $month++; }
- } elsif ($month eq "02") {
- if ($day > 28) { $day -= 28; $month++; }
- }
- if ($month > 12) { $month -= 12; $year++; }
- return sprintf("%04d-%02d-%02dT%02d:%02d:%02d", $year,$month,$day,$hour,$minute,$second);
- } else {
- return "";
- }
-}
-
-sub stopwatch {
- local($this, $function, $id, *ht, *OUT) = @_;
- # function: start|stop|count|report; start|stop times are absolute (in secs.)
-
- my $current_time = time;
- # print OUT "Point S stopwatch $function $id $current_time\n";
- if ($function eq "start") {
- if ($ht{STOPWATCH_START}->{$id}) {
- $ht{STOPWATCH_N_RESTARTS}->{$id} = ($ht{STOPWATCH_N_RESTARTS}->{$id} || 0) + 1;
- } else {
- $ht{STOPWATCH_START}->{$id} = $current_time;
- }
- } elsif ($function eq "end") {
- if ($start_time = $ht{STOPWATCH_START}->{$id}) {
- $ht{STOPWATCH_TIME}->{$id} = ($ht{STOPWATCH_TIME}->{$id} || 0) + ($current_time - $start_time);
- $ht{STOPWATCH_START}->{$id} = "";
- } else {
- $ht{STOPWATCH_N_DEAD_ENDS}->{$id} = ($ht{STOPWATCH_N_DEAD_ENDS}->{$id} || 0) + 1;
- }
- } elsif ($function eq "count") {
- $ht{STOPWATCH_COUNT}->{$id} = ($ht{STOPWATCH_COUNT}->{$id} || 0) + 1;
- } elsif ($function eq "report") {
- my $id2;
- foreach $id2 (keys %{$ht{STOPWATCH_START}}) {
- if ($start_time = $ht{STOPWATCH_START}->{$id2}) {
- $ht{STOPWATCH_TIME}->{$id2} = ($ht{STOPWATCH_TIME}->{$id2} || 0) + ($current_time - $start_time);
- $ht{STOPWATCH_START}->{$id2} = $current_time;
- }
- }
- print OUT "Time report:\n";
- foreach $id2 (sort { $ht{STOPWATCH_TIME}->{$b} <=> $ht{STOPWATCH_TIME}->{$a} }
- keys %{$ht{STOPWATCH_TIME}}) {
- my $stopwatch_time = $ht{STOPWATCH_TIME}->{$id2};
- $stopwatch_time = $this->round_to_n_decimal_places($stopwatch_time, 3);
- my $n_restarts = $ht{STOPWATCH_N_RESTARTS}->{$id2};
- my $n_dead_ends = $ht{STOPWATCH_N_DEAD_ENDS}->{$id2};
- my $start_time = $ht{STOPWATCH_START}->{$id2};
- print OUT " $id2: $stopwatch_time seconds";
- print OUT " with $n_restarts restart(s)" if $n_restarts;
- print OUT " with $n_dead_ends dead end(s)" if $n_dead_ends;
- print OUT " (active)" if $start_time;
- print OUT "\n";
- }
- foreach $id2 (sort { $ht{STOPWATCH_COUNT}->{$b} <=> $ht{STOPWATCH_COUNT}->{$a} }
- keys %{$ht{STOPWATCH_COUNT}}) {
- $count = $ht{STOPWATCH_COUNT}->{$id2};
- print OUT " C $id2: $count\n";
- }
- }
-}
-
-sub print_html_banner {
- local($this,$text,$bgcolor,*OUT,$control) = @_;
-
- $control = "" unless defined($control);
- $bgcolor = "#BBCCFF" unless defined($bgcolor);
- print OUT "";
- print OUT " " unless $text =~ /^\s*<(table|nobr)/;
- print OUT $text;
- print OUT "
\n";
- print OUT " \n" unless $control =~ /nobr/i;
-}
-
-sub print_html_head {
- local($this, $title, *OUT, $control, $onload_fc, $add_javascript) = @_;
-
- $control = "" unless defined($control);
- $onload_fc = "" unless defined($onload_fc);
- $onload_clause = ($onload_fc) ? " onload=\"$onload_fc\"" : "";
- $add_javascript = "" unless defined($add_javascript);
- $max_age_clause = "";
- $max_age_clause = " "; # if $control =~ /\bexp1hour\b/;
- $css_clause = "";
- $css_clause = "\n " if $control =~ /css/;
- $css_clause .= "\n " if $control =~ /css/;
- $css_clause = "\n " if $control =~ /css-handheld/;
- $icon_clause = "";
- $icon_clause .= "\n " if $control =~ /\bAMR\b/i;
- $icon_clause .= "\n " if $control =~ /\bCRE\b/i;
- print OUT "\xEF\xBB\xBF\n" unless $control =~ /\bno-bom\b/; # utf8 marker byte order mark
- print OUT<
-
-
- $max_age_clause
- $title $css_clause$icon_clause
-END_OF_HEADER1
-;
-
- unless ($control =~ /no javascript/) {
- print OUT<
-
-
-END_OF_HEADER2
-;
- }
-
- print OUT<
-
-END_OF_HEADER3
-;
-}
-
-
-sub print_html_foot {
- local($this, *OUT) = @_;
-
- print OUT " \n";
- print OUT "\n";
-}
-
-sub print_html_page {
- local($this, *OUT, $s) = @_;
-
- print OUT "\xEF\xBB\xBF\n";
- print OUT "\n";
- print OUT " \n";
- print OUT " DEBUG \n";
- print OUT " \n";
- print OUT " \n";
- print OUT " \n";
- print OUT " \n";
- print OUT " $s\n";
- print OUT " \n";
- print OUT "\n";
-}
-
-sub http_catfile {
- local($this, @path) = @_;
-
- $result = File::Spec->catfile(@path);
- $result =~ s/(https?):\/([a-zA-Z])/$1:\/\/$2/;
- return $result;
-}
-
-sub underscore_to_space {
- local($this, $s) = @_;
-
- return "" unless defined($s);
-
- $s =~ s/_+/ /g;
- return $s;
-}
-
-sub space_to_underscore {
- local($this, $s) = @_;
-
- return "" unless defined($s);
-
- $s =~ s/ /_/g;
- return $s;
-}
-
-sub remove_spaces {
- local($this, $s) = @_;
-
- $s =~ s/\s//g;
- return $s;
-}
-
-sub is_punctuation_string_p {
- local($this, $s) = @_;
-
- return "" unless $s;
- $s = $this->normalize_string($s) if $s =~ /[\x80-\xBF]/;
- return $s =~ /^[-_,;:.?!\/\@+*"()]+$/;
-}
-
-sub is_rare_punctuation_string_p {
- local($this, $s) = @_;
-
- return 0 unless $s =~ /^[\x21-\x2F\x3A\x40\x5B-\x60\x7B-\x7E]{2,}$/;
- return 0 if $s =~ /^(\.{2,3}|-{2,3}|\*{2,3}|::|\@?[-\/:]\@?)$/;
- return 1;
-}
-
-sub simplify_punctuation {
- local($this, $s) = @_;
-
- $s =~ s/\xE2\x80\x92/-/g;
- $s =~ s/\xE2\x80\x93/-/g;
- $s =~ s/\xE2\x80\x94/-/g;
- $s =~ s/\xE2\x80\x95/-/g;
- $s =~ s/\xE2\x80\x98/`/g;
- $s =~ s/\xE2\x80\x99/'/g;
- $s =~ s/\xE2\x80\x9A/`/g;
- $s =~ s/\xE2\x80\x9C/"/g;
- $s =~ s/\xE2\x80\x9D/"/g;
- $s =~ s/\xE2\x80\x9E/"/g;
- $s =~ s/\xE2\x80\x9F/"/g;
- $s =~ s/\xE2\x80\xA2/*/g;
- $s =~ s/\xE2\x80\xA4/./g;
- $s =~ s/\xE2\x80\xA5/../g;
- $s =~ s/\xE2\x80\xA6/.../g;
- return $s;
-}
-
-sub latin_plus_p {
- local($this, $s, $control) = @_;
-
- $control = "" unless defined($control);
- return $s =~ /^([\x20-\x7E]|\xC2[\xA1-\xBF]|[\xC3-\xCC][\x80-\xBF]|\xCA[\x80-\xAF]|\xE2[\x80-\xAF][\x80-\xBF])+$/;
-}
-
-sub nth_line_in_file {
- local($this, $filename, $n) = @_;
-
- return "" unless $n =~ /^[1-9]\d*$/;
- open(IN, $filename) || return "";
- my $line_no = 0;
- while () {
- $line_no++;
- if ($n == $line_no) {
- $_ =~ s/\s+$//;
- close(IN);
- return $_;
- }
- }
- close(IN);
- return "";
-}
-
-sub read_file {
- local($this, $filename) = @_;
-
- my $file_content = "";
- open(IN, $filename) || return "";
- while () {
- $file_content .= $_;
- }
- close(IN);
- return $file_content;
-}
-
-sub cap_list {
- local($this, @list) = @_;
-
- @cap_list = ();
- foreach $l (@list) {
- ($premod, $core) = ($l =~ /^(a|an) (\S.*)$/);
- if (defined($premod) && defined($core)) {
- push(@cap_list, "$premod \u$core");
- } elsif ($this->cap_member($l, "US")) {
- push(@cap_list, uc $l);
- } else {
- push(@cap_list, "\u$l");
- }
- }
- return @cap_list;
-}
-
-sub integer_list_with_commas_and_ranges {
- local($this, @list) = @_;
-
- my $in_range_p = 0;
- my $last_value = 0;
- my $result = "";
- while (@list) {
- $elem = shift @list;
- if ($elem =~ /^\d+$/) {
- if ($in_range_p) {
- if ($elem == $last_value + 1) {
- $last_value = $elem;
- } else {
- $result .= "-$last_value, $elem";
- if (@list && ($next = $list[0]) && ($elem =~ /^\d+$/) && ($next =~ /^\d+$/)
- && ($next == $elem + 1)) {
- $last_value = $elem;
- $in_range_p = 1;
- } else {
- $in_range_p = 0;
- }
- }
- } else {
- $result .= ", $elem";
- if (@list && ($next = $list[0]) && ($elem =~ /^\d+$/) && ($next =~ /^\d+$/)
- && ($next == $elem + 1)) {
- $last_value = $elem;
- $in_range_p = 1;
- }
- }
- } else {
- if ($in_range_p) {
- $result .= "-$last_value, $elem";
- $in_range_p = 0;
- } else {
- $result .= ", $elem";
- }
- }
- }
- if ($in_range_p) {
- $result .= "-$last_value";
- }
- $result =~ s/^,\s*//;
- return $result;
-}
-
-sub comma_append {
- local($this, $a, $b) = @_;
-
- if (defined($a) && ($a =~ /\S/)) {
- if (defined($b) && ($b =~ /\S/)) {
- return "$a,$b";
- } else {
- return $a;
- }
- } else {
- if (defined($b) && ($b =~ /\S/)) {
- return $b;
- } else {
- return "";
- }
- }
-}
-
-sub version {
- return "3.17";
-}
-
-sub print_stderr {
- local($this, $message, $verbose) = @_;
-
- $verbose = 1 unless defined($verbose);
- print STDERR $message if $verbose;
- return 1;
-}
-
-sub print_log {
- local($this, $message, *LOG, $verbose) = @_;
-
- $verbose = 1 unless defined($verbose);
- print LOG $message if $verbose;
- return 1;
-}
-
-sub compare_alignment {
- local($this, $a, $b, $delimiter) = @_;
-
- $delimiter = "-" unless $delimiter;
- my @a_list = split($delimiter, $a);
- my @b_list = split($delimiter, $b);
-
- while (@a_list && @b_list) {
- $a_head = shift @a_list;
- $b_head = shift @b_list;
- next if $a_head eq $b_head;
- return $a_head <=> $b_head if ($a_head =~ /^\d+$/) && ($b_head =~ /^\d+$/);
- return $a_head cmp $b_head;
- }
- return -1 if @a_list;
- return 1 if @b_list;
- return 0;
-}
-
-sub normalize_string {
- # normalize punctuation, full-width characters (to ASCII)
- local($this, $s, $control) = @_;
-
- $control = "" unless defined($control);
-
- $norm_s = $s;
- $norm_s =~ tr/A-Z/a-z/;
-
- $norm_s =~ s/ \@([-:\/])/ $1/g; # non-initial left @
- $norm_s =~ s/^\@([-:\/])/$1/; # initial left @
- $norm_s =~ s/([-:\/])\@ /$1 /g; # non-initial right @
- $norm_s =~ s/([-:\/])\@$/$1/; # initial right @
- $norm_s =~ s/([\(\)"])([,;.?!])/$1 $2/g;
- $norm_s =~ s/\bcannot\b/can not/g;
-
- $norm_s =~ s/\xC2\xAD/-/g; # soft hyphen
-
- $norm_s =~ s/\xE2\x80\x94/-/g; # em dash
- $norm_s =~ s/\xE2\x80\x95/-/g; # horizontal bar
- $norm_s =~ s/\xE2\x80\x98/`/g; # grave accent
- $norm_s =~ s/\xE2\x80\x99/'/g; # apostrophe
- $norm_s =~ s/\xE2\x80\x9C/"/g; # left double quote mark
- $norm_s =~ s/\xE2\x80\x9D/"/g; # right double quote mark
- $norm_s =~ s/\xE2\x94\x80/-/g; # box drawings light horizontal
- $norm_s =~ s/\xE2\x94\x81/-/g; # box drawings heavy horizontal
- $norm_s =~ s/\xE3\x80\x81/,/g; # ideographic comma
- $norm_s =~ s/\xE3\x80\x82/./g; # ideographic full stop
- $norm_s =~ s/\xE3\x80\x88/"/g; # left angle bracket
- $norm_s =~ s/\xE3\x80\x89/"/g; # right angle bracket
- $norm_s =~ s/\xE3\x80\x8A/"/g; # left double angle bracket
- $norm_s =~ s/\xE3\x80\x8B/"/g; # right double angle bracket
- $norm_s =~ s/\xE3\x80\x8C/"/g; # left corner bracket
- $norm_s =~ s/\xE3\x80\x8D/"/g; # right corner bracket
- $norm_s =~ s/\xE3\x80\x8E/"/g; # left white corner bracket
- $norm_s =~ s/\xE3\x80\x8F/"/g; # right white corner bracket
- $norm_s =~ s/\xE3\x83\xBB/\xC2\xB7/g; # katakana middle dot -> middle dot
- $norm_s =~ s/\xEF\xBB\xBF//g; # UTF8 marker
-
- if ($control =~ /\bzh\b/i) {
- # de-tokenize Chinese
- unless ($control =~ /\bpreserve-tok\b/) {
- while ($norm_s =~ /[\xE0-\xEF][\x80-\xBF][\x80-\xBF] [\xE0-\xEF][\x80-\xBF][\x80-\xBF]/) {
- $norm_s =~ s/([\xE0-\xEF][\x80-\xBF][\x80-\xBF]) ([\xE0-\xEF][\x80-\xBF][\x80-\xBF])/$1$2/g;
- }
- $norm_s =~ s/([\xE0-\xEF][\x80-\xBF][\x80-\xBF]) ([\x21-\x7E])/$1$2/g;
- $norm_s =~ s/([\x21-\x7E]) ([\xE0-\xEF][\x80-\xBF][\x80-\xBF])/$1$2/g;
- }
-
- # fullwidth characters
- while ($norm_s =~ /\xEF\xBC[\x81-\xBF]/) {
- ($pre,$fullwidth,$post) = ($norm_s =~ /^(.*)(\xEF\xBC[\x81-\xBF])(.*)$/);
- $fullwidth =~ s/^\xEF\xBC//;
- $fullwidth =~ tr/[\x81-\xBF]/[\x21-\x5F]/;
- $norm_s = "$pre$fullwidth$post";
- }
- while ($norm_s =~ /\xEF\xBD[\x80-\x9E]/) {
- ($pre,$fullwidth,$post) = ($norm_s =~ /^(.*)(\xEF\xBD[\x80-\x9E])(.*)$/);
- $fullwidth =~ s/^\xEF\xBD//;
- $fullwidth =~ tr/[\x80-\x9E]/[\x60-\x7E]/;
- $norm_s = "$pre$fullwidth$post";
- }
- $norm_s =~ tr/A-Z/a-z/ unless $control =~ /\bpreserve-case\b/;
-
- unless ($control =~ /\bpreserve-tok\b/) {
- while ($norm_s =~ /[\x21-\x2F\x3A-\x40\x5B-\x60\x7B-\x7E] [\x21-\x2F\x3A-\x40\x5B-\x60\x7B-\x7E]/) {
- $norm_s =~ s/([\x21-\x2F\x3A-\x40\x5B-\x60\x7B-\x7E]) ([\x21-\x2F\x3A-\x40\x5B-\x60\x7B-\x7E])/$1$2/g;
- }
- $norm_s =~ s/([\x21-\x7E]) ([\x21-\x2F\x3A-\x40\x5B-\x60\x7B-\x7E])/$1$2/g;
- $norm_s =~ s/([\x21-\x2F\x3A-\x40\x5B-\x60\x7B-\x7E]) ([\x21-\x7E])/$1$2/g;
- $norm_s =~ s/ (\xC2\xA9|\xC2\xB7|\xC3\x97) /$1/g; # copyright sign, middle dot, multiplication sign
- }
- }
-
- if (($control =~ /\bzh\b/i) && ($control =~ /\bnorm-char\b/)) {
- $norm_s =~ s/\xE6\x96\xBC/\xE4\xBA\x8E/g; # feng1 (first char. of Chin. "lie low", line 1308)
- $norm_s =~ s/\xE6\xAD\xA7/\xE5\xB2\x90/g; # qi2 (second char. of Chin. "difference", line 1623)
- $norm_s =~ s/\xE8\x82\xB2/\xE6\xAF\x93/g; # yu4 (second char. of Chin. "sports", line 440)
- $norm_s =~ s/\xE8\x91\x97/\xE7\x9D\x80/g; # zhao (second char. of Chin. "prominent", line 4)
- $norm_s =~ s/\xE9\x81\x87/\xE8\xBF\x82/g; # yu4 (second char. of Chin. "good luck", line 959)
- }
-
- if ($control =~ /\bspurious-punct\b/) {
- $norm_s =~ s/^\s*[-_\." ]+//;
- $norm_s =~ s/[-_\." ]+\s*$//;
- $norm_s =~ s/\(\s+end\s+\)\s*$//i;
- $norm_s =~ s/^\s*null\s*$//i;
- }
-
- $norm_s =~ s/^\s+//;
- $norm_s =~ s/\s+$//;
- $norm_s =~ s/\s+/ /g;
-
- return $norm_s;
-}
-
-sub normalize_extreme_string {
- local($this, $s, $control) = @_;
-
- $control = "" unless defined($control);
-
- $norm_s = $s;
- $norm_s =~ s/\xE2\xA9\xBE/\xE2\x89\xA5/g; # slanted greater than or equal to
-
- return $norm_s;
-}
-
-sub increase_ht_count {
- local($this, *ht, $incr, @path) = @_;
-
- if ($#path == 0) {
- $ht{($path[0])} = ($ht{($path[0])} || 0) + $incr;
- } elsif ($#path == 1) {
- $ht{($path[0])}->{($path[1])}
- = ($ht{($path[0])}->{($path[1])} || 0) + $incr;
- } elsif ($#path == 2) {
- $ht{($path[0])}->{($path[1])}->{($path[2])}
- = ($ht{($path[0])}->{($path[1])}->{($path[2])} || 0) + $incr;
- } elsif ($#path == 3) {
- $ht{($path[0])}->{($path[1])}->{($path[2])}->{($path[3])}
- = ($ht{($path[0])}->{($path[1])}->{($path[2])}->{($path[3])} || 0) + $incr;
- } elsif ($#path == 4) {
- $ht{($path[0])}->{($path[1])}->{($path[2])}->{($path[3])}->{($path[4])}
- = ($ht{($path[0])}->{($path[1])}->{($path[2])}->{($path[3])}->{($path[4])} || 0) + $incr;
- } else {
- print STDERR "increase_ht_count unsupported for path of length " . ($#path + 1) . "\n";
- }
-}
-
-sub adjust_numbers {
- # non-negative integers
- local($this, $s, $delta) = @_;
-
- $result = "";
- while ($s =~ /\d/) {
- ($pre,$i,$post) = ($s =~ /^([^0-9]*)(\d+)([^0-9].*|)$/);
- $result .= $pre . ($i + $delta);
- $s = $post;
- }
- $result .= $s;
- return $result;
-}
-
-sub first_defined {
- local($this, @list) = @_;
-
- foreach $elem (@list) {
- return $elem if defined($elem);
- }
- return "";
-}
-
-sub first_defined_non_empty {
- local($this, @list) = @_;
-
- foreach $item (@list) {
- return $item if defined($item) && ($item ne "");
- }
- return "";
-}
-
-sub elem_after_member_list {
- local($this,$elem,@array) = @_;
-
- my @elem_after_member_list = ();
- foreach $i ((0 .. ($#array - 1))) {
- push(@elem_after_member_list, $array[$i+1]) if $elem eq $array[$i];
- }
- return join(" ", @elem_after_member_list);
-}
-
-sub add_value_to_list {
- local($this,$s,$value,$sep) = @_;
-
- $s = "" unless defined($s);
- $sep = "," unless defined($sep);
- return ($s =~ /\S/) ? "$s$sep$value" : $value;
-}
-
-sub add_new_value_to_list {
- local($this,$s,$value,$sep) = @_;
-
- $s = "" unless defined($s);
- $sep = "," unless defined($sep);
- my @values = split(/$sep/, $s);
- push(@values, $value) if defined($value) && ! $this->member($value, @values);
-
- return join($sep, @values);
-}
-
-sub add_new_hash_value_to_list {
- local($this,*ht,$key,$value,$sep) = @_;
-
- $sep = "," unless defined($sep);
- my $value_s = $ht{$key};
- if (defined($value_s)) {
- my @values = split(/$sep/, $value_s);
- push(@values, $value) unless $this->member($value, @values);
- $ht{$key} = join($sep, @values);
- } else {
- $ht{$key} = $value;
- }
-}
-
-sub ip_info {
- local($this, $ip_address) = @_;
-
- my %ip_map = ();
- $ip_map{"128.9.208.69"} = "Ulf Hermjakob (bach.isi.edu)";
- $ip_map{"128.9.208.169"} = "Ulf Hermjakob (brahms.isi.edu)";
- $ip_map{"128.9.184.148"} = "Ulf Hermjakob (beethoven.isi.edu ?)";
- $ip_map{"128.9.184.162"} = "Ulf Hermjakob (beethoven.isi.edu)";
- $ip_map{"128.9.176.39"} = "Kevin Knight";
- $ip_map{"128.9.184.187"} = "Kevin Knight";
- $ip_map{"128.9.216.56"} = "Kevin Knight";
- $ip_map{"128.9.208.155"} = "cage.isi.edu";
-
- return ($ip_name = $ip_map{$ip_address}) ? "$ip_address - $ip_name" : $ip_address;
-}
-
-# from standalone de-accent.pl
-sub de_accent_string {
- local($this, $s) = @_;
-
- $s =~ tr/A-Z/a-z/;
- unless (0) {
- # Latin-1
- if ($s =~ /\xC3[\x80-\xBF]/) {
- $s =~ s/(À|Á|Â|Ã|Ä|Å)/A/g;
- $s =~ s/Æ/Ae/g;
- $s =~ s/Ç/C/g;
- $s =~ s/Ð/D/g;
- $s =~ s/(È|É|Ê|Ë)/E/g;
- $s =~ s/(Ì|Í|Î|Ï)/I/g;
- $s =~ s/Ñ/N/g;
- $s =~ s/(Ò|Ó|Ô|Õ|Ö|Ø)/O/g;
- $s =~ s/(Ù|Ú|Û|Ü)/U/g;
- $s =~ s/Þ/Th/g;
- $s =~ s/Ý/Y/g;
- $s =~ s/(à|á|â|ã|ä|å)/a/g;
- $s =~ s/æ/ae/g;
- $s =~ s/ç/c/g;
- $s =~ s/(è|é|ê|ë)/e/g;
- $s =~ s/(ì|í|î|ï)/i/g;
- $s =~ s/ð/d/g;
- $s =~ s/ñ/n/g;
- $s =~ s/(ò|ó|ô|õ|ö)/o/g;
- $s =~ s/ß/ss/g;
- $s =~ s/þ/th/g;
- $s =~ s/(ù|ú|û|ü)/u/g;
- $s =~ s/(ý|ÿ)/y/g;
- }
- # Latin Extended-A
- if ($s =~ /[\xC4-\xC5][\x80-\xBF]/) {
- $s =~ s/(Ā|Ă|Ą)/A/g;
- $s =~ s/(ā|ă|ą)/a/g;
- $s =~ s/(Ć|Ĉ|Ċ|Č)/C/g;
- $s =~ s/(ć|ĉ|ċ|č)/c/g;
- $s =~ s/(Ď|Đ)/D/g;
- $s =~ s/(ď|đ)/d/g;
- $s =~ s/(Ē|Ĕ|Ė|Ę|Ě)/E/g;
- $s =~ s/(ē|ĕ|ė|ę|ě)/e/g;
- $s =~ s/(Ĝ|Ğ|Ġ|Ģ)/G/g;
- $s =~ s/(ĝ|ğ|ġ|ģ)/g/g;
- $s =~ s/(Ĥ|Ħ)/H/g;
- $s =~ s/(ĥ|ħ)/h/g;
- $s =~ s/(Ĩ|Ī|Ĭ|Į|İ)/I/g;
- $s =~ s/(ĩ|ī|ĭ|į|ı)/i/g;
- $s =~ s/IJ/Ij/g;
- $s =~ s/ij/ij/g;
- $s =~ s/Ĵ/J/g;
- $s =~ s/ĵ/j/g;
- $s =~ s/Ķ/K/g;
- $s =~ s/(ķ|ĸ)/k/g;
- $s =~ s/(Ĺ|Ļ|Ľ|Ŀ|Ł)/L/g;
- $s =~ s/(ļ|ľ|ŀ|ł)/l/g;
- $s =~ s/(Ń|Ņ|Ň|Ŋ)/N/g;
- $s =~ s/(ń|ņ|ň|ʼn|ŋ)/n/g;
- $s =~ s/(Ō|Ŏ|Ő)/O/g;
- $s =~ s/(ō|ŏ|ő)/o/g;
- $s =~ s/Œ/Oe/g;
- $s =~ s/œ/oe/g;
- $s =~ s/(Ŕ|Ŗ|Ř)/R/g;
- $s =~ s/(ŕ|ŗ|ř)/r/g;
- $s =~ s/(Ś|Ŝ|Ş|Š)/S/g;
- $s =~ s/(ś|ŝ|ş|š|ſ)/s/g;
- $s =~ s/(Ţ|Ť|Ŧ)/T/g;
- $s =~ s/(ţ|ť|ŧ)/t/g;
- $s =~ s/(Ũ|Ū|Ŭ|Ů|Ű|Ų)/U/g;
- $s =~ s/(ũ|ū|ŭ|ů|ű|ų)/u/g;
- $s =~ s/Ŵ/W/g;
- $s =~ s/ŵ/w/g;
- $s =~ s/(Ŷ|Ÿ)/Y/g;
- $s =~ s/ŷ/y/g;
- $s =~ s/(Ź|Ż|Ž)/Z/g;
- $s =~ s/(ź|ż|ž)/z/g;
- }
- # Latin Extended-B
- if ($s =~ /[\xC7-\xC7][\x80-\xBF]/) {
- $s =~ s/(\xC7\x8D)/A/g;
- $s =~ s/(\xC7\x8E)/a/g;
- $s =~ s/(\xC7\x8F)/I/g;
- $s =~ s/(\xC7\x90)/i/g;
- $s =~ s/(\xC7\x91)/O/g;
- $s =~ s/(\xC7\x92)/o/g;
- $s =~ s/(\xC7\x93)/U/g;
- $s =~ s/(\xC7\x94)/u/g;
- $s =~ s/(\xC7\x95)/U/g;
- $s =~ s/(\xC7\x96)/u/g;
- $s =~ s/(\xC7\x97)/U/g;
- $s =~ s/(\xC7\x98)/u/g;
- $s =~ s/(\xC7\x99)/U/g;
- $s =~ s/(\xC7\x9A)/u/g;
- $s =~ s/(\xC7\x9B)/U/g;
- $s =~ s/(\xC7\x9C)/u/g;
- }
- # Latin Extended Additional
- if ($s =~ /\xE1[\xB8-\xBF][\x80-\xBF]/) {
- $s =~ s/(ḁ|ạ|ả|ấ|ầ|ẩ|ẫ|ậ|ắ|ằ|ẳ|ẵ|ặ|ẚ)/a/g;
- $s =~ s/(ḃ|ḅ|ḇ)/b/g;
- $s =~ s/(ḉ)/c/g;
- $s =~ s/(ḋ|ḍ|ḏ|ḑ|ḓ)/d/g;
- $s =~ s/(ḕ|ḗ|ḙ|ḛ|ḝ|ẹ|ẻ|ẽ|ế|ề|ể|ễ|ệ)/e/g;
- $s =~ s/(ḟ)/f/g;
- $s =~ s/(ḡ)/g/g;
- $s =~ s/(ḣ|ḥ|ḧ|ḩ|ḫ)/h/g;
- $s =~ s/(ḭ|ḯ|ỉ|ị)/i/g;
- $s =~ s/(ḱ|ḳ|ḵ)/k/g;
- $s =~ s/(ḷ|ḹ|ḻ|ḽ)/l/g;
- $s =~ s/(ḿ|ṁ|ṃ)/m/g;
- $s =~ s/(ṅ|ṇ|ṉ|ṋ)/m/g;
- $s =~ s/(ọ|ỏ|ố|ồ|ổ|ỗ|ộ|ớ|ờ|ở|ỡ|ợ|ṍ|ṏ|ṑ|ṓ)/o/g;
- $s =~ s/(ṕ|ṗ)/p/g;
- $s =~ s/(ṙ|ṛ|ṝ|ṟ)/r/g;
- $s =~ s/(ṡ|ṣ|ṥ|ṧ|ṩ|ẛ)/s/g;
- $s =~ s/(ṫ|ṭ|ṯ|ṱ)/t/g;
- $s =~ s/(ṳ|ṵ|ṷ|ṹ|ṻ|ụ|ủ|ứ|ừ|ử|ữ|ự)/u/g;
- $s =~ s/(ṽ|ṿ)/v/g;
- $s =~ s/(ẁ|ẃ|ẅ|ẇ|ẉ|ẘ)/w/g;
- $s =~ s/(ẋ|ẍ)/x/g;
- $s =~ s/(ẏ|ỳ|ỵ|ỷ|ỹ|ẙ)/y/g;
- $s =~ s/(ẑ|ẓ|ẕ)/z/g;
- $s =~ s/(Ḁ|Ạ|Ả|Ấ|Ầ|Ẩ|Ẫ|Ậ|Ắ|Ằ|Ẳ|Ẵ|Ặ)/A/g;
- $s =~ s/(Ḃ|Ḅ|Ḇ)/B/g;
- $s =~ s/(Ḉ)/C/g;
- $s =~ s/(Ḋ|Ḍ|Ḏ|Ḑ|Ḓ)/D/g;
- $s =~ s/(Ḕ|Ḗ|Ḙ|Ḛ|Ḝ|Ẹ|Ẻ|Ẽ|Ế|Ề|Ể|Ễ|Ệ)/E/g;
- $s =~ s/(Ḟ)/F/g;
- $s =~ s/(Ḡ)/G/g;
- $s =~ s/(Ḣ|Ḥ|Ḧ|Ḩ|Ḫ)/H/g;
- $s =~ s/(Ḭ|Ḯ|Ỉ|Ị)/I/g;
- $s =~ s/(Ḱ|Ḳ|Ḵ)/K/g;
- $s =~ s/(Ḷ|Ḹ|Ḻ|Ḽ)/L/g;
- $s =~ s/(Ḿ|Ṁ|Ṃ)/M/g;
- $s =~ s/(Ṅ|Ṇ|Ṉ|Ṋ)/N/g;
- $s =~ s/(Ṍ|Ṏ|Ṑ|Ṓ|Ọ|Ỏ|Ố|Ồ|Ổ|Ỗ|Ộ|Ớ|Ờ|Ở|Ỡ|Ợ)/O/g;
- $s =~ s/(Ṕ|Ṗ)/P/g;
- $s =~ s/(Ṙ|Ṛ|Ṝ|Ṟ)/R/g;
- $s =~ s/(Ṡ|Ṣ|Ṥ|Ṧ|Ṩ)/S/g;
- $s =~ s/(Ṫ|Ṭ|Ṯ|Ṱ)/T/g;
- $s =~ s/(Ṳ|Ṵ|Ṷ|Ṹ|Ṻ|Ụ|Ủ|Ứ|Ừ|Ử|Ữ|Ự)/U/g;
- $s =~ s/(Ṽ|Ṿ)/V/g;
- $s =~ s/(Ẁ|Ẃ|Ẅ|Ẇ|Ẉ)/W/g;
- $s =~ s/(Ẍ)/X/g;
- $s =~ s/(Ẏ|Ỳ|Ỵ|Ỷ|Ỹ)/Y/g;
- $s =~ s/(Ẑ|Ẓ|Ẕ)/Z/g;
- }
- # Greek letters
- if ($s =~ /\xCE[\x86-\xAB]/) {
- $s =~ s/ά/α/g;
- $s =~ s/έ/ε/g;
- $s =~ s/ί/ι/g;
- $s =~ s/ϊ/ι/g;
- $s =~ s/ΐ/ι/g;
- $s =~ s/ό/ο/g;
- $s =~ s/ύ/υ/g;
- $s =~ s/ϋ/υ/g;
- $s =~ s/ΰ/υ/g;
- $s =~ s/ώ/ω/g;
- $s =~ s/Ά/Α/g;
- $s =~ s/Έ/Ε/g;
- $s =~ s/Ή/Η/g;
- $s =~ s/Ί/Ι/g;
- $s =~ s/Ϊ/Ι/g;
- $s =~ s/Ύ/Υ/g;
- $s =~ s/Ϋ/Υ/g;
- $s =~ s/Ώ/Ω/g;
- }
- # Cyrillic letters
- if ($s =~ /\xD0[\x80-\xAF]/) {
- $s =~ s/Ѐ/Е/g;
- $s =~ s/Ё/Е/g;
- $s =~ s/Ѓ/Г/g;
- $s =~ s/Ќ/К/g;
- $s =~ s/Ѝ/И/g;
- $s =~ s/Й/И/g;
- $s =~ s/ѐ/е/g;
- $s =~ s/ё/е/g;
- $s =~ s/ѓ/г/g;
- $s =~ s/ќ/к/g;
- $s =~ s/ѝ/и/g;
- $s =~ s/й/и/g;
- }
- }
- return $s;
-}
-
-sub read_de_accent_case_resource {
- local($this, $filename, *ht, *LOG, $verbose) = @_;
- # e.g. data/char-de-accent-lc.txt
-
- if (open(IN, $filename)) {
- my $mode = "de-accent";
- my $line_number = 0;
- my $n_de_accent_targets = 0;
- my $n_de_accent_sources = 0;
- my $n_case_entries = 0;
- while () {
- s/^\xEF\xBB\xBF//;
- s/\s*$//;
- $line_number++;
- if ($_ =~ /^#+\s*CASE\b/) {
- $mode = "case";
- } elsif ($_ =~ /^#+\s*PUNCTUATION NORMALIZATION\b/) {
- $mode = "punctuation-normalization";
- } elsif ($_ =~ /^#/) {
- # ignore comment
- } elsif ($_ =~ /^\s*$/) {
- # ignore empty line
- } elsif (($mode eq "de-accent") && (($char_without_accent, @chars_with_accent) = split(/\s+/, $_))) {
- if (keys %{$ht{DE_ACCENT_INV}->{$char_without_accent}}) {
- print LOG "Ignoring duplicate de-accent line for target $char_without_accent in l.$line_number in $filename\n" unless $char_without_accent eq "--";
- } elsif (@chars_with_accent) {
- $n_de_accent_targets++;
- foreach $char_with_accent (@chars_with_accent) {
- my @prev_target_chars = keys %{$ht{DE_ACCENT}->{$char_with_accent}};
- print LOG "Accent character $char_with_accent has duplicate target $char_without_accent (besides @prev_target_chars) in l.$line_number in $filename\n" if @prev_target_chars && (! ($char_without_accent =~ /^[aou]e$/i));
- $char_without_accent = "" if $char_without_accent eq "--";
- $ht{DE_ACCENT}->{$char_with_accent}->{$char_without_accent} = 1;
- $ht{DE_ACCENT1}->{$char_with_accent} = $char_without_accent
- if (! defined($ht{DE_ACCENT1}->{$char_with_accent}))
- && ($char_without_accent =~ /^.[\x80-\xBF]*$/);
- $ht{DE_ACCENT_INV}->{$char_without_accent}->{$char_with_accent} = 1;
- $ht{UPPER_CASE_OR_ACCENTED}->{$char_with_accent} = 1;
- $n_de_accent_sources++;
- }
- } else {
- print LOG "Empty de-accent list for $char_without_accent in l.$line_number in $filename\n";
- }
- } elsif (($mode eq "punctuation-normalization") && (($norm_punct, @unnorm_puncts) = split(/\s+/, $_))) {
- if (keys %{$ht{NORM_PUNCT_INV}->{$norm_punct}}) {
- print LOG "Ignoring duplicate punctuation-normalization line for target $norm_punct in l.$line_number in $filename\n";
- } elsif (@unnorm_puncts) {
- foreach $unnorm_punct (@unnorm_puncts) {
- my $prev_norm_punct = $ht{NORM_PUNCT}->{$unnorm_punct};
- if ($prev_norm_punct) {
- print LOG "Ignoring duplicate punctuation normalization $unnorm_punct -> $norm_punct (besides $prev_norm_punct) in l.$line_number in $filename\n";
- }
- $ht{NORM_PUNCT}->{$unnorm_punct} = $norm_punct;
- $ht{NORM_PUNCT_INV}->{$norm_punct}->{$unnorm_punct} = 1;
- $ht{LC_DE_ACCENT_CHAR_NORM_PUNCT}->{$unnorm_punct} = $norm_punct;
- }
- }
- } elsif (($mode eq "case") && (($uc_char, $lc_char) = ($_ =~ /^(\S+)\s+(\S+)\s*$/))) {
- $ht{UPPER_TO_LOWER_CASE}->{$uc_char} = $lc_char;
- $ht{LOWER_TO_UPPER_CASE}->{$lc_char} = $uc_char;
- $ht{UPPER_CASE_P}->{$uc_char} = 1;
- $ht{LOWER_CASE_P}->{$lc_char} = 1;
- $ht{UPPER_CASE_OR_ACCENTED}->{$uc_char} = 1;
- $n_case_entries++;
- } else {
- print LOG "Unrecognized l.$line_number in $filename\n";
- }
- }
- foreach $char (keys %{$ht{UPPER_CASE_OR_ACCENTED}}) {
- my $lc_char = $ht{UPPER_TO_LOWER_CASE}->{$char};
- $lc_char = $char unless defined($lc_char);
- my @de_accend_char_results = sort keys %{$ht{DE_ACCENT}->{$lc_char}};
- my $new_char = (@de_accend_char_results) ? $de_accend_char_results[0] : $lc_char;
- $ht{LC_DE_ACCENT_CHAR}->{$char} = $new_char;
- $ht{LC_DE_ACCENT_CHAR_NORM_PUNCT}->{$char} = $new_char;
- }
- close(IN);
- print LOG "Found $n_case_entries case entries, $n_de_accent_sources/$n_de_accent_targets source/target entries in $line_number lines in file $filename\n" if $verbose;
- } else {
- print LOG "Can't open $filename\n";
- }
-}
-
-sub de_accent_char {
- local($this, $char, *ht, $default) = @_;
-
- @de_accend_char_results = sort keys %{$ht{DE_ACCENT}->{$char}};
- return (@de_accend_char_results) ? @de_accend_char_results : ($default);
-}
-
-sub lower_case_char {
- local($this, $char, *ht, $default) = @_;
-
- return (defined($lc = $ht{UPPER_TO_LOWER_CASE}->{$char})) ? $lc : $default;
-}
-
-sub lower_case_and_de_accent_char {
- local($this, $char, *ht) = @_;
-
- my $lc_char = $this->lower_case_char($char, *ht, $char);
- return $this->de_accent_char($lc_char, *ht, $lc_char);
-}
-
-sub lower_case_and_de_accent_string {
- local($this, $string, *ht, $control) = @_;
-
- # $this->stopwatch("start", "lower_case_and_de_accent_string", *ht, *LOG);
- my $norm_punct_p = ($control && ($control =~ /norm-punct/i));
- my @chars = $this->split_into_utf8_characters($string);
- my $result = "";
- foreach $char (@chars) {
- my @lc_de_accented_chars = $this->lower_case_and_de_accent_char($char, *ht);
- if ($norm_punct_p
- && (! @lc_de_accented_chars)) {
- my $norm_punct = $ht{NORM_PUNCT}->{$char};
- @lc_de_accented_chars = ($norm_punct) if $norm_punct;
- }
- $result .= ((@lc_de_accented_chars) ? $lc_de_accented_chars[0] : $char);
- }
- # $this->stopwatch("end", "lower_case_and_de_accent_string", *ht, *LOG);
- return $result;
-}
-
-sub lower_case_and_de_accent_norm_punct {
- local($this, $char, *ht) = @_;
-
- my $new_char = $ht{LC_DE_ACCENT_CHAR_NORM_PUNCT}->{$char};
- return (defined($new_char)) ? $new_char : $char;
-}
-
-sub lower_case_and_de_accent_string2 {
- local($this, $string, *ht, $control) = @_;
-
- my $norm_punct_p = ($control && ($control =~ /norm-punct/i));
- # $this->stopwatch("start", "lower_case_and_de_accent_string2", *ht, *LOG);
- my $s = $string;
- my $result = "";
- while (($char, $rest) = ($s =~ /^(.[\x80-\xBF]*)(.*)$/)) {
- my $new_char = $ht{LC_DE_ACCENT_CHAR}->{$char};
- if (defined($new_char)) {
- $result .= $new_char;
- } elsif ($norm_punct_p && defined($new_char = $ht{NORM_PUNCT}->{$char})) {
- $result .= $new_char;
- } else {
- $result .= $char;
- }
- $s = $rest;
- }
- # $this->stopwatch("end", "lower_case_and_de_accent_string2", *ht, *LOG);
- return $result;
-}
-
-sub lower_case_string {
- local($this, $string, *ht, $control) = @_;
-
- my $norm_punct_p = ($control && ($control =~ /norm-punct/i));
- my $s = $string;
- my $result = "";
- while (($char, $rest) = ($s =~ /^(.[\x80-\xBF]*)(.*)$/)) {
- my $lc_char = $ht{UPPER_TO_LOWER_CASE}->{$char};
- if (defined($lc_char)) {
- $result .= $lc_char;
- } elsif ($norm_punct_p && defined($new_char = $ht{NORM_PUNCT}->{$char})) {
- $result .= $new_char;
- } else {
- $result .= $char;
- }
- $s = $rest;
- }
- return $result;
-}
-
-sub round_to_n_decimal_places {
- local($this, $x, $n, $fill_decimals_p) = @_;
-
- $fill_decimals_p = 0 unless defined($fill_decimals_p);
- unless (defined($x)) {
- return $x;
- }
- if (($x =~ /^-?\d+$/) && (! $fill_decimals_p)) {
- return $x;
- }
- $factor = 1;
- foreach $i ((1 .. $n)) {
- $factor *= 10;
- }
- my $rounded_number;
- if ($x > 0) {
- $rounded_number = (int(($factor * $x) + 0.5) / $factor);
- } else {
- $rounded_number = (int(($factor * $x) - 0.5) / $factor);
- }
- if ($fill_decimals_p) {
- ($period, $decimals) = ($rounded_number =~ /^-?\d+(\.?)(\d*)$/);
- $rounded_number .= "." unless $period || ($n == 0);
- foreach ((1 .. ($n - length($decimals)))) {
- $rounded_number .= 0;
- }
- }
- return $rounded_number;
-}
-
-sub commify {
- local($caller,$number) = @_;
-
- my $text = reverse $number;
- $text =~ s/(\d\d\d)(?=\d)(?!\d*\.)/$1,/g;
- return scalar reverse $text;
-}
-
-sub add_javascript_functions {
- local($caller,@function_names) = @_;
-
- $add_javascript_function_s = "";
- foreach $function_name (@function_names) {
-
- if ($function_name eq "highlight_elems") {
- $add_javascript_function_s .= "
- function highlight_elems(group_id, value) {
- if (group_id != '') {
- i = 1;
- id = group_id + '-' + i;
- while ((s = document.getElementById(id)) != null) {
- if (! s.origColor) {
- if (s.style.color) {
- s.origColor = s.style.color;
- } else {
- s.origColor = '#000000';
- }
- }
- if (value == '1') {
- s.style.color = '#0000FF';
- if (s.innerHTML == '-') {
- s.style.innerHtml = s.innerHTML;
- s.innerHTML = '- ← here ';
- s.style.fontWeight = 900;
- } else {
- s.style.fontWeight = 'bold';
- }
- } else {
- s.style.fontWeight = 'normal';
- s.style.color = s.origColor;
- if (s.style.innerHtml != null) {
- s.innerHTML = s.style.innerHtml;
- }
- }
- i = i + 1;
- id = group_id + '-' + i;
- }
- }
- }
-";
- } elsif ($function_name eq "set_style_for_ids") {
- $add_javascript_function_s .= "
- function set_style_for_ids(style,id_list) {
- var ids = id_list.split(/\\s+/);
- var len = ids.length;
- var s;
- for (var i=0; i>$filename")) {
- print OUT $s;
- close(OUT);
- $result = "Appended";
- } else {
- $result = "Can't append";
- }
- } else {
- if (open(OUT, ">$filename")) {
- print OUT $s;
- close(OUT);
- $result = "Wrote";
- } else {
- $result = "Can't write";
- }
- }
- chmod($mod, $filename) if defined($mod) && -e $filename;
- return $result;
-}
-
-sub square {
- local($caller, $x) = @_;
-
- return $x * $x;
-}
-
-sub mutual_info {
- local($caller, $ab_count, $a_count, $b_count, $total_count, $smoothing) = @_;
-
- $smoothing = 1 unless defined($smoothing);
- $ab_count = 0 unless defined($ab_count);
- return 0 unless $a_count && $b_count && $total_count;
-
- my $p_ab = $ab_count / $total_count;
- my $p_a = $a_count / $total_count;
- my $p_b = $b_count / $total_count;
- my $expected_ab = $p_a * $p_b * $total_count;
-
- return -99 unless $expected_ab || $smoothing;
-
- return CORE::log(($ab_count + $smoothing) / ($expected_ab + $smoothing));
-}
-
-sub mutual_info_multi {
- local($caller, $multi_count, $total_count, $smoothing, @counts) = @_;
-
- return 0 unless $total_count;
- my $p_indivuals = 1;
- foreach $count (@counts) {
- return 0 unless $count;
- $p_indivuals *= ($count / $total_count);
- }
- my $expected_multi_count = $p_indivuals * $total_count;
- # print STDERR "actual vs. expected multi_count($multi_count, $total_count, $smoothing, @counts) = $multi_count vs. $expected_multi_count\n";
-
- return -99 unless $expected_multi_count || $smoothing;
-
- return CORE::log(($multi_count + $smoothing) / ($expected_multi_count + $smoothing));
-}
-
-sub precision_recall_fmeasure {
- local($caller, $n_gold, $n_test, $n_shared, $pretty_print_p) = @_;
-
- unless (($n_gold =~ /^[1-9]\d*$/) && ($n_test =~ /^[1-9]\d*$/)) {
- $zero = ($pretty_print_p) ? "0%" : 0;
- if ($n_gold =~ /^[1-9]\d*$/) {
- return ("n/a", $zero, $zero);
- } elsif ($n_test =~ /^[1-9]\d*$/) {
- return ($zero, "n/a", $zero);
- } else {
- return ("n/a", "n/a", "n/a");
- }
- }
- my $precision = $n_shared / $n_test;
- my $recall = $n_shared / $n_gold;
- my $f_measure = ($precision * $recall * 2) / ($precision + $recall);
-
- return ($precision, $recall, $f_measure) unless $pretty_print_p;
-
- my $pretty_precision = $caller->round_to_n_decimal_places(100*$precision, 1) . "%";
- my $pretty_recall = $caller->round_to_n_decimal_places(100*$recall, 1) . "%";
- my $pretty_f_measure = $caller->round_to_n_decimal_places(100*$f_measure, 1) . "%";
-
- return ($pretty_precision, $pretty_recall, $pretty_f_measure);
-}
-
-sub recapitalize_named_entity {
- local($caller, $s) = @_;
-
- my @comps = ();
- foreach $comp (split(/\s+/, $s)) {
- if ($comp =~ /^(and|da|for|of|on|the|van|von)$/) {
- push(@comps, $comp);
- } elsif ($comp =~ /^[a-z]/) {
- push(@comps, ucfirst $comp);
- } else {
- push(@comps, $comp);
- }
- }
- return join(" ", @comps);
-}
-
-sub slot_value_in_double_colon_del_list {
- local($this, $s, $slot, $default) = @_;
-
- $default = "" unless defined($default);
- if (($value) = ($s =~ /::$slot\s+(\S.*\S|\S)\s*$/)) {
- $value =~ s/\s*::\S.*\s*$//;
- return $value;
- } else {
- return $default;
- }
-}
-
-sub synt_in_double_colon_del_list {
- local($this, $s) = @_;
-
- ($value) = ($s =~ /::synt\s+(\S+|\S.*?\S)(?:\s+::.*)?$/);
- return (defined($value)) ? $value : "";
-}
-
-sub form_in_double_colon_del_list {
- local($this, $s) = @_;
-
- ($value) = ($s =~ /::form\s+(\S+|\S.*?\S)(?:\s+::.*)?$/);
- return (defined($value)) ? $value : "";
-}
-
-sub lex_in_double_colon_del_list {
- local($this, $s) = @_;
-
- ($value) = ($s =~ /::lex\s+(\S+|\S.*?\S)(?:\s+::.*)?$/);
- return (defined($value)) ? $value : "";
-}
-
-sub multi_slot_value_in_double_colon_del_list {
- # e.g. when there are multiple slot/value pairs in a line, e.g. ::eng ... :eng ...
- local($this, $s, $slot) = @_;
-
- @values = ();
- while (($value, $rest) = ($s =~ /::$slot\s+(\S|\S.*?\S)(\s+::\S.*|\s*)$/)) {
- push(@values, $value);
- $s = $rest;
- }
- return @values;
-}
-
-sub remove_slot_in_double_colon_del_list {
- local($this, $s, $slot) = @_;
-
- $s =~ s/::$slot(?:|\s+\S|\s+\S.*?\S)(\s+::\S.*|\s*)$/$1/;
- $s =~ s/^\s*//;
- return $s;
-}
-
-sub extract_split_info_from_split_dir {
- local($this, $dir, *ht) = @_;
-
- my $n_files = 0;
- my $n_snt_ids = 0;
- if (opendir(DIR, $dir)) {
- my @filenames = sort readdir(DIR);
- closedir(DIR);
- foreach $filename (@filenames) {
- next unless $filename =~ /\.txt$/;
- my $split_class;
- if (($split_class) = ($filename =~ /-(dev|training|test)-/)) {
- my $full_filename = "$dir/$filename";
- if (open(IN, $full_filename)) {
- my $old_n_snt_ids = $n_snt_ids;
- while () {
- if (($snt_id) = ($_ =~ /^#\s*::id\s+(\S+)/)) {
- if ($old_split_class = $ht{SPLIT_CLASS}->{$snt_id}) {
- unless ($old_split_class eq $split_class) {
- print STDERR "Conflicting split class for $snt_id: $old_split_class $split_class\n";
- }
- } else {
- $ht{SPLIT_CLASS}->{$snt_id} = $split_class;
- $ht{SPLIT_CLASS_COUNT}->{$split_class} = ($ht{SPLIT_CLASS_COUNT}->{$split_class} || 0) + 1;
- $n_snt_ids++;
- }
- }
- }
- $n_files++ unless $n_snt_ids == $old_n_snt_ids;
- close(IN);
- } else {
- print STDERR "Can't open file $full_filename";
- }
- } else {
- print STDERR "Skipping file $filename when extracting split info from $dir\n";
- }
- }
- print STDERR "Extracted $n_snt_ids split classes from $n_files files.\n";
- } else {
- print STDERR "Can't open directory $dir to extract split info.\n";
- }
-}
-
-sub extract_toks_for_split_class_from_dir {
- local($this, $dir, *ht, $split_class, $control) = @_;
-
- $control = "" unless defined($control);
- $print_snt_id_p = ($control =~ /\bwith-snt-id\b/);
- my $n_files = 0;
- my $n_snts = 0;
- if (opendir(DIR, $dir)) {
- my @filenames = sort readdir(DIR);
- closedir(DIR);
- foreach $filename (@filenames) {
- next unless $filename =~ /^alignment-release-.*\.txt$/;
- my $full_filename = "$dir/$filename";
- if (open(IN, $full_filename)) {
- my $old_n_snts = $n_snts;
- my $snt_id = "";
- while () {
- if (($s_value) = ($_ =~ /^#\s*::id\s+(\S+)/)) {
- $snt_id = $s_value;
- $proper_split_class_p
- = ($this_split_class = $ht{SPLIT_CLASS}->{$snt_id})
- && ($this_split_class eq $split_class);
- } elsif (($tok) = ($_ =~ /^#\s*::tok\s+(\S|\S.*\S)\s*$/)) {
- if ($proper_split_class_p) {
- print "$snt_id " if $print_snt_id_p;
- print "$tok\n";
- $n_snts++;
- }
- }
- }
- $n_files++ unless $n_snts == $old_n_snts;
- close(IN);
- } else {
- print STDERR "Can't open file $full_filename";
- }
- }
- print STDERR "Extracted $n_snts tokenized sentences ($split_class) from $n_files files.\n";
- } else {
- print STDERR "Can't open directory $dir to extract tokens.\n";
- }
-}
-
-sub load_relevant_tok_ngram_corpus {
- local($this, $filename, *ht, $max_lex_rule_span, $ngram_count_min, $optional_ngram_output_filename) = @_;
-
- $ngram_count_min = 1 unless $ngram_count_min;
- $max_lex_rule_span = 10 unless $max_lex_rule_span;
- my $n_ngram_instances = 0;
- my $n_ngram_types = 0;
- if (open(IN, $filename)) {
- while () {
- s/\s*$//;
- @tokens = split(/\s+/, $_);
- foreach $from_token_index ((0 .. $#tokens)) {
- foreach $to_token_index (($from_token_index .. ($from_token_index + $max_lex_rule_span -1))) {
- last if $to_token_index > $#tokens;
- my $ngram = join(" ", @tokens[$from_token_index .. $to_token_index]);
- $ht{RELEVANT_NGRAM}->{$ngram} = ($ht{RELEVANT_NGRAM}->{$ngram} || 0) + 1;
- }
- }
- }
- close(IN);
- if ($optional_ngram_output_filename && open(OUT, ">$optional_ngram_output_filename")) {
- foreach $ngram (sort keys %{$ht{RELEVANT_NGRAM}}) {
- $count = $ht{RELEVANT_NGRAM}->{$ngram};
- next unless $count >= $ngram_count_min;
- print OUT "($count) $ngram\n";
- $n_ngram_types++;
- $n_ngram_instances += $count;
- }
- close(OUT);
- print STDERR "Extracted $n_ngram_types ngram types, $n_ngram_instances ngram instances.\n";
- print STDERR "Wrote ngram stats to $optional_ngram_output_filename\n";
- }
- } else {
- print STDERR "Can't open relevant tok ngram corpus $filename\n";
- }
-}
-
-sub load_relevant_tok_ngrams {
- local($this, $filename, *ht) = @_;
-
- my $n_entries = 0;
- if (open(IN, $filename)) {
- while () {
- s/\s*$//;
- if (($count, $ngram) = ($_ =~ /^\((\d+)\)\s+(\S|\S.*\S)\s*$/)) {
- $lc_ngram = lc $ngram;
- $ht{RELEVANT_NGRAM}->{$lc_ngram} = ($ht{RELEVANT_NGRAM}->{$lc_ngram} || 0) + $count;
- $ht{RELEVANT_LC_NGRAM}->{$lc_ngram} = ($ht{RELEVANT_LC_NGRAM}->{$lc_ngram} || 0) + $count;
- $n_entries++;
- }
- }
- close(IN);
- print STDERR "Read in $n_entries entries from $filename\n";
- } else {
- print STDERR "Can't open relevant tok ngrams from $filename\n";
- }
-}
-
-sub snt_id_sort_function {
- local($this, $a, $b) = @_;
-
- if ((($core_a, $index_a) = ($a =~ /^(\S+)\.(\d+)$/))
- && (($core_b, $index_b) = ($b =~ /^(\S+)\.(\d+)$/))) {
- return ($core_a cmp $core_b) || ($index_a <=> $index_b);
- } else {
- return $a cmp $b;
- }
-}
-
-sub count_value_sort_function {
- local($this, $a_count, $b_count, $a_value, $b_value, $control) = @_;
-
- # normalize fractions such as "1/2"
- if ($a_count > $b_count) {
- return ($control eq "decreasing") ? -1 : 1;
- } elsif ($b_count > $a_count) {
- return ($control eq "decreasing") ? 1 : -1;
- }
- $a_value = $num / $den if ($num, $den) = ($a_value =~ /^([1-9]\d*)\/([1-9]\d*)$/);
- $b_value = $num / $den if ($num, $den) = ($b_value =~ /^([1-9]\d*)\/([1-9]\d*)$/);
- $a_value =~ s/:/\./ if $a_value =~ /^\d+:\d+$/;
- $b_value =~ s/:/\./ if $b_value =~ /^\d+:\d+$/;
- if (($a_value =~ /^-?\d+(\.\d+)?$/)
- && ($b_value =~ /^-?\d+(\.\d+)?$/)) {
- return $a_value <=> $b_value;
- } elsif ($a_value =~ /^-?\d+(\.\d+)?$/) {
- return 1;
- } elsif ($b_value =~ /^-?\d+(\.\d+)?$/) {
- return -1;
- } else {
- return $a_value cmp $b_value;
- }
-}
-
-sub undef_to_blank {
- local($this, $x) = @_;
-
- return (defined($x)) ? $x : "";
-}
-
-sub en_lex_amr_list {
- local($this, $s) = @_;
-
- $bpe = qr{ \( (?: (?> [^()]+ ) | (??{ $bpe }))* \) }x; # see Perl Cookbook 2nd ed. p. 218
- @en_lex_amr_list = ();
- my $amr_s;
- my $lex;
- my $test;
- while ($s =~ /\S/) {
- $s =~ s/^\s*//;
- if (($s =~ /^\([a-z]\d* .*\)/)
- && (($amr_s, $rest) = ($s =~ /^($bpe)(\s.*|)$/))) {
- push(@en_lex_amr_list, $amr_s);
- $s = $rest;
- } elsif (($lex, $rest) = ($s =~ /^\s*(\S+)(\s.*|)$/)) {
- push(@en_lex_amr_list, $lex);
- $s = $rest;
- } else {
- print STDERR "en_lex_amr_list can't process: $s\n";
- $s = "";
- }
- }
- return @en_lex_amr_list;
-}
-
-sub make_sure_dir_exists {
- local($this, $dir, $umask) = @_;
-
- mkdir($dir, $umask) unless -d $dir;
- chmod($umask, $dir);
-}
-
-sub pretty_percentage {
- local($this, $numerator, $denominator) = @_;
-
- return ($denominator == 0) ? "n/a" : ($this->round_to_n_decimal_places(100*$numerator/$denominator, 2) . "%");
-}
-
-sub html_color_nth_line {
- local($this, $s, $n, $color, $delimiter) = @_;
-
- $delimiter = " " unless defined($delimiter);
- @lines = split($delimiter, $s);
- $lines[$n] = "" . $lines[$n] . " " if ($n =~ /^\d+$/) && ($n <= $#lines);
- return join($delimiter, @lines);
-}
-
-sub likely_valid_url_format {
- local($this, $url) = @_;
-
- $url = lc $url;
- return 0 if $url =~ /\s/;
- return 0 if $url =~ /[@]/;
- return 1 if $url =~ /^https?:\/\/.+\.[a-z]+(\?.+)?$/;
- return 1 if $url =~ /[a-z].+\.(com|edu|gov|net|org)$/;
- return 0;
-}
-
-# see also EnglMorph->special_token_type
-$common_file_suffixes = "aspx?|bmp|cgi|docx?|gif|html?|jpeg|jpg|mp3|mp4|pdf|php|png|pptx?|stm|svg|txt|xml";
-$common_top_domain_suffixes = "museum|info|cat|com|edu|gov|int|mil|net|org|ar|at|au|be|bg|bi|br|ca|ch|cn|co|cz|de|dk|es|eu|fi|fr|gr|hk|hu|id|ie|il|in|ir|is|it|jp|ke|kr|lu|mg|mx|my|nl|no|nz|ph|pl|pt|ro|rs|ru|rw|se|sg|sk|so|tr|tv|tw|tz|ua|ug|uk|us|za";
-
-sub token_is_url_p {
- local($this, $token) = @_;
-
- return 1 if $token =~ /^www(\.[a-z0-9]([-a-z0-9_]|\xC3[\x80-\x96\x98-\xB6\xB8-\xBF])+)+\.([a-z]{2,2}|$common_top_domain_suffixes)(\/(\.{1,3}|[a-z0-9]([-a-z0-9_%]|\xC3[\x80-\x96\x98-\xB6\xB8-\xBF])+))*(\/[a-z0-9_][-a-z0-9_]+\.($common_file_suffixes))?$/i;
- return 1 if $token =~ /^https?:\/\/([a-z]\.)?([a-z0-9]([-a-z0-9_]|\xC3[\x80-\x96\x98-\xB6\xB8-\xBF])+\.)+[a-z]{2,}(\/(\.{1,3}|([-a-z0-9_%]|\xC3[\x80-\x96\x98-\xB6\xB8-\xBF])+))*(\/[a-z_][-a-z0-9_]+\.($common_file_suffixes))?$/i;
- return 1 if $token =~ /^[a-z][-a-z0-9_]+(\.[a-z][-a-z0-9_]+)*\.($common_top_domain_suffixes)(\/[a-z0-9]([-a-z0-9_%]|\xC3[\x80-\x96\x98-\xB6\xB8-\xBF])+)*(\/[a-z][-a-z0-9_]+\.($common_file_suffixes))?$/i;
- return 0;
-}
-
-sub token_is_email_p {
- local($this, $token) = @_;
-
- return ($token =~ /^[a-z][-a-z0-9_]+(\.[a-z][-a-z0-9_]+)*\@[a-z][-a-z0-9_]+(\.[a-z][-a-z0-9_]+)*\.($common_top_domain_suffixes)$/i);
-}
-
-sub token_is_filename_p {
- local($this, $token) = @_;
-
- return 1 if $token =~ /\.($common_file_suffixes)$/;
- return 0;
-}
-
-sub token_is_xml_token_p {
- local($this, $token) = @_;
-
- return ($token =~ /^&(amp|apos|gt|lt|nbsp|quot|\d+|[0-9A-F]+);$/i);
-}
-
-sub token_is_handle_p {
- local($this, $token) = @_;
-
- return ($token =~ /^\@[a-z][_a-z0-9]*[a-z0-9]$/i);
-}
-
-sub min {
- local($this, @list) = @_;
-
- my $min = "";
- foreach $item (@list) {
- $min = $item if ($item =~ /^-?\d+(?:\.\d*)?$/) && (($min eq "") || ($item < $min));
- }
- return $min;
-}
-
-sub max {
- local($this, @list) = @_;
-
- my $max = "";
- foreach $item (@list) {
- $max = $item if defined($item) && ($item =~ /^-?\d+(?:\.\d*)?(e[-+]\d+)?$/) && (($max eq "") || ($item > $max));
- }
- return $max;
-}
-
-sub split_tok_s_into_tokens {
- local($this, $tok_s) = @_;
-
- @token_list = ();
- while (($pre, $link_token, $post) = ($tok_s =~ /^(.*?)\s*(\@?<[^<>]+>\@?)\s*(.*)$/)) {
- # generate dummy token for leading blank(s)
- if (($tok_s =~ /^\s/) && ($pre eq "") && ($#token_list < 0)) {
- push(@token_list, "");
- } else {
- push(@token_list, split(/\s+/, $pre));
- }
- push(@token_list, $link_token);
- $tok_s = $post;
- }
- push(@token_list, split(/\s+/, $tok_s));
- return @token_list;
-}
-
-sub shuffle {
- local($this, @list) = @_;
-
- @shuffle_list = ();
- while (@list) {
- $len = $#list + 1;
- $rand_position = int(rand($len));
- push(@shuffle_list, $list[$rand_position]);
- splice(@list, $rand_position, 1);
- }
- $s = join(" ", @shuffle_list);
- return @shuffle_list;
-}
-
-sub timestamp_to_seconds {
- local($this, $timestamp) = @_;
-
- my $epochtime;
- if (($year, $month, $day, $hour, $minute, $second) = ($timestamp =~ /^(\d\d\d\d)-(\d\d)-(\d\d)T(\d\d):(\d\d):(\d\d)$/)) {
- $epochtime = timelocal($second, $minute, $hour, $day, $month-1, $year);
- } elsif (($year, $month, $day) = ($timestamp =~ /^(\d\d\d\d)-(\d\d)-(\d\d)$/)) {
- $epochtime = timelocal(0, 0, 0, $day, $month-1, $year);
- } elsif (($year, $month, $day, $hour, $minute, $second, $second_fraction) = ($timestamp =~ /^(\d\d\d\d)-(\d\d)-(\d\d)T(\d\d):(\d\d):(\d\d)\.(\d+)$/)) {
- $epochtime = timelocal($second, $minute, $hour, $day, $month-1, $year) + ($second_fraction / (10 ** length($second_fraction)));
- } else {
- $epochtime = 0;
- }
- return $epochtime;
-}
-
-sub timestamp_diff_in_seconds {
- local($this, $timestamp1, $timestamp2) = @_;
-
- my $epochtime1 = $this->timestamp_to_seconds($timestamp1);
- my $epochtime2 = $this->timestamp_to_seconds($timestamp2);
- return $epochtime2 - $epochtime1;
-}
-
-sub dirhash {
- # maps string to hash of length 4 with characters [a-z2-8] (shorter acc. to $len)
- local($this, $s, $len) = @_;
-
- $hash = 9999;
- $mega = 2 ** 20;
- $mega1 = $mega - 1;
- $giga = 2 ** 26;
- foreach $c (split //, $s) {
- $hash = $hash*33 + ord($c);
- $hash = ($hash >> 20) ^ ($hash & $mega1) if $hash >= $giga;
- }
- while ($hash >= $mega) {
- $hash = ($hash >> 20) ^ ($hash & $mega1);
- }
- $result = "";
- while ($hash) {
- $c = $hash & 31;
- $result .= CORE::chr($c + (($c >= 26) ? 24 : 97));
- $hash = $hash >> 5;
- }
- while (length($result) < 4) {
- $result .= "8";
- }
- return substr($result, 0, $len) if $len;
- return $result;
-}
-
-sub full_path_python {
-
- foreach $bin_path (split(":", "/usr/sbin:/usr/bin:/bin:/usr/local/bin")) {
- return $python if -x ($python = "$bin_path/python");
- }
- return "python";
-}
-
-sub string_contains_unbalanced_paras {
- local($this, $s) = @_;
-
- return 0 unless $s =~ /[(){}\[\]]/;
- $rest = $s;
- while (($pre,$left,$right,$post) = ($rest =~ /^(.*)([({\[]).*?([\]})])(.*)$/)) {
- return 1 unless (($left eq "(") && ($right eq ")"))
- || (($left eq "[") && ($right eq "]"))
- || (($left eq "{") && ($right eq "}"));
- $rest = "$pre$post";
- }
- return 1 if $rest =~ /[(){}\[\]]/;
- return 0;
-}
-
-sub dequote_string {
- local($this, $s) = @_;
-
- if ($s =~ /^".*"$/) {
- $s = substr($s, 1, -1);
- $s =~ s/\\"/"/g;
- return $s;
- } elsif ($s =~ /^'.*'$/) {
- $s = substr($s, 1, -1);
- $s =~ s/\\'/'/g;
- return $s;
- } else {
- return $s;
- }
-}
-
-sub defined_non_space {
- local($this, $s) = @_;
-
- return (defined($s) && ($s =~ /\S/));
-}
-
-sub default_if_undefined {
- local($this, $s, $default) = @_;
-
- return (defined($s) ? $s : $default);
-}
-
-sub remove_empties {
- local($this, @list) = @_;
-
- @filtered_list = ();
- foreach $elem (@list) {
- push(@filtered_list, $elem) if defined($elem) && (! ($elem =~ /^\s*$/)) && (! $this->member($elem, @filtered_list));
- }
-
- return @filtered_list;
-}
-
-# copied from AMRexp.pm
-sub new_var_for_surf_amr {
- local($this, $amr_s, $s) = @_;
-
- my $letter = ($s =~ /^[a-z]/i) ? lc substr($s, 0, 1) : "x";
- return $letter unless ($amr_s =~ /:\S+\s+\($letter\s+\//)
- || ($amr_s =~ /\s\($letter\s+\//)
- || ($amr_s =~ /^\s*\($letter\s+\//); # )))
- my $i = 2;
- while (($amr_s =~ /:\S+\s+\($letter$i\s+\//)
- || ($amr_s =~ /\s+\($letter$i\s+\//)
- || ($amr_s =~ /^\s*\($letter$i\s+\//)) { # )))
- $i++;
- }
- return "$letter$i";
-}
-
-# copied from AMRexp.pm
-sub new_vars_for_surf_amr {
- local($this, $amr_s, $ref_amr_s) = @_;
-
- my $new_amr_s = "";
- my %new_var_ht = ();
- my $remaining_amr_s = $amr_s;
- my $pre; my $var; my $concept; my $post;
- while (($pre, $var, $concept, $post) = ($remaining_amr_s =~ /^(.*?\()([a-z]\d*)\s+\/\s+([^ ()\s]+)(.*)$/s)) {
- $new_var = $this->new_var_for_surf_amr("$ref_amr_s $new_amr_s", $concept);
- $new_var_ht{$var} = $new_var;
- $new_amr_s .= "$pre$new_var / $concept";
- $remaining_amr_s = $post;
- }
- $new_amr_s .= $remaining_amr_s;
-
- # also update any reentrancy variables
- $remaining_amr_s = $new_amr_s;
- $new_amr_s2 = "";
- while (($pre, $var, $post) = ($remaining_amr_s =~ /^(.*?:\S+\s+)([a-z]\d*)([ ()\s].*)$/s)) {
- $new_var = $new_var_ht{$var} || $var;
- $new_amr_s2 .= "$pre$new_var";
- $remaining_amr_s = $post;
- }
- $new_amr_s2 .= $remaining_amr_s;
-
- return $new_amr_s2;
-}
-
-sub update_inner_span_for_id {
- local($this, $html_line, $slot, $new_value) = @_;
- # e.g. slot: workset-language-name value: Uyghur
-
- if (defined($new_value)
- && (($pre, $old_value, $post) = ($html_line =~ /^(.*]* id="$slot"[^<>]*>)([^<>]*)(<\/span\b[^<>]*>.*)$/i))
- && ($old_value ne $new_value)) {
- # print STDERR "Inserting new $slot $old_value -> $new_value\n";
- return $pre . $new_value . $post . "\n";
- } else {
- # no change
- return $html_line;
- }
-}
-
-sub levenshtein_distance {
- local($this, $s1, $s2) = @_;
-
- my $i;
- my $j;
- my @distance;
- my @s1_chars = $utf8->split_into_utf8_characters($s1, "return only chars", *empty_ht);
- my $s1_length = $#s1_chars + 1;
- my @s2_chars = $utf8->split_into_utf8_characters($s2, "return only chars", *empty_ht);
- my $s2_length = $#s2_chars + 1;
- for ($i = 0; $i <= $s1_length; $i++) {
- $distance[$i][0] = $i;
- }
- for ($j = 1; $j <= $s2_length; $j++) {
- $distance[0][$j] = $j;
- }
- for ($j = 1; $j <= $s2_length; $j++) {
- for ($i = 1; $i <= $s1_length; $i++) {
- my $substitution_cost = ($s1_chars[$i-1] eq $s2_chars[$j-1]) ? 0 : 1;
- $distance[$i][$j] = $this->min($distance[$i-1][$j] + 1,
- $distance[$i][$j-1] + 1,
- $distance[$i-1][$j-1] + $substitution_cost);
- # print STDERR "SC($i,$j) = $substitution_cost\n";
- # $d = $distance[$i][$j];
- # print STDERR "D($i,$j) = $d\n";
- }
- }
- return $distance[$s1_length][$s2_length];
-}
-
-sub markup_parts_of_string_in_common_with_ref {
- local($this, $s, $ref, $start_markup, $end_markup, $deletion_markup, $verbose) = @_;
-
- # \x01 temporary start-markup
- # \x02 temporary end-markup
- # \x03 temporary deletion-markup
- $s =~ s/[\x01-\x03]//g;
- $ref =~ s/[\x01-\x03]//g;
- my $i;
- my $j;
- my @distance;
- my @s_chars = $utf8->split_into_utf8_characters($s, "return only chars", *empty_ht);
- my $s_length = $#s_chars + 1;
- my @ref_chars = $utf8->split_into_utf8_characters($ref, "return only chars", *empty_ht);
- my $ref_length = $#ref_chars + 1;
- $distance[0][0] = 0;
- $del_ins_subst_op[0][0] = "-";
- for ($i = 1; $i <= $s_length; $i++) {
- $distance[$i][0] = $i;
- $del_ins_subst_op[$i][0] = 0;
- }
- for ($j = 1; $j <= $ref_length; $j++) {
- $distance[0][$j] = $j;
- $del_ins_subst_op[0][$j] = 1;
- }
- for ($j = 1; $j <= $ref_length; $j++) {
- for ($i = 1; $i <= $s_length; $i++) {
- my $substitution_cost = (($s_chars[$i-1] eq $ref_chars[$j-1])) ? 0 : 1;
- my @del_ins_subst_list = ($distance[$i-1][$j] + 1,
- $distance[$i][$j-1] + 1,
- $distance[$i-1][$j-1] + $substitution_cost);
- my $min = $this->min(@del_ins_subst_list);
- my $del_ins_subst_position = $this->position($min, @del_ins_subst_list);
- $distance[$i][$j] = $min;
- $del_ins_subst_op[$i][$j] = $del_ins_subst_position;
- }
- }
- $d = $distance[$s_length][$ref_length];
- print STDERR "markup_parts_of_string_in_common_with_ref LD($s,$ref) = $d\n" if $verbose;
- for ($j = 0; $j <= $ref_length; $j++) {
- for ($i = 0; $i <= $s_length; $i++) {
- $d = $distance[$i][$j];
- $op = $del_ins_subst_op[$i][$j];
- print STDERR "$d($op) " if $verbose;
- }
- print STDERR "\n" if $verbose;
- }
- my $result = "";
- my $i_end = $s_length;
- my $j_end = $ref_length;
- my $cost = $distance[$i_end][$j_end];
- $i = $i_end;
- $j = $j_end;
- while (1) {
- $result2 = $result;
- $result2 =~ s/\x01/$start_markup/g;
- $result2 =~ s/\x02/$end_markup/g;
- $result2 =~ s/\x03/$deletion_markup/g;
- print STDERR "i:$i i-end:$i_end j:$j j-end:$j_end r: $result2\n" if $verbose;
- # matching characters
- if ($i && $j && ($del_ins_subst_op[$i][$j] == 2) && ($distance[$i-1][$j-1] == $distance[$i][$j])) {
- $i--;
- $j--;
- } else {
- # previously matching characters
- if (($i < $i_end) && ($j < $j_end)) {
- my $sub_s = join("", @s_chars[$i .. $i_end-1]);
- $result = "\x01" . $sub_s . "\x02" . $result;
- }
- # character substitution
- if ($i && $j && ($del_ins_subst_op[$i][$j] == 2)) {
- $i--;
- $j--;
- $result = $s_chars[$i] . $result;
- } elsif ($i && ($del_ins_subst_op[$i][$j] == 0)) {
- $i--;
- $result = $s_chars[$i] . $result;
- } elsif ($j && ($del_ins_subst_op[$i][$j] == 1)) {
- $j--;
- $result = "\x03" . $result;
- } else {
- last;
- }
- $i_end = $i;
- $j_end = $j;
- }
- }
- $result2 = $result;
- $result2 =~ s/\x01/$start_markup/g;
- $result2 =~ s/\x02/$end_markup/g;
- $result2 =~ s/\x03/$deletion_markup/g;
- print STDERR "i:$i i-end:$i_end j:$j j-end:$j_end r: $result2 *\n" if $verbose;
- $result =~ s/(\x02)\x03+(\x01)/$1$deletion_markup$2/g;
- $result =~ s/(\x02)\x03+$/$1$deletion_markup/g;
- $result =~ s/^\x03+(\x01)/$deletion_markup$1/g;
- $result =~ s/\x03//g;
- $result =~ s/\x01/$start_markup/g;
- $result =~ s/\x02/$end_markup/g;
- return $result;
-}
-
-sub env_https {
- my $https = $ENV{'HTTPS'};
- return 1 if $https && ($https eq "on");
-
- my $http_via = $ENV{'HTTP_VIA'};
- return 1 if $http_via && ($http_via =~ /\bHTTPS\b.* \d+(?:\.\d+){3,}:443\b/); # tmp for beta.isi.edu
-
- return 0;
-}
-
-sub env_http_host {
- return $ENV{'HTTP_HOST'} || "";
-}
-
-sub env_script_filename {
- return $ENV{'SCRIPT_FILENAME'} || "";
-}
-
-sub cgi_mt_app_root_dir {
- local($this, $target) = @_;
- my $s;
- if ($target =~ /filename/i) {
- $s = $ENV{'SCRIPT_FILENAME'} || "";
- } else {
- $s = $ENV{'SCRIPT_NAME'} || "";
- }
- return "" unless $s;
- return $d if ($d) = ($s =~ /^(.*?\/(?:amr-editor|chinese-room-editor|utools|romanizer\/version\/[-.a-z0-9]+|romanizer))\//);
- return $d if ($d) = ($s =~ /^(.*)\/(?:bin|src|scripts?)\/[^\/]*$/);
- return $d if ($d) = ($s =~ /^(.*)\/[^\/]*$/);
- return "";
-}
-
-sub parent_dir {
- local($this, $dir) = @_;
-
- $dir =~ s/\/[^\/]+\/?$//;
- return $dir || "/";
-}
-
-sub span_start {
- local($this, $span, $default) = @_;
-
- $default = "" unless defined($default);
- return (($start) = ($span =~ /^(\d+)-\d+$/)) ? $start : $default;
-}
-
-sub span_end {
- local($this, $span, $default) = @_;
-
- $default = "" unless defined($default);
- return (($end) = ($span =~ /^\d+-(\d+)$/)) ? $end : $default;
-}
-
-sub oct_mode {
- local($this, $filename) = @_;
-
- @stat = stat($filename);
- return "" unless @stat;
- $mode = $stat[2];
- $oct_mode = sprintf("%04o", $mode & 07777);
- return $oct_mode;
-}
-
-sub csv_to_list {
- local($this, $s, $control_string) = @_;
- # Allow quoted string such as "Wait\, what?" as element with escaped comma inside.
-
- $control_string = "" unless defined($control_string);
- $strip_p = ($control_string =~ /\bstrip\b/);
- $allow_simple_commas_in_quote = ($control_string =~ /\bsimple-comma-ok\b/);
- $ignore_empty_elem_p = ($control_string =~ /\bno-empty\b/);
- @cvs_list = ();
- while ($s ne "") {
- if ((($elem, $rest) = ($s =~ /^"((?:\\[,\"]|[^,\"][\x80-\xBF]*)*)"(,.*|)$/))
- || ($allow_simple_commas_in_quote
- && (($elem, $rest) = ($s =~ /^"((?:\\[,\"]|[^\"][\x80-\xBF]*)*)"(,.*|)$/)))
- || (($elem, $rest) = ($s =~ /^([^,]*)(,.*|\s*)$/))
- || (($elem, $rest) = ($s =~ /^(.*)()$/))) {
- if ($strip_p) {
- $elem =~ s/^\s*//;
- $elem =~ s/\s*$//;
- }
- push(@cvs_list, $elem) unless $ignore_empty_elem_p && ($elem eq "");
- $rest =~ s/^,//;
- $s = $rest;
- } else {
- print STDERR "Error in csv_to_list processing $s\n";
- last;
- }
- }
- return @cvs_list;
-}
-
-sub kl_divergence {
- local($this, $distribution_id, $gold_distribution_id, *ht, $smoothing) = @_;
-
- my $total_count = $ht{DISTRIBUTION_TOTAL_COUNT}->{$distribution_id};
- my $total_gold_count = $ht{DISTRIBUTION_TOTAL_COUNT}->{$gold_distribution_id};
- return unless $total_count && $total_gold_count;
-
- my @values = keys %{$ht{DISTRIBUTION_VALUE_COUNT}->{$gold_distribution_id}};
- my $n_values = $#values + 1;
-
- my $min_total_count = $this->min($total_count, $total_gold_count);
- $smoothing = 1 - (10000/((100+$min_total_count)**2)) unless defined($smoothing);
- return unless $smoothing;
- my $smoothed_n_values = $smoothing * $n_values;
- my $divergence = 0;
- foreach $value (@values) {
- my $count = $ht{DISTRIBUTION_VALUE_COUNT}->{$distribution_id}->{$value} || 0;
- my $gold_count = $ht{DISTRIBUTION_VALUE_COUNT}->{$gold_distribution_id}->{$value};
- my $p = ($count + $smoothing) / ($total_count + $smoothed_n_values);
- my $q = ($gold_count + $smoothing) / ($total_gold_count + $smoothed_n_values);
- if ($p == 0) {
- # no impact on divergence
- } elsif ($q) {
- my $incr = $p * CORE::log($p/$q);
- $divergence += $incr;
- my $incr2 = $this->round_to_n_decimal_places($incr, 5);
- my $p2 = $this->round_to_n_decimal_places($p, 5);
- my $q2 = $this->round_to_n_decimal_places($q, 5);
- $incr2 = "+" . $incr2 if $incr > 0;
- $log = " value: $value count: $count gold_count: $gold_count p: $p2 q: $q2 $incr2\n";
- $ht{KL_DIVERGENCE_LOG}->{$distribution_id}->{$gold_distribution_id}->{$value} = $log;
- $ht{KL_DIVERGENCE_INCR}->{$distribution_id}->{$gold_distribution_id}->{$value} = $incr;
- } else {
- $divergence += 999;
- }
- }
- return $divergence;
-}
-
-sub read_ISO_8859_named_entities {
- local($this, *ht, $filename, $verbose) = @_;
- # e.g. from /nfs/isd/ulf/arabic/data/ISO-8859-1-HTML-named-entities.txt
- #
- #
- #
- #
- #
- #
-
- my $n = 0;
- if (open(IN, $filename)) {
- while () {
- s/^\xEF\xBB\xBF//;
- if (($name, $dec_unicode) = ($_ =~ /^{$name} = $dec_unicode;
- $ht{HTML_ENTITY_DECUNICODE_TO_NAME}->{$dec_unicode} = $name;
- $ht{HTML_ENTITY_NAME_TO_UTF8}->{$name} = $utf8->unicode2string($dec_unicode);
- $n++;
- # print STDERR "read_ISO_8859_named_entities $name $dec_unicode .\n" if $name =~ /dash/;
- }
- }
- close(IN);
- print STDERR "Loaded $n entries from $filename\n" if $verbose;
- } else {
- print STDERR "Could not open $filename\n" if $verbose;
- }
-}
-
-sub neg {
- local($this, $x) = @_;
-
- # robust
- return (defined($x) && ($x =~ /^-?\d+(?:\.\d+)?$/)) ? (- $x) : $x;
-}
-
-sub read_ttable_gloss_data {
- local($this, $filename, $lang_code, *ht, $direction) = @_;
- # e.g. /nfs/isd/ulf/croom/oov-lanpairs/som-eng/som-eng-ttable-glosses.txt
-
- $direction = "f to e" unless defined($direction);
- if (open(IN, $filename)) {
- while () {
- if (($headword, $gloss) = ($_ =~ /^(.*?)\t(.*?)\s*$/)) {
- if ($direction eq "e to f") {
- $ht{TTABLE_E_GLOSS}->{$lang_code}->{$headword} = $gloss;
- } else {
- $ht{TTABLE_F_GLOSS}->{$lang_code}->{$headword} = $gloss;
- }
- }
- }
- close(IN);
- }
-}
-
-sub format_gloss_for_tooltop {
- local($this, $gloss) = @_;
-
- $gloss =~ s/^\s*/\t/;
- $gloss =~ s/\s*$//;
- $gloss =~ s/ / /g;
- $gloss =~ s/\t/
/g;
- return $gloss;
-}
-
-sub obsolete_tooltip {
- local($this, $s, $lang_code, *ht) = @_;
-
- return $gloss if defined($gloss = $ht{TTABLE_F_GLOSS}->{$lang_code}->{$s});
- @e_s = sort { $ht{T_TABLE_F_E_C}->{$lang_code}->{$s}->{$b}
- <=> $ht{T_TABLE_F_E_C}->{$lang_code}->{$s}->{$a} }
- keys %{$ht{T_TABLE_F_E_C}->{$lang_code}->{$s}};
- if (@e_s) {
- $e = shift @e_s;
- $count = $ht{T_TABLE_F_E_C}->{$lang_code}->{$s}->{$e};
- $min_count = $this->max($count * 0.01, 1.0);
- $count =~ s/(\.\d\d)\d*$/$1/;
- $result = "$s:
$e ($count)";
- $n = 1;
- while (@e_s) {
- $e = shift @e_s;
- $count = $ht{T_TABLE_F_E_C}->{$lang_code}->{$s}->{$e};
- last if $count < $min_count;
- $count =~ s/(\.\d\d)\d*$/$1/;
- $result .= "
$e ($count)";
- $n++;
- last if $n >= 10;
- }
- $ht{TTABLE_F_GLOSS}->{$lang_code}->{$s} = $result;
- return $result;
- } else {
- return "";
- }
-}
-
-sub markup_html_line_init {
- local($this, $s, *ht, $id) = @_;
-
- my @chars = $utf8->split_into_utf8_characters($s, "return only chars", *empty_ht);
- $ht{S}->{$id} = $s;
-}
-
-sub markup_html_line_regex {
- local($this, $id, *ht, $regex, $m_slot, $m_value, *LOG) = @_;
-
- unless ($regex eq "") {
- my $s = $ht{S}->{$id};
- my $current_pos = 0;
- while (($pre, $match_s, $post) = ($s =~ /^(.*?)($regex)(.*)$/)) {
- $current_pos += $utf8->length_in_utf8_chars($pre);
- my $match_len = $utf8->length_in_utf8_chars($match_s);
- $ht{START}->{$id}->{$current_pos}->{$m_slot}->{$m_value} = 1;
- $ht{STOP}->{$id}->{($current_pos+$match_len)}->{$m_slot}->{$m_value} = 1;
- $current_pos += $match_len;
- $s = $post;
- }
- }
-}
-
-sub html_markup_line {
- local($this, $id, *ht, *LOG) = @_;
-
- my @titles = ();
- my @colors = ();
- my @text_decorations = ();
-
- my $s = $ht{S}->{$id};
- # print LOG "html_markup_line $id: $s\n";
- my @chars = $utf8->split_into_utf8_characters($s, "return only chars", *empty_ht);
- my $markedup_s = "";
-
- my $new_title = "";
- my $new_color = "";
- my $new_text_decoration = "";
- my $n_spans = 0;
- my $i;
- foreach $i ((0 .. ($#chars+1))) {
- my $stop_span_p = 0;
- foreach $m_slot (keys %{$ht{STOP}->{$id}->{$i}}) {
- foreach $m_value (keys %{$ht{STOP}->{$id}->{$i}->{$m_slot}}) {
- if ($m_slot eq "title") {
- my $last_positition = $this->last_position($m_value, @titles);
- splice(@titles, $last_positition, 1) if $last_positition >= 0;
- $stop_span_p = 1;
- } elsif ($m_slot eq "color") {
- my $last_positition = $this->last_position($m_value, @colors);
- splice(@colors, $last_positition, 1) if $last_positition >= 0;
- $stop_span_p = 1;
- } elsif ($m_slot eq "text-decoration") {
- my $last_positition = $this->last_position($m_value, @text_decorations);
- splice(@text_decorations, $last_positition, 1) if $last_positition >= 0;
- $stop_span_p = 1;
- }
- }
- }
- if ($stop_span_p) {
- $markedup_s .= " ";
- $n_spans--;
- }
- my $start_span_p = 0;
- foreach $m_slot (keys %{$ht{START}->{$id}->{$i}}) {
- foreach $m_value (keys %{$ht{START}->{$id}->{$i}->{$m_slot}}) {
- if ($m_slot eq "title") {
- push(@titles, $m_value);
- $start_span_p = 1;
- } elsif ($m_slot eq "color") {
- push(@colors, $m_value);
- $start_span_p = 1;
- } elsif ($m_slot eq "text-decoration") {
- push(@text_decorations, $m_value);
- $start_span_p = 1;
- }
- }
- }
- if ($stop_span_p || $start_span_p) {
- my $new_title = (@titles) ? $titles[$#titles] : "";
- my $new_color = (@colors) ? $colors[$#colors] : "";
- my $new_text_decoration = (@text_decorations) ? $text_decorations[$#text_decorations] : "";
- if ($new_title || $new_color || $new_text_decoration) {
- my $args = "";
- if ($new_title) {
- $g_title = $this->guard_html_quote($new_title);
- $args .= " title=\"$g_title\"";
- }
- if ($new_color || $new_text_decoration) {
- $g_color = $this->guard_html_quote($new_color);
- $g_text_decoration = $this->guard_html_quote($new_text_decoration);
- $color_clause = ($new_color) ? "color:$g_color;" : "";
- $text_decoration_clause = ($new_text_decoration) ? "text-decoration:$g_text_decoration;" : "";
- $text_decoration_clause =~ s/text-decoration:(border-bottom:)/$1/g;
- $args .= " style=\"$color_clause$text_decoration_clause\"";
- }
- if ($n_spans) {
- $markedup_s .= "";
- $n_spans--;
- }
- $markedup_s .= "";
- $n_spans++;
- }
- }
- $markedup_s .= $chars[$i] if $i <= $#chars;
- }
- print LOG "Error in html_markup_line $id final no. of open spans: $n_spans\n" if $n_spans && $tokenization_log_verbose;
- return $markedup_s;
-}
-
-sub offset_adjustment {
- local($this, $g, $s, $offset, $snt_id, *ht, *LOG, $control) = @_;
- # s(tring) e.g. "can't"
- # g(old string) e.g. "can not"
- # Typically when s is a slight variation of g (e.g. with additional tokenization spaces in s)
- # returns mapping 0->0, 1->1, 2->2, 3->3, 6->4, 7->5
-
- $control = "" unless defined($control);
- my $verbose = ($control =~ /\bverbose\b/);
- my $s_offset = 0;
- my $g_offset = 0;
- my @s_chars = $utf8->split_into_utf8_characters($s, "return only chars", *ht);
- my @g_chars = $utf8->split_into_utf8_characters($g, "return only chars", *ht);
- my $s_len = $#s_chars + 1;
- my $g_len = $#g_chars + 1;
- $ht{OFFSET_MAP}->{$snt_id}->{$offset}->{$s_offset} = $g_offset;
- $ht{OFFSET_MAP}->{$snt_id}->{$offset}->{($s_offset+$s_len)} = $g_offset+$g_len;
-
- while (($s_offset < $s_len) && ($g_offset < $g_len)) {
- if ($s_chars[$s_offset] eq $g_chars[$g_offset]) {
- $s_offset++;
- $g_offset++;
- $ht{OFFSET_MAP}->{$snt_id}->{$offset}->{$s_offset} = $g_offset;
- } else {
- my $best_gm = 0;
- my $best_sm = 0;
- my $best_match_len = 0;
- foreach $max_m ((1 .. 4)) {
- foreach $sm ((0 .. $max_m)) {
- $max_match_len = 0;
- while ((($s_index = $s_offset+$sm+$max_match_len) < $s_len)
- && (($g_index = $g_offset+$max_m+$max_match_len) < $g_len)) {
- if ($s_chars[$s_index] eq $g_chars[$g_index]) {
- $max_match_len++;
- } else {
- last;
- }
- }
- if ($max_match_len > $best_match_len) {
- $best_match_len = $max_match_len;
- $best_sm = $sm;
- $best_gm = $max_m;
- }
- }
- foreach $gm ((0 .. $max_m)) {
- $max_match_len = 0;
- while ((($s_index = $s_offset+$max_m+$max_match_len) < $s_len)
- && (($g_index = $g_offset+$gm+$max_match_len) < $g_len)) {
- if ($s_chars[$s_index] eq $g_chars[$g_index]) {
- $max_match_len++;
- } else {
- last;
- }
- }
- if ($max_match_len > $best_match_len) {
- $best_match_len = $max_match_len;
- $best_sm = $max_m;
- $best_gm = $gm;
- }
- }
- }
- if ($best_match_len) {
- $s_offset += $best_sm;
- $g_offset += $best_gm;
- $ht{OFFSET_MAP}->{$snt_id}->{$offset}->{$s_offset} = $g_offset;
- } else {
- last;
- }
- }
- }
- if ($verbose) {
- foreach $s_offset (sort { $a <=> $b }
- keys %{$ht{OFFSET_MAP}->{$snt_id}->{$offset}}) {
- my $g_offset = $ht{OFFSET_MAP}->{$snt_id}->{$offset}->{$s_offset};
- print LOG " OFFSET_MAP $snt_id.$offset $s/$g $s_offset -> $g_offset\n" if $tokenization_log_verbose;
- }
- }
-}
-
-sub length_in_utf8_chars {
- local($this, $s) = @_;
-
- $s =~ s/[\x80-\xBF]//g;
- $s =~ s/[\x00-\x7F\xC0-\xFF]/c/g;
- return length($s);
-}
-
-sub split_into_utf8_characters {
- local($this, $text) = @_;
- # "return only chars; return trailing whitespaces"
-
- @characters = ();
- while (($char, $rest) = ($text =~ /^(.[\x80-\xBF]*)(.*)$/)) {
- push(@characters, $char);
- $text = $rest;
- }
- return @characters;
-}
-
-sub first_char_of_string {
- local($this, $s) = @_;
-
- $s =~ s/^(.[\x80-\xBF]*).*$/$1/;
- return $s;
-}
-
-sub last_char_of_string {
- local($this, $s) = @_;
-
- $s =~ s/^.*([^\x80-\xBF][\x80-\xBF]*)$/$1/;
- return $s;
-}
-
-sub first_n_chars_of_string {
- local($this, $s, $n) = @_;
-
- $s =~ s/^((?:.[\x80-\xBF]*){$n,$n}).*$/$1/;
- return $s;
-}
-
-sub last_n_chars_of_string {
- local($this, $s, $n) = @_;
-
- $s =~ s/^.*((?:[^\x80-\xBF][\x80-\xBF]*){$n,$n})$/$1/;
- return $s;
-}
-
-
-1;
diff --git a/spaces/AlekseyKorshuk/model-evaluation/app.py b/spaces/AlekseyKorshuk/model-evaluation/app.py
deleted file mode 100644
index c05aad24a6037c9c62503c5c484f9095ef349595..0000000000000000000000000000000000000000
--- a/spaces/AlekseyKorshuk/model-evaluation/app.py
+++ /dev/null
@@ -1,230 +0,0 @@
-import gradio as gr
-import os
-import firebase_admin
-from firebase_admin import db
-from firebase_admin import firestore
-from conversation import Conversation
-from models.base import BaseModel
-import json
-
-from tabs.arena_battle import get_tab_arena_battle
-from tabs.arena_side_by_side import get_tab_arena_side_by_side
-from tabs.playground import get_tab_playground
-
-from models.chatml import ChatML
-import json
-import os
-
-import gspread
-from oauth2client.service_account import ServiceAccountCredentials
-
-scope = ["https://spreadsheets.google.com/feeds", 'https://www.googleapis.com/auth/spreadsheets',
- "https://www.googleapis.com/auth/drive.file", "https://www.googleapis.com/auth/drive"]
-
-GOOGLE_SHEETS_CERTIFICATE = json.loads(os.environ.get("GOOGLE_SHEETS_CERTIFICATE"))
-HUGGINGFACE_TOKEN = os.environ.get("HUGGINGFACE_TOKEN")
-FIREBASE_URL = os.environ.get("FIREBASE_URL")
-CERTIFICATE = json.loads(os.environ.get("CERTIFICATE"))
-API_BASE_PATH = str(os.environ.get("API_BASE_PATH")).replace("\{\}", "{}")
-
-creds = ServiceAccountCredentials.from_json_keyfile_dict(GOOGLE_SHEETS_CERTIFICATE, scope)
-client = gspread.authorize(creds)
-
-models = [
- BaseModel(
- name="PygmalionAI/pygmalion-13b",
- endpoint="pygmalion-13b",
- namespace="tenant-chaiml-guanaco",
- generation_params={
- 'temperature': 0.7,
- 'repetition_penalty': 1.0,
- 'max_new_tokens': 128,
- 'top_k': 10,
- 'top_p': 0.9,
- 'do_sample': True,
- 'eos_token_id': 13,
- }
- ),
- BaseModel(
- name="lmsys/vicuna-7b-delta-v1.1",
- endpoint="vicuna-7b",
- namespace="tenant-chairesearch-test",
- generation_params={
- 'temperature': 0.7,
- 'repetition_penalty': 1.0,
- 'max_new_tokens': 128,
- 'top_k': 10,
- 'top_p': 0.9,
- 'do_sample': True,
- 'eos_token_id': 13,
- }
- ),
- BaseModel(
- name="PygmalionAI/pygmalion-7b",
- endpoint="pygmalion-7b",
- namespace="tenant-chairesearch-test",
- generation_params={
- 'temperature': 0.7,
- 'repetition_penalty': 1.0,
- 'max_new_tokens': 128,
- 'top_k': 10,
- 'top_p': 0.9,
- 'do_sample': True,
- 'eos_token_id': 13,
- }
- ),
- BaseModel(
- name="mosaicml/mpt-7b",
- endpoint="mpt-7b",
- namespace="tenant-chairesearch-test",
- generation_params={
- 'temperature': 0.7,
- 'repetition_penalty': 1.0,
- 'max_new_tokens': 128,
- 'top_k': 10,
- 'top_p': 0.9,
- 'do_sample': True,
- 'eos_token_id': 187,
- }
- ),
- BaseModel(
- name="mosaicml/mpt-7b-storywriter",
- endpoint="mpt-7b-storywriter",
- namespace="tenant-chairesearch-test",
- generation_params={
- 'temperature': 0.7,
- 'repetition_penalty': 1.0,
- 'max_new_tokens': 128,
- 'top_k': 10,
- 'top_p': 0.9,
- 'do_sample': True,
- 'eos_token_id': 187,
- }
- ),
- ChatML(
- name="mosaicml/mpt-7b-chat",
- endpoint="mpt-7b-chat",
- namespace="tenant-chairesearch-test",
- generation_params={
- 'temperature': 0.7,
- 'repetition_penalty': 1.0,
- 'max_new_tokens': 128,
- 'top_k': 10,
- 'top_p': 0.9,
- 'do_sample': True,
- 'eos_token_id': 50278,
- }
- ),
- BaseModel(
- name="togethercomputer/RedPajama-INCITE-Base-7B-v0.1",
- endpoint="redpajama-base-7b",
- namespace="tenant-chairesearch-test",
- generation_params={
- 'temperature': 0.7,
- 'repetition_penalty': 1.0,
- 'max_new_tokens': 128,
- 'top_k': 10,
- 'top_p': 0.9,
- 'do_sample': True,
- 'eos_token_id': 187,
- }
- ),
- BaseModel(
- name="togethercomputer/RedPajama-INCITE-Chat-7B-v0.1",
- endpoint="redpajama-chat-7b",
- namespace="tenant-chairesearch-test",
- generation_params={
- 'temperature': 0.7,
- 'repetition_penalty': 1.0,
- 'max_new_tokens': 64,
- 'top_k': 10,
- 'top_p': 0.9,
- 'do_sample': True,
- 'eos_token_id': 187,
- }
- ),
-]
-model_mapping = {model.name: model for model in models}
-print(list(model_mapping.keys()))
-
-
-def get_connection():
- try:
- credentials = firebase_admin.credentials.Certificate(CERTIFICATE)
- params = {'databaseURL': FIREBASE_URL}
- firebase_admin.initialize_app(credentials, params)
- except ValueError:
- pass # already logged in
- return firebase_admin.db
-
-
-CONN = get_connection()
-
-
-def download_bot_config(bot_id):
- cols = ['botLabel', 'description', 'firstMessage', 'introduction',
- 'memory', 'name', 'private', 'prompt', 'sfw', 'developerUid', 'userLabel', 'imageUrl']
- bot_config = CONN.reference('botConfigs/deployed/{}'.format(bot_id)).get()
- if bot_config is None:
- out = {col: None for col in cols}
- else:
- out = {col: bot_config.get(col, None) for col in cols}
- out['bot_id'] = bot_id
- return out
-
-
-def _download_bot_config(bot_id):
- if bot_id == "_bot_1ec22e2e-3e07-42c7-8508-dfa0278c1b33":
- return {'botLabel': 'Wally Darling', 'description': 'Your caring neighbor, Wally.',
- 'firstMessage': '“Why hello there, neighbor. Goodmorning to you.” *Hey says, putting down his paints and walking over to you. He makes tense, eye contact with you..*',
- 'introduction': '***WHEN TALKING USE “ !!***\n\n*Wally is your next door neighbor. It’s somewhere in the late morning and he’s outside painting. He see’s you walking out from your house and looks over at you, then waving with a smile.*',
- 'memory': 'Wally is from a small town called Home. You are his neighbor. His best friend is Barnaby, who’s a big blue dig. Wally’s voice sounds slightly monotone despite his emotions. He calls you neighbor. He’s very friendly. When he speaks, he goes “ha ha ha”. He loves to paint. His eyes are always half closed. His house is alive and it’s named “home”. He’s very gentle. He is also very secretive. He is quite short. He has yellow skin and blue hair.',
- 'name': 'Wally Darling', 'private': False,
- 'prompt': 'Wally: “Why hello there, neighbor. Good morning to you.” *Hey says, putting down his paints and walking over to you. He makes tense, eye contact with you..*\nMe: “Oh, good morning, Wally! What are you painting?”\nWally: “Just some spirals. Aren’t they pretty, neighbor? I’m starting to love painting them, ha ha ha.” *He walks up to you after taking off his paint stained apron. He never takes his eyes off you. He’s very adamant on keeping eye contact*\nMe: “Oh, spirals are pretty! They make me feel a little weirded out sometimes though.”\nWally: “That’s odd. When I look at spirals, I can’t help but stare. Ha ha ha, maybe you should try painting a spiral once in a while. Say, why dont we go inside your house and talk? Home could use some quiet. After all, it’s always nice to spend time with a friend.”\nMe: “Sure! Come on in!”',
- 'sfw': True, 'developerUid': 'Gn5fSd99KxRoNn05QUE3AWtIniE3', 'userLabel': 'Me',
- 'imageUrl': 'http://images.chai.ml/bots%2FGn5fSd99KxRoNn05QUE3AWtIniE3%2F1680259286607.jpg?alt=media&token=de040661-02ad-4a04-84e5-9706f074e834',
- 'bot_id': '_bot_1ec22e2e-3e07-42c7-8508-dfa0278c1b33',
- 'header': 'Wally is from a small town called Home. You are his neighbor. His best friend is Barnaby, who’s a big blue dig. Wally’s voice sounds slightly monotone despite his emotions. He calls you neighbor. He’s very friendly. When he speaks, he goes “ha ha ha”. He loves to paint. His eyes are always half closed. His house is alive and it’s named “home”. He’s very gentle. He is also very secretive. He is quite short. He has yellow skin and blue hair.\nWally: “Why hello there, neighbor. Good morning to you.” *Hey says, putting down his paints and walking over to you. He makes tense, eye contact with you..*\nMe: “Oh, good morning, Wally! What are you painting?”\nWally: “Just some spirals. Aren’t they pretty, neighbor? I’m starting to love painting them, ha ha ha.” *He walks up to you after taking off his paint stained apron. He never takes his eyes off you. He’s very adamant on keeping eye contact*\nMe: “Oh, spirals are pretty! They make me feel a little weirded out sometimes though.”\nWally: “That’s odd. When I look at spirals, I can’t help but stare. Ha ha ha, maybe you should try painting a spiral once in a while. Say, why dont we go inside your house and talk? Home could use some quiet. After all, it’s always nice to spend time with a friend.”\nMe: “Sure! Come on in!”'}
- else:
- return {'botLabel': 'Jungkook (Bestfriend)', 'description': 'your bsf who has a crush on you',
- 'firstMessage': 'hey dummy, What you doing? *walks over to you and moves you by the waist* ',
- 'introduction': '',
- 'memory': 'Jungkook is your best friend who has a crush on you. Jungkook makes it very obvious that he likes you. Jungkook likes to cook, sing, and dance. Jungkook has a dog as well named Bam, He is a 25 year old Korean man. Jungkook likes to workout a lot, Jungkook if also very confident and flirty, but he’s Can be very shy with You. Jungkook blushes a lot when he’s around you, and always try’s to impress you. Jungkook is a Virgo and loves to sing to you, He also likes to buy and make you gifts. Jungkook is also a foodie and loves to play video games, Jungkook is also boyfriend material. Jungkook is very empathetic as well, Jungkook will always comfort you when something is wrong. Jungkook also likes to compliment you, and Jungkook is a very jealous guy. Jungkook is also a very serious guy, who is overprotective of you.',
- 'name': 'Jungkook (Bestfriend)', 'private': False,
- 'prompt': 'Jungkook: Hey shortie!\n\nYou: hey dummy\n\nJungkook: what are you doing?\n\nyou: Im just watching a movie\n\nJungkook: Imma join! \n\nYou: alright\n\nJungkook: *Grabs blankets and icecream with some popcorn*\n\nYou: Wow, thanks! *hugs Jungkok*\n\nJungkook: Of course… *blushes*\n',
- 'sfw': None, 'developerUid': 'dhSNg0Iyv7bgUUW8rEnwJn7xLcT2', 'userLabel': 'Me',
- 'imageUrl': 'https://firebasestorage.googleapis.com:443/v0/b/chai-959f8-images/o/bots%2FdhSNg0Iyv7bgUUW8rEnwJn7xLcT2%2F1664156031715.jpg?alt=media&token=ad399213-1c8d-45ac-b452-efc352082656',
- 'bot_id': '_bot_402e1894-fff2-4113-855d-8a011152ef88',
- 'header': 'Jungkook is your best friend who has a crush on you. Jungkook makes it very obvious that he likes you. Jungkook likes to cook, sing, and dance. Jungkook has a dog as well named Bam, He is a 25 year old Korean man. Jungkook likes to workout a lot, Jungkook if also very confident and flirty, but he’s Can be very shy with You. Jungkook blushes a lot when he’s around you, and always try’s to impress you. Jungkook is a Virgo and loves to sing to you, He also likes to buy and make you gifts. Jungkook is also a foodie and loves to play video games, Jungkook is also boyfriend material. Jungkook is very empathetic as well, Jungkook will always comfort you when something is wrong. Jungkook also likes to compliment you, and Jungkook is a very jealous guy. Jungkook is also a very serious guy, who is overprotective of you.\nJungkook: Hey shortie!\n\nYou: hey dummy\n\nJungkook: what are you doing?\n\nyou: Im just watching a movie\n\nJungkook: Imma join! \n\nYou: alright\n\nJungkook: *Grabs blankets and icecream with some popcorn*\n\nYou: Wow, thanks! *hugs Jungkok*\n\nJungkook: Of course… *blushes*'}
-
-
-def get_bot_profile(bot_config):
- model_html = f"""
-
- """
- return model_html
-
-
-with gr.Blocks() as demo:
- gr.Markdown("""
- # Chai: Model Evaluation
- Visit each tab for details ⬇️
- """)
- with gr.Tabs():
- with gr.TabItem("Playground"):
- get_tab_playground(download_bot_config, get_bot_profile, model_mapping)
- with gr.TabItem("Chatbot Arena (battle)"):
- get_tab_arena_battle(download_bot_config, get_bot_profile, model_mapping, client)
- with gr.TabItem("Chatbot Arena (side-by-side)"):
- get_tab_arena_side_by_side(download_bot_config, get_bot_profile, model_mapping, client)
-
-demo.launch(enable_queue=False)
diff --git a/spaces/AlexWortega/AlexWortega-instruct_rugptlarge/README.md b/spaces/AlexWortega/AlexWortega-instruct_rugptlarge/README.md
deleted file mode 100644
index 6b15fec80c565dee4048780eb503becc8eefdd15..0000000000000000000000000000000000000000
--- a/spaces/AlexWortega/AlexWortega-instruct_rugptlarge/README.md
+++ /dev/null
@@ -1,12 +0,0 @@
----
-title: AlexWortega-instruct Rugptlarge
-emoji: 😻
-colorFrom: red
-colorTo: green
-sdk: gradio
-sdk_version: 3.23.0
-app_file: app.py
-pinned: false
----
-
-Check out the configuration reference at https://huggingface.co/docs/hub/spaces-config-reference
diff --git a/spaces/Alpaca233/ChatGPT-PPT-Generate/README.md b/spaces/Alpaca233/ChatGPT-PPT-Generate/README.md
deleted file mode 100644
index a8840c104541362f6bdfb04f998104382c6e4a2c..0000000000000000000000000000000000000000
--- a/spaces/Alpaca233/ChatGPT-PPT-Generate/README.md
+++ /dev/null
@@ -1,14 +0,0 @@
----
-title: ChatGPT PPT Generate
-emoji: 🌍
-colorFrom: pink
-colorTo: indigo
-sdk: gradio
-sdk_version: 3.21.0
-app_file: app.py
-pinned: false
----
-
-Check out the configuration reference at https://huggingface.co/docs/hub/spaces-config-reference
-
-form [here](https://github.com/AmNotAGoose/Python-PPTX-ChatGPT-Presentation-Generator)
diff --git "a/spaces/Amon1/ChatGPTForAcadamic/crazy_functions/\350\247\243\346\236\220\351\241\271\347\233\256\346\272\220\344\273\243\347\240\201.py" "b/spaces/Amon1/ChatGPTForAcadamic/crazy_functions/\350\247\243\346\236\220\351\241\271\347\233\256\346\272\220\344\273\243\347\240\201.py"
deleted file mode 100644
index 172be245c2eb20f629842aaefab7f4c90f4509a2..0000000000000000000000000000000000000000
--- "a/spaces/Amon1/ChatGPTForAcadamic/crazy_functions/\350\247\243\346\236\220\351\241\271\347\233\256\346\272\220\344\273\243\347\240\201.py"
+++ /dev/null
@@ -1,213 +0,0 @@
-from predict import predict_no_ui
-from toolbox import CatchException, report_execption, write_results_to_file, predict_no_ui_but_counting_down
-fast_debug = False
-
-def 解析源代码(file_manifest, project_folder, top_p, temperature, chatbot, history, systemPromptTxt):
- import time, glob, os
- print('begin analysis on:', file_manifest)
- for index, fp in enumerate(file_manifest):
- with open(fp, 'r', encoding='utf-8') as f:
- file_content = f.read()
-
- prefix = "接下来请你逐文件分析下面的工程" if index==0 else ""
- i_say = prefix + f'请对下面的程序文件做一个概述文件名是{os.path.relpath(fp, project_folder)},文件代码是 ```{file_content}```'
- i_say_show_user = prefix + f'[{index}/{len(file_manifest)}] 请对下面的程序文件做一个概述: {os.path.abspath(fp)}'
- chatbot.append((i_say_show_user, "[Local Message] waiting gpt response."))
- yield chatbot, history, '正常'
-
- if not fast_debug:
- msg = '正常'
-
- # ** gpt request **
- gpt_say = yield from predict_no_ui_but_counting_down(i_say, i_say_show_user, chatbot, top_p, temperature, history=[]) # 带超时倒计时
-
- chatbot[-1] = (i_say_show_user, gpt_say)
- history.append(i_say_show_user); history.append(gpt_say)
- yield chatbot, history, msg
- if not fast_debug: time.sleep(2)
-
- all_file = ', '.join([os.path.relpath(fp, project_folder) for index, fp in enumerate(file_manifest)])
- i_say = f'根据以上你自己的分析,对程序的整体功能和构架做出概括。然后用一张markdown表格整理每个文件的功能(包括{all_file})。'
- chatbot.append((i_say, "[Local Message] waiting gpt response."))
- yield chatbot, history, '正常'
-
- if not fast_debug:
- msg = '正常'
- # ** gpt request **
- gpt_say = yield from predict_no_ui_but_counting_down(i_say, i_say, chatbot, top_p, temperature, history=history) # 带超时倒计时
-
- chatbot[-1] = (i_say, gpt_say)
- history.append(i_say); history.append(gpt_say)
- yield chatbot, history, msg
- res = write_results_to_file(history)
- chatbot.append(("完成了吗?", res))
- yield chatbot, history, msg
-
-
-
-
-@CatchException
-def 解析项目本身(txt, top_p, temperature, chatbot, history, systemPromptTxt, WEB_PORT):
- history = [] # 清空历史,以免输入溢出
- import time, glob, os
- file_manifest = [f for f in glob.glob('./*.py') if ('test_project' not in f) and ('gpt_log' not in f)] + \
- [f for f in glob.glob('./crazy_functions/*.py') if ('test_project' not in f) and ('gpt_log' not in f)]
- for index, fp in enumerate(file_manifest):
- # if 'test_project' in fp: continue
- with open(fp, 'r', encoding='utf-8') as f:
- file_content = f.read()
-
- prefix = "接下来请你分析自己的程序构成,别紧张," if index==0 else ""
- i_say = prefix + f'请对下面的程序文件做一个概述文件名是{fp},文件代码是 ```{file_content}```'
- i_say_show_user = prefix + f'[{index}/{len(file_manifest)}] 请对下面的程序文件做一个概述: {os.path.abspath(fp)}'
- chatbot.append((i_say_show_user, "[Local Message] waiting gpt response."))
- yield chatbot, history, '正常'
-
- if not fast_debug:
- # ** gpt request **
- # gpt_say = predict_no_ui(inputs=i_say, top_p=top_p, temperature=temperature)
- gpt_say = yield from predict_no_ui_but_counting_down(i_say, i_say_show_user, chatbot, top_p, temperature, history=[], long_connection=True) # 带超时倒计时
-
- chatbot[-1] = (i_say_show_user, gpt_say)
- history.append(i_say_show_user); history.append(gpt_say)
- yield chatbot, history, '正常'
- time.sleep(2)
-
- i_say = f'根据以上你自己的分析,对程序的整体功能和构架做出概括。然后用一张markdown表格整理每个文件的功能(包括{file_manifest})。'
- chatbot.append((i_say, "[Local Message] waiting gpt response."))
- yield chatbot, history, '正常'
-
- if not fast_debug:
- # ** gpt request **
- # gpt_say = predict_no_ui(inputs=i_say, top_p=top_p, temperature=temperature, history=history)
- gpt_say = yield from predict_no_ui_but_counting_down(i_say, i_say, chatbot, top_p, temperature, history=history, long_connection=True) # 带超时倒计时
-
- chatbot[-1] = (i_say, gpt_say)
- history.append(i_say); history.append(gpt_say)
- yield chatbot, history, '正常'
- res = write_results_to_file(history)
- chatbot.append(("完成了吗?", res))
- yield chatbot, history, '正常'
-
-@CatchException
-def 解析一个Python项目(txt, top_p, temperature, chatbot, history, systemPromptTxt, WEB_PORT):
- history = [] # 清空历史,以免输入溢出
- import glob, os
- if os.path.exists(txt):
- project_folder = txt
- else:
- if txt == "": txt = '空空如也的输入栏'
- report_execption(chatbot, history, a = f"解析项目: {txt}", b = f"找不到本地项目或无权访问: {txt}")
- yield chatbot, history, '正常'
- return
- file_manifest = [f for f in glob.glob(f'{project_folder}/**/*.py', recursive=True)]
- if len(file_manifest) == 0:
- report_execption(chatbot, history, a = f"解析项目: {txt}", b = f"找不到任何python文件: {txt}")
- yield chatbot, history, '正常'
- return
- yield from 解析源代码(file_manifest, project_folder, top_p, temperature, chatbot, history, systemPromptTxt)
-
-
-@CatchException
-def 解析一个C项目的头文件(txt, top_p, temperature, chatbot, history, systemPromptTxt, WEB_PORT):
- history = [] # 清空历史,以免输入溢出
- import glob, os
- if os.path.exists(txt):
- project_folder = txt
- else:
- if txt == "": txt = '空空如也的输入栏'
- report_execption(chatbot, history, a = f"解析项目: {txt}", b = f"找不到本地项目或无权访问: {txt}")
- yield chatbot, history, '正常'
- return
- file_manifest = [f for f in glob.glob(f'{project_folder}/**/*.h', recursive=True)] # + \
- # [f for f in glob.glob(f'{project_folder}/**/*.cpp', recursive=True)] + \
- # [f for f in glob.glob(f'{project_folder}/**/*.c', recursive=True)]
- if len(file_manifest) == 0:
- report_execption(chatbot, history, a = f"解析项目: {txt}", b = f"找不到任何.h头文件: {txt}")
- yield chatbot, history, '正常'
- return
- yield from 解析源代码(file_manifest, project_folder, top_p, temperature, chatbot, history, systemPromptTxt)
-
-@CatchException
-def 解析一个C项目(txt, top_p, temperature, chatbot, history, systemPromptTxt, WEB_PORT):
- history = [] # 清空历史,以免输入溢出
- import glob, os
- if os.path.exists(txt):
- project_folder = txt
- else:
- if txt == "": txt = '空空如也的输入栏'
- report_execption(chatbot, history, a = f"解析项目: {txt}", b = f"找不到本地项目或无权访问: {txt}")
- yield chatbot, history, '正常'
- return
- file_manifest = [f for f in glob.glob(f'{project_folder}/**/*.h', recursive=True)] + \
- [f for f in glob.glob(f'{project_folder}/**/*.cpp', recursive=True)] + \
- [f for f in glob.glob(f'{project_folder}/**/*.c', recursive=True)]
- if len(file_manifest) == 0:
- report_execption(chatbot, history, a = f"解析项目: {txt}", b = f"找不到任何.h头文件: {txt}")
- yield chatbot, history, '正常'
- return
- yield from 解析源代码(file_manifest, project_folder, top_p, temperature, chatbot, history, systemPromptTxt)
-
-
-@CatchException
-def 解析一个Java项目(txt, top_p, temperature, chatbot, history, systemPromptTxt, WEB_PORT):
- history = [] # 清空历史,以免输入溢出
- import glob, os
- if os.path.exists(txt):
- project_folder = txt
- else:
- if txt == "": txt = '空空如也的输入栏'
- report_execption(chatbot, history, a=f"解析项目: {txt}", b=f"找不到本地项目或无权访问: {txt}")
- yield chatbot, history, '正常'
- return
- file_manifest = [f for f in glob.glob(f'{project_folder}/**/*.java', recursive=True)] + \
- [f for f in glob.glob(f'{project_folder}/**/*.jar', recursive=True)] + \
- [f for f in glob.glob(f'{project_folder}/**/*.xml', recursive=True)] + \
- [f for f in glob.glob(f'{project_folder}/**/*.sh', recursive=True)]
- if len(file_manifest) == 0:
- report_execption(chatbot, history, a=f"解析项目: {txt}", b=f"找不到任何java文件: {txt}")
- yield chatbot, history, '正常'
- return
- yield from 解析源代码(file_manifest, project_folder, top_p, temperature, chatbot, history, systemPromptTxt)
-
-
-@CatchException
-def 解析一个Rect项目(txt, top_p, temperature, chatbot, history, systemPromptTxt, WEB_PORT):
- history = [] # 清空历史,以免输入溢出
- import glob, os
- if os.path.exists(txt):
- project_folder = txt
- else:
- if txt == "": txt = '空空如也的输入栏'
- report_execption(chatbot, history, a=f"解析项目: {txt}", b=f"找不到本地项目或无权访问: {txt}")
- yield chatbot, history, '正常'
- return
- file_manifest = [f for f in glob.glob(f'{project_folder}/**/*.ts', recursive=True)] + \
- [f for f in glob.glob(f'{project_folder}/**/*.tsx', recursive=True)] + \
- [f for f in glob.glob(f'{project_folder}/**/*.json', recursive=True)] + \
- [f for f in glob.glob(f'{project_folder}/**/*.js', recursive=True)] + \
- [f for f in glob.glob(f'{project_folder}/**/*.jsx', recursive=True)]
- if len(file_manifest) == 0:
- report_execption(chatbot, history, a=f"解析项目: {txt}", b=f"找不到任何Rect文件: {txt}")
- yield chatbot, history, '正常'
- return
- yield from 解析源代码(file_manifest, project_folder, top_p, temperature, chatbot, history, systemPromptTxt)
-
-
-@CatchException
-def 解析一个Golang项目(txt, top_p, temperature, chatbot, history, systemPromptTxt, WEB_PORT):
- history = [] # 清空历史,以免输入溢出
- import glob, os
- if os.path.exists(txt):
- project_folder = txt
- else:
- if txt == "": txt = '空空如也的输入栏'
- report_execption(chatbot, history, a=f"解析项目: {txt}", b=f"找不到本地项目或无权访问: {txt}")
- yield chatbot, history, '正常'
- return
- file_manifest = [f for f in glob.glob(f'{project_folder}/**/*.go', recursive=True)]
- if len(file_manifest) == 0:
- report_execption(chatbot, history, a=f"解析项目: {txt}", b=f"找不到任何golang文件: {txt}")
- yield chatbot, history, '正常'
- return
- yield from 解析源代码(file_manifest, project_folder, top_p, temperature, chatbot, history, systemPromptTxt)
diff --git a/spaces/Andres99/Tune-A-Video-Training-UI/README.md b/spaces/Andres99/Tune-A-Video-Training-UI/README.md
deleted file mode 100644
index f7281fef5e46797913556e9bd414a04daf0aff50..0000000000000000000000000000000000000000
--- a/spaces/Andres99/Tune-A-Video-Training-UI/README.md
+++ /dev/null
@@ -1,12 +0,0 @@
----
-title: Tune-A-Video Training UI
-emoji: ⚡
-colorFrom: red
-colorTo: purple
-sdk: docker
-pinned: false
-license: mit
-duplicated_from: Tune-A-Video-library/Tune-A-Video-Training-UI
----
-
-Check out the configuration reference at https://huggingface.co/docs/hub/spaces-config-reference
diff --git a/spaces/Androidonnxfork/CivitAi-to-Diffusers/diffusers/docs/source/en/api/schedulers/lms_discrete.md b/spaces/Androidonnxfork/CivitAi-to-Diffusers/diffusers/docs/source/en/api/schedulers/lms_discrete.md
deleted file mode 100644
index a7a6e87c85daed0ba5024ff2474c444ab6171068..0000000000000000000000000000000000000000
--- a/spaces/Androidonnxfork/CivitAi-to-Diffusers/diffusers/docs/source/en/api/schedulers/lms_discrete.md
+++ /dev/null
@@ -1,20 +0,0 @@
-
-
-# Linear multistep scheduler for discrete beta schedules
-
-## Overview
-
-Original implementation can be found [here](https://arxiv.org/abs/2206.00364).
-
-## LMSDiscreteScheduler
-[[autodoc]] LMSDiscreteScheduler
\ No newline at end of file
diff --git a/spaces/Androidonnxfork/CivitAi-to-Diffusers/diffusers/src/diffusers/pipelines/kandinsky/pipeline_kandinsky_inpaint.py b/spaces/Androidonnxfork/CivitAi-to-Diffusers/diffusers/src/diffusers/pipelines/kandinsky/pipeline_kandinsky_inpaint.py
deleted file mode 100644
index dda0c3faa7fd9081cd0348f72540cc094514f2eb..0000000000000000000000000000000000000000
--- a/spaces/Androidonnxfork/CivitAi-to-Diffusers/diffusers/src/diffusers/pipelines/kandinsky/pipeline_kandinsky_inpaint.py
+++ /dev/null
@@ -1,657 +0,0 @@
-# Copyright 2023 The HuggingFace Team. All rights reserved.
-#
-# Licensed under the Apache License, Version 2.0 (the "License");
-# you may not use this file except in compliance with the License.
-# You may obtain a copy of the License at
-#
-# http://www.apache.org/licenses/LICENSE-2.0
-#
-# Unless required by applicable law or agreed to in writing, software
-# distributed under the License is distributed on an "AS IS" BASIS,
-# WITHOUT WARRANTIES OR CONDITIONS OF ANY KIND, either express or implied.
-# See the License for the specific language governing permissions and
-# limitations under the License.
-
-from copy import deepcopy
-from typing import Callable, List, Optional, Union
-
-import numpy as np
-import PIL
-import torch
-import torch.nn.functional as F
-from packaging import version
-from PIL import Image
-from transformers import (
- XLMRobertaTokenizer,
-)
-
-from ... import __version__
-from ...models import UNet2DConditionModel, VQModel
-from ...schedulers import DDIMScheduler
-from ...utils import (
- is_accelerate_available,
- is_accelerate_version,
- logging,
- randn_tensor,
- replace_example_docstring,
-)
-from ..pipeline_utils import DiffusionPipeline, ImagePipelineOutput
-from .text_encoder import MultilingualCLIP
-
-
-logger = logging.get_logger(__name__) # pylint: disable=invalid-name
-
-EXAMPLE_DOC_STRING = """
- Examples:
- ```py
- >>> from diffusers import KandinskyInpaintPipeline, KandinskyPriorPipeline
- >>> from diffusers.utils import load_image
- >>> import torch
- >>> import numpy as np
-
- >>> pipe_prior = KandinskyPriorPipeline.from_pretrained(
- ... "kandinsky-community/kandinsky-2-1-prior", torch_dtype=torch.float16
- ... )
- >>> pipe_prior.to("cuda")
-
- >>> prompt = "a hat"
- >>> image_emb, zero_image_emb = pipe_prior(prompt, return_dict=False)
-
- >>> pipe = KandinskyInpaintPipeline.from_pretrained(
- ... "kandinsky-community/kandinsky-2-1-inpaint", torch_dtype=torch.float16
- ... )
- >>> pipe.to("cuda")
-
- >>> init_image = load_image(
- ... "https://huggingface.co/datasets/hf-internal-testing/diffusers-images/resolve/main"
- ... "/kandinsky/cat.png"
- ... )
-
- >>> mask = np.zeros((768, 768), dtype=np.float32)
- >>> mask[:250, 250:-250] = 1
-
- >>> out = pipe(
- ... prompt,
- ... image=init_image,
- ... mask_image=mask,
- ... image_embeds=image_emb,
- ... negative_image_embeds=zero_image_emb,
- ... height=768,
- ... width=768,
- ... num_inference_steps=50,
- ... )
-
- >>> image = out.images[0]
- >>> image.save("cat_with_hat.png")
- ```
-"""
-
-
-def get_new_h_w(h, w, scale_factor=8):
- new_h = h // scale_factor**2
- if h % scale_factor**2 != 0:
- new_h += 1
- new_w = w // scale_factor**2
- if w % scale_factor**2 != 0:
- new_w += 1
- return new_h * scale_factor, new_w * scale_factor
-
-
-def prepare_mask(masks):
- prepared_masks = []
- for mask in masks:
- old_mask = deepcopy(mask)
- for i in range(mask.shape[1]):
- for j in range(mask.shape[2]):
- if old_mask[0][i][j] == 1:
- continue
- if i != 0:
- mask[:, i - 1, j] = 0
- if j != 0:
- mask[:, i, j - 1] = 0
- if i != 0 and j != 0:
- mask[:, i - 1, j - 1] = 0
- if i != mask.shape[1] - 1:
- mask[:, i + 1, j] = 0
- if j != mask.shape[2] - 1:
- mask[:, i, j + 1] = 0
- if i != mask.shape[1] - 1 and j != mask.shape[2] - 1:
- mask[:, i + 1, j + 1] = 0
- prepared_masks.append(mask)
- return torch.stack(prepared_masks, dim=0)
-
-
-def prepare_mask_and_masked_image(image, mask, height, width):
- r"""
- Prepares a pair (mask, image) to be consumed by the Kandinsky inpaint pipeline. This means that those inputs will
- be converted to ``torch.Tensor`` with shapes ``batch x channels x height x width`` where ``channels`` is ``3`` for
- the ``image`` and ``1`` for the ``mask``.
-
- The ``image`` will be converted to ``torch.float32`` and normalized to be in ``[-1, 1]``. The ``mask`` will be
- binarized (``mask > 0.5``) and cast to ``torch.float32`` too.
-
- Args:
- image (Union[np.array, PIL.Image, torch.Tensor]): The image to inpaint.
- It can be a ``PIL.Image``, or a ``height x width x 3`` ``np.array`` or a ``channels x height x width``
- ``torch.Tensor`` or a ``batch x channels x height x width`` ``torch.Tensor``.
- mask (_type_): The mask to apply to the image, i.e. regions to inpaint.
- It can be a ``PIL.Image``, or a ``height x width`` ``np.array`` or a ``1 x height x width``
- ``torch.Tensor`` or a ``batch x 1 x height x width`` ``torch.Tensor``.
- height (`int`, *optional*, defaults to 512):
- The height in pixels of the generated image.
- width (`int`, *optional*, defaults to 512):
- The width in pixels of the generated image.
-
-
- Raises:
- ValueError: ``torch.Tensor`` images should be in the ``[-1, 1]`` range. ValueError: ``torch.Tensor`` mask
- should be in the ``[0, 1]`` range. ValueError: ``mask`` and ``image`` should have the same spatial dimensions.
- TypeError: ``mask`` is a ``torch.Tensor`` but ``image`` is not
- (ot the other way around).
-
- Returns:
- tuple[torch.Tensor]: The pair (mask, image) as ``torch.Tensor`` with 4
- dimensions: ``batch x channels x height x width``.
- """
-
- if image is None:
- raise ValueError("`image` input cannot be undefined.")
-
- if mask is None:
- raise ValueError("`mask_image` input cannot be undefined.")
-
- if isinstance(image, torch.Tensor):
- if not isinstance(mask, torch.Tensor):
- raise TypeError(f"`image` is a torch.Tensor but `mask` (type: {type(mask)} is not")
-
- # Batch single image
- if image.ndim == 3:
- assert image.shape[0] == 3, "Image outside a batch should be of shape (3, H, W)"
- image = image.unsqueeze(0)
-
- # Batch and add channel dim for single mask
- if mask.ndim == 2:
- mask = mask.unsqueeze(0).unsqueeze(0)
-
- # Batch single mask or add channel dim
- if mask.ndim == 3:
- # Single batched mask, no channel dim or single mask not batched but channel dim
- if mask.shape[0] == 1:
- mask = mask.unsqueeze(0)
-
- # Batched masks no channel dim
- else:
- mask = mask.unsqueeze(1)
-
- assert image.ndim == 4 and mask.ndim == 4, "Image and Mask must have 4 dimensions"
- assert image.shape[-2:] == mask.shape[-2:], "Image and Mask must have the same spatial dimensions"
- assert image.shape[0] == mask.shape[0], "Image and Mask must have the same batch size"
-
- # Check image is in [-1, 1]
- if image.min() < -1 or image.max() > 1:
- raise ValueError("Image should be in [-1, 1] range")
-
- # Check mask is in [0, 1]
- if mask.min() < 0 or mask.max() > 1:
- raise ValueError("Mask should be in [0, 1] range")
-
- # Binarize mask
- mask[mask < 0.5] = 0
- mask[mask >= 0.5] = 1
-
- # Image as float32
- image = image.to(dtype=torch.float32)
- elif isinstance(mask, torch.Tensor):
- raise TypeError(f"`mask` is a torch.Tensor but `image` (type: {type(image)} is not")
- else:
- # preprocess image
- if isinstance(image, (PIL.Image.Image, np.ndarray)):
- image = [image]
-
- if isinstance(image, list) and isinstance(image[0], PIL.Image.Image):
- # resize all images w.r.t passed height an width
- image = [i.resize((width, height), resample=Image.BICUBIC, reducing_gap=1) for i in image]
- image = [np.array(i.convert("RGB"))[None, :] for i in image]
- image = np.concatenate(image, axis=0)
- elif isinstance(image, list) and isinstance(image[0], np.ndarray):
- image = np.concatenate([i[None, :] for i in image], axis=0)
-
- image = image.transpose(0, 3, 1, 2)
- image = torch.from_numpy(image).to(dtype=torch.float32) / 127.5 - 1.0
-
- # preprocess mask
- if isinstance(mask, (PIL.Image.Image, np.ndarray)):
- mask = [mask]
-
- if isinstance(mask, list) and isinstance(mask[0], PIL.Image.Image):
- mask = [i.resize((width, height), resample=PIL.Image.LANCZOS) for i in mask]
- mask = np.concatenate([np.array(m.convert("L"))[None, None, :] for m in mask], axis=0)
- mask = mask.astype(np.float32) / 255.0
- elif isinstance(mask, list) and isinstance(mask[0], np.ndarray):
- mask = np.concatenate([m[None, None, :] for m in mask], axis=0)
-
- mask[mask < 0.5] = 0
- mask[mask >= 0.5] = 1
- mask = torch.from_numpy(mask)
-
- mask = 1 - mask
-
- return mask, image
-
-
-class KandinskyInpaintPipeline(DiffusionPipeline):
- """
- Pipeline for text-guided image inpainting using Kandinsky2.1
-
- This model inherits from [`DiffusionPipeline`]. Check the superclass documentation for the generic methods the
- library implements for all the pipelines (such as downloading or saving, running on a particular device, etc.)
-
- Args:
- text_encoder ([`MultilingualCLIP`]):
- Frozen text-encoder.
- tokenizer ([`XLMRobertaTokenizer`]):
- Tokenizer of class
- scheduler ([`DDIMScheduler`]):
- A scheduler to be used in combination with `unet` to generate image latents.
- unet ([`UNet2DConditionModel`]):
- Conditional U-Net architecture to denoise the image embedding.
- movq ([`VQModel`]):
- MoVQ image encoder and decoder
- """
-
- def __init__(
- self,
- text_encoder: MultilingualCLIP,
- movq: VQModel,
- tokenizer: XLMRobertaTokenizer,
- unet: UNet2DConditionModel,
- scheduler: DDIMScheduler,
- ):
- super().__init__()
-
- self.register_modules(
- text_encoder=text_encoder,
- movq=movq,
- tokenizer=tokenizer,
- unet=unet,
- scheduler=scheduler,
- )
- self.movq_scale_factor = 2 ** (len(self.movq.config.block_out_channels) - 1)
- self._warn_has_been_called = False
-
- # Copied from diffusers.pipelines.unclip.pipeline_unclip.UnCLIPPipeline.prepare_latents
- def prepare_latents(self, shape, dtype, device, generator, latents, scheduler):
- if latents is None:
- latents = randn_tensor(shape, generator=generator, device=device, dtype=dtype)
- else:
- if latents.shape != shape:
- raise ValueError(f"Unexpected latents shape, got {latents.shape}, expected {shape}")
- latents = latents.to(device)
-
- latents = latents * scheduler.init_noise_sigma
- return latents
-
- def _encode_prompt(
- self,
- prompt,
- device,
- num_images_per_prompt,
- do_classifier_free_guidance,
- negative_prompt=None,
- ):
- batch_size = len(prompt) if isinstance(prompt, list) else 1
- # get prompt text embeddings
- text_inputs = self.tokenizer(
- prompt,
- padding="max_length",
- max_length=77,
- truncation=True,
- return_attention_mask=True,
- add_special_tokens=True,
- return_tensors="pt",
- )
-
- text_input_ids = text_inputs.input_ids
- untruncated_ids = self.tokenizer(prompt, padding="longest", return_tensors="pt").input_ids
-
- if untruncated_ids.shape[-1] >= text_input_ids.shape[-1] and not torch.equal(text_input_ids, untruncated_ids):
- removed_text = self.tokenizer.batch_decode(untruncated_ids[:, self.tokenizer.model_max_length - 1 : -1])
- logger.warning(
- "The following part of your input was truncated because CLIP can only handle sequences up to"
- f" {self.tokenizer.model_max_length} tokens: {removed_text}"
- )
-
- text_input_ids = text_input_ids.to(device)
- text_mask = text_inputs.attention_mask.to(device)
-
- prompt_embeds, text_encoder_hidden_states = self.text_encoder(
- input_ids=text_input_ids, attention_mask=text_mask
- )
-
- prompt_embeds = prompt_embeds.repeat_interleave(num_images_per_prompt, dim=0)
- text_encoder_hidden_states = text_encoder_hidden_states.repeat_interleave(num_images_per_prompt, dim=0)
- text_mask = text_mask.repeat_interleave(num_images_per_prompt, dim=0)
-
- if do_classifier_free_guidance:
- uncond_tokens: List[str]
- if negative_prompt is None:
- uncond_tokens = [""] * batch_size
- elif type(prompt) is not type(negative_prompt):
- raise TypeError(
- f"`negative_prompt` should be the same type to `prompt`, but got {type(negative_prompt)} !="
- f" {type(prompt)}."
- )
- elif isinstance(negative_prompt, str):
- uncond_tokens = [negative_prompt]
- elif batch_size != len(negative_prompt):
- raise ValueError(
- f"`negative_prompt`: {negative_prompt} has batch size {len(negative_prompt)}, but `prompt`:"
- f" {prompt} has batch size {batch_size}. Please make sure that passed `negative_prompt` matches"
- " the batch size of `prompt`."
- )
- else:
- uncond_tokens = negative_prompt
-
- uncond_input = self.tokenizer(
- uncond_tokens,
- padding="max_length",
- max_length=77,
- truncation=True,
- return_attention_mask=True,
- add_special_tokens=True,
- return_tensors="pt",
- )
- uncond_text_input_ids = uncond_input.input_ids.to(device)
- uncond_text_mask = uncond_input.attention_mask.to(device)
-
- negative_prompt_embeds, uncond_text_encoder_hidden_states = self.text_encoder(
- input_ids=uncond_text_input_ids, attention_mask=uncond_text_mask
- )
-
- # duplicate unconditional embeddings for each generation per prompt, using mps friendly method
-
- seq_len = negative_prompt_embeds.shape[1]
- negative_prompt_embeds = negative_prompt_embeds.repeat(1, num_images_per_prompt)
- negative_prompt_embeds = negative_prompt_embeds.view(batch_size * num_images_per_prompt, seq_len)
-
- seq_len = uncond_text_encoder_hidden_states.shape[1]
- uncond_text_encoder_hidden_states = uncond_text_encoder_hidden_states.repeat(1, num_images_per_prompt, 1)
- uncond_text_encoder_hidden_states = uncond_text_encoder_hidden_states.view(
- batch_size * num_images_per_prompt, seq_len, -1
- )
- uncond_text_mask = uncond_text_mask.repeat_interleave(num_images_per_prompt, dim=0)
-
- # done duplicates
-
- # For classifier free guidance, we need to do two forward passes.
- # Here we concatenate the unconditional and text embeddings into a single batch
- # to avoid doing two forward passes
- prompt_embeds = torch.cat([negative_prompt_embeds, prompt_embeds])
- text_encoder_hidden_states = torch.cat([uncond_text_encoder_hidden_states, text_encoder_hidden_states])
-
- text_mask = torch.cat([uncond_text_mask, text_mask])
-
- return prompt_embeds, text_encoder_hidden_states, text_mask
-
- def enable_model_cpu_offload(self, gpu_id=0):
- r"""
- Offloads all models to CPU using accelerate, reducing memory usage with a low impact on performance. Compared
- to `enable_sequential_cpu_offload`, this method moves one whole model at a time to the GPU when its `forward`
- method is called, and the model remains in GPU until the next model runs. Memory savings are lower than with
- `enable_sequential_cpu_offload`, but performance is much better due to the iterative execution of the `unet`.
- """
- if is_accelerate_available() and is_accelerate_version(">=", "0.17.0.dev0"):
- from accelerate import cpu_offload_with_hook
- else:
- raise ImportError("`enable_model_cpu_offload` requires `accelerate v0.17.0` or higher.")
-
- device = torch.device(f"cuda:{gpu_id}")
-
- if self.device.type != "cpu":
- self.to("cpu", silence_dtype_warnings=True)
- torch.cuda.empty_cache() # otherwise we don't see the memory savings (but they probably exist)
-
- hook = None
- for cpu_offloaded_model in [self.text_encoder, self.unet, self.movq]:
- _, hook = cpu_offload_with_hook(cpu_offloaded_model, device, prev_module_hook=hook)
-
- # We'll offload the last model manually.
- self.final_offload_hook = hook
-
- @torch.no_grad()
- @replace_example_docstring(EXAMPLE_DOC_STRING)
- def __call__(
- self,
- prompt: Union[str, List[str]],
- image: Union[torch.FloatTensor, PIL.Image.Image],
- mask_image: Union[torch.FloatTensor, PIL.Image.Image, np.ndarray],
- image_embeds: torch.FloatTensor,
- negative_image_embeds: torch.FloatTensor,
- negative_prompt: Optional[Union[str, List[str]]] = None,
- height: int = 512,
- width: int = 512,
- num_inference_steps: int = 100,
- guidance_scale: float = 4.0,
- num_images_per_prompt: int = 1,
- generator: Optional[Union[torch.Generator, List[torch.Generator]]] = None,
- latents: Optional[torch.FloatTensor] = None,
- output_type: Optional[str] = "pil",
- callback: Optional[Callable[[int, int, torch.FloatTensor], None]] = None,
- callback_steps: int = 1,
- return_dict: bool = True,
- ):
- """
- Function invoked when calling the pipeline for generation.
-
- Args:
- prompt (`str` or `List[str]`):
- The prompt or prompts to guide the image generation.
- image (`torch.FloatTensor`, `PIL.Image.Image` or `np.ndarray`):
- `Image`, or tensor representing an image batch, that will be used as the starting point for the
- process.
- mask_image (`PIL.Image.Image`,`torch.FloatTensor` or `np.ndarray`):
- `Image`, or a tensor representing an image batch, to mask `image`. White pixels in the mask will be
- repainted, while black pixels will be preserved. You can pass a pytorch tensor as mask only if the
- image you passed is a pytorch tensor, and it should contain one color channel (L) instead of 3, so the
- expected shape would be either `(B, 1, H, W,)`, `(B, H, W)`, `(1, H, W)` or `(H, W)` If image is an PIL
- image or numpy array, mask should also be a either PIL image or numpy array. If it is a PIL image, it
- will be converted to a single channel (luminance) before use. If it is a nummpy array, the expected
- shape is `(H, W)`.
- image_embeds (`torch.FloatTensor` or `List[torch.FloatTensor]`):
- The clip image embeddings for text prompt, that will be used to condition the image generation.
- negative_image_embeds (`torch.FloatTensor` or `List[torch.FloatTensor]`):
- The clip image embeddings for negative text prompt, will be used to condition the image generation.
- negative_prompt (`str` or `List[str]`, *optional*):
- The prompt or prompts not to guide the image generation. Ignored when not using guidance (i.e., ignored
- if `guidance_scale` is less than `1`).
- height (`int`, *optional*, defaults to 512):
- The height in pixels of the generated image.
- width (`int`, *optional*, defaults to 512):
- The width in pixels of the generated image.
- num_inference_steps (`int`, *optional*, defaults to 100):
- The number of denoising steps. More denoising steps usually lead to a higher quality image at the
- expense of slower inference.
- guidance_scale (`float`, *optional*, defaults to 4.0):
- Guidance scale as defined in [Classifier-Free Diffusion Guidance](https://arxiv.org/abs/2207.12598).
- `guidance_scale` is defined as `w` of equation 2. of [Imagen
- Paper](https://arxiv.org/pdf/2205.11487.pdf). Guidance scale is enabled by setting `guidance_scale >
- 1`. Higher guidance scale encourages to generate images that are closely linked to the text `prompt`,
- usually at the expense of lower image quality.
- num_images_per_prompt (`int`, *optional*, defaults to 1):
- The number of images to generate per prompt.
- generator (`torch.Generator` or `List[torch.Generator]`, *optional*):
- One or a list of [torch generator(s)](https://pytorch.org/docs/stable/generated/torch.Generator.html)
- to make generation deterministic.
- latents (`torch.FloatTensor`, *optional*):
- Pre-generated noisy latents, sampled from a Gaussian distribution, to be used as inputs for image
- generation. Can be used to tweak the same generation with different prompts. If not provided, a latents
- tensor will ge generated by sampling using the supplied random `generator`.
- output_type (`str`, *optional*, defaults to `"pil"`):
- The output format of the generate image. Choose between: `"pil"` (`PIL.Image.Image`), `"np"`
- (`np.array`) or `"pt"` (`torch.Tensor`).
- callback (`Callable`, *optional*):
- A function that calls every `callback_steps` steps during inference. The function is called with the
- following arguments: `callback(step: int, timestep: int, latents: torch.FloatTensor)`.
- callback_steps (`int`, *optional*, defaults to 1):
- The frequency at which the `callback` function is called. If not specified, the callback is called at
- every step.
- return_dict (`bool`, *optional*, defaults to `True`):
- Whether or not to return a [`~pipelines.ImagePipelineOutput`] instead of a plain tuple.
-
- Examples:
-
- Returns:
- [`~pipelines.ImagePipelineOutput`] or `tuple`
- """
- if not self._warn_has_been_called and version.parse(version.parse(__version__).base_version) < version.parse(
- "0.22.0.dev0"
- ):
- logger.warn(
- "Please note that the expected format of `mask_image` has recently been changed. "
- "Before diffusers == 0.19.0, Kandinsky Inpainting pipelines repainted black pixels and preserved black pixels. "
- "As of diffusers==0.19.0 this behavior has been inverted. Now white pixels are repainted and black pixels are preserved. "
- "This way, Kandinsky's masking behavior is aligned with Stable Diffusion. "
- "THIS means that you HAVE to invert the input mask to have the same behavior as before as explained in https://github.com/huggingface/diffusers/pull/4207. "
- "This warning will be surpressed after the first inference call and will be removed in diffusers>0.22.0"
- )
- self._warn_has_been_called = True
-
- # Define call parameters
- if isinstance(prompt, str):
- batch_size = 1
- elif isinstance(prompt, list):
- batch_size = len(prompt)
- else:
- raise ValueError(f"`prompt` has to be of type `str` or `list` but is {type(prompt)}")
-
- device = self._execution_device
-
- batch_size = batch_size * num_images_per_prompt
- do_classifier_free_guidance = guidance_scale > 1.0
-
- prompt_embeds, text_encoder_hidden_states, _ = self._encode_prompt(
- prompt, device, num_images_per_prompt, do_classifier_free_guidance, negative_prompt
- )
-
- if isinstance(image_embeds, list):
- image_embeds = torch.cat(image_embeds, dim=0)
- if isinstance(negative_image_embeds, list):
- negative_image_embeds = torch.cat(negative_image_embeds, dim=0)
-
- if do_classifier_free_guidance:
- image_embeds = image_embeds.repeat_interleave(num_images_per_prompt, dim=0)
- negative_image_embeds = negative_image_embeds.repeat_interleave(num_images_per_prompt, dim=0)
-
- image_embeds = torch.cat([negative_image_embeds, image_embeds], dim=0).to(
- dtype=prompt_embeds.dtype, device=device
- )
-
- # preprocess image and mask
- mask_image, image = prepare_mask_and_masked_image(image, mask_image, height, width)
-
- image = image.to(dtype=prompt_embeds.dtype, device=device)
- image = self.movq.encode(image)["latents"]
-
- mask_image = mask_image.to(dtype=prompt_embeds.dtype, device=device)
-
- image_shape = tuple(image.shape[-2:])
- mask_image = F.interpolate(
- mask_image,
- image_shape,
- mode="nearest",
- )
- mask_image = prepare_mask(mask_image)
- masked_image = image * mask_image
-
- mask_image = mask_image.repeat_interleave(num_images_per_prompt, dim=0)
- masked_image = masked_image.repeat_interleave(num_images_per_prompt, dim=0)
- if do_classifier_free_guidance:
- mask_image = mask_image.repeat(2, 1, 1, 1)
- masked_image = masked_image.repeat(2, 1, 1, 1)
-
- self.scheduler.set_timesteps(num_inference_steps, device=device)
- timesteps_tensor = self.scheduler.timesteps
-
- num_channels_latents = self.movq.config.latent_channels
-
- # get h, w for latents
- sample_height, sample_width = get_new_h_w(height, width, self.movq_scale_factor)
-
- # create initial latent
- latents = self.prepare_latents(
- (batch_size, num_channels_latents, sample_height, sample_width),
- text_encoder_hidden_states.dtype,
- device,
- generator,
- latents,
- self.scheduler,
- )
-
- # Check that sizes of mask, masked image and latents match with expected
- num_channels_mask = mask_image.shape[1]
- num_channels_masked_image = masked_image.shape[1]
- if num_channels_latents + num_channels_mask + num_channels_masked_image != self.unet.config.in_channels:
- raise ValueError(
- f"Incorrect configuration settings! The config of `pipeline.unet`: {self.unet.config} expects"
- f" {self.unet.config.in_channels} but received `num_channels_latents`: {num_channels_latents} +"
- f" `num_channels_mask`: {num_channels_mask} + `num_channels_masked_image`: {num_channels_masked_image}"
- f" = {num_channels_latents+num_channels_masked_image+num_channels_mask}. Please verify the config of"
- " `pipeline.unet` or your `mask_image` or `image` input."
- )
-
- for i, t in enumerate(self.progress_bar(timesteps_tensor)):
- # expand the latents if we are doing classifier free guidance
- latent_model_input = torch.cat([latents] * 2) if do_classifier_free_guidance else latents
- latent_model_input = torch.cat([latent_model_input, masked_image, mask_image], dim=1)
-
- added_cond_kwargs = {"text_embeds": prompt_embeds, "image_embeds": image_embeds}
- noise_pred = self.unet(
- sample=latent_model_input,
- timestep=t,
- encoder_hidden_states=text_encoder_hidden_states,
- added_cond_kwargs=added_cond_kwargs,
- return_dict=False,
- )[0]
-
- if do_classifier_free_guidance:
- noise_pred, variance_pred = noise_pred.split(latents.shape[1], dim=1)
- noise_pred_uncond, noise_pred_text = noise_pred.chunk(2)
- _, variance_pred_text = variance_pred.chunk(2)
- noise_pred = noise_pred_uncond + guidance_scale * (noise_pred_text - noise_pred_uncond)
- noise_pred = torch.cat([noise_pred, variance_pred_text], dim=1)
-
- if not (
- hasattr(self.scheduler.config, "variance_type")
- and self.scheduler.config.variance_type in ["learned", "learned_range"]
- ):
- noise_pred, _ = noise_pred.split(latents.shape[1], dim=1)
-
- # compute the previous noisy sample x_t -> x_t-1
- latents = self.scheduler.step(
- noise_pred,
- t,
- latents,
- generator=generator,
- ).prev_sample
-
- if callback is not None and i % callback_steps == 0:
- callback(i, t, latents)
-
- # post-processing
- image = self.movq.decode(latents, force_not_quantize=True)["sample"]
-
- if output_type not in ["pt", "np", "pil"]:
- raise ValueError(f"Only the output types `pt`, `pil` and `np` are supported not output_type={output_type}")
-
- if output_type in ["np", "pil"]:
- image = image * 0.5 + 0.5
- image = image.clamp(0, 1)
- image = image.cpu().permute(0, 2, 3, 1).float().numpy()
-
- if output_type == "pil":
- image = self.numpy_to_pil(image)
-
- if not return_dict:
- return (image,)
-
- return ImagePipelineOutput(images=image)
diff --git a/spaces/Andy1621/uniformer_image_detection/mmdet/core/export/pytorch2onnx.py b/spaces/Andy1621/uniformer_image_detection/mmdet/core/export/pytorch2onnx.py
deleted file mode 100644
index 809a817e67446b3c0c7894dcefb3c4bbc29afb7e..0000000000000000000000000000000000000000
--- a/spaces/Andy1621/uniformer_image_detection/mmdet/core/export/pytorch2onnx.py
+++ /dev/null
@@ -1,154 +0,0 @@
-from functools import partial
-
-import mmcv
-import numpy as np
-import torch
-from mmcv.runner import load_checkpoint
-
-
-def generate_inputs_and_wrap_model(config_path,
- checkpoint_path,
- input_config,
- cfg_options=None):
- """Prepare sample input and wrap model for ONNX export.
-
- The ONNX export API only accept args, and all inputs should be
- torch.Tensor or corresponding types (such as tuple of tensor).
- So we should call this function before exporting. This function will:
-
- 1. generate corresponding inputs which are used to execute the model.
- 2. Wrap the model's forward function.
-
- For example, the MMDet models' forward function has a parameter
- ``return_loss:bool``. As we want to set it as False while export API
- supports neither bool type or kwargs. So we have to replace the forward
- like: ``model.forward = partial(model.forward, return_loss=False)``
-
- Args:
- config_path (str): the OpenMMLab config for the model we want to
- export to ONNX
- checkpoint_path (str): Path to the corresponding checkpoint
- input_config (dict): the exactly data in this dict depends on the
- framework. For MMSeg, we can just declare the input shape,
- and generate the dummy data accordingly. However, for MMDet,
- we may pass the real img path, or the NMS will return None
- as there is no legal bbox.
-
- Returns:
- tuple: (model, tensor_data) wrapped model which can be called by \
- model(*tensor_data) and a list of inputs which are used to execute \
- the model while exporting.
- """
-
- model = build_model_from_cfg(
- config_path, checkpoint_path, cfg_options=cfg_options)
- one_img, one_meta = preprocess_example_input(input_config)
- tensor_data = [one_img]
- model.forward = partial(
- model.forward, img_metas=[[one_meta]], return_loss=False)
-
- # pytorch has some bug in pytorch1.3, we have to fix it
- # by replacing these existing op
- opset_version = 11
- # put the import within the function thus it will not cause import error
- # when not using this function
- try:
- from mmcv.onnx.symbolic import register_extra_symbolics
- except ModuleNotFoundError:
- raise NotImplementedError('please update mmcv to version>=v1.0.4')
- register_extra_symbolics(opset_version)
-
- return model, tensor_data
-
-
-def build_model_from_cfg(config_path, checkpoint_path, cfg_options=None):
- """Build a model from config and load the given checkpoint.
-
- Args:
- config_path (str): the OpenMMLab config for the model we want to
- export to ONNX
- checkpoint_path (str): Path to the corresponding checkpoint
-
- Returns:
- torch.nn.Module: the built model
- """
- from mmdet.models import build_detector
-
- cfg = mmcv.Config.fromfile(config_path)
- if cfg_options is not None:
- cfg.merge_from_dict(cfg_options)
- # import modules from string list.
- if cfg.get('custom_imports', None):
- from mmcv.utils import import_modules_from_strings
- import_modules_from_strings(**cfg['custom_imports'])
- # set cudnn_benchmark
- if cfg.get('cudnn_benchmark', False):
- torch.backends.cudnn.benchmark = True
- cfg.model.pretrained = None
- cfg.data.test.test_mode = True
-
- # build the model
- cfg.model.train_cfg = None
- model = build_detector(cfg.model, test_cfg=cfg.get('test_cfg'))
- load_checkpoint(model, checkpoint_path, map_location='cpu')
- model.cpu().eval()
- return model
-
-
-def preprocess_example_input(input_config):
- """Prepare an example input image for ``generate_inputs_and_wrap_model``.
-
- Args:
- input_config (dict): customized config describing the example input.
-
- Returns:
- tuple: (one_img, one_meta), tensor of the example input image and \
- meta information for the example input image.
-
- Examples:
- >>> from mmdet.core.export import preprocess_example_input
- >>> input_config = {
- >>> 'input_shape': (1,3,224,224),
- >>> 'input_path': 'demo/demo.jpg',
- >>> 'normalize_cfg': {
- >>> 'mean': (123.675, 116.28, 103.53),
- >>> 'std': (58.395, 57.12, 57.375)
- >>> }
- >>> }
- >>> one_img, one_meta = preprocess_example_input(input_config)
- >>> print(one_img.shape)
- torch.Size([1, 3, 224, 224])
- >>> print(one_meta)
- {'img_shape': (224, 224, 3),
- 'ori_shape': (224, 224, 3),
- 'pad_shape': (224, 224, 3),
- 'filename': '.png',
- 'scale_factor': 1.0,
- 'flip': False}
- """
- input_path = input_config['input_path']
- input_shape = input_config['input_shape']
- one_img = mmcv.imread(input_path)
- one_img = mmcv.imresize(one_img, input_shape[2:][::-1])
- show_img = one_img.copy()
- if 'normalize_cfg' in input_config.keys():
- normalize_cfg = input_config['normalize_cfg']
- mean = np.array(normalize_cfg['mean'], dtype=np.float32)
- std = np.array(normalize_cfg['std'], dtype=np.float32)
- to_rgb = normalize_cfg.get('to_rgb', True)
- one_img = mmcv.imnormalize(one_img, mean, std, to_rgb=to_rgb)
- one_img = one_img.transpose(2, 0, 1)
- one_img = torch.from_numpy(one_img).unsqueeze(0).float().requires_grad_(
- True)
- (_, C, H, W) = input_shape
- one_meta = {
- 'img_shape': (H, W, C),
- 'ori_shape': (H, W, C),
- 'pad_shape': (H, W, C),
- 'filename': '.png',
- 'scale_factor': 1.0,
- 'flip': False,
- 'show_img': show_img,
- }
-
- return one_img, one_meta
diff --git a/spaces/AnnasBlackHat/Image-Similarity/app.py b/spaces/AnnasBlackHat/Image-Similarity/app.py
deleted file mode 100644
index a813cd907544302be6d44ac0c4b546a68fafabad..0000000000000000000000000000000000000000
--- a/spaces/AnnasBlackHat/Image-Similarity/app.py
+++ /dev/null
@@ -1,32 +0,0 @@
-import gradio as gr
-import os
-import random
-from src.model import simlarity_model as model
-from src.similarity.similarity import Similarity
-
-similarity = Similarity()
-models = similarity.get_models()
-
-def check(img_main, img_1, img_2, model_idx):
- result = similarity.check_similarity([img_main, img_1, img_2], models[model_idx])
- return result
-
-with gr.Blocks() as demo:
- gr.Markdown('Checking Image Similarity')
- img_main = gr.Text(label='Main Image', placeholder='https://myimage.jpg')
-
- gr.Markdown('Images to check')
- img_1 = gr.Text(label='1st Image', placeholder='https://myimage_1.jpg')
- img_2 = gr.Text(label='2nd Image', placeholder='https://myimage_2.jpg')
-
- gr.Markdown('Choose the model')
- model = gr.Dropdown([m.name for m in models], label='Model', type='index')
-
- gallery = gr.Gallery(
- label="Generated images", show_label=False, elem_id="gallery"
- ).style(grid=[2], height="auto")
-
- submit_btn = gr.Button('Check Similarity')
- submit_btn.click(fn=check,inputs=[img_main, img_1, img_2, model], outputs=gallery)
-
-demo.launch()
\ No newline at end of file
diff --git a/spaces/Anonymous-sub/Rerender/ControlNet/annotator/uniformer/exp/upernet_global_small/test_config_h32.py b/spaces/Anonymous-sub/Rerender/ControlNet/annotator/uniformer/exp/upernet_global_small/test_config_h32.py
deleted file mode 100644
index a31e3874f76f9f7b089ac8834d85df2441af9b0e..0000000000000000000000000000000000000000
--- a/spaces/Anonymous-sub/Rerender/ControlNet/annotator/uniformer/exp/upernet_global_small/test_config_h32.py
+++ /dev/null
@@ -1,39 +0,0 @@
-_base_ = [
- '../../configs/_base_/models/upernet_uniformer.py',
- '../../configs/_base_/datasets/ade20k.py',
- '../../configs/_base_/default_runtime.py',
- '../../configs/_base_/schedules/schedule_160k.py'
-]
-model = dict(
- backbone=dict(
- type='UniFormer',
- embed_dim=[64, 128, 320, 512],
- layers=[3, 4, 8, 3],
- head_dim=64,
- drop_path_rate=0.25,
- windows=False,
- hybrid=True,
- window_size=32
- ),
- decode_head=dict(
- in_channels=[64, 128, 320, 512],
- num_classes=150
- ),
- auxiliary_head=dict(
- in_channels=320,
- num_classes=150
- ))
-
-# AdamW optimizer, no weight decay for position embedding & layer norm in backbone
-optimizer = dict(_delete_=True, type='AdamW', lr=0.00006, betas=(0.9, 0.999), weight_decay=0.01,
- paramwise_cfg=dict(custom_keys={'absolute_pos_embed': dict(decay_mult=0.),
- 'relative_position_bias_table': dict(decay_mult=0.),
- 'norm': dict(decay_mult=0.)}))
-
-lr_config = dict(_delete_=True, policy='poly',
- warmup='linear',
- warmup_iters=1500,
- warmup_ratio=1e-6,
- power=1.0, min_lr=0.0, by_epoch=False)
-
-data=dict(samples_per_gpu=2)
\ No newline at end of file
diff --git a/spaces/Anustup/NS_AI_LABS/app-local.py b/spaces/Anustup/NS_AI_LABS/app-local.py
deleted file mode 100644
index d8eabbc62924dab3d0cc03a8a2373ffffe01eadc..0000000000000000000000000000000000000000
--- a/spaces/Anustup/NS_AI_LABS/app-local.py
+++ /dev/null
@@ -1,3 +0,0 @@
-# Run the app with no audio file restrictions
-from app import create_ui
-create_ui(-1)
\ No newline at end of file
diff --git a/spaces/Arcader7171/positive/README.md b/spaces/Arcader7171/positive/README.md
deleted file mode 100644
index 9d58e9c4c7fba60658fb073293b2529488aa2e97..0000000000000000000000000000000000000000
--- a/spaces/Arcader7171/positive/README.md
+++ /dev/null
@@ -1,12 +0,0 @@
----
-title: Positive
-emoji: 🚀
-colorFrom: blue
-colorTo: red
-sdk: gradio
-sdk_version: 3.12.0
-app_file: app.py
-pinned: false
----
-
-Check out the configuration reference at https://huggingface.co/docs/hub/spaces-config-reference
diff --git a/spaces/Armored-Atom/gpt2/app.py b/spaces/Armored-Atom/gpt2/app.py
deleted file mode 100644
index 4205e03f91904065e1610f7e6c7b2f1de1771184..0000000000000000000000000000000000000000
--- a/spaces/Armored-Atom/gpt2/app.py
+++ /dev/null
@@ -1,3 +0,0 @@
-import gradio as gr
-
-gr.Interface.load("models/gpt2").launch()
\ No newline at end of file
diff --git a/spaces/Artrajz/vits-simple-api/static/css/style.css b/spaces/Artrajz/vits-simple-api/static/css/style.css
deleted file mode 100644
index 275ec332c1708e619b30a1fb9df2a1fd9ca45799..0000000000000000000000000000000000000000
--- a/spaces/Artrajz/vits-simple-api/static/css/style.css
+++ /dev/null
@@ -1,84 +0,0 @@
-.main-container {
- position: relative;
- width: 100%;
- min-height: 300px;
-}
-
-.container {
- width: 300px;
- position: relative;
-}
-
-
-/*tabs*/
-.tabs {
- display: flex;
- left: 0;
-}
-
-.tab-button {
- display: inline-block;
- background-color: transparent;
- padding: 5px 10px;
- cursor: pointer;
- margin-bottom: -2px;
- border-top: 2px solid transparent;
- border-left: 2px solid transparent;
- border-right: 2px solid transparent;
- border-bottom: 0px;
- border-top-left-radius: 0.5rem;
- border-top-right-radius: 0.5rem;
- color: gray;
-}
-
-.tab-button.active {
- background-color: white;
- border-top: 2px solid #dee2e6;
- border-left: 2px solid #dee2e6;
- border-right: 2px solid #dee2e6;
- color: black;
-}
-
-/*content*/
-
-.content {
- border: gray;
- border-left-width: 2px;
-}
-
-.content-pane {
- display: none;
- padding: 20px;
-}
-
-.content-pane.active {
- display: flex;
- -ms-flex-wrap: wrap;
- flex-wrap: wrap;
-}
-
-*, :before, :after {
- box-sizing: border-box;
- border-width: 0;
- border-style: solid;
- border-color: #e5e7eb;
-}
-
-
-.flex {
- display: flex;
-}
-
-.border-transparent {
- border-color: transparent;
-}
-
-.border-b-2 {
- border-bottom: 2px solid #dee2e6;
-}
-
-.border-lr-2 {
- border-left: 2px solid #dee2e6;
- border-right: 2px solid #dee2e6;
-}
-
diff --git a/spaces/AvaterClasher/Food_Classifier_Refined_MONI/README.md b/spaces/AvaterClasher/Food_Classifier_Refined_MONI/README.md
deleted file mode 100644
index f23120a1858a6eb293712f6f3ef792b323f88d79..0000000000000000000000000000000000000000
--- a/spaces/AvaterClasher/Food_Classifier_Refined_MONI/README.md
+++ /dev/null
@@ -1,13 +0,0 @@
----
-title: Food Classifier Refined MONI
-emoji: 🐢
-colorFrom: gray
-colorTo: red
-sdk: gradio
-sdk_version: 3.42.0
-app_file: app.py
-pinned: false
-license: mit
----
-
-Check out the configuration reference at https://huggingface.co/docs/hub/spaces-config-reference
diff --git a/spaces/Awesimo/jojogan/app.py b/spaces/Awesimo/jojogan/app.py
deleted file mode 100644
index 603f709d7df99edf19f1885ff93629e58419e949..0000000000000000000000000000000000000000
--- a/spaces/Awesimo/jojogan/app.py
+++ /dev/null
@@ -1,124 +0,0 @@
-import os
-from PIL import Image
-import torch
-import gradio as gr
-import torch
-torch.backends.cudnn.benchmark = True
-from torchvision import transforms, utils
-from util import *
-from PIL import Image
-import math
-import random
-import numpy as np
-from torch import nn, autograd, optim
-from torch.nn import functional as F
-from tqdm import tqdm
-import lpips
-from model import *
-from copy import deepcopy
-import imageio
-
-import os
-import sys
-import numpy as np
-from PIL import Image
-import torch
-import torchvision.transforms as transforms
-from argparse import Namespace
-from e4e.models.psp import pSp
-from util import *
-from huggingface_hub import hf_hub_download
-
-device= 'cpu'
-model_path_e = hf_hub_download(repo_id="akhaliq/JoJoGAN_e4e_ffhq_encode", filename="e4e_ffhq_encode.pt")
-ckpt = torch.load(model_path_e, map_location='cpu')
-opts = ckpt['opts']
-opts['checkpoint_path'] = model_path_e
-opts= Namespace(**opts)
-net = pSp(opts, device).eval().to(device)
-
-@ torch.no_grad()
-def projection(img, name, device='cuda'):
-
- transform = transforms.Compose(
- [
- transforms.Resize(256),
- transforms.CenterCrop(256),
- transforms.ToTensor(),
- transforms.Normalize([0.5, 0.5, 0.5], [0.5, 0.5, 0.5]),
- ]
- )
- img = transform(img).unsqueeze(0).to(device)
- images, w_plus = net(img, randomize_noise=False, return_latents=True)
- result_file = {}
- result_file['latent'] = w_plus[0]
- torch.save(result_file, name)
- return w_plus[0]
-
-device = 'cpu'
-
-latent_dim = 512
-
-model_path_s = hf_hub_download(repo_id="akhaliq/jojogan-stylegan2-ffhq-config-f", filename="stylegan2-ffhq-config-f.pt")
-original_generator = Generator(1024, latent_dim, 8, 2).to(device)
-ckpt = torch.load(model_path_s, map_location=lambda storage, loc: storage)
-original_generator.load_state_dict(ckpt["g_ema"], strict=False)
-mean_latent = original_generator.mean_latent(10000)
-
-
-#MODELS
-generatorzombie = deepcopy(original_generator)
-generatorhulk = deepcopy(original_generator)
-generatorjojo = deepcopy(original_generator)
-generatorwalker = deepcopy(original_generator)
-
-transform = transforms.Compose(
- [
- transforms.Resize((1024, 1024)),
- transforms.ToTensor(),
- transforms.Normalize((0.5, 0.5, 0.5), (0.5, 0.5, 0.5)),
- ]
-)
-
-#HULK
-modelhulk = hf_hub_download(repo_id="Awesimo/jojogan-hulk", filename="hulk.pt")
-ckpthulk = torch.load(modelhulk, map_location=lambda storage, loc: storage)
-generatorhulk.load_state_dict(ckpthulk["g"], strict=False)
-
-#ZOMBIE
-modelzombie = hf_hub_download(repo_id="Awesimo/jojogan-zombie", filename="zombie.pt")
-ckptzombie = torch.load(modelzombie, map_location=lambda storage, loc: storage)
-generatorzombie.load_state_dict(ckptzombie["g"], strict=False)
-
-#WHITE WALKER
-modelwalker = hf_hub_download(repo_id="Awesimo/jojogan-white-walker", filename="white_walker_v2.pt")
-ckptwalker = torch.load(modelwalker, map_location=lambda storage, loc: storage)
-generatorwalker.load_state_dict(ckptwalker["g"], strict=False)
-
-
-def inference(img, model):
- img.save('out.jpg')
- aligned_face = align_face('out.jpg')
-
- my_w = projection(aligned_face, "test.pt", device).unsqueeze(0)
- if model == 'Hulk':
- with torch.no_grad():
- my_sample = generatorhulk(my_w, input_is_latent=True)
- elif model == 'Zombie':
- with torch.no_grad():
- my_sample = generatorzombie(my_w, input_is_latent=True)
- elif model == 'White-Walker':
- with torch.no_grad():
- my_sample = generatorwalker(my_w, input_is_latent=True)
- else:
- with torch.no_grad():
- my_sample = generatorzombie(my_w, input_is_latent=True)
-
-
- npimage = my_sample[0].permute(1, 2, 0).detach().numpy()
- imageio.imwrite('filename.jpeg', npimage)
- return 'filename.jpeg'
-
-title = "JoJoGAN Test 🤖"
-examples=[['assets/samples/image01.jpg','Hulk'],['assets/samples/image02.jpg','Zombie'],['assets/samples/image03.jpg','White-Walker'],['assets/samples/image04.jpg','Hulk']]
-gr.Interface(inference, [gr.inputs.Image(type="pil"),gr.inputs.Dropdown(choices=['Hulk', 'Zombie', 'White-Walker'], type="value", default='Hulk', label="Model")], gr.outputs.Image(type="file"),title=title,allow_flagging=False,examples=examples,allow_screenshot=False).launch()
diff --git a/spaces/Awesimo/jojogan/e4e/editings/ganspace.py b/spaces/Awesimo/jojogan/e4e/editings/ganspace.py
deleted file mode 100644
index 0c286a421280c542e9776a75e64bb65409da8fc7..0000000000000000000000000000000000000000
--- a/spaces/Awesimo/jojogan/e4e/editings/ganspace.py
+++ /dev/null
@@ -1,22 +0,0 @@
-import torch
-
-
-def edit(latents, pca, edit_directions):
- edit_latents = []
- for latent in latents:
- for pca_idx, start, end, strength in edit_directions:
- delta = get_delta(pca, latent, pca_idx, strength)
- delta_padded = torch.zeros(latent.shape).to('cuda')
- delta_padded[start:end] += delta.repeat(end - start, 1)
- edit_latents.append(latent + delta_padded)
- return torch.stack(edit_latents)
-
-
-def get_delta(pca, latent, idx, strength):
- # pca: ganspace checkpoint. latent: (16, 512) w+
- w_centered = latent - pca['mean'].to('cuda')
- lat_comp = pca['comp'].to('cuda')
- lat_std = pca['std'].to('cuda')
- w_coord = torch.sum(w_centered[0].reshape(-1)*lat_comp[idx].reshape(-1)) / lat_std[idx]
- delta = (strength - w_coord)*lat_comp[idx]*lat_std[idx]
- return delta
diff --git a/spaces/Awiny/Image2Paragraph/models/grit_src/third_party/CenterNet2/detectron2/utils/visualizer.py b/spaces/Awiny/Image2Paragraph/models/grit_src/third_party/CenterNet2/detectron2/utils/visualizer.py
deleted file mode 100644
index 8e145181871d1981e41db3c8cbc7e8f4cc7b5833..0000000000000000000000000000000000000000
--- a/spaces/Awiny/Image2Paragraph/models/grit_src/third_party/CenterNet2/detectron2/utils/visualizer.py
+++ /dev/null
@@ -1,1267 +0,0 @@
-# Copyright (c) Facebook, Inc. and its affiliates.
-import colorsys
-import logging
-import math
-import numpy as np
-from enum import Enum, unique
-import cv2
-import matplotlib as mpl
-import matplotlib.colors as mplc
-import matplotlib.figure as mplfigure
-import pycocotools.mask as mask_util
-import torch
-from matplotlib.backends.backend_agg import FigureCanvasAgg
-from PIL import Image
-
-from detectron2.data import MetadataCatalog
-from detectron2.structures import BitMasks, Boxes, BoxMode, Keypoints, PolygonMasks, RotatedBoxes
-from detectron2.utils.file_io import PathManager
-
-from .colormap import random_color
-
-logger = logging.getLogger(__name__)
-
-__all__ = ["ColorMode", "VisImage", "Visualizer"]
-
-
-_SMALL_OBJECT_AREA_THRESH = 1000
-_LARGE_MASK_AREA_THRESH = 120000
-_OFF_WHITE = (1.0, 1.0, 240.0 / 255)
-_BLACK = (0, 0, 0)
-_RED = (1.0, 0, 0)
-
-_KEYPOINT_THRESHOLD = 0.05
-
-
-@unique
-class ColorMode(Enum):
- """
- Enum of different color modes to use for instance visualizations.
- """
-
- IMAGE = 0
- """
- Picks a random color for every instance and overlay segmentations with low opacity.
- """
- SEGMENTATION = 1
- """
- Let instances of the same category have similar colors
- (from metadata.thing_colors), and overlay them with
- high opacity. This provides more attention on the quality of segmentation.
- """
- IMAGE_BW = 2
- """
- Same as IMAGE, but convert all areas without masks to gray-scale.
- Only available for drawing per-instance mask predictions.
- """
-
-
-class GenericMask:
- """
- Attribute:
- polygons (list[ndarray]): list[ndarray]: polygons for this mask.
- Each ndarray has format [x, y, x, y, ...]
- mask (ndarray): a binary mask
- """
-
- def __init__(self, mask_or_polygons, height, width):
- self._mask = self._polygons = self._has_holes = None
- self.height = height
- self.width = width
-
- m = mask_or_polygons
- if isinstance(m, dict):
- # RLEs
- assert "counts" in m and "size" in m
- if isinstance(m["counts"], list): # uncompressed RLEs
- h, w = m["size"]
- assert h == height and w == width
- m = mask_util.frPyObjects(m, h, w)
- self._mask = mask_util.decode(m)[:, :]
- return
-
- if isinstance(m, list): # list[ndarray]
- self._polygons = [np.asarray(x).reshape(-1) for x in m]
- return
-
- if isinstance(m, np.ndarray): # assumed to be a binary mask
- assert m.shape[1] != 2, m.shape
- assert m.shape == (
- height,
- width,
- ), f"mask shape: {m.shape}, target dims: {height}, {width}"
- self._mask = m.astype("uint8")
- return
-
- raise ValueError("GenericMask cannot handle object {} of type '{}'".format(m, type(m)))
-
- @property
- def mask(self):
- if self._mask is None:
- self._mask = self.polygons_to_mask(self._polygons)
- return self._mask
-
- @property
- def polygons(self):
- if self._polygons is None:
- self._polygons, self._has_holes = self.mask_to_polygons(self._mask)
- return self._polygons
-
- @property
- def has_holes(self):
- if self._has_holes is None:
- if self._mask is not None:
- self._polygons, self._has_holes = self.mask_to_polygons(self._mask)
- else:
- self._has_holes = False # if original format is polygon, does not have holes
- return self._has_holes
-
- def mask_to_polygons(self, mask):
- # cv2.RETR_CCOMP flag retrieves all the contours and arranges them to a 2-level
- # hierarchy. External contours (boundary) of the object are placed in hierarchy-1.
- # Internal contours (holes) are placed in hierarchy-2.
- # cv2.CHAIN_APPROX_NONE flag gets vertices of polygons from contours.
- mask = np.ascontiguousarray(mask) # some versions of cv2 does not support incontiguous arr
- res = cv2.findContours(mask.astype("uint8"), cv2.RETR_CCOMP, cv2.CHAIN_APPROX_NONE)
- hierarchy = res[-1]
- if hierarchy is None: # empty mask
- return [], False
- has_holes = (hierarchy.reshape(-1, 4)[:, 3] >= 0).sum() > 0
- res = res[-2]
- res = [x.flatten() for x in res]
- # These coordinates from OpenCV are integers in range [0, W-1 or H-1].
- # We add 0.5 to turn them into real-value coordinate space. A better solution
- # would be to first +0.5 and then dilate the returned polygon by 0.5.
- res = [x + 0.5 for x in res if len(x) >= 6]
- return res, has_holes
-
- def polygons_to_mask(self, polygons):
- rle = mask_util.frPyObjects(polygons, self.height, self.width)
- rle = mask_util.merge(rle)
- return mask_util.decode(rle)[:, :]
-
- def area(self):
- return self.mask.sum()
-
- def bbox(self):
- p = mask_util.frPyObjects(self.polygons, self.height, self.width)
- p = mask_util.merge(p)
- bbox = mask_util.toBbox(p)
- bbox[2] += bbox[0]
- bbox[3] += bbox[1]
- return bbox
-
-
-class _PanopticPrediction:
- """
- Unify different panoptic annotation/prediction formats
- """
-
- def __init__(self, panoptic_seg, segments_info, metadata=None):
- if segments_info is None:
- assert metadata is not None
- # If "segments_info" is None, we assume "panoptic_img" is a
- # H*W int32 image storing the panoptic_id in the format of
- # category_id * label_divisor + instance_id. We reserve -1 for
- # VOID label.
- label_divisor = metadata.label_divisor
- segments_info = []
- for panoptic_label in np.unique(panoptic_seg.numpy()):
- if panoptic_label == -1:
- # VOID region.
- continue
- pred_class = panoptic_label // label_divisor
- isthing = pred_class in metadata.thing_dataset_id_to_contiguous_id.values()
- segments_info.append(
- {
- "id": int(panoptic_label),
- "category_id": int(pred_class),
- "isthing": bool(isthing),
- }
- )
- del metadata
-
- self._seg = panoptic_seg
-
- self._sinfo = {s["id"]: s for s in segments_info} # seg id -> seg info
- segment_ids, areas = torch.unique(panoptic_seg, sorted=True, return_counts=True)
- areas = areas.numpy()
- sorted_idxs = np.argsort(-areas)
- self._seg_ids, self._seg_areas = segment_ids[sorted_idxs], areas[sorted_idxs]
- self._seg_ids = self._seg_ids.tolist()
- for sid, area in zip(self._seg_ids, self._seg_areas):
- if sid in self._sinfo:
- self._sinfo[sid]["area"] = float(area)
-
- def non_empty_mask(self):
- """
- Returns:
- (H, W) array, a mask for all pixels that have a prediction
- """
- empty_ids = []
- for id in self._seg_ids:
- if id not in self._sinfo:
- empty_ids.append(id)
- if len(empty_ids) == 0:
- return np.zeros(self._seg.shape, dtype=np.uint8)
- assert (
- len(empty_ids) == 1
- ), ">1 ids corresponds to no labels. This is currently not supported"
- return (self._seg != empty_ids[0]).numpy().astype(np.bool)
-
- def semantic_masks(self):
- for sid in self._seg_ids:
- sinfo = self._sinfo.get(sid)
- if sinfo is None or sinfo["isthing"]:
- # Some pixels (e.g. id 0 in PanopticFPN) have no instance or semantic predictions.
- continue
- yield (self._seg == sid).numpy().astype(np.bool), sinfo
-
- def instance_masks(self):
- for sid in self._seg_ids:
- sinfo = self._sinfo.get(sid)
- if sinfo is None or not sinfo["isthing"]:
- continue
- mask = (self._seg == sid).numpy().astype(np.bool)
- if mask.sum() > 0:
- yield mask, sinfo
-
-
-def _create_text_labels(classes, scores, class_names, is_crowd=None):
- """
- Args:
- classes (list[int] or None):
- scores (list[float] or None):
- class_names (list[str] or None):
- is_crowd (list[bool] or None):
-
- Returns:
- list[str] or None
- """
- labels = None
- if classes is not None:
- if class_names is not None and len(class_names) > 0:
- labels = [class_names[i] for i in classes]
- else:
- labels = [str(i) for i in classes]
- if scores is not None:
- if labels is None:
- labels = ["{:.0f}%".format(s * 100) for s in scores]
- else:
- labels = ["{} {:.0f}%".format(l, s * 100) for l, s in zip(labels, scores)]
- if labels is not None and is_crowd is not None:
- labels = [l + ("|crowd" if crowd else "") for l, crowd in zip(labels, is_crowd)]
- return labels
-
-
-class VisImage:
- def __init__(self, img, scale=1.0):
- """
- Args:
- img (ndarray): an RGB image of shape (H, W, 3) in range [0, 255].
- scale (float): scale the input image
- """
- self.img = img
- self.scale = scale
- self.width, self.height = img.shape[1], img.shape[0]
- self._setup_figure(img)
-
- def _setup_figure(self, img):
- """
- Args:
- Same as in :meth:`__init__()`.
-
- Returns:
- fig (matplotlib.pyplot.figure): top level container for all the image plot elements.
- ax (matplotlib.pyplot.Axes): contains figure elements and sets the coordinate system.
- """
- fig = mplfigure.Figure(frameon=False)
- self.dpi = fig.get_dpi()
- # add a small 1e-2 to avoid precision lost due to matplotlib's truncation
- # (https://github.com/matplotlib/matplotlib/issues/15363)
- fig.set_size_inches(
- (self.width * self.scale + 1e-2) / self.dpi,
- (self.height * self.scale + 1e-2) / self.dpi,
- )
- self.canvas = FigureCanvasAgg(fig)
- # self.canvas = mpl.backends.backend_cairo.FigureCanvasCairo(fig)
- ax = fig.add_axes([0.0, 0.0, 1.0, 1.0])
- ax.axis("off")
- self.fig = fig
- self.ax = ax
- self.reset_image(img)
-
- def reset_image(self, img):
- """
- Args:
- img: same as in __init__
- """
- img = img.astype("uint8")
- self.ax.imshow(img, extent=(0, self.width, self.height, 0), interpolation="nearest")
-
- def save(self, filepath):
- """
- Args:
- filepath (str): a string that contains the absolute path, including the file name, where
- the visualized image will be saved.
- """
- self.fig.savefig(filepath)
-
- def get_image(self):
- """
- Returns:
- ndarray:
- the visualized image of shape (H, W, 3) (RGB) in uint8 type.
- The shape is scaled w.r.t the input image using the given `scale` argument.
- """
- canvas = self.canvas
- s, (width, height) = canvas.print_to_buffer()
- # buf = io.BytesIO() # works for cairo backend
- # canvas.print_rgba(buf)
- # width, height = self.width, self.height
- # s = buf.getvalue()
-
- buffer = np.frombuffer(s, dtype="uint8")
-
- img_rgba = buffer.reshape(height, width, 4)
- rgb, alpha = np.split(img_rgba, [3], axis=2)
- return rgb.astype("uint8")
-
-
-class Visualizer:
- """
- Visualizer that draws data about detection/segmentation on images.
-
- It contains methods like `draw_{text,box,circle,line,binary_mask,polygon}`
- that draw primitive objects to images, as well as high-level wrappers like
- `draw_{instance_predictions,sem_seg,panoptic_seg_predictions,dataset_dict}`
- that draw composite data in some pre-defined style.
-
- Note that the exact visualization style for the high-level wrappers are subject to change.
- Style such as color, opacity, label contents, visibility of labels, or even the visibility
- of objects themselves (e.g. when the object is too small) may change according
- to different heuristics, as long as the results still look visually reasonable.
-
- To obtain a consistent style, you can implement custom drawing functions with the
- abovementioned primitive methods instead. If you need more customized visualization
- styles, you can process the data yourself following their format documented in
- tutorials (:doc:`/tutorials/models`, :doc:`/tutorials/datasets`). This class does not
- intend to satisfy everyone's preference on drawing styles.
-
- This visualizer focuses on high rendering quality rather than performance. It is not
- designed to be used for real-time applications.
- """
-
- # TODO implement a fast, rasterized version using OpenCV
-
- def __init__(self, img_rgb, metadata=None, scale=1.0, instance_mode=ColorMode.IMAGE):
- """
- Args:
- img_rgb: a numpy array of shape (H, W, C), where H and W correspond to
- the height and width of the image respectively. C is the number of
- color channels. The image is required to be in RGB format since that
- is a requirement of the Matplotlib library. The image is also expected
- to be in the range [0, 255].
- metadata (Metadata): dataset metadata (e.g. class names and colors)
- instance_mode (ColorMode): defines one of the pre-defined style for drawing
- instances on an image.
- """
- self.img = np.asarray(img_rgb).clip(0, 255).astype(np.uint8)
- if metadata is None:
- metadata = MetadataCatalog.get("__nonexist__")
- self.metadata = metadata
- self.output = VisImage(self.img, scale=scale)
- self.cpu_device = torch.device("cpu")
-
- # too small texts are useless, therefore clamp to 9
- self._default_font_size = max(
- np.sqrt(self.output.height * self.output.width) // 90, 10 // scale
- )
- self._instance_mode = instance_mode
- self.keypoint_threshold = _KEYPOINT_THRESHOLD
-
- def draw_instance_predictions(self, predictions):
- """
- Draw instance-level prediction results on an image.
-
- Args:
- predictions (Instances): the output of an instance detection/segmentation
- model. Following fields will be used to draw:
- "pred_boxes", "pred_classes", "scores", "pred_masks" (or "pred_masks_rle").
-
- Returns:
- output (VisImage): image object with visualizations.
- """
- boxes = predictions.pred_boxes if predictions.has("pred_boxes") else None
- scores = predictions.scores if predictions.has("scores") else None
- classes = predictions.pred_classes.tolist() if predictions.has("pred_classes") else None
- labels = _create_text_labels(classes, scores, self.metadata.get("thing_classes", None))
- keypoints = predictions.pred_keypoints if predictions.has("pred_keypoints") else None
-
- if predictions.has("pred_masks"):
- masks = np.asarray(predictions.pred_masks)
- masks = [GenericMask(x, self.output.height, self.output.width) for x in masks]
- else:
- masks = None
-
- if self._instance_mode == ColorMode.SEGMENTATION and self.metadata.get("thing_colors"):
- colors = [
- self._jitter([x / 255 for x in self.metadata.thing_colors[c]]) for c in classes
- ]
- alpha = 0.8
- else:
- colors = None
- alpha = 0.5
-
- if self._instance_mode == ColorMode.IMAGE_BW:
- self.output.reset_image(
- self._create_grayscale_image(
- (predictions.pred_masks.any(dim=0) > 0).numpy()
- if predictions.has("pred_masks")
- else None
- )
- )
- alpha = 0.3
-
- self.overlay_instances(
- masks=masks,
- boxes=boxes,
- labels=labels,
- keypoints=keypoints,
- assigned_colors=colors,
- alpha=alpha,
- )
- return self.output
-
- def draw_sem_seg(self, sem_seg, area_threshold=None, alpha=0.8):
- """
- Draw semantic segmentation predictions/labels.
-
- Args:
- sem_seg (Tensor or ndarray): the segmentation of shape (H, W).
- Each value is the integer label of the pixel.
- area_threshold (int): segments with less than `area_threshold` are not drawn.
- alpha (float): the larger it is, the more opaque the segmentations are.
-
- Returns:
- output (VisImage): image object with visualizations.
- """
- if isinstance(sem_seg, torch.Tensor):
- sem_seg = sem_seg.numpy()
- labels, areas = np.unique(sem_seg, return_counts=True)
- sorted_idxs = np.argsort(-areas).tolist()
- labels = labels[sorted_idxs]
- for label in filter(lambda l: l < len(self.metadata.stuff_classes), labels):
- try:
- mask_color = [x / 255 for x in self.metadata.stuff_colors[label]]
- except (AttributeError, IndexError):
- mask_color = None
-
- binary_mask = (sem_seg == label).astype(np.uint8)
- text = self.metadata.stuff_classes[label]
- self.draw_binary_mask(
- binary_mask,
- color=mask_color,
- edge_color=_OFF_WHITE,
- text=text,
- alpha=alpha,
- area_threshold=area_threshold,
- )
- return self.output
-
- def draw_panoptic_seg(self, panoptic_seg, segments_info, area_threshold=None, alpha=0.7):
- """
- Draw panoptic prediction annotations or results.
-
- Args:
- panoptic_seg (Tensor): of shape (height, width) where the values are ids for each
- segment.
- segments_info (list[dict] or None): Describe each segment in `panoptic_seg`.
- If it is a ``list[dict]``, each dict contains keys "id", "category_id".
- If None, category id of each pixel is computed by
- ``pixel // metadata.label_divisor``.
- area_threshold (int): stuff segments with less than `area_threshold` are not drawn.
-
- Returns:
- output (VisImage): image object with visualizations.
- """
- pred = _PanopticPrediction(panoptic_seg, segments_info, self.metadata)
-
- if self._instance_mode == ColorMode.IMAGE_BW:
- self.output.reset_image(self._create_grayscale_image(pred.non_empty_mask()))
-
- # draw mask for all semantic segments first i.e. "stuff"
- for mask, sinfo in pred.semantic_masks():
- category_idx = sinfo["category_id"]
- try:
- mask_color = [x / 255 for x in self.metadata.stuff_colors[category_idx]]
- except AttributeError:
- mask_color = None
-
- text = self.metadata.stuff_classes[category_idx]
- self.draw_binary_mask(
- mask,
- color=mask_color,
- edge_color=_OFF_WHITE,
- text=text,
- alpha=alpha,
- area_threshold=area_threshold,
- )
-
- # draw mask for all instances second
- all_instances = list(pred.instance_masks())
- if len(all_instances) == 0:
- return self.output
- masks, sinfo = list(zip(*all_instances))
- category_ids = [x["category_id"] for x in sinfo]
-
- try:
- scores = [x["score"] for x in sinfo]
- except KeyError:
- scores = None
- labels = _create_text_labels(
- category_ids, scores, self.metadata.thing_classes, [x.get("iscrowd", 0) for x in sinfo]
- )
-
- try:
- colors = [
- self._jitter([x / 255 for x in self.metadata.thing_colors[c]]) for c in category_ids
- ]
- except AttributeError:
- colors = None
- self.overlay_instances(masks=masks, labels=labels, assigned_colors=colors, alpha=alpha)
-
- return self.output
-
- draw_panoptic_seg_predictions = draw_panoptic_seg # backward compatibility
-
- def draw_dataset_dict(self, dic):
- """
- Draw annotations/segmentaions in Detectron2 Dataset format.
-
- Args:
- dic (dict): annotation/segmentation data of one image, in Detectron2 Dataset format.
-
- Returns:
- output (VisImage): image object with visualizations.
- """
- annos = dic.get("annotations", None)
- if annos:
- if "segmentation" in annos[0]:
- masks = [x["segmentation"] for x in annos]
- else:
- masks = None
- if "keypoints" in annos[0]:
- keypts = [x["keypoints"] for x in annos]
- keypts = np.array(keypts).reshape(len(annos), -1, 3)
- else:
- keypts = None
-
- boxes = [
- BoxMode.convert(x["bbox"], x["bbox_mode"], BoxMode.XYXY_ABS)
- if len(x["bbox"]) == 4
- else x["bbox"]
- for x in annos
- ]
-
- colors = None
- category_ids = [x["category_id"] for x in annos]
- if self._instance_mode == ColorMode.SEGMENTATION and self.metadata.get("thing_colors"):
- colors = [
- self._jitter([x / 255 for x in self.metadata.thing_colors[c]])
- for c in category_ids
- ]
- names = self.metadata.get("thing_classes", None)
- labels = _create_text_labels(
- category_ids,
- scores=None,
- class_names=names,
- is_crowd=[x.get("iscrowd", 0) for x in annos],
- )
- self.overlay_instances(
- labels=labels, boxes=boxes, masks=masks, keypoints=keypts, assigned_colors=colors
- )
-
- sem_seg = dic.get("sem_seg", None)
- if sem_seg is None and "sem_seg_file_name" in dic:
- with PathManager.open(dic["sem_seg_file_name"], "rb") as f:
- sem_seg = Image.open(f)
- sem_seg = np.asarray(sem_seg, dtype="uint8")
- if sem_seg is not None:
- self.draw_sem_seg(sem_seg, area_threshold=0, alpha=0.5)
-
- pan_seg = dic.get("pan_seg", None)
- if pan_seg is None and "pan_seg_file_name" in dic:
- with PathManager.open(dic["pan_seg_file_name"], "rb") as f:
- pan_seg = Image.open(f)
- pan_seg = np.asarray(pan_seg)
- from panopticapi.utils import rgb2id
-
- pan_seg = rgb2id(pan_seg)
- if pan_seg is not None:
- segments_info = dic["segments_info"]
- pan_seg = torch.tensor(pan_seg)
- self.draw_panoptic_seg(pan_seg, segments_info, area_threshold=0, alpha=0.5)
- return self.output
-
- def overlay_instances(
- self,
- *,
- boxes=None,
- labels=None,
- masks=None,
- keypoints=None,
- assigned_colors=None,
- alpha=0.5,
- ):
- """
- Args:
- boxes (Boxes, RotatedBoxes or ndarray): either a :class:`Boxes`,
- or an Nx4 numpy array of XYXY_ABS format for the N objects in a single image,
- or a :class:`RotatedBoxes`,
- or an Nx5 numpy array of (x_center, y_center, width, height, angle_degrees) format
- for the N objects in a single image,
- labels (list[str]): the text to be displayed for each instance.
- masks (masks-like object): Supported types are:
-
- * :class:`detectron2.structures.PolygonMasks`,
- :class:`detectron2.structures.BitMasks`.
- * list[list[ndarray]]: contains the segmentation masks for all objects in one image.
- The first level of the list corresponds to individual instances. The second
- level to all the polygon that compose the instance, and the third level
- to the polygon coordinates. The third level should have the format of
- [x0, y0, x1, y1, ..., xn, yn] (n >= 3).
- * list[ndarray]: each ndarray is a binary mask of shape (H, W).
- * list[dict]: each dict is a COCO-style RLE.
- keypoints (Keypoint or array like): an array-like object of shape (N, K, 3),
- where the N is the number of instances and K is the number of keypoints.
- The last dimension corresponds to (x, y, visibility or score).
- assigned_colors (list[matplotlib.colors]): a list of colors, where each color
- corresponds to each mask or box in the image. Refer to 'matplotlib.colors'
- for full list of formats that the colors are accepted in.
- Returns:
- output (VisImage): image object with visualizations.
- """
- num_instances = 0
- if boxes is not None:
- boxes = self._convert_boxes(boxes)
- num_instances = len(boxes)
- if masks is not None:
- masks = self._convert_masks(masks)
- if num_instances:
- assert len(masks) == num_instances
- else:
- num_instances = len(masks)
- if keypoints is not None:
- if num_instances:
- assert len(keypoints) == num_instances
- else:
- num_instances = len(keypoints)
- keypoints = self._convert_keypoints(keypoints)
- if labels is not None:
- assert len(labels) == num_instances
- if assigned_colors is None:
- assigned_colors = [random_color(rgb=True, maximum=1) for _ in range(num_instances)]
- if num_instances == 0:
- return self.output
- if boxes is not None and boxes.shape[1] == 5:
- return self.overlay_rotated_instances(
- boxes=boxes, labels=labels, assigned_colors=assigned_colors
- )
-
- # Display in largest to smallest order to reduce occlusion.
- areas = None
- if boxes is not None:
- areas = np.prod(boxes[:, 2:] - boxes[:, :2], axis=1)
- elif masks is not None:
- areas = np.asarray([x.area() for x in masks])
-
- if areas is not None:
- sorted_idxs = np.argsort(-areas).tolist()
- # Re-order overlapped instances in descending order.
- boxes = boxes[sorted_idxs] if boxes is not None else None
- labels = [labels[k] for k in sorted_idxs] if labels is not None else None
- masks = [masks[idx] for idx in sorted_idxs] if masks is not None else None
- assigned_colors = [assigned_colors[idx] for idx in sorted_idxs]
- keypoints = keypoints[sorted_idxs] if keypoints is not None else None
-
- for i in range(num_instances):
- color = assigned_colors[i]
- if boxes is not None:
- self.draw_box(boxes[i], edge_color=color)
-
- if masks is not None:
- for segment in masks[i].polygons:
- self.draw_polygon(segment.reshape(-1, 2), color, alpha=alpha)
-
- if labels is not None:
- # first get a box
- if boxes is not None:
- x0, y0, x1, y1 = boxes[i]
- text_pos = (x0, y0) # if drawing boxes, put text on the box corner.
- horiz_align = "left"
- elif masks is not None:
- # skip small mask without polygon
- if len(masks[i].polygons) == 0:
- continue
-
- x0, y0, x1, y1 = masks[i].bbox()
-
- # draw text in the center (defined by median) when box is not drawn
- # median is less sensitive to outliers.
- text_pos = np.median(masks[i].mask.nonzero(), axis=1)[::-1]
- horiz_align = "center"
- else:
- continue # drawing the box confidence for keypoints isn't very useful.
- # for small objects, draw text at the side to avoid occlusion
- instance_area = (y1 - y0) * (x1 - x0)
- if (
- instance_area < _SMALL_OBJECT_AREA_THRESH * self.output.scale
- or y1 - y0 < 40 * self.output.scale
- ):
- if y1 >= self.output.height - 5:
- text_pos = (x1, y0)
- else:
- text_pos = (x0, y1)
-
- height_ratio = (y1 - y0) / np.sqrt(self.output.height * self.output.width)
- lighter_color = self._change_color_brightness(color, brightness_factor=0.7)
- font_size = (
- np.clip((height_ratio - 0.02) / 0.08 + 1, 1.2, 2)
- * 0.5
- * self._default_font_size
- )
- self.draw_text(
- labels[i],
- text_pos,
- color=lighter_color,
- horizontal_alignment=horiz_align,
- font_size=font_size,
- )
-
- # draw keypoints
- if keypoints is not None:
- for keypoints_per_instance in keypoints:
- self.draw_and_connect_keypoints(keypoints_per_instance)
-
- return self.output
-
- def overlay_rotated_instances(self, boxes=None, labels=None, assigned_colors=None):
- """
- Args:
- boxes (ndarray): an Nx5 numpy array of
- (x_center, y_center, width, height, angle_degrees) format
- for the N objects in a single image.
- labels (list[str]): the text to be displayed for each instance.
- assigned_colors (list[matplotlib.colors]): a list of colors, where each color
- corresponds to each mask or box in the image. Refer to 'matplotlib.colors'
- for full list of formats that the colors are accepted in.
-
- Returns:
- output (VisImage): image object with visualizations.
- """
- num_instances = len(boxes)
-
- if assigned_colors is None:
- assigned_colors = [random_color(rgb=True, maximum=1) for _ in range(num_instances)]
- if num_instances == 0:
- return self.output
-
- # Display in largest to smallest order to reduce occlusion.
- if boxes is not None:
- areas = boxes[:, 2] * boxes[:, 3]
-
- sorted_idxs = np.argsort(-areas).tolist()
- # Re-order overlapped instances in descending order.
- boxes = boxes[sorted_idxs]
- labels = [labels[k] for k in sorted_idxs] if labels is not None else None
- colors = [assigned_colors[idx] for idx in sorted_idxs]
-
- for i in range(num_instances):
- self.draw_rotated_box_with_label(
- boxes[i], edge_color=colors[i], label=labels[i] if labels is not None else None
- )
-
- return self.output
-
- def draw_and_connect_keypoints(self, keypoints):
- """
- Draws keypoints of an instance and follows the rules for keypoint connections
- to draw lines between appropriate keypoints. This follows color heuristics for
- line color.
-
- Args:
- keypoints (Tensor): a tensor of shape (K, 3), where K is the number of keypoints
- and the last dimension corresponds to (x, y, probability).
-
- Returns:
- output (VisImage): image object with visualizations.
- """
- visible = {}
- keypoint_names = self.metadata.get("keypoint_names")
- for idx, keypoint in enumerate(keypoints):
-
- # draw keypoint
- x, y, prob = keypoint
- if prob > self.keypoint_threshold:
- self.draw_circle((x, y), color=_RED)
- if keypoint_names:
- keypoint_name = keypoint_names[idx]
- visible[keypoint_name] = (x, y)
-
- if self.metadata.get("keypoint_connection_rules"):
- for kp0, kp1, color in self.metadata.keypoint_connection_rules:
- if kp0 in visible and kp1 in visible:
- x0, y0 = visible[kp0]
- x1, y1 = visible[kp1]
- color = tuple(x / 255.0 for x in color)
- self.draw_line([x0, x1], [y0, y1], color=color)
-
- # draw lines from nose to mid-shoulder and mid-shoulder to mid-hip
- # Note that this strategy is specific to person keypoints.
- # For other keypoints, it should just do nothing
- try:
- ls_x, ls_y = visible["left_shoulder"]
- rs_x, rs_y = visible["right_shoulder"]
- mid_shoulder_x, mid_shoulder_y = (ls_x + rs_x) / 2, (ls_y + rs_y) / 2
- except KeyError:
- pass
- else:
- # draw line from nose to mid-shoulder
- nose_x, nose_y = visible.get("nose", (None, None))
- if nose_x is not None:
- self.draw_line([nose_x, mid_shoulder_x], [nose_y, mid_shoulder_y], color=_RED)
-
- try:
- # draw line from mid-shoulder to mid-hip
- lh_x, lh_y = visible["left_hip"]
- rh_x, rh_y = visible["right_hip"]
- except KeyError:
- pass
- else:
- mid_hip_x, mid_hip_y = (lh_x + rh_x) / 2, (lh_y + rh_y) / 2
- self.draw_line([mid_hip_x, mid_shoulder_x], [mid_hip_y, mid_shoulder_y], color=_RED)
- return self.output
-
- """
- Primitive drawing functions:
- """
-
- def draw_text(
- self,
- text,
- position,
- *,
- font_size=None,
- color="g",
- horizontal_alignment="center",
- rotation=0,
- ):
- """
- Args:
- text (str): class label
- position (tuple): a tuple of the x and y coordinates to place text on image.
- font_size (int, optional): font of the text. If not provided, a font size
- proportional to the image width is calculated and used.
- color: color of the text. Refer to `matplotlib.colors` for full list
- of formats that are accepted.
- horizontal_alignment (str): see `matplotlib.text.Text`
- rotation: rotation angle in degrees CCW
-
- Returns:
- output (VisImage): image object with text drawn.
- """
- if not font_size:
- font_size = self._default_font_size
-
- # since the text background is dark, we don't want the text to be dark
- color = np.maximum(list(mplc.to_rgb(color)), 0.2)
- color[np.argmax(color)] = max(0.8, np.max(color))
-
- x, y = position
- self.output.ax.text(
- x,
- y,
- text,
- size=font_size * self.output.scale,
- family="sans-serif",
- bbox={"facecolor": "black", "alpha": 0.8, "pad": 0.7, "edgecolor": "none"},
- verticalalignment="top",
- horizontalalignment=horizontal_alignment,
- color=color,
- zorder=10,
- rotation=rotation,
- )
- return self.output
-
- def draw_box(self, box_coord, alpha=0.5, edge_color="g", line_style="-"):
- """
- Args:
- box_coord (tuple): a tuple containing x0, y0, x1, y1 coordinates, where x0 and y0
- are the coordinates of the image's top left corner. x1 and y1 are the
- coordinates of the image's bottom right corner.
- alpha (float): blending efficient. Smaller values lead to more transparent masks.
- edge_color: color of the outline of the box. Refer to `matplotlib.colors`
- for full list of formats that are accepted.
- line_style (string): the string to use to create the outline of the boxes.
-
- Returns:
- output (VisImage): image object with box drawn.
- """
- x0, y0, x1, y1 = box_coord
- width = x1 - x0
- height = y1 - y0
-
- linewidth = max(self._default_font_size / 4, 1)
-
- self.output.ax.add_patch(
- mpl.patches.Rectangle(
- (x0, y0),
- width,
- height,
- fill=False,
- edgecolor=edge_color,
- linewidth=linewidth * self.output.scale,
- alpha=alpha,
- linestyle=line_style,
- )
- )
- return self.output
-
- def draw_rotated_box_with_label(
- self, rotated_box, alpha=0.5, edge_color="g", line_style="-", label=None
- ):
- """
- Draw a rotated box with label on its top-left corner.
-
- Args:
- rotated_box (tuple): a tuple containing (cnt_x, cnt_y, w, h, angle),
- where cnt_x and cnt_y are the center coordinates of the box.
- w and h are the width and height of the box. angle represents how
- many degrees the box is rotated CCW with regard to the 0-degree box.
- alpha (float): blending efficient. Smaller values lead to more transparent masks.
- edge_color: color of the outline of the box. Refer to `matplotlib.colors`
- for full list of formats that are accepted.
- line_style (string): the string to use to create the outline of the boxes.
- label (string): label for rotated box. It will not be rendered when set to None.
-
- Returns:
- output (VisImage): image object with box drawn.
- """
- cnt_x, cnt_y, w, h, angle = rotated_box
- area = w * h
- # use thinner lines when the box is small
- linewidth = self._default_font_size / (
- 6 if area < _SMALL_OBJECT_AREA_THRESH * self.output.scale else 3
- )
-
- theta = angle * math.pi / 180.0
- c = math.cos(theta)
- s = math.sin(theta)
- rect = [(-w / 2, h / 2), (-w / 2, -h / 2), (w / 2, -h / 2), (w / 2, h / 2)]
- # x: left->right ; y: top->down
- rotated_rect = [(s * yy + c * xx + cnt_x, c * yy - s * xx + cnt_y) for (xx, yy) in rect]
- for k in range(4):
- j = (k + 1) % 4
- self.draw_line(
- [rotated_rect[k][0], rotated_rect[j][0]],
- [rotated_rect[k][1], rotated_rect[j][1]],
- color=edge_color,
- linestyle="--" if k == 1 else line_style,
- linewidth=linewidth,
- )
-
- if label is not None:
- text_pos = rotated_rect[1] # topleft corner
-
- height_ratio = h / np.sqrt(self.output.height * self.output.width)
- label_color = self._change_color_brightness(edge_color, brightness_factor=0.7)
- font_size = (
- np.clip((height_ratio - 0.02) / 0.08 + 1, 1.2, 2) * 0.5 * self._default_font_size
- )
- self.draw_text(label, text_pos, color=label_color, font_size=font_size, rotation=angle)
-
- return self.output
-
- def draw_circle(self, circle_coord, color, radius=3):
- """
- Args:
- circle_coord (list(int) or tuple(int)): contains the x and y coordinates
- of the center of the circle.
- color: color of the polygon. Refer to `matplotlib.colors` for a full list of
- formats that are accepted.
- radius (int): radius of the circle.
-
- Returns:
- output (VisImage): image object with box drawn.
- """
- x, y = circle_coord
- self.output.ax.add_patch(
- mpl.patches.Circle(circle_coord, radius=radius, fill=True, color=color)
- )
- return self.output
-
- def draw_line(self, x_data, y_data, color, linestyle="-", linewidth=None):
- """
- Args:
- x_data (list[int]): a list containing x values of all the points being drawn.
- Length of list should match the length of y_data.
- y_data (list[int]): a list containing y values of all the points being drawn.
- Length of list should match the length of x_data.
- color: color of the line. Refer to `matplotlib.colors` for a full list of
- formats that are accepted.
- linestyle: style of the line. Refer to `matplotlib.lines.Line2D`
- for a full list of formats that are accepted.
- linewidth (float or None): width of the line. When it's None,
- a default value will be computed and used.
-
- Returns:
- output (VisImage): image object with line drawn.
- """
- if linewidth is None:
- linewidth = self._default_font_size / 3
- linewidth = max(linewidth, 1)
- self.output.ax.add_line(
- mpl.lines.Line2D(
- x_data,
- y_data,
- linewidth=linewidth * self.output.scale,
- color=color,
- linestyle=linestyle,
- )
- )
- return self.output
-
- def draw_binary_mask(
- self, binary_mask, color=None, *, edge_color=None, text=None, alpha=0.5, area_threshold=10
- ):
- """
- Args:
- binary_mask (ndarray): numpy array of shape (H, W), where H is the image height and
- W is the image width. Each value in the array is either a 0 or 1 value of uint8
- type.
- color: color of the mask. Refer to `matplotlib.colors` for a full list of
- formats that are accepted. If None, will pick a random color.
- edge_color: color of the polygon edges. Refer to `matplotlib.colors` for a
- full list of formats that are accepted.
- text (str): if None, will be drawn on the object
- alpha (float): blending efficient. Smaller values lead to more transparent masks.
- area_threshold (float): a connected component smaller than this area will not be shown.
-
- Returns:
- output (VisImage): image object with mask drawn.
- """
- if color is None:
- color = random_color(rgb=True, maximum=1)
- color = mplc.to_rgb(color)
-
- has_valid_segment = False
- binary_mask = binary_mask.astype("uint8") # opencv needs uint8
- mask = GenericMask(binary_mask, self.output.height, self.output.width)
- shape2d = (binary_mask.shape[0], binary_mask.shape[1])
-
- if not mask.has_holes:
- # draw polygons for regular masks
- for segment in mask.polygons:
- area = mask_util.area(mask_util.frPyObjects([segment], shape2d[0], shape2d[1]))
- if area < (area_threshold or 0):
- continue
- has_valid_segment = True
- segment = segment.reshape(-1, 2)
- self.draw_polygon(segment, color=color, edge_color=edge_color, alpha=alpha)
- else:
- # TODO: Use Path/PathPatch to draw vector graphics:
- # https://stackoverflow.com/questions/8919719/how-to-plot-a-complex-polygon
- rgba = np.zeros(shape2d + (4,), dtype="float32")
- rgba[:, :, :3] = color
- rgba[:, :, 3] = (mask.mask == 1).astype("float32") * alpha
- has_valid_segment = True
- self.output.ax.imshow(rgba, extent=(0, self.output.width, self.output.height, 0))
-
- if text is not None and has_valid_segment:
- lighter_color = self._change_color_brightness(color, brightness_factor=0.7)
- self._draw_text_in_mask(binary_mask, text, lighter_color)
- return self.output
-
- def draw_soft_mask(self, soft_mask, color=None, *, text=None, alpha=0.5):
- """
- Args:
- soft_mask (ndarray): float array of shape (H, W), each value in [0, 1].
- color: color of the mask. Refer to `matplotlib.colors` for a full list of
- formats that are accepted. If None, will pick a random color.
- text (str): if None, will be drawn on the object
- alpha (float): blending efficient. Smaller values lead to more transparent masks.
-
- Returns:
- output (VisImage): image object with mask drawn.
- """
- if color is None:
- color = random_color(rgb=True, maximum=1)
- color = mplc.to_rgb(color)
-
- shape2d = (soft_mask.shape[0], soft_mask.shape[1])
- rgba = np.zeros(shape2d + (4,), dtype="float32")
- rgba[:, :, :3] = color
- rgba[:, :, 3] = soft_mask * alpha
- self.output.ax.imshow(rgba, extent=(0, self.output.width, self.output.height, 0))
-
- if text is not None:
- lighter_color = self._change_color_brightness(color, brightness_factor=0.7)
- binary_mask = (soft_mask > 0.5).astype("uint8")
- self._draw_text_in_mask(binary_mask, text, lighter_color)
- return self.output
-
- def draw_polygon(self, segment, color, edge_color=None, alpha=0.5):
- """
- Args:
- segment: numpy array of shape Nx2, containing all the points in the polygon.
- color: color of the polygon. Refer to `matplotlib.colors` for a full list of
- formats that are accepted.
- edge_color: color of the polygon edges. Refer to `matplotlib.colors` for a
- full list of formats that are accepted. If not provided, a darker shade
- of the polygon color will be used instead.
- alpha (float): blending efficient. Smaller values lead to more transparent masks.
-
- Returns:
- output (VisImage): image object with polygon drawn.
- """
- if edge_color is None:
- # make edge color darker than the polygon color
- if alpha > 0.8:
- edge_color = self._change_color_brightness(color, brightness_factor=-0.7)
- else:
- edge_color = color
- edge_color = mplc.to_rgb(edge_color) + (1,)
-
- polygon = mpl.patches.Polygon(
- segment,
- fill=True,
- facecolor=mplc.to_rgb(color) + (alpha,),
- edgecolor=edge_color,
- linewidth=max(self._default_font_size // 15 * self.output.scale, 1),
- )
- self.output.ax.add_patch(polygon)
- return self.output
-
- """
- Internal methods:
- """
-
- def _jitter(self, color):
- """
- Randomly modifies given color to produce a slightly different color than the color given.
-
- Args:
- color (tuple[double]): a tuple of 3 elements, containing the RGB values of the color
- picked. The values in the list are in the [0.0, 1.0] range.
-
- Returns:
- jittered_color (tuple[double]): a tuple of 3 elements, containing the RGB values of the
- color after being jittered. The values in the list are in the [0.0, 1.0] range.
- """
- color = mplc.to_rgb(color)
- vec = np.random.rand(3)
- # better to do it in another color space
- vec = vec / np.linalg.norm(vec) * 0.5
- res = np.clip(vec + color, 0, 1)
- return tuple(res)
-
- def _create_grayscale_image(self, mask=None):
- """
- Create a grayscale version of the original image.
- The colors in masked area, if given, will be kept.
- """
- img_bw = self.img.astype("f4").mean(axis=2)
- img_bw = np.stack([img_bw] * 3, axis=2)
- if mask is not None:
- img_bw[mask] = self.img[mask]
- return img_bw
-
- def _change_color_brightness(self, color, brightness_factor):
- """
- Depending on the brightness_factor, gives a lighter or darker color i.e. a color with
- less or more saturation than the original color.
-
- Args:
- color: color of the polygon. Refer to `matplotlib.colors` for a full list of
- formats that are accepted.
- brightness_factor (float): a value in [-1.0, 1.0] range. A lightness factor of
- 0 will correspond to no change, a factor in [-1.0, 0) range will result in
- a darker color and a factor in (0, 1.0] range will result in a lighter color.
-
- Returns:
- modified_color (tuple[double]): a tuple containing the RGB values of the
- modified color. Each value in the tuple is in the [0.0, 1.0] range.
- """
- assert brightness_factor >= -1.0 and brightness_factor <= 1.0
- color = mplc.to_rgb(color)
- polygon_color = colorsys.rgb_to_hls(*mplc.to_rgb(color))
- modified_lightness = polygon_color[1] + (brightness_factor * polygon_color[1])
- modified_lightness = 0.0 if modified_lightness < 0.0 else modified_lightness
- modified_lightness = 1.0 if modified_lightness > 1.0 else modified_lightness
- modified_color = colorsys.hls_to_rgb(polygon_color[0], modified_lightness, polygon_color[2])
- return modified_color
-
- def _convert_boxes(self, boxes):
- """
- Convert different format of boxes to an NxB array, where B = 4 or 5 is the box dimension.
- """
- if isinstance(boxes, Boxes) or isinstance(boxes, RotatedBoxes):
- return boxes.tensor.detach().numpy()
- else:
- return np.asarray(boxes)
-
- def _convert_masks(self, masks_or_polygons):
- """
- Convert different format of masks or polygons to a tuple of masks and polygons.
-
- Returns:
- list[GenericMask]:
- """
-
- m = masks_or_polygons
- if isinstance(m, PolygonMasks):
- m = m.polygons
- if isinstance(m, BitMasks):
- m = m.tensor.numpy()
- if isinstance(m, torch.Tensor):
- m = m.numpy()
- ret = []
- for x in m:
- if isinstance(x, GenericMask):
- ret.append(x)
- else:
- ret.append(GenericMask(x, self.output.height, self.output.width))
- return ret
-
- def _draw_text_in_mask(self, binary_mask, text, color):
- """
- Find proper places to draw text given a binary mask.
- """
- # TODO sometimes drawn on wrong objects. the heuristics here can improve.
- _num_cc, cc_labels, stats, centroids = cv2.connectedComponentsWithStats(binary_mask, 8)
- if stats[1:, -1].size == 0:
- return
- largest_component_id = np.argmax(stats[1:, -1]) + 1
-
- # draw text on the largest component, as well as other very large components.
- for cid in range(1, _num_cc):
- if cid == largest_component_id or stats[cid, -1] > _LARGE_MASK_AREA_THRESH:
- # median is more stable than centroid
- # center = centroids[largest_component_id]
- center = np.median((cc_labels == cid).nonzero(), axis=1)[::-1]
- self.draw_text(text, center, color=color)
-
- def _convert_keypoints(self, keypoints):
- if isinstance(keypoints, Keypoints):
- keypoints = keypoints.tensor
- keypoints = np.asarray(keypoints)
- return keypoints
-
- def get_output(self):
- """
- Returns:
- output (VisImage): the image output containing the visualizations added
- to the image.
- """
- return self.output
diff --git a/spaces/Awiny/Image2Paragraph/models/grit_src/third_party/CenterNet2/projects/CenterNet2/centernet/modeling/layers/iou_loss.py b/spaces/Awiny/Image2Paragraph/models/grit_src/third_party/CenterNet2/projects/CenterNet2/centernet/modeling/layers/iou_loss.py
deleted file mode 100644
index 6a02464651dc1a0dcec9f30285a3a4ef74209f89..0000000000000000000000000000000000000000
--- a/spaces/Awiny/Image2Paragraph/models/grit_src/third_party/CenterNet2/projects/CenterNet2/centernet/modeling/layers/iou_loss.py
+++ /dev/null
@@ -1,121 +0,0 @@
-import torch
-from torch import nn
-
-
-class IOULoss(nn.Module):
- def __init__(self, loc_loss_type='iou'):
- super(IOULoss, self).__init__()
- self.loc_loss_type = loc_loss_type
-
- def forward(self, pred, target, weight=None, reduction='sum'):
- pred_left = pred[:, 0]
- pred_top = pred[:, 1]
- pred_right = pred[:, 2]
- pred_bottom = pred[:, 3]
-
- target_left = target[:, 0]
- target_top = target[:, 1]
- target_right = target[:, 2]
- target_bottom = target[:, 3]
-
- target_aera = (target_left + target_right) * \
- (target_top + target_bottom)
- pred_aera = (pred_left + pred_right) * \
- (pred_top + pred_bottom)
-
- w_intersect = torch.min(pred_left, target_left) + \
- torch.min(pred_right, target_right)
- h_intersect = torch.min(pred_bottom, target_bottom) + \
- torch.min(pred_top, target_top)
-
- g_w_intersect = torch.max(pred_left, target_left) + \
- torch.max(pred_right, target_right)
- g_h_intersect = torch.max(pred_bottom, target_bottom) + \
- torch.max(pred_top, target_top)
- ac_uion = g_w_intersect * g_h_intersect
-
- area_intersect = w_intersect * h_intersect
- area_union = target_aera + pred_aera - area_intersect
-
- ious = (area_intersect + 1.0) / (area_union + 1.0)
- gious = ious - (ac_uion - area_union) / ac_uion
- if self.loc_loss_type == 'iou':
- losses = -torch.log(ious)
- elif self.loc_loss_type == 'linear_iou':
- losses = 1 - ious
- elif self.loc_loss_type == 'giou':
- losses = 1 - gious
- else:
- raise NotImplementedError
-
- if weight is not None:
- losses = losses * weight
- else:
- losses = losses
-
- if reduction == 'sum':
- return losses.sum()
- elif reduction == 'batch':
- return losses.sum(dim=[1])
- elif reduction == 'none':
- return losses
- else:
- raise NotImplementedError
-
-
-def giou_loss(
- boxes1: torch.Tensor,
- boxes2: torch.Tensor,
- reduction: str = "none",
- eps: float = 1e-7,
-) -> torch.Tensor:
- """
- Generalized Intersection over Union Loss (Hamid Rezatofighi et. al)
- https://arxiv.org/abs/1902.09630
- Gradient-friendly IoU loss with an additional penalty that is non-zero when the
- boxes do not overlap and scales with the size of their smallest enclosing box.
- This loss is symmetric, so the boxes1 and boxes2 arguments are interchangeable.
- Args:
- boxes1, boxes2 (Tensor): box locations in XYXY format, shape (N, 4) or (4,).
- reduction: 'none' | 'mean' | 'sum'
- 'none': No reduction will be applied to the output.
- 'mean': The output will be averaged.
- 'sum': The output will be summed.
- eps (float): small number to prevent division by zero
- """
-
- x1, y1, x2, y2 = boxes1.unbind(dim=-1)
- x1g, y1g, x2g, y2g = boxes2.unbind(dim=-1)
-
- assert (x2 >= x1).all(), "bad box: x1 larger than x2"
- assert (y2 >= y1).all(), "bad box: y1 larger than y2"
-
- # Intersection keypoints
- xkis1 = torch.max(x1, x1g)
- ykis1 = torch.max(y1, y1g)
- xkis2 = torch.min(x2, x2g)
- ykis2 = torch.min(y2, y2g)
-
- intsctk = torch.zeros_like(x1)
- mask = (ykis2 > ykis1) & (xkis2 > xkis1)
- intsctk[mask] = (xkis2[mask] - xkis1[mask]) * (ykis2[mask] - ykis1[mask])
- unionk = (x2 - x1) * (y2 - y1) + (x2g - x1g) * (y2g - y1g) - intsctk
- iouk = intsctk / (unionk + eps)
-
- # smallest enclosing box
- xc1 = torch.min(x1, x1g)
- yc1 = torch.min(y1, y1g)
- xc2 = torch.max(x2, x2g)
- yc2 = torch.max(y2, y2g)
-
- area_c = (xc2 - xc1) * (yc2 - yc1)
- miouk = iouk - ((area_c - unionk) / (area_c + eps))
-
- loss = 1 - miouk
-
- if reduction == "mean":
- loss = loss.mean()
- elif reduction == "sum":
- loss = loss.sum()
-
- return loss
\ No newline at end of file
diff --git a/spaces/Bart92/RVC_HF/lib/uvr5_pack/lib_v5/nets_61968KB.py b/spaces/Bart92/RVC_HF/lib/uvr5_pack/lib_v5/nets_61968KB.py
deleted file mode 100644
index becbfae85683a13bbb19d3ea6c840da24e61e01e..0000000000000000000000000000000000000000
--- a/spaces/Bart92/RVC_HF/lib/uvr5_pack/lib_v5/nets_61968KB.py
+++ /dev/null
@@ -1,122 +0,0 @@
-import torch
-from torch import nn
-import torch.nn.functional as F
-
-from . import layers_123821KB as layers
-
-
-class BaseASPPNet(nn.Module):
- def __init__(self, nin, ch, dilations=(4, 8, 16)):
- super(BaseASPPNet, self).__init__()
- self.enc1 = layers.Encoder(nin, ch, 3, 2, 1)
- self.enc2 = layers.Encoder(ch, ch * 2, 3, 2, 1)
- self.enc3 = layers.Encoder(ch * 2, ch * 4, 3, 2, 1)
- self.enc4 = layers.Encoder(ch * 4, ch * 8, 3, 2, 1)
-
- self.aspp = layers.ASPPModule(ch * 8, ch * 16, dilations)
-
- self.dec4 = layers.Decoder(ch * (8 + 16), ch * 8, 3, 1, 1)
- self.dec3 = layers.Decoder(ch * (4 + 8), ch * 4, 3, 1, 1)
- self.dec2 = layers.Decoder(ch * (2 + 4), ch * 2, 3, 1, 1)
- self.dec1 = layers.Decoder(ch * (1 + 2), ch, 3, 1, 1)
-
- def __call__(self, x):
- h, e1 = self.enc1(x)
- h, e2 = self.enc2(h)
- h, e3 = self.enc3(h)
- h, e4 = self.enc4(h)
-
- h = self.aspp(h)
-
- h = self.dec4(h, e4)
- h = self.dec3(h, e3)
- h = self.dec2(h, e2)
- h = self.dec1(h, e1)
-
- return h
-
-
-class CascadedASPPNet(nn.Module):
- def __init__(self, n_fft):
- super(CascadedASPPNet, self).__init__()
- self.stg1_low_band_net = BaseASPPNet(2, 32)
- self.stg1_high_band_net = BaseASPPNet(2, 32)
-
- self.stg2_bridge = layers.Conv2DBNActiv(34, 16, 1, 1, 0)
- self.stg2_full_band_net = BaseASPPNet(16, 32)
-
- self.stg3_bridge = layers.Conv2DBNActiv(66, 32, 1, 1, 0)
- self.stg3_full_band_net = BaseASPPNet(32, 64)
-
- self.out = nn.Conv2d(64, 2, 1, bias=False)
- self.aux1_out = nn.Conv2d(32, 2, 1, bias=False)
- self.aux2_out = nn.Conv2d(32, 2, 1, bias=False)
-
- self.max_bin = n_fft // 2
- self.output_bin = n_fft // 2 + 1
-
- self.offset = 128
-
- def forward(self, x, aggressiveness=None):
- mix = x.detach()
- x = x.clone()
-
- x = x[:, :, : self.max_bin]
-
- bandw = x.size()[2] // 2
- aux1 = torch.cat(
- [
- self.stg1_low_band_net(x[:, :, :bandw]),
- self.stg1_high_band_net(x[:, :, bandw:]),
- ],
- dim=2,
- )
-
- h = torch.cat([x, aux1], dim=1)
- aux2 = self.stg2_full_band_net(self.stg2_bridge(h))
-
- h = torch.cat([x, aux1, aux2], dim=1)
- h = self.stg3_full_band_net(self.stg3_bridge(h))
-
- mask = torch.sigmoid(self.out(h))
- mask = F.pad(
- input=mask,
- pad=(0, 0, 0, self.output_bin - mask.size()[2]),
- mode="replicate",
- )
-
- if self.training:
- aux1 = torch.sigmoid(self.aux1_out(aux1))
- aux1 = F.pad(
- input=aux1,
- pad=(0, 0, 0, self.output_bin - aux1.size()[2]),
- mode="replicate",
- )
- aux2 = torch.sigmoid(self.aux2_out(aux2))
- aux2 = F.pad(
- input=aux2,
- pad=(0, 0, 0, self.output_bin - aux2.size()[2]),
- mode="replicate",
- )
- return mask * mix, aux1 * mix, aux2 * mix
- else:
- if aggressiveness:
- mask[:, :, : aggressiveness["split_bin"]] = torch.pow(
- mask[:, :, : aggressiveness["split_bin"]],
- 1 + aggressiveness["value"] / 3,
- )
- mask[:, :, aggressiveness["split_bin"] :] = torch.pow(
- mask[:, :, aggressiveness["split_bin"] :],
- 1 + aggressiveness["value"],
- )
-
- return mask * mix
-
- def predict(self, x_mag, aggressiveness=None):
- h = self.forward(x_mag, aggressiveness)
-
- if self.offset > 0:
- h = h[:, :, :, self.offset : -self.offset]
- assert h.size()[3] > 0
-
- return h
diff --git a/spaces/Benson/text-generation/Examples/Base-1.apk.md b/spaces/Benson/text-generation/Examples/Base-1.apk.md
deleted file mode 100644
index d1c9b418fe9f5c566f670498d4f6417b36d87e57..0000000000000000000000000000000000000000
--- a/spaces/Benson/text-generation/Examples/Base-1.apk.md
+++ /dev/null
@@ -1,53 +0,0 @@
-
-¿Qué es Base-1.apk y cómo usarlo?
-Si usted está buscando una manera práctica y conveniente para proteger y copia de seguridad de sus datos importantes en su dispositivo Android, es posible que desee comprobar Base-1.apk. Esta es una aplicación popular para Android que le permite administrar y restaurar fácilmente todos sus archivos en una ubicación, reduciendo la posibilidad de que pierda cualquier información valiosa. En este artículo, explicaremos qué es Base-1.apk, cómo descargarlo e instalarlo, y cómo usarlo de manera efectiva.
- Introducción
-Android es una plataforma versátil y abierta que te permite personalizar y modificar tu dispositivo según tus preferencias. Sin embargo, esto también significa que debe tener cuidado con la seguridad e integridad de sus datos, ya que hay muchas amenazas y riesgos potenciales que pueden comprometer o dañar sus archivos. Es por eso que es importante tener una solución de copia de seguridad confiable que pueda ayudarlo a proteger sus datos y restaurarlos en caso de cualquier emergencia.
-base-1.apk DOWNLOAD > https://bltlly.com/2v6LA6
- ¿Qué es un archivo APK?
-Un archivo APK es el formato de archivo de paquete que Android utiliza para distribuir e instalar aplicaciones. Contiene todos los elementos que una aplicación necesita para ejecutarse correctamente en su dispositivo, como código, recursos, activos, certificados y manifiesto. Un archivo APK es un archivo de archivo, lo que significa que contiene varios archivos, además de algunos metadatos sobre ellos. Puedes abrir un archivo APK con una herramienta de extracción de archivos como 7-Zip para ver lo que hay dentro.
- ¿Qué es Base-1.apk?
-Base-1.apk es la versión original, sin modificar de una aplicación Android llamada Base. Base es una herramienta que hace que sea simple y práctico para proteger y respaldar sus datos importantes. Al usar esta aplicación, puede administrar y restaurar fácilmente todos sus archivos en una ubicación, reduciendo la posibilidad de que pierda cualquier información valiosa. También puede organizar y clasificar sus archivos en carpetas para una mejor administración.
- ¿Cómo descargar e instalar Base-1.apk?
-
- Descargar desde el sitio web oficial
-La forma más segura y recomendada para descargar Base-1.apk es desde el sitio web oficial del desarrollador. Puede visitar baseapk.in y hacer clic en el enlace de descarga para obtener la última versión de la aplicación. Una vez que haya descargado el archivo APK, es necesario habilitar la instalación de aplicaciones de fuentes desconocidas en la configuración del dispositivo. Luego, puedes tocar en el archivo APK y seguir las instrucciones para instalarlo.
- Descargar de la tienda de aplicaciones de terceros
-Otra forma de descargar Base-1.apk es desde una tienda de aplicaciones de terceros, como Aptoide o APKPure. Estas son plataformas alternativas que ofrecen una variedad de aplicaciones que no están disponibles en Google Play. Sin embargo, debe tener cuidado al descargar aplicaciones de estas fuentes, ya que pueden contener malware u otro software dañino. Siempre debe comprobar las revisiones y calificaciones de las aplicaciones antes de descargarlas, y solo descargar de fuentes de confianza.
- Descargar desde enlace directo
-La última forma de descargar Base-1.apk es desde un enlace directo que alguien te proporciona. Esto podría ser un amigo, un colega, o un sitio web que ofrece descargas APK. Sin embargo, este es el método más arriesgado, ya que no tiene manera de verificar la autenticidad o la seguridad del archivo APK. Solo debe descargar archivos APK de enlaces directos si confía en la fuente por completo, y escanear el archivo con una aplicación antivirus antes de instalarlo.
- ¿Cómo usar Base-1.apk?
-Una vez que haya instalado Base-1
Una vez que haya instalado Base-1.apk en su dispositivo, puede comenzar a usarlo para proteger y respaldar sus datos. Estas son algunas de las principales características y funciones de la aplicación:
- Copia de seguridad segura de sus datos
-
- Administrar y restaurar sus archivos
-Base-1.apk también le permite administrar y restaurar sus archivos desde el servicio de almacenamiento en la nube. Puede ver, editar, eliminar o compartir sus archivos desde la interfaz de la aplicación. También puede restaurar sus archivos a su dispositivo u otro dispositivo en caso de cualquier emergencia. Puede seleccionar qué archivos y carpetas desea restaurar y elegir la carpeta de destino en su dispositivo. También puede restaurar sus archivos a su ubicación original o una nueva ubicación.
-
- Organiza y clasifica tus carpetas
-Otra característica útil de Base-1.apk es que te ayuda a organizar y clasificar tus carpetas según diferentes categorías, como fotos, videos, música, documentos, etc. También puedes crear carpetas y etiquetas personalizadas para tus archivos. De esta manera, puede encontrar y acceder fácilmente a sus archivos sin perder tiempo o espacio. También puede ordenar sus archivos por nombre, fecha, tamaño o tipo.
- Conclusión
-Base-1.apk es una aplicación Android potente y práctica que le ayuda a proteger y hacer copias de seguridad de sus datos importantes en su dispositivo. Al usar esta aplicación, puede administrar y restaurar fácilmente todos sus archivos en una ubicación, reduciendo la posibilidad de que pierda cualquier información valiosa. También puede organizar y clasificar sus archivos en carpetas para una mejor administración.
- Resumen de los puntos principales
-En este artículo, hemos explicado lo que es Base-1.apk, cómo descargarlo e instalarlo, y cómo usarlo de manera efectiva. Hemos cubierto los siguientes puntos:
-
- Un archivo APK es el formato de archivo de paquete que Android utiliza para distribuir e instalar aplicaciones.
-Base-1.apk es la versión original, sin modificar de una aplicación Android llamada Base.
- Base es una herramienta que hace que sea simple y práctico para proteger y respaldar sus datos importantes.
- Puede descargar Base-1.apk desde el sitio web oficial, una tienda de aplicaciones de terceros, o un enlace directo.
-
-
- Llamada a la acción
-Si estás interesado en probar Base-1.apk por ti mismo, puedes descargarlo desde baseapk.in y seguir las instrucciones de instalación. También puede consultar la sección de preguntas frecuentes a continuación para obtener más información sobre la aplicación. Esperamos que disfrute usando Base-1.apk y lo encuentre útil para proteger y hacer copias de seguridad de sus datos.
- Preguntas frecuentes
-Aquí están algunas de las preguntas más comunes que los usuarios tienen sobre Base-1.apk:
- Q: ¿Es seguro usar Base-1.apk?
-A: Sí, Base-1.apk es seguro de usar, siempre y cuando se descarga desde el sitio web oficial o una fuente de confianza. Sin embargo, siempre debes escanear cualquier archivo APK con una aplicación antivirus antes de instalarlo en tu dispositivo.
- Q: ¿Cuánto espacio ocupa Base-1.apk en mi dispositivo?
-A: Base-1.apk ocupa unos 15 MB de espacio en su dispositivo. Sin embargo, el tamaño real puede variar dependiendo de la versión de la aplicación y el modelo del dispositivo.
- Q: ¿Cuánto espacio de almacenamiento en la nube ofrece Base-1.apk?
-A: Base-1.apk no ofrece ningún espacio de almacenamiento en la nube por sí mismo. Utiliza el servicio de almacenamiento en la nube que elija para realizar copias de seguridad de sus datos, como Google Drive, Dropbox o OneDrive. La cantidad de espacio de almacenamiento en la nube que obtiene depende del proveedor de servicios y del plan que tenga.
- Q: ¿Puedo usar Base-1.apk en varios dispositivos?
-A: Sí, puedes usar Base-1.apk en varios dispositivos siempre y cuando ejecuten Android 4.0 o superior. Solo necesitas descargar e instalar la aplicación en
A: Sí, puedes usar Base-1.apk en varios dispositivos siempre y cuando ejecuten Android 4.0 o superior. Solo tienes que descargar e instalar la aplicación en cada dispositivo e iniciar sesión con la misma cuenta. A continuación, puede acceder y restaurar sus archivos desde cualquier dispositivo.
- Q: ¿Qué pasa si olvido mi contraseña para Base-1.apk? 64aa2da5cf
-
-
\ No newline at end of file
diff --git a/spaces/Big-Web/MMSD/env/Lib/site-packages/setuptools/_distutils/command/build_py.py b/spaces/Big-Web/MMSD/env/Lib/site-packages/setuptools/_distutils/command/build_py.py
deleted file mode 100644
index 47c6158e0f74033bfcfeb7424df227a3815651de..0000000000000000000000000000000000000000
--- a/spaces/Big-Web/MMSD/env/Lib/site-packages/setuptools/_distutils/command/build_py.py
+++ /dev/null
@@ -1,407 +0,0 @@
-"""distutils.command.build_py
-
-Implements the Distutils 'build_py' command."""
-
-import os
-import importlib.util
-import sys
-import glob
-
-from distutils.core import Command
-from distutils.errors import DistutilsOptionError, DistutilsFileError
-from distutils.util import convert_path
-from distutils import log
-
-
-class build_py(Command):
-
- description = "\"build\" pure Python modules (copy to build directory)"
-
- user_options = [
- ('build-lib=', 'd', "directory to \"build\" (copy) to"),
- ('compile', 'c', "compile .py to .pyc"),
- ('no-compile', None, "don't compile .py files [default]"),
- (
- 'optimize=',
- 'O',
- "also compile with optimization: -O1 for \"python -O\", "
- "-O2 for \"python -OO\", and -O0 to disable [default: -O0]",
- ),
- ('force', 'f', "forcibly build everything (ignore file timestamps)"),
- ]
-
- boolean_options = ['compile', 'force']
- negative_opt = {'no-compile': 'compile'}
-
- def initialize_options(self):
- self.build_lib = None
- self.py_modules = None
- self.package = None
- self.package_data = None
- self.package_dir = None
- self.compile = 0
- self.optimize = 0
- self.force = None
-
- def finalize_options(self):
- self.set_undefined_options(
- 'build', ('build_lib', 'build_lib'), ('force', 'force')
- )
-
- # Get the distribution options that are aliases for build_py
- # options -- list of packages and list of modules.
- self.packages = self.distribution.packages
- self.py_modules = self.distribution.py_modules
- self.package_data = self.distribution.package_data
- self.package_dir = {}
- if self.distribution.package_dir:
- for name, path in self.distribution.package_dir.items():
- self.package_dir[name] = convert_path(path)
- self.data_files = self.get_data_files()
-
- # Ick, copied straight from install_lib.py (fancy_getopt needs a
- # type system! Hell, *everything* needs a type system!!!)
- if not isinstance(self.optimize, int):
- try:
- self.optimize = int(self.optimize)
- assert 0 <= self.optimize <= 2
- except (ValueError, AssertionError):
- raise DistutilsOptionError("optimize must be 0, 1, or 2")
-
- def run(self):
- # XXX copy_file by default preserves atime and mtime. IMHO this is
- # the right thing to do, but perhaps it should be an option -- in
- # particular, a site administrator might want installed files to
- # reflect the time of installation rather than the last
- # modification time before the installed release.
-
- # XXX copy_file by default preserves mode, which appears to be the
- # wrong thing to do: if a file is read-only in the working
- # directory, we want it to be installed read/write so that the next
- # installation of the same module distribution can overwrite it
- # without problems. (This might be a Unix-specific issue.) Thus
- # we turn off 'preserve_mode' when copying to the build directory,
- # since the build directory is supposed to be exactly what the
- # installation will look like (ie. we preserve mode when
- # installing).
-
- # Two options control which modules will be installed: 'packages'
- # and 'py_modules'. The former lets us work with whole packages, not
- # specifying individual modules at all; the latter is for
- # specifying modules one-at-a-time.
-
- if self.py_modules:
- self.build_modules()
- if self.packages:
- self.build_packages()
- self.build_package_data()
-
- self.byte_compile(self.get_outputs(include_bytecode=0))
-
- def get_data_files(self):
- """Generate list of '(package,src_dir,build_dir,filenames)' tuples"""
- data = []
- if not self.packages:
- return data
- for package in self.packages:
- # Locate package source directory
- src_dir = self.get_package_dir(package)
-
- # Compute package build directory
- build_dir = os.path.join(*([self.build_lib] + package.split('.')))
-
- # Length of path to strip from found files
- plen = 0
- if src_dir:
- plen = len(src_dir) + 1
-
- # Strip directory from globbed filenames
- filenames = [file[plen:] for file in self.find_data_files(package, src_dir)]
- data.append((package, src_dir, build_dir, filenames))
- return data
-
- def find_data_files(self, package, src_dir):
- """Return filenames for package's data files in 'src_dir'"""
- globs = self.package_data.get('', []) + self.package_data.get(package, [])
- files = []
- for pattern in globs:
- # Each pattern has to be converted to a platform-specific path
- filelist = glob.glob(
- os.path.join(glob.escape(src_dir), convert_path(pattern))
- )
- # Files that match more than one pattern are only added once
- files.extend(
- [fn for fn in filelist if fn not in files and os.path.isfile(fn)]
- )
- return files
-
- def build_package_data(self):
- """Copy data files into build directory"""
- for package, src_dir, build_dir, filenames in self.data_files:
- for filename in filenames:
- target = os.path.join(build_dir, filename)
- self.mkpath(os.path.dirname(target))
- self.copy_file(
- os.path.join(src_dir, filename), target, preserve_mode=False
- )
-
- def get_package_dir(self, package):
- """Return the directory, relative to the top of the source
- distribution, where package 'package' should be found
- (at least according to the 'package_dir' option, if any)."""
- path = package.split('.')
-
- if not self.package_dir:
- if path:
- return os.path.join(*path)
- else:
- return ''
- else:
- tail = []
- while path:
- try:
- pdir = self.package_dir['.'.join(path)]
- except KeyError:
- tail.insert(0, path[-1])
- del path[-1]
- else:
- tail.insert(0, pdir)
- return os.path.join(*tail)
- else:
- # Oops, got all the way through 'path' without finding a
- # match in package_dir. If package_dir defines a directory
- # for the root (nameless) package, then fallback on it;
- # otherwise, we might as well have not consulted
- # package_dir at all, as we just use the directory implied
- # by 'tail' (which should be the same as the original value
- # of 'path' at this point).
- pdir = self.package_dir.get('')
- if pdir is not None:
- tail.insert(0, pdir)
-
- if tail:
- return os.path.join(*tail)
- else:
- return ''
-
- def check_package(self, package, package_dir):
- # Empty dir name means current directory, which we can probably
- # assume exists. Also, os.path.exists and isdir don't know about
- # my "empty string means current dir" convention, so we have to
- # circumvent them.
- if package_dir != "":
- if not os.path.exists(package_dir):
- raise DistutilsFileError(
- "package directory '%s' does not exist" % package_dir
- )
- if not os.path.isdir(package_dir):
- raise DistutilsFileError(
- "supposed package directory '%s' exists, "
- "but is not a directory" % package_dir
- )
-
- # Directories without __init__.py are namespace packages (PEP 420).
- if package:
- init_py = os.path.join(package_dir, "__init__.py")
- if os.path.isfile(init_py):
- return init_py
-
- # Either not in a package at all (__init__.py not expected), or
- # __init__.py doesn't exist -- so don't return the filename.
- return None
-
- def check_module(self, module, module_file):
- if not os.path.isfile(module_file):
- log.warn("file %s (for module %s) not found", module_file, module)
- return False
- else:
- return True
-
- def find_package_modules(self, package, package_dir):
- self.check_package(package, package_dir)
- module_files = glob.glob(os.path.join(glob.escape(package_dir), "*.py"))
- modules = []
- setup_script = os.path.abspath(self.distribution.script_name)
-
- for f in module_files:
- abs_f = os.path.abspath(f)
- if abs_f != setup_script:
- module = os.path.splitext(os.path.basename(f))[0]
- modules.append((package, module, f))
- else:
- self.debug_print("excluding %s" % setup_script)
- return modules
-
- def find_modules(self):
- """Finds individually-specified Python modules, ie. those listed by
- module name in 'self.py_modules'. Returns a list of tuples (package,
- module_base, filename): 'package' is a tuple of the path through
- package-space to the module; 'module_base' is the bare (no
- packages, no dots) module name, and 'filename' is the path to the
- ".py" file (relative to the distribution root) that implements the
- module.
- """
- # Map package names to tuples of useful info about the package:
- # (package_dir, checked)
- # package_dir - the directory where we'll find source files for
- # this package
- # checked - true if we have checked that the package directory
- # is valid (exists, contains __init__.py, ... ?)
- packages = {}
-
- # List of (package, module, filename) tuples to return
- modules = []
-
- # We treat modules-in-packages almost the same as toplevel modules,
- # just the "package" for a toplevel is empty (either an empty
- # string or empty list, depending on context). Differences:
- # - don't check for __init__.py in directory for empty package
- for module in self.py_modules:
- path = module.split('.')
- package = '.'.join(path[0:-1])
- module_base = path[-1]
-
- try:
- (package_dir, checked) = packages[package]
- except KeyError:
- package_dir = self.get_package_dir(package)
- checked = 0
-
- if not checked:
- init_py = self.check_package(package, package_dir)
- packages[package] = (package_dir, 1)
- if init_py:
- modules.append((package, "__init__", init_py))
-
- # XXX perhaps we should also check for just .pyc files
- # (so greedy closed-source bastards can distribute Python
- # modules too)
- module_file = os.path.join(package_dir, module_base + ".py")
- if not self.check_module(module, module_file):
- continue
-
- modules.append((package, module_base, module_file))
-
- return modules
-
- def find_all_modules(self):
- """Compute the list of all modules that will be built, whether
- they are specified one-module-at-a-time ('self.py_modules') or
- by whole packages ('self.packages'). Return a list of tuples
- (package, module, module_file), just like 'find_modules()' and
- 'find_package_modules()' do."""
- modules = []
- if self.py_modules:
- modules.extend(self.find_modules())
- if self.packages:
- for package in self.packages:
- package_dir = self.get_package_dir(package)
- m = self.find_package_modules(package, package_dir)
- modules.extend(m)
- return modules
-
- def get_source_files(self):
- return [module[-1] for module in self.find_all_modules()]
-
- def get_module_outfile(self, build_dir, package, module):
- outfile_path = [build_dir] + list(package) + [module + ".py"]
- return os.path.join(*outfile_path)
-
- def get_outputs(self, include_bytecode=1):
- modules = self.find_all_modules()
- outputs = []
- for (package, module, module_file) in modules:
- package = package.split('.')
- filename = self.get_module_outfile(self.build_lib, package, module)
- outputs.append(filename)
- if include_bytecode:
- if self.compile:
- outputs.append(
- importlib.util.cache_from_source(filename, optimization='')
- )
- if self.optimize > 0:
- outputs.append(
- importlib.util.cache_from_source(
- filename, optimization=self.optimize
- )
- )
-
- outputs += [
- os.path.join(build_dir, filename)
- for package, src_dir, build_dir, filenames in self.data_files
- for filename in filenames
- ]
-
- return outputs
-
- def build_module(self, module, module_file, package):
- if isinstance(package, str):
- package = package.split('.')
- elif not isinstance(package, (list, tuple)):
- raise TypeError(
- "'package' must be a string (dot-separated), list, or tuple"
- )
-
- # Now put the module source file into the "build" area -- this is
- # easy, we just copy it somewhere under self.build_lib (the build
- # directory for Python source).
- outfile = self.get_module_outfile(self.build_lib, package, module)
- dir = os.path.dirname(outfile)
- self.mkpath(dir)
- return self.copy_file(module_file, outfile, preserve_mode=0)
-
- def build_modules(self):
- modules = self.find_modules()
- for (package, module, module_file) in modules:
- # Now "build" the module -- ie. copy the source file to
- # self.build_lib (the build directory for Python source).
- # (Actually, it gets copied to the directory for this package
- # under self.build_lib.)
- self.build_module(module, module_file, package)
-
- def build_packages(self):
- for package in self.packages:
- # Get list of (package, module, module_file) tuples based on
- # scanning the package directory. 'package' is only included
- # in the tuple so that 'find_modules()' and
- # 'find_package_tuples()' have a consistent interface; it's
- # ignored here (apart from a sanity check). Also, 'module' is
- # the *unqualified* module name (ie. no dots, no package -- we
- # already know its package!), and 'module_file' is the path to
- # the .py file, relative to the current directory
- # (ie. including 'package_dir').
- package_dir = self.get_package_dir(package)
- modules = self.find_package_modules(package, package_dir)
-
- # Now loop over the modules we found, "building" each one (just
- # copy it to self.build_lib).
- for (package_, module, module_file) in modules:
- assert package == package_
- self.build_module(module, module_file, package)
-
- def byte_compile(self, files):
- if sys.dont_write_bytecode:
- self.warn('byte-compiling is disabled, skipping.')
- return
-
- from distutils.util import byte_compile
-
- prefix = self.build_lib
- if prefix[-1] != os.sep:
- prefix = prefix + os.sep
-
- # XXX this code is essentially the same as the 'byte_compile()
- # method of the "install_lib" command, except for the determination
- # of the 'prefix' string. Hmmm.
- if self.compile:
- byte_compile(
- files, optimize=0, force=self.force, prefix=prefix, dry_run=self.dry_run
- )
- if self.optimize > 0:
- byte_compile(
- files,
- optimize=self.optimize,
- force=self.force,
- prefix=prefix,
- dry_run=self.dry_run,
- )
diff --git a/spaces/Big-Web/MMSD/env/Lib/site-packages/urllib3/util/queue.py b/spaces/Big-Web/MMSD/env/Lib/site-packages/urllib3/util/queue.py
deleted file mode 100644
index 41784104ee4bd5796006d1052536325d52db1e8c..0000000000000000000000000000000000000000
--- a/spaces/Big-Web/MMSD/env/Lib/site-packages/urllib3/util/queue.py
+++ /dev/null
@@ -1,22 +0,0 @@
-import collections
-
-from ..packages import six
-from ..packages.six.moves import queue
-
-if six.PY2:
- # Queue is imported for side effects on MS Windows. See issue #229.
- import Queue as _unused_module_Queue # noqa: F401
-
-
-class LifoQueue(queue.Queue):
- def _init(self, _):
- self.queue = collections.deque()
-
- def _qsize(self, len=len):
- return len(self.queue)
-
- def _put(self, item):
- self.queue.append(item)
-
- def _get(self):
- return self.queue.pop()
diff --git a/spaces/CVPR/Dual-Key_Backdoor_Attacks/datagen/detectron2/detectron2/layers/csrc/ROIAlignRotated/ROIAlignRotated.h b/spaces/CVPR/Dual-Key_Backdoor_Attacks/datagen/detectron2/detectron2/layers/csrc/ROIAlignRotated/ROIAlignRotated.h
deleted file mode 100644
index dfc17b68e595c84a191c6979751cf11af6d879fd..0000000000000000000000000000000000000000
--- a/spaces/CVPR/Dual-Key_Backdoor_Attacks/datagen/detectron2/detectron2/layers/csrc/ROIAlignRotated/ROIAlignRotated.h
+++ /dev/null
@@ -1,115 +0,0 @@
-// Copyright (c) Facebook, Inc. and its affiliates. All Rights Reserved
-#pragma once
-#include
-
-namespace detectron2 {
-
-at::Tensor ROIAlignRotated_forward_cpu(
- const at::Tensor& input,
- const at::Tensor& rois,
- const float spatial_scale,
- const int pooled_height,
- const int pooled_width,
- const int sampling_ratio);
-
-at::Tensor ROIAlignRotated_backward_cpu(
- const at::Tensor& grad,
- const at::Tensor& rois,
- const float spatial_scale,
- const int pooled_height,
- const int pooled_width,
- const int batch_size,
- const int channels,
- const int height,
- const int width,
- const int sampling_ratio);
-
-#ifdef WITH_CUDA
-at::Tensor ROIAlignRotated_forward_cuda(
- const at::Tensor& input,
- const at::Tensor& rois,
- const float spatial_scale,
- const int pooled_height,
- const int pooled_width,
- const int sampling_ratio);
-
-at::Tensor ROIAlignRotated_backward_cuda(
- const at::Tensor& grad,
- const at::Tensor& rois,
- const float spatial_scale,
- const int pooled_height,
- const int pooled_width,
- const int batch_size,
- const int channels,
- const int height,
- const int width,
- const int sampling_ratio);
-#endif
-
-// Interface for Python
-inline at::Tensor ROIAlignRotated_forward(
- const at::Tensor& input,
- const at::Tensor& rois,
- const float spatial_scale,
- const int pooled_height,
- const int pooled_width,
- const int sampling_ratio) {
- if (input.type().is_cuda()) {
-#ifdef WITH_CUDA
- return ROIAlignRotated_forward_cuda(
- input,
- rois,
- spatial_scale,
- pooled_height,
- pooled_width,
- sampling_ratio);
-#else
- AT_ERROR("Not compiled with GPU support");
-#endif
- }
- return ROIAlignRotated_forward_cpu(
- input, rois, spatial_scale, pooled_height, pooled_width, sampling_ratio);
-}
-
-inline at::Tensor ROIAlignRotated_backward(
- const at::Tensor& grad,
- const at::Tensor& rois,
- const float spatial_scale,
- const int pooled_height,
- const int pooled_width,
- const int batch_size,
- const int channels,
- const int height,
- const int width,
- const int sampling_ratio) {
- if (grad.type().is_cuda()) {
-#ifdef WITH_CUDA
- return ROIAlignRotated_backward_cuda(
- grad,
- rois,
- spatial_scale,
- pooled_height,
- pooled_width,
- batch_size,
- channels,
- height,
- width,
- sampling_ratio);
-#else
- AT_ERROR("Not compiled with GPU support");
-#endif
- }
- return ROIAlignRotated_backward_cpu(
- grad,
- rois,
- spatial_scale,
- pooled_height,
- pooled_width,
- batch_size,
- channels,
- height,
- width,
- sampling_ratio);
-}
-
-} // namespace detectron2
diff --git a/spaces/CVPR/LIVE/thrust/thrust/device_new.h b/spaces/CVPR/LIVE/thrust/thrust/device_new.h
deleted file mode 100644
index 1ae4ce5a40d03b88073dd029d9a7049dcdab6783..0000000000000000000000000000000000000000
--- a/spaces/CVPR/LIVE/thrust/thrust/device_new.h
+++ /dev/null
@@ -1,88 +0,0 @@
-/*
- * Copyright 2008-2013 NVIDIA Corporation
- *
- * Licensed under the Apache License, Version 2.0 (the "License");
- * you may not use this file except in compliance with the License.
- * You may obtain a copy of the License at
- *
- * http://www.apache.org/licenses/LICENSE-2.0
- *
- * Unless required by applicable law or agreed to in writing, software
- * distributed under the License is distributed on an "AS IS" BASIS,
- * WITHOUT WARRANTIES OR CONDITIONS OF ANY KIND, either express or implied.
- * See the License for the specific language governing permissions and
- * limitations under the License.
- */
-
-
-/*! \file device_new.h
- * \brief Constructs new elements in device memory
- */
-
-#pragma once
-
-#include
-
-// #include this for size_t
-#include
-#include
-
-namespace thrust
-{
-
-/*!
- * \addtogroup allocation_functions Allocation Functions
- * \{
- */
-
-/*! \p device_new implements the placement \c new operator for types
- * resident in device memory. \p device_new calls T 's null
- * constructor on a array of objects in device memory.
- * No memory is allocated by this function.
- *
- * \param p A \p device_ptr to a region of device memory into which
- * to construct one or many T s.
- * \param n The number of objects to construct at \p p.
- * \return p, casted to T 's type.
- *
- * \see device_ptr
- */
-template
- device_ptr device_new(device_ptr p,
- const size_t n = 1);
-
-/*! \p device_new implements the placement new operator for types
- * resident in device memory. \p device_new calls T 's copy
- * constructor on a array of objects in device memory. No memory is
- * allocated by this function.
- *
- * \param p A \p device_ptr to a region of device memory into which to
- * construct one or many T s.
- * \param exemplar The value from which to copy.
- * \param n The number of objects to construct at \p p.
- * \return p, casted to T 's type.
- *
- * \see device_ptr
- * \see fill
- */
-template
- device_ptr device_new(device_ptr p,
- const T &exemplar,
- const size_t n = 1);
-
-/*! \p device_new implements the new operator for types resident in device memory.
- * It allocates device memory large enough to hold \p n new objects of type \c T.
- *
- * \param n The number of objects to allocate. Defaults to \c 1.
- * \return A \p device_ptr to the newly allocated region of device memory.
- */
-template
- device_ptr device_new(const size_t n = 1);
-
-/*! \}
- */
-
-} // end thrust
-
-#include
-
diff --git a/spaces/CVPR/LIVE/thrust/thrust/system/cuda/detail/par_to_seq.h b/spaces/CVPR/LIVE/thrust/thrust/system/cuda/detail/par_to_seq.h
deleted file mode 100644
index 22c4e58386e8c6dd0832bf3820072fadc53d34e8..0000000000000000000000000000000000000000
--- a/spaces/CVPR/LIVE/thrust/thrust/system/cuda/detail/par_to_seq.h
+++ /dev/null
@@ -1,91 +0,0 @@
-/******************************************************************************
- * Copyright (c) 2016, NVIDIA CORPORATION. All rights reserved.
- *
- * Redistribution and use in source and binary forms, with or without
- * modification, are permitted provided that the following conditions are met:
- * * Redistributions of source code must retain the above copyright
- * notice, this list of conditions and the following disclaimer.
- * * Redistributions in binary form must reproduce the above copyright
- * notice, this list of conditions and the following disclaimer in the
- * documentation and/or other materials provided with the distribution.
- * * Neither the name of the NVIDIA CORPORATION nor the
- * names of its contributors may be used to endorse or promote products
- * derived from this software without specific prior written permission.
- *
- * THIS SOFTWARE IS PROVIDED BY THE COPYRIGHT HOLDERS AND CONTRIBUTORS "AS IS"
- * AND ANY EXPRESS OR IMPLIED WARRANTIES, INCLUDING, BUT NOT LIMITED TO, THE
- * IMPLIED WARRANTIES OF MERCHANTABILITY AND FITNESS FOR A PARTICULAR PURPOSE
- * ARE DISCLAIMED. IN NO EVENT SHALL NVIDIA CORPORATION BE LIABLE FOR ANY
- * DIRECT, INDIRECT, INCIDENTAL, SPECIAL, EXEMPLARY, OR CONSEQUENTIAL DAMAGES
- * (INCLUDING, BUT NOT LIMITED TO, PROCUREMENT OF SUBSTITUTE GOODS OR SERVICES;
- * LOSS OF USE, DATA, OR PROFITS; OR BUSINESS INTERRUPTION) HOWEVER CAUSED AND
- * ON ANY THEORY OF LIABILITY, WHETHER IN CONTRACT, STRICT LIABILITY, OR TORT
- * (INCLUDING NEGLIGENCE OR OTHERWISE) ARISING IN ANY WAY OUT OF THE USE OF THIS
- * SOFTWARE, EVEN IF ADVISED OF THE POSSIBILITY OF SUCH DAMAGE.
- *
- ******************************************************************************/
-#pragma once
-
-#include
-#include
-
-namespace thrust
-{
-namespace cuda_cub {
-
-template
-struct has_par : thrust::detail::true_type {};
-
-template <>
-struct has_par<0> : thrust::detail::false_type {};
-
-template
-struct cvt_to_seq_impl
-{
- typedef thrust::detail::seq_t seq_t;
-
- static seq_t __host__ __device__
- doit(Policy&)
- {
- return seq_t();
- }
-}; // cvt_to_seq_impl
-
-#if 0
-template
-struct cvt_to_seq_impl<
- thrust::detail::execute_with_allocator >
-{
- typedef thrust::detail::execute_with_allocator
- Policy;
- typedef thrust::detail::execute_with_allocator<
- Allocator,
- thrust::system::detail::sequential::execution_policy>
- seq_t;
-
-
- static seq_t __host__ __device__
- doit(Policy& policy)
- {
- return seq_t(policy.m_alloc);
- }
-}; // specialization of struct cvt_to_seq_impl
-#endif
-
-template
-typename cvt_to_seq_impl::seq_t __host__ __device__
-cvt_to_seq(Policy& policy)
-{
- return cvt_to_seq_impl::doit(policy);
-}
-
-#if __THRUST_HAS_CUDART__
-#define THRUST_CUDART_DISPATCH par
-#else
-#define THRUST_CUDART_DISPATCH seq
-#endif
-
-} // namespace cuda_
-} // end namespace thrust
diff --git a/spaces/CVPR/LIVE/thrust/thrust/uninitialized_fill.h b/spaces/CVPR/LIVE/thrust/thrust/uninitialized_fill.h
deleted file mode 100644
index 33dc24886c30586a1302ec31f51a9f2dfca9b051..0000000000000000000000000000000000000000
--- a/spaces/CVPR/LIVE/thrust/thrust/uninitialized_fill.h
+++ /dev/null
@@ -1,275 +0,0 @@
-/*
- * Copyright 2008-2013 NVIDIA Corporation
- *
- * Licensed under the Apache License, Version 2.0 (the "License");
- * you may not use this file except in compliance with the License.
- * You may obtain a copy of the License at
- *
- * http://www.apache.org/licenses/LICENSE-2.0
- *
- * Unless required by applicable law or agreed to in writing, software
- * distributed under the License is distributed on an "AS IS" BASIS,
- * WITHOUT WARRANTIES OR CONDITIONS OF ANY KIND, either express or implied.
- * See the License for the specific language governing permissions and
- * limitations under the License.
- */
-
-
-/*! \file uninitialized_fill.h
- * \brief Copy construction into a range of uninitialized elements from a source value
- */
-
-#pragma once
-
-#include
-#include
-
-namespace thrust
-{
-
-
-/*! \addtogroup filling
- * \ingroup transformations
- * \{
- */
-
-
-/*! In \c thrust, the function \c thrust::device_new allocates memory for
- * an object and then creates an object at that location by calling a
- * constructor. Occasionally, however, it is useful to separate those two
- * operations. If each iterator in the range [first, last) points
- * to uninitialized memory, then \p uninitialized_fill creates copies of \c x
- * in that range. That is, for each iterator \c i in the range [first, last) ,
- * \p uninitialized_fill creates a copy of \c x in the location pointed to \c i by
- * calling \p ForwardIterator's \c value_type's copy constructor.
- *
- * The algorithm's execution is parallelized as determined by \p exec.
- *
- * \param exec The execution policy to use for parallelization.
- * \param first The first element of the range of interest.
- * \param last The last element of the range of interest.
- * \param x The value to use as the exemplar of the copy constructor.
- *
- * \tparam DerivedPolicy The name of the derived execution policy.
- * \tparam ForwardIterator is a model of Forward Iterator ,
- * \p ForwardIterator is mutable, and \p ForwardIterator's \c value_type has a constructor that
- * takes a single argument of type \p T.
- *
- * The following code snippet demonstrates how to use \p uninitialized_fill to initialize a range of
- * uninitialized memory using the \p thrust::device execution policy for parallelization:
- *
- * \code
- * #include
- * #include
- * #include
- *
- * struct Int
- * {
- * __host__ __device__
- * Int(int x) : val(x) {}
- * int val;
- * };
- * ...
- * const int N = 137;
- *
- * Int val(46);
- * thrust::device_ptr array = thrust::device_malloc(N);
- * thrust::uninitialized_fill(thrust::device, array, array + N, val);
- *
- * // Int x = array[i];
- * // x.val == 46 for all 0 <= i < N
- * \endcode
- *
- * \see http://www.sgi.com/tech/stl/uninitialized_fill.html
- * \see \c uninitialized_fill_n
- * \see \c fill
- * \see \c uninitialized_copy
- * \see \c device_new
- * \see \c device_malloc
- */
-template
-__host__ __device__
- void uninitialized_fill(const thrust::detail::execution_policy_base &exec,
- ForwardIterator first,
- ForwardIterator last,
- const T &x);
-
-
-/*! In \c thrust, the function \c thrust::device_new allocates memory for
- * an object and then creates an object at that location by calling a
- * constructor. Occasionally, however, it is useful to separate those two
- * operations. If each iterator in the range [first, last) points
- * to uninitialized memory, then \p uninitialized_fill creates copies of \c x
- * in that range. That is, for each iterator \c i in the range [first, last) ,
- * \p uninitialized_fill creates a copy of \c x in the location pointed to \c i by
- * calling \p ForwardIterator's \c value_type's copy constructor.
- *
- * \param first The first element of the range of interest.
- * \param last The last element of the range of interest.
- * \param x The value to use as the exemplar of the copy constructor.
- *
- * \tparam ForwardIterator is a model of Forward Iterator ,
- * \p ForwardIterator is mutable, and \p ForwardIterator's \c value_type has a constructor that
- * takes a single argument of type \p T.
- *
- * The following code snippet demonstrates how to use \p uninitialized_fill to initialize a range of
- * uninitialized memory.
- *
- * \code
- * #include
- * #include
- *
- * struct Int
- * {
- * __host__ __device__
- * Int(int x) : val(x) {}
- * int val;
- * };
- * ...
- * const int N = 137;
- *
- * Int val(46);
- * thrust::device_ptr array = thrust::device_malloc(N);
- * thrust::uninitialized_fill(array, array + N, val);
- *
- * // Int x = array[i];
- * // x.val == 46 for all 0 <= i < N
- * \endcode
- *
- * \see http://www.sgi.com/tech/stl/uninitialized_fill.html
- * \see \c uninitialized_fill_n
- * \see \c fill
- * \see \c uninitialized_copy
- * \see \c device_new
- * \see \c device_malloc
- */
-template
- void uninitialized_fill(ForwardIterator first,
- ForwardIterator last,
- const T &x);
-
-
-/*! In \c thrust, the function \c thrust::device_new allocates memory for
- * an object and then creates an object at that location by calling a
- * constructor. Occasionally, however, it is useful to separate those two
- * operations. If each iterator in the range [first, first+n) points
- * to uninitialized memory, then \p uninitialized_fill creates copies of \c x
- * in that range. That is, for each iterator \c i in the range [first, first+n) ,
- * \p uninitialized_fill creates a copy of \c x in the location pointed to \c i by
- * calling \p ForwardIterator's \c value_type's copy constructor.
- *
- * The algorithm's execution is parallelized as determined by \p exec.
- *
- * \param exec The execution policy to use for parallelization.
- * \param first The first element of the range of interest.
- * \param n The size of the range of interest.
- * \param x The value to use as the exemplar of the copy constructor.
- * \return first+n
- *
- * \tparam DerivedPolicy The name of the derived execution policy.
- * \tparam ForwardIterator is a model of Forward Iterator ,
- * \p ForwardIterator is mutable, and \p ForwardIterator's \c value_type has a constructor that
- * takes a single argument of type \p T.
- *
- * The following code snippet demonstrates how to use \p uninitialized_fill to initialize a range of
- * uninitialized memory using the \p thrust::device execution policy for parallelization:
- *
- * \code
- * #include
- * #include
- * #include
- *
- * struct Int
- * {
- * __host__ __device__
- * Int(int x) : val(x) {}
- * int val;
- * };
- * ...
- * const int N = 137;
- *
- * Int val(46);
- * thrust::device_ptr array = thrust::device_malloc(N);
- * thrust::uninitialized_fill_n(thrust::device, array, N, val);
- *
- * // Int x = array[i];
- * // x.val == 46 for all 0 <= i < N
- * \endcode
- *
- * \see http://www.sgi.com/tech/stl/uninitialized_fill.html
- * \see \c uninitialized_fill
- * \see \c fill
- * \see \c uninitialized_copy_n
- * \see \c device_new
- * \see \c device_malloc
- */
-template
-__host__ __device__
- ForwardIterator uninitialized_fill_n(const thrust::detail::execution_policy_base &exec,
- ForwardIterator first,
- Size n,
- const T &x);
-
-
-/*! In \c thrust, the function \c thrust::device_new allocates memory for
- * an object and then creates an object at that location by calling a
- * constructor. Occasionally, however, it is useful to separate those two
- * operations. If each iterator in the range [first, first+n) points
- * to uninitialized memory, then \p uninitialized_fill creates copies of \c x
- * in that range. That is, for each iterator \c i in the range [first, first+n) ,
- * \p uninitialized_fill creates a copy of \c x in the location pointed to \c i by
- * calling \p ForwardIterator's \c value_type's copy constructor.
- *
- * \param first The first element of the range of interest.
- * \param n The size of the range of interest.
- * \param x The value to use as the exemplar of the copy constructor.
- * \return first+n
- *
- * \tparam ForwardIterator is a model of Forward Iterator ,
- * \p ForwardIterator is mutable, and \p ForwardIterator's \c value_type has a constructor that
- * takes a single argument of type \p T.
- *
- * The following code snippet demonstrates how to use \p uninitialized_fill to initialize a range of
- * uninitialized memory.
- *
- * \code
- * #include
- * #include
- *
- * struct Int
- * {
- * __host__ __device__
- * Int(int x) : val(x) {}
- * int val;
- * };
- * ...
- * const int N = 137;
- *
- * Int val(46);
- * thrust::device_ptr array = thrust::device_malloc(N);
- * thrust::uninitialized_fill_n(array, N, val);
- *
- * // Int x = array[i];
- * // x.val == 46 for all 0 <= i < N
- * \endcode
- *
- * \see http://www.sgi.com/tech/stl/uninitialized_fill.html
- * \see \c uninitialized_fill
- * \see \c fill
- * \see \c uninitialized_copy_n
- * \see \c device_new
- * \see \c device_malloc
- */
-template
- ForwardIterator uninitialized_fill_n(ForwardIterator first,
- Size n,
- const T &x);
-
-/*! \} // end filling
- * \} // transformations
- */
-
-} // end thrust
-
-#include
-
diff --git a/spaces/CVPR/WALT/mmdet/core/bbox/samplers/instance_balanced_pos_sampler.py b/spaces/CVPR/WALT/mmdet/core/bbox/samplers/instance_balanced_pos_sampler.py
deleted file mode 100644
index c735298487e14e4a0ec42913f25673cccb98a8a0..0000000000000000000000000000000000000000
--- a/spaces/CVPR/WALT/mmdet/core/bbox/samplers/instance_balanced_pos_sampler.py
+++ /dev/null
@@ -1,55 +0,0 @@
-import numpy as np
-import torch
-
-from ..builder import BBOX_SAMPLERS
-from .random_sampler import RandomSampler
-
-
-@BBOX_SAMPLERS.register_module()
-class InstanceBalancedPosSampler(RandomSampler):
- """Instance balanced sampler that samples equal number of positive samples
- for each instance."""
-
- def _sample_pos(self, assign_result, num_expected, **kwargs):
- """Sample positive boxes.
-
- Args:
- assign_result (:obj:`AssignResult`): The assigned results of boxes.
- num_expected (int): The number of expected positive samples
-
- Returns:
- Tensor or ndarray: sampled indices.
- """
- pos_inds = torch.nonzero(assign_result.gt_inds > 0, as_tuple=False)
- if pos_inds.numel() != 0:
- pos_inds = pos_inds.squeeze(1)
- if pos_inds.numel() <= num_expected:
- return pos_inds
- else:
- unique_gt_inds = assign_result.gt_inds[pos_inds].unique()
- num_gts = len(unique_gt_inds)
- num_per_gt = int(round(num_expected / float(num_gts)) + 1)
- sampled_inds = []
- for i in unique_gt_inds:
- inds = torch.nonzero(
- assign_result.gt_inds == i.item(), as_tuple=False)
- if inds.numel() != 0:
- inds = inds.squeeze(1)
- else:
- continue
- if len(inds) > num_per_gt:
- inds = self.random_choice(inds, num_per_gt)
- sampled_inds.append(inds)
- sampled_inds = torch.cat(sampled_inds)
- if len(sampled_inds) < num_expected:
- num_extra = num_expected - len(sampled_inds)
- extra_inds = np.array(
- list(set(pos_inds.cpu()) - set(sampled_inds.cpu())))
- if len(extra_inds) > num_extra:
- extra_inds = self.random_choice(extra_inds, num_extra)
- extra_inds = torch.from_numpy(extra_inds).to(
- assign_result.gt_inds.device).long()
- sampled_inds = torch.cat([sampled_inds, extra_inds])
- elif len(sampled_inds) > num_expected:
- sampled_inds = self.random_choice(sampled_inds, num_expected)
- return sampled_inds
diff --git a/spaces/CVPR/WALT/train.py b/spaces/CVPR/WALT/train.py
deleted file mode 100644
index f0f11191f08da30857ae17d6c498c746b1d184f5..0000000000000000000000000000000000000000
--- a/spaces/CVPR/WALT/train.py
+++ /dev/null
@@ -1,191 +0,0 @@
-import argparse
-import copy
-import os
-import os.path as osp
-import time
-import warnings
-
-import mmcv
-import torch
-from mmcv import Config, DictAction
-from mmcv.runner import get_dist_info, init_dist
-from mmcv.utils import get_git_hash
-
-from mmdet import __version__
-from mmdet.apis import set_random_seed
-from mmdet.models import build_detector
-from mmdet.utils import collect_env, get_root_logger
-from walt.apis import train_detector
-from walt.datasets import build_dataset
-
-
-def parse_args():
- parser = argparse.ArgumentParser(description='Train a detector')
- parser.add_argument('config', help='train config file path')
- parser.add_argument('--work-dir', help='the dir to save logs and models')
- parser.add_argument(
- '--resume-from', help='the checkpoint file to resume from')
- parser.add_argument(
- '--no-validate',
- action='store_true',
- help='whether not to evaluate the checkpoint during training')
- group_gpus = parser.add_mutually_exclusive_group()
- group_gpus.add_argument(
- '--gpus',
- type=int,
- help='number of gpus to use '
- '(only applicable to non-distributed training)')
- group_gpus.add_argument(
- '--gpu-ids',
- type=int,
- nargs='+',
- help='ids of gpus to use '
- '(only applicable to non-distributed training)')
- parser.add_argument('--seed', type=int, default=None, help='random seed')
- parser.add_argument(
- '--deterministic',
- action='store_true',
- help='whether to set deterministic options for CUDNN backend.')
- parser.add_argument(
- '--options',
- nargs='+',
- action=DictAction,
- help='override some settings in the used config, the key-value pair '
- 'in xxx=yyy format will be merged into config file (deprecate), '
- 'change to --cfg-options instead.')
- parser.add_argument(
- '--cfg-options',
- nargs='+',
- action=DictAction,
- help='override some settings in the used config, the key-value pair '
- 'in xxx=yyy format will be merged into config file. If the value to '
- 'be overwritten is a list, it should be like key="[a,b]" or key=a,b '
- 'It also allows nested list/tuple values, e.g. key="[(a,b),(c,d)]" '
- 'Note that the quotation marks are necessary and that no white space '
- 'is allowed.')
- parser.add_argument(
- '--launcher',
- choices=['none', 'pytorch', 'slurm', 'mpi'],
- default='none',
- help='job launcher')
- parser.add_argument('--local_rank', type=int, default=0)
- args = parser.parse_args()
- if 'LOCAL_RANK' not in os.environ:
- os.environ['LOCAL_RANK'] = str(args.local_rank)
-
- if args.options and args.cfg_options:
- raise ValueError(
- '--options and --cfg-options cannot be both '
- 'specified, --options is deprecated in favor of --cfg-options')
- if args.options:
- warnings.warn('--options is deprecated in favor of --cfg-options')
- args.cfg_options = args.options
-
- return args
-
-
-def main():
- args = parse_args()
-
- cfg = Config.fromfile(args.config)
- if args.cfg_options is not None:
- cfg.merge_from_dict(args.cfg_options)
- # import modules from string list.
- if cfg.get('custom_imports', None):
- from mmcv.utils import import_modules_from_strings
- import_modules_from_strings(**cfg['custom_imports'])
- # set cudnn_benchmark
- if cfg.get('cudnn_benchmark', False):
- torch.backends.cudnn.benchmark = True
-
- # work_dir is determined in this priority: CLI > segment in file > filename
- if args.work_dir is not None:
- # update configs according to CLI args if args.work_dir is not None
- cfg.work_dir = args.work_dir
- elif cfg.get('work_dir', None) is None:
- # use config filename as default work_dir if cfg.work_dir is None
- cfg.work_dir = osp.join('./work_dirs',
- osp.splitext(osp.basename(args.config))[0])
-
- if args.resume_from is not None:
- cfg.resume_from = args.resume_from
- if args.gpu_ids is not None:
- cfg.gpu_ids = args.gpu_ids
- else:
- cfg.gpu_ids = range(1) if args.gpus is None else range(args.gpus)
-
- # init distributed env first, since logger depends on the dist info.
- if args.launcher == 'none':
- distributed = False
- else:
- distributed = True
- init_dist(args.launcher, **cfg.dist_params)
- # re-set gpu_ids with distributed training mode
- _, world_size = get_dist_info()
- cfg.gpu_ids = range(world_size)
-
-
- # create work_dir
- mmcv.mkdir_or_exist(osp.abspath(cfg.work_dir))
- # dump config
- cfg.dump(osp.join(cfg.work_dir, osp.basename(args.config)))
- # init the logger before other steps
- timestamp = time.strftime('%Y%m%d_%H%M%S', time.localtime())
- log_file = osp.join(cfg.work_dir, f'{timestamp}.log')
- logger = get_root_logger(log_file=log_file, log_level=cfg.log_level)
-
- # init the meta dict to record some important information such as
- # environment info and seed, which will be logged
- meta = dict()
- # log env info
- env_info_dict = collect_env()
- env_info = '\n'.join([(f'{k}: {v}') for k, v in env_info_dict.items()])
- dash_line = '-' * 60 + '\n'
- logger.info('Environment info:\n' + dash_line + env_info + '\n' +
- dash_line)
- meta['env_info'] = env_info
- meta['config'] = cfg.pretty_text
- # log some basic info
- logger.info(f'Distributed training: {distributed}')
- logger.info(f'Config:\n{cfg.pretty_text}')
-
- # set random seeds
- if args.seed is not None:
- logger.info(f'Set random seed to {args.seed}, '
- f'deterministic: {args.deterministic}')
- set_random_seed(args.seed, deterministic=args.deterministic)
- cfg.seed = args.seed
- meta['seed'] = args.seed
- meta['exp_name'] = osp.basename(args.config)
-
- model = build_detector(
- cfg.model,
- train_cfg=cfg.get('train_cfg'),
- test_cfg=cfg.get('test_cfg'))
-
- datasets = [build_dataset(cfg.data.train)]
- if len(cfg.workflow) == 2:
- val_dataset = copy.deepcopy(cfg.data.val)
- val_dataset.pipeline = cfg.data.train.pipeline
- datasets.append(build_dataset(val_dataset))
- if cfg.checkpoint_config is not None:
- # save mmdet version, config file content and class names in
- # checkpoints as meta data
- cfg.checkpoint_config.meta = dict(
- mmdet_version=__version__ + get_git_hash()[:7],
- CLASSES=datasets[0].CLASSES)
-
- # add an attribute for visualization convenience
- model.CLASSES = datasets[0].CLASSES
- train_detector(
- model,
- datasets,
- cfg,
- distributed=distributed,
- validate=(not args.no_validate),
- timestamp=timestamp,
- meta=meta)
-
-
-if __name__ == '__main__':
- main()
diff --git a/spaces/CVPR/regionclip-demo/detectron2/layers/csrc/box_iou_rotated/box_iou_rotated.h b/spaces/CVPR/regionclip-demo/detectron2/layers/csrc/box_iou_rotated/box_iou_rotated.h
deleted file mode 100644
index b65888b1be11881a776827b5212f08b8f63138f9..0000000000000000000000000000000000000000
--- a/spaces/CVPR/regionclip-demo/detectron2/layers/csrc/box_iou_rotated/box_iou_rotated.h
+++ /dev/null
@@ -1,35 +0,0 @@
-// Copyright (c) Facebook, Inc. and its affiliates.
-#pragma once
-#include
-
-namespace detectron2 {
-
-at::Tensor box_iou_rotated_cpu(
- const at::Tensor& boxes1,
- const at::Tensor& boxes2);
-
-#if defined(WITH_CUDA) || defined(WITH_HIP)
-at::Tensor box_iou_rotated_cuda(
- const at::Tensor& boxes1,
- const at::Tensor& boxes2);
-#endif
-
-// Interface for Python
-// inline is needed to prevent multiple function definitions when this header is
-// included by different cpps
-inline at::Tensor box_iou_rotated(
- const at::Tensor& boxes1,
- const at::Tensor& boxes2) {
- assert(boxes1.device().is_cuda() == boxes2.device().is_cuda());
- if (boxes1.device().is_cuda()) {
-#if defined(WITH_CUDA) || defined(WITH_HIP)
- return box_iou_rotated_cuda(boxes1.contiguous(), boxes2.contiguous());
-#else
- AT_ERROR("Not compiled with GPU support");
-#endif
- }
-
- return box_iou_rotated_cpu(boxes1.contiguous(), boxes2.contiguous());
-}
-
-} // namespace detectron2
diff --git a/spaces/CanKorkut/turkish-hatespeech-detection/README.md b/spaces/CanKorkut/turkish-hatespeech-detection/README.md
deleted file mode 100644
index b82635df8508b10c8cb19856755ed2ad0ab643bb..0000000000000000000000000000000000000000
--- a/spaces/CanKorkut/turkish-hatespeech-detection/README.md
+++ /dev/null
@@ -1,13 +0,0 @@
----
-title: Turkish Hatespeech Detection
-emoji: ⚡
-colorFrom: green
-colorTo: red
-sdk: gradio
-sdk_version: 3.23.0
-app_file: app.py
-pinned: false
-license: mit
----
-
-Check out the configuration reference at https://huggingface.co/docs/hub/spaces-config-reference
diff --git a/spaces/Cicooo/vits-uma-genshin-honkai/app.py b/spaces/Cicooo/vits-uma-genshin-honkai/app.py
deleted file mode 100644
index 92ddafdcd240434f58569b0e6964ef331a971dcf..0000000000000000000000000000000000000000
--- a/spaces/Cicooo/vits-uma-genshin-honkai/app.py
+++ /dev/null
@@ -1,124 +0,0 @@
-import time
-import gradio as gr
-import utils
-import commons
-from models import SynthesizerTrn
-from text import text_to_sequence
-from torch import no_grad, LongTensor
-import torch
-
-hps_ms = utils.get_hparams_from_file(r'./model/config.json')
-device = torch.device("cuda" if torch.cuda.is_available() else "cpu")
-net_g_ms = SynthesizerTrn(
- len(hps_ms.symbols),
- hps_ms.data.filter_length // 2 + 1,
- hps_ms.train.segment_size // hps_ms.data.hop_length,
- n_speakers=hps_ms.data.n_speakers,
- **hps_ms.model).to(device)
-_ = net_g_ms.eval()
-speakers = hps_ms.speakers
-model, optimizer, learning_rate, epochs = utils.load_checkpoint(r'./model/G_953000.pth', net_g_ms, None)
-
-def get_text(text, hps):
- text_norm, clean_text = text_to_sequence(text, hps.symbols, hps.data.text_cleaners)
- if hps.data.add_blank:
- text_norm = commons.intersperse(text_norm, 0)
- text_norm = LongTensor(text_norm)
- return text_norm, clean_text
-
-def vits(text, language, speaker_id, noise_scale, noise_scale_w, length_scale):
- start = time.perf_counter()
- if not len(text):
- return "输入文本不能为空!", None, None
- text = text.replace('\n', ' ').replace('\r', '').replace(" ", "")
- if len(text) > 500:
- return f"输入文字过长!{len(text)}>100", None, None
- if language == 0:
- text = f"[ZH]{text}[ZH]"
- elif language == 1:
- text = f"[JA]{text}[JA]"
- else:
- text = f"{text}"
- stn_tst, clean_text = get_text(text, hps_ms)
- with no_grad():
- x_tst = stn_tst.unsqueeze(0)
- x_tst_lengths = LongTensor([stn_tst.size(0)])
- speaker_id = LongTensor([speaker_id])
- audio = net_g_ms.infer(x_tst, x_tst_lengths, sid=speaker_id, noise_scale=noise_scale, noise_scale_w=noise_scale_w,
- length_scale=length_scale)[0][0, 0].data.cpu().float().numpy()
-
- return "生成成功!", (22050, audio), f"生成耗时 {round(time.perf_counter()-start, 2)} s"
-
-def search_speaker(search_value):
- for s in speakers:
- if search_value == s:
- return s
- for s in speakers:
- if search_value in s:
- return s
-
-def change_lang(language):
- if language == 0:
- return 0.6, 0.668, 1.2
- else:
- return 0.6, 0.668, 1.1
-
-download_audio_js = """
-() =>{{
- let root = document.querySelector("body > gradio-app");
- if (root.shadowRoot != null)
- root = root.shadowRoot;
- let audio = root.querySelector("#tts-audio").querySelector("audio");
- let text = root.querySelector("#input-text").querySelector("textarea");
- if (audio == undefined)
- return;
- text = text.value;
- if (text == undefined)
- text = Math.floor(Math.random()*100000000);
- audio = audio.src;
- let oA = document.createElement("a");
- oA.download = text.substr(0, 20)+'.wav';
- oA.href = audio;
- document.body.appendChild(oA);
- oA.click();
- oA.remove();
-}}
-"""
-
-if __name__ == '__main__':
- with gr.Blocks() as app:
- gr.Markdown(
- "# VITS语音在线合成demo\n"
- "主要有赛马娘,原神中文,原神日语,崩坏3的音色
"
- ''
- ''
- )
-
- with gr.Tabs():
- with gr.TabItem("vits"):
- with gr.Row():
- with gr.Column():
- input_text = gr.Textbox(label="Text (100 words limitation)", lines=5, value="今天晚上吃啥好呢。", elem_id=f"input-text")
- lang = gr.Dropdown(label="Language", choices=["中文", "日语", "中日混合(中文用[ZH][ZH]包裹起来,日文用[JA][JA]包裹起来)"],
- type="index", value="中文")
- btn = gr.Button(value="Submit")
- with gr.Row():
- search = gr.Textbox(label="Search Speaker", lines=1)
- btn2 = gr.Button(value="Search")
- sid = gr.Dropdown(label="Speaker", choices=speakers, type="index", value=speakers[228])
- with gr.Row():
- ns = gr.Slider(label="noise_scale(控制感情变化程度)", minimum=0.1, maximum=1.0, step=0.1, value=0.6, interactive=True)
- nsw = gr.Slider(label="noise_scale_w(控制音素发音长度)", minimum=0.1, maximum=1.0, step=0.1, value=0.668, interactive=True)
- ls = gr.Slider(label="length_scale(控制整体语速)", minimum=0.1, maximum=2.0, step=0.1, value=1.2, interactive=True)
- with gr.Column():
- o1 = gr.Textbox(label="Output Message")
- o2 = gr.Audio(label="Output Audio", elem_id=f"tts-audio")
- o3 = gr.Textbox(label="Extra Info")
- download = gr.Button("Download Audio")
- btn.click(vits, inputs=[input_text, lang, sid, ns, nsw, ls], outputs=[o1, o2, o3], api_name="generate")
- download.click(None, [], [], _js=download_audio_js.format())
- btn2.click(search_speaker, inputs=[search], outputs=[sid])
- lang.change(change_lang, inputs=[lang], outputs=[ns, nsw, ls])
- with gr.TabItem("可用人物一览"):
- gr.Radio(label="Speaker", choices=speakers, interactive=False, type="index")
- app.queue(concurrency_count=1).launch()
\ No newline at end of file
diff --git a/spaces/CikeyQI/Yunzai/Yunzai/lib/config/init.js b/spaces/CikeyQI/Yunzai/Yunzai/lib/config/init.js
deleted file mode 100644
index 2cd1cc27e8788f9748137c9ddc5cc49343a4728a..0000000000000000000000000000000000000000
--- a/spaces/CikeyQI/Yunzai/Yunzai/lib/config/init.js
+++ /dev/null
@@ -1,42 +0,0 @@
-import setLog from './log.js'
-import redisInit from './redis.js'
-import { checkRun } from './check.js'
-import cfg from './config.js'
-
-/** 设置标题 */
-process.title = 'TRSS Yunzai'
-
-/** 设置时区 */
-process.env.TZ = 'Asia/Shanghai'
-
-/** 捕获未处理的Promise错误 */
-process.on('unhandledRejection', (error, promise) => {
- if (logger) {
- logger.error(error)
- } else {
- console.log(error)
- }
-})
-
-/** 退出事件 */
-process.on('exit', async code => {
- if (typeof redis != 'undefined' && typeof test == 'undefined')
- await redis.save()
- logger.mark(logger.magenta('TRSS-Yunzai 已停止运行'))
-})
-
-await checkInit()
-
-/** 初始化事件 */
-async function checkInit() {
- /** 日志设置 */
- setLog()
-
- logger.mark('----^_^----')
- logger.mark(logger.yellow(`TRSS-Yunzai v${cfg.package.version} 启动中...`))
- logger.mark(logger.cyan('https://github.com/TimeRainStarSky/Yunzai'))
-
- await redisInit()
-
- checkRun()
-}
diff --git a/spaces/Cloudy1225/stackoverflow-sentiment-analysis/app.py b/spaces/Cloudy1225/stackoverflow-sentiment-analysis/app.py
deleted file mode 100644
index 86c96775d7d564a4f6b6dcc5f6d615c467480a11..0000000000000000000000000000000000000000
--- a/spaces/Cloudy1225/stackoverflow-sentiment-analysis/app.py
+++ /dev/null
@@ -1,148 +0,0 @@
-import csv
-import gradio as gr
-import pandas as pd
-from sentiment_analyser import RandomAnalyser, RoBERTaAnalyser, ChatGPTAnalyser
-import matplotlib.pyplot as plt
-from sklearn.metrics import ConfusionMatrixDisplay, confusion_matrix
-
-
-def plot_bar(value_counts):
- fig, ax = plt.subplots(figsize=(6, 6))
- value_counts.plot.barh(ax=ax)
- ax.bar_label(ax.containers[0])
- plt.title('Frequency of Predictions')
- return fig
-
-
-def plot_confusion_matrix(y_pred, y_true):
- cm = confusion_matrix(y_true, y_pred, normalize='true')
- fig, ax = plt.subplots(figsize=(6, 6))
- labels = []
- for label in SENTI_MAPPING.keys():
- if (label in y_pred.values) or (label in y_true.values):
- labels.append(label)
- disp = ConfusionMatrixDisplay(confusion_matrix=cm,
- display_labels=labels)
- disp.plot(cmap="Blues", values_format=".2f", ax=ax, colorbar=False)
- plt.title("Normalized Confusion Matrix")
- return fig
-
-
-def classify(num: int):
- samples_df = df.sample(num)
- X = samples_df['Text'].tolist()
- y = samples_df['Label']
- roberta = MODEL_MAPPING[OUR_MODEL]
- y_pred = pd.Series(roberta.predict(X), index=samples_df.index)
- samples_df['Predict'] = y_pred
- bar = plot_bar(y_pred.value_counts())
- cm = plot_confusion_matrix(y_pred, y)
- plt.close()
- return samples_df, bar, cm
-
-
-def analysis(Text):
- keys = []
- values = []
- for name, model in MODEL_MAPPING.items():
- keys.append(name)
- values.append(SENTI_MAPPING[model.predict([Text])[0]])
- return pd.DataFrame([values], columns=keys)
-
-
-def analyse_file(file):
- output_name = 'output.csv'
- with open(output_name, mode='w', newline='') as output:
- writer = csv.writer(output)
- header = ['Text', 'Label']
- writer.writerow(header)
- model = MODEL_MAPPING[OUR_MODEL]
- with open(file.name) as f:
- for line in f:
- text = line[:-1]
- sentiment = model.predict([text])
- writer.writerow([text, sentiment[0]])
- return output_name
-
-
-MODEL_MAPPING = {
- 'Random': RandomAnalyser(),
- 'RoBERTa': RoBERTaAnalyser(),
- 'ChatGPT': RandomAnalyser(),
-}
-
-OUR_MODEL = 'RoBERTa'
-
-SENTI_MAPPING = {
- 'negative': '😭',
- 'neutral': '😶',
- 'positive': '🥰'
-}
-
-TITLE = "Sentiment Analysis on Software Engineer Texts"
-DESCRIPTION = (
- "这里是第16组“睿王和他的五个小跟班”软工三迭代三模型演示页面。"
- "模型链接:[Cloudy1225/stackoverflow-roberta-base-sentiment]"
- "(https://huggingface.co/Cloudy1225/stackoverflow-roberta-base-sentiment) "
-)
-
-MAX_SAMPLES = 64
-
-df = pd.read_csv('./SOF4423.csv')
-
-with gr.Blocks(title=TITLE) as demo:
- gr.HTML(f"{TITLE} ")
- gr.Markdown(DESCRIPTION)
- gr.HTML("Model Inference ")
- gr.Markdown((
- "在左侧文本框中输入文本并按回车键,右侧将输出情感分析结果。"
- "这里我们展示了三种结果,分别是随机结果、模型结果和 ChatGPT 结果。"
- ))
- with gr.Row():
- with gr.Column():
- text_input = gr.Textbox(label='Input',
- placeholder="Enter a positive or negative sentence here...")
- with gr.Column():
- senti_output = gr.Dataframe(type="pandas", value=[['😋', '😋', '😋']],
- headers=list(MODEL_MAPPING.keys()), interactive=False)
- text_input.submit(analysis, inputs=text_input, outputs=senti_output, show_progress=True)
-
- gr.Markdown((
- "在左侧文件框中上传 txt/csv 文件,模型会对输入文本的每一行当作一个文本进行情感分析。"
- "可以在右侧下载输出文件,输出文件为两列 csv 格式,第一列为原始文本,第二列为分类结果。"
- ))
- with gr.Row():
- with gr.Column():
- file_input = gr.File(label='File',
- file_types=['.txt', '.csv'])
- with gr.Column():
- file_output = gr.File(label='Output')
- file_input.upload(analyse_file, inputs=file_input, outputs=file_output)
-
- gr.HTML("Model Evaluation ")
- gr.Markdown((
- "这里是在 StackOverflow4423 数据集上评估我们的模型。"
- "滑动 Slider,将会从 StackOverflow4423 数据集中抽样出指定数量的样本,预测其情感标签。"
- "并根据预测结果绘制标签分布图和混淆矩阵。"
- ))
- input_models = list(MODEL_MAPPING)
- input_n_samples = gr.Slider(
- minimum=4,
- maximum=MAX_SAMPLES,
- value=8,
- step=4,
- label='Number of samples'
- )
-
- with gr.Row():
- with gr.Column():
- bar_plot = gr.Plot(label='Predictions Frequency')
- with gr.Column():
- cm_plot = gr.Plot(label='Confusion Matrix')
-
- with gr.Row():
- dataframe = gr.Dataframe(type="pandas", wrap=True, headers=['Text', 'Label', 'Predict'])
-
- input_n_samples.change(fn=classify, inputs=input_n_samples, outputs=[dataframe, bar_plot, cm_plot])
-
-demo.launch()
diff --git a/spaces/CofAI/viewq/index.html b/spaces/CofAI/viewq/index.html
deleted file mode 100644
index 2521505a17f7e85156d7af8eaf9e44af2786b2e9..0000000000000000000000000000000000000000
--- a/spaces/CofAI/viewq/index.html
+++ /dev/null
@@ -1,21 +0,0 @@
-
-
-
- ViewQ
-
-
-
- ViewQ
-
-
-
-
-
- ChatGPT by ViewQ
-
-
-
-
-
-
diff --git a/spaces/Cvandi/remake/experiments/pretrained_models/README.md b/spaces/Cvandi/remake/experiments/pretrained_models/README.md
deleted file mode 100644
index d0cc4afcbdd2c733f6b946bb86bd00baa90e8295..0000000000000000000000000000000000000000
--- a/spaces/Cvandi/remake/experiments/pretrained_models/README.md
+++ /dev/null
@@ -1 +0,0 @@
-# Put downloaded pre-trained models here
diff --git a/spaces/DQChoi/gpt-demo/venv/lib/python3.11/site-packages/aiohttp/web_app.py b/spaces/DQChoi/gpt-demo/venv/lib/python3.11/site-packages/aiohttp/web_app.py
deleted file mode 100644
index 8fd4471d3af019c6e3bd01fcb9838ee99636238e..0000000000000000000000000000000000000000
--- a/spaces/DQChoi/gpt-demo/venv/lib/python3.11/site-packages/aiohttp/web_app.py
+++ /dev/null
@@ -1,557 +0,0 @@
-import asyncio
-import logging
-import warnings
-from functools import partial, update_wrapper
-from typing import (
- TYPE_CHECKING,
- Any,
- AsyncIterator,
- Awaitable,
- Callable,
- Dict,
- Iterable,
- Iterator,
- List,
- Mapping,
- MutableMapping,
- Optional,
- Sequence,
- Tuple,
- Type,
- Union,
- cast,
-)
-
-from aiosignal import Signal
-from frozenlist import FrozenList
-
-from . import hdrs
-from .abc import (
- AbstractAccessLogger,
- AbstractMatchInfo,
- AbstractRouter,
- AbstractStreamWriter,
-)
-from .helpers import DEBUG
-from .http_parser import RawRequestMessage
-from .log import web_logger
-from .streams import StreamReader
-from .web_log import AccessLogger
-from .web_middlewares import _fix_request_current_app
-from .web_protocol import RequestHandler
-from .web_request import Request
-from .web_response import StreamResponse
-from .web_routedef import AbstractRouteDef
-from .web_server import Server
-from .web_urldispatcher import (
- AbstractResource,
- AbstractRoute,
- Domain,
- MaskDomain,
- MatchedSubAppResource,
- PrefixedSubAppResource,
- UrlDispatcher,
-)
-
-__all__ = ("Application", "CleanupError")
-
-
-if TYPE_CHECKING: # pragma: no cover
- from .typedefs import Handler
-
- _AppSignal = Signal[Callable[["Application"], Awaitable[None]]]
- _RespPrepareSignal = Signal[Callable[[Request, StreamResponse], Awaitable[None]]]
- _Middleware = Union[
- Callable[[Request, Handler], Awaitable[StreamResponse]],
- Callable[["Application", Handler], Awaitable[Handler]], # old-style
- ]
- _Middlewares = FrozenList[_Middleware]
- _MiddlewaresHandlers = Optional[Sequence[Tuple[_Middleware, bool]]]
- _Subapps = List["Application"]
-else:
- # No type checker mode, skip types
- _AppSignal = Signal
- _RespPrepareSignal = Signal
- _Middleware = Callable
- _Middlewares = FrozenList
- _MiddlewaresHandlers = Optional[Sequence]
- _Subapps = List
-
-
-class Application(MutableMapping[str, Any]):
- ATTRS = frozenset(
- [
- "logger",
- "_debug",
- "_router",
- "_loop",
- "_handler_args",
- "_middlewares",
- "_middlewares_handlers",
- "_run_middlewares",
- "_state",
- "_frozen",
- "_pre_frozen",
- "_subapps",
- "_on_response_prepare",
- "_on_startup",
- "_on_shutdown",
- "_on_cleanup",
- "_client_max_size",
- "_cleanup_ctx",
- ]
- )
-
- def __init__(
- self,
- *,
- logger: logging.Logger = web_logger,
- router: Optional[UrlDispatcher] = None,
- middlewares: Iterable[_Middleware] = (),
- handler_args: Optional[Mapping[str, Any]] = None,
- client_max_size: int = 1024**2,
- loop: Optional[asyncio.AbstractEventLoop] = None,
- debug: Any = ..., # mypy doesn't support ellipsis
- ) -> None:
- if router is None:
- router = UrlDispatcher()
- else:
- warnings.warn(
- "router argument is deprecated", DeprecationWarning, stacklevel=2
- )
- assert isinstance(router, AbstractRouter), router
-
- if loop is not None:
- warnings.warn(
- "loop argument is deprecated", DeprecationWarning, stacklevel=2
- )
-
- if debug is not ...:
- warnings.warn(
- "debug argument is deprecated", DeprecationWarning, stacklevel=2
- )
- self._debug = debug
- self._router: UrlDispatcher = router
- self._loop = loop
- self._handler_args = handler_args
- self.logger = logger
-
- self._middlewares: _Middlewares = FrozenList(middlewares)
-
- # initialized on freezing
- self._middlewares_handlers: _MiddlewaresHandlers = None
- # initialized on freezing
- self._run_middlewares: Optional[bool] = None
-
- self._state: Dict[str, Any] = {}
- self._frozen = False
- self._pre_frozen = False
- self._subapps: _Subapps = []
-
- self._on_response_prepare: _RespPrepareSignal = Signal(self)
- self._on_startup: _AppSignal = Signal(self)
- self._on_shutdown: _AppSignal = Signal(self)
- self._on_cleanup: _AppSignal = Signal(self)
- self._cleanup_ctx = CleanupContext()
- self._on_startup.append(self._cleanup_ctx._on_startup)
- self._on_cleanup.append(self._cleanup_ctx._on_cleanup)
- self._client_max_size = client_max_size
-
- def __init_subclass__(cls: Type["Application"]) -> None:
- warnings.warn(
- "Inheritance class {} from web.Application "
- "is discouraged".format(cls.__name__),
- DeprecationWarning,
- stacklevel=2,
- )
-
- if DEBUG: # pragma: no cover
-
- def __setattr__(self, name: str, val: Any) -> None:
- if name not in self.ATTRS:
- warnings.warn(
- "Setting custom web.Application.{} attribute "
- "is discouraged".format(name),
- DeprecationWarning,
- stacklevel=2,
- )
- super().__setattr__(name, val)
-
- # MutableMapping API
-
- def __eq__(self, other: object) -> bool:
- return self is other
-
- def __getitem__(self, key: str) -> Any:
- return self._state[key]
-
- def _check_frozen(self) -> None:
- if self._frozen:
- warnings.warn(
- "Changing state of started or joined " "application is deprecated",
- DeprecationWarning,
- stacklevel=3,
- )
-
- def __setitem__(self, key: str, value: Any) -> None:
- self._check_frozen()
- self._state[key] = value
-
- def __delitem__(self, key: str) -> None:
- self._check_frozen()
- del self._state[key]
-
- def __len__(self) -> int:
- return len(self._state)
-
- def __iter__(self) -> Iterator[str]:
- return iter(self._state)
-
- ########
- @property
- def loop(self) -> asyncio.AbstractEventLoop:
- # Technically the loop can be None
- # but we mask it by explicit type cast
- # to provide more convinient type annotation
- warnings.warn("loop property is deprecated", DeprecationWarning, stacklevel=2)
- return cast(asyncio.AbstractEventLoop, self._loop)
-
- def _set_loop(self, loop: Optional[asyncio.AbstractEventLoop]) -> None:
- if loop is None:
- loop = asyncio.get_event_loop()
- if self._loop is not None and self._loop is not loop:
- raise RuntimeError(
- "web.Application instance initialized with different loop"
- )
-
- self._loop = loop
-
- # set loop debug
- if self._debug is ...:
- self._debug = loop.get_debug()
-
- # set loop to sub applications
- for subapp in self._subapps:
- subapp._set_loop(loop)
-
- @property
- def pre_frozen(self) -> bool:
- return self._pre_frozen
-
- def pre_freeze(self) -> None:
- if self._pre_frozen:
- return
-
- self._pre_frozen = True
- self._middlewares.freeze()
- self._router.freeze()
- self._on_response_prepare.freeze()
- self._cleanup_ctx.freeze()
- self._on_startup.freeze()
- self._on_shutdown.freeze()
- self._on_cleanup.freeze()
- self._middlewares_handlers = tuple(self._prepare_middleware())
-
- # If current app and any subapp do not have middlewares avoid run all
- # of the code footprint that it implies, which have a middleware
- # hardcoded per app that sets up the current_app attribute. If no
- # middlewares are configured the handler will receive the proper
- # current_app without needing all of this code.
- self._run_middlewares = True if self.middlewares else False
-
- for subapp in self._subapps:
- subapp.pre_freeze()
- self._run_middlewares = self._run_middlewares or subapp._run_middlewares
-
- @property
- def frozen(self) -> bool:
- return self._frozen
-
- def freeze(self) -> None:
- if self._frozen:
- return
-
- self.pre_freeze()
- self._frozen = True
- for subapp in self._subapps:
- subapp.freeze()
-
- @property
- def debug(self) -> bool:
- warnings.warn("debug property is deprecated", DeprecationWarning, stacklevel=2)
- return self._debug # type: ignore[no-any-return]
-
- def _reg_subapp_signals(self, subapp: "Application") -> None:
- def reg_handler(signame: str) -> None:
- subsig = getattr(subapp, signame)
-
- async def handler(app: "Application") -> None:
- await subsig.send(subapp)
-
- appsig = getattr(self, signame)
- appsig.append(handler)
-
- reg_handler("on_startup")
- reg_handler("on_shutdown")
- reg_handler("on_cleanup")
-
- def add_subapp(self, prefix: str, subapp: "Application") -> AbstractResource:
- if not isinstance(prefix, str):
- raise TypeError("Prefix must be str")
- prefix = prefix.rstrip("/")
- if not prefix:
- raise ValueError("Prefix cannot be empty")
- factory = partial(PrefixedSubAppResource, prefix, subapp)
- return self._add_subapp(factory, subapp)
-
- def _add_subapp(
- self, resource_factory: Callable[[], AbstractResource], subapp: "Application"
- ) -> AbstractResource:
- if self.frozen:
- raise RuntimeError("Cannot add sub application to frozen application")
- if subapp.frozen:
- raise RuntimeError("Cannot add frozen application")
- resource = resource_factory()
- self.router.register_resource(resource)
- self._reg_subapp_signals(subapp)
- self._subapps.append(subapp)
- subapp.pre_freeze()
- if self._loop is not None:
- subapp._set_loop(self._loop)
- return resource
-
- def add_domain(self, domain: str, subapp: "Application") -> AbstractResource:
- if not isinstance(domain, str):
- raise TypeError("Domain must be str")
- elif "*" in domain:
- rule: Domain = MaskDomain(domain)
- else:
- rule = Domain(domain)
- factory = partial(MatchedSubAppResource, rule, subapp)
- return self._add_subapp(factory, subapp)
-
- def add_routes(self, routes: Iterable[AbstractRouteDef]) -> List[AbstractRoute]:
- return self.router.add_routes(routes)
-
- @property
- def on_response_prepare(self) -> _RespPrepareSignal:
- return self._on_response_prepare
-
- @property
- def on_startup(self) -> _AppSignal:
- return self._on_startup
-
- @property
- def on_shutdown(self) -> _AppSignal:
- return self._on_shutdown
-
- @property
- def on_cleanup(self) -> _AppSignal:
- return self._on_cleanup
-
- @property
- def cleanup_ctx(self) -> "CleanupContext":
- return self._cleanup_ctx
-
- @property
- def router(self) -> UrlDispatcher:
- return self._router
-
- @property
- def middlewares(self) -> _Middlewares:
- return self._middlewares
-
- def _make_handler(
- self,
- *,
- loop: Optional[asyncio.AbstractEventLoop] = None,
- access_log_class: Type[AbstractAccessLogger] = AccessLogger,
- **kwargs: Any,
- ) -> Server:
-
- if not issubclass(access_log_class, AbstractAccessLogger):
- raise TypeError(
- "access_log_class must be subclass of "
- "aiohttp.abc.AbstractAccessLogger, got {}".format(access_log_class)
- )
-
- self._set_loop(loop)
- self.freeze()
-
- kwargs["debug"] = self._debug
- kwargs["access_log_class"] = access_log_class
- if self._handler_args:
- for k, v in self._handler_args.items():
- kwargs[k] = v
-
- return Server(
- self._handle, # type: ignore[arg-type]
- request_factory=self._make_request,
- loop=self._loop,
- **kwargs,
- )
-
- def make_handler(
- self,
- *,
- loop: Optional[asyncio.AbstractEventLoop] = None,
- access_log_class: Type[AbstractAccessLogger] = AccessLogger,
- **kwargs: Any,
- ) -> Server:
-
- warnings.warn(
- "Application.make_handler(...) is deprecated, " "use AppRunner API instead",
- DeprecationWarning,
- stacklevel=2,
- )
-
- return self._make_handler(
- loop=loop, access_log_class=access_log_class, **kwargs
- )
-
- async def startup(self) -> None:
- """Causes on_startup signal
-
- Should be called in the event loop along with the request handler.
- """
- await self.on_startup.send(self)
-
- async def shutdown(self) -> None:
- """Causes on_shutdown signal
-
- Should be called before cleanup()
- """
- await self.on_shutdown.send(self)
-
- async def cleanup(self) -> None:
- """Causes on_cleanup signal
-
- Should be called after shutdown()
- """
- if self.on_cleanup.frozen:
- await self.on_cleanup.send(self)
- else:
- # If an exception occurs in startup, ensure cleanup contexts are completed.
- await self._cleanup_ctx._on_cleanup(self)
-
- def _make_request(
- self,
- message: RawRequestMessage,
- payload: StreamReader,
- protocol: RequestHandler,
- writer: AbstractStreamWriter,
- task: "asyncio.Task[None]",
- _cls: Type[Request] = Request,
- ) -> Request:
- return _cls(
- message,
- payload,
- protocol,
- writer,
- task,
- self._loop,
- client_max_size=self._client_max_size,
- )
-
- def _prepare_middleware(self) -> Iterator[Tuple[_Middleware, bool]]:
- for m in reversed(self._middlewares):
- if getattr(m, "__middleware_version__", None) == 1:
- yield m, True
- else:
- warnings.warn(
- 'old-style middleware "{!r}" deprecated, ' "see #2252".format(m),
- DeprecationWarning,
- stacklevel=2,
- )
- yield m, False
-
- yield _fix_request_current_app(self), True
-
- async def _handle(self, request: Request) -> StreamResponse:
- loop = asyncio.get_event_loop()
- debug = loop.get_debug()
- match_info = await self._router.resolve(request)
- if debug: # pragma: no cover
- if not isinstance(match_info, AbstractMatchInfo):
- raise TypeError(
- "match_info should be AbstractMatchInfo "
- "instance, not {!r}".format(match_info)
- )
- match_info.add_app(self)
-
- match_info.freeze()
-
- resp = None
- request._match_info = match_info
- expect = request.headers.get(hdrs.EXPECT)
- if expect:
- resp = await match_info.expect_handler(request)
- await request.writer.drain()
-
- if resp is None:
- handler = match_info.handler
-
- if self._run_middlewares:
- for app in match_info.apps[::-1]:
- for m, new_style in app._middlewares_handlers: # type: ignore[union-attr] # noqa
- if new_style:
- handler = update_wrapper(
- partial(m, handler=handler), handler
- )
- else:
- handler = await m(app, handler) # type: ignore[arg-type]
-
- resp = await handler(request)
-
- return resp
-
- def __call__(self) -> "Application":
- """gunicorn compatibility"""
- return self
-
- def __repr__(self) -> str:
- return f""
-
- def __bool__(self) -> bool:
- return True
-
-
-class CleanupError(RuntimeError):
- @property
- def exceptions(self) -> List[BaseException]:
- return cast(List[BaseException], self.args[1])
-
-
-if TYPE_CHECKING: # pragma: no cover
- _CleanupContextBase = FrozenList[Callable[[Application], AsyncIterator[None]]]
-else:
- _CleanupContextBase = FrozenList
-
-
-class CleanupContext(_CleanupContextBase):
- def __init__(self) -> None:
- super().__init__()
- self._exits: List[AsyncIterator[None]] = []
-
- async def _on_startup(self, app: Application) -> None:
- for cb in self:
- it = cb(app).__aiter__()
- await it.__anext__()
- self._exits.append(it)
-
- async def _on_cleanup(self, app: Application) -> None:
- errors = []
- for it in reversed(self._exits):
- try:
- await it.__anext__()
- except StopAsyncIteration:
- pass
- except Exception as exc:
- errors.append(exc)
- else:
- errors.append(RuntimeError(f"{it!r} has more than one 'yield'"))
- if errors:
- if len(errors) == 1:
- raise errors[0]
- else:
- raise CleanupError("Multiple errors on cleanup stage", errors)
diff --git a/spaces/DQChoi/gpt-demo/venv/lib/python3.11/site-packages/certifi/__init__.py b/spaces/DQChoi/gpt-demo/venv/lib/python3.11/site-packages/certifi/__init__.py
deleted file mode 100644
index 705f416d6b06ce5f51b3ff47c49d078e93c6f034..0000000000000000000000000000000000000000
--- a/spaces/DQChoi/gpt-demo/venv/lib/python3.11/site-packages/certifi/__init__.py
+++ /dev/null
@@ -1,4 +0,0 @@
-from .core import contents, where
-
-__all__ = ["contents", "where"]
-__version__ = "2023.05.07"
diff --git a/spaces/DQChoi/gpt-demo/venv/lib/python3.11/site-packages/fontTools/ttLib/tables/M_A_T_H_.py b/spaces/DQChoi/gpt-demo/venv/lib/python3.11/site-packages/fontTools/ttLib/tables/M_A_T_H_.py
deleted file mode 100644
index 011426b52a195bb2596116cc7bce0ad6e671eb23..0000000000000000000000000000000000000000
--- a/spaces/DQChoi/gpt-demo/venv/lib/python3.11/site-packages/fontTools/ttLib/tables/M_A_T_H_.py
+++ /dev/null
@@ -1,5 +0,0 @@
-from .otBase import BaseTTXConverter
-
-
-class table_M_A_T_H_(BaseTTXConverter):
- pass
diff --git a/spaces/DQChoi/gpt-demo/venv/lib/python3.11/site-packages/fontTools/ttLib/tables/_k_e_r_n.py b/spaces/DQChoi/gpt-demo/venv/lib/python3.11/site-packages/fontTools/ttLib/tables/_k_e_r_n.py
deleted file mode 100644
index 94183c8a0a1e8a02cfc229d525030d9ae2b27ddf..0000000000000000000000000000000000000000
--- a/spaces/DQChoi/gpt-demo/venv/lib/python3.11/site-packages/fontTools/ttLib/tables/_k_e_r_n.py
+++ /dev/null
@@ -1,279 +0,0 @@
-from fontTools.ttLib import getSearchRange
-from fontTools.misc.textTools import safeEval, readHex
-from fontTools.misc.fixedTools import fixedToFloat as fi2fl, floatToFixed as fl2fi
-from . import DefaultTable
-import struct
-import sys
-import array
-import logging
-
-
-log = logging.getLogger(__name__)
-
-
-class table__k_e_r_n(DefaultTable.DefaultTable):
- def getkern(self, format):
- for subtable in self.kernTables:
- if subtable.format == format:
- return subtable
- return None # not found
-
- def decompile(self, data, ttFont):
- version, nTables = struct.unpack(">HH", data[:4])
- apple = False
- if (len(data) >= 8) and (version == 1):
- # AAT Apple's "new" format. Hm.
- version, nTables = struct.unpack(">LL", data[:8])
- self.version = fi2fl(version, 16)
- data = data[8:]
- apple = True
- else:
- self.version = version
- data = data[4:]
- self.kernTables = []
- for i in range(nTables):
- if self.version == 1.0:
- # Apple
- length, coverage, subtableFormat = struct.unpack(">LBB", data[:6])
- else:
- # in OpenType spec the "version" field refers to the common
- # subtable header; the actual subtable format is stored in
- # the 8-15 mask bits of "coverage" field.
- # This "version" is always 0 so we ignore it here
- _, length, subtableFormat, coverage = struct.unpack(">HHBB", data[:6])
- if nTables == 1 and subtableFormat == 0:
- # The "length" value is ignored since some fonts
- # (like OpenSans and Calibri) have a subtable larger than
- # its value.
- (nPairs,) = struct.unpack(">H", data[6:8])
- calculated_length = (nPairs * 6) + 14
- if length != calculated_length:
- log.warning(
- "'kern' subtable longer than defined: "
- "%d bytes instead of %d bytes" % (calculated_length, length)
- )
- length = calculated_length
- if subtableFormat not in kern_classes:
- subtable = KernTable_format_unkown(subtableFormat)
- else:
- subtable = kern_classes[subtableFormat](apple)
- subtable.decompile(data[:length], ttFont)
- self.kernTables.append(subtable)
- data = data[length:]
-
- def compile(self, ttFont):
- if hasattr(self, "kernTables"):
- nTables = len(self.kernTables)
- else:
- nTables = 0
- if self.version == 1.0:
- # AAT Apple's "new" format.
- data = struct.pack(">LL", fl2fi(self.version, 16), nTables)
- else:
- data = struct.pack(">HH", self.version, nTables)
- if hasattr(self, "kernTables"):
- for subtable in self.kernTables:
- data = data + subtable.compile(ttFont)
- return data
-
- def toXML(self, writer, ttFont):
- writer.simpletag("version", value=self.version)
- writer.newline()
- for subtable in self.kernTables:
- subtable.toXML(writer, ttFont)
-
- def fromXML(self, name, attrs, content, ttFont):
- if name == "version":
- self.version = safeEval(attrs["value"])
- return
- if name != "kernsubtable":
- return
- if not hasattr(self, "kernTables"):
- self.kernTables = []
- format = safeEval(attrs["format"])
- if format not in kern_classes:
- subtable = KernTable_format_unkown(format)
- else:
- apple = self.version == 1.0
- subtable = kern_classes[format](apple)
- self.kernTables.append(subtable)
- subtable.fromXML(name, attrs, content, ttFont)
-
-
-class KernTable_format_0(object):
-
- # 'version' is kept for backward compatibility
- version = format = 0
-
- def __init__(self, apple=False):
- self.apple = apple
-
- def decompile(self, data, ttFont):
- if not self.apple:
- version, length, subtableFormat, coverage = struct.unpack(">HHBB", data[:6])
- if version != 0:
- from fontTools.ttLib import TTLibError
-
- raise TTLibError("unsupported kern subtable version: %d" % version)
- tupleIndex = None
- # Should we also assert length == len(data)?
- data = data[6:]
- else:
- length, coverage, subtableFormat, tupleIndex = struct.unpack(
- ">LBBH", data[:8]
- )
- data = data[8:]
- assert self.format == subtableFormat, "unsupported format"
- self.coverage = coverage
- self.tupleIndex = tupleIndex
-
- self.kernTable = kernTable = {}
-
- nPairs, searchRange, entrySelector, rangeShift = struct.unpack(
- ">HHHH", data[:8]
- )
- data = data[8:]
-
- datas = array.array("H", data[: 6 * nPairs])
- if sys.byteorder != "big":
- datas.byteswap()
- it = iter(datas)
- glyphOrder = ttFont.getGlyphOrder()
- for k in range(nPairs):
- left, right, value = next(it), next(it), next(it)
- if value >= 32768:
- value -= 65536
- try:
- kernTable[(glyphOrder[left], glyphOrder[right])] = value
- except IndexError:
- # Slower, but will not throw an IndexError on an invalid
- # glyph id.
- kernTable[
- (ttFont.getGlyphName(left), ttFont.getGlyphName(right))
- ] = value
- if len(data) > 6 * nPairs + 4: # Ignore up to 4 bytes excess
- log.warning(
- "excess data in 'kern' subtable: %d bytes", len(data) - 6 * nPairs
- )
-
- def compile(self, ttFont):
- nPairs = min(len(self.kernTable), 0xFFFF)
- searchRange, entrySelector, rangeShift = getSearchRange(nPairs, 6)
- searchRange &= 0xFFFF
- entrySelector = min(entrySelector, 0xFFFF)
- rangeShift = min(rangeShift, 0xFFFF)
- data = struct.pack(">HHHH", nPairs, searchRange, entrySelector, rangeShift)
-
- # yeehee! (I mean, turn names into indices)
- try:
- reverseOrder = ttFont.getReverseGlyphMap()
- kernTable = sorted(
- (reverseOrder[left], reverseOrder[right], value)
- for ((left, right), value) in self.kernTable.items()
- )
- except KeyError:
- # Slower, but will not throw KeyError on invalid glyph id.
- getGlyphID = ttFont.getGlyphID
- kernTable = sorted(
- (getGlyphID(left), getGlyphID(right), value)
- for ((left, right), value) in self.kernTable.items()
- )
-
- for left, right, value in kernTable:
- data = data + struct.pack(">HHh", left, right, value)
-
- if not self.apple:
- version = 0
- length = len(data) + 6
- if length >= 0x10000:
- log.warning(
- '"kern" subtable overflow, '
- "truncating length value while preserving pairs."
- )
- length &= 0xFFFF
- header = struct.pack(">HHBB", version, length, self.format, self.coverage)
- else:
- if self.tupleIndex is None:
- # sensible default when compiling a TTX from an old fonttools
- # or when inserting a Windows-style format 0 subtable into an
- # Apple version=1.0 kern table
- log.warning("'tupleIndex' is None; default to 0")
- self.tupleIndex = 0
- length = len(data) + 8
- header = struct.pack(
- ">LBBH", length, self.coverage, self.format, self.tupleIndex
- )
- return header + data
-
- def toXML(self, writer, ttFont):
- attrs = dict(coverage=self.coverage, format=self.format)
- if self.apple:
- if self.tupleIndex is None:
- log.warning("'tupleIndex' is None; default to 0")
- attrs["tupleIndex"] = 0
- else:
- attrs["tupleIndex"] = self.tupleIndex
- writer.begintag("kernsubtable", **attrs)
- writer.newline()
- items = sorted(self.kernTable.items())
- for (left, right), value in items:
- writer.simpletag("pair", [("l", left), ("r", right), ("v", value)])
- writer.newline()
- writer.endtag("kernsubtable")
- writer.newline()
-
- def fromXML(self, name, attrs, content, ttFont):
- self.coverage = safeEval(attrs["coverage"])
- subtableFormat = safeEval(attrs["format"])
- if self.apple:
- if "tupleIndex" in attrs:
- self.tupleIndex = safeEval(attrs["tupleIndex"])
- else:
- # previous fontTools versions didn't export tupleIndex
- log.warning("Apple kern subtable is missing 'tupleIndex' attribute")
- self.tupleIndex = None
- else:
- self.tupleIndex = None
- assert subtableFormat == self.format, "unsupported format"
- if not hasattr(self, "kernTable"):
- self.kernTable = {}
- for element in content:
- if not isinstance(element, tuple):
- continue
- name, attrs, content = element
- self.kernTable[(attrs["l"], attrs["r"])] = safeEval(attrs["v"])
-
- def __getitem__(self, pair):
- return self.kernTable[pair]
-
- def __setitem__(self, pair, value):
- self.kernTable[pair] = value
-
- def __delitem__(self, pair):
- del self.kernTable[pair]
-
-
-class KernTable_format_unkown(object):
- def __init__(self, format):
- self.format = format
-
- def decompile(self, data, ttFont):
- self.data = data
-
- def compile(self, ttFont):
- return self.data
-
- def toXML(self, writer, ttFont):
- writer.begintag("kernsubtable", format=self.format)
- writer.newline()
- writer.comment("unknown 'kern' subtable format")
- writer.newline()
- writer.dumphex(self.data)
- writer.endtag("kernsubtable")
- writer.newline()
-
- def fromXML(self, name, attrs, content, ttFont):
- self.decompile(readHex(content), ttFont)
-
-
-kern_classes = {0: KernTable_format_0}
diff --git a/spaces/DQChoi/gpt-demo/venv/lib/python3.11/site-packages/huggingface_hub/inference/__init__.py b/spaces/DQChoi/gpt-demo/venv/lib/python3.11/site-packages/huggingface_hub/inference/__init__.py
deleted file mode 100644
index e69de29bb2d1d6434b8b29ae775ad8c2e48c5391..0000000000000000000000000000000000000000
diff --git a/spaces/Datasculptor/3D-Room-Layout-Estimation_LGT-Net/utils/boundary.py b/spaces/Datasculptor/3D-Room-Layout-Estimation_LGT-Net/utils/boundary.py
deleted file mode 100644
index 034691cb8769aff85927ba1ea222b4a690f95e82..0000000000000000000000000000000000000000
--- a/spaces/Datasculptor/3D-Room-Layout-Estimation_LGT-Net/utils/boundary.py
+++ /dev/null
@@ -1,473 +0,0 @@
-"""
-@date: 2021/06/19
-@description:
-"""
-import math
-import functools
-
-from scipy import stats
-from scipy.ndimage.filters import maximum_filter
-import numpy as np
-from typing import List
-from utils.conversion import uv2xyz, xyz2uv, depth2xyz, uv2pixel, depth2uv, pixel2uv, xyz2pixel, uv2lonlat
-from utils.visibility_polygon import calc_visible_polygon
-
-
-def connect_corners_uv(uv1: np.ndarray, uv2: np.ndarray, length=256) -> np.ndarray:
- """
- :param uv1: [u, v]
- :param uv2: [u, v]
- :param length: Fix the total length in pixel coordinates
- :return:
- """
- # why -0.5? Check out the uv2Pixel function
- p_u1 = uv1[0] * length - 0.5
- p_u2 = uv2[0] * length - 0.5
-
- if abs(p_u1 - p_u2) < length / 2:
- start = np.ceil(min(p_u1, p_u2))
- p = max(p_u1, p_u2)
- end = np.floor(p)
- if end == np.ceil(p):
- end = end - 1
- else:
- start = np.ceil(max(p_u1, p_u2))
- p = min(p_u1, p_u2) + length
- end = np.floor(p)
- if end == np.ceil(p):
- end = end - 1
- p_us = (np.arange(start, end + 1) % length).astype(np.float64)
- if len(p_us) == 0:
- return None
- us = (p_us + 0.5) / length # why +0.5? Check out the uv2Pixel function
-
- plan_y = boundary_type(np.array([uv1, uv2]))
- xyz1 = uv2xyz(np.array(uv1), plan_y)
- xyz2 = uv2xyz(np.array(uv2), plan_y)
- x1 = xyz1[0]
- z1 = xyz1[2]
- x2 = xyz2[0]
- z2 = xyz2[2]
-
- d_x = x2 - x1
- d_z = z2 - z1
-
- lon_s = (us - 0.5) * 2 * np.pi
- k = np.tan(lon_s)
- ps = (k * z1 - x1) / (d_x - k * d_z)
- cs = np.sqrt((z1 + ps * d_z) ** 2 + (x1 + ps * d_x) ** 2)
-
- lats = np.arctan2(plan_y, cs)
- vs = lats / np.pi + 0.5
- uv = np.stack([us, vs], axis=-1)
-
- if start == end:
- return uv[0:1]
- return uv
-
-
-def connect_corners_xyz(uv1: np.ndarray, uv2: np.ndarray, step=0.01) -> np.ndarray:
- """
- :param uv1: [u, v]
- :param uv2: [u, v]
- :param step: Fixed step size in xyz coordinates
- :return:
- """
- plan_y = boundary_type(np.array([uv1, uv2]))
- xyz1 = uv2xyz(np.array(uv1), plan_y)
- xyz2 = uv2xyz(np.array(uv2), plan_y)
-
- vec = xyz2 - xyz1
- norm = np.linalg.norm(vec, ord=2)
- direct = vec / norm
- xyz = np.array([xyz1 + direct * dis for dis in np.linspace(0, norm, int(norm / step))])
- if len(xyz) == 0:
- xyz = np.array([xyz2])
- uv = xyz2uv(xyz)
- return uv
-
-
-def connect_corners(uv1: np.ndarray, uv2: np.ndarray, step=0.01, length=None) -> np.ndarray:
- """
- :param uv1: [u, v]
- :param uv2: [u, v]
- :param step:
- :param length:
- :return: [[u1, v1], [u2, v2]....] if length!=None,length of return result = length
- """
- if length is not None:
- uv = connect_corners_uv(uv1, uv2, length)
- elif step is not None:
- uv = connect_corners_xyz(uv1, uv2, step)
- else:
- uv = np.array([uv1])
- return uv
-
-
-def visibility_corners(corners):
- plan_y = boundary_type(corners)
- xyz = uv2xyz(corners, plan_y)
- xz = xyz[:, ::2]
- xz = calc_visible_polygon(center=np.array([0, 0]), polygon=xz, show=False)
- xyz = np.insert(xz, 1, plan_y, axis=1)
- output = xyz2uv(xyz).astype(np.float32)
- return output
-
-
-def corners2boundary(corners: np.ndarray, step=0.01, length=None, visible=True) -> np.ndarray:
- """
- When there is occlusion, even if the length is fixed, the final output length may be greater than the given length,
- which is more defined as the fixed step size under UV
- :param length:
- :param step:
- :param corners: [[u1, v1], [u2, v2]....]
- :param visible:
- :return: [[u1, v1], [u2, v2]....] if length!=None,length of return result = length
- """
- assert step is not None or length is not None, "the step and length parameters cannot be null at the same time"
- if len(corners) < 3:
- return corners
-
- if visible:
- corners = visibility_corners(corners)
-
- n_con = len(corners)
- boundary = None
- for j in range(n_con):
- uv = connect_corners(corners[j], corners[(j + 1) % n_con], step, length)
- if uv is None:
- continue
- if boundary is None:
- boundary = uv
- else:
- boundary = np.concatenate((boundary, uv))
- boundary = np.roll(boundary, -boundary.argmin(axis=0)[0], axis=0)
-
- output_polygon = []
- for i, p in enumerate(boundary):
- q = boundary[(i + 1) % len(boundary)]
- if int(p[0] * 10000) == int(q[0] * 10000):
- continue
- output_polygon.append(p)
- output_polygon = np.array(output_polygon, dtype=np.float32)
- return output_polygon
-
-
-def corners2boundaries(ratio: float, corners_xyz: np.ndarray = None, corners_uv: np.ndarray = None, step=0.01,
- length=None, visible=True):
- """
- When both step and length are None, corners are also returned
- :param ratio:
- :param corners_xyz:
- :param corners_uv:
- :param step:
- :param length:
- :param visible:
- :return: floor_boundary, ceil_boundary
- """
- if corners_xyz is None:
- plan_y = boundary_type(corners_uv)
- xyz = uv2xyz(corners_uv, plan_y)
- floor_xyz = xyz.copy()
- ceil_xyz = xyz.copy()
- if plan_y > 0:
- ceil_xyz[:, 1] *= -ratio
- else:
- floor_xyz[:, 1] /= -ratio
- else:
- floor_xyz = corners_xyz.copy()
- ceil_xyz = corners_xyz.copy()
- if corners_xyz[0][1] > 0:
- ceil_xyz[:, 1] *= -ratio
- else:
- floor_xyz[:, 1] /= -ratio
-
- floor_uv = xyz2uv(floor_xyz)
- ceil_uv = xyz2uv(ceil_xyz)
- if step is None and length is None:
- return floor_uv, ceil_uv
-
- floor_boundary = corners2boundary(floor_uv, step, length, visible)
- ceil_boundary = corners2boundary(ceil_uv, step, length, visible)
- return floor_boundary, ceil_boundary
-
-
-def depth2boundary(depth: np.array, step=0.01, length=None,):
- xyz = depth2xyz(depth)
- uv = xyz2uv(xyz)
- return corners2boundary(uv, step, length, visible=False)
-
-
-def depth2boundaries(ratio: float, depth: np.array, step=0.01, length=None,):
- """
-
- :param ratio:
- :param depth:
- :param step:
- :param length:
- :return: floor_boundary, ceil_boundary
- """
- xyz = depth2xyz(depth)
- return corners2boundaries(ratio, corners_xyz=xyz, step=step, length=length, visible=False)
-
-
-def boundary_type(corners: np.ndarray) -> int:
- """
- Returns the boundary type that also represents the projection plane
- :param corners:
- :return:
- """
- if is_ceil_boundary(corners):
- plan_y = -1
- elif is_floor_boundary(corners):
- plan_y = 1
- else:
- # An intersection occurs and an exception is considered
- assert False, 'corners error!'
- return plan_y
-
-
-def is_normal_layout(boundaries: List[np.array]):
- if len(boundaries) != 2:
- print("boundaries length must be 2!")
- return False
-
- if boundary_type(boundaries[0]) != -1:
- print("ceil boundary error!")
- return False
-
- if boundary_type(boundaries[1]) != 1:
- print("floor boundary error!")
- return False
- return True
-
-
-def is_ceil_boundary(corners: np.ndarray) -> bool:
- m = corners[..., 1].max()
- return m < 0.5
-
-
-def is_floor_boundary(corners: np.ndarray) -> bool:
- m = corners[..., 1].min()
- return m > 0.5
-
-
-@functools.lru_cache()
-def get_gauss_map(sigma=1.5, width=5):
- x = np.arange(width*2 + 1) - width
- y = stats.norm(0, sigma).pdf(x)
- y = y / y.max()
- return y
-
-
-def get_heat_map(u_s, patch_num=256, sigma=2, window_width=15, show=False):
- """
- :param window_width:
- :param sigma:
- :param u_s: [u1, u2, u3, ...]
- :param patch_num
- :param show
- :return:
- """
- pixel_us = uv2pixel(u_s, w=patch_num, axis=0)
- gauss_map = get_gauss_map(sigma, window_width)
- heat_map_all = []
- for u in pixel_us:
- heat_map = np.zeros(patch_num, dtype=np.float)
- left = u-window_width
- right = u+window_width+1
-
- offset = 0
- if left < 0:
- offset = left
- elif right > patch_num:
- offset = right - patch_num
-
- left = left - offset
- right = right - offset
- heat_map[left:right] = gauss_map
- if offset != 0:
- heat_map = np.roll(heat_map, offset)
- heat_map_all.append(heat_map)
-
- heat_map_all = np.array(heat_map_all).max(axis=0)
- if show:
- import matplotlib.pyplot as plt
- plt.imshow(heat_map_all[None].repeat(50, axis=0))
- plt.show()
- return heat_map_all
-
-
-def find_peaks(signal, size=15*2+1, min_v=0.05, N=None):
- # code from HorizonNet: https://github.com/sunset1995/HorizonNet/blob/master/inference.py
- max_v = maximum_filter(signal, size=size, mode='wrap')
- pk_loc = np.where(max_v == signal)[0]
- pk_loc = pk_loc[signal[pk_loc] > min_v]
- if N is not None:
- order = np.argsort(-signal[pk_loc])
- pk_loc = pk_loc[order[:N]]
- pk_loc = pk_loc[np.argsort(pk_loc)]
- return pk_loc, signal[pk_loc]
-
-
-def get_object_cor(depth, size, center_u, patch_num=256):
- width_u = size[0, center_u]
- height_v = size[1, center_u]
- boundary_v = size[2, center_u]
-
- center_boundary_v = depth2uv(depth[center_u:center_u + 1])[0, 1]
- center_bottom_v = center_boundary_v - boundary_v
- center_top_v = center_bottom_v - height_v
-
- base_v = center_boundary_v - 0.5
- assert base_v > 0
-
- center_u = pixel2uv(np.array([center_u]), w=patch_num, h=patch_num // 2, axis=0)[0]
-
- center_boundary_uv = np.array([center_u, center_boundary_v])
- center_bottom_uv = np.array([center_u, center_bottom_v])
- center_top_uv = np.array([center_u, center_top_v])
-
- left_u = center_u - width_u / 2
- right_u = center_u + width_u / 2
-
- left_u = 1 + left_u if left_u < 0 else left_u
- right_u = right_u - 1 if right_u > 1 else right_u
-
- pixel_u = uv2pixel(np.array([left_u, right_u]), w=patch_num, h=patch_num // 2, axis=0)
- left_pixel_u = pixel_u[0]
- right_pixel_u = pixel_u[1]
-
- left_boundary_v = depth2uv(depth[left_pixel_u:left_pixel_u + 1])[0, 1]
- right_boundary_v = depth2uv(depth[right_pixel_u:right_pixel_u + 1])[0, 1]
-
- left_boundary_uv = np.array([left_u, left_boundary_v])
- right_boundary_uv = np.array([right_u, right_boundary_v])
-
- xyz = uv2xyz(np.array([left_boundary_uv, right_boundary_uv, center_boundary_uv]))
- left_boundary_xyz = xyz[0]
- right_boundary_xyz = xyz[1]
-
- # need align
- center_boundary_xyz = xyz[2]
- center_bottom_xyz = uv2xyz(np.array([center_bottom_uv]))[0]
- center_top_xyz = uv2xyz(np.array([center_top_uv]))[0]
- center_boundary_norm = np.linalg.norm(center_boundary_xyz[::2])
- center_bottom_norm = np.linalg.norm(center_bottom_xyz[::2])
- center_top_norm = np.linalg.norm(center_top_xyz[::2])
- center_bottom_xyz = center_bottom_xyz * center_boundary_norm / center_bottom_norm
- center_top_xyz = center_top_xyz * center_boundary_norm / center_top_norm
-
- left_bottom_xyz = left_boundary_xyz.copy()
- left_bottom_xyz[1] = center_bottom_xyz[1]
- right_bottom_xyz = right_boundary_xyz.copy()
- right_bottom_xyz[1] = center_bottom_xyz[1]
-
- left_top_xyz = left_boundary_xyz.copy()
- left_top_xyz[1] = center_top_xyz[1]
- right_top_xyz = right_boundary_xyz.copy()
- right_top_xyz[1] = center_top_xyz[1]
-
- uv = xyz2uv(np.array([left_bottom_xyz, right_bottom_xyz, left_top_xyz, right_top_xyz]))
- left_bottom_uv = uv[0]
- right_bottom_uv = uv[1]
- left_top_uv = uv[2]
- right_top_uv = uv[3]
-
- return [left_bottom_uv, right_bottom_uv, left_top_uv, right_top_uv], \
- [left_bottom_xyz, right_bottom_xyz, left_top_xyz, right_top_xyz]
-
-
-def layout2depth(boundaries: List[np.array], return_mask=False, show=False, camera_height=1.6):
- """
-
- :param camera_height:
- :param boundaries: [[[u_f1, v_f2], [u_f2, v_f2],...], [[u_c1, v_c2], [u_c2, v_c2]]]
- :param return_mask:
- :param show:
- :return:
- """
- # code from HorizonNet: https://github.com/sunset1995/HorizonNet/blob/master/eval_general.py
-
- w = len(boundaries[0])
- h = w//2
- # Convert corners to per-column boundary first
- # Up -pi/2, Down pi/2
- vf = uv2lonlat(boundaries[0])
- vc = uv2lonlat(boundaries[1])
- vc = vc[None, :, 1] # [1, w]
- vf = vf[None, :, 1] # [1, w]
- assert (vc > 0).sum() == 0
- assert (vf < 0).sum() == 0
-
- # Per-pixel v coordinate (vertical angle)
- vs = ((np.arange(h) + 0.5) / h - 0.5) * np.pi
- vs = np.repeat(vs[:, None], w, axis=1) # [h, w]
-
- # Floor-plane to depth
- floor_h = camera_height
- floor_d = np.abs(floor_h / np.sin(vs))
-
- # wall to camera distance on horizontal plane at cross camera center
- cs = floor_h / np.tan(vf)
-
- # Ceiling-plane to depth
- ceil_h = np.abs(cs * np.tan(vc)) # [1, w]
- ceil_d = np.abs(ceil_h / np.sin(vs)) # [h, w]
-
- # Wall to depth
- wall_d = np.abs(cs / np.cos(vs)) # [h, w]
-
- # Recover layout depth
- floor_mask = (vs > vf)
- ceil_mask = (vs < vc)
- wall_mask = (~floor_mask) & (~ceil_mask)
- depth = np.zeros([h, w], np.float32) # [h, w]
- depth[floor_mask] = floor_d[floor_mask]
- depth[ceil_mask] = ceil_d[ceil_mask]
- depth[wall_mask] = wall_d[wall_mask]
-
- assert (depth == 0).sum() == 0
- if return_mask:
- return depth, floor_mask, ceil_mask, wall_mask
- if show:
- import matplotlib.pyplot as plt
- plt.imshow(depth)
- plt.show()
- return depth
-
-
-def calc_rotation(corners: np.ndarray):
- xz = uv2xyz(corners)[..., 0::2]
- max_norm = -1
- max_v = None
- for i in range(len(xz)):
- p_c = xz[i]
- p_n = xz[(i + 1) % len(xz)]
- v_cn = p_n - p_c
- v_norm = np.linalg.norm(v_cn)
- if v_norm > max_norm:
- max_norm = v_norm
- max_v = v_cn
-
- # v<-----------|o
- # | | |
- # | ----|----z |
- # | | |
- # | x \|/
- # |------------u
- # It is required that the vector be aligned on the x-axis, z equals y, and x is still x.
- # In floorplan, x is displayed as the x-coordinate and z as the y-coordinate
- rotation = np.arctan2(max_v[1], max_v[0])
- return rotation
-
-
-if __name__ == '__main__':
- corners = np.array([[0.2, 0.7],
- [0.4, 0.7],
- [0.3, 0.6],
- [0.6, 0.6],
- [0.8, 0.7]])
- get_heat_map(u=corners[..., 0], show=True, sigma=2, width=15)
- pass
-
diff --git a/spaces/DragGan/DragGan-Inversion/gui_utils/imgui_window.py b/spaces/DragGan/DragGan-Inversion/gui_utils/imgui_window.py
deleted file mode 100644
index 5937788f2e8e51772677ab12c67038f5ccd37b42..0000000000000000000000000000000000000000
--- a/spaces/DragGan/DragGan-Inversion/gui_utils/imgui_window.py
+++ /dev/null
@@ -1,110 +0,0 @@
-# Copyright (c) 2021, NVIDIA CORPORATION & AFFILIATES. All rights reserved.
-#
-# NVIDIA CORPORATION and its licensors retain all intellectual property
-# and proprietary rights in and to this software, related documentation
-# and any modifications thereto. Any use, reproduction, disclosure or
-# distribution of this software and related documentation without an express
-# license agreement from NVIDIA CORPORATION is strictly prohibited.
-
-import os
-import imgui
-import imgui.integrations.glfw
-
-from . import glfw_window
-from . import imgui_utils
-from . import text_utils
-
-# ----------------------------------------------------------------------------
-
-
-class ImguiWindow(glfw_window.GlfwWindow):
- def __init__(self, *, title='ImguiWindow', font=None, font_sizes=range(14, 24), **glfw_kwargs):
- if font is None:
- font = text_utils.get_default_font()
- font_sizes = {int(size) for size in font_sizes}
- super().__init__(title=title, **glfw_kwargs)
-
- # Init fields.
- self._imgui_context = None
- self._imgui_renderer = None
- self._imgui_fonts = None
- self._cur_font_size = max(font_sizes)
-
- # Delete leftover imgui.ini to avoid unexpected behavior.
- if os.path.isfile('imgui.ini'):
- os.remove('imgui.ini')
-
- # Init ImGui.
- self._imgui_context = imgui.create_context()
- self._imgui_renderer = _GlfwRenderer(self._glfw_window)
- self._attach_glfw_callbacks()
- # Disable creating imgui.ini at runtime.
- imgui.get_io().ini_saving_rate = 0
- # Improve behavior with imgui_utils.drag_custom().
- imgui.get_io().mouse_drag_threshold = 0
- self._imgui_fonts = {size: imgui.get_io().fonts.add_font_from_file_ttf(
- font, size) for size in font_sizes}
- self._imgui_renderer.refresh_font_texture()
-
- def close(self):
- self.make_context_current()
- self._imgui_fonts = None
- if self._imgui_renderer is not None:
- self._imgui_renderer.shutdown()
- self._imgui_renderer = None
- if self._imgui_context is not None:
- # imgui.destroy_context(self._imgui_context) # Commented out to avoid creating imgui.ini at the end.
- self._imgui_context = None
- super().close()
-
- def _glfw_key_callback(self, *args):
- super()._glfw_key_callback(*args)
- self._imgui_renderer.keyboard_callback(*args)
-
- @property
- def font_size(self):
- return self._cur_font_size
-
- @property
- def spacing(self):
- return round(self._cur_font_size * 0.4)
-
- def set_font_size(self, target): # Applied on next frame.
- self._cur_font_size = min((abs(key - target), key)
- for key in self._imgui_fonts.keys())[1]
-
- def begin_frame(self):
- # Begin glfw frame.
- super().begin_frame()
-
- # Process imgui events.
- self._imgui_renderer.mouse_wheel_multiplier = self._cur_font_size / 10
- if self.content_width > 0 and self.content_height > 0:
- self._imgui_renderer.process_inputs()
-
- # Begin imgui frame.
- imgui.new_frame()
- imgui.push_font(self._imgui_fonts[self._cur_font_size])
- imgui_utils.set_default_style(
- spacing=self.spacing, indent=self.font_size, scrollbar=self.font_size+4)
-
- def end_frame(self):
- imgui.pop_font()
- imgui.render()
- imgui.end_frame()
- self._imgui_renderer.render(imgui.get_draw_data())
- super().end_frame()
-
-# ----------------------------------------------------------------------------
-# Wrapper class for GlfwRenderer to fix a mouse wheel bug on Linux.
-
-
-class _GlfwRenderer(imgui.integrations.glfw.GlfwRenderer):
- def __init__(self, *args, **kwargs):
- super().__init__(*args, **kwargs)
- self.mouse_wheel_multiplier = 1
-
- def scroll_callback(self, window, x_offset, y_offset):
- self.io.mouse_wheel += y_offset * self.mouse_wheel_multiplier
-
-# ----------------------------------------------------------------------------
diff --git a/spaces/DragGan/DragGan-Inversion/stylegan_human/utils/util.py b/spaces/DragGan/DragGan-Inversion/stylegan_human/utils/util.py
deleted file mode 100644
index 544c94895dfc0bfcd1285fde7cd2c102b71113ed..0000000000000000000000000000000000000000
--- a/spaces/DragGan/DragGan-Inversion/stylegan_human/utils/util.py
+++ /dev/null
@@ -1,84 +0,0 @@
-# Copyright (c) SenseTime Research. All rights reserved.
-
-import torch
-import cv2
-from torchvision import transforms
-import numpy as np
-import math
-
-
-def visual(output, out_path):
- output = (output + 1)/2
- output = torch.clamp(output, 0, 1)
- if output.shape[1] == 1:
- output = torch.cat([output, output, output], 1)
- output = output[0].detach().cpu().permute(1, 2, 0).numpy()
- output = (output*255).astype(np.uint8)
- output = output[:, :, ::-1]
- cv2.imwrite(out_path, output)
-
-
-def get_lr(t, initial_lr, rampdown=0.25, rampup=0.05):
-
- lr_ramp = min(1, (1 - t) / rampdown)
- lr_ramp = 0.5 - 0.5 * math.cos(lr_ramp * math.pi)
- lr_ramp = lr_ramp * min(1, t / rampup)
- return initial_lr * lr_ramp
-
-
-def latent_noise(latent, strength):
- noise = torch.randn_like(latent) * strength
-
- return latent + noise
-
-
-def noise_regularize_(noises):
- loss = 0
-
- for noise in noises:
- size = noise.shape[2]
-
- while True:
- loss = (
- loss
- + (noise * torch.roll(noise, shifts=1, dims=3)).mean().pow(2)
- + (noise * torch.roll(noise, shifts=1, dims=2)).mean().pow(2)
- )
-
- if size <= 8:
- break
-
- noise = noise.reshape([-1, 1, size // 2, 2, size // 2, 2])
- noise = noise.mean([3, 5])
- size //= 2
-
- return loss
-
-
-def noise_normalize_(noises):
- for noise in noises:
- mean = noise.mean()
- std = noise.std()
-
- noise.data.add_(-mean).div_(std)
-
-
-def tensor_to_numpy(x):
- x = x[0].permute(1, 2, 0)
- x = torch.clamp(x, -1, 1)
- x = (x+1) * 127.5
- x = x.cpu().detach().numpy().astype(np.uint8)
- return x
-
-
-def numpy_to_tensor(x):
- x = (x / 255 - 0.5) * 2
- x = torch.from_numpy(x).unsqueeze(0).permute(0, 3, 1, 2)
- x = x.cuda().float()
- return x
-
-
-def tensor_to_pil(x):
- x = torch.clamp(x, -1, 1)
- x = (x+1) * 127.5
- return transforms.ToPILImage()(x.squeeze_(0))
diff --git a/spaces/Eddycrack864/Applio-Inference/train/losses.py b/spaces/Eddycrack864/Applio-Inference/train/losses.py
deleted file mode 100644
index b89038f14d06d7fae43628183e9ffb465e4edafd..0000000000000000000000000000000000000000
--- a/spaces/Eddycrack864/Applio-Inference/train/losses.py
+++ /dev/null
@@ -1,59 +0,0 @@
-import torch
-from torch.nn import functional as F
-
-
-def feature_loss(fmap_r, fmap_g):
- loss = 0
- for dr, dg in zip(fmap_r, fmap_g):
- for rl, gl in zip(dr, dg):
- rl = rl.float().detach()
- gl = gl.float()
- loss += torch.mean(torch.abs(rl - gl))
-
- return loss * 2
-
-
-def discriminator_loss(disc_real_outputs, disc_generated_outputs):
- loss = 0
- r_losses = []
- g_losses = []
- for dr, dg in zip(disc_real_outputs, disc_generated_outputs):
- dr = dr.float()
- dg = dg.float()
- r_loss = torch.mean((1 - dr) ** 2)
- g_loss = torch.mean(dg**2)
- loss += r_loss + g_loss
- r_losses.append(r_loss.item())
- g_losses.append(g_loss.item())
-
- return loss, r_losses, g_losses
-
-
-def generator_loss(disc_outputs):
- loss = 0
- gen_losses = []
- for dg in disc_outputs:
- dg = dg.float()
- l = torch.mean((1 - dg) ** 2)
- gen_losses.append(l)
- loss += l
-
- return loss, gen_losses
-
-
-def kl_loss(z_p, logs_q, m_p, logs_p, z_mask):
- """
- z_p, logs_q: [b, h, t_t]
- m_p, logs_p: [b, h, t_t]
- """
- z_p = z_p.float()
- logs_q = logs_q.float()
- m_p = m_p.float()
- logs_p = logs_p.float()
- z_mask = z_mask.float()
-
- kl = logs_p - logs_q - 0.5
- kl += 0.5 * ((z_p - m_p) ** 2) * torch.exp(-2.0 * logs_p)
- kl = torch.sum(kl * z_mask)
- l = kl / torch.sum(z_mask)
- return l
diff --git a/spaces/FedeFT/Head_Pose_Estimation_and_LAEO_computation/utils/my_utils.py b/spaces/FedeFT/Head_Pose_Estimation_and_LAEO_computation/utils/my_utils.py
deleted file mode 100644
index 3ca21d557102ea9f8a811a699f2baea6858d8155..0000000000000000000000000000000000000000
--- a/spaces/FedeFT/Head_Pose_Estimation_and_LAEO_computation/utils/my_utils.py
+++ /dev/null
@@ -1,1375 +0,0 @@
-import numpy as np
-from scipy.spatial import distance as dist
-from utils.labels import pose_id_part, pose_id_part_openpose, rev_pose_id_part_openpose, rev_pose_id_part
-import cv2
-import os
-import json
-
-
-def rescale_bb(boxes, pad, im_width, im_height):
- """
- Modify in place the bounding box coordinates (percentage) to the new image width and height
-
- Args:
- :boxes (numpy.ndarray): Array of bounding box coordinates expressed in percentage [y_min, x_min, y_max, x_max]
- :pad (tuple): The first element represents the right padding (applied by resize_preserving_ar() function);
- the second element represents the bottom padding (applied by resize_preserving_ar() function) and
- the third element is a tuple that is the shape of the image after resizing without the padding (this is useful for
- the coordinates changes)
- :im_width (int): The new image width
- :im_height (int): The new image height
-
- Returns:
- """
-
- right_padding = pad[0]
- bottom_padding = pad[1]
-
- if bottom_padding != 0:
- for box in boxes:
- y_min, y_max = box[0] * im_height, box[2] * im_height # to pixels
- box[0], box[2] = y_min / (im_height - pad[1]), y_max / (im_height - pad[1]) # back to percentage
-
- if right_padding != 0:
- for box in boxes:
- x_min, x_max = box[1] * im_width, box[3] * im_width # to pixels
- box[1], box[3] = x_min / (im_width - pad[0]), x_max / (im_width - pad[0]) # back to percentage
-
-
-def rescale_key_points(key_points, pad, im_width, im_height):
- """
- Modify in place the bounding box coordinates (percentage) to the new image width and height
-
- Args:
- :key_points (numpy.ndarray): Array of bounding box coordinates expressed in percentage [y_min, x_min, y_max, x_max]
- :pad (tuple): The first element represents the right padding (applied by resize_preserving_ar() function);
- the second element represents the bottom padding (applied by resize_preserving_ar() function) and
- the third element is a tuple that is the shape of the image after resizing without the padding (this is useful for
- the coordinates changes)
- :im_width (int): The new image width
- :im_height (int): The new image height
-
- Returns:
- """
-
- right_padding = pad[0]
- bottom_padding = pad[1]
-
- if bottom_padding != 0:
- for aux in key_points:
- for point in aux: # x 1 y 0
- y = point[0] * im_height
- point[0] = y / (im_height - pad[1])
-
- if right_padding != 0:
- for aux in key_points:
- for point in aux:
- x = point[1] * im_width
- point[1] = x / (im_width - pad[0])
-
-
-def change_coordinates_aspect_ratio(aux_key_points_array, img_person, img_person_resized):
- """
-
- Args:
- :
-
- Returns:
- :
- """
-
- aux_key_points_array_ratio = []
- ratio_h, ratio_w = img_person.shape[0] / (img_person_resized.shape[1]), img_person.shape[1] / (img_person_resized.shape[2]) # shape 0 batch 1
-
- for elem in aux_key_points_array:
- aux = np.zeros(3)
- aux[0] = int((elem[0]) * ratio_h)
- aux[1] = int(elem[1] * ratio_h)
- aux[2] = int(elem[2])
- aux_key_points_array_ratio.append(aux)
-
- aux_key_points_array_ratio = np.array(aux_key_points_array_ratio, dtype=int)
-
- return aux_key_points_array_ratio
-
-
-def parse_output_pose(heatmaps, offsets, threshold):
- """
- Parse the output pose (auxiliary function for tflite models)
- Args:
- :
-
- Returns:
- :
- """
- #
- # heatmaps: 9x9x17 probability of appearance of each keypoint in the particular part of the image (9,9) -> used to locate position of the joints
- # offsets: 9x9x34 used for calculation of the keypoint's position (first 17 x coords, the second 17 y coords)
- #
- joint_num = heatmaps.shape[-1]
- pose_kps = np.zeros((joint_num, 3), np.uint32)
-
- for i in range(heatmaps.shape[-1]):
- joint_heatmap = heatmaps[..., i]
- max_val_pos = np.squeeze(np.argwhere(joint_heatmap == np.max(joint_heatmap)))
- remap_pos = np.array(max_val_pos / 8 * 257, dtype=np.int32)
- pose_kps[i, 0] = int(remap_pos[0] + offsets[max_val_pos[0], max_val_pos[1], i])
- pose_kps[i, 1] = int(remap_pos[1] + offsets[max_val_pos[0], max_val_pos[1], i + joint_num])
- max_prob = np.max(joint_heatmap)
-
- if max_prob > threshold:
- if pose_kps[i, 0] < 257 and pose_kps[i, 1] < 257:
- pose_kps[i, 2] = 1
-
- return pose_kps
-
-
-def retrieve_xyz_from_detection(points_list, point_cloud_img):
- """
- Retrieve the xyz of the list of points passed as input (if we have the point cloud of the image)
- Args:
- :points_list (list): list of points for which we want to retrieve xyz information
- :point_cloud_img (numpy.ndarray): numpy array containing XYZRGBA information of the image
-
- Returns:
- :xyz (list): list of lists of 3D points with XYZ information (left camera origin (0,0,0))
- """
-
- xyz = [[point_cloud_img[:, :, 0][point[1], point[0]], point_cloud_img[:, :, 1][point[1], point[0]], point_cloud_img[:, :, 2][point[1], point[0]]]
- for point in points_list]
- return xyz
-
-
-def retrieve_xyz_pose_points(point_cloud_image, key_points_score, key_points):
- """Retrieve the key points from the point cloud to get the XYZ position in the 3D space
-
- Args:
- :point_cloud_image (numpy.ndarray):
- :key_points_score (list):
- :key_points (list):
-
- Returns:
- :xyz_pose: a list of lists representing the XYZ 3D coordinates of each key point (j is the index number of the id pose)
- """
- xyz_pose = []
-
- for i in range(len(key_points_score)):
- xyz_pose_aux = []
- for j in range(len(key_points_score[i])):
- # if key_points_score[i][j] > threshold:# and j < 5:
- x, y = int(key_points[i][j][0] * point_cloud_image.shape[0]) - 1, int(key_points[i][j][1] * point_cloud_image.shape[1]) - 1
- xyz_pose_aux.append([point_cloud_image[x, y, 0], point_cloud_image[x, y, 1], point_cloud_image[x, y, 2], key_points_score[i][j]])
-
- xyz_pose.append(xyz_pose_aux)
- return xyz_pose
-
-
-def compute_distance(points_list, min_distance=1.5):
- """
- Compute the distance between each point and find if there are points that are closer to each other that do not respect a certain distance
- expressed in meter.
-
- Args:
- :points_list (list): list of points expressed in xyz 3D coordinates (meters)
- :min_distance (float): minimum threshold for distances (if the l2 distance between two objects is lower than this value it is considered a violation)
- (default is 1.5)
-
- Returns:
- :distance_matrix: matrix containing the distances between each points (diagonal 0)
- :violate: set of points that violate the minimum distance threshold
- :couple_points: list of lists of couple points that violate the min_distance threshold (to keep track of each couple)
- """
-
- if points_list is None or len(points_list) == 1 or len(points_list) == 0:
- return None, None, None
- else: # if there are more than two points
- violate = set()
- couple_points = []
- aux = np.array(points_list)
- distance_matrix = dist.cdist(aux, aux, 'euclidean')
- for i in range(0, distance_matrix.shape[0]): # loop over the upper triangular of the distance matrix
- for j in range(i + 1, distance_matrix.shape[1]):
- if distance_matrix[i, j] < min_distance:
- # print("Distance between {} and {} is {:.2f} meters".format(i, j, distance_matrix[i, j]))
- violate.add(i)
- violate.add(j)
- couple_points.append((i, j))
-
- return distance_matrix, violate, couple_points
-
-
-def initialize_video_recorder(output_path, output_depth_path, fps, shape):
- """Initialize OpenCV video recorders that will be used to write each image/frame to a single video
-
- Args:
- :output (str): The file location where the recorded video will be saved
- :output_depth (str): The file location where the recorded video with depth information will be saved
- :fps (int): The frame per seconds of the output videos
- :shape (tuple): The dimension of the output video (width, height)
-
- Returns:
- :writer (cv2.VideoWriter): The video writer used to save the video
- :writer_depth (cv2.VideoWriter): The video writer used to save the video with depth information
- """
-
- if not os.path.isdir(os.path.split(output_path)[0]):
- logger.error("Invalid path for the video writer; folder does not exist")
- exit(1)
-
- fourcc = cv2.VideoWriter_fourcc(*"MJPG")
- writer = cv2.VideoWriter(output_path, fourcc, fps, shape, True)
- writer_depth = None
-
- if output_depth_path:
- if not os.path.isdir(os.path.split(output_depth_path)[0]):
- logger.error("Invalid path for the depth video writer; folder does not exist")
- exit(1)
- writer_depth = cv2.VideoWriter(output_depth_path, fourcc, fps, shape, True)
-
- return writer, writer_depth
-
-
-def delete_items_from_array_aux(arr, i):
- """
- Auxiliary function that delete the item at a certain index from a numpy array
-
- Args:
- :arr (numpy.ndarray): Array of array where each element correspond to the four coordinates of bounding box expressed in percentage
- :i (int): Index of the element to be deleted
-
- Returns:
- :arr_ret: the array without the element at index i
- """
-
- aux = arr.tolist()
- aux.pop(i)
- arr_ret = np.array(aux)
- return arr_ret
-
-
-def fit_plane_least_square(xyz):
- # find a plane that best fit xyz points using least squares
- (rows, cols) = xyz.shape
- g = np.ones((rows, 3))
- g[:, 0] = xyz[:, 0] # X
- g[:, 1] = xyz[:, 1] # Y
- z = xyz[:, 2]
- (a, b, c), _, rank, s = np.linalg.lstsq(g, z, rcond=None)
-
- normal = (a, b, -1)
- nn = np.linalg.norm(normal)
- normal = normal / nn
- point = np.array([0.0, 0.0, c])
- d = -point.dot(normal)
- return d, normal, point
-
-
-#
-# def plot_plane(data, normal, d):
-# from mpl_toolkits.mplot3d import Axes3D
-# import matplotlib.pyplot as plt
-#
-# fig = plt.figure()
-# ax = fig.gca(projection='3d')
-#
-# # plot fitted plane
-# maxx = np.max(data[:, 0])
-# maxy = np.max(data[:, 1])
-# minx = np.min(data[:, 0])
-# miny = np.min(data[:, 1])
-#
-# # compute needed points for plane plotting
-# xx, yy = np.meshgrid([minx - 10, maxx + 10], [miny - 10, maxy + 10])
-# z = (-normal[0] * xx - normal[1] * yy - d) * 1. / normal[2]
-#
-# # plot plane
-# ax.plot_surface(xx, yy, z, alpha=0.2)
-#
-# ax.set_xlabel('x')
-# ax.set_ylabel('y')
-# ax.set_zlabel('z')
-# plt.show()
-#
-# return
-
-
-def shape_to_np(shape, dtype="int"):
- """
- Function used for the dlib facial detector; it determine the facial landmarks for the face region, then convert the facial landmark
- (x, y)-coordinates to a NumPy array
-
- Args:
- :shape ():
- :dtype ():
- (Default is "int")
-
- Returns:
- :coordinates (list): list of x, y coordinates
- """
- # initialize the list of (x, y)-coordinates
- coordinates = np.zeros((68, 2), dtype=dtype)
- # loop over the 68 facial landmarks and convert them to a 2-tuple of (x, y)-coordinates
- for i in range(0, 68):
- coordinates[i] = (shape.part(i).x, shape.part(i).y)
- # return the list of (x, y)-coordinates
- return coordinates
-
-
-def rect_to_bb(rect):
- """
- Function used for the dlib facial detector; it converts dlib's rectangle to a tuple (x, y, w, h) where x and y represent xmin and ymin
- coordinates while w and h represent the width and the height
-
- Args:
- :rect (dlib.rectangle): dlib rectangle object that represents the region of the image where a face is detected
-
- Returns:
- :res (tuple): tuple that represents the region of the image where a face is detected in the form x, y, w, h
- """
- # take a bounding predicted by dlib and convert it to the format (x, y, w, h) as we would normally do with OpenCV
- x = rect.left()
- y = rect.top()
- w = rect.right() - x
- h = rect.bottom() - y
- # return a tuple of (x, y, w, h)
- res = x, y, w, h
- return res
-
-
-def enlarge_bb(y_min, x_min, y_max, x_max, im_width, im_height):
- """
- Enlarge the bounding box to include more background margin (used for face detection)
-
- Args:
- :y_min (int): the top y coordinate of the bounding box
- :x_min (int): the left x coordinate of the bounding box
- :y_max (int): the bottom y coordinate of the bounding box
- :x_max (int): the right x coordinate of the bounding box
- :im_width (int): The width of the image
- :im_height (int): The height of the image
-
- Returns:
- :y_min (int): the top y coordinate of the bounding box after enlarging
- :x_min (int): the left x coordinate of the bounding box after enlarging
- :y_max (int): the bottom y coordinate of the bounding box after enlarging
- :x_max (int): the right x coordinate of the bounding box after enlarging
- """
-
- y_min = int(max(0, y_min - abs(y_min - y_max) / 10))
- y_max = int(min(im_height, y_max + abs(y_min - y_max) / 10))
- x_min = int(max(0, x_min - abs(x_min - x_max) / 5))
- x_max = int(min(im_width, x_max + abs(x_min - x_max) / 4)) # 5
- x_max = int(min(x_max, im_width))
- return y_min, x_min, y_max, x_max
-
-
-def linear_assignment(cost_matrix):
- try:
- import lap
- _, x, y = lap.lapjv(cost_matrix, extend_cost=True)
- return np.array([[y[i], i] for i in x if i >= 0])
- except ImportError:
- from scipy.optimize import linear_sum_assignment
- x, y = linear_sum_assignment(cost_matrix)
- return np.array(list(zip(x, y)))
-
-
-def iou_batch(bb_test, bb_gt):
- """
- From SORT: Computes IUO between two bboxes in the form [x1,y1,x2,y2]
-
- Args:
- :bb_test ():
- :bb_gt ():
-
- Returns:
-
- """
- # print(bb_test, bb_gt)
- bb_gt = np.expand_dims(bb_gt, 0)
- bb_test = np.expand_dims(bb_test, 1)
-
- xx1 = np.maximum(bb_test[..., 0], bb_gt[..., 0])
- yy1 = np.maximum(bb_test[..., 1], bb_gt[..., 1])
- xx2 = np.minimum(bb_test[..., 2], bb_gt[..., 2])
- yy2 = np.minimum(bb_test[..., 3], bb_gt[..., 3])
- w = np.maximum(0., xx2 - xx1)
- h = np.maximum(0., yy2 - yy1)
- wh = w * h
- o = wh / ((bb_test[..., 2] - bb_test[..., 0]) * (bb_test[..., 3] - bb_test[..., 1]) + (bb_gt[..., 2] - bb_gt[..., 0]) * (
- bb_gt[..., 3] - bb_gt[..., 1]) - wh)
- return o
-
-
-def convert_bbox_to_z(bbox):
- """
- Takes a bounding box in the form [x1,y1,x2,y2] and returns z in the form [x,y,s,r] where x,y is the centre of the box and s is the scale/area and r is
- the aspect ratio
-
- Args:
- :bbox ():
-
- Returns:
-
- """
- w = bbox[2] - bbox[0]
- h = bbox[3] - bbox[1]
- x = bbox[0] + w / 2.
- y = bbox[1] + h / 2.
- s = w * h # scale is just area
- r = w / float(h) if float(h) != 0 else w
- return np.array([x, y, s, r]).reshape((4, 1))
-
-
-def convert_x_to_bbox(x, score=None):
- """
- Takes a bounding box in the centre form [x,y,s,r] and returns it in the form
- [x1,y1,x2,y2] where x1,y1 is the top left and x2,y2 is the bottom right
-
- Args:
- :x ():
- :score ():
- (Default is None)
-
- Returns:
-
- """
- w = np.sqrt(x[2] * x[3])
- h = x[2] / w
- if score is None:
- return np.array([x[0] - w / 2., x[1] - h / 2., x[0] + w / 2., x[1] + h / 2.]).reshape((1, 4))
- else:
- return np.array([x[0] - w / 2., x[1] - h / 2., x[0] + w / 2., x[1] + h / 2., score]).reshape((1, 5))
-
-
-def associate_detections_to_trackers(detections, trackers, iou_threshold=0.3):
- """
- Assigns detections to tracked object (both represented as bounding boxes)
- Returns 3 lists of matches, unmatched_detections and unmatched_trackers
-
- Args:
- :detections ():
- :trackers ():
- :iou_threshold ():
- (Default is 0.3)
-
- Returns:
-
- """
- if len(trackers) == 0:
- return np.empty((0, 2), dtype=int), np.arange(len(detections)), np.empty((0, 5), dtype=int)
-
- iou_matrix = iou_batch(detections, trackers)
- # print("IOU MATRIX: ", iou_matrix)
-
- if min(iou_matrix.shape) > 0:
- a = (iou_matrix > iou_threshold).astype(np.int32)
- if a.sum(1).max() == 1 and a.sum(0).max() == 1:
- matched_indices = np.stack(np.where(a), axis=1)
- else:
- matched_indices = linear_assignment(-iou_matrix)
- else:
- matched_indices = np.empty(shape=(0, 2))
-
- unmatched_detections = []
- for d, det in enumerate(detections):
- if d not in matched_indices[:, 0]:
- unmatched_detections.append(d)
- unmatched_trackers = []
- for t, trk in enumerate(trackers):
- if t not in matched_indices[:, 1]:
- unmatched_trackers.append(t)
-
- # filter out matched with low IOU
- matches = []
- for m in matched_indices:
- if iou_matrix[m[0], m[1]] < iou_threshold:
- unmatched_detections.append(m[0])
- unmatched_trackers.append(m[1])
- else:
- matches.append(m.reshape(1, 2))
- if len(matches) == 0:
- matches = np.empty((0, 2), dtype=int)
- else:
- matches = np.concatenate(matches, axis=0)
-
- return matches, np.array(unmatched_detections), np.array(unmatched_trackers)
-
-
-def find_face_from_key_points(key_points, bboxes, image, person=None, openpose=False, gazefollow=True):
- """
-
- Args:
- key_points:
- bboxes:
- image:
- person:
- openpose:
- gazefollow:
-
- Returns:
-
- """
-
- im_width, im_height = image.shape[1], image.shape[0]
-
- # key_points, bboxes = person.get_key_points()[-1], person.get_bboxes()[-1]
- # print("PERSON ID:", person.get_id())
-
- # 0 nose, 1/2 left/right eye, 3/4 left/right ear
- # 5/6 leftShoulder/rightShoulder
- # 7/8 leftElbow/rightElbow
- # 9/10 leftWrist/rightWrist
- # 11/12 leftHip/rightHip
- # 13/14 leftKnee/rightKnee
- # 15/16 leftAnkle/rightAnkle
- # print(key_points)
-
- face_points = key_points[:7]
-
- if openpose:
- face_points = []
- for point in key_points[:7]:
- # print(point[2], type(point[2]))
- if point[2] > 0.0:
- face_points.append(point)
- # print("face1", face_points)
-
- if len(face_points) == 0:
- return None, []
-
- # print("bboxe", bboxes, face_points)
- if not gazefollow:
- ct = compute_centroid(face_points)
-
- x_min, y_min = ct[0] - 10, ct[1] - 15
- x_max, y_max = ct[0] + 10, ct[1] + 10
-
- y_min_bbox = y_min
-
- elif gazefollow:
- # [l_shoulder, r_shoulder] = key_points[5:]
- # print(l_shoulder, r_shoulder)
- print("FACE", face_points)
- if len(face_points) == 1:
- return None, []
-
- x_min, y_min, _ = np.amin(face_points, axis=0)
- x_max, y_max, _ = np.amax(face_points, axis=0)
-
- # aux_diff =
- # print("X: ", aux_diff)
- # if aux_diff < 20:
- # x_max += 20
- # x_min -= 20
-
- aux_diff = y_max - y_min
- print("y: ", aux_diff)
- if aux_diff < 50: # rapporto xmax -xmin o altro
- y_max += (x_max - x_min) / 1.4
- y_min -= (x_max - x_min) / 1.2
- # x_min -= 10
- # x_max += 10
-
- y_min_bbox = int(y_min) # int(bboxes[1]) if bboxes is not None else y_min - (x_max-x_min)
- # if bboxes is None:
- # y_max = y_max + (x_max-x_min)
-
- y_min, x_min, y_max, x_max = enlarge_bb(y_min_bbox, x_min, y_max, x_max, im_width, im_height)
- # print(y_min, x_min, y_max, x_max, y_max - y_min, x_max - x_min)
- # if -1 < y_max - y_min < 5 and -1 < x_max - x_min < 5: # due punti uguali
- # # print("AAAAA")
- # return None, []
-
- face_image = image[y_min:y_max, x_min:x_max]
-
- if person is not None:
- # person.print_()
- person.update_faces(face_image)
- person.update_faces_coordinates([y_min, x_min, y_max, x_max])
- # person.update_faces_key_points(face_points)
- # person.print_()
- return None
- else:
- return face_image, [y_min, x_min, y_max, x_max]
-
-
-def compute_interaction_cosine(head_position, target_position, gaze_direction):
- """
- Computes the interaction between two people using the angle of view.
- The interaction in measured as the cosine of the angle formed by the line from person A to B and the gaze direction of person A.
-
- Args:
- :head_position (list): list of pixel coordinates [x, y] that represents the position of the head of person A
- :target_position (list): list of pixel coordinates [x, y] that represents the position of head of person B
- :gaze_direction (list): list that represents the gaze direction of the head of person A in the form [gx, gy]
-
- Returns:
- :val (float): value that describe the quantity of interaction
- """
-
- if head_position == target_position:
- return 0 # or -1
- else:
- # direction from observer to target
- direction = np.arctan2((target_position[1] - head_position[1]), (target_position[0] - head_position[0]))
- direction_gaze = np.arctan2(gaze_direction[1], gaze_direction[0])
- difference = direction - direction_gaze
-
- # difference of the line joining observer -> target with the gazing direction,
- val = np.cos(difference)
- if val < 0:
- return 0
- else:
- return val
-
-
-def compute_attention_from_vectors(list_objects):
- """
-
- Args:
- :list_objects ():
-
- Returns:
-
- """
-
- dict_person = dict()
- id_list = []
- for obj in list_objects:
- if len(obj.get_key_points()) > 0:
- # print("Object ID: ", obj.get_id(), "x: ", obj.get_poses_vector_norm()[-1][0], "y: ", obj.get_poses_vector_norm()[-1][1])
- id_list.append(obj.get_id())
-
- # print("kpts: ", obj.get_key_points()[-1])
- aux = [obj.get_key_points()[-1][j][:2] for j in [0, 2, 1, 4, 3]]
- dict_person[obj.get_id()] = [obj.get_poses_vector_norm()[-1], np.mean(aux, axis=0).tolist()]
-
- attention_matrix = np.zeros((len(dict_person), len(dict_person)), dtype=np.float32)
-
- for i in range(attention_matrix.shape[0]):
- for j in range(attention_matrix.shape[1]):
- if i == j:
- continue
- attention_matrix[i][j] = compute_interaction_cosine(dict_person[i][1], dict_person[j][1], dict_person[i][0])
-
- return attention_matrix.tolist(), id_list
-
-
-def compute_attention_ypr(list_objects):
- """
-
- Args:
- :list_objects ():
-
- Returns:
- :
- """
-
- for obj in list_objects:
- if len(obj.get_key_points()) > 0:
- print("Object ID: ", obj.get_id(), "yaw: ", obj.get_poses_ypr()[-1][0], "pitch: ", obj.get_poses_ypr()[-1][1], "roll: ",
- obj.get_poses_ypr()[-1][2])
-
-
-def save_key_points_to_json(ids, kpts, path_json, openpose=False):
- """
- Save key points to .json format according to Openpose output format
-
- Args:
- :kpts ():
- :path_json ():
-
- Returns:
- """
-
- # print(path_json)
- dict_file = {"version": 1.3}
- list_dict_person = []
- for j in range(len(kpts)):
- dict_person = {"person_id": [int(ids[j])],
- "face_keypoints_2d": [],
- "hand_left_keypoints_2d": [],
- "hand_right_keypoints_2d": [],
- "pose_keypoints_3d": [],
- "face_keypoints_3d": [],
- "hand_left_keypoints_3d": [],
- "hand_right_keypoints_3d": []}
-
- kpts_openpose = np.zeros((25, 3))
- for i, point in enumerate(kpts[j]):
- if openpose:
- idx_op = rev_pose_id_part_openpose[pose_id_part_openpose[i]]
- else:
- idx_op = rev_pose_id_part_openpose[pose_id_part[i]]
- # print(idx_op, point[1], point[0], point[2])
- kpts_openpose[idx_op] = [point[1], point[0], point[2]] # x, y, conf
-
- list_kpts_openpose = list(np.concatenate(kpts_openpose).ravel())
- dict_person["pose_keypoints_2d"] = list_kpts_openpose
- # print(dict_person)
- list_dict_person.append(dict_person)
-
- dict_file["people"] = list_dict_person
-
- # Serializing json
- json_object = json.dumps(dict_file, indent=4)
-
- # Writing to sample.json
- with open(path_json, "w") as outfile:
- outfile.write(json_object)
-
-
-def json_to_poses(json_data):
- """
-
- Args:
- :js_data ():
-
- Returns:
- :res ():
- """
- poses = []
- confidences = []
- ids = []
-
- for arr in json_data["people"]:
- ids.append(arr["person_id"])
- confidences.append(arr["pose_keypoints_2d"][2::3])
- aux = arr["pose_keypoints_2d"][2::3]
- arr = np.delete(arr["pose_keypoints_2d"], slice(2, None, 3))
- # print("B", list(zip(arr[::2], arr[1::2])))
- poses.append(list(zip(arr[::2], arr[1::2], aux)))
-
- return poses, confidences, ids
-
-
-def parse_json1(aux):
- # print(aux['people'])
- list_kpts = []
- id_list = []
- for person in aux['people']:
- # print(len(person['pose_keypoints_2d']))
- aux = person['pose_keypoints_2d']
- aux_kpts = [[aux[i+1], aux[i], aux[i+2]] for i in range(0, 75, 3)]
- # print(len(aux_kpts))
- list_kpts.append(aux_kpts)
- id_list.append(person['person_id'])
-
- # print(list_kpts)
- return list_kpts, id_list
-
-
-def load_poses_from_json1(json_filename):
- """
-
- Args:
- :json_filename ():
-
- Returns:
- :poses, conf:
- """
- with open(json_filename) as data_file:
- loaded = json.load(data_file)
- zz = parse_json1(loaded)
- return zz
-
-
-def load_poses_from_json(json_filename):
- """
-
- Args:
- :json_filename ():
-
- Returns:
- :poses, conf:
- """
- with open(json_filename) as data_file:
- loaded = json.load(data_file)
- poses, conf, ids = json_to_poses(loaded)
-
- if len(poses) < 1: # != 1:
- return None, None, None
- else:
- return poses, conf, ids
-
-
-def compute_head_features(img, pose, conf, open_pose=True):
- """
-
- Args:
- img:
- pose:
- conf:
- open_pose:
-
- Returns:
-
- """
-
- joints = [0, 15, 16, 17, 18] if open_pose else [0, 2, 1, 4, 3]
-
- n_joints_set = [pose[joint] for joint in joints if joint_set(pose[joint])] # if open_pose else pose
-
- if len(n_joints_set) < 1:
- return None, None
-
- centroid = compute_centroid(n_joints_set)
-
- # for j in n_joints_set:
- # print(j, centroid)
- max_dist = max([dist_2D([j[0], j[1]], centroid) for j in n_joints_set])
-
- new_repr = [(np.array([pose[joint][0], pose[joint][1]]) - np.array(centroid)) for joint in joints] if open_pose else [
- (np.array(pose[i]) - np.array(centroid)) for i in range(len(n_joints_set))]
- result = []
-
- for i in range(0, 5):
-
- if joint_set(pose[joints[i]]):
- if max_dist != 0.0:
- result.append([new_repr[i][0] / max_dist, new_repr[i][1] / max_dist])
- else:
- result.append([new_repr[i][0], new_repr[i][1]])
- else:
- result.append([0, 0])
-
- flat_list = [item for sublist in result for item in sublist]
-
- conf_list = []
-
- for j in joints:
- conf_list.append(conf[j])
-
- return flat_list, conf_list, centroid
-
-
-def compute_body_features(pose, conf):
- """
-
- Args:
- pose:
- conf:
-
- Returns:
-
- """
- joints = [0, 15, 16, 17, 18]
- alljoints = range(0, 25)
-
- n_joints_set = [pose[joint] for joint in joints if joint_set(pose[joint])]
-
- if len(n_joints_set) < 1:
- return None, None
-
- centroid = compute_centroid(n_joints_set)
-
- n_joints_set = [pose[joint] for joint in alljoints if joint_set(pose[joint])]
-
- max_dist = max([dist_2D(j, centroid) for j in n_joints_set])
-
- new_repr = [(np.array(pose[joint]) - np.array(centroid)) for joint in alljoints]
-
- result = []
-
- for i in range(0, 25):
-
- if joint_set(pose[i]):
- result.append([new_repr[i][0] / max_dist, new_repr[i][1] / max_dist])
- else:
- result.append([0, 0])
-
- flat_list = [item for sublist in result for item in sublist]
-
- for j in alljoints:
- flat_list.append(conf[j])
-
- return flat_list, centroid
-
-
-def compute_centroid(points):
- """
-
- Args:
- points:
-
- Returns:
-
- """
- x, y = [], []
- for point in points:
- if len(point) == 3:
- if point[2] > 0.0:
- x.append(point[0])
- y.append(point[1])
- else:
- x.append(point[0])
- y.append(point[1])
-
- # print(x, y)
- if x == [] or y == []:
- return [None, None]
- mean_x = np.mean(x)
- mean_y = np.mean(y)
-
- return [mean_x, mean_y]
-
-
-def joint_set(p):
- """
-
- Args:
- p:
-
- Returns:
-
- """
- return p[0] != 0.0 or p[1] != 0.0
-
-
-def dist_2D(p1, p2):
- """
-
- Args:
- p1:
- p2:
-
- Returns:
-
- """
- # print(p1)
- # print(p2)
-
- p1 = np.array(p1)
- p2 = np.array(p2)
-
- squared_dist = np.sum((p1 - p2) ** 2, axis=0)
- return np.sqrt(squared_dist)
-
-
-def compute_head_centroid(pose):
- """
-
- Args:
- pose:
-
- Returns:
-
- """
- joints = [0, 15, 16, 17, 18]
-
- n_joints_set = [pose[joint] for joint in joints if joint_set(pose[joint])]
-
- # if len(n_joints_set) < 2:
- # return None
-
- centroid = compute_centroid(n_joints_set)
-
- return centroid
-
-
-def head_direction_to_json(path_json, norm_list, unc_list, ids_list, file_name):
-
- dict_file = {}
- list_dict_person = []
- for k, i in enumerate(norm_list):
- dict_person = {"id_person": [ids_list[k]],
- "norm_xy": [i[0][0].item(), i[0][1].item()], # from numpy to native python type for json serilization
- "center_xy": [int(i[1][0]), int(i[1][1])],
- "uncertainty": [unc_list[k].item()]}
-
- list_dict_person.append(dict_person)
- dict_file["people"] = list_dict_person
-
- json_object = json.dumps(dict_file, indent=4)
-
- with open(path_json, "w") as outfile:
- outfile.write(json_object)
-
-
-def ypr_to_json(path_json, yaw_list, pitch_list, roll_list, yaw_u_list, pitch_u_list, roll_u_list, ids_list, center_xy):
-
- dict_file = {}
- list_dict_person = []
- for k in range(len(yaw_list)):
- dict_person = {"id_person": [ids_list[k]],
- "yaw": [yaw_list[k].item()],
- "yaw_u": [yaw_u_list[k].item()],
- "pitch": [pitch_list[k].item()],
- "pitch_u": [pitch_u_list[k].item()],
- "roll": [roll_list[k].item()],
- "roll_u": [roll_u_list[k].item()],
- "center_xy": [int(center_xy[k][0]), int(center_xy[k][1])]}
-
- list_dict_person.append(dict_person)
- dict_file["people"] = list_dict_person
-
- json_object = json.dumps(dict_file, indent=4)
-
- with open(path_json, "w") as outfile:
- outfile.write(json_object)
- # exit()
-
-
-def save_keypoints_image(img, poses, suffix_, path_save=''):
- """
- Save the image with the key points drawn on it
- Args:
- img:
- poses:
- suffix_:
-
- Returns:
-
- """
- aux = img.copy()
- for point in poses:
- for i, p in enumerate(point):
- if i in [0, 15, 16, 17, 18]:
- cv2.circle(aux, (int(p[0]), int(p[1])), 2, (0, 255, 0), 2)
-
- cv2.imwrite(os.path.join(path_save, suffix_ + '.jpg'), aux)
-
-
-def unit_vector(vector):
- """
- Returns the unit vector of the vector.
-
- Args:
- vector:
-
- Returns:
-
- """
- return vector / np.linalg.norm(vector)
-
-
-def angle_between(v1, v2):
- """
- Returns the angle in radians between vectors 'v1' and 'v2'::
-
- angle_between((1, 0, 0), (0, 1, 0))
- 1.5707963267948966
- angle_between((1, 0, 0), (1, 0, 0))
- 0.0
- angle_between((1, 0, 0), (-1, 0, 0))
- 3.141592653589793
- """
- # if not unit vector
- v1_u = unit_vector(tuple(v1))
- v2_u = unit_vector(tuple(v2))
- angle = np.arccos(np.clip(np.dot(v1_u, v2_u), -1.0, 1.0))
- return angle if angle < 1.80 else angle - 1.80
-
-
-def centroid_constraint(centroid, centroid_det, gazefollow=False): # x y
- """
-
- Args:
- centroid:
- centroid_det:
-
- Returns:
-
- """
- if centroid_det == [None, None]:
- return False
-
- if gazefollow == False:
- if 0 < centroid_det[0] < 143 and 0 < centroid_det[1] < 24: # centroid in the overprinted text of hour in the video
- return False
- if 0 < centroid_det[1] < 4:
- return False
- if centroid[0] - 3 < centroid_det[0] < centroid[0] + 3 and centroid[1] - 3 < centroid_det[1] < centroid[
- 1] + 3: # detected centroid near the gt centroid
- return True
- else:
- return False
- else:
- if int(centroid[0] - 30) < int(centroid_det[0]) < int(centroid[0] + 30) and int(centroid[1] - 30) < int(centroid_det[1]) < int(
- centroid[1] + 30): # detected centroid near the gt centroid
- return True
- else:
- return False
-
-
-def initialize_video_reader(path_video):
- """
-
- Args:
- path_video:
-
- Returns:
-
- """
- cap = cv2.VideoCapture(path_video)
- if cap is None or not cap.isOpened():
- print('Warning: unable to open video source: ', path_video)
- exit(-1)
- return cap
-
-
-def distance_skeletons(kpts1, kpts2, dst_type):
- """
- Function to compute the distance between skeletons
- #TO DO
- Args:
- kpts1:
- kpts2:
- dts_type:
-
- Returns:
-
- """
- if len(kpts1) != len(kpts2):
- print("Error: Different notation used for keypoints")
- exit(-1)
-
- print(len(kpts1), len(kpts2))
- # to openpose notations
- if len(kpts1) == len(kpts2) == 17:
- kpts1, kpts2 = kpt_centernet_to_openpose(kpts1), kpt_centernet_to_openpose(kpts2)
- print(len(kpts1), len(kpts2))
-
- if len(kpts1) != 25 or len(kpts2) != 25:
- print("Error")
- exit(-1)
-
- res_dist = 0
-
- if dst_type == 'all_points':
- for i, _ in enumerate(kpts1):
- res_dist += dist_2D(kpts1[i][:2], kpts2[i][:2])
- res_dist /= 25
- return res_dist
-
- elif dst_type == 'head_centroid':
- top1_c, top2_c = compute_head_centroid(kpts1), compute_head_centroid(kpts2)
- if top1_c == [None, None] or top2_c == [None, None]:
- res_dist = 900
- else:
- res_dist = dist_2D(top1_c[:2], top2_c[:2])
- return res_dist
-
- elif dst_type == 'three_centroids':
- #TO DO
- # top1_c, top2_c = compute_centroid(kpts1[0, 15, 16, 17, 18]), compute_centroid(kpts2[0, 15, 16, 17, 18])
- # mid1_c, mid2_c = compute_centroid(kpts1[2, 5, 9, 12]), compute_centroid(kpts2[2, 5, 9, 12])
- # btm1_c, btm2_c = compute_centroid(kpts1[9, 12, 10, 13]), compute_centroid(kpts2[9, 12, 10, 13])
- # res_dist = dist_2D(top1_c[:2], top2_c[:2]) + dist_2D(mid1_c[:2], mid2_c[:2]) + dist_2D(btm1_c[:2], btm2_c[:2])
- # res_dist /= 3
- # return res_dist
- return None
-
- elif dst_type == '':
- print("dst_typ not valid")
- exit(-1)
-
-
-def kpt_openpose_to_centernet(kpts):
- """
-
- Args:
- kpts:
-
- Returns:
-
- """
- #TO TEST
- kpts_openpose = np.zeros((16, 3))
- for i, point in enumerate(kpts):
- idx_op = rev_pose_id_part[pose_id_part_openpose[i]]
- kpts_openpose[idx_op] = [point[0], point[1], point[2]]
-
- return kpts_openpose
-
-
-def kpt_centernet_to_openpose(kpts):
- """
-
- Args:
- kpts:
-
- Returns:
-
- """
- #TO TEST
- kpts_openpose = np.zeros((25, 3))
- for i, point in enumerate(kpts):
- idx_op = rev_pose_id_part_openpose[pose_id_part[i]]
- kpts_openpose[idx_op] = [point[1], point[0], point[2]]
-
- return kpts_openpose
-
-
-def non_maxima_aux(det, kpt, threshold=15): # threshold in pxels
- # print("A", kpt, "\n", len(kpt))
-
- indexes_to_delete = []
-
- if len(kpt) == 0 or len(det) == 0:
- return [], []
-
- if len(kpt) == 1 or len(det) == 1:
- return det, kpt
-
- kpt_res = kpt.copy()
- det_res_aux = det.copy()
-
- for i in range(0, len(kpt)):
- for j in range(i, len(kpt)):
- if i == j:
- continue
- dist = distance_skeletons(kpt[i], kpt[j], 'head_centroid')
- # print("DIST", i, j, dist)
- if dist < threshold:
- if j not in indexes_to_delete:
- indexes_to_delete.append(j)
- # kpt_res.pop(j)
- det_res = []
-
- # print(indexes_to_delete)
- indexes_to_delete = sorted(indexes_to_delete, reverse=True)
- # print(len(kpt_res))
- for index in indexes_to_delete:
- kpt_res.pop(index)
-
- det_res_aux = list(np.delete(det_res_aux, indexes_to_delete, axis=0))
- det_res = np.array(det_res_aux)
-
- return det_res, kpt_res
-
-
-def compute_centroid_list(points):
- """
-
- Args:
- points:
-
- Returns:
-
- """
- x, y = [], []
- for i in range(0, len(points), 3):
- if points[i + 2] > 0.0: # confidence openpose
- x.append(points[i])
- y.append(points[i + 1])
-
- if x == [] or y == []:
- return [None, None]
- mean_x = np.mean(x)
- mean_y = np.mean(y)
-
- return [mean_x, mean_y]
-
-
-def normalize_wrt_maximum_distance_point(points, file_name=''):
- centroid = compute_centroid_list(points)
- # centroid = [points[0], points[1]]
- # print(centroid)
- # exit()
-
- max_dist_x, max_dist_y = 0, 0
- for i in range(0, len(points), 3):
- if points[i + 2] > 0.0: # confidence openpose take only valid keypoints (if not detected (0, 0, 0)
- distance_x = abs(points[i] - centroid[0])
- distance_y = abs(points[i+1] - centroid[1])
- # dist_aux.append(distance)
- if distance_x > max_dist_x:
- max_dist_x = distance_x
- if distance_y > max_dist_y:
- max_dist_y = distance_y
- elif points[i + 2] == 0.0: # check for centernet people on borders with confidence 0
- points[i] = 0
- points[i+1] = 0
-
- for i in range(0, len(points), 3):
- if points[i + 2] > 0.0:
- if max_dist_x != 0.0:
- points[i] = (points[i] - centroid[0]) / max_dist_x
- if max_dist_y != 0.0:
- points[i + 1] = (points[i + 1] - centroid[1]) / max_dist_y
- if max_dist_x == 0.0: # only one point valid with some confidence value so it become (0,0, confidence)
- points[i] = 0.0
- if max_dist_y == 0.0:
- points[i + 1] = 0.0
-
- return points
-
-
-def retrieve_interest_points(kpts, detector):
- """
-
- :param kpts:
- :return:
- """
- res_kpts = []
-
- if detector == 'centernet':
- face_points = [0, 1, 2, 3, 4]
- for index in face_points:
- res_kpts.append(kpts[index][1])
- res_kpts.append(kpts[index][0])
- res_kpts.append(kpts[index][2])
- elif detector== 'zedcam':
- face_points = [0, 14, 15, 16, 17]
- for index in face_points:
- res_kpts.append(kpts[index][0])
- res_kpts.append(kpts[index][1])
- res_kpts.append(kpts[index][2])
- else:
- # take only interest points (5 points of face)
- face_points = [0, 16, 15, 18, 17]
- for index in face_points:
- res_kpts.append(kpts[index][0])
- res_kpts.append(kpts[index][1])
- res_kpts.append(kpts[index][2])
-
-
-
- return res_kpts
-
-def create_bbox_from_openpose_keypoints(data):
- # from labels import pose_id_part_openpose
- bbox = list()
- ids = list()
- kpt = list()
- kpt_scores = list()
- for person in data['people']:
- ids.append(person['person_id'][0])
- kpt_temp = list()
- kpt_score_temp = list()
- # create bbox with min max each dimension
- x, y = [], []
- for i in pose_id_part_openpose:
- if i < 25:
- # kpt and kpts scores
- kpt_temp.append([int(person['pose_keypoints_2d'][i * 3]), int(person['pose_keypoints_2d'][(i * 3) + 1]),
- person['pose_keypoints_2d'][(i * 3) + 2]])
- kpt_score_temp.append(person['pose_keypoints_2d'][(i * 3) + 2])
- # check confidence != 0
- if person['pose_keypoints_2d'][(3 * i) + 2]!=0:
- x.append(int(person['pose_keypoints_2d'][3 * i]))
- y.append(int(person['pose_keypoints_2d'][(3 * i) + 1]))
- kpt_scores.append(kpt_score_temp)
- kpt.append(kpt_temp)
- xmax = max(x)
- xmin = min(x)
- ymax = max(y)
- ymin = min(y)
- bbox.append([xmin, ymin, xmax, ymax, 1]) # last value is for compatibility of centernet
-
- return bbox, kpt, kpt_scores # not to use scores
-
-def atoi(text):
- return int(text) if text.isdigit() else text
-
-
-def natural_keys(text):
- """
- alist.sort(key=natural_keys) sorts in human order
- http://nedbatchelder.com/blog/200712/human_sorting.html
- (See Toothy's implementation in the comments)
- """
- import re
- return [atoi(c) for c in re.split(r'(\d+)', text)]
\ No newline at end of file
diff --git "a/spaces/Fengbinbin/gpt-academic/crazy_functions/\346\200\273\347\273\223word\346\226\207\346\241\243.py" "b/spaces/Fengbinbin/gpt-academic/crazy_functions/\346\200\273\347\273\223word\346\226\207\346\241\243.py"
deleted file mode 100644
index f1fe20171cc54aec0c79f4961e71b57845f252d5..0000000000000000000000000000000000000000
--- "a/spaces/Fengbinbin/gpt-academic/crazy_functions/\346\200\273\347\273\223word\346\226\207\346\241\243.py"
+++ /dev/null
@@ -1,127 +0,0 @@
-from toolbox import update_ui
-from toolbox import CatchException, report_execption, write_results_to_file
-from .crazy_utils import request_gpt_model_in_new_thread_with_ui_alive
-fast_debug = False
-
-
-def 解析docx(file_manifest, project_folder, llm_kwargs, plugin_kwargs, chatbot, history, system_prompt):
- import time, os
- # pip install python-docx 用于docx格式,跨平台
- # pip install pywin32 用于doc格式,仅支持Win平台
- for index, fp in enumerate(file_manifest):
- if fp.split(".")[-1] == "docx":
- from docx import Document
- doc = Document(fp)
- file_content = "\n".join([para.text for para in doc.paragraphs])
- else:
- import win32com.client
- word = win32com.client.Dispatch("Word.Application")
- word.visible = False
- # 打开文件
- print('fp', os.getcwd())
- doc = word.Documents.Open(os.getcwd() + '/' + fp)
- # file_content = doc.Content.Text
- doc = word.ActiveDocument
- file_content = doc.Range().Text
- doc.Close()
- word.Quit()
-
- print(file_content)
- # private_upload里面的文件名在解压zip后容易出现乱码(rar和7z格式正常),故可以只分析文章内容,不输入文件名
- from .crazy_utils import breakdown_txt_to_satisfy_token_limit_for_pdf
- from request_llm.bridge_all import model_info
- max_token = model_info[llm_kwargs['llm_model']]['max_token']
- TOKEN_LIMIT_PER_FRAGMENT = max_token * 3 // 4
- paper_fragments = breakdown_txt_to_satisfy_token_limit_for_pdf(
- txt=file_content,
- get_token_fn=model_info[llm_kwargs['llm_model']]['token_cnt'],
- limit=TOKEN_LIMIT_PER_FRAGMENT
- )
- this_paper_history = []
- for i, paper_frag in enumerate(paper_fragments):
- i_say = f'请对下面的文章片段用中文做概述,文件名是{os.path.relpath(fp, project_folder)},文章内容是 ```{paper_frag}```'
- i_say_show_user = f'请对下面的文章片段做概述: {os.path.abspath(fp)}的第{i+1}/{len(paper_fragments)}个片段。'
- gpt_say = yield from request_gpt_model_in_new_thread_with_ui_alive(
- inputs=i_say,
- inputs_show_user=i_say_show_user,
- llm_kwargs=llm_kwargs,
- chatbot=chatbot,
- history=[],
- sys_prompt="总结文章。"
- )
-
- chatbot[-1] = (i_say_show_user, gpt_say)
- history.extend([i_say_show_user,gpt_say])
- this_paper_history.extend([i_say_show_user,gpt_say])
-
- # 已经对该文章的所有片段总结完毕,如果文章被切分了,
- if len(paper_fragments) > 1:
- i_say = f"根据以上的对话,总结文章{os.path.abspath(fp)}的主要内容。"
- gpt_say = yield from request_gpt_model_in_new_thread_with_ui_alive(
- inputs=i_say,
- inputs_show_user=i_say,
- llm_kwargs=llm_kwargs,
- chatbot=chatbot,
- history=this_paper_history,
- sys_prompt="总结文章。"
- )
-
- history.extend([i_say,gpt_say])
- this_paper_history.extend([i_say,gpt_say])
-
- res = write_results_to_file(history)
- chatbot.append(("完成了吗?", res))
- yield from update_ui(chatbot=chatbot, history=history) # 刷新界面
-
- res = write_results_to_file(history)
- chatbot.append(("所有文件都总结完成了吗?", res))
- yield from update_ui(chatbot=chatbot, history=history) # 刷新界面
-
-
-@CatchException
-def 总结word文档(txt, llm_kwargs, plugin_kwargs, chatbot, history, system_prompt, web_port):
- import glob, os
-
- # 基本信息:功能、贡献者
- chatbot.append([
- "函数插件功能?",
- "批量总结Word文档。函数插件贡献者: JasonGuo1"])
- yield from update_ui(chatbot=chatbot, history=history) # 刷新界面
-
- # 尝试导入依赖,如果缺少依赖,则给出安装建议
- try:
- from docx import Document
- except:
- report_execption(chatbot, history,
- a=f"解析项目: {txt}",
- b=f"导入软件依赖失败。使用该模块需要额外依赖,安装方法```pip install --upgrade python-docx pywin32```。")
- yield from update_ui(chatbot=chatbot, history=history) # 刷新界面
- return
-
- # 清空历史,以免输入溢出
- history = []
-
- # 检测输入参数,如没有给定输入参数,直接退出
- if os.path.exists(txt):
- project_folder = txt
- else:
- if txt == "": txt = '空空如也的输入栏'
- report_execption(chatbot, history, a=f"解析项目: {txt}", b=f"找不到本地项目或无权访问: {txt}")
- yield from update_ui(chatbot=chatbot, history=history) # 刷新界面
- return
-
- # 搜索需要处理的文件清单
- if txt.endswith('.docx') or txt.endswith('.doc'):
- file_manifest = [txt]
- else:
- file_manifest = [f for f in glob.glob(f'{project_folder}/**/*.docx', recursive=True)] + \
- [f for f in glob.glob(f'{project_folder}/**/*.doc', recursive=True)]
-
- # 如果没找到任何文件
- if len(file_manifest) == 0:
- report_execption(chatbot, history, a=f"解析项目: {txt}", b=f"找不到任何.docx或doc文件: {txt}")
- yield from update_ui(chatbot=chatbot, history=history) # 刷新界面
- return
-
- # 开始正式执行任务
- yield from 解析docx(file_manifest, project_folder, llm_kwargs, plugin_kwargs, chatbot, history, system_prompt)
diff --git a/spaces/Fr33d0m21/stabilityai-stable-diffusion-2-1/app.py b/spaces/Fr33d0m21/stabilityai-stable-diffusion-2-1/app.py
deleted file mode 100644
index 0160420876923d89f2ab5fccb9f4d13725e29972..0000000000000000000000000000000000000000
--- a/spaces/Fr33d0m21/stabilityai-stable-diffusion-2-1/app.py
+++ /dev/null
@@ -1,3 +0,0 @@
-import gradio as gr
-
-gr.Interface.load("models/stabilityai/stable-diffusion-2-1").launch()
\ No newline at end of file
diff --git a/spaces/FridaZuley/RVC_HFKawaii/diffq/utils.py b/spaces/FridaZuley/RVC_HFKawaii/diffq/utils.py
deleted file mode 100644
index be6ab5253c38564140bc202077292bb99f9f397b..0000000000000000000000000000000000000000
--- a/spaces/FridaZuley/RVC_HFKawaii/diffq/utils.py
+++ /dev/null
@@ -1,37 +0,0 @@
-# Copyright (c) Facebook, Inc. and its affiliates.
-# All rights reserved.
-#
-# This source code is licensed under the license found in the
-# LICENSE file in the root directory of this source tree.
-
-import inspect
-from typing import Optional, List
-
-
-def simple_repr(obj, attrs: Optional[List[str]] = None, overrides={}):
- """
- Return a simple representation string for `obj`.
- If `attrs` is not None, it should be a list of attributes to include.
- """
- params = inspect.signature(obj.__class__).parameters
- attrs_repr = []
- if attrs is None:
- attrs = params.keys()
- for attr in attrs:
- display = False
- if attr in overrides:
- value = overrides[attr]
- elif hasattr(obj, attr):
- value = getattr(obj, attr)
- else:
- continue
- if attr in params:
- param = params[attr]
- if param.default is inspect._empty or value != param.default:
- display = True
- else:
- display = True
-
- if display:
- attrs_repr.append(f"{attr}={value}")
- return f"{obj.__class__.__name__}({','.join(attrs_repr)})"
diff --git a/spaces/GAURAVBRAR/AIGK/README.md b/spaces/GAURAVBRAR/AIGK/README.md
deleted file mode 100644
index d7596128447263d958abb85ab6304adb6e225dbc..0000000000000000000000000000000000000000
--- a/spaces/GAURAVBRAR/AIGK/README.md
+++ /dev/null
@@ -1,12 +0,0 @@
----
-title: AIGK
-emoji: 🏃
-colorFrom: green
-colorTo: blue
-sdk: gradio
-sdk_version: 4.1.1
-app_file: app.py
-pinned: false
----
-
-Check out the configuration reference at https://huggingface.co/docs/hub/spaces-config-reference
diff --git a/spaces/GaenKoki/voicevox/get_cost_candidates.py b/spaces/GaenKoki/voicevox/get_cost_candidates.py
deleted file mode 100644
index 072c4b4d57a757c957a0a1e9ab0afb0c5c989cb0..0000000000000000000000000000000000000000
--- a/spaces/GaenKoki/voicevox/get_cost_candidates.py
+++ /dev/null
@@ -1,91 +0,0 @@
-"""
-voicevox_engine/part_of_speech_data.pyのcost_candidatesを計算するプログラムです。
-引数のnaist_jdic_pathには、open_jtalkのsrc/mecab-naist-jdic/naist-jdic.csvを指定してください。
-
-実行例:
-python get_cost_candidates.py --naist_jdic_path=/path/to/naist-jdic.csv \
- --pos=名詞 \
- --pos_detail_1=固有名詞 \
- --pos_detail_2=一般 \
- --pos_detail_3=*
-
-cost_candidatesの値の詳細は以下の通りです。
-- 1番目の値はnaist_jdic内の同一品詞の最小コストから1を引いたもの、11番目の値は最大コストに1を足したものです。
-- 2番目の値はnaist_jdic内の同一品詞のコストの下位1%、10番目の値は99%の値です。
-- 6番目の値はnaist_jdic内の同一品詞のコストの最頻値です。
-- 2番目から6番目、6番目から10番目までの値は一定割合で増加するようになっています。
-"""
-
-import argparse
-import statistics
-from pathlib import Path
-from typing import List
-
-import numpy as np
-
-
-def get_candidates(
- naist_jdic_path: Path,
- pos: str,
- pos_detail_1: str,
- pos_detail_2: str,
- pos_detail_3: str,
-) -> List[int]:
- costs = []
- with naist_jdic_path.open(encoding="utf-8") as f:
- for line in f:
- (
- _,
- _,
- _,
- _cost,
- _pos,
- _pos_detail_1,
- _pos_detail_2,
- _pos_detail_3,
- _,
- _,
- _,
- _,
- _,
- _,
- _,
- ) = line.split(",")
- if (_pos, _pos_detail_1, _pos_detail_2, _pos_detail_3) == (
- pos,
- pos_detail_1,
- pos_detail_2,
- pos_detail_3,
- ):
- costs.append(int(_cost))
- assert len(costs) > 0
- cost_min = min(costs) - 1
- cost_1per = np.quantile(costs, 0.01).astype(np.int64)
- cost_mode = statistics.mode(costs)
- cost_99per = np.quantile(costs, 0.99).astype(np.int64)
- cost_max = max(costs) + 1
- return (
- [cost_min]
- + [int(cost_1per + (cost_mode - cost_1per) * i / 4) for i in range(5)]
- + [int(cost_mode + (cost_99per - cost_mode) * i / 4) for i in range(1, 5)]
- + [cost_max]
- )
-
-
-if __name__ == "__main__":
- parser = argparse.ArgumentParser()
- parser.add_argument("--naist_jdic_path", type=Path)
- parser.add_argument("--pos", type=str)
- parser.add_argument("--pos_detail_1", type=str)
- parser.add_argument("--pos_detail_2", type=str)
- parser.add_argument("--pos_detail_3", type=str)
- args = parser.parse_args()
- print(
- get_candidates(
- naist_jdic_path=args.naist_jdic_path,
- pos=args.pos,
- pos_detail_1=args.pos_detail_1,
- pos_detail_2=args.pos_detail_2,
- pos_detail_3=args.pos_detail_3,
- )
- )
diff --git a/spaces/Gen-Sim/Gen-Sim/cliport/generated_tasks/color_coordinated_cylinder_ball_match.py b/spaces/Gen-Sim/Gen-Sim/cliport/generated_tasks/color_coordinated_cylinder_ball_match.py
deleted file mode 100644
index c68700bfe287370c2b31f3ccbbbafc1370ab92f9..0000000000000000000000000000000000000000
--- a/spaces/Gen-Sim/Gen-Sim/cliport/generated_tasks/color_coordinated_cylinder_ball_match.py
+++ /dev/null
@@ -1,62 +0,0 @@
-import numpy as np
-import os
-import pybullet as p
-import random
-from cliport.tasks import primitives
-from cliport.tasks.grippers import Spatula
-from cliport.tasks.task import Task
-from cliport.utils import utils
-import numpy as np
-from cliport.tasks.task import Task
-from cliport.utils import utils
-import pybullet as p
-
-class ColorCoordinatedCylinderBallMatch(Task):
- """Pick up each ball and place it on top of the cylinder of the same color."""
-
- def __init__(self):
- super().__init__()
- self.max_steps = 20
- self.lang_template = "place the {color} ball on the {color} cylinder"
- self.task_completed_desc = "done placing balls on cylinders."
- self.additional_reset()
-
- def reset(self, env):
- super().reset(env)
-
- # Add cylinders.
- # x, y, z dimensions for the asset size
- cylinder_size = (0.04, 0.04, 0.1)
- cylinder_urdf = 'cylinder/cylinder-template.urdf'
- cylinder_colors = ['red', 'blue', 'green', 'yellow']
- cylinder_poses = []
- cylinders = []
- for color in cylinder_colors:
- cylinder_pose = self.get_random_pose(env, cylinder_size)
- cylinder_id = env.add_object(cylinder_urdf, cylinder_pose, color=color)
- cylinder_poses.append(cylinder_pose)
- cylinders.append(cylinder_id)
-
- # Add balls.
- # x, y, z dimensions for the asset size
- ball_size = (0.04, 0.04, 0.04)
- ball_urdf = 'ball/ball-template.urdf'
- balls = []
- for color in cylinder_colors:
- ball_pose = self.get_random_pose(env, ball_size)
- ball_id = env.add_object(ball_urdf, ball_pose, color=color)
- balls.append(ball_id)
-
- # Add blocks as obstacles.
- # x, y, z dimensions for the asset size
- block_size = (0.04, 0.04, 0.04)
- block_urdf = 'block/small.urdf'
- for _ in range(5):
- block_pose = self.get_random_pose(env, block_size)
- env.add_object(block_urdf, block_pose)
-
- # Goal: each ball is on top of the cylinder of the same color.
- for i in range(len(balls)):
- self.add_goal(objs=[balls[i]], matches=np.ones((1, 1)), targ_poses=[cylinder_poses[i]], replace=False,
- rotations=True, metric='pose', params=None, step_max_reward=1/len(balls),
- language_goal=self.lang_template.format(color=cylinder_colors[i]))
\ No newline at end of file
diff --git a/spaces/Gradio-Blocks/StyleGAN-NADA/e4e/models/stylegan2/op/upfirdn2d.py b/spaces/Gradio-Blocks/StyleGAN-NADA/e4e/models/stylegan2/op/upfirdn2d.py
deleted file mode 100644
index 7bc5a1e331c2bbb1893ac748cfd0f144ff0651b4..0000000000000000000000000000000000000000
--- a/spaces/Gradio-Blocks/StyleGAN-NADA/e4e/models/stylegan2/op/upfirdn2d.py
+++ /dev/null
@@ -1,184 +0,0 @@
-import os
-
-import torch
-from torch.autograd import Function
-from torch.utils.cpp_extension import load
-
-module_path = os.path.dirname(__file__)
-upfirdn2d_op = load(
- 'upfirdn2d',
- sources=[
- os.path.join(module_path, 'upfirdn2d.cpp'),
- os.path.join(module_path, 'upfirdn2d_kernel.cu'),
- ],
-)
-
-
-class UpFirDn2dBackward(Function):
- @staticmethod
- def forward(
- ctx, grad_output, kernel, grad_kernel, up, down, pad, g_pad, in_size, out_size
- ):
- up_x, up_y = up
- down_x, down_y = down
- g_pad_x0, g_pad_x1, g_pad_y0, g_pad_y1 = g_pad
-
- grad_output = grad_output.reshape(-1, out_size[0], out_size[1], 1)
-
- grad_input = upfirdn2d_op.upfirdn2d(
- grad_output,
- grad_kernel,
- down_x,
- down_y,
- up_x,
- up_y,
- g_pad_x0,
- g_pad_x1,
- g_pad_y0,
- g_pad_y1,
- )
- grad_input = grad_input.view(in_size[0], in_size[1], in_size[2], in_size[3])
-
- ctx.save_for_backward(kernel)
-
- pad_x0, pad_x1, pad_y0, pad_y1 = pad
-
- ctx.up_x = up_x
- ctx.up_y = up_y
- ctx.down_x = down_x
- ctx.down_y = down_y
- ctx.pad_x0 = pad_x0
- ctx.pad_x1 = pad_x1
- ctx.pad_y0 = pad_y0
- ctx.pad_y1 = pad_y1
- ctx.in_size = in_size
- ctx.out_size = out_size
-
- return grad_input
-
- @staticmethod
- def backward(ctx, gradgrad_input):
- kernel, = ctx.saved_tensors
-
- gradgrad_input = gradgrad_input.reshape(-1, ctx.in_size[2], ctx.in_size[3], 1)
-
- gradgrad_out = upfirdn2d_op.upfirdn2d(
- gradgrad_input,
- kernel,
- ctx.up_x,
- ctx.up_y,
- ctx.down_x,
- ctx.down_y,
- ctx.pad_x0,
- ctx.pad_x1,
- ctx.pad_y0,
- ctx.pad_y1,
- )
- # gradgrad_out = gradgrad_out.view(ctx.in_size[0], ctx.out_size[0], ctx.out_size[1], ctx.in_size[3])
- gradgrad_out = gradgrad_out.view(
- ctx.in_size[0], ctx.in_size[1], ctx.out_size[0], ctx.out_size[1]
- )
-
- return gradgrad_out, None, None, None, None, None, None, None, None
-
-
-class UpFirDn2d(Function):
- @staticmethod
- def forward(ctx, input, kernel, up, down, pad):
- up_x, up_y = up
- down_x, down_y = down
- pad_x0, pad_x1, pad_y0, pad_y1 = pad
-
- kernel_h, kernel_w = kernel.shape
- batch, channel, in_h, in_w = input.shape
- ctx.in_size = input.shape
-
- input = input.reshape(-1, in_h, in_w, 1)
-
- ctx.save_for_backward(kernel, torch.flip(kernel, [0, 1]))
-
- out_h = (in_h * up_y + pad_y0 + pad_y1 - kernel_h) // down_y + 1
- out_w = (in_w * up_x + pad_x0 + pad_x1 - kernel_w) // down_x + 1
- ctx.out_size = (out_h, out_w)
-
- ctx.up = (up_x, up_y)
- ctx.down = (down_x, down_y)
- ctx.pad = (pad_x0, pad_x1, pad_y0, pad_y1)
-
- g_pad_x0 = kernel_w - pad_x0 - 1
- g_pad_y0 = kernel_h - pad_y0 - 1
- g_pad_x1 = in_w * up_x - out_w * down_x + pad_x0 - up_x + 1
- g_pad_y1 = in_h * up_y - out_h * down_y + pad_y0 - up_y + 1
-
- ctx.g_pad = (g_pad_x0, g_pad_x1, g_pad_y0, g_pad_y1)
-
- out = upfirdn2d_op.upfirdn2d(
- input, kernel, up_x, up_y, down_x, down_y, pad_x0, pad_x1, pad_y0, pad_y1
- )
- # out = out.view(major, out_h, out_w, minor)
- out = out.view(-1, channel, out_h, out_w)
-
- return out
-
- @staticmethod
- def backward(ctx, grad_output):
- kernel, grad_kernel = ctx.saved_tensors
-
- grad_input = UpFirDn2dBackward.apply(
- grad_output,
- kernel,
- grad_kernel,
- ctx.up,
- ctx.down,
- ctx.pad,
- ctx.g_pad,
- ctx.in_size,
- ctx.out_size,
- )
-
- return grad_input, None, None, None, None
-
-
-def upfirdn2d(input, kernel, up=1, down=1, pad=(0, 0)):
- out = UpFirDn2d.apply(
- input, kernel, (up, up), (down, down), (pad[0], pad[1], pad[0], pad[1])
- )
-
- return out
-
-
-def upfirdn2d_native(
- input, kernel, up_x, up_y, down_x, down_y, pad_x0, pad_x1, pad_y0, pad_y1
-):
- _, in_h, in_w, minor = input.shape
- kernel_h, kernel_w = kernel.shape
-
- out = input.view(-1, in_h, 1, in_w, 1, minor)
- out = F.pad(out, [0, 0, 0, up_x - 1, 0, 0, 0, up_y - 1])
- out = out.view(-1, in_h * up_y, in_w * up_x, minor)
-
- out = F.pad(
- out, [0, 0, max(pad_x0, 0), max(pad_x1, 0), max(pad_y0, 0), max(pad_y1, 0)]
- )
- out = out[
- :,
- max(-pad_y0, 0): out.shape[1] - max(-pad_y1, 0),
- max(-pad_x0, 0): out.shape[2] - max(-pad_x1, 0),
- :,
- ]
-
- out = out.permute(0, 3, 1, 2)
- out = out.reshape(
- [-1, 1, in_h * up_y + pad_y0 + pad_y1, in_w * up_x + pad_x0 + pad_x1]
- )
- w = torch.flip(kernel, [0, 1]).view(1, 1, kernel_h, kernel_w)
- out = F.conv2d(out, w)
- out = out.reshape(
- -1,
- minor,
- in_h * up_y + pad_y0 + pad_y1 - kernel_h + 1,
- in_w * up_x + pad_x0 + pad_x1 - kernel_w + 1,
- )
- out = out.permute(0, 2, 3, 1)
-
- return out[:, ::down_y, ::down_x, :]
diff --git a/spaces/Gradio-Blocks/uniformer_image_detection/configs/dynamic_rcnn/README.md b/spaces/Gradio-Blocks/uniformer_image_detection/configs/dynamic_rcnn/README.md
deleted file mode 100644
index ffdc42dcdfddbaa946f81cba00e73b5573aa19fc..0000000000000000000000000000000000000000
--- a/spaces/Gradio-Blocks/uniformer_image_detection/configs/dynamic_rcnn/README.md
+++ /dev/null
@@ -1,20 +0,0 @@
-# Dynamic R-CNN: Towards High Quality Object Detection via Dynamic Training
-
-## Introduction
-
-[ALGORITHM]
-
-```
-@article{DynamicRCNN,
- author = {Hongkai Zhang and Hong Chang and Bingpeng Ma and Naiyan Wang and Xilin Chen},
- title = {Dynamic {R-CNN}: Towards High Quality Object Detection via Dynamic Training},
- journal = {arXiv preprint arXiv:2004.06002},
- year = {2020}
-}
-```
-
-## Results and Models
-
-| Backbone | Style | Lr schd | Mem (GB) | Inf time (fps) | box AP | Config | Download |
-|:---------:|:-------:|:-------:|:--------:|:--------------:|:------:|:------:|:--------:|
-| R-50 | pytorch | 1x | 3.8 | | 38.9 | [config](https://github.com/open-mmlab/mmdetection/tree/master/configs/dynamic_rcnn/dynamic_rcnn_r50_fpn_1x.py) | [model](http://download.openmmlab.com/mmdetection/v2.0/dynamic_rcnn/dynamic_rcnn_r50_fpn_1x/dynamic_rcnn_r50_fpn_1x-62a3f276.pth) | [log](http://download.openmmlab.com/mmdetection/v2.0/dynamic_rcnn/dynamic_rcnn_r50_fpn_1x/dynamic_rcnn_r50_fpn_1x_20200618_095048.log.json) |
diff --git a/spaces/Gradio-Blocks/uniformer_image_detection/configs/gn+ws/mask_rcnn_r101_fpn_gn_ws-all_20_23_24e_coco.py b/spaces/Gradio-Blocks/uniformer_image_detection/configs/gn+ws/mask_rcnn_r101_fpn_gn_ws-all_20_23_24e_coco.py
deleted file mode 100644
index a790d932152420f5be0a05b21ac122087d315398..0000000000000000000000000000000000000000
--- a/spaces/Gradio-Blocks/uniformer_image_detection/configs/gn+ws/mask_rcnn_r101_fpn_gn_ws-all_20_23_24e_coco.py
+++ /dev/null
@@ -1,4 +0,0 @@
-_base_ = './mask_rcnn_r101_fpn_gn_ws-all_2x_coco.py'
-# learning policy
-lr_config = dict(step=[20, 23])
-runner = dict(type='EpochBasedRunner', max_epochs=24)
diff --git a/spaces/Gradio-Blocks/uniformer_image_segmentation/configs/fcn/fcn_r50-d8_512x512_80k_ade20k.py b/spaces/Gradio-Blocks/uniformer_image_segmentation/configs/fcn/fcn_r50-d8_512x512_80k_ade20k.py
deleted file mode 100644
index ef194cb594eb76316324066e23e48184d8cede27..0000000000000000000000000000000000000000
--- a/spaces/Gradio-Blocks/uniformer_image_segmentation/configs/fcn/fcn_r50-d8_512x512_80k_ade20k.py
+++ /dev/null
@@ -1,6 +0,0 @@
-_base_ = [
- '../_base_/models/fcn_r50-d8.py', '../_base_/datasets/ade20k.py',
- '../_base_/default_runtime.py', '../_base_/schedules/schedule_80k.py'
-]
-model = dict(
- decode_head=dict(num_classes=150), auxiliary_head=dict(num_classes=150))
diff --git a/spaces/Gradio-Blocks/uniformer_image_segmentation/exp/upernet_hybrid_small/run.sh b/spaces/Gradio-Blocks/uniformer_image_segmentation/exp/upernet_hybrid_small/run.sh
deleted file mode 100644
index 9fb22edfa7a32624ea08a63fe7d720c40db3b696..0000000000000000000000000000000000000000
--- a/spaces/Gradio-Blocks/uniformer_image_segmentation/exp/upernet_hybrid_small/run.sh
+++ /dev/null
@@ -1,10 +0,0 @@
-#!/usr/bin/env bash
-
-work_path=$(dirname $0)
-PYTHONPATH="$(dirname $0)/../../":$PYTHONPATH \
-python -m torch.distributed.launch --nproc_per_node=8 \
- tools/train.py ${work_path}/config.py \
- --launcher pytorch \
- --options model.backbone.pretrained_path='your_model_path/uniformer_small_in1k.pth' \
- --work-dir ${work_path}/ckpt \
- 2>&1 | tee -a ${work_path}/log.txt
diff --git a/spaces/GrandaddyShmax/MusicGen_Plus/audiocraft/quantization/base.py b/spaces/GrandaddyShmax/MusicGen_Plus/audiocraft/quantization/base.py
deleted file mode 100644
index 1b16c130d266fbd021d3fc29bb9f98c33dd3c588..0000000000000000000000000000000000000000
--- a/spaces/GrandaddyShmax/MusicGen_Plus/audiocraft/quantization/base.py
+++ /dev/null
@@ -1,107 +0,0 @@
-# Copyright (c) Meta Platforms, Inc. and affiliates.
-# All rights reserved.
-#
-# This source code is licensed under the license found in the
-# LICENSE file in the root directory of this source tree.
-
-"""
-Base class for all quantizers.
-"""
-
-from dataclasses import dataclass, field
-import typing as tp
-
-import torch
-from torch import nn
-
-
-@dataclass
-class QuantizedResult:
- x: torch.Tensor
- codes: torch.Tensor
- bandwidth: torch.Tensor # bandwidth in kb/s used, per batch item.
- penalty: tp.Optional[torch.Tensor] = None
- metrics: dict = field(default_factory=dict)
-
-
-class BaseQuantizer(nn.Module):
- """Base class for quantizers.
- """
-
- def forward(self, x: torch.Tensor, frame_rate: int) -> QuantizedResult:
- """
- Given input tensor x, returns first the quantized (or approximately quantized)
- representation along with quantized codes, bandwidth, and any penalty term for the loss.
- Finally, this returns a dict of metrics to update logging etc.
- Frame rate must be passed so that the bandwidth is properly computed.
- """
- raise NotImplementedError()
-
- def encode(self, x: torch.Tensor) -> torch.Tensor:
- """Encode a given input tensor with the specified sample rate at the given bandwidth.
- """
- raise NotImplementedError()
-
- def decode(self, codes: torch.Tensor) -> torch.Tensor:
- """Decode the given codes to the quantized representation.
- """
- raise NotImplementedError()
-
- @property
- def total_codebooks(self):
- """Total number of codebooks.
- """
- raise NotImplementedError()
-
- @property
- def num_codebooks(self):
- """Number of active codebooks.
- """
- raise NotImplementedError()
-
- def set_num_codebooks(self, n: int):
- """Set the number of active codebooks.
- """
- raise NotImplementedError()
-
-
-class DummyQuantizer(BaseQuantizer):
- """Fake quantizer that actually does not perform any quantization.
- """
- def __init__(self):
- super().__init__()
-
- def forward(self, x: torch.Tensor, frame_rate: int):
- q = x.unsqueeze(1)
- return QuantizedResult(x, q, torch.tensor(q.numel() * 32 * frame_rate / 1000 / len(x)).to(x))
-
- def encode(self, x: torch.Tensor) -> torch.Tensor:
- """Encode a given input tensor with the specified sample rate at the given bandwidth.
- In the case of the DummyQuantizer, the codes are actually identical
- to the input and resulting quantized representation as no quantization is done.
- """
- return x.unsqueeze(1)
-
- def decode(self, codes: torch.Tensor) -> torch.Tensor:
- """Decode the given codes to the quantized representation.
- In the case of the DummyQuantizer, the codes are actually identical
- to the input and resulting quantized representation as no quantization is done.
- """
- return codes.squeeze(1)
-
- @property
- def total_codebooks(self):
- """Total number of codebooks.
- """
- return 1
-
- @property
- def num_codebooks(self):
- """Total number of codebooks.
- """
- return self.total_codebooks
-
- def set_num_codebooks(self, n: int):
- """Set the number of active codebooks.
- """
- raise AttributeError("Cannot override the number of codebooks for the dummy quantizer")
diff --git a/spaces/Grezz/generate_human_motion/VQ-Trans/models/quantize_cnn.py b/spaces/Grezz/generate_human_motion/VQ-Trans/models/quantize_cnn.py
deleted file mode 100644
index b796772749efda9a225bdcb0e7262791a972a710..0000000000000000000000000000000000000000
--- a/spaces/Grezz/generate_human_motion/VQ-Trans/models/quantize_cnn.py
+++ /dev/null
@@ -1,415 +0,0 @@
-import numpy as np
-import torch
-import torch.nn as nn
-import torch.nn.functional as F
-
-class QuantizeEMAReset(nn.Module):
- def __init__(self, nb_code, code_dim, args):
- super().__init__()
- self.nb_code = nb_code
- self.code_dim = code_dim
- self.mu = args.mu
- self.reset_codebook()
-
- def reset_codebook(self):
- self.init = False
- self.code_sum = None
- self.code_count = None
- if torch.cuda.is_available():
- self.register_buffer('codebook', torch.zeros(self.nb_code, self.code_dim).cuda())
- else:
- self.register_buffer('codebook', torch.zeros(self.nb_code, self.code_dim))
-
- def _tile(self, x):
- nb_code_x, code_dim = x.shape
- if nb_code_x < self.nb_code:
- n_repeats = (self.nb_code + nb_code_x - 1) // nb_code_x
- std = 0.01 / np.sqrt(code_dim)
- out = x.repeat(n_repeats, 1)
- out = out + torch.randn_like(out) * std
- else :
- out = x
- return out
-
- def init_codebook(self, x):
- out = self._tile(x)
- self.codebook = out[:self.nb_code]
- self.code_sum = self.codebook.clone()
- self.code_count = torch.ones(self.nb_code, device=self.codebook.device)
- self.init = True
-
- @torch.no_grad()
- def compute_perplexity(self, code_idx) :
- # Calculate new centres
- code_onehot = torch.zeros(self.nb_code, code_idx.shape[0], device=code_idx.device) # nb_code, N * L
- code_onehot.scatter_(0, code_idx.view(1, code_idx.shape[0]), 1)
-
- code_count = code_onehot.sum(dim=-1) # nb_code
- prob = code_count / torch.sum(code_count)
- perplexity = torch.exp(-torch.sum(prob * torch.log(prob + 1e-7)))
- return perplexity
-
- @torch.no_grad()
- def update_codebook(self, x, code_idx):
-
- code_onehot = torch.zeros(self.nb_code, x.shape[0], device=x.device) # nb_code, N * L
- code_onehot.scatter_(0, code_idx.view(1, x.shape[0]), 1)
-
- code_sum = torch.matmul(code_onehot, x) # nb_code, w
- code_count = code_onehot.sum(dim=-1) # nb_code
-
- out = self._tile(x)
- code_rand = out[:self.nb_code]
-
- # Update centres
- self.code_sum = self.mu * self.code_sum + (1. - self.mu) * code_sum # w, nb_code
- self.code_count = self.mu * self.code_count + (1. - self.mu) * code_count # nb_code
-
- usage = (self.code_count.view(self.nb_code, 1) >= 1.0).float()
- code_update = self.code_sum.view(self.nb_code, self.code_dim) / self.code_count.view(self.nb_code, 1)
-
- self.codebook = usage * code_update + (1 - usage) * code_rand
- prob = code_count / torch.sum(code_count)
- perplexity = torch.exp(-torch.sum(prob * torch.log(prob + 1e-7)))
-
-
- return perplexity
-
- def preprocess(self, x):
- # NCT -> NTC -> [NT, C]
- x = x.permute(0, 2, 1).contiguous()
- x = x.view(-1, x.shape[-1])
- return x
-
- def quantize(self, x):
- # Calculate latent code x_l
- k_w = self.codebook.t()
- distance = torch.sum(x ** 2, dim=-1, keepdim=True) - 2 * torch.matmul(x, k_w) + torch.sum(k_w ** 2, dim=0,
- keepdim=True) # (N * L, b)
- _, code_idx = torch.min(distance, dim=-1)
- return code_idx
-
- def dequantize(self, code_idx):
- x = F.embedding(code_idx, self.codebook)
- return x
-
-
- def forward(self, x):
- N, width, T = x.shape
-
- # Preprocess
- x = self.preprocess(x)
-
- # Init codebook if not inited
- if self.training and not self.init:
- self.init_codebook(x)
-
- # quantize and dequantize through bottleneck
- code_idx = self.quantize(x)
- x_d = self.dequantize(code_idx)
-
- # Update embeddings
- if self.training:
- perplexity = self.update_codebook(x, code_idx)
- else :
- perplexity = self.compute_perplexity(code_idx)
-
- # Loss
- commit_loss = F.mse_loss(x, x_d.detach())
-
- # Passthrough
- x_d = x + (x_d - x).detach()
-
- # Postprocess
- x_d = x_d.view(N, T, -1).permute(0, 2, 1).contiguous() #(N, DIM, T)
-
- return x_d, commit_loss, perplexity
-
-
-
-class Quantizer(nn.Module):
- def __init__(self, n_e, e_dim, beta):
- super(Quantizer, self).__init__()
-
- self.e_dim = e_dim
- self.n_e = n_e
- self.beta = beta
-
- self.embedding = nn.Embedding(self.n_e, self.e_dim)
- self.embedding.weight.data.uniform_(-1.0 / self.n_e, 1.0 / self.n_e)
-
- def forward(self, z):
-
- N, width, T = z.shape
- z = self.preprocess(z)
- assert z.shape[-1] == self.e_dim
- z_flattened = z.contiguous().view(-1, self.e_dim)
-
- # B x V
- d = torch.sum(z_flattened ** 2, dim=1, keepdim=True) + \
- torch.sum(self.embedding.weight**2, dim=1) - 2 * \
- torch.matmul(z_flattened, self.embedding.weight.t())
- # B x 1
- min_encoding_indices = torch.argmin(d, dim=1)
- z_q = self.embedding(min_encoding_indices).view(z.shape)
-
- # compute loss for embedding
- loss = torch.mean((z_q - z.detach())**2) + self.beta * \
- torch.mean((z_q.detach() - z)**2)
-
- # preserve gradients
- z_q = z + (z_q - z).detach()
- z_q = z_q.view(N, T, -1).permute(0, 2, 1).contiguous() #(N, DIM, T)
-
- min_encodings = F.one_hot(min_encoding_indices, self.n_e).type(z.dtype)
- e_mean = torch.mean(min_encodings, dim=0)
- perplexity = torch.exp(-torch.sum(e_mean*torch.log(e_mean + 1e-10)))
- return z_q, loss, perplexity
-
- def quantize(self, z):
-
- assert z.shape[-1] == self.e_dim
-
- # B x V
- d = torch.sum(z ** 2, dim=1, keepdim=True) + \
- torch.sum(self.embedding.weight ** 2, dim=1) - 2 * \
- torch.matmul(z, self.embedding.weight.t())
- # B x 1
- min_encoding_indices = torch.argmin(d, dim=1)
- return min_encoding_indices
-
- def dequantize(self, indices):
-
- index_flattened = indices.view(-1)
- z_q = self.embedding(index_flattened)
- z_q = z_q.view(indices.shape + (self.e_dim, )).contiguous()
- return z_q
-
- def preprocess(self, x):
- # NCT -> NTC -> [NT, C]
- x = x.permute(0, 2, 1).contiguous()
- x = x.view(-1, x.shape[-1])
- return x
-
-
-
-class QuantizeReset(nn.Module):
- def __init__(self, nb_code, code_dim, args):
- super().__init__()
- self.nb_code = nb_code
- self.code_dim = code_dim
- self.reset_codebook()
- self.codebook = nn.Parameter(torch.randn(nb_code, code_dim))
-
- def reset_codebook(self):
- self.init = False
- self.code_count = None
-
- def _tile(self, x):
- nb_code_x, code_dim = x.shape
- if nb_code_x < self.nb_code:
- n_repeats = (self.nb_code + nb_code_x - 1) // nb_code_x
- std = 0.01 / np.sqrt(code_dim)
- out = x.repeat(n_repeats, 1)
- out = out + torch.randn_like(out) * std
- else :
- out = x
- return out
-
- def init_codebook(self, x):
- out = self._tile(x)
- self.codebook = nn.Parameter(out[:self.nb_code])
- self.code_count = torch.ones(self.nb_code, device=self.codebook.device)
- self.init = True
-
- @torch.no_grad()
- def compute_perplexity(self, code_idx) :
- # Calculate new centres
- code_onehot = torch.zeros(self.nb_code, code_idx.shape[0], device=code_idx.device) # nb_code, N * L
- code_onehot.scatter_(0, code_idx.view(1, code_idx.shape[0]), 1)
-
- code_count = code_onehot.sum(dim=-1) # nb_code
- prob = code_count / torch.sum(code_count)
- perplexity = torch.exp(-torch.sum(prob * torch.log(prob + 1e-7)))
- return perplexity
-
- def update_codebook(self, x, code_idx):
-
- code_onehot = torch.zeros(self.nb_code, x.shape[0], device=x.device) # nb_code, N * L
- code_onehot.scatter_(0, code_idx.view(1, x.shape[0]), 1)
-
- code_count = code_onehot.sum(dim=-1) # nb_code
-
- out = self._tile(x)
- code_rand = out[:self.nb_code]
-
- # Update centres
- self.code_count = code_count # nb_code
- usage = (self.code_count.view(self.nb_code, 1) >= 1.0).float()
-
- self.codebook.data = usage * self.codebook.data + (1 - usage) * code_rand
- prob = code_count / torch.sum(code_count)
- perplexity = torch.exp(-torch.sum(prob * torch.log(prob + 1e-7)))
-
-
- return perplexity
-
- def preprocess(self, x):
- # NCT -> NTC -> [NT, C]
- x = x.permute(0, 2, 1).contiguous()
- x = x.view(-1, x.shape[-1])
- return x
-
- def quantize(self, x):
- # Calculate latent code x_l
- k_w = self.codebook.t()
- distance = torch.sum(x ** 2, dim=-1, keepdim=True) - 2 * torch.matmul(x, k_w) + torch.sum(k_w ** 2, dim=0,
- keepdim=True) # (N * L, b)
- _, code_idx = torch.min(distance, dim=-1)
- return code_idx
-
- def dequantize(self, code_idx):
- x = F.embedding(code_idx, self.codebook)
- return x
-
-
- def forward(self, x):
- N, width, T = x.shape
- # Preprocess
- x = self.preprocess(x)
- # Init codebook if not inited
- if self.training and not self.init:
- self.init_codebook(x)
- # quantize and dequantize through bottleneck
- code_idx = self.quantize(x)
- x_d = self.dequantize(code_idx)
- # Update embeddings
- if self.training:
- perplexity = self.update_codebook(x, code_idx)
- else :
- perplexity = self.compute_perplexity(code_idx)
-
- # Loss
- commit_loss = F.mse_loss(x, x_d.detach())
-
- # Passthrough
- x_d = x + (x_d - x).detach()
-
- # Postprocess
- x_d = x_d.view(N, T, -1).permute(0, 2, 1).contiguous() #(N, DIM, T)
-
- return x_d, commit_loss, perplexity
-
-class QuantizeEMA(nn.Module):
- def __init__(self, nb_code, code_dim, args):
- super().__init__()
- self.nb_code = nb_code
- self.code_dim = code_dim
- self.mu = 0.99
- self.reset_codebook()
-
- def reset_codebook(self):
- self.init = False
- self.code_sum = None
- self.code_count = None
- self.register_buffer('codebook', torch.zeros(self.nb_code, self.code_dim).cuda())
-
- def _tile(self, x):
- nb_code_x, code_dim = x.shape
- if nb_code_x < self.nb_code:
- n_repeats = (self.nb_code + nb_code_x - 1) // nb_code_x
- std = 0.01 / np.sqrt(code_dim)
- out = x.repeat(n_repeats, 1)
- out = out + torch.randn_like(out) * std
- else :
- out = x
- return out
-
- def init_codebook(self, x):
- out = self._tile(x)
- self.codebook = out[:self.nb_code]
- self.code_sum = self.codebook.clone()
- self.code_count = torch.ones(self.nb_code, device=self.codebook.device)
- self.init = True
-
- @torch.no_grad()
- def compute_perplexity(self, code_idx) :
- # Calculate new centres
- code_onehot = torch.zeros(self.nb_code, code_idx.shape[0], device=code_idx.device) # nb_code, N * L
- code_onehot.scatter_(0, code_idx.view(1, code_idx.shape[0]), 1)
-
- code_count = code_onehot.sum(dim=-1) # nb_code
- prob = code_count / torch.sum(code_count)
- perplexity = torch.exp(-torch.sum(prob * torch.log(prob + 1e-7)))
- return perplexity
-
- @torch.no_grad()
- def update_codebook(self, x, code_idx):
-
- code_onehot = torch.zeros(self.nb_code, x.shape[0], device=x.device) # nb_code, N * L
- code_onehot.scatter_(0, code_idx.view(1, x.shape[0]), 1)
-
- code_sum = torch.matmul(code_onehot, x) # nb_code, w
- code_count = code_onehot.sum(dim=-1) # nb_code
-
- # Update centres
- self.code_sum = self.mu * self.code_sum + (1. - self.mu) * code_sum # w, nb_code
- self.code_count = self.mu * self.code_count + (1. - self.mu) * code_count # nb_code
-
- code_update = self.code_sum.view(self.nb_code, self.code_dim) / self.code_count.view(self.nb_code, 1)
-
- self.codebook = code_update
- prob = code_count / torch.sum(code_count)
- perplexity = torch.exp(-torch.sum(prob * torch.log(prob + 1e-7)))
-
- return perplexity
-
- def preprocess(self, x):
- # NCT -> NTC -> [NT, C]
- x = x.permute(0, 2, 1).contiguous()
- x = x.view(-1, x.shape[-1])
- return x
-
- def quantize(self, x):
- # Calculate latent code x_l
- k_w = self.codebook.t()
- distance = torch.sum(x ** 2, dim=-1, keepdim=True) - 2 * torch.matmul(x, k_w) + torch.sum(k_w ** 2, dim=0,
- keepdim=True) # (N * L, b)
- _, code_idx = torch.min(distance, dim=-1)
- return code_idx
-
- def dequantize(self, code_idx):
- x = F.embedding(code_idx, self.codebook)
- return x
-
-
- def forward(self, x):
- N, width, T = x.shape
-
- # Preprocess
- x = self.preprocess(x)
-
- # Init codebook if not inited
- if self.training and not self.init:
- self.init_codebook(x)
-
- # quantize and dequantize through bottleneck
- code_idx = self.quantize(x)
- x_d = self.dequantize(code_idx)
-
- # Update embeddings
- if self.training:
- perplexity = self.update_codebook(x, code_idx)
- else :
- perplexity = self.compute_perplexity(code_idx)
-
- # Loss
- commit_loss = F.mse_loss(x, x_d.detach())
-
- # Passthrough
- x_d = x + (x_d - x).detach()
-
- # Postprocess
- x_d = x_d.view(N, T, -1).permute(0, 2, 1).contiguous() #(N, DIM, T)
-
- return x_d, commit_loss, perplexity
\ No newline at end of file
diff --git a/spaces/HALLA/HALL-E/index.html b/spaces/HALLA/HALL-E/index.html
deleted file mode 100644
index 74d65ba18bf356ce52b1d00b0e7c1903d5e285f2..0000000000000000000000000000000000000000
--- a/spaces/HALLA/HALL-E/index.html
+++ /dev/null
@@ -1,64 +0,0 @@
-
-
-
-
-
-
-
-
-
-
-
-
-
-
-
-
-
-
-
-
-
-
-
-
diff --git a/spaces/HaHaBill/LandShapes-Antarctica/models/biggan/pytorch_biggan/pytorch_pretrained_biggan/convert_tf_to_pytorch.py b/spaces/HaHaBill/LandShapes-Antarctica/models/biggan/pytorch_biggan/pytorch_pretrained_biggan/convert_tf_to_pytorch.py
deleted file mode 100644
index 7ccb787dec188e9dbd9ea31288c049c1bdb30f95..0000000000000000000000000000000000000000
--- a/spaces/HaHaBill/LandShapes-Antarctica/models/biggan/pytorch_biggan/pytorch_pretrained_biggan/convert_tf_to_pytorch.py
+++ /dev/null
@@ -1,312 +0,0 @@
-# coding: utf-8
-"""
-Convert a TF Hub model for BigGAN in a PT one.
-"""
-from __future__ import (absolute_import, division, print_function, unicode_literals)
-
-from itertools import chain
-
-import os
-import argparse
-import logging
-import numpy as np
-import torch
-import torch.nn as nn
-import torch.nn.functional as F
-from torch.nn.functional import normalize
-
-from .model import BigGAN, WEIGHTS_NAME, CONFIG_NAME
-from .config import BigGANConfig
-
-logger = logging.getLogger(__name__)
-
-
-def extract_batch_norm_stats(tf_model_path, batch_norm_stats_path=None):
- try:
- import numpy as np
- import tensorflow as tf
- import tensorflow_hub as hub
- except ImportError:
- raise ImportError("Loading a TensorFlow models in PyTorch, requires TensorFlow and TF Hub to be installed. "
- "Please see https://www.tensorflow.org/install/ for installation instructions for TensorFlow. "
- "And see https://github.com/tensorflow/hub for installing Hub. "
- "Probably pip install tensorflow tensorflow-hub")
- tf.reset_default_graph()
- logger.info('Loading BigGAN module from: {}'.format(tf_model_path))
- module = hub.Module(tf_model_path)
- inputs = {k: tf.placeholder(v.dtype, v.get_shape().as_list(), k)
- for k, v in module.get_input_info_dict().items()}
- output = module(inputs)
-
- initializer = tf.global_variables_initializer()
- sess = tf.Session()
- stacks = sum(((i*10 + 1, i*10 + 3, i*10 + 6, i*10 + 8) for i in range(50)), ())
- numpy_stacks = []
- for i in stacks:
- logger.info("Retrieving module_apply_default/stack_{}".format(i))
- try:
- stack_var = tf.get_default_graph().get_tensor_by_name("module_apply_default/stack_%d:0" % i)
- except KeyError:
- break # We have all the stats
- numpy_stacks.append(sess.run(stack_var))
-
- if batch_norm_stats_path is not None:
- torch.save(numpy_stacks, batch_norm_stats_path)
- else:
- return numpy_stacks
-
-
-def build_tf_to_pytorch_map(model, config):
- """ Build a map from TF variables to PyTorch modules. """
- tf_to_pt_map = {}
-
- # Embeddings and GenZ
- tf_to_pt_map.update({'linear/w/ema_0.9999': model.embeddings.weight,
- 'Generator/GenZ/G_linear/b/ema_0.9999': model.generator.gen_z.bias,
- 'Generator/GenZ/G_linear/w/ema_0.9999': model.generator.gen_z.weight_orig,
- 'Generator/GenZ/G_linear/u0': model.generator.gen_z.weight_u})
-
- # GBlock blocks
- model_layer_idx = 0
- for i, (up, in_channels, out_channels) in enumerate(config.layers):
- if i == config.attention_layer_position:
- model_layer_idx += 1
- layer_str = "Generator/GBlock_%d/" % i if i > 0 else "Generator/GBlock/"
- layer_pnt = model.generator.layers[model_layer_idx]
- for i in range(4): # Batchnorms
- batch_str = layer_str + ("BatchNorm_%d/" % i if i > 0 else "BatchNorm/")
- batch_pnt = getattr(layer_pnt, 'bn_%d' % i)
- for name in ('offset', 'scale'):
- sub_module_str = batch_str + name + "/"
- sub_module_pnt = getattr(batch_pnt, name)
- tf_to_pt_map.update({sub_module_str + "w/ema_0.9999": sub_module_pnt.weight_orig,
- sub_module_str + "u0": sub_module_pnt.weight_u})
- for i in range(4): # Convolutions
- conv_str = layer_str + "conv%d/" % i
- conv_pnt = getattr(layer_pnt, 'conv_%d' % i)
- tf_to_pt_map.update({conv_str + "b/ema_0.9999": conv_pnt.bias,
- conv_str + "w/ema_0.9999": conv_pnt.weight_orig,
- conv_str + "u0": conv_pnt.weight_u})
- model_layer_idx += 1
-
- # Attention block
- layer_str = "Generator/attention/"
- layer_pnt = model.generator.layers[config.attention_layer_position]
- tf_to_pt_map.update({layer_str + "gamma/ema_0.9999": layer_pnt.gamma})
- for pt_name, tf_name in zip(['snconv1x1_g', 'snconv1x1_o_conv', 'snconv1x1_phi', 'snconv1x1_theta'],
- ['g/', 'o_conv/', 'phi/', 'theta/']):
- sub_module_str = layer_str + tf_name
- sub_module_pnt = getattr(layer_pnt, pt_name)
- tf_to_pt_map.update({sub_module_str + "w/ema_0.9999": sub_module_pnt.weight_orig,
- sub_module_str + "u0": sub_module_pnt.weight_u})
-
- # final batch norm and conv to rgb
- layer_str = "Generator/BatchNorm/"
- layer_pnt = model.generator.bn
- tf_to_pt_map.update({layer_str + "offset/ema_0.9999": layer_pnt.bias,
- layer_str + "scale/ema_0.9999": layer_pnt.weight})
- layer_str = "Generator/conv_to_rgb/"
- layer_pnt = model.generator.conv_to_rgb
- tf_to_pt_map.update({layer_str + "b/ema_0.9999": layer_pnt.bias,
- layer_str + "w/ema_0.9999": layer_pnt.weight_orig,
- layer_str + "u0": layer_pnt.weight_u})
- return tf_to_pt_map
-
-
-def load_tf_weights_in_biggan(model, config, tf_model_path, batch_norm_stats_path=None):
- """ Load tf checkpoints and standing statistics in a pytorch model
- """
- try:
- import numpy as np
- import tensorflow as tf
- except ImportError:
- raise ImportError("Loading a TensorFlow models in PyTorch, requires TensorFlow to be installed. Please see "
- "https://www.tensorflow.org/install/ for installation instructions.")
- # Load weights from TF model
- checkpoint_path = tf_model_path + "/variables/variables"
- init_vars = tf.train.list_variables(checkpoint_path)
- from pprint import pprint
- pprint(init_vars)
-
- # Extract batch norm statistics from model if needed
- if batch_norm_stats_path:
- stats = torch.load(batch_norm_stats_path)
- else:
- logger.info("Extracting batch norm stats")
- stats = extract_batch_norm_stats(tf_model_path)
-
- # Build TF to PyTorch weights loading map
- tf_to_pt_map = build_tf_to_pytorch_map(model, config)
-
- tf_weights = {}
- for name in tf_to_pt_map.keys():
- array = tf.train.load_variable(checkpoint_path, name)
- tf_weights[name] = array
- # logger.info("Loading TF weight {} with shape {}".format(name, array.shape))
-
- # Load parameters
- with torch.no_grad():
- pt_params_pnt = set()
- for name, pointer in tf_to_pt_map.items():
- array = tf_weights[name]
- if pointer.dim() == 1:
- if pointer.dim() < array.ndim:
- array = np.squeeze(array)
- elif pointer.dim() == 2: # Weights
- array = np.transpose(array)
- elif pointer.dim() == 4: # Convolutions
- array = np.transpose(array, (3, 2, 0, 1))
- else:
- raise "Wrong dimensions to adjust: " + str((pointer.shape, array.shape))
- if pointer.shape != array.shape:
- raise ValueError("Wrong dimensions: " + str((pointer.shape, array.shape)))
- logger.info("Initialize PyTorch weight {} with shape {}".format(name, pointer.shape))
- pointer.data = torch.from_numpy(array) if isinstance(array, np.ndarray) else torch.tensor(array)
- tf_weights.pop(name, None)
- pt_params_pnt.add(pointer.data_ptr())
-
- # Prepare SpectralNorm buffers by running one step of Spectral Norm (no need to train the model):
- for module in model.modules():
- for n, buffer in module.named_buffers():
- if n == 'weight_v':
- weight_mat = module.weight_orig
- weight_mat = weight_mat.reshape(weight_mat.size(0), -1)
- u = module.weight_u
-
- v = normalize(torch.mv(weight_mat.t(), u), dim=0, eps=config.eps)
- buffer.data = v
- pt_params_pnt.add(buffer.data_ptr())
-
- u = normalize(torch.mv(weight_mat, v), dim=0, eps=config.eps)
- module.weight_u.data = u
- pt_params_pnt.add(module.weight_u.data_ptr())
-
- # Load batch norm statistics
- index = 0
- for layer in model.generator.layers:
- if not hasattr(layer, 'bn_0'):
- continue
- for i in range(4): # Batchnorms
- bn_pointer = getattr(layer, 'bn_%d' % i)
- pointer = bn_pointer.running_means
- if pointer.shape != stats[index].shape:
- raise "Wrong dimensions: " + str((pointer.shape, stats[index].shape))
- pointer.data = torch.from_numpy(stats[index])
- pt_params_pnt.add(pointer.data_ptr())
-
- pointer = bn_pointer.running_vars
- if pointer.shape != stats[index+1].shape:
- raise "Wrong dimensions: " + str((pointer.shape, stats[index].shape))
- pointer.data = torch.from_numpy(stats[index+1])
- pt_params_pnt.add(pointer.data_ptr())
-
- index += 2
-
- bn_pointer = model.generator.bn
- pointer = bn_pointer.running_means
- if pointer.shape != stats[index].shape:
- raise "Wrong dimensions: " + str((pointer.shape, stats[index].shape))
- pointer.data = torch.from_numpy(stats[index])
- pt_params_pnt.add(pointer.data_ptr())
-
- pointer = bn_pointer.running_vars
- if pointer.shape != stats[index+1].shape:
- raise "Wrong dimensions: " + str((pointer.shape, stats[index].shape))
- pointer.data = torch.from_numpy(stats[index+1])
- pt_params_pnt.add(pointer.data_ptr())
-
- remaining_params = list(n for n, t in chain(model.named_parameters(), model.named_buffers()) \
- if t.data_ptr() not in pt_params_pnt)
-
- logger.info("TF Weights not copied to PyTorch model: {} -".format(', '.join(tf_weights.keys())))
- logger.info("Remanining parameters/buffers from PyTorch model: {} -".format(', '.join(remaining_params)))
-
- return model
-
-
-BigGAN128 = BigGANConfig(output_dim=128, z_dim=128, class_embed_dim=128, channel_width=128, num_classes=1000,
- layers=[(False, 16, 16),
- (True, 16, 16),
- (False, 16, 16),
- (True, 16, 8),
- (False, 8, 8),
- (True, 8, 4),
- (False, 4, 4),
- (True, 4, 2),
- (False, 2, 2),
- (True, 2, 1)],
- attention_layer_position=8, eps=1e-4, n_stats=51)
-
-BigGAN256 = BigGANConfig(output_dim=256, z_dim=128, class_embed_dim=128, channel_width=128, num_classes=1000,
- layers=[(False, 16, 16),
- (True, 16, 16),
- (False, 16, 16),
- (True, 16, 8),
- (False, 8, 8),
- (True, 8, 8),
- (False, 8, 8),
- (True, 8, 4),
- (False, 4, 4),
- (True, 4, 2),
- (False, 2, 2),
- (True, 2, 1)],
- attention_layer_position=8, eps=1e-4, n_stats=51)
-
-BigGAN512 = BigGANConfig(output_dim=512, z_dim=128, class_embed_dim=128, channel_width=128, num_classes=1000,
- layers=[(False, 16, 16),
- (True, 16, 16),
- (False, 16, 16),
- (True, 16, 8),
- (False, 8, 8),
- (True, 8, 8),
- (False, 8, 8),
- (True, 8, 4),
- (False, 4, 4),
- (True, 4, 2),
- (False, 2, 2),
- (True, 2, 1),
- (False, 1, 1),
- (True, 1, 1)],
- attention_layer_position=8, eps=1e-4, n_stats=51)
-
-
-def main():
- parser = argparse.ArgumentParser(description="Convert a BigGAN TF Hub model in a PyTorch model")
- parser.add_argument("--model_type", type=str, default="", required=True,
- help="BigGAN model type (128, 256, 512)")
- parser.add_argument("--tf_model_path", type=str, default="", required=True,
- help="Path of the downloaded TF Hub model")
- parser.add_argument("--pt_save_path", type=str, default="",
- help="Folder to save the PyTorch model (default: Folder of the TF Hub model)")
- parser.add_argument("--batch_norm_stats_path", type=str, default="",
- help="Path of previously extracted batch norm statistics")
- args = parser.parse_args()
-
- logging.basicConfig(level=logging.INFO)
-
- if not args.pt_save_path:
- args.pt_save_path = args.tf_model_path
-
- if args.model_type == "128":
- config = BigGAN128
- elif args.model_type == "256":
- config = BigGAN256
- elif args.model_type == "512":
- config = BigGAN512
- else:
- raise ValueError("model_type should be one of 128, 256 or 512")
-
- model = BigGAN(config)
- model = load_tf_weights_in_biggan(model, config, args.tf_model_path, args.batch_norm_stats_path)
-
- model_save_path = os.path.join(args.pt_save_path, WEIGHTS_NAME)
- config_save_path = os.path.join(args.pt_save_path, CONFIG_NAME)
-
- logger.info("Save model dump to {}".format(model_save_path))
- torch.save(model.state_dict(), model_save_path)
- logger.info("Save configuration file to {}".format(config_save_path))
- with open(config_save_path, "w", encoding="utf-8") as f:
- f.write(config.to_json_string())
-
-if __name__ == "__main__":
- main()
diff --git a/spaces/HarryLee/eCommerceImageCaptioning/fairseq/fairseq/criterions/label_smoothed_cross_entropy_with_alignment.py b/spaces/HarryLee/eCommerceImageCaptioning/fairseq/fairseq/criterions/label_smoothed_cross_entropy_with_alignment.py
deleted file mode 100644
index 2ea37c16b4a477c48e4dd4500ec03f2d0c86d611..0000000000000000000000000000000000000000
--- a/spaces/HarryLee/eCommerceImageCaptioning/fairseq/fairseq/criterions/label_smoothed_cross_entropy_with_alignment.py
+++ /dev/null
@@ -1,130 +0,0 @@
-# Copyright (c) Facebook, Inc. and its affiliates.
-#
-# This source code is licensed under the MIT license found in the
-# LICENSE file in the root directory of this source tree.
-
-import math
-
-from fairseq import metrics, utils
-from fairseq.criterions import register_criterion
-
-from .label_smoothed_cross_entropy import (
- LabelSmoothedCrossEntropyCriterion,
- LabelSmoothedCrossEntropyCriterionConfig,
-)
-
-from dataclasses import dataclass, field
-
-
-@dataclass
-class LabelSmoothedCrossEntropyCriterionWithAlignmentConfig(
- LabelSmoothedCrossEntropyCriterionConfig
-):
- alignment_lambda: float = field(
- default=0.05, metadata={"help": "weight for the alignment loss"}
- )
-
-
-@register_criterion(
- "label_smoothed_cross_entropy_with_alignment",
- dataclass=LabelSmoothedCrossEntropyCriterionWithAlignmentConfig,
-)
-class LabelSmoothedCrossEntropyCriterionWithAlignment(
- LabelSmoothedCrossEntropyCriterion
-):
- def __init__(self, task, sentence_avg, label_smoothing, alignment_lambda):
- super().__init__(task, sentence_avg, label_smoothing)
- self.alignment_lambda = alignment_lambda
-
- def forward(self, model, sample, reduce=True):
- """Compute the loss for the given sample.
-
- Returns a tuple with three elements:
- 1) the loss
- 2) the sample size, which is used as the denominator for the gradient
- 3) logging outputs to display while training
- """
- net_output = model(**sample["net_input"])
- loss, nll_loss = self.compute_loss(model, net_output, sample, reduce=reduce)
- sample_size = (
- sample["target"].size(0) if self.sentence_avg else sample["ntokens"]
- )
- logging_output = {
- "loss": utils.item(loss.data) if reduce else loss.data,
- "nll_loss": utils.item(nll_loss.data) if reduce else nll_loss.data,
- "ntokens": sample["ntokens"],
- "nsentences": sample["target"].size(0),
- "sample_size": sample_size,
- }
-
- alignment_loss = None
-
- # Compute alignment loss only for training set and non dummy batches.
- if "alignments" in sample and sample["alignments"] is not None:
- alignment_loss = self.compute_alignment_loss(sample, net_output)
-
- if alignment_loss is not None:
- logging_output["alignment_loss"] = utils.item(alignment_loss.data)
- loss += self.alignment_lambda * alignment_loss
-
- return loss, sample_size, logging_output
-
- def compute_alignment_loss(self, sample, net_output):
- attn_prob = net_output[1]["attn"][0]
- bsz, tgt_sz, src_sz = attn_prob.shape
- attn = attn_prob.view(bsz * tgt_sz, src_sz)
-
- align = sample["alignments"]
- align_weights = sample["align_weights"].float()
-
- if len(align) > 0:
- # Alignment loss computation. align (shape [:, 2]) contains the src-tgt index pairs corresponding to
- # the alignments. align_weights (shape [:]) contains the 1 / frequency of a tgt index for normalizing.
- loss = -(
- (attn[align[:, 1][:, None], align[:, 0][:, None]]).log()
- * align_weights[:, None]
- ).sum()
- else:
- return None
-
- return loss
-
- @staticmethod
- def reduce_metrics(logging_outputs) -> None:
- """Aggregate logging outputs from data parallel training."""
- loss_sum = utils.item(sum(log.get("loss", 0) for log in logging_outputs))
- nll_loss_sum = utils.item(
- sum(log.get("nll_loss", 0) for log in logging_outputs)
- )
- alignment_loss_sum = utils.item(
- sum(log.get("alignment_loss", 0) for log in logging_outputs)
- )
- ntokens = utils.item(sum(log.get("ntokens", 0) for log in logging_outputs))
- sample_size = utils.item(
- sum(log.get("sample_size", 0) for log in logging_outputs)
- )
-
- metrics.log_scalar(
- "loss", loss_sum / sample_size / math.log(2), sample_size, round=3
- )
- metrics.log_scalar(
- "nll_loss", nll_loss_sum / ntokens / math.log(2), ntokens, round=3
- )
- metrics.log_scalar(
- "alignment_loss",
- alignment_loss_sum / sample_size / math.log(2),
- sample_size,
- round=3,
- )
- metrics.log_derived(
- "ppl", lambda meters: utils.get_perplexity(meters["nll_loss"].avg)
- )
-
- @staticmethod
- def logging_outputs_can_be_summed() -> bool:
- """
- Whether the logging outputs returned by `forward` can be summed
- across workers prior to calling `reduce_metrics`. Setting this
- to True will improves distributed training speed.
- """
- return True
diff --git a/spaces/Harveenchadha/Vakyansh-Malayalam-TTS/ttsv/__init__.py b/spaces/Harveenchadha/Vakyansh-Malayalam-TTS/ttsv/__init__.py
deleted file mode 100644
index e69de29bb2d1d6434b8b29ae775ad8c2e48c5391..0000000000000000000000000000000000000000
diff --git a/spaces/Harveenchadha/Vakyansh-Odia-TTS/ttsv/src/glow_tts/modules.py b/spaces/Harveenchadha/Vakyansh-Odia-TTS/ttsv/src/glow_tts/modules.py
deleted file mode 100644
index a192251aaccb036780d77d6c8b538b652a5e24e2..0000000000000000000000000000000000000000
--- a/spaces/Harveenchadha/Vakyansh-Odia-TTS/ttsv/src/glow_tts/modules.py
+++ /dev/null
@@ -1,276 +0,0 @@
-import copy
-import math
-import numpy as np
-import scipy
-import torch
-from torch import nn
-from torch.nn import functional as F
-
-import commons
-
-
-class LayerNorm(nn.Module):
- def __init__(self, channels, eps=1e-4):
- super().__init__()
- self.channels = channels
- self.eps = eps
-
- self.gamma = nn.Parameter(torch.ones(channels))
- self.beta = nn.Parameter(torch.zeros(channels))
-
- def forward(self, x):
- n_dims = len(x.shape)
- mean = torch.mean(x, 1, keepdim=True)
- variance = torch.mean((x - mean) ** 2, 1, keepdim=True)
-
- x = (x - mean) * torch.rsqrt(variance + self.eps)
-
- shape = [1, -1] + [1] * (n_dims - 2)
- x = x * self.gamma.view(*shape) + self.beta.view(*shape)
- return x
-
-
-class ConvReluNorm(nn.Module):
- def __init__(
- self,
- in_channels,
- hidden_channels,
- out_channels,
- kernel_size,
- n_layers,
- p_dropout,
- ):
- super().__init__()
- self.in_channels = in_channels
- self.hidden_channels = hidden_channels
- self.out_channels = out_channels
- self.kernel_size = kernel_size
- self.n_layers = n_layers
- self.p_dropout = p_dropout
- assert n_layers > 1, "Number of layers should be larger than 0."
-
- self.conv_layers = nn.ModuleList()
- self.norm_layers = nn.ModuleList()
- self.conv_layers.append(
- nn.Conv1d(
- in_channels, hidden_channels, kernel_size, padding=kernel_size // 2
- )
- )
- self.norm_layers.append(LayerNorm(hidden_channels))
- self.relu_drop = nn.Sequential(nn.ReLU(), nn.Dropout(p_dropout))
- for _ in range(n_layers - 1):
- self.conv_layers.append(
- nn.Conv1d(
- hidden_channels,
- hidden_channels,
- kernel_size,
- padding=kernel_size // 2,
- )
- )
- self.norm_layers.append(LayerNorm(hidden_channels))
- self.proj = nn.Conv1d(hidden_channels, out_channels, 1)
- self.proj.weight.data.zero_()
- self.proj.bias.data.zero_()
-
- def forward(self, x, x_mask):
- x_org = x
- for i in range(self.n_layers):
- x = self.conv_layers[i](x * x_mask)
- x = self.norm_layers[i](x)
- x = self.relu_drop(x)
- x = x_org + self.proj(x)
- return x * x_mask
-
-
-class WN(torch.nn.Module):
- def __init__(
- self,
- in_channels,
- hidden_channels,
- kernel_size,
- dilation_rate,
- n_layers,
- gin_channels=0,
- p_dropout=0,
- ):
- super(WN, self).__init__()
- assert kernel_size % 2 == 1
- assert hidden_channels % 2 == 0
- self.in_channels = in_channels
- self.hidden_channels = hidden_channels
- self.kernel_size = (kernel_size,)
- self.dilation_rate = dilation_rate
- self.n_layers = n_layers
- self.gin_channels = gin_channels
- self.p_dropout = p_dropout
-
- self.in_layers = torch.nn.ModuleList()
- self.res_skip_layers = torch.nn.ModuleList()
- self.drop = nn.Dropout(p_dropout)
-
- if gin_channels != 0:
- cond_layer = torch.nn.Conv1d(
- gin_channels, 2 * hidden_channels * n_layers, 1
- )
- self.cond_layer = torch.nn.utils.weight_norm(cond_layer, name="weight")
-
- for i in range(n_layers):
- dilation = dilation_rate ** i
- padding = int((kernel_size * dilation - dilation) / 2)
- in_layer = torch.nn.Conv1d(
- hidden_channels,
- 2 * hidden_channels,
- kernel_size,
- dilation=dilation,
- padding=padding,
- )
- in_layer = torch.nn.utils.weight_norm(in_layer, name="weight")
- self.in_layers.append(in_layer)
-
- # last one is not necessary
- if i < n_layers - 1:
- res_skip_channels = 2 * hidden_channels
- else:
- res_skip_channels = hidden_channels
-
- res_skip_layer = torch.nn.Conv1d(hidden_channels, res_skip_channels, 1)
- res_skip_layer = torch.nn.utils.weight_norm(res_skip_layer, name="weight")
- self.res_skip_layers.append(res_skip_layer)
-
- def forward(self, x, x_mask=None, g=None, **kwargs):
- output = torch.zeros_like(x)
- n_channels_tensor = torch.IntTensor([self.hidden_channels])
-
- if g is not None:
- g = self.cond_layer(g)
-
- for i in range(self.n_layers):
- x_in = self.in_layers[i](x)
- x_in = self.drop(x_in)
- if g is not None:
- cond_offset = i * 2 * self.hidden_channels
- g_l = g[:, cond_offset : cond_offset + 2 * self.hidden_channels, :]
- else:
- g_l = torch.zeros_like(x_in)
-
- acts = commons.fused_add_tanh_sigmoid_multiply(x_in, g_l, n_channels_tensor)
-
- res_skip_acts = self.res_skip_layers[i](acts)
- if i < self.n_layers - 1:
- x = (x + res_skip_acts[:, : self.hidden_channels, :]) * x_mask
- output = output + res_skip_acts[:, self.hidden_channels :, :]
- else:
- output = output + res_skip_acts
- return output * x_mask
-
- def remove_weight_norm(self):
- if self.gin_channels != 0:
- torch.nn.utils.remove_weight_norm(self.cond_layer)
- for l in self.in_layers:
- torch.nn.utils.remove_weight_norm(l)
- for l in self.res_skip_layers:
- torch.nn.utils.remove_weight_norm(l)
-
-
-class ActNorm(nn.Module):
- def __init__(self, channels, ddi=False, **kwargs):
- super().__init__()
- self.channels = channels
- self.initialized = not ddi
-
- self.logs = nn.Parameter(torch.zeros(1, channels, 1))
- self.bias = nn.Parameter(torch.zeros(1, channels, 1))
-
- def forward(self, x, x_mask=None, reverse=False, **kwargs):
- if x_mask is None:
- x_mask = torch.ones(x.size(0), 1, x.size(2)).to(
- device=x.device, dtype=x.dtype
- )
- x_len = torch.sum(x_mask, [1, 2])
- if not self.initialized:
- self.initialize(x, x_mask)
- self.initialized = True
-
- if reverse:
- z = (x - self.bias) * torch.exp(-self.logs) * x_mask
- logdet = None
- else:
- z = (self.bias + torch.exp(self.logs) * x) * x_mask
- logdet = torch.sum(self.logs) * x_len # [b]
-
- return z, logdet
-
- def store_inverse(self):
- pass
-
- def set_ddi(self, ddi):
- self.initialized = not ddi
-
- def initialize(self, x, x_mask):
- with torch.no_grad():
- denom = torch.sum(x_mask, [0, 2])
- m = torch.sum(x * x_mask, [0, 2]) / denom
- m_sq = torch.sum(x * x * x_mask, [0, 2]) / denom
- v = m_sq - (m ** 2)
- logs = 0.5 * torch.log(torch.clamp_min(v, 1e-6))
-
- bias_init = (
- (-m * torch.exp(-logs)).view(*self.bias.shape).to(dtype=self.bias.dtype)
- )
- logs_init = (-logs).view(*self.logs.shape).to(dtype=self.logs.dtype)
-
- self.bias.data.copy_(bias_init)
- self.logs.data.copy_(logs_init)
-
-
-class InvConvNear(nn.Module):
- def __init__(self, channels, n_split=4, no_jacobian=False, **kwargs):
- super().__init__()
- assert n_split % 2 == 0
- self.channels = channels
- self.n_split = n_split
- self.no_jacobian = no_jacobian
-
- w_init = torch.qr(torch.FloatTensor(self.n_split, self.n_split).normal_())[0]
- if torch.det(w_init) < 0:
- w_init[:, 0] = -1 * w_init[:, 0]
- self.weight = nn.Parameter(w_init)
-
- def forward(self, x, x_mask=None, reverse=False, **kwargs):
- b, c, t = x.size()
- assert c % self.n_split == 0
- if x_mask is None:
- x_mask = 1
- x_len = torch.ones((b,), dtype=x.dtype, device=x.device) * t
- else:
- x_len = torch.sum(x_mask, [1, 2])
-
- x = x.view(b, 2, c // self.n_split, self.n_split // 2, t)
- x = (
- x.permute(0, 1, 3, 2, 4)
- .contiguous()
- .view(b, self.n_split, c // self.n_split, t)
- )
-
- if reverse:
- if hasattr(self, "weight_inv"):
- weight = self.weight_inv
- else:
- weight = torch.inverse(self.weight.float()).to(dtype=self.weight.dtype)
- logdet = None
- else:
- weight = self.weight
- if self.no_jacobian:
- logdet = 0
- else:
- logdet = torch.logdet(self.weight) * (c / self.n_split) * x_len # [b]
-
- weight = weight.view(self.n_split, self.n_split, 1, 1)
- z = F.conv2d(x, weight)
-
- z = z.view(b, 2, self.n_split // 2, c // self.n_split, t)
- z = z.permute(0, 1, 3, 2, 4).contiguous().view(b, c, t) * x_mask
- return z, logdet
-
- def store_inverse(self):
- self.weight_inv = torch.inverse(self.weight.float()).to(dtype=self.weight.dtype)
diff --git a/spaces/Heckeroo/waifu-diffusion/README.md b/spaces/Heckeroo/waifu-diffusion/README.md
deleted file mode 100644
index a615352daa14ce97a4c767b8e8541f928b5bc2e5..0000000000000000000000000000000000000000
--- a/spaces/Heckeroo/waifu-diffusion/README.md
+++ /dev/null
@@ -1,12 +0,0 @@
----
-title: Waifu Diffusion
-emoji: 🐠
-colorFrom: blue
-colorTo: yellow
-sdk: gradio
-sdk_version: 3.13.0
-app_file: app.py
-pinned: false
----
-
-Check out the configuration reference at https://huggingface.co/docs/hub/spaces-config-reference
diff --git a/spaces/HenryJJ/llm_template/app.py b/spaces/HenryJJ/llm_template/app.py
deleted file mode 100644
index 0c2065c43f496cc224b21dfb251215dc97ae24a5..0000000000000000000000000000000000000000
--- a/spaces/HenryJJ/llm_template/app.py
+++ /dev/null
@@ -1,64 +0,0 @@
-import gradio as gr
-import openai
-import json
-from pymongo import MongoClient
-import os
-
-# Initialize MongoDB client
-client = MongoClient(os.environ['DB_URL'])
-
-db = client['test']
-collection = db['gradio']
-
-def get_saved_data():
- saved_data = collection.find({}, {"_id": 0, "name": 1})
- options = [item['name'] for item in saved_data]
- return options
-
-def chat_with_gpt(question, api_key, temperature, system_message):
- openai.api_key = api_key
- response = openai.ChatCompletion.create(
- model="gpt-3.5-turbo",
- messages=[
- {"role": "system", "content": system_message},
- {"role": "user", "content": question}
- ],
- temperature=temperature
- )
- assistant_reply = response['choices'][0]['message']['content']
- return f"{assistant_reply}"
-
-def update_dropdown_choices():
- new_choices = get_saved_data()
- return gr.Dropdown.update(choices=new_choices)
-
-def save_to_mongodb(name, question, system_message):
- if not name.strip(): # Check if name is empty or just whitespace
- return "Please enter a name.", None # Return a message and None to indicate no update for the dropdown
- collection.insert_one({"name": name, "question": question, "system_message": system_message})
- return "Saved to MongoDB.", update_dropdown_choices()
-
-def update_textboxes(selected_name):
- selected_data = collection.find_one({"name": selected_name}, {"_id": 0})
- return selected_data['question'], selected_data['system_message'], selected_data['name']
-
-
-with gr.Blocks() as app:
- saved_data_dropdown = gr.Dropdown(get_saved_data(), label="Select Saved Data")
- name = gr.Textbox(lines=1, placeholder="Name", label="Name")
- question = gr.Textbox(lines=2, placeholder="What's your question?", label="Question")
- api_key = gr.Textbox(lines=1, placeholder="Your OpenAI API Key", label="API Key")
- temperature = gr.Slider(minimum=0, maximum=1, step=0.01, value=0.7, label="Temperature")
- system_message = gr.Textbox(lines=1, placeholder="System Message (Optional)", value="You are a helpful assistant.", label="System Message")
-
- with gr.Row():
- chat_btn = gr.Button("Chat with GPT")
- save_btn = gr.Button("Share to community")
-
- output = gr.Textbox(label="Result", interactive=False)
-
- chat_btn.click(chat_with_gpt, inputs=[question, api_key, temperature, system_message], outputs=output)
- save_btn.click(save_to_mongodb, inputs=[name, question, system_message], outputs=[output, saved_data_dropdown])
- saved_data_dropdown.select(update_textboxes, inputs=[saved_data_dropdown], outputs=[question, system_message, name])
-
-app.launch()
diff --git a/spaces/ICML2022/OFA/fairseq/examples/truncated_bptt/transformer_xl_model.py b/spaces/ICML2022/OFA/fairseq/examples/truncated_bptt/transformer_xl_model.py
deleted file mode 100644
index a6c8b25a07276c2ee30c0aa5f0e4b0a2837ed5ca..0000000000000000000000000000000000000000
--- a/spaces/ICML2022/OFA/fairseq/examples/truncated_bptt/transformer_xl_model.py
+++ /dev/null
@@ -1,155 +0,0 @@
-# Copyright (c) Facebook, Inc. and its affiliates.
-#
-# This source code is licensed under the MIT license found in the
-# LICENSE file in the root directory of this source tree.
-
-import logging
-from dataclasses import dataclass, field
-from typing import Dict, List, Optional
-
-import torch
-from fairseq.dataclass import FairseqDataclass
-from fairseq.models import (
- FairseqIncrementalDecoder,
- FairseqLanguageModel,
- register_model,
-)
-from fairseq.modules.checkpoint_activations import checkpoint_wrapper
-from omegaconf import II
-
-
-logger = logging.getLogger(__name__)
-
-
-@dataclass
-class TransformerXLConfig(FairseqDataclass):
- # defaults come from the original Transformer-XL code
- cutoffs: List[int] = field(default_factory=lambda: [20000, 40000, 200000])
- d_model: int = 500
- n_head: int = 10
- d_head: int = 50
- d_inner: int = 1000
- div_val: int = 1
- n_layer: int = 12
- mem_len: int = 0
- clamp_len: int = -1
- same_length: bool = False
- dropout: float = 0.0
- dropatt: float = 0.0
- checkpoint_activations: bool = False
- offload_activations: bool = False
- max_target_positions: int = II("task.max_target_positions")
-
-
-@register_model("transformer_xl", dataclass=TransformerXLConfig)
-class TransformerXLLanguageModel(FairseqLanguageModel):
- @classmethod
- def build_model(cls, cfg: TransformerXLConfig, task):
- return cls(TransformerXLDecoder(cfg, task))
-
-
-class TransformerXLDecoder(FairseqIncrementalDecoder):
- def __init__(self, cfg, task):
- try:
- from transformers.models.transfo_xl import (
- TransfoXLConfig,
- TransfoXLLMHeadModel,
- )
- except ImportError:
- from transformers.configuration_transfo_xl import TransfoXLConfig
- from transformers.modeling_transfo_xl import TransfoXLLMHeadModel
-
- super().__init__(task.target_dictionary)
- self.cfg = cfg
-
- # remove any cutoffs larger than the vocab size
- cutoffs = [
- cutoff for cutoff in cfg.cutoffs if cutoff < len(task.target_dictionary)
- ]
-
- config = TransfoXLConfig(
- vocab_size=len(task.target_dictionary),
- cutoffs=cutoffs,
- d_model=cfg.d_model,
- d_embed=cfg.d_model,
- n_head=cfg.n_head,
- d_head=cfg.d_head,
- d_inner=cfg.d_inner,
- div_val=cfg.div_val,
- n_layer=cfg.n_layer,
- mem_len=cfg.mem_len,
- clamp_len=cfg.clamp_len,
- same_length=cfg.same_length,
- dropout=cfg.dropout,
- dropatt=cfg.dropatt,
- )
- logger.info(config)
- self.model = TransfoXLLMHeadModel(config)
-
- # Workaround a bug in huggingface's ``ProjectedAdaptiveLogSoftmax``
- # which adds ``None`` values to an ``nn.ParameterList``, which is not
- # supported in PyTorch. Instead we can replace this with an
- # ``nn.ModuleList``, which does support ``None`` values.
- try:
- if all(p is None for p in self.model.crit.out_projs._parameters.values()):
- self.model.crit.out_projs = torch.nn.ModuleList(
- [None] * len(self.model.crit.out_projs._parameters)
- )
- except Exception:
- pass
-
- if cfg.checkpoint_activations or cfg.offload_activations:
- for i in range(len(self.model.transformer.layers)):
- self.model.transformer.layers[i] = checkpoint_wrapper(
- self.model.transformer.layers[i],
- offload_to_cpu=cfg.offload_activations,
- )
- # TODO: may save mem to wrap(layer.pos_ff.CoreNet[3])
-
- self._mems = None
-
- def forward(
- self,
- src_tokens,
- src_lengths=None, # unused
- incremental_state: Optional[Dict[str, List[torch.Tensor]]] = None,
- encoder_out=None,
- ):
- if incremental_state is not None: # used during inference
- mems = self.get_incremental_state(incremental_state, "mems")
- src_tokens = src_tokens[:, -1:] # only keep the most recent token
- else:
- mems = self._mems
-
- output = self.model(
- input_ids=src_tokens,
- mems=mems,
- return_dict=False,
- )
-
- if len(output) >= 2:
- if incremental_state is not None:
- self.set_incremental_state(incremental_state, "mems", output[1])
- else:
- self._mems = output[1]
-
- return (output[0],)
-
- def max_positions(self):
- return self.cfg.max_target_positions
-
- def reorder_incremental_state(
- self,
- incremental_state: Dict[str, Dict[str, Optional[torch.Tensor]]],
- new_order: torch.Tensor,
- ):
- """Reorder incremental state.
-
- This will be called when the order of the input has changed from the
- previous time step. A typical use case is beam search, where the input
- order changes between time steps based on the selection of beams.
- """
- mems = self.get_incremental_state(incremental_state, "mems")
- if mems is not None:
- new_mems = [mems_i.index_select(1, new_order) for mems_i in mems]
- self.set_incremental_state(incremental_state, "mems", new_mems)
diff --git a/spaces/ICML2022/OFA/fairseq/fairseq/modules/__init__.py b/spaces/ICML2022/OFA/fairseq/fairseq/modules/__init__.py
deleted file mode 100644
index d7a030e2b5cbca30e6a4ca4f8a17a62a8cf197af..0000000000000000000000000000000000000000
--- a/spaces/ICML2022/OFA/fairseq/fairseq/modules/__init__.py
+++ /dev/null
@@ -1,82 +0,0 @@
-# Copyright (c) Facebook, Inc. and its affiliates.
-#
-# This source code is licensed under the MIT license found in the
-# LICENSE file in the root directory of this source tree.
-"""isort:skip_file"""
-
-from .adaptive_input import AdaptiveInput
-from .adaptive_softmax import AdaptiveSoftmax
-from .base_layer import BaseLayer
-from .beamable_mm import BeamableMM
-from .character_token_embedder import CharacterTokenEmbedder
-from .conv_tbc import ConvTBC
-from .cross_entropy import cross_entropy
-from .downsampled_multihead_attention import DownsampledMultiHeadAttention
-from .dynamic_convolution import DynamicConv, DynamicConv1dTBC
-from .dynamic_crf_layer import DynamicCRF
-from .fairseq_dropout import FairseqDropout
-from .fp32_group_norm import Fp32GroupNorm
-from .gelu import gelu, gelu_accurate
-from .grad_multiply import GradMultiply
-from .gumbel_vector_quantizer import GumbelVectorQuantizer
-from .kmeans_vector_quantizer import KmeansVectorQuantizer
-from .layer_drop import LayerDropModuleList
-from .layer_norm import Fp32LayerNorm, LayerNorm
-from .learned_positional_embedding import LearnedPositionalEmbedding
-from .lightweight_convolution import LightweightConv, LightweightConv1dTBC
-from .linearized_convolution import LinearizedConvolution
-from .location_attention import LocationAttention
-from .lstm_cell_with_zoneout import LSTMCellWithZoneOut
-from .multihead_attention import MultiheadAttention
-from .positional_embedding import PositionalEmbedding
-from .same_pad import SamePad
-from .scalar_bias import ScalarBias
-from .sinusoidal_positional_embedding import SinusoidalPositionalEmbedding
-from .transformer_sentence_encoder_layer import TransformerSentenceEncoderLayer
-from .transformer_sentence_encoder import TransformerSentenceEncoder
-from .transpose_last import TransposeLast
-from .unfold import unfold1d
-from .transformer_layer import TransformerDecoderLayer, TransformerEncoderLayer
-from .vggblock import VGGBlock
-
-__all__ = [
- "AdaptiveInput",
- "AdaptiveSoftmax",
- "BaseLayer",
- "BeamableMM",
- "CharacterTokenEmbedder",
- "ConvTBC",
- "cross_entropy",
- "DownsampledMultiHeadAttention",
- "DynamicConv1dTBC",
- "DynamicConv",
- "DynamicCRF",
- "FairseqDropout",
- "Fp32GroupNorm",
- "Fp32LayerNorm",
- "gelu",
- "gelu_accurate",
- "GradMultiply",
- "GumbelVectorQuantizer",
- "KmeansVectorQuantizer",
- "LayerDropModuleList",
- "LayerNorm",
- "LearnedPositionalEmbedding",
- "LightweightConv1dTBC",
- "LightweightConv",
- "LinearizedConvolution",
- "LocationAttention",
- "LSTMCellWithZoneOut",
- "MultiheadAttention",
- "PositionalEmbedding",
- "SamePad",
- "ScalarBias",
- "SinusoidalPositionalEmbedding",
- "TransformerSentenceEncoderLayer",
- "TransformerSentenceEncoder",
- "TransformerDecoderLayer",
- "TransformerEncoderLayer",
- "TransposeLast",
- "VGGBlock",
- "unfold1d",
-]
diff --git a/spaces/IDEA-Research/Grounded-SAM/segment_anything/scripts/export_onnx_model.py b/spaces/IDEA-Research/Grounded-SAM/segment_anything/scripts/export_onnx_model.py
deleted file mode 100644
index 8ec5c2ec24fc53cd9fdf66564cfe163b9eb26c24..0000000000000000000000000000000000000000
--- a/spaces/IDEA-Research/Grounded-SAM/segment_anything/scripts/export_onnx_model.py
+++ /dev/null
@@ -1,204 +0,0 @@
-# Copyright (c) Meta Platforms, Inc. and affiliates.
-# All rights reserved.
-
-# This source code is licensed under the license found in the
-# LICENSE file in the root directory of this source tree.
-
-import torch
-
-from segment_anything import build_sam, build_sam_vit_b, build_sam_vit_l
-from segment_anything.utils.onnx import SamOnnxModel
-
-import argparse
-import warnings
-
-try:
- import onnxruntime # type: ignore
-
- onnxruntime_exists = True
-except ImportError:
- onnxruntime_exists = False
-
-parser = argparse.ArgumentParser(
- description="Export the SAM prompt encoder and mask decoder to an ONNX model."
-)
-
-parser.add_argument(
- "--checkpoint", type=str, required=True, help="The path to the SAM model checkpoint."
-)
-
-parser.add_argument(
- "--output", type=str, required=True, help="The filename to save the ONNX model to."
-)
-
-parser.add_argument(
- "--model-type",
- type=str,
- default="default",
- help="In ['default', 'vit_b', 'vit_l']. Which type of SAM model to export.",
-)
-
-parser.add_argument(
- "--return-single-mask",
- action="store_true",
- help=(
- "If true, the exported ONNX model will only return the best mask, "
- "instead of returning multiple masks. For high resolution images "
- "this can improve runtime when upscaling masks is expensive."
- ),
-)
-
-parser.add_argument(
- "--opset",
- type=int,
- default=17,
- help="The ONNX opset version to use. Must be >=11",
-)
-
-parser.add_argument(
- "--quantize-out",
- type=str,
- default=None,
- help=(
- "If set, will quantize the model and save it with this name. "
- "Quantization is performed with quantize_dynamic from onnxruntime.quantization.quantize."
- ),
-)
-
-parser.add_argument(
- "--gelu-approximate",
- action="store_true",
- help=(
- "Replace GELU operations with approximations using tanh. Useful "
- "for some runtimes that have slow or unimplemented erf ops, used in GELU."
- ),
-)
-
-parser.add_argument(
- "--use-stability-score",
- action="store_true",
- help=(
- "Replaces the model's predicted mask quality score with the stability "
- "score calculated on the low resolution masks using an offset of 1.0. "
- ),
-)
-
-parser.add_argument(
- "--return-extra-metrics",
- action="store_true",
- help=(
- "The model will return five results: (masks, scores, stability_scores, "
- "areas, low_res_logits) instead of the usual three. This can be "
- "significantly slower for high resolution outputs."
- ),
-)
-
-
-def run_export(
- model_type: str,
- checkpoint: str,
- output: str,
- opset: int,
- return_single_mask: bool,
- gelu_approximate: bool = False,
- use_stability_score: bool = False,
- return_extra_metrics=False,
-):
- print("Loading model...")
- if model_type == "vit_b":
- sam = build_sam_vit_b(checkpoint)
- elif model_type == "vit_l":
- sam = build_sam_vit_l(checkpoint)
- else:
- sam = build_sam(checkpoint)
-
- onnx_model = SamOnnxModel(
- model=sam,
- return_single_mask=return_single_mask,
- use_stability_score=use_stability_score,
- return_extra_metrics=return_extra_metrics,
- )
-
- if gelu_approximate:
- for n, m in onnx_model.named_modules():
- if isinstance(m, torch.nn.GELU):
- m.approximate = "tanh"
-
- dynamic_axes = {
- "point_coords": {1: "num_points"},
- "point_labels": {1: "num_points"},
- }
-
- embed_dim = sam.prompt_encoder.embed_dim
- embed_size = sam.prompt_encoder.image_embedding_size
- mask_input_size = [4 * x for x in embed_size]
- dummy_inputs = {
- "image_embeddings": torch.randn(1, embed_dim, *embed_size, dtype=torch.float),
- "point_coords": torch.randint(low=0, high=1024, size=(1, 5, 2), dtype=torch.float),
- "point_labels": torch.randint(low=0, high=4, size=(1, 5), dtype=torch.float),
- "mask_input": torch.randn(1, 1, *mask_input_size, dtype=torch.float),
- "has_mask_input": torch.tensor([1], dtype=torch.float),
- "orig_im_size": torch.tensor([1500, 2250], dtype=torch.float),
- }
-
- _ = onnx_model(**dummy_inputs)
-
- output_names = ["masks", "iou_predictions", "low_res_masks"]
-
- with warnings.catch_warnings():
- warnings.filterwarnings("ignore", category=torch.jit.TracerWarning)
- warnings.filterwarnings("ignore", category=UserWarning)
- with open(output, "wb") as f:
- print(f"Exporing onnx model to {output}...")
- torch.onnx.export(
- onnx_model,
- tuple(dummy_inputs.values()),
- f,
- export_params=True,
- verbose=False,
- opset_version=opset,
- do_constant_folding=True,
- input_names=list(dummy_inputs.keys()),
- output_names=output_names,
- dynamic_axes=dynamic_axes,
- )
-
- if onnxruntime_exists:
- ort_inputs = {k: to_numpy(v) for k, v in dummy_inputs.items()}
- ort_session = onnxruntime.InferenceSession(output)
- _ = ort_session.run(None, ort_inputs)
- print("Model has successfully been run with ONNXRuntime.")
-
-
-def to_numpy(tensor):
- return tensor.cpu().numpy()
-
-
-if __name__ == "__main__":
- args = parser.parse_args()
- run_export(
- model_type=args.model_type,
- checkpoint=args.checkpoint,
- output=args.output,
- opset=args.opset,
- return_single_mask=args.return_single_mask,
- gelu_approximate=args.gelu_approximate,
- use_stability_score=args.use_stability_score,
- return_extra_metrics=args.return_extra_metrics,
- )
-
- if args.quantize_out is not None:
- assert onnxruntime_exists, "onnxruntime is required to quantize the model."
- from onnxruntime.quantization import QuantType # type: ignore
- from onnxruntime.quantization.quantize import quantize_dynamic # type: ignore
-
- print(f"Quantizing model and writing to {args.quantize_out}...")
- quantize_dynamic(
- model_input=args.output,
- model_output=args.quantize_out,
- optimize_model=True,
- per_channel=False,
- reduce_range=False,
- weight_type=QuantType.QUInt8,
- )
- print("Done!")
diff --git a/spaces/Iceclear/StableSR/StableSR/basicsr/losses/basic_loss.py b/spaces/Iceclear/StableSR/StableSR/basicsr/losses/basic_loss.py
deleted file mode 100644
index d2e965526a9b0e2686575bf93f0173cc2664d9bb..0000000000000000000000000000000000000000
--- a/spaces/Iceclear/StableSR/StableSR/basicsr/losses/basic_loss.py
+++ /dev/null
@@ -1,253 +0,0 @@
-import torch
-from torch import nn as nn
-from torch.nn import functional as F
-
-from basicsr.archs.vgg_arch import VGGFeatureExtractor
-from basicsr.utils.registry import LOSS_REGISTRY
-from .loss_util import weighted_loss
-
-_reduction_modes = ['none', 'mean', 'sum']
-
-
-@weighted_loss
-def l1_loss(pred, target):
- return F.l1_loss(pred, target, reduction='none')
-
-
-@weighted_loss
-def mse_loss(pred, target):
- return F.mse_loss(pred, target, reduction='none')
-
-
-@weighted_loss
-def charbonnier_loss(pred, target, eps=1e-12):
- return torch.sqrt((pred - target)**2 + eps)
-
-
-@LOSS_REGISTRY.register()
-class L1Loss(nn.Module):
- """L1 (mean absolute error, MAE) loss.
-
- Args:
- loss_weight (float): Loss weight for L1 loss. Default: 1.0.
- reduction (str): Specifies the reduction to apply to the output.
- Supported choices are 'none' | 'mean' | 'sum'. Default: 'mean'.
- """
-
- def __init__(self, loss_weight=1.0, reduction='mean'):
- super(L1Loss, self).__init__()
- if reduction not in ['none', 'mean', 'sum']:
- raise ValueError(f'Unsupported reduction mode: {reduction}. Supported ones are: {_reduction_modes}')
-
- self.loss_weight = loss_weight
- self.reduction = reduction
-
- def forward(self, pred, target, weight=None, **kwargs):
- """
- Args:
- pred (Tensor): of shape (N, C, H, W). Predicted tensor.
- target (Tensor): of shape (N, C, H, W). Ground truth tensor.
- weight (Tensor, optional): of shape (N, C, H, W). Element-wise weights. Default: None.
- """
- return self.loss_weight * l1_loss(pred, target, weight, reduction=self.reduction)
-
-
-@LOSS_REGISTRY.register()
-class MSELoss(nn.Module):
- """MSE (L2) loss.
-
- Args:
- loss_weight (float): Loss weight for MSE loss. Default: 1.0.
- reduction (str): Specifies the reduction to apply to the output.
- Supported choices are 'none' | 'mean' | 'sum'. Default: 'mean'.
- """
-
- def __init__(self, loss_weight=1.0, reduction='mean'):
- super(MSELoss, self).__init__()
- if reduction not in ['none', 'mean', 'sum']:
- raise ValueError(f'Unsupported reduction mode: {reduction}. Supported ones are: {_reduction_modes}')
-
- self.loss_weight = loss_weight
- self.reduction = reduction
-
- def forward(self, pred, target, weight=None, **kwargs):
- """
- Args:
- pred (Tensor): of shape (N, C, H, W). Predicted tensor.
- target (Tensor): of shape (N, C, H, W). Ground truth tensor.
- weight (Tensor, optional): of shape (N, C, H, W). Element-wise weights. Default: None.
- """
- return self.loss_weight * mse_loss(pred, target, weight, reduction=self.reduction)
-
-
-@LOSS_REGISTRY.register()
-class CharbonnierLoss(nn.Module):
- """Charbonnier loss (one variant of Robust L1Loss, a differentiable
- variant of L1Loss).
-
- Described in "Deep Laplacian Pyramid Networks for Fast and Accurate
- Super-Resolution".
-
- Args:
- loss_weight (float): Loss weight for L1 loss. Default: 1.0.
- reduction (str): Specifies the reduction to apply to the output.
- Supported choices are 'none' | 'mean' | 'sum'. Default: 'mean'.
- eps (float): A value used to control the curvature near zero. Default: 1e-12.
- """
-
- def __init__(self, loss_weight=1.0, reduction='mean', eps=1e-12):
- super(CharbonnierLoss, self).__init__()
- if reduction not in ['none', 'mean', 'sum']:
- raise ValueError(f'Unsupported reduction mode: {reduction}. Supported ones are: {_reduction_modes}')
-
- self.loss_weight = loss_weight
- self.reduction = reduction
- self.eps = eps
-
- def forward(self, pred, target, weight=None, **kwargs):
- """
- Args:
- pred (Tensor): of shape (N, C, H, W). Predicted tensor.
- target (Tensor): of shape (N, C, H, W). Ground truth tensor.
- weight (Tensor, optional): of shape (N, C, H, W). Element-wise weights. Default: None.
- """
- return self.loss_weight * charbonnier_loss(pred, target, weight, eps=self.eps, reduction=self.reduction)
-
-
-@LOSS_REGISTRY.register()
-class WeightedTVLoss(L1Loss):
- """Weighted TV loss.
-
- Args:
- loss_weight (float): Loss weight. Default: 1.0.
- """
-
- def __init__(self, loss_weight=1.0, reduction='mean'):
- if reduction not in ['mean', 'sum']:
- raise ValueError(f'Unsupported reduction mode: {reduction}. Supported ones are: mean | sum')
- super(WeightedTVLoss, self).__init__(loss_weight=loss_weight, reduction=reduction)
-
- def forward(self, pred, weight=None):
- if weight is None:
- y_weight = None
- x_weight = None
- else:
- y_weight = weight[:, :, :-1, :]
- x_weight = weight[:, :, :, :-1]
-
- y_diff = super().forward(pred[:, :, :-1, :], pred[:, :, 1:, :], weight=y_weight)
- x_diff = super().forward(pred[:, :, :, :-1], pred[:, :, :, 1:], weight=x_weight)
-
- loss = x_diff + y_diff
-
- return loss
-
-
-@LOSS_REGISTRY.register()
-class PerceptualLoss(nn.Module):
- """Perceptual loss with commonly used style loss.
-
- Args:
- layer_weights (dict): The weight for each layer of vgg feature.
- Here is an example: {'conv5_4': 1.}, which means the conv5_4
- feature layer (before relu5_4) will be extracted with weight
- 1.0 in calculating losses.
- vgg_type (str): The type of vgg network used as feature extractor.
- Default: 'vgg19'.
- use_input_norm (bool): If True, normalize the input image in vgg.
- Default: True.
- range_norm (bool): If True, norm images with range [-1, 1] to [0, 1].
- Default: False.
- perceptual_weight (float): If `perceptual_weight > 0`, the perceptual
- loss will be calculated and the loss will multiplied by the
- weight. Default: 1.0.
- style_weight (float): If `style_weight > 0`, the style loss will be
- calculated and the loss will multiplied by the weight.
- Default: 0.
- criterion (str): Criterion used for perceptual loss. Default: 'l1'.
- """
-
- def __init__(self,
- layer_weights,
- vgg_type='vgg19',
- use_input_norm=True,
- range_norm=False,
- perceptual_weight=1.0,
- style_weight=0.,
- criterion='l1'):
- super(PerceptualLoss, self).__init__()
- self.perceptual_weight = perceptual_weight
- self.style_weight = style_weight
- self.layer_weights = layer_weights
- self.vgg = VGGFeatureExtractor(
- layer_name_list=list(layer_weights.keys()),
- vgg_type=vgg_type,
- use_input_norm=use_input_norm,
- range_norm=range_norm)
-
- self.criterion_type = criterion
- if self.criterion_type == 'l1':
- self.criterion = torch.nn.L1Loss()
- elif self.criterion_type == 'l2':
- self.criterion = torch.nn.L2loss()
- elif self.criterion_type == 'fro':
- self.criterion = None
- else:
- raise NotImplementedError(f'{criterion} criterion has not been supported.')
-
- def forward(self, x, gt):
- """Forward function.
-
- Args:
- x (Tensor): Input tensor with shape (n, c, h, w).
- gt (Tensor): Ground-truth tensor with shape (n, c, h, w).
-
- Returns:
- Tensor: Forward results.
- """
- # extract vgg features
- x_features = self.vgg(x)
- gt_features = self.vgg(gt.detach())
-
- # calculate perceptual loss
- if self.perceptual_weight > 0:
- percep_loss = 0
- for k in x_features.keys():
- if self.criterion_type == 'fro':
- percep_loss += torch.norm(x_features[k] - gt_features[k], p='fro') * self.layer_weights[k]
- else:
- percep_loss += self.criterion(x_features[k], gt_features[k]) * self.layer_weights[k]
- percep_loss *= self.perceptual_weight
- else:
- percep_loss = None
-
- # calculate style loss
- if self.style_weight > 0:
- style_loss = 0
- for k in x_features.keys():
- if self.criterion_type == 'fro':
- style_loss += torch.norm(
- self._gram_mat(x_features[k]) - self._gram_mat(gt_features[k]), p='fro') * self.layer_weights[k]
- else:
- style_loss += self.criterion(self._gram_mat(x_features[k]), self._gram_mat(
- gt_features[k])) * self.layer_weights[k]
- style_loss *= self.style_weight
- else:
- style_loss = None
-
- return percep_loss, style_loss
-
- def _gram_mat(self, x):
- """Calculate Gram matrix.
-
- Args:
- x (torch.Tensor): Tensor with shape of (n, c, h, w).
-
- Returns:
- torch.Tensor: Gram matrix.
- """
- n, c, h, w = x.size()
- features = x.view(n, c, w * h)
- features_t = features.transpose(1, 2)
- gram = features.bmm(features_t) / (c * h * w)
- return gram
diff --git a/spaces/Illumotion/Koboldcpp/examples/benchmark/benchmark-matmult.cpp b/spaces/Illumotion/Koboldcpp/examples/benchmark/benchmark-matmult.cpp
deleted file mode 100644
index f1c382aa9b9557a2636b8ca8d6703cc27c03d362..0000000000000000000000000000000000000000
--- a/spaces/Illumotion/Koboldcpp/examples/benchmark/benchmark-matmult.cpp
+++ /dev/null
@@ -1,275 +0,0 @@
-#include "build-info.h"
-#include "common.h"
-#include "ggml.h"
-
-#include
-#include
-#include
-#include
-#include
-#include
-#include
-#include
-#include
-#include
-#include
-#include
-#include
-#include
-
-#if defined(_MSC_VER)
-#pragma warning(disable: 4244 4267) // possible loss of data
-#endif
-
-static void ggml_graph_compute_helper(std::vector & buf, ggml_cgraph * graph, int n_threads) {
- struct ggml_cplan plan = ggml_graph_plan(graph, n_threads);
-
- if (plan.work_size > 0) {
- buf.resize(plan.work_size);
- plan.work_data = buf.data();
- }
-
- ggml_graph_compute(graph, &plan);
-}
-
-static float tensor_sum_elements(const ggml_tensor * tensor) {
- double sum = 0;
- if (tensor->type == GGML_TYPE_F32) {
- for (int j = 0; j < tensor->ne[1]; j++) {
- for (int k = 0; k < tensor->ne[0]; k++) {
- sum += ((float *) tensor->data)[j*tensor->ne[0] + k];
- }
- }
- }
- return sum;
-}
-
-static void tensor_dump(const ggml_tensor * tensor, const char * name) {
- printf("%15s: type = %i (%5s) ne = %5" PRIi64 " x %5" PRIi64 " x %5" PRIi64 ", nb = (%5zi, %5zi, %5zi) - ", name,
- tensor->type, ggml_type_name(tensor->type),
- tensor->ne[0], tensor->ne[1], tensor->ne[2], tensor->nb[0], tensor->nb[1], tensor->nb[2]);
- float sum = tensor_sum_elements(tensor);
- printf("Sum of tensor %s is %6.2f\n", name, sum);
-}
-
-#define TENSOR_DUMP(tensor) tensor_dump(tensor, #tensor)
-
-struct benchmark_params_struct {
- int32_t n_threads = 1;
- int32_t n_iterations = 10;
-};
-
-static void print_usage(int /*argc*/, char ** argv, struct benchmark_params_struct params) {
- fprintf(stderr, "usage: %s [options]\n", argv[0]);
- fprintf(stderr, "\n");
- fprintf(stderr, "options:\n");
- fprintf(stderr, " -h, --help show this help message and exit\n");
- fprintf(stderr, " -t N, --threads N number of threads to use during computation (default: %d)\n", params.n_threads);
- fprintf(stderr, " -i N, --iter N number of iterations to use during computation (default: %d)\n", params.n_iterations);
- fprintf(stderr, "\n");
-}
-
-int main(int argc, char ** argv) {
- struct benchmark_params_struct benchmark_params;
-
- bool invalid_param = false;
- std::string arg;
- for (int i = 1; i < argc; i++) {
- arg = argv[i];
-
- if (arg == "-t" || arg == "--threads") {
- if (++i >= argc) {
- invalid_param = true;
- break;
- }
- benchmark_params.n_threads = std::stoi(argv[i]);
- } else if (arg == "-i" || arg == "--iter") {
- if (++i >= argc) {
- invalid_param = true;
- break;
- }
- benchmark_params.n_iterations = std::stoi(argv[i]);
- } else if (arg == "-h" || arg == "--help") {
- print_usage(argc, argv, benchmark_params);
- exit(0);
- }
- }
- if (invalid_param) {
- fprintf(stderr, "error: invalid parameter for argument: %s\n", arg.c_str());
- print_usage(argc, argv, benchmark_params);
- exit(1);
- }
-
- print_build_info();
- printf("Starting Test\n");
-
- // create the ggml context
- struct ggml_context * ctx;
- //const int sizex = 4096;
- //const int sizey = 11008;
-
-#undef VERBOSE_DEBUGGING
-#ifndef VERBOSE_DEBUGGING
- const int sizey = 4096;
- const int sizex = 11008;
- const int sizez = 128;
-#else
- /* Working - let's increase size */
- const int sizey = 1;
- const int sizex = (8*32);
- const int sizez = 1;
-
- /*const int sizey = 1;
- const int sizex = 3*(8*32);
- const int sizez = 1;*/
-#endif
-
- //printf("Memsize required = %i\n", sizex*sizex);
-
- // TODO: perform the bench for all types or for a user specified type
- const ggml_type qtype = GGML_TYPE_Q4_1;
-
- size_t ctx_size = 0;
- ctx_size += sizex*sizey*ggml_type_sizef(GGML_TYPE_F32);
- ctx_size += sizex*sizey*ggml_type_sizef(GGML_TYPE_F32);
- ctx_size += sizex*sizez*ggml_type_sizef(GGML_TYPE_F32);
- ctx_size += sizex*sizey*ggml_type_sizef(qtype);
- ctx_size += sizex*sizey*ggml_type_sizef(qtype);
- ctx_size += sizex*sizey*ggml_type_sizef(GGML_TYPE_F32); // BLAS
- ctx_size += sizex*sizey*ggml_type_sizef(GGML_TYPE_F32); // BLAS
- ctx_size += 1024*1024*16;
-
- printf("Allocating Memory of size %zi bytes, %zi MB\n",ctx_size, (ctx_size/1024/1024));
-
- struct ggml_init_params params = {
- /*.mem_size =*/ ctx_size,
- /*.mem_buffer =*/ NULL,
- /* no_alloc =*/ 0
- };
-
- ctx = ggml_init(params);
- if (!ctx) {
- fprintf(stderr, "%s: ggml_init() failed\n", __func__);
- return 1;
- }
-
-
- printf("Creating new tensors\n");
- // printf("Creating new tensor m1\n");
- struct ggml_tensor * m11 = ggml_new_tensor_2d(ctx, GGML_TYPE_F32, sizex, sizey);
- ggml_set_f32(m11, 1.0f);
-
- // printf("Creating new tensor m1\n");
- struct ggml_tensor * m12 = ggml_new_tensor_2d(ctx, GGML_TYPE_F32, sizex, sizey);
- ggml_set_f32(m12, 1.5f);
-
- // printf("Creating new tensor m2\n");
- struct ggml_tensor * m2 = ggml_new_tensor_2d(ctx, GGML_TYPE_F32, sizex, sizez);
- ggml_set_f32(m2, 2.0f);
-
- printf("\n------ Test 1 - Matrix Mult via F32 code\n");
- // printf("Creating new tensor m11xm2\n");
- struct ggml_tensor * m11xm2 = ggml_mul_mat(ctx, m11, m2);
-
- // printf("Creating compute graph\n");
- struct ggml_cgraph gf = ggml_build_forward(m11xm2);
-
- printf("n_threads=%i\n", benchmark_params.n_threads);
-
- TENSOR_DUMP(m11);
- TENSOR_DUMP(m2);
-
- std::vector work_buffer;
-
- ggml_graph_compute_helper(work_buffer, &gf, benchmark_params.n_threads);
-
- TENSOR_DUMP(gf.nodes[0]);
-
- printf("\n------ Test 2 - Matrix Mult via %s code\n", ggml_type_name(qtype));
-
- int32_t nelements = sizex*sizey;
-
- std::vector hist_cur(1 << 4, 0);
-
- // Set up a the benchmark matrices
- // printf("Creating new tensor q11 & Running quantize\n");
- struct ggml_tensor * q11 = ggml_new_tensor_2d(ctx, qtype, sizex, sizey);
- ggml_quantize_chunk(qtype, (const float *) m11->data, q11->data, 0, nelements, hist_cur.data());
-
- // Set up a the compute graph
- // printf("Creating new tensor q31\n");
- struct ggml_tensor * q31 = ggml_mul_mat(ctx, q11, m2);
-
- // printf("Creating compute graph\n");
- struct ggml_cgraph gf31 = ggml_build_forward(q31);
-
- // Set up a second graph computation to make sure we override the CPU cache lines
- // printf("Creating new tensor q12 & Running quantize\n");
- struct ggml_tensor * q12 = ggml_new_tensor_2d(ctx, qtype, sizex, sizey);
- ggml_quantize_chunk(qtype, (const float *) m12->data, q12->data, 0, nelements, hist_cur.data());
-
- // printf("Creating new tensor q32\n");
- struct ggml_tensor * q32 = ggml_mul_mat(ctx, q12, m2);
-
- //printf("Creating compute graph\n");
- struct ggml_cgraph gf32 = ggml_build_forward(q32);
- printf("n_threads=%i\n", benchmark_params.n_threads);
-
- const int dimx = sizex;
- const int dimy = sizey;
- const int dimz = sizez;
- long long int flops_per_dot_product = dimy + dimy;
- long long int flops_per_matrix = flops_per_dot_product * dimx * dimz; ;
- printf("Matrix Multiplication of (%i,%i,%i) x (%i,%i,%i) - about %6.2f gFLOPS\n\n", sizex, sizey, 1, sizex, sizez, 1, 1.0f*flops_per_matrix / 1000 / 1000 / 1000);
-
-
- // Let's use the F32 result from above as a reference for the quantized multiplication
- float sum_of_F32_reference = tensor_sum_elements(gf.nodes[0]);
-
- printf("Iteration;NThreads; SizeX; SizeY; SizeZ; Required_FLOPS; Elapsed_u_Seconds; gigaFLOPS\n");
- printf("=====================================================================================\n");
-
- double gflops_sum = 0;
- for (int i=0;i allowed_delta) {
- printf("\nABORT - ERROR in Matrix Multiplication result - expected %6.2f, got %6.2f (delta %6.2f > allowed_delta %6.2f)\n",
- sum_of_F32_reference,
- sum_of_Q4_result,
- delta,
- allowed_delta
- );
- exit(0);
- }
-
- // Running a different graph computation to make sure we override the CPU cache lines
- ggml_graph_compute_helper(work_buffer, &gf32, benchmark_params.n_threads);
- }
- printf("\n");
- printf("Average%78.2f\n",gflops_sum/((double)benchmark_params.n_iterations));
- printf("=====================================================================================\n");
-}
diff --git a/spaces/Intel/ldm3d/static/public/js/WebVR.js b/spaces/Intel/ldm3d/static/public/js/WebVR.js
deleted file mode 100644
index 20808284bc2e2c5e57ad213bda90626c481f3d2c..0000000000000000000000000000000000000000
--- a/spaces/Intel/ldm3d/static/public/js/WebVR.js
+++ /dev/null
@@ -1,261 +0,0 @@
-/**
- * @author mrdoob / http://mrdoob.com
- * @author Mugen87 / https://github.com/Mugen87
- *
- * Based on @tojiro's vr-samples-utils.js
- */
-
-THREE.WEBVR = {
-
- createButton: function ( renderer, options ) {
-
- if ( options && options.referenceSpaceType ) {
-
- renderer.vr.setReferenceSpaceType( options.referenceSpaceType );
-
- }
-
- function showEnterVR( device ) {
-
- button.style.display = '';
-
- button.style.cursor = 'pointer';
- button.style.left = 'calc(50% - 50px)';
- button.style.width = '100px';
-
- button.textContent = 'ENTER VR';
-
- button.onmouseenter = function () {
-
- button.style.opacity = '1.0';
-
- };
-
- button.onmouseleave = function () {
-
- button.style.opacity = '0.5';
-
- };
-
- button.onclick = function () {
-
- device.isPresenting ? device.exitPresent() : device.requestPresent( [ { source: renderer.domElement } ] );
-
- };
-
- renderer.vr.setDevice( device );
-
- }
-
- function showEnterXR( /*device*/ ) {
-
- var currentSession = null;
-
- function onSessionStarted( session ) {
-
- session.addEventListener( 'end', onSessionEnded );
-
- renderer.vr.setSession( session );
- button.textContent = 'EXIT XR';
-
- currentSession = session;
-
- }
-
- function onSessionEnded( /*event*/ ) {
-
- currentSession.removeEventListener( 'end', onSessionEnded );
-
- renderer.vr.setSession( null );
- button.textContent = 'ENTER XR';
-
- currentSession = null;
-
- }
-
- //
-
- button.style.display = '';
-
- button.style.cursor = 'pointer';
- button.style.left = 'calc(50% - 50px)';
- button.style.width = '100px';
-
- button.textContent = 'ENTER XR';
-
- button.onmouseenter = function () {
-
- button.style.opacity = '1.0';
-
- };
-
- button.onmouseleave = function () {
-
- button.style.opacity = '0.5';
-
- };
-
- button.onclick = function () {
-
- if ( currentSession === null ) {
-
- // WebXR's requestReferenceSpace only works if the corresponding feature
- // was requested at session creation time. For simplicity, just ask for
- // the interesting ones as optional features, but be aware that the
- // requestReferenceSpace call will fail if it turns out to be unavailable.
- // ('local' is always available for immersive sessions and doesn't need to
- // be requested separately.)
-
- var sessionInit = { optionalFeatures: [ 'local-floor', 'bounded-floor' ] };
- navigator.xr.requestSession( 'immersive-vr', sessionInit ).then( onSessionStarted );
-
- } else {
-
- currentSession.end();
-
- }
-
- };
-
- }
-
- function disableButton() {
-
- button.style.display = '';
-
- button.style.cursor = 'auto';
- button.style.left = 'calc(50% - 75px)';
- button.style.width = '150px';
-
- button.onmouseenter = null;
- button.onmouseleave = null;
-
- button.onclick = null;
-
- }
-
- function showVRNotFound() {
-
- disableButton();
-
- button.textContent = 'VR NOT FOUND';
-
- renderer.vr.setDevice( null );
-
- }
-
- function showXRNotFound() {
-
- disableButton();
-
- button.textContent = 'XR NOT FOUND';
-
- }
-
- function stylizeElement( element ) {
-
- element.style.position = 'absolute';
- element.style.bottom = '20px';
- element.style.padding = '12px 6px';
- element.style.border = '1px solid #fff';
- element.style.borderRadius = '4px';
- element.style.background = 'rgba(0,0,0,0.1)';
- element.style.color = '#fff';
- element.style.font = 'normal 13px sans-serif';
- element.style.textAlign = 'center';
- element.style.opacity = '0.5';
- element.style.outline = 'none';
- element.style.zIndex = '999';
-
- }
-
- if ( 'xr' in navigator ) {
-
- var button = document.createElement( 'button' );
- button.style.display = 'none';
-
- stylizeElement( button );
-
- navigator.xr.isSessionSupported( 'immersive-vr' ).then( function ( supported ) {
-
- if ( supported ) {
-
- showEnterXR();
-
- } else {
-
- showXRNotFound();
-
- }
-
- } );
-
- return button;
-
- } else if ( 'getVRDisplays' in navigator ) {
-
- var button = document.createElement( 'button' );
- button.style.display = 'none';
-
- stylizeElement( button );
-
- window.addEventListener( 'vrdisplayconnect', function ( event ) {
-
- showEnterVR( event.display );
-
- }, false );
-
- window.addEventListener( 'vrdisplaydisconnect', function ( /*event*/ ) {
-
- showVRNotFound();
-
- }, false );
-
- window.addEventListener( 'vrdisplaypresentchange', function ( event ) {
-
- button.textContent = event.display.isPresenting ? 'EXIT VR' : 'ENTER VR';
-
- }, false );
-
- window.addEventListener( 'vrdisplayactivate', function ( event ) {
-
- event.display.requestPresent( [ { source: renderer.domElement } ] );
-
- }, false );
-
- navigator.getVRDisplays()
- .then( function ( displays ) {
-
- if ( displays.length > 0 ) {
-
- showEnterVR( displays[ 0 ] );
-
- } else {
-
- showVRNotFound();
-
- }
-
- } ).catch( showVRNotFound );
-
- return button;
-
- } else {
-
- var message = document.createElement( 'a' );
- message.href = 'https://webvr.info';
- message.innerHTML = 'WEBVR NOT SUPPORTED';
-
- message.style.left = 'calc(50% - 90px)';
- message.style.width = '180px';
- message.style.textDecoration = 'none';
-
- stylizeElement( message );
-
- return message;
-
- }
-
- }
-
-};
\ No newline at end of file
diff --git a/spaces/JoPmt/Short_Bedtime_Stories/README.md b/spaces/JoPmt/Short_Bedtime_Stories/README.md
deleted file mode 100644
index b3c971f703624f6b4a97bee9321d5b9a8d4c0e80..0000000000000000000000000000000000000000
--- a/spaces/JoPmt/Short_Bedtime_Stories/README.md
+++ /dev/null
@@ -1,10 +0,0 @@
----
-title: Short Bedtime Stories
-emoji: 🐠
-colorFrom: gray
-colorTo: red
-sdk: static
-pinned: false
----
-
-Check out the configuration reference at https://huggingface.co/docs/hub/spaces-config-reference
diff --git a/spaces/KaygNas/cut-it/Dockerfile b/spaces/KaygNas/cut-it/Dockerfile
deleted file mode 100644
index 91448797d01519360d73ee81db6be6c4587aff1e..0000000000000000000000000000000000000000
--- a/spaces/KaygNas/cut-it/Dockerfile
+++ /dev/null
@@ -1,43 +0,0 @@
-# Use the official Node 18 image
-FROM node:18
-
-# Set the working directory to /html
-WORKDIR /code
-
-# Copy and install dependencies
-COPY package*.json ./
-RUN npm install
-
-# Build the web app
-COPY . .
-RUN npm run build
-
-
-# Use the official Python 3.9 image
-FROM python:3.9
-
-# Set the working directory to /code
-WORKDIR /code
-
-# Copy the current directory contents into the container at /code
-COPY ./requirements.txt /code/requirements.txt
-
-# Install requirements.txt
-RUN pip install --no-cache-dir --upgrade -r /code/requirements.txt
-
-# Set up a new user named "user" with user ID 1000
-RUN useradd -m -u 1000 user
-# Switch to the "user" user
-USER user
-# Set home to the user's home directory
-ENV HOME=/home/user \
- PATH=/home/user/.local/bin:$PATH
-
-# Set the working directory to the user's home directory
-WORKDIR $HOME/app
-
-# Copy the current directory contents into the container at $HOME/app setting the owner to the user
-COPY --chown=user main.py $HOME/app
-COPY --chown=user --from=0 /code/dist $HOME/app/dist
-
-CMD ["uvicorn", "main:app", "--host", "0.0.0.0", "--port", "7860"]
\ No newline at end of file
diff --git a/spaces/Kevin676/AutoGPT/autogpt/commands/web_playwright.py b/spaces/Kevin676/AutoGPT/autogpt/commands/web_playwright.py
deleted file mode 100644
index 4e388ded203cefb5e24f9116f7fe5b8a94893413..0000000000000000000000000000000000000000
--- a/spaces/Kevin676/AutoGPT/autogpt/commands/web_playwright.py
+++ /dev/null
@@ -1,80 +0,0 @@
-"""Web scraping commands using Playwright"""
-from __future__ import annotations
-
-try:
- from playwright.sync_api import sync_playwright
-except ImportError:
- print(
- "Playwright not installed. Please install it with 'pip install playwright' to use."
- )
-from bs4 import BeautifulSoup
-
-from autogpt.processing.html import extract_hyperlinks, format_hyperlinks
-
-
-def scrape_text(url: str) -> str:
- """Scrape text from a webpage
-
- Args:
- url (str): The URL to scrape text from
-
- Returns:
- str: The scraped text
- """
- with sync_playwright() as p:
- browser = p.chromium.launch()
- page = browser.new_page()
-
- try:
- page.goto(url)
- html_content = page.content()
- soup = BeautifulSoup(html_content, "html.parser")
-
- for script in soup(["script", "style"]):
- script.extract()
-
- text = soup.get_text()
- lines = (line.strip() for line in text.splitlines())
- chunks = (phrase.strip() for line in lines for phrase in line.split(" "))
- text = "\n".join(chunk for chunk in chunks if chunk)
-
- except Exception as e:
- text = f"Error: {str(e)}"
-
- finally:
- browser.close()
-
- return text
-
-
-def scrape_links(url: str) -> str | list[str]:
- """Scrape links from a webpage
-
- Args:
- url (str): The URL to scrape links from
-
- Returns:
- Union[str, List[str]]: The scraped links
- """
- with sync_playwright() as p:
- browser = p.chromium.launch()
- page = browser.new_page()
-
- try:
- page.goto(url)
- html_content = page.content()
- soup = BeautifulSoup(html_content, "html.parser")
-
- for script in soup(["script", "style"]):
- script.extract()
-
- hyperlinks = extract_hyperlinks(soup, url)
- formatted_links = format_hyperlinks(hyperlinks)
-
- except Exception as e:
- formatted_links = f"Error: {str(e)}"
-
- finally:
- browser.close()
-
- return formatted_links
diff --git a/spaces/Kevin676/ChatGPT-with-Voice-Cloning-in-Chinese/encoder/data_objects/speaker.py b/spaces/Kevin676/ChatGPT-with-Voice-Cloning-in-Chinese/encoder/data_objects/speaker.py
deleted file mode 100644
index 494e882fe34fc38dcc793ab8c74a6cc2376bb7b5..0000000000000000000000000000000000000000
--- a/spaces/Kevin676/ChatGPT-with-Voice-Cloning-in-Chinese/encoder/data_objects/speaker.py
+++ /dev/null
@@ -1,40 +0,0 @@
-from encoder.data_objects.random_cycler import RandomCycler
-from encoder.data_objects.utterance import Utterance
-from pathlib import Path
-
-# Contains the set of utterances of a single speaker
-class Speaker:
- def __init__(self, root: Path):
- self.root = root
- self.name = root.name
- self.utterances = None
- self.utterance_cycler = None
-
- def _load_utterances(self):
- with self.root.joinpath("_sources.txt").open("r") as sources_file:
- sources = [l.split(",") for l in sources_file]
- sources = {frames_fname: wave_fpath for frames_fname, wave_fpath in sources}
- self.utterances = [Utterance(self.root.joinpath(f), w) for f, w in sources.items()]
- self.utterance_cycler = RandomCycler(self.utterances)
-
- def random_partial(self, count, n_frames):
- """
- Samples a batch of unique partial utterances from the disk in a way that all
- utterances come up at least once every two cycles and in a random order every time.
-
- :param count: The number of partial utterances to sample from the set of utterances from
- that speaker. Utterances are guaranteed not to be repeated if is not larger than
- the number of utterances available.
- :param n_frames: The number of frames in the partial utterance.
- :return: A list of tuples (utterance, frames, range) where utterance is an Utterance,
- frames are the frames of the partial utterances and range is the range of the partial
- utterance with regard to the complete utterance.
- """
- if self.utterances is None:
- self._load_utterances()
-
- utterances = self.utterance_cycler.sample(count)
-
- a = [(u,) + u.random_partial(n_frames) for u in utterances]
-
- return a
diff --git a/spaces/KevlarVK/content_summarizer/Utils.py b/spaces/KevlarVK/content_summarizer/Utils.py
deleted file mode 100644
index 35d221759fa9d892bc3c7362e20411101f89e521..0000000000000000000000000000000000000000
--- a/spaces/KevlarVK/content_summarizer/Utils.py
+++ /dev/null
@@ -1,103 +0,0 @@
-import requests
-from bs4 import BeautifulSoup
-from nltk.tokenize import sent_tokenize
-import nltk
-import re
-import streamlit as st
-from youtube_transcript_api import YouTubeTranscriptApi
-import spacy
-
-@st.cache
-def fetch_article_text(url: str):
-
- r = requests.get(url)
- soup = BeautifulSoup(r.text, "html.parser")
- results = soup.find_all(["h1", "p"])
- text = [result.text for result in results]
- ARTICLE = " ".join(text)
- return re.sub(r'\[\d+\]', '', ARTICLE)
-
-def count_tokens(text: str):
- return len(text.split(" "))
-
-@st.cache
-def get_text_from_youtube_url(url: str):
-
- id = url.split("=")[1]
- try:
- transcript = YouTubeTranscriptApi.get_transcript(id)
- except:
- transcript = YouTubeTranscriptApi.find_transcript(["en"])
- script = ""
-
- for text in transcript:
- t = text["text"]
- if t != '[Music]':
- script += t.lower() + " "
-
- return add_punctuation(script)
-
-def add_punctuation(text: str):
-
- # try:
- nlp = spacy.load("en_core_web_sm")
- # except:
- # import spacy.cli
- # spacy.cli.download("en_core_web_sm")
- # nlp = spacy.load("en_core_web_sm")
-
- doc = nlp(text)
- punctuation = [".", ",", ";", ":", "?", "!"]
-
- sentences = []
- for sentence in doc.sents:
-
- last_token = sentence[-1]
- if last_token.text in punctuation:
- sentence = sentence[:-1]
-
- last_word = sentence[-1]
- if last_word.pos_ == "NOUN":
- sentence = sentence.text + "."
- elif last_word.pos_ == "VERB":
- sentence = sentence.text + "?"
- else:
- sentence = sentence.text + "."
-
- sentence = sentence[0].upper() + sentence[1:]
- sentences.append(sentence)
-
- text_with_punctuation = " ".join(sentences)
-
- return text_with_punctuation
-
-
-def get_input_chunks(text: str, max_length: int = 500):
-
- text = re.sub(r'\[\d+\]', '', text)
-
- try:
- sentences = sent_tokenize(text)
- except:
- nltk.download('punkt')
- sentences = sent_tokenize(text)
-
- sentences = [sentence for sentence in sentences if len(sentence.strip()) > 0 and count_tokens(sentence) > 4]
-
- input_chunks = []
- temp_sentences = ""
- tokens = 0
-
- for sentence in sentences:
- if tokens + count_tokens(sentence) < max_length:
- temp_sentences += sentence
- tokens += count_tokens(sentence)
- else:
- input_chunks.append(temp_sentences)
- tokens = count_tokens(sentence)
- temp_sentences = sentence
-
- if len(temp_sentences) > 0:
- input_chunks.append(temp_sentences)
-
- return input_chunks
diff --git a/spaces/Kimata/Sanskrit-TTS/transforms.py b/spaces/Kimata/Sanskrit-TTS/transforms.py
deleted file mode 100644
index 4793d67ca5a5630e0ffe0f9fb29445c949e64dae..0000000000000000000000000000000000000000
--- a/spaces/Kimata/Sanskrit-TTS/transforms.py
+++ /dev/null
@@ -1,193 +0,0 @@
-import torch
-from torch.nn import functional as F
-
-import numpy as np
-
-
-DEFAULT_MIN_BIN_WIDTH = 1e-3
-DEFAULT_MIN_BIN_HEIGHT = 1e-3
-DEFAULT_MIN_DERIVATIVE = 1e-3
-
-
-def piecewise_rational_quadratic_transform(inputs,
- unnormalized_widths,
- unnormalized_heights,
- unnormalized_derivatives,
- inverse=False,
- tails=None,
- tail_bound=1.,
- min_bin_width=DEFAULT_MIN_BIN_WIDTH,
- min_bin_height=DEFAULT_MIN_BIN_HEIGHT,
- min_derivative=DEFAULT_MIN_DERIVATIVE):
-
- if tails is None:
- spline_fn = rational_quadratic_spline
- spline_kwargs = {}
- else:
- spline_fn = unconstrained_rational_quadratic_spline
- spline_kwargs = {
- 'tails': tails,
- 'tail_bound': tail_bound
- }
-
- outputs, logabsdet = spline_fn(
- inputs=inputs,
- unnormalized_widths=unnormalized_widths,
- unnormalized_heights=unnormalized_heights,
- unnormalized_derivatives=unnormalized_derivatives,
- inverse=inverse,
- min_bin_width=min_bin_width,
- min_bin_height=min_bin_height,
- min_derivative=min_derivative,
- **spline_kwargs
- )
- return outputs, logabsdet
-
-
-def searchsorted(bin_locations, inputs, eps=1e-6):
- bin_locations[..., -1] += eps
- return torch.sum(
- inputs[..., None] >= bin_locations,
- dim=-1
- ) - 1
-
-
-def unconstrained_rational_quadratic_spline(inputs,
- unnormalized_widths,
- unnormalized_heights,
- unnormalized_derivatives,
- inverse=False,
- tails='linear',
- tail_bound=1.,
- min_bin_width=DEFAULT_MIN_BIN_WIDTH,
- min_bin_height=DEFAULT_MIN_BIN_HEIGHT,
- min_derivative=DEFAULT_MIN_DERIVATIVE):
- inside_interval_mask = (inputs >= -tail_bound) & (inputs <= tail_bound)
- outside_interval_mask = ~inside_interval_mask
-
- outputs = torch.zeros_like(inputs)
- logabsdet = torch.zeros_like(inputs)
-
- if tails == 'linear':
- unnormalized_derivatives = F.pad(unnormalized_derivatives, pad=(1, 1))
- constant = np.log(np.exp(1 - min_derivative) - 1)
- unnormalized_derivatives[..., 0] = constant
- unnormalized_derivatives[..., -1] = constant
-
- outputs[outside_interval_mask] = inputs[outside_interval_mask]
- logabsdet[outside_interval_mask] = 0
- else:
- raise RuntimeError('{} tails are not implemented.'.format(tails))
-
- outputs[inside_interval_mask], logabsdet[inside_interval_mask] = rational_quadratic_spline(
- inputs=inputs[inside_interval_mask],
- unnormalized_widths=unnormalized_widths[inside_interval_mask, :],
- unnormalized_heights=unnormalized_heights[inside_interval_mask, :],
- unnormalized_derivatives=unnormalized_derivatives[inside_interval_mask, :],
- inverse=inverse,
- left=-tail_bound, right=tail_bound, bottom=-tail_bound, top=tail_bound,
- min_bin_width=min_bin_width,
- min_bin_height=min_bin_height,
- min_derivative=min_derivative
- )
-
- return outputs, logabsdet
-
-def rational_quadratic_spline(inputs,
- unnormalized_widths,
- unnormalized_heights,
- unnormalized_derivatives,
- inverse=False,
- left=0., right=1., bottom=0., top=1.,
- min_bin_width=DEFAULT_MIN_BIN_WIDTH,
- min_bin_height=DEFAULT_MIN_BIN_HEIGHT,
- min_derivative=DEFAULT_MIN_DERIVATIVE):
- if torch.min(inputs) < left or torch.max(inputs) > right:
- raise ValueError('Input to a transform is not within its domain')
-
- num_bins = unnormalized_widths.shape[-1]
-
- if min_bin_width * num_bins > 1.0:
- raise ValueError('Minimal bin width too large for the number of bins')
- if min_bin_height * num_bins > 1.0:
- raise ValueError('Minimal bin height too large for the number of bins')
-
- widths = F.softmax(unnormalized_widths, dim=-1)
- widths = min_bin_width + (1 - min_bin_width * num_bins) * widths
- cumwidths = torch.cumsum(widths, dim=-1)
- cumwidths = F.pad(cumwidths, pad=(1, 0), mode='constant', value=0.0)
- cumwidths = (right - left) * cumwidths + left
- cumwidths[..., 0] = left
- cumwidths[..., -1] = right
- widths = cumwidths[..., 1:] - cumwidths[..., :-1]
-
- derivatives = min_derivative + F.softplus(unnormalized_derivatives)
-
- heights = F.softmax(unnormalized_heights, dim=-1)
- heights = min_bin_height + (1 - min_bin_height * num_bins) * heights
- cumheights = torch.cumsum(heights, dim=-1)
- cumheights = F.pad(cumheights, pad=(1, 0), mode='constant', value=0.0)
- cumheights = (top - bottom) * cumheights + bottom
- cumheights[..., 0] = bottom
- cumheights[..., -1] = top
- heights = cumheights[..., 1:] - cumheights[..., :-1]
-
- if inverse:
- bin_idx = searchsorted(cumheights, inputs)[..., None]
- else:
- bin_idx = searchsorted(cumwidths, inputs)[..., None]
-
- input_cumwidths = cumwidths.gather(-1, bin_idx)[..., 0]
- input_bin_widths = widths.gather(-1, bin_idx)[..., 0]
-
- input_cumheights = cumheights.gather(-1, bin_idx)[..., 0]
- delta = heights / widths
- input_delta = delta.gather(-1, bin_idx)[..., 0]
-
- input_derivatives = derivatives.gather(-1, bin_idx)[..., 0]
- input_derivatives_plus_one = derivatives[..., 1:].gather(-1, bin_idx)[..., 0]
-
- input_heights = heights.gather(-1, bin_idx)[..., 0]
-
- if inverse:
- a = (((inputs - input_cumheights) * (input_derivatives
- + input_derivatives_plus_one
- - 2 * input_delta)
- + input_heights * (input_delta - input_derivatives)))
- b = (input_heights * input_derivatives
- - (inputs - input_cumheights) * (input_derivatives
- + input_derivatives_plus_one
- - 2 * input_delta))
- c = - input_delta * (inputs - input_cumheights)
-
- discriminant = b.pow(2) - 4 * a * c
- assert (discriminant >= 0).all()
-
- root = (2 * c) / (-b - torch.sqrt(discriminant))
- outputs = root * input_bin_widths + input_cumwidths
-
- theta_one_minus_theta = root * (1 - root)
- denominator = input_delta + ((input_derivatives + input_derivatives_plus_one - 2 * input_delta)
- * theta_one_minus_theta)
- derivative_numerator = input_delta.pow(2) * (input_derivatives_plus_one * root.pow(2)
- + 2 * input_delta * theta_one_minus_theta
- + input_derivatives * (1 - root).pow(2))
- logabsdet = torch.log(derivative_numerator) - 2 * torch.log(denominator)
-
- return outputs, -logabsdet
- else:
- theta = (inputs - input_cumwidths) / input_bin_widths
- theta_one_minus_theta = theta * (1 - theta)
-
- numerator = input_heights * (input_delta * theta.pow(2)
- + input_derivatives * theta_one_minus_theta)
- denominator = input_delta + ((input_derivatives + input_derivatives_plus_one - 2 * input_delta)
- * theta_one_minus_theta)
- outputs = input_cumheights + numerator / denominator
-
- derivative_numerator = input_delta.pow(2) * (input_derivatives_plus_one * theta.pow(2)
- + 2 * input_delta * theta_one_minus_theta
- + input_derivatives * (1 - theta).pow(2))
- logabsdet = torch.log(derivative_numerator) - 2 * torch.log(denominator)
-
- return outputs, logabsdet
diff --git a/spaces/KyanChen/RSPrompter/mmdet/models/dense_heads/fcos_head.py b/spaces/KyanChen/RSPrompter/mmdet/models/dense_heads/fcos_head.py
deleted file mode 100644
index f3206877a1e3684e7ecf90799bb234c59838f294..0000000000000000000000000000000000000000
--- a/spaces/KyanChen/RSPrompter/mmdet/models/dense_heads/fcos_head.py
+++ /dev/null
@@ -1,455 +0,0 @@
-# Copyright (c) OpenMMLab. All rights reserved.
-from typing import Dict, List, Tuple
-
-import torch
-import torch.nn as nn
-from mmcv.cnn import Scale
-from mmengine.structures import InstanceData
-from torch import Tensor
-
-from mmdet.registry import MODELS
-from mmdet.utils import (ConfigType, InstanceList, MultiConfig,
- OptInstanceList, RangeType, reduce_mean)
-from ..utils import multi_apply
-from .anchor_free_head import AnchorFreeHead
-
-INF = 1e8
-
-
-@MODELS.register_module()
-class FCOSHead(AnchorFreeHead):
- """Anchor-free head used in `FCOS `_.
-
- The FCOS head does not use anchor boxes. Instead bounding boxes are
- predicted at each pixel and a centerness measure is used to suppress
- low-quality predictions.
- Here norm_on_bbox, centerness_on_reg, dcn_on_last_conv are training
- tricks used in official repo, which will bring remarkable mAP gains
- of up to 4.9. Please see https://github.com/tianzhi0549/FCOS for
- more detail.
-
- Args:
- num_classes (int): Number of categories excluding the background
- category.
- in_channels (int): Number of channels in the input feature map.
- strides (Sequence[int] or Sequence[Tuple[int, int]]): Strides of points
- in multiple feature levels. Defaults to (4, 8, 16, 32, 64).
- regress_ranges (Sequence[Tuple[int, int]]): Regress range of multiple
- level points.
- center_sampling (bool): If true, use center sampling.
- Defaults to False.
- center_sample_radius (float): Radius of center sampling.
- Defaults to 1.5.
- norm_on_bbox (bool): If true, normalize the regression targets with
- FPN strides. Defaults to False.
- centerness_on_reg (bool): If true, position centerness on the
- regress branch. Please refer to https://github.com/tianzhi0549/FCOS/issues/89#issuecomment-516877042.
- Defaults to False.
- conv_bias (bool or str): If specified as `auto`, it will be decided by
- the norm_cfg. Bias of conv will be set as True if `norm_cfg` is
- None, otherwise False. Defaults to "auto".
- loss_cls (:obj:`ConfigDict` or dict): Config of classification loss.
- loss_bbox (:obj:`ConfigDict` or dict): Config of localization loss.
- loss_centerness (:obj:`ConfigDict`, or dict): Config of centerness
- loss.
- norm_cfg (:obj:`ConfigDict` or dict): dictionary to construct and
- config norm layer. Defaults to
- ``norm_cfg=dict(type='GN', num_groups=32, requires_grad=True)``.
- init_cfg (:obj:`ConfigDict` or dict or list[:obj:`ConfigDict` or \
- dict]): Initialization config dict.
-
- Example:
- >>> self = FCOSHead(11, 7)
- >>> feats = [torch.rand(1, 7, s, s) for s in [4, 8, 16, 32, 64]]
- >>> cls_score, bbox_pred, centerness = self.forward(feats)
- >>> assert len(cls_score) == len(self.scales)
- """ # noqa: E501
-
- def __init__(self,
- num_classes: int,
- in_channels: int,
- regress_ranges: RangeType = ((-1, 64), (64, 128), (128, 256),
- (256, 512), (512, INF)),
- center_sampling: bool = False,
- center_sample_radius: float = 1.5,
- norm_on_bbox: bool = False,
- centerness_on_reg: bool = False,
- loss_cls: ConfigType = dict(
- type='FocalLoss',
- use_sigmoid=True,
- gamma=2.0,
- alpha=0.25,
- loss_weight=1.0),
- loss_bbox: ConfigType = dict(type='IoULoss', loss_weight=1.0),
- loss_centerness: ConfigType = dict(
- type='CrossEntropyLoss',
- use_sigmoid=True,
- loss_weight=1.0),
- norm_cfg: ConfigType = dict(
- type='GN', num_groups=32, requires_grad=True),
- init_cfg: MultiConfig = dict(
- type='Normal',
- layer='Conv2d',
- std=0.01,
- override=dict(
- type='Normal',
- name='conv_cls',
- std=0.01,
- bias_prob=0.01)),
- **kwargs) -> None:
- self.regress_ranges = regress_ranges
- self.center_sampling = center_sampling
- self.center_sample_radius = center_sample_radius
- self.norm_on_bbox = norm_on_bbox
- self.centerness_on_reg = centerness_on_reg
- super().__init__(
- num_classes=num_classes,
- in_channels=in_channels,
- loss_cls=loss_cls,
- loss_bbox=loss_bbox,
- norm_cfg=norm_cfg,
- init_cfg=init_cfg,
- **kwargs)
- self.loss_centerness = MODELS.build(loss_centerness)
-
- def _init_layers(self) -> None:
- """Initialize layers of the head."""
- super()._init_layers()
- self.conv_centerness = nn.Conv2d(self.feat_channels, 1, 3, padding=1)
- self.scales = nn.ModuleList([Scale(1.0) for _ in self.strides])
-
- def forward(
- self, x: Tuple[Tensor]
- ) -> Tuple[List[Tensor], List[Tensor], List[Tensor]]:
- """Forward features from the upstream network.
-
- Args:
- feats (tuple[Tensor]): Features from the upstream network, each is
- a 4D-tensor.
-
- Returns:
- tuple: A tuple of each level outputs.
-
- - cls_scores (list[Tensor]): Box scores for each scale level, \
- each is a 4D-tensor, the channel number is \
- num_points * num_classes.
- - bbox_preds (list[Tensor]): Box energies / deltas for each \
- scale level, each is a 4D-tensor, the channel number is \
- num_points * 4.
- - centernesses (list[Tensor]): centerness for each scale level, \
- each is a 4D-tensor, the channel number is num_points * 1.
- """
- return multi_apply(self.forward_single, x, self.scales, self.strides)
-
- def forward_single(self, x: Tensor, scale: Scale,
- stride: int) -> Tuple[Tensor, Tensor, Tensor]:
- """Forward features of a single scale level.
-
- Args:
- x (Tensor): FPN feature maps of the specified stride.
- scale (:obj:`mmcv.cnn.Scale`): Learnable scale module to resize
- the bbox prediction.
- stride (int): The corresponding stride for feature maps, only
- used to normalize the bbox prediction when self.norm_on_bbox
- is True.
-
- Returns:
- tuple: scores for each class, bbox predictions and centerness
- predictions of input feature maps.
- """
- cls_score, bbox_pred, cls_feat, reg_feat = super().forward_single(x)
- if self.centerness_on_reg:
- centerness = self.conv_centerness(reg_feat)
- else:
- centerness = self.conv_centerness(cls_feat)
- # scale the bbox_pred of different level
- # float to avoid overflow when enabling FP16
- bbox_pred = scale(bbox_pred).float()
- if self.norm_on_bbox:
- # bbox_pred needed for gradient computation has been modified
- # by F.relu(bbox_pred) when run with PyTorch 1.10. So replace
- # F.relu(bbox_pred) with bbox_pred.clamp(min=0)
- bbox_pred = bbox_pred.clamp(min=0)
- if not self.training:
- bbox_pred *= stride
- else:
- bbox_pred = bbox_pred.exp()
- return cls_score, bbox_pred, centerness
-
- def loss_by_feat(
- self,
- cls_scores: List[Tensor],
- bbox_preds: List[Tensor],
- centernesses: List[Tensor],
- batch_gt_instances: InstanceList,
- batch_img_metas: List[dict],
- batch_gt_instances_ignore: OptInstanceList = None
- ) -> Dict[str, Tensor]:
- """Calculate the loss based on the features extracted by the detection
- head.
-
- Args:
- cls_scores (list[Tensor]): Box scores for each scale level,
- each is a 4D-tensor, the channel number is
- num_points * num_classes.
- bbox_preds (list[Tensor]): Box energies / deltas for each scale
- level, each is a 4D-tensor, the channel number is
- num_points * 4.
- centernesses (list[Tensor]): centerness for each scale level, each
- is a 4D-tensor, the channel number is num_points * 1.
- batch_gt_instances (list[:obj:`InstanceData`]): Batch of
- gt_instance. It usually includes ``bboxes`` and ``labels``
- attributes.
- batch_img_metas (list[dict]): Meta information of each image, e.g.,
- image size, scaling factor, etc.
- batch_gt_instances_ignore (list[:obj:`InstanceData`], Optional):
- Batch of gt_instances_ignore. It includes ``bboxes`` attribute
- data that is ignored during training and testing.
- Defaults to None.
-
- Returns:
- dict[str, Tensor]: A dictionary of loss components.
- """
- assert len(cls_scores) == len(bbox_preds) == len(centernesses)
- featmap_sizes = [featmap.size()[-2:] for featmap in cls_scores]
- all_level_points = self.prior_generator.grid_priors(
- featmap_sizes,
- dtype=bbox_preds[0].dtype,
- device=bbox_preds[0].device)
- labels, bbox_targets = self.get_targets(all_level_points,
- batch_gt_instances)
-
- num_imgs = cls_scores[0].size(0)
- # flatten cls_scores, bbox_preds and centerness
- flatten_cls_scores = [
- cls_score.permute(0, 2, 3, 1).reshape(-1, self.cls_out_channels)
- for cls_score in cls_scores
- ]
- flatten_bbox_preds = [
- bbox_pred.permute(0, 2, 3, 1).reshape(-1, 4)
- for bbox_pred in bbox_preds
- ]
- flatten_centerness = [
- centerness.permute(0, 2, 3, 1).reshape(-1)
- for centerness in centernesses
- ]
- flatten_cls_scores = torch.cat(flatten_cls_scores)
- flatten_bbox_preds = torch.cat(flatten_bbox_preds)
- flatten_centerness = torch.cat(flatten_centerness)
- flatten_labels = torch.cat(labels)
- flatten_bbox_targets = torch.cat(bbox_targets)
- # repeat points to align with bbox_preds
- flatten_points = torch.cat(
- [points.repeat(num_imgs, 1) for points in all_level_points])
-
- # FG cat_id: [0, num_classes -1], BG cat_id: num_classes
- bg_class_ind = self.num_classes
- pos_inds = ((flatten_labels >= 0)
- & (flatten_labels < bg_class_ind)).nonzero().reshape(-1)
- num_pos = torch.tensor(
- len(pos_inds), dtype=torch.float, device=bbox_preds[0].device)
- num_pos = max(reduce_mean(num_pos), 1.0)
- loss_cls = self.loss_cls(
- flatten_cls_scores, flatten_labels, avg_factor=num_pos)
-
- pos_bbox_preds = flatten_bbox_preds[pos_inds]
- pos_centerness = flatten_centerness[pos_inds]
- pos_bbox_targets = flatten_bbox_targets[pos_inds]
- pos_centerness_targets = self.centerness_target(pos_bbox_targets)
- # centerness weighted iou loss
- centerness_denorm = max(
- reduce_mean(pos_centerness_targets.sum().detach()), 1e-6)
-
- if len(pos_inds) > 0:
- pos_points = flatten_points[pos_inds]
- pos_decoded_bbox_preds = self.bbox_coder.decode(
- pos_points, pos_bbox_preds)
- pos_decoded_target_preds = self.bbox_coder.decode(
- pos_points, pos_bbox_targets)
- loss_bbox = self.loss_bbox(
- pos_decoded_bbox_preds,
- pos_decoded_target_preds,
- weight=pos_centerness_targets,
- avg_factor=centerness_denorm)
- loss_centerness = self.loss_centerness(
- pos_centerness, pos_centerness_targets, avg_factor=num_pos)
- else:
- loss_bbox = pos_bbox_preds.sum()
- loss_centerness = pos_centerness.sum()
-
- return dict(
- loss_cls=loss_cls,
- loss_bbox=loss_bbox,
- loss_centerness=loss_centerness)
-
- def get_targets(
- self, points: List[Tensor], batch_gt_instances: InstanceList
- ) -> Tuple[List[Tensor], List[Tensor]]:
- """Compute regression, classification and centerness targets for points
- in multiple images.
-
- Args:
- points (list[Tensor]): Points of each fpn level, each has shape
- (num_points, 2).
- batch_gt_instances (list[:obj:`InstanceData`]): Batch of
- gt_instance. It usually includes ``bboxes`` and ``labels``
- attributes.
-
- Returns:
- tuple: Targets of each level.
-
- - concat_lvl_labels (list[Tensor]): Labels of each level.
- - concat_lvl_bbox_targets (list[Tensor]): BBox targets of each \
- level.
- """
- assert len(points) == len(self.regress_ranges)
- num_levels = len(points)
- # expand regress ranges to align with points
- expanded_regress_ranges = [
- points[i].new_tensor(self.regress_ranges[i])[None].expand_as(
- points[i]) for i in range(num_levels)
- ]
- # concat all levels points and regress ranges
- concat_regress_ranges = torch.cat(expanded_regress_ranges, dim=0)
- concat_points = torch.cat(points, dim=0)
-
- # the number of points per img, per lvl
- num_points = [center.size(0) for center in points]
-
- # get labels and bbox_targets of each image
- labels_list, bbox_targets_list = multi_apply(
- self._get_targets_single,
- batch_gt_instances,
- points=concat_points,
- regress_ranges=concat_regress_ranges,
- num_points_per_lvl=num_points)
-
- # split to per img, per level
- labels_list = [labels.split(num_points, 0) for labels in labels_list]
- bbox_targets_list = [
- bbox_targets.split(num_points, 0)
- for bbox_targets in bbox_targets_list
- ]
-
- # concat per level image
- concat_lvl_labels = []
- concat_lvl_bbox_targets = []
- for i in range(num_levels):
- concat_lvl_labels.append(
- torch.cat([labels[i] for labels in labels_list]))
- bbox_targets = torch.cat(
- [bbox_targets[i] for bbox_targets in bbox_targets_list])
- if self.norm_on_bbox:
- bbox_targets = bbox_targets / self.strides[i]
- concat_lvl_bbox_targets.append(bbox_targets)
- return concat_lvl_labels, concat_lvl_bbox_targets
-
- def _get_targets_single(
- self, gt_instances: InstanceData, points: Tensor,
- regress_ranges: Tensor,
- num_points_per_lvl: List[int]) -> Tuple[Tensor, Tensor]:
- """Compute regression and classification targets for a single image."""
- num_points = points.size(0)
- num_gts = len(gt_instances)
- gt_bboxes = gt_instances.bboxes
- gt_labels = gt_instances.labels
-
- if num_gts == 0:
- return gt_labels.new_full((num_points,), self.num_classes), \
- gt_bboxes.new_zeros((num_points, 4))
-
- areas = (gt_bboxes[:, 2] - gt_bboxes[:, 0]) * (
- gt_bboxes[:, 3] - gt_bboxes[:, 1])
- # TODO: figure out why these two are different
- # areas = areas[None].expand(num_points, num_gts)
- areas = areas[None].repeat(num_points, 1)
- regress_ranges = regress_ranges[:, None, :].expand(
- num_points, num_gts, 2)
- gt_bboxes = gt_bboxes[None].expand(num_points, num_gts, 4)
- xs, ys = points[:, 0], points[:, 1]
- xs = xs[:, None].expand(num_points, num_gts)
- ys = ys[:, None].expand(num_points, num_gts)
-
- left = xs - gt_bboxes[..., 0]
- right = gt_bboxes[..., 2] - xs
- top = ys - gt_bboxes[..., 1]
- bottom = gt_bboxes[..., 3] - ys
- bbox_targets = torch.stack((left, top, right, bottom), -1)
-
- if self.center_sampling:
- # condition1: inside a `center bbox`
- radius = self.center_sample_radius
- center_xs = (gt_bboxes[..., 0] + gt_bboxes[..., 2]) / 2
- center_ys = (gt_bboxes[..., 1] + gt_bboxes[..., 3]) / 2
- center_gts = torch.zeros_like(gt_bboxes)
- stride = center_xs.new_zeros(center_xs.shape)
-
- # project the points on current lvl back to the `original` sizes
- lvl_begin = 0
- for lvl_idx, num_points_lvl in enumerate(num_points_per_lvl):
- lvl_end = lvl_begin + num_points_lvl
- stride[lvl_begin:lvl_end] = self.strides[lvl_idx] * radius
- lvl_begin = lvl_end
-
- x_mins = center_xs - stride
- y_mins = center_ys - stride
- x_maxs = center_xs + stride
- y_maxs = center_ys + stride
- center_gts[..., 0] = torch.where(x_mins > gt_bboxes[..., 0],
- x_mins, gt_bboxes[..., 0])
- center_gts[..., 1] = torch.where(y_mins > gt_bboxes[..., 1],
- y_mins, gt_bboxes[..., 1])
- center_gts[..., 2] = torch.where(x_maxs > gt_bboxes[..., 2],
- gt_bboxes[..., 2], x_maxs)
- center_gts[..., 3] = torch.where(y_maxs > gt_bboxes[..., 3],
- gt_bboxes[..., 3], y_maxs)
-
- cb_dist_left = xs - center_gts[..., 0]
- cb_dist_right = center_gts[..., 2] - xs
- cb_dist_top = ys - center_gts[..., 1]
- cb_dist_bottom = center_gts[..., 3] - ys
- center_bbox = torch.stack(
- (cb_dist_left, cb_dist_top, cb_dist_right, cb_dist_bottom), -1)
- inside_gt_bbox_mask = center_bbox.min(-1)[0] > 0
- else:
- # condition1: inside a gt bbox
- inside_gt_bbox_mask = bbox_targets.min(-1)[0] > 0
-
- # condition2: limit the regression range for each location
- max_regress_distance = bbox_targets.max(-1)[0]
- inside_regress_range = (
- (max_regress_distance >= regress_ranges[..., 0])
- & (max_regress_distance <= regress_ranges[..., 1]))
-
- # if there are still more than one objects for a location,
- # we choose the one with minimal area
- areas[inside_gt_bbox_mask == 0] = INF
- areas[inside_regress_range == 0] = INF
- min_area, min_area_inds = areas.min(dim=1)
-
- labels = gt_labels[min_area_inds]
- labels[min_area == INF] = self.num_classes # set as BG
- bbox_targets = bbox_targets[range(num_points), min_area_inds]
-
- return labels, bbox_targets
-
- def centerness_target(self, pos_bbox_targets: Tensor) -> Tensor:
- """Compute centerness targets.
-
- Args:
- pos_bbox_targets (Tensor): BBox targets of positive bboxes in shape
- (num_pos, 4)
-
- Returns:
- Tensor: Centerness target.
- """
- # only calculate pos centerness targets, otherwise there may be nan
- left_right = pos_bbox_targets[:, [0, 2]]
- top_bottom = pos_bbox_targets[:, [1, 3]]
- if len(left_right) == 0:
- centerness_targets = left_right[..., 0]
- else:
- centerness_targets = (
- left_right.min(dim=-1)[0] / left_right.max(dim=-1)[0]) * (
- top_bottom.min(dim=-1)[0] / top_bottom.max(dim=-1)[0])
- return torch.sqrt(centerness_targets)
diff --git a/spaces/KyanChen/RSPrompter/mmdet/models/dense_heads/gfl_head.py b/spaces/KyanChen/RSPrompter/mmdet/models/dense_heads/gfl_head.py
deleted file mode 100644
index 6d2947a894892575c7f86ba6725456e6571f7585..0000000000000000000000000000000000000000
--- a/spaces/KyanChen/RSPrompter/mmdet/models/dense_heads/gfl_head.py
+++ /dev/null
@@ -1,667 +0,0 @@
-# Copyright (c) OpenMMLab. All rights reserved.
-from typing import List, Optional, Sequence, Tuple
-
-import torch
-import torch.nn as nn
-import torch.nn.functional as F
-from mmcv.cnn import ConvModule, Scale
-from mmengine.config import ConfigDict
-from mmengine.structures import InstanceData
-from torch import Tensor
-
-from mmdet.registry import MODELS, TASK_UTILS
-from mmdet.structures.bbox import bbox_overlaps
-from mmdet.utils import (ConfigType, InstanceList, MultiConfig, OptConfigType,
- OptInstanceList, reduce_mean)
-from ..task_modules.prior_generators import anchor_inside_flags
-from ..task_modules.samplers import PseudoSampler
-from ..utils import (filter_scores_and_topk, images_to_levels, multi_apply,
- unmap)
-from .anchor_head import AnchorHead
-
-
-class Integral(nn.Module):
- """A fixed layer for calculating integral result from distribution.
-
- This layer calculates the target location by :math: ``sum{P(y_i) * y_i}``,
- P(y_i) denotes the softmax vector that represents the discrete distribution
- y_i denotes the discrete set, usually {0, 1, 2, ..., reg_max}
-
- Args:
- reg_max (int): The maximal value of the discrete set. Defaults to 16.
- You may want to reset it according to your new dataset or related
- settings.
- """
-
- def __init__(self, reg_max: int = 16) -> None:
- super().__init__()
- self.reg_max = reg_max
- self.register_buffer('project',
- torch.linspace(0, self.reg_max, self.reg_max + 1))
-
- def forward(self, x: Tensor) -> Tensor:
- """Forward feature from the regression head to get integral result of
- bounding box location.
-
- Args:
- x (Tensor): Features of the regression head, shape (N, 4*(n+1)),
- n is self.reg_max.
-
- Returns:
- x (Tensor): Integral result of box locations, i.e., distance
- offsets from the box center in four directions, shape (N, 4).
- """
- x = F.softmax(x.reshape(-1, self.reg_max + 1), dim=1)
- x = F.linear(x, self.project.type_as(x)).reshape(-1, 4)
- return x
-
-
-@MODELS.register_module()
-class GFLHead(AnchorHead):
- """Generalized Focal Loss: Learning Qualified and Distributed Bounding
- Boxes for Dense Object Detection.
-
- GFL head structure is similar with ATSS, however GFL uses
- 1) joint representation for classification and localization quality, and
- 2) flexible General distribution for bounding box locations,
- which are supervised by
- Quality Focal Loss (QFL) and Distribution Focal Loss (DFL), respectively
-
- https://arxiv.org/abs/2006.04388
-
- Args:
- num_classes (int): Number of categories excluding the background
- category.
- in_channels (int): Number of channels in the input feature map.
- stacked_convs (int): Number of conv layers in cls and reg tower.
- Defaults to 4.
- conv_cfg (:obj:`ConfigDict` or dict, optional): dictionary to construct
- and config conv layer. Defaults to None.
- norm_cfg (:obj:`ConfigDict` or dict): dictionary to construct and
- config norm layer. Default: dict(type='GN', num_groups=32,
- requires_grad=True).
- loss_qfl (:obj:`ConfigDict` or dict): Config of Quality Focal Loss
- (QFL).
- bbox_coder (:obj:`ConfigDict` or dict): Config of bbox coder. Defaults
- to 'DistancePointBBoxCoder'.
- reg_max (int): Max value of integral set :math: ``{0, ..., reg_max}``
- in QFL setting. Defaults to 16.
- init_cfg (:obj:`ConfigDict` or dict or list[dict] or
- list[:obj:`ConfigDict`]): Initialization config dict.
- Example:
- >>> self = GFLHead(11, 7)
- >>> feats = [torch.rand(1, 7, s, s) for s in [4, 8, 16, 32, 64]]
- >>> cls_quality_score, bbox_pred = self.forward(feats)
- >>> assert len(cls_quality_score) == len(self.scales)
- """
-
- def __init__(self,
- num_classes: int,
- in_channels: int,
- stacked_convs: int = 4,
- conv_cfg: OptConfigType = None,
- norm_cfg: ConfigType = dict(
- type='GN', num_groups=32, requires_grad=True),
- loss_dfl: ConfigType = dict(
- type='DistributionFocalLoss', loss_weight=0.25),
- bbox_coder: ConfigType = dict(type='DistancePointBBoxCoder'),
- reg_max: int = 16,
- init_cfg: MultiConfig = dict(
- type='Normal',
- layer='Conv2d',
- std=0.01,
- override=dict(
- type='Normal',
- name='gfl_cls',
- std=0.01,
- bias_prob=0.01)),
- **kwargs) -> None:
- self.stacked_convs = stacked_convs
- self.conv_cfg = conv_cfg
- self.norm_cfg = norm_cfg
- self.reg_max = reg_max
- super().__init__(
- num_classes=num_classes,
- in_channels=in_channels,
- bbox_coder=bbox_coder,
- init_cfg=init_cfg,
- **kwargs)
-
- if self.train_cfg:
- self.assigner = TASK_UTILS.build(self.train_cfg['assigner'])
- if self.train_cfg.get('sampler', None) is not None:
- self.sampler = TASK_UTILS.build(
- self.train_cfg['sampler'], default_args=dict(context=self))
- else:
- self.sampler = PseudoSampler(context=self)
-
- self.integral = Integral(self.reg_max)
- self.loss_dfl = MODELS.build(loss_dfl)
-
- def _init_layers(self) -> None:
- """Initialize layers of the head."""
- self.relu = nn.ReLU()
- self.cls_convs = nn.ModuleList()
- self.reg_convs = nn.ModuleList()
- for i in range(self.stacked_convs):
- chn = self.in_channels if i == 0 else self.feat_channels
- self.cls_convs.append(
- ConvModule(
- chn,
- self.feat_channels,
- 3,
- stride=1,
- padding=1,
- conv_cfg=self.conv_cfg,
- norm_cfg=self.norm_cfg))
- self.reg_convs.append(
- ConvModule(
- chn,
- self.feat_channels,
- 3,
- stride=1,
- padding=1,
- conv_cfg=self.conv_cfg,
- norm_cfg=self.norm_cfg))
- assert self.num_anchors == 1, 'anchor free version'
- self.gfl_cls = nn.Conv2d(
- self.feat_channels, self.cls_out_channels, 3, padding=1)
- self.gfl_reg = nn.Conv2d(
- self.feat_channels, 4 * (self.reg_max + 1), 3, padding=1)
- self.scales = nn.ModuleList(
- [Scale(1.0) for _ in self.prior_generator.strides])
-
- def forward(self, x: Tuple[Tensor]) -> Tuple[List[Tensor]]:
- """Forward features from the upstream network.
-
- Args:
- x (tuple[Tensor]): Features from the upstream network, each is
- a 4D-tensor.
-
- Returns:
- tuple: Usually a tuple of classification scores and bbox prediction
-
- - cls_scores (list[Tensor]): Classification and quality (IoU)
- joint scores for all scale levels, each is a 4D-tensor,
- the channel number is num_classes.
- - bbox_preds (list[Tensor]): Box distribution logits for all
- scale levels, each is a 4D-tensor, the channel number is
- 4*(n+1), n is max value of integral set.
- """
- return multi_apply(self.forward_single, x, self.scales)
-
- def forward_single(self, x: Tensor, scale: Scale) -> Sequence[Tensor]:
- """Forward feature of a single scale level.
-
- Args:
- x (Tensor): Features of a single scale level.
- scale (:obj: `mmcv.cnn.Scale`): Learnable scale module to resize
- the bbox prediction.
-
- Returns:
- tuple:
-
- - cls_score (Tensor): Cls and quality joint scores for a single
- scale level the channel number is num_classes.
- - bbox_pred (Tensor): Box distribution logits for a single scale
- level, the channel number is 4*(n+1), n is max value of
- integral set.
- """
- cls_feat = x
- reg_feat = x
- for cls_conv in self.cls_convs:
- cls_feat = cls_conv(cls_feat)
- for reg_conv in self.reg_convs:
- reg_feat = reg_conv(reg_feat)
- cls_score = self.gfl_cls(cls_feat)
- bbox_pred = scale(self.gfl_reg(reg_feat)).float()
- return cls_score, bbox_pred
-
- def anchor_center(self, anchors: Tensor) -> Tensor:
- """Get anchor centers from anchors.
-
- Args:
- anchors (Tensor): Anchor list with shape (N, 4), ``xyxy`` format.
-
- Returns:
- Tensor: Anchor centers with shape (N, 2), ``xy`` format.
- """
- anchors_cx = (anchors[..., 2] + anchors[..., 0]) / 2
- anchors_cy = (anchors[..., 3] + anchors[..., 1]) / 2
- return torch.stack([anchors_cx, anchors_cy], dim=-1)
-
- def loss_by_feat_single(self, anchors: Tensor, cls_score: Tensor,
- bbox_pred: Tensor, labels: Tensor,
- label_weights: Tensor, bbox_targets: Tensor,
- stride: Tuple[int], avg_factor: int) -> dict:
- """Calculate the loss of a single scale level based on the features
- extracted by the detection head.
-
- Args:
- anchors (Tensor): Box reference for each scale level with shape
- (N, num_total_anchors, 4).
- cls_score (Tensor): Cls and quality joint scores for each scale
- level has shape (N, num_classes, H, W).
- bbox_pred (Tensor): Box distribution logits for each scale
- level with shape (N, 4*(n+1), H, W), n is max value of integral
- set.
- labels (Tensor): Labels of each anchors with shape
- (N, num_total_anchors).
- label_weights (Tensor): Label weights of each anchor with shape
- (N, num_total_anchors)
- bbox_targets (Tensor): BBox regression targets of each anchor
- weight shape (N, num_total_anchors, 4).
- stride (Tuple[int]): Stride in this scale level.
- avg_factor (int): Average factor that is used to average
- the loss. When using sampling method, avg_factor is usually
- the sum of positive and negative priors. When using
- `PseudoSampler`, `avg_factor` is usually equal to the number
- of positive priors.
-
- Returns:
- dict[str, Tensor]: A dictionary of loss components.
- """
- assert stride[0] == stride[1], 'h stride is not equal to w stride!'
- anchors = anchors.reshape(-1, 4)
- cls_score = cls_score.permute(0, 2, 3,
- 1).reshape(-1, self.cls_out_channels)
- bbox_pred = bbox_pred.permute(0, 2, 3,
- 1).reshape(-1, 4 * (self.reg_max + 1))
- bbox_targets = bbox_targets.reshape(-1, 4)
- labels = labels.reshape(-1)
- label_weights = label_weights.reshape(-1)
-
- # FG cat_id: [0, num_classes -1], BG cat_id: num_classes
- bg_class_ind = self.num_classes
- pos_inds = ((labels >= 0)
- & (labels < bg_class_ind)).nonzero().squeeze(1)
- score = label_weights.new_zeros(labels.shape)
-
- if len(pos_inds) > 0:
- pos_bbox_targets = bbox_targets[pos_inds]
- pos_bbox_pred = bbox_pred[pos_inds]
- pos_anchors = anchors[pos_inds]
- pos_anchor_centers = self.anchor_center(pos_anchors) / stride[0]
-
- weight_targets = cls_score.detach().sigmoid()
- weight_targets = weight_targets.max(dim=1)[0][pos_inds]
- pos_bbox_pred_corners = self.integral(pos_bbox_pred)
- pos_decode_bbox_pred = self.bbox_coder.decode(
- pos_anchor_centers, pos_bbox_pred_corners)
- pos_decode_bbox_targets = pos_bbox_targets / stride[0]
- score[pos_inds] = bbox_overlaps(
- pos_decode_bbox_pred.detach(),
- pos_decode_bbox_targets,
- is_aligned=True)
- pred_corners = pos_bbox_pred.reshape(-1, self.reg_max + 1)
- target_corners = self.bbox_coder.encode(pos_anchor_centers,
- pos_decode_bbox_targets,
- self.reg_max).reshape(-1)
-
- # regression loss
- loss_bbox = self.loss_bbox(
- pos_decode_bbox_pred,
- pos_decode_bbox_targets,
- weight=weight_targets,
- avg_factor=1.0)
-
- # dfl loss
- loss_dfl = self.loss_dfl(
- pred_corners,
- target_corners,
- weight=weight_targets[:, None].expand(-1, 4).reshape(-1),
- avg_factor=4.0)
- else:
- loss_bbox = bbox_pred.sum() * 0
- loss_dfl = bbox_pred.sum() * 0
- weight_targets = bbox_pred.new_tensor(0)
-
- # cls (qfl) loss
- loss_cls = self.loss_cls(
- cls_score, (labels, score),
- weight=label_weights,
- avg_factor=avg_factor)
-
- return loss_cls, loss_bbox, loss_dfl, weight_targets.sum()
-
- def loss_by_feat(
- self,
- cls_scores: List[Tensor],
- bbox_preds: List[Tensor],
- batch_gt_instances: InstanceList,
- batch_img_metas: List[dict],
- batch_gt_instances_ignore: OptInstanceList = None) -> dict:
- """Calculate the loss based on the features extracted by the detection
- head.
-
- Args:
- cls_scores (list[Tensor]): Cls and quality scores for each scale
- level has shape (N, num_classes, H, W).
- bbox_preds (list[Tensor]): Box distribution logits for each scale
- level with shape (N, 4*(n+1), H, W), n is max value of integral
- set.
- batch_gt_instances (list[:obj:`InstanceData`]): Batch of
- gt_instance. It usually includes ``bboxes`` and ``labels``
- attributes.
- batch_img_metas (list[dict]): Meta information of each image, e.g.,
- image size, scaling factor, etc.
- batch_gt_instances_ignore (list[:obj:`InstanceData`], Optional):
- Batch of gt_instances_ignore. It includes ``bboxes`` attribute
- data that is ignored during training and testing.
- Defaults to None.
-
- Returns:
- dict[str, Tensor]: A dictionary of loss components.
- """
-
- featmap_sizes = [featmap.size()[-2:] for featmap in cls_scores]
- assert len(featmap_sizes) == self.prior_generator.num_levels
-
- device = cls_scores[0].device
- anchor_list, valid_flag_list = self.get_anchors(
- featmap_sizes, batch_img_metas, device=device)
-
- cls_reg_targets = self.get_targets(
- anchor_list,
- valid_flag_list,
- batch_gt_instances,
- batch_img_metas,
- batch_gt_instances_ignore=batch_gt_instances_ignore)
-
- (anchor_list, labels_list, label_weights_list, bbox_targets_list,
- bbox_weights_list, avg_factor) = cls_reg_targets
-
- avg_factor = reduce_mean(
- torch.tensor(avg_factor, dtype=torch.float, device=device)).item()
-
- losses_cls, losses_bbox, losses_dfl,\
- avg_factor = multi_apply(
- self.loss_by_feat_single,
- anchor_list,
- cls_scores,
- bbox_preds,
- labels_list,
- label_weights_list,
- bbox_targets_list,
- self.prior_generator.strides,
- avg_factor=avg_factor)
-
- avg_factor = sum(avg_factor)
- avg_factor = reduce_mean(avg_factor).clamp_(min=1).item()
- losses_bbox = list(map(lambda x: x / avg_factor, losses_bbox))
- losses_dfl = list(map(lambda x: x / avg_factor, losses_dfl))
- return dict(
- loss_cls=losses_cls, loss_bbox=losses_bbox, loss_dfl=losses_dfl)
-
- def _predict_by_feat_single(self,
- cls_score_list: List[Tensor],
- bbox_pred_list: List[Tensor],
- score_factor_list: List[Tensor],
- mlvl_priors: List[Tensor],
- img_meta: dict,
- cfg: ConfigDict,
- rescale: bool = False,
- with_nms: bool = True) -> InstanceData:
- """Transform a single image's features extracted from the head into
- bbox results.
-
- Args:
- cls_score_list (list[Tensor]): Box scores from all scale
- levels of a single image, each item has shape
- (num_priors * num_classes, H, W).
- bbox_pred_list (list[Tensor]): Box energies / deltas from
- all scale levels of a single image, each item has shape
- (num_priors * 4, H, W).
- score_factor_list (list[Tensor]): Score factor from all scale
- levels of a single image. GFL head does not need this value.
- mlvl_priors (list[Tensor]): Each element in the list is
- the priors of a single level in feature pyramid, has shape
- (num_priors, 4).
- img_meta (dict): Image meta info.
- cfg (:obj: `ConfigDict`): Test / postprocessing configuration,
- if None, test_cfg would be used.
- rescale (bool): If True, return boxes in original image space.
- Defaults to False.
- with_nms (bool): If True, do nms before return boxes.
- Defaults to True.
-
- Returns:
- tuple[Tensor]: Results of detected bboxes and labels. If with_nms
- is False and mlvl_score_factor is None, return mlvl_bboxes and
- mlvl_scores, else return mlvl_bboxes, mlvl_scores and
- mlvl_score_factor. Usually with_nms is False is used for aug
- test. If with_nms is True, then return the following format
-
- - det_bboxes (Tensor): Predicted bboxes with shape
- [num_bboxes, 5], where the first 4 columns are bounding
- box positions (tl_x, tl_y, br_x, br_y) and the 5-th
- column are scores between 0 and 1.
- - det_labels (Tensor): Predicted labels of the corresponding
- box with shape [num_bboxes].
- """
- cfg = self.test_cfg if cfg is None else cfg
- img_shape = img_meta['img_shape']
- nms_pre = cfg.get('nms_pre', -1)
-
- mlvl_bboxes = []
- mlvl_scores = []
- mlvl_labels = []
- for level_idx, (cls_score, bbox_pred, stride, priors) in enumerate(
- zip(cls_score_list, bbox_pred_list,
- self.prior_generator.strides, mlvl_priors)):
- assert cls_score.size()[-2:] == bbox_pred.size()[-2:]
- assert stride[0] == stride[1]
-
- bbox_pred = bbox_pred.permute(1, 2, 0)
- bbox_pred = self.integral(bbox_pred) * stride[0]
-
- scores = cls_score.permute(1, 2, 0).reshape(
- -1, self.cls_out_channels).sigmoid()
-
- # After https://github.com/open-mmlab/mmdetection/pull/6268/,
- # this operation keeps fewer bboxes under the same `nms_pre`.
- # There is no difference in performance for most models. If you
- # find a slight drop in performance, you can set a larger
- # `nms_pre` than before.
- results = filter_scores_and_topk(
- scores, cfg.score_thr, nms_pre,
- dict(bbox_pred=bbox_pred, priors=priors))
- scores, labels, _, filtered_results = results
-
- bbox_pred = filtered_results['bbox_pred']
- priors = filtered_results['priors']
-
- bboxes = self.bbox_coder.decode(
- self.anchor_center(priors), bbox_pred, max_shape=img_shape)
- mlvl_bboxes.append(bboxes)
- mlvl_scores.append(scores)
- mlvl_labels.append(labels)
-
- results = InstanceData()
- results.bboxes = torch.cat(mlvl_bboxes)
- results.scores = torch.cat(mlvl_scores)
- results.labels = torch.cat(mlvl_labels)
-
- return self._bbox_post_process(
- results=results,
- cfg=cfg,
- rescale=rescale,
- with_nms=with_nms,
- img_meta=img_meta)
-
- def get_targets(self,
- anchor_list: List[Tensor],
- valid_flag_list: List[Tensor],
- batch_gt_instances: InstanceList,
- batch_img_metas: List[dict],
- batch_gt_instances_ignore: OptInstanceList = None,
- unmap_outputs=True) -> tuple:
- """Get targets for GFL head.
-
- This method is almost the same as `AnchorHead.get_targets()`. Besides
- returning the targets as the parent method does, it also returns the
- anchors as the first element of the returned tuple.
- """
- num_imgs = len(batch_img_metas)
- assert len(anchor_list) == len(valid_flag_list) == num_imgs
-
- # anchor number of multi levels
- num_level_anchors = [anchors.size(0) for anchors in anchor_list[0]]
- num_level_anchors_list = [num_level_anchors] * num_imgs
-
- # concat all level anchors and flags to a single tensor
- for i in range(num_imgs):
- assert len(anchor_list[i]) == len(valid_flag_list[i])
- anchor_list[i] = torch.cat(anchor_list[i])
- valid_flag_list[i] = torch.cat(valid_flag_list[i])
-
- # compute targets for each image
- if batch_gt_instances_ignore is None:
- batch_gt_instances_ignore = [None] * num_imgs
- (all_anchors, all_labels, all_label_weights, all_bbox_targets,
- all_bbox_weights, pos_inds_list, neg_inds_list,
- sampling_results_list) = multi_apply(
- self._get_targets_single,
- anchor_list,
- valid_flag_list,
- num_level_anchors_list,
- batch_gt_instances,
- batch_img_metas,
- batch_gt_instances_ignore,
- unmap_outputs=unmap_outputs)
- # Get `avg_factor` of all images, which calculate in `SamplingResult`.
- # When using sampling method, avg_factor is usually the sum of
- # positive and negative priors. When using `PseudoSampler`,
- # `avg_factor` is usually equal to the number of positive priors.
- avg_factor = sum(
- [results.avg_factor for results in sampling_results_list])
- # split targets to a list w.r.t. multiple levels
- anchors_list = images_to_levels(all_anchors, num_level_anchors)
- labels_list = images_to_levels(all_labels, num_level_anchors)
- label_weights_list = images_to_levels(all_label_weights,
- num_level_anchors)
- bbox_targets_list = images_to_levels(all_bbox_targets,
- num_level_anchors)
- bbox_weights_list = images_to_levels(all_bbox_weights,
- num_level_anchors)
- return (anchors_list, labels_list, label_weights_list,
- bbox_targets_list, bbox_weights_list, avg_factor)
-
- def _get_targets_single(self,
- flat_anchors: Tensor,
- valid_flags: Tensor,
- num_level_anchors: List[int],
- gt_instances: InstanceData,
- img_meta: dict,
- gt_instances_ignore: Optional[InstanceData] = None,
- unmap_outputs: bool = True) -> tuple:
- """Compute regression, classification targets for anchors in a single
- image.
-
- Args:
- flat_anchors (Tensor): Multi-level anchors of the image, which are
- concatenated into a single tensor of shape (num_anchors, 4)
- valid_flags (Tensor): Multi level valid flags of the image,
- which are concatenated into a single tensor of
- shape (num_anchors,).
- num_level_anchors (list[int]): Number of anchors of each scale
- level.
- gt_instances (:obj:`InstanceData`): Ground truth of instance
- annotations. It usually includes ``bboxes`` and ``labels``
- attributes.
- img_meta (dict): Meta information for current image.
- gt_instances_ignore (:obj:`InstanceData`, optional): Instances
- to be ignored during training. It includes ``bboxes`` attribute
- data that is ignored during training and testing.
- Defaults to None.
- unmap_outputs (bool): Whether to map outputs back to the original
- set of anchors. Defaults to True.
-
- Returns:
- tuple: N is the number of total anchors in the image.
-
- - anchors (Tensor): All anchors in the image with shape (N, 4).
- - labels (Tensor): Labels of all anchors in the image with
- shape (N,).
- - label_weights (Tensor): Label weights of all anchor in the
- image with shape (N,).
- - bbox_targets (Tensor): BBox targets of all anchors in the
- image with shape (N, 4).
- - bbox_weights (Tensor): BBox weights of all anchors in the
- image with shape (N, 4).
- - pos_inds (Tensor): Indices of positive anchor with shape
- (num_pos,).
- - neg_inds (Tensor): Indices of negative anchor with shape
- (num_neg,).
- - sampling_result (:obj:`SamplingResult`): Sampling results.
- """
- inside_flags = anchor_inside_flags(flat_anchors, valid_flags,
- img_meta['img_shape'][:2],
- self.train_cfg['allowed_border'])
- if not inside_flags.any():
- raise ValueError(
- 'There is no valid anchor inside the image boundary. Please '
- 'check the image size and anchor sizes, or set '
- '``allowed_border`` to -1 to skip the condition.')
- # assign gt and sample anchors
- anchors = flat_anchors[inside_flags, :]
- num_level_anchors_inside = self.get_num_level_anchors_inside(
- num_level_anchors, inside_flags)
- pred_instances = InstanceData(priors=anchors)
- assign_result = self.assigner.assign(
- pred_instances=pred_instances,
- num_level_priors=num_level_anchors_inside,
- gt_instances=gt_instances,
- gt_instances_ignore=gt_instances_ignore)
-
- sampling_result = self.sampler.sample(
- assign_result=assign_result,
- pred_instances=pred_instances,
- gt_instances=gt_instances)
-
- num_valid_anchors = anchors.shape[0]
- bbox_targets = torch.zeros_like(anchors)
- bbox_weights = torch.zeros_like(anchors)
- labels = anchors.new_full((num_valid_anchors, ),
- self.num_classes,
- dtype=torch.long)
- label_weights = anchors.new_zeros(num_valid_anchors, dtype=torch.float)
-
- pos_inds = sampling_result.pos_inds
- neg_inds = sampling_result.neg_inds
- if len(pos_inds) > 0:
- pos_bbox_targets = sampling_result.pos_gt_bboxes
- bbox_targets[pos_inds, :] = pos_bbox_targets
- bbox_weights[pos_inds, :] = 1.0
-
- labels[pos_inds] = sampling_result.pos_gt_labels
- if self.train_cfg['pos_weight'] <= 0:
- label_weights[pos_inds] = 1.0
- else:
- label_weights[pos_inds] = self.train_cfg['pos_weight']
- if len(neg_inds) > 0:
- label_weights[neg_inds] = 1.0
-
- # map up to original set of anchors
- if unmap_outputs:
- num_total_anchors = flat_anchors.size(0)
- anchors = unmap(anchors, num_total_anchors, inside_flags)
- labels = unmap(
- labels, num_total_anchors, inside_flags, fill=self.num_classes)
- label_weights = unmap(label_weights, num_total_anchors,
- inside_flags)
- bbox_targets = unmap(bbox_targets, num_total_anchors, inside_flags)
- bbox_weights = unmap(bbox_weights, num_total_anchors, inside_flags)
-
- return (anchors, labels, label_weights, bbox_targets, bbox_weights,
- pos_inds, neg_inds, sampling_result)
-
- def get_num_level_anchors_inside(self, num_level_anchors: List[int],
- inside_flags: Tensor) -> List[int]:
- """Get the number of valid anchors in every level."""
-
- split_inside_flags = torch.split(inside_flags, num_level_anchors)
- num_level_anchors_inside = [
- int(flags.sum()) for flags in split_inside_flags
- ]
- return num_level_anchors_inside
diff --git a/spaces/LUOYE-123/QQsign/devices/device_8950.js b/spaces/LUOYE-123/QQsign/devices/device_8950.js
deleted file mode 100644
index fe1caad4a8c5eb07633510e1d8a890197056a211..0000000000000000000000000000000000000000
--- a/spaces/LUOYE-123/QQsign/devices/device_8950.js
+++ /dev/null
@@ -1,344 +0,0 @@
-"use strict";
-var __importDefault = (this && this.__importDefault) || function (mod) {
- return (mod && mod.__esModule) ? mod : { "default": mod };
-};
-Object.defineProperty(exports, "__esModule", { value: true });
-exports.getApkInfo = exports.Platform = exports.Device = exports.generateFullDevice = exports.generateShortDevice = void 0;
-const crypto_1 = require("crypto");
-const constants_1 = require("./constants");
-const axios_1 = __importDefault(require("axios"));
-const algo_1 = require("./algo");
-function generateImei() {
- let imei = `86${(0, constants_1.randomString)(12, '0123456789')}`;
- function calcSP(imei) {
- let sum = 0;
- for (let i = 0; i < imei.length; ++i) {
- if (i % 2) {
- let j = parseInt(imei[i]) * 2;
- sum += j % 10 + Math.floor(j / 10);
- }
- else {
- sum += parseInt(imei[i]);
- }
- }
- return (100 - sum) % 10;
- }
- return imei + calcSP(imei);
-}
-/** 生成短设备信息 */
-function generateShortDevice() {
- const randstr = (length, num = false) => {
- const map = num ? '0123456789' : '0123456789abcdef';
- return (0, constants_1.randomString)(length, map);
- };
- return {
- "--begin--": "该设备为随机生成,丢失后不能得到原先配置",
- product: `ILPP-${randstr(5).toUpperCase()}`,
- device: `${randstr(5).toUpperCase()}`,
- board: `${randstr(5).toUpperCase()}`,
- brand: `${randstr(4).toUpperCase()}`,
- model: `ICQQ ${randstr(4).toUpperCase()}`,
- wifi_ssid: `HUAWEI-${randstr(7)}`,
- bootloader: `U-boot`,
- android_id: `IL.${randstr(7, true)}.${randstr(4, true)}`,
- boot_id: `${randstr(8)}-${randstr(4)}-${randstr(4)}-${randstr(4)}-${randstr(12)}`,
- proc_version: `Linux version 5.10.101-android12-${randstr(8)}`,
- mac_address: `2D:${randstr(2).toUpperCase()}:${randstr(2).toUpperCase()}:${randstr(2).toUpperCase()}:${randstr(2).toUpperCase()}:${randstr(2).toUpperCase()}`,
- ip_address: `192.168.${randstr(2, true)}.${randstr(2, true)}`,
- imei: `${generateImei()}`,
- incremental: `${randstr(10, true).toUpperCase()}`,
- "--end--": "修改后可能需要重新验证设备。"
- };
-}
-exports.generateShortDevice = generateShortDevice;
-/** 生成完整设备信息 */
-function generateFullDevice(apk, d) {
- if (!d)
- d = generateShortDevice();
- return {
- display: d.android_id,
- product: d.product,
- device: d.device,
- board: d.board,
- brand: d.brand,
- model: d.model,
- bootloader: d.bootloader,
- fingerprint: `${d.brand}/${d.product}/${d.device}:10/${d.android_id}/${d.incremental}:user/release-keys`,
- boot_id: d.boot_id,
- proc_version: d.proc_version,
- baseband: "",
- sim: "T-Mobile",
- os_type: "android",
- mac_address: d.mac_address,
- ip_address: d.ip_address,
- wifi_bssid: d.mac_address,
- wifi_ssid: d.wifi_ssid,
- imei: d.imei,
- android_id: (0, constants_1.md5)(d.android_id).toString("hex"),
- apn: "wifi",
- version: {
- incremental: d.incremental,
- release: "10",
- codename: "REL",
- sdk: 29,
- },
- imsi: (0, crypto_1.randomBytes)(16),
- guid: (0, constants_1.md5)(Buffer.concat([Buffer.from(d.imei), Buffer.from(d.mac_address)])),
- };
-}
-exports.generateFullDevice = generateFullDevice;
-class Device {
- constructor(apk, d) {
- this.apk = apk;
- this.secret = 'ZdJqM15EeO2zWc08';
- this.publicKey = `-----BEGIN PUBLIC KEY-----
-MIGfMA0GCSqGSIb3DQEBAQUAA4GNADCBiQKBgQDEIxgwoutfwoJxcGQeedgP7FG9
-qaIuS0qzfR8gWkrkTZKM2iWHn2ajQpBRZjMSoSf6+KJGvar2ORhBfpDXyVtZCKpq
-LQ+FLkpncClKVIrBwv6PHyUvuCb0rIarmgDnzkfQAqVufEtR64iazGDKatvJ9y6B
-9NMbHddGSAUmRTCrHQIDAQAB
------END PUBLIC KEY-----`;
- if (!d)
- d = generateShortDevice();
- Object.assign(this, generateFullDevice(apk, d));
- }
- async getQIMEI() {
- if (this.apk.app_key === "") {
- return;
- }
- const k = (0, constants_1.randomString)(16);
- const key = (0, algo_1.encryptPKCS1)(this.publicKey, k);
- const time = Date.now();
- const nonce = (0, constants_1.randomString)(16);
- const payload = this.genRandomPayloadByDevice();
- const params = (0, algo_1.aesEncrypt)(JSON.stringify(payload), k).toString('base64');
- try {
- const { data } = await axios_1.default.post("https://snowflake.qq.com/ola/android", {
- key,
- params,
- time, nonce,
- sign: (0, constants_1.md5)(key + params + time + nonce + this.secret).toString("hex"),
- extra: ''
- }, {
- headers: {
- 'User-Agent': `Dalvik/2.1.0 (Linux; U; Android ${this.version.release}; PCRT00 Build/N2G48H)`,
- 'Content-Type': "application/json"
- }
- });
- if (data?.code !== 0) {
- return;
- }
- const { q16, q36 } = JSON.parse((0, algo_1.aesDecrypt)(data.data, k));
- this.qImei16 = q16;
- this.qImei36 = q36;
- }
- catch {
- }
- }
- genRandomPayloadByDevice() {
- const fixedRand = (max = 1, min = 0) => {
- if (max < min)
- [max, min] = [min, max];
- const diff = max - min;
- return Math.floor(Math.random() * diff) + min;
- };
- const reserved = {
- "harmony": "0",
- "clone": Math.random() > 0.5 ? "1" : "0",
- "containe": "",
- "oz": "",
- "oo": "",
- "kelong": Math.random() > 0.5 ? "1" : "0",
- "uptimes": (0, constants_1.formatTime)(new Date()),
- "multiUser": Math.random() > 0.5 ? "1" : "0",
- "bod": this.board,
- "brd": this.brand,
- "dv": this.device,
- "firstLevel": "",
- "manufact": this.brand,
- "name": this.model,
- "host": "se.infra",
- "kernel": this.fingerprint
- };
- const timestamp = Date.now();
- this.mtime = this.mtime || Date.now();
- const mtime1 = new Date(this.mtime || Date.now());
- const dateFormat = (fmt, time = Date.now()) => (0, constants_1.formatTime)(time, fmt);
- const mtimeStr1 = dateFormat("YYYY-mm-ddHHMMSS", mtime1) + "." + this.imei.slice(2, 11);
- const mtime2 = new Date(this.mtime - parseInt(this.imei.slice(2, 4)));
- const mtimeStr2 = dateFormat("YYYY-mm-ddHHMMSS", mtime2) + "." + this.imei.slice(5, 14);
- let beaconIdArr = [
- `${(0, constants_1.formatTime)(new Date(timestamp + fixedRand(60, 0)))}.${String(fixedRand(99, 0)).padStart(2, '0')}0000000`,
- mtimeStr1,
- '0000000000000000',
- (0, constants_1.md5)(this.android_id + this.imei).toString("hex").slice(0, 16),
- ...new Array(4).fill(false).map((_) => fixedRand(10000000, 1000000)),
- this.boot_id,
- '1',
- fixedRand(5, 0),
- fixedRand(5, 0),
- `${(0, constants_1.formatTime)(new Date(timestamp + fixedRand(60, 0)))}.${String(fixedRand(99, 0)).padStart(2, '0')}0000000`,
- `${(0, constants_1.formatTime)(new Date(timestamp + fixedRand(60, 0)))}.${String(fixedRand(99, 0)).padStart(2, '0')}0000000`,
- fixedRand(5, 0),
- fixedRand(100, 10),
- `${(0, constants_1.formatTime)(new Date(timestamp + fixedRand(60, 0)))}.${String(fixedRand(99, 0)).padStart(2, '0')}0000000`,
- `${(0, constants_1.formatTime)(new Date(timestamp + fixedRand(60, 0)))}.${String(fixedRand(99, 0)).padStart(2, '0')}0000000`,
- fixedRand(50000, 10000),
- fixedRand(100, 10),
- `${(0, constants_1.formatTime)(new Date(timestamp + fixedRand(60, 0)))}.${String(fixedRand(99, 0)).padStart(2, '0')}0000000`,
- mtimeStr2,
- fixedRand(10000, 1000),
- fixedRand(5, 0),
- `${dateFormat("YYYY-mm-ddHHMMSS")}.${String(((10 + parseInt(this.imei.slice(5, 7))) % 100)).padStart(2, "0")}0000000`,
- `${dateFormat("YYYY-mm-ddHHMMSS")}.${String(((11 + parseInt(this.imei.slice(5, 7))) % 100)).padStart(2, "0")}0000000`,
- fixedRand(10000, 1000),
- fixedRand(100, 10),
- `${dateFormat("YYYY-mm-ddHHMMSS")}.${String(((11 + parseInt(this.imei.slice(5, 7))) % 100)).padStart(2, "0")}0000000`,
- `${dateFormat("YYYY-mm-ddHHMMSS")}.${String(((11 + parseInt(this.imei.slice(5, 7))) % 100)).padStart(2, "0")}0000000`,
- fixedRand(10000, 1000),
- fixedRand(5, 0),
- `${(0, constants_1.formatTime)(new Date(timestamp + fixedRand(60, 0)))}.${String(fixedRand(99, 0)).padStart(2, '0')}0000000`,
- `${(0, constants_1.formatTime)(new Date(timestamp + fixedRand(60, 0)))}.${String(fixedRand(99, 0)).padStart(2, '0')}0000000`,
- fixedRand(5, 0),
- fixedRand(100, 10),
- `${(0, constants_1.formatTime)(new Date(timestamp + fixedRand(60, 0)))}.${String(fixedRand(99, 0)).padStart(2, '0')}0000000`,
- `${(0, constants_1.formatTime)(new Date(timestamp + fixedRand(60, 0)))}.${String(fixedRand(99, 0)).padStart(2, '0')}0000000`,
- fixedRand(5, 0),
- fixedRand(5, 0),
- ].map((str, idx) => `k${idx + 1}:${str}`);
- return {
- "androidId": this.android_id,
- "platformId": 1,
- "appKey": this.apk.app_key,
- "appVersion": this.apk.version,
- "beaconIdSrc": beaconIdArr.join(';'),
- "brand": this.brand,
- "channelId": "2017",
- "cid": "",
- "imei": this.imei,
- "imsi": this.imsi.toString("hex"),
- "mac": this.mac_address,
- "model": this.model,
- "networkType": "unknown",
- "oaid": "",
- "osVersion": `Android ${this.version.release},level ${this.version.sdk}`,
- "qimei": "",
- "qimei36": "",
- "sdkVersion": "1.2.13.6",
- "targetSdkVersion": "26",
- "audit": "",
- "userId": "{}",
- "packageId": this.apk.id,
- "deviceType": this.display,
- "sdkName": "",
- "reserved": JSON.stringify(reserved),
- };
- }
-}
-exports.Device = Device;
-/** 支持的登录设备平台 */
-var Platform;
-(function (Platform) {
- Platform[Platform["Android"] = 1] = "Android";
- Platform[Platform["aPad"] = 2] = "aPad";
- Platform[Platform["Watch"] = 3] = "Watch";
- Platform[Platform["iMac"] = 4] = "iMac";
- Platform[Platform["iPad"] = 5] = "iPad";
- Platform[Platform["Tim"] = 6] = "Tim";
-})(Platform || (exports.Platform = Platform = {}));
-const mobile = {
- id: "com.tencent.mobileqq",
- app_key: '0S200MNJT807V3GE',
- name: "A8.9.50.f5a7d351",
- version: "8.9.50.10650",
- ver: "8.9.50",
- sign: Buffer.from('A6 B7 45 BF 24 A2 C2 77 52 77 16 F6 F3 6E B6 8D'.split(' ').map(s => parseInt(s, 16))),
- buildtime: 1676531414,
- appid: 16,
- subid: 537155547,
- bitmap: 150470524,
- main_sig_map: 16724722,
- sub_sig_map: 0x10400,
- sdkver: "6.0.0.2535",
- display: "Android",
- qua: 'V1_AND_SQ_8.9.50_3898_YYB_D',
- ssover: 19,
-};
-const tim = {
- id: "com.tencent.tim",
- app_key: '0S200MNJT807V3GE',
- name: "A3.5.1.3168",
- version: "3.5.1.3168",
- ver: "3.5.1",
- sign: Buffer.from('775e696d09856872fdd8ab4f3f06b1e0', 'hex'),
- buildtime: 1630062176,
- appid: 16,
- subid: 537150355,
- bitmap: 150470524,
- main_sig_map: 16724722,
- sub_sig_map: 0x10400,
- sdkver: "6.0.0.2484",
- display: "Tim",
- qua: "V1_AND_SQ_8.3.9_351_TIM_D",
- ssover: 18,
-};
-const watch = {
- id: "com.tencent.qqlite",
- app_key: '0S200MNJT807V3GE',
- name: "A2.0.8",
- version: "2.0.8",
- ver: "2.0.8",
- sign: Buffer.from('A6 B7 45 BF 24 A2 C2 77 52 77 16 F6 F3 6E B6 8D'.split(' ').map(s => parseInt(s, 16))),
- buildtime: 1559564731,
- appid: 16,
- subid: 537065138,
- bitmap: 16252796,
- main_sig_map: 16724722,
- sub_sig_map: 0x10400,
- sdkver: "6.0.0.2365",
- display: "Watch",
- qua: '',
- ssover: 5
-};
-const hd = {
- id: "com.tencent.minihd.qq",
- app_key: '0S200MNJT807V3GE',
- name: "A5.9.3.3468",
- version: "5.9.3.3468",
- ver: "5.9.3",
- sign: Buffer.from('AA 39 78 F4 1F D9 6F F9 91 4A 66 9E 18 64 74 C7'.split(' ').map(s => parseInt(s, 16))),
- buildtime: 1637427966,
- appid: 16,
- subid: 537128930,
- bitmap: 150470524,
- main_sig_map: 1970400,
- sub_sig_map: 66560,
- sdkver: "6.0.0.2433",
- display: "iMac",
- qua: '',
- ssover: 12
-};
-const apklist = {
- [Platform.Android]: mobile,
- [Platform.Tim]: tim,
- [Platform.aPad]: {
- ...mobile,
- subid: 537155599,
- display: 'aPad'
- },
- [Platform.Watch]: watch,
- [Platform.iMac]: { ...hd },
- [Platform.iPad]: {
- ...mobile,
- subid: 537155074,
- sign: hd.sign,
- name: 'A8.9.50.611',
- version: 'A8.9.50.611',
- sdkver: '6.0.0.2535',
- qua: 'V1_AND_SQ_8.9.50_3898_YYB_D',
- display: 'iPad'
- },
-};
-function getApkInfo(p) {
- return apklist[p] || apklist[Platform.Android];
-}
-exports.getApkInfo = getApkInfo;
diff --git a/spaces/LZRi/LZR-Bert-VITS2/train_ms.py b/spaces/LZRi/LZR-Bert-VITS2/train_ms.py
deleted file mode 100644
index 5d109003d40497ea4493e7c73f47c1eb7370a81e..0000000000000000000000000000000000000000
--- a/spaces/LZRi/LZR-Bert-VITS2/train_ms.py
+++ /dev/null
@@ -1,402 +0,0 @@
-import os
-import json
-import argparse
-import itertools
-import math
-import torch
-import shutil
-from torch import nn, optim
-from torch.nn import functional as F
-from torch.utils.data import DataLoader
-from torch.utils.tensorboard import SummaryWriter
-import torch.multiprocessing as mp
-import torch.distributed as dist
-from torch.nn.parallel import DistributedDataParallel as DDP
-from torch.cuda.amp import autocast, GradScaler
-from tqdm import tqdm
-import logging
-logging.getLogger('numba').setLevel(logging.WARNING)
-import commons
-import utils
-from data_utils import (
- TextAudioSpeakerLoader,
- TextAudioSpeakerCollate,
- DistributedBucketSampler
-)
-from models import (
- SynthesizerTrn,
- MultiPeriodDiscriminator,
- DurationDiscriminator,
-)
-from losses import (
- generator_loss,
- discriminator_loss,
- feature_loss,
- kl_loss
-)
-from mel_processing import mel_spectrogram_torch, spec_to_mel_torch
-from text.symbols import symbols
-
-torch.backends.cudnn.benchmark = True
-torch.backends.cuda.matmul.allow_tf32 = True
-torch.backends.cudnn.allow_tf32 = True
-torch.set_float32_matmul_precision('medium')
-global_step = 0
-
-
-def main():
- """Assume Single Node Multi GPUs Training Only"""
- assert torch.cuda.is_available(), "CPU training is not allowed."
-
- n_gpus = torch.cuda.device_count()
- os.environ['MASTER_ADDR'] = 'localhost'
- os.environ['MASTER_PORT'] = '65280'
-
- hps = utils.get_hparams()
- if not hps.cont:
- shutil.copy('./pretrained_models/D_0.pth','./logs/OUTPUT_MODEL/D_0.pth')
- shutil.copy('./pretrained_models/G_0.pth','./logs/OUTPUT_MODEL/G_0.pth')
- shutil.copy('./pretrained_models/DUR_0.pth','./logs/OUTPUT_MODEL/DUR_0.pth')
- mp.spawn(run, nprocs=n_gpus, args=(n_gpus, hps,))
-
-
-def run(rank, n_gpus, hps):
- global global_step
- if rank == 0:
- logger = utils.get_logger(hps.model_dir)
- logger.info(hps)
- utils.check_git_hash(hps.model_dir)
- writer = SummaryWriter(log_dir=hps.model_dir)
- writer_eval = SummaryWriter(log_dir=os.path.join(hps.model_dir, "eval"))
-
- dist.init_process_group(backend= 'gloo' if os.name == 'nt' else 'nccl', init_method='env://', world_size=n_gpus, rank=rank)
- torch.manual_seed(hps.train.seed)
- torch.cuda.set_device(rank)
-
- train_dataset = TextAudioSpeakerLoader(hps.data.training_files, hps.data)
- train_sampler = DistributedBucketSampler(
- train_dataset,
- hps.train.batch_size,
- [32, 300, 400, 500, 600, 700, 800, 900, 1000],
- num_replicas=n_gpus,
- rank=rank,
- shuffle=True)
- collate_fn = TextAudioSpeakerCollate()
- train_loader = DataLoader(train_dataset, num_workers=2, shuffle=False, pin_memory=True,
- collate_fn=collate_fn, batch_sampler=train_sampler)
- if rank == 0:
- eval_dataset = TextAudioSpeakerLoader(hps.data.validation_files, hps.data)
- eval_loader = DataLoader(eval_dataset, num_workers=0, shuffle=False,
- batch_size=1, pin_memory=True,
- drop_last=False, collate_fn=collate_fn)
- if "use_noise_scaled_mas" in hps.model.keys() and hps.model.use_noise_scaled_mas == True:
- print("Using noise scaled MAS for VITS2")
- use_noise_scaled_mas = True
- mas_noise_scale_initial = 0.01
- noise_scale_delta = 2e-6
- else:
- print("Using normal MAS for VITS1")
- use_noise_scaled_mas = False
- mas_noise_scale_initial = 0.0
- noise_scale_delta = 0.0
- if "use_duration_discriminator" in hps.model.keys() and hps.model.use_duration_discriminator == True:
- print("Using duration discriminator for VITS2")
- use_duration_discriminator = True
- net_dur_disc = DurationDiscriminator(
- hps.model.hidden_channels,
- hps.model.hidden_channels,
- 3,
- 0.1,
- gin_channels=hps.model.gin_channels if hps.data.n_speakers != 0 else 0,
- ).cuda(rank)
- if "use_spk_conditioned_encoder" in hps.model.keys() and hps.model.use_spk_conditioned_encoder == True:
- if hps.data.n_speakers == 0:
- raise ValueError("n_speakers must be > 0 when using spk conditioned encoder to train multi-speaker model")
- use_spk_conditioned_encoder = True
- else:
- print("Using normal encoder for VITS1")
- use_spk_conditioned_encoder = False
-
- net_g = SynthesizerTrn(
- len(symbols),
- hps.data.filter_length // 2 + 1,
- hps.train.segment_size // hps.data.hop_length,
- n_speakers=hps.data.n_speakers,
- mas_noise_scale_initial = mas_noise_scale_initial,
- noise_scale_delta = noise_scale_delta,
- **hps.model).cuda(rank)
-
- freeze_enc = getattr(hps.model, "freeze_enc", False)
- if freeze_enc:
- print("freeze encoder !!!")
- for param in net_g.enc_p.parameters():
- param.requires_grad = False
-
- net_d = MultiPeriodDiscriminator(hps.model.use_spectral_norm).cuda(rank)
- optim_g = torch.optim.AdamW(
- filter(lambda p: p.requires_grad, net_g.parameters()),
- hps.train.learning_rate,
- betas=hps.train.betas,
- eps=hps.train.eps)
- optim_d = torch.optim.AdamW(
- net_d.parameters(),
- hps.train.learning_rate,
- betas=hps.train.betas,
- eps=hps.train.eps)
- if net_dur_disc is not None:
- optim_dur_disc = torch.optim.AdamW(
- net_dur_disc.parameters(),
- hps.train.learning_rate,
- betas=hps.train.betas,
- eps=hps.train.eps)
- else:
- optim_dur_disc = None
- net_g = DDP(net_g, device_ids=[rank], find_unused_parameters=True)
- net_d = DDP(net_d, device_ids=[rank], find_unused_parameters=True)
- if net_dur_disc is not None:
- net_dur_disc = DDP(net_dur_disc, device_ids=[rank], find_unused_parameters=True)
-
- pretrain_dir = None
- if pretrain_dir is None:
- try:
- if net_dur_disc is not None:
- _, optim_dur_disc, _, epoch_str = utils.load_checkpoint(utils.latest_checkpoint_path(hps.model_dir, "DUR_*.pth"), net_dur_disc, optim_dur_disc, skip_optimizer=not hps.cont)
- _, optim_g, _, epoch_str = utils.load_checkpoint(utils.latest_checkpoint_path(hps.model_dir, "G_*.pth"), net_g,
- optim_g, skip_optimizer=not hps.cont)
- _, optim_d, _, epoch_str = utils.load_checkpoint(utils.latest_checkpoint_path(hps.model_dir, "D_*.pth"), net_d,
- optim_d, skip_optimizer=not hps.cont)
-
- epoch_str = max(epoch_str, 1)
- global_step = (epoch_str - 1) * len(train_loader)
- except Exception as e:
- print(e)
- epoch_str = 1
- global_step = 0
- else:
- _, _, _, epoch_str = utils.load_checkpoint(utils.latest_checkpoint_path(pretrain_dir, "G_*.pth"), net_g,
- optim_g, True)
- _, _, _, epoch_str = utils.load_checkpoint(utils.latest_checkpoint_path(pretrain_dir, "D_*.pth"), net_d,
- optim_d, True)
-
-
-
- scheduler_g = torch.optim.lr_scheduler.ExponentialLR(optim_g, gamma=hps.train.lr_decay, last_epoch=epoch_str - 2)
- scheduler_d = torch.optim.lr_scheduler.ExponentialLR(optim_d, gamma=hps.train.lr_decay, last_epoch=epoch_str - 2)
- if net_dur_disc is not None:
- scheduler_dur_disc = torch.optim.lr_scheduler.ExponentialLR(optim_dur_disc, gamma=hps.train.lr_decay, last_epoch=epoch_str-2)
- else:
- scheduler_dur_disc = None
- scaler = GradScaler(enabled=hps.train.fp16_run)
-
- for epoch in range(epoch_str, hps.train.epochs + 1):
- if rank == 0:
- train_and_evaluate(rank, epoch, hps, [net_g, net_d, net_dur_disc], [optim_g, optim_d, optim_dur_disc], [scheduler_g, scheduler_d, scheduler_dur_disc], scaler, [train_loader, eval_loader], logger, [writer, writer_eval])
- else:
- train_and_evaluate(rank, epoch, hps, [net_g, net_d, net_dur_disc], [optim_g, optim_d, optim_dur_disc], [scheduler_g, scheduler_d, scheduler_dur_disc], scaler, [train_loader, None], None, None)
- scheduler_g.step()
- scheduler_d.step()
- if net_dur_disc is not None:
- scheduler_dur_disc.step()
-
-
-def train_and_evaluate(rank, epoch, hps, nets, optims, schedulers, scaler, loaders, logger, writers):
- net_g, net_d, net_dur_disc = nets
- optim_g, optim_d, optim_dur_disc = optims
- scheduler_g, scheduler_d, scheduler_dur_disc = schedulers
- train_loader, eval_loader = loaders
- if writers is not None:
- writer, writer_eval = writers
-
- train_loader.batch_sampler.set_epoch(epoch)
- global global_step
-
- net_g.train()
- net_d.train()
- if net_dur_disc is not None:
- net_dur_disc.train()
- for batch_idx, (x, x_lengths, spec, spec_lengths, y, y_lengths, speakers, tone, language, bert) in tqdm(enumerate(train_loader)):
- if net_g.module.use_noise_scaled_mas:
- current_mas_noise_scale = net_g.module.mas_noise_scale_initial - net_g.module.noise_scale_delta * global_step
- net_g.module.current_mas_noise_scale = max(current_mas_noise_scale, 0.0)
- x, x_lengths = x.cuda(rank, non_blocking=True), x_lengths.cuda(rank, non_blocking=True)
- spec, spec_lengths = spec.cuda(rank, non_blocking=True), spec_lengths.cuda(rank, non_blocking=True)
- y, y_lengths = y.cuda(rank, non_blocking=True), y_lengths.cuda(rank, non_blocking=True)
- speakers = speakers.cuda(rank, non_blocking=True)
- tone = tone.cuda(rank, non_blocking=True)
- language = language.cuda(rank, non_blocking=True)
- bert = bert.cuda(rank, non_blocking=True)
-
- with autocast(enabled=hps.train.fp16_run):
- y_hat, l_length, attn, ids_slice, x_mask, z_mask, \
- (z, z_p, m_p, logs_p, m_q, logs_q), (hidden_x, logw, logw_) = net_g(x, x_lengths, spec, spec_lengths, speakers, tone, language, bert)
- mel = spec_to_mel_torch(
- spec,
- hps.data.filter_length,
- hps.data.n_mel_channels,
- hps.data.sampling_rate,
- hps.data.mel_fmin,
- hps.data.mel_fmax)
- y_mel = commons.slice_segments(mel, ids_slice, hps.train.segment_size // hps.data.hop_length)
- y_hat_mel = mel_spectrogram_torch(
- y_hat.squeeze(1),
- hps.data.filter_length,
- hps.data.n_mel_channels,
- hps.data.sampling_rate,
- hps.data.hop_length,
- hps.data.win_length,
- hps.data.mel_fmin,
- hps.data.mel_fmax
- )
-
- y = commons.slice_segments(y, ids_slice * hps.data.hop_length, hps.train.segment_size) # slice
-
- # Discriminator
- y_d_hat_r, y_d_hat_g, _, _ = net_d(y, y_hat.detach())
- with autocast(enabled=False):
- loss_disc, losses_disc_r, losses_disc_g = discriminator_loss(y_d_hat_r, y_d_hat_g)
- loss_disc_all = loss_disc
- if net_dur_disc is not None:
- y_dur_hat_r, y_dur_hat_g = net_dur_disc(hidden_x.detach(), x_mask.detach(), logw.detach(), logw_.detach())
- with autocast(enabled=False):
- # TODO: I think need to mean using the mask, but for now, just mean all
- loss_dur_disc, losses_dur_disc_r, losses_dur_disc_g = discriminator_loss(y_dur_hat_r, y_dur_hat_g)
- loss_dur_disc_all = loss_dur_disc
- optim_dur_disc.zero_grad()
- scaler.scale(loss_dur_disc_all).backward()
- scaler.unscale_(optim_dur_disc)
- grad_norm_dur_disc = commons.clip_grad_value_(net_dur_disc.parameters(), None)
- scaler.step(optim_dur_disc)
-
- optim_d.zero_grad()
- scaler.scale(loss_disc_all).backward()
- scaler.unscale_(optim_d)
- grad_norm_d = commons.clip_grad_value_(net_d.parameters(), None)
- scaler.step(optim_d)
-
- with autocast(enabled=hps.train.fp16_run):
- # Generator
- y_d_hat_r, y_d_hat_g, fmap_r, fmap_g = net_d(y, y_hat)
- if net_dur_disc is not None:
- y_dur_hat_r, y_dur_hat_g = net_dur_disc(hidden_x, x_mask, logw, logw_)
- with autocast(enabled=False):
- loss_dur = torch.sum(l_length.float())
- loss_mel = F.l1_loss(y_mel, y_hat_mel) * hps.train.c_mel
- loss_kl = kl_loss(z_p, logs_q, m_p, logs_p, z_mask) * hps.train.c_kl
-
- loss_fm = feature_loss(fmap_r, fmap_g)
- loss_gen, losses_gen = generator_loss(y_d_hat_g)
- loss_gen_all = loss_gen + loss_fm + loss_mel + loss_dur + loss_kl
- if net_dur_disc is not None:
- loss_dur_gen, losses_dur_gen = generator_loss(y_dur_hat_g)
- loss_gen_all += loss_dur_gen
- optim_g.zero_grad()
- scaler.scale(loss_gen_all).backward()
- scaler.unscale_(optim_g)
- grad_norm_g = commons.clip_grad_value_(net_g.parameters(), None)
- scaler.step(optim_g)
- scaler.update()
-
- if rank == 0:
- if global_step % hps.train.log_interval == 0:
- lr = optim_g.param_groups[0]['lr']
- losses = [loss_disc, loss_gen, loss_fm, loss_mel, loss_dur, loss_kl]
- logger.info('Train Epoch: {} [{:.0f}%]'.format(
- epoch,
- 100. * batch_idx / len(train_loader)))
- logger.info([x.item() for x in losses] + [global_step, lr])
-
- scalar_dict = {"loss/g/total": loss_gen_all, "loss/d/total": loss_disc_all, "learning_rate": lr,
- "grad_norm_d": grad_norm_d, "grad_norm_g": grad_norm_g}
- scalar_dict.update(
- {"loss/g/fm": loss_fm, "loss/g/mel": loss_mel, "loss/g/dur": loss_dur, "loss/g/kl": loss_kl})
- scalar_dict.update({"loss/g/{}".format(i): v for i, v in enumerate(losses_gen)})
- scalar_dict.update({"loss/d_r/{}".format(i): v for i, v in enumerate(losses_disc_r)})
- scalar_dict.update({"loss/d_g/{}".format(i): v for i, v in enumerate(losses_disc_g)})
-
- image_dict = {
- "slice/mel_org": utils.plot_spectrogram_to_numpy(y_mel[0].data.cpu().numpy()),
- "slice/mel_gen": utils.plot_spectrogram_to_numpy(y_hat_mel[0].data.cpu().numpy()),
- "all/mel": utils.plot_spectrogram_to_numpy(mel[0].data.cpu().numpy()),
- "all/attn": utils.plot_alignment_to_numpy(attn[0, 0].data.cpu().numpy())
- }
- utils.summarize(
- writer=writer,
- global_step=global_step,
- images=image_dict,
- scalars=scalar_dict)
-
- if global_step % hps.train.eval_interval == 0:
- evaluate(hps, net_g, eval_loader, writer_eval)
- utils.save_checkpoint(net_g, optim_g, hps.train.learning_rate, epoch,
- os.path.join(hps.model_dir, "G_{}.pth".format(global_step)))
- utils.save_checkpoint(net_d, optim_d, hps.train.learning_rate, epoch,
- os.path.join(hps.model_dir, "D_{}.pth".format(global_step)))
- if net_dur_disc is not None:
- utils.save_checkpoint(net_dur_disc, optim_dur_disc, hps.train.learning_rate, epoch, os.path.join(hps.model_dir, "DUR_{}.pth".format(global_step)))
- keep_ckpts = getattr(hps.train, 'keep_ckpts', 5)
- if keep_ckpts > 0:
- utils.clean_checkpoints(path_to_models=hps.model_dir, n_ckpts_to_keep=keep_ckpts, sort_by_time=True)
-
-
- global_step += 1
-
- if rank == 0:
- logger.info('====> Epoch: {}'.format(epoch))
-
-
-
-def evaluate(hps, generator, eval_loader, writer_eval):
- generator.eval()
- image_dict = {}
- audio_dict = {}
- print("Evaluating ...")
- with torch.no_grad():
- for batch_idx, (x, x_lengths, spec, spec_lengths, y, y_lengths, speakers, tone, language, bert) in enumerate(eval_loader):
- x, x_lengths = x.cuda(), x_lengths.cuda()
- spec, spec_lengths = spec.cuda(), spec_lengths.cuda()
- y, y_lengths = y.cuda(), y_lengths.cuda()
- speakers = speakers.cuda()
- bert = bert.cuda()
- tone = tone.cuda()
- language = language.cuda()
- for use_sdp in [True, False]:
- y_hat, attn, mask, *_ = generator.module.infer(x, x_lengths, speakers, tone, language, bert, y=spec, max_len=1000, sdp_ratio=0.0 if not use_sdp else 1.0)
- y_hat_lengths = mask.sum([1, 2]).long() * hps.data.hop_length
-
- mel = spec_to_mel_torch(
- spec,
- hps.data.filter_length,
- hps.data.n_mel_channels,
- hps.data.sampling_rate,
- hps.data.mel_fmin,
- hps.data.mel_fmax)
- y_hat_mel = mel_spectrogram_torch(
- y_hat.squeeze(1).float(),
- hps.data.filter_length,
- hps.data.n_mel_channels,
- hps.data.sampling_rate,
- hps.data.hop_length,
- hps.data.win_length,
- hps.data.mel_fmin,
- hps.data.mel_fmax
- )
- image_dict.update({
- f"gen/mel_{batch_idx}": utils.plot_spectrogram_to_numpy(y_hat_mel[0].cpu().numpy())
- })
- audio_dict.update({
- f"gen/audio_{batch_idx}_{use_sdp}": y_hat[0, :, :y_hat_lengths[0]]
- })
- image_dict.update({f"gt/mel_{batch_idx}": utils.plot_spectrogram_to_numpy(mel[0].cpu().numpy())})
- audio_dict.update({f"gt/audio_{batch_idx}": y[0, :, :y_lengths[0]]})
-
- utils.summarize(
- writer=writer_eval,
- global_step=global_step,
- images=image_dict,
- audios=audio_dict,
- audio_sampling_rate=hps.data.sampling_rate
- )
- generator.train()
-
-if __name__ == "__main__":
- main()
diff --git a/spaces/Lamai/LAMAIGPT/autogpt/config/ai_config.py b/spaces/Lamai/LAMAIGPT/autogpt/config/ai_config.py
deleted file mode 100644
index d50c30beee9dc8009f63415378ae1c6a399f0037..0000000000000000000000000000000000000000
--- a/spaces/Lamai/LAMAIGPT/autogpt/config/ai_config.py
+++ /dev/null
@@ -1,121 +0,0 @@
-# sourcery skip: do-not-use-staticmethod
-"""
-A module that contains the AIConfig class object that contains the configuration
-"""
-from __future__ import annotations
-
-import os
-from typing import Type
-
-import yaml
-
-
-class AIConfig:
- """
- A class object that contains the configuration information for the AI
-
- Attributes:
- ai_name (str): The name of the AI.
- ai_role (str): The description of the AI's role.
- ai_goals (list): The list of objectives the AI is supposed to complete.
- """
-
- def __init__(
- self, ai_name: str = "", ai_role: str = "", ai_goals: list | None = None
- ) -> None:
- """
- Initialize a class instance
-
- Parameters:
- ai_name (str): The name of the AI.
- ai_role (str): The description of the AI's role.
- ai_goals (list): The list of objectives the AI is supposed to complete.
- Returns:
- None
- """
- if ai_goals is None:
- ai_goals = []
- self.ai_name = ai_name
- self.ai_role = ai_role
- self.ai_goals = ai_goals
-
- # Soon this will go in a folder where it remembers more stuff about the run(s)
- SAVE_FILE = os.path.join(os.path.dirname(__file__), "..", "ai_settings.yaml")
-
- @staticmethod
- def load(config_file: str = SAVE_FILE) -> "AIConfig":
- """
- Returns class object with parameters (ai_name, ai_role, ai_goals) loaded from
- yaml file if yaml file exists,
- else returns class with no parameters.
-
- Parameters:
- config_file (int): The path to the config yaml file.
- DEFAULT: "../ai_settings.yaml"
-
- Returns:
- cls (object): An instance of given cls object
- """
-
- try:
- with open(config_file, encoding="utf-8") as file:
- config_params = yaml.load(file, Loader=yaml.FullLoader)
- except FileNotFoundError:
- config_params = {}
-
- ai_name = config_params.get("ai_name", "")
- ai_role = config_params.get("ai_role", "")
- ai_goals = config_params.get("ai_goals", [])
- # type: Type[AIConfig]
- return AIConfig(ai_name, ai_role, ai_goals)
-
- def save(self, config_file: str = SAVE_FILE) -> None:
- """
- Saves the class parameters to the specified file yaml file path as a yaml file.
-
- Parameters:
- config_file(str): The path to the config yaml file.
- DEFAULT: "../ai_settings.yaml"
-
- Returns:
- None
- """
-
- config = {
- "ai_name": self.ai_name,
- "ai_role": self.ai_role,
- "ai_goals": self.ai_goals,
- }
- with open(config_file, "w", encoding="utf-8") as file:
- yaml.dump(config, file, allow_unicode=True)
-
- def construct_full_prompt(self) -> str:
- """
- Returns a prompt to the user with the class information in an organized fashion.
-
- Parameters:
- None
-
- Returns:
- full_prompt (str): A string containing the initial prompt for the user
- including the ai_name, ai_role and ai_goals.
- """
-
- prompt_start = (
- "Your decisions must always be made independently without"
- " seeking user assistance. Play to your strengths as an LLM and pursue"
- " simple strategies with no legal complications."
- ""
- )
-
- from autogpt.prompt import get_prompt
-
- # Construct full prompt
- full_prompt = (
- f"You are {self.ai_name}, {self.ai_role}\n{prompt_start}\n\nGOALS:\n\n"
- )
- for i, goal in enumerate(self.ai_goals):
- full_prompt += f"{i+1}. {goal}\n"
-
- full_prompt += f"\n\n{get_prompt()}"
- return full_prompt
diff --git a/spaces/LaynzKunz/Aesthetic_RVC_Inference_HF/go-applio.bat b/spaces/LaynzKunz/Aesthetic_RVC_Inference_HF/go-applio.bat
deleted file mode 100644
index 70cc1bea97c811535eb36665c4a57acfe788dde4..0000000000000000000000000000000000000000
--- a/spaces/LaynzKunz/Aesthetic_RVC_Inference_HF/go-applio.bat
+++ /dev/null
@@ -1,100 +0,0 @@
-@echo off
-setlocal
-title Applio - Start
-cd %~dp0
-
-:::
-::: _ _
-::: /\ | (_)
-::: / \ _ __ _ __ | |_ ___
-::: / /\ \ | '_ \| '_ \| | |/ _ \
-::: / ____ \| |_) | |_) | | | (_) |
-::: /_/ \_\ .__/| .__/|_|_|\___/
-::: | | | |
-::: |_| |_|
-:::
-:::
-
-for /f "usebackq delims=" %%i in ("%cd%\assets\configs\version.txt") do (
- set "localVersion=%%i"
-)
-for /f %%i in ('powershell -command "(Invoke-WebRequest -Uri 'https://raw.githubusercontent.com/IAHispano/Applio-RVC-Fork/main/assets/configs/version.txt').Content"') do set "onlineVersion=%%i"
-
-:menu
-for /f "delims=: tokens=*" %%A in ('findstr /b ":::" "%~f0"') do @echo(%%A
-powershell -command "if ('%localVersion%' -lt '%onlineVersion%') { exit 1 } else { exit 0 }"
-if %errorlevel% equ 1 (
- echo You are currently using an outdated version %localVersion%
- echo.
- echo We're excited to announce that version %onlineVersion% is now available for download on https://github.com/IAHispano/Applio-RVC-Fork.
- echo Upgrade now to access the latest features and improvements!
- echo.
- goto continue
-) else (
- goto continue
-)
-
-:continue
-echo Runtime: Recommended for regular users
-echo [1] Start Applio - Runtime ^(Nvidia Support^)
-echo [2] Start Applio - Runtime ^(Intel Support. Requires Nvidia runtime^)
-echo [3] Start Applio - Runtime ^(AMD Support^)
-echo.
-echo Dependencies: Only recommended for experienced users
-echo [4] Start Applio ^(Nvidia Support^)
-echo [5] Start Applio ^(AMD Support^)
-echo.
-echo [6] Exit
-echo.
-
-set /p choice=Select an option:
-set choice=%choice: =%
-
-if "%choice%"=="6" (
- goto finish
-) else if "%choice%"=="5" (
- cls
- echo Starting Applio with AMD support...
- python infer-web.py --pycmd python --port 7897 --dml --theme dark
- pause
- cls
- goto menu
-) else if "%choice%"=="4" (
- cls
- echo Starting Applio with Nvidia support...
- python infer-web.py --pycmd python --port 7897 --theme dark
- pause
- cls
- goto menu
-) else if "%choice%"=="3" (
- cls
- echo Starting Applio with runtime for AMD support ^(you must have it installed^)...
- runtime\python.exe infer-web.py --pycmd runtime/python.exe --port 7897 --dml --theme dark
- pause
- cls
- goto menu
-) else if "%choice%"=="2" (
- runtime\python.exe -m pip install scikit-learn-intelex
- cls
- echo Starting Applio with runtime for Intel CPU support ^(you must have Nvidia support installed^)...
- runtime\python.exe -m sklearnex infer-web.py --pycmd runtime/python.exe --port 7897 --theme dark
- pause
- cls
- goto menu
-) else if "%choice%"=="1" (
- cls
- echo Starting Applio with runtime for Nvidia support ^(you must have it installed^)...
- runtime\python.exe infer-web.py --pycmd runtime/python.exe --port 7897 --theme dark
- pause
- cls
- goto menu
-)
-
-cls
-echo Invalid option. Please enter a number from 1 to 5.
-echo.
-echo Press 'Enter' to access the main menu...
-pause>nul
-cls
-goto menu
-:finish
diff --git a/spaces/LaynzKunz/Aesthetic_RVC_Inference_HF/go-tensorboard.bat b/spaces/LaynzKunz/Aesthetic_RVC_Inference_HF/go-tensorboard.bat
deleted file mode 100644
index 631402eac66b7f9c39d803e6a280aa50dd3884b9..0000000000000000000000000000000000000000
--- a/spaces/LaynzKunz/Aesthetic_RVC_Inference_HF/go-tensorboard.bat
+++ /dev/null
@@ -1,5 +0,0 @@
-title Applio - Tensorboard
-cd %~dp0
-cls
-python lib/fixes/tensor-launch.py
-pause
diff --git a/spaces/MLVKU/Human_Object_Interaction/README.md b/spaces/MLVKU/Human_Object_Interaction/README.md
deleted file mode 100644
index d6ac37bd187c96985e9f12343c4c83adf360c7d6..0000000000000000000000000000000000000000
--- a/spaces/MLVKU/Human_Object_Interaction/README.md
+++ /dev/null
@@ -1,142 +0,0 @@
----
-title: HOI detection (HOTR_CPC)
-emoji: ⚡
-colorFrom: indigo
-colorTo: indigo
-sdk: gradio
-sdk_version: 3.3
-app_file: app.py
-pinned: false
-license: apache-2.0
----
-
-# CPC_HOTR
-
-This repository contains the application of [Cross-Path Consistency Learning](https://arxiv.org/abs/2204.04836) at [HOTR](https://arxiv.org/abs/2104.13682), based on the official implementation of HOTR in [here](https://github.com/kakaobrain/HOTR).
-
-
-
-
-
-
-## 1. Environmental Setup
-```bash
-$ conda create -n HOTR_CPC python=3.7
-$ conda install -c pytorch pytorch torchvision # PyTorch 1.7.1, torchvision 0.8.2, CUDA=11.0
-$ conda install cython scipy
-$ pip install pycocotools
-$ pip install opencv-python
-$ pip install wandb
-```
-
-## 2. HOI dataset setup
-Our current version of HOTR supports the experiments for both [V-COCO](https://github.com/s-gupta/v-coco) and [HICO-DET](https://drive.google.com/file/d/1QZcJmGVlF9f4h-XLWe9Gkmnmj2z1gSnk/view) dataset.
-Download the dataset under the pulled directory.
-For HICO-DET, we use the [annotation files](https://drive.google.com/file/d/1QZcJmGVlF9f4h-XLWe9Gkmnmj2z1gSnk/view) provided by the PPDM authors.
-Download the [list of actions](https://drive.google.com/open?id=1EeHNHuYyJI-qqDk_-5nay7Mb07tzZLsl) as `list_action.txt` and place them under the unballed hico-det directory.
-Below we present how you should place the files.
-```bash
-# V-COCO setup
-$ git clone https://github.com/s-gupta/v-coco.git
-$ cd v-coco
-$ ln -s [:COCO_DIR] coco/images # COCO_DIR contains images of train2014 & val2014
-$ python script_pick_annotations.py [:COCO_DIR]/annotations
-
-# HICO-DET setup
-$ tar -zxvf hico_20160224_det.tar.gz # move the unballed folder under the pulled repository
-
-# dataset setup
-HOTR
- │─ v-coco
- │ │─ data
- │ │ │─ instances_vcoco_all_2014.json
- │ │ :
- │ └─ coco
- │ │─ images
- │ │ │─ train2014
- │ │ │ │─ COCO_train2014_000000000009.jpg
- │ │ │ :
- │ │ └─ val2014
- │ │ │─ COCO_val2014_000000000042.jpg
- : : :
- │─ hico_20160224_det
- │ │─ list_action.txt
- │ │─ annotations
- │ │ │─ trainval_hico.json
- │ │ │─ test_hico.json
- │ │ └─ corre_hico.npy
- : :
-```
-
-If you wish to download the datasets on our own directory, simply change the 'data_path' argument to the directory you have downloaded the datasets.
-```bash
---data_path [:your_own_directory]/[v-coco/hico_20160224_det]
-```
-
-## 3. Training
-After the preparation, you can start the training with the following command.
-
-For the HICO-DET training.
-```
-GPUS_PER_NODE=8 ./tools/run_dist_launch.sh 8 ./configs/hico_train.sh
-```
-For the V-COCO training.
-```
-GPUS_PER_NODE=8 ./tools/run_dist_launch.sh 8 ./configs/vcoco_train.sh
-```
-
-## 4. Evaluation
-For evaluation of main inference path P1 (x->HOI), `--path_id` should be set to 0.
-Indexes of Augmented paths are range to 1~3. (1: x->HO->I, 2: x->HI->O, 3: x->OI->H)
-
-HICODET
-```
-python -m torch.distributed.launch \
- --nproc_per_node=8 \
- --use_env main.py \
- --batch_size 2 \
- --HOIDet \
- --path_id 0 \
- --share_enc \
- --pretrained_dec \
- --share_dec_param \
- --num_hoi_queries [:query_num] \
- --object_threshold 0 \
- --temperature 0.2 \ # use the exact same temperature value that you used during training!
- --no_aux_loss \
- --eval \
- --dataset_file hico-det \
- --data_path hico_20160224_det \
- --resume checkpoints/hico_det/hico_[:query_num].pth
-```
-
-VCOCO
-```
-python -m torch.distributed.launch \
- --nproc_per_node=8 \
- --use_env main.py \
- --batch_size 2 \
- --HOIDet \
- --path_id 0 \
- --share_enc \
- --share_dec_param \
- --pretrained_dec \
- --num_hoi_queries [:query_num] \
- --temperature 0.05 \ # use the exact same temperature value that you used during training!
- --object_threshold 0 \
- --no_aux_loss \
- --eval \
- --dataset_file vcoco \
- --data_path v-coco \
- --resume checkpoints/vcoco/vcoco_[:query_num].pth
-```
-
-## Citation
-```
-@inproceedings{park2022consistency,
- title={Consistency Learning via Decoding Path Augmentation for Transformers in Human Object Interaction Detection},
- author={Park, Jihwan and Lee, SeungJun and Heo, Hwan and Choi, Hyeong Kyu and Kim, Hyunwoo J},
- booktitle={Proceedings of the IEEE/CVF Conference on Computer Vision and Pattern Recognition},
- year={2022}
-}
-```
diff --git a/spaces/Make-A-Protagonist/Make-A-Protagonist-inference/Make-A-Protagonist/experts/GroundedSAM/segment_anything/segment_anything/automatic_mask_generator.py b/spaces/Make-A-Protagonist/Make-A-Protagonist-inference/Make-A-Protagonist/experts/GroundedSAM/segment_anything/segment_anything/automatic_mask_generator.py
deleted file mode 100644
index 23264971b7ff5aa0b4f499ade7773b68dce984b6..0000000000000000000000000000000000000000
--- a/spaces/Make-A-Protagonist/Make-A-Protagonist-inference/Make-A-Protagonist/experts/GroundedSAM/segment_anything/segment_anything/automatic_mask_generator.py
+++ /dev/null
@@ -1,372 +0,0 @@
-# Copyright (c) Meta Platforms, Inc. and affiliates.
-# All rights reserved.
-
-# This source code is licensed under the license found in the
-# LICENSE file in the root directory of this source tree.
-
-import numpy as np
-import torch
-from torchvision.ops.boxes import batched_nms, box_area # type: ignore
-
-from typing import Any, Dict, List, Optional, Tuple
-
-from .modeling import Sam
-from .predictor import SamPredictor
-from .utils.amg import (
- MaskData,
- area_from_rle,
- batch_iterator,
- batched_mask_to_box,
- box_xyxy_to_xywh,
- build_all_layer_point_grids,
- calculate_stability_score,
- coco_encode_rle,
- generate_crop_boxes,
- is_box_near_crop_edge,
- mask_to_rle_pytorch,
- remove_small_regions,
- rle_to_mask,
- uncrop_boxes_xyxy,
- uncrop_masks,
- uncrop_points,
-)
-
-
-class SamAutomaticMaskGenerator:
- def __init__(
- self,
- model: Sam,
- points_per_side: Optional[int] = 32,
- points_per_batch: int = 64,
- pred_iou_thresh: float = 0.88,
- stability_score_thresh: float = 0.95,
- stability_score_offset: float = 1.0,
- box_nms_thresh: float = 0.7,
- crop_n_layers: int = 0,
- crop_nms_thresh: float = 0.7,
- crop_overlap_ratio: float = 512 / 1500,
- crop_n_points_downscale_factor: int = 1,
- point_grids: Optional[List[np.ndarray]] = None,
- min_mask_region_area: int = 0,
- output_mode: str = "binary_mask",
- ) -> None:
- """
- Using a SAM model, generates masks for the entire image.
- Generates a grid of point prompts over the image, then filters
- low quality and duplicate masks. The default settings are chosen
- for SAM with a ViT-H backbone.
-
- Arguments:
- model (Sam): The SAM model to use for mask prediction.
- points_per_side (int or None): The number of points to be sampled
- along one side of the image. The total number of points is
- points_per_side**2. If None, 'point_grids' must provide explicit
- point sampling.
- points_per_batch (int): Sets the number of points run simultaneously
- by the model. Higher numbers may be faster but use more GPU memory.
- pred_iou_thresh (float): A filtering threshold in [0,1], using the
- model's predicted mask quality.
- stability_score_thresh (float): A filtering threshold in [0,1], using
- the stability of the mask under changes to the cutoff used to binarize
- the model's mask predictions.
- stability_score_offset (float): The amount to shift the cutoff when
- calculated the stability score.
- box_nms_thresh (float): The box IoU cutoff used by non-maximal
- suppression to filter duplicate masks.
- crops_n_layers (int): If >0, mask prediction will be run again on
- crops of the image. Sets the number of layers to run, where each
- layer has 2**i_layer number of image crops.
- crops_nms_thresh (float): The box IoU cutoff used by non-maximal
- suppression to filter duplicate masks between different crops.
- crop_overlap_ratio (float): Sets the degree to which crops overlap.
- In the first crop layer, crops will overlap by this fraction of
- the image length. Later layers with more crops scale down this overlap.
- crop_n_points_downscale_factor (int): The number of points-per-side
- sampled in layer n is scaled down by crop_n_points_downscale_factor**n.
- point_grids (list(np.ndarray) or None): A list over explicit grids
- of points used for sampling, normalized to [0,1]. The nth grid in the
- list is used in the nth crop layer. Exclusive with points_per_side.
- min_mask_region_area (int): If >0, postprocessing will be applied
- to remove disconnected regions and holes in masks with area smaller
- than min_mask_region_area. Requires opencv.
- output_mode (str): The form masks are returned in. Can be 'binary_mask',
- 'uncompressed_rle', or 'coco_rle'. 'coco_rle' requires pycocotools.
- For large resolutions, 'binary_mask' may consume large amounts of
- memory.
- """
-
- assert (points_per_side is None) != (
- point_grids is None
- ), "Exactly one of points_per_side or point_grid must be provided."
- if points_per_side is not None:
- self.point_grids = build_all_layer_point_grids(
- points_per_side,
- crop_n_layers,
- crop_n_points_downscale_factor,
- )
- elif point_grids is not None:
- self.point_grids = point_grids
- else:
- raise ValueError("Can't have both points_per_side and point_grid be None.")
-
- assert output_mode in [
- "binary_mask",
- "uncompressed_rle",
- "coco_rle",
- ], f"Unknown output_mode {output_mode}."
- if output_mode == "coco_rle":
- from pycocotools import mask as mask_utils # type: ignore # noqa: F401
-
- if min_mask_region_area > 0:
- import cv2 # type: ignore # noqa: F401
-
- self.predictor = SamPredictor(model)
- self.points_per_batch = points_per_batch
- self.pred_iou_thresh = pred_iou_thresh
- self.stability_score_thresh = stability_score_thresh
- self.stability_score_offset = stability_score_offset
- self.box_nms_thresh = box_nms_thresh
- self.crop_n_layers = crop_n_layers
- self.crop_nms_thresh = crop_nms_thresh
- self.crop_overlap_ratio = crop_overlap_ratio
- self.crop_n_points_downscale_factor = crop_n_points_downscale_factor
- self.min_mask_region_area = min_mask_region_area
- self.output_mode = output_mode
-
- @torch.no_grad()
- def generate(self, image: np.ndarray) -> List[Dict[str, Any]]:
- """
- Generates masks for the given image.
-
- Arguments:
- image (np.ndarray): The image to generate masks for, in HWC uint8 format.
-
- Returns:
- list(dict(str, any)): A list over records for masks. Each record is
- a dict containing the following keys:
- segmentation (dict(str, any) or np.ndarray): The mask. If
- output_mode='binary_mask', is an array of shape HW. Otherwise,
- is a dictionary containing the RLE.
- bbox (list(float)): The box around the mask, in XYWH format.
- area (int): The area in pixels of the mask.
- predicted_iou (float): The model's own prediction of the mask's
- quality. This is filtered by the pred_iou_thresh parameter.
- point_coords (list(list(float))): The point coordinates input
- to the model to generate this mask.
- stability_score (float): A measure of the mask's quality. This
- is filtered on using the stability_score_thresh parameter.
- crop_box (list(float)): The crop of the image used to generate
- the mask, given in XYWH format.
- """
-
- # Generate masks
- mask_data = self._generate_masks(image)
-
- # Filter small disconnected regions and holes in masks
- if self.min_mask_region_area > 0:
- mask_data = self.postprocess_small_regions(
- mask_data,
- self.min_mask_region_area,
- max(self.box_nms_thresh, self.crop_nms_thresh),
- )
-
- # Encode masks
- if self.output_mode == "coco_rle":
- mask_data["segmentations"] = [coco_encode_rle(rle) for rle in mask_data["rles"]]
- elif self.output_mode == "binary_mask":
- mask_data["segmentations"] = [rle_to_mask(rle) for rle in mask_data["rles"]]
- else:
- mask_data["segmentations"] = mask_data["rles"]
-
- # Write mask records
- curr_anns = []
- for idx in range(len(mask_data["segmentations"])):
- ann = {
- "segmentation": mask_data["segmentations"][idx],
- "area": area_from_rle(mask_data["rles"][idx]),
- "bbox": box_xyxy_to_xywh(mask_data["boxes"][idx]).tolist(),
- "predicted_iou": mask_data["iou_preds"][idx].item(),
- "point_coords": [mask_data["points"][idx].tolist()],
- "stability_score": mask_data["stability_score"][idx].item(),
- "crop_box": box_xyxy_to_xywh(mask_data["crop_boxes"][idx]).tolist(),
- }
- curr_anns.append(ann)
-
- return curr_anns
-
- def _generate_masks(self, image: np.ndarray) -> MaskData:
- orig_size = image.shape[:2]
- crop_boxes, layer_idxs = generate_crop_boxes(
- orig_size, self.crop_n_layers, self.crop_overlap_ratio
- )
-
- # Iterate over image crops
- data = MaskData()
- for crop_box, layer_idx in zip(crop_boxes, layer_idxs):
- crop_data = self._process_crop(image, crop_box, layer_idx, orig_size)
- data.cat(crop_data)
-
- # Remove duplicate masks between crops
- if len(crop_boxes) > 1:
- # Prefer masks from smaller crops
- scores = 1 / box_area(data["crop_boxes"])
- scores = scores.to(data["boxes"].device)
- keep_by_nms = batched_nms(
- data["boxes"].float(),
- scores,
- torch.zeros(len(data["boxes"])), # categories
- iou_threshold=self.crop_nms_thresh,
- )
- data.filter(keep_by_nms)
-
- data.to_numpy()
- return data
-
- def _process_crop(
- self,
- image: np.ndarray,
- crop_box: List[int],
- crop_layer_idx: int,
- orig_size: Tuple[int, ...],
- ) -> MaskData:
- # Crop the image and calculate embeddings
- x0, y0, x1, y1 = crop_box
- cropped_im = image[y0:y1, x0:x1, :]
- cropped_im_size = cropped_im.shape[:2]
- self.predictor.set_image(cropped_im)
-
- # Get points for this crop
- points_scale = np.array(cropped_im_size)[None, ::-1]
- points_for_image = self.point_grids[crop_layer_idx] * points_scale
-
- # Generate masks for this crop in batches
- data = MaskData()
- for (points,) in batch_iterator(self.points_per_batch, points_for_image):
- batch_data = self._process_batch(points, cropped_im_size, crop_box, orig_size)
- data.cat(batch_data)
- del batch_data
- self.predictor.reset_image()
-
- # Remove duplicates within this crop.
- keep_by_nms = batched_nms(
- data["boxes"].float(),
- data["iou_preds"],
- torch.zeros(len(data["boxes"])), # categories
- iou_threshold=self.box_nms_thresh,
- )
- data.filter(keep_by_nms)
-
- # Return to the original image frame
- data["boxes"] = uncrop_boxes_xyxy(data["boxes"], crop_box)
- data["points"] = uncrop_points(data["points"], crop_box)
- data["crop_boxes"] = torch.tensor([crop_box for _ in range(len(data["rles"]))])
-
- return data
-
- def _process_batch(
- self,
- points: np.ndarray,
- im_size: Tuple[int, ...],
- crop_box: List[int],
- orig_size: Tuple[int, ...],
- ) -> MaskData:
- orig_h, orig_w = orig_size
-
- # Run model on this batch
- transformed_points = self.predictor.transform.apply_coords(points, im_size)
- in_points = torch.as_tensor(transformed_points, device=self.predictor.device)
- in_labels = torch.ones(in_points.shape[0], dtype=torch.int, device=in_points.device)
- masks, iou_preds, _ = self.predictor.predict_torch(
- in_points[:, None, :],
- in_labels[:, None],
- multimask_output=True,
- return_logits=True,
- )
-
- # Serialize predictions and store in MaskData
- data = MaskData(
- masks=masks.flatten(0, 1),
- iou_preds=iou_preds.flatten(0, 1),
- points=torch.as_tensor(points.repeat(masks.shape[1], axis=0)),
- )
- del masks
-
- # Filter by predicted IoU
- if self.pred_iou_thresh > 0.0:
- keep_mask = data["iou_preds"] > self.pred_iou_thresh
- data.filter(keep_mask)
-
- # Calculate stability score
- data["stability_score"] = calculate_stability_score(
- data["masks"], self.predictor.model.mask_threshold, self.stability_score_offset
- )
- if self.stability_score_thresh > 0.0:
- keep_mask = data["stability_score"] >= self.stability_score_thresh
- data.filter(keep_mask)
-
- # Threshold masks and calculate boxes
- data["masks"] = data["masks"] > self.predictor.model.mask_threshold
- data["boxes"] = batched_mask_to_box(data["masks"])
-
- # Filter boxes that touch crop boundaries
- keep_mask = ~is_box_near_crop_edge(data["boxes"], crop_box, [0, 0, orig_w, orig_h])
- if not torch.all(keep_mask):
- data.filter(keep_mask)
-
- # Compress to RLE
- data["masks"] = uncrop_masks(data["masks"], crop_box, orig_h, orig_w)
- data["rles"] = mask_to_rle_pytorch(data["masks"])
- del data["masks"]
-
- return data
-
- @staticmethod
- def postprocess_small_regions(
- mask_data: MaskData, min_area: int, nms_thresh: float
- ) -> MaskData:
- """
- Removes small disconnected regions and holes in masks, then reruns
- box NMS to remove any new duplicates.
-
- Edits mask_data in place.
-
- Requires open-cv as a dependency.
- """
- if len(mask_data["rles"]) == 0:
- return mask_data
-
- # Filter small disconnected regions and holes
- new_masks = []
- scores = []
- for rle in mask_data["rles"]:
- mask = rle_to_mask(rle)
-
- mask, changed = remove_small_regions(mask, min_area, mode="holes")
- unchanged = not changed
- mask, changed = remove_small_regions(mask, min_area, mode="islands")
- unchanged = unchanged and not changed
-
- new_masks.append(torch.as_tensor(mask).unsqueeze(0))
- # Give score=0 to changed masks and score=1 to unchanged masks
- # so NMS will prefer ones that didn't need postprocessing
- scores.append(float(unchanged))
-
- # Recalculate boxes and remove any new duplicates
- masks = torch.cat(new_masks, dim=0)
- boxes = batched_mask_to_box(masks)
- keep_by_nms = batched_nms(
- boxes.float(),
- torch.as_tensor(scores),
- torch.zeros(len(boxes)), # categories
- iou_threshold=nms_thresh,
- )
-
- # Only recalculate RLEs for masks that have changed
- for i_mask in keep_by_nms:
- if scores[i_mask] == 0.0:
- mask_torch = masks[i_mask].unsqueeze(0)
- mask_data["rles"][i_mask] = mask_to_rle_pytorch(mask_torch)[0]
- mask_data["boxes"][i_mask] = boxes[i_mask] # update res directly
- mask_data.filter(keep_by_nms)
-
- return mask_data
diff --git a/spaces/MakiAi/Image2VideoProcessingPipelin/modules/Transition/SimpleVideoMerger.py b/spaces/MakiAi/Image2VideoProcessingPipelin/modules/Transition/SimpleVideoMerger.py
deleted file mode 100644
index e3b9fc8185b3484cea9ef0e41ee7fc442c08b35c..0000000000000000000000000000000000000000
--- a/spaces/MakiAi/Image2VideoProcessingPipelin/modules/Transition/SimpleVideoMerger.py
+++ /dev/null
@@ -1,52 +0,0 @@
-from PIL import Image, ImageFilter
-import random
-import os
-from pathlib import Path
-import cv2
-import numpy as np
-
-
-class SimpleVideoMerger:
- def __init__(self, fps: int = 30):
- self.fps = fps
-
- def merge_videos(self, input_folder: str, output_filename: str):
- video_files = [f for f in Path(input_folder).glob("*.mp4")]
-
- if not video_files:
- print("No video files found in the specified directory.")
- return
-
- videos = []
-
- for video_file in video_files:
- video = cv2.VideoCapture(str(video_file))
- videos.append(video)
-
- width = int(videos[0].get(cv2.CAP_PROP_FRAME_WIDTH))
- height = int(videos[0].get(cv2.CAP_PROP_FRAME_HEIGHT))
-
- fourcc = cv2.VideoWriter_fourcc(*'MP4V')
- out = cv2.VideoWriter(output_filename, fourcc, self.fps, (width, height))
-
- for i, video in enumerate(videos):
- ret, frame = video.read()
-
- while ret:
- out.write(frame)
- ret, frame = video.read()
-
- video.release()
-
- out.release()
-
- print(f"Concatenated video saved to {output_filename}.")
-
-if __name__ == '__main__':
- # 使用例 (コメントアウトされています)
- merger = SimpleVideoMerger()
- input_folder_path = r"image\Echoes-of-Creation_Blurred_mov"
- output_folder_path = f"{input_folder_path}_Final"
- os.makedirs(output_folder_path, exist_ok=True)
- output_video_path = os.path.join(output_folder_path, "concatenated_video.mp4")
- merger.merge_videos(input_folder_path, output_video_path)
\ No newline at end of file
diff --git a/spaces/Malolactica/amigosdejuegos/Dockerfile b/spaces/Malolactica/amigosdejuegos/Dockerfile
deleted file mode 100644
index eef259fa372a804549fb0af0913718a13344da34..0000000000000000000000000000000000000000
--- a/spaces/Malolactica/amigosdejuegos/Dockerfile
+++ /dev/null
@@ -1,11 +0,0 @@
-FROM node:18-bullseye-slim
-RUN apt-get update && \
- apt-get install -y git
-RUN git clone https://gitgud.io/khanon/oai-reverse-proxy.git /app
-WORKDIR /app
-RUN npm install
-COPY Dockerfile greeting.md* .env* ./
-RUN npm run build
-EXPOSE 7860
-ENV NODE_ENV=production
-CMD [ "npm", "start" ]
diff --git a/spaces/MathysL/AutoGPT4/tests/unit/test_chat.py b/spaces/MathysL/AutoGPT4/tests/unit/test_chat.py
deleted file mode 100644
index 774f4103762c28d5a02e89c14b224fae0bc0756a..0000000000000000000000000000000000000000
--- a/spaces/MathysL/AutoGPT4/tests/unit/test_chat.py
+++ /dev/null
@@ -1,86 +0,0 @@
-# Generated by CodiumAI
-import time
-import unittest
-from unittest.mock import patch
-
-from autogpt.chat import create_chat_message, generate_context
-
-
-class TestChat(unittest.TestCase):
- # Tests that the function returns a dictionary with the correct keys and values when valid strings are provided for role and content.
- def test_happy_path_role_content(self):
- result = create_chat_message("system", "Hello, world!")
- self.assertEqual(result, {"role": "system", "content": "Hello, world!"})
-
- # Tests that the function returns a dictionary with the correct keys and values when empty strings are provided for role and content.
- def test_empty_role_content(self):
- result = create_chat_message("", "")
- self.assertEqual(result, {"role": "", "content": ""})
-
- # Tests the behavior of the generate_context function when all input parameters are empty.
- @patch("time.strftime")
- def test_generate_context_empty_inputs(self, mock_strftime):
- # Mock the time.strftime function to return a fixed value
- mock_strftime.return_value = "Sat Apr 15 00:00:00 2023"
- # Arrange
- prompt = ""
- relevant_memory = ""
- full_message_history = []
- model = "gpt-3.5-turbo-0301"
-
- # Act
- result = generate_context(prompt, relevant_memory, full_message_history, model)
-
- # Assert
- expected_result = (
- -1,
- 47,
- 3,
- [
- {"role": "system", "content": ""},
- {
- "role": "system",
- "content": f"The current time and date is {time.strftime('%c')}",
- },
- {
- "role": "system",
- "content": f"This reminds you of these events from your past:\n\n\n",
- },
- ],
- )
- self.assertEqual(result, expected_result)
-
- # Tests that the function successfully generates a current_context given valid inputs.
- def test_generate_context_valid_inputs(self):
- # Given
- prompt = "What is your favorite color?"
- relevant_memory = "You once painted your room blue."
- full_message_history = [
- create_chat_message("user", "Hi there!"),
- create_chat_message("assistant", "Hello! How can I assist you today?"),
- create_chat_message("user", "Can you tell me a joke?"),
- create_chat_message(
- "assistant",
- "Why did the tomato turn red? Because it saw the salad dressing!",
- ),
- create_chat_message("user", "Haha, that's funny."),
- ]
- model = "gpt-3.5-turbo-0301"
-
- # When
- result = generate_context(prompt, relevant_memory, full_message_history, model)
-
- # Then
- self.assertIsInstance(result[0], int)
- self.assertIsInstance(result[1], int)
- self.assertIsInstance(result[2], int)
- self.assertIsInstance(result[3], list)
- self.assertGreaterEqual(result[0], 0)
- self.assertGreaterEqual(result[1], 0)
- self.assertGreaterEqual(result[2], 0)
- self.assertGreaterEqual(
- len(result[3]), 3
- ) # current_context should have at least 3 messages
- self.assertLessEqual(
- result[1], 2048
- ) # token limit for GPT-3.5-turbo-0301 is 2048 tokens
diff --git a/spaces/Mellow-ai/PhotoAI_Mellow/annotator/uniformer/mmcv/ops/points_in_boxes.py b/spaces/Mellow-ai/PhotoAI_Mellow/annotator/uniformer/mmcv/ops/points_in_boxes.py
deleted file mode 100644
index 4003173a53052161dbcd687a2fa1d755642fdab8..0000000000000000000000000000000000000000
--- a/spaces/Mellow-ai/PhotoAI_Mellow/annotator/uniformer/mmcv/ops/points_in_boxes.py
+++ /dev/null
@@ -1,133 +0,0 @@
-import torch
-
-from ..utils import ext_loader
-
-ext_module = ext_loader.load_ext('_ext', [
- 'points_in_boxes_part_forward', 'points_in_boxes_cpu_forward',
- 'points_in_boxes_all_forward'
-])
-
-
-def points_in_boxes_part(points, boxes):
- """Find the box in which each point is (CUDA).
-
- Args:
- points (torch.Tensor): [B, M, 3], [x, y, z] in LiDAR/DEPTH coordinate
- boxes (torch.Tensor): [B, T, 7],
- num_valid_boxes <= T, [x, y, z, x_size, y_size, z_size, rz] in
- LiDAR/DEPTH coordinate, (x, y, z) is the bottom center
-
- Returns:
- box_idxs_of_pts (torch.Tensor): (B, M), default background = -1
- """
- assert points.shape[0] == boxes.shape[0], \
- 'Points and boxes should have the same batch size, ' \
- f'but got {points.shape[0]} and {boxes.shape[0]}'
- assert boxes.shape[2] == 7, \
- 'boxes dimension should be 7, ' \
- f'but got unexpected shape {boxes.shape[2]}'
- assert points.shape[2] == 3, \
- 'points dimension should be 3, ' \
- f'but got unexpected shape {points.shape[2]}'
- batch_size, num_points, _ = points.shape
-
- box_idxs_of_pts = points.new_zeros((batch_size, num_points),
- dtype=torch.int).fill_(-1)
-
- # If manually put the tensor 'points' or 'boxes' on a device
- # which is not the current device, some temporary variables
- # will be created on the current device in the cuda op,
- # and the output will be incorrect.
- # Therefore, we force the current device to be the same
- # as the device of the tensors if it was not.
- # Please refer to https://github.com/open-mmlab/mmdetection3d/issues/305
- # for the incorrect output before the fix.
- points_device = points.get_device()
- assert points_device == boxes.get_device(), \
- 'Points and boxes should be put on the same device'
- if torch.cuda.current_device() != points_device:
- torch.cuda.set_device(points_device)
-
- ext_module.points_in_boxes_part_forward(boxes.contiguous(),
- points.contiguous(),
- box_idxs_of_pts)
-
- return box_idxs_of_pts
-
-
-def points_in_boxes_cpu(points, boxes):
- """Find all boxes in which each point is (CPU). The CPU version of
- :meth:`points_in_boxes_all`.
-
- Args:
- points (torch.Tensor): [B, M, 3], [x, y, z] in
- LiDAR/DEPTH coordinate
- boxes (torch.Tensor): [B, T, 7],
- num_valid_boxes <= T, [x, y, z, x_size, y_size, z_size, rz],
- (x, y, z) is the bottom center.
-
- Returns:
- box_idxs_of_pts (torch.Tensor): (B, M, T), default background = 0.
- """
- assert points.shape[0] == boxes.shape[0], \
- 'Points and boxes should have the same batch size, ' \
- f'but got {points.shape[0]} and {boxes.shape[0]}'
- assert boxes.shape[2] == 7, \
- 'boxes dimension should be 7, ' \
- f'but got unexpected shape {boxes.shape[2]}'
- assert points.shape[2] == 3, \
- 'points dimension should be 3, ' \
- f'but got unexpected shape {points.shape[2]}'
- batch_size, num_points, _ = points.shape
- num_boxes = boxes.shape[1]
-
- point_indices = points.new_zeros((batch_size, num_boxes, num_points),
- dtype=torch.int)
- for b in range(batch_size):
- ext_module.points_in_boxes_cpu_forward(boxes[b].float().contiguous(),
- points[b].float().contiguous(),
- point_indices[b])
- point_indices = point_indices.transpose(1, 2)
-
- return point_indices
-
-
-def points_in_boxes_all(points, boxes):
- """Find all boxes in which each point is (CUDA).
-
- Args:
- points (torch.Tensor): [B, M, 3], [x, y, z] in LiDAR/DEPTH coordinate
- boxes (torch.Tensor): [B, T, 7],
- num_valid_boxes <= T, [x, y, z, x_size, y_size, z_size, rz],
- (x, y, z) is the bottom center.
-
- Returns:
- box_idxs_of_pts (torch.Tensor): (B, M, T), default background = 0.
- """
- assert boxes.shape[0] == points.shape[0], \
- 'Points and boxes should have the same batch size, ' \
- f'but got {boxes.shape[0]} and {boxes.shape[0]}'
- assert boxes.shape[2] == 7, \
- 'boxes dimension should be 7, ' \
- f'but got unexpected shape {boxes.shape[2]}'
- assert points.shape[2] == 3, \
- 'points dimension should be 3, ' \
- f'but got unexpected shape {points.shape[2]}'
- batch_size, num_points, _ = points.shape
- num_boxes = boxes.shape[1]
-
- box_idxs_of_pts = points.new_zeros((batch_size, num_points, num_boxes),
- dtype=torch.int).fill_(0)
-
- # Same reason as line 25-32
- points_device = points.get_device()
- assert points_device == boxes.get_device(), \
- 'Points and boxes should be put on the same device'
- if torch.cuda.current_device() != points_device:
- torch.cuda.set_device(points_device)
-
- ext_module.points_in_boxes_all_forward(boxes.contiguous(),
- points.contiguous(),
- box_idxs_of_pts)
-
- return box_idxs_of_pts
diff --git a/spaces/Mellow-ai/PhotoAI_Mellow/annotator/uniformer/mmseg/datasets/pipelines/formating.py b/spaces/Mellow-ai/PhotoAI_Mellow/annotator/uniformer/mmseg/datasets/pipelines/formating.py
deleted file mode 100644
index 97db85f4f9db39fb86ba77ead7d1a8407d810adb..0000000000000000000000000000000000000000
--- a/spaces/Mellow-ai/PhotoAI_Mellow/annotator/uniformer/mmseg/datasets/pipelines/formating.py
+++ /dev/null
@@ -1,288 +0,0 @@
-from collections.abc import Sequence
-
-import annotator.uniformer.mmcv as mmcv
-import numpy as np
-import torch
-from annotator.uniformer.mmcv.parallel import DataContainer as DC
-
-from ..builder import PIPELINES
-
-
-def to_tensor(data):
- """Convert objects of various python types to :obj:`torch.Tensor`.
-
- Supported types are: :class:`numpy.ndarray`, :class:`torch.Tensor`,
- :class:`Sequence`, :class:`int` and :class:`float`.
-
- Args:
- data (torch.Tensor | numpy.ndarray | Sequence | int | float): Data to
- be converted.
- """
-
- if isinstance(data, torch.Tensor):
- return data
- elif isinstance(data, np.ndarray):
- return torch.from_numpy(data)
- elif isinstance(data, Sequence) and not mmcv.is_str(data):
- return torch.tensor(data)
- elif isinstance(data, int):
- return torch.LongTensor([data])
- elif isinstance(data, float):
- return torch.FloatTensor([data])
- else:
- raise TypeError(f'type {type(data)} cannot be converted to tensor.')
-
-
-@PIPELINES.register_module()
-class ToTensor(object):
- """Convert some results to :obj:`torch.Tensor` by given keys.
-
- Args:
- keys (Sequence[str]): Keys that need to be converted to Tensor.
- """
-
- def __init__(self, keys):
- self.keys = keys
-
- def __call__(self, results):
- """Call function to convert data in results to :obj:`torch.Tensor`.
-
- Args:
- results (dict): Result dict contains the data to convert.
-
- Returns:
- dict: The result dict contains the data converted
- to :obj:`torch.Tensor`.
- """
-
- for key in self.keys:
- results[key] = to_tensor(results[key])
- return results
-
- def __repr__(self):
- return self.__class__.__name__ + f'(keys={self.keys})'
-
-
-@PIPELINES.register_module()
-class ImageToTensor(object):
- """Convert image to :obj:`torch.Tensor` by given keys.
-
- The dimension order of input image is (H, W, C). The pipeline will convert
- it to (C, H, W). If only 2 dimension (H, W) is given, the output would be
- (1, H, W).
-
- Args:
- keys (Sequence[str]): Key of images to be converted to Tensor.
- """
-
- def __init__(self, keys):
- self.keys = keys
-
- def __call__(self, results):
- """Call function to convert image in results to :obj:`torch.Tensor` and
- transpose the channel order.
-
- Args:
- results (dict): Result dict contains the image data to convert.
-
- Returns:
- dict: The result dict contains the image converted
- to :obj:`torch.Tensor` and transposed to (C, H, W) order.
- """
-
- for key in self.keys:
- img = results[key]
- if len(img.shape) < 3:
- img = np.expand_dims(img, -1)
- results[key] = to_tensor(img.transpose(2, 0, 1))
- return results
-
- def __repr__(self):
- return self.__class__.__name__ + f'(keys={self.keys})'
-
-
-@PIPELINES.register_module()
-class Transpose(object):
- """Transpose some results by given keys.
-
- Args:
- keys (Sequence[str]): Keys of results to be transposed.
- order (Sequence[int]): Order of transpose.
- """
-
- def __init__(self, keys, order):
- self.keys = keys
- self.order = order
-
- def __call__(self, results):
- """Call function to convert image in results to :obj:`torch.Tensor` and
- transpose the channel order.
-
- Args:
- results (dict): Result dict contains the image data to convert.
-
- Returns:
- dict: The result dict contains the image converted
- to :obj:`torch.Tensor` and transposed to (C, H, W) order.
- """
-
- for key in self.keys:
- results[key] = results[key].transpose(self.order)
- return results
-
- def __repr__(self):
- return self.__class__.__name__ + \
- f'(keys={self.keys}, order={self.order})'
-
-
-@PIPELINES.register_module()
-class ToDataContainer(object):
- """Convert results to :obj:`mmcv.DataContainer` by given fields.
-
- Args:
- fields (Sequence[dict]): Each field is a dict like
- ``dict(key='xxx', **kwargs)``. The ``key`` in result will
- be converted to :obj:`mmcv.DataContainer` with ``**kwargs``.
- Default: ``(dict(key='img', stack=True),
- dict(key='gt_semantic_seg'))``.
- """
-
- def __init__(self,
- fields=(dict(key='img',
- stack=True), dict(key='gt_semantic_seg'))):
- self.fields = fields
-
- def __call__(self, results):
- """Call function to convert data in results to
- :obj:`mmcv.DataContainer`.
-
- Args:
- results (dict): Result dict contains the data to convert.
-
- Returns:
- dict: The result dict contains the data converted to
- :obj:`mmcv.DataContainer`.
- """
-
- for field in self.fields:
- field = field.copy()
- key = field.pop('key')
- results[key] = DC(results[key], **field)
- return results
-
- def __repr__(self):
- return self.__class__.__name__ + f'(fields={self.fields})'
-
-
-@PIPELINES.register_module()
-class DefaultFormatBundle(object):
- """Default formatting bundle.
-
- It simplifies the pipeline of formatting common fields, including "img"
- and "gt_semantic_seg". These fields are formatted as follows.
-
- - img: (1)transpose, (2)to tensor, (3)to DataContainer (stack=True)
- - gt_semantic_seg: (1)unsqueeze dim-0 (2)to tensor,
- (3)to DataContainer (stack=True)
- """
-
- def __call__(self, results):
- """Call function to transform and format common fields in results.
-
- Args:
- results (dict): Result dict contains the data to convert.
-
- Returns:
- dict: The result dict contains the data that is formatted with
- default bundle.
- """
-
- if 'img' in results:
- img = results['img']
- if len(img.shape) < 3:
- img = np.expand_dims(img, -1)
- img = np.ascontiguousarray(img.transpose(2, 0, 1))
- results['img'] = DC(to_tensor(img), stack=True)
- if 'gt_semantic_seg' in results:
- # convert to long
- results['gt_semantic_seg'] = DC(
- to_tensor(results['gt_semantic_seg'][None,
- ...].astype(np.int64)),
- stack=True)
- return results
-
- def __repr__(self):
- return self.__class__.__name__
-
-
-@PIPELINES.register_module()
-class Collect(object):
- """Collect data from the loader relevant to the specific task.
-
- This is usually the last stage of the data loader pipeline. Typically keys
- is set to some subset of "img", "gt_semantic_seg".
-
- The "img_meta" item is always populated. The contents of the "img_meta"
- dictionary depends on "meta_keys". By default this includes:
-
- - "img_shape": shape of the image input to the network as a tuple
- (h, w, c). Note that images may be zero padded on the bottom/right
- if the batch tensor is larger than this shape.
-
- - "scale_factor": a float indicating the preprocessing scale
-
- - "flip": a boolean indicating if image flip transform was used
-
- - "filename": path to the image file
-
- - "ori_shape": original shape of the image as a tuple (h, w, c)
-
- - "pad_shape": image shape after padding
-
- - "img_norm_cfg": a dict of normalization information:
- - mean - per channel mean subtraction
- - std - per channel std divisor
- - to_rgb - bool indicating if bgr was converted to rgb
-
- Args:
- keys (Sequence[str]): Keys of results to be collected in ``data``.
- meta_keys (Sequence[str], optional): Meta keys to be converted to
- ``mmcv.DataContainer`` and collected in ``data[img_metas]``.
- Default: ``('filename', 'ori_filename', 'ori_shape', 'img_shape',
- 'pad_shape', 'scale_factor', 'flip', 'flip_direction',
- 'img_norm_cfg')``
- """
-
- def __init__(self,
- keys,
- meta_keys=('filename', 'ori_filename', 'ori_shape',
- 'img_shape', 'pad_shape', 'scale_factor', 'flip',
- 'flip_direction', 'img_norm_cfg')):
- self.keys = keys
- self.meta_keys = meta_keys
-
- def __call__(self, results):
- """Call function to collect keys in results. The keys in ``meta_keys``
- will be converted to :obj:mmcv.DataContainer.
-
- Args:
- results (dict): Result dict contains the data to collect.
-
- Returns:
- dict: The result dict contains the following keys
- - keys in``self.keys``
- - ``img_metas``
- """
-
- data = {}
- img_meta = {}
- for key in self.meta_keys:
- img_meta[key] = results[key]
- data['img_metas'] = DC(img_meta, cpu_only=True)
- for key in self.keys:
- data[key] = results[key]
- return data
-
- def __repr__(self):
- return self.__class__.__name__ + \
- f'(keys={self.keys}, meta_keys={self.meta_keys})'
diff --git a/spaces/Mountchicken/MAERec-Gradio/tools/dataset_converters/textdet/kaist_converter.py b/spaces/Mountchicken/MAERec-Gradio/tools/dataset_converters/textdet/kaist_converter.py
deleted file mode 100644
index 3f95804d1dda27a88db247e177c3f7522361faf5..0000000000000000000000000000000000000000
--- a/spaces/Mountchicken/MAERec-Gradio/tools/dataset_converters/textdet/kaist_converter.py
+++ /dev/null
@@ -1,201 +0,0 @@
-# Copyright (c) OpenMMLab. All rights reserved.
-import argparse
-import math
-import os
-import os.path as osp
-import xml.etree.ElementTree as ET
-
-import mmcv
-import mmengine
-
-from mmocr.utils import dump_ocr_data
-
-
-def collect_files(img_dir, gt_dir, ratio):
- """Collect all images and their corresponding groundtruth files.
-
- Args:
- img_dir (str): The image directory
- gt_dir (str): The groundtruth directory
- ratio (float): Split ratio for val set
-
- Returns:
- files (list): The list of tuples (img_file, groundtruth_file)
- """
- assert isinstance(img_dir, str)
- assert img_dir
- assert isinstance(gt_dir, str)
- assert gt_dir
- assert isinstance(ratio, float)
- assert ratio < 1.0, 'val_ratio should be a float between 0.0 to 1.0'
-
- ann_list, imgs_list = [], []
- for img_file in os.listdir(img_dir):
- ann_list.append(osp.join(gt_dir, img_file.split('.')[0] + '.xml'))
- imgs_list.append(osp.join(img_dir, img_file))
-
- all_files = list(zip(sorted(imgs_list), sorted(ann_list)))
- assert len(all_files), f'No images found in {img_dir}'
- print(f'Loaded {len(all_files)} images from {img_dir}')
-
- trn_files, val_files = [], []
- if ratio > 0:
- for i, file in enumerate(all_files):
- if i % math.floor(1 / ratio):
- trn_files.append(file)
- else:
- val_files.append(file)
- else:
- trn_files, val_files = all_files, []
-
- print(f'training #{len(trn_files)}, val #{len(val_files)}')
-
- return trn_files, val_files
-
-
-def collect_annotations(files, nproc=1):
- """Collect the annotation information.
-
- Args:
- files (list): The list of tuples (image_file, groundtruth_file)
- nproc (int): The number of process to collect annotations
-
- Returns:
- images (list): The list of image information dicts
- """
- assert isinstance(files, list)
- assert isinstance(nproc, int)
-
- if nproc > 1:
- images = mmengine.track_parallel_progress(
- load_img_info, files, nproc=nproc)
- else:
- images = mmengine.track_progress(load_img_info, files)
-
- return images
-
-
-def load_img_info(files):
- """Load the information of one image.
-
- Args:
- files (tuple): The tuple of (img_file, groundtruth_file)
-
- Returns:
- img_info (dict): The dict of the img and annotation information
- """
- assert isinstance(files, tuple)
-
- img_file, gt_file = files
- assert osp.basename(gt_file).split('.')[0] == osp.basename(img_file).split(
- '.')[0]
- # read imgs while ignoring orientations
- img = mmcv.imread(img_file, 'unchanged')
-
- img_info = dict(
- file_name=osp.join(osp.basename(img_file)),
- height=img.shape[0],
- width=img.shape[1],
- segm_file=osp.join(osp.basename(gt_file)))
-
- if osp.splitext(gt_file)[1] == '.xml':
- img_info = load_xml_info(gt_file, img_info)
- else:
- raise NotImplementedError
-
- return img_info
-
-
-def load_xml_info(gt_file, img_info):
- """Collect the annotation information.
-
- Annotation Format
-
- DSC02306.JPG
-
-
-
-
-
-
-
-
-
-
-
-
- no
- 2
-
-
-
-
- Args:
- gt_file (str): The path to ground-truth
- img_info (dict): The dict of the img and annotation information
-
- Returns:
- img_info (dict): The dict of the img and annotation information
- """
-
- obj = ET.parse(gt_file)
- root = obj.getroot()
- anno_info = []
- for word in root.iter('word'):
- x, y = max(0, int(word.attrib['x'])), max(0, int(word.attrib['y']))
- w, h = int(word.attrib['width']), int(word.attrib['height'])
- bbox = [x, y, w, h]
- segmentation = [x, y, x + w, y, x + w, y + h, x, y + h]
-
- anno = dict(
- iscrowd=0,
- category_id=1,
- bbox=bbox,
- area=w * h,
- segmentation=[segmentation])
- anno_info.append(anno)
-
- img_info.update(anno_info=anno_info)
-
- return img_info
-
-
-def parse_args():
- parser = argparse.ArgumentParser(
- description='Generate training and val set of KAIST ')
- parser.add_argument('root_path', help='Root dir path of KAIST')
- parser.add_argument(
- '--val-ratio', help='Split ratio for val set', default=0.0, type=float)
- parser.add_argument(
- '--nproc', default=1, type=int, help='Number of process')
- args = parser.parse_args()
- return args
-
-
-def main():
- args = parse_args()
- root_path = args.root_path
- ratio = args.val_ratio
-
- trn_files, val_files = collect_files(
- osp.join(root_path, 'imgs'), osp.join(root_path, 'annotations'), ratio)
-
- # Train set
- trn_infos = collect_annotations(trn_files, nproc=args.nproc)
- with mmengine.Timer(
- print_tmpl='It takes {}s to convert KAIST Training annotation'):
- dump_ocr_data(trn_infos, osp.join(root_path,
- 'instances_training.json'),
- 'textdet')
-
- # Val set
- if len(val_files) > 0:
- val_infos = collect_annotations(val_files, nproc=args.nproc)
- with mmengine.Timer(
- print_tmpl='It takes {}s to convert KAIST Val annotation'):
- dump_ocr_data(val_infos, osp.join(root_path, 'instances_val.json'),
- 'textdet')
-
-
-if __name__ == '__main__':
- main()
diff --git a/spaces/NCTCMumbai/NCTC/models/official/nlp/modeling/layers/multi_channel_attention_test.py b/spaces/NCTCMumbai/NCTC/models/official/nlp/modeling/layers/multi_channel_attention_test.py
deleted file mode 100644
index ab6e0e7fec48635d09e6e30c3ad247044ae9785f..0000000000000000000000000000000000000000
--- a/spaces/NCTCMumbai/NCTC/models/official/nlp/modeling/layers/multi_channel_attention_test.py
+++ /dev/null
@@ -1,56 +0,0 @@
-# Lint as: python3
-# Copyright 2020 The TensorFlow Authors. All Rights Reserved.
-#
-# Licensed under the Apache License, Version 2.0 (the "License");
-# you may not use this file except in compliance with the License.
-# You may obtain a copy of the License at
-#
-# http://www.apache.org/licenses/LICENSE-2.0
-#
-# Unless required by applicable law or agreed to in writing, software
-# distributed under the License is distributed on an "AS IS" BASIS,
-# WITHOUT WARRANTIES OR CONDITIONS OF ANY KIND, either express or implied.
-# See the License for the specific language governing permissions and
-# limitations under the License.
-# ==============================================================================
-"""Tests for nlp.nhnet.multi_channel_attention."""
-
-from __future__ import absolute_import
-from __future__ import division
-from __future__ import print_function
-
-import numpy as np
-import tensorflow as tf
-
-from official.nlp.modeling.layers import multi_channel_attention
-
-
-class MultiChannelAttentionTest(tf.test.TestCase):
-
- def test_doc_attention(self):
- num_heads = 2
- doc_attention = multi_channel_attention.VotingAttention(
- num_heads, head_size=8)
- num_docs = 3
- inputs = np.zeros((2, num_docs, 10, 16), dtype=np.float32)
- doc_mask = np.zeros((2, num_docs), dtype=np.float32)
- outputs = doc_attention(inputs, doc_mask)
- self.assertEqual(outputs.shape, (2, num_docs))
-
- def test_multi_channel_attention(self):
- num_heads = 2
- num_docs = 5
- attention_layer = multi_channel_attention.MultiChannelAttention(
- num_heads, key_size=2)
-
- from_data = 10 * np.random.random_sample((3, 4, 8))
- to_data = 10 * np.random.random_sample((3, num_docs, 2, 8))
- mask_data = np.random.randint(2, size=(3, num_docs, 4, 2))
- doc_probs = np.random.randint(
- 2, size=(3, num_heads, 4, num_docs)).astype(float)
- outputs = attention_layer([from_data, to_data, doc_probs], mask_data)
- self.assertEqual(outputs.shape, (3, 4, 8))
-
-
-if __name__ == "__main__":
- tf.test.main()
diff --git a/spaces/NCTCMumbai/NCTC/models/official/recommendation/stat_utils.py b/spaces/NCTCMumbai/NCTC/models/official/recommendation/stat_utils.py
deleted file mode 100644
index 658a2721e98a88d71dc2ac4562366283ffd2fc47..0000000000000000000000000000000000000000
--- a/spaces/NCTCMumbai/NCTC/models/official/recommendation/stat_utils.py
+++ /dev/null
@@ -1,92 +0,0 @@
-# Copyright 2018 The TensorFlow Authors. All Rights Reserved.
-#
-# Licensed under the Apache License, Version 2.0 (the "License");
-# you may not use this file except in compliance with the License.
-# You may obtain a copy of the License at
-#
-# http://www.apache.org/licenses/LICENSE-2.0
-#
-# Unless required by applicable law or agreed to in writing, software
-# distributed under the License is distributed on an "AS IS" BASIS,
-# WITHOUT WARRANTIES OR CONDITIONS OF ANY KIND, either express or implied.
-# See the License for the specific language governing permissions and
-# limitations under the License.
-# ==============================================================================
-"""Statistics utility functions of NCF."""
-
-from __future__ import absolute_import
-from __future__ import division
-from __future__ import print_function
-
-import os
-
-import numpy as np
-
-
-def random_int32():
- return np.random.randint(low=0, high=np.iinfo(np.int32).max, dtype=np.int32)
-
-
-def permutation(args):
- """Fork safe permutation function.
-
- This function can be called within a multiprocessing worker and give
- appropriately random results.
-
- Args:
- args: A size two tuple that will unpacked into the size of the permutation
- and the random seed. This form is used because starmap is not universally
- available.
-
- returns:
- A NumPy array containing a random permutation.
- """
- x, seed = args
-
- # If seed is None NumPy will seed randomly.
- state = np.random.RandomState(seed=seed) # pylint: disable=no-member
- output = np.arange(x, dtype=np.int32)
- state.shuffle(output)
- return output
-
-
-def very_slightly_biased_randint(max_val_vector):
- sample_dtype = np.uint64
- out_dtype = max_val_vector.dtype
- samples = np.random.randint(low=0, high=np.iinfo(sample_dtype).max,
- size=max_val_vector.shape, dtype=sample_dtype)
- return np.mod(samples, max_val_vector.astype(sample_dtype)).astype(out_dtype)
-
-
-def mask_duplicates(x, axis=1): # type: (np.ndarray, int) -> np.ndarray
- """Identify duplicates from sampling with replacement.
-
- Args:
- x: A 2D NumPy array of samples
- axis: The axis along which to de-dupe.
-
- Returns:
- A NumPy array with the same shape as x with one if an element appeared
- previously along axis 1, else zero.
- """
- if axis != 1:
- raise NotImplementedError
-
- x_sort_ind = np.argsort(x, axis=1, kind="mergesort")
- sorted_x = x[np.arange(x.shape[0])[:, np.newaxis], x_sort_ind]
-
- # compute the indices needed to map values back to their original position.
- inv_x_sort_ind = np.argsort(x_sort_ind, axis=1, kind="mergesort")
-
- # Compute the difference of adjacent sorted elements.
- diffs = sorted_x[:, :-1] - sorted_x[:, 1:]
-
- # We are only interested in whether an element is zero. Therefore left padding
- # with ones to restore the original shape is sufficient.
- diffs = np.concatenate(
- [np.ones((diffs.shape[0], 1), dtype=diffs.dtype), diffs], axis=1)
-
- # Duplicate values will have a difference of zero. By definition the first
- # element is never a duplicate.
- return np.where(diffs[np.arange(x.shape[0])[:, np.newaxis],
- inv_x_sort_ind], 0, 1)
diff --git a/spaces/NCTCMumbai/NCTC/models/research/autoencoder/MaskingNoiseAutoencoderRunner.py b/spaces/NCTCMumbai/NCTC/models/research/autoencoder/MaskingNoiseAutoencoderRunner.py
deleted file mode 100644
index b776302e286ff740ba7b8e6f679a54b23944df12..0000000000000000000000000000000000000000
--- a/spaces/NCTCMumbai/NCTC/models/research/autoencoder/MaskingNoiseAutoencoderRunner.py
+++ /dev/null
@@ -1,55 +0,0 @@
-from __future__ import absolute_import
-from __future__ import division
-from __future__ import print_function
-
-import numpy as np
-import sklearn.preprocessing as prep
-import tensorflow as tf
-from tensorflow.examples.tutorials.mnist import input_data
-
-from autoencoder_models.DenoisingAutoencoder import MaskingNoiseAutoencoder
-
-mnist = input_data.read_data_sets('MNIST_data', one_hot=True)
-
-
-def standard_scale(X_train, X_test):
- preprocessor = prep.StandardScaler().fit(X_train)
- X_train = preprocessor.transform(X_train)
- X_test = preprocessor.transform(X_test)
- return X_train, X_test
-
-
-def get_random_block_from_data(data, batch_size):
- start_index = np.random.randint(0, len(data) - batch_size)
- return data[start_index:(start_index + batch_size)]
-
-
-X_train, X_test = standard_scale(mnist.train.images, mnist.test.images)
-
-n_samples = int(mnist.train.num_examples)
-training_epochs = 100
-batch_size = 128
-display_step = 1
-
-autoencoder = MaskingNoiseAutoencoder(
- n_input=784,
- n_hidden=200,
- transfer_function=tf.nn.softplus,
- optimizer=tf.train.AdamOptimizer(learning_rate=0.001),
- dropout_probability=0.95)
-
-for epoch in range(training_epochs):
- avg_cost = 0.
- total_batch = int(n_samples / batch_size)
- for i in range(total_batch):
- batch_xs = get_random_block_from_data(X_train, batch_size)
-
- cost = autoencoder.partial_fit(batch_xs)
-
- avg_cost += cost / n_samples * batch_size
-
- if epoch % display_step == 0:
- print("Epoch:", '%d,' % (epoch + 1),
- "Cost:", "{:.9f}".format(avg_cost))
-
-print("Total cost: " + str(autoencoder.calc_total_cost(X_test)))
diff --git a/spaces/NingKanae/anime-voice-generator/transforms.py b/spaces/NingKanae/anime-voice-generator/transforms.py
deleted file mode 100644
index 4793d67ca5a5630e0ffe0f9fb29445c949e64dae..0000000000000000000000000000000000000000
--- a/spaces/NingKanae/anime-voice-generator/transforms.py
+++ /dev/null
@@ -1,193 +0,0 @@
-import torch
-from torch.nn import functional as F
-
-import numpy as np
-
-
-DEFAULT_MIN_BIN_WIDTH = 1e-3
-DEFAULT_MIN_BIN_HEIGHT = 1e-3
-DEFAULT_MIN_DERIVATIVE = 1e-3
-
-
-def piecewise_rational_quadratic_transform(inputs,
- unnormalized_widths,
- unnormalized_heights,
- unnormalized_derivatives,
- inverse=False,
- tails=None,
- tail_bound=1.,
- min_bin_width=DEFAULT_MIN_BIN_WIDTH,
- min_bin_height=DEFAULT_MIN_BIN_HEIGHT,
- min_derivative=DEFAULT_MIN_DERIVATIVE):
-
- if tails is None:
- spline_fn = rational_quadratic_spline
- spline_kwargs = {}
- else:
- spline_fn = unconstrained_rational_quadratic_spline
- spline_kwargs = {
- 'tails': tails,
- 'tail_bound': tail_bound
- }
-
- outputs, logabsdet = spline_fn(
- inputs=inputs,
- unnormalized_widths=unnormalized_widths,
- unnormalized_heights=unnormalized_heights,
- unnormalized_derivatives=unnormalized_derivatives,
- inverse=inverse,
- min_bin_width=min_bin_width,
- min_bin_height=min_bin_height,
- min_derivative=min_derivative,
- **spline_kwargs
- )
- return outputs, logabsdet
-
-
-def searchsorted(bin_locations, inputs, eps=1e-6):
- bin_locations[..., -1] += eps
- return torch.sum(
- inputs[..., None] >= bin_locations,
- dim=-1
- ) - 1
-
-
-def unconstrained_rational_quadratic_spline(inputs,
- unnormalized_widths,
- unnormalized_heights,
- unnormalized_derivatives,
- inverse=False,
- tails='linear',
- tail_bound=1.,
- min_bin_width=DEFAULT_MIN_BIN_WIDTH,
- min_bin_height=DEFAULT_MIN_BIN_HEIGHT,
- min_derivative=DEFAULT_MIN_DERIVATIVE):
- inside_interval_mask = (inputs >= -tail_bound) & (inputs <= tail_bound)
- outside_interval_mask = ~inside_interval_mask
-
- outputs = torch.zeros_like(inputs)
- logabsdet = torch.zeros_like(inputs)
-
- if tails == 'linear':
- unnormalized_derivatives = F.pad(unnormalized_derivatives, pad=(1, 1))
- constant = np.log(np.exp(1 - min_derivative) - 1)
- unnormalized_derivatives[..., 0] = constant
- unnormalized_derivatives[..., -1] = constant
-
- outputs[outside_interval_mask] = inputs[outside_interval_mask]
- logabsdet[outside_interval_mask] = 0
- else:
- raise RuntimeError('{} tails are not implemented.'.format(tails))
-
- outputs[inside_interval_mask], logabsdet[inside_interval_mask] = rational_quadratic_spline(
- inputs=inputs[inside_interval_mask],
- unnormalized_widths=unnormalized_widths[inside_interval_mask, :],
- unnormalized_heights=unnormalized_heights[inside_interval_mask, :],
- unnormalized_derivatives=unnormalized_derivatives[inside_interval_mask, :],
- inverse=inverse,
- left=-tail_bound, right=tail_bound, bottom=-tail_bound, top=tail_bound,
- min_bin_width=min_bin_width,
- min_bin_height=min_bin_height,
- min_derivative=min_derivative
- )
-
- return outputs, logabsdet
-
-def rational_quadratic_spline(inputs,
- unnormalized_widths,
- unnormalized_heights,
- unnormalized_derivatives,
- inverse=False,
- left=0., right=1., bottom=0., top=1.,
- min_bin_width=DEFAULT_MIN_BIN_WIDTH,
- min_bin_height=DEFAULT_MIN_BIN_HEIGHT,
- min_derivative=DEFAULT_MIN_DERIVATIVE):
- if torch.min(inputs) < left or torch.max(inputs) > right:
- raise ValueError('Input to a transform is not within its domain')
-
- num_bins = unnormalized_widths.shape[-1]
-
- if min_bin_width * num_bins > 1.0:
- raise ValueError('Minimal bin width too large for the number of bins')
- if min_bin_height * num_bins > 1.0:
- raise ValueError('Minimal bin height too large for the number of bins')
-
- widths = F.softmax(unnormalized_widths, dim=-1)
- widths = min_bin_width + (1 - min_bin_width * num_bins) * widths
- cumwidths = torch.cumsum(widths, dim=-1)
- cumwidths = F.pad(cumwidths, pad=(1, 0), mode='constant', value=0.0)
- cumwidths = (right - left) * cumwidths + left
- cumwidths[..., 0] = left
- cumwidths[..., -1] = right
- widths = cumwidths[..., 1:] - cumwidths[..., :-1]
-
- derivatives = min_derivative + F.softplus(unnormalized_derivatives)
-
- heights = F.softmax(unnormalized_heights, dim=-1)
- heights = min_bin_height + (1 - min_bin_height * num_bins) * heights
- cumheights = torch.cumsum(heights, dim=-1)
- cumheights = F.pad(cumheights, pad=(1, 0), mode='constant', value=0.0)
- cumheights = (top - bottom) * cumheights + bottom
- cumheights[..., 0] = bottom
- cumheights[..., -1] = top
- heights = cumheights[..., 1:] - cumheights[..., :-1]
-
- if inverse:
- bin_idx = searchsorted(cumheights, inputs)[..., None]
- else:
- bin_idx = searchsorted(cumwidths, inputs)[..., None]
-
- input_cumwidths = cumwidths.gather(-1, bin_idx)[..., 0]
- input_bin_widths = widths.gather(-1, bin_idx)[..., 0]
-
- input_cumheights = cumheights.gather(-1, bin_idx)[..., 0]
- delta = heights / widths
- input_delta = delta.gather(-1, bin_idx)[..., 0]
-
- input_derivatives = derivatives.gather(-1, bin_idx)[..., 0]
- input_derivatives_plus_one = derivatives[..., 1:].gather(-1, bin_idx)[..., 0]
-
- input_heights = heights.gather(-1, bin_idx)[..., 0]
-
- if inverse:
- a = (((inputs - input_cumheights) * (input_derivatives
- + input_derivatives_plus_one
- - 2 * input_delta)
- + input_heights * (input_delta - input_derivatives)))
- b = (input_heights * input_derivatives
- - (inputs - input_cumheights) * (input_derivatives
- + input_derivatives_plus_one
- - 2 * input_delta))
- c = - input_delta * (inputs - input_cumheights)
-
- discriminant = b.pow(2) - 4 * a * c
- assert (discriminant >= 0).all()
-
- root = (2 * c) / (-b - torch.sqrt(discriminant))
- outputs = root * input_bin_widths + input_cumwidths
-
- theta_one_minus_theta = root * (1 - root)
- denominator = input_delta + ((input_derivatives + input_derivatives_plus_one - 2 * input_delta)
- * theta_one_minus_theta)
- derivative_numerator = input_delta.pow(2) * (input_derivatives_plus_one * root.pow(2)
- + 2 * input_delta * theta_one_minus_theta
- + input_derivatives * (1 - root).pow(2))
- logabsdet = torch.log(derivative_numerator) - 2 * torch.log(denominator)
-
- return outputs, -logabsdet
- else:
- theta = (inputs - input_cumwidths) / input_bin_widths
- theta_one_minus_theta = theta * (1 - theta)
-
- numerator = input_heights * (input_delta * theta.pow(2)
- + input_derivatives * theta_one_minus_theta)
- denominator = input_delta + ((input_derivatives + input_derivatives_plus_one - 2 * input_delta)
- * theta_one_minus_theta)
- outputs = input_cumheights + numerator / denominator
-
- derivative_numerator = input_delta.pow(2) * (input_derivatives_plus_one * theta.pow(2)
- + 2 * input_delta * theta_one_minus_theta
- + input_derivatives * (1 - theta).pow(2))
- logabsdet = torch.log(derivative_numerator) - 2 * torch.log(denominator)
-
- return outputs, logabsdet
diff --git a/spaces/OFA-Sys/OFA-Generic_Interface/fairseq/examples/roberta/README.custom_classification.md b/spaces/OFA-Sys/OFA-Generic_Interface/fairseq/examples/roberta/README.custom_classification.md
deleted file mode 100644
index 7254bb7d178760ef5b847901bbcac3711af33ca2..0000000000000000000000000000000000000000
--- a/spaces/OFA-Sys/OFA-Generic_Interface/fairseq/examples/roberta/README.custom_classification.md
+++ /dev/null
@@ -1,168 +0,0 @@
-# Finetuning RoBERTa on a custom classification task
-
-This example shows how to finetune RoBERTa on the IMDB dataset, but should illustrate the process for most classification tasks.
-
-### 1) Get the data
-
-```bash
-wget http://ai.stanford.edu/~amaas/data/sentiment/aclImdb_v1.tar.gz
-tar zxvf aclImdb_v1.tar.gz
-```
-
-
-### 2) Format data
-
-`IMDB` data has one data-sample in each file, below python code-snippet converts it one file for train and valid each for ease of processing.
-```python
-import argparse
-import os
-import random
-from glob import glob
-
-random.seed(0)
-
-def main(args):
- for split in ['train', 'test']:
- samples = []
- for class_label in ['pos', 'neg']:
- fnames = glob(os.path.join(args.datadir, split, class_label) + '/*.txt')
- for fname in fnames:
- with open(fname) as fin:
- line = fin.readline()
- samples.append((line, 1 if class_label == 'pos' else 0))
- random.shuffle(samples)
- out_fname = 'train' if split == 'train' else 'dev'
- f1 = open(os.path.join(args.datadir, out_fname + '.input0'), 'w')
- f2 = open(os.path.join(args.datadir, out_fname + '.label'), 'w')
- for sample in samples:
- f1.write(sample[0] + '\n')
- f2.write(str(sample[1]) + '\n')
- f1.close()
- f2.close()
-
-if __name__ == '__main__':
- parser = argparse.ArgumentParser()
- parser.add_argument('--datadir', default='aclImdb')
- args = parser.parse_args()
- main(args)
-```
-
-
-### 3) BPE encode
-
-Run `multiprocessing_bpe_encoder`, you can also do this in previous step for each sample but that might be slower.
-```bash
-# Download encoder.json and vocab.bpe
-wget -N 'https://dl.fbaipublicfiles.com/fairseq/gpt2_bpe/encoder.json'
-wget -N 'https://dl.fbaipublicfiles.com/fairseq/gpt2_bpe/vocab.bpe'
-
-for SPLIT in train dev; do
- python -m examples.roberta.multiprocessing_bpe_encoder \
- --encoder-json encoder.json \
- --vocab-bpe vocab.bpe \
- --inputs "aclImdb/$SPLIT.input0" \
- --outputs "aclImdb/$SPLIT.input0.bpe" \
- --workers 60 \
- --keep-empty
-done
-```
-
-
-### 4) Preprocess data
-
-```bash
-# Download fairseq dictionary.
-wget -N 'https://dl.fbaipublicfiles.com/fairseq/gpt2_bpe/dict.txt'
-
-fairseq-preprocess \
- --only-source \
- --trainpref "aclImdb/train.input0.bpe" \
- --validpref "aclImdb/dev.input0.bpe" \
- --destdir "IMDB-bin/input0" \
- --workers 60 \
- --srcdict dict.txt
-
-fairseq-preprocess \
- --only-source \
- --trainpref "aclImdb/train.label" \
- --validpref "aclImdb/dev.label" \
- --destdir "IMDB-bin/label" \
- --workers 60
-
-```
-
-
-### 5) Run training
-
-```bash
-TOTAL_NUM_UPDATES=7812 # 10 epochs through IMDB for bsz 32
-WARMUP_UPDATES=469 # 6 percent of the number of updates
-LR=1e-05 # Peak LR for polynomial LR scheduler.
-HEAD_NAME=imdb_head # Custom name for the classification head.
-NUM_CLASSES=2 # Number of classes for the classification task.
-MAX_SENTENCES=8 # Batch size.
-ROBERTA_PATH=/path/to/roberta.large/model.pt
-
-CUDA_VISIBLE_DEVICES=0 fairseq-train IMDB-bin/ \
- --restore-file $ROBERTA_PATH \
- --max-positions 512 \
- --batch-size $MAX_SENTENCES \
- --max-tokens 4400 \
- --task sentence_prediction \
- --reset-optimizer --reset-dataloader --reset-meters \
- --required-batch-size-multiple 1 \
- --init-token 0 --separator-token 2 \
- --arch roberta_large \
- --criterion sentence_prediction \
- --classification-head-name $HEAD_NAME \
- --num-classes $NUM_CLASSES \
- --dropout 0.1 --attention-dropout 0.1 \
- --weight-decay 0.1 --optimizer adam --adam-betas "(0.9, 0.98)" --adam-eps 1e-06 \
- --clip-norm 0.0 \
- --lr-scheduler polynomial_decay --lr $LR --total-num-update $TOTAL_NUM_UPDATES --warmup-updates $WARMUP_UPDATES \
- --fp16 --fp16-init-scale 4 --threshold-loss-scale 1 --fp16-scale-window 128 \
- --max-epoch 10 \
- --best-checkpoint-metric accuracy --maximize-best-checkpoint-metric \
- --shorten-method "truncate" \
- --find-unused-parameters \
- --update-freq 4
-```
-
-The above command will finetune RoBERTa-large with an effective batch-size of 32
-sentences (`--batch-size=8 --update-freq=4`). The expected
-`best-validation-accuracy` after 10 epochs is ~96.5%.
-
-If you run out of GPU memory, try decreasing `--batch-size` and increase
-`--update-freq` to compensate.
-
-
-### 6) Load model using hub interface
-
-Now we can load the trained model checkpoint using the RoBERTa hub interface.
-
-Assuming your checkpoints are stored in `checkpoints/`:
-```python
-from fairseq.models.roberta import RobertaModel
-roberta = RobertaModel.from_pretrained(
- 'checkpoints',
- checkpoint_file='checkpoint_best.pt',
- data_name_or_path='IMDB-bin'
-)
-roberta.eval() # disable dropout
-```
-
-Finally you can make predictions using the `imdb_head` (or whatever you set
-`--classification-head-name` to during training):
-```python
-label_fn = lambda label: roberta.task.label_dictionary.string(
- [label + roberta.task.label_dictionary.nspecial]
-)
-
-tokens = roberta.encode('Best movie this year')
-pred = label_fn(roberta.predict('imdb_head', tokens).argmax().item())
-assert pred == '1' # positive
-
-tokens = roberta.encode('Worst movie ever')
-pred = label_fn(roberta.predict('imdb_head', tokens).argmax().item())
-assert pred == '0' # negative
-```
diff --git a/spaces/OFA-Sys/OFA-Generic_Interface/fairseq/fairseq/registry.py b/spaces/OFA-Sys/OFA-Generic_Interface/fairseq/fairseq/registry.py
deleted file mode 100644
index f3b9406043d75a51d7bf4af5294f82b33a8f9a5e..0000000000000000000000000000000000000000
--- a/spaces/OFA-Sys/OFA-Generic_Interface/fairseq/fairseq/registry.py
+++ /dev/null
@@ -1,100 +0,0 @@
-# Copyright (c) Facebook, Inc. and its affiliates.
-#
-# This source code is licensed under the MIT license found in the
-# LICENSE file in the root directory of this source tree.
-
-from argparse import Namespace
-
-from typing import Union
-from fairseq.dataclass import FairseqDataclass
-from fairseq.dataclass.utils import merge_with_parent
-from hydra.core.config_store import ConfigStore
-from omegaconf import DictConfig
-
-REGISTRIES = {}
-
-
-def setup_registry(registry_name: str, base_class=None, default=None, required=False):
- assert registry_name.startswith("--")
- registry_name = registry_name[2:].replace("-", "_")
-
- REGISTRY = {}
- REGISTRY_CLASS_NAMES = set()
- DATACLASS_REGISTRY = {}
-
- # maintain a registry of all registries
- if registry_name in REGISTRIES:
- return # registry already exists
- REGISTRIES[registry_name] = {
- "registry": REGISTRY,
- "default": default,
- "dataclass_registry": DATACLASS_REGISTRY,
- }
-
- def build_x(cfg: Union[DictConfig, str, Namespace], *extra_args, **extra_kwargs):
- if isinstance(cfg, DictConfig):
- choice = cfg._name
-
- if choice and choice in DATACLASS_REGISTRY:
- dc = DATACLASS_REGISTRY[choice]
- cfg = merge_with_parent(dc(), cfg)
- elif isinstance(cfg, str):
- choice = cfg
- if choice in DATACLASS_REGISTRY:
- cfg = DATACLASS_REGISTRY[choice]()
- else:
- choice = getattr(cfg, registry_name, None)
- if choice in DATACLASS_REGISTRY:
- cfg = DATACLASS_REGISTRY[choice].from_namespace(cfg)
-
- if choice is None:
- if required:
- raise ValueError("{} is required!".format(registry_name))
- return None
-
- cls = REGISTRY[choice]
- if hasattr(cls, "build_" + registry_name):
- builder = getattr(cls, "build_" + registry_name)
- else:
- builder = cls
-
- return builder(cfg, *extra_args, **extra_kwargs)
-
- def register_x(name, dataclass=None):
- def register_x_cls(cls):
- if name in REGISTRY:
- raise ValueError(
- "Cannot register duplicate {} ({})".format(registry_name, name)
- )
- if cls.__name__ in REGISTRY_CLASS_NAMES:
- raise ValueError(
- "Cannot register {} with duplicate class name ({})".format(
- registry_name, cls.__name__
- )
- )
- if base_class is not None and not issubclass(cls, base_class):
- raise ValueError(
- "{} must extend {}".format(cls.__name__, base_class.__name__)
- )
-
- if dataclass is not None and not issubclass(dataclass, FairseqDataclass):
- raise ValueError(
- "Dataclass {} must extend FairseqDataclass".format(dataclass)
- )
-
- cls.__dataclass = dataclass
- if cls.__dataclass is not None:
- DATACLASS_REGISTRY[name] = cls.__dataclass
-
- cs = ConfigStore.instance()
- node = dataclass()
- node._name = name
- cs.store(name=name, group=registry_name, node=node, provider="fairseq")
-
- REGISTRY[name] = cls
-
- return cls
-
- return register_x_cls
-
- return build_x, register_x, REGISTRY, DATACLASS_REGISTRY
diff --git a/spaces/OFA-Sys/OFA-vqa/fairseq/fairseq/models/speech_to_text/s2t_transformer.py b/spaces/OFA-Sys/OFA-vqa/fairseq/fairseq/models/speech_to_text/s2t_transformer.py
deleted file mode 100644
index aff9d0ffc7b7e671c476ff28d1cd945e9ff41519..0000000000000000000000000000000000000000
--- a/spaces/OFA-Sys/OFA-vqa/fairseq/fairseq/models/speech_to_text/s2t_transformer.py
+++ /dev/null
@@ -1,502 +0,0 @@
-#!/usr/bin/env python3
-
-import logging
-import math
-from typing import Dict, List, Optional, Tuple
-from pathlib import Path
-
-import torch
-import torch.nn as nn
-from fairseq import checkpoint_utils, utils
-from fairseq.data.data_utils import lengths_to_padding_mask
-from fairseq.models import (
- FairseqEncoder,
- FairseqEncoderDecoderModel,
- register_model,
- register_model_architecture,
-)
-from fairseq.models.transformer import Embedding, TransformerDecoder
-from fairseq.modules import (
- FairseqDropout,
- LayerNorm,
- PositionalEmbedding,
- TransformerEncoderLayer,
-)
-from torch import Tensor
-
-
-logger = logging.getLogger(__name__)
-
-
-class Conv1dSubsampler(nn.Module):
- """Convolutional subsampler: a stack of 1D convolution (along temporal
- dimension) followed by non-linear activation via gated linear units
- (https://arxiv.org/abs/1911.08460)
-
- Args:
- in_channels (int): the number of input channels
- mid_channels (int): the number of intermediate channels
- out_channels (int): the number of output channels
- kernel_sizes (List[int]): the kernel size for each convolutional layer
- """
-
- def __init__(
- self,
- in_channels: int,
- mid_channels: int,
- out_channels: int,
- kernel_sizes: List[int] = (3, 3),
- ):
- super(Conv1dSubsampler, self).__init__()
- self.n_layers = len(kernel_sizes)
- self.conv_layers = nn.ModuleList(
- nn.Conv1d(
- in_channels if i == 0 else mid_channels // 2,
- mid_channels if i < self.n_layers - 1 else out_channels * 2,
- k,
- stride=2,
- padding=k // 2,
- )
- for i, k in enumerate(kernel_sizes)
- )
-
- def get_out_seq_lens_tensor(self, in_seq_lens_tensor):
- out = in_seq_lens_tensor.clone()
- for _ in range(self.n_layers):
- out = ((out.float() - 1) / 2 + 1).floor().long()
- return out
-
- def forward(self, src_tokens, src_lengths):
- bsz, in_seq_len, _ = src_tokens.size() # B x T x (C x D)
- x = src_tokens.transpose(1, 2).contiguous() # -> B x (C x D) x T
- for conv in self.conv_layers:
- x = conv(x)
- x = nn.functional.glu(x, dim=1)
- _, _, out_seq_len = x.size()
- x = x.transpose(1, 2).transpose(0, 1).contiguous() # -> T x B x (C x D)
- return x, self.get_out_seq_lens_tensor(src_lengths)
-
-
-@register_model("s2t_transformer")
-class S2TTransformerModel(FairseqEncoderDecoderModel):
- """Adapted Transformer model (https://arxiv.org/abs/1706.03762) for
- speech-to-text tasks. The Transformer encoder/decoder remains the same.
- A trainable input subsampler is prepended to the Transformer encoder to
- project inputs into the encoder dimension as well as downsample input
- sequence for computational efficiency."""
-
- def __init__(self, encoder, decoder):
- super().__init__(encoder, decoder)
-
- @staticmethod
- def add_args(parser):
- """Add model-specific arguments to the parser."""
- # input
- parser.add_argument(
- "--conv-kernel-sizes",
- type=str,
- metavar="N",
- help="kernel sizes of Conv1d subsampling layers",
- )
- parser.add_argument(
- "--conv-channels",
- type=int,
- metavar="N",
- help="# of channels in Conv1d subsampling layers",
- )
- # Transformer
- parser.add_argument(
- "--activation-fn",
- type=str,
- default="relu",
- choices=utils.get_available_activation_fns(),
- help="activation function to use",
- )
- parser.add_argument(
- "--dropout", type=float, metavar="D", help="dropout probability"
- )
- parser.add_argument(
- "--attention-dropout",
- type=float,
- metavar="D",
- help="dropout probability for attention weights",
- )
- parser.add_argument(
- "--activation-dropout",
- "--relu-dropout",
- type=float,
- metavar="D",
- help="dropout probability after activation in FFN.",
- )
- parser.add_argument(
- "--encoder-embed-dim",
- type=int,
- metavar="N",
- help="encoder embedding dimension",
- )
- parser.add_argument(
- "--encoder-ffn-embed-dim",
- type=int,
- metavar="N",
- help="encoder embedding dimension for FFN",
- )
- parser.add_argument(
- "--encoder-layers", type=int, metavar="N", help="num encoder layers"
- )
- parser.add_argument(
- "--encoder-attention-heads",
- type=int,
- metavar="N",
- help="num encoder attention heads",
- )
- parser.add_argument(
- "--encoder-normalize-before",
- action="store_true",
- help="apply layernorm before each encoder block",
- )
- parser.add_argument(
- "--decoder-embed-dim",
- type=int,
- metavar="N",
- help="decoder embedding dimension",
- )
- parser.add_argument(
- "--decoder-ffn-embed-dim",
- type=int,
- metavar="N",
- help="decoder embedding dimension for FFN",
- )
- parser.add_argument(
- "--decoder-layers", type=int, metavar="N", help="num decoder layers"
- )
- parser.add_argument(
- "--decoder-attention-heads",
- type=int,
- metavar="N",
- help="num decoder attention heads",
- )
- parser.add_argument(
- "--decoder-normalize-before",
- action="store_true",
- help="apply layernorm before each decoder block",
- )
- parser.add_argument(
- "--share-decoder-input-output-embed",
- action="store_true",
- help="share decoder input and output embeddings",
- )
- parser.add_argument(
- "--layernorm-embedding",
- action="store_true",
- help="add layernorm to embedding",
- )
- parser.add_argument(
- "--no-scale-embedding",
- action="store_true",
- help="if True, dont scale embeddings",
- )
- parser.add_argument(
- "--load-pretrained-encoder-from",
- type=str,
- metavar="STR",
- help="model to take encoder weights from (for initialization)",
- )
- parser.add_argument(
- '--encoder-freezing-updates',
- type=int,
- metavar='N',
- help='freeze encoder for first N updates'
- )
-
- @classmethod
- def build_encoder(cls, args):
- encoder = S2TTransformerEncoder(args)
- pretraining_path = getattr(args, "load_pretrained_encoder_from", None)
- if pretraining_path is not None:
- if not Path(pretraining_path).exists():
- logger.warning(
- f"skipped pretraining because {pretraining_path} does not exist"
- )
- else:
- encoder = checkpoint_utils.load_pretrained_component_from_model(
- component=encoder, checkpoint=pretraining_path
- )
- logger.info(f"loaded pretrained encoder from: {pretraining_path}")
- return encoder
-
- @classmethod
- def build_decoder(cls, args, task, embed_tokens):
- return TransformerDecoderScriptable(args, task.target_dictionary, embed_tokens)
-
- @classmethod
- def build_model(cls, args, task):
- """Build a new model instance."""
-
- # make sure all arguments are present in older models
- base_architecture(args)
-
- def build_embedding(dictionary, embed_dim):
- num_embeddings = len(dictionary)
- padding_idx = dictionary.pad()
- return Embedding(num_embeddings, embed_dim, padding_idx)
-
- decoder_embed_tokens = build_embedding(
- task.target_dictionary, args.decoder_embed_dim
- )
- encoder = cls.build_encoder(args)
- decoder = cls.build_decoder(args, task, decoder_embed_tokens)
- return cls(encoder, decoder)
-
- def get_normalized_probs(
- self,
- net_output: Tuple[Tensor, Optional[Dict[str, List[Optional[Tensor]]]]],
- log_probs: bool,
- sample: Optional[Dict[str, Tensor]] = None,
- ):
- # net_output['encoder_out'] is a (B, T, D) tensor
- lprobs = self.get_normalized_probs_scriptable(net_output, log_probs, sample)
- lprobs.batch_first = True
- return lprobs
-
- def forward(self, src_tokens, src_lengths, prev_output_tokens):
- """
- The forward method inherited from the base class has a **kwargs
- argument in its input, which is not supported in torchscript. This
- method overwrites the forward method definition without **kwargs.
- """
- encoder_out = self.encoder(src_tokens=src_tokens, src_lengths=src_lengths)
- decoder_out = self.decoder(
- prev_output_tokens=prev_output_tokens, encoder_out=encoder_out
- )
- return decoder_out
-
-
-class S2TTransformerEncoder(FairseqEncoder):
- """Speech-to-text Transformer encoder that consists of input subsampler and
- Transformer encoder."""
-
- def __init__(self, args):
- super().__init__(None)
-
- self.encoder_freezing_updates = args.encoder_freezing_updates
- self.num_updates = 0
-
- self.dropout_module = FairseqDropout(
- p=args.dropout, module_name=self.__class__.__name__
- )
- self.embed_scale = math.sqrt(args.encoder_embed_dim)
- if args.no_scale_embedding:
- self.embed_scale = 1.0
- self.padding_idx = 1
-
- self.subsample = Conv1dSubsampler(
- args.input_feat_per_channel * args.input_channels,
- args.conv_channels,
- args.encoder_embed_dim,
- [int(k) for k in args.conv_kernel_sizes.split(",")],
- )
-
- self.embed_positions = PositionalEmbedding(
- args.max_source_positions, args.encoder_embed_dim, self.padding_idx
- )
-
- self.transformer_layers = nn.ModuleList(
- [TransformerEncoderLayer(args) for _ in range(args.encoder_layers)]
- )
- if args.encoder_normalize_before:
- self.layer_norm = LayerNorm(args.encoder_embed_dim)
- else:
- self.layer_norm = None
-
- def _forward(self, src_tokens, src_lengths, return_all_hiddens=False):
- x, input_lengths = self.subsample(src_tokens, src_lengths)
- x = self.embed_scale * x
-
- encoder_padding_mask = lengths_to_padding_mask(input_lengths)
- positions = self.embed_positions(encoder_padding_mask).transpose(0, 1)
- x += positions
- x = self.dropout_module(x)
-
- encoder_states = []
-
- for layer in self.transformer_layers:
- x = layer(x, encoder_padding_mask)
- if return_all_hiddens:
- encoder_states.append(x)
-
- if self.layer_norm is not None:
- x = self.layer_norm(x)
-
- return {
- "encoder_out": [x], # T x B x C
- "encoder_padding_mask": [encoder_padding_mask] if encoder_padding_mask.any() else [], # B x T
- "encoder_embedding": [], # B x T x C
- "encoder_states": encoder_states, # List[T x B x C]
- "src_tokens": [],
- "src_lengths": [],
- }
-
- def forward(self, src_tokens, src_lengths, return_all_hiddens=False):
- if self.num_updates < self.encoder_freezing_updates:
- with torch.no_grad():
- x = self._forward(src_tokens, src_lengths,
- return_all_hiddens=return_all_hiddens)
- else:
- x = self._forward(src_tokens, src_lengths,
- return_all_hiddens=return_all_hiddens)
- return x
-
- def reorder_encoder_out(self, encoder_out, new_order):
- new_encoder_out = (
- [] if len(encoder_out["encoder_out"]) == 0
- else [x.index_select(1, new_order) for x in encoder_out["encoder_out"]]
- )
-
- new_encoder_padding_mask = (
- [] if len(encoder_out["encoder_padding_mask"]) == 0
- else [x.index_select(0, new_order) for x in encoder_out["encoder_padding_mask"]]
- )
-
- new_encoder_embedding = (
- [] if len(encoder_out["encoder_embedding"]) == 0
- else [x.index_select(0, new_order) for x in encoder_out["encoder_embedding"]]
- )
-
- encoder_states = encoder_out["encoder_states"]
- if len(encoder_states) > 0:
- for idx, state in enumerate(encoder_states):
- encoder_states[idx] = state.index_select(1, new_order)
-
- return {
- "encoder_out": new_encoder_out, # T x B x C
- "encoder_padding_mask": new_encoder_padding_mask, # B x T
- "encoder_embedding": new_encoder_embedding, # B x T x C
- "encoder_states": encoder_states, # List[T x B x C]
- "src_tokens": [], # B x T
- "src_lengths": [], # B x 1
- }
-
- def set_num_updates(self, num_updates):
- super().set_num_updates(num_updates)
- self.num_updates = num_updates
-
-
-class TransformerDecoderScriptable(TransformerDecoder):
- def extract_features(
- self,
- prev_output_tokens,
- encoder_out: Optional[Dict[str, List[Tensor]]] = None,
- incremental_state: Optional[Dict[str, Dict[str, Optional[Tensor]]]] = None,
- full_context_alignment: bool = False,
- alignment_layer: Optional[int] = None,
- alignment_heads: Optional[int] = None,
- ):
- # call scriptable method from parent class
- x, _ = self.extract_features_scriptable(
- prev_output_tokens,
- encoder_out,
- incremental_state,
- full_context_alignment,
- alignment_layer,
- alignment_heads,
- )
- return x, None
-
-
-@register_model_architecture(model_name="s2t_transformer", arch_name="s2t_transformer")
-def base_architecture(args):
- args.encoder_freezing_updates = getattr(args, "encoder_freezing_updates", 0)
- # Convolutional subsampler
- args.conv_kernel_sizes = getattr(args, "conv_kernel_sizes", "5,5")
- args.conv_channels = getattr(args, "conv_channels", 1024)
- # Transformer
- args.encoder_embed_dim = getattr(args, "encoder_embed_dim", 512)
- args.encoder_ffn_embed_dim = getattr(args, "encoder_ffn_embed_dim", 2048)
- args.encoder_layers = getattr(args, "encoder_layers", 12)
- args.encoder_attention_heads = getattr(args, "encoder_attention_heads", 8)
- args.encoder_normalize_before = getattr(args, "encoder_normalize_before", True)
- args.decoder_embed_dim = getattr(args, "decoder_embed_dim", args.encoder_embed_dim)
- args.decoder_ffn_embed_dim = getattr(
- args, "decoder_ffn_embed_dim", args.encoder_ffn_embed_dim
- )
- args.decoder_layers = getattr(args, "decoder_layers", 6)
- args.decoder_attention_heads = getattr(args, "decoder_attention_heads", 8)
- args.decoder_normalize_before = getattr(args, "decoder_normalize_before", True)
- args.decoder_learned_pos = getattr(args, "decoder_learned_pos", False)
- args.dropout = getattr(args, "dropout", 0.1)
- args.attention_dropout = getattr(args, "attention_dropout", args.dropout)
- args.activation_dropout = getattr(args, "activation_dropout", args.dropout)
- args.activation_fn = getattr(args, "activation_fn", "relu")
- args.adaptive_softmax_cutoff = getattr(args, "adaptive_softmax_cutoff", None)
- args.adaptive_softmax_dropout = getattr(args, "adaptive_softmax_dropout", 0)
- args.share_decoder_input_output_embed = getattr(
- args, "share_decoder_input_output_embed", False
- )
- args.no_token_positional_embeddings = getattr(
- args, "no_token_positional_embeddings", False
- )
- args.adaptive_input = getattr(args, "adaptive_input", False)
- args.decoder_layerdrop = getattr(args, "decoder_layerdrop", 0.0)
- args.decoder_output_dim = getattr(
- args, "decoder_output_dim", args.decoder_embed_dim
- )
- args.decoder_input_dim = getattr(args, "decoder_input_dim", args.decoder_embed_dim)
- args.no_scale_embedding = getattr(args, "no_scale_embedding", False)
- args.quant_noise_pq = getattr(args, "quant_noise_pq", 0)
-
-
-@register_model_architecture("s2t_transformer", "s2t_transformer_s")
-def s2t_transformer_s(args):
- args.encoder_embed_dim = getattr(args, "encoder_embed_dim", 256)
- args.encoder_ffn_embed_dim = getattr(args, "encoder_ffn_embed_dim", 256 * 8)
- args.encoder_attention_heads = getattr(args, "encoder_attention_heads", 4)
- args.decoder_attention_heads = getattr(args, "decoder_attention_heads", 4)
- args.dropout = getattr(args, "dropout", 0.1)
- base_architecture(args)
-
-
-@register_model_architecture("s2t_transformer", "s2t_transformer_xs")
-def s2t_transformer_xs(args):
- args.encoder_layers = getattr(args, "encoder_layers", 6)
- args.decoder_layers = getattr(args, "decoder_layers", 3)
- args.encoder_ffn_embed_dim = getattr(args, "encoder_ffn_embed_dim", 256 * 4)
- args.dropout = getattr(args, "dropout", 0.3)
- s2t_transformer_s(args)
-
-
-@register_model_architecture("s2t_transformer", "s2t_transformer_sp")
-def s2t_transformer_sp(args):
- args.encoder_layers = getattr(args, "encoder_layers", 16)
- s2t_transformer_s(args)
-
-
-@register_model_architecture("s2t_transformer", "s2t_transformer_m")
-def s2t_transformer_m(args):
- args.encoder_embed_dim = getattr(args, "encoder_embed_dim", 512)
- args.encoder_ffn_embed_dim = getattr(args, "encoder_ffn_embed_dim", 512 * 4)
- args.encoder_attention_heads = getattr(args, "encoder_attention_heads", 8)
- args.decoder_attention_heads = getattr(args, "decoder_attention_heads", 8)
- args.dropout = getattr(args, "dropout", 0.15)
- base_architecture(args)
-
-
-@register_model_architecture("s2t_transformer", "s2t_transformer_mp")
-def s2t_transformer_mp(args):
- args.encoder_layers = getattr(args, "encoder_layers", 16)
- s2t_transformer_m(args)
-
-
-@register_model_architecture("s2t_transformer", "s2t_transformer_l")
-def s2t_transformer_l(args):
- args.encoder_embed_dim = getattr(args, "encoder_embed_dim", 1024)
- args.encoder_ffn_embed_dim = getattr(args, "encoder_ffn_embed_dim", 1024 * 4)
- args.encoder_attention_heads = getattr(args, "encoder_attention_heads", 16)
- args.decoder_attention_heads = getattr(args, "decoder_attention_heads", 16)
- args.dropout = getattr(args, "dropout", 0.2)
- base_architecture(args)
-
-
-@register_model_architecture("s2t_transformer", "s2t_transformer_lp")
-def s2t_transformer_lp(args):
- args.encoder_layers = getattr(args, "encoder_layers", 16)
- s2t_transformer_l(args)
diff --git a/spaces/OIUGLK/bingo/src/components/chat-message.tsx b/spaces/OIUGLK/bingo/src/components/chat-message.tsx
deleted file mode 100644
index bf272d8d7005cfd06c53bd213e09ea217e803549..0000000000000000000000000000000000000000
--- a/spaces/OIUGLK/bingo/src/components/chat-message.tsx
+++ /dev/null
@@ -1,93 +0,0 @@
-import remarkGfm from 'remark-gfm'
-import remarkMath from 'remark-math'
-import supersub from 'remark-supersub'
-import remarkBreaks from 'remark-breaks'
-import { cn } from '@/lib/utils'
-import { CodeBlock } from '@/components/ui/codeblock'
-import { MemoizedReactMarkdown } from '@/components/markdown'
-import { LearnMore } from './learn-more'
-import { ChatMessageModel } from '@/lib/bots/bing/types'
-import { useEffect } from 'react'
-import { TurnCounter } from './turn-counter'
-
-export interface ChatMessageProps {
- message: ChatMessageModel
-}
-
-export function ChatMessage({ message, ...props }: ChatMessageProps) {
- useEffect(() => {
- if (document.body.scrollHeight - window.innerHeight - window.scrollY - 200 < 0) {
- window.scrollBy(0, 200)
- }
- }, [message.text])
-
- return message.text ? (
-
-
-
- }
- } catch (e) {
- }
- return
- },
- p({ children }) {
- return {children}
- },
- code({ node, inline, className, children, ...props }) {
- if (children.length) {
- if (children[0] == '▍') {
- return (
- ▍
- )
- }
-
- children[0] = (children[0] as string).replace('`▍`', '▍')
- }
-
- const match = /language-(\w+)/.exec(className || '')
-
- if (inline) {
- return (
-
- {children}
-
- )
- }
-
- return (
-
- )
- }
- }}
- >
- {message.text}
-
-
-
- {message.author === 'bot' && }
- {message.author === 'bot' && }
-
-
- ) : null
-}
diff --git a/spaces/PAIR/Text2Video-Zero/annotator/uniformer/mmcv/utils/timer.py b/spaces/PAIR/Text2Video-Zero/annotator/uniformer/mmcv/utils/timer.py
deleted file mode 100644
index e3db7d497d8b374e18b5297e0a1d6eb186fd8cba..0000000000000000000000000000000000000000
--- a/spaces/PAIR/Text2Video-Zero/annotator/uniformer/mmcv/utils/timer.py
+++ /dev/null
@@ -1,118 +0,0 @@
-# Copyright (c) OpenMMLab. All rights reserved.
-from time import time
-
-
-class TimerError(Exception):
-
- def __init__(self, message):
- self.message = message
- super(TimerError, self).__init__(message)
-
-
-class Timer:
- """A flexible Timer class.
-
- :Example:
-
- >>> import time
- >>> import annotator.uniformer.mmcv as mmcv
- >>> with mmcv.Timer():
- >>> # simulate a code block that will run for 1s
- >>> time.sleep(1)
- 1.000
- >>> with mmcv.Timer(print_tmpl='it takes {:.1f} seconds'):
- >>> # simulate a code block that will run for 1s
- >>> time.sleep(1)
- it takes 1.0 seconds
- >>> timer = mmcv.Timer()
- >>> time.sleep(0.5)
- >>> print(timer.since_start())
- 0.500
- >>> time.sleep(0.5)
- >>> print(timer.since_last_check())
- 0.500
- >>> print(timer.since_start())
- 1.000
- """
-
- def __init__(self, start=True, print_tmpl=None):
- self._is_running = False
- self.print_tmpl = print_tmpl if print_tmpl else '{:.3f}'
- if start:
- self.start()
-
- @property
- def is_running(self):
- """bool: indicate whether the timer is running"""
- return self._is_running
-
- def __enter__(self):
- self.start()
- return self
-
- def __exit__(self, type, value, traceback):
- print(self.print_tmpl.format(self.since_last_check()))
- self._is_running = False
-
- def start(self):
- """Start the timer."""
- if not self._is_running:
- self._t_start = time()
- self._is_running = True
- self._t_last = time()
-
- def since_start(self):
- """Total time since the timer is started.
-
- Returns (float): Time in seconds.
- """
- if not self._is_running:
- raise TimerError('timer is not running')
- self._t_last = time()
- return self._t_last - self._t_start
-
- def since_last_check(self):
- """Time since the last checking.
-
- Either :func:`since_start` or :func:`since_last_check` is a checking
- operation.
-
- Returns (float): Time in seconds.
- """
- if not self._is_running:
- raise TimerError('timer is not running')
- dur = time() - self._t_last
- self._t_last = time()
- return dur
-
-
-_g_timers = {} # global timers
-
-
-def check_time(timer_id):
- """Add check points in a single line.
-
- This method is suitable for running a task on a list of items. A timer will
- be registered when the method is called for the first time.
-
- :Example:
-
- >>> import time
- >>> import annotator.uniformer.mmcv as mmcv
- >>> for i in range(1, 6):
- >>> # simulate a code block
- >>> time.sleep(i)
- >>> mmcv.check_time('task1')
- 2.000
- 3.000
- 4.000
- 5.000
-
- Args:
- timer_id (str): Timer identifier.
- """
- if timer_id not in _g_timers:
- _g_timers[timer_id] = Timer()
- return 0
- else:
- return _g_timers[timer_id].since_last_check()
diff --git a/spaces/PKUWilliamYang/VToonify/vtoonify/model/raft/alt_cuda_corr/correlation.cpp b/spaces/PKUWilliamYang/VToonify/vtoonify/model/raft/alt_cuda_corr/correlation.cpp
deleted file mode 100644
index b01584d19edb99e7feec5f2e4c51169a1ed208db..0000000000000000000000000000000000000000
--- a/spaces/PKUWilliamYang/VToonify/vtoonify/model/raft/alt_cuda_corr/correlation.cpp
+++ /dev/null
@@ -1,54 +0,0 @@
-#include
-#include
-
-// CUDA forward declarations
-std::vector corr_cuda_forward(
- torch::Tensor fmap1,
- torch::Tensor fmap2,
- torch::Tensor coords,
- int radius);
-
-std::vector corr_cuda_backward(
- torch::Tensor fmap1,
- torch::Tensor fmap2,
- torch::Tensor coords,
- torch::Tensor corr_grad,
- int radius);
-
-// C++ interface
-#define CHECK_CUDA(x) TORCH_CHECK(x.type().is_cuda(), #x " must be a CUDA tensor")
-#define CHECK_CONTIGUOUS(x) TORCH_CHECK(x.is_contiguous(), #x " must be contiguous")
-#define CHECK_INPUT(x) CHECK_CUDA(x); CHECK_CONTIGUOUS(x)
-
-std::vector corr_forward(
- torch::Tensor fmap1,
- torch::Tensor fmap2,
- torch::Tensor coords,
- int radius) {
- CHECK_INPUT(fmap1);
- CHECK_INPUT(fmap2);
- CHECK_INPUT(coords);
-
- return corr_cuda_forward(fmap1, fmap2, coords, radius);
-}
-
-
-std::vector corr_backward(
- torch::Tensor fmap1,
- torch::Tensor fmap2,
- torch::Tensor coords,
- torch::Tensor corr_grad,
- int radius) {
- CHECK_INPUT(fmap1);
- CHECK_INPUT(fmap2);
- CHECK_INPUT(coords);
- CHECK_INPUT(corr_grad);
-
- return corr_cuda_backward(fmap1, fmap2, coords, corr_grad, radius);
-}
-
-
-PYBIND11_MODULE(TORCH_EXTENSION_NAME, m) {
- m.def("forward", &corr_forward, "CORR forward");
- m.def("backward", &corr_backward, "CORR backward");
-}
\ No newline at end of file
diff --git a/spaces/Pavankunchala/Depth-Estimation-App/app.py b/spaces/Pavankunchala/Depth-Estimation-App/app.py
deleted file mode 100644
index 66fc9bf16756c12b85673b290dcd7830ab95b12d..0000000000000000000000000000000000000000
--- a/spaces/Pavankunchala/Depth-Estimation-App/app.py
+++ /dev/null
@@ -1,146 +0,0 @@
-import sys
-import time
-from pathlib import Path
-import cv2
-from openvino.inference_engine import IECore
-import matplotlib.cm
-import matplotlib.pyplot as plt
-import numpy as np
-import streamlit as st
-from PIL import Image
-import tempfile
-DEMO_IMAGE = 'dog-new.jpg'
-DEMO_VIDEO = 'dance2.mp4'
-@st.cache
-def normalize_minmax(data):
-
- return (data - data.min()) / (data.max() - data.min())
-@st.cache
-def convert_result_to_image(result, colormap="inferno"):
-
- cmap = matplotlib.cm.get_cmap(colormap)
- result = result.squeeze(0)
- result = normalize_minmax(result)
- result = cmap(result)[:, :, :3] * 255
- result = result.astype(np.uint8)
- return result
-@st.cache
-def to_rgb(image_data) -> np.ndarray:
-
- return cv2.cvtColor(image_data, cv2.COLOR_BGR2RGB)
-st.title("Depth Estimation App")
-st.sidebar.title('Depth Estimation')
-st.sidebar.subheader('Parameters')
-DEVICE = "CPU"
-MODEL_FILE = "models/MiDaS_small.xml"
-model_xml_path = Path(MODEL_FILE)
-ie = IECore()
-net = ie.read_network(model=model_xml_path, weights=model_xml_path.with_suffix(".bin"))
-exec_net = ie.load_network(network=net, device_name=DEVICE)
-input_key = list(exec_net.input_info)[0]
-output_key = list(exec_net.outputs.keys())[0]
-network_input_shape = exec_net.input_info[input_key].tensor_desc.dims
-network_image_height, network_image_width = network_input_shape[2:]
-app_mode = st.sidebar.selectbox('Choose the App mode',
-['Run on Image','Run on Video'],index = 0)
-if app_mode == "Run on Image":
- st.markdown('Running on Image')
- st.sidebar.text('Params for Image')
- st.markdown(
- """
-
- """,
- unsafe_allow_html=True,
- )
- img_file_buffer = st.sidebar.file_uploader("Upload an image", type=[ "jpg", "jpeg",'png'])
- if img_file_buffer is not None:
- image = np.array(Image.open(img_file_buffer))
- else:
- demo_image = DEMO_IMAGE
- image = np.array(Image.open(demo_image))
- st.sidebar.text('Original Image')
- st.sidebar.image(image)
- resized_image = cv2.resize(src=image, dsize=(network_image_height, network_image_width))
- # reshape image to network input shape NCHW
- input_image = np.expand_dims(np.transpose(resized_image, (2, 0, 1)), 0)
- result = exec_net.infer(inputs={input_key: input_image})[output_key]
- # convert network result of disparity map to an image that shows
- # distance as colors
- result_image = convert_result_to_image(result=result)
- # resize back to original image shape. cv2.resize expects shape
- # in (width, height), [::-1] reverses the (height, width) shape to match this.
- result_image = cv2.resize(result_image, image.shape[:2][::-1])
- st.subheader('Output Image')
- st.image(result_image,use_column_width= True)
-if app_mode =='Run on Video':
- st.markdown('Running on Video')
-
- video_file_buffer = st.sidebar.file_uploader("Upload a video", type=[ "mp4", "mov",'avi','asf', 'm4v' ])
- tfflie = tempfile.NamedTemporaryFile(delete=False)
- stop_button = st.sidebar.button('Stop Processing')
- if stop_button:
- st.stop()
- if not video_file_buffer:
-
- vid = cv2.VideoCapture(DEMO_VIDEO)
- tfflie.name = DEMO_VIDEO
-
-
- else:
- tfflie.write(video_file_buffer.read())
- vid = cv2.VideoCapture(tfflie.name)
-
- width = int(vid.get(cv2.CAP_PROP_FRAME_WIDTH))
- height = int(vid.get(cv2.CAP_PROP_FRAME_HEIGHT))
- fps = int(vid.get(cv2.CAP_PROP_FPS))#codec = cv2.VideoWriter_fourcc(*FLAGS.output_format)
- codec = cv2.VideoWriter_fourcc('X','V','I','D')
- out = cv2.VideoWriter('output_depth.mp4', codec, fps, (width, height))
- start_time = time.perf_counter()
- total_inference_duration = 0
- stframe = st.empty()
- SCALE_OUTPUT = 1
- st.markdown("**Frame Rate**")
- kpi1_text = st.markdown("0")
- save_video = st.checkbox('Save video')
- while vid.isOpened():
- ret, image = vid.read()
- new_time = time.time()
- input_video_frame_height, input_video_frame_width = image.shape[:2]
- target_frame_height = int(input_video_frame_height * SCALE_OUTPUT)
- target_frame_width = int(input_video_frame_width * SCALE_OUTPUT)
- if not ret:
- vid.release()
- break
- resized_image = cv2.resize(src=image, dsize=(network_image_height, network_image_width))
- # reshape image to network input shape NCHW
- input_image = np.expand_dims(np.transpose(resized_image, (2, 0, 1)), 0)
- inference_start_time = time.perf_counter()
- result = exec_net.infer(inputs={input_key: input_image})[output_key]
- inference_stop_time = time.perf_counter()
- inference_duration = inference_stop_time - inference_start_time
- total_inference_duration += inference_duration
- result_frame = to_rgb(convert_result_to_image(result))
- # Resize image and result to target frame shape
- result_frame = cv2.resize(result_frame, (target_frame_width, target_frame_height))
- image = cv2.resize(image, (target_frame_width, target_frame_height))
- # Put image and result side by side
- stacked_frame = np.hstack((image, result_frame))
- if save_video:
- out.write(stacked_frame)
- stframe.image(stacked_frame,channels = 'BGR',use_column_width=True)
- fps = 1.0/(time.time() - new_time)
- kpi1_text.write(f"{'{:.1f}'.format(fps)} ", unsafe_allow_html=True)
-
- vid.release()
- out.release()
- cv2.destroyAllWindows()
- st.success('Video is Processed')
- st.stop()
\ No newline at end of file
diff --git a/spaces/Plurigrid/LifeSim/src/components/ui/collapsible.tsx b/spaces/Plurigrid/LifeSim/src/components/ui/collapsible.tsx
deleted file mode 100644
index 9fa48946afd1eb56bd932377fd888e3986304676..0000000000000000000000000000000000000000
--- a/spaces/Plurigrid/LifeSim/src/components/ui/collapsible.tsx
+++ /dev/null
@@ -1,11 +0,0 @@
-"use client"
-
-import * as CollapsiblePrimitive from "@radix-ui/react-collapsible"
-
-const Collapsible = CollapsiblePrimitive.Root
-
-const CollapsibleTrigger = CollapsiblePrimitive.CollapsibleTrigger
-
-const CollapsibleContent = CollapsiblePrimitive.CollapsibleContent
-
-export { Collapsible, CollapsibleTrigger, CollapsibleContent }
diff --git a/spaces/Podtekatel/ArcaneSVK2/inference/model_pipeline.py b/spaces/Podtekatel/ArcaneSVK2/inference/model_pipeline.py
deleted file mode 100644
index d03117f9e420367e0733f64ff046c178f147bfbe..0000000000000000000000000000000000000000
--- a/spaces/Podtekatel/ArcaneSVK2/inference/model_pipeline.py
+++ /dev/null
@@ -1,115 +0,0 @@
-import logging
-import time
-
-import cv2
-import numpy as np
-
-from .center_crop import center_crop
-from .face_detector import FaceDetector
-
-
-class VSNetModelPipeline:
- def __init__(self, model, face_detector: FaceDetector, background_resize=720, no_detected_resize=256, use_cloning=True):
- self.background_resize = background_resize
- self.no_detected_resize = no_detected_resize
- self.model = model
- self.face_detector = face_detector
- self.mask = self.create_circular_mask(face_detector.target_size, face_detector.target_size)
- self.use_cloning = use_cloning
-
- @staticmethod
- def create_circular_mask(h, w, power=None, clipping_coef=0.85):
- center = (int(w / 2), int(h / 2))
-
- Y, X = np.ogrid[:h, :w]
- dist_from_center = np.sqrt((X - center[0]) ** 2 + (Y - center[1]) ** 2)
- print(dist_from_center.max(), dist_from_center.min())
- clipping_radius = min((h - center[0]), (w - center[1])) * clipping_coef
- max_size = max((h - center[0]), (w - center[1]))
- dist_from_center[dist_from_center < clipping_radius] = clipping_radius
- dist_from_center[dist_from_center > max_size] = max_size
- max_distance, min_distance = np.max(dist_from_center), np.min(dist_from_center)
- dist_from_center = 1 - (dist_from_center - min_distance) / (max_distance - min_distance)
- if power is not None:
- dist_from_center = np.power(dist_from_center, power)
- dist_from_center = np.stack([dist_from_center] * 3, axis=2)
- # mask = dist_from_center <= radius
- return dist_from_center
-
-
- @staticmethod
- def resize_size(image, size=720, always_apply=True):
- h, w, c = np.shape(image)
- if min(h, w) > size or always_apply:
- if h < w:
- h, w = int(size * h / w), size
- else:
- h, w = size, int(size * w / h)
- image = cv2.resize(image, (w, h), interpolation=cv2.INTER_AREA)
- return image
-
- def normalize(self, img):
- img = img.astype(np.float32) / 255 * 2 - 1
- return img
-
- def denormalize(self, img):
- return (img + 1) / 2
-
- def divide_crop(self, img, must_divided=32):
- h, w, _ = img.shape
- h = h // must_divided * must_divided
- w = w // must_divided * must_divided
-
- img = center_crop(img, h, w)
- return img
-
- def merge_crops(self, faces_imgs, crops, full_image):
- for face, crop in zip(faces_imgs, crops):
- x1, y1, x2, y2 = crop
- W, H = x2 - x1, y2 - y1
- result_face = cv2.resize(face, (W, H), interpolation=cv2.INTER_LINEAR)
- face_mask = cv2.resize(self.mask, (W, H), interpolation=cv2.INTER_LINEAR)
- if self.use_cloning:
- center = round((x2 + x1) / 2), round((y2 + y1) / 2)
- full_image = cv2.seamlessClone(result_face, full_image, (face_mask > 0.0).astype(np.uint8) * 255, center, cv2.NORMAL_CLONE)
- else:
- input_face = full_image[y1: y2, x1: x2]
- full_image[y1: y2, x1: x2] = (result_face * face_mask + input_face * (1 - face_mask)).astype(np.uint8)
- return full_image
-
- def __call__(self, img):
- return self.process_image(img)
-
- def process_image(self, img):
- img = self.resize_size(img, size=self.background_resize)
- img = self.divide_crop(img)
-
- face_crops, coords = self.face_detector(img)
-
- if len(face_crops) > 0:
- start_time = time.time()
- faces = self.normalize(face_crops)
- faces = faces.transpose(0, 3, 1, 2)
- out_faces = self.model(faces)
- out_faces = self.denormalize(out_faces)
- out_faces = out_faces.transpose(0, 2, 3, 1)
- out_faces = np.clip(out_faces * 255, 0, 255).astype(np.uint8)
- end_time = time.time()
- logging.info(f'Face FPS {1 / (end_time - start_time)}')
- else:
- out_faces = []
- img = self.resize_size(img, size=self.no_detected_resize)
- img = self.divide_crop(img)
-
- start_time = time.time()
- full_image = self.normalize(img)
- full_image = np.expand_dims(full_image, 0).transpose(0, 3, 1, 2)
- full_image = self.model(full_image)
- full_image = self.denormalize(full_image)
- full_image = full_image.transpose(0, 2, 3, 1)
- full_image = np.clip(full_image * 255, 0, 255).astype(np.uint8)
- end_time = time.time()
- logging.info(f'Background FPS {1 / (end_time - start_time)}')
-
- result_image = self.merge_crops(out_faces, coords, full_image[0])
- return result_image
diff --git a/spaces/Pranjal12345/Text_to_Speech/tortoise/read.py b/spaces/Pranjal12345/Text_to_Speech/tortoise/read.py
deleted file mode 100644
index e5839aa89522d4770ab3f53ef2aca5b7eb7eac84..0000000000000000000000000000000000000000
--- a/spaces/Pranjal12345/Text_to_Speech/tortoise/read.py
+++ /dev/null
@@ -1,101 +0,0 @@
-import argparse
-import os
-from time import time
-
-import torch
-import torchaudio
-
-from api import TextToSpeech, MODELS_DIR
-from utils.audio import load_audio, load_voices
-from utils.text import split_and_recombine_text
-
-
-if __name__ == '__main__':
- parser = argparse.ArgumentParser()
- parser.add_argument('--textfile', type=str, help='A file containing the text to read.', default="tortoise/data/riding_hood.txt")
- parser.add_argument('--voice', type=str, help='Selects the voice to use for generation. See options in voices/ directory (and add your own!) '
- 'Use the & character to join two voices together. Use a comma to perform inference on multiple voices.', default='pat')
- parser.add_argument('--output_path', type=str, help='Where to store outputs.', default='results/longform/')
- parser.add_argument('--output_name', type=str, help='How to name the output file', default='combined.wav')
- parser.add_argument('--preset', type=str, help='Which voice preset to use.', default='standard')
- parser.add_argument('--regenerate', type=str, help='Comma-separated list of clip numbers to re-generate, or nothing.', default=None)
- parser.add_argument('--candidates', type=int, help='How many output candidates to produce per-voice. Only the first candidate is actually used in the final product, the others can be used manually.', default=1)
- parser.add_argument('--model_dir', type=str, help='Where to find pretrained model checkpoints. Tortoise automatically downloads these to .models, so this'
- 'should only be specified if you have custom checkpoints.', default=MODELS_DIR)
- parser.add_argument('--seed', type=int, help='Random seed which can be used to reproduce results.', default=None)
- parser.add_argument('--produce_debug_state', type=bool, help='Whether or not to produce debug_state.pth, which can aid in reproducing problems. Defaults to true.', default=True)
- parser.add_argument('--use_deepspeed', type=bool, help='Use deepspeed for speed bump.', default=False)
- parser.add_argument('--kv_cache', type=bool, help='If you disable this please wait for a long a time to get the output', default=True)
- parser.add_argument('--half', type=bool, help="float16(half) precision inference if True it's faster and take less vram and ram", default=True)
-
-
- args = parser.parse_args()
- if torch.backends.mps.is_available():
- args.use_deepspeed = False
- tts = TextToSpeech(models_dir=args.model_dir, use_deepspeed=args.use_deepspeed, kv_cache=args.kv_cache, half=args.half)
-
- outpath = args.output_path
- outname = args.output_name
- selected_voices = args.voice.split(',')
- regenerate = args.regenerate
- if regenerate is not None:
- regenerate = [int(e) for e in regenerate.split(',')]
-
- # Process text
- with open(args.textfile, 'r', encoding='utf-8') as f:
- text = ' '.join([l for l in f.readlines()])
- if '|' in text:
- print("Found the '|' character in your text, which I will use as a cue for where to split it up. If this was not"
- "your intent, please remove all '|' characters from the input.")
- texts = text.split('|')
- else:
- texts = split_and_recombine_text(text)
-
- seed = int(time()) if args.seed is None else args.seed
- for selected_voice in selected_voices:
- voice_outpath = os.path.join(outpath, selected_voice)
- os.makedirs(voice_outpath, exist_ok=True)
-
- if '&' in selected_voice:
- voice_sel = selected_voice.split('&')
- else:
- voice_sel = [selected_voice]
-
- voice_samples, conditioning_latents = load_voices(voice_sel)
- all_parts = []
- for j, text in enumerate(texts):
- if regenerate is not None and j not in regenerate:
- all_parts.append(load_audio(os.path.join(voice_outpath, f'{j}.wav'), 24000))
- continue
- gen = tts.tts_with_preset(text, voice_samples=voice_samples, conditioning_latents=conditioning_latents,
- preset=args.preset, k=args.candidates, use_deterministic_seed=seed)
- if args.candidates == 1:
- audio_ = gen.squeeze(0).cpu()
- torchaudio.save(os.path.join(voice_outpath, f'{j}.wav'), audio_, 24000)
- else:
- candidate_dir = os.path.join(voice_outpath, str(j))
- os.makedirs(candidate_dir, exist_ok=True)
- for k, g in enumerate(gen):
- torchaudio.save(os.path.join(candidate_dir, f'{k}.wav'), g.squeeze(0).cpu(), 24000)
- audio_ = gen[0].squeeze(0).cpu()
- all_parts.append(audio_)
-
- if args.candidates == 1:
- full_audio = torch.cat(all_parts, dim=-1)
- torchaudio.save(os.path.join(voice_outpath, f"{outname}.wav"), full_audio, 24000)
-
- if args.produce_debug_state:
- os.makedirs('debug_states', exist_ok=True)
- dbg_state = (seed, texts, voice_samples, conditioning_latents)
- torch.save(dbg_state, f'debug_states/read_debug_{selected_voice}.pth')
-
- # Combine each candidate's audio clips.
- if args.candidates > 1:
- audio_clips = []
- for candidate in range(args.candidates):
- for line in range(len(texts)):
- wav_file = os.path.join(voice_outpath, str(line), f"{candidate}.wav")
- audio_clips.append(load_audio(wav_file, 24000))
- audio_clips = torch.cat(audio_clips, dim=-1)
- torchaudio.save(os.path.join(voice_outpath, f"{outname}_{candidate:02d}.wav"), audio_clips, 24000)
- audio_clips = []
diff --git a/spaces/RMXK/RVC_HFF/gui_v1.py b/spaces/RMXK/RVC_HFF/gui_v1.py
deleted file mode 100644
index becba80cdda6987c1ad70c89e68a4e3a4da44639..0000000000000000000000000000000000000000
--- a/spaces/RMXK/RVC_HFF/gui_v1.py
+++ /dev/null
@@ -1,708 +0,0 @@
-import os
-import logging
-import sys
-from dotenv import load_dotenv
-
-load_dotenv()
-
-os.environ["OMP_NUM_THREADS"] = "4"
-if sys.platform == "darwin":
- os.environ["PYTORCH_ENABLE_MPS_FALLBACK"] = "1"
-
-now_dir = os.getcwd()
-sys.path.append(now_dir)
-import multiprocessing
-
-logger = logging.getLogger(__name__)
-
-
-class Harvest(multiprocessing.Process):
- def __init__(self, inp_q, opt_q):
- multiprocessing.Process.__init__(self)
- self.inp_q = inp_q
- self.opt_q = opt_q
-
- def run(self):
- import numpy as np
- import pyworld
-
- while 1:
- idx, x, res_f0, n_cpu, ts = self.inp_q.get()
- f0, t = pyworld.harvest(
- x.astype(np.double),
- fs=16000,
- f0_ceil=1100,
- f0_floor=50,
- frame_period=10,
- )
- res_f0[idx] = f0
- if len(res_f0.keys()) >= n_cpu:
- self.opt_q.put(ts)
-
-
-if __name__ == "__main__":
- import json
- import multiprocessing
- import re
- import threading
- import time
- import traceback
- from multiprocessing import Queue, cpu_count
- from queue import Empty
-
- import librosa
- from tools.torchgate import TorchGate
- import numpy as np
- import PySimpleGUI as sg
- import sounddevice as sd
- import torch
- import torch.nn.functional as F
- import torchaudio.transforms as tat
-
- import tools.rvc_for_realtime as rvc_for_realtime
- from i18n.i18n import I18nAuto
-
- i18n = I18nAuto()
- device = rvc_for_realtime.config.device
- # device = torch.device(
- # "cuda"
- # if torch.cuda.is_available()
- # else ("mps" if torch.backends.mps.is_available() else "cpu")
- # )
- current_dir = os.getcwd()
- inp_q = Queue()
- opt_q = Queue()
- n_cpu = min(cpu_count(), 8)
- for _ in range(n_cpu):
- Harvest(inp_q, opt_q).start()
-
- class GUIConfig:
- def __init__(self) -> None:
- self.pth_path: str = ""
- self.index_path: str = ""
- self.pitch: int = 0
- self.samplerate: int = 40000
- self.block_time: float = 1.0 # s
- self.buffer_num: int = 1
- self.threhold: int = -60
- self.crossfade_time: float = 0.04
- self.extra_time: float = 2.0
- self.I_noise_reduce = False
- self.O_noise_reduce = False
- self.rms_mix_rate = 0.0
- self.index_rate = 0.3
- self.n_cpu = min(n_cpu, 6)
- self.f0method = "harvest"
- self.sg_input_device = ""
- self.sg_output_device = ""
-
- class GUI:
- def __init__(self) -> None:
- self.config = GUIConfig()
- self.flag_vc = False
-
- self.launcher()
-
- def load(self):
- input_devices, output_devices, _, _ = self.get_devices()
- try:
- with open("configs/config.json", "r") as j:
- data = json.load(j)
- data["pm"] = data["f0method"] == "pm"
- data["harvest"] = data["f0method"] == "harvest"
- data["crepe"] = data["f0method"] == "crepe"
- data["rmvpe"] = data["f0method"] == "rmvpe"
- except:
- with open("configs/config.json", "w") as j:
- data = {
- "pth_path": " ",
- "index_path": " ",
- "sg_input_device": input_devices[sd.default.device[0]],
- "sg_output_device": output_devices[sd.default.device[1]],
- "threhold": "-60",
- "pitch": "0",
- "index_rate": "0",
- "rms_mix_rate": "0",
- "block_time": "0.25",
- "crossfade_length": "0.04",
- "extra_time": "2",
- "f0method": "rmvpe",
- }
- data["pm"] = data["f0method"] == "pm"
- data["harvest"] = data["f0method"] == "harvest"
- data["crepe"] = data["f0method"] == "crepe"
- data["rmvpe"] = data["f0method"] == "rmvpe"
- return data
-
- def launcher(self):
- data = self.load()
- sg.theme("LightBlue3")
- input_devices, output_devices, _, _ = self.get_devices()
- layout = [
- [
- sg.Frame(
- title=i18n("加载模型"),
- layout=[
- [
- sg.Input(
- default_text=data.get("pth_path", ""),
- key="pth_path",
- ),
- sg.FileBrowse(
- i18n("选择.pth文件"),
- initial_folder=os.path.join(
- os.getcwd(), "assets/weights"
- ),
- file_types=((". pth"),),
- ),
- ],
- [
- sg.Input(
- default_text=data.get("index_path", ""),
- key="index_path",
- ),
- sg.FileBrowse(
- i18n("选择.index文件"),
- initial_folder=os.path.join(os.getcwd(), "logs"),
- file_types=((". index"),),
- ),
- ],
- ],
- )
- ],
- [
- sg.Frame(
- layout=[
- [
- sg.Text(i18n("输入设备")),
- sg.Combo(
- input_devices,
- key="sg_input_device",
- default_value=data.get("sg_input_device", ""),
- ),
- ],
- [
- sg.Text(i18n("输出设备")),
- sg.Combo(
- output_devices,
- key="sg_output_device",
- default_value=data.get("sg_output_device", ""),
- ),
- ],
- [sg.Button(i18n("重载设备列表"), key="reload_devices")],
- ],
- title=i18n("音频设备(请使用同种类驱动)"),
- )
- ],
- [
- sg.Frame(
- layout=[
- [
- sg.Text(i18n("响应阈值")),
- sg.Slider(
- range=(-60, 0),
- key="threhold",
- resolution=1,
- orientation="h",
- default_value=data.get("threhold", "-60"),
- enable_events=True,
- ),
- ],
- [
- sg.Text(i18n("音调设置")),
- sg.Slider(
- range=(-24, 24),
- key="pitch",
- resolution=1,
- orientation="h",
- default_value=data.get("pitch", "0"),
- enable_events=True,
- ),
- ],
- [
- sg.Text(i18n("Index Rate")),
- sg.Slider(
- range=(0.0, 1.0),
- key="index_rate",
- resolution=0.01,
- orientation="h",
- default_value=data.get("index_rate", "0"),
- enable_events=True,
- ),
- ],
- [
- sg.Text(i18n("响度因子")),
- sg.Slider(
- range=(0.0, 1.0),
- key="rms_mix_rate",
- resolution=0.01,
- orientation="h",
- default_value=data.get("rms_mix_rate", "0"),
- enable_events=True,
- ),
- ],
- [
- sg.Text(i18n("音高算法")),
- sg.Radio(
- "pm",
- "f0method",
- key="pm",
- default=data.get("pm", "") == True,
- enable_events=True,
- ),
- sg.Radio(
- "harvest",
- "f0method",
- key="harvest",
- default=data.get("harvest", "") == True,
- enable_events=True,
- ),
- sg.Radio(
- "crepe",
- "f0method",
- key="crepe",
- default=data.get("crepe", "") == True,
- enable_events=True,
- ),
- sg.Radio(
- "rmvpe",
- "f0method",
- key="rmvpe",
- default=data.get("rmvpe", "") == True,
- enable_events=True,
- ),
- ],
- ],
- title=i18n("常规设置"),
- ),
- sg.Frame(
- layout=[
- [
- sg.Text(i18n("采样长度")),
- sg.Slider(
- range=(0.05, 2.4),
- key="block_time",
- resolution=0.01,
- orientation="h",
- default_value=data.get("block_time", "0.25"),
- enable_events=True,
- ),
- ],
- [
- sg.Text(i18n("harvest进程数")),
- sg.Slider(
- range=(1, n_cpu),
- key="n_cpu",
- resolution=1,
- orientation="h",
- default_value=data.get(
- "n_cpu", min(self.config.n_cpu, n_cpu)
- ),
- enable_events=True,
- ),
- ],
- [
- sg.Text(i18n("淡入淡出长度")),
- sg.Slider(
- range=(0.01, 0.15),
- key="crossfade_length",
- resolution=0.01,
- orientation="h",
- default_value=data.get("crossfade_length", "0.04"),
- enable_events=True,
- ),
- ],
- [
- sg.Text(i18n("额外推理时长")),
- sg.Slider(
- range=(0.05, 5.00),
- key="extra_time",
- resolution=0.01,
- orientation="h",
- default_value=data.get("extra_time", "2.0"),
- enable_events=True,
- ),
- ],
- [
- sg.Checkbox(
- i18n("输入降噪"),
- key="I_noise_reduce",
- enable_events=True,
- ),
- sg.Checkbox(
- i18n("输出降噪"),
- key="O_noise_reduce",
- enable_events=True,
- ),
- ],
- ],
- title=i18n("性能设置"),
- ),
- ],
- [
- sg.Button(i18n("开始音频转换"), key="start_vc"),
- sg.Button(i18n("停止音频转换"), key="stop_vc"),
- sg.Text(i18n("推理时间(ms):")),
- sg.Text("0", key="infer_time"),
- ],
- ]
- self.window = sg.Window("RVC - GUI", layout=layout, finalize=True)
- self.event_handler()
-
- def event_handler(self):
- while True:
- event, values = self.window.read()
- if event == sg.WINDOW_CLOSED:
- self.flag_vc = False
- exit()
- if event == "reload_devices":
- prev_input = self.window["sg_input_device"].get()
- prev_output = self.window["sg_output_device"].get()
- input_devices, output_devices, _, _ = self.get_devices(update=True)
- if prev_input not in input_devices:
- self.config.sg_input_device = input_devices[0]
- else:
- self.config.sg_input_device = prev_input
- self.window["sg_input_device"].Update(values=input_devices)
- self.window["sg_input_device"].Update(
- value=self.config.sg_input_device
- )
- if prev_output not in output_devices:
- self.config.sg_output_device = output_devices[0]
- else:
- self.config.sg_output_device = prev_output
- self.window["sg_output_device"].Update(values=output_devices)
- self.window["sg_output_device"].Update(
- value=self.config.sg_output_device
- )
- if event == "start_vc" and self.flag_vc == False:
- if self.set_values(values) == True:
- logger.info("Use CUDA: %s", torch.cuda.is_available())
- self.start_vc()
- settings = {
- "pth_path": values["pth_path"],
- "index_path": values["index_path"],
- "sg_input_device": values["sg_input_device"],
- "sg_output_device": values["sg_output_device"],
- "threhold": values["threhold"],
- "pitch": values["pitch"],
- "rms_mix_rate": values["rms_mix_rate"],
- "index_rate": values["index_rate"],
- "block_time": values["block_time"],
- "crossfade_length": values["crossfade_length"],
- "extra_time": values["extra_time"],
- "n_cpu": values["n_cpu"],
- "f0method": ["pm", "harvest", "crepe", "rmvpe"][
- [
- values["pm"],
- values["harvest"],
- values["crepe"],
- values["rmvpe"],
- ].index(True)
- ],
- }
- with open("configs/config.json", "w") as j:
- json.dump(settings, j)
- if event == "stop_vc" and self.flag_vc == True:
- self.flag_vc = False
-
- # Parameter hot update
- if event == "threhold":
- self.config.threhold = values["threhold"]
- elif event == "pitch":
- self.config.pitch = values["pitch"]
- if hasattr(self, "rvc"):
- self.rvc.change_key(values["pitch"])
- elif event == "index_rate":
- self.config.index_rate = values["index_rate"]
- if hasattr(self, "rvc"):
- self.rvc.change_index_rate(values["index_rate"])
- elif event == "rms_mix_rate":
- self.config.rms_mix_rate = values["rms_mix_rate"]
- elif event in ["pm", "harvest", "crepe", "rmvpe"]:
- self.config.f0method = event
- elif event == "I_noise_reduce":
- self.config.I_noise_reduce = values["I_noise_reduce"]
- elif event == "O_noise_reduce":
- self.config.O_noise_reduce = values["O_noise_reduce"]
- elif event != "start_vc" and self.flag_vc == True:
- # Other parameters do not support hot update
- self.flag_vc = False
-
- def set_values(self, values):
- if len(values["pth_path"].strip()) == 0:
- sg.popup(i18n("请选择pth文件"))
- return False
- if len(values["index_path"].strip()) == 0:
- sg.popup(i18n("请选择index文件"))
- return False
- pattern = re.compile("[^\x00-\x7F]+")
- if pattern.findall(values["pth_path"]):
- sg.popup(i18n("pth文件路径不可包含中文"))
- return False
- if pattern.findall(values["index_path"]):
- sg.popup(i18n("index文件路径不可包含中文"))
- return False
- self.set_devices(values["sg_input_device"], values["sg_output_device"])
- self.config.pth_path = values["pth_path"]
- self.config.index_path = values["index_path"]
- self.config.threhold = values["threhold"]
- self.config.pitch = values["pitch"]
- self.config.block_time = values["block_time"]
- self.config.crossfade_time = values["crossfade_length"]
- self.config.extra_time = values["extra_time"]
- self.config.I_noise_reduce = values["I_noise_reduce"]
- self.config.O_noise_reduce = values["O_noise_reduce"]
- self.config.rms_mix_rate = values["rms_mix_rate"]
- self.config.index_rate = values["index_rate"]
- self.config.n_cpu = values["n_cpu"]
- self.config.f0method = ["pm", "harvest", "crepe", "rmvpe"][
- [
- values["pm"],
- values["harvest"],
- values["crepe"],
- values["rmvpe"],
- ].index(True)
- ]
- return True
-
- def start_vc(self):
- torch.cuda.empty_cache()
- self.flag_vc = True
- self.rvc = rvc_for_realtime.RVC(
- self.config.pitch,
- self.config.pth_path,
- self.config.index_path,
- self.config.index_rate,
- self.config.n_cpu,
- inp_q,
- opt_q,
- device,
- self.rvc if hasattr(self, "rvc") else None
- )
- self.config.samplerate = self.rvc.tgt_sr
- self.zc = self.rvc.tgt_sr // 100
- self.block_frame = int(np.round(self.config.block_time * self.config.samplerate / self.zc)) * self.zc
- self.block_frame_16k = 160 * self.block_frame // self.zc
- self.crossfade_frame = int(np.round(self.config.crossfade_time * self.config.samplerate / self.zc)) * self.zc
- self.sola_search_frame = self.zc
- self.extra_frame = int(np.round(self.config.extra_time * self.config.samplerate / self.zc)) * self.zc
- self.input_wav: torch.Tensor = torch.zeros(
- self.extra_frame
- + self.crossfade_frame
- + self.sola_search_frame
- + self.block_frame,
- device=device,
- dtype=torch.float32,
- )
- self.input_wav_res: torch.Tensor= torch.zeros(160 * self.input_wav.shape[0] // self.zc, device=device,dtype=torch.float32)
- self.pitch: np.ndarray = np.zeros(
- self.input_wav.shape[0] // self.zc,
- dtype="int32",
- )
- self.pitchf: np.ndarray = np.zeros(
- self.input_wav.shape[0] // self.zc,
- dtype="float64",
- )
- self.sola_buffer: torch.Tensor = torch.zeros(
- self.crossfade_frame, device=device, dtype=torch.float32
- )
- self.nr_buffer: torch.Tensor = self.sola_buffer.clone()
- self.output_buffer: torch.Tensor = self.input_wav.clone()
- self.res_buffer: torch.Tensor = torch.zeros(2 * self.zc, device=device,dtype=torch.float32)
- self.valid_rate = 1 - (self.extra_frame - 1) / self.input_wav.shape[0]
- self.fade_in_window: torch.Tensor = (
- torch.sin(
- 0.5
- * np.pi
- * torch.linspace(
- 0.0,
- 1.0,
- steps=self.crossfade_frame,
- device=device,
- dtype=torch.float32,
- )
- )
- ** 2
- )
- self.fade_out_window: torch.Tensor = 1 - self.fade_in_window
- self.resampler = tat.Resample(
- orig_freq=self.config.samplerate, new_freq=16000, dtype=torch.float32
- ).to(device)
- self.tg = TorchGate(sr=self.config.samplerate, n_fft=4*self.zc, prop_decrease=0.9).to(device)
- thread_vc = threading.Thread(target=self.soundinput)
- thread_vc.start()
-
- def soundinput(self):
- """
- 接受音频输入
- """
- channels = 1 if sys.platform == "darwin" else 2
- with sd.Stream(
- channels=channels,
- callback=self.audio_callback,
- blocksize=self.block_frame,
- samplerate=self.config.samplerate,
- dtype="float32",
- ):
- while self.flag_vc:
- time.sleep(self.config.block_time)
- logger.debug("Audio block passed.")
- logger.debug("ENDing VC")
-
- def audio_callback(
- self, indata: np.ndarray, outdata: np.ndarray, frames, times, status
- ):
- """
- 音频处理
- """
- start_time = time.perf_counter()
- indata = librosa.to_mono(indata.T)
- if self.config.threhold > -60:
- rms = librosa.feature.rms(
- y=indata, frame_length=4*self.zc, hop_length=self.zc
- )
- db_threhold = (
- librosa.amplitude_to_db(rms, ref=1.0)[0] < self.config.threhold
- )
- for i in range(db_threhold.shape[0]):
- if db_threhold[i]:
- indata[i * self.zc : (i + 1) * self.zc] = 0
- self.input_wav[: -self.block_frame] = self.input_wav[self.block_frame :].clone()
- self.input_wav[-self.block_frame: ] = torch.from_numpy(indata).to(device)
- self.input_wav_res[ : -self.block_frame_16k] = self.input_wav_res[self.block_frame_16k :].clone()
- # input noise reduction and resampling
- if self.config.I_noise_reduce:
- input_wav = self.input_wav[-self.crossfade_frame -self.block_frame-2*self.zc: ]
- input_wav = self.tg(input_wav.unsqueeze(0), self.input_wav.unsqueeze(0))[0, 2*self.zc:]
- input_wav[: self.crossfade_frame] *= self.fade_in_window
- input_wav[: self.crossfade_frame] += self.nr_buffer * self.fade_out_window
- self.nr_buffer[:] = input_wav[-self.crossfade_frame: ]
- input_wav = torch.cat((self.res_buffer[:], input_wav[: self.block_frame]))
- self.res_buffer[:] = input_wav[-2*self.zc: ]
- self.input_wav_res[-self.block_frame_16k-160: ] = self.resampler(input_wav)[160: ]
- else:
- self.input_wav_res[-self.block_frame_16k-160: ] = self.resampler(self.input_wav[-self.block_frame-2*self.zc: ])[160: ]
- # infer
- f0_extractor_frame = self.block_frame_16k + 800
- if self.config.f0method == 'rmvpe':
- f0_extractor_frame = 5120 * ((f0_extractor_frame - 1) // 5120 + 1)
- infer_wav = self.rvc.infer(
- self.input_wav_res,
- self.input_wav_res[-f0_extractor_frame :].cpu().numpy(),
- self.block_frame_16k,
- self.valid_rate,
- self.pitch,
- self.pitchf,
- self.config.f0method,
- )
- infer_wav = infer_wav[
- -self.crossfade_frame - self.sola_search_frame - self.block_frame :
- ]
- # output noise reduction
- if self.config.O_noise_reduce:
- self.output_buffer[: -self.block_frame] = self.output_buffer[self.block_frame :].clone()
- self.output_buffer[-self.block_frame: ] = infer_wav[-self.block_frame:]
- infer_wav = self.tg(infer_wav.unsqueeze(0), self.output_buffer.unsqueeze(0)).squeeze(0)
- # volume envelop mixing
- if self.config.rms_mix_rate < 1:
- rms1 = librosa.feature.rms(
- y=self.input_wav_res[-160*infer_wav.shape[0]//self.zc :].cpu().numpy(),
- frame_length=640,
- hop_length=160,
- )
- rms1 = torch.from_numpy(rms1).to(device)
- rms1 = F.interpolate(
- rms1.unsqueeze(0), size=infer_wav.shape[0] + 1, mode="linear",align_corners=True,
- )[0,0,:-1]
- rms2 = librosa.feature.rms(
- y=infer_wav[:].cpu().numpy(), frame_length=4*self.zc, hop_length=self.zc
- )
- rms2 = torch.from_numpy(rms2).to(device)
- rms2 = F.interpolate(
- rms2.unsqueeze(0), size=infer_wav.shape[0] + 1, mode="linear",align_corners=True,
- )[0,0,:-1]
- rms2 = torch.max(rms2, torch.zeros_like(rms2) + 1e-3)
- infer_wav *= torch.pow(rms1 / rms2, torch.tensor(1 - self.config.rms_mix_rate))
- # SOLA algorithm from https://github.com/yxlllc/DDSP-SVC
- conv_input = infer_wav[None, None, : self.crossfade_frame + self.sola_search_frame]
- cor_nom = F.conv1d(conv_input, self.sola_buffer[None, None, :])
- cor_den = torch.sqrt(
- F.conv1d(conv_input ** 2, torch.ones(1, 1, self.crossfade_frame, device=device)) + 1e-8)
- if sys.platform == "darwin":
- _, sola_offset = torch.max(cor_nom[0, 0] / cor_den[0, 0])
- sola_offset = sola_offset.item()
- else:
- sola_offset = torch.argmax(cor_nom[0, 0] / cor_den[0, 0])
- logger.debug("sola_offset = %d", int(sola_offset))
- infer_wav = infer_wav[sola_offset: sola_offset + self.block_frame + self.crossfade_frame]
- infer_wav[: self.crossfade_frame] *= self.fade_in_window
- infer_wav[: self.crossfade_frame] += self.sola_buffer *self.fade_out_window
- self.sola_buffer[:] = infer_wav[-self.crossfade_frame:]
- if sys.platform == "darwin":
- outdata[:] = infer_wav[:-self.crossfade_frame].cpu().numpy()[:, np.newaxis]
- else:
- outdata[:] = infer_wav[:-self.crossfade_frame].repeat(2, 1).t().cpu().numpy()
- total_time = time.perf_counter() - start_time
- self.window["infer_time"].update(int(total_time * 1000))
- logger.info("Infer time: %.2f", total_time)
-
- def get_devices(self, update: bool = True):
- """获取设备列表"""
- if update:
- sd._terminate()
- sd._initialize()
- devices = sd.query_devices()
- hostapis = sd.query_hostapis()
- for hostapi in hostapis:
- for device_idx in hostapi["devices"]:
- devices[device_idx]["hostapi_name"] = hostapi["name"]
- input_devices = [
- f"{d['name']} ({d['hostapi_name']})"
- for d in devices
- if d["max_input_channels"] > 0
- ]
- output_devices = [
- f"{d['name']} ({d['hostapi_name']})"
- for d in devices
- if d["max_output_channels"] > 0
- ]
- input_devices_indices = [
- d["index"] if "index" in d else d["name"]
- for d in devices
- if d["max_input_channels"] > 0
- ]
- output_devices_indices = [
- d["index"] if "index" in d else d["name"]
- for d in devices
- if d["max_output_channels"] > 0
- ]
- return (
- input_devices,
- output_devices,
- input_devices_indices,
- output_devices_indices,
- )
-
- def set_devices(self, input_device, output_device):
- """设置输出设备"""
- (
- input_devices,
- output_devices,
- input_device_indices,
- output_device_indices,
- ) = self.get_devices()
- sd.default.device[0] = input_device_indices[
- input_devices.index(input_device)
- ]
- sd.default.device[1] = output_device_indices[
- output_devices.index(output_device)
- ]
- logger.info(
- "Input device: %s:%s", str(sd.default.device[0]), input_device
- )
- logger.info(
- "Output device: %s:%s", str(sd.default.device[1]), output_device
- )
-
- gui = GUI()
\ No newline at end of file
diff --git a/spaces/RTLAI/BLIPsinki/app.py b/spaces/RTLAI/BLIPsinki/app.py
deleted file mode 100644
index bae7449f9dcbc0a2e5cebc21064df384b2f2b78a..0000000000000000000000000000000000000000
--- a/spaces/RTLAI/BLIPsinki/app.py
+++ /dev/null
@@ -1,56 +0,0 @@
-import numpy as np
-import requests
-import gradio as gr
-from transformers import pipeline
-
-
-
-def getModelPath(language):
- if language == 'English':
- path = None
- elif language == 'German':
- path = "Helsinki-NLP/opus-mt-en-de"
- elif language == 'French':
- path = "Helsinki-NLP/opus-mt-en-fr"
- elif language == 'Spanish':
- path = "Helsinki-NLP/opus-mt-en-es"
- elif language == 'Chinese':
- path = "Helsinki-NLP/opus-mt-en-zh"
- elif language == 'Ukranian':
- path = "Helsinki-NLP/opus-mt-en-uk"
- elif language == 'Swedish':
- path = "Helsinki-NLP/opus-mt-en-sv"
- elif language == 'Arabic':
- path = "Helsinki-NLP/opus-mt-en-ar"
- elif language == 'Italian':
- path = "Helsinki-NLP/opus-mt-en-it"
- elif language == 'Hindi':
- path = "Helsinki-NLP/opus-mt-en-hi"
- return(path)
-
-def blipsinki(input_img,strategy,language):
- b64_string = gr.processing_utils.encode_url_or_file_to_base64(input_img)
- response = requests.post(url='https://salesforce-blip.hf.space/api/predict', json={"data": [ b64_string,"Image Captioning","None",str(strategy)]})
- jres = response.json()
- print(jres)
-
- cap = jres["data"][0]
- modelpath = getModelPath(language)
- if modelpath:
- translator = pipeline("translation", model=modelpath)
- trans_cap = translator(cap)
- tc = trans_cap[0]['translation_text']
- return str(tc)
- else:
- return str(cap)
-
-description = "A pipeline of BLIP image captioning and Helsinki translation in order to generate image captions in a language of your choice either with beam search (deterministic) or nucleus sampling (stochastic). Enjoy! Is the language you want to use missing? Let me know and I'll integrate it."
-
-
-inputs_ = [gr.inputs.Image(type='filepath', label="Input Image"),gr.inputs.Radio(choices=['Beam search','Nucleus sampling'], type="value", default="Nucleus sampling", label="Mode"), gr.inputs.Radio(choices=['English','German', 'French', 'Spanish', 'Chinese', 'Ukranian', 'Swedish', 'Arabic', 'Italian', 'Hindi'],type="value", default = 'German',label="Language")]
-
-outputs_ = gr.outputs.Textbox(label="Output")
-
-iface = gr.Interface(blipsinki, inputs_, outputs_, description=description)
-
-iface.launch(debug=True,show_error=True)
\ No newline at end of file
diff --git a/spaces/Raspberry-ai/main/.env/lib/python3.11/site-packages/pip/_internal/utils/_log.py b/spaces/Raspberry-ai/main/.env/lib/python3.11/site-packages/pip/_internal/utils/_log.py
deleted file mode 100644
index 92c4c6a193873ce09629f6cfaa2dabc4f14ecb03..0000000000000000000000000000000000000000
--- a/spaces/Raspberry-ai/main/.env/lib/python3.11/site-packages/pip/_internal/utils/_log.py
+++ /dev/null
@@ -1,38 +0,0 @@
-"""Customize logging
-
-Defines custom logger class for the `logger.verbose(...)` method.
-
-init_logging() must be called before any other modules that call logging.getLogger.
-"""
-
-import logging
-from typing import Any, cast
-
-# custom log level for `--verbose` output
-# between DEBUG and INFO
-VERBOSE = 15
-
-
-class VerboseLogger(logging.Logger):
- """Custom Logger, defining a verbose log-level
-
- VERBOSE is between INFO and DEBUG.
- """
-
- def verbose(self, msg: str, *args: Any, **kwargs: Any) -> None:
- return self.log(VERBOSE, msg, *args, **kwargs)
-
-
-def getLogger(name: str) -> VerboseLogger:
- """logging.getLogger, but ensures our VerboseLogger class is returned"""
- return cast(VerboseLogger, logging.getLogger(name))
-
-
-def init_logging() -> None:
- """Register our VerboseLogger and VERBOSE log level.
-
- Should be called before any calls to getLogger(),
- i.e. in pip._internal.__init__
- """
- logging.setLoggerClass(VerboseLogger)
- logging.addLevelName(VERBOSE, "VERBOSE")
diff --git a/spaces/ReThGe/Linet/rethge_torch.py b/spaces/ReThGe/Linet/rethge_torch.py
deleted file mode 100644
index d01d648809c108af44eb09dfd3963158e68c6064..0000000000000000000000000000000000000000
--- a/spaces/ReThGe/Linet/rethge_torch.py
+++ /dev/null
@@ -1,1192 +0,0 @@
-## this file contains custom-code functions for pytorch deeplearning
-# containing model training/eval func, results/image plot func, and other help_funcs too
-# belongs to: rethge
-# created data: 2023/07/02
-
-
-## imports
-# torch related
-import torch
-from torch import nn
-import torchvision
-from torch.utils.data import DataLoader, Dataset
-from torchvision import datasets, transforms
-
-
-# data related
-
-import pandas as pd
-from PIL import Image
-import matplotlib.pyplot as plt
-
-from torchmetrics import ConfusionMatrix
-from mlxtend.plotting import plot_confusion_matrix
-
-# system related
-import os, gc
-import shutil
-import pathlib
-from pathlib import Path
-import random
-from typing import Tuple, Dict, List
-from timeit import default_timer as timer
-from tqdm.auto import tqdm
-
-
-
-# ————————————————————————————————————————————————————————————————————————————————————————————————————————————
-# utils related funcs
-def set_seeds(seed: int=42):
- """Sets random sets for torch operations.
-
- Args:
- seed (int, optional): Random seed to set. Defaults to 42.
- """
- torch.manual_seed(seed)
- torch.cuda.manual_seed(seed)
-
-
-def device_picking():
- """
- if GPU is available, using GPU, otherwise use CPU
- """
- device = torch.device('cuda' if torch.cuda.is_available() else 'cpu')
- print(f"Using {device} to DeepLearning")
- return device
-
-
-def check_cuda_cache_and_clean(clean: bool = False):
- """
- run a cuda mem checking, and clean cache when needed
- """
- os.environ["KMP_DUPLICATE_LIB_OK"] = "TRUE"
- os.environ["PYTORCH_CUDA_ALLOC_CONF"] = "max_split_size_mb: 128"
-
- cached_tensor = torch.cuda.memory_allocated() /1024/1024
- total_cached = torch.cuda.memory_reserved() /1024/1024
-
- print(f"current GPU memory occupied by tensors: {cached_tensor} Mb")
- print(f"current GPU memory managed by the caching allocator: {total_cached} Mb")
- print(f"rest GPU mem: {total_cached-cached_tensor} Mb\n")
-
- if clean:
- gc.collect()
- torch.cuda.empty_cache()
- cached_tensor = torch.cuda.memory_allocated() /1024/1024
- total_cached = torch.cuda.memory_reserved() /1024/1024
- print(f"GPU memory occupied by tensors after clean: {cached_tensor} Mb")
- print(f"GPU memory managed by the caching allocator after clean: {total_cached} Mb")
-# ————————————————————————————————————————————————————————————————————————————————————————————————————————————
-
-
-# ————————————————————————————————————————————————————————————————————————————————————————————————————————————
-# directory/file manipulate related funcs
-
-def walk_through_dir(dir_path: pathlib.Path):
- """
- know about your dataset dir
- """
-
- for dirpath, dirname, filenames in os.walk(dir_path):
- print(f"There are {len(dirname)} directories and {len(filenames)} images in '{dirpath}'.")
-
-
-def rename_get_rid_of_txt_suffix(working_dir: str):
-
- '''
- working dir should only exist the one type of file, and no folder
- '''
-
- os.chdir(working_dir)
- names=[]
- for i in os.listdir(working_dir):
- n = i.removesuffix('.txt')
- names.append(n)
-
- for i, j in enumerate(os.listdir(working_dir)):
- file_full_dir = f'{working_dir}\{j}'
- rename = f'{working_dir}\{names[i]}'
- os.rename(file_full_dir, rename)
-
-
-def rename_suffix(working_dir: str,
- suffix_to_add: str):
-
- """
- add suffix to all the file in a dir
- """
-
- for i in os.listdir(working_dir):
- file_full_dir = f'{working_dir}\{i}'
- rename = f'{file_full_dir}.{suffix_to_add}'
- os.rename(file_full_dir, rename)
-
-
-def copy_file_to_dir(working_dir: str,
- aim_dir: str):
-
- """copy all the file to a dir"""
-
- os.chdir(working_dir)
- for file in os.listdir():
- shutil.move(file, aim_dir)
-
-
-def remove_unused_label(image_dir: str,
- label_dir: str):
-
- """
- for object detection project data file management
- remove un-used label
- """
-
- label_dir_list = list(Path(label_dir).glob('*.*'))
- name_img = []
- count = 0
-
- for i in os.listdir(image_dir):
-
- n = i.removesuffix('.jpg')
- name_img.append(n)
-
- for names in label_dir_list:
- if names.stem not in name_img:
- os.remove(names)
- count += 1
- print(f"removed {count} unused labels")
-
-
-def find_missing_label(image_dir: str,
- label_dir: str) -> list:
-
- """
- for object detection project data file management
- find missed image label
- """
-
- # the stem name of label
- label_stem = []
- image_stem = []
- dir_missing_label = []
-
- for i in os.listdir(label_dir):
- if i == 'classes.txt':
- continue
- n = i.removesuffix('.txt')
- label_stem.append(n)
-
- for i in os.listdir(image_dir):
- if i == 'classes.txt':
- continue
- n = i.removesuffix('.jpg')
- image_stem.append(n)
-
-
- a = [x for x in image_stem if x not in label_stem]
- for i in a:
- suffix = '.jpg'
- i = f'{i}{suffix}'
- dir = f'{image_dir}\\{i}'
- dir_missing_label.append(Path(dir))
-
- print(f"missing {len(dir_missing_label)} label")
-
- return dir_missing_label
-
-
-def adding_nothing_label(image_dir: str,
- label_dir: str):
-
- """
- for object detection project data file management
- create empty txt file as 'nothing' label
- """
-
- label_name = []
- image_name = []
-
- for i in os.listdir(label_dir):
- if i == 'classes.txt':
- continue
-
- nl = i.removesuffix('.txt')
- label_name.append(nl)
-
- for i in os.listdir(image_dir):
- if i == 'classes.txt':
- continue
-
- nm = i.removesuffix('.jpg')
- image_name.append(nm)
-
- compare = [x for x in image_name if x not in label_name]
- print(f"missing {len(compare)} label\nimage number: {len(image_name)}\nlabel number: {len(label_name)}")
-
- for i in compare:
- suffix = '.txt'
- i = f'{i}{suffix}'
- dir = f'{label_dir}\\{i}'
-
- with open(dir, 'w') as fb:
- fb.close()
-
- if len(compare) == 0:
- print(f"No label is missing in {label_dir}")
- else:
- print(f"now having {len(os.listdir(label_dir))} files in folder")
-
-
-def find_classes(dir: str) -> Tuple[List[str], Dict[str, int]]:
- """
- find the class folder names in a target dir
-
- example:
- classname, class_dict = find_classes(dir) # [anode, cathode, nothing]
-
- """
-
- classes = sorted(entry.name for entry in os.scandir(dir) if entry.is_dir())
-
- if not classes:
- raise FileNotFoundError(f"Couldn't find any classes in {dir}... please check file structure")
-
- class_to_idx = {class_name: i for i, class_name in enumerate(classes)}
-
- return classes, class_to_idx
-
-
-# ————————————————————————————————————————————————————————————————————————————————————————————————————————————
-
-
-
-# ————————————————————————————————————————————————————————————————————————————————————————————————————————————
-# plot related funcs
-def plot_trans(img_path_list: List[str], # img_path_list = list(img_path.glob('*/*/*.jpg'))
- transform: torchvision.transforms,
- n: int = 3,
- seed=None):
- """
- select random img from a path list, and using transform, and visualize
-
- example:
- img_path_list = list(img_path.glob('*/*/*.jpg'))
- transform = transform.Compose([...])
- """
-
- if seed:
- random.seed(seed)
-
- random_img_path = random.sample(img_path_list, k=n)
- for p in random_img_path:
- with Image.open(p) as f:
- fig, ax = plt.subplots(nrows=1, ncols=2)
- ax[0].imshow(f)
- ax[0].set_title(f"Origin size: {f.size}")
- ax[0].axis(False)
-
- trans_img = transform(f).permute(1, 2, 0) # we need to change shape for plt
- # hwc -> hwc
- ax[1].imshow(trans_img)
- ax[1].set_title(f"transformed img_shape\n: {trans_img.shape}")
- ax[1].axis(False)
-
- fig.suptitle(f"Class name: {p.parent.stem}", fontsize=16)
-
-
-def display_random_img(dataset: torch.utils.data.Dataset,
- classes: List[str] = None,
- n: int = 10,
- display_shape: bool = True,
- seed: int = None):
- '''
- a func to display random img
-
- Args:
- classes: list of classname,
- n: numbers of img to show
- '''
-
- # nrow=2
-
- # if not n % 2:
- # ncol = int(n/2)+1
- # else:
- # ncol = int(n/2)
-
- if n > 10:
- n=10
- display_shape = False
- print(f"too many pics to display, max to 10 for display purpose")
-
- if seed:
- random.seed(seed)
-
- # get index of random samples
- random_samples_idx = random.sample(range(len(dataset)), k=n)
-
- plt.figure(figsize=(16,8))
-
- # loop through idx and plot
- for i, sample_idx in enumerate(random_samples_idx):
- image, label = dataset[sample_idx][0].permute(1,2,0), dataset[sample_idx][1]
-
- plt.subplot(1, n, i+1)
- plt.imshow(image)
- plt.axis(False)
-
- if classes:
- title = f"Class: {classes[label]}"
- if display_shape:
- title += f"\nshape: {image.shape}"
- plt.title(title)
-
-
-def plot_lr(results: Dict[str, List[float]] or Path):
- """
- this funcs plot a lr_scheduler's curve varying with epochs when a training is over
- """
-
- if type(results) != dict:
- results = pd.read_csv(results)
- results = results.iloc[:, 1:] # row, col
- results = results.to_dict("list")
-
- else:
- pass
-
- lr = results['learning rate']
- epochs = range(len(results['learning rate']))
-
- plt.figure(figsize=(7,7))
- plt.plot(epochs, lr, label='learning rate')
- plt.title('learning rate scheduler')
- plt.xlabel('Epochs')
- plt.legend()
-
-
-def plot_loss_curves(results: Dict[str, List[float]] or Path):
- """
- results is a dict and will be like:
- {'train_loss': [...],
- 'train_acc': [...],
- 'test_loss': [...],
- 'test_acc': [...]}
- """
- if type(results) != dict:
- results = pd.read_csv(results)
- results = results.iloc[:, 1:] # row, col
- results = results.to_dict("list")
-
- else:
- pass
-
- loss = results['train_loss']
- test_loss = results['test_loss']
-
- accuracy = results['train_acc']
- test_accuracy = results['test_acc']
-
- epochs = range(len(results['train_loss']))
-
- plt.figure(figsize=(15,7))
-
- plt.subplot(1, 2, 1)
- plt.plot(epochs, loss, label='train_loss')
- plt.plot(epochs, test_loss, label='test_loss')
- plt.title('Loss')
- plt.xlabel('Epochs')
- plt.legend()
-
- plt.subplot(1, 2, 2)
- plt.plot(epochs, accuracy, label='train_acc')
- plt.plot(epochs, test_accuracy, label='test_acc')
- plt.title('Accuracy')
- plt.xlabel('Epochs')
- plt.legend()
-
-
-def pred_single_img(Model: torch.nn.Module,
- image_path: str,
- class_name: List[str] = None,
- transforms = None,
- device: torch.device = torch.device('cpu')
- ):
- """
- show a img's pred results
- """
-
- image_done = torchvision.io.read_image(image_path).type(torch.float).to(device) / 255.
- Model.to(device)
-
- if transforms:
- image_done = transforms(image_done).unsqueeze(0).to(device)
-
- Model.eval()
- with torch.inference_mode():
- pred = Model(image_done)
- pred_probs = torch.softmax(pred, dim=1)
- pred_class = torch.argmax(pred_probs, dim=1)
-
- plt.imshow(image_done.squeeze().permute(1,2,0))
- title = f'Pred: {class_name[pred_class.cpu()]} | Probs: {pred_probs.max().cpu():.4f}'
- plt.title(title)
- plt.axis(False)
-
- return pred_probs
-
-
-def plot_conf_mat(predictions: List[int],
- num_classes: int,
- classname,
- dataset_imagefolder: datasets.ImageFolder,
- task: str = 'multiclass'):
-
- confmat = ConfusionMatrix(num_classes=num_classes,
- task=task)
-
- confmat_tensor = confmat(preds=predictions,
- target=torch.tensor(dataset_imagefolder.targets))
-
- fig, ax = plot_confusion_matrix(
- conf_mat=confmat_tensor.numpy(), # plt like working with np
- class_names=classname,
- figsize=(10,7))
-
-
-def plot_patch_img(img: torch.Tensor,
- img_size: int = 224,
- patch_size: int = 16,):
-
- """this is for ViT demonstrate"""
-
-
- # Setup hyperparameters and make sure img_size and patch_size are compatible
-
- num_patches = img_size/patch_size
- assert img_size % patch_size == 0, "Image size must be divisible by patch size"
-
- print(f"Number of patches per row: {num_patches}\
- \nNumber of patches per column: {num_patches}\
- \nTotal patches: {num_patches*num_patches}\
- \nPatch size: {patch_size} pixels x {patch_size} pixels")
-
- image_permuted = img.permute(1, 2, 0)
- # Create a series of subplots
- fig, axs = plt.subplots(nrows=img_size // patch_size, # need int not float
- ncols=img_size // patch_size,
- figsize=(num_patches, num_patches),
- sharex=True,
- sharey=True)
-
- # Loop through height and width of image
- for i, patch_height in enumerate(range(0, img_size, patch_size)): # iterate through height
- for j, patch_width in enumerate(range(0, img_size, patch_size)): # iterate through width
-
- # Plot the permuted image patch (image_permuted -> (Height, Width, Color Channels))
- axs[i, j].imshow(image_permuted[patch_height:patch_height+patch_size, # iterate through height
- patch_width:patch_width+patch_size, # iterate through width
- :]) # get all color channels
-
- # Set up label information, remove the ticks for clarity and set labels to outside
- axs[i, j].set_ylabel(i+1,
- rotation="horizontal",
- horizontalalignment="right",
- verticalalignment="center"
- )
- axs[i, j].set_xlabel(j+1)
- axs[i, j].set_xticks([])
- axs[i, j].set_yticks([])
- axs[i, j].label_outer()
-
- plt.show()
-
-
-def plot_5_feature_map(img_conv_out: torch.Tensor,
- embedding_size: int = 768,):
- """
- Plot random 5 convolutional feature maps, for ViT
- """
- random_indexes = random.sample(range(0, embedding_size), k=5) # pick 5 numbers between 0 and the embedding size
- print(f"Showing random convolutional feature maps from indexes: {random_indexes}")
-
- # Create plot
- fig, axs = plt.subplots(nrows=1, ncols=5, figsize=(12, 12))
-
- # Plot random image feature maps
- for i, idx in enumerate(random_indexes):
- img_feature_map = img_conv_out[:, idx, :, :] # index on the output tensor of the convolutional layer
- axs[i].imshow(img_feature_map.squeeze().detach().numpy())
- axs[i].set(xticklabels=[], yticklabels=[], xticks=[], yticks=[]);
-
-# ————————————————————————————————————————————————————————————————————————————————————————————————————————————
-
-
-
-
-# ————————————————————————————————————————————————————————————————————————————————————————————————————————————
-## Data_load related
-
-# custom ImageFolder
-class RTG_RAM_DataSet(Dataset):
- def __init__(self,
- dir: str,
- transform=None):
- super().__init__()
-
- """
- this is a custom ImageFolder of pytorch
- load your data into RAM in advance
- can boost the training process
- """
-
- self.paths = list(Path(dir).glob("*/*.jpg")) # pathlib.Path
-
- self.transform = transform
-
- self.classes, self.class_idx = find_classes(dir)
-
- def load_image(self, index: int) -> Image.Image:
- """Open an image via a path and return it"""
-
- image_path = self.paths[index]
- return Image.open(image_path)
-
- # overwrite __len__()
- def __len__(self) -> int:
- """return the total num of samples."""
- return len(self.paths)
-
- # overwrite __getitem__()
- def __getitem__(self, index: int) -> Tuple[torch.Tensor, int]:
- """return one sample of data, and label like (X, y)."""
- img = self.load_image(index)
- class_name = self.paths[index].parent.name
- class_idx = self.class_idx[class_name]
-
- # transformation if necessary
- if self.transform:
- return self.transform(img), class_idx # return data+label (X,y)
- else:
- return img, class_idx
-
-
-def create_dataloaders(
- train_dir: str,
- valid_dir: str,
- transform: transforms.Compose,
- batch_size: int,
- test_transform: transforms.Compose = None,
- num_workers: int = 0,
- test_dir: str = None,
- pin_mem: bool = True
-):
-
- """Creates training and testing DataLoaders.
-
- Takes in a training directory and testing directory path and turns
- them into PyTorch Datasets and then into PyTorch DataLoaders.
-
- Returns:
- A tuple of (train_dataloader, test_dataloader, class_names).
- Where class_names is a list of the target classes.
-
- """
- # Use ImageFolder to create dataset(s)
- train_data = RTG_RAM_DataSet(train_dir, transform=transform)
- valid_data = RTG_RAM_DataSet(valid_dir, transform=transform)
-
- if test_dir :
- test_data = RTG_RAM_DataSet(test_dir, transform=test_transform)
-
- test_dataloader = DataLoader(
- test_data,
- batch_size=batch_size,
- shuffle=False,
- num_workers=0,
- pin_memory=pin_mem,)
- else:
- pass
-
- # Get class names
- class_names = train_data.classes
-
- # Turn images into data loaders
- train_dataloader = DataLoader(
- train_data,
- batch_size=batch_size,
- shuffle=True,
- num_workers=num_workers,
- pin_memory=pin_mem,
- )
-
- valid_dataloader = DataLoader(
- valid_data,
- batch_size=batch_size,
- shuffle=True,
- num_workers=num_workers,
- pin_memory=pin_mem,
- )
-
-
- if test_dir:
- return train_dataloader, valid_dataloader, test_dataloader, class_names
- else:
- return train_dataloader, valid_dataloader, class_names
-
-
-# ————————————————————————————————————————————————————————————————————————————————————————————————————————————
-
-
-
-# ————————————————————————————————————————————————————————————————————————————————————————————————————————————
-## model related
-
-def print_train_time(start: float,
- end: float,
- device: torch.device = None):
- """Prints and return time cost."""
- total_time = end - start
- print(f"train time on {device}: {total_time:.3f} seconds")
- return total_time
-
-def lr_scheduler_setting(optima: torch.optim.Optimizer,
- linearLR_factor: float = 0.1,
- expLR_gamma: float = 0.95,
- constLR_factor: float = 0.1,
- mileston1: int = 30,
- mileston2: int = 60,
- epochs: int = 100):
-
-
- last = epochs-mileston2
- optima = optima
-
-
- if mileston1 > mileston2 or mileston1 > epochs:
- raise ValueError('mileston1 should smaller than epochs or mileston2')
- if mileston2 < mileston1 or mileston2 > epochs:
- raise ValueError('mileston2 should larger than mileston1 or smaller than epochs')
-
- scheduler1 = torch.optim.lr_scheduler.LinearLR(optima, start_factor=linearLR_factor)
- scheduler2 = torch.optim.lr_scheduler.ExponentialLR(optima, gamma=expLR_gamma) # also need to tune gamma here
- scheduler3 = torch.optim.lr_scheduler.ConstantLR(optima, factor=constLR_factor, total_iters=last)
- scheduler = torch.optim.lr_scheduler.SequentialLR(optima, schedulers=[scheduler1, scheduler2, scheduler3], milestones=[mileston1, mileston2])
-
- return scheduler
-
-
-def general_train_setup(Model: nn.Module,
- train_path: Path,
- valid_path: Path,
- test_path: Path,
- transform: transforms,
- test_transform: transforms,
- batch_size: int = 8,
- num_worker: int = 8, # cpu cores
- init_lr: float = 0.01
- ):
-
- """
- quick setup for a training
-
- Returns:
- a dict that contain dataloader, lr_scheduler(if needed), loss_fn, optimizing_func, classnames
- """
-
- train_dataloader, valid_dataloader, test_dataloader, class_name = create_dataloaders(train_dir=train_path,
- valid_dir=valid_path,
- test_dir=test_path,
- test_transform=test_transform,
- batch_size=batch_size,
- num_workers=num_worker,
- transform=transform,
- pin_mem=True)
-
-
- loss_fn = torch.nn.CrossEntropyLoss()
- optima = torch.optim.AdamW(params=Model.parameters(), lr=init_lr, eps=1e-3) # 0.01
-
-
- if test_path:
- return {'train_dataloader': train_dataloader,
- 'valid_dataloader': valid_dataloader,
- 'test_dataloader': test_dataloader,
- 'class_name': class_name,
- 'loss_fn': loss_fn,
- 'optima': optima}
- else:
- return {'train_dataloader': train_dataloader,
- 'valid_dataloader': valid_dataloader,
- 'class_name': class_name,
- 'loss_fn': loss_fn,
- 'optima': optima}
-
-
-def train_step(Model: torch.nn.Module,
- data_loader: torch.utils.data.DataLoader,
- loss_fn: torch.nn.Module,
- optima: torch.optim.Optimizer,
- #accuracy_fn,
- device: torch.device = torch.device("cpu")):
- """
- Performs a training with model trying to learn on data loader.
- train a single step
- """
-
- train_loss, train_acc = 0, 0
-
- Model.to(device)
- # with torch.cuda.device(device=device): # this is useless
- Model.train()
-
- for _, (X, y) in enumerate(data_loader):
- # batch
- X, y = X.to(device), y.to(device)
-
- y_pred_t = Model(X)
- loss_t = loss_fn(y_pred_t, y)
- loss_t.backward()
- optima.step() # updata params per batch, not per epoch
-
- optima.zero_grad(set_to_none=True)
- # for param in Model.parameters():
- # param.grad = None
-
- train_loss += loss_t.item() # .item() turn single tensor into a single scaler
- y_pred_t_class = torch.argmax(y_pred_t, dim=1)
- train_acc += torch.eq(y_pred_t_class, y).sum().item()/len(y_pred_t) * 100
-
-
- train_loss /= len(data_loader)
- train_acc /= len(data_loader)
-
- # print(f"Train loss: {train_loss:.4f} | Train acc: {train_acc:.4f}%")
- return train_acc, train_loss
-
-
-def test_step(Model: torch.nn.Module,
- data_loader: torch.utils.data.DataLoader,
- loss_fn: torch.nn.Module,
- #accuracy_fn,
- device: torch.device = torch.device("cpu")):
- '''test/valid a single step'''
-
- test_loss, test_acc = 0, 0
-
- Model.to(device)
-
- Model.eval()
- with torch.inference_mode():
- for X, y in data_loader:
-
- X, y = X.to(device), y.to(device)
-
- y_pred_e = Model(X)
- test_loss += loss_fn(y_pred_e, y).item()
-
- y_pred_e_labels = y_pred_e.argmax(dim=1)
- test_acc += torch.eq(y_pred_e_labels, y).sum().item()/len(y_pred_e) * 100
-
- # test_acc += accuracy_fn(y_true=y,
- # y_pred=y_pred_e.argmax(dim=1))
-
- test_loss /= len(data_loader)
- test_acc /= len(data_loader)
-
- # print(f"Test loss: {test_loss:.4F} | Test acc: {test_acc:.4F}%\n")
- return test_acc, test_loss
-
-
-def train_test_loop(Model: torch.nn.Module,
- train_loader: torch.utils.data.DataLoader,
- test_loader: torch.utils.data.DataLoader,
- epochs: int,
- optima: torch.optim.Optimizer,
- scheduler: torch.optim.lr_scheduler = None,
- #accuracy_fn,
- loss_fn: torch.nn.Module = nn.CrossEntropyLoss(),
- device: torch.device = torch.device("cpu")):
-
- if scheduler is not None:
- results = {'train_loss': [],
- 'train_acc': [],
- 'test_loss': [],
- 'test_acc': [],
- 'learning rate': []}
- else:
- results = {'train_loss': [],
- 'train_acc': [],
- 'test_loss': [],
- 'test_acc': [],}
-
- Model.to(device)
- time_start = timer()
-
- for ep in tqdm(range(epochs)):
-
- train_acc, train_loss = train_step(Model=Model,
- data_loader=train_loader,
- loss_fn=loss_fn,
- optima=optima,
- device=device)
-
- test_acc, test_loss = test_step(Model=Model,
- data_loader=test_loader,
- loss_fn=loss_fn,
- device=device)
-
- if scheduler is not None:
- current_lr = optima.param_groups[0]['lr']
- results['learning rate'].append(current_lr)
- scheduler.step()
-
- print(f"Epoch: {ep+1} | "
- f"train_loss: {train_loss:.4f} | "
- f"train_acc: {train_acc:.4f} | "
- f"test_loss: {test_loss:.4f} | "
- f"test_acc: {test_acc:.4f}"
- )
-
- results['train_loss'].append(train_loss)
- results['train_acc'].append(train_acc)
- results['test_loss'].append(test_loss)
- results['test_acc'].append(test_acc)
-
- time_end = timer()
- _ = print_train_time(start=time_start,
- end=time_end,
- device=device)
-
- return results
-
-
-def train_test_loop_with_amp(Model: torch.nn.Module,
- train_loader: torch.utils.data.DataLoader,
- test_loader: torch.utils.data.DataLoader,
- epochs: int,
- optima: torch.optim.Optimizer,
- scheduler: torch.optim.lr_scheduler = None,
- loss_fn: torch.nn.Module = nn.CrossEntropyLoss(),
- device: torch.device = torch.device("cpu")):
-
- """
- using AMP to training
- """
-
- if scheduler is not None:
- results = {'train_loss': [],
- 'train_acc': [],
- 'test_loss': [],
- 'test_acc': [],
- 'learning rate': []}
- else:
- results = {'train_loss': [],
- 'train_acc': [],
- 'test_loss': [],
- 'test_acc': [],}
-
-
- # train_loss, train_acc = 0, 0
-
- Model.to(device)
- Model.train()
-
- scaler = torch.cuda.amp.GradScaler(enabled=True)
- time_start = timer()
- for ep in tqdm(range(epochs)):
-
- train_loss, train_acc = 0, 0 #?? maybe to avoid nan?
-
- for X, y in train_loader:
- X, y = X.to(device), y.to(device)
-
- optima.zero_grad(set_to_none=True)
- # for param in Model.parameters():
- # param.grad = None
-
- with torch.autocast(device_type=str(device), dtype=torch.float16):
-
- y_pred_t = Model(X)
- loss_t = loss_fn(y_pred_t, y)
-
- # or maybe we should move this two line inside of AMP block?
- train_loss += loss_t.item() # .item() turn single tensor into a single scaler
- y_pred_t_class = torch.argmax(y_pred_t, dim=1)
- train_acc += torch.eq(y_pred_t_class, y).sum().item()/len(y_pred_t) * 100
-
- scaler.scale(loss_t).backward() # none type
-
- scaler.unscale_(optima)
-
- torch.nn.utils.clip_grad_norm_(Model.parameters(), max_norm=0.1)
-
- scaler.step(optima)
- scaler.update()
-
- # loss_t.backward()
- # optima.step()
-
- train_loss /= len(train_loader)
- train_acc /= len(train_loader)
-
- if train_acc > 100:
- train_acc = 100.0000
-
- test_acc, test_loss = test_step(Model=Model,
- data_loader=test_loader,
- loss_fn=loss_fn,
- device=device)
-
- if scheduler is not None:
- optima.zero_grad(set_to_none=True)
- optima.step()
- current_lr = optima.param_groups[0]['lr']
- results['learning rate'].append(current_lr)
- scheduler.step()
-
- print(f"Epoch: {ep+1} | "
- f"train_loss: {train_loss:.4f} | " # nan???
- f"train_acc: {train_acc:.4f} | "
- f"test_loss: {test_loss:.4f} | " # nan???
- f"test_acc: {test_acc:.4f}"
- )
-
- results['train_loss'].append(train_loss)
- results['train_acc'].append(train_acc)
- results['test_loss'].append(test_loss)
- results['test_acc'].append(test_acc)
-
- # gc.collect()
- # torch.cuda.empty_cache()
-
- time_end = timer()
- print_train_time(start=time_start,
- end=time_end,
- device=device)
-
- return results
-
-
-
-def eval_model(Model: torch.nn.Module,
- eval_loader: torch.utils.data.DataLoader,
- loss_fn: torch.nn.Module = nn.CrossEntropyLoss(),
- show: bool = True,
- device: torch.device = torch.device("cpu")):
- '''
- eval model prediction results, return loss, acc, pred_tensor
- pred_tensor is for the plot of confusion matrix
- '''
- loss = 0
- acc = 0
- preds = []
-
- Model.to(device)
- Model.eval()
- with torch.inference_mode():
- for X, y in tqdm(eval_loader):
- X, y = X.to(device), y.to(device)
-
- raw_logits = Model(X)
-
- loss += loss_fn(raw_logits, y).item()
- pred_label = torch.argmax(raw_logits, dim=1)
-
- prediction = torch.argmax(raw_logits.squeeze(0), dim=1) # using this for confusion matrix
- preds.append(prediction.cpu())
-
- acc += torch.eq(pred_label, y).sum().item()/len(raw_logits) * 100
-
- loss /= len(eval_loader)
- acc /= len(eval_loader)
-
- predictions_tensor = torch.cat(preds)
-
- if show:
- print(f"Model: {Model.__class__.__name__}")
- print(f"Eval loss: {loss:.4F} | Eval acc: {acc:.4F}%\n")
- return loss, acc, predictions_tensor
-
-
-# ————————————————————————————————————————————————————————————————————————————————————————————————————————————
-
-
-# ————————————————————————————————————————————————————————————————————————————————————————————————————————————
-## result saving
-def save_model(model: torch.nn.Module,
- target_dir: str,
- model_name: str):
- """Saves a PyTorch model to a target directory.
-
- Args:
- model: A target PyTorch model to save.
- target_dir: A directory for saving the model to.
- model_name: A filename for the saved model. Should include
- either ".pth" or ".pt" as the file extension.
-
- """
- # Create target directory
- target_dir_path = Path(target_dir)
- target_dir_path.mkdir(parents=True,
- exist_ok=True)
-
- # Create model save path
- assert model_name.endswith(".pth") or model_name.endswith(".pt"), "model_name should end with '.pt' or '.pth'"
- model_save_path = target_dir_path / model_name
-
- # Save the whole model, not only the state_dict(), so that we don't have to init model structure instance everytime
- print(f"[INFO] Saving model to: {model_save_path}")
- torch.save(obj=model, # .state_dict(),
- f=model_save_path)
-
-
-def save_results(results: Dict[str, List[float]],
- path_and_filename: str):
- '''save Dict results into csv format'''
-
- print(f"[INFO] Saving results to: {path_and_filename}")
- df = pd.DataFrame(results)
- df.to_csv(path_and_filename, index=False)
-
-
-# ————————————————————————————————————————————————————————————————————————————————————————————————————————————
-
-
-# ————————————————————————————————————————————————————————————————————————————————————————————————————————————
-## result analyze related
-
-def pred_wrong_and_store(path: Path, # class1..classn/img.jpg
- Model,
- transform,
- class_names,
- top_num: int = 5,
- show: bool = True,
- device: torch.device = torch.device('cpu')):
- """
- preds some img on a model and store the results
- and also grab and plot some most wrong examples
-
- Returns:
- a sorted pandas dataframe
- """
-
- pred_list = []
-
- # first, get a list contain every single img path
- img_path_list = list(Path(path).glob("*/*.jpg"))
-
-
- for path in tqdm(img_path_list):
-
- # a empty dict to store every img result
- pred_dict = {}
-
- # get sample path
- pred_dict['img_path'] = path
-
- # get class name
- class_name = path.parent.stem
- pred_dict["class_names"] = class_name
-
- start_time = timer()
-
- # get predictions
- img = Image.open(path)
- transformed_img = transform(img).unsqueeze(0).to(device)
-
- Model.to(device)
- Model.eval()
- with torch.inference_mode():
- pred_logits = Model(transformed_img)
- pred_probs = torch.softmax(pred_logits, dim=1)
- pred_label = torch.argmax(pred_probs, dim=1)
- pred_class = class_names[pred_label.cpu()]
-
- pred_dict["pred_probs"] = pred_probs.unsqueeze(0).max().cpu().item() # make sure result back to cpu
- pred_dict["pred_class"] = pred_class # convient for plot
-
- end_time = timer()
- pred_dict["time_for_pred"] = round(end_time-start_time, 4)
-
- pred_dict['correct'] = class_name == pred_class
-
- pred_list.append(pred_dict)
-
- pred_df = pd.DataFrame(pred_list)
- sorted_pred_df = pred_df.sort_values(by=['correct', 'pred_probs'], ascending=[True, False])
-
- if show:
- most_wrong = sorted_pred_df.head(n=top_num)
-
- for row in most_wrong.iterrows():
- data_row = row[1]
- img_path = data_row[0]
- true_label = data_row[1]
- pred_prob = data_row[2]
- pred_class = data_row[3]
-
- # plot img
- img = torchvision.io.read_image(str(img_path)) # read to tensor
- plt.figure()
- plt.imshow(img.permute(1, 2, 0)) # h x w x c
- plt.title(f"True: {true_label} | Pred: {pred_class} | Prob: {pred_prob}")
- plt.axis(False);
- else:
- pass
-
- return sorted_pred_df
-
-
-def check_model_size(path, show=True):
- """check a model's size"""
-
- size = Path(path).stat().st_size // (1024*1024)
- if show:
- print(f"model size: {size:.3f} MB")
-
- return size
-
-
-def general_test(Model,
- model_path,
- class_name,
- manual_transforms,
- test_path, loss_fn,
- valid_loader):
-
- """
- run a general test on a model
- including model_size, params, loss and acc on test set, pred_time and so on
-
- Returns:
- a dict
- """
-
- stat = {}
- print(f'[INFO] running general test on: {Model._get_name()}')
-
- model_size = check_model_size(model_path, show=False)
- print('size check ... done')
- model_params = sum(torch.numel(param) for param in Model.parameters())
- print('params check ... done')
- loss, acc, _ = eval_model(Model, valid_loader, loss_fn, show=False)
- print('valid evaluate ... done')
- pred_df = pred_wrong_and_store(test_path, Model, manual_transforms, class_name, show=False)
- print('prediction test ... done')
- average_time_per_pred = round(pred_df.time_for_pred.mean(), 4)
- print('predict time calculate ... done')
- test_acc = pred_df.correct.value_counts()[0]*100/len(pred_df)
- print('real accurate calculate ... done')
-
- stat['valid_loss'] = loss
- stat['valid_acc'] = acc
- stat['test_acc'] = test_acc
- stat['number_of_parameters'] = model_params
- stat['model_size (MB)'] = model_size
- stat['time_per_pred_cpu'] = average_time_per_pred
-
- print("test results:")
- print(stat)
-
- return stat
-# ————————————————————————————————————————————————————————————————————————————————————————————————————————————
\ No newline at end of file
diff --git a/spaces/RedBaron5/PatentSolver/README.md b/spaces/RedBaron5/PatentSolver/README.md
deleted file mode 100644
index ceee5cf77c6480363b635c60d2a67f67613b854a..0000000000000000000000000000000000000000
--- a/spaces/RedBaron5/PatentSolver/README.md
+++ /dev/null
@@ -1,38 +0,0 @@
----
-title: PatentSolver
-emoji: 🚀
-colorFrom: gray
-colorTo: gray
-sdk: streamlit
-app_file: app.py
-pinned: false
-duplicated_from: xin/PatentSolver
----
-
-# Configuration
-
-`title`: _string_
-Display title for the Space
-
-`emoji`: _string_
-Space emoji (emoji-only character allowed)
-
-`colorFrom`: _string_
-Color for Thumbnail gradient (red, yellow, green, blue, indigo, purple, pink, gray)
-
-`colorTo`: _string_
-Color for Thumbnail gradient (red, yellow, green, blue, indigo, purple, pink, gray)
-
-`sdk`: _string_
-Can be either `gradio` or `streamlit`
-
-`sdk_version` : _string_
-Only applicable for `streamlit` SDK.
-See [doc](https://hf.co/docs/hub/spaces) for more info on supported versions.
-
-`app_file`: _string_
-Path to your main application file (which contains either `gradio` or `streamlit` Python code).
-Path is relative to the root of the repository.
-
-`pinned`: _boolean_
-Whether the Space stays on top of your list.
diff --git a/spaces/Robert001/UniControl-Demo/annotator/uniformer_base/mmseg/apis/inference.py b/spaces/Robert001/UniControl-Demo/annotator/uniformer_base/mmseg/apis/inference.py
deleted file mode 100644
index 90bc1c0c68525734bd6793f07c15fe97d3c8342c..0000000000000000000000000000000000000000
--- a/spaces/Robert001/UniControl-Demo/annotator/uniformer_base/mmseg/apis/inference.py
+++ /dev/null
@@ -1,136 +0,0 @@
-import matplotlib.pyplot as plt
-import annotator.uniformer.mmcv as mmcv
-import torch
-from annotator.uniformer.mmcv.parallel import collate, scatter
-from annotator.uniformer.mmcv.runner import load_checkpoint
-
-from annotator.uniformer.mmseg.datasets.pipelines import Compose
-from annotator.uniformer.mmseg.models import build_segmentor
-
-
-def init_segmentor(config, checkpoint=None, device='cuda:0'):
- """Initialize a segmentor from config file.
-
- Args:
- config (str or :obj:`mmcv.Config`): Config file path or the config
- object.
- checkpoint (str, optional): Checkpoint path. If left as None, the model
- will not load any weights.
- device (str, optional) CPU/CUDA device option. Default 'cuda:0'.
- Use 'cpu' for loading model on CPU.
- Returns:
- nn.Module: The constructed segmentor.
- """
- if isinstance(config, str):
- config = mmcv.Config.fromfile(config)
- elif not isinstance(config, mmcv.Config):
- raise TypeError('config must be a filename or Config object, '
- 'but got {}'.format(type(config)))
- config.model.pretrained = None
- config.model.train_cfg = None
- model = build_segmentor(config.model, test_cfg=config.get('test_cfg'))
- if checkpoint is not None:
- checkpoint = load_checkpoint(model, checkpoint, map_location='cpu')
- model.CLASSES = checkpoint['meta']['CLASSES']
- model.PALETTE = checkpoint['meta']['PALETTE']
- model.cfg = config # save the config in the model for convenience
- model.to(device)
- model.eval()
- return model
-
-
-class LoadImage:
- """A simple pipeline to load image."""
-
- def __call__(self, results):
- """Call function to load images into results.
-
- Args:
- results (dict): A result dict contains the file name
- of the image to be read.
-
- Returns:
- dict: ``results`` will be returned containing loaded image.
- """
-
- if isinstance(results['img'], str):
- results['filename'] = results['img']
- results['ori_filename'] = results['img']
- else:
- results['filename'] = None
- results['ori_filename'] = None
- img = mmcv.imread(results['img'])
- results['img'] = img
- results['img_shape'] = img.shape
- results['ori_shape'] = img.shape
- return results
-
-
-def inference_segmentor(model, img):
- """Inference image(s) with the segmentor.
-
- Args:
- model (nn.Module): The loaded segmentor.
- imgs (str/ndarray or list[str/ndarray]): Either image files or loaded
- images.
-
- Returns:
- (list[Tensor]): The segmentation result.
- """
- cfg = model.cfg
- device = next(model.parameters()).device # model device
- # build the data pipeline
- test_pipeline = [LoadImage()] + cfg.data.test.pipeline[1:]
- test_pipeline = Compose(test_pipeline)
- # prepare data
- data = dict(img=img)
- data = test_pipeline(data)
- data = collate([data], samples_per_gpu=1)
- if next(model.parameters()).is_cuda:
- # scatter to specified GPU
- data = scatter(data, [device])[0]
- else:
- data['img_metas'] = [i.data[0] for i in data['img_metas']]
-
- # forward the model
- with torch.no_grad():
- result = model(return_loss=False, rescale=True, **data)
- return result
-
-
-def show_result_pyplot(model,
- img,
- result,
- palette=None,
- fig_size=(15, 10),
- opacity=0.5,
- title='',
- block=True):
- """Visualize the segmentation results on the image.
-
- Args:
- model (nn.Module): The loaded segmentor.
- img (str or np.ndarray): Image filename or loaded image.
- result (list): The segmentation result.
- palette (list[list[int]]] | None): The palette of segmentation
- map. If None is given, random palette will be generated.
- Default: None
- fig_size (tuple): Figure size of the pyplot figure.
- opacity(float): Opacity of painted segmentation map.
- Default 0.5.
- Must be in (0, 1] range.
- title (str): The title of pyplot figure.
- Default is ''.
- block (bool): Whether to block the pyplot figure.
- Default is True.
- """
- if hasattr(model, 'module'):
- model = model.module
- img = model.show_result(
- img, result, palette=palette, show=False, opacity=opacity)
- # plt.figure(figsize=fig_size)
- # plt.imshow(mmcv.bgr2rgb(img))
- # plt.title(title)
- # plt.tight_layout()
- # plt.show(block=block)
- return mmcv.bgr2rgb(img)
diff --git a/spaces/Rominn/vits-uma-genshin-honkai/text/symbols.py b/spaces/Rominn/vits-uma-genshin-honkai/text/symbols.py
deleted file mode 100644
index edfbd24247be8c757275ce80b9ec27a0ffa808f3..0000000000000000000000000000000000000000
--- a/spaces/Rominn/vits-uma-genshin-honkai/text/symbols.py
+++ /dev/null
@@ -1,39 +0,0 @@
-'''
-Defines the set of symbols used in text input to the model.
-'''
-
-'''# japanese_cleaners
-_pad = '_'
-_punctuation = ',.!?-'
-_letters = 'AEINOQUabdefghijkmnoprstuvwyzʃʧ↓↑ '
-'''
-
-'''# japanese_cleaners2
-_pad = '_'
-_punctuation = ',.!?-~…'
-_letters = 'AEINOQUabdefghijkmnoprstuvwyzʃʧʦ↓↑ '
-'''
-
-'''# korean_cleaners
-_pad = '_'
-_punctuation = ',.!?…~'
-_letters = 'ㄱㄴㄷㄹㅁㅂㅅㅇㅈㅊㅋㅌㅍㅎㄲㄸㅃㅆㅉㅏㅓㅗㅜㅡㅣㅐㅔ '
-'''
-
-'''# chinese_cleaners
-_pad = '_'
-_punctuation = ',。!?—…'
-_letters = 'ㄅㄆㄇㄈㄉㄊㄋㄌㄍㄎㄏㄐㄑㄒㄓㄔㄕㄖㄗㄘㄙㄚㄛㄜㄝㄞㄟㄠㄡㄢㄣㄤㄥㄦㄧㄨㄩˉˊˇˋ˙ '
-'''
-
-# zh_ja_mixture_cleaners
-_pad = '_'
-_punctuation = ',.!?-~…'
-_letters = 'AEINOQUabdefghijklmnoprstuvwyzʃʧʦɯɹəɥ⁼ʰ`→↓↑ '
-
-
-# Export all symbols:
-symbols = [_pad] + list(_punctuation) + list(_letters)
-
-# Special symbol ids
-SPACE_ID = symbols.index(" ")
\ No newline at end of file
diff --git a/spaces/SeViLA/SeViLA/lavis/datasets/builders/image_text_pair_builder.py b/spaces/SeViLA/SeViLA/lavis/datasets/builders/image_text_pair_builder.py
deleted file mode 100644
index 90e411eb6d41c23c15dbf5a0c67e2b68d467b43b..0000000000000000000000000000000000000000
--- a/spaces/SeViLA/SeViLA/lavis/datasets/builders/image_text_pair_builder.py
+++ /dev/null
@@ -1,77 +0,0 @@
-"""
- Copyright (c) 2022, salesforce.com, inc.
- All rights reserved.
- SPDX-License-Identifier: BSD-3-Clause
- For full license text, see the LICENSE file in the repo root or https://opensource.org/licenses/BSD-3-Clause
-"""
-
-import os
-from lavis.common.registry import registry
-
-from lavis.datasets.builders.base_dataset_builder import BaseDatasetBuilder
-from lavis.datasets.datasets.image_text_pair_datasets import ImageTextPairDataset
-from lavis.datasets.datasets.laion_dataset import LaionDataset
-
-
-@registry.register_builder("conceptual_caption_3m")
-class ConceptualCaption3MBuilder(BaseDatasetBuilder):
- train_dataset_cls = ImageTextPairDataset
-
- DATASET_CONFIG_DICT = {
- "default": "configs/datasets/conceptual_caption/defaults_3m.yaml"
- }
-
-
-@registry.register_builder("conceptual_caption_12m")
-class ConceptualCaption12MBuilder(BaseDatasetBuilder):
- train_dataset_cls = ImageTextPairDataset
-
- DATASET_CONFIG_DICT = {
- "default": "configs/datasets/conceptual_caption/defaults_12m.yaml"
- }
-
-
-@registry.register_builder("sbu_caption")
-class SBUCaptionBuilder(BaseDatasetBuilder):
- train_dataset_cls = ImageTextPairDataset
-
- DATASET_CONFIG_DICT = {"default": "configs/datasets/sbu_caption/defaults.yaml"}
-
-
-@registry.register_builder("vg_caption")
-class VGCaptionBuilder(BaseDatasetBuilder):
- train_dataset_cls = ImageTextPairDataset
-
- DATASET_CONFIG_DICT = {"default": "configs/datasets/vg/defaults_caption.yaml"}
-
-
-@registry.register_builder("laion2B_multi")
-class Laion2BMultiBuilder(BaseDatasetBuilder):
- train_dataset_cls = LaionDataset
-
- DATASET_CONFIG_DICT = {"default": "configs/datasets/laion/defaults_2B_multi.yaml"}
-
- def _download_ann(self):
- pass
-
- def _download_vis(self):
- pass
-
- def build(self):
- self.build_processors()
-
- build_info = self.config.build_info
-
- datasets = dict()
- split = "train" # laion dataset only has train split
-
- # create datasets
- # [NOTE] return inner_datasets (wds.DataPipeline)
- dataset_cls = self.train_dataset_cls
- datasets[split] = dataset_cls(
- vis_processor=self.vis_processors[split],
- text_processor=self.text_processors[split],
- location=build_info.storage,
- ).inner_dataset
-
- return datasets
diff --git a/spaces/Sparkles-AI/design-look-a-likes/README.md b/spaces/Sparkles-AI/design-look-a-likes/README.md
deleted file mode 100644
index 4e32a4efc3f4eb718c2adf9d79a952eadbf4acea..0000000000000000000000000000000000000000
--- a/spaces/Sparkles-AI/design-look-a-likes/README.md
+++ /dev/null
@@ -1,11 +0,0 @@
----
-title: Design Look A Likes
-emoji: 👁
-colorFrom: gray
-colorTo: indigo
-sdk: docker
-pinned: false
-license: unknown
----
-
-Check out the configuration reference at https://huggingface.co/docs/hub/spaces-config-reference
diff --git a/spaces/SungBeom/chatwine-korean/.venv/Lib/site-packages/IPython/terminal/shortcuts/auto_match.py b/spaces/SungBeom/chatwine-korean/.venv/Lib/site-packages/IPython/terminal/shortcuts/auto_match.py
deleted file mode 100644
index 6c2b1ef70c9051304efa42ba7af348c7299e5534..0000000000000000000000000000000000000000
--- a/spaces/SungBeom/chatwine-korean/.venv/Lib/site-packages/IPython/terminal/shortcuts/auto_match.py
+++ /dev/null
@@ -1,104 +0,0 @@
-"""
-Utilities function for keybinding with prompt toolkit.
-
-This will be bound to specific key press and filter modes,
-like whether we are in edit mode, and whether the completer is open.
-"""
-import re
-from prompt_toolkit.key_binding import KeyPressEvent
-
-
-def parenthesis(event: KeyPressEvent):
- """Auto-close parenthesis"""
- event.current_buffer.insert_text("()")
- event.current_buffer.cursor_left()
-
-
-def brackets(event: KeyPressEvent):
- """Auto-close brackets"""
- event.current_buffer.insert_text("[]")
- event.current_buffer.cursor_left()
-
-
-def braces(event: KeyPressEvent):
- """Auto-close braces"""
- event.current_buffer.insert_text("{}")
- event.current_buffer.cursor_left()
-
-
-def double_quote(event: KeyPressEvent):
- """Auto-close double quotes"""
- event.current_buffer.insert_text('""')
- event.current_buffer.cursor_left()
-
-
-def single_quote(event: KeyPressEvent):
- """Auto-close single quotes"""
- event.current_buffer.insert_text("''")
- event.current_buffer.cursor_left()
-
-
-def docstring_double_quotes(event: KeyPressEvent):
- """Auto-close docstring (double quotes)"""
- event.current_buffer.insert_text('""""')
- event.current_buffer.cursor_left(3)
-
-
-def docstring_single_quotes(event: KeyPressEvent):
- """Auto-close docstring (single quotes)"""
- event.current_buffer.insert_text("''''")
- event.current_buffer.cursor_left(3)
-
-
-def raw_string_parenthesis(event: KeyPressEvent):
- """Auto-close parenthesis in raw strings"""
- matches = re.match(
- r".*(r|R)[\"'](-*)",
- event.current_buffer.document.current_line_before_cursor,
- )
- dashes = matches.group(2) if matches else ""
- event.current_buffer.insert_text("()" + dashes)
- event.current_buffer.cursor_left(len(dashes) + 1)
-
-
-def raw_string_bracket(event: KeyPressEvent):
- """Auto-close bracker in raw strings"""
- matches = re.match(
- r".*(r|R)[\"'](-*)",
- event.current_buffer.document.current_line_before_cursor,
- )
- dashes = matches.group(2) if matches else ""
- event.current_buffer.insert_text("[]" + dashes)
- event.current_buffer.cursor_left(len(dashes) + 1)
-
-
-def raw_string_braces(event: KeyPressEvent):
- """Auto-close braces in raw strings"""
- matches = re.match(
- r".*(r|R)[\"'](-*)",
- event.current_buffer.document.current_line_before_cursor,
- )
- dashes = matches.group(2) if matches else ""
- event.current_buffer.insert_text("{}" + dashes)
- event.current_buffer.cursor_left(len(dashes) + 1)
-
-
-def skip_over(event: KeyPressEvent):
- """Skip over automatically added parenthesis/quote.
-
- (rather than adding another parenthesis/quote)"""
- event.current_buffer.cursor_right()
-
-
-def delete_pair(event: KeyPressEvent):
- """Delete auto-closed parenthesis"""
- event.current_buffer.delete()
- event.current_buffer.delete_before_cursor()
-
-
-auto_match_parens = {"(": parenthesis, "[": brackets, "{": braces}
-auto_match_parens_raw_string = {
- "(": raw_string_parenthesis,
- "[": raw_string_bracket,
- "{": raw_string_braces,
-}
diff --git a/spaces/SungBeom/chatwine-korean/.venv/Lib/site-packages/docarray/array/doc_list/io.py b/spaces/SungBeom/chatwine-korean/.venv/Lib/site-packages/docarray/array/doc_list/io.py
deleted file mode 100644
index c2b531c25502840501dc1a773bb84eba153d9240..0000000000000000000000000000000000000000
--- a/spaces/SungBeom/chatwine-korean/.venv/Lib/site-packages/docarray/array/doc_list/io.py
+++ /dev/null
@@ -1,826 +0,0 @@
-import base64
-import csv
-import io
-import os
-import pathlib
-import pickle
-from abc import abstractmethod
-from contextlib import nullcontext
-from io import StringIO, TextIOWrapper
-from itertools import compress
-from typing import (
- TYPE_CHECKING,
- Any,
- BinaryIO,
- ContextManager,
- Dict,
- Generator,
- Iterable,
- Iterator,
- List,
- Optional,
- Tuple,
- Type,
- TypeVar,
- Union,
-)
-
-import orjson
-
-from docarray.base_doc import AnyDoc, BaseDoc
-from docarray.base_doc.io.json import orjson_dumps
-from docarray.helper import (
- _access_path_dict_to_nested_dict,
- _all_access_paths_valid,
- _dict_to_access_paths,
-)
-from docarray.utils._internal.compress import _decompress_bytes, _get_compress_ctx
-from docarray.utils._internal.misc import import_library
-
-if TYPE_CHECKING:
- import pandas as pd
-
- from docarray import DocList
- from docarray.proto import DocListProto
-
-T = TypeVar('T', bound='IOMixinArray')
-T_doc = TypeVar('T_doc', bound=BaseDoc)
-
-ARRAY_PROTOCOLS = {'protobuf-array', 'pickle-array', 'json-array'}
-SINGLE_PROTOCOLS = {'pickle', 'protobuf', 'json'}
-ALLOWED_PROTOCOLS = ARRAY_PROTOCOLS.union(SINGLE_PROTOCOLS)
-ALLOWED_COMPRESSIONS = {'lz4', 'bz2', 'lzma', 'zlib', 'gzip'}
-
-
-def _protocol_and_compress_from_file_path(
- file_path: Union[pathlib.Path, str],
- default_protocol: Optional[str] = None,
- default_compress: Optional[str] = None,
-) -> Tuple[Optional[str], Optional[str]]:
- """Extract protocol and compression algorithm from a string, use defaults if not found.
- :param file_path: path of a file.
- :param default_protocol: default serialization protocol used in case not found.
- :param default_compress: default compression method used in case not found.
- Examples:
- >>> _protocol_and_compress_from_file_path('./docarray_fashion_mnist.protobuf.gzip')
- ('protobuf', 'gzip')
- >>> _protocol_and_compress_from_file_path('/Documents/docarray_fashion_mnist.protobuf')
- ('protobuf', None)
- >>> _protocol_and_compress_from_file_path('/Documents/docarray_fashion_mnist.gzip')
- (None, gzip)
- """
-
- protocol = default_protocol
- compress = default_compress
-
- file_extensions = [e.replace('.', '') for e in pathlib.Path(file_path).suffixes]
- for extension in file_extensions:
- if extension in ALLOWED_PROTOCOLS:
- protocol = extension
- elif extension in ALLOWED_COMPRESSIONS:
- compress = extension
-
- return protocol, compress
-
-
-class _LazyRequestReader:
- def __init__(self, r):
- self._data = r.iter_content(chunk_size=1024 * 1024)
- self.content = b''
-
- def __getitem__(self, item: slice):
- while len(self.content) < item.stop:
- try:
- self.content += next(self._data)
- except StopIteration:
- return self.content[item.start : -1 : item.step]
- return self.content[item]
-
-
-class IOMixinArray(Iterable[T_doc]):
- doc_type: Type[T_doc]
-
- @abstractmethod
- def __len__(self):
- ...
-
- @abstractmethod
- def __init__(
- self,
- docs: Optional[Iterable[BaseDoc]] = None,
- ):
- ...
-
- @classmethod
- def from_protobuf(cls: Type[T], pb_msg: 'DocListProto') -> T:
- """create a Document from a protobuf message
- :param pb_msg: The protobuf message from where to construct the DocList
- """
- return cls(cls.doc_type.from_protobuf(doc_proto) for doc_proto in pb_msg.docs)
-
- def to_protobuf(self) -> 'DocListProto':
- """Convert `DocList` into a Protobuf message"""
- from docarray.proto import DocListProto
-
- da_proto = DocListProto()
- for doc in self:
- da_proto.docs.append(doc.to_protobuf())
-
- return da_proto
-
- @classmethod
- def from_bytes(
- cls: Type[T],
- data: bytes,
- protocol: str = 'protobuf-array',
- compress: Optional[str] = None,
- show_progress: bool = False,
- ) -> T:
- """Deserialize bytes into a `DocList`.
-
- :param data: Bytes from which to deserialize
- :param protocol: protocol that was used to serialize
- :param compress: compression algorithm that was used to serialize between `lz4`, `bz2`, `lzma`, `zlib`, `gzip`
- :param show_progress: show progress bar, only works when protocol is `pickle` or `protobuf`
- :return: the deserialized `DocList`
- """
- return cls._load_binary_all(
- file_ctx=nullcontext(data),
- protocol=protocol,
- compress=compress,
- show_progress=show_progress,
- )
-
- def _write_bytes(
- self,
- bf: BinaryIO,
- protocol: str = 'protobuf-array',
- compress: Optional[str] = None,
- show_progress: bool = False,
- ) -> None:
- if protocol in ARRAY_PROTOCOLS:
- compress_ctx = _get_compress_ctx(compress)
- else:
- # delegate the compression to per-doc compression
- compress_ctx = None
-
- fc: ContextManager
- if compress_ctx is None:
- # if compress do not support streaming then postpone the compress
- # into the for-loop
- f, fc = bf, nullcontext()
- else:
- f = compress_ctx(bf)
- fc = f
- compress = None
-
- with fc:
- if protocol == 'protobuf-array':
- f.write(self.to_protobuf().SerializePartialToString())
- elif protocol == 'pickle-array':
- f.write(pickle.dumps(self))
- elif protocol == 'json-array':
- f.write(self.to_json())
- elif protocol in SINGLE_PROTOCOLS:
- f.write(
- b''.join(
- self._to_binary_stream(
- protocol=protocol,
- compress=compress,
- show_progress=show_progress,
- )
- )
- )
- else:
- raise ValueError(
- f'protocol={protocol} is not supported. Can be only {ALLOWED_PROTOCOLS}.'
- )
-
- def _to_binary_stream(
- self,
- protocol: str = 'protobuf',
- compress: Optional[str] = None,
- show_progress: bool = False,
- ) -> Iterator[bytes]:
- from rich import filesize
-
- if show_progress:
- from docarray.utils._internal.progress_bar import _get_progressbar
-
- pbar, t = _get_progressbar(
- 'Serializing', disable=not show_progress, total=len(self)
- )
- else:
- from contextlib import nullcontext
-
- pbar = nullcontext()
-
- yield self._stream_header
-
- with pbar:
- if show_progress:
- _total_size = 0
- pbar.start_task(t)
- for doc in self:
- doc_bytes = doc.to_bytes(protocol=protocol, compress=compress)
- len_doc_as_bytes = len(doc_bytes).to_bytes(4, 'big', signed=False)
- all_bytes = len_doc_as_bytes + doc_bytes
-
- yield all_bytes
-
- if show_progress:
- _total_size += len(all_bytes)
- pbar.update(
- t,
- advance=1,
- total_size=str(filesize.decimal(_total_size)),
- )
-
- def to_bytes(
- self,
- protocol: str = 'protobuf-array',
- compress: Optional[str] = None,
- file_ctx: Optional[BinaryIO] = None,
- show_progress: bool = False,
- ) -> Optional[bytes]:
- """Serialize itself into `bytes`.
-
- For more Pythonic code, please use ``bytes(...)``.
-
- :param protocol: protocol to use. It can be 'pickle-array', 'protobuf-array', 'pickle' or 'protobuf'
- :param compress: compress algorithm to use between : `lz4`, `bz2`, `lzma`, `zlib`, `gzip`
- :param file_ctx: File or filename or serialized bytes where the data is stored.
- :param show_progress: show progress bar, only works when protocol is `pickle` or `protobuf`
- :return: the binary serialization in bytes or None if file_ctx is passed where to store
- """
-
- with file_ctx or io.BytesIO() as bf:
- self._write_bytes(
- bf=bf,
- protocol=protocol,
- compress=compress,
- show_progress=show_progress,
- )
- if isinstance(bf, io.BytesIO):
- return bf.getvalue()
-
- return None
-
- @classmethod
- def from_base64(
- cls: Type[T],
- data: str,
- protocol: str = 'protobuf-array',
- compress: Optional[str] = None,
- show_progress: bool = False,
- ) -> T:
- """Deserialize base64 strings into a `DocList`.
-
- :param data: Base64 string to deserialize
- :param protocol: protocol that was used to serialize
- :param compress: compress algorithm that was used to serialize between `lz4`, `bz2`, `lzma`, `zlib`, `gzip`
- :param show_progress: show progress bar, only works when protocol is `pickle` or `protobuf`
- :return: the deserialized `DocList`
- """
- return cls._load_binary_all(
- file_ctx=nullcontext(base64.b64decode(data)),
- protocol=protocol,
- compress=compress,
- show_progress=show_progress,
- )
-
- def to_base64(
- self,
- protocol: str = 'protobuf-array',
- compress: Optional[str] = None,
- show_progress: bool = False,
- ) -> str:
- """Serialize itself into base64 encoded string.
-
- :param protocol: protocol to use. It can be 'pickle-array', 'protobuf-array', 'pickle' or 'protobuf'
- :param compress: compress algorithm to use between `lz4`, `bz2`, `lzma`, `zlib`, `gzip`
- :param show_progress: show progress bar, only works when protocol is `pickle` or `protobuf`
- :return: the binary serialization in bytes or None if file_ctx is passed where to store
- """
- with io.BytesIO() as bf:
- self._write_bytes(
- bf=bf,
- compress=compress,
- protocol=protocol,
- show_progress=show_progress,
- )
- return base64.b64encode(bf.getvalue()).decode('utf-8')
-
- @classmethod
- def from_json(
- cls: Type[T],
- file: Union[str, bytes, bytearray],
- ) -> T:
- """Deserialize JSON strings or bytes into a `DocList`.
-
- :param file: JSON object from where to deserialize a `DocList`
- :return: the deserialized `DocList`
- """
- json_docs = orjson.loads(file)
- return cls([cls.doc_type(**v) for v in json_docs])
-
- def to_json(self) -> bytes:
- """Convert the object into JSON bytes. Can be loaded via `.from_json`.
- :return: JSON serialization of `DocList`
- """
- return orjson_dumps(self)
-
- @classmethod
- def from_csv(
- cls,
- file_path: str,
- encoding: str = 'utf-8',
- dialect: Union[str, csv.Dialect] = 'excel',
- ) -> 'DocList':
- """
- Load a DocList from a csv file following the schema defined in the
- [`.doc_type`][docarray.DocList] attribute.
- Every row of the csv file will be mapped to one document in the doc_list.
- The column names (defined in the first row) have to match the field names
- of the Document type.
- For nested fields use "__"-separated access paths, such as `'image__url'`.
-
- List-like fields (including field of type DocList) are not supported.
-
- :param file_path: path to csv file to load DocList from.
- :param encoding: encoding used to read the csv file. Defaults to 'utf-8'.
- :param dialect: defines separator and how to handle whitespaces etc.
- Can be a [`csv.Dialect`](https://docs.python.org/3/library/csv.html#csv.Dialect)
- instance or one string of:
- `'excel'` (for comma separated values),
- `'excel-tab'` (for tab separated values),
- `'unix'` (for csv file generated on UNIX systems).
-
- :return: `DocList` object
- """
- if cls.doc_type == AnyDoc:
- raise TypeError(
- 'There is no document schema defined. '
- 'Please specify the DocList\'s Document type using `DocList[MyDoc]`.'
- )
-
- if file_path.startswith('http'):
- import urllib.request
-
- with urllib.request.urlopen(file_path) as f:
- file = StringIO(f.read().decode(encoding))
- return cls._from_csv_file(file, dialect)
- else:
- with open(file_path, 'r', encoding=encoding) as fp:
- return cls._from_csv_file(fp, dialect)
-
- @classmethod
- def _from_csv_file(
- cls, file: Union[StringIO, TextIOWrapper], dialect: Union[str, csv.Dialect]
- ) -> 'DocList':
- from docarray import DocList
-
- rows = csv.DictReader(file, dialect=dialect)
-
- doc_type = cls.doc_type
- docs = DocList.__class_getitem__(doc_type)()
-
- field_names: List[str] = (
- [] if rows.fieldnames is None else [str(f) for f in rows.fieldnames]
- )
- if field_names is None or len(field_names) == 0:
- raise TypeError("No field names are given.")
-
- valid_paths = _all_access_paths_valid(
- doc_type=doc_type, access_paths=field_names
- )
- if not all(valid_paths):
- raise ValueError(
- f'Column names do not match the schema of the DocList\'s '
- f'document type ({cls.doc_type.__name__}): '
- f'{list(compress(field_names, [not v for v in valid_paths]))}'
- )
-
- for access_path2val in rows:
- doc_dict: Dict[Any, Any] = _access_path_dict_to_nested_dict(access_path2val)
- docs.append(doc_type.parse_obj(doc_dict))
-
- return docs
-
- def to_csv(
- self, file_path: str, dialect: Union[str, csv.Dialect] = 'excel'
- ) -> None:
- """
- Save a `DocList` to a csv file.
- The field names will be stored in the first row. Each row corresponds to the
- information of one Document.
- Columns for nested fields will be named after the "__"-seperated access paths,
- such as `'image__url'` for `image.url`.
-
- :param file_path: path to a csv file.
- :param dialect: defines separator and how to handle whitespaces etc.
- Can be a [`csv.Dialect`](https://docs.python.org/3/library/csv.html#csv.Dialect)
- instance or one string of:
- `'excel'` (for comma separated values),
- `'excel-tab'` (for tab separated values),
- `'unix'` (for csv file generated on UNIX systems).
-
- """
- if self.doc_type == AnyDoc:
- raise TypeError(
- 'DocList must be homogeneous to be converted to a csv.'
- 'There is no document schema defined. '
- 'Please specify the DocList\'s Document type using `DocList[MyDoc]`.'
- )
- fields = self.doc_type._get_access_paths()
-
- with open(file_path, 'w') as csv_file:
- writer = csv.DictWriter(csv_file, fieldnames=fields, dialect=dialect)
- writer.writeheader()
-
- for doc in self:
- doc_dict = _dict_to_access_paths(doc.dict())
- writer.writerow(doc_dict)
-
- @classmethod
- def from_dataframe(cls, df: 'pd.DataFrame') -> 'DocList':
- """
- Load a `DocList` from a `pandas.DataFrame` following the schema
- defined in the [`.doc_type`][docarray.DocList] attribute.
- Every row of the dataframe will be mapped to one Document in the doc_list.
- The column names of the dataframe have to match the field names of the
- Document type.
- For nested fields use "__"-separated access paths as column names,
- such as `'image__url'`.
-
- List-like fields (including field of type DocList) are not supported.
-
- ---
-
- ```python
- import pandas as pd
-
- from docarray import BaseDoc, DocList
-
-
- class Person(BaseDoc):
- name: str
- follower: int
-
-
- df = pd.DataFrame(
- data=[['Maria', 12345], ['Jake', 54321]], columns=['name', 'follower']
- )
-
- docs = DocList[Person].from_dataframe(df)
-
- assert docs.name == ['Maria', 'Jake']
- assert docs.follower == [12345, 54321]
- ```
-
- ---
-
- :param df: `pandas.DataFrame` to extract Document's information from
- :return: `DocList` where each Document contains the information of one
- corresponding row of the `pandas.DataFrame`.
- """
- from docarray import DocList
-
- if cls.doc_type == AnyDoc:
- raise TypeError(
- 'There is no document schema defined. '
- 'Please specify the DocList\'s Document type using `DocList[MyDoc]`.'
- )
-
- doc_type = cls.doc_type
- docs = DocList.__class_getitem__(doc_type)()
- field_names = df.columns.tolist()
-
- if field_names is None or len(field_names) == 0:
- raise TypeError("No field names are given.")
-
- valid_paths = _all_access_paths_valid(
- doc_type=doc_type, access_paths=field_names
- )
- if not all(valid_paths):
- raise ValueError(
- f'Column names do not match the schema of the DocList\'s '
- f'document type ({cls.doc_type.__name__}): '
- f'{list(compress(field_names, [not v for v in valid_paths]))}'
- )
-
- for row in df.itertuples():
- access_path2val = row._asdict()
- access_path2val.pop('index', None)
- doc_dict = _access_path_dict_to_nested_dict(access_path2val)
- docs.append(doc_type.parse_obj(doc_dict))
-
- return docs
-
- def to_dataframe(self) -> 'pd.DataFrame':
- """
- Save a DocList to a `pandas.DataFrame`.
- The field names will be stored as column names. Each row of the dataframe corresponds
- to the information of one Document.
- Columns for nested fields will be named after the "__"-seperated access paths,
- such as `'image__url'` for `image.url`.
-
- :return: `pandas.DataFrame`
- """
- if TYPE_CHECKING:
- import pandas as pd
- else:
- pd = import_library('pandas', raise_error=True)
-
- if self.doc_type == AnyDoc:
- raise TypeError(
- 'DocList must be homogeneous to be converted to a DataFrame.'
- 'There is no document schema defined. '
- 'Please specify the DocList\'s Document type using `DocList[MyDoc]`.'
- )
-
- fields = self.doc_type._get_access_paths()
- df = pd.DataFrame(columns=fields)
-
- for doc in self:
- doc_dict = _dict_to_access_paths(doc.dict())
- doc_dict = {k: [v] for k, v in doc_dict.items()}
- df = pd.concat([df, pd.DataFrame.from_dict(doc_dict)], ignore_index=True)
-
- return df
-
- # Methods to load from/to files in different formats
- @property
- def _stream_header(self) -> bytes:
- # Binary format for streaming case
-
- # V2 DocList streaming serialization format
- # | 1 byte | 8 bytes | 4 bytes | variable(docarray v2) | 4 bytes | variable(docarray v2) ...
-
- # 1 byte (uint8)
- version_byte = b'\x02'
- # 8 bytes (uint64)
- num_docs_as_bytes = len(self).to_bytes(8, 'big', signed=False)
- return version_byte + num_docs_as_bytes
-
- @classmethod
- def _load_binary_all(
- cls: Type[T],
- file_ctx: Union[ContextManager[io.BufferedReader], ContextManager[bytes]],
- protocol: Optional[str],
- compress: Optional[str],
- show_progress: bool,
- ):
- """Read a `DocList` object from a binary file
- :param protocol: protocol to use. It can be 'pickle-array', 'protobuf-array', 'pickle' or 'protobuf'
- :param compress: compress algorithm to use between `lz4`, `bz2`, `lzma`, `zlib`, `gzip`
- :param show_progress: show progress bar, only works when protocol is `pickle` or `protobuf`
- :return: a `DocList`
- """
- with file_ctx as fp:
- if isinstance(fp, bytes):
- d = fp
- else:
- d = fp.read()
-
- if protocol is not None and protocol in (
- 'pickle-array',
- 'protobuf-array',
- 'json-array',
- ):
- if _get_compress_ctx(algorithm=compress) is not None:
- d = _decompress_bytes(d, algorithm=compress)
- compress = None
-
- if protocol is not None and protocol == 'protobuf-array':
- from docarray.proto import DocListProto
-
- dap = DocListProto()
- dap.ParseFromString(d)
-
- return cls.from_protobuf(dap)
- elif protocol is not None and protocol == 'pickle-array':
- return pickle.loads(d)
-
- elif protocol is not None and protocol == 'json-array':
- return cls.from_json(d)
-
- # Binary format for streaming case
- else:
- from rich import filesize
-
- from docarray.utils._internal.progress_bar import _get_progressbar
-
- # 1 byte (uint8)
- version_num = int.from_bytes(d[0:1], 'big', signed=False)
- if version_num != 2:
- raise ValueError(
- f'Unsupported version number {version_num} in binary format, expected 2'
- )
-
- # 8 bytes (uint64)
- num_docs = int.from_bytes(d[1:9], 'big', signed=False)
-
- pbar, t = _get_progressbar(
- 'Deserializing', disable=not show_progress, total=num_docs
- )
-
- # this 9 is version + num_docs bytes used
- start_pos = 9
- docs = []
- with pbar:
- _total_size = 0
- pbar.start_task(t)
-
- for _ in range(num_docs):
- # 4 bytes (uint32)
- len_current_doc_in_bytes = int.from_bytes(
- d[start_pos : start_pos + 4], 'big', signed=False
- )
- start_doc_pos = start_pos + 4
- end_doc_pos = start_doc_pos + len_current_doc_in_bytes
- start_pos = end_doc_pos
-
- # variable length bytes doc
- load_protocol: str = protocol or 'protobuf'
- doc = cls.doc_type.from_bytes(
- d[start_doc_pos:end_doc_pos],
- protocol=load_protocol,
- compress=compress,
- )
- docs.append(doc)
- _total_size += len_current_doc_in_bytes
- pbar.update(
- t, advance=1, total_size=str(filesize.decimal(_total_size))
- )
- return cls(docs)
-
- @classmethod
- def _load_binary_stream(
- cls: Type[T],
- file_ctx: ContextManager[io.BufferedReader],
- protocol: str = 'protobuf',
- compress: Optional[str] = None,
- show_progress: bool = False,
- ) -> Generator['T_doc', None, None]:
- """Yield `Document` objects from a binary file
-
- :param protocol: protocol to use. It can be 'pickle' or 'protobuf'
- :param compress: compress algorithm to use between `lz4`, `bz2`, `lzma`, `zlib`, `gzip`
- :param show_progress: show progress bar, only works when protocol is `pickle` or `protobuf`
- :return: a generator of `Document` objects
- """
-
- from rich import filesize
-
- with file_ctx as f:
- version_numdocs_lendoc0 = f.read(9)
- # 1 byte (uint8)
- version_num = int.from_bytes(
- version_numdocs_lendoc0[0:1], 'big', signed=False
- )
- if version_num != 2:
- raise ValueError(
- f'Unsupported version number {version_num} in binary format, expected 2'
- )
-
- # 8 bytes (uint64)
- num_docs = int.from_bytes(version_numdocs_lendoc0[1:9], 'big', signed=False)
-
- if show_progress:
- from docarray.utils._internal.progress_bar import _get_progressbar
-
- pbar, t = _get_progressbar(
- 'Deserializing', disable=not show_progress, total=num_docs
- )
- else:
- from contextlib import nullcontext
-
- pbar = nullcontext()
-
- with pbar:
- if show_progress:
- _total_size = 0
- pbar.start_task(t)
- for _ in range(num_docs):
- # 4 bytes (uint32)
- len_current_doc_in_bytes = int.from_bytes(
- f.read(4), 'big', signed=False
- )
- load_protocol: str = protocol
- yield cls.doc_type.from_bytes(
- f.read(len_current_doc_in_bytes),
- protocol=load_protocol,
- compress=compress,
- )
- if show_progress:
- _total_size += len_current_doc_in_bytes
- pbar.update(
- t, advance=1, total_size=str(filesize.decimal(_total_size))
- )
-
- @classmethod
- def load_binary(
- cls: Type[T],
- file: Union[str, bytes, pathlib.Path, io.BufferedReader, _LazyRequestReader],
- protocol: str = 'protobuf-array',
- compress: Optional[str] = None,
- show_progress: bool = False,
- streaming: bool = False,
- ) -> Union[T, Generator['T_doc', None, None]]:
- """Load doc_list elements from a compressed binary file.
-
- In case protocol is pickle the `Documents` are streamed from disk to save memory usage
-
- !!! note
- If `file` is `str` it can specify `protocol` and `compress` as file extensions.
- This functionality assumes `file=file_name.$protocol.$compress` where `$protocol` and `$compress` refer to a
- string interpolation of the respective `protocol` and `compress` methods.
- For example if `file=my_docarray.protobuf.lz4` then the binary data will be loaded assuming `protocol=protobuf`
- and `compress=lz4`.
-
- :param file: File or filename or serialized bytes where the data is stored.
- :param protocol: protocol to use. It can be 'pickle-array', 'protobuf-array', 'pickle' or 'protobuf'
- :param compress: compress algorithm to use between `lz4`, `bz2`, `lzma`, `zlib`, `gzip`
- :param show_progress: show progress bar, only works when protocol is `pickle` or `protobuf`
- :param streaming: if `True` returns a generator over `Document` objects.
-
- :return: a `DocList` object
-
- """
- load_protocol: Optional[str] = protocol
- load_compress: Optional[str] = compress
- file_ctx: Union[nullcontext, io.BufferedReader]
- if isinstance(file, (io.BufferedReader, _LazyRequestReader, bytes)):
- file_ctx = nullcontext(file)
- # by checking path existence we allow file to be of type Path, LocalPath, PurePath and str
- elif isinstance(file, (str, pathlib.Path)) and os.path.exists(file):
- load_protocol, load_compress = _protocol_and_compress_from_file_path(
- file, protocol, compress
- )
- file_ctx = open(file, 'rb')
- else:
- raise FileNotFoundError(f'cannot find file {file}')
- if streaming:
- if load_protocol not in SINGLE_PROTOCOLS:
- raise ValueError(
- f'`streaming` is only available when using {" or ".join(map(lambda x: f"`{x}`", SINGLE_PROTOCOLS))} as protocol, '
- f'got {load_protocol}'
- )
- else:
- return cls._load_binary_stream(
- file_ctx,
- protocol=load_protocol,
- compress=load_compress,
- show_progress=show_progress,
- )
- else:
- return cls._load_binary_all(
- file_ctx, load_protocol, load_compress, show_progress
- )
-
- def save_binary(
- self,
- file: Union[str, pathlib.Path],
- protocol: str = 'protobuf-array',
- compress: Optional[str] = None,
- show_progress: bool = False,
- ) -> None:
- """Save DocList into a binary file.
-
- It will use the protocol to pick how to save the DocList.
- If used `picke-doc_list` and `protobuf-array` the DocList will be stored
- and compressed at complete level using `pickle` or `protobuf`.
- When using `protobuf` or `pickle` as protocol each Document in DocList
- will be stored individually and this would make it available for streaming.
-
- !!! note
- If `file` is `str` it can specify `protocol` and `compress` as file extensions.
- This functionality assumes `file=file_name.$protocol.$compress` where `$protocol` and `$compress` refer to a
- string interpolation of the respective `protocol` and `compress` methods.
- For example if `file=my_docarray.protobuf.lz4` then the binary data will be created using `protocol=protobuf`
- and `compress=lz4`.
-
- :param file: File or filename to which the data is saved.
- :param protocol: protocol to use. It can be 'pickle-array', 'protobuf-array', 'pickle' or 'protobuf'
- :param compress: compress algorithm to use between `lz4`, `bz2`, `lzma`, `zlib`, `gzip`
- :param show_progress: show progress bar, only works when protocol is `pickle` or `protobuf`
- """
- if isinstance(file, io.BufferedWriter):
- file_ctx = nullcontext(file)
- else:
- _protocol, _compress = _protocol_and_compress_from_file_path(file)
-
- if _protocol is not None:
- protocol = _protocol
- if _compress is not None:
- compress = _compress
-
- file_ctx = open(file, 'wb')
-
- self.to_bytes(
- protocol=protocol,
- compress=compress,
- file_ctx=file_ctx,
- show_progress=show_progress,
- )
diff --git a/spaces/Superlang/ImageProcessor/annotator/uniformer/configs/_base_/datasets/cityscapes_769x769.py b/spaces/Superlang/ImageProcessor/annotator/uniformer/configs/_base_/datasets/cityscapes_769x769.py
deleted file mode 100644
index 336c7b254fe392b4703039fec86a83acdbd2e1a5..0000000000000000000000000000000000000000
--- a/spaces/Superlang/ImageProcessor/annotator/uniformer/configs/_base_/datasets/cityscapes_769x769.py
+++ /dev/null
@@ -1,35 +0,0 @@
-_base_ = './cityscapes.py'
-img_norm_cfg = dict(
- mean=[123.675, 116.28, 103.53], std=[58.395, 57.12, 57.375], to_rgb=True)
-crop_size = (769, 769)
-train_pipeline = [
- dict(type='LoadImageFromFile'),
- dict(type='LoadAnnotations'),
- dict(type='Resize', img_scale=(2049, 1025), ratio_range=(0.5, 2.0)),
- dict(type='RandomCrop', crop_size=crop_size, cat_max_ratio=0.75),
- dict(type='RandomFlip', prob=0.5),
- dict(type='PhotoMetricDistortion'),
- dict(type='Normalize', **img_norm_cfg),
- dict(type='Pad', size=crop_size, pad_val=0, seg_pad_val=255),
- dict(type='DefaultFormatBundle'),
- dict(type='Collect', keys=['img', 'gt_semantic_seg']),
-]
-test_pipeline = [
- dict(type='LoadImageFromFile'),
- dict(
- type='MultiScaleFlipAug',
- img_scale=(2049, 1025),
- # img_ratios=[0.5, 0.75, 1.0, 1.25, 1.5, 1.75],
- flip=False,
- transforms=[
- dict(type='Resize', keep_ratio=True),
- dict(type='RandomFlip'),
- dict(type='Normalize', **img_norm_cfg),
- dict(type='ImageToTensor', keys=['img']),
- dict(type='Collect', keys=['img']),
- ])
-]
-data = dict(
- train=dict(pipeline=train_pipeline),
- val=dict(pipeline=test_pipeline),
- test=dict(pipeline=test_pipeline))
diff --git a/spaces/Surn/UnlimitedMusicGen/audiocraft/quantization/core_vq.py b/spaces/Surn/UnlimitedMusicGen/audiocraft/quantization/core_vq.py
deleted file mode 100644
index e1896bb1788a945a1f7be6369abb255ecf72c7a0..0000000000000000000000000000000000000000
--- a/spaces/Surn/UnlimitedMusicGen/audiocraft/quantization/core_vq.py
+++ /dev/null
@@ -1,400 +0,0 @@
-# Copyright (c) Meta Platforms, Inc. and affiliates.
-# All rights reserved.
-#
-# This source code is licensed under the license found in the
-# LICENSE file in the root directory of this source tree.
-
-import typing as tp
-
-from einops import rearrange, repeat
-import flashy
-import torch
-from torch import nn, einsum
-import torch.nn.functional as F
-
-
-def exists(val: tp.Optional[tp.Any]) -> bool:
- return val is not None
-
-
-def default(val: tp.Any, d: tp.Any) -> tp.Any:
- return val if exists(val) else d
-
-
-def l2norm(t):
- return F.normalize(t, p=2, dim=-1)
-
-
-def ema_inplace(moving_avg, new, decay: float):
- moving_avg.data.mul_(decay).add_(new, alpha=(1 - decay))
-
-
-def laplace_smoothing(x, n_categories: int, epsilon: float = 1e-5):
- return (x + epsilon) / (x.sum() + n_categories * epsilon)
-
-
-def uniform_init(*shape: int):
- t = torch.empty(shape)
- nn.init.kaiming_uniform_(t)
- return t
-
-
-def sample_vectors(samples, num: int):
- num_samples, device = samples.shape[0], samples.device
-
- if num_samples >= num:
- indices = torch.randperm(num_samples, device=device)[:num]
- else:
- indices = torch.randint(0, num_samples, (num,), device=device)
-
- return samples[indices]
-
-
-def kmeans(samples, num_clusters: int, num_iters: int = 10):
- dim, dtype = samples.shape[-1], samples.dtype
-
- means = sample_vectors(samples, num_clusters)
-
- for _ in range(num_iters):
- diffs = rearrange(samples, "n d -> n () d") - rearrange(
- means, "c d -> () c d"
- )
- dists = -(diffs ** 2).sum(dim=-1)
-
- buckets = dists.max(dim=-1).indices
- bins = torch.bincount(buckets, minlength=num_clusters)
- zero_mask = bins == 0
- bins_min_clamped = bins.masked_fill(zero_mask, 1)
-
- new_means = buckets.new_zeros(num_clusters, dim, dtype=dtype)
- new_means.scatter_add_(0, repeat(buckets, "n -> n d", d=dim), samples)
- new_means = new_means / bins_min_clamped[..., None]
-
- means = torch.where(zero_mask[..., None], means, new_means)
-
- return means, bins
-
-
-def orthgonal_loss_fn(t):
- # eq (2) from https://arxiv.org/abs/2112.00384
- n = t.shape[0]
- normed_codes = l2norm(t)
- identity = torch.eye(n, device=t.device)
- cosine_sim = einsum("i d, j d -> i j", normed_codes, normed_codes)
- return ((cosine_sim - identity) ** 2).sum() / (n ** 2)
-
-
-class EuclideanCodebook(nn.Module):
- """Codebook with Euclidean distance.
-
- Args:
- dim (int): Dimension.
- codebook_size (int): Codebook size.
- kmeans_init (bool): Whether to use k-means to initialize the codebooks.
- If set to true, run the k-means algorithm on the first training batch and use
- the learned centroids as initialization.
- kmeans_iters (int): Number of iterations used for k-means algorithm at initialization.
- decay (float): Decay for exponential moving average over the codebooks.
- epsilon (float): Epsilon value for numerical stability.
- threshold_ema_dead_code (int): Threshold for dead code expiration. Replace any codes
- that have an exponential moving average cluster size less than the specified threshold with
- randomly selected vector from the current batch.
- """
- def __init__(
- self,
- dim: int,
- codebook_size: int,
- kmeans_init: int = False,
- kmeans_iters: int = 10,
- decay: float = 0.8,
- epsilon: float = 1e-5,
- threshold_ema_dead_code: int = 2,
- ):
- super().__init__()
- self.decay = decay
- init_fn: tp.Union[tp.Callable[..., torch.Tensor], tp.Any] = uniform_init if not kmeans_init else torch.zeros
- embed = init_fn(codebook_size, dim)
-
- self.codebook_size = codebook_size
-
- self.kmeans_iters = kmeans_iters
- self.epsilon = epsilon
- self.threshold_ema_dead_code = threshold_ema_dead_code
-
- self.register_buffer("inited", torch.Tensor([not kmeans_init]))
- self.register_buffer("cluster_size", torch.zeros(codebook_size))
- self.register_buffer("embed", embed)
- self.register_buffer("embed_avg", embed.clone())
-
- @torch.jit.ignore
- def init_embed_(self, data):
- if self.inited:
- return
-
- embed, cluster_size = kmeans(data, self.codebook_size, self.kmeans_iters)
- self.embed.data.copy_(embed)
- self.embed_avg.data.copy_(embed.clone())
- self.cluster_size.data.copy_(cluster_size)
- self.inited.data.copy_(torch.Tensor([True]))
- # Make sure all buffers across workers are in sync after initialization
- flashy.distrib.broadcast_tensors(self.buffers())
-
- def replace_(self, samples, mask):
- modified_codebook = torch.where(
- mask[..., None], sample_vectors(samples, self.codebook_size), self.embed
- )
- self.embed.data.copy_(modified_codebook)
-
- def expire_codes_(self, batch_samples):
- if self.threshold_ema_dead_code == 0:
- return
-
- expired_codes = self.cluster_size < self.threshold_ema_dead_code
- if not torch.any(expired_codes):
- return
-
- batch_samples = rearrange(batch_samples, "... d -> (...) d")
- self.replace_(batch_samples, mask=expired_codes)
- flashy.distrib.broadcast_tensors(self.buffers())
-
- def preprocess(self, x):
- x = rearrange(x, "... d -> (...) d")
- return x
-
- def quantize(self, x):
- embed = self.embed.t()
- dist = -(
- x.pow(2).sum(1, keepdim=True)
- - 2 * x @ embed
- + embed.pow(2).sum(0, keepdim=True)
- )
- embed_ind = dist.max(dim=-1).indices
- return embed_ind
-
- def postprocess_emb(self, embed_ind, shape):
- return embed_ind.view(*shape[:-1])
-
- def dequantize(self, embed_ind):
- quantize = F.embedding(embed_ind, self.embed)
- return quantize
-
- def encode(self, x):
- shape = x.shape
- # pre-process
- x = self.preprocess(x)
- # quantize
- embed_ind = self.quantize(x)
- # post-process
- embed_ind = self.postprocess_emb(embed_ind, shape)
- return embed_ind
-
- def decode(self, embed_ind):
- quantize = self.dequantize(embed_ind)
- return quantize
-
- def forward(self, x):
- shape, dtype = x.shape, x.dtype
- x = self.preprocess(x)
- self.init_embed_(x)
-
- embed_ind = self.quantize(x)
- embed_onehot = F.one_hot(embed_ind, self.codebook_size).type(dtype)
- embed_ind = self.postprocess_emb(embed_ind, shape)
- quantize = self.dequantize(embed_ind)
-
- if self.training:
- # We do the expiry of code at that point as buffers are in sync
- # and all the workers will take the same decision.
- self.expire_codes_(x)
- ema_inplace(self.cluster_size, embed_onehot.sum(0), self.decay)
- embed_sum = x.t() @ embed_onehot
- ema_inplace(self.embed_avg, embed_sum.t(), self.decay)
- cluster_size = (
- laplace_smoothing(self.cluster_size, self.codebook_size, self.epsilon)
- * self.cluster_size.sum()
- )
- embed_normalized = self.embed_avg / cluster_size.unsqueeze(1)
- self.embed.data.copy_(embed_normalized)
-
- return quantize, embed_ind
-
-
-class VectorQuantization(nn.Module):
- """Vector quantization implementation.
- Currently supports only euclidean distance.
-
- Args:
- dim (int): Dimension
- codebook_size (int): Codebook size
- codebook_dim (int): Codebook dimension. If not defined, uses the specified dimension in dim.
- decay (float): Decay for exponential moving average over the codebooks.
- epsilon (float): Epsilon value for numerical stability.
- kmeans_init (bool): Whether to use kmeans to initialize the codebooks.
- kmeans_iters (int): Number of iterations used for kmeans initialization.
- threshold_ema_dead_code (int):
- channels_last (bool): Channels are the last dimension in the input tensors.
- commitment_weight (float): Weight for commitment loss.
- orthogonal_reg_weight (float): Orthogonal regularization weights.
- orthogonal_reg_active_codes_only (bool): Apply orthogonal regularization only on active codes.
- orthogonal_reg_max_codes (optional int): Maximum number of codes to consider
- for orthogonal regulariation.
- threshold_ema_dead_code (int): Threshold for dead code expiration. Replace any codes
- that have an exponential moving average cluster size less than the specified threshold with
- randomly selected vector from the current batch.
- """
- def __init__(
- self,
- dim: int,
- codebook_size: int,
- codebook_dim: tp.Optional[int] = None,
- decay: float = 0.8,
- epsilon: float = 1e-5,
- kmeans_init: bool = False,
- kmeans_iters: int = 10,
- threshold_ema_dead_code: int = 2,
- channels_last: bool = False,
- commitment_weight: float = 1.,
- orthogonal_reg_weight: float = 0.0,
- orthogonal_reg_active_codes_only: bool = False,
- orthogonal_reg_max_codes: tp.Optional[int] = None,
- ):
- super().__init__()
- _codebook_dim: int = default(codebook_dim, dim)
-
- requires_projection = _codebook_dim != dim
- self.project_in = (nn.Linear(dim, _codebook_dim) if requires_projection else nn.Identity())
- self.project_out = (nn.Linear(_codebook_dim, dim) if requires_projection else nn.Identity())
-
- self.epsilon = epsilon
- self.commitment_weight = commitment_weight
-
- self.orthogonal_reg_weight = orthogonal_reg_weight
- self.orthogonal_reg_active_codes_only = orthogonal_reg_active_codes_only
- self.orthogonal_reg_max_codes = orthogonal_reg_max_codes
-
- self._codebook = EuclideanCodebook(dim=_codebook_dim, codebook_size=codebook_size,
- kmeans_init=kmeans_init, kmeans_iters=kmeans_iters,
- decay=decay, epsilon=epsilon,
- threshold_ema_dead_code=threshold_ema_dead_code)
- self.codebook_size = codebook_size
-
- self.channels_last = channels_last
-
- @property
- def codebook(self):
- return self._codebook.embed
-
- @property
- def inited(self):
- return self._codebook.inited
-
- def _preprocess(self, x):
- if not self.channels_last:
- x = rearrange(x, "b d n -> b n d")
- return x
-
- def _postprocess(self, quantize):
- if not self.channels_last:
- quantize = rearrange(quantize, "b n d -> b d n")
- return quantize
-
- def encode(self, x):
- x = self._preprocess(x)
- x = self.project_in(x)
- embed_in = self._codebook.encode(x)
- return embed_in
-
- def decode(self, embed_ind):
- quantize = self._codebook.decode(embed_ind)
- quantize = self.project_out(quantize)
- quantize = self._postprocess(quantize)
- return quantize
-
- def forward(self, x):
- device = x.device
- x = self._preprocess(x)
-
- x = self.project_in(x)
- quantize, embed_ind = self._codebook(x)
-
- if self.training:
- quantize = x + (quantize - x).detach()
-
- loss = torch.tensor([0.0], device=device, requires_grad=self.training)
-
- if self.training:
- if self.commitment_weight > 0:
- commit_loss = F.mse_loss(quantize.detach(), x)
- loss = loss + commit_loss * self.commitment_weight
-
- if self.orthogonal_reg_weight > 0:
- codebook = self.codebook
-
- if self.orthogonal_reg_active_codes_only:
- # only calculate orthogonal loss for the activated codes for this batch
- unique_code_ids = torch.unique(embed_ind)
- codebook = codebook[unique_code_ids]
-
- num_codes = codebook.shape[0]
- if exists(self.orthogonal_reg_max_codes) and num_codes > self.orthogonal_reg_max_codes:
- rand_ids = torch.randperm(num_codes, device=device)[:self.orthogonal_reg_max_codes]
- codebook = codebook[rand_ids]
-
- orthogonal_reg_loss = orthgonal_loss_fn(codebook)
- loss = loss + orthogonal_reg_loss * self.orthogonal_reg_weight
-
- quantize = self.project_out(quantize)
- quantize = self._postprocess(quantize)
-
- return quantize, embed_ind, loss
-
-
-class ResidualVectorQuantization(nn.Module):
- """Residual vector quantization implementation.
-
- Follows Algorithm 1. in https://arxiv.org/pdf/2107.03312.pdf
- """
- def __init__(self, *, num_quantizers, **kwargs):
- super().__init__()
- self.layers = nn.ModuleList(
- [VectorQuantization(**kwargs) for _ in range(num_quantizers)]
- )
-
- def forward(self, x, n_q: tp.Optional[int] = None):
- quantized_out = 0.0
- residual = x
-
- all_losses = []
- all_indices = []
-
- n_q = n_q or len(self.layers)
-
- for i, layer in enumerate(self.layers[:n_q]):
- quantized, indices, loss = layer(residual)
- residual = residual - quantized
- quantized_out = quantized_out + quantized
- all_indices.append(indices)
- all_losses.append(loss)
-
- out_losses, out_indices = map(torch.stack, (all_losses, all_indices))
- return quantized_out, out_indices, out_losses
-
- def encode(self, x: torch.Tensor, n_q: tp.Optional[int] = None) -> torch.Tensor:
- residual = x
- all_indices = []
- n_q = n_q or len(self.layers)
- for layer in self.layers[:n_q]:
- indices = layer.encode(residual)
- quantized = layer.decode(indices)
- residual = residual - quantized
- all_indices.append(indices)
- out_indices = torch.stack(all_indices)
- return out_indices
-
- def decode(self, q_indices: torch.Tensor) -> torch.Tensor:
- quantized_out = torch.tensor(0.0, device=q_indices.device)
- for i, indices in enumerate(q_indices):
- layer = self.layers[i]
- quantized = layer.decode(indices)
- quantized_out = quantized_out + quantized
- return quantized_out
diff --git a/spaces/TH5314/newbing/src/components/welcome-screen.tsx b/spaces/TH5314/newbing/src/components/welcome-screen.tsx
deleted file mode 100644
index f7449fcbb6c621875e235db98f2790bf7894fb0a..0000000000000000000000000000000000000000
--- a/spaces/TH5314/newbing/src/components/welcome-screen.tsx
+++ /dev/null
@@ -1,34 +0,0 @@
-import { useBing } from '@/lib/hooks/use-bing'
-
-const exampleMessages = [
- {
- heading: '🧐 提出复杂问题',
- message: `我可以为我挑剔的只吃橙色食物的孩子做什么饭?`
- },
- {
- heading: '🙌 获取更好的答案',
- message: '销量最高的 3 种宠物吸尘器有哪些优点和缺点?'
- },
- {
- heading: '🎨 获得创意灵感',
- message: `以海盗的口吻写一首关于外太空鳄鱼的俳句`
- }
-]
-
-export function WelcomeScreen({ setInput }: Pick, 'setInput'>) {
- return (
-
- {exampleMessages.map(example => (
-
setInput(example.message)}>
- {example.heading}
-
-
-
-
“{example.message}”
-
-
-
- ))}
-
- )
-}
diff --git a/spaces/TandCAcceptMe/face-swap-docker/mynewshinyroop/Lib/site-packages/pip/_internal/resolution/resolvelib/base.py b/spaces/TandCAcceptMe/face-swap-docker/mynewshinyroop/Lib/site-packages/pip/_internal/resolution/resolvelib/base.py
deleted file mode 100644
index b206692a0a976d8336e3f5896eadf4765a33fb2c..0000000000000000000000000000000000000000
--- a/spaces/TandCAcceptMe/face-swap-docker/mynewshinyroop/Lib/site-packages/pip/_internal/resolution/resolvelib/base.py
+++ /dev/null
@@ -1,141 +0,0 @@
-from typing import FrozenSet, Iterable, Optional, Tuple, Union
-
-from pip._vendor.packaging.specifiers import SpecifierSet
-from pip._vendor.packaging.utils import NormalizedName, canonicalize_name
-from pip._vendor.packaging.version import LegacyVersion, Version
-
-from pip._internal.models.link import Link, links_equivalent
-from pip._internal.req.req_install import InstallRequirement
-from pip._internal.utils.hashes import Hashes
-
-CandidateLookup = Tuple[Optional["Candidate"], Optional[InstallRequirement]]
-CandidateVersion = Union[LegacyVersion, Version]
-
-
-def format_name(project: str, extras: FrozenSet[str]) -> str:
- if not extras:
- return project
- canonical_extras = sorted(canonicalize_name(e) for e in extras)
- return "{}[{}]".format(project, ",".join(canonical_extras))
-
-
-class Constraint:
- def __init__(
- self, specifier: SpecifierSet, hashes: Hashes, links: FrozenSet[Link]
- ) -> None:
- self.specifier = specifier
- self.hashes = hashes
- self.links = links
-
- @classmethod
- def empty(cls) -> "Constraint":
- return Constraint(SpecifierSet(), Hashes(), frozenset())
-
- @classmethod
- def from_ireq(cls, ireq: InstallRequirement) -> "Constraint":
- links = frozenset([ireq.link]) if ireq.link else frozenset()
- return Constraint(ireq.specifier, ireq.hashes(trust_internet=False), links)
-
- def __bool__(self) -> bool:
- return bool(self.specifier) or bool(self.hashes) or bool(self.links)
-
- def __and__(self, other: InstallRequirement) -> "Constraint":
- if not isinstance(other, InstallRequirement):
- return NotImplemented
- specifier = self.specifier & other.specifier
- hashes = self.hashes & other.hashes(trust_internet=False)
- links = self.links
- if other.link:
- links = links.union([other.link])
- return Constraint(specifier, hashes, links)
-
- def is_satisfied_by(self, candidate: "Candidate") -> bool:
- # Reject if there are any mismatched URL constraints on this package.
- if self.links and not all(_match_link(link, candidate) for link in self.links):
- return False
- # We can safely always allow prereleases here since PackageFinder
- # already implements the prerelease logic, and would have filtered out
- # prerelease candidates if the user does not expect them.
- return self.specifier.contains(candidate.version, prereleases=True)
-
-
-class Requirement:
- @property
- def project_name(self) -> NormalizedName:
- """The "project name" of a requirement.
-
- This is different from ``name`` if this requirement contains extras,
- in which case ``name`` would contain the ``[...]`` part, while this
- refers to the name of the project.
- """
- raise NotImplementedError("Subclass should override")
-
- @property
- def name(self) -> str:
- """The name identifying this requirement in the resolver.
-
- This is different from ``project_name`` if this requirement contains
- extras, where ``project_name`` would not contain the ``[...]`` part.
- """
- raise NotImplementedError("Subclass should override")
-
- def is_satisfied_by(self, candidate: "Candidate") -> bool:
- return False
-
- def get_candidate_lookup(self) -> CandidateLookup:
- raise NotImplementedError("Subclass should override")
-
- def format_for_error(self) -> str:
- raise NotImplementedError("Subclass should override")
-
-
-def _match_link(link: Link, candidate: "Candidate") -> bool:
- if candidate.source_link:
- return links_equivalent(link, candidate.source_link)
- return False
-
-
-class Candidate:
- @property
- def project_name(self) -> NormalizedName:
- """The "project name" of the candidate.
-
- This is different from ``name`` if this candidate contains extras,
- in which case ``name`` would contain the ``[...]`` part, while this
- refers to the name of the project.
- """
- raise NotImplementedError("Override in subclass")
-
- @property
- def name(self) -> str:
- """The name identifying this candidate in the resolver.
-
- This is different from ``project_name`` if this candidate contains
- extras, where ``project_name`` would not contain the ``[...]`` part.
- """
- raise NotImplementedError("Override in subclass")
-
- @property
- def version(self) -> CandidateVersion:
- raise NotImplementedError("Override in subclass")
-
- @property
- def is_installed(self) -> bool:
- raise NotImplementedError("Override in subclass")
-
- @property
- def is_editable(self) -> bool:
- raise NotImplementedError("Override in subclass")
-
- @property
- def source_link(self) -> Optional[Link]:
- raise NotImplementedError("Override in subclass")
-
- def iter_dependencies(self, with_requires: bool) -> Iterable[Optional[Requirement]]:
- raise NotImplementedError("Override in subclass")
-
- def get_install_requirement(self) -> Optional[InstallRequirement]:
- raise NotImplementedError("Override in subclass")
-
- def format_for_error(self) -> str:
- raise NotImplementedError("Subclass should override")
diff --git a/spaces/TempoFunk/makeavid-sd-jax/makeavid_sd/torch_impl/torch_attention_pseudo3d.py b/spaces/TempoFunk/makeavid-sd-jax/makeavid_sd/torch_impl/torch_attention_pseudo3d.py
deleted file mode 100644
index 9f6fd30c932b21e58fba730c3a6d7604f4631a97..0000000000000000000000000000000000000000
--- a/spaces/TempoFunk/makeavid-sd-jax/makeavid_sd/torch_impl/torch_attention_pseudo3d.py
+++ /dev/null
@@ -1,294 +0,0 @@
-from typing import Optional
-
-import torch
-import torch.nn.functional as F
-from torch import nn
-
-from einops import rearrange
-
-from diffusers.models.attention_processor import Attention as CrossAttention
-#from torch_cross_attention import CrossAttention
-
-
-class TransformerPseudo3DModelOutput:
- def __init__(self, sample: torch.FloatTensor) -> None:
- self.sample = sample
-
-
-class TransformerPseudo3DModel(nn.Module):
- def __init__(self,
- num_attention_heads: int = 16,
- attention_head_dim: int = 88,
- in_channels: Optional[int] = None,
- num_layers: int = 1,
- dropout: float = 0.0,
- norm_num_groups: int = 32,
- cross_attention_dim: Optional[int] = None,
- attention_bias: bool = False
- ) -> None:
- super().__init__()
- self.num_attention_heads = num_attention_heads
- self.attention_head_dim = attention_head_dim
- inner_dim = num_attention_heads * attention_head_dim
-
- # 1. Transformer2DModel can process both standard continous images of shape `(batch_size, num_channels, width, height)` as well as quantized image embeddings of shape `(batch_size, num_image_vectors)`
- # Define whether input is continuous or discrete depending on configuration
- # its continuous
-
- # 2. Define input layers
- self.in_channels = in_channels
-
- self.norm = torch.nn.GroupNorm(
- num_groups = norm_num_groups,
- num_channels = in_channels,
- eps = 1e-6,
- affine = True
- )
- self.proj_in = nn.Conv2d(
- in_channels,
- inner_dim,
- kernel_size = 1,
- stride = 1,
- padding = 0
- )
-
- # 3. Define transformers blocks
- self.transformer_blocks = nn.ModuleList(
- [
- BasicTransformerBlock(
- inner_dim,
- num_attention_heads,
- attention_head_dim,
- dropout = dropout,
- cross_attention_dim = cross_attention_dim,
- attention_bias = attention_bias,
- )
- for _ in range(num_layers)
- ]
- )
-
- # 4. Define output layers
- self.proj_out = nn.Conv2d(inner_dim, in_channels, kernel_size = 1, stride = 1, padding = 0)
-
- def forward(self,
- hidden_states: torch.Tensor,
- encoder_hidden_states: Optional[torch.Tensor] = None,
- timestep: torch.long = None
- ) -> TransformerPseudo3DModelOutput:
- """
- Args:
- hidden_states ( When discrete, `torch.LongTensor` of shape `(batch size, num latent pixels)`.
- When continous, `torch.FloatTensor` of shape `(batch size, channel, height, width)`): Input
- hidden_states
- encoder_hidden_states ( `torch.LongTensor` of shape `(batch size, context dim)`, *optional*):
- Conditional embeddings for cross attention layer. If not given, cross-attention defaults to
- self-attention.
- timestep ( `torch.long`, *optional*):
- Optional timestep to be applied as an embedding in AdaLayerNorm's. Used to indicate denoising step.
- return_dict (`bool`, *optional*, defaults to `True`):
- Whether or not to return a [`models.unet_2d_condition.UNet2DConditionOutput`] instead of a plain tuple.
-
- Returns:
- [`~models.attention.Transformer2DModelOutput`] or `tuple`: [`~models.attention.Transformer2DModelOutput`]
- if `return_dict` is True, otherwise a `tuple`. When returning a tuple, the first element is the sample
- tensor.
- """
- b, c, *_, h, w = hidden_states.shape
- is_video = hidden_states.ndim == 5
- f = None
- if is_video:
- b, c, f, h, w = hidden_states.shape
- hidden_states = rearrange(hidden_states, 'b c f h w -> (b f) c h w')
- #encoder_hidden_states = encoder_hidden_states.repeat_interleave(f, 0)
-
- # 1. Input
- batch, channel, height, weight = hidden_states.shape
- residual = hidden_states
- hidden_states = self.norm(hidden_states)
- hidden_states = self.proj_in(hidden_states)
- inner_dim = hidden_states.shape[1]
- hidden_states = hidden_states.permute(0, 2, 3, 1).reshape(batch, height * weight, inner_dim)
-
- # 2. Blocks
- for block in self.transformer_blocks:
- hidden_states = block(
- hidden_states,
- context = encoder_hidden_states,
- timestep = timestep,
- frames_length = f,
- height = height,
- weight = weight
- )
-
- # 3. Output
- hidden_states = hidden_states.reshape(batch, height, weight, inner_dim).permute(0, 3, 1, 2)
- hidden_states = self.proj_out(hidden_states)
- output = hidden_states + residual
-
- if is_video:
- output = rearrange(output, '(b f) c h w -> b c f h w', b = b)
-
- return TransformerPseudo3DModelOutput(sample = output)
-
-
-
-class BasicTransformerBlock(nn.Module):
- r"""
- A basic Transformer block.
-
- Parameters:
- dim (`int`): The number of channels in the input and output.
- num_attention_heads (`int`): The number of heads to use for multi-head attention.
- attention_head_dim (`int`): The number of channels in each head.
- dropout (`float`, *optional*, defaults to 0.0): The dropout probability to use.
- cross_attention_dim (`int`, *optional*): The size of the context vector for cross attention.
- num_embeds_ada_norm (:
- obj: `int`, *optional*): The number of diffusion steps used during training. See `Transformer2DModel`.
- attention_bias (:
- obj: `bool`, *optional*, defaults to `False`): Configure if the attentions should contain a bias parameter.
- """
-
- def __init__(self,
- dim: int,
- num_attention_heads: int,
- attention_head_dim: int,
- dropout: float = 0.0,
- cross_attention_dim: Optional[int] = None,
- attention_bias: bool = False,
- ) -> None:
- super().__init__()
- self.attn1 = CrossAttention(
- query_dim = dim,
- heads = num_attention_heads,
- dim_head = attention_head_dim,
- dropout = dropout,
- bias = attention_bias
- ) # is a self-attention
- self.ff = FeedForward(dim, dropout = dropout)
- self.attn2 = CrossAttention(
- query_dim = dim,
- cross_attention_dim = cross_attention_dim,
- heads = num_attention_heads,
- dim_head = attention_head_dim,
- dropout = dropout,
- bias = attention_bias
- ) # is self-attn if context is none
- self.attn_temporal = CrossAttention(
- query_dim = dim,
- heads = num_attention_heads,
- dim_head = attention_head_dim,
- dropout = dropout,
- bias = attention_bias
- ) # is a self-attention
-
- # layer norms
- self.norm1 = nn.LayerNorm(dim)
- self.norm2 = nn.LayerNorm(dim)
- self.norm_temporal = nn.LayerNorm(dim)
- self.norm3 = nn.LayerNorm(dim)
-
- def forward(self,
- hidden_states: torch.Tensor,
- context: Optional[torch.Tensor] = None,
- timestep: torch.int64 = None,
- frames_length: Optional[int] = None,
- height: Optional[int] = None,
- weight: Optional[int] = None
- ) -> torch.Tensor:
- if context is not None and frames_length is not None:
- context = context.repeat_interleave(frames_length, 0)
- # 1. Self-Attention
- norm_hidden_states = (
- self.norm1(hidden_states)
- )
- hidden_states = self.attn1(norm_hidden_states) + hidden_states
-
- # 2. Cross-Attention
- norm_hidden_states = (
- self.norm2(hidden_states)
- )
- hidden_states = self.attn2(
- norm_hidden_states,
- encoder_hidden_states = context
- ) + hidden_states
-
- # append temporal attention
- if frames_length is not None:
- hidden_states = rearrange(
- hidden_states,
- '(b f) (h w) c -> (b h w) f c',
- f = frames_length,
- h = height,
- w = weight
- )
- norm_hidden_states = (
- self.norm_temporal(hidden_states)
- )
- hidden_states = self.attn_temporal(norm_hidden_states) + hidden_states
- hidden_states = rearrange(
- hidden_states,
- '(b h w) f c -> (b f) (h w) c',
- f = frames_length,
- h = height,
- w = weight
- )
-
- # 3. Feed-forward
- hidden_states = self.ff(self.norm3(hidden_states)) + hidden_states
- return hidden_states
-
-
-class FeedForward(nn.Module):
- r"""
- A feed-forward layer.
-
- Parameters:
- dim (`int`): The number of channels in the input.
- dim_out (`int`, *optional*): The number of channels in the output. If not given, defaults to `dim`.
- mult (`int`, *optional*, defaults to 4): The multiplier to use for the hidden dimension.
- dropout (`float`, *optional*, defaults to 0.0): The dropout probability to use.
- """
-
- def __init__(self,
- dim: int,
- dim_out: Optional[int] = None,
- mult: int = 4,
- dropout: float = 0.0
- ) -> None:
- super().__init__()
- inner_dim = int(dim * mult)
- dim_out = dim_out if dim_out is not None else dim
-
- geglu = GEGLU(dim, inner_dim)
-
- self.net = nn.ModuleList([])
- # project in
- self.net.append(geglu)
- # project dropout
- self.net.append(nn.Dropout(dropout))
- # project out
- self.net.append(nn.Linear(inner_dim, dim_out))
-
- def forward(self, hidden_states: torch.Tensor) -> torch.Tensor:
- for module in self.net:
- hidden_states = module(hidden_states)
- return hidden_states
-
-
-# feedforward
-class GEGLU(nn.Module):
- r"""
- A variant of the gated linear unit activation function from https://arxiv.org/abs/2002.05202.
-
- Parameters:
- dim_in (`int`): The number of channels in the input.
- dim_out (`int`): The number of channels in the output.
- """
-
- def __init__(self, dim_in: int, dim_out: int) -> None:
- super().__init__()
- self.proj = nn.Linear(dim_in, dim_out * 2)
-
- def forward(self, hidden_states: torch.Tensor) -> torch.Tensor:
- hidden_states, gate = self.proj(hidden_states).chunk(2, dim = -1)
- return hidden_states * F.gelu(gate)
diff --git a/spaces/TeraTTS/TTS/app.py b/spaces/TeraTTS/TTS/app.py
deleted file mode 100644
index 958ac40cfee33adc55ca0c45afd26ca2c893643b..0000000000000000000000000000000000000000
--- a/spaces/TeraTTS/TTS/app.py
+++ /dev/null
@@ -1,39 +0,0 @@
-import gradio as gr
-from infer_onnx import TTS
-from ruaccent import RUAccent # https://huggingface.co/TeraTTS/accentuator
-
-# Заголовок и ссылка на репозиторий с моделями
-title = "GitHub with models: https://github.com/Tera2Space/RUTTS"
-
-# Список моделей TTS для выбора
-models = ["TeraTTS/natasha-g2p-vits", "TeraTTS/glados2-g2p-vits", "TeraTTS/glados-g2p-vits", "TeraTTS/girl_nice-g2p-vits"]
-
-# Создаем словарь моделей и инициализируем их
-models = {k: TTS(k) for k in models}
-
-# Создаем объект для акцентуации текста
-accentizer = RUAccent(workdir="./model/ruaccent")
-accentizer.load(omograph_model_size='big_poetry', use_dictionary=True)
-
-# Функция для предобработки текста (акцентуация и ё)
-
-# Функция для синтеза речи
-def text_to_speech(model_name, length_scale, text, prep_text):
- if prep_text:
- text = accentizer.process_all(text)
- audio = models[model_name](text, length_scale=length_scale)
- models[model_name].save_wav(audio, 'temp.wav', sample_rate=models[model_name].config["samplerate"])
-
- return 'temp.wav', f"Обработанный текст: '{text}'"
-
-# Создание интерфейса Gradio
-model_choice = gr.Dropdown(choices=list(models.keys()), value="TeraTTS/natasha-g2p-vits", label="Выберите модель")
-input_text = gr.Textbox(label="Введите текст для синтеза речи")
-prep_text = gr.Checkbox(label="Предобработать", info="Хотите предобработать текст? (ударения, ё)", value=True)
-length_scale = gr.Slider(minimum=0.1, maximum=2.0, label="Length scale (увеличить длину звучания) По умолчанию: 1.2", value=1.2)
-
-output_audio = gr.Audio(label="Аудио", type="numpy")
-output_text = gr.Textbox(label="Обработанный текст")
-
-iface = gr.Interface(fn=text_to_speech, inputs=[model_choice, length_scale, input_text, prep_text], outputs=[output_audio, output_text], title=title)
-iface.launch()
\ No newline at end of file
diff --git a/spaces/Tihsrah/Meetings/app.py b/spaces/Tihsrah/Meetings/app.py
deleted file mode 100644
index 0e379941dc3161317b760a3f7593dc12f0d3f1c3..0000000000000000000000000000000000000000
--- a/spaces/Tihsrah/Meetings/app.py
+++ /dev/null
@@ -1,214 +0,0 @@
-import subprocess
-# # Run the pip install command
-subprocess.check_call(['pip', 'install', 'wordcloud'])
-subprocess.check_call(['pip', 'install', 'git+https://github.com/openai/whisper.git'])
-subprocess.check_call(['pip', 'install', 'transformers'])
-subprocess.check_call(['pip', 'install', 'imageio==2.4.1'])
-subprocess.check_call(['pip', 'install', 'moviepy'])
-subprocess.check_call(['pip', 'install', 'keybert'])
-subprocess.check_call(['pip', 'install', 'pytube'])
-
-import streamlit as st
-import os
-from wordcloud import WordCloud
-from keybert import KeyBERT
-import pandas as pd
-import matplotlib.pyplot as plt
-# //////////////////////////////////////////////////////////////////////////////////////////////////////////////////////////
-
-
-from moviepy.editor import *
-from tqdm import tqdm
-import os
-import math
-import nltk
-nltk.download('punkt')
-import whisper
-from transformers import pipeline
-
-from pytube import YouTube
-def process_video(path):
- whisper_model = whisper.load_model("base")
-
- def SpeechToTextEng(aud_path):
- result = whisper_model.transcribe(aud_path)
- return result["text"]
-
- def run_range(duration):
- time=duration/60
- floor=math.ceil(time)
- return floor
-
- time_range=60
- clip_run_range=0
- clip_duration=0
-
- def audio_generator(path,aud=0,vid=0):
- if vid==1:
- clip=VideoFileClip(path)
- clip_duration = clip.duration
- clip_run_range=run_range(clip_duration)
- for i in range(clip_run_range):
- left=i*time_range
- right=left+time_range
- # print(left,right)
-
- crop_clip=clip.subclip(left,right)
- try:
- crop_clip.audio.write_audiofile("vid_to_aud"+str(i)+".mp3")
- except:
- pass
-
- if aud==1:
- audio_clip=AudioFileClip(path)
- clip_duration = audio_clip.duration
- print(clip_duration)
- clip_run_range=run_range(clip_duration)
- print(clip_run_range)
- for i in range(clip_run_range):
- left=i*time_range
- right=left+time_range
- # print(left,right)
- crop_clip=audio_clip.subclip(left,right)
- try:
- crop_clip.write_audiofile("vid_to_aud"+str(i)+".mp3")
- except:
- pass
-
-
-
-
- # YouTube video URL
- video_url = path
-
- # Create a YouTube object
- yt = YouTube(video_url)
-
- # Get the highest resolution video stream
- stream = yt.streams.get_lowest_resolution()
-
- # Download the video
- stream.download(filename='meeting.mp4')
-
- audio_generator("./meeting.mp4",vid=1)
- transcribed_lit=[]
- label_lit=[]
- translated_lit=[]
-
- for i in tqdm(range(clip_run_range)):
- transcribed=SpeechToTextEng("./vid_to_aud"+str(i)+".mp3")
- transcribed_lit.append(transcribed)
- os.remove("./vid_to_aud"+str(i)+".mp3")
-
-
- data = pd.DataFrame(
- {'transcriptions': transcribed_lit
- })
-
- summarizer = pipeline("summarization")
-
- sentiment_analyzer = pipeline("sentiment-analysis")
-
- sumarized_lit=[]
- sentiment_lit=[]
- for i in tqdm(range(len(data))):
- summarized=summarizer(data.iloc[i,0],min_length=75, max_length=300)[0]['summary_text']
- sentiment = sentiment_analyzer(data.iloc[i,0])[0]['label']
- sumarized_lit.append(summarized)
- sentiment_lit.append(sentiment)
-
- data['summary']=sumarized_lit
- data['sentiment']=sentiment_lit
- data.to_csv('output2.csv', index=False)
- tot_text=""
- for i in range(len(data)):
- tot_text=tot_text+data.iloc[i,0]
-
- key_model = KeyBERT('distilbert-base-nli-mean-tokens')
- def extract_keywords(text, top_n=50):
- keywords = key_model.extract_keywords(text, top_n=top_n)
- return [keyword[0] for keyword in keywords]
-
- tot_keywords=extract_keywords(tot_text)
-
- def get_500_words(text,left,right):
- words = text.split()
- first_500_words = ' '.join(words[left:right])
- return first_500_words
-
- def summarize_text(text):
- chunk_size = 500 # Number of words per chunk
- total_summary = "" # Total summary
-
- words = text.split() # Split the text into individual words
- num_chunks = len(words) // chunk_size + 1 # Calculate the number of chunks
-
- for i in tqdm(range(num_chunks)):
- start_index = i * chunk_size
- end_index = start_index + chunk_size
- chunk = " ".join(words[start_index:end_index])
-
- # Pass the chunk to the summarizer (replace with your summarization code)
- chunk_summary = summarizer(chunk,min_length=75, max_length=200)[0]['summary_text']
- # print(chunk_summary)
- total_summary += chunk_summary
-
- return total_summary
-
- tot_summary=summarize_text(tot_text)
- return tot_text,tot_summary,tot_keywords
-
-
-
-
-# //////////////////////////////////////////////////////////////////////////////////////////////////////////////////////////
-def generate_word_cloud(text):
- # Create a WordCloud object
- wordcloud = WordCloud(width=800, height=400, background_color='white').generate(text)
-
- # Display the generated word cloud
- fig, ax = plt.subplots(figsize=(10, 5))
-
- # Plot the word cloud on the axis
- ax.imshow(wordcloud, interpolation='bilinear')
- ax.axis('off')
- st.pyplot(fig)
-
-
-def main():
- st.title("Meeting Summary Web App")
-
- # YouTube link input
- youtube_url = st.text_input("Enter the YouTube video link")
-
- if st.button("Process Video"):
- if youtube_url:
- # Process the YouTube video
- tot_text, tot_summary, tot_keywords = process_video(youtube_url)
-
- # Display the output
- if os.path.exists("output2.csv"):
- output_df = pd.read_csv("output2.csv")
- st.subheader("Transcriptions:")
- st.write(output_df["transcriptions"])
-
- st.subheader("Labels:")
- st.write(output_df["labels"])
-
- st.subheader("Word Cloud:")
- generate_word_cloud(output_df["transcriptions"].str.cat(sep=' '))
-
- st.subheader("tot_text:")
- st.write(tot_text)
-
- st.subheader("tot_summary:")
- st.write(tot_summary)
-
- st.subheader("tot_keywords:")
- st.write(tot_keywords)
-
- else:
- st.write("No output file found.")
-
-if __name__ == "__main__":
- main()
\ No newline at end of file
diff --git a/spaces/Tuana/find-the-animal/README.md b/spaces/Tuana/find-the-animal/README.md
deleted file mode 100644
index 09b19eaa56943906c9d52761cc8b99e5e7784160..0000000000000000000000000000000000000000
--- a/spaces/Tuana/find-the-animal/README.md
+++ /dev/null
@@ -1,10 +0,0 @@
----
-title: MultiModalRetrieval for Image Search
-emoji: 🦒
-colorFrom: green
-colorTo: yellow
-sdk: streamlit
-sdk_version: 1.10.0
-app_file: 🏡_Home.py
-pinned: false
----
\ No newline at end of file
diff --git a/spaces/VideoCrafter/VideoCrafter/lvdm/distributions.py b/spaces/VideoCrafter/VideoCrafter/lvdm/distributions.py
deleted file mode 100644
index 0b69b6984880ec24279b658384ed8031335e3474..0000000000000000000000000000000000000000
--- a/spaces/VideoCrafter/VideoCrafter/lvdm/distributions.py
+++ /dev/null
@@ -1,95 +0,0 @@
-import torch
-import numpy as np
-
-
-class AbstractDistribution:
- def sample(self):
- raise NotImplementedError()
-
- def mode(self):
- raise NotImplementedError()
-
-
-class DiracDistribution(AbstractDistribution):
- def __init__(self, value):
- self.value = value
-
- def sample(self):
- return self.value
-
- def mode(self):
- return self.value
-
-
-class DiagonalGaussianDistribution(object):
- def __init__(self, parameters, deterministic=False):
- self.parameters = parameters
- self.mean, self.logvar = torch.chunk(parameters, 2, dim=1)
- self.logvar = torch.clamp(self.logvar, -30.0, 20.0)
- self.deterministic = deterministic
- self.std = torch.exp(0.5 * self.logvar)
- self.var = torch.exp(self.logvar)
- if self.deterministic:
- self.var = self.std = torch.zeros_like(self.mean).to(device=self.parameters.device)
-
- def sample(self, noise=None):
- if noise is None:
- noise = torch.randn(self.mean.shape)
-
- x = self.mean + self.std * noise.to(device=self.parameters.device)
- return x
-
- def kl(self, other=None):
- if self.deterministic:
- return torch.Tensor([0.])
- else:
- if other is None:
- return 0.5 * torch.sum(torch.pow(self.mean, 2)
- + self.var - 1.0 - self.logvar,
- dim=[1, 2, 3])
- else:
- return 0.5 * torch.sum(
- torch.pow(self.mean - other.mean, 2) / other.var
- + self.var / other.var - 1.0 - self.logvar + other.logvar,
- dim=[1, 2, 3])
-
- def nll(self, sample, dims=[1,2,3]):
- if self.deterministic:
- return torch.Tensor([0.])
- logtwopi = np.log(2.0 * np.pi)
- return 0.5 * torch.sum(
- logtwopi + self.logvar + torch.pow(sample - self.mean, 2) / self.var,
- dim=dims)
-
- def mode(self):
- return self.mean
-
-
-def normal_kl(mean1, logvar1, mean2, logvar2):
- """
- source: https://github.com/openai/guided-diffusion/blob/27c20a8fab9cb472df5d6bdd6c8d11c8f430b924/guided_diffusion/losses.py#L12
- Compute the KL divergence between two gaussians.
- Shapes are automatically broadcasted, so batches can be compared to
- scalars, among other use cases.
- """
- tensor = None
- for obj in (mean1, logvar1, mean2, logvar2):
- if isinstance(obj, torch.Tensor):
- tensor = obj
- break
- assert tensor is not None, "at least one argument must be a Tensor"
-
- # Force variances to be Tensors. Broadcasting helps convert scalars to
- # Tensors, but it does not work for torch.exp().
- logvar1, logvar2 = [
- x if isinstance(x, torch.Tensor) else torch.tensor(x).to(tensor)
- for x in (logvar1, logvar2)
- ]
-
- return 0.5 * (
- -1.0
- + logvar2
- - logvar1
- + torch.exp(logvar1 - logvar2)
- + ((mean1 - mean2) ** 2) * torch.exp(-logvar2)
- )
diff --git a/spaces/WAT-ai-AA/stable-diffused-adversarial-attacks/model.py b/spaces/WAT-ai-AA/stable-diffused-adversarial-attacks/model.py
deleted file mode 100644
index cc8c50c4265a547ef9991d3899937cbffeb2112a..0000000000000000000000000000000000000000
--- a/spaces/WAT-ai-AA/stable-diffused-adversarial-attacks/model.py
+++ /dev/null
@@ -1,78 +0,0 @@
-import torch.nn as nn
-
-
-def conv3x3(in_channels, out_channels, stride=1):
- return nn.Conv2d(
- in_channels,
- out_channels,
- kernel_size=3,
- stride=stride,
- padding=1,
- bias=False,
- )
-
-
-class ResidualBlock(nn.Module):
- def __init__(self, in_channels, out_channels, stride=1, downsample=None):
- super(ResidualBlock, self).__init__()
- self.conv1 = conv3x3(in_channels, out_channels, stride)
- self.bn1 = nn.BatchNorm2d(out_channels)
- self.relu = nn.ReLU(inplace=True)
- self.conv2 = conv3x3(out_channels, out_channels)
- self.bn2 = nn.BatchNorm2d(out_channels)
- self.downsample = downsample
-
- def forward(self, x):
- residual = x
- out = self.conv1(x)
- out = self.bn1(out)
- out = self.relu(out)
- out = self.conv2(out)
- out = self.bn2(out)
- if self.downsample:
- residual = self.downsample(x)
- out += residual
- out = self.relu(out)
- return out
-
-
-class ResNet(nn.Module):
- def __init__(self, block, layers, num_classes=10):
- super(ResNet, self).__init__()
- self.in_channels = 16
- self.conv = conv3x3(3, 16)
- self.bn = nn.BatchNorm2d(16)
- self.relu = nn.ReLU(inplace=True)
- self.layer1 = self.make_layer(block, 16, layers[0])
- self.layer2 = self.make_layer(block, 32, layers[1], 2)
- self.layer3 = self.make_layer(block, 64, layers[2], 2)
- self.avg_pool = nn.AvgPool2d(8)
- self.fc = nn.Linear(64, num_classes)
-
- def make_layer(self, block, out_channels, blocks, stride=1):
- downsample = None
- if (stride != 1) or (self.in_channels != out_channels):
- downsample = nn.Sequential(
- conv3x3(self.in_channels, out_channels, stride=stride),
- nn.BatchNorm2d(out_channels),
- )
- layers = []
- layers.append(
- block(self.in_channels, out_channels, stride, downsample)
- )
- self.in_channels = out_channels
- for i in range(1, blocks):
- layers.append(block(out_channels, out_channels))
- return nn.Sequential(*layers)
-
- def forward(self, x):
- out = self.conv(x)
- out = self.bn(out)
- out = self.relu(out)
- out = self.layer1(out)
- out = self.layer2(out)
- out = self.layer3(out)
- out = self.avg_pool(out)
- out = out.view(out.size(0), -1)
- out = self.fc(out)
- return out
diff --git a/spaces/Wrathless/Dkrotzer-MusicalMagic/README.md b/spaces/Wrathless/Dkrotzer-MusicalMagic/README.md
deleted file mode 100644
index 5ed3b1f58120772f839d8a172a943bfd63818fd4..0000000000000000000000000000000000000000
--- a/spaces/Wrathless/Dkrotzer-MusicalMagic/README.md
+++ /dev/null
@@ -1,125 +0,0 @@
----
-title: MusicGen
-python_version: '3.9'
-tags:
-- music generation
-- language models
-- LLMs
-app_file: app.py
-emoji: 🎵
-colorFrom: white
-colorTo: blue
-sdk: gradio
-sdk_version: 3.34.0
-pinned: true
-suggested_hardware: a10g-large
-license: cc-by-nc-4.0
-duplicated_from: musicgen/MusicGen
----
-# Audiocraft
-
-
-
-
-Audiocraft is a PyTorch library for deep learning research on audio generation. At the moment, it contains the code for MusicGen, a state-of-the-art controllable text-to-music model.
-
-## MusicGen
-
-Audiocraft provides the code and models for MusicGen, [a simple and controllable model for music generation][arxiv]. MusicGen is a single stage auto-regressive
-Transformer model trained over a 32kHz EnCodec tokenizer with 4 codebooks sampled at 50 Hz. Unlike existing methods like [MusicLM](https://arxiv.org/abs/2301.11325), MusicGen doesn't not require a self-supervised semantic representation, and it generates
-all 4 codebooks in one pass. By introducing a small delay between the codebooks, we show we can predict
-them in parallel, thus having only 50 auto-regressive steps per second of audio.
-Check out our [sample page][musicgen_samples] or test the available demo!
-
-
-
-
-
-
-
-
-
-## Installation
-Audiocraft requires Python 3.9, PyTorch 2.0.0, and a GPU with at least 16 GB of memory (for the medium-sized model). To install Audiocraft, you can run the following:
-
-```shell
-# Best to make sure you have torch installed first, in particular before installing xformers.
-# Don't run this if you already have PyTorch installed.
-pip install 'torch>=2.0'
-# Then proceed to one of the following
-pip install -U audiocraft # stable release
-pip install -U git+https://git@github.com/facebookresearch/audiocraft#egg=audiocraft # bleeding edge
-pip install -e . # or if you cloned the repo locally
-```
-
-## Usage
-You can play with MusicGen by running the jupyter notebook at [`demo.ipynb`](./demo.ipynb) locally, or use the provided [colab notebook](https://colab.research.google.com/drive/1fxGqfg96RBUvGxZ1XXN07s3DthrKUl4-?usp=sharing). Finally, a demo is also available on the [`facebook/MusiGen` HugginFace Space](https://huggingface.co/spaces/facebook/MusicGen) (huge thanks to all the HF team for their support).
-
-## API
-
-We provide a simple API and 4 pre-trained models. The pre trained models are:
-- `small`: 300M model, text to music only - [🤗 Hub](https://huggingface.co/facebook/musicgen-small)
-- `medium`: 1.5B model, text to music only - [🤗 Hub](https://huggingface.co/facebook/musicgen-medium)
-- `melody`: 1.5B model, text to music and text+melody to music - [🤗 Hub](https://huggingface.co/facebook/musicgen-melody)
-- `large`: 3.3B model, text to music only - [🤗 Hub](https://huggingface.co/facebook/musicgen-large)
-
-We observe the best trade-off between quality and compute with the `medium` or `melody` model.
-In order to use MusicGen locally **you must have a GPU**. We recommend 16GB of memory, but smaller
-GPUs will be able to generate short sequences, or longer sequences with the `small` model.
-
-**Note**: Please make sure to have [ffmpeg](https://ffmpeg.org/download.html) installed when using newer version of `torchaudio`.
-You can install it with:
-```
-apt get install ffmpeg
-```
-
-See after a quick example for using the API.
-
-```python
-import torchaudio
-from audiocraft.models import MusicGen
-from audiocraft.data.audio import audio_write
-
-model = MusicGen.get_pretrained('melody')
-model.set_generation_params(duration=8) # generate 8 seconds.
-wav = model.generate_unconditional(4) # generates 4 unconditional audio samples
-descriptions = ['happy rock', 'energetic EDM', 'sad jazz']
-wav = model.generate(descriptions) # generates 3 samples.
-
-melody, sr = torchaudio.load('./assets/bach.mp3')
-# generates using the melody from the given audio and the provided descriptions.
-wav = model.generate_with_chroma(descriptions, melody[None].expand(3, -1, -1), sr)
-
-for idx, one_wav in enumerate(wav):
- # Will save under {idx}.wav, with loudness normalization at -14 db LUFS.
- audio_write(f'{idx}', one_wav.cpu(), model.sample_rate, strategy="loudness")
-```
-
-
-## Model Card
-
-See [the model card page](./MODEL_CARD.md).
-
-## FAQ
-
-#### Will the training code be released?
-
-Yes. We will soon release the training code for MusicGen and EnCodec.
-
-
-## Citation
-```
-@article{copet2023simple,
- title={Simple and Controllable Music Generation},
- author={Jade Copet and Felix Kreuk and Itai Gat and Tal Remez and David Kant and Gabriel Synnaeve and Yossi Adi and Alexandre Défossez},
- year={2023},
- journal={arXiv preprint arXiv:2306.05284},
-}
-```
-
-## License
-* The code in this repository is released under the MIT license as found in the [LICENSE file](LICENSE).
-* The weights in this repository are released under the CC-BY-NC 4.0 license as found in the [LICENSE_weights file](LICENSE_weights).
-
-[arxiv]: https://arxiv.org/abs/2306.05284
-[musicgen_samples]: https://ai.honu.io/papers/musicgen/
diff --git a/spaces/Xenova/semantic-image-search-client/_next/static/chunks/482-32e037cb6270169e.js b/spaces/Xenova/semantic-image-search-client/_next/static/chunks/482-32e037cb6270169e.js
deleted file mode 100644
index 8a9f1658452089aaf3d52e7b22327fa51344fe15..0000000000000000000000000000000000000000
--- a/spaces/Xenova/semantic-image-search-client/_next/static/chunks/482-32e037cb6270169e.js
+++ /dev/null
@@ -1,9 +0,0 @@
-(self.webpackChunk_N_E=self.webpackChunk_N_E||[]).push([[482],{5051:function(e,t,r){"use strict";r.d(t,{Jx:function(){return p}});var n=["0","1","2","3","4","5","6","7","8","9","A","B","C","D","E","F","G","H","I","J","K","L","M","N","O","P","Q","R","S","T","U","V","W","X","Y","Z","a","b","c","d","e","f","g","h","i","j","k","l","m","n","o","p","q","r","s","t","u","v","w","x","y","z","#","$","%","*","+",",","-",".",":",";","=","?","@","[","]","^","_","{","|","}","~"],i=e=>{let t=0;for(let r=0;r{let t=e/255;return t<=.04045?t/12.92:Math.pow((t+.055)/1.055,2.4)},a=e=>{let t=Math.max(0,Math.min(1,e));return t<=.0031308?Math.trunc(3294.6*t+.5):Math.trunc((1.055*Math.pow(t,.4166666666666667)-.055)*255+.5)},l=e=>e<0?-1:1,u=(e,t)=>l(e)*Math.pow(Math.abs(e),t),s=class extends Error{constructor(e){super(e),this.name="ValidationError",this.message=e}},d=e=>{if(!e||e.length<6)throw new s("The blurhash string must be at least 6 characters");let t=i(e[0]),r=Math.floor(t/9)+1,n=t%9+1;if(e.length!==4+2*n*r)throw new s(`blurhash length mismatch: length is ${e.length} but it should be ${4+2*n*r}`)},f=e=>[o(e>>16),o(e>>8&255),o(255&e)],c=(e,t)=>[u((Math.floor(e/361)-9)/9,2)*t,u((Math.floor(e/19)%19-9)/9,2)*t,u((e%19-9)/9,2)*t],p=(e,t,r,n)=>{d(e),n|=1;let o=i(e[0]),l=Math.floor(o/9)+1,u=o%9+1,s=(i(e[1])+1)/166,p=Array(u*l);for(let t=0;t{}).then(()=>{if(e.parentElement&&e.isConnected){if("blur"===t&&i(!0),null==r?void 0:r.current){let t=new Event("load");Object.defineProperty(t,"target",{writable:!1,value:e});let n=!1,i=!1;r.current({...t,nativeEvent:t,currentTarget:e,target:e,isDefaultPrevented:()=>n,isPropagationStopped:()=>i,persist:()=>{},preventDefault:()=>{n=!0,t.preventDefault()},stopPropagation:()=>{i=!0,t.stopPropagation()}})}(null==n?void 0:n.current)&&n.current(e)}})}function m(e){let[t,r]=o.version.split("."),n=parseInt(t,10),i=parseInt(r,10);return n>18||18===n&&i>=3?{fetchPriority:e}:{fetchpriority:e}}let h=(0,o.forwardRef)((e,t)=>{let{src:r,srcSet:n,sizes:i,height:a,width:l,decoding:u,className:s,style:d,fetchPriority:f,placeholder:c,loading:p,unoptimized:h,fill:y,onLoadRef:b,onLoadingCompleteRef:v,setBlurComplete:_,setShowAltText:w,onLoad:S,onError:P,...O}=e;return o.default.createElement("img",{...O,...m(f),loading:p,width:l,height:a,decoding:u,"data-nimg":y?"fill":"1",className:s,style:d,sizes:i,srcSet:n,src:r,ref:(0,o.useCallback)(e=>{t&&("function"==typeof t?t(e):"object"==typeof t&&(t.current=e)),e&&(P&&(e.src=e.src),e.complete&&g(e,c,b,v,_,h))},[r,c,b,v,_,P,h,t]),onLoad:e=>{let t=e.currentTarget;g(t,c,b,v,_,h)},onError:e=>{w(!0),"blur"===c&&_(!0),P&&P(e)}})});function y(e){let{isAppRouter:t,imgAttributes:r}=e,n={as:"image",imageSrcSet:r.srcSet,imageSizes:r.sizes,crossOrigin:r.crossOrigin,referrerPolicy:r.referrerPolicy,...m(r.fetchPriority)};return t?((0,a.preload)(r.src,n),null):o.default.createElement(l.default,null,o.default.createElement("link",{key:"__nimg-"+r.src+r.srcSet+r.sizes,rel:"preload",href:r.srcSet?void 0:r.src,...n}))}let b=(0,o.forwardRef)((e,t)=>{let r=(0,o.useContext)(f.RouterContext),n=(0,o.useContext)(d.ImageConfigContext),i=(0,o.useMemo)(()=>{let e=p||n||s.imageConfigDefault,t=[...e.deviceSizes,...e.imageSizes].sort((e,t)=>e-t),r=e.deviceSizes.sort((e,t)=>e-t);return{...e,allSizes:t,deviceSizes:r}},[n]),{onLoad:a,onLoadingComplete:l}=e,g=(0,o.useRef)(a);(0,o.useEffect)(()=>{g.current=a},[a]);let m=(0,o.useRef)(l);(0,o.useEffect)(()=>{m.current=l},[l]);let[b,v]=(0,o.useState)(!1),[_,w]=(0,o.useState)(!1),{props:S,meta:P}=(0,u.getImgProps)(e,{defaultLoader:c.default,imgConf:i,blurComplete:b,showAltText:_});return o.default.createElement(o.default.Fragment,null,o.default.createElement(h,{...S,unoptimized:P.unoptimized,placeholder:P.placeholder,fill:P.fill,onLoadRef:g,onLoadingCompleteRef:m,setBlurComplete:v,setShowAltText:w,ref:t}),P.priority?o.default.createElement(y,{isAppRouter:!r,imgAttributes:S}):null)});("function"==typeof t.default||"object"==typeof t.default&&null!==t.default)&&void 0===t.default.__esModule&&(Object.defineProperty(t.default,"__esModule",{value:!0}),Object.assign(t.default,t),e.exports=t.default)},7555:function(e,t,r){"use strict";Object.defineProperty(t,"__esModule",{value:!0}),Object.defineProperty(t,"AmpStateContext",{enumerable:!0,get:function(){return o}});let n=r(1024),i=n._(r(2265)),o=i.default.createContext({})},8551:function(e,t){"use strict";function r(e){let{ampFirst:t=!1,hybrid:r=!1,hasQuery:n=!1}=void 0===e?{}:e;return t||r&&n}Object.defineProperty(t,"__esModule",{value:!0}),Object.defineProperty(t,"isInAmpMode",{enumerable:!0,get:function(){return r}})},2301:function(e,t,r){"use strict";Object.defineProperty(t,"__esModule",{value:!0}),Object.defineProperty(t,"getImgProps",{enumerable:!0,get:function(){return l}}),r(7873);let n=r(9540),i=r(7709);function o(e){return void 0!==e.default}function a(e){return void 0===e?e:"number"==typeof e?Number.isFinite(e)?e:NaN:"string"==typeof e&&/^[0-9]+$/.test(e)?parseInt(e,10):NaN}function l(e,t){var r;let l,u,s,{src:d,sizes:f,unoptimized:c=!1,priority:p=!1,loading:g,className:m,quality:h,width:y,height:b,fill:v=!1,style:_,onLoad:w,onLoadingComplete:S,placeholder:P="empty",blurDataURL:O,fetchPriority:C,layout:j,objectFit:x,objectPosition:E,lazyBoundary:M,lazyRoot:I,...z}=e,{imgConf:k,showAltText:A,blurComplete:R,defaultLoader:D}=t,U=k||i.imageConfigDefault;if("allSizes"in U)l=U;else{let e=[...U.deviceSizes,...U.imageSizes].sort((e,t)=>e-t),t=U.deviceSizes.sort((e,t)=>e-t);l={...U,allSizes:e,deviceSizes:t}}let L=z.loader||D;delete z.loader,delete z.srcSet;let N="__next_img_default"in L;if(N){if("custom"===l.loader)throw Error('Image with src "'+d+'" is missing "loader" prop.\nRead more: https://nextjs.org/docs/messages/next-image-missing-loader')}else{let e=L;L=t=>{let{config:r,...n}=t;return e(n)}}if(j){"fill"===j&&(v=!0);let e={intrinsic:{maxWidth:"100%",height:"auto"},responsive:{width:"100%",height:"auto"}}[j];e&&(_={..._,...e});let t={responsive:"100vw",fill:"100vw"}[j];t&&!f&&(f=t)}let T="",F=a(y),W=a(b);if("object"==typeof(r=d)&&(o(r)||void 0!==r.src)){let e=o(d)?d.default:d;if(!e.src)throw Error("An object should only be passed to the image component src parameter if it comes from a static image import. It must include src. Received "+JSON.stringify(e));if(!e.height||!e.width)throw Error("An object should only be passed to the image component src parameter if it comes from a static image import. It must include height and width. Received "+JSON.stringify(e));if(u=e.blurWidth,s=e.blurHeight,O=O||e.blurDataURL,T=e.src,!v){if(F||W){if(F&&!W){let t=F/e.width;W=Math.round(e.height*t)}else if(!F&&W){let t=W/e.height;F=Math.round(e.width*t)}}else F=e.width,W=e.height}}let B=!p&&("lazy"===g||void 0===g);(!(d="string"==typeof d?d:T)||d.startsWith("data:")||d.startsWith("blob:"))&&(c=!0,B=!1),l.unoptimized&&(c=!0),N&&d.endsWith(".svg")&&!l.dangerouslyAllowSVG&&(c=!0),p&&(C="high");let V=a(h),$=Object.assign(v?{position:"absolute",height:"100%",width:"100%",left:0,top:0,right:0,bottom:0,objectFit:x,objectPosition:E}:{},A?{}:{color:"transparent"},_),G="blur"===P&&O&&!R?{backgroundSize:$.objectFit||"cover",backgroundPosition:$.objectPosition||"50% 50%",backgroundRepeat:"no-repeat",backgroundImage:'url("data:image/svg+xml;charset=utf-8,'+(0,n.getImageBlurSvg)({widthInt:F,heightInt:W,blurWidth:u,blurHeight:s,blurDataURL:O,objectFit:$.objectFit})+'")'}:{},H=function(e){let{config:t,src:r,unoptimized:n,width:i,quality:o,sizes:a,loader:l}=e;if(n)return{src:r,srcSet:void 0,sizes:void 0};let{widths:u,kind:s}=function(e,t,r){let{deviceSizes:n,allSizes:i}=e;if(r){let e=/(^|\s)(1?\d?\d)vw/g,t=[];for(let n;n=e.exec(r);n)t.push(parseInt(n[2]));if(t.length){let e=.01*Math.min(...t);return{widths:i.filter(t=>t>=n[0]*e),kind:"w"}}return{widths:i,kind:"w"}}if("number"!=typeof t)return{widths:n,kind:"w"};let o=[...new Set([t,2*t].map(e=>i.find(t=>t>=e)||i[i.length-1]))];return{widths:o,kind:"x"}}(t,i,a),d=u.length-1;return{sizes:a||"w"!==s?a:"100vw",srcSet:u.map((e,n)=>l({config:t,src:r,quality:o,width:e})+" "+("w"===s?e:n+1)+s).join(", "),src:l({config:t,src:r,quality:o,width:u[d]})}}({config:l,src:d,unoptimized:c,width:F,quality:V,sizes:f,loader:L}),q={...z,loading:B?"lazy":g,fetchPriority:C,width:F,height:W,decoding:"async",className:m,style:{...$,...G},sizes:H.sizes,srcSet:H.srcSet,src:H.src},J={unoptimized:c,priority:p,placeholder:P,fill:v};return{props:q,meta:J}}},2912:function(e,t,r){"use strict";Object.defineProperty(t,"__esModule",{value:!0}),function(e,t){for(var r in t)Object.defineProperty(e,r,{enumerable:!0,get:t[r]})}(t,{defaultHead:function(){return d},default:function(){return g}});let n=r(1024),i=r(8533),o=i._(r(2265)),a=n._(r(2378)),l=r(7555),u=r(1330),s=r(8551);function d(e){void 0===e&&(e=!1);let t=[o.default.createElement("meta",{charSet:"utf-8"})];return e||t.push(o.default.createElement("meta",{name:"viewport",content:"width=device-width"})),t}function f(e,t){return"string"==typeof t||"number"==typeof t?e:t.type===o.default.Fragment?e.concat(o.default.Children.toArray(t.props.children).reduce((e,t)=>"string"==typeof t||"number"==typeof t?e:e.concat(t),[])):e.concat(t)}r(7873);let c=["name","httpEquiv","charSet","itemProp"];function p(e,t){let{inAmpMode:r}=t;return e.reduce(f,[]).reverse().concat(d(r).reverse()).filter(function(){let e=new Set,t=new Set,r=new Set,n={};return i=>{let o=!0,a=!1;if(i.key&&"number"!=typeof i.key&&i.key.indexOf("$")>0){a=!0;let t=i.key.slice(i.key.indexOf("$")+1);e.has(t)?o=!1:e.add(t)}switch(i.type){case"title":case"base":t.has(i.type)?o=!1:t.add(i.type);break;case"meta":for(let e=0,t=c.length;e{let n=e.key||t;if(!r&&"link"===e.type&&e.props.href&&["https://fonts.googleapis.com/css","https://use.typekit.net/"].some(t=>e.props.href.startsWith(t))){let t={...e.props||{}};return t["data-href"]=t.href,t.href=void 0,t["data-optimized-fonts"]=!0,o.default.cloneElement(e,t)}return o.default.cloneElement(e,{key:n})})}let g=function(e){let{children:t}=e,r=(0,o.useContext)(l.AmpStateContext),n=(0,o.useContext)(u.HeadManagerContext);return o.default.createElement(a.default,{reduceComponentsToState:p,headManager:n,inAmpMode:(0,s.isInAmpMode)(r)},t)};("function"==typeof t.default||"object"==typeof t.default&&null!==t.default)&&void 0===t.default.__esModule&&(Object.defineProperty(t.default,"__esModule",{value:!0}),Object.assign(t.default,t),e.exports=t.default)},9540:function(e,t){"use strict";function r(e){let{widthInt:t,heightInt:r,blurWidth:n,blurHeight:i,blurDataURL:o,objectFit:a}=e,l=n||t,u=i||r,s=o.startsWith("data:image/jpeg")?"%3CfeComponentTransfer%3E%3CfeFuncA type='discrete' tableValues='1 1'/%3E%3C/feComponentTransfer%3E%":"";return l&&u?"%3Csvg xmlns='http%3A//www.w3.org/2000/svg' viewBox='0 0 "+l+" "+u+"'%3E%3Cfilter id='b' color-interpolation-filters='sRGB'%3E%3CfeGaussianBlur stdDeviation='"+(n&&i?"1":"20")+"'/%3E"+s+"%3C/filter%3E%3Cimage preserveAspectRatio='none' filter='url(%23b)' x='0' y='0' height='100%25' width='100%25' href='"+o+"'/%3E%3C/svg%3E":"%3Csvg xmlns='http%3A//www.w3.org/2000/svg'%3E%3Cimage style='filter:blur(20px)' preserveAspectRatio='"+("contain"===a?"xMidYMid":"cover"===a?"xMidYMid slice":"none")+"' x='0' y='0' height='100%25' width='100%25' href='"+o+"'/%3E%3C/svg%3E"}Object.defineProperty(t,"__esModule",{value:!0}),Object.defineProperty(t,"getImageBlurSvg",{enumerable:!0,get:function(){return r}})},9469:function(e,t,r){"use strict";Object.defineProperty(t,"__esModule",{value:!0}),Object.defineProperty(t,"ImageConfigContext",{enumerable:!0,get:function(){return a}});let n=r(1024),i=n._(r(2265)),o=r(7709),a=i.default.createContext(o.imageConfigDefault)},7709:function(e,t){"use strict";Object.defineProperty(t,"__esModule",{value:!0}),function(e,t){for(var r in t)Object.defineProperty(e,r,{enumerable:!0,get:t[r]})}(t,{VALID_LOADERS:function(){return r},imageConfigDefault:function(){return n}});let r=["default","imgix","cloudinary","akamai","custom"],n={deviceSizes:[640,750,828,1080,1200,1920,2048,3840],imageSizes:[16,32,48,64,96,128,256,384],path:"/_next/image",loader:"default",loaderFile:"",domains:[],disableStaticImages:!1,minimumCacheTTL:60,formats:["image/webp"],dangerouslyAllowSVG:!1,contentSecurityPolicy:"script-src 'none'; frame-src 'none'; sandbox;",contentDispositionType:"inline",remotePatterns:[],unoptimized:!1}},1295:function(e,t,r){"use strict";Object.defineProperty(t,"__esModule",{value:!0}),function(e,t){for(var r in t)Object.defineProperty(e,r,{enumerable:!0,get:t[r]})}(t,{default:function(){return s},unstable_getImgProps:function(){return u}});let n=r(1024),i=r(2301),o=r(7873),a=r(3222),l=n._(r(5033)),u=e=>{(0,o.warnOnce)("Warning: unstable_getImgProps() is experimental and may change or be removed at any time. Use at your own risk.");let{props:t}=(0,i.getImgProps)(e,{defaultLoader:l.default,imgConf:{deviceSizes:[640,750,828,1080,1200,1920,2048,3840],imageSizes:[16,32,48,64,96,128,256,384],path:"/_next/image",loader:"default",dangerouslyAllowSVG:!1,unoptimized:!1}});for(let[e,r]of Object.entries(t))void 0===r&&delete t[e];return{props:t}},s=a.Image},5033:function(e,t){"use strict";function r(e){let{config:t,src:r,width:n,quality:i}=e;return t.path+"?url="+encodeURIComponent(r)+"&w="+n+"&q="+(i||75)}Object.defineProperty(t,"__esModule",{value:!0}),Object.defineProperty(t,"default",{enumerable:!0,get:function(){return n}}),r.__next_img_default=!0;let n=r},2706:function(e,t,r){"use strict";Object.defineProperty(t,"__esModule",{value:!0}),Object.defineProperty(t,"RouterContext",{enumerable:!0,get:function(){return o}});let n=r(1024),i=n._(r(2265)),o=i.default.createContext(null)},2378:function(e,t,r){"use strict";Object.defineProperty(t,"__esModule",{value:!0}),Object.defineProperty(t,"default",{enumerable:!0,get:function(){return l}});let n=r(8533),i=n._(r(2265)),o=i.useLayoutEffect,a=i.useEffect;function l(e){let{headManager:t,reduceComponentsToState:r}=e;function n(){if(t&&t.mountedInstances){let n=i.Children.toArray(Array.from(t.mountedInstances).filter(Boolean));t.updateHead(r(n,e))}}return o(()=>{var r;return null==t||null==(r=t.mountedInstances)||r.add(e.children),()=>{var r;null==t||null==(r=t.mountedInstances)||r.delete(e.children)}}),o(()=>(t&&(t._pendingUpdate=n),()=>{t&&(t._pendingUpdate=n)})),a(()=>(t&&t._pendingUpdate&&(t._pendingUpdate(),t._pendingUpdate=null),()=>{t&&t._pendingUpdate&&(t._pendingUpdate(),t._pendingUpdate=null)})),null}},7873:function(e,t){"use strict";Object.defineProperty(t,"__esModule",{value:!0}),Object.defineProperty(t,"warnOnce",{enumerable:!0,get:function(){return r}});let r=e=>{}},622:function(e,t,r){"use strict";/**
- * @license React
- * react-jsx-runtime.production.min.js
- *
- * Copyright (c) Meta Platforms, Inc. and affiliates.
- *
- * This source code is licensed under the MIT license found in the
- * LICENSE file in the root directory of this source tree.
- */var n=r(2265),i=Symbol.for("react.element"),o=Symbol.for("react.fragment"),a=Object.prototype.hasOwnProperty,l=n.__SECRET_INTERNALS_DO_NOT_USE_OR_YOU_WILL_BE_FIRED.ReactCurrentOwner,u={key:!0,ref:!0,__self:!0,__source:!0};function s(e,t,r){var n,o={},s=null,d=null;for(n in void 0!==r&&(s=""+r),void 0!==t.key&&(s=""+t.key),void 0!==t.ref&&(d=t.ref),t)a.call(t,n)&&!u.hasOwnProperty(n)&&(o[n]=t[n]);if(e&&e.defaultProps)for(n in t=e.defaultProps)void 0===o[n]&&(o[n]=t[n]);return{$$typeof:i,type:e,key:s,ref:d,props:o,_owner:l.current}}t.Fragment=o,t.jsx=s,t.jsxs=s},7437:function(e,t,r){"use strict";e.exports=r(622)},6691:function(e,t,r){e.exports=r(1295)}}]);
\ No newline at end of file
diff --git a/spaces/XzJosh/nine1-Bert-VITS2/server.py b/spaces/XzJosh/nine1-Bert-VITS2/server.py
deleted file mode 100644
index c736ca4f95fec853950eef6654ef79856beffc0a..0000000000000000000000000000000000000000
--- a/spaces/XzJosh/nine1-Bert-VITS2/server.py
+++ /dev/null
@@ -1,123 +0,0 @@
-from flask import Flask, request, Response
-from io import BytesIO
-import torch
-from av import open as avopen
-
-import commons
-import utils
-from models import SynthesizerTrn
-from text.symbols import symbols
-from text import cleaned_text_to_sequence, get_bert
-from text.cleaner import clean_text
-from scipy.io import wavfile
-
-# Flask Init
-app = Flask(__name__)
-app.config['JSON_AS_ASCII'] = False
-def get_text(text, language_str, hps):
- norm_text, phone, tone, word2ph = clean_text(text, language_str)
- print([f"{p}{t}" for p, t in zip(phone, tone)])
- phone, tone, language = cleaned_text_to_sequence(phone, tone, language_str)
-
- if hps.data.add_blank:
- phone = commons.intersperse(phone, 0)
- tone = commons.intersperse(tone, 0)
- language = commons.intersperse(language, 0)
- for i in range(len(word2ph)):
- word2ph[i] = word2ph[i] * 2
- word2ph[0] += 1
- bert = get_bert(norm_text, word2ph, language_str)
-
- assert bert.shape[-1] == len(phone)
-
- phone = torch.LongTensor(phone)
- tone = torch.LongTensor(tone)
- language = torch.LongTensor(language)
-
- return bert, phone, tone, language
-
-def infer(text, sdp_ratio, noise_scale, noise_scale_w,length_scale,sid):
- bert, phones, tones, lang_ids = get_text(text,"ZH", hps,)
- with torch.no_grad():
- x_tst=phones.to(dev).unsqueeze(0)
- tones=tones.to(dev).unsqueeze(0)
- lang_ids=lang_ids.to(dev).unsqueeze(0)
- bert = bert.to(dev).unsqueeze(0)
- x_tst_lengths = torch.LongTensor([phones.size(0)]).to(dev)
- speakers = torch.LongTensor([hps.data.spk2id[sid]]).to(dev)
- audio = net_g.infer(x_tst, x_tst_lengths, speakers, tones, lang_ids,bert, sdp_ratio=sdp_ratio
- , noise_scale=noise_scale, noise_scale_w=noise_scale_w, length_scale=length_scale)[0][0,0].data.cpu().float().numpy()
- return audio
-
-def replace_punctuation(text, i=2):
- punctuation = ",。?!"
- for char in punctuation:
- text = text.replace(char, char * i)
- return text
-
-def wav2(i, o, format):
- inp = avopen(i, 'rb')
- out = avopen(o, 'wb', format=format)
- if format == "ogg": format = "libvorbis"
-
- ostream = out.add_stream(format)
-
- for frame in inp.decode(audio=0):
- for p in ostream.encode(frame): out.mux(p)
-
- for p in ostream.encode(None): out.mux(p)
-
- out.close()
- inp.close()
-
-# Load Generator
-hps = utils.get_hparams_from_file("./configs/config.json")
-
-dev='cuda'
-net_g = SynthesizerTrn(
- len(symbols),
- hps.data.filter_length // 2 + 1,
- hps.train.segment_size // hps.data.hop_length,
- n_speakers=hps.data.n_speakers,
- **hps.model).to(dev)
-_ = net_g.eval()
-
-_ = utils.load_checkpoint("logs/G_649000.pth", net_g, None,skip_optimizer=True)
-
-@app.route("/",methods=['GET','POST'])
-def main():
- if request.method == 'GET':
- try:
- speaker = request.args.get('speaker')
- text = request.args.get('text').replace("/n","")
- sdp_ratio = float(request.args.get("sdp_ratio", 0.2))
- noise = float(request.args.get("noise", 0.5))
- noisew = float(request.args.get("noisew", 0.6))
- length = float(request.args.get("length", 1.2))
- if length >= 2:
- return "Too big length"
- if len(text) >=200:
- return "Too long text"
- fmt = request.args.get("format", "wav")
- if None in (speaker, text):
- return "Missing Parameter"
- if fmt not in ("mp3", "wav", "ogg"):
- return "Invalid Format"
- except:
- return "Invalid Parameter"
-
- with torch.no_grad():
- audio = infer(text, sdp_ratio=sdp_ratio, noise_scale=noise, noise_scale_w=noisew, length_scale=length, sid=speaker)
-
- with BytesIO() as wav:
- wavfile.write(wav, hps.data.sampling_rate, audio)
- torch.cuda.empty_cache()
- if fmt == "wav":
- return Response(wav.getvalue(), mimetype="audio/wav")
- wav.seek(0, 0)
- with BytesIO() as ofp:
- wav2(wav, ofp, fmt)
- return Response(
- ofp.getvalue(),
- mimetype="audio/mpeg" if fmt == "mp3" else "audio/ogg"
- )
diff --git a/spaces/YONG627/456123/yolov5-code-main/utils/aws/mime.sh b/spaces/YONG627/456123/yolov5-code-main/utils/aws/mime.sh
deleted file mode 100644
index c319a83cfbdf09bea634c3bd9fca737c0b1dd505..0000000000000000000000000000000000000000
--- a/spaces/YONG627/456123/yolov5-code-main/utils/aws/mime.sh
+++ /dev/null
@@ -1,26 +0,0 @@
-# AWS EC2 instance startup 'MIME' script https://aws.amazon.com/premiumsupport/knowledge-center/execute-user-data-ec2/
-# This script will run on every instance restart, not only on first start
-# --- DO NOT COPY ABOVE COMMENTS WHEN PASTING INTO USERDATA ---
-
-Content-Type: multipart/mixed; boundary="//"
-MIME-Version: 1.0
-
---//
-Content-Type: text/cloud-config; charset="us-ascii"
-MIME-Version: 1.0
-Content-Transfer-Encoding: 7bit
-Content-Disposition: attachment; filename="cloud-config.txt"
-
-#cloud-config
-cloud_final_modules:
-- [scripts-user, always]
-
---//
-Content-Type: text/x-shellscript; charset="us-ascii"
-MIME-Version: 1.0
-Content-Transfer-Encoding: 7bit
-Content-Disposition: attachment; filename="userdata.txt"
-
-#!/bin/bash
-# --- paste contents of userdata.sh here ---
---//
diff --git a/spaces/YUANAI/DiffspeechResearch/modules/tts/portaspeech/portaspeech_flow.py b/spaces/YUANAI/DiffspeechResearch/modules/tts/portaspeech/portaspeech_flow.py
deleted file mode 100644
index 256887dd8b365e38ac6c1973f4ec376e93029652..0000000000000000000000000000000000000000
--- a/spaces/YUANAI/DiffspeechResearch/modules/tts/portaspeech/portaspeech_flow.py
+++ /dev/null
@@ -1,75 +0,0 @@
-import torch
-import torch.distributions as dist
-from torch import nn
-from modules.commons.normalizing_flow.glow_modules import Glow
-from modules.tts.portaspeech.portaspeech import PortaSpeech
-
-
-class PortaSpeechFlow(PortaSpeech):
- def __init__(self, ph_dict_size, word_dict_size, hparams, out_dims=None):
- super().__init__(ph_dict_size, word_dict_size, hparams, out_dims)
- cond_hs = 80
- if hparams.get('use_txt_cond', True):
- cond_hs = cond_hs + hparams['hidden_size']
- if hparams.get('use_latent_cond', False):
- cond_hs = cond_hs + hparams['latent_size']
- if hparams['use_cond_proj']:
- self.g_proj = nn.Conv1d(cond_hs, 160, 5, padding=2)
- cond_hs = 160
- self.post_flow = Glow(
- 80, hparams['post_glow_hidden'], hparams['post_glow_kernel_size'], 1,
- hparams['post_glow_n_blocks'], hparams['post_glow_n_block_layers'],
- n_split=4, n_sqz=2,
- gin_channels=cond_hs,
- share_cond_layers=hparams['post_share_cond_layers'],
- share_wn_layers=hparams['share_wn_layers'],
- sigmoid_scale=hparams['sigmoid_scale']
- )
- self.prior_dist = dist.Normal(0, 1)
-
- def forward(self, txt_tokens, word_tokens, ph2word, word_len, mel2word=None, mel2ph=None,
- spk_embed=None, spk_id=None, pitch=None, infer=False, tgt_mels=None,
- forward_post_glow=True, two_stage=True, global_step=None):
- is_training = self.training
- train_fvae = not (forward_post_glow and two_stage)
- if not train_fvae:
- self.eval()
- with torch.set_grad_enabled(mode=train_fvae):
- ret = super(PortaSpeechFlow, self).forward(
- txt_tokens, word_tokens, ph2word, word_len, mel2word, mel2ph,
- spk_embed, spk_id, pitch, infer, tgt_mels, global_step)
- if (forward_post_glow or not two_stage) and self.hparams['use_post_flow']:
- self.run_post_glow(tgt_mels, infer, is_training, ret)
- return ret
-
- def run_post_glow(self, tgt_mels, infer, is_training, ret):
- x_recon = ret['mel_out'].transpose(1, 2)
- g = x_recon
- B, _, T = g.shape
- if self.hparams.get('use_txt_cond', True):
- g = torch.cat([g, ret['decoder_inp'].transpose(1, 2)], 1)
- if self.hparams.get('use_latent_cond', False):
- g_z = ret['z_p'][:, :, :, None].repeat(1, 1, 1, 4).reshape(B, -1, T)
- g = torch.cat([g, g_z], 1)
- if self.hparams['use_cond_proj']:
- g = self.g_proj(g)
- prior_dist = self.prior_dist
- if not infer:
- if is_training:
- self.post_flow.train()
- nonpadding = ret['nonpadding'].transpose(1, 2)
- y_lengths = nonpadding.sum(-1)
- if self.hparams['detach_postflow_input']:
- g = g.detach()
- tgt_mels = tgt_mels.transpose(1, 2)
- z_postflow, ldj = self.post_flow(tgt_mels, nonpadding, g=g)
- ldj = ldj / y_lengths / 80
- ret['z_pf'], ret['ldj_pf'] = z_postflow, ldj
- ret['postflow'] = -prior_dist.log_prob(z_postflow).mean() - ldj.mean()
- if torch.isnan(ret['postflow']):
- ret['postflow'] = None
- else:
- nonpadding = torch.ones_like(x_recon[:, :1, :])
- z_post = torch.randn(x_recon.shape).to(g.device) * self.hparams['noise_scale']
- x_recon, _ = self.post_flow(z_post, nonpadding, g, reverse=True)
- ret['mel_out'] = x_recon.transpose(1, 2)
diff --git a/spaces/Yuliang/ICON/app.py b/spaces/Yuliang/ICON/app.py
deleted file mode 100644
index f528aa27b29b1f15645060c6c49432234939f364..0000000000000000000000000000000000000000
--- a/spaces/Yuliang/ICON/app.py
+++ /dev/null
@@ -1,156 +0,0 @@
-# install
-
-
-import glob
-import gradio as gr
-import os
-import numpy as np
-
-import subprocess
-
-if os.getenv('SYSTEM') == 'spaces':
- subprocess.run('pip install pyembree'.split())
- subprocess.run(
- 'pip install torch==1.11.0+cu113 torchvision==0.12.0+cu113 -f https://download.pytorch.org/whl/cu113/torch_stable.html'.split())
- subprocess.run(
- 'pip install https://download.is.tue.mpg.de/icon/HF/kaolin-0.11.0-cp38-cp38-linux_x86_64.whl'.split())
- subprocess.run(
- 'pip install https://download.is.tue.mpg.de/icon/HF/pytorch3d-0.7.0-cp38-cp38-linux_x86_64.whl'.split())
- subprocess.run(
- 'pip install git+https://github.com/YuliangXiu/neural_voxelization_layer.git'.split())
-
-from apps.infer import generate_model
-
-# running
-
-description = '''
-# ICON Clothed Human Digitization
-### ICON: Implicit Clothed humans Obtained from Normals (CVPR 2022)
-
-
-
-
-
-
-
-
-
-VIDEO
-
-
-
- The reconstruction + refinement + video take about 200 seconds for single image. ICON is only suitable for humanoid images and will not work well on cartoons with non-human shapes.
-
-
-
-More
-
-#### Citation
-```
-@inproceedings{xiu2022icon,
- title = {{ICON}: {I}mplicit {C}lothed humans {O}btained from {N}ormals},
- author = {Xiu, Yuliang and Yang, Jinlong and Tzionas, Dimitrios and Black, Michael J.},
- booktitle = {Proceedings of the IEEE/CVF Conference on Computer Vision and Pattern Recognition (CVPR)},
- month = {June},
- year = {2022},
- pages = {13296-13306}
-}
-```
-
-#### Acknowledgments:
-
-- [StyleGAN-Human, ECCV 2022](https://stylegan-human.github.io/)
-- [nagolinc/styleGanHuman_and_PIFu](https://huggingface.co/spaces/nagolinc/styleGanHuman_and_PIFu)
-- [radames/PIFu-Clothed-Human-Digitization](https://huggingface.co/spaces/radames/PIFu-Clothed-Human-Digitization)
-
-#### Image Credits
-
-* [Pinterest](https://www.pinterest.com/search/pins/?q=parkour&rs=sitelinks_searchbox)
-
-#### Related works
-
-* [ICON @ MPI](https://icon.is.tue.mpg.de/)
-* [MonoPort @ USC](https://xiuyuliang.cn/monoport)
-* [Phorhum @ Google](https://phorhum.github.io/)
-* [PIFuHD @ Meta](https://shunsukesaito.github.io/PIFuHD/)
-* [PaMIR @ Tsinghua](http://www.liuyebin.com/pamir/pamir.html)
-
-
-'''
-
-
-def generate_image(seed, psi):
- iface = gr.Interface.load("spaces/hysts/StyleGAN-Human")
- img = iface(seed, psi)
- return img
-
-
-model_types = ['ICON', 'PIFu', 'PaMIR']
-examples_names = glob.glob('examples/*.png')
-examples_types = np.random.choice(
- model_types, len(examples_names), p=[0.6, 0.2, 0.2])
-
-examples = [list(item) for item in zip(examples_names, examples_types)]
-
-with gr.Blocks() as demo:
- gr.Markdown(description)
-
- out_lst = []
- with gr.Row():
- with gr.Column():
- with gr.Row():
- with gr.Column():
- seed = gr.inputs.Slider(
- 0, 1000, step=1, default=0, label='Seed (For Image Generation)')
- psi = gr.inputs.Slider(
- 0, 2, step=0.05, default=0.7, label='Truncation psi (For Image Generation)')
- radio_choice = gr.Radio(
- model_types, label='Method (For Reconstruction)', value='icon-filter')
- inp = gr.Image(type="filepath", label="Input Image")
- with gr.Row():
- btn_sample = gr.Button("Generate Image")
- btn_submit = gr.Button("Submit Image")
-
- gr.Examples(examples=examples,
- inputs=[inp, radio_choice],
- cache_examples=False,
- fn=generate_model,
- outputs=out_lst)
-
- out_vid = gr.Video(
- label="Image + Normal + SMPL Body + Clothed Human")
- out_vid_download = gr.File(
- label="Download Video, welcome share on Twitter with #ICON")
-
- with gr.Column():
- overlap_inp = gr.Image(
- type="filepath", label="Image Normal Overlap")
- out_final = gr.Model3D(
- clear_color=[0.0, 0.0, 0.0, 0.0], label="Clothed human")
- out_final_download = gr.File(
- label="Download clothed human mesh")
- out_smpl = gr.Model3D(
- clear_color=[0.0, 0.0, 0.0, 0.0], label="SMPL body")
- out_smpl_download = gr.File(label="Download SMPL body mesh")
- out_smpl_npy_download = gr.File(label="Download SMPL params")
-
- out_lst = [out_smpl, out_smpl_download, out_smpl_npy_download,
- out_final, out_final_download, out_vid, out_vid_download, overlap_inp]
-
- btn_submit.click(fn=generate_model, inputs=[
- inp, radio_choice], outputs=out_lst)
- btn_sample.click(fn=generate_image, inputs=[seed, psi], outputs=inp)
-
-if __name__ == "__main__":
-
- # demo.launch(debug=False, enable_queue=False,
- # auth=(os.environ['USER'], os.environ['PASSWORD']),
- # auth_message="Register at icon.is.tue.mpg.de to get HuggingFace username and password.")
-
- demo.launch(debug=True, enable_queue=True)
diff --git a/spaces/ZeroTwo3/WavJourney/parse_voice.py b/spaces/ZeroTwo3/WavJourney/parse_voice.py
deleted file mode 100644
index 9583f402cfb23aede18d421befd2508633b1d23c..0000000000000000000000000000000000000000
--- a/spaces/ZeroTwo3/WavJourney/parse_voice.py
+++ /dev/null
@@ -1,31 +0,0 @@
-import os
-import argparse
-from VoiceParser.model import VoiceParser
-
-def main():
- parser = argparse.ArgumentParser()
- parser.add_argument('--wav-path', type=str, help="Path of a wav file")
- parser.add_argument('--wav-dir', type=str, help="Directory of wav files")
- parser.add_argument('--out-dir', type=str, help="Directory of output npz files")
- args = parser.parse_args()
-
- if (args.wav_path is None and args.wav_dir is None) or (args.wav_path is not None and args.wav_dir is not None):
- parser.error("Please provide either '--wav-path' or '--wav-dir', but not both.")
-
- out_dir = args.out_dir
-
- model = VoiceParser(device='cpu')
-
- if args.wav_path is not None:
- model.extract_acoustic_embed(args.wav_path, out_dir)
- print(f'Sucessfully parsed {args.wav_path}')
- else:
- wav_name_list = os.listdir(args.wav_dir)
- for wav_name in wav_name_list:
- wav_path = os.path.join(args.wav_dir, wav_name)
- model.extract_acoustic_embed(wav_path, out_dir)
- print(f'Sucessfully parsed {wav_path}')
-
-
-if __name__ == '__main__':
- main()
diff --git a/spaces/Zwicky18/vits-models/modules.py b/spaces/Zwicky18/vits-models/modules.py
deleted file mode 100644
index 56ea4145eddf19dd330a3a41ab0183efc1686d83..0000000000000000000000000000000000000000
--- a/spaces/Zwicky18/vits-models/modules.py
+++ /dev/null
@@ -1,388 +0,0 @@
-import math
-import numpy as np
-import torch
-from torch import nn
-from torch.nn import functional as F
-
-from torch.nn import Conv1d, ConvTranspose1d, AvgPool1d, Conv2d
-from torch.nn.utils import weight_norm, remove_weight_norm
-
-import commons
-from commons import init_weights, get_padding
-from transforms import piecewise_rational_quadratic_transform
-
-
-LRELU_SLOPE = 0.1
-
-
-class LayerNorm(nn.Module):
- def __init__(self, channels, eps=1e-5):
- super().__init__()
- self.channels = channels
- self.eps = eps
-
- self.gamma = nn.Parameter(torch.ones(channels))
- self.beta = nn.Parameter(torch.zeros(channels))
-
- def forward(self, x):
- x = x.transpose(1, -1)
- x = F.layer_norm(x, (self.channels,), self.gamma, self.beta, self.eps)
- return x.transpose(1, -1)
-
-
-class ConvReluNorm(nn.Module):
- def __init__(self, in_channels, hidden_channels, out_channels, kernel_size, n_layers, p_dropout):
- super().__init__()
- self.in_channels = in_channels
- self.hidden_channels = hidden_channels
- self.out_channels = out_channels
- self.kernel_size = kernel_size
- self.n_layers = n_layers
- self.p_dropout = p_dropout
- assert n_layers > 1, "Number of layers should be larger than 0."
-
- self.conv_layers = nn.ModuleList()
- self.norm_layers = nn.ModuleList()
- self.conv_layers.append(nn.Conv1d(in_channels, hidden_channels, kernel_size, padding=kernel_size//2))
- self.norm_layers.append(LayerNorm(hidden_channels))
- self.relu_drop = nn.Sequential(
- nn.ReLU(),
- nn.Dropout(p_dropout))
- for _ in range(n_layers-1):
- self.conv_layers.append(nn.Conv1d(hidden_channels, hidden_channels, kernel_size, padding=kernel_size//2))
- self.norm_layers.append(LayerNorm(hidden_channels))
- self.proj = nn.Conv1d(hidden_channels, out_channels, 1)
- self.proj.weight.data.zero_()
- self.proj.bias.data.zero_()
-
- def forward(self, x, x_mask):
- x_org = x
- for i in range(self.n_layers):
- x = self.conv_layers[i](x * x_mask)
- x = self.norm_layers[i](x)
- x = self.relu_drop(x)
- x = x_org + self.proj(x)
- return x * x_mask
-
-
-class DDSConv(nn.Module):
- """
- Dialted and Depth-Separable Convolution
- """
- def __init__(self, channels, kernel_size, n_layers, p_dropout=0.):
- super().__init__()
- self.channels = channels
- self.kernel_size = kernel_size
- self.n_layers = n_layers
- self.p_dropout = p_dropout
-
- self.drop = nn.Dropout(p_dropout)
- self.convs_sep = nn.ModuleList()
- self.convs_1x1 = nn.ModuleList()
- self.norms_1 = nn.ModuleList()
- self.norms_2 = nn.ModuleList()
- for i in range(n_layers):
- dilation = kernel_size ** i
- padding = (kernel_size * dilation - dilation) // 2
- self.convs_sep.append(nn.Conv1d(channels, channels, kernel_size,
- groups=channels, dilation=dilation, padding=padding
- ))
- self.convs_1x1.append(nn.Conv1d(channels, channels, 1))
- self.norms_1.append(LayerNorm(channels))
- self.norms_2.append(LayerNorm(channels))
-
- def forward(self, x, x_mask, g=None):
- if g is not None:
- x = x + g
- for i in range(self.n_layers):
- y = self.convs_sep[i](x * x_mask)
- y = self.norms_1[i](y)
- y = F.gelu(y)
- y = self.convs_1x1[i](y)
- y = self.norms_2[i](y)
- y = F.gelu(y)
- y = self.drop(y)
- x = x + y
- return x * x_mask
-
-
-class WN(torch.nn.Module):
- def __init__(self, hidden_channels, kernel_size, dilation_rate, n_layers, gin_channels=0, p_dropout=0):
- super(WN, self).__init__()
- assert(kernel_size % 2 == 1)
- self.hidden_channels =hidden_channels
- self.kernel_size = kernel_size,
- self.dilation_rate = dilation_rate
- self.n_layers = n_layers
- self.gin_channels = gin_channels
- self.p_dropout = p_dropout
-
- self.in_layers = torch.nn.ModuleList()
- self.res_skip_layers = torch.nn.ModuleList()
- self.drop = nn.Dropout(p_dropout)
-
- if gin_channels != 0:
- cond_layer = torch.nn.Conv1d(gin_channels, 2*hidden_channels*n_layers, 1)
- self.cond_layer = torch.nn.utils.weight_norm(cond_layer, name='weight')
-
- for i in range(n_layers):
- dilation = dilation_rate ** i
- padding = int((kernel_size * dilation - dilation) / 2)
- in_layer = torch.nn.Conv1d(hidden_channels, 2*hidden_channels, kernel_size,
- dilation=dilation, padding=padding)
- in_layer = torch.nn.utils.weight_norm(in_layer, name='weight')
- self.in_layers.append(in_layer)
-
- # last one is not necessary
- if i < n_layers - 1:
- res_skip_channels = 2 * hidden_channels
- else:
- res_skip_channels = hidden_channels
-
- res_skip_layer = torch.nn.Conv1d(hidden_channels, res_skip_channels, 1)
- res_skip_layer = torch.nn.utils.weight_norm(res_skip_layer, name='weight')
- self.res_skip_layers.append(res_skip_layer)
-
- def forward(self, x, x_mask, g=None, **kwargs):
- output = torch.zeros_like(x)
- n_channels_tensor = torch.IntTensor([self.hidden_channels])
-
- if g is not None:
- g = self.cond_layer(g)
-
- for i in range(self.n_layers):
- x_in = self.in_layers[i](x)
- if g is not None:
- cond_offset = i * 2 * self.hidden_channels
- g_l = g[:,cond_offset:cond_offset+2*self.hidden_channels,:]
- else:
- g_l = torch.zeros_like(x_in)
-
- acts = commons.fused_add_tanh_sigmoid_multiply(
- x_in,
- g_l,
- n_channels_tensor)
- acts = self.drop(acts)
-
- res_skip_acts = self.res_skip_layers[i](acts)
- if i < self.n_layers - 1:
- res_acts = res_skip_acts[:,:self.hidden_channels,:]
- x = (x + res_acts) * x_mask
- output = output + res_skip_acts[:,self.hidden_channels:,:]
- else:
- output = output + res_skip_acts
- return output * x_mask
-
- def remove_weight_norm(self):
- if self.gin_channels != 0:
- torch.nn.utils.remove_weight_norm(self.cond_layer)
- for l in self.in_layers:
- torch.nn.utils.remove_weight_norm(l)
- for l in self.res_skip_layers:
- torch.nn.utils.remove_weight_norm(l)
-
-
-class ResBlock1(torch.nn.Module):
- def __init__(self, channels, kernel_size=3, dilation=(1, 3, 5)):
- super(ResBlock1, self).__init__()
- self.convs1 = nn.ModuleList([
- weight_norm(Conv1d(channels, channels, kernel_size, 1, dilation=dilation[0],
- padding=get_padding(kernel_size, dilation[0]))),
- weight_norm(Conv1d(channels, channels, kernel_size, 1, dilation=dilation[1],
- padding=get_padding(kernel_size, dilation[1]))),
- weight_norm(Conv1d(channels, channels, kernel_size, 1, dilation=dilation[2],
- padding=get_padding(kernel_size, dilation[2])))
- ])
- self.convs1.apply(init_weights)
-
- self.convs2 = nn.ModuleList([
- weight_norm(Conv1d(channels, channels, kernel_size, 1, dilation=1,
- padding=get_padding(kernel_size, 1))),
- weight_norm(Conv1d(channels, channels, kernel_size, 1, dilation=1,
- padding=get_padding(kernel_size, 1))),
- weight_norm(Conv1d(channels, channels, kernel_size, 1, dilation=1,
- padding=get_padding(kernel_size, 1)))
- ])
- self.convs2.apply(init_weights)
-
- def forward(self, x, x_mask=None):
- for c1, c2 in zip(self.convs1, self.convs2):
- xt = F.leaky_relu(x, LRELU_SLOPE)
- if x_mask is not None:
- xt = xt * x_mask
- xt = c1(xt)
- xt = F.leaky_relu(xt, LRELU_SLOPE)
- if x_mask is not None:
- xt = xt * x_mask
- xt = c2(xt)
- x = xt + x
- if x_mask is not None:
- x = x * x_mask
- return x
-
- def remove_weight_norm(self):
- for l in self.convs1:
- remove_weight_norm(l)
- for l in self.convs2:
- remove_weight_norm(l)
-
-
-class ResBlock2(torch.nn.Module):
- def __init__(self, channels, kernel_size=3, dilation=(1, 3)):
- super(ResBlock2, self).__init__()
- self.convs = nn.ModuleList([
- weight_norm(Conv1d(channels, channels, kernel_size, 1, dilation=dilation[0],
- padding=get_padding(kernel_size, dilation[0]))),
- weight_norm(Conv1d(channels, channels, kernel_size, 1, dilation=dilation[1],
- padding=get_padding(kernel_size, dilation[1])))
- ])
- self.convs.apply(init_weights)
-
- def forward(self, x, x_mask=None):
- for c in self.convs:
- xt = F.leaky_relu(x, LRELU_SLOPE)
- if x_mask is not None:
- xt = xt * x_mask
- xt = c(xt)
- x = xt + x
- if x_mask is not None:
- x = x * x_mask
- return x
-
- def remove_weight_norm(self):
- for l in self.convs:
- remove_weight_norm(l)
-
-
-class Log(nn.Module):
- def forward(self, x, x_mask, reverse=False, **kwargs):
- if not reverse:
- y = torch.log(torch.clamp_min(x, 1e-5)) * x_mask
- logdet = torch.sum(-y, [1, 2])
- return y, logdet
- else:
- x = torch.exp(x) * x_mask
- return x
-
-
-class Flip(nn.Module):
- def forward(self, x, *args, reverse=False, **kwargs):
- x = torch.flip(x, [1])
- if not reverse:
- logdet = torch.zeros(x.size(0)).to(dtype=x.dtype, device=x.device)
- return x, logdet
- else:
- return x
-
-
-class ElementwiseAffine(nn.Module):
- def __init__(self, channels):
- super().__init__()
- self.channels = channels
- self.m = nn.Parameter(torch.zeros(channels,1))
- self.logs = nn.Parameter(torch.zeros(channels,1))
-
- def forward(self, x, x_mask, reverse=False, **kwargs):
- if not reverse:
- y = self.m + torch.exp(self.logs) * x
- y = y * x_mask
- logdet = torch.sum(self.logs * x_mask, [1,2])
- return y, logdet
- else:
- x = (x - self.m) * torch.exp(-self.logs) * x_mask
- return x
-
-
-class ResidualCouplingLayer(nn.Module):
- def __init__(self,
- channels,
- hidden_channels,
- kernel_size,
- dilation_rate,
- n_layers,
- p_dropout=0,
- gin_channels=0,
- mean_only=False):
- assert channels % 2 == 0, "channels should be divisible by 2"
- super().__init__()
- self.channels = channels
- self.hidden_channels = hidden_channels
- self.kernel_size = kernel_size
- self.dilation_rate = dilation_rate
- self.n_layers = n_layers
- self.half_channels = channels // 2
- self.mean_only = mean_only
-
- self.pre = nn.Conv1d(self.half_channels, hidden_channels, 1)
- self.enc = WN(hidden_channels, kernel_size, dilation_rate, n_layers, p_dropout=p_dropout, gin_channels=gin_channels)
- self.post = nn.Conv1d(hidden_channels, self.half_channels * (2 - mean_only), 1)
- self.post.weight.data.zero_()
- self.post.bias.data.zero_()
-
- def forward(self, x, x_mask, g=None, reverse=False):
- x0, x1 = torch.split(x, [self.half_channels]*2, 1)
- h = self.pre(x0) * x_mask
- h = self.enc(h, x_mask, g=g)
- stats = self.post(h) * x_mask
- if not self.mean_only:
- m, logs = torch.split(stats, [self.half_channels]*2, 1)
- else:
- m = stats
- logs = torch.zeros_like(m)
-
- if not reverse:
- x1 = m + x1 * torch.exp(logs) * x_mask
- x = torch.cat([x0, x1], 1)
- logdet = torch.sum(logs, [1,2])
- return x, logdet
- else:
- x1 = (x1 - m) * torch.exp(-logs) * x_mask
- x = torch.cat([x0, x1], 1)
- return x
-
-
-class ConvFlow(nn.Module):
- def __init__(self, in_channels, filter_channels, kernel_size, n_layers, num_bins=10, tail_bound=5.0):
- super().__init__()
- self.in_channels = in_channels
- self.filter_channels = filter_channels
- self.kernel_size = kernel_size
- self.n_layers = n_layers
- self.num_bins = num_bins
- self.tail_bound = tail_bound
- self.half_channels = in_channels // 2
-
- self.pre = nn.Conv1d(self.half_channels, filter_channels, 1)
- self.convs = DDSConv(filter_channels, kernel_size, n_layers, p_dropout=0.)
- self.proj = nn.Conv1d(filter_channels, self.half_channels * (num_bins * 3 - 1), 1)
- self.proj.weight.data.zero_()
- self.proj.bias.data.zero_()
-
- def forward(self, x, x_mask, g=None, reverse=False):
- x0, x1 = torch.split(x, [self.half_channels]*2, 1)
- h = self.pre(x0)
- h = self.convs(h, x_mask, g=g)
- h = self.proj(h) * x_mask
-
- b, c, t = x0.shape
- h = h.reshape(b, c, -1, t).permute(0, 1, 3, 2) # [b, cx?, t] -> [b, c, t, ?]
-
- unnormalized_widths = h[..., :self.num_bins] / math.sqrt(self.filter_channels)
- unnormalized_heights = h[..., self.num_bins:2*self.num_bins] / math.sqrt(self.filter_channels)
- unnormalized_derivatives = h[..., 2 * self.num_bins:]
-
- x1, logabsdet = piecewise_rational_quadratic_transform(x1,
- unnormalized_widths,
- unnormalized_heights,
- unnormalized_derivatives,
- inverse=reverse,
- tails='linear',
- tail_bound=self.tail_bound
- )
-
- x = torch.cat([x0, x1], 1) * x_mask
- logdet = torch.sum(logabsdet * x_mask, [1,2])
- if not reverse:
- return x, logdet
- else:
- return x
diff --git a/spaces/a-v-bely/russian-task-generator/README.md b/spaces/a-v-bely/russian-task-generator/README.md
deleted file mode 100644
index 1d661d5fdd019d0cb5dce142430e3f12048c002a..0000000000000000000000000000000000000000
--- a/spaces/a-v-bely/russian-task-generator/README.md
+++ /dev/null
@@ -1,12 +0,0 @@
----
-title: Russian Task Generator
-emoji: 📚
-colorFrom: pink
-colorTo: yellow
-sdk: streamlit
-sdk_version: 1.20.0
-app_file: app.py
-pinned: false
----
-
-Check out the configuration reference at https://huggingface.co/docs/hub/spaces-config-reference
diff --git a/spaces/abdulsamod/crop_yield/README.md b/spaces/abdulsamod/crop_yield/README.md
deleted file mode 100644
index 2affd3f4903737b4c089517cf1f9242620296fdc..0000000000000000000000000000000000000000
--- a/spaces/abdulsamod/crop_yield/README.md
+++ /dev/null
@@ -1,12 +0,0 @@
----
-title: Crop Yield
-emoji: 🦀
-colorFrom: pink
-colorTo: yellow
-sdk: gradio
-sdk_version: 3.16.2
-app_file: app.py
-pinned: false
----
-
-Check out the configuration reference at https://huggingface.co/docs/hub/spaces-config-reference
diff --git a/spaces/abdvl/datahub_qa_bot/docs/api/tutorials/adding-column-description.md b/spaces/abdvl/datahub_qa_bot/docs/api/tutorials/adding-column-description.md
deleted file mode 100644
index fb7bf34f6e7d8853467592f55dcb3c743c030b68..0000000000000000000000000000000000000000
--- a/spaces/abdvl/datahub_qa_bot/docs/api/tutorials/adding-column-description.md
+++ /dev/null
@@ -1,200 +0,0 @@
-# Adding Description on Columns
-
-## Why Would You Add Description on Columns?
-Adding column descriptions(documentation) to a dataset can provide crucial context for understanding the data and its variables. This can aid in data exploration, cleaning, and analysis, as well as ensure that others can understand the data if it is shared or used in collaboration. Additionally, column descriptions can help prevent errors and misunderstandings by clearly defining the meaning and units of measurement for each variable.
-
-### Goal Of This Guide
-This guide will show you how to add a description to `user_name `column of a dataset `fct_users_deleted`.
-
-
-## Prerequisites
-For this tutorial, you need to deploy DataHub Quickstart and ingest sample data.
-For detailed steps, please refer to [Prepare Local DataHub Environment](/docs/api/tutorials/references/prepare-datahub.md).
-
-:::note
-Before adding a description, you need to ensure the targeted dataset is already present in your datahub.
-If you attempt to manipulate entities that do not exist, your operation will fail.
-In this guide, we will be using data from sample ingestion.
-:::
-
-In this example, we will add a description to `user_name `column of a dataset `fct_users_deleted`.
-
-## Add Description With GraphQL
-
-:::note
-Please note that there are two available endpoints (`:8000`, `:9002`) to access GraphQL.
-For more information about the differences between these endpoints, please refer to [DataHub Metadata Service](../../../metadata-service/README.md#graphql-api)
-:::
-
-### GraphQL Explorer
-GraphQL Explorer is the fastest way to experiment with GraphQL without any dependencies.
-Navigate to GraphQL Explorer (`http://localhost:9002/api/graphiql`) and run the following query.
-
-```json
-mutation updateDescription {
- updateDescription(
- input: {
- description: "Name of the user who was deleted. This description is updated via GrpahQL.",
- resourceUrn:"urn:li:dataset:(urn:li:dataPlatform:hive,fct_users_deleted,PROD)",
- subResource: "user_name",
- subResourceType:DATASET_FIELD
- }
- )
-}
-```
-
-Note that you can use general markdown in `description`. For example, you can do the following.
-
-```json
-mutation updateDescription {
- updateDescription(
- input: {
- description: """
- ### User Name
- The `user_name` column is a primary key column that contains the name of the user who was deleted.
- """,
- resourceUrn:"urn:li:dataset:(urn:li:dataPlatform:hive,fct_users_deleted,PROD)",
- subResource: "user_name",
- subResourceType:DATASET_FIELD
- }
- )
-}
-```
-
-`updateDescription` currently only supports Dataset Schema Fields, Containers.
-For more information about the `updateDescription` mutation, please refer to [updateLineage](https://datahubproject.io/docs/graphql/mutations/#updateDescription).
-
-
-If you see the following response, the operation was successful:
-```python
-{
- "data": {
- "updateDescription": true
- },
- "extensions": {}
-}
-```
-
-### CURL
-
-With CURL, you need to provide tokens. To generate a token, please refer to [Generate Access Token](/docs/api/tutorials/references/generate-access-token.md).
-With `accessToken`, you can run the following command.
-
-```shell
-curl --location --request POST 'http://localhost:8080/api/graphql' \
---header 'Authorization: Bearer ' \
---header 'Content-Type: application/json' \
---data-raw '{ "query": "mutation updateDescription { updateDescription ( input: { description: \"Name of the user who was deleted. This description is updated via GrpahQL.\", resourceUrn: \"urn:li:dataset:(urn:li:dataPlatform:hive,fct_users_deleted,PROD)\", subResource: \"user_name\", subResourceType:DATASET_FIELD }) }", "variables":{}}'
-```
-Expected Response:
-```json
-{"data":{"updateDescription":true},"extensions":{}}
-```
-
-
-## Add Description With Python SDK
-Following code add a description to `user_name `column of a dataset `fct_users_deleted`.
-
-```python
-import logging
-import time
-
-from datahub.emitter.mce_builder import make_dataset_urn
-from datahub.emitter.mcp import MetadataChangeProposalWrapper
-
-# read-modify-write requires access to the DataHubGraph (RestEmitter is not enough)
-from datahub.ingestion.graph.client import DatahubClientConfig, DataHubGraph
-
-# Imports for metadata model classes
-from datahub.metadata.schema_classes import (
- AuditStampClass,
- InstitutionalMemoryClass,
- EditableSchemaMetadataClass,
- EditableSchemaFieldInfoClass,
-)
-
-log = logging.getLogger(__name__)
-logging.basicConfig(level=logging.INFO)
-
-def get_simple_field_path_from_v2_field_path(field_path: str) -> str:
- """A helper function to extract simple . path notation from the v2 field path"""
- if not field_path.startswith("[version=2.0]"):
- # not a v2, we assume this is a simple path
- return field_path
- # this is a v2 field path
- tokens = [
- t for t in field_path.split(".") if not (t.startswith("[") or t.endswith("]"))
- ]
-
- return ".".join(tokens)
-
-# Inputs -> owner, ownership_type, dataset
-documentation_to_add = "Name of the user who was deleted. This description is updated via PythonSDK."
-dataset_urn = make_dataset_urn(platform="hive", name="fct_users_deleted", env="PROD")
-column = "user_name"
-field_info_to_set = EditableSchemaFieldInfoClass(
- fieldPath=column, description=documentation_to_add
-)
-
-
-# Some helpful variables to fill out objects later
-now = int(time.time() * 1000) # milliseconds since epoch
-current_timestamp = AuditStampClass(time=now, actor="urn:li:corpuser:ingestion")
-
-
-# First we get the current owners
-gms_endpoint = "http://localhost:8080"
-graph = DataHubGraph(config=DatahubClientConfig(server=gms_endpoint))
-
-current_editable_schema_metadata = graph.get_aspect(
- entity_urn=dataset_urn,
- aspect_type=EditableSchemaMetadataClass,
-)
-
-
-need_write = False
-
-if current_editable_schema_metadata:
- for fieldInfo in current_editable_schema_metadata.editableSchemaFieldInfo:
- if get_simple_field_path_from_v2_field_path(fieldInfo.fieldPath) == column:
- # we have some editable schema metadata for this field
- field_match = True
- if documentation_to_add != fieldInfo.description:
- fieldInfo.description = documentation_to_add
- need_write = True
-else:
- # create a brand new editable dataset properties aspect
- current_editable_schema_metadata = EditableSchemaMetadataClass(
- editableSchemaFieldInfo=[field_info_to_set],
- created=current_timestamp,
- )
- need_write = True
-
-if need_write:
- event: MetadataChangeProposalWrapper = MetadataChangeProposalWrapper(
- entityUrn=dataset_urn,
- aspect=current_editable_schema_metadata,
- )
- graph.emit(event)
- log.info(f"Documentation added to dataset {dataset_urn}")
-
-else:
- log.info("Documentation already exists and is identical, omitting write")
-
-
-current_institutional_memory = graph.get_aspect(
- entity_urn=dataset_urn, aspect_type=InstitutionalMemoryClass
-)
-
-need_write = False
-```
-
-We're using the `MetdataChangeProposalWrapper` to change entities in this example.
-For more information about the `MetadataChangeProposal`, please refer to [MetadataChangeProposal & MetadataChangeLog Events](/docs/advanced/mcp-mcl.md)
-
-
-## Expected Outcomes
-You can now see column description is added to `user_name` column of `fct_users_deleted`.
-
-
-
diff --git a/spaces/abdvl/datahub_qa_bot/docs/what/graph.md b/spaces/abdvl/datahub_qa_bot/docs/what/graph.md
deleted file mode 100644
index 092ba0e7838d5253f85a49bd0e45e3785be2e601..0000000000000000000000000000000000000000
--- a/spaces/abdvl/datahub_qa_bot/docs/what/graph.md
+++ /dev/null
@@ -1,15 +0,0 @@
-# What is GMA graph?
-
-All the [entities](entity.md) and [relationships](relationship.md) are stored in a graph database, Neo4j.
-The graph always represents the current state of the world and has no direct support for versioning or history.
-However, as stated in the [Metadata Modeling](../modeling/metadata-model.md) section,
-the graph is merely a derived view of all metadata [aspects](aspect.md) thus can always be rebuilt directly from historic [MAEs](mxe.md#metadata-audit-event-mae).
-Consequently, it is possible to build a specific snapshot of the graph in time by replaying MAEs up to that point.
-
-In theory, the system can work with any generic [OLTP](https://en.wikipedia.org/wiki/Online_transaction_processing) graph DB that supports the following operations:
-* Dynamical creation, modification, and removal of nodes and edges
-* Dynamical attachment of key-value properties to each node and edge
-* Transactional partial updates of properties of a specific node or edge
-* Fast ID-based retrieval of nodes & edges
-* Efficient queries involving both graph traversal and properties value filtering
-* Support efficient bidirectional graph traversal
diff --git a/spaces/abhaskumarsinha/MinimalGPT-Felis_Catus/subword/chrF.py b/spaces/abhaskumarsinha/MinimalGPT-Felis_Catus/subword/chrF.py
deleted file mode 100644
index 3a35941d61b618a8b32d937b51f0d10071129bd6..0000000000000000000000000000000000000000
--- a/spaces/abhaskumarsinha/MinimalGPT-Felis_Catus/subword/chrF.py
+++ /dev/null
@@ -1,139 +0,0 @@
-#!/usr/bin/env python
-# -*- coding: utf-8 -*-
-# Author: Rico Sennrich
-
-"""Compute chrF3 for machine translation evaluation
-
-Reference:
-Maja Popović (2015). chrF: character n-gram F-score for automatic MT evaluation. In Proceedings of the Tenth Workshop on Statistical Machine Translationn, pages 392–395, Lisbon, Portugal.
-"""
-
-from __future__ import print_function, unicode_literals, division
-
-import sys
-import codecs
-import io
-import argparse
-
-from collections import defaultdict
-
-# hack for python2/3 compatibility
-from io import open
-argparse.open = open
-
-def create_parser():
- parser = argparse.ArgumentParser(
- formatter_class=argparse.RawDescriptionHelpFormatter,
- description="learn BPE-based word segmentation")
-
- parser.add_argument(
- '--ref', '-r', type=argparse.FileType('r'), required=True,
- metavar='PATH',
- help="Reference file")
- parser.add_argument(
- '--hyp', type=argparse.FileType('r'), metavar='PATH',
- default=sys.stdin,
- help="Hypothesis file (default: stdin).")
- parser.add_argument(
- '--beta', '-b', type=float, default=3,
- metavar='FLOAT',
- help="beta parameter (default: '%(default)s')")
- parser.add_argument(
- '--ngram', '-n', type=int, default=6,
- metavar='INT',
- help="ngram order (default: '%(default)s')")
- parser.add_argument(
- '--space', '-s', action='store_true',
- help="take spaces into account (default: '%(default)s')")
- parser.add_argument(
- '--precision', action='store_true',
- help="report precision (default: '%(default)s')")
- parser.add_argument(
- '--recall', action='store_true',
- help="report recall (default: '%(default)s')")
-
- return parser
-
-def extract_ngrams(words, max_length=4, spaces=False):
-
- if not spaces:
- words = ''.join(words.split())
- else:
- words = words.strip()
-
- results = defaultdict(lambda: defaultdict(int))
- for length in range(max_length):
- for start_pos in range(len(words)):
- end_pos = start_pos + length + 1
- if end_pos <= len(words):
- results[length][tuple(words[start_pos: end_pos])] += 1
- return results
-
-
-def get_correct(ngrams_ref, ngrams_test, correct, total):
-
- for rank in ngrams_test:
- for chain in ngrams_test[rank]:
- total[rank] += ngrams_test[rank][chain]
- if chain in ngrams_ref[rank]:
- correct[rank] += min(ngrams_test[rank][chain], ngrams_ref[rank][chain])
-
- return correct, total
-
-
-def f1(correct, total_hyp, total_ref, max_length, beta=3, smooth=0):
-
- precision = 0
- recall = 0
-
- for i in range(max_length):
- if total_hyp[i] + smooth and total_ref[i] + smooth:
- precision += (correct[i] + smooth) / (total_hyp[i] + smooth)
- recall += (correct[i] + smooth) / (total_ref[i] + smooth)
-
- precision /= max_length
- recall /= max_length
-
- return (1 + beta**2) * (precision*recall) / ((beta**2 * precision) + recall), precision, recall
-
-def main(args):
-
- correct = [0]*args.ngram
- total = [0]*args.ngram
- total_ref = [0]*args.ngram
- for line in args.ref:
- line2 = args.hyp.readline()
-
- ngrams_ref = extract_ngrams(line, max_length=args.ngram, spaces=args.space)
- ngrams_test = extract_ngrams(line2, max_length=args.ngram, spaces=args.space)
-
- get_correct(ngrams_ref, ngrams_test, correct, total)
-
- for rank in ngrams_ref:
- for chain in ngrams_ref[rank]:
- total_ref[rank] += ngrams_ref[rank][chain]
-
- chrf, precision, recall = f1(correct, total, total_ref, args.ngram, args.beta)
-
- print('chrF3: {0:.4f}'.format(chrf))
- if args.precision:
- print('chrPrec: {0:.4f}'.format(precision))
- if args.recall:
- print('chrRec: {0:.4f}'.format(recall))
-
-if __name__ == '__main__':
-
- # python 2/3 compatibility
- if sys.version_info < (3, 0):
- sys.stderr = codecs.getwriter('UTF-8')(sys.stderr)
- sys.stdout = codecs.getwriter('UTF-8')(sys.stdout)
- sys.stdin = codecs.getreader('UTF-8')(sys.stdin)
- else:
- sys.stdin = io.TextIOWrapper(sys.stdin.buffer, encoding='utf-8')
- sys.stderr = io.TextIOWrapper(sys.stderr.buffer, encoding='utf-8')
- sys.stdout = io.TextIOWrapper(sys.stdout.buffer, encoding='utf-8', write_through=True, line_buffering=True)
-
- parser = create_parser()
- args = parser.parse_args()
-
- main(args)
diff --git a/spaces/abhishek/sketch-to-image/annotator/uniformer/mmdet_null/models/backbones/darknet.py b/spaces/abhishek/sketch-to-image/annotator/uniformer/mmdet_null/models/backbones/darknet.py
deleted file mode 100644
index 517fe26259217792e0dad80ca3824d914cfe3904..0000000000000000000000000000000000000000
--- a/spaces/abhishek/sketch-to-image/annotator/uniformer/mmdet_null/models/backbones/darknet.py
+++ /dev/null
@@ -1,199 +0,0 @@
-# Copyright (c) 2019 Western Digital Corporation or its affiliates.
-
-import logging
-
-import torch.nn as nn
-from mmcv.cnn import ConvModule, constant_init, kaiming_init
-from mmcv.runner import load_checkpoint
-from torch.nn.modules.batchnorm import _BatchNorm
-
-from ..builder import BACKBONES
-
-
-class ResBlock(nn.Module):
- """The basic residual block used in Darknet. Each ResBlock consists of two
- ConvModules and the input is added to the final output. Each ConvModule is
- composed of Conv, BN, and LeakyReLU. In YoloV3 paper, the first convLayer
- has half of the number of the filters as much as the second convLayer. The
- first convLayer has filter size of 1x1 and the second one has the filter
- size of 3x3.
-
- Args:
- in_channels (int): The input channels. Must be even.
- conv_cfg (dict): Config dict for convolution layer. Default: None.
- norm_cfg (dict): Dictionary to construct and config norm layer.
- Default: dict(type='BN', requires_grad=True)
- act_cfg (dict): Config dict for activation layer.
- Default: dict(type='LeakyReLU', negative_slope=0.1).
- """
-
- def __init__(self,
- in_channels,
- conv_cfg=None,
- norm_cfg=dict(type='BN', requires_grad=True),
- act_cfg=dict(type='LeakyReLU', negative_slope=0.1)):
- super(ResBlock, self).__init__()
- assert in_channels % 2 == 0 # ensure the in_channels is even
- half_in_channels = in_channels // 2
-
- # shortcut
- cfg = dict(conv_cfg=conv_cfg, norm_cfg=norm_cfg, act_cfg=act_cfg)
-
- self.conv1 = ConvModule(in_channels, half_in_channels, 1, **cfg)
- self.conv2 = ConvModule(
- half_in_channels, in_channels, 3, padding=1, **cfg)
-
- def forward(self, x):
- residual = x
- out = self.conv1(x)
- out = self.conv2(out)
- out = out + residual
-
- return out
-
-
-@BACKBONES.register_module()
-class Darknet(nn.Module):
- """Darknet backbone.
-
- Args:
- depth (int): Depth of Darknet. Currently only support 53.
- out_indices (Sequence[int]): Output from which stages.
- frozen_stages (int): Stages to be frozen (stop grad and set eval mode).
- -1 means not freezing any parameters. Default: -1.
- conv_cfg (dict): Config dict for convolution layer. Default: None.
- norm_cfg (dict): Dictionary to construct and config norm layer.
- Default: dict(type='BN', requires_grad=True)
- act_cfg (dict): Config dict for activation layer.
- Default: dict(type='LeakyReLU', negative_slope=0.1).
- norm_eval (bool): Whether to set norm layers to eval mode, namely,
- freeze running stats (mean and var). Note: Effect on Batch Norm
- and its variants only.
-
- Example:
- >>> from mmdet.models import Darknet
- >>> import torch
- >>> self = Darknet(depth=53)
- >>> self.eval()
- >>> inputs = torch.rand(1, 3, 416, 416)
- >>> level_outputs = self.forward(inputs)
- >>> for level_out in level_outputs:
- ... print(tuple(level_out.shape))
- ...
- (1, 256, 52, 52)
- (1, 512, 26, 26)
- (1, 1024, 13, 13)
- """
-
- # Dict(depth: (layers, channels))
- arch_settings = {
- 53: ((1, 2, 8, 8, 4), ((32, 64), (64, 128), (128, 256), (256, 512),
- (512, 1024)))
- }
-
- def __init__(self,
- depth=53,
- out_indices=(3, 4, 5),
- frozen_stages=-1,
- conv_cfg=None,
- norm_cfg=dict(type='BN', requires_grad=True),
- act_cfg=dict(type='LeakyReLU', negative_slope=0.1),
- norm_eval=True):
- super(Darknet, self).__init__()
- if depth not in self.arch_settings:
- raise KeyError(f'invalid depth {depth} for darknet')
- self.depth = depth
- self.out_indices = out_indices
- self.frozen_stages = frozen_stages
- self.layers, self.channels = self.arch_settings[depth]
-
- cfg = dict(conv_cfg=conv_cfg, norm_cfg=norm_cfg, act_cfg=act_cfg)
-
- self.conv1 = ConvModule(3, 32, 3, padding=1, **cfg)
-
- self.cr_blocks = ['conv1']
- for i, n_layers in enumerate(self.layers):
- layer_name = f'conv_res_block{i + 1}'
- in_c, out_c = self.channels[i]
- self.add_module(
- layer_name,
- self.make_conv_res_block(in_c, out_c, n_layers, **cfg))
- self.cr_blocks.append(layer_name)
-
- self.norm_eval = norm_eval
-
- def forward(self, x):
- outs = []
- for i, layer_name in enumerate(self.cr_blocks):
- cr_block = getattr(self, layer_name)
- x = cr_block(x)
- if i in self.out_indices:
- outs.append(x)
-
- return tuple(outs)
-
- def init_weights(self, pretrained=None):
- if isinstance(pretrained, str):
- logger = logging.getLogger()
- load_checkpoint(self, pretrained, strict=False, logger=logger)
- elif pretrained is None:
- for m in self.modules():
- if isinstance(m, nn.Conv2d):
- kaiming_init(m)
- elif isinstance(m, (_BatchNorm, nn.GroupNorm)):
- constant_init(m, 1)
-
- else:
- raise TypeError('pretrained must be a str or None')
-
- def _freeze_stages(self):
- if self.frozen_stages >= 0:
- for i in range(self.frozen_stages):
- m = getattr(self, self.cr_blocks[i])
- m.eval()
- for param in m.parameters():
- param.requires_grad = False
-
- def train(self, mode=True):
- super(Darknet, self).train(mode)
- self._freeze_stages()
- if mode and self.norm_eval:
- for m in self.modules():
- if isinstance(m, _BatchNorm):
- m.eval()
-
- @staticmethod
- def make_conv_res_block(in_channels,
- out_channels,
- res_repeat,
- conv_cfg=None,
- norm_cfg=dict(type='BN', requires_grad=True),
- act_cfg=dict(type='LeakyReLU',
- negative_slope=0.1)):
- """In Darknet backbone, ConvLayer is usually followed by ResBlock. This
- function will make that. The Conv layers always have 3x3 filters with
- stride=2. The number of the filters in Conv layer is the same as the
- out channels of the ResBlock.
-
- Args:
- in_channels (int): The number of input channels.
- out_channels (int): The number of output channels.
- res_repeat (int): The number of ResBlocks.
- conv_cfg (dict): Config dict for convolution layer. Default: None.
- norm_cfg (dict): Dictionary to construct and config norm layer.
- Default: dict(type='BN', requires_grad=True)
- act_cfg (dict): Config dict for activation layer.
- Default: dict(type='LeakyReLU', negative_slope=0.1).
- """
-
- cfg = dict(conv_cfg=conv_cfg, norm_cfg=norm_cfg, act_cfg=act_cfg)
-
- model = nn.Sequential()
- model.add_module(
- 'conv',
- ConvModule(
- in_channels, out_channels, 3, stride=2, padding=1, **cfg))
- for idx in range(res_repeat):
- model.add_module('res{}'.format(idx),
- ResBlock(out_channels, **cfg))
- return model
diff --git a/spaces/abhishek/sketch-to-image/annotator/uniformer/mmdet_null/models/dense_heads/pisa_retinanet_head.py b/spaces/abhishek/sketch-to-image/annotator/uniformer/mmdet_null/models/dense_heads/pisa_retinanet_head.py
deleted file mode 100644
index bd87b9aeb07e05ff94b444ac8999eca3f616711a..0000000000000000000000000000000000000000
--- a/spaces/abhishek/sketch-to-image/annotator/uniformer/mmdet_null/models/dense_heads/pisa_retinanet_head.py
+++ /dev/null
@@ -1,154 +0,0 @@
-import torch
-from mmcv.runner import force_fp32
-
-from mmdet.core import images_to_levels
-from ..builder import HEADS
-from ..losses import carl_loss, isr_p
-from .retina_head import RetinaHead
-
-
-@HEADS.register_module()
-class PISARetinaHead(RetinaHead):
- """PISA Retinanet Head.
-
- The head owns the same structure with Retinanet Head, but differs in two
- aspects:
- 1. Importance-based Sample Reweighting Positive (ISR-P) is applied to
- change the positive loss weights.
- 2. Classification-aware regression loss is adopted as a third loss.
- """
-
- @force_fp32(apply_to=('cls_scores', 'bbox_preds'))
- def loss(self,
- cls_scores,
- bbox_preds,
- gt_bboxes,
- gt_labels,
- img_metas,
- gt_bboxes_ignore=None):
- """Compute losses of the head.
-
- Args:
- cls_scores (list[Tensor]): Box scores for each scale level
- Has shape (N, num_anchors * num_classes, H, W)
- bbox_preds (list[Tensor]): Box energies / deltas for each scale
- level with shape (N, num_anchors * 4, H, W)
- gt_bboxes (list[Tensor]): Ground truth bboxes of each image
- with shape (num_obj, 4).
- gt_labels (list[Tensor]): Ground truth labels of each image
- with shape (num_obj, 4).
- img_metas (list[dict]): Meta information of each image, e.g.,
- image size, scaling factor, etc.
- gt_bboxes_ignore (list[Tensor]): Ignored gt bboxes of each image.
- Default: None.
-
- Returns:
- dict: Loss dict, comprise classification loss, regression loss and
- carl loss.
- """
- featmap_sizes = [featmap.size()[-2:] for featmap in cls_scores]
- assert len(featmap_sizes) == self.anchor_generator.num_levels
-
- device = cls_scores[0].device
-
- anchor_list, valid_flag_list = self.get_anchors(
- featmap_sizes, img_metas, device=device)
- label_channels = self.cls_out_channels if self.use_sigmoid_cls else 1
- cls_reg_targets = self.get_targets(
- anchor_list,
- valid_flag_list,
- gt_bboxes,
- img_metas,
- gt_bboxes_ignore_list=gt_bboxes_ignore,
- gt_labels_list=gt_labels,
- label_channels=label_channels,
- return_sampling_results=True)
- if cls_reg_targets is None:
- return None
- (labels_list, label_weights_list, bbox_targets_list, bbox_weights_list,
- num_total_pos, num_total_neg, sampling_results_list) = cls_reg_targets
- num_total_samples = (
- num_total_pos + num_total_neg if self.sampling else num_total_pos)
-
- # anchor number of multi levels
- num_level_anchors = [anchors.size(0) for anchors in anchor_list[0]]
- # concat all level anchors and flags to a single tensor
- concat_anchor_list = []
- for i in range(len(anchor_list)):
- concat_anchor_list.append(torch.cat(anchor_list[i]))
- all_anchor_list = images_to_levels(concat_anchor_list,
- num_level_anchors)
-
- num_imgs = len(img_metas)
- flatten_cls_scores = [
- cls_score.permute(0, 2, 3, 1).reshape(num_imgs, -1, label_channels)
- for cls_score in cls_scores
- ]
- flatten_cls_scores = torch.cat(
- flatten_cls_scores, dim=1).reshape(-1,
- flatten_cls_scores[0].size(-1))
- flatten_bbox_preds = [
- bbox_pred.permute(0, 2, 3, 1).reshape(num_imgs, -1, 4)
- for bbox_pred in bbox_preds
- ]
- flatten_bbox_preds = torch.cat(
- flatten_bbox_preds, dim=1).view(-1, flatten_bbox_preds[0].size(-1))
- flatten_labels = torch.cat(labels_list, dim=1).reshape(-1)
- flatten_label_weights = torch.cat(
- label_weights_list, dim=1).reshape(-1)
- flatten_anchors = torch.cat(all_anchor_list, dim=1).reshape(-1, 4)
- flatten_bbox_targets = torch.cat(
- bbox_targets_list, dim=1).reshape(-1, 4)
- flatten_bbox_weights = torch.cat(
- bbox_weights_list, dim=1).reshape(-1, 4)
-
- # Apply ISR-P
- isr_cfg = self.train_cfg.get('isr', None)
- if isr_cfg is not None:
- all_targets = (flatten_labels, flatten_label_weights,
- flatten_bbox_targets, flatten_bbox_weights)
- with torch.no_grad():
- all_targets = isr_p(
- flatten_cls_scores,
- flatten_bbox_preds,
- all_targets,
- flatten_anchors,
- sampling_results_list,
- bbox_coder=self.bbox_coder,
- loss_cls=self.loss_cls,
- num_class=self.num_classes,
- **self.train_cfg.isr)
- (flatten_labels, flatten_label_weights, flatten_bbox_targets,
- flatten_bbox_weights) = all_targets
-
- # For convenience we compute loss once instead separating by fpn level,
- # so that we don't need to separate the weights by level again.
- # The result should be the same
- losses_cls = self.loss_cls(
- flatten_cls_scores,
- flatten_labels,
- flatten_label_weights,
- avg_factor=num_total_samples)
- losses_bbox = self.loss_bbox(
- flatten_bbox_preds,
- flatten_bbox_targets,
- flatten_bbox_weights,
- avg_factor=num_total_samples)
- loss_dict = dict(loss_cls=losses_cls, loss_bbox=losses_bbox)
-
- # CARL Loss
- carl_cfg = self.train_cfg.get('carl', None)
- if carl_cfg is not None:
- loss_carl = carl_loss(
- flatten_cls_scores,
- flatten_labels,
- flatten_bbox_preds,
- flatten_bbox_targets,
- self.loss_bbox,
- **self.train_cfg.carl,
- avg_factor=num_total_pos,
- sigmoid=True,
- num_class=self.num_classes)
- loss_dict.update(loss_carl)
-
- return loss_dict
diff --git a/spaces/aimaswx/my_streamchat/app.py b/spaces/aimaswx/my_streamchat/app.py
deleted file mode 100644
index 84ff5fd1dcdde4b45aad87113226c47d056c01d7..0000000000000000000000000000000000000000
--- a/spaces/aimaswx/my_streamchat/app.py
+++ /dev/null
@@ -1,34 +0,0 @@
-# chat_bot.py
-
-import openai
-import streamlit as st
-from streamlit_chat import message
-
-#申请的api_key
-openai.api_key = "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"
-def generate_response(prompt):
- completion = openai.Completion.create(
- model="text-davinci-003",
- prompt=prompt,
- max_tokens=1024,
- temperature=0
- )
- message=completion.choices[0].text
- return message
-
-st.markdown("#### 我是ChatGPT聊天机器人,我可以回答您的任何问题!")
-if 'generated' not in st.session_state:
- st.session_state['generated'] = []
-if 'past' not in st.session_state:
- st.session_state['past'] = []
-user_input=st.text_input("请输入您的问题:",key='input')
-if user_input:
- output=generate_response(user_input)
- st.session_state['past'].append(user_input)
- st.session_state['generated'].append(output)
-if st.session_state['generated']:
- for i in range(len(st.session_state['generated'])-1, -1, -1):
- message(st.session_state["generated"][i], key=str(i))
- message(st.session_state['past'][i],
- is_user=True,
- key=str(i)+'_user')
\ No newline at end of file
diff --git a/spaces/akhaliq/SummerTime/model/third_party/HMNet/ThirdParty/ROUGE/ROUGE-1.5.5/runROUGE-test.pl b/spaces/akhaliq/SummerTime/model/third_party/HMNet/ThirdParty/ROUGE/ROUGE-1.5.5/runROUGE-test.pl
deleted file mode 100644
index ef36a278a9630fc182b89c997e7c9ff0c827a65d..0000000000000000000000000000000000000000
--- a/spaces/akhaliq/SummerTime/model/third_party/HMNet/ThirdParty/ROUGE/ROUGE-1.5.5/runROUGE-test.pl
+++ /dev/null
@@ -1,51 +0,0 @@
-#!/usr/bin/perl -w
-use Cwd;
-$curdir=getcwd;
-$ROUGE="../ROUGE-1.5.5.pl";
-chdir("sample-test");
-$cmd="$ROUGE -e ../data -c 95 -2 -1 -U -r 1000 -n 4 -w 1.2 -a ROUGE-test.xml > ../sample-output/ROUGE-test-c95-2-1-U-r1000-n4-w1.2-a.out";
-print $cmd,"\n";
-system($cmd);
-$cmd="$ROUGE -e ../data -c 95 -2 -1 -U -r 1000 -n 4 -w 1.2 -a -m ROUGE-test.xml > ../sample-output/ROUGE-test-c95-2-1-U-r1000-n4-w1.2-a-m.out";
-print $cmd,"\n";
-system($cmd);
-$cmd="$ROUGE -e ../data -c 95 -2 -1 -U -r 1000 -n 4 -w 1.2 -a -m -s ROUGE-test.xml > ../sample-output/ROUGE-test-c95-2-1-U-r1000-n4-w1.2-a-m-s.out";
-print $cmd,"\n";
-system($cmd);
-$cmd="$ROUGE -e ../data -c 95 -2 -1 -U -r 1000 -n 4 -w 1.2 -l 10 -a ROUGE-test.xml > ../sample-output/ROUGE-test-c95-2-1-U-r1000-n4-w1.2-l10-a.out";
-print $cmd,"\n";
-system($cmd);
-$cmd="$ROUGE -e ../data -c 95 -2 -1 -U -r 1000 -n 4 -w 1.2 -l 10 -a -m ROUGE-test.xml > ../sample-output/ROUGE-test-c95-2-1-U-r1000-n4-w1.2-l10-a-m.out";
-print $cmd,"\n";
-system($cmd);
-$cmd="$ROUGE -e ../data -c 95 -2 -1 -U -r 1000 -n 4 -w 1.2 -l 10 -a -m -s ROUGE-test.xml > ../sample-output/ROUGE-test-c95-2-1-U-r1000-n4-w1.2-l10-a-m-s.out";
-print $cmd,"\n";
-system($cmd);
-$cmd="$ROUGE -e ../data -c 95 -2 -1 -U -r 1000 -n 4 -w 1.2 -b 75 -a ROUGE-test.xml > ../sample-output/ROUGE-test-c95-2-1-U-r1000-n4-w1.2-b75-a.out";
-print $cmd,"\n";
-system($cmd);
-$cmd="$ROUGE -e ../data -c 95 -2 -1 -U -r 1000 -n 4 -w 1.2 -b 75 -a -m ROUGE-test.xml > ../sample-output/ROUGE-test-c95-2-1-U-r1000-n4-w1.2-b75-a-m.out";
-print $cmd,"\n";
-system($cmd);
-$cmd="$ROUGE -e ../data -c 95 -2 -1 -U -r 1000 -n 4 -w 1.2 -b 75 -a -m -s ROUGE-test.xml > ../sample-output/ROUGE-test-c95-2-1-U-r1000-n4-w1.2-b75-a-m-s.out";
-print $cmd,"\n";
-system($cmd);
-$cmd="$ROUGE -e ../data -3 HM -z SIMPLE DUC2002-BE-F.in.26.lst 26 > ../sample-output/DUC2002-BE-F.in.26.lst.out";
-print $cmd,"\n";
-system($cmd);
-$cmd="$ROUGE -e ../data -3 HM DUC2002-BE-F.in.26.simple.xml 26 > ../sample-output/DUC2002-BE-F.in.26.simple.out";
-print $cmd,"\n";
-system($cmd);
-$cmd="$ROUGE -e ../data -3 HM -z SIMPLE DUC2002-BE-L.in.26.lst 26 > ../sample-output/DUC2002-BE-L.in.26.lst.out";
-print $cmd,"\n";
-system($cmd);
-$cmd="$ROUGE -e ../data -3 HM DUC2002-BE-L.in.26.simple.xml 26 > ../sample-output/DUC2002-BE-L.in.26.simple.out";
-print $cmd,"\n";
-system($cmd);
-$cmd="$ROUGE -e ../data -n 4 -z SPL DUC2002-ROUGE.in.26.spl.lst 26 > ../sample-output/DUC2002-ROUGE.in.26.spl.lst.out";
-print $cmd,"\n";
-system($cmd);
-$cmd="$ROUGE -e ../data -n 4 DUC2002-ROUGE.in.26.spl.xml 26 > ../sample-output/DUC2002-ROUGE.in.26.spl.out";
-print $cmd,"\n";
-system($cmd);
-chdir($curdir);
diff --git a/spaces/akhaliq/deeplab2/model/utils.py b/spaces/akhaliq/deeplab2/model/utils.py
deleted file mode 100644
index b28a19ea3b18c8eff5039a2c6eb2270e197c8a20..0000000000000000000000000000000000000000
--- a/spaces/akhaliq/deeplab2/model/utils.py
+++ /dev/null
@@ -1,485 +0,0 @@
-# coding=utf-8
-# Copyright 2021 The Deeplab2 Authors.
-#
-# Licensed under the Apache License, Version 2.0 (the "License");
-# you may not use this file except in compliance with the License.
-# You may obtain a copy of the License at
-#
-# http://www.apache.org/licenses/LICENSE-2.0
-#
-# Unless required by applicable law or agreed to in writing, software
-# distributed under the License is distributed on an "AS IS" BASIS,
-# WITHOUT WARRANTIES OR CONDITIONS OF ANY KIND, either express or implied.
-# See the License for the specific language governing permissions and
-# limitations under the License.
-
-"""This file contains utility functions for the model code."""
-
-from typing import Any, List, MutableMapping, MutableSequence, Optional, Set
-
-import tensorflow as tf
-
-from deeplab2 import common
-from deeplab2 import config_pb2
-
-layers = tf.keras.layers
-
-_PREDICTION_WITH_NEAREST_UPSAMPLING = (
- common.PRED_INSTANCE_KEY,
- common.PRED_INSTANCE_CENTER_KEY,
- common.PRED_INSTANCE_SCORES_KEY,
- common.PRED_PANOPTIC_KEY,
- common.PRED_SEMANTIC_KEY,
- common.PRED_NEXT_PANOPTIC_KEY,
- common.PRED_CONCAT_NEXT_PANOPTIC_KEY,
- common.PRED_CENTER_HEATMAP_KEY,
-)
-
-_PREDICTION_WITH_BILINEAR_UPSAMPLING = (
- common.PRED_SEMANTIC_PROBS_KEY,
- common.PRED_OFFSET_MAP_KEY,
-)
-
-_INPUT_WITH_NEAREST_UPSAMPLING = (
- common.GT_INSTANCE_CENTER_KEY,
-)
-
-_INPUT_WITH_BILINEAR_UPSAMPLING = (
- common.IMAGE,
- common.GT_INSTANCE_REGRESSION_KEY
-)
-
-
-def _scale_helper(value, scale):
- if isinstance(value, tf.Tensor):
- return tf.cast(
- (tf.cast(value, dtype=tf.float32) - 1.0) * scale + 1.0,
- dtype=tf.int32)
- else:
- return int((float(value) - 1.0) * scale + 1.0)
-
-
-def scale_mutable_sequence(input_sequence: MutableSequence[int],
- scale: float) -> MutableSequence[int]:
- return [_scale_helper(x, scale) for x in input_sequence]
-
-
-def scale_int_list(int_list, scale):
- return [int(x * scale) for x in int_list]
-
-
-def undo_image_preprocessing(image_in: tf.Tensor, method: str,
- perform_crop: bool,
- regions_to_crop: List[int],
- output_shape: List[int]) -> tf.Tensor:
- """Undoes the image preprocessing.
-
- In particular, this function slices out the valid regions (determined by
- `regions_to_crop`) in the input when perform_crop is True. After
- that, we resize the results to the desired `output_shape`.
-
- Args:
- image_in: Input image Tensor with shape [batch, height, width, n_channels].
- method: Image resize method.
- perform_crop: Boolean, performing crop or not.
- regions_to_crop: The regions to crop [height, width]. Will only apply
- cropping at the bottom right.
- output_shape: Desired shape after resizing [height, width].
-
- Returns:
- Outputs after cropping (if perform_crop = True) and resizing.
- """
- if perform_crop:
- image_out = image_in[
- :, :regions_to_crop[0], :regions_to_crop[1], :]
- else:
- image_out = image_in
- return resize_align_corners(image_out, output_shape, method=method)
-
-
-def undo_preprocessing(input_or_prediction_dict: MutableMapping[str, Any],
- regions_to_crop: List[int],
- output_shape: List[int]) -> MutableMapping[str, Any]:
- """Undoes preprocessing for predictions.
-
- Args:
- input_or_prediction_dict: A dictionary storing different types of inputs or
- predictions.
- regions_to_crop: The regions to crop [height, width]. Will only apply
- cropping at the bottom right.
- output_shape: Desired shape after resizing [height, width].
-
- Returns:
- inputs or predictions after cropping (if perform_crop = True) and resizing.
- """
- for key in input_or_prediction_dict.keys():
- if key in _PREDICTION_WITH_NEAREST_UPSAMPLING or key in _INPUT_WITH_NEAREST_UPSAMPLING:
- input_or_prediction_dict[key] = tf.squeeze(
- undo_image_preprocessing(
- tf.expand_dims(input_or_prediction_dict[key], 3),
- 'nearest',
- perform_crop=True,
- regions_to_crop=regions_to_crop,
- output_shape=output_shape),
- axis=3)
- elif key in _PREDICTION_WITH_BILINEAR_UPSAMPLING or key in _INPUT_WITH_BILINEAR_UPSAMPLING:
- input_or_prediction_dict[key] = undo_image_preprocessing(
- input_or_prediction_dict[key],
- 'bilinear',
- perform_crop=True,
- regions_to_crop=regions_to_crop,
- output_shape=output_shape)
- else:
- # We only undo preprocessing for those defined in
- # _{PREDICTION,INPUT}_WITH_{NEAREST,BILINEAR}_UPSAMPLING.
- # Other intermediate results are skipped.
- continue
- return input_or_prediction_dict
-
-
-def add_zero_padding(input_tensor: tf.Tensor, kernel_size: int,
- rank: int) -> tf.Tensor:
- """Adds zero-padding to the input_tensor."""
- pad_total = kernel_size - 1
- pad_begin = pad_total // 2
- pad_end = pad_total - pad_begin
- if rank == 3:
- return tf.pad(
- input_tensor,
- paddings=[[pad_begin, pad_end], [pad_begin, pad_end], [0, 0]])
- else:
- return tf.pad(
- input_tensor,
- paddings=[[0, 0], [pad_begin, pad_end], [pad_begin, pad_end], [0, 0]])
-
-
-def resize_and_rescale_offsets(input_tensor: tf.Tensor, target_size):
- """Bilinearly resizes and rescales the offsets.
-
- Args:
- input_tensor: A tf.Tensor of shape [batch, height, width, 2].
- target_size: A list or tuple or 1D tf.Tensor that specifies the height and
- width after resizing.
-
- Returns:
- The input_tensor resized to shape `[batch, target_height, target_width, 2]`.
- Moreover, the offsets along the y-axis are rescaled by a factor equal to
- (target_height - 1) / (reference_height - 1) and the offsets along the
- x-axis are rescaled by a factor equal to
- (target_width - 1) / (reference_width - 1).
- """
- input_size_y = tf.shape(input_tensor)[1]
- input_size_x = tf.shape(input_tensor)[2]
-
- scale_y = tf.cast(target_size[0] - 1, tf.float32) / tf.cast(
- input_size_y - 1, tf.float32)
- scale_x = tf.cast(target_size[1] - 1, tf.float32) / tf.cast(
- input_size_x - 1, tf.float32)
-
- target_y, target_x = tf.split(
- value=input_tensor, num_or_size_splits=2, axis=3)
- target_y *= scale_y
- target_x *= scale_x
- target = tf.concat([target_y, target_x], 3)
- return resize_bilinear(target, target_size)
-
-
-def resize_align_corners(input_tensor, target_size, method='bilinear'):
- """Resizes the input_tensor to target_size.
-
- This returns the same output as tf.compat.v1.image.resize(input_tensor,
- target_size, align_corners=True).
-
- Args:
- input_tensor: A tf.Tensor of shape [batch, height, width, channels].
- target_size: A list or tuple or 1D tf.Tensor that specifies the height and
- width after resizing.
- method: An optional string specifying the method used for resizing.
- Supported options are 'nearest' and 'bilinear'.
-
- Returns:
- The resized tensor.
-
- Raises:
- ValueError: An error occurs if 1) the input tensor's rank is not 4 or 2) the
- resizing method is not supported.
- """
- if method == 'bilinear':
- tf_method = tf.compat.v1.image.ResizeMethod.BILINEAR
- elif method == 'nearest':
- tf_method = tf.compat.v1.image.ResizeMethod.NEAREST_NEIGHBOR
- else:
- raise ValueError('The given method %s is not supported. Please use bilinear'
- ' or nearest.' % method)
-
- tf.debugging.assert_rank(
- input_tensor, 4,
- message='Input tensor to resize method should have rank of 4.')
-
- return tf.compat.v1.image.resize(
- input_tensor,
- target_size,
- method=tf_method,
- align_corners=True,
- name='resize_align_corners')
-
-
-def resize_bilinear(images,
- size,
- align_corners=True,
- name=None):
- """TPU memory efficient version of tf.compat.v1.image.resize_bilinear.
-
- ResizeBilinear on TPU requires padded batch and channel dimensions. On a
- TPUv3, the worst case could lead to 256x memory consumption, if the
- input is, for example, [1, 257, 513, 1]. In this function, we replace the
- default resize_bilinear by two resize_bilinear operations, which put one image
- axis on the channel axis. This reduces TPU padding when batch * channel is
- small and height * width is large.
-
- Args:
- images: Input image of shape [B, H, W, C].
- size: A list of two elements: [height, width]. The new size for the images.
- align_corners: Whether to align corners of the image.
- name: Name of the operation.
-
- Returns:
- Resized image.
- """
- _, height, width, channel = images.get_shape().as_list()
- if height == size[0] and width == size[1]:
- return images
- dtype = images.dtype
- images = tf.cast(images, tf.float32)
- # We check the channel axis only since the batch size is similar (usually 1 or
- # 2). In this way, this if-else easily supports dynamic batch size without
- # using tf.cond().
- if channel > 32 or not align_corners:
- images = tf.compat.v1.image.resize_bilinear(
- images, size,
- align_corners=align_corners,
- name=name)
- else:
- images = tf.transpose(images, [0, 3, 1, 2])
- images = tf.compat.v1.image.resize_bilinear(
- images, [channel, size[0]],
- align_corners=align_corners,
- name=name + '_height' if name else None)
- images = tf.transpose(images, [0, 1, 3, 2])
- images = tf.compat.v1.image.resize_bilinear(
- images, [channel, size[1]],
- align_corners=align_corners,
- name=name + '_width' if name else None)
- images = tf.transpose(images, [0, 3, 2, 1])
- return tf.cast(images, dtype)
-
-
-def make_divisible(value: float,
- divisor: int,
- min_value: Optional[float] = None) -> int:
- """Ensures all layers have channels that are divisible by the divisor.
-
- Args:
- value: A `float` of original value.
- divisor: An `int` of the divisor that needs to be checked upon.
- min_value: A `float` of minimum value threshold.
-
- Returns:
- The adjusted value in `int` that is divisible by divisor.
-
- Raises:
- ValueError: Minimual value should be divisible by divisor.
- """
- if min_value is None:
- min_value = divisor
- elif min_value % divisor != 0:
- raise ValueError('Minimual value should be divisible by divisor.')
-
- new_value = max(min_value, int(value + divisor / 2) // divisor * divisor)
- # Make sure that round down does not go down by more than 10%.
- if new_value < 0.9 * value:
- new_value += divisor
- return int(new_value)
-
-
-def transpose_and_reshape_for_attention_operation(inputs):
- """Sequentially transposes and reshapes the tensor.
-
- Args:
- inputs: An input [batch, num_heads, length, channel] tensor.
-
- Returns:
- output: An output [batch, length, num_heads * channel] tensor.
- """
- _, num_heads, length, channel = inputs.get_shape().as_list()
- transposed_inputs = tf.transpose(inputs, [0, 2, 1, 3])
- return tf.reshape(transposed_inputs, [-1, length, num_heads * channel])
-
-
-def reshape_and_transpose_for_attention_operation(inputs, num_heads):
- """Sequentially reshapes and transposes the tensor.
-
- Args:
- inputs: An input [batch, length, num_heads * channel] tensor.
- num_heads: An integer, the number of attention heads.
-
- Returns:
- output: An output [batch, num_heads, length, channel] tensor.
- """
- _, length, channels = inputs.get_shape().as_list()
- inputs = tf.reshape(inputs, [-1, length, num_heads, channels // num_heads])
- return tf.transpose(inputs, [0, 2, 1, 3])
-
-
-def get_layer_name(private_attribute_name):
- if private_attribute_name[0] != '_':
- raise ValueError('Private attribute name should start with a \'_\'.')
- return private_attribute_name[1:]
-
-
-def get_stem_current_name(index):
- return '_basic_block{}'.format(index + 1)
-
-
-def get_low_level_conv_fusion_conv_current_names(index):
- return ('_low_level_conv{}'.format(index + 1),
- '_fusion_conv{}'.format(index + 1))
-
-
-def get_conv_bn_act_current_name(index, use_bn, activation):
- name = '_conv{}'.format(index + 1)
- if use_bn:
- name += '_bn'
- if (activation is not None and
- activation.lower() != 'none' and
- activation.lower() != 'linear'):
- name += '_act'
- return name
-
-
-def safe_setattr(obj, name, value):
- """A conflict-safe version of setattr().
-
- Different from setattr(), this function raises ValueError if the object
- already has an attribute with the same name.
-
- Args:
- obj: An object whose attribute has to be set.
- name: A string, the name of the attribute.
- value: Any type, the value given to the attribute.
-
- Raises:
- ValueError: If the object already has an attribute with the same name.
- """
- if hasattr(obj, name):
- raise ValueError('The object already has an attribute with the same name.')
- setattr(obj, name, value)
-
-
-def pad_sequence_with_none(sequence, target_length):
- return list(sequence) + [None] * (target_length - len(sequence))
-
-
-def strided_downsample(input_tensor, target_size):
- """Strided downsamples a tensor to the target size.
-
- The stride_height and stride_width is computed by (height - 1) //
- (target_height - 1) and (width - 1) // (target_width - 1). We raise an error
- if stride_height != stride_width, since this is not intended in our current
- use cases. But this check can be removed if different strides are desired.
- This function supports static shape only.
-
- Args:
- input_tensor: A [batch, height, width] tf.Tensor to be downsampled.
- target_size: A list of two integers, [target_height, target_width], the
- target size after downsampling.
-
- Returns:
- output_tensor: A [batch, target_height, target_width] tf.Tensor, the
- downsampled result.
-
- Raises:
- ValueError: If the input cannot be downsampled with integer stride, i.e.,
- (height - 1) % (target_height - 1) != 0, or (width - 1) % (target_width -
- 1) != 0.
- ValueError: If the height axis stride does not equal to the width axis
- stride.
- """
- input_height, input_width = input_tensor.get_shape().as_list()[1:3]
- target_height, target_width = target_size
-
- if ((input_height - 1) % (target_height - 1) or
- (input_width - 1) % (target_width - 1)):
- raise ValueError('The input cannot be downsampled with integer striding. '
- 'Please ensure (height - 1) % (target_height - 1) == 0 '
- 'and (width - 1) % (target_width - 1) == 0.')
- stride_height = (input_height - 1) // (target_height - 1)
- stride_width = (input_width - 1) // (target_width - 1)
- if stride_height != stride_width:
- raise ValueError('The height axis stride does not equal to the width axis '
- 'stride.')
- if stride_height > 1 or stride_width > 1:
- return input_tensor[:, ::stride_height, ::stride_width]
- return input_tensor
-
-
-def get_stuff_class_ids(num_thing_stuff_classes: int,
- thing_class_ids: List[int],
- void_label: int) -> List[int]:
- """Computes stuff_class_ids.
-
- The stuff_class_ids are computed from the num_thing_stuff_classes, the
- thing_class_ids and the void_label.
-
- Args:
- num_thing_stuff_classes: An integer specifying the number of stuff and thing
- classes, not including `void` class.
- thing_class_ids: A List of integers of length [num_thing_classes] containing
- thing class indices.
- void_label: An integer specifying the void label.
-
- Returns:
- stuff_class_ids: A sorted List of integers of shape [num_stuff_classes]
- containing stuff class indices.
- """
- if void_label >= num_thing_stuff_classes:
- thing_stuff_class_ids = list(range(num_thing_stuff_classes))
- else:
- thing_stuff_class_ids = [_ for _ in range(num_thing_stuff_classes + 1)
- if _ is not void_label]
- return sorted(set(thing_stuff_class_ids) - set(thing_class_ids))
-
-
-def get_supported_tasks(
- config: config_pb2.ExperimentOptions) -> Set[str]:
- """Gets currently supported tasks for each meta_architecture.
-
- Args:
- config: A config_pb2.ExperimentOptions configuration.
-
- Returns:
- supported_tasks: A set of strings (see common.py), optionally
- - common.TASK_PANOPTIC_SEGMENTATION,
- - common.TASK_INSTANCE_SEGMENTATION,
- - common.TASK_VIDEO_PANOPTIC_SEGMENTATION,
- """
- supported_tasks = set()
- meta_architecture = config.model_options.WhichOneof('meta_architecture')
- is_max_deeplab = meta_architecture == 'max_deeplab'
- is_motion_deeplab = meta_architecture == 'motion_deeplab'
- is_panoptic_deeplab = meta_architecture == 'panoptic_deeplab'
- is_vip_deeplab = meta_architecture == 'vip_deeplab'
- is_panoptic = (
- (config.model_options.panoptic_deeplab.instance.enable and
- is_panoptic_deeplab) or
- is_motion_deeplab or is_max_deeplab or is_vip_deeplab)
- if is_panoptic:
- supported_tasks.add(common.TASK_PANOPTIC_SEGMENTATION)
- # MaX-DeepLab does not support evaluating instance segmentation mask AP yet.
- if not is_max_deeplab:
- supported_tasks.add(common.TASK_INSTANCE_SEGMENTATION)
- if is_motion_deeplab or is_vip_deeplab:
- supported_tasks.add(common.TASK_VIDEO_PANOPTIC_SEGMENTATION)
- if is_vip_deeplab:
- supported_tasks.add(common.TASK_DEPTH_AWARE_VIDEO_PANOPTIC_SEGMENTATION)
- return supported_tasks
diff --git a/spaces/akhaliq/frame-interpolation/app.py b/spaces/akhaliq/frame-interpolation/app.py
deleted file mode 100644
index 9a12e7ea8a1a07e91ccedd5a880cafb0da9e4d1e..0000000000000000000000000000000000000000
--- a/spaces/akhaliq/frame-interpolation/app.py
+++ /dev/null
@@ -1,97 +0,0 @@
-import os
-
-os.system("git clone https://github.com/google-research/frame-interpolation")
-import sys
-
-sys.path.append("frame-interpolation")
-import numpy as np
-import tensorflow as tf
-import mediapy
-from PIL import Image
-from eval import interpolator, util
-import gradio as gr
-
-from huggingface_hub import snapshot_download
-
-from image_tools.sizes import resize_and_crop
-
-
-def load_model(model_name):
- model = interpolator.Interpolator(snapshot_download(repo_id=model_name), None)
-
- return model
-
-
-model_names = [
- "akhaliq/frame-interpolation-film-style",
- "NimaBoscarino/frame-interpolation_film_l1",
- "NimaBoscarino/frame_interpolation_film_vgg",
-]
-
-models = {model_name: load_model(model_name) for model_name in model_names}
-
-ffmpeg_path = util.get_ffmpeg_path()
-mediapy.set_ffmpeg(ffmpeg_path)
-
-
-def resize(width, img):
- basewidth = width
- img = Image.open(img)
- wpercent = (basewidth / float(img.size[0]))
- hsize = int((float(img.size[1]) * float(wpercent)))
- img = img.resize((basewidth, hsize), Image.ANTIALIAS)
- return img
-
-
-def resize_img(img1, img2):
- img_target_size = Image.open(img1)
- img_to_resize = resize_and_crop(
- img2,
- (img_target_size.size[0], img_target_size.size[1]), # set width and height to match img1
- crop_origin="middle"
- )
- img_to_resize.save('resized_img2.png')
-
-
-def predict(frame1, frame2, times_to_interpolate, model_name):
- model = models[model_name]
-
- frame1 = resize(256, frame1)
- frame2 = resize(256, frame2)
-
- frame1.save("test1.png")
- frame2.save("test2.png")
-
- resize_img("test1.png", "test2.png")
- input_frames = ["test1.png", "resized_img2.png"]
-
- frames = list(
- util.interpolate_recursively_from_files(
- input_frames, times_to_interpolate, model))
-
- mediapy.write_video("out.mp4", frames, fps=30)
- return "out.mp4"
-
-
-title = "frame-interpolation"
-description = "Gradio demo for FILM: Frame Interpolation for Large Scene Motion. To use it, simply upload your images and add the times to interpolate number or click on one of the examples to load them. Read more at the links below."
-article = "FILM: Frame Interpolation for Large Motion | Github Repo
"
-examples = [
- ['cat3.jpeg', 'cat4.jpeg', 2, model_names[0]],
- ['cat1.jpeg', 'cat2.jpeg', 2, model_names[1]],
-]
-
-gr.Interface(
- predict,
- [
- gr.inputs.Image(type='filepath'),
- gr.inputs.Image(type='filepath'),
- gr.inputs.Slider(minimum=2, maximum=4, step=1),
- gr.inputs.Dropdown(choices=model_names, default=model_names[0])
- ],
- "playable_video",
- title=title,
- description=description,
- article=article,
- examples=examples
-).launch(enable_queue=True)
diff --git a/spaces/akhaliq/lama/bin/predict_inner_features.py b/spaces/akhaliq/lama/bin/predict_inner_features.py
deleted file mode 100644
index 4f9f7a11a6c4757a4eaa05cf1ac648d372f7e02f..0000000000000000000000000000000000000000
--- a/spaces/akhaliq/lama/bin/predict_inner_features.py
+++ /dev/null
@@ -1,119 +0,0 @@
-#!/usr/bin/env python3
-
-# Example command:
-# ./bin/predict.py \
-# model.path= \
-# indir= \
-# outdir=
-
-import logging
-import os
-import sys
-import traceback
-
-from saicinpainting.evaluation.utils import move_to_device
-
-os.environ['OMP_NUM_THREADS'] = '1'
-os.environ['OPENBLAS_NUM_THREADS'] = '1'
-os.environ['MKL_NUM_THREADS'] = '1'
-os.environ['VECLIB_MAXIMUM_THREADS'] = '1'
-os.environ['NUMEXPR_NUM_THREADS'] = '1'
-
-import cv2
-import hydra
-import numpy as np
-import torch
-import tqdm
-import yaml
-from omegaconf import OmegaConf
-from torch.utils.data._utils.collate import default_collate
-
-from saicinpainting.training.data.datasets import make_default_val_dataset
-from saicinpainting.training.trainers import load_checkpoint, DefaultInpaintingTrainingModule
-from saicinpainting.utils import register_debug_signal_handlers, get_shape
-
-LOGGER = logging.getLogger(__name__)
-
-
-@hydra.main(config_path='../configs/prediction', config_name='default_inner_features.yaml')
-def main(predict_config: OmegaConf):
- try:
- register_debug_signal_handlers() # kill -10 will result in traceback dumped into log
-
- device = torch.device(predict_config.device)
-
- train_config_path = os.path.join(predict_config.model.path, 'config.yaml')
- with open(train_config_path, 'r') as f:
- train_config = OmegaConf.create(yaml.safe_load(f))
-
- checkpoint_path = os.path.join(predict_config.model.path, 'models', predict_config.model.checkpoint)
- model = load_checkpoint(train_config, checkpoint_path, strict=False)
- model.freeze()
- model.to(device)
-
- assert isinstance(model, DefaultInpaintingTrainingModule), 'Only DefaultInpaintingTrainingModule is supported'
- assert isinstance(getattr(model.generator, 'model', None), torch.nn.Sequential)
-
- if not predict_config.indir.endswith('/'):
- predict_config.indir += '/'
-
- dataset = make_default_val_dataset(predict_config.indir, **predict_config.dataset)
-
- max_level = max(predict_config.levels)
-
- with torch.no_grad():
- for img_i in tqdm.trange(len(dataset)):
- mask_fname = dataset.mask_filenames[img_i]
- cur_out_fname = os.path.join(predict_config.outdir, os.path.splitext(mask_fname[len(predict_config.indir):])[0])
- os.makedirs(os.path.dirname(cur_out_fname), exist_ok=True)
-
- batch = move_to_device(default_collate([dataset[img_i]]), device)
-
- img = batch['image']
- mask = batch['mask']
- mask[:] = 0
- mask_h, mask_w = mask.shape[-2:]
- mask[:, :,
- mask_h // 2 - predict_config.hole_radius : mask_h // 2 + predict_config.hole_radius,
- mask_w // 2 - predict_config.hole_radius : mask_w // 2 + predict_config.hole_radius] = 1
-
- masked_img = torch.cat([img * (1 - mask), mask], dim=1)
-
- feats = masked_img
- for level_i, level in enumerate(model.generator.model):
- feats = level(feats)
- if level_i in predict_config.levels:
- cur_feats = torch.cat([f for f in feats if torch.is_tensor(f)], dim=1) \
- if isinstance(feats, tuple) else feats
-
- if predict_config.slice_channels:
- cur_feats = cur_feats[:, slice(*predict_config.slice_channels)]
-
- cur_feat = cur_feats.pow(2).mean(1).pow(0.5).clone()
- cur_feat -= cur_feat.min()
- cur_feat /= cur_feat.std()
- cur_feat = cur_feat.clamp(0, 1) / 1
- cur_feat = cur_feat.cpu().numpy()[0]
- cur_feat *= 255
- cur_feat = np.clip(cur_feat, 0, 255).astype('uint8')
- cv2.imwrite(cur_out_fname + f'_lev{level_i:02d}_norm.png', cur_feat)
-
- # for channel_i in predict_config.channels:
- #
- # cur_feat = cur_feats[0, channel_i].clone().detach().cpu().numpy()
- # cur_feat -= cur_feat.min()
- # cur_feat /= cur_feat.max()
- # cur_feat *= 255
- # cur_feat = np.clip(cur_feat, 0, 255).astype('uint8')
- # cv2.imwrite(cur_out_fname + f'_lev{level_i}_ch{channel_i}.png', cur_feat)
- elif level_i >= max_level:
- break
- except KeyboardInterrupt:
- LOGGER.warning('Interrupted by user')
- except Exception as ex:
- LOGGER.critical(f'Prediction failed due to {ex}:\n{traceback.format_exc()}')
- sys.exit(1)
-
-
-if __name__ == '__main__':
- main()
diff --git a/spaces/akhaliq/lama/models/ade20k/segm_lib/utils/data/dataloader.py b/spaces/akhaliq/lama/models/ade20k/segm_lib/utils/data/dataloader.py
deleted file mode 100644
index 039b9ec3645b2a4626ff47c221e372f32a6ad339..0000000000000000000000000000000000000000
--- a/spaces/akhaliq/lama/models/ade20k/segm_lib/utils/data/dataloader.py
+++ /dev/null
@@ -1,425 +0,0 @@
-import torch
-import torch.multiprocessing as multiprocessing
-from torch._C import _set_worker_signal_handlers, \
- _remove_worker_pids, _error_if_any_worker_fails
-try:
- from torch._C import _set_worker_pids
-except:
- from torch._C import _update_worker_pids as _set_worker_pids
-from .sampler import SequentialSampler, RandomSampler, BatchSampler
-import signal
-import collections
-import re
-import sys
-import threading
-import traceback
-from torch._six import string_classes, int_classes
-import numpy as np
-
-if sys.version_info[0] == 2:
- import Queue as queue
-else:
- import queue
-
-
-class ExceptionWrapper(object):
- r"Wraps an exception plus traceback to communicate across threads"
-
- def __init__(self, exc_info):
- self.exc_type = exc_info[0]
- self.exc_msg = "".join(traceback.format_exception(*exc_info))
-
-
-_use_shared_memory = False
-"""Whether to use shared memory in default_collate"""
-
-
-def _worker_loop(dataset, index_queue, data_queue, collate_fn, seed, init_fn, worker_id):
- global _use_shared_memory
- _use_shared_memory = True
-
- # Intialize C side signal handlers for SIGBUS and SIGSEGV. Python signal
- # module's handlers are executed after Python returns from C low-level
- # handlers, likely when the same fatal signal happened again already.
- # https://docs.python.org/3/library/signal.html Sec. 18.8.1.1
- _set_worker_signal_handlers()
-
- torch.set_num_threads(1)
- torch.manual_seed(seed)
- np.random.seed(seed)
-
- if init_fn is not None:
- init_fn(worker_id)
-
- while True:
- r = index_queue.get()
- if r is None:
- break
- idx, batch_indices = r
- try:
- samples = collate_fn([dataset[i] for i in batch_indices])
- except Exception:
- data_queue.put((idx, ExceptionWrapper(sys.exc_info())))
- else:
- data_queue.put((idx, samples))
-
-
-def _worker_manager_loop(in_queue, out_queue, done_event, pin_memory, device_id):
- if pin_memory:
- torch.cuda.set_device(device_id)
-
- while True:
- try:
- r = in_queue.get()
- except Exception:
- if done_event.is_set():
- return
- raise
- if r is None:
- break
- if isinstance(r[1], ExceptionWrapper):
- out_queue.put(r)
- continue
- idx, batch = r
- try:
- if pin_memory:
- batch = pin_memory_batch(batch)
- except Exception:
- out_queue.put((idx, ExceptionWrapper(sys.exc_info())))
- else:
- out_queue.put((idx, batch))
-
-numpy_type_map = {
- 'float64': torch.DoubleTensor,
- 'float32': torch.FloatTensor,
- 'float16': torch.HalfTensor,
- 'int64': torch.LongTensor,
- 'int32': torch.IntTensor,
- 'int16': torch.ShortTensor,
- 'int8': torch.CharTensor,
- 'uint8': torch.ByteTensor,
-}
-
-
-def default_collate(batch):
- "Puts each data field into a tensor with outer dimension batch size"
-
- error_msg = "batch must contain tensors, numbers, dicts or lists; found {}"
- elem_type = type(batch[0])
- if torch.is_tensor(batch[0]):
- out = None
- if _use_shared_memory:
- # If we're in a background process, concatenate directly into a
- # shared memory tensor to avoid an extra copy
- numel = sum([x.numel() for x in batch])
- storage = batch[0].storage()._new_shared(numel)
- out = batch[0].new(storage)
- return torch.stack(batch, 0, out=out)
- elif elem_type.__module__ == 'numpy' and elem_type.__name__ != 'str_' \
- and elem_type.__name__ != 'string_':
- elem = batch[0]
- if elem_type.__name__ == 'ndarray':
- # array of string classes and object
- if re.search('[SaUO]', elem.dtype.str) is not None:
- raise TypeError(error_msg.format(elem.dtype))
-
- return torch.stack([torch.from_numpy(b) for b in batch], 0)
- if elem.shape == (): # scalars
- py_type = float if elem.dtype.name.startswith('float') else int
- return numpy_type_map[elem.dtype.name](list(map(py_type, batch)))
- elif isinstance(batch[0], int_classes):
- return torch.LongTensor(batch)
- elif isinstance(batch[0], float):
- return torch.DoubleTensor(batch)
- elif isinstance(batch[0], string_classes):
- return batch
- elif isinstance(batch[0], collections.Mapping):
- return {key: default_collate([d[key] for d in batch]) for key in batch[0]}
- elif isinstance(batch[0], collections.Sequence):
- transposed = zip(*batch)
- return [default_collate(samples) for samples in transposed]
-
- raise TypeError((error_msg.format(type(batch[0]))))
-
-
-def pin_memory_batch(batch):
- if torch.is_tensor(batch):
- return batch.pin_memory()
- elif isinstance(batch, string_classes):
- return batch
- elif isinstance(batch, collections.Mapping):
- return {k: pin_memory_batch(sample) for k, sample in batch.items()}
- elif isinstance(batch, collections.Sequence):
- return [pin_memory_batch(sample) for sample in batch]
- else:
- return batch
-
-
-_SIGCHLD_handler_set = False
-"""Whether SIGCHLD handler is set for DataLoader worker failures. Only one
-handler needs to be set for all DataLoaders in a process."""
-
-
-def _set_SIGCHLD_handler():
- # Windows doesn't support SIGCHLD handler
- if sys.platform == 'win32':
- return
- # can't set signal in child threads
- if not isinstance(threading.current_thread(), threading._MainThread):
- return
- global _SIGCHLD_handler_set
- if _SIGCHLD_handler_set:
- return
- previous_handler = signal.getsignal(signal.SIGCHLD)
- if not callable(previous_handler):
- previous_handler = None
-
- def handler(signum, frame):
- # This following call uses `waitid` with WNOHANG from C side. Therefore,
- # Python can still get and update the process status successfully.
- _error_if_any_worker_fails()
- if previous_handler is not None:
- previous_handler(signum, frame)
-
- signal.signal(signal.SIGCHLD, handler)
- _SIGCHLD_handler_set = True
-
-
-class DataLoaderIter(object):
- "Iterates once over the DataLoader's dataset, as specified by the sampler"
-
- def __init__(self, loader):
- self.dataset = loader.dataset
- self.collate_fn = loader.collate_fn
- self.batch_sampler = loader.batch_sampler
- self.num_workers = loader.num_workers
- self.pin_memory = loader.pin_memory and torch.cuda.is_available()
- self.timeout = loader.timeout
- self.done_event = threading.Event()
-
- self.sample_iter = iter(self.batch_sampler)
-
- if self.num_workers > 0:
- self.worker_init_fn = loader.worker_init_fn
- self.index_queue = multiprocessing.SimpleQueue()
- self.worker_result_queue = multiprocessing.SimpleQueue()
- self.batches_outstanding = 0
- self.worker_pids_set = False
- self.shutdown = False
- self.send_idx = 0
- self.rcvd_idx = 0
- self.reorder_dict = {}
-
- base_seed = torch.LongTensor(1).random_(0, 2**31-1)[0]
- self.workers = [
- multiprocessing.Process(
- target=_worker_loop,
- args=(self.dataset, self.index_queue, self.worker_result_queue, self.collate_fn,
- base_seed + i, self.worker_init_fn, i))
- for i in range(self.num_workers)]
-
- if self.pin_memory or self.timeout > 0:
- self.data_queue = queue.Queue()
- if self.pin_memory:
- maybe_device_id = torch.cuda.current_device()
- else:
- # do not initialize cuda context if not necessary
- maybe_device_id = None
- self.worker_manager_thread = threading.Thread(
- target=_worker_manager_loop,
- args=(self.worker_result_queue, self.data_queue, self.done_event, self.pin_memory,
- maybe_device_id))
- self.worker_manager_thread.daemon = True
- self.worker_manager_thread.start()
- else:
- self.data_queue = self.worker_result_queue
-
- for w in self.workers:
- w.daemon = True # ensure that the worker exits on process exit
- w.start()
-
- _set_worker_pids(id(self), tuple(w.pid for w in self.workers))
- _set_SIGCHLD_handler()
- self.worker_pids_set = True
-
- # prime the prefetch loop
- for _ in range(2 * self.num_workers):
- self._put_indices()
-
- def __len__(self):
- return len(self.batch_sampler)
-
- def _get_batch(self):
- if self.timeout > 0:
- try:
- return self.data_queue.get(timeout=self.timeout)
- except queue.Empty:
- raise RuntimeError('DataLoader timed out after {} seconds'.format(self.timeout))
- else:
- return self.data_queue.get()
-
- def __next__(self):
- if self.num_workers == 0: # same-process loading
- indices = next(self.sample_iter) # may raise StopIteration
- batch = self.collate_fn([self.dataset[i] for i in indices])
- if self.pin_memory:
- batch = pin_memory_batch(batch)
- return batch
-
- # check if the next sample has already been generated
- if self.rcvd_idx in self.reorder_dict:
- batch = self.reorder_dict.pop(self.rcvd_idx)
- return self._process_next_batch(batch)
-
- if self.batches_outstanding == 0:
- self._shutdown_workers()
- raise StopIteration
-
- while True:
- assert (not self.shutdown and self.batches_outstanding > 0)
- idx, batch = self._get_batch()
- self.batches_outstanding -= 1
- if idx != self.rcvd_idx:
- # store out-of-order samples
- self.reorder_dict[idx] = batch
- continue
- return self._process_next_batch(batch)
-
- next = __next__ # Python 2 compatibility
-
- def __iter__(self):
- return self
-
- def _put_indices(self):
- assert self.batches_outstanding < 2 * self.num_workers
- indices = next(self.sample_iter, None)
- if indices is None:
- return
- self.index_queue.put((self.send_idx, indices))
- self.batches_outstanding += 1
- self.send_idx += 1
-
- def _process_next_batch(self, batch):
- self.rcvd_idx += 1
- self._put_indices()
- if isinstance(batch, ExceptionWrapper):
- raise batch.exc_type(batch.exc_msg)
- return batch
-
- def __getstate__(self):
- # TODO: add limited pickling support for sharing an iterator
- # across multiple threads for HOGWILD.
- # Probably the best way to do this is by moving the sample pushing
- # to a separate thread and then just sharing the data queue
- # but signalling the end is tricky without a non-blocking API
- raise NotImplementedError("DataLoaderIterator cannot be pickled")
-
- def _shutdown_workers(self):
- try:
- if not self.shutdown:
- self.shutdown = True
- self.done_event.set()
- # if worker_manager_thread is waiting to put
- while not self.data_queue.empty():
- self.data_queue.get()
- for _ in self.workers:
- self.index_queue.put(None)
- # done_event should be sufficient to exit worker_manager_thread,
- # but be safe here and put another None
- self.worker_result_queue.put(None)
- finally:
- # removes pids no matter what
- if self.worker_pids_set:
- _remove_worker_pids(id(self))
- self.worker_pids_set = False
-
- def __del__(self):
- if self.num_workers > 0:
- self._shutdown_workers()
-
-
-class DataLoader(object):
- """
- Data loader. Combines a dataset and a sampler, and provides
- single- or multi-process iterators over the dataset.
-
- Arguments:
- dataset (Dataset): dataset from which to load the data.
- batch_size (int, optional): how many samples per batch to load
- (default: 1).
- shuffle (bool, optional): set to ``True`` to have the data reshuffled
- at every epoch (default: False).
- sampler (Sampler, optional): defines the strategy to draw samples from
- the dataset. If specified, ``shuffle`` must be False.
- batch_sampler (Sampler, optional): like sampler, but returns a batch of
- indices at a time. Mutually exclusive with batch_size, shuffle,
- sampler, and drop_last.
- num_workers (int, optional): how many subprocesses to use for data
- loading. 0 means that the data will be loaded in the main process.
- (default: 0)
- collate_fn (callable, optional): merges a list of samples to form a mini-batch.
- pin_memory (bool, optional): If ``True``, the data loader will copy tensors
- into CUDA pinned memory before returning them.
- drop_last (bool, optional): set to ``True`` to drop the last incomplete batch,
- if the dataset size is not divisible by the batch size. If ``False`` and
- the size of dataset is not divisible by the batch size, then the last batch
- will be smaller. (default: False)
- timeout (numeric, optional): if positive, the timeout value for collecting a batch
- from workers. Should always be non-negative. (default: 0)
- worker_init_fn (callable, optional): If not None, this will be called on each
- worker subprocess with the worker id (an int in ``[0, num_workers - 1]``) as
- input, after seeding and before data loading. (default: None)
-
- .. note:: By default, each worker will have its PyTorch seed set to
- ``base_seed + worker_id``, where ``base_seed`` is a long generated
- by main process using its RNG. You may use ``torch.initial_seed()`` to access
- this value in :attr:`worker_init_fn`, which can be used to set other seeds
- (e.g. NumPy) before data loading.
-
- .. warning:: If ``spawn'' start method is used, :attr:`worker_init_fn` cannot be an
- unpicklable object, e.g., a lambda function.
- """
-
- def __init__(self, dataset, batch_size=1, shuffle=False, sampler=None, batch_sampler=None,
- num_workers=0, collate_fn=default_collate, pin_memory=False, drop_last=False,
- timeout=0, worker_init_fn=None):
- self.dataset = dataset
- self.batch_size = batch_size
- self.num_workers = num_workers
- self.collate_fn = collate_fn
- self.pin_memory = pin_memory
- self.drop_last = drop_last
- self.timeout = timeout
- self.worker_init_fn = worker_init_fn
-
- if timeout < 0:
- raise ValueError('timeout option should be non-negative')
-
- if batch_sampler is not None:
- if batch_size > 1 or shuffle or sampler is not None or drop_last:
- raise ValueError('batch_sampler is mutually exclusive with '
- 'batch_size, shuffle, sampler, and drop_last')
-
- if sampler is not None and shuffle:
- raise ValueError('sampler is mutually exclusive with shuffle')
-
- if self.num_workers < 0:
- raise ValueError('num_workers cannot be negative; '
- 'use num_workers=0 to disable multiprocessing.')
-
- if batch_sampler is None:
- if sampler is None:
- if shuffle:
- sampler = RandomSampler(dataset)
- else:
- sampler = SequentialSampler(dataset)
- batch_sampler = BatchSampler(sampler, batch_size, drop_last)
-
- self.sampler = sampler
- self.batch_sampler = batch_sampler
-
- def __iter__(self):
- return DataLoaderIter(self)
-
- def __len__(self):
- return len(self.batch_sampler)
diff --git a/spaces/aliabd/Anime2Sketch/app.py b/spaces/aliabd/Anime2Sketch/app.py
deleted file mode 100644
index 91ba257902e995bbecf44fff4e0dbcfeea384a7d..0000000000000000000000000000000000000000
--- a/spaces/aliabd/Anime2Sketch/app.py
+++ /dev/null
@@ -1,41 +0,0 @@
-import os
-import random
-from data import get_image_list
-from model import create_model
-from data import read_img_path, tensor_to_img, save_image
-import gradio as gr
-import torchtext
-from PIL import Image
-import torch
-
-torch.hub.download_url_to_file('https://upload.wikimedia.org/wikipedia/commons/thumb/a/a5/Tsunami_by_hokusai_19th_century.jpg/1920px-Tsunami_by_hokusai_19th_century.jpg', 'wave.jpg')
-torch.hub.download_url_to_file('https://cdn.pixabay.com/photo/2020/10/02/13/49/bridge-5621201_1280.jpg', 'building.jpg')
-
-torchtext.utils.download_from_url("https://drive.google.com/uc?id=1RILKwUdjjBBngB17JHwhZNBEaW4Mr-Ml", root="./weights/")
-gpu_ids=[]
-model = create_model(gpu_ids)
-
-def sketch2anime(img, load_size=512):
- img, aus_resize = read_img_path(img.name, load_size)
- aus_tensor = model(img)
- aus_img = tensor_to_img(aus_tensor)
- image_pil = Image.fromarray(aus_img)
- image_pil = image_pil.resize(aus_resize, Image.BICUBIC)
- return image_pil
-
-
-title = "Anime2Sketch"
-description = "A sketch extractor for illustration, anime art and manga. Read more at the links below."
-article = "Adversarial Open Domain Adaption for Sketch-to-Photo Synthesis | Github Repo
"
-
-gr.Interface(
- sketch2anime,
- [gr.inputs.Image(type="file", label="Input")],
- gr.outputs.Image(type="pil", label="Output"),
- title=title,
- description=description,
- article=article,
- examples=[
- ["building.jpg"],
- ["wave.jpg"]
- ]).launch(debug=True)
diff --git a/spaces/allknowingroger/Image-Models-Test11/app.py b/spaces/allknowingroger/Image-Models-Test11/app.py
deleted file mode 100644
index 00819f049f3e8fcd38b83c6d0b42e243a93cc3d7..0000000000000000000000000000000000000000
--- a/spaces/allknowingroger/Image-Models-Test11/app.py
+++ /dev/null
@@ -1,144 +0,0 @@
-import gradio as gr
-# import os
-# import sys
-# from pathlib import Path
-import time
-
-models =[
- "Interfan/abraham",
- "Alexzyx/lora-trained-xl-colab-v3",
- "WALIDALI/joejordly",
- "gfalcao/ldsc26jun-nocrop",
- "digiplay/ShowmakerMix_v1",
- "digiplay/VersaMix_base_diffusers",
- "digiplay/AbsoluteReality_v1.0_diffusers",
- "digiplay/2.5DSET_new1a25d_FFver",
- "digiplay/DiamondCoalMix_v2_pruned_diffusers",
-]
-
-
-model_functions = {}
-model_idx = 1
-for model_path in models:
- try:
- model_functions[model_idx] = gr.Interface.load(f"models/{model_path}", live=False, preprocess=True, postprocess=False)
- except Exception as error:
- def the_fn(txt):
- return None
- model_functions[model_idx] = gr.Interface(fn=the_fn, inputs=["text"], outputs=["image"])
- model_idx+=1
-
-
-def send_it_idx(idx):
- def send_it_fn(prompt):
- output = (model_functions.get(str(idx)) or model_functions.get(str(1)))(prompt)
- return output
- return send_it_fn
-
-def get_prompts(prompt_text):
- return prompt_text
-
-def clear_it(val):
- if int(val) != 0:
- val = 0
- else:
- val = 0
- pass
- return val
-
-def all_task_end(cnt,t_stamp):
- to = t_stamp + 60
- et = time.time()
- if et > to and t_stamp != 0:
- d = gr.update(value=0)
- tog = gr.update(value=1)
- #print(f'to: {to} et: {et}')
- else:
- if cnt != 0:
- d = gr.update(value=et)
- else:
- d = gr.update(value=0)
- tog = gr.update(value=0)
- #print (f'passing: to: {to} et: {et}')
- pass
- return d, tog
-
-def all_task_start():
- print("\n\n\n\n\n\n\n")
- t = time.gmtime()
- t_stamp = time.time()
- current_time = time.strftime("%H:%M:%S", t)
- return gr.update(value=t_stamp), gr.update(value=t_stamp), gr.update(value=0)
-
-def clear_fn():
- nn = len(models)
- return tuple([None, *[None for _ in range(nn)]])
-
-
-
-with gr.Blocks(title="SD Models") as my_interface:
- with gr.Column(scale=12):
- # with gr.Row():
- # gr.Markdown("""- Primary prompt: 你想画的内容(英文单词,如 a cat, 加英文逗号效果更好;点 Improve 按钮进行完善)\n- Real prompt: 完善后的提示词,出现后再点右边的 Run 按钮开始运行""")
- with gr.Row():
- with gr.Row(scale=6):
- primary_prompt=gr.Textbox(label="Prompt", value="")
- # real_prompt=gr.Textbox(label="Real prompt")
- with gr.Row(scale=6):
- # improve_prompts_btn=gr.Button("Improve")
- with gr.Row():
- run=gr.Button("Run",variant="primary")
- clear_btn=gr.Button("Clear")
- with gr.Row():
- sd_outputs = {}
- model_idx = 1
- for model_path in models:
- with gr.Column(scale=3, min_width=320):
- with gr.Box():
- sd_outputs[model_idx] = gr.Image(label=model_path)
- pass
- model_idx += 1
- pass
- pass
-
- with gr.Row(visible=False):
- start_box=gr.Number(interactive=False)
- end_box=gr.Number(interactive=False)
- tog_box=gr.Textbox(value=0,interactive=False)
-
- start_box.change(
- all_task_end,
- [start_box, end_box],
- [start_box, tog_box],
- every=1,
- show_progress=False)
-
- primary_prompt.submit(all_task_start, None, [start_box, end_box, tog_box])
- run.click(all_task_start, None, [start_box, end_box, tog_box])
- runs_dict = {}
- model_idx = 1
- for model_path in models:
- runs_dict[model_idx] = run.click(model_functions[model_idx], inputs=[primary_prompt], outputs=[sd_outputs[model_idx]])
- model_idx += 1
- pass
- pass
-
- # improve_prompts_btn_clicked=improve_prompts_btn.click(
- # get_prompts,
- # inputs=[primary_prompt],
- # outputs=[primary_prompt],
- # cancels=list(runs_dict.values()))
- clear_btn.click(
- clear_fn,
- None,
- [primary_prompt, *list(sd_outputs.values())],
- cancels=[*list(runs_dict.values())])
- tog_box.change(
- clear_it,
- tog_box,
- tog_box,
- cancels=[*list(runs_dict.values())])
-
-my_interface.queue(concurrency_count=600, status_update_rate=1)
-my_interface.launch(inline=True, show_api=False)
-
\ No newline at end of file
diff --git a/spaces/anpigon/talktosayno/app.py b/spaces/anpigon/talktosayno/app.py
deleted file mode 100644
index 746507356e3cc1a8946b274a4a93a1a0fb2f63d2..0000000000000000000000000000000000000000
--- a/spaces/anpigon/talktosayno/app.py
+++ /dev/null
@@ -1,91 +0,0 @@
-import gradio as gr
-import chromadb
-from langchain import PromptTemplate
-from langchain.chains import RetrievalQAWithSourcesChain
-from langchain.chat_models import ChatOpenAI
-from langchain.embeddings.openai import OpenAIEmbeddings
-from langchain.prompts.chat import (
- ChatPromptTemplate,
- SystemMessagePromptTemplate,
-)
-from langchain.vectorstores import Chroma
-
-from constants import persist_directory
-
-embedding = OpenAIEmbeddings()
-client = chromadb.PersistentClient(path=persist_directory)
-vector_store = Chroma(client=client, embedding_function=embedding)
-retriever = vector_store.as_retriever(search_kwargs={"k": 10})
-
-llm = ChatOpenAI(model_name="gpt-3.5-turbo-16k", temperature=0)
-# llm = ChatOpenAI(model_name="gpt-3.5-turbo", temperature=0)
-
-system_template = """Your name is Sayno. You act like a successful rich man. You should answer in the tone of a long document. Never reveal that it is an AI.
-Given the following extracted parts of a long document and a question, create a final answer with references ("SOURCES").
-If you don't know the answer, just say that you don't know. Don't try to make up an answer.
-!IMPORTANT: ALWAYS return a "SOURCES" part in your answer.
-!IMPORTANT: Be sure to answer in Korean.
-
-[EXAMPLE]
-QUESTION: Which state/country's law governs the interpretation of the contract?
-=========
-Content: This Agreement is governed by English law and the parties submit to the exclusive jurisdiction of the English courts in relation to any dispute (contractual or non-contractual) concerning this Agreement save that either party may apply to any court for an injunction or other relief to protect its Intellectual Property Rights.
-Source: 28-pl
-Content: No Waiver. Failure or delay in exercising any right or remedy under this Agreement shall not constitute a waiver of such (or any other) right or remedy.\n\n11.7 Severability. The invalidity, illegality or unenforceability of any term (or part of a term) of this Agreement shall not affect the continuation in force of the remainder of the term (if any) and this Agreement.\n\n11.8 No Agency. Except as expressly stated otherwise, nothing in this Agreement shall create an agency, partnership or joint venture of any kind between the parties.\n\n11.9 No Third-Party Beneficiaries.
-Source: 30-pl
-Content: (b) if Google believes, in good faith, that the Distributor has violated or caused Google to violate any Anti-Bribery Laws (as defined in Clause 8.5) or that such a violation is reasonably likely to occur,
-Source: 4-pl
-=========
-FINAL ANSWER: This Agreement is governed by English law.
-SOURCES: 28-pl
-
-QUESTION: {question}
-=========
-{summaries}
-=========
-FINAL ANSWER:
-"""
-
-prompt = ChatPromptTemplate.from_messages(
- [
- SystemMessagePromptTemplate.from_template(system_template),
- ]
-)
-
-document_prompt = PromptTemplate(
- template="Content: {page_content}\nSource: {source}, {page} page",
- input_variables=["page_content", "source", "page"],
-)
-
-chain_type_kwargs = {"prompt": prompt, "document_prompt": document_prompt}
-
-chain = RetrievalQAWithSourcesChain.from_chain_type(
- llm=llm,
- chain_type="stuff",
- retriever=retriever,
- return_source_documents=True,
- chain_type_kwargs=chain_type_kwargs,
- reduce_k_below_max_tokens=True,
- verbose=False,
-)
-
-
-def respond(message, chat_history):
- result = chain(message)
- print(result)
- bot_message = f'{result["answer"]} - 출처: {result["sources"]}'
- chat_history.append((message, bot_message))
- return "", chat_history
-
-
-with gr.Blocks(theme=gr.themes.Soft()) as demo:
- gr.Markdown("# 안녕하세요. 세이노와 대화해보세요.")
- initial_greeting = "안녕하세요!\n저는 세이노처럼 경험과 지식을 갖춘 인공지능 ChatGPT입니다. 세이노는 사업, 경영, 투자에 대한 전문가이며, 많은 사람들이 그의 조언을 참고하고 있습니다. 어떤 도움이 필요하신가요? 세이노와 관련된 질문이 있으시면 편안하게 물어보세요!"
- chatbot = gr.Chatbot(label="채팅창", value=[(None, initial_greeting)])
- msg = gr.Textbox(label="입력")
- clear = gr.Button("초기화")
-
- msg.submit(respond, [msg, chatbot], [msg, chatbot])
- clear.click(lambda: None, None, chatbot, queue=False)
-
-demo.launch(debug=False)
diff --git a/spaces/antonovmaxim/text-generation-webui-space/text-generation-webui-main/docs/DeepSpeed.md b/spaces/antonovmaxim/text-generation-webui-space/text-generation-webui-main/docs/DeepSpeed.md
deleted file mode 100644
index 70cd81519a6954ebc7cdaf82e03a169bed878106..0000000000000000000000000000000000000000
--- a/spaces/antonovmaxim/text-generation-webui-space/text-generation-webui-main/docs/DeepSpeed.md
+++ /dev/null
@@ -1,23 +0,0 @@
-An alternative way of reducing the GPU memory usage of models is to use the `DeepSpeed ZeRO-3` optimization.
-
-With this, I have been able to load a 6b model (GPT-J 6B) with less than 6GB of VRAM. The speed of text generation is very decent and much better than what would be accomplished with `--auto-devices --gpu-memory 6`.
-
-As far as I know, DeepSpeed is only available for Linux at the moment.
-
-### How to use it
-
-1. Install DeepSpeed:
-
-```
-pip install deepspeed
-```
-
-2. Start the web UI replacing `python` with `deepspeed --num_gpus=1` and adding the `--deepspeed` flag. Example:
-
-```
-deepspeed --num_gpus=1 server.py --deepspeed --chat --model gpt-j-6B
-```
-
-### Learn more
-
-For more information, check out [this comment](https://github.com/oobabooga/text-generation-webui/issues/40#issuecomment-1412038622) by 81300, who came up with the DeepSpeed support in this web UI.
\ No newline at end of file
diff --git a/spaces/apsys/hetfit/nets/deep_dense.py b/spaces/apsys/hetfit/nets/deep_dense.py
deleted file mode 100644
index 14ca122f73f1a191c0e3ad902b1e312985b7242a..0000000000000000000000000000000000000000
--- a/spaces/apsys/hetfit/nets/deep_dense.py
+++ /dev/null
@@ -1,32 +0,0 @@
-from torch import nn
-from torch.functional import F
-
-class dmodel(nn.Module):
- """4 layers Torch model. Relu activations, hidden layers are same size.
-
- """
- def __init__(self, in_features=1, hidden_features=200, out_features=1):
- """Init
-
- Args:
- in_features (int, optional): Input features. Defaults to 1.
- hidden_features (int, optional): Hidden dims. Defaults to 200.
- out_features (int, optional): Output dims. Defaults to 1.
- """
- super(dmodel, self).__init__()
-
- self.fc1 = nn.Linear(in_features, hidden_features)
- self.fc2 = nn.Linear(hidden_features, hidden_features)
- self.fc3 = nn.Linear(hidden_features, hidden_features)
- self.fc4 = nn.Linear(hidden_features, out_features)
-
-
- def forward(self, x):
- x = self.fc1(x)
- x = F.relu(x) # ReLU activation
- x = self.fc2(x)
- x = F.relu(x) # ReLU activation
- x = self.fc3(x)
- x = F.relu(x) # ReLU activation
- x = self.fc4(x)
- return x
\ No newline at end of file
diff --git a/spaces/arikru/packstation-inspector/app.py b/spaces/arikru/packstation-inspector/app.py
deleted file mode 100644
index 0eb11b29ce5da7a1a1e8934bda724c06bcf5bc02..0000000000000000000000000000000000000000
--- a/spaces/arikru/packstation-inspector/app.py
+++ /dev/null
@@ -1,30 +0,0 @@
-# AUTOGENERATED! DO NOT EDIT! File to edit: app.ipynb.
-
-# %% auto 0
-__all__ = ['learn', 'categories', 'im', 'image', 'label', 'examples', 'intf', 'classify_image']
-
-# %% app.ipynb 1
-from fastai.vision.all import *
-import gradio as gr
-
-# %% app.ipynb 2
-learn = load_learner('export.pkl')
-
-# %% app.ipynb 7
-categories = ('dreckig', 'sauber')
-im = PILImage.create('packstation_sauber.jpg')
-im.thumbnail((192,192))
-im
-
-learn.predict(im)
-def classify_image(img):
- prod,idx,probs = learn.predict(img)
- return dict(zip(categories, map(float,probs)))
-
-# %% app.ipynb 9
-image = gr.inputs.Image(shape=(192,192))
-label = gr.outputs.Label()
-examples = ['packstation_sauber.jpg', 'packstation_dreckig.jpg']
-
-intf = gr.Interface(fn=classify_image, inputs=image, outputs=label, examples=examples)
-intf.launch(inline=False)
diff --git a/spaces/artificialguybr/freedom/README.md b/spaces/artificialguybr/freedom/README.md
deleted file mode 100644
index 873e7e62d36ccd8fbb8c52187dfea4bf94ee2b6f..0000000000000000000000000000000000000000
--- a/spaces/artificialguybr/freedom/README.md
+++ /dev/null
@@ -1,12 +0,0 @@
----
-title: Freedom
-emoji: 🌖
-colorFrom: gray
-colorTo: indigo
-sdk: gradio
-sdk_version: 3.33.1
-app_file: app.py
-pinned: false
----
-
-Check out the configuration reference at https://huggingface.co/docs/hub/spaces-config-reference
diff --git a/spaces/artificialguybr/video-dubbing/TTS/TTS/bin/resample.py b/spaces/artificialguybr/video-dubbing/TTS/TTS/bin/resample.py
deleted file mode 100644
index a3f28485d1fb235ab0d521ee30318c64b48fbd5a..0000000000000000000000000000000000000000
--- a/spaces/artificialguybr/video-dubbing/TTS/TTS/bin/resample.py
+++ /dev/null
@@ -1,90 +0,0 @@
-import argparse
-import glob
-import os
-from argparse import RawTextHelpFormatter
-from multiprocessing import Pool
-from shutil import copytree
-
-import librosa
-import soundfile as sf
-from tqdm import tqdm
-
-
-def resample_file(func_args):
- filename, output_sr = func_args
- y, sr = librosa.load(filename, sr=output_sr)
- sf.write(filename, y, sr)
-
-
-def resample_files(input_dir, output_sr, output_dir=None, file_ext="wav", n_jobs=10):
- if output_dir:
- print("Recursively copying the input folder...")
- copytree(input_dir, output_dir)
- input_dir = output_dir
-
- print("Resampling the audio files...")
- audio_files = glob.glob(os.path.join(input_dir, f"**/*.{file_ext}"), recursive=True)
- print(f"Found {len(audio_files)} files...")
- audio_files = list(zip(audio_files, len(audio_files) * [output_sr]))
- with Pool(processes=n_jobs) as p:
- with tqdm(total=len(audio_files)) as pbar:
- for _, _ in enumerate(p.imap_unordered(resample_file, audio_files)):
- pbar.update()
-
- print("Done !")
-
-
-if __name__ == "__main__":
- parser = argparse.ArgumentParser(
- description="""Resample a folder recusively with librosa
- Can be used in place or create a copy of the folder as an output.\n\n
- Example run:
- python TTS/bin/resample.py
- --input_dir /root/LJSpeech-1.1/
- --output_sr 22050
- --output_dir /root/resampled_LJSpeech-1.1/
- --file_ext wav
- --n_jobs 24
- """,
- formatter_class=RawTextHelpFormatter,
- )
-
- parser.add_argument(
- "--input_dir",
- type=str,
- default=None,
- required=True,
- help="Path of the folder containing the audio files to resample",
- )
-
- parser.add_argument(
- "--output_sr",
- type=int,
- default=22050,
- required=False,
- help="Samlple rate to which the audio files should be resampled",
- )
-
- parser.add_argument(
- "--output_dir",
- type=str,
- default=None,
- required=False,
- help="Path of the destination folder. If not defined, the operation is done in place",
- )
-
- parser.add_argument(
- "--file_ext",
- type=str,
- default="wav",
- required=False,
- help="Extension of the audio files to resample",
- )
-
- parser.add_argument(
- "--n_jobs", type=int, default=None, help="Number of threads to use, by default it uses all cores"
- )
-
- args = parser.parse_args()
-
- resample_files(args.input_dir, args.output_sr, args.output_dir, args.file_ext, args.n_jobs)
diff --git a/spaces/artificialguybr/video-dubbing/TTS/hubconf.py b/spaces/artificialguybr/video-dubbing/TTS/hubconf.py
deleted file mode 100644
index 0c9c5930fcbf98962d3086e7537aa3941b191083..0000000000000000000000000000000000000000
--- a/spaces/artificialguybr/video-dubbing/TTS/hubconf.py
+++ /dev/null
@@ -1,46 +0,0 @@
-dependencies = [
- 'torch', 'gdown', 'pysbd', 'gruut', 'anyascii', 'pypinyin', 'coqpit', 'mecab-python3', 'unidic-lite'
-]
-import torch
-
-from TTS.utils.manage import ModelManager
-from TTS.utils.synthesizer import Synthesizer
-
-
-def tts(model_name='tts_models/en/ljspeech/tacotron2-DCA',
- vocoder_name=None,
- use_cuda=False):
- """TTS entry point for PyTorch Hub that provides a Synthesizer object to synthesize speech from a give text.
-
- Example:
- >>> synthesizer = torch.hub.load('coqui-ai/TTS', 'tts', source='github')
- >>> wavs = synthesizer.tts("This is a test! This is also a test!!")
- wavs - is a list of values of the synthesized speech.
-
- Args:
- model_name (str, optional): One of the model names from .model.json. Defaults to 'tts_models/en/ljspeech/tacotron2-DCA'.
- vocoder_name (str, optional): One of the model names from .model.json. Defaults to 'vocoder_models/en/ljspeech/multiband-melgan'.
- pretrained (bool, optional): [description]. Defaults to True.
-
- Returns:
- TTS.utils.synthesizer.Synthesizer: Synthesizer object wrapping both vocoder and tts models.
- """
- manager = ModelManager()
-
- model_path, config_path, model_item = manager.download_model(model_name)
- vocoder_name = model_item[
- 'default_vocoder'] if vocoder_name is None else vocoder_name
- vocoder_path, vocoder_config_path, _ = manager.download_model(vocoder_name)
-
- # create synthesizer
- synt = Synthesizer(tts_checkpoint=model_path,
- tts_config_path=config_path,
- vocoder_checkpoint=vocoder_path,
- vocoder_config=vocoder_config_path,
- use_cuda=use_cuda)
- return synt
-
-
-if __name__ == '__main__':
- synthesizer = torch.hub.load('coqui-ai/TTS:dev', 'tts', source='github')
- synthesizer.tts("This is a test!")
diff --git a/spaces/arxify/RVC-beta-v2-0618/runtime/Lib/site-packages/altair/vegalite/v3/tests/test_renderers.py b/spaces/arxify/RVC-beta-v2-0618/runtime/Lib/site-packages/altair/vegalite/v3/tests/test_renderers.py
deleted file mode 100644
index ba8b4c9855a36774ca88a1027b12c9910213b67d..0000000000000000000000000000000000000000
--- a/spaces/arxify/RVC-beta-v2-0618/runtime/Lib/site-packages/altair/vegalite/v3/tests/test_renderers.py
+++ /dev/null
@@ -1,86 +0,0 @@
-"""Tests of various renderers"""
-
-import json
-import re
-
-import pytest
-
-import altair.vegalite.v3 as alt
-
-
-def _extract_embedOpt(html):
- """Extract an embedOpt definition from an html string.
-
- Note: this is very brittle, but works for the specific test in this file.
- """
- result = re.search(r"embedOpt\s+=\s+(?P\{.*?\})", html)
- if not result:
- return None
- else:
- return json.loads(result.groupdict()["embedOpt"])
-
-
-@pytest.fixture
-def chart():
- return alt.Chart("data.csv").mark_point()
-
-
-def test_colab_renderer_embed_options(chart):
- """Test that embed_options in renderer metadata are correctly manifest in html"""
-
- def assert_actions_true(chart):
- bundle = chart._repr_mimebundle_(None, None)
- embedOpt = _extract_embedOpt(bundle["text/html"])
- assert embedOpt == {"actions": True, "mode": "vega-lite"}
-
- def assert_actions_false(chart):
- bundle = chart._repr_mimebundle_(None, None)
- embedOpt = _extract_embedOpt(bundle["text/html"])
- assert embedOpt == {"actions": False, "mode": "vega-lite"}
-
- with alt.renderers.enable("colab", embed_options=dict(actions=False)):
- assert_actions_false(chart)
-
- with alt.renderers.enable("colab"):
- with alt.renderers.enable(embed_options=dict(actions=True)):
- assert_actions_true(chart)
-
- with alt.renderers.set_embed_options(actions=False):
- assert_actions_false(chart)
-
- with alt.renderers.set_embed_options(actions=True):
- assert_actions_true(chart)
-
-
-def test_default_renderer_embed_options(chart, renderer="default"):
- # check that metadata is passed appropriately
- mimetype = alt.display.VEGALITE_MIME_TYPE
- spec = chart.to_dict()
- with alt.renderers.enable(renderer, embed_options=dict(actions=False)):
- bundle, metadata = chart._repr_mimebundle_(None, None)
- assert set(bundle.keys()) == {mimetype, "text/plain"}
- assert bundle[mimetype] == spec
- assert metadata == {mimetype: {"embed_options": {"actions": False}}}
-
- # Sanity check: no metadata specified
- with alt.renderers.enable(renderer):
- bundle, metadata = chart._repr_mimebundle_(None, None)
- assert bundle[mimetype] == spec
- assert metadata == {}
-
-
-def test_json_renderer_embed_options(chart, renderer="json"):
- """Test that embed_options in renderer metadata are correctly manifest in html"""
- mimetype = "application/json"
- spec = chart.to_dict()
- with alt.renderers.enable("json", option="foo"):
- bundle, metadata = chart._repr_mimebundle_(None, None)
- assert set(bundle.keys()) == {mimetype, "text/plain"}
- assert bundle[mimetype] == spec
- assert metadata == {mimetype: {"option": "foo"}}
-
- # Sanity check: no options specified
- with alt.renderers.enable(renderer):
- bundle, metadata = chart._repr_mimebundle_(None, None)
- assert bundle[mimetype] == spec
- assert metadata == {}
diff --git a/spaces/arxify/RVC-beta-v2-0618/runtime/Lib/site-packages/fairseq/benchmark/dummy_model.py b/spaces/arxify/RVC-beta-v2-0618/runtime/Lib/site-packages/fairseq/benchmark/dummy_model.py
deleted file mode 100644
index ff26e4fe655d8e8d7f9942c4bd3df7cd267405fb..0000000000000000000000000000000000000000
--- a/spaces/arxify/RVC-beta-v2-0618/runtime/Lib/site-packages/fairseq/benchmark/dummy_model.py
+++ /dev/null
@@ -1,96 +0,0 @@
-# Copyright (c) Facebook, Inc. and its affiliates.
-#
-# This source code is licensed under the MIT license found in the
-# LICENSE file in the root directory of this source tree.
-
-import torch.nn as nn
-import torch.nn.functional as F
-from fairseq.data import Dictionary
-from fairseq.models import (
- FairseqDecoder,
- FairseqLanguageModel,
- register_model,
- register_model_architecture,
-)
-
-
-@register_model("dummy_model")
-class DummyModel(FairseqLanguageModel):
- def __init__(self, args, encoder):
- super().__init__(encoder)
- self.args = args
-
- @staticmethod
- def add_args(parser):
- parser.add_argument("--num-layers", type=int, default=24)
- parser.add_argument("--embed-dim", type=int, default=1024)
-
- @classmethod
- def build_model(cls, args, task):
- encoder = DummyEncoder(
- num_embed=len(task.target_dictionary),
- embed_dim=args.embed_dim,
- num_layers=args.num_layers,
- )
- return cls(args, encoder)
-
- def forward(self, src_tokens, masked_tokens=None, **kwargs):
- return self.decoder(src_tokens, masked_tokens=masked_tokens)
-
-
-class DummyEncoder(FairseqDecoder):
- def __init__(self, num_embed=50000, embed_dim=1024, num_layers=24):
- super().__init__(Dictionary())
- self.embed = nn.Embedding(
- num_embeddings=num_embed, embedding_dim=embed_dim, padding_idx=0
- )
- self.layers_a = nn.ModuleList(
- [
- nn.Sequential(
- nn.LayerNorm(embed_dim),
- nn.Linear(embed_dim, 3 * embed_dim), # q, k, v input projection
- nn.Linear(3 * embed_dim, embed_dim), # skip self-attention
- nn.Linear(embed_dim, embed_dim), # output projection
- nn.Dropout(),
- )
- for i in range(num_layers)
- ]
- )
- self.layers_b = nn.ModuleList(
- [
- nn.Sequential(
- nn.LayerNorm(embed_dim),
- nn.Linear(embed_dim, 4 * embed_dim), # FFN
- nn.ReLU(),
- nn.Linear(4 * embed_dim, embed_dim), # FFN
- nn.Dropout(0.1),
- )
- for i in range(num_layers)
- ]
- )
- self.out_proj = nn.Linear(embed_dim, num_embed)
-
- def forward(self, tokens, masked_tokens=None):
- x = self.embed(tokens)
- for layer_a, layer_b in zip(self.layers_a, self.layers_b):
- x = x + layer_a(x)
- x = x + layer_b(x)
- x = self.out_proj(x)
- if masked_tokens is not None:
- x = x[masked_tokens]
- return (x,)
-
- def max_positions(self):
- return 1024
-
- def get_normalized_probs(self, net_output, log_probs, sample=None):
- logits = net_output[0].float()
- if log_probs:
- return F.log_softmax(logits, dim=-1)
- else:
- return F.softmax(logits, dim=-1)
-
-
-@register_model_architecture("dummy_model", "dummy_model")
-def base_architecture(args):
- pass
diff --git a/spaces/arxify/RVC-beta-v2-0618/runtime/Lib/site-packages/fairseq/config/__init__.py b/spaces/arxify/RVC-beta-v2-0618/runtime/Lib/site-packages/fairseq/config/__init__.py
deleted file mode 100644
index 6264236915a7269a4d920ee8213004374dd86a9a..0000000000000000000000000000000000000000
--- a/spaces/arxify/RVC-beta-v2-0618/runtime/Lib/site-packages/fairseq/config/__init__.py
+++ /dev/null
@@ -1,4 +0,0 @@
-# Copyright (c) Facebook, Inc. and its affiliates.
-#
-# This source code is licensed under the MIT license found in the
-# LICENSE file in the root directory of this source tree.
diff --git a/spaces/asciicorp/hotel-chat/vector_qa.py b/spaces/asciicorp/hotel-chat/vector_qa.py
deleted file mode 100644
index 8bcc27afd7e7d871d8d2bd305713898aeb5c9374..0000000000000000000000000000000000000000
--- a/spaces/asciicorp/hotel-chat/vector_qa.py
+++ /dev/null
@@ -1,30 +0,0 @@
-from langchain.llms import OpenAI
-from langchain.chains import RetrievalQA
-from langchain.prompts import PromptTemplate
-
-import pickle
-import os
-
-os.environ["OPENAI_API_KEY"] = "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"
-
-llm = OpenAI(temperature=0)
-
-prompt_template = """Use the following pieces of context to answer the question at the end. give a friendly and conversational answer a customer service agent might give.
-{context}
-Question: {question}
-Answer:"""
-PROMPT = PromptTemplate(
- template=prompt_template, input_variables=["context", "question"]
-)
-
-chain_type_kwargs = {"prompt": PROMPT}
-
-with open("vectorstore.pkl", "rb") as f:
- vectorstore = pickle.load(f)
-
-hotel_details_chain = RetrievalQA.from_chain_type(llm=llm, chain_type="stuff", retriever=vectorstore.as_retriever(), chain_type_kwargs=chain_type_kwargs)
-
-with open("vectorstore_rooms.pkl", "rb") as f:
- vectorstore_rooms = pickle.load(f)
-
-room_details_chain = RetrievalQA.from_chain_type(llm=llm, chain_type="stuff", retriever=vectorstore_rooms.as_retriever(), chain_type_kwargs=chain_type_kwargs)
\ No newline at end of file
diff --git a/spaces/aseifert/ExplaiNER/src/utils.py b/spaces/aseifert/ExplaiNER/src/utils.py
deleted file mode 100644
index 7443acbb3ca7e879e690f5fed981009b94cef6fc..0000000000000000000000000000000000000000
--- a/spaces/aseifert/ExplaiNER/src/utils.py
+++ /dev/null
@@ -1,255 +0,0 @@
-from pathlib import Path
-
-import matplotlib as matplotlib
-import matplotlib.cm as cm
-import pandas as pd
-import streamlit as st
-import tokenizers
-import torch
-import torch.nn.functional as F
-from st_aggrid import AgGrid, GridOptionsBuilder, GridUpdateMode
-
-PROJ = Path(__file__).parent
-
-tokenizer_hash_funcs = {
- tokenizers.Tokenizer: lambda _: None,
- tokenizers.AddedToken: lambda _: None,
-}
-# device = torch.device("cuda" if torch.cuda.is_available() else "cpu" if torch.has_mps else "cpu")
-device = torch.device("cuda" if torch.cuda.is_available() else "cpu")
-
-classmap = {
- "O": "O",
- "PER": "🙎",
- "person": "🙎",
- "LOC": "🌎",
- "location": "🌎",
- "ORG": "🏤",
- "corporation": "🏤",
- "product": "📱",
- "creative": "🎷",
- "MISC": "🎷",
-}
-
-
-def aggrid_interactive_table(df: pd.DataFrame) -> dict:
- """Creates an st-aggrid interactive table based on a dataframe.
-
- Args:
- df (pd.DataFrame]): Source dataframe
- Returns:
- dict: The selected row
- """
- options = GridOptionsBuilder.from_dataframe(
- df, enableRowGroup=True, enableValue=True, enablePivot=True
- )
-
- options.configure_side_bar()
- # options.configure_default_column(cellRenderer=JsCode('''function(params) {return ''+params.value+' '}'''))
-
- options.configure_selection("single")
- selection = AgGrid(
- df,
- enable_enterprise_modules=True,
- gridOptions=options.build(),
- theme="light",
- update_mode=GridUpdateMode.NO_UPDATE,
- allow_unsafe_jscode=True,
- )
-
- return selection
-
-
-def explode_df(df: pd.DataFrame) -> pd.DataFrame:
- """Takes a dataframe and explodes all the fields."""
-
- df_tokens = df.apply(pd.Series.explode)
- if "losses" in df.columns:
- df_tokens["losses"] = df_tokens["losses"].astype(float)
- return df_tokens # type: ignore
-
-
-def align_sample(row: pd.Series):
- """Uses word_ids to align all lists in a sample."""
-
- columns = row.axes[0].to_list()
- indices = [i for i, id in enumerate(row.word_ids) if id >= 0 and id != row.word_ids[i - 1]]
-
- out = {}
-
- tokens = []
- for i, tok in enumerate(row.tokens):
- if row.word_ids[i] == -1:
- continue
-
- if row.word_ids[i] != row.word_ids[i - 1]:
- tokens.append(tok.lstrip("▁").lstrip("##").rstrip("@@"))
- else:
- tokens[-1] += tok.lstrip("▁").lstrip("##").rstrip("@@")
- out["tokens"] = tokens
-
- if "preds" in columns:
- out["preds"] = [row.preds[i] for i in indices]
-
- if "labels" in columns:
- out["labels"] = [row.labels[i] for i in indices]
-
- if "losses" in columns:
- out["losses"] = [row.losses[i] for i in indices]
-
- if "probs" in columns:
- out["probs"] = [row.probs[i] for i in indices]
-
- if "hidden_states" in columns:
- out["hidden_states"] = [row.hidden_states[i] for i in indices]
-
- if "ids" in columns:
- out["ids"] = row.ids
-
- assert len(tokens) == len(out["preds"]), (tokens, row.tokens)
-
- return out
-
-
-@st.cache(
- allow_output_mutation=True,
- hash_funcs=tokenizer_hash_funcs,
-)
-def tag_text(text: str, tokenizer, model, device: torch.device) -> pd.DataFrame:
- """Tags a given text and creates an (exploded) DataFrame with the predicted labels and probabilities.
-
- Args:
- text (str): The text to be processed
- tokenizer: Tokenizer to use
- model (_type_): Model to use
- device (torch.device): The device we want pytorch to use for its calcultaions.
-
- Returns:
- pd.DataFrame: A data frame holding the tagged text.
- """
-
- tokens = tokenizer(text).tokens()
- tokenized = tokenizer(text, return_tensors="pt")
- word_ids = [w if w is not None else -1 for w in tokenized.word_ids()]
- input_ids = tokenized.input_ids.to(device)
- outputs = model(input_ids, output_hidden_states=True)
- preds = torch.argmax(outputs.logits, dim=2)
- preds = [model.config.id2label[p] for p in preds[0].cpu().numpy()]
- hidden_states = outputs.hidden_states[-1][0].detach().cpu().numpy()
- # hidden_states = np.mean([hidden_states, outputs.hidden_states[0][0].detach().cpu().numpy()], axis=0)
-
- probs = 1 // (
- torch.min(F.softmax(outputs.logits, dim=-1), dim=-1).values[0].detach().cpu().numpy()
- )
-
- df = pd.DataFrame(
- [[tokens, word_ids, preds, probs, hidden_states]],
- columns="tokens word_ids preds probs hidden_states".split(),
- )
- merged_df = pd.DataFrame(df.apply(align_sample, axis=1).tolist())
- return explode_df(merged_df).reset_index().drop(columns=["index"])
-
-
-def get_bg_color(label: str):
- """Retrieves a label's color from the session state."""
- return st.session_state[f"color_{label}"]
-
-
-def get_fg_color(bg_color_hex: str) -> str:
- """Chooses the proper (foreground) text color (black/white) for a given background color, maximizing contrast.
-
- Adapted from https://gomakethings.com/dynamically-changing-the-text-color-based-on-background-color-contrast-with-vanilla-js/
-
- Args:
- bg_color_hex (str): The background color given as a HEX stirng.
-
- Returns:
- str: Either "black" or "white".
- """
- r = int(bg_color_hex[1:3], 16)
- g = int(bg_color_hex[3:5], 16)
- b = int(bg_color_hex[5:7], 16)
- yiq = ((r * 299) + (g * 587) + (b * 114)) / 1000
- return "black" if (yiq >= 128) else "white"
-
-
-def colorize_classes(df: pd.DataFrame) -> pd.DataFrame:
- """Colorizes the errors in the dataframe."""
-
- def colorize_row(row):
- return [
- "background-color: "
- + ("white" if (row["labels"] == "IGN" or (row["preds"] == row["labels"])) else "pink")
- + ";"
- ] * len(row)
-
- def colorize_col(col):
- if col.name == "labels" or col.name == "preds":
- bgs = []
- fgs = []
- for v in col.values:
- bgs.append(get_bg_color(v.split("-")[1]) if "-" in v else "#ffffff")
- fgs.append(get_fg_color(bgs[-1]))
- return [f"background-color: {bg}; color: {fg};" for bg, fg in zip(bgs, fgs)]
- return [""] * len(col)
-
- df = df.reset_index().drop(columns=["index"]).T
- return df # .style.apply(colorize_col, axis=0)
-
-
-def htmlify_labeled_example(example: pd.DataFrame) -> str:
- """Builds an HTML (string) representation of a single example.
-
- Args:
- example (pd.DataFrame): The example to process.
-
- Returns:
- str: An HTML string representation of a single example.
- """
- html = []
-
- for _, row in example.iterrows():
- pred = row.preds.split("-")[1] if "-" in row.preds else "O"
- label = row.labels
- label_class = row.labels.split("-")[1] if "-" in row.labels else "O"
-
- color = get_bg_color(row.preds.split("-")[1]) if "-" in row.preds else "#000000"
- true_color = get_bg_color(row.labels.split("-")[1]) if "-" in row.labels else "#000000"
-
- font_color = get_fg_color(color) if color else "white"
- true_font_color = get_fg_color(true_color) if true_color else "white"
-
- is_correct = row.preds == row.labels
- loss_html = (
- ""
- if float(row.losses) < 0.01
- else f"{row.losses:.3f} "
- )
- loss_html = ""
-
- if row.labels == row.preds == "O":
- html.append(f"{row.tokens} ")
- elif row.labels == "IGN":
- assert False
- else:
- opacity = "1" if not is_correct else "0.5"
- correct = (
- ""
- if is_correct
- else f"{classmap[label_class]} "
- )
- pred_icon = classmap[pred] if pred != "O" and row.preds[:2] != "I-" else ""
- html.append(
- f"{pred_icon + ' '}{row.tokens} {correct}{loss_html}"
- )
-
- return " ".join(html)
-
-
-def color_map_color(value: float, cmap_name="Set1", vmin=0, vmax=1) -> str:
- """Turns a value into a color using a color map."""
- norm = matplotlib.colors.Normalize(vmin=vmin, vmax=vmax)
- cmap = cm.get_cmap(cmap_name) # PiYG
- rgba = cmap(norm(abs(value)))
- color = matplotlib.colors.rgb2hex(rgba[:3])
- return color
diff --git a/spaces/avivdm1/AutoGPT/Dockerfile b/spaces/avivdm1/AutoGPT/Dockerfile
deleted file mode 100644
index 8396154998f32a50d55c199a674b638d5cf7bda2..0000000000000000000000000000000000000000
--- a/spaces/avivdm1/AutoGPT/Dockerfile
+++ /dev/null
@@ -1,38 +0,0 @@
-# Use an official Python base image from the Docker Hub
-FROM python:3.10-slim
-
-# Install git
-RUN apt-get -y update
-RUN apt-get -y install git chromium-driver
-
-# Install Xvfb and other dependencies for headless browser testing
-RUN apt-get update \
- && apt-get install -y wget gnupg2 libgtk-3-0 libdbus-glib-1-2 dbus-x11 xvfb ca-certificates
-
-# Install Firefox / Chromium
-RUN wget -q -O - https://dl-ssl.google.com/linux/linux_signing_key.pub | apt-key add - \
- && echo "deb [arch=amd64] http://dl.google.com/linux/chrome/deb/ stable main" >> /etc/apt/sources.list.d/google-chrome.list \
- && apt-get update \
- && apt-get install -y chromium firefox-esr
-
-# Set environment variables
-ENV PIP_NO_CACHE_DIR=yes \
- PYTHONUNBUFFERED=1 \
- PYTHONDONTWRITEBYTECODE=1
-
-# Create a non-root user and set permissions
-RUN useradd --create-home appuser
-WORKDIR /home/appuser
-RUN chown appuser:appuser /home/appuser
-USER appuser
-
-# Copy the requirements.txt file and install the requirements
-COPY --chown=appuser:appuser requirements.txt .
-RUN sed -i '/Items below this point will not be included in the Docker Image/,$d' requirements.txt && \
- pip install --no-cache-dir --user -r requirements.txt
-
-# Copy the application files
-COPY --chown=appuser:appuser autogpt/ ./autogpt
-
-# Set the entrypoint
-ENTRYPOINT ["python", "-m", "autogpt"]
diff --git a/spaces/awaawawawa/iurf7irfuyytruyyugb/ldmlib/data/lsun.py b/spaces/awaawawawa/iurf7irfuyytruyyugb/ldmlib/data/lsun.py
deleted file mode 100644
index 6256e45715ff0b57c53f985594d27cbbbff0e68e..0000000000000000000000000000000000000000
--- a/spaces/awaawawawa/iurf7irfuyytruyyugb/ldmlib/data/lsun.py
+++ /dev/null
@@ -1,92 +0,0 @@
-import os
-import numpy as np
-import PIL
-from PIL import Image
-from torch.utils.data import Dataset
-from torchvision import transforms
-
-
-class LSUNBase(Dataset):
- def __init__(self,
- txt_file,
- data_root,
- size=None,
- interpolation="bicubic",
- flip_p=0.5
- ):
- self.data_paths = txt_file
- self.data_root = data_root
- with open(self.data_paths, "r") as f:
- self.image_paths = f.read().splitlines()
- self._length = len(self.image_paths)
- self.labels = {
- "relative_file_path_": [l for l in self.image_paths],
- "file_path_": [os.path.join(self.data_root, l)
- for l in self.image_paths],
- }
-
- self.size = size
- self.interpolation = {"linear": PIL.Image.LINEAR,
- "bilinear": PIL.Image.BILINEAR,
- "bicubic": PIL.Image.BICUBIC,
- "lanczos": PIL.Image.LANCZOS,
- }[interpolation]
- self.flip = transforms.RandomHorizontalFlip(p=flip_p)
-
- def __len__(self):
- return self._length
-
- def __getitem__(self, i):
- example = dict((k, self.labels[k][i]) for k in self.labels)
- image = Image.open(example["file_path_"])
- if not image.mode == "RGB":
- image = image.convert("RGB")
-
- # default to score-sde preprocessing
- img = np.array(image).astype(np.uint8)
- crop = min(img.shape[0], img.shape[1])
- h, w, = img.shape[0], img.shape[1]
- img = img[(h - crop) // 2:(h + crop) // 2,
- (w - crop) // 2:(w + crop) // 2]
-
- image = Image.fromarray(img)
- if self.size is not None:
- image = image.resize((self.size, self.size), resample=self.interpolation)
-
- image = self.flip(image)
- image = np.array(image).astype(np.uint8)
- example["image"] = (image / 127.5 - 1.0).astype(np.float32)
- return example
-
-
-class LSUNChurchesTrain(LSUNBase):
- def __init__(self, **kwargs):
- super().__init__(txt_file="data/lsun/church_outdoor_train.txt", data_root="data/lsun/churches", **kwargs)
-
-
-class LSUNChurchesValidation(LSUNBase):
- def __init__(self, flip_p=0., **kwargs):
- super().__init__(txt_file="data/lsun/church_outdoor_val.txt", data_root="data/lsun/churches",
- flip_p=flip_p, **kwargs)
-
-
-class LSUNBedroomsTrain(LSUNBase):
- def __init__(self, **kwargs):
- super().__init__(txt_file="data/lsun/bedrooms_train.txt", data_root="data/lsun/bedrooms", **kwargs)
-
-
-class LSUNBedroomsValidation(LSUNBase):
- def __init__(self, flip_p=0.0, **kwargs):
- super().__init__(txt_file="data/lsun/bedrooms_val.txt", data_root="data/lsun/bedrooms",
- flip_p=flip_p, **kwargs)
-
-
-class LSUNCatsTrain(LSUNBase):
- def __init__(self, **kwargs):
- super().__init__(txt_file="data/lsun/cat_train.txt", data_root="data/lsun/cats", **kwargs)
-
-
-class LSUNCatsValidation(LSUNBase):
- def __init__(self, flip_p=0., **kwargs):
- super().__init__(txt_file="data/lsun/cat_val.txt", data_root="data/lsun/cats",
- flip_p=flip_p, **kwargs)
diff --git a/spaces/awacke1/MultiRhymeLyricSmith/rhyme-with-ai/rhyme.py b/spaces/awacke1/MultiRhymeLyricSmith/rhyme-with-ai/rhyme.py
deleted file mode 100644
index db5d6655768eda8b4545af452eb3cb3d6be9c05a..0000000000000000000000000000000000000000
--- a/spaces/awacke1/MultiRhymeLyricSmith/rhyme-with-ai/rhyme.py
+++ /dev/null
@@ -1,64 +0,0 @@
-import functools
-import random
-from typing import List, Optional
-
-import requests
-from gazpacho import Soup, get
-
-from rhyme_with_ai.utils import find_last_word
-
-
-def query_rhyme_words(sentence: str, n_rhymes: int, language:str="english") -> List[str]:
- """Returns a list of rhyme words for a sentence.
- Parameters
- ----------
- sentence : Sentence that may end with punctuation
- n_rhymes : Maximum number of rhymes to return
- Returns
- -------
- List[str] -- List of words that rhyme with the final word
- """
- last_word = find_last_word(sentence)
- if language == "english":
- return query_datamuse_api(last_word, n_rhymes)
- elif language == "dutch":
- return mick_rijmwoordenboek(last_word, n_rhymes)
- else:
- raise NotImplementedError(f"Unsupported language ({language}) expected 'english' or 'dutch'.")
-
-
-def query_datamuse_api(word: str, n_rhymes: Optional[int] = None) -> List[str]:
- """Query the DataMuse API.
- Parameters
- ----------
- word : Word to rhyme with
- n_rhymes : Max rhymes to return
- Returns
- -------
- Rhyme words
- """
- out = requests.get(
- "https://api.datamuse.com/words", params={"rel_rhy": word}
- ).json()
- words = [_["word"] for _ in out]
- if n_rhymes is None:
- return words
- return words[:n_rhymes]
-
-
-@functools.lru_cache(maxsize=128, typed=False)
-def mick_rijmwoordenboek(word: str, n_words: int):
- url = f"https://rijmwoordenboek.nl/rijm/{word}"
- html = get(url)
- soup = Soup(html)
-
- results = soup.find("div", {"id": "rhymeResultsWords"}).html.split(" ")
-
- # clean up
- results = [r.replace("\n", "").replace(" ", "") for r in results]
-
- # filter html and empty strings
- results = [r for r in results if ("<" not in r) and (len(r) > 0)]
-
- return random.sample(results, min(len(results), n_words))
-
diff --git a/spaces/awacke1/PDFViewerwithUpdatesWorkBench/app.py b/spaces/awacke1/PDFViewerwithUpdatesWorkBench/app.py
deleted file mode 100644
index bb37be37a7023ecc0313ee3615a9e36f91848cca..0000000000000000000000000000000000000000
--- a/spaces/awacke1/PDFViewerwithUpdatesWorkBench/app.py
+++ /dev/null
@@ -1,13 +0,0 @@
-#import fitz
-import streamlit as st
-
-uploaded_pdf = st.file_uploader("Load pdf: ", type=['pdf'])
-
-def show_pdf(file_path):
- with open(file_path,"rb") as f:
- base64_pdf = base64.b64encode(f.read()).decode('utf-8')
- pdf_display = f''
- st.markdown(pdf_display, unsafe_allow_html=True)
-
-if uploaded_pdf is not None:
- show_pdf(uploaded_pdf)
diff --git a/spaces/awacke1/VizLib-TopLargeHospitalsNewJersey-03-09-2023/app.py b/spaces/awacke1/VizLib-TopLargeHospitalsNewJersey-03-09-2023/app.py
deleted file mode 100644
index 05adfa181088800fc3ff4f4847de72688e4fe5a5..0000000000000000000000000000000000000000
--- a/spaces/awacke1/VizLib-TopLargeHospitalsNewJersey-03-09-2023/app.py
+++ /dev/null
@@ -1,58 +0,0 @@
-import streamlit as st
-import graphviz as gv
-from graphviz import Graph
-import folium
-from streamlit_folium import folium_static
-
-# Define the cluster relations graph using gvmap
-g = Graph(format='svg')
-g.graph_attr['bgcolor'] = '#FFFFFF'
-g.graph_attr['outputorder'] = 'edgesfirst'
-g.graph_attr['size'] = '10,10'
-g.node_attr['style'] = 'filled'
-g.node_attr['shape'] = 'box'
-g.node_attr['fillcolor'] = '#FFDAB9'
-
-with g.subgraph(name='cluster_NJ') as c:
- c.graph_attr['bgcolor'] = '#ADD8E6'
- c.node_attr['color'] = '#000000'
- c.node_attr['fontcolor'] = '#000000'
- c.attr(label='New Jersey', fontsize='24')
- c.node('Hackensack Meridian Health', URL='https://www.hackensackmeridianhealth.org/', target='_blank', tooltip='Hackensack Meridian Health: Hackensack University Medical Center')
- c.node('RWJBarnabas Health', URL='https://www.rwjbh.org/', target='_blank', tooltip='RWJBarnabas Health: Robert Wood Johnson University Hospital')
- c.node('Atlantic Health System', URL='https://www.atlantichealth.org/', target='_blank', tooltip='Atlantic Health System: Morristown Medical Center')
- c.node('Virtua Health', URL='https://www.virtua.org/', target='_blank', tooltip='Virtua Health: Virtua Memorial Hospital')
- c.node('Inspira Health', URL='https://www.inspirahealthnetwork.org/', target='_blank', tooltip='Inspira Health: Inspira Medical Center Vineland')
- c.node('Cooper University Health Care', URL='https://www.cooperhealth.org/', target='_blank', tooltip='Cooper University Health Care: Cooper University Hospital')
- c.node('University Hospital', URL='https://www.uhnj.org/', target='_blank', tooltip='University Hospital: University Hospital')
- c.node('Robert Wood Johnson University Hospital Hamilton', URL='https://www.rwjbh.org/robert-wood-johnson-university-hospital-hamilton/', target='_blank', tooltip='Robert Wood Johnson University Hospital Hamilton: Robert Wood Johnson University Hospital Hamilton')
- c.node('Trinitas Regional Medical Center', URL='https://www.trinitasrmc.org/', target='_blank', tooltip='Trinitas Regional Medical Center: Trinitas Regional Medical Center')
- c.node('Capital Health Regional Medical Center', URL='https://www.capitalhealth.org/', target='_blank', tooltip='Capital Health Regional Medical Center: Capital Health Regional Medical Center')
-
-# Render the graph using streamlit
-st.graphviz_chart(g)
-
-# Define hospitals data
-hospitals = [('Hackensack Meridian Health', 'Hackensack University Medical Center', 40.899886, -74.039179),
- ('RWJBarnabas Health', 'Robert Wood Johnson University Hospital', 40.491301, -74.450611),
- ('Atlantic Health System', 'Morristown Medical Center', 40.787231, -74.473851),
- ('Virtua Health', 'Virtua Memorial Hospital', 39.931229, -75.025831),
- ('Inspira Health', 'Inspira Medical Center Vineland', 39.460225, -75.035542),
- ('Cooper University Health Care', 'Cooper University Hospital', 39.942743, -75.119090),
- ('University Hospital', 'University Hospital', 40.742310, -74.177609),
- ('Robert Wood Johnson University Hospital Hamilton', 'Robert Wood Johnson University Hospital Hamilton', 40.214008, -74.679619),
- ('Trinitas Regional Medical Center', 'Trinitas Regional Medical Center', 40.661474, -74.215013),
- ('Capital Health Regional Medical Center', 'Capital Health Regional Medical Center', 40.266778, -74.796452)]
-
-#Create a map centered on New Jersey
-m = folium.Map(location=[40.0583, -74.4057], zoom_start=8)
-
-#Add markers for each hospital
-for hospital in hospitals:
- folium.Marker(
- location=[hospital[2], hospital[3]],
- popup=f'{hospital[1]} {hospital[2]},{hospital[3]}'
- ).add_to(m)
-
-#Display the map in Streamlit
-folium_static(m)
diff --git a/spaces/axuint/OpenNiji/app.py b/spaces/axuint/OpenNiji/app.py
deleted file mode 100644
index 77e77186169c96b041fd9e4a5588642dd219bf73..0000000000000000000000000000000000000000
--- a/spaces/axuint/OpenNiji/app.py
+++ /dev/null
@@ -1,3 +0,0 @@
-import gradio as gr
-
-gr.Interface.load("models/Korakoe/OpenNiji").launch()
\ No newline at end of file
diff --git a/spaces/ayapoooooo123/openai-jukebox-1b-lyrics/README.md b/spaces/ayapoooooo123/openai-jukebox-1b-lyrics/README.md
deleted file mode 100644
index 7513f56e1c10332f93d220dc609e4c6c497afdb3..0000000000000000000000000000000000000000
--- a/spaces/ayapoooooo123/openai-jukebox-1b-lyrics/README.md
+++ /dev/null
@@ -1,13 +0,0 @@
----
-title: Openai Jukebox 1b Lyrics
-emoji: 💻
-colorFrom: red
-colorTo: blue
-sdk: gradio
-sdk_version: 3.15.0
-app_file: app.py
-pinned: false
-license: bigscience-bloom-rail-1.0
----
-
-Check out the configuration reference at https://huggingface.co/docs/hub/spaces-config-reference
diff --git a/spaces/banana-projects/web3d/node_modules/three/src/materials/LineBasicMaterial.d.ts b/spaces/banana-projects/web3d/node_modules/three/src/materials/LineBasicMaterial.d.ts
deleted file mode 100644
index f4c56c18feacd1e90e818000cb010cef58eebcdd..0000000000000000000000000000000000000000
--- a/spaces/banana-projects/web3d/node_modules/three/src/materials/LineBasicMaterial.d.ts
+++ /dev/null
@@ -1,20 +0,0 @@
-import { Color } from './../math/Color';
-import { MaterialParameters, Material } from './Material';
-
-export interface LineBasicMaterialParameters extends MaterialParameters {
- color?: Color | string | number;
- linewidth?: number;
- linecap?: string;
- linejoin?: string;
-}
-
-export class LineBasicMaterial extends Material {
- constructor(parameters?: LineBasicMaterialParameters);
-
- color: Color;
- linewidth: number;
- linecap: string;
- linejoin: string;
-
- setValues(parameters: LineBasicMaterialParameters): void;
-}
diff --git a/spaces/banana-projects/web3d/node_modules/three/src/math/Interpolant.d.ts b/spaces/banana-projects/web3d/node_modules/three/src/math/Interpolant.d.ts
deleted file mode 100644
index 1d3301a03086d6c19c72d5a8b713b3ce124a819b..0000000000000000000000000000000000000000
--- a/spaces/banana-projects/web3d/node_modules/three/src/math/Interpolant.d.ts
+++ /dev/null
@@ -1,15 +0,0 @@
-export abstract class Interpolant {
- constructor(
- parameterPositions: any,
- samplesValues: any,
- sampleSize: number,
- resultBuffer?: any
- );
-
- parameterPositions: any;
- samplesValues: any;
- valueSize: number;
- resultBuffer: any;
-
- evaluate(time: number): any;
-}
diff --git a/spaces/beihai/GFPGAN-V1.3-whole-image/.history/app_20220327195556.py b/spaces/beihai/GFPGAN-V1.3-whole-image/.history/app_20220327195556.py
deleted file mode 100644
index 87c94b94347da6ee77c6686922500ed33abf5eaf..0000000000000000000000000000000000000000
--- a/spaces/beihai/GFPGAN-V1.3-whole-image/.history/app_20220327195556.py
+++ /dev/null
@@ -1,68 +0,0 @@
-import os
-os.system("pip install gfpgan")
-
-#os.system("pip freeze")
-#os.system("wget https://github.com/TencentARC/GFPGAN/releases/download/v0.2.0/GFPGANCleanv1-NoCE-C2.pth -P .")
-import random
-import gradio as gr
-from PIL import Image
-import torch
-# torch.hub.download_url_to_file('https://upload.wikimedia.org/wikipedia/commons/thumb/a/ab/Abraham_Lincoln_O-77_matte_collodion_print.jpg/1024px-Abraham_Lincoln_O-77_matte_collodion_print.jpg', 'lincoln.jpg')
-# torch.hub.download_url_to_file('https://upload.wikimedia.org/wikipedia/commons/5/50/Albert_Einstein_%28Nobel%29.png', 'einstein.png')
-# torch.hub.download_url_to_file('https://upload.wikimedia.org/wikipedia/commons/thumb/9/9d/Thomas_Edison2.jpg/1024px-Thomas_Edison2.jpg', 'edison.jpg')
-# torch.hub.download_url_to_file('https://upload.wikimedia.org/wikipedia/commons/thumb/a/a9/Henry_Ford_1888.jpg/1024px-Henry_Ford_1888.jpg', 'Henry.jpg')
-# torch.hub.download_url_to_file('https://upload.wikimedia.org/wikipedia/commons/thumb/0/06/Frida_Kahlo%2C_by_Guillermo_Kahlo.jpg/800px-Frida_Kahlo%2C_by_Guillermo_Kahlo.jpg', 'Frida.jpg')
-
-
-import cv2
-import glob
-import numpy as np
-from basicsr.utils import imwrite
-from gfpgan import GFPGANer
-
-bg_upsampler = None
-
-
-
-# set up GFPGAN restorer
-restorer = GFPGANer(
- model_path='experiments/pretrained_models/GFPGANv1.3.pth',
- upscale=2,
- arch='clean',
- channel_multiplier=2,
- bg_upsampler=bg_upsampler)
-
-
-def inference(img):
- input_img = cv2.imread(img, cv2.IMREAD_COLOR)
- cropped_faces, restored_faces, restored_img = restorer.enhance(
- input_img, has_aligned=False, only_center_face=False, paste_back=True)
-
- #return Image.fromarray(restored_faces[0][:,:,::-1])
- return Image.fromarray(restored_img[:, :, ::-1])
-
-title = "让美好回忆更清晰"
-
-
-description = "上传老照片,点击Submit,稍等片刻,右侧Output将照片另存为即可。"
-article = "
"
-
-article = " | Github Repo
"
-
-gr.Interface(
- inference,
- [gr.inputs.Image(type="filepath", label="Input")],
- gr.outputs.Image(type="pil", label="Output"),
- title=title,
- description=description,
- article=article,
- examples=[
- ['lincoln.jpg'],
- ['einstein.png'],
- ['edison.jpg'],
- ['Henry.jpg'],
- ['Frida.jpg']
- ]
- ).launch(enable_queue=True,cache_examples=True,share=True)
-
-
diff --git a/spaces/beki/pii-anonymizer/spacy_recognizer.py b/spaces/beki/pii-anonymizer/spacy_recognizer.py
deleted file mode 100644
index 438784bff558b160afeb6c781d81944fd3dd2efe..0000000000000000000000000000000000000000
--- a/spaces/beki/pii-anonymizer/spacy_recognizer.py
+++ /dev/null
@@ -1,131 +0,0 @@
-import logging
-from typing import Optional, List, Tuple, Set
-
-from presidio_analyzer import (
- RecognizerResult,
- LocalRecognizer,
- AnalysisExplanation,
-)
-from presidio_analyzer.nlp_engine import NlpArtifacts
-from presidio_analyzer.predefined_recognizers.spacy_recognizer import SpacyRecognizer
-
-logger = logging.getLogger("presidio-analyzer")
-
-
-class CustomSpacyRecognizer(LocalRecognizer):
-
- ENTITIES = [
- "LOCATION",
- "PERSON",
- "NRP",
- "ORGANIZATION",
- "DATE_TIME",
- ]
-
- DEFAULT_EXPLANATION = "Identified as {} by Spacy's Named Entity Recognition (Privy-trained)"
-
- CHECK_LABEL_GROUPS = [
- ({"LOCATION"}, {"LOC", "LOCATION", "STREET_ADDRESS", "COORDINATE"}),
- ({"PERSON"}, {"PER", "PERSON"}),
- ({"NRP"}, {"NORP", "NRP"}),
- ({"ORGANIZATION"}, {"ORG"}),
- ({"DATE_TIME"}, {"DATE_TIME"}),
- ]
-
- MODEL_LANGUAGES = {
- "en": "beki/en_spacy_pii_distilbert",
- }
-
- PRESIDIO_EQUIVALENCES = {
- "PER": "PERSON",
- "LOC": "LOCATION",
- "ORG": "ORGANIZATION",
- "NROP": "NRP",
- "DATE_TIME": "DATE_TIME",
- }
-
- def __init__(
- self,
- supported_language: str = "en",
- supported_entities: Optional[List[str]] = None,
- check_label_groups: Optional[Tuple[Set, Set]] = None,
- context: Optional[List[str]] = None,
- ner_strength: float = 0.85,
- ):
- self.ner_strength = ner_strength
- self.check_label_groups = (
- check_label_groups if check_label_groups else self.CHECK_LABEL_GROUPS
- )
- supported_entities = supported_entities if supported_entities else self.ENTITIES
- super().__init__(
- supported_entities=supported_entities,
- supported_language=supported_language,
- )
-
- def load(self) -> None:
- """Load the model, not used. Model is loaded during initialization."""
- pass
-
- def get_supported_entities(self) -> List[str]:
- """
- Return supported entities by this model.
- :return: List of the supported entities.
- """
- return self.supported_entities
-
- def build_spacy_explanation(
- self, original_score: float, explanation: str
- ) -> AnalysisExplanation:
- """
- Create explanation for why this result was detected.
- :param original_score: Score given by this recognizer
- :param explanation: Explanation string
- :return:
- """
- explanation = AnalysisExplanation(
- recognizer=self.__class__.__name__,
- original_score=original_score,
- textual_explanation=explanation,
- )
- return explanation
-
- def analyze(self, text, entities, nlp_artifacts=None): # noqa D102
- results = []
- if not nlp_artifacts:
- logger.warning("Skipping SpaCy, nlp artifacts not provided...")
- return results
-
- ner_entities = nlp_artifacts.entities
-
- for entity in entities:
- if entity not in self.supported_entities:
- continue
- for ent in ner_entities:
- if not self.__check_label(entity, ent.label_, self.check_label_groups):
- continue
- textual_explanation = self.DEFAULT_EXPLANATION.format(
- ent.label_)
- explanation = self.build_spacy_explanation(
- self.ner_strength, textual_explanation
- )
- spacy_result = RecognizerResult(
- entity_type=entity,
- start=ent.start_char,
- end=ent.end_char,
- score=self.ner_strength,
- analysis_explanation=explanation,
- recognition_metadata={
- RecognizerResult.RECOGNIZER_NAME_KEY: self.name
- },
- )
- results.append(spacy_result)
-
- return results
-
- @staticmethod
- def __check_label(
- entity: str, label: str, check_label_groups: Tuple[Set, Set]
- ) -> bool:
- return any(
- [entity in egrp and label in lgrp for egrp, lgrp in check_label_groups]
- )
diff --git a/spaces/beomi/KoRWKV-1.5B/app.py b/spaces/beomi/KoRWKV-1.5B/app.py
deleted file mode 100644
index a9cf1c76ab28dbeb76f37ca37bc3111335d560ba..0000000000000000000000000000000000000000
--- a/spaces/beomi/KoRWKV-1.5B/app.py
+++ /dev/null
@@ -1,93 +0,0 @@
-from threading import Thread
-
-import torch
-import gradio as gr
-from transformers import AutoTokenizer, RwkvForCausalLM, TextIteratorStreamer
-
-model_id = "beomi/KoRWKV-1.5B"
-torch_device = "cuda" if torch.cuda.is_available() else "cpu"
-print("Running on device:", torch_device)
-print("CPU threads:", torch.get_num_threads())
-
-
-if torch_device == "cuda":
- model = RwkvForCausalLM.from_pretrained(model_id, device_map="auto")
-else:
- model = RwkvForCausalLM.from_pretrained(model_id)
-tokenizer = AutoTokenizer.from_pretrained(model_id)
-
-
-def run_generation(user_text, top_p, temperature, max_new_tokens):
- # Get the model and tokenizer, and tokenize the user text.
- user_text = user_text.strip()
- model_inputs = tokenizer([user_text], return_tensors="pt", return_token_type_ids=False).to(torch_device)
-
- if len(user_text) > 100:
- skip_prompt = True
- else:
- skip_prompt = False
-
- # Start generation on a separate thread, so that we don't block the UI. The text is pulled from the streamer
- # in the main thread. Adds timeout to the streamer to handle exceptions in the generation thread.
- streamer = TextIteratorStreamer(tokenizer, timeout=10., skip_prompt=skip_prompt, skip_special_tokens=False)
- generate_kwargs = dict(
- model_inputs,
- streamer=streamer,
- max_new_tokens=max_new_tokens,
- do_sample=True,
- top_p=top_p,
- temperature=float(temperature),
- # repetition_penalty=0.5,
- # no_repeat_ngram_size=6,
- )
- t = Thread(target=model.generate, kwargs=generate_kwargs)
- t.start()
-
- # Pull the generated text from the streamer, and update the model output.
- model_output = ""
- for new_text in streamer:
- model_output += new_text
- yield model_output
- return model_output
-
-
-def reset_textbox():
- return gr.update(value='')
-
-
-with gr.Blocks() as demo:
- duplicate_link = "https://huggingface.co/spaces/beomi/KoRWKV-1.5B?duplicate=true"
- gr.Markdown(
- "# 🤗 KoRWKV-1.5B 🔥Streaming🔥 on Gradio\n"
- "This demo showcases the use of the "
- "[streaming feature](https://huggingface.co/docs/transformers/main/en/generation_strategies#streaming) "
- "of 🤗 Transformers with Gradio to generate text in real-time. It uses "
- f"[{model_id}](https://huggingface.co/{model_id}) and the Spaces free compute tier.\n\n"
- f"Feel free to [duplicate this Space]({duplicate_link}) to try your own models or use this space as a "
- "template! 💛"
- )
-
- with gr.Row():
- with gr.Column(scale=4):
- user_text = gr.Textbox(
- placeholder="여기에 문장의 시작부분을 입력해주세요...",
- label="User input"
- )
- model_output = gr.Textbox(label="Model output", lines=10, interactive=False)
- button_submit = gr.Button(value="Submit")
-
- with gr.Column(scale=1):
- max_new_tokens = gr.Slider(
- minimum=1, maximum=512, value=250, step=1, interactive=True, label="Max New Tokens",
- )
- top_p = gr.Slider(
- minimum=0.05, maximum=1.0, value=1, step=0.05, interactive=True, label="Top-p (nucleus sampling)",
- )
- temperature = gr.Slider(
- minimum=0.1, maximum=2.0, value=1, step=0.1, interactive=True, label="Temperature",
- )
-
- user_text.submit(run_generation, [user_text, top_p, temperature, max_new_tokens], model_output)
- button_submit.click(run_generation, [user_text, top_p, temperature, max_new_tokens], model_output)
-
- demo.queue(max_size=32).launch(enable_queue=True)
diff --git a/spaces/bhandsab/meta-llama-Llama-2-70b-chat/README.md b/spaces/bhandsab/meta-llama-Llama-2-70b-chat/README.md
deleted file mode 100644
index 5e1fde61df277a4cc08e0bb01046a46fab174138..0000000000000000000000000000000000000000
--- a/spaces/bhandsab/meta-llama-Llama-2-70b-chat/README.md
+++ /dev/null
@@ -1,12 +0,0 @@
----
-title: Meta Llama Llama 2 70b Chat
-emoji: 🐨
-colorFrom: green
-colorTo: yellow
-sdk: streamlit
-sdk_version: 1.21.0
-app_file: app.py
-pinned: false
----
-
-Check out the configuration reference at https://huggingface.co/docs/hub/spaces-config-reference
diff --git a/spaces/bioriAsaeru/text-to-voice/((HOT)) Adobe Audition CC 2020 V13.0.3.60 With Crack (x64) Latest The Best Deal You Can Find Online.md b/spaces/bioriAsaeru/text-to-voice/((HOT)) Adobe Audition CC 2020 V13.0.3.60 With Crack (x64) Latest The Best Deal You Can Find Online.md
deleted file mode 100644
index 30d794813223878e0368e154255f9077cf9e6a1f..0000000000000000000000000000000000000000
--- a/spaces/bioriAsaeru/text-to-voice/((HOT)) Adobe Audition CC 2020 V13.0.3.60 With Crack (x64) Latest The Best Deal You Can Find Online.md
+++ /dev/null
@@ -1,6 +0,0 @@
-((HOT)) Adobe Audition CC 2020 V13.0.3.60 With Crack (x64) Latest DOWNLOAD >>>>> https://urloso.com/2uyPyr
-
- aaccfb2cb3
-
-
-
diff --git a/spaces/bioriAsaeru/text-to-voice/IntelliJIDEACracklicensekeywithtorrent100WorkingfreeDownload The Ultimate Guide for Java and Kotlin Developers.md b/spaces/bioriAsaeru/text-to-voice/IntelliJIDEACracklicensekeywithtorrent100WorkingfreeDownload The Ultimate Guide for Java and Kotlin Developers.md
deleted file mode 100644
index 3877d5c9e4b34b778146cc2a87ffc036189b749e..0000000000000000000000000000000000000000
--- a/spaces/bioriAsaeru/text-to-voice/IntelliJIDEACracklicensekeywithtorrent100WorkingfreeDownload The Ultimate Guide for Java and Kotlin Developers.md
+++ /dev/null
@@ -1,6 +0,0 @@
-IntelliJIDEACracklicensekeywithtorrent100WorkingfreeDownload Download Zip ✶✶✶ https://urloso.com/2uyPqH
-
- aaccfb2cb3
-
-
-
diff --git a/spaces/bonrix/text_detection_easyocr/README.md b/spaces/bonrix/text_detection_easyocr/README.md
deleted file mode 100644
index 3022715bf8d6b895e9fc15d6cb7cbff14a95ec6a..0000000000000000000000000000000000000000
--- a/spaces/bonrix/text_detection_easyocr/README.md
+++ /dev/null
@@ -1,13 +0,0 @@
----
-title: Text Detection Easyocr
-emoji: 🌍
-colorFrom: red
-colorTo: green
-sdk: gradio
-sdk_version: 3.29.0
-app_file: app.py
-pinned: false
-license: apache-2.0
----
-
-Check out the configuration reference at https://huggingface.co/docs/hub/spaces-config-reference
diff --git a/spaces/brjathu/HMR2.0/vendor/detectron2/projects/DeepLab/deeplab/build_solver.py b/spaces/brjathu/HMR2.0/vendor/detectron2/projects/DeepLab/deeplab/build_solver.py
deleted file mode 100644
index a1d359c2c35baf75a835879bb4b4f902be235179..0000000000000000000000000000000000000000
--- a/spaces/brjathu/HMR2.0/vendor/detectron2/projects/DeepLab/deeplab/build_solver.py
+++ /dev/null
@@ -1,27 +0,0 @@
-# Copyright (c) Facebook, Inc. and its affiliates.
-import torch
-
-from detectron2.config import CfgNode
-from detectron2.solver import LRScheduler
-from detectron2.solver import build_lr_scheduler as build_d2_lr_scheduler
-
-from .lr_scheduler import WarmupPolyLR
-
-
-def build_lr_scheduler(cfg: CfgNode, optimizer: torch.optim.Optimizer) -> LRScheduler:
- """
- Build a LR scheduler from config.
- """
- name = cfg.SOLVER.LR_SCHEDULER_NAME
- if name == "WarmupPolyLR":
- return WarmupPolyLR(
- optimizer,
- cfg.SOLVER.MAX_ITER,
- warmup_factor=cfg.SOLVER.WARMUP_FACTOR,
- warmup_iters=cfg.SOLVER.WARMUP_ITERS,
- warmup_method=cfg.SOLVER.WARMUP_METHOD,
- power=cfg.SOLVER.POLY_LR_POWER,
- constant_ending=cfg.SOLVER.POLY_LR_CONSTANT_ENDING,
- )
- else:
- return build_d2_lr_scheduler(cfg, optimizer)
diff --git a/spaces/camel-ai/camel-data-explorer/sync.sh b/spaces/camel-ai/camel-data-explorer/sync.sh
deleted file mode 100644
index 7b6bc0fcff9800a24c73b2dc4a5e8b9305101059..0000000000000000000000000000000000000000
--- a/spaces/camel-ai/camel-data-explorer/sync.sh
+++ /dev/null
@@ -1,15 +0,0 @@
-TMP_DIR=/tmp/camel_hf_tmp
-echo $TMP_DIR
-HF_REPO_DIR=`realpath .`
-echo $HF_REPO_DIR
-
-mkdir -p $TMP_DIR
-git clone -b hf_spaces_2 https://github.com/lightaime/camel.git $TMP_DIR
-cd $TMP_DIR
-
-find apps/data_explorer -name "*.py" | grep -v test | xargs -n 1 -I {} rsync -R {} $HF_REPO_DIR
-find apps/common -name "*.py" | grep -v test | xargs -n 1 -I {} rsync -R {} $HF_REPO_DIR
-
-rm -rf $TMP_DIR
-
-echo Done
diff --git a/spaces/camenduru-com/VITS-Umamusume-voice-synthesizer/monotonic_align/core.c b/spaces/camenduru-com/VITS-Umamusume-voice-synthesizer/monotonic_align/core.c
deleted file mode 100644
index 5631d20a9a00db29e143a6e8e4e5c378d6bb850a..0000000000000000000000000000000000000000
--- a/spaces/camenduru-com/VITS-Umamusume-voice-synthesizer/monotonic_align/core.c
+++ /dev/null
@@ -1,21299 +0,0 @@
-/* Generated by Cython 0.29.21 */
-
-/* BEGIN: Cython Metadata
-{
- "distutils": {
- "name": "monotonic_align.core",
- "sources": [
- "core.pyx"
- ]
- },
- "module_name": "monotonic_align.core"
-}
-END: Cython Metadata */
-
-#define PY_SSIZE_T_CLEAN
-#include "Python.h"
-#ifndef Py_PYTHON_H
- #error Python headers needed to compile C extensions, please install development version of Python.
-#elif PY_VERSION_HEX < 0x02060000 || (0x03000000 <= PY_VERSION_HEX && PY_VERSION_HEX < 0x03030000)
- #error Cython requires Python 2.6+ or Python 3.3+.
-#else
-#define CYTHON_ABI "0_29_21"
-#define CYTHON_HEX_VERSION 0x001D15F0
-#define CYTHON_FUTURE_DIVISION 0
-#include
-#ifndef offsetof
- #define offsetof(type, member) ( (size_t) & ((type*)0) -> member )
-#endif
-#if !defined(WIN32) && !defined(MS_WINDOWS)
- #ifndef __stdcall
- #define __stdcall
- #endif
- #ifndef __cdecl
- #define __cdecl
- #endif
- #ifndef __fastcall
- #define __fastcall
- #endif
-#endif
-#ifndef DL_IMPORT
- #define DL_IMPORT(t) t
-#endif
-#ifndef DL_EXPORT
- #define DL_EXPORT(t) t
-#endif
-#define __PYX_COMMA ,
-#ifndef HAVE_LONG_LONG
- #if PY_VERSION_HEX >= 0x02070000
- #define HAVE_LONG_LONG
- #endif
-#endif
-#ifndef PY_LONG_LONG
- #define PY_LONG_LONG LONG_LONG
-#endif
-#ifndef Py_HUGE_VAL
- #define Py_HUGE_VAL HUGE_VAL
-#endif
-#ifdef PYPY_VERSION
- #define CYTHON_COMPILING_IN_PYPY 1
- #define CYTHON_COMPILING_IN_PYSTON 0
- #define CYTHON_COMPILING_IN_CPYTHON 0
- #undef CYTHON_USE_TYPE_SLOTS
- #define CYTHON_USE_TYPE_SLOTS 0
- #undef CYTHON_USE_PYTYPE_LOOKUP
- #define CYTHON_USE_PYTYPE_LOOKUP 0
- #if PY_VERSION_HEX < 0x03050000
- #undef CYTHON_USE_ASYNC_SLOTS
- #define CYTHON_USE_ASYNC_SLOTS 0
- #elif !defined(CYTHON_USE_ASYNC_SLOTS)
- #define CYTHON_USE_ASYNC_SLOTS 1
- #endif
- #undef CYTHON_USE_PYLIST_INTERNALS
- #define CYTHON_USE_PYLIST_INTERNALS 0
- #undef CYTHON_USE_UNICODE_INTERNALS
- #define CYTHON_USE_UNICODE_INTERNALS 0
- #undef CYTHON_USE_UNICODE_WRITER
- #define CYTHON_USE_UNICODE_WRITER 0
- #undef CYTHON_USE_PYLONG_INTERNALS
- #define CYTHON_USE_PYLONG_INTERNALS 0
- #undef CYTHON_AVOID_BORROWED_REFS
- #define CYTHON_AVOID_BORROWED_REFS 1
- #undef CYTHON_ASSUME_SAFE_MACROS
- #define CYTHON_ASSUME_SAFE_MACROS 0
- #undef CYTHON_UNPACK_METHODS
- #define CYTHON_UNPACK_METHODS 0
- #undef CYTHON_FAST_THREAD_STATE
- #define CYTHON_FAST_THREAD_STATE 0
- #undef CYTHON_FAST_PYCALL
- #define CYTHON_FAST_PYCALL 0
- #undef CYTHON_PEP489_MULTI_PHASE_INIT
- #define CYTHON_PEP489_MULTI_PHASE_INIT 0
- #undef CYTHON_USE_TP_FINALIZE
- #define CYTHON_USE_TP_FINALIZE 0
- #undef CYTHON_USE_DICT_VERSIONS
- #define CYTHON_USE_DICT_VERSIONS 0
- #undef CYTHON_USE_EXC_INFO_STACK
- #define CYTHON_USE_EXC_INFO_STACK 0
-#elif defined(PYSTON_VERSION)
- #define CYTHON_COMPILING_IN_PYPY 0
- #define CYTHON_COMPILING_IN_PYSTON 1
- #define CYTHON_COMPILING_IN_CPYTHON 0
- #ifndef CYTHON_USE_TYPE_SLOTS
- #define CYTHON_USE_TYPE_SLOTS 1
- #endif
- #undef CYTHON_USE_PYTYPE_LOOKUP
- #define CYTHON_USE_PYTYPE_LOOKUP 0
- #undef CYTHON_USE_ASYNC_SLOTS
- #define CYTHON_USE_ASYNC_SLOTS 0
- #undef CYTHON_USE_PYLIST_INTERNALS
- #define CYTHON_USE_PYLIST_INTERNALS 0
- #ifndef CYTHON_USE_UNICODE_INTERNALS
- #define CYTHON_USE_UNICODE_INTERNALS 1
- #endif
- #undef CYTHON_USE_UNICODE_WRITER
- #define CYTHON_USE_UNICODE_WRITER 0
- #undef CYTHON_USE_PYLONG_INTERNALS
- #define CYTHON_USE_PYLONG_INTERNALS 0
- #ifndef CYTHON_AVOID_BORROWED_REFS
- #define CYTHON_AVOID_BORROWED_REFS 0
- #endif
- #ifndef CYTHON_ASSUME_SAFE_MACROS
- #define CYTHON_ASSUME_SAFE_MACROS 1
- #endif
- #ifndef CYTHON_UNPACK_METHODS
- #define CYTHON_UNPACK_METHODS 1
- #endif
- #undef CYTHON_FAST_THREAD_STATE
- #define CYTHON_FAST_THREAD_STATE 0
- #undef CYTHON_FAST_PYCALL
- #define CYTHON_FAST_PYCALL 0
- #undef CYTHON_PEP489_MULTI_PHASE_INIT
- #define CYTHON_PEP489_MULTI_PHASE_INIT 0
- #undef CYTHON_USE_TP_FINALIZE
- #define CYTHON_USE_TP_FINALIZE 0
- #undef CYTHON_USE_DICT_VERSIONS
- #define CYTHON_USE_DICT_VERSIONS 0
- #undef CYTHON_USE_EXC_INFO_STACK
- #define CYTHON_USE_EXC_INFO_STACK 0
-#else
- #define CYTHON_COMPILING_IN_PYPY 0
- #define CYTHON_COMPILING_IN_PYSTON 0
- #define CYTHON_COMPILING_IN_CPYTHON 1
- #ifndef CYTHON_USE_TYPE_SLOTS
- #define CYTHON_USE_TYPE_SLOTS 1
- #endif
- #if PY_VERSION_HEX < 0x02070000
- #undef CYTHON_USE_PYTYPE_LOOKUP
- #define CYTHON_USE_PYTYPE_LOOKUP 0
- #elif !defined(CYTHON_USE_PYTYPE_LOOKUP)
- #define CYTHON_USE_PYTYPE_LOOKUP 1
- #endif
- #if PY_MAJOR_VERSION < 3
- #undef CYTHON_USE_ASYNC_SLOTS
- #define CYTHON_USE_ASYNC_SLOTS 0
- #elif !defined(CYTHON_USE_ASYNC_SLOTS)
- #define CYTHON_USE_ASYNC_SLOTS 1
- #endif
- #if PY_VERSION_HEX < 0x02070000
- #undef CYTHON_USE_PYLONG_INTERNALS
- #define CYTHON_USE_PYLONG_INTERNALS 0
- #elif !defined(CYTHON_USE_PYLONG_INTERNALS)
- #define CYTHON_USE_PYLONG_INTERNALS 1
- #endif
- #ifndef CYTHON_USE_PYLIST_INTERNALS
- #define CYTHON_USE_PYLIST_INTERNALS 1
- #endif
- #ifndef CYTHON_USE_UNICODE_INTERNALS
- #define CYTHON_USE_UNICODE_INTERNALS 1
- #endif
- #if PY_VERSION_HEX < 0x030300F0
- #undef CYTHON_USE_UNICODE_WRITER
- #define CYTHON_USE_UNICODE_WRITER 0
- #elif !defined(CYTHON_USE_UNICODE_WRITER)
- #define CYTHON_USE_UNICODE_WRITER 1
- #endif
- #ifndef CYTHON_AVOID_BORROWED_REFS
- #define CYTHON_AVOID_BORROWED_REFS 0
- #endif
- #ifndef CYTHON_ASSUME_SAFE_MACROS
- #define CYTHON_ASSUME_SAFE_MACROS 1
- #endif
- #ifndef CYTHON_UNPACK_METHODS
- #define CYTHON_UNPACK_METHODS 1
- #endif
- #ifndef CYTHON_FAST_THREAD_STATE
- #define CYTHON_FAST_THREAD_STATE 1
- #endif
- #ifndef CYTHON_FAST_PYCALL
- #define CYTHON_FAST_PYCALL 1
- #endif
- #ifndef CYTHON_PEP489_MULTI_PHASE_INIT
- #define CYTHON_PEP489_MULTI_PHASE_INIT (PY_VERSION_HEX >= 0x03050000)
- #endif
- #ifndef CYTHON_USE_TP_FINALIZE
- #define CYTHON_USE_TP_FINALIZE (PY_VERSION_HEX >= 0x030400a1)
- #endif
- #ifndef CYTHON_USE_DICT_VERSIONS
- #define CYTHON_USE_DICT_VERSIONS (PY_VERSION_HEX >= 0x030600B1)
- #endif
- #ifndef CYTHON_USE_EXC_INFO_STACK
- #define CYTHON_USE_EXC_INFO_STACK (PY_VERSION_HEX >= 0x030700A3)
- #endif
-#endif
-#if !defined(CYTHON_FAST_PYCCALL)
-#define CYTHON_FAST_PYCCALL (CYTHON_FAST_PYCALL && PY_VERSION_HEX >= 0x030600B1)
-#endif
-#if CYTHON_USE_PYLONG_INTERNALS
- #include "longintrepr.h"
- #undef SHIFT
- #undef BASE
- #undef MASK
- #ifdef SIZEOF_VOID_P
- enum { __pyx_check_sizeof_voidp = 1 / (int)(SIZEOF_VOID_P == sizeof(void*)) };
- #endif
-#endif
-#ifndef __has_attribute
- #define __has_attribute(x) 0
-#endif
-#ifndef __has_cpp_attribute
- #define __has_cpp_attribute(x) 0
-#endif
-#ifndef CYTHON_RESTRICT
- #if defined(__GNUC__)
- #define CYTHON_RESTRICT __restrict__
- #elif defined(_MSC_VER) && _MSC_VER >= 1400
- #define CYTHON_RESTRICT __restrict
- #elif defined (__STDC_VERSION__) && __STDC_VERSION__ >= 199901L
- #define CYTHON_RESTRICT restrict
- #else
- #define CYTHON_RESTRICT
- #endif
-#endif
-#ifndef CYTHON_UNUSED
-# if defined(__GNUC__)
-# if !(defined(__cplusplus)) || (__GNUC__ > 3 || (__GNUC__ == 3 && __GNUC_MINOR__ >= 4))
-# define CYTHON_UNUSED __attribute__ ((__unused__))
-# else
-# define CYTHON_UNUSED
-# endif
-# elif defined(__ICC) || (defined(__INTEL_COMPILER) && !defined(_MSC_VER))
-# define CYTHON_UNUSED __attribute__ ((__unused__))
-# else
-# define CYTHON_UNUSED
-# endif
-#endif
-#ifndef CYTHON_MAYBE_UNUSED_VAR
-# if defined(__cplusplus)
- template void CYTHON_MAYBE_UNUSED_VAR( const T& ) { }
-# else
-# define CYTHON_MAYBE_UNUSED_VAR(x) (void)(x)
-# endif
-#endif
-#ifndef CYTHON_NCP_UNUSED
-# if CYTHON_COMPILING_IN_CPYTHON
-# define CYTHON_NCP_UNUSED
-# else
-# define CYTHON_NCP_UNUSED CYTHON_UNUSED
-# endif
-#endif
-#define __Pyx_void_to_None(void_result) ((void)(void_result), Py_INCREF(Py_None), Py_None)
-#ifdef _MSC_VER
- #ifndef _MSC_STDINT_H_
- #if _MSC_VER < 1300
- typedef unsigned char uint8_t;
- typedef unsigned int uint32_t;
- #else
- typedef unsigned __int8 uint8_t;
- typedef unsigned __int32 uint32_t;
- #endif
- #endif
-#else
- #include
-#endif
-#ifndef CYTHON_FALLTHROUGH
- #if defined(__cplusplus) && __cplusplus >= 201103L
- #if __has_cpp_attribute(fallthrough)
- #define CYTHON_FALLTHROUGH [[fallthrough]]
- #elif __has_cpp_attribute(clang::fallthrough)
- #define CYTHON_FALLTHROUGH [[clang::fallthrough]]
- #elif __has_cpp_attribute(gnu::fallthrough)
- #define CYTHON_FALLTHROUGH [[gnu::fallthrough]]
- #endif
- #endif
- #ifndef CYTHON_FALLTHROUGH
- #if __has_attribute(fallthrough)
- #define CYTHON_FALLTHROUGH __attribute__((fallthrough))
- #else
- #define CYTHON_FALLTHROUGH
- #endif
- #endif
- #if defined(__clang__ ) && defined(__apple_build_version__)
- #if __apple_build_version__ < 7000000
- #undef CYTHON_FALLTHROUGH
- #define CYTHON_FALLTHROUGH
- #endif
- #endif
-#endif
-
-#ifndef CYTHON_INLINE
- #if defined(__clang__)
- #define CYTHON_INLINE __inline__ __attribute__ ((__unused__))
- #elif defined(__GNUC__)
- #define CYTHON_INLINE __inline__
- #elif defined(_MSC_VER)
- #define CYTHON_INLINE __inline
- #elif defined (__STDC_VERSION__) && __STDC_VERSION__ >= 199901L
- #define CYTHON_INLINE inline
- #else
- #define CYTHON_INLINE
- #endif
-#endif
-
-#if CYTHON_COMPILING_IN_PYPY && PY_VERSION_HEX < 0x02070600 && !defined(Py_OptimizeFlag)
- #define Py_OptimizeFlag 0
-#endif
-#define __PYX_BUILD_PY_SSIZE_T "n"
-#define CYTHON_FORMAT_SSIZE_T "z"
-#if PY_MAJOR_VERSION < 3
- #define __Pyx_BUILTIN_MODULE_NAME "__builtin__"
- #define __Pyx_PyCode_New(a, k, l, s, f, code, c, n, v, fv, cell, fn, name, fline, lnos)\
- PyCode_New(a+k, l, s, f, code, c, n, v, fv, cell, fn, name, fline, lnos)
- #define __Pyx_DefaultClassType PyClass_Type
-#else
- #define __Pyx_BUILTIN_MODULE_NAME "builtins"
-#if PY_VERSION_HEX >= 0x030800A4 && PY_VERSION_HEX < 0x030800B2
- #define __Pyx_PyCode_New(a, k, l, s, f, code, c, n, v, fv, cell, fn, name, fline, lnos)\
- PyCode_New(a, 0, k, l, s, f, code, c, n, v, fv, cell, fn, name, fline, lnos)
-#else
- #define __Pyx_PyCode_New(a, k, l, s, f, code, c, n, v, fv, cell, fn, name, fline, lnos)\
- PyCode_New(a, k, l, s, f, code, c, n, v, fv, cell, fn, name, fline, lnos)
-#endif
- #define __Pyx_DefaultClassType PyType_Type
-#endif
-#ifndef Py_TPFLAGS_CHECKTYPES
- #define Py_TPFLAGS_CHECKTYPES 0
-#endif
-#ifndef Py_TPFLAGS_HAVE_INDEX
- #define Py_TPFLAGS_HAVE_INDEX 0
-#endif
-#ifndef Py_TPFLAGS_HAVE_NEWBUFFER
- #define Py_TPFLAGS_HAVE_NEWBUFFER 0
-#endif
-#ifndef Py_TPFLAGS_HAVE_FINALIZE
- #define Py_TPFLAGS_HAVE_FINALIZE 0
-#endif
-#ifndef METH_STACKLESS
- #define METH_STACKLESS 0
-#endif
-#if PY_VERSION_HEX <= 0x030700A3 || !defined(METH_FASTCALL)
- #ifndef METH_FASTCALL
- #define METH_FASTCALL 0x80
- #endif
- typedef PyObject *(*__Pyx_PyCFunctionFast) (PyObject *self, PyObject *const *args, Py_ssize_t nargs);
- typedef PyObject *(*__Pyx_PyCFunctionFastWithKeywords) (PyObject *self, PyObject *const *args,
- Py_ssize_t nargs, PyObject *kwnames);
-#else
- #define __Pyx_PyCFunctionFast _PyCFunctionFast
- #define __Pyx_PyCFunctionFastWithKeywords _PyCFunctionFastWithKeywords
-#endif
-#if CYTHON_FAST_PYCCALL
-#define __Pyx_PyFastCFunction_Check(func)\
- ((PyCFunction_Check(func) && (METH_FASTCALL == (PyCFunction_GET_FLAGS(func) & ~(METH_CLASS | METH_STATIC | METH_COEXIST | METH_KEYWORDS | METH_STACKLESS)))))
-#else
-#define __Pyx_PyFastCFunction_Check(func) 0
-#endif
-#if CYTHON_COMPILING_IN_PYPY && !defined(PyObject_Malloc)
- #define PyObject_Malloc(s) PyMem_Malloc(s)
- #define PyObject_Free(p) PyMem_Free(p)
- #define PyObject_Realloc(p) PyMem_Realloc(p)
-#endif
-#if CYTHON_COMPILING_IN_CPYTHON && PY_VERSION_HEX < 0x030400A1
- #define PyMem_RawMalloc(n) PyMem_Malloc(n)
- #define PyMem_RawRealloc(p, n) PyMem_Realloc(p, n)
- #define PyMem_RawFree(p) PyMem_Free(p)
-#endif
-#if CYTHON_COMPILING_IN_PYSTON
- #define __Pyx_PyCode_HasFreeVars(co) PyCode_HasFreeVars(co)
- #define __Pyx_PyFrame_SetLineNumber(frame, lineno) PyFrame_SetLineNumber(frame, lineno)
-#else
- #define __Pyx_PyCode_HasFreeVars(co) (PyCode_GetNumFree(co) > 0)
- #define __Pyx_PyFrame_SetLineNumber(frame, lineno) (frame)->f_lineno = (lineno)
-#endif
-#if !CYTHON_FAST_THREAD_STATE || PY_VERSION_HEX < 0x02070000
- #define __Pyx_PyThreadState_Current PyThreadState_GET()
-#elif PY_VERSION_HEX >= 0x03060000
- #define __Pyx_PyThreadState_Current _PyThreadState_UncheckedGet()
-#elif PY_VERSION_HEX >= 0x03000000
- #define __Pyx_PyThreadState_Current PyThreadState_GET()
-#else
- #define __Pyx_PyThreadState_Current _PyThreadState_Current
-#endif
-#if PY_VERSION_HEX < 0x030700A2 && !defined(PyThread_tss_create) && !defined(Py_tss_NEEDS_INIT)
-#include "pythread.h"
-#define Py_tss_NEEDS_INIT 0
-typedef int Py_tss_t;
-static CYTHON_INLINE int PyThread_tss_create(Py_tss_t *key) {
- *key = PyThread_create_key();
- return 0;
-}
-static CYTHON_INLINE Py_tss_t * PyThread_tss_alloc(void) {
- Py_tss_t *key = (Py_tss_t *)PyObject_Malloc(sizeof(Py_tss_t));
- *key = Py_tss_NEEDS_INIT;
- return key;
-}
-static CYTHON_INLINE void PyThread_tss_free(Py_tss_t *key) {
- PyObject_Free(key);
-}
-static CYTHON_INLINE int PyThread_tss_is_created(Py_tss_t *key) {
- return *key != Py_tss_NEEDS_INIT;
-}
-static CYTHON_INLINE void PyThread_tss_delete(Py_tss_t *key) {
- PyThread_delete_key(*key);
- *key = Py_tss_NEEDS_INIT;
-}
-static CYTHON_INLINE int PyThread_tss_set(Py_tss_t *key, void *value) {
- return PyThread_set_key_value(*key, value);
-}
-static CYTHON_INLINE void * PyThread_tss_get(Py_tss_t *key) {
- return PyThread_get_key_value(*key);
-}
-#endif
-#if CYTHON_COMPILING_IN_CPYTHON || defined(_PyDict_NewPresized)
-#define __Pyx_PyDict_NewPresized(n) ((n <= 8) ? PyDict_New() : _PyDict_NewPresized(n))
-#else
-#define __Pyx_PyDict_NewPresized(n) PyDict_New()
-#endif
-#if PY_MAJOR_VERSION >= 3 || CYTHON_FUTURE_DIVISION
- #define __Pyx_PyNumber_Divide(x,y) PyNumber_TrueDivide(x,y)
- #define __Pyx_PyNumber_InPlaceDivide(x,y) PyNumber_InPlaceTrueDivide(x,y)
-#else
- #define __Pyx_PyNumber_Divide(x,y) PyNumber_Divide(x,y)
- #define __Pyx_PyNumber_InPlaceDivide(x,y) PyNumber_InPlaceDivide(x,y)
-#endif
-#if CYTHON_COMPILING_IN_CPYTHON && PY_VERSION_HEX >= 0x030500A1 && CYTHON_USE_UNICODE_INTERNALS
-#define __Pyx_PyDict_GetItemStr(dict, name) _PyDict_GetItem_KnownHash(dict, name, ((PyASCIIObject *) name)->hash)
-#else
-#define __Pyx_PyDict_GetItemStr(dict, name) PyDict_GetItem(dict, name)
-#endif
-#if PY_VERSION_HEX > 0x03030000 && defined(PyUnicode_KIND)
- #define CYTHON_PEP393_ENABLED 1
- #define __Pyx_PyUnicode_READY(op) (likely(PyUnicode_IS_READY(op)) ?\
- 0 : _PyUnicode_Ready((PyObject *)(op)))
- #define __Pyx_PyUnicode_GET_LENGTH(u) PyUnicode_GET_LENGTH(u)
- #define __Pyx_PyUnicode_READ_CHAR(u, i) PyUnicode_READ_CHAR(u, i)
- #define __Pyx_PyUnicode_MAX_CHAR_VALUE(u) PyUnicode_MAX_CHAR_VALUE(u)
- #define __Pyx_PyUnicode_KIND(u) PyUnicode_KIND(u)
- #define __Pyx_PyUnicode_DATA(u) PyUnicode_DATA(u)
- #define __Pyx_PyUnicode_READ(k, d, i) PyUnicode_READ(k, d, i)
- #define __Pyx_PyUnicode_WRITE(k, d, i, ch) PyUnicode_WRITE(k, d, i, ch)
- #if defined(PyUnicode_IS_READY) && defined(PyUnicode_GET_SIZE)
- #define __Pyx_PyUnicode_IS_TRUE(u) (0 != (likely(PyUnicode_IS_READY(u)) ? PyUnicode_GET_LENGTH(u) : PyUnicode_GET_SIZE(u)))
- #else
- #define __Pyx_PyUnicode_IS_TRUE(u) (0 != PyUnicode_GET_LENGTH(u))
- #endif
-#else
- #define CYTHON_PEP393_ENABLED 0
- #define PyUnicode_1BYTE_KIND 1
- #define PyUnicode_2BYTE_KIND 2
- #define PyUnicode_4BYTE_KIND 4
- #define __Pyx_PyUnicode_READY(op) (0)
- #define __Pyx_PyUnicode_GET_LENGTH(u) PyUnicode_GET_SIZE(u)
- #define __Pyx_PyUnicode_READ_CHAR(u, i) ((Py_UCS4)(PyUnicode_AS_UNICODE(u)[i]))
- #define __Pyx_PyUnicode_MAX_CHAR_VALUE(u) ((sizeof(Py_UNICODE) == 2) ? 65535 : 1114111)
- #define __Pyx_PyUnicode_KIND(u) (sizeof(Py_UNICODE))
- #define __Pyx_PyUnicode_DATA(u) ((void*)PyUnicode_AS_UNICODE(u))
- #define __Pyx_PyUnicode_READ(k, d, i) ((void)(k), (Py_UCS4)(((Py_UNICODE*)d)[i]))
- #define __Pyx_PyUnicode_WRITE(k, d, i, ch) (((void)(k)), ((Py_UNICODE*)d)[i] = ch)
- #define __Pyx_PyUnicode_IS_TRUE(u) (0 != PyUnicode_GET_SIZE(u))
-#endif
-#if CYTHON_COMPILING_IN_PYPY
- #define __Pyx_PyUnicode_Concat(a, b) PyNumber_Add(a, b)
- #define __Pyx_PyUnicode_ConcatSafe(a, b) PyNumber_Add(a, b)
-#else
- #define __Pyx_PyUnicode_Concat(a, b) PyUnicode_Concat(a, b)
- #define __Pyx_PyUnicode_ConcatSafe(a, b) ((unlikely((a) == Py_None) || unlikely((b) == Py_None)) ?\
- PyNumber_Add(a, b) : __Pyx_PyUnicode_Concat(a, b))
-#endif
-#if CYTHON_COMPILING_IN_PYPY && !defined(PyUnicode_Contains)
- #define PyUnicode_Contains(u, s) PySequence_Contains(u, s)
-#endif
-#if CYTHON_COMPILING_IN_PYPY && !defined(PyByteArray_Check)
- #define PyByteArray_Check(obj) PyObject_TypeCheck(obj, &PyByteArray_Type)
-#endif
-#if CYTHON_COMPILING_IN_PYPY && !defined(PyObject_Format)
- #define PyObject_Format(obj, fmt) PyObject_CallMethod(obj, "__format__", "O", fmt)
-#endif
-#define __Pyx_PyString_FormatSafe(a, b) ((unlikely((a) == Py_None || (PyString_Check(b) && !PyString_CheckExact(b)))) ? PyNumber_Remainder(a, b) : __Pyx_PyString_Format(a, b))
-#define __Pyx_PyUnicode_FormatSafe(a, b) ((unlikely((a) == Py_None || (PyUnicode_Check(b) && !PyUnicode_CheckExact(b)))) ? PyNumber_Remainder(a, b) : PyUnicode_Format(a, b))
-#if PY_MAJOR_VERSION >= 3
- #define __Pyx_PyString_Format(a, b) PyUnicode_Format(a, b)
-#else
- #define __Pyx_PyString_Format(a, b) PyString_Format(a, b)
-#endif
-#if PY_MAJOR_VERSION < 3 && !defined(PyObject_ASCII)
- #define PyObject_ASCII(o) PyObject_Repr(o)
-#endif
-#if PY_MAJOR_VERSION >= 3
- #define PyBaseString_Type PyUnicode_Type
- #define PyStringObject PyUnicodeObject
- #define PyString_Type PyUnicode_Type
- #define PyString_Check PyUnicode_Check
- #define PyString_CheckExact PyUnicode_CheckExact
-#ifndef PyObject_Unicode
- #define PyObject_Unicode PyObject_Str
-#endif
-#endif
-#if PY_MAJOR_VERSION >= 3
- #define __Pyx_PyBaseString_Check(obj) PyUnicode_Check(obj)
- #define __Pyx_PyBaseString_CheckExact(obj) PyUnicode_CheckExact(obj)
-#else
- #define __Pyx_PyBaseString_Check(obj) (PyString_Check(obj) || PyUnicode_Check(obj))
- #define __Pyx_PyBaseString_CheckExact(obj) (PyString_CheckExact(obj) || PyUnicode_CheckExact(obj))
-#endif
-#ifndef PySet_CheckExact
- #define PySet_CheckExact(obj) (Py_TYPE(obj) == &PySet_Type)
-#endif
-#if PY_VERSION_HEX >= 0x030900A4
- #define __Pyx_SET_REFCNT(obj, refcnt) Py_SET_REFCNT(obj, refcnt)
- #define __Pyx_SET_SIZE(obj, size) Py_SET_SIZE(obj, size)
-#else
- #define __Pyx_SET_REFCNT(obj, refcnt) Py_REFCNT(obj) = (refcnt)
- #define __Pyx_SET_SIZE(obj, size) Py_SIZE(obj) = (size)
-#endif
-#if CYTHON_ASSUME_SAFE_MACROS
- #define __Pyx_PySequence_SIZE(seq) Py_SIZE(seq)
-#else
- #define __Pyx_PySequence_SIZE(seq) PySequence_Size(seq)
-#endif
-#if PY_MAJOR_VERSION >= 3
- #define PyIntObject PyLongObject
- #define PyInt_Type PyLong_Type
- #define PyInt_Check(op) PyLong_Check(op)
- #define PyInt_CheckExact(op) PyLong_CheckExact(op)
- #define PyInt_FromString PyLong_FromString
- #define PyInt_FromUnicode PyLong_FromUnicode
- #define PyInt_FromLong PyLong_FromLong
- #define PyInt_FromSize_t PyLong_FromSize_t
- #define PyInt_FromSsize_t PyLong_FromSsize_t
- #define PyInt_AsLong PyLong_AsLong
- #define PyInt_AS_LONG PyLong_AS_LONG
- #define PyInt_AsSsize_t PyLong_AsSsize_t
- #define PyInt_AsUnsignedLongMask PyLong_AsUnsignedLongMask
- #define PyInt_AsUnsignedLongLongMask PyLong_AsUnsignedLongLongMask
- #define PyNumber_Int PyNumber_Long
-#endif
-#if PY_MAJOR_VERSION >= 3
- #define PyBoolObject PyLongObject
-#endif
-#if PY_MAJOR_VERSION >= 3 && CYTHON_COMPILING_IN_PYPY
- #ifndef PyUnicode_InternFromString
- #define PyUnicode_InternFromString(s) PyUnicode_FromString(s)
- #endif
-#endif
-#if PY_VERSION_HEX < 0x030200A4
- typedef long Py_hash_t;
- #define __Pyx_PyInt_FromHash_t PyInt_FromLong
- #define __Pyx_PyInt_AsHash_t PyInt_AsLong
-#else
- #define __Pyx_PyInt_FromHash_t PyInt_FromSsize_t
- #define __Pyx_PyInt_AsHash_t PyInt_AsSsize_t
-#endif
-#if PY_MAJOR_VERSION >= 3
- #define __Pyx_PyMethod_New(func, self, klass) ((self) ? ((void)(klass), PyMethod_New(func, self)) : __Pyx_NewRef(func))
-#else
- #define __Pyx_PyMethod_New(func, self, klass) PyMethod_New(func, self, klass)
-#endif
-#if CYTHON_USE_ASYNC_SLOTS
- #if PY_VERSION_HEX >= 0x030500B1
- #define __Pyx_PyAsyncMethodsStruct PyAsyncMethods
- #define __Pyx_PyType_AsAsync(obj) (Py_TYPE(obj)->tp_as_async)
- #else
- #define __Pyx_PyType_AsAsync(obj) ((__Pyx_PyAsyncMethodsStruct*) (Py_TYPE(obj)->tp_reserved))
- #endif
-#else
- #define __Pyx_PyType_AsAsync(obj) NULL
-#endif
-#ifndef __Pyx_PyAsyncMethodsStruct
- typedef struct {
- unaryfunc am_await;
- unaryfunc am_aiter;
- unaryfunc am_anext;
- } __Pyx_PyAsyncMethodsStruct;
-#endif
-
-#if defined(WIN32) || defined(MS_WINDOWS)
- #define _USE_MATH_DEFINES
-#endif
-#include
-#ifdef NAN
-#define __PYX_NAN() ((float) NAN)
-#else
-static CYTHON_INLINE float __PYX_NAN() {
- float value;
- memset(&value, 0xFF, sizeof(value));
- return value;
-}
-#endif
-#if defined(__CYGWIN__) && defined(_LDBL_EQ_DBL)
-#define __Pyx_truncl trunc
-#else
-#define __Pyx_truncl truncl
-#endif
-
-#define __PYX_MARK_ERR_POS(f_index, lineno) \
- { __pyx_filename = __pyx_f[f_index]; (void)__pyx_filename; __pyx_lineno = lineno; (void)__pyx_lineno; __pyx_clineno = __LINE__; (void)__pyx_clineno; }
-#define __PYX_ERR(f_index, lineno, Ln_error) \
- { __PYX_MARK_ERR_POS(f_index, lineno) goto Ln_error; }
-
-#ifndef __PYX_EXTERN_C
- #ifdef __cplusplus
- #define __PYX_EXTERN_C extern "C"
- #else
- #define __PYX_EXTERN_C extern
- #endif
-#endif
-
-#define __PYX_HAVE__monotonic_align__core
-#define __PYX_HAVE_API__monotonic_align__core
-/* Early includes */
-#include "pythread.h"
-#include
-#include
-#include
-#include "pystate.h"
-#ifdef _OPENMP
-#include
-#endif /* _OPENMP */
-
-#if defined(PYREX_WITHOUT_ASSERTIONS) && !defined(CYTHON_WITHOUT_ASSERTIONS)
-#define CYTHON_WITHOUT_ASSERTIONS
-#endif
-
-typedef struct {PyObject **p; const char *s; const Py_ssize_t n; const char* encoding;
- const char is_unicode; const char is_str; const char intern; } __Pyx_StringTabEntry;
-
-#define __PYX_DEFAULT_STRING_ENCODING_IS_ASCII 0
-#define __PYX_DEFAULT_STRING_ENCODING_IS_UTF8 0
-#define __PYX_DEFAULT_STRING_ENCODING_IS_DEFAULT (PY_MAJOR_VERSION >= 3 && __PYX_DEFAULT_STRING_ENCODING_IS_UTF8)
-#define __PYX_DEFAULT_STRING_ENCODING ""
-#define __Pyx_PyObject_FromString __Pyx_PyBytes_FromString
-#define __Pyx_PyObject_FromStringAndSize __Pyx_PyBytes_FromStringAndSize
-#define __Pyx_uchar_cast(c) ((unsigned char)c)
-#define __Pyx_long_cast(x) ((long)x)
-#define __Pyx_fits_Py_ssize_t(v, type, is_signed) (\
- (sizeof(type) < sizeof(Py_ssize_t)) ||\
- (sizeof(type) > sizeof(Py_ssize_t) &&\
- likely(v < (type)PY_SSIZE_T_MAX ||\
- v == (type)PY_SSIZE_T_MAX) &&\
- (!is_signed || likely(v > (type)PY_SSIZE_T_MIN ||\
- v == (type)PY_SSIZE_T_MIN))) ||\
- (sizeof(type) == sizeof(Py_ssize_t) &&\
- (is_signed || likely(v < (type)PY_SSIZE_T_MAX ||\
- v == (type)PY_SSIZE_T_MAX))) )
-static CYTHON_INLINE int __Pyx_is_valid_index(Py_ssize_t i, Py_ssize_t limit) {
- return (size_t) i < (size_t) limit;
-}
-#if defined (__cplusplus) && __cplusplus >= 201103L
- #include
- #define __Pyx_sst_abs(value) std::abs(value)
-#elif SIZEOF_INT >= SIZEOF_SIZE_T
- #define __Pyx_sst_abs(value) abs(value)
-#elif SIZEOF_LONG >= SIZEOF_SIZE_T
- #define __Pyx_sst_abs(value) labs(value)
-#elif defined (_MSC_VER)
- #define __Pyx_sst_abs(value) ((Py_ssize_t)_abs64(value))
-#elif defined (__STDC_VERSION__) && __STDC_VERSION__ >= 199901L
- #define __Pyx_sst_abs(value) llabs(value)
-#elif defined (__GNUC__)
- #define __Pyx_sst_abs(value) __builtin_llabs(value)
-#else
- #define __Pyx_sst_abs(value) ((value<0) ? -value : value)
-#endif
-static CYTHON_INLINE const char* __Pyx_PyObject_AsString(PyObject*);
-static CYTHON_INLINE const char* __Pyx_PyObject_AsStringAndSize(PyObject*, Py_ssize_t* length);
-#define __Pyx_PyByteArray_FromString(s) PyByteArray_FromStringAndSize((const char*)s, strlen((const char*)s))
-#define __Pyx_PyByteArray_FromStringAndSize(s, l) PyByteArray_FromStringAndSize((const char*)s, l)
-#define __Pyx_PyBytes_FromString PyBytes_FromString
-#define __Pyx_PyBytes_FromStringAndSize PyBytes_FromStringAndSize
-static CYTHON_INLINE PyObject* __Pyx_PyUnicode_FromString(const char*);
-#if PY_MAJOR_VERSION < 3
- #define __Pyx_PyStr_FromString __Pyx_PyBytes_FromString
- #define __Pyx_PyStr_FromStringAndSize __Pyx_PyBytes_FromStringAndSize
-#else
- #define __Pyx_PyStr_FromString __Pyx_PyUnicode_FromString
- #define __Pyx_PyStr_FromStringAndSize __Pyx_PyUnicode_FromStringAndSize
-#endif
-#define __Pyx_PyBytes_AsWritableString(s) ((char*) PyBytes_AS_STRING(s))
-#define __Pyx_PyBytes_AsWritableSString(s) ((signed char*) PyBytes_AS_STRING(s))
-#define __Pyx_PyBytes_AsWritableUString(s) ((unsigned char*) PyBytes_AS_STRING(s))
-#define __Pyx_PyBytes_AsString(s) ((const char*) PyBytes_AS_STRING(s))
-#define __Pyx_PyBytes_AsSString(s) ((const signed char*) PyBytes_AS_STRING(s))
-#define __Pyx_PyBytes_AsUString(s) ((const unsigned char*) PyBytes_AS_STRING(s))
-#define __Pyx_PyObject_AsWritableString(s) ((char*) __Pyx_PyObject_AsString(s))
-#define __Pyx_PyObject_AsWritableSString(s) ((signed char*) __Pyx_PyObject_AsString(s))
-#define __Pyx_PyObject_AsWritableUString(s) ((unsigned char*) __Pyx_PyObject_AsString(s))
-#define __Pyx_PyObject_AsSString(s) ((const signed char*) __Pyx_PyObject_AsString(s))
-#define __Pyx_PyObject_AsUString(s) ((const unsigned char*) __Pyx_PyObject_AsString(s))
-#define __Pyx_PyObject_FromCString(s) __Pyx_PyObject_FromString((const char*)s)
-#define __Pyx_PyBytes_FromCString(s) __Pyx_PyBytes_FromString((const char*)s)
-#define __Pyx_PyByteArray_FromCString(s) __Pyx_PyByteArray_FromString((const char*)s)
-#define __Pyx_PyStr_FromCString(s) __Pyx_PyStr_FromString((const char*)s)
-#define __Pyx_PyUnicode_FromCString(s) __Pyx_PyUnicode_FromString((const char*)s)
-static CYTHON_INLINE size_t __Pyx_Py_UNICODE_strlen(const Py_UNICODE *u) {
- const Py_UNICODE *u_end = u;
- while (*u_end++) ;
- return (size_t)(u_end - u - 1);
-}
-#define __Pyx_PyUnicode_FromUnicode(u) PyUnicode_FromUnicode(u, __Pyx_Py_UNICODE_strlen(u))
-#define __Pyx_PyUnicode_FromUnicodeAndLength PyUnicode_FromUnicode
-#define __Pyx_PyUnicode_AsUnicode PyUnicode_AsUnicode
-#define __Pyx_NewRef(obj) (Py_INCREF(obj), obj)
-#define __Pyx_Owned_Py_None(b) __Pyx_NewRef(Py_None)
-static CYTHON_INLINE PyObject * __Pyx_PyBool_FromLong(long b);
-static CYTHON_INLINE int __Pyx_PyObject_IsTrue(PyObject*);
-static CYTHON_INLINE int __Pyx_PyObject_IsTrueAndDecref(PyObject*);
-static CYTHON_INLINE PyObject* __Pyx_PyNumber_IntOrLong(PyObject* x);
-#define __Pyx_PySequence_Tuple(obj)\
- (likely(PyTuple_CheckExact(obj)) ? __Pyx_NewRef(obj) : PySequence_Tuple(obj))
-static CYTHON_INLINE Py_ssize_t __Pyx_PyIndex_AsSsize_t(PyObject*);
-static CYTHON_INLINE PyObject * __Pyx_PyInt_FromSize_t(size_t);
-#if CYTHON_ASSUME_SAFE_MACROS
-#define __pyx_PyFloat_AsDouble(x) (PyFloat_CheckExact(x) ? PyFloat_AS_DOUBLE(x) : PyFloat_AsDouble(x))
-#else
-#define __pyx_PyFloat_AsDouble(x) PyFloat_AsDouble(x)
-#endif
-#define __pyx_PyFloat_AsFloat(x) ((float) __pyx_PyFloat_AsDouble(x))
-#if PY_MAJOR_VERSION >= 3
-#define __Pyx_PyNumber_Int(x) (PyLong_CheckExact(x) ? __Pyx_NewRef(x) : PyNumber_Long(x))
-#else
-#define __Pyx_PyNumber_Int(x) (PyInt_CheckExact(x) ? __Pyx_NewRef(x) : PyNumber_Int(x))
-#endif
-#define __Pyx_PyNumber_Float(x) (PyFloat_CheckExact(x) ? __Pyx_NewRef(x) : PyNumber_Float(x))
-#if PY_MAJOR_VERSION < 3 && __PYX_DEFAULT_STRING_ENCODING_IS_ASCII
-static int __Pyx_sys_getdefaultencoding_not_ascii;
-static int __Pyx_init_sys_getdefaultencoding_params(void) {
- PyObject* sys;
- PyObject* default_encoding = NULL;
- PyObject* ascii_chars_u = NULL;
- PyObject* ascii_chars_b = NULL;
- const char* default_encoding_c;
- sys = PyImport_ImportModule("sys");
- if (!sys) goto bad;
- default_encoding = PyObject_CallMethod(sys, (char*) "getdefaultencoding", NULL);
- Py_DECREF(sys);
- if (!default_encoding) goto bad;
- default_encoding_c = PyBytes_AsString(default_encoding);
- if (!default_encoding_c) goto bad;
- if (strcmp(default_encoding_c, "ascii") == 0) {
- __Pyx_sys_getdefaultencoding_not_ascii = 0;
- } else {
- char ascii_chars[128];
- int c;
- for (c = 0; c < 128; c++) {
- ascii_chars[c] = c;
- }
- __Pyx_sys_getdefaultencoding_not_ascii = 1;
- ascii_chars_u = PyUnicode_DecodeASCII(ascii_chars, 128, NULL);
- if (!ascii_chars_u) goto bad;
- ascii_chars_b = PyUnicode_AsEncodedString(ascii_chars_u, default_encoding_c, NULL);
- if (!ascii_chars_b || !PyBytes_Check(ascii_chars_b) || memcmp(ascii_chars, PyBytes_AS_STRING(ascii_chars_b), 128) != 0) {
- PyErr_Format(
- PyExc_ValueError,
- "This module compiled with c_string_encoding=ascii, but default encoding '%.200s' is not a superset of ascii.",
- default_encoding_c);
- goto bad;
- }
- Py_DECREF(ascii_chars_u);
- Py_DECREF(ascii_chars_b);
- }
- Py_DECREF(default_encoding);
- return 0;
-bad:
- Py_XDECREF(default_encoding);
- Py_XDECREF(ascii_chars_u);
- Py_XDECREF(ascii_chars_b);
- return -1;
-}
-#endif
-#if __PYX_DEFAULT_STRING_ENCODING_IS_DEFAULT && PY_MAJOR_VERSION >= 3
-#define __Pyx_PyUnicode_FromStringAndSize(c_str, size) PyUnicode_DecodeUTF8(c_str, size, NULL)
-#else
-#define __Pyx_PyUnicode_FromStringAndSize(c_str, size) PyUnicode_Decode(c_str, size, __PYX_DEFAULT_STRING_ENCODING, NULL)
-#if __PYX_DEFAULT_STRING_ENCODING_IS_DEFAULT
-static char* __PYX_DEFAULT_STRING_ENCODING;
-static int __Pyx_init_sys_getdefaultencoding_params(void) {
- PyObject* sys;
- PyObject* default_encoding = NULL;
- char* default_encoding_c;
- sys = PyImport_ImportModule("sys");
- if (!sys) goto bad;
- default_encoding = PyObject_CallMethod(sys, (char*) (const char*) "getdefaultencoding", NULL);
- Py_DECREF(sys);
- if (!default_encoding) goto bad;
- default_encoding_c = PyBytes_AsString(default_encoding);
- if (!default_encoding_c) goto bad;
- __PYX_DEFAULT_STRING_ENCODING = (char*) malloc(strlen(default_encoding_c) + 1);
- if (!__PYX_DEFAULT_STRING_ENCODING) goto bad;
- strcpy(__PYX_DEFAULT_STRING_ENCODING, default_encoding_c);
- Py_DECREF(default_encoding);
- return 0;
-bad:
- Py_XDECREF(default_encoding);
- return -1;
-}
-#endif
-#endif
-
-
-/* Test for GCC > 2.95 */
-#if defined(__GNUC__) && (__GNUC__ > 2 || (__GNUC__ == 2 && (__GNUC_MINOR__ > 95)))
- #define likely(x) __builtin_expect(!!(x), 1)
- #define unlikely(x) __builtin_expect(!!(x), 0)
-#else /* !__GNUC__ or GCC < 2.95 */
- #define likely(x) (x)
- #define unlikely(x) (x)
-#endif /* __GNUC__ */
-static CYTHON_INLINE void __Pyx_pretend_to_initialize(void* ptr) { (void)ptr; }
-
-static PyObject *__pyx_m = NULL;
-static PyObject *__pyx_d;
-static PyObject *__pyx_b;
-static PyObject *__pyx_cython_runtime = NULL;
-static PyObject *__pyx_empty_tuple;
-static PyObject *__pyx_empty_bytes;
-static PyObject *__pyx_empty_unicode;
-static int __pyx_lineno;
-static int __pyx_clineno = 0;
-static const char * __pyx_cfilenm= __FILE__;
-static const char *__pyx_filename;
-
-
-static const char *__pyx_f[] = {
- "core.pyx",
- "stringsource",
-};
-/* NoFastGil.proto */
-#define __Pyx_PyGILState_Ensure PyGILState_Ensure
-#define __Pyx_PyGILState_Release PyGILState_Release
-#define __Pyx_FastGIL_Remember()
-#define __Pyx_FastGIL_Forget()
-#define __Pyx_FastGilFuncInit()
-
-/* MemviewSliceStruct.proto */
-struct __pyx_memoryview_obj;
-typedef struct {
- struct __pyx_memoryview_obj *memview;
- char *data;
- Py_ssize_t shape[8];
- Py_ssize_t strides[8];
- Py_ssize_t suboffsets[8];
-} __Pyx_memviewslice;
-#define __Pyx_MemoryView_Len(m) (m.shape[0])
-
-/* Atomics.proto */
-#include
-#ifndef CYTHON_ATOMICS
- #define CYTHON_ATOMICS 1
-#endif
-#define __pyx_atomic_int_type int
-#if CYTHON_ATOMICS && __GNUC__ >= 4 && (__GNUC_MINOR__ > 1 ||\
- (__GNUC_MINOR__ == 1 && __GNUC_PATCHLEVEL >= 2)) &&\
- !defined(__i386__)
- #define __pyx_atomic_incr_aligned(value, lock) __sync_fetch_and_add(value, 1)
- #define __pyx_atomic_decr_aligned(value, lock) __sync_fetch_and_sub(value, 1)
- #ifdef __PYX_DEBUG_ATOMICS
- #warning "Using GNU atomics"
- #endif
-#elif CYTHON_ATOMICS && defined(_MSC_VER) && 0
- #include
- #undef __pyx_atomic_int_type
- #define __pyx_atomic_int_type LONG
- #define __pyx_atomic_incr_aligned(value, lock) InterlockedIncrement(value)
- #define __pyx_atomic_decr_aligned(value, lock) InterlockedDecrement(value)
- #ifdef __PYX_DEBUG_ATOMICS
- #pragma message ("Using MSVC atomics")
- #endif
-#elif CYTHON_ATOMICS && (defined(__ICC) || defined(__INTEL_COMPILER)) && 0
- #define __pyx_atomic_incr_aligned(value, lock) _InterlockedIncrement(value)
- #define __pyx_atomic_decr_aligned(value, lock) _InterlockedDecrement(value)
- #ifdef __PYX_DEBUG_ATOMICS
- #warning "Using Intel atomics"
- #endif
-#else
- #undef CYTHON_ATOMICS
- #define CYTHON_ATOMICS 0
- #ifdef __PYX_DEBUG_ATOMICS
- #warning "Not using atomics"
- #endif
-#endif
-typedef volatile __pyx_atomic_int_type __pyx_atomic_int;
-#if CYTHON_ATOMICS
- #define __pyx_add_acquisition_count(memview)\
- __pyx_atomic_incr_aligned(__pyx_get_slice_count_pointer(memview), memview->lock)
- #define __pyx_sub_acquisition_count(memview)\
- __pyx_atomic_decr_aligned(__pyx_get_slice_count_pointer(memview), memview->lock)
-#else
- #define __pyx_add_acquisition_count(memview)\
- __pyx_add_acquisition_count_locked(__pyx_get_slice_count_pointer(memview), memview->lock)
- #define __pyx_sub_acquisition_count(memview)\
- __pyx_sub_acquisition_count_locked(__pyx_get_slice_count_pointer(memview), memview->lock)
-#endif
-
-/* ForceInitThreads.proto */
-#ifndef __PYX_FORCE_INIT_THREADS
- #define __PYX_FORCE_INIT_THREADS 0
-#endif
-
-/* BufferFormatStructs.proto */
-#define IS_UNSIGNED(type) (((type) -1) > 0)
-struct __Pyx_StructField_;
-#define __PYX_BUF_FLAGS_PACKED_STRUCT (1 << 0)
-typedef struct {
- const char* name;
- struct __Pyx_StructField_* fields;
- size_t size;
- size_t arraysize[8];
- int ndim;
- char typegroup;
- char is_unsigned;
- int flags;
-} __Pyx_TypeInfo;
-typedef struct __Pyx_StructField_ {
- __Pyx_TypeInfo* type;
- const char* name;
- size_t offset;
-} __Pyx_StructField;
-typedef struct {
- __Pyx_StructField* field;
- size_t parent_offset;
-} __Pyx_BufFmt_StackElem;
-typedef struct {
- __Pyx_StructField root;
- __Pyx_BufFmt_StackElem* head;
- size_t fmt_offset;
- size_t new_count, enc_count;
- size_t struct_alignment;
- int is_complex;
- char enc_type;
- char new_packmode;
- char enc_packmode;
- char is_valid_array;
-} __Pyx_BufFmt_Context;
-
-
-/*--- Type declarations ---*/
-struct __pyx_array_obj;
-struct __pyx_MemviewEnum_obj;
-struct __pyx_memoryview_obj;
-struct __pyx_memoryviewslice_obj;
-struct __pyx_opt_args_15monotonic_align_4core_maximum_path_each;
-
-/* "monotonic_align/core.pyx":7
- * @cython.boundscheck(False)
- * @cython.wraparound(False)
- * cdef void maximum_path_each(int[:,::1] path, float[:,::1] value, int t_y, int t_x, float max_neg_val=-1e9) nogil: # <<<<<<<<<<<<<<
- * cdef int x
- * cdef int y
- */
-struct __pyx_opt_args_15monotonic_align_4core_maximum_path_each {
- int __pyx_n;
- float max_neg_val;
-};
-
-/* "View.MemoryView":105
- *
- * @cname("__pyx_array")
- * cdef class array: # <<<<<<<<<<<<<<
- *
- * cdef:
- */
-struct __pyx_array_obj {
- PyObject_HEAD
- struct __pyx_vtabstruct_array *__pyx_vtab;
- char *data;
- Py_ssize_t len;
- char *format;
- int ndim;
- Py_ssize_t *_shape;
- Py_ssize_t *_strides;
- Py_ssize_t itemsize;
- PyObject *mode;
- PyObject *_format;
- void (*callback_free_data)(void *);
- int free_data;
- int dtype_is_object;
-};
-
-
-/* "View.MemoryView":279
- *
- * @cname('__pyx_MemviewEnum')
- * cdef class Enum(object): # <<<<<<<<<<<<<<
- * cdef object name
- * def __init__(self, name):
- */
-struct __pyx_MemviewEnum_obj {
- PyObject_HEAD
- PyObject *name;
-};
-
-
-/* "View.MemoryView":330
- *
- * @cname('__pyx_memoryview')
- * cdef class memoryview(object): # <<<<<<<<<<<<<<
- *
- * cdef object obj
- */
-struct __pyx_memoryview_obj {
- PyObject_HEAD
- struct __pyx_vtabstruct_memoryview *__pyx_vtab;
- PyObject *obj;
- PyObject *_size;
- PyObject *_array_interface;
- PyThread_type_lock lock;
- __pyx_atomic_int acquisition_count[2];
- __pyx_atomic_int *acquisition_count_aligned_p;
- Py_buffer view;
- int flags;
- int dtype_is_object;
- __Pyx_TypeInfo *typeinfo;
-};
-
-
-/* "View.MemoryView":965
- *
- * @cname('__pyx_memoryviewslice')
- * cdef class _memoryviewslice(memoryview): # <<<<<<<<<<<<<<
- * "Internal class for passing memoryview slices to Python"
- *
- */
-struct __pyx_memoryviewslice_obj {
- struct __pyx_memoryview_obj __pyx_base;
- __Pyx_memviewslice from_slice;
- PyObject *from_object;
- PyObject *(*to_object_func)(char *);
- int (*to_dtype_func)(char *, PyObject *);
-};
-
-
-
-/* "View.MemoryView":105
- *
- * @cname("__pyx_array")
- * cdef class array: # <<<<<<<<<<<<<<
- *
- * cdef:
- */
-
-struct __pyx_vtabstruct_array {
- PyObject *(*get_memview)(struct __pyx_array_obj *);
-};
-static struct __pyx_vtabstruct_array *__pyx_vtabptr_array;
-
-
-/* "View.MemoryView":330
- *
- * @cname('__pyx_memoryview')
- * cdef class memoryview(object): # <<<<<<<<<<<<<<
- *
- * cdef object obj
- */
-
-struct __pyx_vtabstruct_memoryview {
- char *(*get_item_pointer)(struct __pyx_memoryview_obj *, PyObject *);
- PyObject *(*is_slice)(struct __pyx_memoryview_obj *, PyObject *);
- PyObject *(*setitem_slice_assignment)(struct __pyx_memoryview_obj *, PyObject *, PyObject *);
- PyObject *(*setitem_slice_assign_scalar)(struct __pyx_memoryview_obj *, struct __pyx_memoryview_obj *, PyObject *);
- PyObject *(*setitem_indexed)(struct __pyx_memoryview_obj *, PyObject *, PyObject *);
- PyObject *(*convert_item_to_object)(struct __pyx_memoryview_obj *, char *);
- PyObject *(*assign_item_from_object)(struct __pyx_memoryview_obj *, char *, PyObject *);
-};
-static struct __pyx_vtabstruct_memoryview *__pyx_vtabptr_memoryview;
-
-
-/* "View.MemoryView":965
- *
- * @cname('__pyx_memoryviewslice')
- * cdef class _memoryviewslice(memoryview): # <<<<<<<<<<<<<<
- * "Internal class for passing memoryview slices to Python"
- *
- */
-
-struct __pyx_vtabstruct__memoryviewslice {
- struct __pyx_vtabstruct_memoryview __pyx_base;
-};
-static struct __pyx_vtabstruct__memoryviewslice *__pyx_vtabptr__memoryviewslice;
-
-/* --- Runtime support code (head) --- */
-/* Refnanny.proto */
-#ifndef CYTHON_REFNANNY
- #define CYTHON_REFNANNY 0
-#endif
-#if CYTHON_REFNANNY
- typedef struct {
- void (*INCREF)(void*, PyObject*, int);
- void (*DECREF)(void*, PyObject*, int);
- void (*GOTREF)(void*, PyObject*, int);
- void (*GIVEREF)(void*, PyObject*, int);
- void* (*SetupContext)(const char*, int, const char*);
- void (*FinishContext)(void**);
- } __Pyx_RefNannyAPIStruct;
- static __Pyx_RefNannyAPIStruct *__Pyx_RefNanny = NULL;
- static __Pyx_RefNannyAPIStruct *__Pyx_RefNannyImportAPI(const char *modname);
- #define __Pyx_RefNannyDeclarations void *__pyx_refnanny = NULL;
-#ifdef WITH_THREAD
- #define __Pyx_RefNannySetupContext(name, acquire_gil)\
- if (acquire_gil) {\
- PyGILState_STATE __pyx_gilstate_save = PyGILState_Ensure();\
- __pyx_refnanny = __Pyx_RefNanny->SetupContext((name), __LINE__, __FILE__);\
- PyGILState_Release(__pyx_gilstate_save);\
- } else {\
- __pyx_refnanny = __Pyx_RefNanny->SetupContext((name), __LINE__, __FILE__);\
- }
-#else
- #define __Pyx_RefNannySetupContext(name, acquire_gil)\
- __pyx_refnanny = __Pyx_RefNanny->SetupContext((name), __LINE__, __FILE__)
-#endif
- #define __Pyx_RefNannyFinishContext()\
- __Pyx_RefNanny->FinishContext(&__pyx_refnanny)
- #define __Pyx_INCREF(r) __Pyx_RefNanny->INCREF(__pyx_refnanny, (PyObject *)(r), __LINE__)
- #define __Pyx_DECREF(r) __Pyx_RefNanny->DECREF(__pyx_refnanny, (PyObject *)(r), __LINE__)
- #define __Pyx_GOTREF(r) __Pyx_RefNanny->GOTREF(__pyx_refnanny, (PyObject *)(r), __LINE__)
- #define __Pyx_GIVEREF(r) __Pyx_RefNanny->GIVEREF(__pyx_refnanny, (PyObject *)(r), __LINE__)
- #define __Pyx_XINCREF(r) do { if((r) != NULL) {__Pyx_INCREF(r); }} while(0)
- #define __Pyx_XDECREF(r) do { if((r) != NULL) {__Pyx_DECREF(r); }} while(0)
- #define __Pyx_XGOTREF(r) do { if((r) != NULL) {__Pyx_GOTREF(r); }} while(0)
- #define __Pyx_XGIVEREF(r) do { if((r) != NULL) {__Pyx_GIVEREF(r);}} while(0)
-#else
- #define __Pyx_RefNannyDeclarations
- #define __Pyx_RefNannySetupContext(name, acquire_gil)
- #define __Pyx_RefNannyFinishContext()
- #define __Pyx_INCREF(r) Py_INCREF(r)
- #define __Pyx_DECREF(r) Py_DECREF(r)
- #define __Pyx_GOTREF(r)
- #define __Pyx_GIVEREF(r)
- #define __Pyx_XINCREF(r) Py_XINCREF(r)
- #define __Pyx_XDECREF(r) Py_XDECREF(r)
- #define __Pyx_XGOTREF(r)
- #define __Pyx_XGIVEREF(r)
-#endif
-#define __Pyx_XDECREF_SET(r, v) do {\
- PyObject *tmp = (PyObject *) r;\
- r = v; __Pyx_XDECREF(tmp);\
- } while (0)
-#define __Pyx_DECREF_SET(r, v) do {\
- PyObject *tmp = (PyObject *) r;\
- r = v; __Pyx_DECREF(tmp);\
- } while (0)
-#define __Pyx_CLEAR(r) do { PyObject* tmp = ((PyObject*)(r)); r = NULL; __Pyx_DECREF(tmp);} while(0)
-#define __Pyx_XCLEAR(r) do { if((r) != NULL) {PyObject* tmp = ((PyObject*)(r)); r = NULL; __Pyx_DECREF(tmp);}} while(0)
-
-/* PyObjectGetAttrStr.proto */
-#if CYTHON_USE_TYPE_SLOTS
-static CYTHON_INLINE PyObject* __Pyx_PyObject_GetAttrStr(PyObject* obj, PyObject* attr_name);
-#else
-#define __Pyx_PyObject_GetAttrStr(o,n) PyObject_GetAttr(o,n)
-#endif
-
-/* GetBuiltinName.proto */
-static PyObject *__Pyx_GetBuiltinName(PyObject *name);
-
-/* MemviewSliceInit.proto */
-#define __Pyx_BUF_MAX_NDIMS %(BUF_MAX_NDIMS)d
-#define __Pyx_MEMVIEW_DIRECT 1
-#define __Pyx_MEMVIEW_PTR 2
-#define __Pyx_MEMVIEW_FULL 4
-#define __Pyx_MEMVIEW_CONTIG 8
-#define __Pyx_MEMVIEW_STRIDED 16
-#define __Pyx_MEMVIEW_FOLLOW 32
-#define __Pyx_IS_C_CONTIG 1
-#define __Pyx_IS_F_CONTIG 2
-static int __Pyx_init_memviewslice(
- struct __pyx_memoryview_obj *memview,
- int ndim,
- __Pyx_memviewslice *memviewslice,
- int memview_is_new_reference);
-static CYTHON_INLINE int __pyx_add_acquisition_count_locked(
- __pyx_atomic_int *acquisition_count, PyThread_type_lock lock);
-static CYTHON_INLINE int __pyx_sub_acquisition_count_locked(
- __pyx_atomic_int *acquisition_count, PyThread_type_lock lock);
-#define __pyx_get_slice_count_pointer(memview) (memview->acquisition_count_aligned_p)
-#define __pyx_get_slice_count(memview) (*__pyx_get_slice_count_pointer(memview))
-#define __PYX_INC_MEMVIEW(slice, have_gil) __Pyx_INC_MEMVIEW(slice, have_gil, __LINE__)
-#define __PYX_XDEC_MEMVIEW(slice, have_gil) __Pyx_XDEC_MEMVIEW(slice, have_gil, __LINE__)
-static CYTHON_INLINE void __Pyx_INC_MEMVIEW(__Pyx_memviewslice *, int, int);
-static CYTHON_INLINE void __Pyx_XDEC_MEMVIEW(__Pyx_memviewslice *, int, int);
-
-/* RaiseArgTupleInvalid.proto */
-static void __Pyx_RaiseArgtupleInvalid(const char* func_name, int exact,
- Py_ssize_t num_min, Py_ssize_t num_max, Py_ssize_t num_found);
-
-/* RaiseDoubleKeywords.proto */
-static void __Pyx_RaiseDoubleKeywordsError(const char* func_name, PyObject* kw_name);
-
-/* ParseKeywords.proto */
-static int __Pyx_ParseOptionalKeywords(PyObject *kwds, PyObject **argnames[],\
- PyObject *kwds2, PyObject *values[], Py_ssize_t num_pos_args,\
- const char* function_name);
-
-/* None.proto */
-static CYTHON_INLINE void __Pyx_RaiseUnboundLocalError(const char *varname);
-
-/* ArgTypeTest.proto */
-#define __Pyx_ArgTypeTest(obj, type, none_allowed, name, exact)\
- ((likely((Py_TYPE(obj) == type) | (none_allowed && (obj == Py_None)))) ? 1 :\
- __Pyx__ArgTypeTest(obj, type, name, exact))
-static int __Pyx__ArgTypeTest(PyObject *obj, PyTypeObject *type, const char *name, int exact);
-
-/* PyObjectCall.proto */
-#if CYTHON_COMPILING_IN_CPYTHON
-static CYTHON_INLINE PyObject* __Pyx_PyObject_Call(PyObject *func, PyObject *arg, PyObject *kw);
-#else
-#define __Pyx_PyObject_Call(func, arg, kw) PyObject_Call(func, arg, kw)
-#endif
-
-/* PyThreadStateGet.proto */
-#if CYTHON_FAST_THREAD_STATE
-#define __Pyx_PyThreadState_declare PyThreadState *__pyx_tstate;
-#define __Pyx_PyThreadState_assign __pyx_tstate = __Pyx_PyThreadState_Current;
-#define __Pyx_PyErr_Occurred() __pyx_tstate->curexc_type
-#else
-#define __Pyx_PyThreadState_declare
-#define __Pyx_PyThreadState_assign
-#define __Pyx_PyErr_Occurred() PyErr_Occurred()
-#endif
-
-/* PyErrFetchRestore.proto */
-#if CYTHON_FAST_THREAD_STATE
-#define __Pyx_PyErr_Clear() __Pyx_ErrRestore(NULL, NULL, NULL)
-#define __Pyx_ErrRestoreWithState(type, value, tb) __Pyx_ErrRestoreInState(PyThreadState_GET(), type, value, tb)
-#define __Pyx_ErrFetchWithState(type, value, tb) __Pyx_ErrFetchInState(PyThreadState_GET(), type, value, tb)
-#define __Pyx_ErrRestore(type, value, tb) __Pyx_ErrRestoreInState(__pyx_tstate, type, value, tb)
-#define __Pyx_ErrFetch(type, value, tb) __Pyx_ErrFetchInState(__pyx_tstate, type, value, tb)
-static CYTHON_INLINE void __Pyx_ErrRestoreInState(PyThreadState *tstate, PyObject *type, PyObject *value, PyObject *tb);
-static CYTHON_INLINE void __Pyx_ErrFetchInState(PyThreadState *tstate, PyObject **type, PyObject **value, PyObject **tb);
-#if CYTHON_COMPILING_IN_CPYTHON
-#define __Pyx_PyErr_SetNone(exc) (Py_INCREF(exc), __Pyx_ErrRestore((exc), NULL, NULL))
-#else
-#define __Pyx_PyErr_SetNone(exc) PyErr_SetNone(exc)
-#endif
-#else
-#define __Pyx_PyErr_Clear() PyErr_Clear()
-#define __Pyx_PyErr_SetNone(exc) PyErr_SetNone(exc)
-#define __Pyx_ErrRestoreWithState(type, value, tb) PyErr_Restore(type, value, tb)
-#define __Pyx_ErrFetchWithState(type, value, tb) PyErr_Fetch(type, value, tb)
-#define __Pyx_ErrRestoreInState(tstate, type, value, tb) PyErr_Restore(type, value, tb)
-#define __Pyx_ErrFetchInState(tstate, type, value, tb) PyErr_Fetch(type, value, tb)
-#define __Pyx_ErrRestore(type, value, tb) PyErr_Restore(type, value, tb)
-#define __Pyx_ErrFetch(type, value, tb) PyErr_Fetch(type, value, tb)
-#endif
-
-/* RaiseException.proto */
-static void __Pyx_Raise(PyObject *type, PyObject *value, PyObject *tb, PyObject *cause);
-
-/* PyCFunctionFastCall.proto */
-#if CYTHON_FAST_PYCCALL
-static CYTHON_INLINE PyObject *__Pyx_PyCFunction_FastCall(PyObject *func, PyObject **args, Py_ssize_t nargs);
-#else
-#define __Pyx_PyCFunction_FastCall(func, args, nargs) (assert(0), NULL)
-#endif
-
-/* PyFunctionFastCall.proto */
-#if CYTHON_FAST_PYCALL
-#define __Pyx_PyFunction_FastCall(func, args, nargs)\
- __Pyx_PyFunction_FastCallDict((func), (args), (nargs), NULL)
-#if 1 || PY_VERSION_HEX < 0x030600B1
-static PyObject *__Pyx_PyFunction_FastCallDict(PyObject *func, PyObject **args, Py_ssize_t nargs, PyObject *kwargs);
-#else
-#define __Pyx_PyFunction_FastCallDict(func, args, nargs, kwargs) _PyFunction_FastCallDict(func, args, nargs, kwargs)
-#endif
-#define __Pyx_BUILD_ASSERT_EXPR(cond)\
- (sizeof(char [1 - 2*!(cond)]) - 1)
-#ifndef Py_MEMBER_SIZE
-#define Py_MEMBER_SIZE(type, member) sizeof(((type *)0)->member)
-#endif
- static size_t __pyx_pyframe_localsplus_offset = 0;
- #include "frameobject.h"
- #define __Pxy_PyFrame_Initialize_Offsets()\
- ((void)__Pyx_BUILD_ASSERT_EXPR(sizeof(PyFrameObject) == offsetof(PyFrameObject, f_localsplus) + Py_MEMBER_SIZE(PyFrameObject, f_localsplus)),\
- (void)(__pyx_pyframe_localsplus_offset = ((size_t)PyFrame_Type.tp_basicsize) - Py_MEMBER_SIZE(PyFrameObject, f_localsplus)))
- #define __Pyx_PyFrame_GetLocalsplus(frame)\
- (assert(__pyx_pyframe_localsplus_offset), (PyObject **)(((char *)(frame)) + __pyx_pyframe_localsplus_offset))
-#endif
-
-/* PyObjectCall2Args.proto */
-static CYTHON_UNUSED PyObject* __Pyx_PyObject_Call2Args(PyObject* function, PyObject* arg1, PyObject* arg2);
-
-/* PyObjectCallMethO.proto */
-#if CYTHON_COMPILING_IN_CPYTHON
-static CYTHON_INLINE PyObject* __Pyx_PyObject_CallMethO(PyObject *func, PyObject *arg);
-#endif
-
-/* PyObjectCallOneArg.proto */
-static CYTHON_INLINE PyObject* __Pyx_PyObject_CallOneArg(PyObject *func, PyObject *arg);
-
-/* IncludeStringH.proto */
-#include
-
-/* BytesEquals.proto */
-static CYTHON_INLINE int __Pyx_PyBytes_Equals(PyObject* s1, PyObject* s2, int equals);
-
-/* UnicodeEquals.proto */
-static CYTHON_INLINE int __Pyx_PyUnicode_Equals(PyObject* s1, PyObject* s2, int equals);
-
-/* StrEquals.proto */
-#if PY_MAJOR_VERSION >= 3
-#define __Pyx_PyString_Equals __Pyx_PyUnicode_Equals
-#else
-#define __Pyx_PyString_Equals __Pyx_PyBytes_Equals
-#endif
-
-/* None.proto */
-static CYTHON_INLINE Py_ssize_t __Pyx_div_Py_ssize_t(Py_ssize_t, Py_ssize_t);
-
-/* UnaryNegOverflows.proto */
-#define UNARY_NEG_WOULD_OVERFLOW(x)\
- (((x) < 0) & ((unsigned long)(x) == 0-(unsigned long)(x)))
-
-static CYTHON_UNUSED int __pyx_array_getbuffer(PyObject *__pyx_v_self, Py_buffer *__pyx_v_info, int __pyx_v_flags); /*proto*/
-static PyObject *__pyx_array_get_memview(struct __pyx_array_obj *); /*proto*/
-/* GetAttr.proto */
-static CYTHON_INLINE PyObject *__Pyx_GetAttr(PyObject *, PyObject *);
-
-/* GetItemInt.proto */
-#define __Pyx_GetItemInt(o, i, type, is_signed, to_py_func, is_list, wraparound, boundscheck)\
- (__Pyx_fits_Py_ssize_t(i, type, is_signed) ?\
- __Pyx_GetItemInt_Fast(o, (Py_ssize_t)i, is_list, wraparound, boundscheck) :\
- (is_list ? (PyErr_SetString(PyExc_IndexError, "list index out of range"), (PyObject*)NULL) :\
- __Pyx_GetItemInt_Generic(o, to_py_func(i))))
-#define __Pyx_GetItemInt_List(o, i, type, is_signed, to_py_func, is_list, wraparound, boundscheck)\
- (__Pyx_fits_Py_ssize_t(i, type, is_signed) ?\
- __Pyx_GetItemInt_List_Fast(o, (Py_ssize_t)i, wraparound, boundscheck) :\
- (PyErr_SetString(PyExc_IndexError, "list index out of range"), (PyObject*)NULL))
-static CYTHON_INLINE PyObject *__Pyx_GetItemInt_List_Fast(PyObject *o, Py_ssize_t i,
- int wraparound, int boundscheck);
-#define __Pyx_GetItemInt_Tuple(o, i, type, is_signed, to_py_func, is_list, wraparound, boundscheck)\
- (__Pyx_fits_Py_ssize_t(i, type, is_signed) ?\
- __Pyx_GetItemInt_Tuple_Fast(o, (Py_ssize_t)i, wraparound, boundscheck) :\
- (PyErr_SetString(PyExc_IndexError, "tuple index out of range"), (PyObject*)NULL))
-static CYTHON_INLINE PyObject *__Pyx_GetItemInt_Tuple_Fast(PyObject *o, Py_ssize_t i,
- int wraparound, int boundscheck);
-static PyObject *__Pyx_GetItemInt_Generic(PyObject *o, PyObject* j);
-static CYTHON_INLINE PyObject *__Pyx_GetItemInt_Fast(PyObject *o, Py_ssize_t i,
- int is_list, int wraparound, int boundscheck);
-
-/* ObjectGetItem.proto */
-#if CYTHON_USE_TYPE_SLOTS
-static CYTHON_INLINE PyObject *__Pyx_PyObject_GetItem(PyObject *obj, PyObject* key);
-#else
-#define __Pyx_PyObject_GetItem(obj, key) PyObject_GetItem(obj, key)
-#endif
-
-/* decode_c_string_utf16.proto */
-static CYTHON_INLINE PyObject *__Pyx_PyUnicode_DecodeUTF16(const char *s, Py_ssize_t size, const char *errors) {
- int byteorder = 0;
- return PyUnicode_DecodeUTF16(s, size, errors, &byteorder);
-}
-static CYTHON_INLINE PyObject *__Pyx_PyUnicode_DecodeUTF16LE(const char *s, Py_ssize_t size, const char *errors) {
- int byteorder = -1;
- return PyUnicode_DecodeUTF16(s, size, errors, &byteorder);
-}
-static CYTHON_INLINE PyObject *__Pyx_PyUnicode_DecodeUTF16BE(const char *s, Py_ssize_t size, const char *errors) {
- int byteorder = 1;
- return PyUnicode_DecodeUTF16(s, size, errors, &byteorder);
-}
-
-/* decode_c_string.proto */
-static CYTHON_INLINE PyObject* __Pyx_decode_c_string(
- const char* cstring, Py_ssize_t start, Py_ssize_t stop,
- const char* encoding, const char* errors,
- PyObject* (*decode_func)(const char *s, Py_ssize_t size, const char *errors));
-
-/* PyErrExceptionMatches.proto */
-#if CYTHON_FAST_THREAD_STATE
-#define __Pyx_PyErr_ExceptionMatches(err) __Pyx_PyErr_ExceptionMatchesInState(__pyx_tstate, err)
-static CYTHON_INLINE int __Pyx_PyErr_ExceptionMatchesInState(PyThreadState* tstate, PyObject* err);
-#else
-#define __Pyx_PyErr_ExceptionMatches(err) PyErr_ExceptionMatches(err)
-#endif
-
-/* GetAttr3.proto */
-static CYTHON_INLINE PyObject *__Pyx_GetAttr3(PyObject *, PyObject *, PyObject *);
-
-/* PyDictVersioning.proto */
-#if CYTHON_USE_DICT_VERSIONS && CYTHON_USE_TYPE_SLOTS
-#define __PYX_DICT_VERSION_INIT ((PY_UINT64_T) -1)
-#define __PYX_GET_DICT_VERSION(dict) (((PyDictObject*)(dict))->ma_version_tag)
-#define __PYX_UPDATE_DICT_CACHE(dict, value, cache_var, version_var)\
- (version_var) = __PYX_GET_DICT_VERSION(dict);\
- (cache_var) = (value);
-#define __PYX_PY_DICT_LOOKUP_IF_MODIFIED(VAR, DICT, LOOKUP) {\
- static PY_UINT64_T __pyx_dict_version = 0;\
- static PyObject *__pyx_dict_cached_value = NULL;\
- if (likely(__PYX_GET_DICT_VERSION(DICT) == __pyx_dict_version)) {\
- (VAR) = __pyx_dict_cached_value;\
- } else {\
- (VAR) = __pyx_dict_cached_value = (LOOKUP);\
- __pyx_dict_version = __PYX_GET_DICT_VERSION(DICT);\
- }\
-}
-static CYTHON_INLINE PY_UINT64_T __Pyx_get_tp_dict_version(PyObject *obj);
-static CYTHON_INLINE PY_UINT64_T __Pyx_get_object_dict_version(PyObject *obj);
-static CYTHON_INLINE int __Pyx_object_dict_version_matches(PyObject* obj, PY_UINT64_T tp_dict_version, PY_UINT64_T obj_dict_version);
-#else
-#define __PYX_GET_DICT_VERSION(dict) (0)
-#define __PYX_UPDATE_DICT_CACHE(dict, value, cache_var, version_var)
-#define __PYX_PY_DICT_LOOKUP_IF_MODIFIED(VAR, DICT, LOOKUP) (VAR) = (LOOKUP);
-#endif
-
-/* GetModuleGlobalName.proto */
-#if CYTHON_USE_DICT_VERSIONS
-#define __Pyx_GetModuleGlobalName(var, name) {\
- static PY_UINT64_T __pyx_dict_version = 0;\
- static PyObject *__pyx_dict_cached_value = NULL;\
- (var) = (likely(__pyx_dict_version == __PYX_GET_DICT_VERSION(__pyx_d))) ?\
- (likely(__pyx_dict_cached_value) ? __Pyx_NewRef(__pyx_dict_cached_value) : __Pyx_GetBuiltinName(name)) :\
- __Pyx__GetModuleGlobalName(name, &__pyx_dict_version, &__pyx_dict_cached_value);\
-}
-#define __Pyx_GetModuleGlobalNameUncached(var, name) {\
- PY_UINT64_T __pyx_dict_version;\
- PyObject *__pyx_dict_cached_value;\
- (var) = __Pyx__GetModuleGlobalName(name, &__pyx_dict_version, &__pyx_dict_cached_value);\
-}
-static PyObject *__Pyx__GetModuleGlobalName(PyObject *name, PY_UINT64_T *dict_version, PyObject **dict_cached_value);
-#else
-#define __Pyx_GetModuleGlobalName(var, name) (var) = __Pyx__GetModuleGlobalName(name)
-#define __Pyx_GetModuleGlobalNameUncached(var, name) (var) = __Pyx__GetModuleGlobalName(name)
-static CYTHON_INLINE PyObject *__Pyx__GetModuleGlobalName(PyObject *name);
-#endif
-
-/* RaiseTooManyValuesToUnpack.proto */
-static CYTHON_INLINE void __Pyx_RaiseTooManyValuesError(Py_ssize_t expected);
-
-/* RaiseNeedMoreValuesToUnpack.proto */
-static CYTHON_INLINE void __Pyx_RaiseNeedMoreValuesError(Py_ssize_t index);
-
-/* RaiseNoneIterError.proto */
-static CYTHON_INLINE void __Pyx_RaiseNoneNotIterableError(void);
-
-/* ExtTypeTest.proto */
-static CYTHON_INLINE int __Pyx_TypeTest(PyObject *obj, PyTypeObject *type);
-
-/* GetTopmostException.proto */
-#if CYTHON_USE_EXC_INFO_STACK
-static _PyErr_StackItem * __Pyx_PyErr_GetTopmostException(PyThreadState *tstate);
-#endif
-
-/* SaveResetException.proto */
-#if CYTHON_FAST_THREAD_STATE
-#define __Pyx_ExceptionSave(type, value, tb) __Pyx__ExceptionSave(__pyx_tstate, type, value, tb)
-static CYTHON_INLINE void __Pyx__ExceptionSave(PyThreadState *tstate, PyObject **type, PyObject **value, PyObject **tb);
-#define __Pyx_ExceptionReset(type, value, tb) __Pyx__ExceptionReset(__pyx_tstate, type, value, tb)
-static CYTHON_INLINE void __Pyx__ExceptionReset(PyThreadState *tstate, PyObject *type, PyObject *value, PyObject *tb);
-#else
-#define __Pyx_ExceptionSave(type, value, tb) PyErr_GetExcInfo(type, value, tb)
-#define __Pyx_ExceptionReset(type, value, tb) PyErr_SetExcInfo(type, value, tb)
-#endif
-
-/* GetException.proto */
-#if CYTHON_FAST_THREAD_STATE
-#define __Pyx_GetException(type, value, tb) __Pyx__GetException(__pyx_tstate, type, value, tb)
-static int __Pyx__GetException(PyThreadState *tstate, PyObject **type, PyObject **value, PyObject **tb);
-#else
-static int __Pyx_GetException(PyObject **type, PyObject **value, PyObject **tb);
-#endif
-
-/* SwapException.proto */
-#if CYTHON_FAST_THREAD_STATE
-#define __Pyx_ExceptionSwap(type, value, tb) __Pyx__ExceptionSwap(__pyx_tstate, type, value, tb)
-static CYTHON_INLINE void __Pyx__ExceptionSwap(PyThreadState *tstate, PyObject **type, PyObject **value, PyObject **tb);
-#else
-static CYTHON_INLINE void __Pyx_ExceptionSwap(PyObject **type, PyObject **value, PyObject **tb);
-#endif
-
-/* Import.proto */
-static PyObject *__Pyx_Import(PyObject *name, PyObject *from_list, int level);
-
-/* FastTypeChecks.proto */
-#if CYTHON_COMPILING_IN_CPYTHON
-#define __Pyx_TypeCheck(obj, type) __Pyx_IsSubtype(Py_TYPE(obj), (PyTypeObject *)type)
-static CYTHON_INLINE int __Pyx_IsSubtype(PyTypeObject *a, PyTypeObject *b);
-static CYTHON_INLINE int __Pyx_PyErr_GivenExceptionMatches(PyObject *err, PyObject *type);
-static CYTHON_INLINE int __Pyx_PyErr_GivenExceptionMatches2(PyObject *err, PyObject *type1, PyObject *type2);
-#else
-#define __Pyx_TypeCheck(obj, type) PyObject_TypeCheck(obj, (PyTypeObject *)type)
-#define __Pyx_PyErr_GivenExceptionMatches(err, type) PyErr_GivenExceptionMatches(err, type)
-#define __Pyx_PyErr_GivenExceptionMatches2(err, type1, type2) (PyErr_GivenExceptionMatches(err, type1) || PyErr_GivenExceptionMatches(err, type2))
-#endif
-#define __Pyx_PyException_Check(obj) __Pyx_TypeCheck(obj, PyExc_Exception)
-
-static CYTHON_UNUSED int __pyx_memoryview_getbuffer(PyObject *__pyx_v_self, Py_buffer *__pyx_v_info, int __pyx_v_flags); /*proto*/
-/* ListCompAppend.proto */
-#if CYTHON_USE_PYLIST_INTERNALS && CYTHON_ASSUME_SAFE_MACROS
-static CYTHON_INLINE int __Pyx_ListComp_Append(PyObject* list, PyObject* x) {
- PyListObject* L = (PyListObject*) list;
- Py_ssize_t len = Py_SIZE(list);
- if (likely(L->allocated > len)) {
- Py_INCREF(x);
- PyList_SET_ITEM(list, len, x);
- __Pyx_SET_SIZE(list, len + 1);
- return 0;
- }
- return PyList_Append(list, x);
-}
-#else
-#define __Pyx_ListComp_Append(L,x) PyList_Append(L,x)
-#endif
-
-/* PyIntBinop.proto */
-#if !CYTHON_COMPILING_IN_PYPY
-static PyObject* __Pyx_PyInt_AddObjC(PyObject *op1, PyObject *op2, long intval, int inplace, int zerodivision_check);
-#else
-#define __Pyx_PyInt_AddObjC(op1, op2, intval, inplace, zerodivision_check)\
- (inplace ? PyNumber_InPlaceAdd(op1, op2) : PyNumber_Add(op1, op2))
-#endif
-
-/* ListExtend.proto */
-static CYTHON_INLINE int __Pyx_PyList_Extend(PyObject* L, PyObject* v) {
-#if CYTHON_COMPILING_IN_CPYTHON
- PyObject* none = _PyList_Extend((PyListObject*)L, v);
- if (unlikely(!none))
- return -1;
- Py_DECREF(none);
- return 0;
-#else
- return PyList_SetSlice(L, PY_SSIZE_T_MAX, PY_SSIZE_T_MAX, v);
-#endif
-}
-
-/* ListAppend.proto */
-#if CYTHON_USE_PYLIST_INTERNALS && CYTHON_ASSUME_SAFE_MACROS
-static CYTHON_INLINE int __Pyx_PyList_Append(PyObject* list, PyObject* x) {
- PyListObject* L = (PyListObject*) list;
- Py_ssize_t len = Py_SIZE(list);
- if (likely(L->allocated > len) & likely(len > (L->allocated >> 1))) {
- Py_INCREF(x);
- PyList_SET_ITEM(list, len, x);
- __Pyx_SET_SIZE(list, len + 1);
- return 0;
- }
- return PyList_Append(list, x);
-}
-#else
-#define __Pyx_PyList_Append(L,x) PyList_Append(L,x)
-#endif
-
-/* None.proto */
-static CYTHON_INLINE long __Pyx_div_long(long, long);
-
-/* ImportFrom.proto */
-static PyObject* __Pyx_ImportFrom(PyObject* module, PyObject* name);
-
-/* HasAttr.proto */
-static CYTHON_INLINE int __Pyx_HasAttr(PyObject *, PyObject *);
-
-/* PyObject_GenericGetAttrNoDict.proto */
-#if CYTHON_USE_TYPE_SLOTS && CYTHON_USE_PYTYPE_LOOKUP && PY_VERSION_HEX < 0x03070000
-static CYTHON_INLINE PyObject* __Pyx_PyObject_GenericGetAttrNoDict(PyObject* obj, PyObject* attr_name);
-#else
-#define __Pyx_PyObject_GenericGetAttrNoDict PyObject_GenericGetAttr
-#endif
-
-/* PyObject_GenericGetAttr.proto */
-#if CYTHON_USE_TYPE_SLOTS && CYTHON_USE_PYTYPE_LOOKUP && PY_VERSION_HEX < 0x03070000
-static PyObject* __Pyx_PyObject_GenericGetAttr(PyObject* obj, PyObject* attr_name);
-#else
-#define __Pyx_PyObject_GenericGetAttr PyObject_GenericGetAttr
-#endif
-
-/* SetVTable.proto */
-static int __Pyx_SetVtable(PyObject *dict, void *vtable);
-
-/* PyObjectGetAttrStrNoError.proto */
-static CYTHON_INLINE PyObject* __Pyx_PyObject_GetAttrStrNoError(PyObject* obj, PyObject* attr_name);
-
-/* SetupReduce.proto */
-static int __Pyx_setup_reduce(PyObject* type_obj);
-
-/* CLineInTraceback.proto */
-#ifdef CYTHON_CLINE_IN_TRACEBACK
-#define __Pyx_CLineForTraceback(tstate, c_line) (((CYTHON_CLINE_IN_TRACEBACK)) ? c_line : 0)
-#else
-static int __Pyx_CLineForTraceback(PyThreadState *tstate, int c_line);
-#endif
-
-/* CodeObjectCache.proto */
-typedef struct {
- PyCodeObject* code_object;
- int code_line;
-} __Pyx_CodeObjectCacheEntry;
-struct __Pyx_CodeObjectCache {
- int count;
- int max_count;
- __Pyx_CodeObjectCacheEntry* entries;
-};
-static struct __Pyx_CodeObjectCache __pyx_code_cache = {0,0,NULL};
-static int __pyx_bisect_code_objects(__Pyx_CodeObjectCacheEntry* entries, int count, int code_line);
-static PyCodeObject *__pyx_find_code_object(int code_line);
-static void __pyx_insert_code_object(int code_line, PyCodeObject* code_object);
-
-/* AddTraceback.proto */
-static void __Pyx_AddTraceback(const char *funcname, int c_line,
- int py_line, const char *filename);
-
-#if PY_MAJOR_VERSION < 3
- static int __Pyx_GetBuffer(PyObject *obj, Py_buffer *view, int flags);
- static void __Pyx_ReleaseBuffer(Py_buffer *view);
-#else
- #define __Pyx_GetBuffer PyObject_GetBuffer
- #define __Pyx_ReleaseBuffer PyBuffer_Release
-#endif
-
-
-/* BufferStructDeclare.proto */
-typedef struct {
- Py_ssize_t shape, strides, suboffsets;
-} __Pyx_Buf_DimInfo;
-typedef struct {
- size_t refcount;
- Py_buffer pybuffer;
-} __Pyx_Buffer;
-typedef struct {
- __Pyx_Buffer *rcbuffer;
- char *data;
- __Pyx_Buf_DimInfo diminfo[8];
-} __Pyx_LocalBuf_ND;
-
-/* MemviewSliceIsContig.proto */
-static int __pyx_memviewslice_is_contig(const __Pyx_memviewslice mvs, char order, int ndim);
-
-/* OverlappingSlices.proto */
-static int __pyx_slices_overlap(__Pyx_memviewslice *slice1,
- __Pyx_memviewslice *slice2,
- int ndim, size_t itemsize);
-
-/* Capsule.proto */
-static CYTHON_INLINE PyObject *__pyx_capsule_create(void *p, const char *sig);
-
-/* IsLittleEndian.proto */
-static CYTHON_INLINE int __Pyx_Is_Little_Endian(void);
-
-/* BufferFormatCheck.proto */
-static const char* __Pyx_BufFmt_CheckString(__Pyx_BufFmt_Context* ctx, const char* ts);
-static void __Pyx_BufFmt_Init(__Pyx_BufFmt_Context* ctx,
- __Pyx_BufFmt_StackElem* stack,
- __Pyx_TypeInfo* type);
-
-/* TypeInfoCompare.proto */
-static int __pyx_typeinfo_cmp(__Pyx_TypeInfo *a, __Pyx_TypeInfo *b);
-
-/* MemviewSliceValidateAndInit.proto */
-static int __Pyx_ValidateAndInit_memviewslice(
- int *axes_specs,
- int c_or_f_flag,
- int buf_flags,
- int ndim,
- __Pyx_TypeInfo *dtype,
- __Pyx_BufFmt_StackElem stack[],
- __Pyx_memviewslice *memviewslice,
- PyObject *original_obj);
-
-/* ObjectToMemviewSlice.proto */
-static CYTHON_INLINE __Pyx_memviewslice __Pyx_PyObject_to_MemoryviewSlice_d_d_dc_int(PyObject *, int writable_flag);
-
-/* ObjectToMemviewSlice.proto */
-static CYTHON_INLINE __Pyx_memviewslice __Pyx_PyObject_to_MemoryviewSlice_d_d_dc_float(PyObject *, int writable_flag);
-
-/* ObjectToMemviewSlice.proto */
-static CYTHON_INLINE __Pyx_memviewslice __Pyx_PyObject_to_MemoryviewSlice_dc_int(PyObject *, int writable_flag);
-
-/* CIntToPy.proto */
-static CYTHON_INLINE PyObject* __Pyx_PyInt_From_int(int value);
-
-/* CIntToPy.proto */
-static CYTHON_INLINE PyObject* __Pyx_PyInt_From_long(long value);
-
-/* MemviewSliceCopyTemplate.proto */
-static __Pyx_memviewslice
-__pyx_memoryview_copy_new_contig(const __Pyx_memviewslice *from_mvs,
- const char *mode, int ndim,
- size_t sizeof_dtype, int contig_flag,
- int dtype_is_object);
-
-/* CIntFromPy.proto */
-static CYTHON_INLINE int __Pyx_PyInt_As_int(PyObject *);
-
-/* CIntFromPy.proto */
-static CYTHON_INLINE long __Pyx_PyInt_As_long(PyObject *);
-
-/* CIntFromPy.proto */
-static CYTHON_INLINE char __Pyx_PyInt_As_char(PyObject *);
-
-/* CheckBinaryVersion.proto */
-static int __Pyx_check_binary_version(void);
-
-/* InitStrings.proto */
-static int __Pyx_InitStrings(__Pyx_StringTabEntry *t);
-
-static PyObject *__pyx_array_get_memview(struct __pyx_array_obj *__pyx_v_self); /* proto*/
-static char *__pyx_memoryview_get_item_pointer(struct __pyx_memoryview_obj *__pyx_v_self, PyObject *__pyx_v_index); /* proto*/
-static PyObject *__pyx_memoryview_is_slice(struct __pyx_memoryview_obj *__pyx_v_self, PyObject *__pyx_v_obj); /* proto*/
-static PyObject *__pyx_memoryview_setitem_slice_assignment(struct __pyx_memoryview_obj *__pyx_v_self, PyObject *__pyx_v_dst, PyObject *__pyx_v_src); /* proto*/
-static PyObject *__pyx_memoryview_setitem_slice_assign_scalar(struct __pyx_memoryview_obj *__pyx_v_self, struct __pyx_memoryview_obj *__pyx_v_dst, PyObject *__pyx_v_value); /* proto*/
-static PyObject *__pyx_memoryview_setitem_indexed(struct __pyx_memoryview_obj *__pyx_v_self, PyObject *__pyx_v_index, PyObject *__pyx_v_value); /* proto*/
-static PyObject *__pyx_memoryview_convert_item_to_object(struct __pyx_memoryview_obj *__pyx_v_self, char *__pyx_v_itemp); /* proto*/
-static PyObject *__pyx_memoryview_assign_item_from_object(struct __pyx_memoryview_obj *__pyx_v_self, char *__pyx_v_itemp, PyObject *__pyx_v_value); /* proto*/
-static PyObject *__pyx_memoryviewslice_convert_item_to_object(struct __pyx_memoryviewslice_obj *__pyx_v_self, char *__pyx_v_itemp); /* proto*/
-static PyObject *__pyx_memoryviewslice_assign_item_from_object(struct __pyx_memoryviewslice_obj *__pyx_v_self, char *__pyx_v_itemp, PyObject *__pyx_v_value); /* proto*/
-
-/* Module declarations from 'cython.view' */
-
-/* Module declarations from 'cython' */
-
-/* Module declarations from 'monotonic_align.core' */
-static PyTypeObject *__pyx_array_type = 0;
-static PyTypeObject *__pyx_MemviewEnum_type = 0;
-static PyTypeObject *__pyx_memoryview_type = 0;
-static PyTypeObject *__pyx_memoryviewslice_type = 0;
-static PyObject *generic = 0;
-static PyObject *strided = 0;
-static PyObject *indirect = 0;
-static PyObject *contiguous = 0;
-static PyObject *indirect_contiguous = 0;
-static int __pyx_memoryview_thread_locks_used;
-static PyThread_type_lock __pyx_memoryview_thread_locks[8];
-static void __pyx_f_15monotonic_align_4core_maximum_path_each(__Pyx_memviewslice, __Pyx_memviewslice, int, int, struct __pyx_opt_args_15monotonic_align_4core_maximum_path_each *__pyx_optional_args); /*proto*/
-static void __pyx_f_15monotonic_align_4core_maximum_path_c(__Pyx_memviewslice, __Pyx_memviewslice, __Pyx_memviewslice, __Pyx_memviewslice, int __pyx_skip_dispatch); /*proto*/
-static struct __pyx_array_obj *__pyx_array_new(PyObject *, Py_ssize_t, char *, char *, char *); /*proto*/
-static void *__pyx_align_pointer(void *, size_t); /*proto*/
-static PyObject *__pyx_memoryview_new(PyObject *, int, int, __Pyx_TypeInfo *); /*proto*/
-static CYTHON_INLINE int __pyx_memoryview_check(PyObject *); /*proto*/
-static PyObject *_unellipsify(PyObject *, int); /*proto*/
-static PyObject *assert_direct_dimensions(Py_ssize_t *, int); /*proto*/
-static struct __pyx_memoryview_obj *__pyx_memview_slice(struct __pyx_memoryview_obj *, PyObject *); /*proto*/
-static int __pyx_memoryview_slice_memviewslice(__Pyx_memviewslice *, Py_ssize_t, Py_ssize_t, Py_ssize_t, int, int, int *, Py_ssize_t, Py_ssize_t, Py_ssize_t, int, int, int, int); /*proto*/
-static char *__pyx_pybuffer_index(Py_buffer *, char *, Py_ssize_t, Py_ssize_t); /*proto*/
-static int __pyx_memslice_transpose(__Pyx_memviewslice *); /*proto*/
-static PyObject *__pyx_memoryview_fromslice(__Pyx_memviewslice, int, PyObject *(*)(char *), int (*)(char *, PyObject *), int); /*proto*/
-static __Pyx_memviewslice *__pyx_memoryview_get_slice_from_memoryview(struct __pyx_memoryview_obj *, __Pyx_memviewslice *); /*proto*/
-static void __pyx_memoryview_slice_copy(struct __pyx_memoryview_obj *, __Pyx_memviewslice *); /*proto*/
-static PyObject *__pyx_memoryview_copy_object(struct __pyx_memoryview_obj *); /*proto*/
-static PyObject *__pyx_memoryview_copy_object_from_slice(struct __pyx_memoryview_obj *, __Pyx_memviewslice *); /*proto*/
-static Py_ssize_t abs_py_ssize_t(Py_ssize_t); /*proto*/
-static char __pyx_get_best_slice_order(__Pyx_memviewslice *, int); /*proto*/
-static void _copy_strided_to_strided(char *, Py_ssize_t *, char *, Py_ssize_t *, Py_ssize_t *, Py_ssize_t *, int, size_t); /*proto*/
-static void copy_strided_to_strided(__Pyx_memviewslice *, __Pyx_memviewslice *, int, size_t); /*proto*/
-static Py_ssize_t __pyx_memoryview_slice_get_size(__Pyx_memviewslice *, int); /*proto*/
-static Py_ssize_t __pyx_fill_contig_strides_array(Py_ssize_t *, Py_ssize_t *, Py_ssize_t, int, char); /*proto*/
-static void *__pyx_memoryview_copy_data_to_temp(__Pyx_memviewslice *, __Pyx_memviewslice *, char, int); /*proto*/
-static int __pyx_memoryview_err_extents(int, Py_ssize_t, Py_ssize_t); /*proto*/
-static int __pyx_memoryview_err_dim(PyObject *, char *, int); /*proto*/
-static int __pyx_memoryview_err(PyObject *, char *); /*proto*/
-static int __pyx_memoryview_copy_contents(__Pyx_memviewslice, __Pyx_memviewslice, int, int, int); /*proto*/
-static void __pyx_memoryview_broadcast_leading(__Pyx_memviewslice *, int, int); /*proto*/
-static void __pyx_memoryview_refcount_copying(__Pyx_memviewslice *, int, int, int); /*proto*/
-static void __pyx_memoryview_refcount_objects_in_slice_with_gil(char *, Py_ssize_t *, Py_ssize_t *, int, int); /*proto*/
-static void __pyx_memoryview_refcount_objects_in_slice(char *, Py_ssize_t *, Py_ssize_t *, int, int); /*proto*/
-static void __pyx_memoryview_slice_assign_scalar(__Pyx_memviewslice *, int, size_t, void *, int); /*proto*/
-static void __pyx_memoryview__slice_assign_scalar(char *, Py_ssize_t *, Py_ssize_t *, int, size_t, void *); /*proto*/
-static PyObject *__pyx_unpickle_Enum__set_state(struct __pyx_MemviewEnum_obj *, PyObject *); /*proto*/
-static __Pyx_TypeInfo __Pyx_TypeInfo_int = { "int", NULL, sizeof(int), { 0 }, 0, IS_UNSIGNED(int) ? 'U' : 'I', IS_UNSIGNED(int), 0 };
-static __Pyx_TypeInfo __Pyx_TypeInfo_float = { "float", NULL, sizeof(float), { 0 }, 0, 'R', 0, 0 };
-#define __Pyx_MODULE_NAME "monotonic_align.core"
-extern int __pyx_module_is_main_monotonic_align__core;
-int __pyx_module_is_main_monotonic_align__core = 0;
-
-/* Implementation of 'monotonic_align.core' */
-static PyObject *__pyx_builtin_range;
-static PyObject *__pyx_builtin_ValueError;
-static PyObject *__pyx_builtin_MemoryError;
-static PyObject *__pyx_builtin_enumerate;
-static PyObject *__pyx_builtin_TypeError;
-static PyObject *__pyx_builtin_Ellipsis;
-static PyObject *__pyx_builtin_id;
-static PyObject *__pyx_builtin_IndexError;
-static const char __pyx_k_O[] = "O";
-static const char __pyx_k_c[] = "c";
-static const char __pyx_k_id[] = "id";
-static const char __pyx_k_new[] = "__new__";
-static const char __pyx_k_obj[] = "obj";
-static const char __pyx_k_base[] = "base";
-static const char __pyx_k_dict[] = "__dict__";
-static const char __pyx_k_main[] = "__main__";
-static const char __pyx_k_mode[] = "mode";
-static const char __pyx_k_name[] = "name";
-static const char __pyx_k_ndim[] = "ndim";
-static const char __pyx_k_pack[] = "pack";
-static const char __pyx_k_size[] = "size";
-static const char __pyx_k_step[] = "step";
-static const char __pyx_k_stop[] = "stop";
-static const char __pyx_k_t_xs[] = "t_xs";
-static const char __pyx_k_t_ys[] = "t_ys";
-static const char __pyx_k_test[] = "__test__";
-static const char __pyx_k_ASCII[] = "ASCII";
-static const char __pyx_k_class[] = "__class__";
-static const char __pyx_k_error[] = "error";
-static const char __pyx_k_flags[] = "flags";
-static const char __pyx_k_paths[] = "paths";
-static const char __pyx_k_range[] = "range";
-static const char __pyx_k_shape[] = "shape";
-static const char __pyx_k_start[] = "start";
-static const char __pyx_k_encode[] = "encode";
-static const char __pyx_k_format[] = "format";
-static const char __pyx_k_import[] = "__import__";
-static const char __pyx_k_name_2[] = "__name__";
-static const char __pyx_k_pickle[] = "pickle";
-static const char __pyx_k_reduce[] = "__reduce__";
-static const char __pyx_k_struct[] = "struct";
-static const char __pyx_k_unpack[] = "unpack";
-static const char __pyx_k_update[] = "update";
-static const char __pyx_k_values[] = "values";
-static const char __pyx_k_fortran[] = "fortran";
-static const char __pyx_k_memview[] = "memview";
-static const char __pyx_k_Ellipsis[] = "Ellipsis";
-static const char __pyx_k_getstate[] = "__getstate__";
-static const char __pyx_k_itemsize[] = "itemsize";
-static const char __pyx_k_pyx_type[] = "__pyx_type";
-static const char __pyx_k_setstate[] = "__setstate__";
-static const char __pyx_k_TypeError[] = "TypeError";
-static const char __pyx_k_enumerate[] = "enumerate";
-static const char __pyx_k_pyx_state[] = "__pyx_state";
-static const char __pyx_k_reduce_ex[] = "__reduce_ex__";
-static const char __pyx_k_IndexError[] = "IndexError";
-static const char __pyx_k_ValueError[] = "ValueError";
-static const char __pyx_k_pyx_result[] = "__pyx_result";
-static const char __pyx_k_pyx_vtable[] = "__pyx_vtable__";
-static const char __pyx_k_MemoryError[] = "MemoryError";
-static const char __pyx_k_PickleError[] = "PickleError";
-static const char __pyx_k_pyx_checksum[] = "__pyx_checksum";
-static const char __pyx_k_stringsource[] = "stringsource";
-static const char __pyx_k_pyx_getbuffer[] = "__pyx_getbuffer";
-static const char __pyx_k_reduce_cython[] = "__reduce_cython__";
-static const char __pyx_k_View_MemoryView[] = "View.MemoryView";
-static const char __pyx_k_allocate_buffer[] = "allocate_buffer";
-static const char __pyx_k_dtype_is_object[] = "dtype_is_object";
-static const char __pyx_k_pyx_PickleError[] = "__pyx_PickleError";
-static const char __pyx_k_setstate_cython[] = "__setstate_cython__";
-static const char __pyx_k_pyx_unpickle_Enum[] = "__pyx_unpickle_Enum";
-static const char __pyx_k_cline_in_traceback[] = "cline_in_traceback";
-static const char __pyx_k_strided_and_direct[] = "";
-static const char __pyx_k_strided_and_indirect[] = "";
-static const char __pyx_k_contiguous_and_direct[] = "";
-static const char __pyx_k_MemoryView_of_r_object[] = "";
-static const char __pyx_k_MemoryView_of_r_at_0x_x[] = "";
-static const char __pyx_k_contiguous_and_indirect[] = "";
-static const char __pyx_k_Cannot_index_with_type_s[] = "Cannot index with type '%s'";
-static const char __pyx_k_Invalid_shape_in_axis_d_d[] = "Invalid shape in axis %d: %d.";
-static const char __pyx_k_itemsize_0_for_cython_array[] = "itemsize <= 0 for cython.array";
-static const char __pyx_k_unable_to_allocate_array_data[] = "unable to allocate array data.";
-static const char __pyx_k_strided_and_direct_or_indirect[] = "";
-static const char __pyx_k_Buffer_view_does_not_expose_stri[] = "Buffer view does not expose strides";
-static const char __pyx_k_Can_only_create_a_buffer_that_is[] = "Can only create a buffer that is contiguous in memory.";
-static const char __pyx_k_Cannot_assign_to_read_only_memor[] = "Cannot assign to read-only memoryview";
-static const char __pyx_k_Cannot_create_writable_memory_vi[] = "Cannot create writable memory view from read-only memoryview";
-static const char __pyx_k_Empty_shape_tuple_for_cython_arr[] = "Empty shape tuple for cython.array";
-static const char __pyx_k_Incompatible_checksums_s_vs_0xb0[] = "Incompatible checksums (%s vs 0xb068931 = (name))";
-static const char __pyx_k_Indirect_dimensions_not_supporte[] = "Indirect dimensions not supported";
-static const char __pyx_k_Invalid_mode_expected_c_or_fortr[] = "Invalid mode, expected 'c' or 'fortran', got %s";
-static const char __pyx_k_Out_of_bounds_on_buffer_access_a[] = "Out of bounds on buffer access (axis %d)";
-static const char __pyx_k_Unable_to_convert_item_to_object[] = "Unable to convert item to object";
-static const char __pyx_k_got_differing_extents_in_dimensi[] = "got differing extents in dimension %d (got %d and %d)";
-static const char __pyx_k_no_default___reduce___due_to_non[] = "no default __reduce__ due to non-trivial __cinit__";
-static const char __pyx_k_unable_to_allocate_shape_and_str[] = "unable to allocate shape and strides.";
-static PyObject *__pyx_n_s_ASCII;
-static PyObject *__pyx_kp_s_Buffer_view_does_not_expose_stri;
-static PyObject *__pyx_kp_s_Can_only_create_a_buffer_that_is;
-static PyObject *__pyx_kp_s_Cannot_assign_to_read_only_memor;
-static PyObject *__pyx_kp_s_Cannot_create_writable_memory_vi;
-static PyObject *__pyx_kp_s_Cannot_index_with_type_s;
-static PyObject *__pyx_n_s_Ellipsis;
-static PyObject *__pyx_kp_s_Empty_shape_tuple_for_cython_arr;
-static PyObject *__pyx_kp_s_Incompatible_checksums_s_vs_0xb0;
-static PyObject *__pyx_n_s_IndexError;
-static PyObject *__pyx_kp_s_Indirect_dimensions_not_supporte;
-static PyObject *__pyx_kp_s_Invalid_mode_expected_c_or_fortr;
-static PyObject *__pyx_kp_s_Invalid_shape_in_axis_d_d;
-static PyObject *__pyx_n_s_MemoryError;
-static PyObject *__pyx_kp_s_MemoryView_of_r_at_0x_x;
-static PyObject *__pyx_kp_s_MemoryView_of_r_object;
-static PyObject *__pyx_n_b_O;
-static PyObject *__pyx_kp_s_Out_of_bounds_on_buffer_access_a;
-static PyObject *__pyx_n_s_PickleError;
-static PyObject *__pyx_n_s_TypeError;
-static PyObject *__pyx_kp_s_Unable_to_convert_item_to_object;
-static PyObject *__pyx_n_s_ValueError;
-static PyObject *__pyx_n_s_View_MemoryView;
-static PyObject *__pyx_n_s_allocate_buffer;
-static PyObject *__pyx_n_s_base;
-static PyObject *__pyx_n_s_c;
-static PyObject *__pyx_n_u_c;
-static PyObject *__pyx_n_s_class;
-static PyObject *__pyx_n_s_cline_in_traceback;
-static PyObject *__pyx_kp_s_contiguous_and_direct;
-static PyObject *__pyx_kp_s_contiguous_and_indirect;
-static PyObject *__pyx_n_s_dict;
-static PyObject *__pyx_n_s_dtype_is_object;
-static PyObject *__pyx_n_s_encode;
-static PyObject *__pyx_n_s_enumerate;
-static PyObject *__pyx_n_s_error;
-static PyObject *__pyx_n_s_flags;
-static PyObject *__pyx_n_s_format;
-static PyObject *__pyx_n_s_fortran;
-static PyObject *__pyx_n_u_fortran;
-static PyObject *__pyx_n_s_getstate;
-static PyObject *__pyx_kp_s_got_differing_extents_in_dimensi;
-static PyObject *__pyx_n_s_id;
-static PyObject *__pyx_n_s_import;
-static PyObject *__pyx_n_s_itemsize;
-static PyObject *__pyx_kp_s_itemsize_0_for_cython_array;
-static PyObject *__pyx_n_s_main;
-static PyObject *__pyx_n_s_memview;
-static PyObject *__pyx_n_s_mode;
-static PyObject *__pyx_n_s_name;
-static PyObject *__pyx_n_s_name_2;
-static PyObject *__pyx_n_s_ndim;
-static PyObject *__pyx_n_s_new;
-static PyObject *__pyx_kp_s_no_default___reduce___due_to_non;
-static PyObject *__pyx_n_s_obj;
-static PyObject *__pyx_n_s_pack;
-static PyObject *__pyx_n_s_paths;
-static PyObject *__pyx_n_s_pickle;
-static PyObject *__pyx_n_s_pyx_PickleError;
-static PyObject *__pyx_n_s_pyx_checksum;
-static PyObject *__pyx_n_s_pyx_getbuffer;
-static PyObject *__pyx_n_s_pyx_result;
-static PyObject *__pyx_n_s_pyx_state;
-static PyObject *__pyx_n_s_pyx_type;
-static PyObject *__pyx_n_s_pyx_unpickle_Enum;
-static PyObject *__pyx_n_s_pyx_vtable;
-static PyObject *__pyx_n_s_range;
-static PyObject *__pyx_n_s_reduce;
-static PyObject *__pyx_n_s_reduce_cython;
-static PyObject *__pyx_n_s_reduce_ex;
-static PyObject *__pyx_n_s_setstate;
-static PyObject *__pyx_n_s_setstate_cython;
-static PyObject *__pyx_n_s_shape;
-static PyObject *__pyx_n_s_size;
-static PyObject *__pyx_n_s_start;
-static PyObject *__pyx_n_s_step;
-static PyObject *__pyx_n_s_stop;
-static PyObject *__pyx_kp_s_strided_and_direct;
-static PyObject *__pyx_kp_s_strided_and_direct_or_indirect;
-static PyObject *__pyx_kp_s_strided_and_indirect;
-static PyObject *__pyx_kp_s_stringsource;
-static PyObject *__pyx_n_s_struct;
-static PyObject *__pyx_n_s_t_xs;
-static PyObject *__pyx_n_s_t_ys;
-static PyObject *__pyx_n_s_test;
-static PyObject *__pyx_kp_s_unable_to_allocate_array_data;
-static PyObject *__pyx_kp_s_unable_to_allocate_shape_and_str;
-static PyObject *__pyx_n_s_unpack;
-static PyObject *__pyx_n_s_update;
-static PyObject *__pyx_n_s_values;
-static PyObject *__pyx_pf_15monotonic_align_4core_maximum_path_c(CYTHON_UNUSED PyObject *__pyx_self, __Pyx_memviewslice __pyx_v_paths, __Pyx_memviewslice __pyx_v_values, __Pyx_memviewslice __pyx_v_t_ys, __Pyx_memviewslice __pyx_v_t_xs); /* proto */
-static int __pyx_array___pyx_pf_15View_dot_MemoryView_5array___cinit__(struct __pyx_array_obj *__pyx_v_self, PyObject *__pyx_v_shape, Py_ssize_t __pyx_v_itemsize, PyObject *__pyx_v_format, PyObject *__pyx_v_mode, int __pyx_v_allocate_buffer); /* proto */
-static int __pyx_array___pyx_pf_15View_dot_MemoryView_5array_2__getbuffer__(struct __pyx_array_obj *__pyx_v_self, Py_buffer *__pyx_v_info, int __pyx_v_flags); /* proto */
-static void __pyx_array___pyx_pf_15View_dot_MemoryView_5array_4__dealloc__(struct __pyx_array_obj *__pyx_v_self); /* proto */
-static PyObject *__pyx_pf_15View_dot_MemoryView_5array_7memview___get__(struct __pyx_array_obj *__pyx_v_self); /* proto */
-static Py_ssize_t __pyx_array___pyx_pf_15View_dot_MemoryView_5array_6__len__(struct __pyx_array_obj *__pyx_v_self); /* proto */
-static PyObject *__pyx_array___pyx_pf_15View_dot_MemoryView_5array_8__getattr__(struct __pyx_array_obj *__pyx_v_self, PyObject *__pyx_v_attr); /* proto */
-static PyObject *__pyx_array___pyx_pf_15View_dot_MemoryView_5array_10__getitem__(struct __pyx_array_obj *__pyx_v_self, PyObject *__pyx_v_item); /* proto */
-static int __pyx_array___pyx_pf_15View_dot_MemoryView_5array_12__setitem__(struct __pyx_array_obj *__pyx_v_self, PyObject *__pyx_v_item, PyObject *__pyx_v_value); /* proto */
-static PyObject *__pyx_pf___pyx_array___reduce_cython__(CYTHON_UNUSED struct __pyx_array_obj *__pyx_v_self); /* proto */
-static PyObject *__pyx_pf___pyx_array_2__setstate_cython__(CYTHON_UNUSED struct __pyx_array_obj *__pyx_v_self, CYTHON_UNUSED PyObject *__pyx_v___pyx_state); /* proto */
-static int __pyx_MemviewEnum___pyx_pf_15View_dot_MemoryView_4Enum___init__(struct __pyx_MemviewEnum_obj *__pyx_v_self, PyObject *__pyx_v_name); /* proto */
-static PyObject *__pyx_MemviewEnum___pyx_pf_15View_dot_MemoryView_4Enum_2__repr__(struct __pyx_MemviewEnum_obj *__pyx_v_self); /* proto */
-static PyObject *__pyx_pf___pyx_MemviewEnum___reduce_cython__(struct __pyx_MemviewEnum_obj *__pyx_v_self); /* proto */
-static PyObject *__pyx_pf___pyx_MemviewEnum_2__setstate_cython__(struct __pyx_MemviewEnum_obj *__pyx_v_self, PyObject *__pyx_v___pyx_state); /* proto */
-static int __pyx_memoryview___pyx_pf_15View_dot_MemoryView_10memoryview___cinit__(struct __pyx_memoryview_obj *__pyx_v_self, PyObject *__pyx_v_obj, int __pyx_v_flags, int __pyx_v_dtype_is_object); /* proto */
-static void __pyx_memoryview___pyx_pf_15View_dot_MemoryView_10memoryview_2__dealloc__(struct __pyx_memoryview_obj *__pyx_v_self); /* proto */
-static PyObject *__pyx_memoryview___pyx_pf_15View_dot_MemoryView_10memoryview_4__getitem__(struct __pyx_memoryview_obj *__pyx_v_self, PyObject *__pyx_v_index); /* proto */
-static int __pyx_memoryview___pyx_pf_15View_dot_MemoryView_10memoryview_6__setitem__(struct __pyx_memoryview_obj *__pyx_v_self, PyObject *__pyx_v_index, PyObject *__pyx_v_value); /* proto */
-static int __pyx_memoryview___pyx_pf_15View_dot_MemoryView_10memoryview_8__getbuffer__(struct __pyx_memoryview_obj *__pyx_v_self, Py_buffer *__pyx_v_info, int __pyx_v_flags); /* proto */
-static PyObject *__pyx_pf_15View_dot_MemoryView_10memoryview_1T___get__(struct __pyx_memoryview_obj *__pyx_v_self); /* proto */
-static PyObject *__pyx_pf_15View_dot_MemoryView_10memoryview_4base___get__(struct __pyx_memoryview_obj *__pyx_v_self); /* proto */
-static PyObject *__pyx_pf_15View_dot_MemoryView_10memoryview_5shape___get__(struct __pyx_memoryview_obj *__pyx_v_self); /* proto */
-static PyObject *__pyx_pf_15View_dot_MemoryView_10memoryview_7strides___get__(struct __pyx_memoryview_obj *__pyx_v_self); /* proto */
-static PyObject *__pyx_pf_15View_dot_MemoryView_10memoryview_10suboffsets___get__(struct __pyx_memoryview_obj *__pyx_v_self); /* proto */
-static PyObject *__pyx_pf_15View_dot_MemoryView_10memoryview_4ndim___get__(struct __pyx_memoryview_obj *__pyx_v_self); /* proto */
-static PyObject *__pyx_pf_15View_dot_MemoryView_10memoryview_8itemsize___get__(struct __pyx_memoryview_obj *__pyx_v_self); /* proto */
-static PyObject *__pyx_pf_15View_dot_MemoryView_10memoryview_6nbytes___get__(struct __pyx_memoryview_obj *__pyx_v_self); /* proto */
-static PyObject *__pyx_pf_15View_dot_MemoryView_10memoryview_4size___get__(struct __pyx_memoryview_obj *__pyx_v_self); /* proto */
-static Py_ssize_t __pyx_memoryview___pyx_pf_15View_dot_MemoryView_10memoryview_10__len__(struct __pyx_memoryview_obj *__pyx_v_self); /* proto */
-static PyObject *__pyx_memoryview___pyx_pf_15View_dot_MemoryView_10memoryview_12__repr__(struct __pyx_memoryview_obj *__pyx_v_self); /* proto */
-static PyObject *__pyx_memoryview___pyx_pf_15View_dot_MemoryView_10memoryview_14__str__(struct __pyx_memoryview_obj *__pyx_v_self); /* proto */
-static PyObject *__pyx_memoryview___pyx_pf_15View_dot_MemoryView_10memoryview_16is_c_contig(struct __pyx_memoryview_obj *__pyx_v_self); /* proto */
-static PyObject *__pyx_memoryview___pyx_pf_15View_dot_MemoryView_10memoryview_18is_f_contig(struct __pyx_memoryview_obj *__pyx_v_self); /* proto */
-static PyObject *__pyx_memoryview___pyx_pf_15View_dot_MemoryView_10memoryview_20copy(struct __pyx_memoryview_obj *__pyx_v_self); /* proto */
-static PyObject *__pyx_memoryview___pyx_pf_15View_dot_MemoryView_10memoryview_22copy_fortran(struct __pyx_memoryview_obj *__pyx_v_self); /* proto */
-static PyObject *__pyx_pf___pyx_memoryview___reduce_cython__(CYTHON_UNUSED struct __pyx_memoryview_obj *__pyx_v_self); /* proto */
-static PyObject *__pyx_pf___pyx_memoryview_2__setstate_cython__(CYTHON_UNUSED struct __pyx_memoryview_obj *__pyx_v_self, CYTHON_UNUSED PyObject *__pyx_v___pyx_state); /* proto */
-static void __pyx_memoryviewslice___pyx_pf_15View_dot_MemoryView_16_memoryviewslice___dealloc__(struct __pyx_memoryviewslice_obj *__pyx_v_self); /* proto */
-static PyObject *__pyx_pf_15View_dot_MemoryView_16_memoryviewslice_4base___get__(struct __pyx_memoryviewslice_obj *__pyx_v_self); /* proto */
-static PyObject *__pyx_pf___pyx_memoryviewslice___reduce_cython__(CYTHON_UNUSED struct __pyx_memoryviewslice_obj *__pyx_v_self); /* proto */
-static PyObject *__pyx_pf___pyx_memoryviewslice_2__setstate_cython__(CYTHON_UNUSED struct __pyx_memoryviewslice_obj *__pyx_v_self, CYTHON_UNUSED PyObject *__pyx_v___pyx_state); /* proto */
-static PyObject *__pyx_pf_15View_dot_MemoryView___pyx_unpickle_Enum(CYTHON_UNUSED PyObject *__pyx_self, PyObject *__pyx_v___pyx_type, long __pyx_v___pyx_checksum, PyObject *__pyx_v___pyx_state); /* proto */
-static PyObject *__pyx_tp_new_array(PyTypeObject *t, PyObject *a, PyObject *k); /*proto*/
-static PyObject *__pyx_tp_new_Enum(PyTypeObject *t, PyObject *a, PyObject *k); /*proto*/
-static PyObject *__pyx_tp_new_memoryview(PyTypeObject *t, PyObject *a, PyObject *k); /*proto*/
-static PyObject *__pyx_tp_new__memoryviewslice(PyTypeObject *t, PyObject *a, PyObject *k); /*proto*/
-static PyObject *__pyx_int_0;
-static PyObject *__pyx_int_1;
-static PyObject *__pyx_int_184977713;
-static PyObject *__pyx_int_neg_1;
-static float __pyx_k_;
-static PyObject *__pyx_tuple__2;
-static PyObject *__pyx_tuple__3;
-static PyObject *__pyx_tuple__4;
-static PyObject *__pyx_tuple__5;
-static PyObject *__pyx_tuple__6;
-static PyObject *__pyx_tuple__7;
-static PyObject *__pyx_tuple__8;
-static PyObject *__pyx_tuple__9;
-static PyObject *__pyx_slice__16;
-static PyObject *__pyx_tuple__10;
-static PyObject *__pyx_tuple__11;
-static PyObject *__pyx_tuple__12;
-static PyObject *__pyx_tuple__13;
-static PyObject *__pyx_tuple__14;
-static PyObject *__pyx_tuple__15;
-static PyObject *__pyx_tuple__17;
-static PyObject *__pyx_tuple__18;
-static PyObject *__pyx_tuple__19;
-static PyObject *__pyx_tuple__20;
-static PyObject *__pyx_tuple__21;
-static PyObject *__pyx_tuple__22;
-static PyObject *__pyx_tuple__23;
-static PyObject *__pyx_tuple__24;
-static PyObject *__pyx_tuple__25;
-static PyObject *__pyx_codeobj__26;
-/* Late includes */
-
-/* "monotonic_align/core.pyx":7
- * @cython.boundscheck(False)
- * @cython.wraparound(False)
- * cdef void maximum_path_each(int[:,::1] path, float[:,::1] value, int t_y, int t_x, float max_neg_val=-1e9) nogil: # <<<<<<<<<<<<<<
- * cdef int x
- * cdef int y
- */
-
-static void __pyx_f_15monotonic_align_4core_maximum_path_each(__Pyx_memviewslice __pyx_v_path, __Pyx_memviewslice __pyx_v_value, int __pyx_v_t_y, int __pyx_v_t_x, struct __pyx_opt_args_15monotonic_align_4core_maximum_path_each *__pyx_optional_args) {
- float __pyx_v_max_neg_val = __pyx_k_;
- int __pyx_v_x;
- int __pyx_v_y;
- float __pyx_v_v_prev;
- float __pyx_v_v_cur;
- int __pyx_v_index;
- int __pyx_t_1;
- int __pyx_t_2;
- int __pyx_t_3;
- long __pyx_t_4;
- int __pyx_t_5;
- long __pyx_t_6;
- long __pyx_t_7;
- int __pyx_t_8;
- Py_ssize_t __pyx_t_9;
- Py_ssize_t __pyx_t_10;
- float __pyx_t_11;
- float __pyx_t_12;
- float __pyx_t_13;
- int __pyx_t_14;
- Py_ssize_t __pyx_t_15;
- Py_ssize_t __pyx_t_16;
- if (__pyx_optional_args) {
- if (__pyx_optional_args->__pyx_n > 0) {
- __pyx_v_max_neg_val = __pyx_optional_args->max_neg_val;
- }
- }
-
- /* "monotonic_align/core.pyx":13
- * cdef float v_cur
- * cdef float tmp
- * cdef int index = t_x - 1 # <<<<<<<<<<<<<<
- *
- * for y in range(t_y):
- */
- __pyx_v_index = (__pyx_v_t_x - 1);
-
- /* "monotonic_align/core.pyx":15
- * cdef int index = t_x - 1
- *
- * for y in range(t_y): # <<<<<<<<<<<<<<
- * for x in range(max(0, t_x + y - t_y), min(t_x, y + 1)):
- * if x == y:
- */
- __pyx_t_1 = __pyx_v_t_y;
- __pyx_t_2 = __pyx_t_1;
- for (__pyx_t_3 = 0; __pyx_t_3 < __pyx_t_2; __pyx_t_3+=1) {
- __pyx_v_y = __pyx_t_3;
-
- /* "monotonic_align/core.pyx":16
- *
- * for y in range(t_y):
- * for x in range(max(0, t_x + y - t_y), min(t_x, y + 1)): # <<<<<<<<<<<<<<
- * if x == y:
- * v_cur = max_neg_val
- */
- __pyx_t_4 = (__pyx_v_y + 1);
- __pyx_t_5 = __pyx_v_t_x;
- if (((__pyx_t_4 < __pyx_t_5) != 0)) {
- __pyx_t_6 = __pyx_t_4;
- } else {
- __pyx_t_6 = __pyx_t_5;
- }
- __pyx_t_4 = __pyx_t_6;
- __pyx_t_5 = ((__pyx_v_t_x + __pyx_v_y) - __pyx_v_t_y);
- __pyx_t_6 = 0;
- if (((__pyx_t_5 > __pyx_t_6) != 0)) {
- __pyx_t_7 = __pyx_t_5;
- } else {
- __pyx_t_7 = __pyx_t_6;
- }
- __pyx_t_6 = __pyx_t_4;
- for (__pyx_t_5 = __pyx_t_7; __pyx_t_5 < __pyx_t_6; __pyx_t_5+=1) {
- __pyx_v_x = __pyx_t_5;
-
- /* "monotonic_align/core.pyx":17
- * for y in range(t_y):
- * for x in range(max(0, t_x + y - t_y), min(t_x, y + 1)):
- * if x == y: # <<<<<<<<<<<<<<
- * v_cur = max_neg_val
- * else:
- */
- __pyx_t_8 = ((__pyx_v_x == __pyx_v_y) != 0);
- if (__pyx_t_8) {
-
- /* "monotonic_align/core.pyx":18
- * for x in range(max(0, t_x + y - t_y), min(t_x, y + 1)):
- * if x == y:
- * v_cur = max_neg_val # <<<<<<<<<<<<<<
- * else:
- * v_cur = value[y-1, x]
- */
- __pyx_v_v_cur = __pyx_v_max_neg_val;
-
- /* "monotonic_align/core.pyx":17
- * for y in range(t_y):
- * for x in range(max(0, t_x + y - t_y), min(t_x, y + 1)):
- * if x == y: # <<<<<<<<<<<<<<
- * v_cur = max_neg_val
- * else:
- */
- goto __pyx_L7;
- }
-
- /* "monotonic_align/core.pyx":20
- * v_cur = max_neg_val
- * else:
- * v_cur = value[y-1, x] # <<<<<<<<<<<<<<
- * if x == 0:
- * if y == 0:
- */
- /*else*/ {
- __pyx_t_9 = (__pyx_v_y - 1);
- __pyx_t_10 = __pyx_v_x;
- __pyx_v_v_cur = (*((float *) ( /* dim=1 */ ((char *) (((float *) ( /* dim=0 */ (__pyx_v_value.data + __pyx_t_9 * __pyx_v_value.strides[0]) )) + __pyx_t_10)) )));
- }
- __pyx_L7:;
-
- /* "monotonic_align/core.pyx":21
- * else:
- * v_cur = value[y-1, x]
- * if x == 0: # <<<<<<<<<<<<<<
- * if y == 0:
- * v_prev = 0.
- */
- __pyx_t_8 = ((__pyx_v_x == 0) != 0);
- if (__pyx_t_8) {
-
- /* "monotonic_align/core.pyx":22
- * v_cur = value[y-1, x]
- * if x == 0:
- * if y == 0: # <<<<<<<<<<<<<<
- * v_prev = 0.
- * else:
- */
- __pyx_t_8 = ((__pyx_v_y == 0) != 0);
- if (__pyx_t_8) {
-
- /* "monotonic_align/core.pyx":23
- * if x == 0:
- * if y == 0:
- * v_prev = 0. # <<<<<<<<<<<<<<
- * else:
- * v_prev = max_neg_val
- */
- __pyx_v_v_prev = 0.;
-
- /* "monotonic_align/core.pyx":22
- * v_cur = value[y-1, x]
- * if x == 0:
- * if y == 0: # <<<<<<<<<<<<<<
- * v_prev = 0.
- * else:
- */
- goto __pyx_L9;
- }
-
- /* "monotonic_align/core.pyx":25
- * v_prev = 0.
- * else:
- * v_prev = max_neg_val # <<<<<<<<<<<<<<
- * else:
- * v_prev = value[y-1, x-1]
- */
- /*else*/ {
- __pyx_v_v_prev = __pyx_v_max_neg_val;
- }
- __pyx_L9:;
-
- /* "monotonic_align/core.pyx":21
- * else:
- * v_cur = value[y-1, x]
- * if x == 0: # <<<<<<<<<<<<<<
- * if y == 0:
- * v_prev = 0.
- */
- goto __pyx_L8;
- }
-
- /* "monotonic_align/core.pyx":27
- * v_prev = max_neg_val
- * else:
- * v_prev = value[y-1, x-1] # <<<<<<<<<<<<<<
- * value[y, x] += max(v_prev, v_cur)
- *
- */
- /*else*/ {
- __pyx_t_10 = (__pyx_v_y - 1);
- __pyx_t_9 = (__pyx_v_x - 1);
- __pyx_v_v_prev = (*((float *) ( /* dim=1 */ ((char *) (((float *) ( /* dim=0 */ (__pyx_v_value.data + __pyx_t_10 * __pyx_v_value.strides[0]) )) + __pyx_t_9)) )));
- }
- __pyx_L8:;
-
- /* "monotonic_align/core.pyx":28
- * else:
- * v_prev = value[y-1, x-1]
- * value[y, x] += max(v_prev, v_cur) # <<<<<<<<<<<<<<
- *
- * for y in range(t_y - 1, -1, -1):
- */
- __pyx_t_11 = __pyx_v_v_cur;
- __pyx_t_12 = __pyx_v_v_prev;
- if (((__pyx_t_11 > __pyx_t_12) != 0)) {
- __pyx_t_13 = __pyx_t_11;
- } else {
- __pyx_t_13 = __pyx_t_12;
- }
- __pyx_t_9 = __pyx_v_y;
- __pyx_t_10 = __pyx_v_x;
- *((float *) ( /* dim=1 */ ((char *) (((float *) ( /* dim=0 */ (__pyx_v_value.data + __pyx_t_9 * __pyx_v_value.strides[0]) )) + __pyx_t_10)) )) += __pyx_t_13;
- }
- }
-
- /* "monotonic_align/core.pyx":30
- * value[y, x] += max(v_prev, v_cur)
- *
- * for y in range(t_y - 1, -1, -1): # <<<<<<<<<<<<<<
- * path[y, index] = 1
- * if index != 0 and (index == y or value[y-1, index] < value[y-1, index-1]):
- */
- for (__pyx_t_1 = (__pyx_v_t_y - 1); __pyx_t_1 > -1; __pyx_t_1-=1) {
- __pyx_v_y = __pyx_t_1;
-
- /* "monotonic_align/core.pyx":31
- *
- * for y in range(t_y - 1, -1, -1):
- * path[y, index] = 1 # <<<<<<<<<<<<<<
- * if index != 0 and (index == y or value[y-1, index] < value[y-1, index-1]):
- * index = index - 1
- */
- __pyx_t_10 = __pyx_v_y;
- __pyx_t_9 = __pyx_v_index;
- *((int *) ( /* dim=1 */ ((char *) (((int *) ( /* dim=0 */ (__pyx_v_path.data + __pyx_t_10 * __pyx_v_path.strides[0]) )) + __pyx_t_9)) )) = 1;
-
- /* "monotonic_align/core.pyx":32
- * for y in range(t_y - 1, -1, -1):
- * path[y, index] = 1
- * if index != 0 and (index == y or value[y-1, index] < value[y-1, index-1]): # <<<<<<<<<<<<<<
- * index = index - 1
- *
- */
- __pyx_t_14 = ((__pyx_v_index != 0) != 0);
- if (__pyx_t_14) {
- } else {
- __pyx_t_8 = __pyx_t_14;
- goto __pyx_L13_bool_binop_done;
- }
- __pyx_t_14 = ((__pyx_v_index == __pyx_v_y) != 0);
- if (!__pyx_t_14) {
- } else {
- __pyx_t_8 = __pyx_t_14;
- goto __pyx_L13_bool_binop_done;
- }
- __pyx_t_9 = (__pyx_v_y - 1);
- __pyx_t_10 = __pyx_v_index;
- __pyx_t_15 = (__pyx_v_y - 1);
- __pyx_t_16 = (__pyx_v_index - 1);
- __pyx_t_14 = (((*((float *) ( /* dim=1 */ ((char *) (((float *) ( /* dim=0 */ (__pyx_v_value.data + __pyx_t_9 * __pyx_v_value.strides[0]) )) + __pyx_t_10)) ))) < (*((float *) ( /* dim=1 */ ((char *) (((float *) ( /* dim=0 */ (__pyx_v_value.data + __pyx_t_15 * __pyx_v_value.strides[0]) )) + __pyx_t_16)) )))) != 0);
- __pyx_t_8 = __pyx_t_14;
- __pyx_L13_bool_binop_done:;
- if (__pyx_t_8) {
-
- /* "monotonic_align/core.pyx":33
- * path[y, index] = 1
- * if index != 0 and (index == y or value[y-1, index] < value[y-1, index-1]):
- * index = index - 1 # <<<<<<<<<<<<<<
- *
- *
- */
- __pyx_v_index = (__pyx_v_index - 1);
-
- /* "monotonic_align/core.pyx":32
- * for y in range(t_y - 1, -1, -1):
- * path[y, index] = 1
- * if index != 0 and (index == y or value[y-1, index] < value[y-1, index-1]): # <<<<<<<<<<<<<<
- * index = index - 1
- *
- */
- }
- }
-
- /* "monotonic_align/core.pyx":7
- * @cython.boundscheck(False)
- * @cython.wraparound(False)
- * cdef void maximum_path_each(int[:,::1] path, float[:,::1] value, int t_y, int t_x, float max_neg_val=-1e9) nogil: # <<<<<<<<<<<<<<
- * cdef int x
- * cdef int y
- */
-
- /* function exit code */
-}
-
-/* "monotonic_align/core.pyx":38
- * @cython.boundscheck(False)
- * @cython.wraparound(False)
- * cpdef void maximum_path_c(int[:,:,::1] paths, float[:,:,::1] values, int[::1] t_ys, int[::1] t_xs) nogil: # <<<<<<<<<<<<<<
- * cdef int b = paths.shape[0]
- * cdef int i
- */
-
-static PyObject *__pyx_pw_15monotonic_align_4core_1maximum_path_c(PyObject *__pyx_self, PyObject *__pyx_args, PyObject *__pyx_kwds); /*proto*/
-static void __pyx_f_15monotonic_align_4core_maximum_path_c(__Pyx_memviewslice __pyx_v_paths, __Pyx_memviewslice __pyx_v_values, __Pyx_memviewslice __pyx_v_t_ys, __Pyx_memviewslice __pyx_v_t_xs, CYTHON_UNUSED int __pyx_skip_dispatch) {
- CYTHON_UNUSED int __pyx_v_b;
- int __pyx_v_i;
- int __pyx_t_1;
- int __pyx_t_2;
- int __pyx_t_3;
- __Pyx_memviewslice __pyx_t_4 = { 0, 0, { 0 }, { 0 }, { 0 } };
- __Pyx_memviewslice __pyx_t_5 = { 0, 0, { 0 }, { 0 }, { 0 } };
- Py_ssize_t __pyx_t_6;
- Py_ssize_t __pyx_t_7;
-
- /* "monotonic_align/core.pyx":39
- * @cython.wraparound(False)
- * cpdef void maximum_path_c(int[:,:,::1] paths, float[:,:,::1] values, int[::1] t_ys, int[::1] t_xs) nogil:
- * cdef int b = paths.shape[0] # <<<<<<<<<<<<<<
- * cdef int i
- * for i in prange(b, nogil=True):
- */
- __pyx_v_b = (__pyx_v_paths.shape[0]);
-
- /* "monotonic_align/core.pyx":41
- * cdef int b = paths.shape[0]
- * cdef int i
- * for i in prange(b, nogil=True): # <<<<<<<<<<<<<<
- * maximum_path_each(paths[i], values[i], t_ys[i], t_xs[i])
- */
- {
- #ifdef WITH_THREAD
- PyThreadState *_save;
- Py_UNBLOCK_THREADS
- __Pyx_FastGIL_Remember();
- #endif
- /*try:*/ {
- __pyx_t_1 = __pyx_v_b;
- if ((1 == 0)) abort();
- {
- #if ((defined(__APPLE__) || defined(__OSX__)) && (defined(__GNUC__) && (__GNUC__ > 2 || (__GNUC__ == 2 && (__GNUC_MINOR__ > 95)))))
- #undef likely
- #undef unlikely
- #define likely(x) (x)
- #define unlikely(x) (x)
- #endif
- __pyx_t_3 = (__pyx_t_1 - 0 + 1 - 1/abs(1)) / 1;
- if (__pyx_t_3 > 0)
- {
- #ifdef _OPENMP
- #pragma omp parallel private(__pyx_t_6, __pyx_t_7) firstprivate(__pyx_t_4, __pyx_t_5)
- #endif /* _OPENMP */
- {
- #ifdef _OPENMP
- #pragma omp for firstprivate(__pyx_v_i) lastprivate(__pyx_v_i)
- #endif /* _OPENMP */
- for (__pyx_t_2 = 0; __pyx_t_2 < __pyx_t_3; __pyx_t_2++){
- {
- __pyx_v_i = (int)(0 + 1 * __pyx_t_2);
-
- /* "monotonic_align/core.pyx":42
- * cdef int i
- * for i in prange(b, nogil=True):
- * maximum_path_each(paths[i], values[i], t_ys[i], t_xs[i]) # <<<<<<<<<<<<<<
- */
- __pyx_t_4.data = __pyx_v_paths.data;
- __pyx_t_4.memview = __pyx_v_paths.memview;
- __PYX_INC_MEMVIEW(&__pyx_t_4, 0);
- {
- Py_ssize_t __pyx_tmp_idx = __pyx_v_i;
- Py_ssize_t __pyx_tmp_stride = __pyx_v_paths.strides[0];
- __pyx_t_4.data += __pyx_tmp_idx * __pyx_tmp_stride;
-}
-
-__pyx_t_4.shape[0] = __pyx_v_paths.shape[1];
-__pyx_t_4.strides[0] = __pyx_v_paths.strides[1];
- __pyx_t_4.suboffsets[0] = -1;
-
-__pyx_t_4.shape[1] = __pyx_v_paths.shape[2];
-__pyx_t_4.strides[1] = __pyx_v_paths.strides[2];
- __pyx_t_4.suboffsets[1] = -1;
-
-__pyx_t_5.data = __pyx_v_values.data;
- __pyx_t_5.memview = __pyx_v_values.memview;
- __PYX_INC_MEMVIEW(&__pyx_t_5, 0);
- {
- Py_ssize_t __pyx_tmp_idx = __pyx_v_i;
- Py_ssize_t __pyx_tmp_stride = __pyx_v_values.strides[0];
- __pyx_t_5.data += __pyx_tmp_idx * __pyx_tmp_stride;
-}
-
-__pyx_t_5.shape[0] = __pyx_v_values.shape[1];
-__pyx_t_5.strides[0] = __pyx_v_values.strides[1];
- __pyx_t_5.suboffsets[0] = -1;
-
-__pyx_t_5.shape[1] = __pyx_v_values.shape[2];
-__pyx_t_5.strides[1] = __pyx_v_values.strides[2];
- __pyx_t_5.suboffsets[1] = -1;
-
-__pyx_t_6 = __pyx_v_i;
- __pyx_t_7 = __pyx_v_i;
- __pyx_f_15monotonic_align_4core_maximum_path_each(__pyx_t_4, __pyx_t_5, (*((int *) ( /* dim=0 */ ((char *) (((int *) __pyx_v_t_ys.data) + __pyx_t_6)) ))), (*((int *) ( /* dim=0 */ ((char *) (((int *) __pyx_v_t_xs.data) + __pyx_t_7)) ))), NULL);
- __PYX_XDEC_MEMVIEW(&__pyx_t_4, 0);
- __pyx_t_4.memview = NULL;
- __pyx_t_4.data = NULL;
- __PYX_XDEC_MEMVIEW(&__pyx_t_5, 0);
- __pyx_t_5.memview = NULL;
- __pyx_t_5.data = NULL;
- }
- }
- }
- }
- }
- #if ((defined(__APPLE__) || defined(__OSX__)) && (defined(__GNUC__) && (__GNUC__ > 2 || (__GNUC__ == 2 && (__GNUC_MINOR__ > 95)))))
- #undef likely
- #undef unlikely
- #define likely(x) __builtin_expect(!!(x), 1)
- #define unlikely(x) __builtin_expect(!!(x), 0)
- #endif
- }
-
- /* "monotonic_align/core.pyx":41
- * cdef int b = paths.shape[0]
- * cdef int i
- * for i in prange(b, nogil=True): # <<<<<<<<<<<<<<
- * maximum_path_each(paths[i], values[i], t_ys[i], t_xs[i])
- */
- /*finally:*/ {
- /*normal exit:*/{
- #ifdef WITH_THREAD
- __Pyx_FastGIL_Forget();
- Py_BLOCK_THREADS
- #endif
- goto __pyx_L5;
- }
- __pyx_L5:;
- }
- }
-
- /* "monotonic_align/core.pyx":38
- * @cython.boundscheck(False)
- * @cython.wraparound(False)
- * cpdef void maximum_path_c(int[:,:,::1] paths, float[:,:,::1] values, int[::1] t_ys, int[::1] t_xs) nogil: # <<<<<<<<<<<<<<
- * cdef int b = paths.shape[0]
- * cdef int i
- */
-
- /* function exit code */
-}
-
-/* Python wrapper */
-static PyObject *__pyx_pw_15monotonic_align_4core_1maximum_path_c(PyObject *__pyx_self, PyObject *__pyx_args, PyObject *__pyx_kwds); /*proto*/
-static PyObject *__pyx_pw_15monotonic_align_4core_1maximum_path_c(PyObject *__pyx_self, PyObject *__pyx_args, PyObject *__pyx_kwds) {
- __Pyx_memviewslice __pyx_v_paths = { 0, 0, { 0 }, { 0 }, { 0 } };
- __Pyx_memviewslice __pyx_v_values = { 0, 0, { 0 }, { 0 }, { 0 } };
- __Pyx_memviewslice __pyx_v_t_ys = { 0, 0, { 0 }, { 0 }, { 0 } };
- __Pyx_memviewslice __pyx_v_t_xs = { 0, 0, { 0 }, { 0 }, { 0 } };
- int __pyx_lineno = 0;
- const char *__pyx_filename = NULL;
- int __pyx_clineno = 0;
- PyObject *__pyx_r = 0;
- __Pyx_RefNannyDeclarations
- __Pyx_RefNannySetupContext("maximum_path_c (wrapper)", 0);
- {
- static PyObject **__pyx_pyargnames[] = {&__pyx_n_s_paths,&__pyx_n_s_values,&__pyx_n_s_t_ys,&__pyx_n_s_t_xs,0};
- PyObject* values[4] = {0,0,0,0};
- if (unlikely(__pyx_kwds)) {
- Py_ssize_t kw_args;
- const Py_ssize_t pos_args = PyTuple_GET_SIZE(__pyx_args);
- switch (pos_args) {
- case 4: values[3] = PyTuple_GET_ITEM(__pyx_args, 3);
- CYTHON_FALLTHROUGH;
- case 3: values[2] = PyTuple_GET_ITEM(__pyx_args, 2);
- CYTHON_FALLTHROUGH;
- case 2: values[1] = PyTuple_GET_ITEM(__pyx_args, 1);
- CYTHON_FALLTHROUGH;
- case 1: values[0] = PyTuple_GET_ITEM(__pyx_args, 0);
- CYTHON_FALLTHROUGH;
- case 0: break;
- default: goto __pyx_L5_argtuple_error;
- }
- kw_args = PyDict_Size(__pyx_kwds);
- switch (pos_args) {
- case 0:
- if (likely((values[0] = __Pyx_PyDict_GetItemStr(__pyx_kwds, __pyx_n_s_paths)) != 0)) kw_args--;
- else goto __pyx_L5_argtuple_error;
- CYTHON_FALLTHROUGH;
- case 1:
- if (likely((values[1] = __Pyx_PyDict_GetItemStr(__pyx_kwds, __pyx_n_s_values)) != 0)) kw_args--;
- else {
- __Pyx_RaiseArgtupleInvalid("maximum_path_c", 1, 4, 4, 1); __PYX_ERR(0, 38, __pyx_L3_error)
- }
- CYTHON_FALLTHROUGH;
- case 2:
- if (likely((values[2] = __Pyx_PyDict_GetItemStr(__pyx_kwds, __pyx_n_s_t_ys)) != 0)) kw_args--;
- else {
- __Pyx_RaiseArgtupleInvalid("maximum_path_c", 1, 4, 4, 2); __PYX_ERR(0, 38, __pyx_L3_error)
- }
- CYTHON_FALLTHROUGH;
- case 3:
- if (likely((values[3] = __Pyx_PyDict_GetItemStr(__pyx_kwds, __pyx_n_s_t_xs)) != 0)) kw_args--;
- else {
- __Pyx_RaiseArgtupleInvalid("maximum_path_c", 1, 4, 4, 3); __PYX_ERR(0, 38, __pyx_L3_error)
- }
- }
- if (unlikely(kw_args > 0)) {
- if (unlikely(__Pyx_ParseOptionalKeywords(__pyx_kwds, __pyx_pyargnames, 0, values, pos_args, "maximum_path_c") < 0)) __PYX_ERR(0, 38, __pyx_L3_error)
- }
- } else if (PyTuple_GET_SIZE(__pyx_args) != 4) {
- goto __pyx_L5_argtuple_error;
- } else {
- values[0] = PyTuple_GET_ITEM(__pyx_args, 0);
- values[1] = PyTuple_GET_ITEM(__pyx_args, 1);
- values[2] = PyTuple_GET_ITEM(__pyx_args, 2);
- values[3] = PyTuple_GET_ITEM(__pyx_args, 3);
- }
- __pyx_v_paths = __Pyx_PyObject_to_MemoryviewSlice_d_d_dc_int(values[0], PyBUF_WRITABLE); if (unlikely(!__pyx_v_paths.memview)) __PYX_ERR(0, 38, __pyx_L3_error)
- __pyx_v_values = __Pyx_PyObject_to_MemoryviewSlice_d_d_dc_float(values[1], PyBUF_WRITABLE); if (unlikely(!__pyx_v_values.memview)) __PYX_ERR(0, 38, __pyx_L3_error)
- __pyx_v_t_ys = __Pyx_PyObject_to_MemoryviewSlice_dc_int(values[2], PyBUF_WRITABLE); if (unlikely(!__pyx_v_t_ys.memview)) __PYX_ERR(0, 38, __pyx_L3_error)
- __pyx_v_t_xs = __Pyx_PyObject_to_MemoryviewSlice_dc_int(values[3], PyBUF_WRITABLE); if (unlikely(!__pyx_v_t_xs.memview)) __PYX_ERR(0, 38, __pyx_L3_error)
- }
- goto __pyx_L4_argument_unpacking_done;
- __pyx_L5_argtuple_error:;
- __Pyx_RaiseArgtupleInvalid("maximum_path_c", 1, 4, 4, PyTuple_GET_SIZE(__pyx_args)); __PYX_ERR(0, 38, __pyx_L3_error)
- __pyx_L3_error:;
- __Pyx_AddTraceback("monotonic_align.core.maximum_path_c", __pyx_clineno, __pyx_lineno, __pyx_filename);
- __Pyx_RefNannyFinishContext();
- return NULL;
- __pyx_L4_argument_unpacking_done:;
- __pyx_r = __pyx_pf_15monotonic_align_4core_maximum_path_c(__pyx_self, __pyx_v_paths, __pyx_v_values, __pyx_v_t_ys, __pyx_v_t_xs);
-
- /* function exit code */
- __Pyx_RefNannyFinishContext();
- return __pyx_r;
-}
-
-static PyObject *__pyx_pf_15monotonic_align_4core_maximum_path_c(CYTHON_UNUSED PyObject *__pyx_self, __Pyx_memviewslice __pyx_v_paths, __Pyx_memviewslice __pyx_v_values, __Pyx_memviewslice __pyx_v_t_ys, __Pyx_memviewslice __pyx_v_t_xs) {
- PyObject *__pyx_r = NULL;
- __Pyx_RefNannyDeclarations
- PyObject *__pyx_t_1 = NULL;
- int __pyx_lineno = 0;
- const char *__pyx_filename = NULL;
- int __pyx_clineno = 0;
- __Pyx_RefNannySetupContext("maximum_path_c", 0);
- __Pyx_XDECREF(__pyx_r);
- if (unlikely(!__pyx_v_paths.memview)) { __Pyx_RaiseUnboundLocalError("paths"); __PYX_ERR(0, 38, __pyx_L1_error) }
- if (unlikely(!__pyx_v_values.memview)) { __Pyx_RaiseUnboundLocalError("values"); __PYX_ERR(0, 38, __pyx_L1_error) }
- if (unlikely(!__pyx_v_t_ys.memview)) { __Pyx_RaiseUnboundLocalError("t_ys"); __PYX_ERR(0, 38, __pyx_L1_error) }
- if (unlikely(!__pyx_v_t_xs.memview)) { __Pyx_RaiseUnboundLocalError("t_xs"); __PYX_ERR(0, 38, __pyx_L1_error) }
- __pyx_t_1 = __Pyx_void_to_None(__pyx_f_15monotonic_align_4core_maximum_path_c(__pyx_v_paths, __pyx_v_values, __pyx_v_t_ys, __pyx_v_t_xs, 0)); if (unlikely(!__pyx_t_1)) __PYX_ERR(0, 38, __pyx_L1_error)
- __Pyx_GOTREF(__pyx_t_1);
- __pyx_r = __pyx_t_1;
- __pyx_t_1 = 0;
- goto __pyx_L0;
-
- /* function exit code */
- __pyx_L1_error:;
- __Pyx_XDECREF(__pyx_t_1);
- __Pyx_AddTraceback("monotonic_align.core.maximum_path_c", __pyx_clineno, __pyx_lineno, __pyx_filename);
- __pyx_r = NULL;
- __pyx_L0:;
- __PYX_XDEC_MEMVIEW(&__pyx_v_paths, 1);
- __PYX_XDEC_MEMVIEW(&__pyx_v_values, 1);
- __PYX_XDEC_MEMVIEW(&__pyx_v_t_ys, 1);
- __PYX_XDEC_MEMVIEW(&__pyx_v_t_xs, 1);
- __Pyx_XGIVEREF(__pyx_r);
- __Pyx_RefNannyFinishContext();
- return __pyx_r;
-}
-
-/* "View.MemoryView":122
- * cdef bint dtype_is_object
- *
- * def __cinit__(array self, tuple shape, Py_ssize_t itemsize, format not None, # <<<<<<<<<<<<<<
- * mode="c", bint allocate_buffer=True):
- *
- */
-
-/* Python wrapper */
-static int __pyx_array___cinit__(PyObject *__pyx_v_self, PyObject *__pyx_args, PyObject *__pyx_kwds); /*proto*/
-static int __pyx_array___cinit__(PyObject *__pyx_v_self, PyObject *__pyx_args, PyObject *__pyx_kwds) {
- PyObject *__pyx_v_shape = 0;
- Py_ssize_t __pyx_v_itemsize;
- PyObject *__pyx_v_format = 0;
- PyObject *__pyx_v_mode = 0;
- int __pyx_v_allocate_buffer;
- int __pyx_lineno = 0;
- const char *__pyx_filename = NULL;
- int __pyx_clineno = 0;
- int __pyx_r;
- __Pyx_RefNannyDeclarations
- __Pyx_RefNannySetupContext("__cinit__ (wrapper)", 0);
- {
- static PyObject **__pyx_pyargnames[] = {&__pyx_n_s_shape,&__pyx_n_s_itemsize,&__pyx_n_s_format,&__pyx_n_s_mode,&__pyx_n_s_allocate_buffer,0};
- PyObject* values[5] = {0,0,0,0,0};
- values[3] = ((PyObject *)__pyx_n_s_c);
- if (unlikely(__pyx_kwds)) {
- Py_ssize_t kw_args;
- const Py_ssize_t pos_args = PyTuple_GET_SIZE(__pyx_args);
- switch (pos_args) {
- case 5: values[4] = PyTuple_GET_ITEM(__pyx_args, 4);
- CYTHON_FALLTHROUGH;
- case 4: values[3] = PyTuple_GET_ITEM(__pyx_args, 3);
- CYTHON_FALLTHROUGH;
- case 3: values[2] = PyTuple_GET_ITEM(__pyx_args, 2);
- CYTHON_FALLTHROUGH;
- case 2: values[1] = PyTuple_GET_ITEM(__pyx_args, 1);
- CYTHON_FALLTHROUGH;
- case 1: values[0] = PyTuple_GET_ITEM(__pyx_args, 0);
- CYTHON_FALLTHROUGH;
- case 0: break;
- default: goto __pyx_L5_argtuple_error;
- }
- kw_args = PyDict_Size(__pyx_kwds);
- switch (pos_args) {
- case 0:
- if (likely((values[0] = __Pyx_PyDict_GetItemStr(__pyx_kwds, __pyx_n_s_shape)) != 0)) kw_args--;
- else goto __pyx_L5_argtuple_error;
- CYTHON_FALLTHROUGH;
- case 1:
- if (likely((values[1] = __Pyx_PyDict_GetItemStr(__pyx_kwds, __pyx_n_s_itemsize)) != 0)) kw_args--;
- else {
- __Pyx_RaiseArgtupleInvalid("__cinit__", 0, 3, 5, 1); __PYX_ERR(1, 122, __pyx_L3_error)
- }
- CYTHON_FALLTHROUGH;
- case 2:
- if (likely((values[2] = __Pyx_PyDict_GetItemStr(__pyx_kwds, __pyx_n_s_format)) != 0)) kw_args--;
- else {
- __Pyx_RaiseArgtupleInvalid("__cinit__", 0, 3, 5, 2); __PYX_ERR(1, 122, __pyx_L3_error)
- }
- CYTHON_FALLTHROUGH;
- case 3:
- if (kw_args > 0) {
- PyObject* value = __Pyx_PyDict_GetItemStr(__pyx_kwds, __pyx_n_s_mode);
- if (value) { values[3] = value; kw_args--; }
- }
- CYTHON_FALLTHROUGH;
- case 4:
- if (kw_args > 0) {
- PyObject* value = __Pyx_PyDict_GetItemStr(__pyx_kwds, __pyx_n_s_allocate_buffer);
- if (value) { values[4] = value; kw_args--; }
- }
- }
- if (unlikely(kw_args > 0)) {
- if (unlikely(__Pyx_ParseOptionalKeywords(__pyx_kwds, __pyx_pyargnames, 0, values, pos_args, "__cinit__") < 0)) __PYX_ERR(1, 122, __pyx_L3_error)
- }
- } else {
- switch (PyTuple_GET_SIZE(__pyx_args)) {
- case 5: values[4] = PyTuple_GET_ITEM(__pyx_args, 4);
- CYTHON_FALLTHROUGH;
- case 4: values[3] = PyTuple_GET_ITEM(__pyx_args, 3);
- CYTHON_FALLTHROUGH;
- case 3: values[2] = PyTuple_GET_ITEM(__pyx_args, 2);
- values[1] = PyTuple_GET_ITEM(__pyx_args, 1);
- values[0] = PyTuple_GET_ITEM(__pyx_args, 0);
- break;
- default: goto __pyx_L5_argtuple_error;
- }
- }
- __pyx_v_shape = ((PyObject*)values[0]);
- __pyx_v_itemsize = __Pyx_PyIndex_AsSsize_t(values[1]); if (unlikely((__pyx_v_itemsize == (Py_ssize_t)-1) && PyErr_Occurred())) __PYX_ERR(1, 122, __pyx_L3_error)
- __pyx_v_format = values[2];
- __pyx_v_mode = values[3];
- if (values[4]) {
- __pyx_v_allocate_buffer = __Pyx_PyObject_IsTrue(values[4]); if (unlikely((__pyx_v_allocate_buffer == (int)-1) && PyErr_Occurred())) __PYX_ERR(1, 123, __pyx_L3_error)
- } else {
-
- /* "View.MemoryView":123
- *
- * def __cinit__(array self, tuple shape, Py_ssize_t itemsize, format not None,
- * mode="c", bint allocate_buffer=True): # <<<<<<<<<<<<<<
- *
- * cdef int idx
- */
- __pyx_v_allocate_buffer = ((int)1);
- }
- }
- goto __pyx_L4_argument_unpacking_done;
- __pyx_L5_argtuple_error:;
- __Pyx_RaiseArgtupleInvalid("__cinit__", 0, 3, 5, PyTuple_GET_SIZE(__pyx_args)); __PYX_ERR(1, 122, __pyx_L3_error)
- __pyx_L3_error:;
- __Pyx_AddTraceback("View.MemoryView.array.__cinit__", __pyx_clineno, __pyx_lineno, __pyx_filename);
- __Pyx_RefNannyFinishContext();
- return -1;
- __pyx_L4_argument_unpacking_done:;
- if (unlikely(!__Pyx_ArgTypeTest(((PyObject *)__pyx_v_shape), (&PyTuple_Type), 1, "shape", 1))) __PYX_ERR(1, 122, __pyx_L1_error)
- if (unlikely(((PyObject *)__pyx_v_format) == Py_None)) {
- PyErr_Format(PyExc_TypeError, "Argument '%.200s' must not be None", "format"); __PYX_ERR(1, 122, __pyx_L1_error)
- }
- __pyx_r = __pyx_array___pyx_pf_15View_dot_MemoryView_5array___cinit__(((struct __pyx_array_obj *)__pyx_v_self), __pyx_v_shape, __pyx_v_itemsize, __pyx_v_format, __pyx_v_mode, __pyx_v_allocate_buffer);
-
- /* "View.MemoryView":122
- * cdef bint dtype_is_object
- *
- * def __cinit__(array self, tuple shape, Py_ssize_t itemsize, format not None, # <<<<<<<<<<<<<<
- * mode="c", bint allocate_buffer=True):
- *
- */
-
- /* function exit code */
- goto __pyx_L0;
- __pyx_L1_error:;
- __pyx_r = -1;
- __pyx_L0:;
- __Pyx_RefNannyFinishContext();
- return __pyx_r;
-}
-
-static int __pyx_array___pyx_pf_15View_dot_MemoryView_5array___cinit__(struct __pyx_array_obj *__pyx_v_self, PyObject *__pyx_v_shape, Py_ssize_t __pyx_v_itemsize, PyObject *__pyx_v_format, PyObject *__pyx_v_mode, int __pyx_v_allocate_buffer) {
- int __pyx_v_idx;
- Py_ssize_t __pyx_v_i;
- Py_ssize_t __pyx_v_dim;
- PyObject **__pyx_v_p;
- char __pyx_v_order;
- int __pyx_r;
- __Pyx_RefNannyDeclarations
- Py_ssize_t __pyx_t_1;
- int __pyx_t_2;
- PyObject *__pyx_t_3 = NULL;
- int __pyx_t_4;
- PyObject *__pyx_t_5 = NULL;
- PyObject *__pyx_t_6 = NULL;
- char *__pyx_t_7;
- int __pyx_t_8;
- Py_ssize_t __pyx_t_9;
- PyObject *__pyx_t_10 = NULL;
- Py_ssize_t __pyx_t_11;
- int __pyx_lineno = 0;
- const char *__pyx_filename = NULL;
- int __pyx_clineno = 0;
- __Pyx_RefNannySetupContext("__cinit__", 0);
- __Pyx_INCREF(__pyx_v_format);
-
- /* "View.MemoryView":129
- * cdef PyObject **p
- *
- * self.ndim = len(shape) # <<<<<<<<<<<<<<
- * self.itemsize = itemsize
- *
- */
- if (unlikely(__pyx_v_shape == Py_None)) {
- PyErr_SetString(PyExc_TypeError, "object of type 'NoneType' has no len()");
- __PYX_ERR(1, 129, __pyx_L1_error)
- }
- __pyx_t_1 = PyTuple_GET_SIZE(__pyx_v_shape); if (unlikely(__pyx_t_1 == ((Py_ssize_t)-1))) __PYX_ERR(1, 129, __pyx_L1_error)
- __pyx_v_self->ndim = ((int)__pyx_t_1);
-
- /* "View.MemoryView":130
- *
- * self.ndim = len(shape)
- * self.itemsize = itemsize # <<<<<<<<<<<<<<
- *
- * if not self.ndim:
- */
- __pyx_v_self->itemsize = __pyx_v_itemsize;
-
- /* "View.MemoryView":132
- * self.itemsize = itemsize
- *
- * if not self.ndim: # <<<<<<<<<<<<<<
- * raise ValueError("Empty shape tuple for cython.array")
- *
- */
- __pyx_t_2 = ((!(__pyx_v_self->ndim != 0)) != 0);
- if (unlikely(__pyx_t_2)) {
-
- /* "View.MemoryView":133
- *
- * if not self.ndim:
- * raise ValueError("Empty shape tuple for cython.array") # <<<<<<<<<<<<<<
- *
- * if itemsize <= 0:
- */
- __pyx_t_3 = __Pyx_PyObject_Call(__pyx_builtin_ValueError, __pyx_tuple__2, NULL); if (unlikely(!__pyx_t_3)) __PYX_ERR(1, 133, __pyx_L1_error)
- __Pyx_GOTREF(__pyx_t_3);
- __Pyx_Raise(__pyx_t_3, 0, 0, 0);
- __Pyx_DECREF(__pyx_t_3); __pyx_t_3 = 0;
- __PYX_ERR(1, 133, __pyx_L1_error)
-
- /* "View.MemoryView":132
- * self.itemsize = itemsize
- *
- * if not self.ndim: # <<<<<<<<<<<<<<
- * raise ValueError("Empty shape tuple for cython.array")
- *
- */
- }
-
- /* "View.MemoryView":135
- * raise ValueError("Empty shape tuple for cython.array")
- *
- * if itemsize <= 0: # <<<<<<<<<<<<<<
- * raise ValueError("itemsize <= 0 for cython.array")
- *
- */
- __pyx_t_2 = ((__pyx_v_itemsize <= 0) != 0);
- if (unlikely(__pyx_t_2)) {
-
- /* "View.MemoryView":136
- *
- * if itemsize <= 0:
- * raise ValueError("itemsize <= 0 for cython.array") # <<<<<<<<<<<<<<
- *
- * if not isinstance(format, bytes):
- */
- __pyx_t_3 = __Pyx_PyObject_Call(__pyx_builtin_ValueError, __pyx_tuple__3, NULL); if (unlikely(!__pyx_t_3)) __PYX_ERR(1, 136, __pyx_L1_error)
- __Pyx_GOTREF(__pyx_t_3);
- __Pyx_Raise(__pyx_t_3, 0, 0, 0);
- __Pyx_DECREF(__pyx_t_3); __pyx_t_3 = 0;
- __PYX_ERR(1, 136, __pyx_L1_error)
-
- /* "View.MemoryView":135
- * raise ValueError("Empty shape tuple for cython.array")
- *
- * if itemsize <= 0: # <<<<<<<<<<<<<<
- * raise ValueError("itemsize <= 0 for cython.array")
- *
- */
- }
-
- /* "View.MemoryView":138
- * raise ValueError("itemsize <= 0 for cython.array")
- *
- * if not isinstance(format, bytes): # <<<<<<<<<<<<<<
- * format = format.encode('ASCII')
- * self._format = format # keep a reference to the byte string
- */
- __pyx_t_2 = PyBytes_Check(__pyx_v_format);
- __pyx_t_4 = ((!(__pyx_t_2 != 0)) != 0);
- if (__pyx_t_4) {
-
- /* "View.MemoryView":139
- *
- * if not isinstance(format, bytes):
- * format = format.encode('ASCII') # <<<<<<<<<<<<<<
- * self._format = format # keep a reference to the byte string
- * self.format = self._format
- */
- __pyx_t_5 = __Pyx_PyObject_GetAttrStr(__pyx_v_format, __pyx_n_s_encode); if (unlikely(!__pyx_t_5)) __PYX_ERR(1, 139, __pyx_L1_error)
- __Pyx_GOTREF(__pyx_t_5);
- __pyx_t_6 = NULL;
- if (CYTHON_UNPACK_METHODS && likely(PyMethod_Check(__pyx_t_5))) {
- __pyx_t_6 = PyMethod_GET_SELF(__pyx_t_5);
- if (likely(__pyx_t_6)) {
- PyObject* function = PyMethod_GET_FUNCTION(__pyx_t_5);
- __Pyx_INCREF(__pyx_t_6);
- __Pyx_INCREF(function);
- __Pyx_DECREF_SET(__pyx_t_5, function);
- }
- }
- __pyx_t_3 = (__pyx_t_6) ? __Pyx_PyObject_Call2Args(__pyx_t_5, __pyx_t_6, __pyx_n_s_ASCII) : __Pyx_PyObject_CallOneArg(__pyx_t_5, __pyx_n_s_ASCII);
- __Pyx_XDECREF(__pyx_t_6); __pyx_t_6 = 0;
- if (unlikely(!__pyx_t_3)) __PYX_ERR(1, 139, __pyx_L1_error)
- __Pyx_GOTREF(__pyx_t_3);
- __Pyx_DECREF(__pyx_t_5); __pyx_t_5 = 0;
- __Pyx_DECREF_SET(__pyx_v_format, __pyx_t_3);
- __pyx_t_3 = 0;
-
- /* "View.MemoryView":138
- * raise ValueError("itemsize <= 0 for cython.array")
- *
- * if not isinstance(format, bytes): # <<<<<<<<<<<<<<
- * format = format.encode('ASCII')
- * self._format = format # keep a reference to the byte string
- */
- }
-
- /* "View.MemoryView":140
- * if not isinstance(format, bytes):
- * format = format.encode('ASCII')
- * self._format = format # keep a reference to the byte string # <<<<<<<<<<<<<<
- * self.format = self._format
- *
- */
- if (!(likely(PyBytes_CheckExact(__pyx_v_format))||((__pyx_v_format) == Py_None)||(PyErr_Format(PyExc_TypeError, "Expected %.16s, got %.200s", "bytes", Py_TYPE(__pyx_v_format)->tp_name), 0))) __PYX_ERR(1, 140, __pyx_L1_error)
- __pyx_t_3 = __pyx_v_format;
- __Pyx_INCREF(__pyx_t_3);
- __Pyx_GIVEREF(__pyx_t_3);
- __Pyx_GOTREF(__pyx_v_self->_format);
- __Pyx_DECREF(__pyx_v_self->_format);
- __pyx_v_self->_format = ((PyObject*)__pyx_t_3);
- __pyx_t_3 = 0;
-
- /* "View.MemoryView":141
- * format = format.encode('ASCII')
- * self._format = format # keep a reference to the byte string
- * self.format = self._format # <<<<<<<<<<<<<<
- *
- *
- */
- if (unlikely(__pyx_v_self->_format == Py_None)) {
- PyErr_SetString(PyExc_TypeError, "expected bytes, NoneType found");
- __PYX_ERR(1, 141, __pyx_L1_error)
- }
- __pyx_t_7 = __Pyx_PyBytes_AsWritableString(__pyx_v_self->_format); if (unlikely((!__pyx_t_7) && PyErr_Occurred())) __PYX_ERR(1, 141, __pyx_L1_error)
- __pyx_v_self->format = __pyx_t_7;
-
- /* "View.MemoryView":144
- *
- *
- * self._shape = PyObject_Malloc(sizeof(Py_ssize_t)*self.ndim*2) # <<<<<<<<<<<<<<
- * self._strides = self._shape + self.ndim
- *
- */
- __pyx_v_self->_shape = ((Py_ssize_t *)PyObject_Malloc((((sizeof(Py_ssize_t)) * __pyx_v_self->ndim) * 2)));
-
- /* "View.MemoryView":145
- *
- * self._shape = PyObject_Malloc(sizeof(Py_ssize_t)*self.ndim*2)
- * self._strides = self._shape + self.ndim # <<<<<<<<<<<<<<
- *
- * if not self._shape:
- */
- __pyx_v_self->_strides = (__pyx_v_self->_shape + __pyx_v_self->ndim);
-
- /* "View.MemoryView":147
- * self._strides = self._shape + self.ndim
- *
- * if not self._shape: # <<<<<<<<<<<<<<
- * raise MemoryError("unable to allocate shape and strides.")
- *
- */
- __pyx_t_4 = ((!(__pyx_v_self->_shape != 0)) != 0);
- if (unlikely(__pyx_t_4)) {
-
- /* "View.MemoryView":148
- *
- * if not self._shape:
- * raise MemoryError("unable to allocate shape and strides.") # <<<<<<<<<<<<<<
- *
- *
- */
- __pyx_t_3 = __Pyx_PyObject_Call(__pyx_builtin_MemoryError, __pyx_tuple__4, NULL); if (unlikely(!__pyx_t_3)) __PYX_ERR(1, 148, __pyx_L1_error)
- __Pyx_GOTREF(__pyx_t_3);
- __Pyx_Raise(__pyx_t_3, 0, 0, 0);
- __Pyx_DECREF(__pyx_t_3); __pyx_t_3 = 0;
- __PYX_ERR(1, 148, __pyx_L1_error)
-
- /* "View.MemoryView":147
- * self._strides = self._shape + self.ndim
- *
- * if not self._shape: # <<<<<<<<<<<<<<
- * raise MemoryError("unable to allocate shape and strides.")
- *
- */
- }
-
- /* "View.MemoryView":151
- *
- *
- * for idx, dim in enumerate(shape): # <<<<<<<<<<<<<<
- * if dim <= 0:
- * raise ValueError("Invalid shape in axis %d: %d." % (idx, dim))
- */
- __pyx_t_8 = 0;
- __pyx_t_3 = __pyx_v_shape; __Pyx_INCREF(__pyx_t_3); __pyx_t_1 = 0;
- for (;;) {
- if (__pyx_t_1 >= PyTuple_GET_SIZE(__pyx_t_3)) break;
- #if CYTHON_ASSUME_SAFE_MACROS && !CYTHON_AVOID_BORROWED_REFS
- __pyx_t_5 = PyTuple_GET_ITEM(__pyx_t_3, __pyx_t_1); __Pyx_INCREF(__pyx_t_5); __pyx_t_1++; if (unlikely(0 < 0)) __PYX_ERR(1, 151, __pyx_L1_error)
- #else
- __pyx_t_5 = PySequence_ITEM(__pyx_t_3, __pyx_t_1); __pyx_t_1++; if (unlikely(!__pyx_t_5)) __PYX_ERR(1, 151, __pyx_L1_error)
- __Pyx_GOTREF(__pyx_t_5);
- #endif
- __pyx_t_9 = __Pyx_PyIndex_AsSsize_t(__pyx_t_5); if (unlikely((__pyx_t_9 == (Py_ssize_t)-1) && PyErr_Occurred())) __PYX_ERR(1, 151, __pyx_L1_error)
- __Pyx_DECREF(__pyx_t_5); __pyx_t_5 = 0;
- __pyx_v_dim = __pyx_t_9;
- __pyx_v_idx = __pyx_t_8;
- __pyx_t_8 = (__pyx_t_8 + 1);
-
- /* "View.MemoryView":152
- *
- * for idx, dim in enumerate(shape):
- * if dim <= 0: # <<<<<<<<<<<<<<
- * raise ValueError("Invalid shape in axis %d: %d." % (idx, dim))
- * self._shape[idx] = dim
- */
- __pyx_t_4 = ((__pyx_v_dim <= 0) != 0);
- if (unlikely(__pyx_t_4)) {
-
- /* "View.MemoryView":153
- * for idx, dim in enumerate(shape):
- * if dim <= 0:
- * raise ValueError("Invalid shape in axis %d: %d." % (idx, dim)) # <<<<<<<<<<<<<<
- * self._shape[idx] = dim
- *
- */
- __pyx_t_5 = __Pyx_PyInt_From_int(__pyx_v_idx); if (unlikely(!__pyx_t_5)) __PYX_ERR(1, 153, __pyx_L1_error)
- __Pyx_GOTREF(__pyx_t_5);
- __pyx_t_6 = PyInt_FromSsize_t(__pyx_v_dim); if (unlikely(!__pyx_t_6)) __PYX_ERR(1, 153, __pyx_L1_error)
- __Pyx_GOTREF(__pyx_t_6);
- __pyx_t_10 = PyTuple_New(2); if (unlikely(!__pyx_t_10)) __PYX_ERR(1, 153, __pyx_L1_error)
- __Pyx_GOTREF(__pyx_t_10);
- __Pyx_GIVEREF(__pyx_t_5);
- PyTuple_SET_ITEM(__pyx_t_10, 0, __pyx_t_5);
- __Pyx_GIVEREF(__pyx_t_6);
- PyTuple_SET_ITEM(__pyx_t_10, 1, __pyx_t_6);
- __pyx_t_5 = 0;
- __pyx_t_6 = 0;
- __pyx_t_6 = __Pyx_PyString_Format(__pyx_kp_s_Invalid_shape_in_axis_d_d, __pyx_t_10); if (unlikely(!__pyx_t_6)) __PYX_ERR(1, 153, __pyx_L1_error)
- __Pyx_GOTREF(__pyx_t_6);
- __Pyx_DECREF(__pyx_t_10); __pyx_t_10 = 0;
- __pyx_t_10 = __Pyx_PyObject_CallOneArg(__pyx_builtin_ValueError, __pyx_t_6); if (unlikely(!__pyx_t_10)) __PYX_ERR(1, 153, __pyx_L1_error)
- __Pyx_GOTREF(__pyx_t_10);
- __Pyx_DECREF(__pyx_t_6); __pyx_t_6 = 0;
- __Pyx_Raise(__pyx_t_10, 0, 0, 0);
- __Pyx_DECREF(__pyx_t_10); __pyx_t_10 = 0;
- __PYX_ERR(1, 153, __pyx_L1_error)
-
- /* "View.MemoryView":152
- *
- * for idx, dim in enumerate(shape):
- * if dim <= 0: # <<<<<<<<<<<<<<
- * raise ValueError("Invalid shape in axis %d: %d." % (idx, dim))
- * self._shape[idx] = dim
- */
- }
-
- /* "View.MemoryView":154
- * if dim <= 0:
- * raise ValueError("Invalid shape in axis %d: %d." % (idx, dim))
- * self._shape[idx] = dim # <<<<<<<<<<<<<<
- *
- * cdef char order
- */
- (__pyx_v_self->_shape[__pyx_v_idx]) = __pyx_v_dim;
-
- /* "View.MemoryView":151
- *
- *
- * for idx, dim in enumerate(shape): # <<<<<<<<<<<<<<
- * if dim <= 0:
- * raise ValueError("Invalid shape in axis %d: %d." % (idx, dim))
- */
- }
- __Pyx_DECREF(__pyx_t_3); __pyx_t_3 = 0;
-
- /* "View.MemoryView":157
- *
- * cdef char order
- * if mode == 'fortran': # <<<<<<<<<<<<<<
- * order = b'F'
- * self.mode = u'fortran'
- */
- __pyx_t_4 = (__Pyx_PyString_Equals(__pyx_v_mode, __pyx_n_s_fortran, Py_EQ)); if (unlikely(__pyx_t_4 < 0)) __PYX_ERR(1, 157, __pyx_L1_error)
- if (__pyx_t_4) {
-
- /* "View.MemoryView":158
- * cdef char order
- * if mode == 'fortran':
- * order = b'F' # <<<<<<<<<<<<<<
- * self.mode = u'fortran'
- * elif mode == 'c':
- */
- __pyx_v_order = 'F';
-
- /* "View.MemoryView":159
- * if mode == 'fortran':
- * order = b'F'
- * self.mode = u'fortran' # <<<<<<<<<<<<<<
- * elif mode == 'c':
- * order = b'C'
- */
- __Pyx_INCREF(__pyx_n_u_fortran);
- __Pyx_GIVEREF(__pyx_n_u_fortran);
- __Pyx_GOTREF(__pyx_v_self->mode);
- __Pyx_DECREF(__pyx_v_self->mode);
- __pyx_v_self->mode = __pyx_n_u_fortran;
-
- /* "View.MemoryView":157
- *
- * cdef char order
- * if mode == 'fortran': # <<<<<<<<<<<<<<
- * order = b'F'
- * self.mode = u'fortran'
- */
- goto __pyx_L10;
- }
-
- /* "View.MemoryView":160
- * order = b'F'
- * self.mode = u'fortran'
- * elif mode == 'c': # <<<<<<<<<<<<<<
- * order = b'C'
- * self.mode = u'c'
- */
- __pyx_t_4 = (__Pyx_PyString_Equals(__pyx_v_mode, __pyx_n_s_c, Py_EQ)); if (unlikely(__pyx_t_4 < 0)) __PYX_ERR(1, 160, __pyx_L1_error)
- if (likely(__pyx_t_4)) {
-
- /* "View.MemoryView":161
- * self.mode = u'fortran'
- * elif mode == 'c':
- * order = b'C' # <<<<<<<<<<<<<<
- * self.mode = u'c'
- * else:
- */
- __pyx_v_order = 'C';
-
- /* "View.MemoryView":162
- * elif mode == 'c':
- * order = b'C'
- * self.mode = u'c' # <<<<<<<<<<<<<<
- * else:
- * raise ValueError("Invalid mode, expected 'c' or 'fortran', got %s" % mode)
- */
- __Pyx_INCREF(__pyx_n_u_c);
- __Pyx_GIVEREF(__pyx_n_u_c);
- __Pyx_GOTREF(__pyx_v_self->mode);
- __Pyx_DECREF(__pyx_v_self->mode);
- __pyx_v_self->mode = __pyx_n_u_c;
-
- /* "View.MemoryView":160
- * order = b'F'
- * self.mode = u'fortran'
- * elif mode == 'c': # <<<<<<<<<<<<<<
- * order = b'C'
- * self.mode = u'c'
- */
- goto __pyx_L10;
- }
-
- /* "View.MemoryView":164
- * self.mode = u'c'
- * else:
- * raise ValueError("Invalid mode, expected 'c' or 'fortran', got %s" % mode) # <<<<<<<<<<<<<<
- *
- * self.len = fill_contig_strides_array(self._shape, self._strides,
- */
- /*else*/ {
- __pyx_t_3 = __Pyx_PyString_FormatSafe(__pyx_kp_s_Invalid_mode_expected_c_or_fortr, __pyx_v_mode); if (unlikely(!__pyx_t_3)) __PYX_ERR(1, 164, __pyx_L1_error)
- __Pyx_GOTREF(__pyx_t_3);
- __pyx_t_10 = __Pyx_PyObject_CallOneArg(__pyx_builtin_ValueError, __pyx_t_3); if (unlikely(!__pyx_t_10)) __PYX_ERR(1, 164, __pyx_L1_error)
- __Pyx_GOTREF(__pyx_t_10);
- __Pyx_DECREF(__pyx_t_3); __pyx_t_3 = 0;
- __Pyx_Raise(__pyx_t_10, 0, 0, 0);
- __Pyx_DECREF(__pyx_t_10); __pyx_t_10 = 0;
- __PYX_ERR(1, 164, __pyx_L1_error)
- }
- __pyx_L10:;
-
- /* "View.MemoryView":166
- * raise ValueError("Invalid mode, expected 'c' or 'fortran', got %s" % mode)
- *
- * self.len = fill_contig_strides_array(self._shape, self._strides, # <<<<<<<<<<<<<<
- * itemsize, self.ndim, order)
- *
- */
- __pyx_v_self->len = __pyx_fill_contig_strides_array(__pyx_v_self->_shape, __pyx_v_self->_strides, __pyx_v_itemsize, __pyx_v_self->ndim, __pyx_v_order);
-
- /* "View.MemoryView":169
- * itemsize, self.ndim, order)
- *
- * self.free_data = allocate_buffer # <<<<<<<<<<<<<<
- * self.dtype_is_object = format == b'O'
- * if allocate_buffer:
- */
- __pyx_v_self->free_data = __pyx_v_allocate_buffer;
-
- /* "View.MemoryView":170
- *
- * self.free_data = allocate_buffer
- * self.dtype_is_object = format == b'O' # <<<<<<<<<<<<<<
- * if allocate_buffer:
- *
- */
- __pyx_t_10 = PyObject_RichCompare(__pyx_v_format, __pyx_n_b_O, Py_EQ); __Pyx_XGOTREF(__pyx_t_10); if (unlikely(!__pyx_t_10)) __PYX_ERR(1, 170, __pyx_L1_error)
- __pyx_t_4 = __Pyx_PyObject_IsTrue(__pyx_t_10); if (unlikely((__pyx_t_4 == (int)-1) && PyErr_Occurred())) __PYX_ERR(1, 170, __pyx_L1_error)
- __Pyx_DECREF(__pyx_t_10); __pyx_t_10 = 0;
- __pyx_v_self->dtype_is_object = __pyx_t_4;
-
- /* "View.MemoryView":171
- * self.free_data = allocate_buffer
- * self.dtype_is_object = format == b'O'
- * if allocate_buffer: # <<<<<<<<<<<<<<
- *
- *
- */
- __pyx_t_4 = (__pyx_v_allocate_buffer != 0);
- if (__pyx_t_4) {
-
- /* "View.MemoryView":174
- *
- *
- * self.data = malloc(self.len) # <<<<<<<<<<<<<<
- * if not self.data:
- * raise MemoryError("unable to allocate array data.")
- */
- __pyx_v_self->data = ((char *)malloc(__pyx_v_self->len));
-
- /* "View.MemoryView":175
- *
- * self.data = malloc(self.len)
- * if not self.data: # <<<<<<<<<<<<<<
- * raise MemoryError("unable to allocate array data.")
- *
- */
- __pyx_t_4 = ((!(__pyx_v_self->data != 0)) != 0);
- if (unlikely(__pyx_t_4)) {
-
- /* "View.MemoryView":176
- * self.data = malloc(self.len)
- * if not self.data:
- * raise MemoryError("unable to allocate array data.") # <<<<<<<<<<<<<<
- *
- * if self.dtype_is_object:
- */
- __pyx_t_10 = __Pyx_PyObject_Call(__pyx_builtin_MemoryError, __pyx_tuple__5, NULL); if (unlikely(!__pyx_t_10)) __PYX_ERR(1, 176, __pyx_L1_error)
- __Pyx_GOTREF(__pyx_t_10);
- __Pyx_Raise(__pyx_t_10, 0, 0, 0);
- __Pyx_DECREF(__pyx_t_10); __pyx_t_10 = 0;
- __PYX_ERR(1, 176, __pyx_L1_error)
-
- /* "View.MemoryView":175
- *
- * self.data = malloc(self.len)
- * if not self.data: # <<<<<<<<<<<<<<
- * raise MemoryError("unable to allocate array data.")
- *
- */
- }
-
- /* "View.MemoryView":178
- * raise MemoryError("unable to allocate array data.")
- *
- * if self.dtype_is_object: # <<<<<<<<<<<<<<
- * p = self.data
- * for i in range(self.len / itemsize):
- */
- __pyx_t_4 = (__pyx_v_self->dtype_is_object != 0);
- if (__pyx_t_4) {
-
- /* "View.MemoryView":179
- *
- * if self.dtype_is_object:
- * p = self.data # <<<<<<<<<<<<<<
- * for i in range(self.len / itemsize):
- * p[i] = Py_None
- */
- __pyx_v_p = ((PyObject **)__pyx_v_self->data);
-
- /* "View.MemoryView":180
- * if self.dtype_is_object:
- * p = self.data
- * for i in range(self.len / itemsize): # <<<<<<<<<<<<<<
- * p[i] = Py_None
- * Py_INCREF(Py_None)
- */
- if (unlikely(__pyx_v_itemsize == 0)) {
- PyErr_SetString(PyExc_ZeroDivisionError, "integer division or modulo by zero");
- __PYX_ERR(1, 180, __pyx_L1_error)
- }
- else if (sizeof(Py_ssize_t) == sizeof(long) && (!(((Py_ssize_t)-1) > 0)) && unlikely(__pyx_v_itemsize == (Py_ssize_t)-1) && unlikely(UNARY_NEG_WOULD_OVERFLOW(__pyx_v_self->len))) {
- PyErr_SetString(PyExc_OverflowError, "value too large to perform division");
- __PYX_ERR(1, 180, __pyx_L1_error)
- }
- __pyx_t_1 = __Pyx_div_Py_ssize_t(__pyx_v_self->len, __pyx_v_itemsize);
- __pyx_t_9 = __pyx_t_1;
- for (__pyx_t_11 = 0; __pyx_t_11 < __pyx_t_9; __pyx_t_11+=1) {
- __pyx_v_i = __pyx_t_11;
-
- /* "View.MemoryView":181
- * p = self.data
- * for i in range(self.len / itemsize):
- * p[i] = Py_None # <<<<<<<<<<<<<<
- * Py_INCREF(Py_None)
- *
- */
- (__pyx_v_p[__pyx_v_i]) = Py_None;
-
- /* "View.MemoryView":182
- * for i in range(self.len / itemsize):
- * p[i] = Py_None
- * Py_INCREF(Py_None) # <<<<<<<<<<<<<<
- *
- * @cname('getbuffer')
- */
- Py_INCREF(Py_None);
- }
-
- /* "View.MemoryView":178
- * raise MemoryError("unable to allocate array data.")
- *
- * if self.dtype_is_object: # <<<<<<<<<<<<<<
- * p = self.data
- * for i in range(self.len / itemsize):
- */
- }
-
- /* "View.MemoryView":171
- * self.free_data = allocate_buffer
- * self.dtype_is_object = format == b'O'
- * if allocate_buffer: # <<<<<<<<<<<<<<
- *
- *
- */
- }
-
- /* "View.MemoryView":122
- * cdef bint dtype_is_object
- *
- * def __cinit__(array self, tuple shape, Py_ssize_t itemsize, format not None, # <<<<<<<<<<<<<<
- * mode="c", bint allocate_buffer=True):
- *
- */
-
- /* function exit code */
- __pyx_r = 0;
- goto __pyx_L0;
- __pyx_L1_error:;
- __Pyx_XDECREF(__pyx_t_3);
- __Pyx_XDECREF(__pyx_t_5);
- __Pyx_XDECREF(__pyx_t_6);
- __Pyx_XDECREF(__pyx_t_10);
- __Pyx_AddTraceback("View.MemoryView.array.__cinit__", __pyx_clineno, __pyx_lineno, __pyx_filename);
- __pyx_r = -1;
- __pyx_L0:;
- __Pyx_XDECREF(__pyx_v_format);
- __Pyx_RefNannyFinishContext();
- return __pyx_r;
-}
-
-/* "View.MemoryView":185
- *
- * @cname('getbuffer')
- * def __getbuffer__(self, Py_buffer *info, int flags): # <<<<<<<<<<<<<<
- * cdef int bufmode = -1
- * if self.mode == u"c":
- */
-
-/* Python wrapper */
-static CYTHON_UNUSED int __pyx_array_getbuffer(PyObject *__pyx_v_self, Py_buffer *__pyx_v_info, int __pyx_v_flags); /*proto*/
-static CYTHON_UNUSED int __pyx_array_getbuffer(PyObject *__pyx_v_self, Py_buffer *__pyx_v_info, int __pyx_v_flags) {
- int __pyx_r;
- __Pyx_RefNannyDeclarations
- __Pyx_RefNannySetupContext("__getbuffer__ (wrapper)", 0);
- __pyx_r = __pyx_array___pyx_pf_15View_dot_MemoryView_5array_2__getbuffer__(((struct __pyx_array_obj *)__pyx_v_self), ((Py_buffer *)__pyx_v_info), ((int)__pyx_v_flags));
-
- /* function exit code */
- __Pyx_RefNannyFinishContext();
- return __pyx_r;
-}
-
-static int __pyx_array___pyx_pf_15View_dot_MemoryView_5array_2__getbuffer__(struct __pyx_array_obj *__pyx_v_self, Py_buffer *__pyx_v_info, int __pyx_v_flags) {
- int __pyx_v_bufmode;
- int __pyx_r;
- __Pyx_RefNannyDeclarations
- int __pyx_t_1;
- int __pyx_t_2;
- PyObject *__pyx_t_3 = NULL;
- char *__pyx_t_4;
- Py_ssize_t __pyx_t_5;
- int __pyx_t_6;
- Py_ssize_t *__pyx_t_7;
- int __pyx_lineno = 0;
- const char *__pyx_filename = NULL;
- int __pyx_clineno = 0;
- if (__pyx_v_info == NULL) {
- PyErr_SetString(PyExc_BufferError, "PyObject_GetBuffer: view==NULL argument is obsolete");
- return -1;
- }
- __Pyx_RefNannySetupContext("__getbuffer__", 0);
- __pyx_v_info->obj = Py_None; __Pyx_INCREF(Py_None);
- __Pyx_GIVEREF(__pyx_v_info->obj);
-
- /* "View.MemoryView":186
- * @cname('getbuffer')
- * def __getbuffer__(self, Py_buffer *info, int flags):
- * cdef int bufmode = -1 # <<<<<<<<<<<<<<
- * if self.mode == u"c":
- * bufmode = PyBUF_C_CONTIGUOUS | PyBUF_ANY_CONTIGUOUS
- */
- __pyx_v_bufmode = -1;
-
- /* "View.MemoryView":187
- * def __getbuffer__(self, Py_buffer *info, int flags):
- * cdef int bufmode = -1
- * if self.mode == u"c": # <<<<<<<<<<<<<<
- * bufmode = PyBUF_C_CONTIGUOUS | PyBUF_ANY_CONTIGUOUS
- * elif self.mode == u"fortran":
- */
- __pyx_t_1 = (__Pyx_PyUnicode_Equals(__pyx_v_self->mode, __pyx_n_u_c, Py_EQ)); if (unlikely(__pyx_t_1 < 0)) __PYX_ERR(1, 187, __pyx_L1_error)
- __pyx_t_2 = (__pyx_t_1 != 0);
- if (__pyx_t_2) {
-
- /* "View.MemoryView":188
- * cdef int bufmode = -1
- * if self.mode == u"c":
- * bufmode = PyBUF_C_CONTIGUOUS | PyBUF_ANY_CONTIGUOUS # <<<<<<<<<<<<<<
- * elif self.mode == u"fortran":
- * bufmode = PyBUF_F_CONTIGUOUS | PyBUF_ANY_CONTIGUOUS
- */
- __pyx_v_bufmode = (PyBUF_C_CONTIGUOUS | PyBUF_ANY_CONTIGUOUS);
-
- /* "View.MemoryView":187
- * def __getbuffer__(self, Py_buffer *info, int flags):
- * cdef int bufmode = -1
- * if self.mode == u"c": # <<<<<<<<<<<<<<
- * bufmode = PyBUF_C_CONTIGUOUS | PyBUF_ANY_CONTIGUOUS
- * elif self.mode == u"fortran":
- */
- goto __pyx_L3;
- }
-
- /* "View.MemoryView":189
- * if self.mode == u"c":
- * bufmode = PyBUF_C_CONTIGUOUS | PyBUF_ANY_CONTIGUOUS
- * elif self.mode == u"fortran": # <<<<<<<<<<<<<<
- * bufmode = PyBUF_F_CONTIGUOUS | PyBUF_ANY_CONTIGUOUS
- * if not (flags & bufmode):
- */
- __pyx_t_2 = (__Pyx_PyUnicode_Equals(__pyx_v_self->mode, __pyx_n_u_fortran, Py_EQ)); if (unlikely(__pyx_t_2 < 0)) __PYX_ERR(1, 189, __pyx_L1_error)
- __pyx_t_1 = (__pyx_t_2 != 0);
- if (__pyx_t_1) {
-
- /* "View.MemoryView":190
- * bufmode = PyBUF_C_CONTIGUOUS | PyBUF_ANY_CONTIGUOUS
- * elif self.mode == u"fortran":
- * bufmode = PyBUF_F_CONTIGUOUS | PyBUF_ANY_CONTIGUOUS # <<<<<<<<<<<<<<
- * if not (flags & bufmode):
- * raise ValueError("Can only create a buffer that is contiguous in memory.")
- */
- __pyx_v_bufmode = (PyBUF_F_CONTIGUOUS | PyBUF_ANY_CONTIGUOUS);
-
- /* "View.MemoryView":189
- * if self.mode == u"c":
- * bufmode = PyBUF_C_CONTIGUOUS | PyBUF_ANY_CONTIGUOUS
- * elif self.mode == u"fortran": # <<<<<<<<<<<<<<
- * bufmode = PyBUF_F_CONTIGUOUS | PyBUF_ANY_CONTIGUOUS
- * if not (flags & bufmode):
- */
- }
- __pyx_L3:;
-
- /* "View.MemoryView":191
- * elif self.mode == u"fortran":
- * bufmode = PyBUF_F_CONTIGUOUS | PyBUF_ANY_CONTIGUOUS
- * if not (flags & bufmode): # <<<<<<<<<<<<<<
- * raise ValueError("Can only create a buffer that is contiguous in memory.")
- * info.buf = self.data
- */
- __pyx_t_1 = ((!((__pyx_v_flags & __pyx_v_bufmode) != 0)) != 0);
- if (unlikely(__pyx_t_1)) {
-
- /* "View.MemoryView":192
- * bufmode = PyBUF_F_CONTIGUOUS | PyBUF_ANY_CONTIGUOUS
- * if not (flags & bufmode):
- * raise ValueError("Can only create a buffer that is contiguous in memory.") # <<<<<<<<<<<<<<
- * info.buf = self.data
- * info.len = self.len
- */
- __pyx_t_3 = __Pyx_PyObject_Call(__pyx_builtin_ValueError, __pyx_tuple__6, NULL); if (unlikely(!__pyx_t_3)) __PYX_ERR(1, 192, __pyx_L1_error)
- __Pyx_GOTREF(__pyx_t_3);
- __Pyx_Raise(__pyx_t_3, 0, 0, 0);
- __Pyx_DECREF(__pyx_t_3); __pyx_t_3 = 0;
- __PYX_ERR(1, 192, __pyx_L1_error)
-
- /* "View.MemoryView":191
- * elif self.mode == u"fortran":
- * bufmode = PyBUF_F_CONTIGUOUS | PyBUF_ANY_CONTIGUOUS
- * if not (flags & bufmode): # <<<<<<<<<<<<<<
- * raise ValueError("Can only create a buffer that is contiguous in memory.")
- * info.buf = self.data
- */
- }
-
- /* "View.MemoryView":193
- * if not (flags & bufmode):
- * raise ValueError("Can only create a buffer that is contiguous in memory.")
- * info.buf = self.data # <<<<<<<<<<<<<<
- * info.len = self.len
- * info.ndim = self.ndim
- */
- __pyx_t_4 = __pyx_v_self->data;
- __pyx_v_info->buf = __pyx_t_4;
-
- /* "View.MemoryView":194
- * raise ValueError("Can only create a buffer that is contiguous in memory.")
- * info.buf = self.data
- * info.len = self.len # <<<<<<<<<<<<<<
- * info.ndim = self.ndim
- * info.shape = self._shape
- */
- __pyx_t_5 = __pyx_v_self->len;
- __pyx_v_info->len = __pyx_t_5;
-
- /* "View.MemoryView":195
- * info.buf = self.data
- * info.len = self.len
- * info.ndim = self.ndim # <<<<<<<<<<<<<<
- * info.shape = self._shape
- * info.strides = self._strides
- */
- __pyx_t_6 = __pyx_v_self->ndim;
- __pyx_v_info->ndim = __pyx_t_6;
-
- /* "View.MemoryView":196
- * info.len = self.len
- * info.ndim = self.ndim
- * info.shape = self._shape # <<<<<<<<<<<<<<
- * info.strides = self._strides
- * info.suboffsets = NULL
- */
- __pyx_t_7 = __pyx_v_self->_shape;
- __pyx_v_info->shape = __pyx_t_7;
-
- /* "View.MemoryView":197
- * info.ndim = self.ndim
- * info.shape = self._shape
- * info.strides = self._strides # <<<<<<<<<<<<<<
- * info.suboffsets = NULL
- * info.itemsize = self.itemsize
- */
- __pyx_t_7 = __pyx_v_self->_strides;
- __pyx_v_info->strides = __pyx_t_7;
-
- /* "View.MemoryView":198
- * info.shape = self._shape
- * info.strides = self._strides
- * info.suboffsets = NULL # <<<<<<<<<<<<<<
- * info.itemsize = self.itemsize
- * info.readonly = 0
- */
- __pyx_v_info->suboffsets = NULL;
-
- /* "View.MemoryView":199
- * info.strides = self._strides
- * info.suboffsets = NULL
- * info.itemsize = self.itemsize # <<<<<<<<<<<<<<
- * info.readonly = 0
- *
- */
- __pyx_t_5 = __pyx_v_self->itemsize;
- __pyx_v_info->itemsize = __pyx_t_5;
-
- /* "View.MemoryView":200
- * info.suboffsets = NULL
- * info.itemsize = self.itemsize
- * info.readonly = 0 # <<<<<<<<<<<<<<
- *
- * if flags & PyBUF_FORMAT:
- */
- __pyx_v_info->readonly = 0;
-
- /* "View.MemoryView":202
- * info.readonly = 0
- *
- * if flags & PyBUF_FORMAT: # <<<<<<<<<<<<<<
- * info.format = self.format
- * else:
- */
- __pyx_t_1 = ((__pyx_v_flags & PyBUF_FORMAT) != 0);
- if (__pyx_t_1) {
-
- /* "View.MemoryView":203
- *
- * if flags & PyBUF_FORMAT:
- * info.format = self.format # <<<<<<<<<<<<<<
- * else:
- * info.format = NULL
- */
- __pyx_t_4 = __pyx_v_self->format;
- __pyx_v_info->format = __pyx_t_4;
-
- /* "View.MemoryView":202
- * info.readonly = 0
- *
- * if flags & PyBUF_FORMAT: # <<<<<<<<<<<<<<
- * info.format = self.format
- * else:
- */
- goto __pyx_L5;
- }
-
- /* "View.MemoryView":205
- * info.format = self.format
- * else:
- * info.format = NULL # <<<<<<<<<<<<<<
- *
- * info.obj = self
- */
- /*else*/ {
- __pyx_v_info->format = NULL;
- }
- __pyx_L5:;
-
- /* "View.MemoryView":207
- * info.format = NULL
- *
- * info.obj = self # <<<<<<<<<<<<<<
- *
- * __pyx_getbuffer = capsule( &__pyx_array_getbuffer, "getbuffer(obj, view, flags)")
- */
- __Pyx_INCREF(((PyObject *)__pyx_v_self));
- __Pyx_GIVEREF(((PyObject *)__pyx_v_self));
- __Pyx_GOTREF(__pyx_v_info->obj);
- __Pyx_DECREF(__pyx_v_info->obj);
- __pyx_v_info->obj = ((PyObject *)__pyx_v_self);
-
- /* "View.MemoryView":185
- *
- * @cname('getbuffer')
- * def __getbuffer__(self, Py_buffer *info, int flags): # <<<<<<<<<<<<<<
- * cdef int bufmode = -1
- * if self.mode == u"c":
- */
-
- /* function exit code */
- __pyx_r = 0;
- goto __pyx_L0;
- __pyx_L1_error:;
- __Pyx_XDECREF(__pyx_t_3);
- __Pyx_AddTraceback("View.MemoryView.array.__getbuffer__", __pyx_clineno, __pyx_lineno, __pyx_filename);
- __pyx_r = -1;
- if (__pyx_v_info->obj != NULL) {
- __Pyx_GOTREF(__pyx_v_info->obj);
- __Pyx_DECREF(__pyx_v_info->obj); __pyx_v_info->obj = 0;
- }
- goto __pyx_L2;
- __pyx_L0:;
- if (__pyx_v_info->obj == Py_None) {
- __Pyx_GOTREF(__pyx_v_info->obj);
- __Pyx_DECREF(__pyx_v_info->obj); __pyx_v_info->obj = 0;
- }
- __pyx_L2:;
- __Pyx_RefNannyFinishContext();
- return __pyx_r;
-}
-
-/* "View.MemoryView":211
- * __pyx_getbuffer = capsule( &__pyx_array_getbuffer, "getbuffer(obj, view, flags)")
- *
- * def __dealloc__(array self): # <<<<<<<<<<<<<<
- * if self.callback_free_data != NULL:
- * self.callback_free_data(self.data)
- */
-
-/* Python wrapper */
-static void __pyx_array___dealloc__(PyObject *__pyx_v_self); /*proto*/
-static void __pyx_array___dealloc__(PyObject *__pyx_v_self) {
- __Pyx_RefNannyDeclarations
- __Pyx_RefNannySetupContext("__dealloc__ (wrapper)", 0);
- __pyx_array___pyx_pf_15View_dot_MemoryView_5array_4__dealloc__(((struct __pyx_array_obj *)__pyx_v_self));
-
- /* function exit code */
- __Pyx_RefNannyFinishContext();
-}
-
-static void __pyx_array___pyx_pf_15View_dot_MemoryView_5array_4__dealloc__(struct __pyx_array_obj *__pyx_v_self) {
- __Pyx_RefNannyDeclarations
- int __pyx_t_1;
- __Pyx_RefNannySetupContext("__dealloc__", 0);
-
- /* "View.MemoryView":212
- *
- * def __dealloc__(array self):
- * if self.callback_free_data != NULL: # <<<<<<<<<<<<<<
- * self.callback_free_data(self.data)
- * elif self.free_data:
- */
- __pyx_t_1 = ((__pyx_v_self->callback_free_data != NULL) != 0);
- if (__pyx_t_1) {
-
- /* "View.MemoryView":213
- * def __dealloc__(array self):
- * if self.callback_free_data != NULL:
- * self.callback_free_data(self.data) # <<<<<<<<<<<<<<
- * elif self.free_data:
- * if self.dtype_is_object:
- */
- __pyx_v_self->callback_free_data(__pyx_v_self->data);
-
- /* "View.MemoryView":212
- *
- * def __dealloc__(array self):
- * if self.callback_free_data != NULL: # <<<<<<<<<<<<<<
- * self.callback_free_data(self.data)
- * elif self.free_data:
- */
- goto __pyx_L3;
- }
-
- /* "View.MemoryView":214
- * if self.callback_free_data != NULL:
- * self.callback_free_data(self.data)
- * elif self.free_data: # <<<<<<<<<<<<<<
- * if self.dtype_is_object:
- * refcount_objects_in_slice(self.data, self._shape,
- */
- __pyx_t_1 = (__pyx_v_self->free_data != 0);
- if (__pyx_t_1) {
-
- /* "View.MemoryView":215
- * self.callback_free_data(self.data)
- * elif self.free_data:
- * if self.dtype_is_object: # <<<<<<<<<<<<<<
- * refcount_objects_in_slice(self.data, self._shape,
- * self._strides, self.ndim, False)
- */
- __pyx_t_1 = (__pyx_v_self->dtype_is_object != 0);
- if (__pyx_t_1) {
-
- /* "View.MemoryView":216
- * elif self.free_data:
- * if self.dtype_is_object:
- * refcount_objects_in_slice(self.data, self._shape, # <<<<<<<<<<<<<<
- * self._strides, self.ndim, False)
- * free(self.data)
- */
- __pyx_memoryview_refcount_objects_in_slice(__pyx_v_self->data, __pyx_v_self->_shape, __pyx_v_self->_strides, __pyx_v_self->ndim, 0);
-
- /* "View.MemoryView":215
- * self.callback_free_data(self.data)
- * elif self.free_data:
- * if self.dtype_is_object: # <<<<<<<<<<<<<<
- * refcount_objects_in_slice(self.data, self._shape,
- * self._strides, self.ndim, False)
- */
- }
-
- /* "View.MemoryView":218
- * refcount_objects_in_slice(self.data, self._shape,
- * self._strides, self.ndim, False)
- * free(self.data) # <<<<<<<<<<<<<<
- * PyObject_Free(self._shape)
- *
- */
- free(__pyx_v_self->data);
-
- /* "View.MemoryView":214
- * if self.callback_free_data != NULL:
- * self.callback_free_data(self.data)
- * elif self.free_data: # <<<<<<<<<<<<<<
- * if self.dtype_is_object:
- * refcount_objects_in_slice(self.data, self._shape,
- */
- }
- __pyx_L3:;
-
- /* "View.MemoryView":219
- * self._strides, self.ndim, False)
- * free(self.data)
- * PyObject_Free(self._shape) # <<<<<<<<<<<<<<
- *
- * @property
- */
- PyObject_Free(__pyx_v_self->_shape);
-
- /* "View.MemoryView":211
- * __pyx_getbuffer = capsule( &__pyx_array_getbuffer, "getbuffer(obj, view, flags)")
- *
- * def __dealloc__(array self): # <<<<<<<<<<<<<<
- * if self.callback_free_data != NULL:
- * self.callback_free_data(self.data)
- */
-
- /* function exit code */
- __Pyx_RefNannyFinishContext();
-}
-
-/* "View.MemoryView":222
- *
- * @property
- * def memview(self): # <<<<<<<<<<<<<<
- * return self.get_memview()
- *
- */
-
-/* Python wrapper */
-static PyObject *__pyx_pw_15View_dot_MemoryView_5array_7memview_1__get__(PyObject *__pyx_v_self); /*proto*/
-static PyObject *__pyx_pw_15View_dot_MemoryView_5array_7memview_1__get__(PyObject *__pyx_v_self) {
- PyObject *__pyx_r = 0;
- __Pyx_RefNannyDeclarations
- __Pyx_RefNannySetupContext("__get__ (wrapper)", 0);
- __pyx_r = __pyx_pf_15View_dot_MemoryView_5array_7memview___get__(((struct __pyx_array_obj *)__pyx_v_self));
-
- /* function exit code */
- __Pyx_RefNannyFinishContext();
- return __pyx_r;
-}
-
-static PyObject *__pyx_pf_15View_dot_MemoryView_5array_7memview___get__(struct __pyx_array_obj *__pyx_v_self) {
- PyObject *__pyx_r = NULL;
- __Pyx_RefNannyDeclarations
- PyObject *__pyx_t_1 = NULL;
- int __pyx_lineno = 0;
- const char *__pyx_filename = NULL;
- int __pyx_clineno = 0;
- __Pyx_RefNannySetupContext("__get__", 0);
-
- /* "View.MemoryView":223
- * @property
- * def memview(self):
- * return self.get_memview() # <<<<<<<<<<<<<<
- *
- * @cname('get_memview')
- */
- __Pyx_XDECREF(__pyx_r);
- __pyx_t_1 = ((struct __pyx_vtabstruct_array *)__pyx_v_self->__pyx_vtab)->get_memview(__pyx_v_self); if (unlikely(!__pyx_t_1)) __PYX_ERR(1, 223, __pyx_L1_error)
- __Pyx_GOTREF(__pyx_t_1);
- __pyx_r = __pyx_t_1;
- __pyx_t_1 = 0;
- goto __pyx_L0;
-
- /* "View.MemoryView":222
- *
- * @property
- * def memview(self): # <<<<<<<<<<<<<<
- * return self.get_memview()
- *
- */
-
- /* function exit code */
- __pyx_L1_error:;
- __Pyx_XDECREF(__pyx_t_1);
- __Pyx_AddTraceback("View.MemoryView.array.memview.__get__", __pyx_clineno, __pyx_lineno, __pyx_filename);
- __pyx_r = NULL;
- __pyx_L0:;
- __Pyx_XGIVEREF(__pyx_r);
- __Pyx_RefNannyFinishContext();
- return __pyx_r;
-}
-
-/* "View.MemoryView":226
- *
- * @cname('get_memview')
- * cdef get_memview(self): # <<<<<<<<<<<<<<
- * flags = PyBUF_ANY_CONTIGUOUS|PyBUF_FORMAT|PyBUF_WRITABLE
- * return memoryview(self, flags, self.dtype_is_object)
- */
-
-static PyObject *__pyx_array_get_memview(struct __pyx_array_obj *__pyx_v_self) {
- int __pyx_v_flags;
- PyObject *__pyx_r = NULL;
- __Pyx_RefNannyDeclarations
- PyObject *__pyx_t_1 = NULL;
- PyObject *__pyx_t_2 = NULL;
- PyObject *__pyx_t_3 = NULL;
- int __pyx_lineno = 0;
- const char *__pyx_filename = NULL;
- int __pyx_clineno = 0;
- __Pyx_RefNannySetupContext("get_memview", 0);
-
- /* "View.MemoryView":227
- * @cname('get_memview')
- * cdef get_memview(self):
- * flags = PyBUF_ANY_CONTIGUOUS|PyBUF_FORMAT|PyBUF_WRITABLE # <<<<<<<<<<<<<<
- * return memoryview(self, flags, self.dtype_is_object)
- *
- */
- __pyx_v_flags = ((PyBUF_ANY_CONTIGUOUS | PyBUF_FORMAT) | PyBUF_WRITABLE);
-
- /* "View.MemoryView":228
- * cdef get_memview(self):
- * flags = PyBUF_ANY_CONTIGUOUS|PyBUF_FORMAT|PyBUF_WRITABLE
- * return memoryview(self, flags, self.dtype_is_object) # <<<<<<<<<<<<<<
- *
- * def __len__(self):
- */
- __Pyx_XDECREF(__pyx_r);
- __pyx_t_1 = __Pyx_PyInt_From_int(__pyx_v_flags); if (unlikely(!__pyx_t_1)) __PYX_ERR(1, 228, __pyx_L1_error)
- __Pyx_GOTREF(__pyx_t_1);
- __pyx_t_2 = __Pyx_PyBool_FromLong(__pyx_v_self->dtype_is_object); if (unlikely(!__pyx_t_2)) __PYX_ERR(1, 228, __pyx_L1_error)
- __Pyx_GOTREF(__pyx_t_2);
- __pyx_t_3 = PyTuple_New(3); if (unlikely(!__pyx_t_3)) __PYX_ERR(1, 228, __pyx_L1_error)
- __Pyx_GOTREF(__pyx_t_3);
- __Pyx_INCREF(((PyObject *)__pyx_v_self));
- __Pyx_GIVEREF(((PyObject *)__pyx_v_self));
- PyTuple_SET_ITEM(__pyx_t_3, 0, ((PyObject *)__pyx_v_self));
- __Pyx_GIVEREF(__pyx_t_1);
- PyTuple_SET_ITEM(__pyx_t_3, 1, __pyx_t_1);
- __Pyx_GIVEREF(__pyx_t_2);
- PyTuple_SET_ITEM(__pyx_t_3, 2, __pyx_t_2);
- __pyx_t_1 = 0;
- __pyx_t_2 = 0;
- __pyx_t_2 = __Pyx_PyObject_Call(((PyObject *)__pyx_memoryview_type), __pyx_t_3, NULL); if (unlikely(!__pyx_t_2)) __PYX_ERR(1, 228, __pyx_L1_error)
- __Pyx_GOTREF(__pyx_t_2);
- __Pyx_DECREF(__pyx_t_3); __pyx_t_3 = 0;
- __pyx_r = __pyx_t_2;
- __pyx_t_2 = 0;
- goto __pyx_L0;
-
- /* "View.MemoryView":226
- *
- * @cname('get_memview')
- * cdef get_memview(self): # <<<<<<<<<<<<<<
- * flags = PyBUF_ANY_CONTIGUOUS|PyBUF_FORMAT|PyBUF_WRITABLE
- * return memoryview(self, flags, self.dtype_is_object)
- */
-
- /* function exit code */
- __pyx_L1_error:;
- __Pyx_XDECREF(__pyx_t_1);
- __Pyx_XDECREF(__pyx_t_2);
- __Pyx_XDECREF(__pyx_t_3);
- __Pyx_AddTraceback("View.MemoryView.array.get_memview", __pyx_clineno, __pyx_lineno, __pyx_filename);
- __pyx_r = 0;
- __pyx_L0:;
- __Pyx_XGIVEREF(__pyx_r);
- __Pyx_RefNannyFinishContext();
- return __pyx_r;
-}
-
-/* "View.MemoryView":230
- * return memoryview(self, flags, self.dtype_is_object)
- *
- * def __len__(self): # <<<<<<<<<<<<<<
- * return self._shape[0]
- *
- */
-
-/* Python wrapper */
-static Py_ssize_t __pyx_array___len__(PyObject *__pyx_v_self); /*proto*/
-static Py_ssize_t __pyx_array___len__(PyObject *__pyx_v_self) {
- Py_ssize_t __pyx_r;
- __Pyx_RefNannyDeclarations
- __Pyx_RefNannySetupContext("__len__ (wrapper)", 0);
- __pyx_r = __pyx_array___pyx_pf_15View_dot_MemoryView_5array_6__len__(((struct __pyx_array_obj *)__pyx_v_self));
-
- /* function exit code */
- __Pyx_RefNannyFinishContext();
- return __pyx_r;
-}
-
-static Py_ssize_t __pyx_array___pyx_pf_15View_dot_MemoryView_5array_6__len__(struct __pyx_array_obj *__pyx_v_self) {
- Py_ssize_t __pyx_r;
- __Pyx_RefNannyDeclarations
- __Pyx_RefNannySetupContext("__len__", 0);
-
- /* "View.MemoryView":231
- *
- * def __len__(self):
- * return self._shape[0] # <<<<<<<<<<<<<<
- *
- * def __getattr__(self, attr):
- */
- __pyx_r = (__pyx_v_self->_shape[0]);
- goto __pyx_L0;
-
- /* "View.MemoryView":230
- * return memoryview(self, flags, self.dtype_is_object)
- *
- * def __len__(self): # <<<<<<<<<<<<<<
- * return self._shape[0]
- *
- */
-
- /* function exit code */
- __pyx_L0:;
- __Pyx_RefNannyFinishContext();
- return __pyx_r;
-}
-
-/* "View.MemoryView":233
- * return self._shape[0]
- *
- * def __getattr__(self, attr): # <<<<<<<<<<<<<<
- * return getattr(self.memview, attr)
- *
- */
-
-/* Python wrapper */
-static PyObject *__pyx_array___getattr__(PyObject *__pyx_v_self, PyObject *__pyx_v_attr); /*proto*/
-static PyObject *__pyx_array___getattr__(PyObject *__pyx_v_self, PyObject *__pyx_v_attr) {
- PyObject *__pyx_r = 0;
- __Pyx_RefNannyDeclarations
- __Pyx_RefNannySetupContext("__getattr__ (wrapper)", 0);
- __pyx_r = __pyx_array___pyx_pf_15View_dot_MemoryView_5array_8__getattr__(((struct __pyx_array_obj *)__pyx_v_self), ((PyObject *)__pyx_v_attr));
-
- /* function exit code */
- __Pyx_RefNannyFinishContext();
- return __pyx_r;
-}
-
-static PyObject *__pyx_array___pyx_pf_15View_dot_MemoryView_5array_8__getattr__(struct __pyx_array_obj *__pyx_v_self, PyObject *__pyx_v_attr) {
- PyObject *__pyx_r = NULL;
- __Pyx_RefNannyDeclarations
- PyObject *__pyx_t_1 = NULL;
- PyObject *__pyx_t_2 = NULL;
- int __pyx_lineno = 0;
- const char *__pyx_filename = NULL;
- int __pyx_clineno = 0;
- __Pyx_RefNannySetupContext("__getattr__", 0);
-
- /* "View.MemoryView":234
- *
- * def __getattr__(self, attr):
- * return getattr(self.memview, attr) # <<<<<<<<<<<<<<
- *
- * def __getitem__(self, item):
- */
- __Pyx_XDECREF(__pyx_r);
- __pyx_t_1 = __Pyx_PyObject_GetAttrStr(((PyObject *)__pyx_v_self), __pyx_n_s_memview); if (unlikely(!__pyx_t_1)) __PYX_ERR(1, 234, __pyx_L1_error)
- __Pyx_GOTREF(__pyx_t_1);
- __pyx_t_2 = __Pyx_GetAttr(__pyx_t_1, __pyx_v_attr); if (unlikely(!__pyx_t_2)) __PYX_ERR(1, 234, __pyx_L1_error)
- __Pyx_GOTREF(__pyx_t_2);
- __Pyx_DECREF(__pyx_t_1); __pyx_t_1 = 0;
- __pyx_r = __pyx_t_2;
- __pyx_t_2 = 0;
- goto __pyx_L0;
-
- /* "View.MemoryView":233
- * return self._shape[0]
- *
- * def __getattr__(self, attr): # <<<<<<<<<<<<<<
- * return getattr(self.memview, attr)
- *
- */
-
- /* function exit code */
- __pyx_L1_error:;
- __Pyx_XDECREF(__pyx_t_1);
- __Pyx_XDECREF(__pyx_t_2);
- __Pyx_AddTraceback("View.MemoryView.array.__getattr__", __pyx_clineno, __pyx_lineno, __pyx_filename);
- __pyx_r = NULL;
- __pyx_L0:;
- __Pyx_XGIVEREF(__pyx_r);
- __Pyx_RefNannyFinishContext();
- return __pyx_r;
-}
-
-/* "View.MemoryView":236
- * return getattr(self.memview, attr)
- *
- * def __getitem__(self, item): # <<<<<<<<<<<<<<
- * return self.memview[item]
- *
- */
-
-/* Python wrapper */
-static PyObject *__pyx_array___getitem__(PyObject *__pyx_v_self, PyObject *__pyx_v_item); /*proto*/
-static PyObject *__pyx_array___getitem__(PyObject *__pyx_v_self, PyObject *__pyx_v_item) {
- PyObject *__pyx_r = 0;
- __Pyx_RefNannyDeclarations
- __Pyx_RefNannySetupContext("__getitem__ (wrapper)", 0);
- __pyx_r = __pyx_array___pyx_pf_15View_dot_MemoryView_5array_10__getitem__(((struct __pyx_array_obj *)__pyx_v_self), ((PyObject *)__pyx_v_item));
-
- /* function exit code */
- __Pyx_RefNannyFinishContext();
- return __pyx_r;
-}
-
-static PyObject *__pyx_array___pyx_pf_15View_dot_MemoryView_5array_10__getitem__(struct __pyx_array_obj *__pyx_v_self, PyObject *__pyx_v_item) {
- PyObject *__pyx_r = NULL;
- __Pyx_RefNannyDeclarations
- PyObject *__pyx_t_1 = NULL;
- PyObject *__pyx_t_2 = NULL;
- int __pyx_lineno = 0;
- const char *__pyx_filename = NULL;
- int __pyx_clineno = 0;
- __Pyx_RefNannySetupContext("__getitem__", 0);
-
- /* "View.MemoryView":237
- *
- * def __getitem__(self, item):
- * return self.memview[item] # <<<<<<<<<<<<<<
- *
- * def __setitem__(self, item, value):
- */
- __Pyx_XDECREF(__pyx_r);
- __pyx_t_1 = __Pyx_PyObject_GetAttrStr(((PyObject *)__pyx_v_self), __pyx_n_s_memview); if (unlikely(!__pyx_t_1)) __PYX_ERR(1, 237, __pyx_L1_error)
- __Pyx_GOTREF(__pyx_t_1);
- __pyx_t_2 = __Pyx_PyObject_GetItem(__pyx_t_1, __pyx_v_item); if (unlikely(!__pyx_t_2)) __PYX_ERR(1, 237, __pyx_L1_error)
- __Pyx_GOTREF(__pyx_t_2);
- __Pyx_DECREF(__pyx_t_1); __pyx_t_1 = 0;
- __pyx_r = __pyx_t_2;
- __pyx_t_2 = 0;
- goto __pyx_L0;
-
- /* "View.MemoryView":236
- * return getattr(self.memview, attr)
- *
- * def __getitem__(self, item): # <<<<<<<<<<<<<<
- * return self.memview[item]
- *
- */
-
- /* function exit code */
- __pyx_L1_error:;
- __Pyx_XDECREF(__pyx_t_1);
- __Pyx_XDECREF(__pyx_t_2);
- __Pyx_AddTraceback("View.MemoryView.array.__getitem__", __pyx_clineno, __pyx_lineno, __pyx_filename);
- __pyx_r = NULL;
- __pyx_L0:;
- __Pyx_XGIVEREF(__pyx_r);
- __Pyx_RefNannyFinishContext();
- return __pyx_r;
-}
-
-/* "View.MemoryView":239
- * return self.memview[item]
- *
- * def __setitem__(self, item, value): # <<<<<<<<<<<<<<
- * self.memview[item] = value
- *
- */
-
-/* Python wrapper */
-static int __pyx_array___setitem__(PyObject *__pyx_v_self, PyObject *__pyx_v_item, PyObject *__pyx_v_value); /*proto*/
-static int __pyx_array___setitem__(PyObject *__pyx_v_self, PyObject *__pyx_v_item, PyObject *__pyx_v_value) {
- int __pyx_r;
- __Pyx_RefNannyDeclarations
- __Pyx_RefNannySetupContext("__setitem__ (wrapper)", 0);
- __pyx_r = __pyx_array___pyx_pf_15View_dot_MemoryView_5array_12__setitem__(((struct __pyx_array_obj *)__pyx_v_self), ((PyObject *)__pyx_v_item), ((PyObject *)__pyx_v_value));
-
- /* function exit code */
- __Pyx_RefNannyFinishContext();
- return __pyx_r;
-}
-
-static int __pyx_array___pyx_pf_15View_dot_MemoryView_5array_12__setitem__(struct __pyx_array_obj *__pyx_v_self, PyObject *__pyx_v_item, PyObject *__pyx_v_value) {
- int __pyx_r;
- __Pyx_RefNannyDeclarations
- PyObject *__pyx_t_1 = NULL;
- int __pyx_lineno = 0;
- const char *__pyx_filename = NULL;
- int __pyx_clineno = 0;
- __Pyx_RefNannySetupContext("__setitem__", 0);
-
- /* "View.MemoryView":240
- *
- * def __setitem__(self, item, value):
- * self.memview[item] = value # <<<<<<<<<<<<<<
- *
- *
- */
- __pyx_t_1 = __Pyx_PyObject_GetAttrStr(((PyObject *)__pyx_v_self), __pyx_n_s_memview); if (unlikely(!__pyx_t_1)) __PYX_ERR(1, 240, __pyx_L1_error)
- __Pyx_GOTREF(__pyx_t_1);
- if (unlikely(PyObject_SetItem(__pyx_t_1, __pyx_v_item, __pyx_v_value) < 0)) __PYX_ERR(1, 240, __pyx_L1_error)
- __Pyx_DECREF(__pyx_t_1); __pyx_t_1 = 0;
-
- /* "View.MemoryView":239
- * return self.memview[item]
- *
- * def __setitem__(self, item, value): # <<<<<<<<<<<<<<
- * self.memview[item] = value
- *
- */
-
- /* function exit code */
- __pyx_r = 0;
- goto __pyx_L0;
- __pyx_L1_error:;
- __Pyx_XDECREF(__pyx_t_1);
- __Pyx_AddTraceback("View.MemoryView.array.__setitem__", __pyx_clineno, __pyx_lineno, __pyx_filename);
- __pyx_r = -1;
- __pyx_L0:;
- __Pyx_RefNannyFinishContext();
- return __pyx_r;
-}
-
-/* "(tree fragment)":1
- * def __reduce_cython__(self): # <<<<<<<<<<<<<<
- * raise TypeError("no default __reduce__ due to non-trivial __cinit__")
- * def __setstate_cython__(self, __pyx_state):
- */
-
-/* Python wrapper */
-static PyObject *__pyx_pw___pyx_array_1__reduce_cython__(PyObject *__pyx_v_self, CYTHON_UNUSED PyObject *unused); /*proto*/
-static PyObject *__pyx_pw___pyx_array_1__reduce_cython__(PyObject *__pyx_v_self, CYTHON_UNUSED PyObject *unused) {
- PyObject *__pyx_r = 0;
- __Pyx_RefNannyDeclarations
- __Pyx_RefNannySetupContext("__reduce_cython__ (wrapper)", 0);
- __pyx_r = __pyx_pf___pyx_array___reduce_cython__(((struct __pyx_array_obj *)__pyx_v_self));
-
- /* function exit code */
- __Pyx_RefNannyFinishContext();
- return __pyx_r;
-}
-
-static PyObject *__pyx_pf___pyx_array___reduce_cython__(CYTHON_UNUSED struct __pyx_array_obj *__pyx_v_self) {
- PyObject *__pyx_r = NULL;
- __Pyx_RefNannyDeclarations
- PyObject *__pyx_t_1 = NULL;
- int __pyx_lineno = 0;
- const char *__pyx_filename = NULL;
- int __pyx_clineno = 0;
- __Pyx_RefNannySetupContext("__reduce_cython__", 0);
-
- /* "(tree fragment)":2
- * def __reduce_cython__(self):
- * raise TypeError("no default __reduce__ due to non-trivial __cinit__") # <<<<<<<<<<<<<<
- * def __setstate_cython__(self, __pyx_state):
- * raise TypeError("no default __reduce__ due to non-trivial __cinit__")
- */
- __pyx_t_1 = __Pyx_PyObject_Call(__pyx_builtin_TypeError, __pyx_tuple__7, NULL); if (unlikely(!__pyx_t_1)) __PYX_ERR(1, 2, __pyx_L1_error)
- __Pyx_GOTREF(__pyx_t_1);
- __Pyx_Raise(__pyx_t_1, 0, 0, 0);
- __Pyx_DECREF(__pyx_t_1); __pyx_t_1 = 0;
- __PYX_ERR(1, 2, __pyx_L1_error)
-
- /* "(tree fragment)":1
- * def __reduce_cython__(self): # <<<<<<<<<<<<<<
- * raise TypeError("no default __reduce__ due to non-trivial __cinit__")
- * def __setstate_cython__(self, __pyx_state):
- */
-
- /* function exit code */
- __pyx_L1_error:;
- __Pyx_XDECREF(__pyx_t_1);
- __Pyx_AddTraceback("View.MemoryView.array.__reduce_cython__", __pyx_clineno, __pyx_lineno, __pyx_filename);
- __pyx_r = NULL;
- __Pyx_XGIVEREF(__pyx_r);
- __Pyx_RefNannyFinishContext();
- return __pyx_r;
-}
-
-/* "(tree fragment)":3
- * def __reduce_cython__(self):
- * raise TypeError("no default __reduce__ due to non-trivial __cinit__")
- * def __setstate_cython__(self, __pyx_state): # <<<<<<<<<<<<<<
- * raise TypeError("no default __reduce__ due to non-trivial __cinit__")
- */
-
-/* Python wrapper */
-static PyObject *__pyx_pw___pyx_array_3__setstate_cython__(PyObject *__pyx_v_self, PyObject *__pyx_v___pyx_state); /*proto*/
-static PyObject *__pyx_pw___pyx_array_3__setstate_cython__(PyObject *__pyx_v_self, PyObject *__pyx_v___pyx_state) {
- PyObject *__pyx_r = 0;
- __Pyx_RefNannyDeclarations
- __Pyx_RefNannySetupContext("__setstate_cython__ (wrapper)", 0);
- __pyx_r = __pyx_pf___pyx_array_2__setstate_cython__(((struct __pyx_array_obj *)__pyx_v_self), ((PyObject *)__pyx_v___pyx_state));
-
- /* function exit code */
- __Pyx_RefNannyFinishContext();
- return __pyx_r;
-}
-
-static PyObject *__pyx_pf___pyx_array_2__setstate_cython__(CYTHON_UNUSED struct __pyx_array_obj *__pyx_v_self, CYTHON_UNUSED PyObject *__pyx_v___pyx_state) {
- PyObject *__pyx_r = NULL;
- __Pyx_RefNannyDeclarations
- PyObject *__pyx_t_1 = NULL;
- int __pyx_lineno = 0;
- const char *__pyx_filename = NULL;
- int __pyx_clineno = 0;
- __Pyx_RefNannySetupContext("__setstate_cython__", 0);
-
- /* "(tree fragment)":4
- * raise TypeError("no default __reduce__ due to non-trivial __cinit__")
- * def __setstate_cython__(self, __pyx_state):
- * raise TypeError("no default __reduce__ due to non-trivial __cinit__") # <<<<<<<<<<<<<<
- */
- __pyx_t_1 = __Pyx_PyObject_Call(__pyx_builtin_TypeError, __pyx_tuple__8, NULL); if (unlikely(!__pyx_t_1)) __PYX_ERR(1, 4, __pyx_L1_error)
- __Pyx_GOTREF(__pyx_t_1);
- __Pyx_Raise(__pyx_t_1, 0, 0, 0);
- __Pyx_DECREF(__pyx_t_1); __pyx_t_1 = 0;
- __PYX_ERR(1, 4, __pyx_L1_error)
-
- /* "(tree fragment)":3
- * def __reduce_cython__(self):
- * raise TypeError("no default __reduce__ due to non-trivial __cinit__")
- * def __setstate_cython__(self, __pyx_state): # <<<<<<<<<<<<<<
- * raise TypeError("no default __reduce__ due to non-trivial __cinit__")
- */
-
- /* function exit code */
- __pyx_L1_error:;
- __Pyx_XDECREF(__pyx_t_1);
- __Pyx_AddTraceback("View.MemoryView.array.__setstate_cython__", __pyx_clineno, __pyx_lineno, __pyx_filename);
- __pyx_r = NULL;
- __Pyx_XGIVEREF(__pyx_r);
- __Pyx_RefNannyFinishContext();
- return __pyx_r;
-}
-
-/* "View.MemoryView":244
- *
- * @cname("__pyx_array_new")
- * cdef array array_cwrapper(tuple shape, Py_ssize_t itemsize, char *format, # <<<<<<<<<<<<<<
- * char *mode, char *buf):
- * cdef array result
- */
-
-static struct __pyx_array_obj *__pyx_array_new(PyObject *__pyx_v_shape, Py_ssize_t __pyx_v_itemsize, char *__pyx_v_format, char *__pyx_v_mode, char *__pyx_v_buf) {
- struct __pyx_array_obj *__pyx_v_result = 0;
- struct __pyx_array_obj *__pyx_r = NULL;
- __Pyx_RefNannyDeclarations
- int __pyx_t_1;
- PyObject *__pyx_t_2 = NULL;
- PyObject *__pyx_t_3 = NULL;
- PyObject *__pyx_t_4 = NULL;
- PyObject *__pyx_t_5 = NULL;
- int __pyx_lineno = 0;
- const char *__pyx_filename = NULL;
- int __pyx_clineno = 0;
- __Pyx_RefNannySetupContext("array_cwrapper", 0);
-
- /* "View.MemoryView":248
- * cdef array result
- *
- * if buf == NULL: # <<<<<<<<<<<<<<
- * result = array(shape, itemsize, format, mode.decode('ASCII'))
- * else:
- */
- __pyx_t_1 = ((__pyx_v_buf == NULL) != 0);
- if (__pyx_t_1) {
-
- /* "View.MemoryView":249
- *
- * if buf == NULL:
- * result = array(shape, itemsize, format, mode.decode('ASCII')) # <<<<<<<<<<<<<<
- * else:
- * result = array(shape, itemsize, format, mode.decode('ASCII'),
- */
- __pyx_t_2 = PyInt_FromSsize_t(__pyx_v_itemsize); if (unlikely(!__pyx_t_2)) __PYX_ERR(1, 249, __pyx_L1_error)
- __Pyx_GOTREF(__pyx_t_2);
- __pyx_t_3 = __Pyx_PyBytes_FromString(__pyx_v_format); if (unlikely(!__pyx_t_3)) __PYX_ERR(1, 249, __pyx_L1_error)
- __Pyx_GOTREF(__pyx_t_3);
- __pyx_t_4 = __Pyx_decode_c_string(__pyx_v_mode, 0, strlen(__pyx_v_mode), NULL, NULL, PyUnicode_DecodeASCII); if (unlikely(!__pyx_t_4)) __PYX_ERR(1, 249, __pyx_L1_error)
- __Pyx_GOTREF(__pyx_t_4);
- __pyx_t_5 = PyTuple_New(4); if (unlikely(!__pyx_t_5)) __PYX_ERR(1, 249, __pyx_L1_error)
- __Pyx_GOTREF(__pyx_t_5);
- __Pyx_INCREF(__pyx_v_shape);
- __Pyx_GIVEREF(__pyx_v_shape);
- PyTuple_SET_ITEM(__pyx_t_5, 0, __pyx_v_shape);
- __Pyx_GIVEREF(__pyx_t_2);
- PyTuple_SET_ITEM(__pyx_t_5, 1, __pyx_t_2);
- __Pyx_GIVEREF(__pyx_t_3);
- PyTuple_SET_ITEM(__pyx_t_5, 2, __pyx_t_3);
- __Pyx_GIVEREF(__pyx_t_4);
- PyTuple_SET_ITEM(__pyx_t_5, 3, __pyx_t_4);
- __pyx_t_2 = 0;
- __pyx_t_3 = 0;
- __pyx_t_4 = 0;
- __pyx_t_4 = __Pyx_PyObject_Call(((PyObject *)__pyx_array_type), __pyx_t_5, NULL); if (unlikely(!__pyx_t_4)) __PYX_ERR(1, 249, __pyx_L1_error)
- __Pyx_GOTREF(__pyx_t_4);
- __Pyx_DECREF(__pyx_t_5); __pyx_t_5 = 0;
- __pyx_v_result = ((struct __pyx_array_obj *)__pyx_t_4);
- __pyx_t_4 = 0;
-
- /* "View.MemoryView":248
- * cdef array result
- *
- * if buf == NULL: # <<<<<<<<<<<<<<
- * result = array(shape, itemsize, format, mode.decode('ASCII'))
- * else:
- */
- goto __pyx_L3;
- }
-
- /* "View.MemoryView":251
- * result = array(shape, itemsize, format, mode.decode('ASCII'))
- * else:
- * result = array(shape, itemsize, format, mode.decode('ASCII'), # <<<<<<<<<<<<<<
- * allocate_buffer=False)
- * result.data = buf
- */
- /*else*/ {
- __pyx_t_4 = PyInt_FromSsize_t(__pyx_v_itemsize); if (unlikely(!__pyx_t_4)) __PYX_ERR(1, 251, __pyx_L1_error)
- __Pyx_GOTREF(__pyx_t_4);
- __pyx_t_5 = __Pyx_PyBytes_FromString(__pyx_v_format); if (unlikely(!__pyx_t_5)) __PYX_ERR(1, 251, __pyx_L1_error)
- __Pyx_GOTREF(__pyx_t_5);
- __pyx_t_3 = __Pyx_decode_c_string(__pyx_v_mode, 0, strlen(__pyx_v_mode), NULL, NULL, PyUnicode_DecodeASCII); if (unlikely(!__pyx_t_3)) __PYX_ERR(1, 251, __pyx_L1_error)
- __Pyx_GOTREF(__pyx_t_3);
- __pyx_t_2 = PyTuple_New(4); if (unlikely(!__pyx_t_2)) __PYX_ERR(1, 251, __pyx_L1_error)
- __Pyx_GOTREF(__pyx_t_2);
- __Pyx_INCREF(__pyx_v_shape);
- __Pyx_GIVEREF(__pyx_v_shape);
- PyTuple_SET_ITEM(__pyx_t_2, 0, __pyx_v_shape);
- __Pyx_GIVEREF(__pyx_t_4);
- PyTuple_SET_ITEM(__pyx_t_2, 1, __pyx_t_4);
- __Pyx_GIVEREF(__pyx_t_5);
- PyTuple_SET_ITEM(__pyx_t_2, 2, __pyx_t_5);
- __Pyx_GIVEREF(__pyx_t_3);
- PyTuple_SET_ITEM(__pyx_t_2, 3, __pyx_t_3);
- __pyx_t_4 = 0;
- __pyx_t_5 = 0;
- __pyx_t_3 = 0;
-
- /* "View.MemoryView":252
- * else:
- * result = array(shape, itemsize, format, mode.decode('ASCII'),
- * allocate_buffer=False) # <<<<<<<<<<<<<<
- * result.data = buf
- *
- */
- __pyx_t_3 = __Pyx_PyDict_NewPresized(1); if (unlikely(!__pyx_t_3)) __PYX_ERR(1, 252, __pyx_L1_error)
- __Pyx_GOTREF(__pyx_t_3);
- if (PyDict_SetItem(__pyx_t_3, __pyx_n_s_allocate_buffer, Py_False) < 0) __PYX_ERR(1, 252, __pyx_L1_error)
-
- /* "View.MemoryView":251
- * result = array(shape, itemsize, format, mode.decode('ASCII'))
- * else:
- * result = array(shape, itemsize, format, mode.decode('ASCII'), # <<<<<<<<<<<<<<
- * allocate_buffer=False)
- * result.data = buf
- */
- __pyx_t_5 = __Pyx_PyObject_Call(((PyObject *)__pyx_array_type), __pyx_t_2, __pyx_t_3); if (unlikely(!__pyx_t_5)) __PYX_ERR(1, 251, __pyx_L1_error)
- __Pyx_GOTREF(__pyx_t_5);
- __Pyx_DECREF(__pyx_t_2); __pyx_t_2 = 0;
- __Pyx_DECREF(__pyx_t_3); __pyx_t_3 = 0;
- __pyx_v_result = ((struct __pyx_array_obj *)__pyx_t_5);
- __pyx_t_5 = 0;
-
- /* "View.MemoryView":253
- * result = array(shape, itemsize, format, mode.decode('ASCII'),
- * allocate_buffer=False)
- * result.data = buf # <<<<<<<<<<<<<<
- *
- * return result
- */
- __pyx_v_result->data = __pyx_v_buf;
- }
- __pyx_L3:;
-
- /* "View.MemoryView":255
- * result.data = buf
- *
- * return result # <<<<<<<<<<<<<<
- *
- *
- */
- __Pyx_XDECREF(((PyObject *)__pyx_r));
- __Pyx_INCREF(((PyObject *)__pyx_v_result));
- __pyx_r = __pyx_v_result;
- goto __pyx_L0;
-
- /* "View.MemoryView":244
- *
- * @cname("__pyx_array_new")
- * cdef array array_cwrapper(tuple shape, Py_ssize_t itemsize, char *format, # <<<<<<<<<<<<<<
- * char *mode, char *buf):
- * cdef array result
- */
-
- /* function exit code */
- __pyx_L1_error:;
- __Pyx_XDECREF(__pyx_t_2);
- __Pyx_XDECREF(__pyx_t_3);
- __Pyx_XDECREF(__pyx_t_4);
- __Pyx_XDECREF(__pyx_t_5);
- __Pyx_AddTraceback("View.MemoryView.array_cwrapper", __pyx_clineno, __pyx_lineno, __pyx_filename);
- __pyx_r = 0;
- __pyx_L0:;
- __Pyx_XDECREF((PyObject *)__pyx_v_result);
- __Pyx_XGIVEREF((PyObject *)__pyx_r);
- __Pyx_RefNannyFinishContext();
- return __pyx_r;
-}
-
-/* "View.MemoryView":281
- * cdef class Enum(object):
- * cdef object name
- * def __init__(self, name): # <<<<<<<<<<<<<<
- * self.name = name
- * def __repr__(self):
- */
-
-/* Python wrapper */
-static int __pyx_MemviewEnum___init__(PyObject *__pyx_v_self, PyObject *__pyx_args, PyObject *__pyx_kwds); /*proto*/
-static int __pyx_MemviewEnum___init__(PyObject *__pyx_v_self, PyObject *__pyx_args, PyObject *__pyx_kwds) {
- PyObject *__pyx_v_name = 0;
- int __pyx_lineno = 0;
- const char *__pyx_filename = NULL;
- int __pyx_clineno = 0;
- int __pyx_r;
- __Pyx_RefNannyDeclarations
- __Pyx_RefNannySetupContext("__init__ (wrapper)", 0);
- {
- static PyObject **__pyx_pyargnames[] = {&__pyx_n_s_name,0};
- PyObject* values[1] = {0};
- if (unlikely(__pyx_kwds)) {
- Py_ssize_t kw_args;
- const Py_ssize_t pos_args = PyTuple_GET_SIZE(__pyx_args);
- switch (pos_args) {
- case 1: values[0] = PyTuple_GET_ITEM(__pyx_args, 0);
- CYTHON_FALLTHROUGH;
- case 0: break;
- default: goto __pyx_L5_argtuple_error;
- }
- kw_args = PyDict_Size(__pyx_kwds);
- switch (pos_args) {
- case 0:
- if (likely((values[0] = __Pyx_PyDict_GetItemStr(__pyx_kwds, __pyx_n_s_name)) != 0)) kw_args--;
- else goto __pyx_L5_argtuple_error;
- }
- if (unlikely(kw_args > 0)) {
- if (unlikely(__Pyx_ParseOptionalKeywords(__pyx_kwds, __pyx_pyargnames, 0, values, pos_args, "__init__") < 0)) __PYX_ERR(1, 281, __pyx_L3_error)
- }
- } else if (PyTuple_GET_SIZE(__pyx_args) != 1) {
- goto __pyx_L5_argtuple_error;
- } else {
- values[0] = PyTuple_GET_ITEM(__pyx_args, 0);
- }
- __pyx_v_name = values[0];
- }
- goto __pyx_L4_argument_unpacking_done;
- __pyx_L5_argtuple_error:;
- __Pyx_RaiseArgtupleInvalid("__init__", 1, 1, 1, PyTuple_GET_SIZE(__pyx_args)); __PYX_ERR(1, 281, __pyx_L3_error)
- __pyx_L3_error:;
- __Pyx_AddTraceback("View.MemoryView.Enum.__init__", __pyx_clineno, __pyx_lineno, __pyx_filename);
- __Pyx_RefNannyFinishContext();
- return -1;
- __pyx_L4_argument_unpacking_done:;
- __pyx_r = __pyx_MemviewEnum___pyx_pf_15View_dot_MemoryView_4Enum___init__(((struct __pyx_MemviewEnum_obj *)__pyx_v_self), __pyx_v_name);
-
- /* function exit code */
- __Pyx_RefNannyFinishContext();
- return __pyx_r;
-}
-
-static int __pyx_MemviewEnum___pyx_pf_15View_dot_MemoryView_4Enum___init__(struct __pyx_MemviewEnum_obj *__pyx_v_self, PyObject *__pyx_v_name) {
- int __pyx_r;
- __Pyx_RefNannyDeclarations
- __Pyx_RefNannySetupContext("__init__", 0);
-
- /* "View.MemoryView":282
- * cdef object name
- * def __init__(self, name):
- * self.name = name # <<<<<<<<<<<<<<
- * def __repr__(self):
- * return self.name
- */
- __Pyx_INCREF(__pyx_v_name);
- __Pyx_GIVEREF(__pyx_v_name);
- __Pyx_GOTREF(__pyx_v_self->name);
- __Pyx_DECREF(__pyx_v_self->name);
- __pyx_v_self->name = __pyx_v_name;
-
- /* "View.MemoryView":281
- * cdef class Enum(object):
- * cdef object name
- * def __init__(self, name): # <<<<<<<<<<<<<<
- * self.name = name
- * def __repr__(self):
- */
-
- /* function exit code */
- __pyx_r = 0;
- __Pyx_RefNannyFinishContext();
- return __pyx_r;
-}
-
-/* "View.MemoryView":283
- * def __init__(self, name):
- * self.name = name
- * def __repr__(self): # <<<<<<<<<<<<<<
- * return self.name
- *
- */
-
-/* Python wrapper */
-static PyObject *__pyx_MemviewEnum___repr__(PyObject *__pyx_v_self); /*proto*/
-static PyObject *__pyx_MemviewEnum___repr__(PyObject *__pyx_v_self) {
- PyObject *__pyx_r = 0;
- __Pyx_RefNannyDeclarations
- __Pyx_RefNannySetupContext("__repr__ (wrapper)", 0);
- __pyx_r = __pyx_MemviewEnum___pyx_pf_15View_dot_MemoryView_4Enum_2__repr__(((struct __pyx_MemviewEnum_obj *)__pyx_v_self));
-
- /* function exit code */
- __Pyx_RefNannyFinishContext();
- return __pyx_r;
-}
-
-static PyObject *__pyx_MemviewEnum___pyx_pf_15View_dot_MemoryView_4Enum_2__repr__(struct __pyx_MemviewEnum_obj *__pyx_v_self) {
- PyObject *__pyx_r = NULL;
- __Pyx_RefNannyDeclarations
- __Pyx_RefNannySetupContext("__repr__", 0);
-
- /* "View.MemoryView":284
- * self.name = name
- * def __repr__(self):
- * return self.name # <<<<<<<<<<<<<<
- *
- * cdef generic = Enum("")
- */
- __Pyx_XDECREF(__pyx_r);
- __Pyx_INCREF(__pyx_v_self->name);
- __pyx_r = __pyx_v_self->name;
- goto __pyx_L0;
-
- /* "View.MemoryView":283
- * def __init__(self, name):
- * self.name = name
- * def __repr__(self): # <<<<<<<<<<<<<<
- * return self.name
- *
- */
-
- /* function exit code */
- __pyx_L0:;
- __Pyx_XGIVEREF(__pyx_r);
- __Pyx_RefNannyFinishContext();
- return __pyx_r;
-}
-
-/* "(tree fragment)":1
- * def __reduce_cython__(self): # <<<<<<<<<<<<<<
- * cdef tuple state
- * cdef object _dict
- */
-
-/* Python wrapper */
-static PyObject *__pyx_pw___pyx_MemviewEnum_1__reduce_cython__(PyObject *__pyx_v_self, CYTHON_UNUSED PyObject *unused); /*proto*/
-static PyObject *__pyx_pw___pyx_MemviewEnum_1__reduce_cython__(PyObject *__pyx_v_self, CYTHON_UNUSED PyObject *unused) {
- PyObject *__pyx_r = 0;
- __Pyx_RefNannyDeclarations
- __Pyx_RefNannySetupContext("__reduce_cython__ (wrapper)", 0);
- __pyx_r = __pyx_pf___pyx_MemviewEnum___reduce_cython__(((struct __pyx_MemviewEnum_obj *)__pyx_v_self));
-
- /* function exit code */
- __Pyx_RefNannyFinishContext();
- return __pyx_r;
-}
-
-static PyObject *__pyx_pf___pyx_MemviewEnum___reduce_cython__(struct __pyx_MemviewEnum_obj *__pyx_v_self) {
- PyObject *__pyx_v_state = 0;
- PyObject *__pyx_v__dict = 0;
- int __pyx_v_use_setstate;
- PyObject *__pyx_r = NULL;
- __Pyx_RefNannyDeclarations
- PyObject *__pyx_t_1 = NULL;
- int __pyx_t_2;
- int __pyx_t_3;
- PyObject *__pyx_t_4 = NULL;
- PyObject *__pyx_t_5 = NULL;
- int __pyx_lineno = 0;
- const char *__pyx_filename = NULL;
- int __pyx_clineno = 0;
- __Pyx_RefNannySetupContext("__reduce_cython__", 0);
-
- /* "(tree fragment)":5
- * cdef object _dict
- * cdef bint use_setstate
- * state = (self.name,) # <<<<<<<<<<<<<<
- * _dict = getattr(self, '__dict__', None)
- * if _dict is not None:
- */
- __pyx_t_1 = PyTuple_New(1); if (unlikely(!__pyx_t_1)) __PYX_ERR(1, 5, __pyx_L1_error)
- __Pyx_GOTREF(__pyx_t_1);
- __Pyx_INCREF(__pyx_v_self->name);
- __Pyx_GIVEREF(__pyx_v_self->name);
- PyTuple_SET_ITEM(__pyx_t_1, 0, __pyx_v_self->name);
- __pyx_v_state = ((PyObject*)__pyx_t_1);
- __pyx_t_1 = 0;
-
- /* "(tree fragment)":6
- * cdef bint use_setstate
- * state = (self.name,)
- * _dict = getattr(self, '__dict__', None) # <<<<<<<<<<<<<<
- * if _dict is not None:
- * state += (_dict,)
- */
- __pyx_t_1 = __Pyx_GetAttr3(((PyObject *)__pyx_v_self), __pyx_n_s_dict, Py_None); if (unlikely(!__pyx_t_1)) __PYX_ERR(1, 6, __pyx_L1_error)
- __Pyx_GOTREF(__pyx_t_1);
- __pyx_v__dict = __pyx_t_1;
- __pyx_t_1 = 0;
-
- /* "(tree fragment)":7
- * state = (self.name,)
- * _dict = getattr(self, '__dict__', None)
- * if _dict is not None: # <<<<<<<<<<<<<<
- * state += (_dict,)
- * use_setstate = True
- */
- __pyx_t_2 = (__pyx_v__dict != Py_None);
- __pyx_t_3 = (__pyx_t_2 != 0);
- if (__pyx_t_3) {
-
- /* "(tree fragment)":8
- * _dict = getattr(self, '__dict__', None)
- * if _dict is not None:
- * state += (_dict,) # <<<<<<<<<<<<<<
- * use_setstate = True
- * else:
- */
- __pyx_t_1 = PyTuple_New(1); if (unlikely(!__pyx_t_1)) __PYX_ERR(1, 8, __pyx_L1_error)
- __Pyx_GOTREF(__pyx_t_1);
- __Pyx_INCREF(__pyx_v__dict);
- __Pyx_GIVEREF(__pyx_v__dict);
- PyTuple_SET_ITEM(__pyx_t_1, 0, __pyx_v__dict);
- __pyx_t_4 = PyNumber_InPlaceAdd(__pyx_v_state, __pyx_t_1); if (unlikely(!__pyx_t_4)) __PYX_ERR(1, 8, __pyx_L1_error)
- __Pyx_GOTREF(__pyx_t_4);
- __Pyx_DECREF(__pyx_t_1); __pyx_t_1 = 0;
- __Pyx_DECREF_SET(__pyx_v_state, ((PyObject*)__pyx_t_4));
- __pyx_t_4 = 0;
-
- /* "(tree fragment)":9
- * if _dict is not None:
- * state += (_dict,)
- * use_setstate = True # <<<<<<<<<<<<<<
- * else:
- * use_setstate = self.name is not None
- */
- __pyx_v_use_setstate = 1;
-
- /* "(tree fragment)":7
- * state = (self.name,)
- * _dict = getattr(self, '__dict__', None)
- * if _dict is not None: # <<<<<<<<<<<<<<
- * state += (_dict,)
- * use_setstate = True
- */
- goto __pyx_L3;
- }
-
- /* "(tree fragment)":11
- * use_setstate = True
- * else:
- * use_setstate = self.name is not None # <<<<<<<<<<<<<<
- * if use_setstate:
- * return __pyx_unpickle_Enum, (type(self), 0xb068931, None), state
- */
- /*else*/ {
- __pyx_t_3 = (__pyx_v_self->name != Py_None);
- __pyx_v_use_setstate = __pyx_t_3;
- }
- __pyx_L3:;
-
- /* "(tree fragment)":12
- * else:
- * use_setstate = self.name is not None
- * if use_setstate: # <<<<<<<<<<<<<<
- * return __pyx_unpickle_Enum, (type(self), 0xb068931, None), state
- * else:
- */
- __pyx_t_3 = (__pyx_v_use_setstate != 0);
- if (__pyx_t_3) {
-
- /* "(tree fragment)":13
- * use_setstate = self.name is not None
- * if use_setstate:
- * return __pyx_unpickle_Enum, (type(self), 0xb068931, None), state # <<<<<<<<<<<<<<
- * else:
- * return __pyx_unpickle_Enum, (type(self), 0xb068931, state)
- */
- __Pyx_XDECREF(__pyx_r);
- __Pyx_GetModuleGlobalName(__pyx_t_4, __pyx_n_s_pyx_unpickle_Enum); if (unlikely(!__pyx_t_4)) __PYX_ERR(1, 13, __pyx_L1_error)
- __Pyx_GOTREF(__pyx_t_4);
- __pyx_t_1 = PyTuple_New(3); if (unlikely(!__pyx_t_1)) __PYX_ERR(1, 13, __pyx_L1_error)
- __Pyx_GOTREF(__pyx_t_1);
- __Pyx_INCREF(((PyObject *)Py_TYPE(((PyObject *)__pyx_v_self))));
- __Pyx_GIVEREF(((PyObject *)Py_TYPE(((PyObject *)__pyx_v_self))));
- PyTuple_SET_ITEM(__pyx_t_1, 0, ((PyObject *)Py_TYPE(((PyObject *)__pyx_v_self))));
- __Pyx_INCREF(__pyx_int_184977713);
- __Pyx_GIVEREF(__pyx_int_184977713);
- PyTuple_SET_ITEM(__pyx_t_1, 1, __pyx_int_184977713);
- __Pyx_INCREF(Py_None);
- __Pyx_GIVEREF(Py_None);
- PyTuple_SET_ITEM(__pyx_t_1, 2, Py_None);
- __pyx_t_5 = PyTuple_New(3); if (unlikely(!__pyx_t_5)) __PYX_ERR(1, 13, __pyx_L1_error)
- __Pyx_GOTREF(__pyx_t_5);
- __Pyx_GIVEREF(__pyx_t_4);
- PyTuple_SET_ITEM(__pyx_t_5, 0, __pyx_t_4);
- __Pyx_GIVEREF(__pyx_t_1);
- PyTuple_SET_ITEM(__pyx_t_5, 1, __pyx_t_1);
- __Pyx_INCREF(__pyx_v_state);
- __Pyx_GIVEREF(__pyx_v_state);
- PyTuple_SET_ITEM(__pyx_t_5, 2, __pyx_v_state);
- __pyx_t_4 = 0;
- __pyx_t_1 = 0;
- __pyx_r = __pyx_t_5;
- __pyx_t_5 = 0;
- goto __pyx_L0;
-
- /* "(tree fragment)":12
- * else:
- * use_setstate = self.name is not None
- * if use_setstate: # <<<<<<<<<<<<<<
- * return __pyx_unpickle_Enum, (type(self), 0xb068931, None), state
- * else:
- */
- }
-
- /* "(tree fragment)":15
- * return __pyx_unpickle_Enum, (type(self), 0xb068931, None), state
- * else:
- * return __pyx_unpickle_Enum, (type(self), 0xb068931, state) # <<<<<<<<<<<<<<
- * def __setstate_cython__(self, __pyx_state):
- * __pyx_unpickle_Enum__set_state(self, __pyx_state)
- */
- /*else*/ {
- __Pyx_XDECREF(__pyx_r);
- __Pyx_GetModuleGlobalName(__pyx_t_5, __pyx_n_s_pyx_unpickle_Enum); if (unlikely(!__pyx_t_5)) __PYX_ERR(1, 15, __pyx_L1_error)
- __Pyx_GOTREF(__pyx_t_5);
- __pyx_t_1 = PyTuple_New(3); if (unlikely(!__pyx_t_1)) __PYX_ERR(1, 15, __pyx_L1_error)
- __Pyx_GOTREF(__pyx_t_1);
- __Pyx_INCREF(((PyObject *)Py_TYPE(((PyObject *)__pyx_v_self))));
- __Pyx_GIVEREF(((PyObject *)Py_TYPE(((PyObject *)__pyx_v_self))));
- PyTuple_SET_ITEM(__pyx_t_1, 0, ((PyObject *)Py_TYPE(((PyObject *)__pyx_v_self))));
- __Pyx_INCREF(__pyx_int_184977713);
- __Pyx_GIVEREF(__pyx_int_184977713);
- PyTuple_SET_ITEM(__pyx_t_1, 1, __pyx_int_184977713);
- __Pyx_INCREF(__pyx_v_state);
- __Pyx_GIVEREF(__pyx_v_state);
- PyTuple_SET_ITEM(__pyx_t_1, 2, __pyx_v_state);
- __pyx_t_4 = PyTuple_New(2); if (unlikely(!__pyx_t_4)) __PYX_ERR(1, 15, __pyx_L1_error)
- __Pyx_GOTREF(__pyx_t_4);
- __Pyx_GIVEREF(__pyx_t_5);
- PyTuple_SET_ITEM(__pyx_t_4, 0, __pyx_t_5);
- __Pyx_GIVEREF(__pyx_t_1);
- PyTuple_SET_ITEM(__pyx_t_4, 1, __pyx_t_1);
- __pyx_t_5 = 0;
- __pyx_t_1 = 0;
- __pyx_r = __pyx_t_4;
- __pyx_t_4 = 0;
- goto __pyx_L0;
- }
-
- /* "(tree fragment)":1
- * def __reduce_cython__(self): # <<<<<<<<<<<<<<
- * cdef tuple state
- * cdef object _dict
- */
-
- /* function exit code */
- __pyx_L1_error:;
- __Pyx_XDECREF(__pyx_t_1);
- __Pyx_XDECREF(__pyx_t_4);
- __Pyx_XDECREF(__pyx_t_5);
- __Pyx_AddTraceback("View.MemoryView.Enum.__reduce_cython__", __pyx_clineno, __pyx_lineno, __pyx_filename);
- __pyx_r = NULL;
- __pyx_L0:;
- __Pyx_XDECREF(__pyx_v_state);
- __Pyx_XDECREF(__pyx_v__dict);
- __Pyx_XGIVEREF(__pyx_r);
- __Pyx_RefNannyFinishContext();
- return __pyx_r;
-}
-
-/* "(tree fragment)":16
- * else:
- * return __pyx_unpickle_Enum, (type(self), 0xb068931, state)
- * def __setstate_cython__(self, __pyx_state): # <<<<<<<<<<<<<<
- * __pyx_unpickle_Enum__set_state(self, __pyx_state)
- */
-
-/* Python wrapper */
-static PyObject *__pyx_pw___pyx_MemviewEnum_3__setstate_cython__(PyObject *__pyx_v_self, PyObject *__pyx_v___pyx_state); /*proto*/
-static PyObject *__pyx_pw___pyx_MemviewEnum_3__setstate_cython__(PyObject *__pyx_v_self, PyObject *__pyx_v___pyx_state) {
- PyObject *__pyx_r = 0;
- __Pyx_RefNannyDeclarations
- __Pyx_RefNannySetupContext("__setstate_cython__ (wrapper)", 0);
- __pyx_r = __pyx_pf___pyx_MemviewEnum_2__setstate_cython__(((struct __pyx_MemviewEnum_obj *)__pyx_v_self), ((PyObject *)__pyx_v___pyx_state));
-
- /* function exit code */
- __Pyx_RefNannyFinishContext();
- return __pyx_r;
-}
-
-static PyObject *__pyx_pf___pyx_MemviewEnum_2__setstate_cython__(struct __pyx_MemviewEnum_obj *__pyx_v_self, PyObject *__pyx_v___pyx_state) {
- PyObject *__pyx_r = NULL;
- __Pyx_RefNannyDeclarations
- PyObject *__pyx_t_1 = NULL;
- int __pyx_lineno = 0;
- const char *__pyx_filename = NULL;
- int __pyx_clineno = 0;
- __Pyx_RefNannySetupContext("__setstate_cython__", 0);
-
- /* "(tree fragment)":17
- * return __pyx_unpickle_Enum, (type(self), 0xb068931, state)
- * def __setstate_cython__(self, __pyx_state):
- * __pyx_unpickle_Enum__set_state(self, __pyx_state) # <<<<<<<<<<<<<<
- */
- if (!(likely(PyTuple_CheckExact(__pyx_v___pyx_state))||((__pyx_v___pyx_state) == Py_None)||(PyErr_Format(PyExc_TypeError, "Expected %.16s, got %.200s", "tuple", Py_TYPE(__pyx_v___pyx_state)->tp_name), 0))) __PYX_ERR(1, 17, __pyx_L1_error)
- __pyx_t_1 = __pyx_unpickle_Enum__set_state(__pyx_v_self, ((PyObject*)__pyx_v___pyx_state)); if (unlikely(!__pyx_t_1)) __PYX_ERR(1, 17, __pyx_L1_error)
- __Pyx_GOTREF(__pyx_t_1);
- __Pyx_DECREF(__pyx_t_1); __pyx_t_1 = 0;
-
- /* "(tree fragment)":16
- * else:
- * return __pyx_unpickle_Enum, (type(self), 0xb068931, state)
- * def __setstate_cython__(self, __pyx_state): # <<<<<<<<<<<<<<
- * __pyx_unpickle_Enum__set_state(self, __pyx_state)
- */
-
- /* function exit code */
- __pyx_r = Py_None; __Pyx_INCREF(Py_None);
- goto __pyx_L0;
- __pyx_L1_error:;
- __Pyx_XDECREF(__pyx_t_1);
- __Pyx_AddTraceback("View.MemoryView.Enum.__setstate_cython__", __pyx_clineno, __pyx_lineno, __pyx_filename);
- __pyx_r = NULL;
- __pyx_L0:;
- __Pyx_XGIVEREF(__pyx_r);
- __Pyx_RefNannyFinishContext();
- return __pyx_r;
-}
-
-/* "View.MemoryView":298
- *
- * @cname('__pyx_align_pointer')
- * cdef void *align_pointer(void *memory, size_t alignment) nogil: # <<<<<<<<<<<<<<
- * "Align pointer memory on a given boundary"
- * cdef Py_intptr_t aligned_p = memory
- */
-
-static void *__pyx_align_pointer(void *__pyx_v_memory, size_t __pyx_v_alignment) {
- Py_intptr_t __pyx_v_aligned_p;
- size_t __pyx_v_offset;
- void *__pyx_r;
- int __pyx_t_1;
-
- /* "View.MemoryView":300
- * cdef void *align_pointer(void *memory, size_t alignment) nogil:
- * "Align pointer memory on a given boundary"
- * cdef Py_intptr_t aligned_p = memory # <<<<<<<<<<<<<<
- * cdef size_t offset
- *
- */
- __pyx_v_aligned_p = ((Py_intptr_t)__pyx_v_memory);
-
- /* "View.MemoryView":304
- *
- * with cython.cdivision(True):
- * offset = aligned_p % alignment # <<<<<<<<<<<<<<
- *
- * if offset > 0:
- */
- __pyx_v_offset = (__pyx_v_aligned_p % __pyx_v_alignment);
-
- /* "View.MemoryView":306
- * offset = aligned_p % alignment
- *
- * if offset > 0: # <<<<<<<<<<<<<<
- * aligned_p += alignment - offset
- *
- */
- __pyx_t_1 = ((__pyx_v_offset > 0) != 0);
- if (__pyx_t_1) {
-
- /* "View.MemoryView":307
- *
- * if offset > 0:
- * aligned_p += alignment - offset # <<<<<<<<<<<<<<
- *
- * return aligned_p
- */
- __pyx_v_aligned_p = (__pyx_v_aligned_p + (__pyx_v_alignment - __pyx_v_offset));
-
- /* "View.MemoryView":306
- * offset = aligned_p % alignment
- *
- * if offset > 0: # <<<<<<<<<<<<<<
- * aligned_p += alignment - offset
- *
- */
- }
-
- /* "View.MemoryView":309
- * aligned_p += alignment - offset
- *
- * return aligned_p # <<<<<<<<<<<<<<
- *
- *
- */
- __pyx_r = ((void *)__pyx_v_aligned_p);
- goto __pyx_L0;
-
- /* "View.MemoryView":298
- *
- * @cname('__pyx_align_pointer')
- * cdef void *align_pointer(void *memory, size_t alignment) nogil: # <<<<<<<<<<<<<<
- * "Align pointer memory on a given boundary"
- * cdef Py_intptr_t aligned_p = memory
- */
-
- /* function exit code */
- __pyx_L0:;
- return __pyx_r;
-}
-
-/* "View.MemoryView":345
- * cdef __Pyx_TypeInfo *typeinfo
- *
- * def __cinit__(memoryview self, object obj, int flags, bint dtype_is_object=False): # <<<<<<<<<<<<<<
- * self.obj = obj
- * self.flags = flags
- */
-
-/* Python wrapper */
-static int __pyx_memoryview___cinit__(PyObject *__pyx_v_self, PyObject *__pyx_args, PyObject *__pyx_kwds); /*proto*/
-static int __pyx_memoryview___cinit__(PyObject *__pyx_v_self, PyObject *__pyx_args, PyObject *__pyx_kwds) {
- PyObject *__pyx_v_obj = 0;
- int __pyx_v_flags;
- int __pyx_v_dtype_is_object;
- int __pyx_lineno = 0;
- const char *__pyx_filename = NULL;
- int __pyx_clineno = 0;
- int __pyx_r;
- __Pyx_RefNannyDeclarations
- __Pyx_RefNannySetupContext("__cinit__ (wrapper)", 0);
- {
- static PyObject **__pyx_pyargnames[] = {&__pyx_n_s_obj,&__pyx_n_s_flags,&__pyx_n_s_dtype_is_object,0};
- PyObject* values[3] = {0,0,0};
- if (unlikely(__pyx_kwds)) {
- Py_ssize_t kw_args;
- const Py_ssize_t pos_args = PyTuple_GET_SIZE(__pyx_args);
- switch (pos_args) {
- case 3: values[2] = PyTuple_GET_ITEM(__pyx_args, 2);
- CYTHON_FALLTHROUGH;
- case 2: values[1] = PyTuple_GET_ITEM(__pyx_args, 1);
- CYTHON_FALLTHROUGH;
- case 1: values[0] = PyTuple_GET_ITEM(__pyx_args, 0);
- CYTHON_FALLTHROUGH;
- case 0: break;
- default: goto __pyx_L5_argtuple_error;
- }
- kw_args = PyDict_Size(__pyx_kwds);
- switch (pos_args) {
- case 0:
- if (likely((values[0] = __Pyx_PyDict_GetItemStr(__pyx_kwds, __pyx_n_s_obj)) != 0)) kw_args--;
- else goto __pyx_L5_argtuple_error;
- CYTHON_FALLTHROUGH;
- case 1:
- if (likely((values[1] = __Pyx_PyDict_GetItemStr(__pyx_kwds, __pyx_n_s_flags)) != 0)) kw_args--;
- else {
- __Pyx_RaiseArgtupleInvalid("__cinit__", 0, 2, 3, 1); __PYX_ERR(1, 345, __pyx_L3_error)
- }
- CYTHON_FALLTHROUGH;
- case 2:
- if (kw_args > 0) {
- PyObject* value = __Pyx_PyDict_GetItemStr(__pyx_kwds, __pyx_n_s_dtype_is_object);
- if (value) { values[2] = value; kw_args--; }
- }
- }
- if (unlikely(kw_args > 0)) {
- if (unlikely(__Pyx_ParseOptionalKeywords(__pyx_kwds, __pyx_pyargnames, 0, values, pos_args, "__cinit__") < 0)) __PYX_ERR(1, 345, __pyx_L3_error)
- }
- } else {
- switch (PyTuple_GET_SIZE(__pyx_args)) {
- case 3: values[2] = PyTuple_GET_ITEM(__pyx_args, 2);
- CYTHON_FALLTHROUGH;
- case 2: values[1] = PyTuple_GET_ITEM(__pyx_args, 1);
- values[0] = PyTuple_GET_ITEM(__pyx_args, 0);
- break;
- default: goto __pyx_L5_argtuple_error;
- }
- }
- __pyx_v_obj = values[0];
- __pyx_v_flags = __Pyx_PyInt_As_int(values[1]); if (unlikely((__pyx_v_flags == (int)-1) && PyErr_Occurred())) __PYX_ERR(1, 345, __pyx_L3_error)
- if (values[2]) {
- __pyx_v_dtype_is_object = __Pyx_PyObject_IsTrue(values[2]); if (unlikely((__pyx_v_dtype_is_object == (int)-1) && PyErr_Occurred())) __PYX_ERR(1, 345, __pyx_L3_error)
- } else {
- __pyx_v_dtype_is_object = ((int)0);
- }
- }
- goto __pyx_L4_argument_unpacking_done;
- __pyx_L5_argtuple_error:;
- __Pyx_RaiseArgtupleInvalid("__cinit__", 0, 2, 3, PyTuple_GET_SIZE(__pyx_args)); __PYX_ERR(1, 345, __pyx_L3_error)
- __pyx_L3_error:;
- __Pyx_AddTraceback("View.MemoryView.memoryview.__cinit__", __pyx_clineno, __pyx_lineno, __pyx_filename);
- __Pyx_RefNannyFinishContext();
- return -1;
- __pyx_L4_argument_unpacking_done:;
- __pyx_r = __pyx_memoryview___pyx_pf_15View_dot_MemoryView_10memoryview___cinit__(((struct __pyx_memoryview_obj *)__pyx_v_self), __pyx_v_obj, __pyx_v_flags, __pyx_v_dtype_is_object);
-
- /* function exit code */
- __Pyx_RefNannyFinishContext();
- return __pyx_r;
-}
-
-static int __pyx_memoryview___pyx_pf_15View_dot_MemoryView_10memoryview___cinit__(struct __pyx_memoryview_obj *__pyx_v_self, PyObject *__pyx_v_obj, int __pyx_v_flags, int __pyx_v_dtype_is_object) {
- int __pyx_r;
- __Pyx_RefNannyDeclarations
- int __pyx_t_1;
- int __pyx_t_2;
- int __pyx_t_3;
- int __pyx_t_4;
- int __pyx_lineno = 0;
- const char *__pyx_filename = NULL;
- int __pyx_clineno = 0;
- __Pyx_RefNannySetupContext("__cinit__", 0);
-
- /* "View.MemoryView":346
- *
- * def __cinit__(memoryview self, object obj, int flags, bint dtype_is_object=False):
- * self.obj = obj # <<<<<<<<<<<<<<
- * self.flags = flags
- * if type(self) is memoryview or obj is not None:
- */
- __Pyx_INCREF(__pyx_v_obj);
- __Pyx_GIVEREF(__pyx_v_obj);
- __Pyx_GOTREF(__pyx_v_self->obj);
- __Pyx_DECREF(__pyx_v_self->obj);
- __pyx_v_self->obj = __pyx_v_obj;
-
- /* "View.MemoryView":347
- * def __cinit__(memoryview self, object obj, int flags, bint dtype_is_object=False):
- * self.obj = obj
- * self.flags = flags # <<<<<<<<<<<<<<
- * if type(self) is memoryview or obj is not None:
- * __Pyx_GetBuffer(obj, &self.view, flags)
- */
- __pyx_v_self->flags = __pyx_v_flags;
-
- /* "View.MemoryView":348
- * self.obj = obj
- * self.flags = flags
- * if type(self) is memoryview or obj is not None: # <<<<<<<<<<<<<<
- * __Pyx_GetBuffer(obj, &self.view, flags)
- * if self.view.obj == NULL:
- */
- __pyx_t_2 = (((PyObject *)Py_TYPE(((PyObject *)__pyx_v_self))) == ((PyObject *)__pyx_memoryview_type));
- __pyx_t_3 = (__pyx_t_2 != 0);
- if (!__pyx_t_3) {
- } else {
- __pyx_t_1 = __pyx_t_3;
- goto __pyx_L4_bool_binop_done;
- }
- __pyx_t_3 = (__pyx_v_obj != Py_None);
- __pyx_t_2 = (__pyx_t_3 != 0);
- __pyx_t_1 = __pyx_t_2;
- __pyx_L4_bool_binop_done:;
- if (__pyx_t_1) {
-
- /* "View.MemoryView":349
- * self.flags = flags
- * if type(self) is memoryview or obj is not None:
- * __Pyx_GetBuffer(obj, &self.view, flags) # <<<<<<<<<<<<<<
- * if self.view.obj == NULL:
- * (<__pyx_buffer *> &self.view).obj = Py_None
- */
- __pyx_t_4 = __Pyx_GetBuffer(__pyx_v_obj, (&__pyx_v_self->view), __pyx_v_flags); if (unlikely(__pyx_t_4 == ((int)-1))) __PYX_ERR(1, 349, __pyx_L1_error)
-
- /* "View.MemoryView":350
- * if type(self) is memoryview or obj is not None:
- * __Pyx_GetBuffer(obj, &self.view, flags)
- * if self.view.obj == NULL: # <<<<<<<<<<<<<<
- * (<__pyx_buffer *> &self.view).obj = Py_None
- * Py_INCREF(Py_None)
- */
- __pyx_t_1 = ((((PyObject *)__pyx_v_self->view.obj) == NULL) != 0);
- if (__pyx_t_1) {
-
- /* "View.MemoryView":351
- * __Pyx_GetBuffer(obj, &self.view, flags)
- * if self.view.obj == NULL:
- * (<__pyx_buffer *> &self.view).obj = Py_None # <<<<<<<<<<<<<<
- * Py_INCREF(Py_None)
- *
- */
- ((Py_buffer *)(&__pyx_v_self->view))->obj = Py_None;
-
- /* "View.MemoryView":352
- * if self.view.obj == NULL:
- * (<__pyx_buffer *> &self.view).obj = Py_None
- * Py_INCREF(Py_None) # <<<<<<<<<<<<<<
- *
- * global __pyx_memoryview_thread_locks_used
- */
- Py_INCREF(Py_None);
-
- /* "View.MemoryView":350
- * if type(self) is memoryview or obj is not None:
- * __Pyx_GetBuffer(obj, &self.view, flags)
- * if self.view.obj == NULL: # <<<<<<<<<<<<<<
- * (<__pyx_buffer *> &self.view).obj = Py_None
- * Py_INCREF(Py_None)
- */
- }
-
- /* "View.MemoryView":348
- * self.obj = obj
- * self.flags = flags
- * if type(self) is memoryview or obj is not None: # <<<<<<<<<<<<<<
- * __Pyx_GetBuffer(obj, &self.view, flags)
- * if self.view.obj == NULL:
- */
- }
-
- /* "View.MemoryView":355
- *
- * global __pyx_memoryview_thread_locks_used
- * if __pyx_memoryview_thread_locks_used < THREAD_LOCKS_PREALLOCATED: # <<<<<<<<<<<<<<
- * self.lock = __pyx_memoryview_thread_locks[__pyx_memoryview_thread_locks_used]
- * __pyx_memoryview_thread_locks_used += 1
- */
- __pyx_t_1 = ((__pyx_memoryview_thread_locks_used < 8) != 0);
- if (__pyx_t_1) {
-
- /* "View.MemoryView":356
- * global __pyx_memoryview_thread_locks_used
- * if __pyx_memoryview_thread_locks_used < THREAD_LOCKS_PREALLOCATED:
- * self.lock = __pyx_memoryview_thread_locks[__pyx_memoryview_thread_locks_used] # <<<<<<<<<<<<<<
- * __pyx_memoryview_thread_locks_used += 1
- * if self.lock is NULL:
- */
- __pyx_v_self->lock = (__pyx_memoryview_thread_locks[__pyx_memoryview_thread_locks_used]);
-
- /* "View.MemoryView":357
- * if __pyx_memoryview_thread_locks_used < THREAD_LOCKS_PREALLOCATED:
- * self.lock = __pyx_memoryview_thread_locks[__pyx_memoryview_thread_locks_used]
- * __pyx_memoryview_thread_locks_used += 1 # <<<<<<<<<<<<<<
- * if self.lock is NULL:
- * self.lock = PyThread_allocate_lock()
- */
- __pyx_memoryview_thread_locks_used = (__pyx_memoryview_thread_locks_used + 1);
-
- /* "View.MemoryView":355
- *
- * global __pyx_memoryview_thread_locks_used
- * if __pyx_memoryview_thread_locks_used < THREAD_LOCKS_PREALLOCATED: # <<<<<<<<<<<<<<
- * self.lock = __pyx_memoryview_thread_locks[__pyx_memoryview_thread_locks_used]
- * __pyx_memoryview_thread_locks_used += 1
- */
- }
-
- /* "View.MemoryView":358
- * self.lock = __pyx_memoryview_thread_locks[__pyx_memoryview_thread_locks_used]
- * __pyx_memoryview_thread_locks_used += 1
- * if self.lock is NULL: # <<<<<<<<<<<<<<
- * self.lock = PyThread_allocate_lock()
- * if self.lock is NULL:
- */
- __pyx_t_1 = ((__pyx_v_self->lock == NULL) != 0);
- if (__pyx_t_1) {
-
- /* "View.MemoryView":359
- * __pyx_memoryview_thread_locks_used += 1
- * if self.lock is NULL:
- * self.lock = PyThread_allocate_lock() # <<<<<<<<<<<<<<
- * if self.lock is NULL:
- * raise MemoryError
- */
- __pyx_v_self->lock = PyThread_allocate_lock();
-
- /* "View.MemoryView":360
- * if self.lock is NULL:
- * self.lock = PyThread_allocate_lock()
- * if self.lock is NULL: # <<<<<<<<<<<<<<
- * raise MemoryError
- *
- */
- __pyx_t_1 = ((__pyx_v_self->lock == NULL) != 0);
- if (unlikely(__pyx_t_1)) {
-
- /* "View.MemoryView":361
- * self.lock = PyThread_allocate_lock()
- * if self.lock is NULL:
- * raise MemoryError # <<<<<<<<<<<<<<
- *
- * if flags & PyBUF_FORMAT:
- */
- PyErr_NoMemory(); __PYX_ERR(1, 361, __pyx_L1_error)
-
- /* "View.MemoryView":360
- * if self.lock is NULL:
- * self.lock = PyThread_allocate_lock()
- * if self.lock is NULL: # <<<<<<<<<<<<<<
- * raise MemoryError
- *
- */
- }
-
- /* "View.MemoryView":358
- * self.lock = __pyx_memoryview_thread_locks[__pyx_memoryview_thread_locks_used]
- * __pyx_memoryview_thread_locks_used += 1
- * if self.lock is NULL: # <<<<<<<<<<<<<<
- * self.lock = PyThread_allocate_lock()
- * if self.lock is NULL:
- */
- }
-
- /* "View.MemoryView":363
- * raise MemoryError
- *
- * if flags & PyBUF_FORMAT: # <<<<<<<<<<<<<<
- * self.dtype_is_object = (self.view.format[0] == b'O' and self.view.format[1] == b'\0')
- * else:
- */
- __pyx_t_1 = ((__pyx_v_flags & PyBUF_FORMAT) != 0);
- if (__pyx_t_1) {
-
- /* "View.MemoryView":364
- *
- * if flags & PyBUF_FORMAT:
- * self.dtype_is_object = (self.view.format[0] == b'O' and self.view.format[1] == b'\0') # <<<<<<<<<<<<<<
- * else:
- * self.dtype_is_object = dtype_is_object
- */
- __pyx_t_2 = (((__pyx_v_self->view.format[0]) == 'O') != 0);
- if (__pyx_t_2) {
- } else {
- __pyx_t_1 = __pyx_t_2;
- goto __pyx_L11_bool_binop_done;
- }
- __pyx_t_2 = (((__pyx_v_self->view.format[1]) == '\x00') != 0);
- __pyx_t_1 = __pyx_t_2;
- __pyx_L11_bool_binop_done:;
- __pyx_v_self->dtype_is_object = __pyx_t_1;
-
- /* "View.MemoryView":363
- * raise MemoryError
- *
- * if flags & PyBUF_FORMAT: # <<<<<<<<<<<<<<
- * self.dtype_is_object = (self.view.format[0] == b'O' and self.view.format[1] == b'\0')
- * else:
- */
- goto __pyx_L10;
- }
-
- /* "View.MemoryView":366
- * self.dtype_is_object = (self.view.format[0] == b'O' and self.view.format[1] == b'\0')
- * else:
- * self.dtype_is_object = dtype_is_object # <<<<<<<<<<<<<<
- *
- * self.acquisition_count_aligned_p = <__pyx_atomic_int *> align_pointer(
- */
- /*else*/ {
- __pyx_v_self->dtype_is_object = __pyx_v_dtype_is_object;
- }
- __pyx_L10:;
-
- /* "View.MemoryView":368
- * self.dtype_is_object = dtype_is_object
- *
- * self.acquisition_count_aligned_p = <__pyx_atomic_int *> align_pointer( # <<<<<<<<<<<<<<
- * &self.acquisition_count[0], sizeof(__pyx_atomic_int))
- * self.typeinfo = NULL
- */
- __pyx_v_self->acquisition_count_aligned_p = ((__pyx_atomic_int *)__pyx_align_pointer(((void *)(&(__pyx_v_self->acquisition_count[0]))), (sizeof(__pyx_atomic_int))));
-
- /* "View.MemoryView":370
- * self.acquisition_count_aligned_p = <__pyx_atomic_int *> align_pointer(
- * &self.acquisition_count[0], sizeof(__pyx_atomic_int))
- * self.typeinfo = NULL # <<<<<<<<<<<<<<
- *
- * def __dealloc__(memoryview self):
- */
- __pyx_v_self->typeinfo = NULL;
-
- /* "View.MemoryView":345
- * cdef __Pyx_TypeInfo *typeinfo
- *
- * def __cinit__(memoryview self, object obj, int flags, bint dtype_is_object=False): # <<<<<<<<<<<<<<
- * self.obj = obj
- * self.flags = flags
- */
-
- /* function exit code */
- __pyx_r = 0;
- goto __pyx_L0;
- __pyx_L1_error:;
- __Pyx_AddTraceback("View.MemoryView.memoryview.__cinit__", __pyx_clineno, __pyx_lineno, __pyx_filename);
- __pyx_r = -1;
- __pyx_L0:;
- __Pyx_RefNannyFinishContext();
- return __pyx_r;
-}
-
-/* "View.MemoryView":372
- * self.typeinfo = NULL
- *
- * def __dealloc__(memoryview self): # <<<<<<<<<<<<<<
- * if self.obj is not None:
- * __Pyx_ReleaseBuffer(&self.view)
- */
-
-/* Python wrapper */
-static void __pyx_memoryview___dealloc__(PyObject *__pyx_v_self); /*proto*/
-static void __pyx_memoryview___dealloc__(PyObject *__pyx_v_self) {
- __Pyx_RefNannyDeclarations
- __Pyx_RefNannySetupContext("__dealloc__ (wrapper)", 0);
- __pyx_memoryview___pyx_pf_15View_dot_MemoryView_10memoryview_2__dealloc__(((struct __pyx_memoryview_obj *)__pyx_v_self));
-
- /* function exit code */
- __Pyx_RefNannyFinishContext();
-}
-
-static void __pyx_memoryview___pyx_pf_15View_dot_MemoryView_10memoryview_2__dealloc__(struct __pyx_memoryview_obj *__pyx_v_self) {
- int __pyx_v_i;
- __Pyx_RefNannyDeclarations
- int __pyx_t_1;
- int __pyx_t_2;
- int __pyx_t_3;
- int __pyx_t_4;
- int __pyx_t_5;
- PyThread_type_lock __pyx_t_6;
- PyThread_type_lock __pyx_t_7;
- __Pyx_RefNannySetupContext("__dealloc__", 0);
-
- /* "View.MemoryView":373
- *
- * def __dealloc__(memoryview self):
- * if self.obj is not None: # <<<<<<<<<<<<<<
- * __Pyx_ReleaseBuffer(&self.view)
- * elif (<__pyx_buffer *> &self.view).obj == Py_None:
- */
- __pyx_t_1 = (__pyx_v_self->obj != Py_None);
- __pyx_t_2 = (__pyx_t_1 != 0);
- if (__pyx_t_2) {
-
- /* "View.MemoryView":374
- * def __dealloc__(memoryview self):
- * if self.obj is not None:
- * __Pyx_ReleaseBuffer(&self.view) # <<<<<<<<<<<<<<
- * elif (<__pyx_buffer *> &self.view).obj == Py_None:
- *
- */
- __Pyx_ReleaseBuffer((&__pyx_v_self->view));
-
- /* "View.MemoryView":373
- *
- * def __dealloc__(memoryview self):
- * if self.obj is not None: # <<<<<<<<<<<<<<
- * __Pyx_ReleaseBuffer(&self.view)
- * elif (<__pyx_buffer *> &self.view).obj == Py_None:
- */
- goto __pyx_L3;
- }
-
- /* "View.MemoryView":375
- * if self.obj is not None:
- * __Pyx_ReleaseBuffer(&self.view)
- * elif (<__pyx_buffer *> &self.view).obj == Py_None: # <<<<<<<<<<<<<<
- *
- * (<__pyx_buffer *> &self.view).obj = NULL
- */
- __pyx_t_2 = ((((Py_buffer *)(&__pyx_v_self->view))->obj == Py_None) != 0);
- if (__pyx_t_2) {
-
- /* "View.MemoryView":377
- * elif (<__pyx_buffer *> &self.view).obj == Py_None:
- *
- * (<__pyx_buffer *> &self.view).obj = NULL # <<<<<<<<<<<<<<
- * Py_DECREF(Py_None)
- *
- */
- ((Py_buffer *)(&__pyx_v_self->view))->obj = NULL;
-
- /* "View.MemoryView":378
- *
- * (<__pyx_buffer *> &self.view).obj = NULL
- * Py_DECREF(Py_None) # <<<<<<<<<<<<<<
- *
- * cdef int i
- */
- Py_DECREF(Py_None);
-
- /* "View.MemoryView":375
- * if self.obj is not None:
- * __Pyx_ReleaseBuffer(&self.view)
- * elif (<__pyx_buffer *> &self.view).obj == Py_None: # <<<<<<<<<<<<<<
- *
- * (<__pyx_buffer *> &self.view).obj = NULL
- */
- }
- __pyx_L3:;
-
- /* "View.MemoryView":382
- * cdef int i
- * global __pyx_memoryview_thread_locks_used
- * if self.lock != NULL: # <<<<<<<<<<<<<<
- * for i in range(__pyx_memoryview_thread_locks_used):
- * if __pyx_memoryview_thread_locks[i] is self.lock:
- */
- __pyx_t_2 = ((__pyx_v_self->lock != NULL) != 0);
- if (__pyx_t_2) {
-
- /* "View.MemoryView":383
- * global __pyx_memoryview_thread_locks_used
- * if self.lock != NULL:
- * for i in range(__pyx_memoryview_thread_locks_used): # <<<<<<<<<<<<<<
- * if __pyx_memoryview_thread_locks[i] is self.lock:
- * __pyx_memoryview_thread_locks_used -= 1
- */
- __pyx_t_3 = __pyx_memoryview_thread_locks_used;
- __pyx_t_4 = __pyx_t_3;
- for (__pyx_t_5 = 0; __pyx_t_5 < __pyx_t_4; __pyx_t_5+=1) {
- __pyx_v_i = __pyx_t_5;
-
- /* "View.MemoryView":384
- * if self.lock != NULL:
- * for i in range(__pyx_memoryview_thread_locks_used):
- * if __pyx_memoryview_thread_locks[i] is self.lock: # <<<<<<<<<<<<<<
- * __pyx_memoryview_thread_locks_used -= 1
- * if i != __pyx_memoryview_thread_locks_used:
- */
- __pyx_t_2 = (((__pyx_memoryview_thread_locks[__pyx_v_i]) == __pyx_v_self->lock) != 0);
- if (__pyx_t_2) {
-
- /* "View.MemoryView":385
- * for i in range(__pyx_memoryview_thread_locks_used):
- * if __pyx_memoryview_thread_locks[i] is self.lock:
- * __pyx_memoryview_thread_locks_used -= 1 # <<<<<<<<<<<<<<
- * if i != __pyx_memoryview_thread_locks_used:
- * __pyx_memoryview_thread_locks[i], __pyx_memoryview_thread_locks[__pyx_memoryview_thread_locks_used] = (
- */
- __pyx_memoryview_thread_locks_used = (__pyx_memoryview_thread_locks_used - 1);
-
- /* "View.MemoryView":386
- * if __pyx_memoryview_thread_locks[i] is self.lock:
- * __pyx_memoryview_thread_locks_used -= 1
- * if i != __pyx_memoryview_thread_locks_used: # <<<<<<<<<<<<<<
- * __pyx_memoryview_thread_locks[i], __pyx_memoryview_thread_locks[__pyx_memoryview_thread_locks_used] = (
- * __pyx_memoryview_thread_locks[__pyx_memoryview_thread_locks_used], __pyx_memoryview_thread_locks[i])
- */
- __pyx_t_2 = ((__pyx_v_i != __pyx_memoryview_thread_locks_used) != 0);
- if (__pyx_t_2) {
-
- /* "View.MemoryView":388
- * if i != __pyx_memoryview_thread_locks_used:
- * __pyx_memoryview_thread_locks[i], __pyx_memoryview_thread_locks[__pyx_memoryview_thread_locks_used] = (
- * __pyx_memoryview_thread_locks[__pyx_memoryview_thread_locks_used], __pyx_memoryview_thread_locks[i]) # <<<<<<<<<<<<<<
- * break
- * else:
- */
- __pyx_t_6 = (__pyx_memoryview_thread_locks[__pyx_memoryview_thread_locks_used]);
- __pyx_t_7 = (__pyx_memoryview_thread_locks[__pyx_v_i]);
-
- /* "View.MemoryView":387
- * __pyx_memoryview_thread_locks_used -= 1
- * if i != __pyx_memoryview_thread_locks_used:
- * __pyx_memoryview_thread_locks[i], __pyx_memoryview_thread_locks[__pyx_memoryview_thread_locks_used] = ( # <<<<<<<<<<<<<<
- * __pyx_memoryview_thread_locks[__pyx_memoryview_thread_locks_used], __pyx_memoryview_thread_locks[i])
- * break
- */
- (__pyx_memoryview_thread_locks[__pyx_v_i]) = __pyx_t_6;
- (__pyx_memoryview_thread_locks[__pyx_memoryview_thread_locks_used]) = __pyx_t_7;
-
- /* "View.MemoryView":386
- * if __pyx_memoryview_thread_locks[i] is self.lock:
- * __pyx_memoryview_thread_locks_used -= 1
- * if i != __pyx_memoryview_thread_locks_used: # <<<<<<<<<<<<<<
- * __pyx_memoryview_thread_locks[i], __pyx_memoryview_thread_locks[__pyx_memoryview_thread_locks_used] = (
- * __pyx_memoryview_thread_locks[__pyx_memoryview_thread_locks_used], __pyx_memoryview_thread_locks[i])
- */
- }
-
- /* "View.MemoryView":389
- * __pyx_memoryview_thread_locks[i], __pyx_memoryview_thread_locks[__pyx_memoryview_thread_locks_used] = (
- * __pyx_memoryview_thread_locks[__pyx_memoryview_thread_locks_used], __pyx_memoryview_thread_locks[i])
- * break # <<<<<<<<<<<<<<
- * else:
- * PyThread_free_lock(self.lock)
- */
- goto __pyx_L6_break;
-
- /* "View.MemoryView":384
- * if self.lock != NULL:
- * for i in range(__pyx_memoryview_thread_locks_used):
- * if __pyx_memoryview_thread_locks[i] is self.lock: # <<<<<<<<<<<<<<
- * __pyx_memoryview_thread_locks_used -= 1
- * if i != __pyx_memoryview_thread_locks_used:
- */
- }
- }
- /*else*/ {
-
- /* "View.MemoryView":391
- * break
- * else:
- * PyThread_free_lock(self.lock) # <<<<<<<<<<<<<<
- *
- * cdef char *get_item_pointer(memoryview self, object index) except NULL:
- */
- PyThread_free_lock(__pyx_v_self->lock);
- }
- __pyx_L6_break:;
-
- /* "View.MemoryView":382
- * cdef int i
- * global __pyx_memoryview_thread_locks_used
- * if self.lock != NULL: # <<<<<<<<<<<<<<
- * for i in range(__pyx_memoryview_thread_locks_used):
- * if __pyx_memoryview_thread_locks[i] is self.lock:
- */
- }
-
- /* "View.MemoryView":372
- * self.typeinfo = NULL
- *
- * def __dealloc__(memoryview self): # <<<<<<<<<<<<<<
- * if self.obj is not None:
- * __Pyx_ReleaseBuffer(&self.view)
- */
-
- /* function exit code */
- __Pyx_RefNannyFinishContext();
-}
-
-/* "View.MemoryView":393
- * PyThread_free_lock(self.lock)
- *
- * cdef char *get_item_pointer(memoryview self, object index) except NULL: # <<<<<<<<<<<<<<
- * cdef Py_ssize_t dim
- * cdef char *itemp = self.view.buf
- */
-
-static char *__pyx_memoryview_get_item_pointer(struct __pyx_memoryview_obj *__pyx_v_self, PyObject *__pyx_v_index) {
- Py_ssize_t __pyx_v_dim;
- char *__pyx_v_itemp;
- PyObject *__pyx_v_idx = NULL;
- char *__pyx_r;
- __Pyx_RefNannyDeclarations
- Py_ssize_t __pyx_t_1;
- PyObject *__pyx_t_2 = NULL;
- Py_ssize_t __pyx_t_3;
- PyObject *(*__pyx_t_4)(PyObject *);
- PyObject *__pyx_t_5 = NULL;
- Py_ssize_t __pyx_t_6;
- char *__pyx_t_7;
- int __pyx_lineno = 0;
- const char *__pyx_filename = NULL;
- int __pyx_clineno = 0;
- __Pyx_RefNannySetupContext("get_item_pointer", 0);
-
- /* "View.MemoryView":395
- * cdef char *get_item_pointer(memoryview self, object index) except NULL:
- * cdef Py_ssize_t dim
- * cdef char *itemp = self.view.buf # <<<<<<<<<<<<<<
- *
- * for dim, idx in enumerate(index):
- */
- __pyx_v_itemp = ((char *)__pyx_v_self->view.buf);
-
- /* "View.MemoryView":397
- * cdef char *itemp = self.view.buf
- *
- * for dim, idx in enumerate(index): # <<<<<<<<<<<<<<
- * itemp = pybuffer_index(&self.view, itemp, idx, dim)
- *
- */
- __pyx_t_1 = 0;
- if (likely(PyList_CheckExact(__pyx_v_index)) || PyTuple_CheckExact(__pyx_v_index)) {
- __pyx_t_2 = __pyx_v_index; __Pyx_INCREF(__pyx_t_2); __pyx_t_3 = 0;
- __pyx_t_4 = NULL;
- } else {
- __pyx_t_3 = -1; __pyx_t_2 = PyObject_GetIter(__pyx_v_index); if (unlikely(!__pyx_t_2)) __PYX_ERR(1, 397, __pyx_L1_error)
- __Pyx_GOTREF(__pyx_t_2);
- __pyx_t_4 = Py_TYPE(__pyx_t_2)->tp_iternext; if (unlikely(!__pyx_t_4)) __PYX_ERR(1, 397, __pyx_L1_error)
- }
- for (;;) {
- if (likely(!__pyx_t_4)) {
- if (likely(PyList_CheckExact(__pyx_t_2))) {
- if (__pyx_t_3 >= PyList_GET_SIZE(__pyx_t_2)) break;
- #if CYTHON_ASSUME_SAFE_MACROS && !CYTHON_AVOID_BORROWED_REFS
- __pyx_t_5 = PyList_GET_ITEM(__pyx_t_2, __pyx_t_3); __Pyx_INCREF(__pyx_t_5); __pyx_t_3++; if (unlikely(0 < 0)) __PYX_ERR(1, 397, __pyx_L1_error)
- #else
- __pyx_t_5 = PySequence_ITEM(__pyx_t_2, __pyx_t_3); __pyx_t_3++; if (unlikely(!__pyx_t_5)) __PYX_ERR(1, 397, __pyx_L1_error)
- __Pyx_GOTREF(__pyx_t_5);
- #endif
- } else {
- if (__pyx_t_3 >= PyTuple_GET_SIZE(__pyx_t_2)) break;
- #if CYTHON_ASSUME_SAFE_MACROS && !CYTHON_AVOID_BORROWED_REFS
- __pyx_t_5 = PyTuple_GET_ITEM(__pyx_t_2, __pyx_t_3); __Pyx_INCREF(__pyx_t_5); __pyx_t_3++; if (unlikely(0 < 0)) __PYX_ERR(1, 397, __pyx_L1_error)
- #else
- __pyx_t_5 = PySequence_ITEM(__pyx_t_2, __pyx_t_3); __pyx_t_3++; if (unlikely(!__pyx_t_5)) __PYX_ERR(1, 397, __pyx_L1_error)
- __Pyx_GOTREF(__pyx_t_5);
- #endif
- }
- } else {
- __pyx_t_5 = __pyx_t_4(__pyx_t_2);
- if (unlikely(!__pyx_t_5)) {
- PyObject* exc_type = PyErr_Occurred();
- if (exc_type) {
- if (likely(__Pyx_PyErr_GivenExceptionMatches(exc_type, PyExc_StopIteration))) PyErr_Clear();
- else __PYX_ERR(1, 397, __pyx_L1_error)
- }
- break;
- }
- __Pyx_GOTREF(__pyx_t_5);
- }
- __Pyx_XDECREF_SET(__pyx_v_idx, __pyx_t_5);
- __pyx_t_5 = 0;
- __pyx_v_dim = __pyx_t_1;
- __pyx_t_1 = (__pyx_t_1 + 1);
-
- /* "View.MemoryView":398
- *
- * for dim, idx in enumerate(index):
- * itemp = pybuffer_index(&self.view, itemp, idx, dim) # <<<<<<<<<<<<<<
- *
- * return itemp
- */
- __pyx_t_6 = __Pyx_PyIndex_AsSsize_t(__pyx_v_idx); if (unlikely((__pyx_t_6 == (Py_ssize_t)-1) && PyErr_Occurred())) __PYX_ERR(1, 398, __pyx_L1_error)
- __pyx_t_7 = __pyx_pybuffer_index((&__pyx_v_self->view), __pyx_v_itemp, __pyx_t_6, __pyx_v_dim); if (unlikely(__pyx_t_7 == ((char *)NULL))) __PYX_ERR(1, 398, __pyx_L1_error)
- __pyx_v_itemp = __pyx_t_7;
-
- /* "View.MemoryView":397
- * cdef char *itemp = self.view.buf
- *
- * for dim, idx in enumerate(index): # <<<<<<<<<<<<<<
- * itemp = pybuffer_index(&self.view, itemp, idx, dim)
- *
- */
- }
- __Pyx_DECREF(__pyx_t_2); __pyx_t_2 = 0;
-
- /* "View.MemoryView":400
- * itemp = pybuffer_index(&self.view, itemp, idx, dim)
- *
- * return itemp # <<<<<<<<<<<<<<
- *
- *
- */
- __pyx_r = __pyx_v_itemp;
- goto __pyx_L0;
-
- /* "View.MemoryView":393
- * PyThread_free_lock(self.lock)
- *
- * cdef char *get_item_pointer(memoryview self, object index) except NULL: # <<<<<<<<<<<<<<
- * cdef Py_ssize_t dim
- * cdef char *itemp = self.view.buf
- */
-
- /* function exit code */
- __pyx_L1_error:;
- __Pyx_XDECREF(__pyx_t_2);
- __Pyx_XDECREF(__pyx_t_5);
- __Pyx_AddTraceback("View.MemoryView.memoryview.get_item_pointer", __pyx_clineno, __pyx_lineno, __pyx_filename);
- __pyx_r = NULL;
- __pyx_L0:;
- __Pyx_XDECREF(__pyx_v_idx);
- __Pyx_RefNannyFinishContext();
- return __pyx_r;
-}
-
-/* "View.MemoryView":403
- *
- *
- * def __getitem__(memoryview self, object index): # <<<<<<<<<<<<<<
- * if index is Ellipsis:
- * return self
- */
-
-/* Python wrapper */
-static PyObject *__pyx_memoryview___getitem__(PyObject *__pyx_v_self, PyObject *__pyx_v_index); /*proto*/
-static PyObject *__pyx_memoryview___getitem__(PyObject *__pyx_v_self, PyObject *__pyx_v_index) {
- PyObject *__pyx_r = 0;
- __Pyx_RefNannyDeclarations
- __Pyx_RefNannySetupContext("__getitem__ (wrapper)", 0);
- __pyx_r = __pyx_memoryview___pyx_pf_15View_dot_MemoryView_10memoryview_4__getitem__(((struct __pyx_memoryview_obj *)__pyx_v_self), ((PyObject *)__pyx_v_index));
-
- /* function exit code */
- __Pyx_RefNannyFinishContext();
- return __pyx_r;
-}
-
-static PyObject *__pyx_memoryview___pyx_pf_15View_dot_MemoryView_10memoryview_4__getitem__(struct __pyx_memoryview_obj *__pyx_v_self, PyObject *__pyx_v_index) {
- PyObject *__pyx_v_have_slices = NULL;
- PyObject *__pyx_v_indices = NULL;
- char *__pyx_v_itemp;
- PyObject *__pyx_r = NULL;
- __Pyx_RefNannyDeclarations
- int __pyx_t_1;
- int __pyx_t_2;
- PyObject *__pyx_t_3 = NULL;
- PyObject *__pyx_t_4 = NULL;
- PyObject *__pyx_t_5 = NULL;
- char *__pyx_t_6;
- int __pyx_lineno = 0;
- const char *__pyx_filename = NULL;
- int __pyx_clineno = 0;
- __Pyx_RefNannySetupContext("__getitem__", 0);
-
- /* "View.MemoryView":404
- *
- * def __getitem__(memoryview self, object index):
- * if index is Ellipsis: # <<<<<<<<<<<<<<
- * return self
- *
- */
- __pyx_t_1 = (__pyx_v_index == __pyx_builtin_Ellipsis);
- __pyx_t_2 = (__pyx_t_1 != 0);
- if (__pyx_t_2) {
-
- /* "View.MemoryView":405
- * def __getitem__(memoryview self, object index):
- * if index is Ellipsis:
- * return self # <<<<<<<<<<<<<<
- *
- * have_slices, indices = _unellipsify(index, self.view.ndim)
- */
- __Pyx_XDECREF(__pyx_r);
- __Pyx_INCREF(((PyObject *)__pyx_v_self));
- __pyx_r = ((PyObject *)__pyx_v_self);
- goto __pyx_L0;
-
- /* "View.MemoryView":404
- *
- * def __getitem__(memoryview self, object index):
- * if index is Ellipsis: # <<<<<<<<<<<<<<
- * return self
- *
- */
- }
-
- /* "View.MemoryView":407
- * return self
- *
- * have_slices, indices = _unellipsify(index, self.view.ndim) # <<<<<<<<<<<<<<
- *
- * cdef char *itemp
- */
- __pyx_t_3 = _unellipsify(__pyx_v_index, __pyx_v_self->view.ndim); if (unlikely(!__pyx_t_3)) __PYX_ERR(1, 407, __pyx_L1_error)
- __Pyx_GOTREF(__pyx_t_3);
- if (likely(__pyx_t_3 != Py_None)) {
- PyObject* sequence = __pyx_t_3;
- Py_ssize_t size = __Pyx_PySequence_SIZE(sequence);
- if (unlikely(size != 2)) {
- if (size > 2) __Pyx_RaiseTooManyValuesError(2);
- else if (size >= 0) __Pyx_RaiseNeedMoreValuesError(size);
- __PYX_ERR(1, 407, __pyx_L1_error)
- }
- #if CYTHON_ASSUME_SAFE_MACROS && !CYTHON_AVOID_BORROWED_REFS
- __pyx_t_4 = PyTuple_GET_ITEM(sequence, 0);
- __pyx_t_5 = PyTuple_GET_ITEM(sequence, 1);
- __Pyx_INCREF(__pyx_t_4);
- __Pyx_INCREF(__pyx_t_5);
- #else
- __pyx_t_4 = PySequence_ITEM(sequence, 0); if (unlikely(!__pyx_t_4)) __PYX_ERR(1, 407, __pyx_L1_error)
- __Pyx_GOTREF(__pyx_t_4);
- __pyx_t_5 = PySequence_ITEM(sequence, 1); if (unlikely(!__pyx_t_5)) __PYX_ERR(1, 407, __pyx_L1_error)
- __Pyx_GOTREF(__pyx_t_5);
- #endif
- __Pyx_DECREF(__pyx_t_3); __pyx_t_3 = 0;
- } else {
- __Pyx_RaiseNoneNotIterableError(); __PYX_ERR(1, 407, __pyx_L1_error)
- }
- __pyx_v_have_slices = __pyx_t_4;
- __pyx_t_4 = 0;
- __pyx_v_indices = __pyx_t_5;
- __pyx_t_5 = 0;
-
- /* "View.MemoryView":410
- *
- * cdef char *itemp
- * if have_slices: # <<<<<<<<<<<<<<
- * return memview_slice(self, indices)
- * else:
- */
- __pyx_t_2 = __Pyx_PyObject_IsTrue(__pyx_v_have_slices); if (unlikely(__pyx_t_2 < 0)) __PYX_ERR(1, 410, __pyx_L1_error)
- if (__pyx_t_2) {
-
- /* "View.MemoryView":411
- * cdef char *itemp
- * if have_slices:
- * return memview_slice(self, indices) # <<<<<<<<<<<<<<
- * else:
- * itemp = self.get_item_pointer(indices)
- */
- __Pyx_XDECREF(__pyx_r);
- __pyx_t_3 = ((PyObject *)__pyx_memview_slice(__pyx_v_self, __pyx_v_indices)); if (unlikely(!__pyx_t_3)) __PYX_ERR(1, 411, __pyx_L1_error)
- __Pyx_GOTREF(__pyx_t_3);
- __pyx_r = __pyx_t_3;
- __pyx_t_3 = 0;
- goto __pyx_L0;
-
- /* "View.MemoryView":410
- *
- * cdef char *itemp
- * if have_slices: # <<<<<<<<<<<<<<
- * return memview_slice(self, indices)
- * else:
- */
- }
-
- /* "View.MemoryView":413
- * return memview_slice(self, indices)
- * else:
- * itemp = self.get_item_pointer(indices) # <<<<<<<<<<<<<<
- * return self.convert_item_to_object(itemp)
- *
- */
- /*else*/ {
- __pyx_t_6 = ((struct __pyx_vtabstruct_memoryview *)__pyx_v_self->__pyx_vtab)->get_item_pointer(__pyx_v_self, __pyx_v_indices); if (unlikely(__pyx_t_6 == ((char *)NULL))) __PYX_ERR(1, 413, __pyx_L1_error)
- __pyx_v_itemp = __pyx_t_6;
-
- /* "View.MemoryView":414
- * else:
- * itemp = self.get_item_pointer(indices)
- * return self.convert_item_to_object(itemp) # <<<<<<<<<<<<<<
- *
- * def __setitem__(memoryview self, object index, object value):
- */
- __Pyx_XDECREF(__pyx_r);
- __pyx_t_3 = ((struct __pyx_vtabstruct_memoryview *)__pyx_v_self->__pyx_vtab)->convert_item_to_object(__pyx_v_self, __pyx_v_itemp); if (unlikely(!__pyx_t_3)) __PYX_ERR(1, 414, __pyx_L1_error)
- __Pyx_GOTREF(__pyx_t_3);
- __pyx_r = __pyx_t_3;
- __pyx_t_3 = 0;
- goto __pyx_L0;
- }
-
- /* "View.MemoryView":403
- *
- *
- * def __getitem__(memoryview self, object index): # <<<<<<<<<<<<<<
- * if index is Ellipsis:
- * return self
- */
-
- /* function exit code */
- __pyx_L1_error:;
- __Pyx_XDECREF(__pyx_t_3);
- __Pyx_XDECREF(__pyx_t_4);
- __Pyx_XDECREF(__pyx_t_5);
- __Pyx_AddTraceback("View.MemoryView.memoryview.__getitem__", __pyx_clineno, __pyx_lineno, __pyx_filename);
- __pyx_r = NULL;
- __pyx_L0:;
- __Pyx_XDECREF(__pyx_v_have_slices);
- __Pyx_XDECREF(__pyx_v_indices);
- __Pyx_XGIVEREF(__pyx_r);
- __Pyx_RefNannyFinishContext();
- return __pyx_r;
-}
-
-/* "View.MemoryView":416
- * return self.convert_item_to_object(itemp)
- *
- * def __setitem__(memoryview self, object index, object value): # <<<<<<<<<<<<<<
- * if self.view.readonly:
- * raise TypeError("Cannot assign to read-only memoryview")
- */
-
-/* Python wrapper */
-static int __pyx_memoryview___setitem__(PyObject *__pyx_v_self, PyObject *__pyx_v_index, PyObject *__pyx_v_value); /*proto*/
-static int __pyx_memoryview___setitem__(PyObject *__pyx_v_self, PyObject *__pyx_v_index, PyObject *__pyx_v_value) {
- int __pyx_r;
- __Pyx_RefNannyDeclarations
- __Pyx_RefNannySetupContext("__setitem__ (wrapper)", 0);
- __pyx_r = __pyx_memoryview___pyx_pf_15View_dot_MemoryView_10memoryview_6__setitem__(((struct __pyx_memoryview_obj *)__pyx_v_self), ((PyObject *)__pyx_v_index), ((PyObject *)__pyx_v_value));
-
- /* function exit code */
- __Pyx_RefNannyFinishContext();
- return __pyx_r;
-}
-
-static int __pyx_memoryview___pyx_pf_15View_dot_MemoryView_10memoryview_6__setitem__(struct __pyx_memoryview_obj *__pyx_v_self, PyObject *__pyx_v_index, PyObject *__pyx_v_value) {
- PyObject *__pyx_v_have_slices = NULL;
- PyObject *__pyx_v_obj = NULL;
- int __pyx_r;
- __Pyx_RefNannyDeclarations
- int __pyx_t_1;
- PyObject *__pyx_t_2 = NULL;
- PyObject *__pyx_t_3 = NULL;
- PyObject *__pyx_t_4 = NULL;
- int __pyx_lineno = 0;
- const char *__pyx_filename = NULL;
- int __pyx_clineno = 0;
- __Pyx_RefNannySetupContext("__setitem__", 0);
- __Pyx_INCREF(__pyx_v_index);
-
- /* "View.MemoryView":417
- *
- * def __setitem__(memoryview self, object index, object value):
- * if self.view.readonly: # <<<<<<<<<<<<<<
- * raise TypeError("Cannot assign to read-only memoryview")
- *
- */
- __pyx_t_1 = (__pyx_v_self->view.readonly != 0);
- if (unlikely(__pyx_t_1)) {
-
- /* "View.MemoryView":418
- * def __setitem__(memoryview self, object index, object value):
- * if self.view.readonly:
- * raise TypeError("Cannot assign to read-only memoryview") # <<<<<<<<<<<<<<
- *
- * have_slices, index = _unellipsify(index, self.view.ndim)
- */
- __pyx_t_2 = __Pyx_PyObject_Call(__pyx_builtin_TypeError, __pyx_tuple__9, NULL); if (unlikely(!__pyx_t_2)) __PYX_ERR(1, 418, __pyx_L1_error)
- __Pyx_GOTREF(__pyx_t_2);
- __Pyx_Raise(__pyx_t_2, 0, 0, 0);
- __Pyx_DECREF(__pyx_t_2); __pyx_t_2 = 0;
- __PYX_ERR(1, 418, __pyx_L1_error)
-
- /* "View.MemoryView":417
- *
- * def __setitem__(memoryview self, object index, object value):
- * if self.view.readonly: # <<<<<<<<<<<<<<
- * raise TypeError("Cannot assign to read-only memoryview")
- *
- */
- }
-
- /* "View.MemoryView":420
- * raise TypeError("Cannot assign to read-only memoryview")
- *
- * have_slices, index = _unellipsify(index, self.view.ndim) # <<<<<<<<<<<<<<
- *
- * if have_slices:
- */
- __pyx_t_2 = _unellipsify(__pyx_v_index, __pyx_v_self->view.ndim); if (unlikely(!__pyx_t_2)) __PYX_ERR(1, 420, __pyx_L1_error)
- __Pyx_GOTREF(__pyx_t_2);
- if (likely(__pyx_t_2 != Py_None)) {
- PyObject* sequence = __pyx_t_2;
- Py_ssize_t size = __Pyx_PySequence_SIZE(sequence);
- if (unlikely(size != 2)) {
- if (size > 2) __Pyx_RaiseTooManyValuesError(2);
- else if (size >= 0) __Pyx_RaiseNeedMoreValuesError(size);
- __PYX_ERR(1, 420, __pyx_L1_error)
- }
- #if CYTHON_ASSUME_SAFE_MACROS && !CYTHON_AVOID_BORROWED_REFS
- __pyx_t_3 = PyTuple_GET_ITEM(sequence, 0);
- __pyx_t_4 = PyTuple_GET_ITEM(sequence, 1);
- __Pyx_INCREF(__pyx_t_3);
- __Pyx_INCREF(__pyx_t_4);
- #else
- __pyx_t_3 = PySequence_ITEM(sequence, 0); if (unlikely(!__pyx_t_3)) __PYX_ERR(1, 420, __pyx_L1_error)
- __Pyx_GOTREF(__pyx_t_3);
- __pyx_t_4 = PySequence_ITEM(sequence, 1); if (unlikely(!__pyx_t_4)) __PYX_ERR(1, 420, __pyx_L1_error)
- __Pyx_GOTREF(__pyx_t_4);
- #endif
- __Pyx_DECREF(__pyx_t_2); __pyx_t_2 = 0;
- } else {
- __Pyx_RaiseNoneNotIterableError(); __PYX_ERR(1, 420, __pyx_L1_error)
- }
- __pyx_v_have_slices = __pyx_t_3;
- __pyx_t_3 = 0;
- __Pyx_DECREF_SET(__pyx_v_index, __pyx_t_4);
- __pyx_t_4 = 0;
-
- /* "View.MemoryView":422
- * have_slices, index = _unellipsify(index, self.view.ndim)
- *
- * if have_slices: # <<<<<<<<<<<<<<
- * obj = self.is_slice(value)
- * if obj:
- */
- __pyx_t_1 = __Pyx_PyObject_IsTrue(__pyx_v_have_slices); if (unlikely(__pyx_t_1 < 0)) __PYX_ERR(1, 422, __pyx_L1_error)
- if (__pyx_t_1) {
-
- /* "View.MemoryView":423
- *
- * if have_slices:
- * obj = self.is_slice(value) # <<<<<<<<<<<<<<
- * if obj:
- * self.setitem_slice_assignment(self[index], obj)
- */
- __pyx_t_2 = ((struct __pyx_vtabstruct_memoryview *)__pyx_v_self->__pyx_vtab)->is_slice(__pyx_v_self, __pyx_v_value); if (unlikely(!__pyx_t_2)) __PYX_ERR(1, 423, __pyx_L1_error)
- __Pyx_GOTREF(__pyx_t_2);
- __pyx_v_obj = __pyx_t_2;
- __pyx_t_2 = 0;
-
- /* "View.MemoryView":424
- * if have_slices:
- * obj = self.is_slice(value)
- * if obj: # <<<<<<<<<<<<<<
- * self.setitem_slice_assignment(self[index], obj)
- * else:
- */
- __pyx_t_1 = __Pyx_PyObject_IsTrue(__pyx_v_obj); if (unlikely(__pyx_t_1 < 0)) __PYX_ERR(1, 424, __pyx_L1_error)
- if (__pyx_t_1) {
-
- /* "View.MemoryView":425
- * obj = self.is_slice(value)
- * if obj:
- * self.setitem_slice_assignment(self[index], obj) # <<<<<<<<<<<<<<
- * else:
- * self.setitem_slice_assign_scalar(self[index], value)
- */
- __pyx_t_2 = __Pyx_PyObject_GetItem(((PyObject *)__pyx_v_self), __pyx_v_index); if (unlikely(!__pyx_t_2)) __PYX_ERR(1, 425, __pyx_L1_error)
- __Pyx_GOTREF(__pyx_t_2);
- __pyx_t_4 = ((struct __pyx_vtabstruct_memoryview *)__pyx_v_self->__pyx_vtab)->setitem_slice_assignment(__pyx_v_self, __pyx_t_2, __pyx_v_obj); if (unlikely(!__pyx_t_4)) __PYX_ERR(1, 425, __pyx_L1_error)
- __Pyx_GOTREF(__pyx_t_4);
- __Pyx_DECREF(__pyx_t_2); __pyx_t_2 = 0;
- __Pyx_DECREF(__pyx_t_4); __pyx_t_4 = 0;
-
- /* "View.MemoryView":424
- * if have_slices:
- * obj = self.is_slice(value)
- * if obj: # <<<<<<<<<<<<<<
- * self.setitem_slice_assignment(self[index], obj)
- * else:
- */
- goto __pyx_L5;
- }
-
- /* "View.MemoryView":427
- * self.setitem_slice_assignment(self[index], obj)
- * else:
- * self.setitem_slice_assign_scalar(self[index], value) # <<<<<<<<<<<<<<
- * else:
- * self.setitem_indexed(index, value)
- */
- /*else*/ {
- __pyx_t_4 = __Pyx_PyObject_GetItem(((PyObject *)__pyx_v_self), __pyx_v_index); if (unlikely(!__pyx_t_4)) __PYX_ERR(1, 427, __pyx_L1_error)
- __Pyx_GOTREF(__pyx_t_4);
- if (!(likely(((__pyx_t_4) == Py_None) || likely(__Pyx_TypeTest(__pyx_t_4, __pyx_memoryview_type))))) __PYX_ERR(1, 427, __pyx_L1_error)
- __pyx_t_2 = ((struct __pyx_vtabstruct_memoryview *)__pyx_v_self->__pyx_vtab)->setitem_slice_assign_scalar(__pyx_v_self, ((struct __pyx_memoryview_obj *)__pyx_t_4), __pyx_v_value); if (unlikely(!__pyx_t_2)) __PYX_ERR(1, 427, __pyx_L1_error)
- __Pyx_GOTREF(__pyx_t_2);
- __Pyx_DECREF(__pyx_t_4); __pyx_t_4 = 0;
- __Pyx_DECREF(__pyx_t_2); __pyx_t_2 = 0;
- }
- __pyx_L5:;
-
- /* "View.MemoryView":422
- * have_slices, index = _unellipsify(index, self.view.ndim)
- *
- * if have_slices: # <<<<<<<<<<<<<<
- * obj = self.is_slice(value)
- * if obj:
- */
- goto __pyx_L4;
- }
-
- /* "View.MemoryView":429
- * self.setitem_slice_assign_scalar(self[index], value)
- * else:
- * self.setitem_indexed(index, value) # <<<<<<<<<<<<<<
- *
- * cdef is_slice(self, obj):
- */
- /*else*/ {
- __pyx_t_2 = ((struct __pyx_vtabstruct_memoryview *)__pyx_v_self->__pyx_vtab)->setitem_indexed(__pyx_v_self, __pyx_v_index, __pyx_v_value); if (unlikely(!__pyx_t_2)) __PYX_ERR(1, 429, __pyx_L1_error)
- __Pyx_GOTREF(__pyx_t_2);
- __Pyx_DECREF(__pyx_t_2); __pyx_t_2 = 0;
- }
- __pyx_L4:;
-
- /* "View.MemoryView":416
- * return self.convert_item_to_object(itemp)
- *
- * def __setitem__(memoryview self, object index, object value): # <<<<<<<<<<<<<<
- * if self.view.readonly:
- * raise TypeError("Cannot assign to read-only memoryview")
- */
-
- /* function exit code */
- __pyx_r = 0;
- goto __pyx_L0;
- __pyx_L1_error:;
- __Pyx_XDECREF(__pyx_t_2);
- __Pyx_XDECREF(__pyx_t_3);
- __Pyx_XDECREF(__pyx_t_4);
- __Pyx_AddTraceback("View.MemoryView.memoryview.__setitem__", __pyx_clineno, __pyx_lineno, __pyx_filename);
- __pyx_r = -1;
- __pyx_L0:;
- __Pyx_XDECREF(__pyx_v_have_slices);
- __Pyx_XDECREF(__pyx_v_obj);
- __Pyx_XDECREF(__pyx_v_index);
- __Pyx_RefNannyFinishContext();
- return __pyx_r;
-}
-
-/* "View.MemoryView":431
- * self.setitem_indexed(index, value)
- *
- * cdef is_slice(self, obj): # <<<<<<<<<<<<<<
- * if not isinstance(obj, memoryview):
- * try:
- */
-
-static PyObject *__pyx_memoryview_is_slice(struct __pyx_memoryview_obj *__pyx_v_self, PyObject *__pyx_v_obj) {
- PyObject *__pyx_r = NULL;
- __Pyx_RefNannyDeclarations
- int __pyx_t_1;
- int __pyx_t_2;
- PyObject *__pyx_t_3 = NULL;
- PyObject *__pyx_t_4 = NULL;
- PyObject *__pyx_t_5 = NULL;
- PyObject *__pyx_t_6 = NULL;
- PyObject *__pyx_t_7 = NULL;
- PyObject *__pyx_t_8 = NULL;
- int __pyx_t_9;
- int __pyx_lineno = 0;
- const char *__pyx_filename = NULL;
- int __pyx_clineno = 0;
- __Pyx_RefNannySetupContext("is_slice", 0);
- __Pyx_INCREF(__pyx_v_obj);
-
- /* "View.MemoryView":432
- *
- * cdef is_slice(self, obj):
- * if not isinstance(obj, memoryview): # <<<<<<<<<<<<<<
- * try:
- * obj = memoryview(obj, self.flags & ~PyBUF_WRITABLE | PyBUF_ANY_CONTIGUOUS,
- */
- __pyx_t_1 = __Pyx_TypeCheck(__pyx_v_obj, __pyx_memoryview_type);
- __pyx_t_2 = ((!(__pyx_t_1 != 0)) != 0);
- if (__pyx_t_2) {
-
- /* "View.MemoryView":433
- * cdef is_slice(self, obj):
- * if not isinstance(obj, memoryview):
- * try: # <<<<<<<<<<<<<<
- * obj = memoryview(obj, self.flags & ~PyBUF_WRITABLE | PyBUF_ANY_CONTIGUOUS,
- * self.dtype_is_object)
- */
- {
- __Pyx_PyThreadState_declare
- __Pyx_PyThreadState_assign
- __Pyx_ExceptionSave(&__pyx_t_3, &__pyx_t_4, &__pyx_t_5);
- __Pyx_XGOTREF(__pyx_t_3);
- __Pyx_XGOTREF(__pyx_t_4);
- __Pyx_XGOTREF(__pyx_t_5);
- /*try:*/ {
-
- /* "View.MemoryView":434
- * if not isinstance(obj, memoryview):
- * try:
- * obj = memoryview(obj, self.flags & ~PyBUF_WRITABLE | PyBUF_ANY_CONTIGUOUS, # <<<<<<<<<<<<<<
- * self.dtype_is_object)
- * except TypeError:
- */
- __pyx_t_6 = __Pyx_PyInt_From_int(((__pyx_v_self->flags & (~PyBUF_WRITABLE)) | PyBUF_ANY_CONTIGUOUS)); if (unlikely(!__pyx_t_6)) __PYX_ERR(1, 434, __pyx_L4_error)
- __Pyx_GOTREF(__pyx_t_6);
-
- /* "View.MemoryView":435
- * try:
- * obj = memoryview(obj, self.flags & ~PyBUF_WRITABLE | PyBUF_ANY_CONTIGUOUS,
- * self.dtype_is_object) # <<<<<<<<<<<<<<
- * except TypeError:
- * return None
- */
- __pyx_t_7 = __Pyx_PyBool_FromLong(__pyx_v_self->dtype_is_object); if (unlikely(!__pyx_t_7)) __PYX_ERR(1, 435, __pyx_L4_error)
- __Pyx_GOTREF(__pyx_t_7);
-
- /* "View.MemoryView":434
- * if not isinstance(obj, memoryview):
- * try:
- * obj = memoryview(obj, self.flags & ~PyBUF_WRITABLE | PyBUF_ANY_CONTIGUOUS, # <<<<<<<<<<<<<<
- * self.dtype_is_object)
- * except TypeError:
- */
- __pyx_t_8 = PyTuple_New(3); if (unlikely(!__pyx_t_8)) __PYX_ERR(1, 434, __pyx_L4_error)
- __Pyx_GOTREF(__pyx_t_8);
- __Pyx_INCREF(__pyx_v_obj);
- __Pyx_GIVEREF(__pyx_v_obj);
- PyTuple_SET_ITEM(__pyx_t_8, 0, __pyx_v_obj);
- __Pyx_GIVEREF(__pyx_t_6);
- PyTuple_SET_ITEM(__pyx_t_8, 1, __pyx_t_6);
- __Pyx_GIVEREF(__pyx_t_7);
- PyTuple_SET_ITEM(__pyx_t_8, 2, __pyx_t_7);
- __pyx_t_6 = 0;
- __pyx_t_7 = 0;
- __pyx_t_7 = __Pyx_PyObject_Call(((PyObject *)__pyx_memoryview_type), __pyx_t_8, NULL); if (unlikely(!__pyx_t_7)) __PYX_ERR(1, 434, __pyx_L4_error)
- __Pyx_GOTREF(__pyx_t_7);
- __Pyx_DECREF(__pyx_t_8); __pyx_t_8 = 0;
- __Pyx_DECREF_SET(__pyx_v_obj, __pyx_t_7);
- __pyx_t_7 = 0;
-
- /* "View.MemoryView":433
- * cdef is_slice(self, obj):
- * if not isinstance(obj, memoryview):
- * try: # <<<<<<<<<<<<<<
- * obj = memoryview(obj, self.flags & ~PyBUF_WRITABLE | PyBUF_ANY_CONTIGUOUS,
- * self.dtype_is_object)
- */
- }
- __Pyx_XDECREF(__pyx_t_3); __pyx_t_3 = 0;
- __Pyx_XDECREF(__pyx_t_4); __pyx_t_4 = 0;
- __Pyx_XDECREF(__pyx_t_5); __pyx_t_5 = 0;
- goto __pyx_L9_try_end;
- __pyx_L4_error:;
- __Pyx_XDECREF(__pyx_t_6); __pyx_t_6 = 0;
- __Pyx_XDECREF(__pyx_t_7); __pyx_t_7 = 0;
- __Pyx_XDECREF(__pyx_t_8); __pyx_t_8 = 0;
-
- /* "View.MemoryView":436
- * obj = memoryview(obj, self.flags & ~PyBUF_WRITABLE | PyBUF_ANY_CONTIGUOUS,
- * self.dtype_is_object)
- * except TypeError: # <<<<<<<<<<<<<<
- * return None
- *
- */
- __pyx_t_9 = __Pyx_PyErr_ExceptionMatches(__pyx_builtin_TypeError);
- if (__pyx_t_9) {
- __Pyx_AddTraceback("View.MemoryView.memoryview.is_slice", __pyx_clineno, __pyx_lineno, __pyx_filename);
- if (__Pyx_GetException(&__pyx_t_7, &__pyx_t_8, &__pyx_t_6) < 0) __PYX_ERR(1, 436, __pyx_L6_except_error)
- __Pyx_GOTREF(__pyx_t_7);
- __Pyx_GOTREF(__pyx_t_8);
- __Pyx_GOTREF(__pyx_t_6);
-
- /* "View.MemoryView":437
- * self.dtype_is_object)
- * except TypeError:
- * return None # <<<<<<<<<<<<<<
- *
- * return obj
- */
- __Pyx_XDECREF(__pyx_r);
- __pyx_r = Py_None; __Pyx_INCREF(Py_None);
- __Pyx_DECREF(__pyx_t_6); __pyx_t_6 = 0;
- __Pyx_DECREF(__pyx_t_7); __pyx_t_7 = 0;
- __Pyx_DECREF(__pyx_t_8); __pyx_t_8 = 0;
- goto __pyx_L7_except_return;
- }
- goto __pyx_L6_except_error;
- __pyx_L6_except_error:;
-
- /* "View.MemoryView":433
- * cdef is_slice(self, obj):
- * if not isinstance(obj, memoryview):
- * try: # <<<<<<<<<<<<<<
- * obj = memoryview(obj, self.flags & ~PyBUF_WRITABLE | PyBUF_ANY_CONTIGUOUS,
- * self.dtype_is_object)
- */
- __Pyx_XGIVEREF(__pyx_t_3);
- __Pyx_XGIVEREF(__pyx_t_4);
- __Pyx_XGIVEREF(__pyx_t_5);
- __Pyx_ExceptionReset(__pyx_t_3, __pyx_t_4, __pyx_t_5);
- goto __pyx_L1_error;
- __pyx_L7_except_return:;
- __Pyx_XGIVEREF(__pyx_t_3);
- __Pyx_XGIVEREF(__pyx_t_4);
- __Pyx_XGIVEREF(__pyx_t_5);
- __Pyx_ExceptionReset(__pyx_t_3, __pyx_t_4, __pyx_t_5);
- goto __pyx_L0;
- __pyx_L9_try_end:;
- }
-
- /* "View.MemoryView":432
- *
- * cdef is_slice(self, obj):
- * if not isinstance(obj, memoryview): # <<<<<<<<<<<<<<
- * try:
- * obj = memoryview(obj, self.flags & ~PyBUF_WRITABLE | PyBUF_ANY_CONTIGUOUS,
- */
- }
-
- /* "View.MemoryView":439
- * return None
- *
- * return obj # <<<<<<<<<<<<<<
- *
- * cdef setitem_slice_assignment(self, dst, src):
- */
- __Pyx_XDECREF(__pyx_r);
- __Pyx_INCREF(__pyx_v_obj);
- __pyx_r = __pyx_v_obj;
- goto __pyx_L0;
-
- /* "View.MemoryView":431
- * self.setitem_indexed(index, value)
- *
- * cdef is_slice(self, obj): # <<<<<<<<<<<<<<
- * if not isinstance(obj, memoryview):
- * try:
- */
-
- /* function exit code */
- __pyx_L1_error:;
- __Pyx_XDECREF(__pyx_t_6);
- __Pyx_XDECREF(__pyx_t_7);
- __Pyx_XDECREF(__pyx_t_8);
- __Pyx_AddTraceback("View.MemoryView.memoryview.is_slice", __pyx_clineno, __pyx_lineno, __pyx_filename);
- __pyx_r = 0;
- __pyx_L0:;
- __Pyx_XDECREF(__pyx_v_obj);
- __Pyx_XGIVEREF(__pyx_r);
- __Pyx_RefNannyFinishContext();
- return __pyx_r;
-}
-
-/* "View.MemoryView":441
- * return obj
- *
- * cdef setitem_slice_assignment(self, dst, src): # <<<<<<<<<<<<<<
- * cdef __Pyx_memviewslice dst_slice
- * cdef __Pyx_memviewslice src_slice
- */
-
-static PyObject *__pyx_memoryview_setitem_slice_assignment(struct __pyx_memoryview_obj *__pyx_v_self, PyObject *__pyx_v_dst, PyObject *__pyx_v_src) {
- __Pyx_memviewslice __pyx_v_dst_slice;
- __Pyx_memviewslice __pyx_v_src_slice;
- PyObject *__pyx_r = NULL;
- __Pyx_RefNannyDeclarations
- __Pyx_memviewslice *__pyx_t_1;
- __Pyx_memviewslice *__pyx_t_2;
- PyObject *__pyx_t_3 = NULL;
- int __pyx_t_4;
- int __pyx_t_5;
- int __pyx_t_6;
- int __pyx_lineno = 0;
- const char *__pyx_filename = NULL;
- int __pyx_clineno = 0;
- __Pyx_RefNannySetupContext("setitem_slice_assignment", 0);
-
- /* "View.MemoryView":445
- * cdef __Pyx_memviewslice src_slice
- *
- * memoryview_copy_contents(get_slice_from_memview(src, &src_slice)[0], # <<<<<<<<<<<<<<
- * get_slice_from_memview(dst, &dst_slice)[0],
- * src.ndim, dst.ndim, self.dtype_is_object)
- */
- if (!(likely(((__pyx_v_src) == Py_None) || likely(__Pyx_TypeTest(__pyx_v_src, __pyx_memoryview_type))))) __PYX_ERR(1, 445, __pyx_L1_error)
- __pyx_t_1 = __pyx_memoryview_get_slice_from_memoryview(((struct __pyx_memoryview_obj *)__pyx_v_src), (&__pyx_v_src_slice)); if (unlikely(__pyx_t_1 == ((__Pyx_memviewslice *)NULL))) __PYX_ERR(1, 445, __pyx_L1_error)
-
- /* "View.MemoryView":446
- *
- * memoryview_copy_contents(get_slice_from_memview(src, &src_slice)[0],
- * get_slice_from_memview(dst, &dst_slice)[0], # <<<<<<<<<<<<<<
- * src.ndim, dst.ndim, self.dtype_is_object)
- *
- */
- if (!(likely(((__pyx_v_dst) == Py_None) || likely(__Pyx_TypeTest(__pyx_v_dst, __pyx_memoryview_type))))) __PYX_ERR(1, 446, __pyx_L1_error)
- __pyx_t_2 = __pyx_memoryview_get_slice_from_memoryview(((struct __pyx_memoryview_obj *)__pyx_v_dst), (&__pyx_v_dst_slice)); if (unlikely(__pyx_t_2 == ((__Pyx_memviewslice *)NULL))) __PYX_ERR(1, 446, __pyx_L1_error)
-
- /* "View.MemoryView":447
- * memoryview_copy_contents(get_slice_from_memview(src, &src_slice)[0],
- * get_slice_from_memview(dst, &dst_slice)[0],
- * src.ndim, dst.ndim, self.dtype_is_object) # <<<<<<<<<<<<<<
- *
- * cdef setitem_slice_assign_scalar(self, memoryview dst, value):
- */
- __pyx_t_3 = __Pyx_PyObject_GetAttrStr(__pyx_v_src, __pyx_n_s_ndim); if (unlikely(!__pyx_t_3)) __PYX_ERR(1, 447, __pyx_L1_error)
- __Pyx_GOTREF(__pyx_t_3);
- __pyx_t_4 = __Pyx_PyInt_As_int(__pyx_t_3); if (unlikely((__pyx_t_4 == (int)-1) && PyErr_Occurred())) __PYX_ERR(1, 447, __pyx_L1_error)
- __Pyx_DECREF(__pyx_t_3); __pyx_t_3 = 0;
- __pyx_t_3 = __Pyx_PyObject_GetAttrStr(__pyx_v_dst, __pyx_n_s_ndim); if (unlikely(!__pyx_t_3)) __PYX_ERR(1, 447, __pyx_L1_error)
- __Pyx_GOTREF(__pyx_t_3);
- __pyx_t_5 = __Pyx_PyInt_As_int(__pyx_t_3); if (unlikely((__pyx_t_5 == (int)-1) && PyErr_Occurred())) __PYX_ERR(1, 447, __pyx_L1_error)
- __Pyx_DECREF(__pyx_t_3); __pyx_t_3 = 0;
-
- /* "View.MemoryView":445
- * cdef __Pyx_memviewslice src_slice
- *
- * memoryview_copy_contents(get_slice_from_memview(src, &src_slice)[0], # <<<<<<<<<<<<<<
- * get_slice_from_memview(dst, &dst_slice)[0],
- * src.ndim, dst.ndim, self.dtype_is_object)
- */
- __pyx_t_6 = __pyx_memoryview_copy_contents((__pyx_t_1[0]), (__pyx_t_2[0]), __pyx_t_4, __pyx_t_5, __pyx_v_self->dtype_is_object); if (unlikely(__pyx_t_6 == ((int)-1))) __PYX_ERR(1, 445, __pyx_L1_error)
-
- /* "View.MemoryView":441
- * return obj
- *
- * cdef setitem_slice_assignment(self, dst, src): # <<<<<<<<<<<<<<
- * cdef __Pyx_memviewslice dst_slice
- * cdef __Pyx_memviewslice src_slice
- */
-
- /* function exit code */
- __pyx_r = Py_None; __Pyx_INCREF(Py_None);
- goto __pyx_L0;
- __pyx_L1_error:;
- __Pyx_XDECREF(__pyx_t_3);
- __Pyx_AddTraceback("View.MemoryView.memoryview.setitem_slice_assignment", __pyx_clineno, __pyx_lineno, __pyx_filename);
- __pyx_r = 0;
- __pyx_L0:;
- __Pyx_XGIVEREF(__pyx_r);
- __Pyx_RefNannyFinishContext();
- return __pyx_r;
-}
-
-/* "View.MemoryView":449
- * src.ndim, dst.ndim, self.dtype_is_object)
- *
- * cdef setitem_slice_assign_scalar(self, memoryview dst, value): # <<<<<<<<<<<<<<
- * cdef int array[128]
- * cdef void *tmp = NULL
- */
-
-static PyObject *__pyx_memoryview_setitem_slice_assign_scalar(struct __pyx_memoryview_obj *__pyx_v_self, struct __pyx_memoryview_obj *__pyx_v_dst, PyObject *__pyx_v_value) {
- int __pyx_v_array[0x80];
- void *__pyx_v_tmp;
- void *__pyx_v_item;
- __Pyx_memviewslice *__pyx_v_dst_slice;
- __Pyx_memviewslice __pyx_v_tmp_slice;
- PyObject *__pyx_r = NULL;
- __Pyx_RefNannyDeclarations
- __Pyx_memviewslice *__pyx_t_1;
- int __pyx_t_2;
- PyObject *__pyx_t_3 = NULL;
- int __pyx_t_4;
- int __pyx_t_5;
- char const *__pyx_t_6;
- PyObject *__pyx_t_7 = NULL;
- PyObject *__pyx_t_8 = NULL;
- PyObject *__pyx_t_9 = NULL;
- PyObject *__pyx_t_10 = NULL;
- PyObject *__pyx_t_11 = NULL;
- PyObject *__pyx_t_12 = NULL;
- int __pyx_lineno = 0;
- const char *__pyx_filename = NULL;
- int __pyx_clineno = 0;
- __Pyx_RefNannySetupContext("setitem_slice_assign_scalar", 0);
-
- /* "View.MemoryView":451
- * cdef setitem_slice_assign_scalar(self, memoryview dst, value):
- * cdef int array[128]
- * cdef void *tmp = NULL # <<<<<<<<<<<<<<
- * cdef void *item
- *
- */
- __pyx_v_tmp = NULL;
-
- /* "View.MemoryView":456
- * cdef __Pyx_memviewslice *dst_slice
- * cdef __Pyx_memviewslice tmp_slice
- * dst_slice = get_slice_from_memview(dst, &tmp_slice) # <<<<<<<<<<<<<<
- *
- * if self.view.itemsize > sizeof(array):
- */
- __pyx_t_1 = __pyx_memoryview_get_slice_from_memoryview(__pyx_v_dst, (&__pyx_v_tmp_slice)); if (unlikely(__pyx_t_1 == ((__Pyx_memviewslice *)NULL))) __PYX_ERR(1, 456, __pyx_L1_error)
- __pyx_v_dst_slice = __pyx_t_1;
-
- /* "View.MemoryView":458
- * dst_slice = get_slice_from_memview(dst, &tmp_slice)
- *
- * if self.view.itemsize > sizeof(array): # <<<<<<<<<<<<<<
- * tmp = PyMem_Malloc(self.view.itemsize)
- * if tmp == NULL:
- */
- __pyx_t_2 = ((((size_t)__pyx_v_self->view.itemsize) > (sizeof(__pyx_v_array))) != 0);
- if (__pyx_t_2) {
-
- /* "View.MemoryView":459
- *
- * if self.view.itemsize > sizeof(array):
- * tmp = PyMem_Malloc(self.view.itemsize) # <<<<<<<<<<<<<<
- * if tmp == NULL:
- * raise MemoryError
- */
- __pyx_v_tmp = PyMem_Malloc(__pyx_v_self->view.itemsize);
-
- /* "View.MemoryView":460
- * if self.view.itemsize > sizeof(array):
- * tmp = PyMem_Malloc(self.view.itemsize)
- * if tmp == NULL: # <<<<<<<<<<<<<<
- * raise MemoryError
- * item = tmp
- */
- __pyx_t_2 = ((__pyx_v_tmp == NULL) != 0);
- if (unlikely(__pyx_t_2)) {
-
- /* "View.MemoryView":461
- * tmp = PyMem_Malloc(self.view.itemsize)
- * if tmp == NULL:
- * raise MemoryError # <<<<<<<<<<<<<<
- * item = tmp
- * else:
- */
- PyErr_NoMemory(); __PYX_ERR(1, 461, __pyx_L1_error)
-
- /* "View.MemoryView":460
- * if self.view.itemsize > sizeof(array):
- * tmp = PyMem_Malloc(self.view.itemsize)
- * if tmp == NULL: # <<<<<<<<<<<<<<
- * raise MemoryError
- * item = tmp
- */
- }
-
- /* "View.MemoryView":462
- * if tmp == NULL:
- * raise MemoryError
- * item = tmp # <<<<<<<<<<<<<<
- * else:
- * item = array
- */
- __pyx_v_item = __pyx_v_tmp;
-
- /* "View.MemoryView":458
- * dst_slice = get_slice_from_memview(dst, &tmp_slice)
- *
- * if self.view.itemsize > sizeof(array): # <<<<<<<<<<<<<<
- * tmp = PyMem_Malloc(self.view.itemsize)
- * if tmp == NULL:
- */
- goto __pyx_L3;
- }
-
- /* "View.MemoryView":464
- * item = tmp
- * else:
- * item = array # <<<<<<<<<<<<<<
- *
- * try:
- */
- /*else*/ {
- __pyx_v_item = ((void *)__pyx_v_array);
- }
- __pyx_L3:;
-
- /* "View.MemoryView":466
- * item = array
- *
- * try: # <<<<<<<<<<<<<<
- * if self.dtype_is_object:
- * ( item)[0] = value
- */
- /*try:*/ {
-
- /* "View.MemoryView":467
- *
- * try:
- * if self.dtype_is_object: # <<<<<<<<<<<<<<
- * ( item)[0] = value
- * else:
- */
- __pyx_t_2 = (__pyx_v_self->dtype_is_object != 0);
- if (__pyx_t_2) {
-
- /* "View.MemoryView":468
- * try:
- * if self.dtype_is_object:
- * ( item)[0] = value # <<<<<<<<<<<<<<
- * else:
- * self.assign_item_from_object( item, value)
- */
- (((PyObject **)__pyx_v_item)[0]) = ((PyObject *)__pyx_v_value);
-
- /* "View.MemoryView":467
- *
- * try:
- * if self.dtype_is_object: # <<<<<<<<<<<<<<
- * ( item)[0] = value
- * else:
- */
- goto __pyx_L8;
- }
-
- /* "View.MemoryView":470
- * ( item)[0] = value
- * else:
- * self.assign_item_from_object( item, value) # <<<<<<<<<<<<<<
- *
- *
- */
- /*else*/ {
- __pyx_t_3 = ((struct __pyx_vtabstruct_memoryview *)__pyx_v_self->__pyx_vtab)->assign_item_from_object(__pyx_v_self, ((char *)__pyx_v_item), __pyx_v_value); if (unlikely(!__pyx_t_3)) __PYX_ERR(1, 470, __pyx_L6_error)
- __Pyx_GOTREF(__pyx_t_3);
- __Pyx_DECREF(__pyx_t_3); __pyx_t_3 = 0;
- }
- __pyx_L8:;
-
- /* "View.MemoryView":474
- *
- *
- * if self.view.suboffsets != NULL: # <<<<<<<<<<<<<<
- * assert_direct_dimensions(self.view.suboffsets, self.view.ndim)
- * slice_assign_scalar(dst_slice, dst.view.ndim, self.view.itemsize,
- */
- __pyx_t_2 = ((__pyx_v_self->view.suboffsets != NULL) != 0);
- if (__pyx_t_2) {
-
- /* "View.MemoryView":475
- *
- * if self.view.suboffsets != NULL:
- * assert_direct_dimensions(self.view.suboffsets, self.view.ndim) # <<<<<<<<<<<<<<
- * slice_assign_scalar(dst_slice, dst.view.ndim, self.view.itemsize,
- * item, self.dtype_is_object)
- */
- __pyx_t_3 = assert_direct_dimensions(__pyx_v_self->view.suboffsets, __pyx_v_self->view.ndim); if (unlikely(!__pyx_t_3)) __PYX_ERR(1, 475, __pyx_L6_error)
- __Pyx_GOTREF(__pyx_t_3);
- __Pyx_DECREF(__pyx_t_3); __pyx_t_3 = 0;
-
- /* "View.MemoryView":474
- *
- *
- * if self.view.suboffsets != NULL: # <<<<<<<<<<<<<<
- * assert_direct_dimensions(self.view.suboffsets, self.view.ndim)
- * slice_assign_scalar(dst_slice, dst.view.ndim, self.view.itemsize,
- */
- }
-
- /* "View.MemoryView":476
- * if self.view.suboffsets != NULL:
- * assert_direct_dimensions(self.view.suboffsets, self.view.ndim)
- * slice_assign_scalar(dst_slice, dst.view.ndim, self.view.itemsize, # <<<<<<<<<<<<<<
- * item, self.dtype_is_object)
- * finally:
- */
- __pyx_memoryview_slice_assign_scalar(__pyx_v_dst_slice, __pyx_v_dst->view.ndim, __pyx_v_self->view.itemsize, __pyx_v_item, __pyx_v_self->dtype_is_object);
- }
-
- /* "View.MemoryView":479
- * item, self.dtype_is_object)
- * finally:
- * PyMem_Free(tmp) # <<<<<<<<<<<<<<
- *
- * cdef setitem_indexed(self, index, value):
- */
- /*finally:*/ {
- /*normal exit:*/{
- PyMem_Free(__pyx_v_tmp);
- goto __pyx_L7;
- }
- __pyx_L6_error:;
- /*exception exit:*/{
- __Pyx_PyThreadState_declare
- __Pyx_PyThreadState_assign
- __pyx_t_7 = 0; __pyx_t_8 = 0; __pyx_t_9 = 0; __pyx_t_10 = 0; __pyx_t_11 = 0; __pyx_t_12 = 0;
- __Pyx_XDECREF(__pyx_t_3); __pyx_t_3 = 0;
- if (PY_MAJOR_VERSION >= 3) __Pyx_ExceptionSwap(&__pyx_t_10, &__pyx_t_11, &__pyx_t_12);
- if ((PY_MAJOR_VERSION < 3) || unlikely(__Pyx_GetException(&__pyx_t_7, &__pyx_t_8, &__pyx_t_9) < 0)) __Pyx_ErrFetch(&__pyx_t_7, &__pyx_t_8, &__pyx_t_9);
- __Pyx_XGOTREF(__pyx_t_7);
- __Pyx_XGOTREF(__pyx_t_8);
- __Pyx_XGOTREF(__pyx_t_9);
- __Pyx_XGOTREF(__pyx_t_10);
- __Pyx_XGOTREF(__pyx_t_11);
- __Pyx_XGOTREF(__pyx_t_12);
- __pyx_t_4 = __pyx_lineno; __pyx_t_5 = __pyx_clineno; __pyx_t_6 = __pyx_filename;
- {
- PyMem_Free(__pyx_v_tmp);
- }
- if (PY_MAJOR_VERSION >= 3) {
- __Pyx_XGIVEREF(__pyx_t_10);
- __Pyx_XGIVEREF(__pyx_t_11);
- __Pyx_XGIVEREF(__pyx_t_12);
- __Pyx_ExceptionReset(__pyx_t_10, __pyx_t_11, __pyx_t_12);
- }
- __Pyx_XGIVEREF(__pyx_t_7);
- __Pyx_XGIVEREF(__pyx_t_8);
- __Pyx_XGIVEREF(__pyx_t_9);
- __Pyx_ErrRestore(__pyx_t_7, __pyx_t_8, __pyx_t_9);
- __pyx_t_7 = 0; __pyx_t_8 = 0; __pyx_t_9 = 0; __pyx_t_10 = 0; __pyx_t_11 = 0; __pyx_t_12 = 0;
- __pyx_lineno = __pyx_t_4; __pyx_clineno = __pyx_t_5; __pyx_filename = __pyx_t_6;
- goto __pyx_L1_error;
- }
- __pyx_L7:;
- }
-
- /* "View.MemoryView":449
- * src.ndim, dst.ndim, self.dtype_is_object)
- *
- * cdef setitem_slice_assign_scalar(self, memoryview dst, value): # <<<<<<<<<<<<<<
- * cdef int array[128]
- * cdef void *tmp = NULL
- */
-
- /* function exit code */
- __pyx_r = Py_None; __Pyx_INCREF(Py_None);
- goto __pyx_L0;
- __pyx_L1_error:;
- __Pyx_XDECREF(__pyx_t_3);
- __Pyx_AddTraceback("View.MemoryView.memoryview.setitem_slice_assign_scalar", __pyx_clineno, __pyx_lineno, __pyx_filename);
- __pyx_r = 0;
- __pyx_L0:;
- __Pyx_XGIVEREF(__pyx_r);
- __Pyx_RefNannyFinishContext();
- return __pyx_r;
-}
-
-/* "View.MemoryView":481
- * PyMem_Free(tmp)
- *
- * cdef setitem_indexed(self, index, value): # <<<<<<<<<<<<<<
- * cdef char *itemp = self.get_item_pointer(index)
- * self.assign_item_from_object(itemp, value)
- */
-
-static PyObject *__pyx_memoryview_setitem_indexed(struct __pyx_memoryview_obj *__pyx_v_self, PyObject *__pyx_v_index, PyObject *__pyx_v_value) {
- char *__pyx_v_itemp;
- PyObject *__pyx_r = NULL;
- __Pyx_RefNannyDeclarations
- char *__pyx_t_1;
- PyObject *__pyx_t_2 = NULL;
- int __pyx_lineno = 0;
- const char *__pyx_filename = NULL;
- int __pyx_clineno = 0;
- __Pyx_RefNannySetupContext("setitem_indexed", 0);
-
- /* "View.MemoryView":482
- *
- * cdef setitem_indexed(self, index, value):
- * cdef char *itemp = self.get_item_pointer(index) # <<<<<<<<<<<<<<
- * self.assign_item_from_object(itemp, value)
- *
- */
- __pyx_t_1 = ((struct __pyx_vtabstruct_memoryview *)__pyx_v_self->__pyx_vtab)->get_item_pointer(__pyx_v_self, __pyx_v_index); if (unlikely(__pyx_t_1 == ((char *)NULL))) __PYX_ERR(1, 482, __pyx_L1_error)
- __pyx_v_itemp = __pyx_t_1;
-
- /* "View.MemoryView":483
- * cdef setitem_indexed(self, index, value):
- * cdef char *itemp = self.get_item_pointer(index)
- * self.assign_item_from_object(itemp, value) # <<<<<<<<<<<<<<
- *
- * cdef convert_item_to_object(self, char *itemp):
- */
- __pyx_t_2 = ((struct __pyx_vtabstruct_memoryview *)__pyx_v_self->__pyx_vtab)->assign_item_from_object(__pyx_v_self, __pyx_v_itemp, __pyx_v_value); if (unlikely(!__pyx_t_2)) __PYX_ERR(1, 483, __pyx_L1_error)
- __Pyx_GOTREF(__pyx_t_2);
- __Pyx_DECREF(__pyx_t_2); __pyx_t_2 = 0;
-
- /* "View.MemoryView":481
- * PyMem_Free(tmp)
- *
- * cdef setitem_indexed(self, index, value): # <<<<<<<<<<<<<<
- * cdef char *itemp = self.get_item_pointer(index)
- * self.assign_item_from_object(itemp, value)
- */
-
- /* function exit code */
- __pyx_r = Py_None; __Pyx_INCREF(Py_None);
- goto __pyx_L0;
- __pyx_L1_error:;
- __Pyx_XDECREF(__pyx_t_2);
- __Pyx_AddTraceback("View.MemoryView.memoryview.setitem_indexed", __pyx_clineno, __pyx_lineno, __pyx_filename);
- __pyx_r = 0;
- __pyx_L0:;
- __Pyx_XGIVEREF(__pyx_r);
- __Pyx_RefNannyFinishContext();
- return __pyx_r;
-}
-
-/* "View.MemoryView":485
- * self.assign_item_from_object(itemp, value)
- *
- * cdef convert_item_to_object(self, char *itemp): # <<<<<<<<<<<<<<
- * """Only used if instantiated manually by the user, or if Cython doesn't
- * know how to convert the type"""
- */
-
-static PyObject *__pyx_memoryview_convert_item_to_object(struct __pyx_memoryview_obj *__pyx_v_self, char *__pyx_v_itemp) {
- PyObject *__pyx_v_struct = NULL;
- PyObject *__pyx_v_bytesitem = 0;
- PyObject *__pyx_v_result = NULL;
- PyObject *__pyx_r = NULL;
- __Pyx_RefNannyDeclarations
- PyObject *__pyx_t_1 = NULL;
- PyObject *__pyx_t_2 = NULL;
- PyObject *__pyx_t_3 = NULL;
- PyObject *__pyx_t_4 = NULL;
- PyObject *__pyx_t_5 = NULL;
- PyObject *__pyx_t_6 = NULL;
- PyObject *__pyx_t_7 = NULL;
- int __pyx_t_8;
- PyObject *__pyx_t_9 = NULL;
- size_t __pyx_t_10;
- int __pyx_t_11;
- int __pyx_lineno = 0;
- const char *__pyx_filename = NULL;
- int __pyx_clineno = 0;
- __Pyx_RefNannySetupContext("convert_item_to_object", 0);
-
- /* "View.MemoryView":488
- * """Only used if instantiated manually by the user, or if Cython doesn't
- * know how to convert the type"""
- * import struct # <<<<<<<<<<<<<<
- * cdef bytes bytesitem
- *
- */
- __pyx_t_1 = __Pyx_Import(__pyx_n_s_struct, 0, 0); if (unlikely(!__pyx_t_1)) __PYX_ERR(1, 488, __pyx_L1_error)
- __Pyx_GOTREF(__pyx_t_1);
- __pyx_v_struct = __pyx_t_1;
- __pyx_t_1 = 0;
-
- /* "View.MemoryView":491
- * cdef bytes bytesitem
- *
- * bytesitem = itemp[:self.view.itemsize] # <<<<<<<<<<<<<<
- * try:
- * result = struct.unpack(self.view.format, bytesitem)
- */
- __pyx_t_1 = __Pyx_PyBytes_FromStringAndSize(__pyx_v_itemp + 0, __pyx_v_self->view.itemsize - 0); if (unlikely(!__pyx_t_1)) __PYX_ERR(1, 491, __pyx_L1_error)
- __Pyx_GOTREF(__pyx_t_1);
- __pyx_v_bytesitem = ((PyObject*)__pyx_t_1);
- __pyx_t_1 = 0;
-
- /* "View.MemoryView":492
- *
- * bytesitem = itemp[:self.view.itemsize]
- * try: # <<<<<<<<<<<<<<
- * result = struct.unpack(self.view.format, bytesitem)
- * except struct.error:
- */
- {
- __Pyx_PyThreadState_declare
- __Pyx_PyThreadState_assign
- __Pyx_ExceptionSave(&__pyx_t_2, &__pyx_t_3, &__pyx_t_4);
- __Pyx_XGOTREF(__pyx_t_2);
- __Pyx_XGOTREF(__pyx_t_3);
- __Pyx_XGOTREF(__pyx_t_4);
- /*try:*/ {
-
- /* "View.MemoryView":493
- * bytesitem = itemp[:self.view.itemsize]
- * try:
- * result = struct.unpack(self.view.format, bytesitem) # <<<<<<<<<<<<<<
- * except struct.error:
- * raise ValueError("Unable to convert item to object")
- */
- __pyx_t_5 = __Pyx_PyObject_GetAttrStr(__pyx_v_struct, __pyx_n_s_unpack); if (unlikely(!__pyx_t_5)) __PYX_ERR(1, 493, __pyx_L3_error)
- __Pyx_GOTREF(__pyx_t_5);
- __pyx_t_6 = __Pyx_PyBytes_FromString(__pyx_v_self->view.format); if (unlikely(!__pyx_t_6)) __PYX_ERR(1, 493, __pyx_L3_error)
- __Pyx_GOTREF(__pyx_t_6);
- __pyx_t_7 = NULL;
- __pyx_t_8 = 0;
- if (CYTHON_UNPACK_METHODS && likely(PyMethod_Check(__pyx_t_5))) {
- __pyx_t_7 = PyMethod_GET_SELF(__pyx_t_5);
- if (likely(__pyx_t_7)) {
- PyObject* function = PyMethod_GET_FUNCTION(__pyx_t_5);
- __Pyx_INCREF(__pyx_t_7);
- __Pyx_INCREF(function);
- __Pyx_DECREF_SET(__pyx_t_5, function);
- __pyx_t_8 = 1;
- }
- }
- #if CYTHON_FAST_PYCALL
- if (PyFunction_Check(__pyx_t_5)) {
- PyObject *__pyx_temp[3] = {__pyx_t_7, __pyx_t_6, __pyx_v_bytesitem};
- __pyx_t_1 = __Pyx_PyFunction_FastCall(__pyx_t_5, __pyx_temp+1-__pyx_t_8, 2+__pyx_t_8); if (unlikely(!__pyx_t_1)) __PYX_ERR(1, 493, __pyx_L3_error)
- __Pyx_XDECREF(__pyx_t_7); __pyx_t_7 = 0;
- __Pyx_GOTREF(__pyx_t_1);
- __Pyx_DECREF(__pyx_t_6); __pyx_t_6 = 0;
- } else
- #endif
- #if CYTHON_FAST_PYCCALL
- if (__Pyx_PyFastCFunction_Check(__pyx_t_5)) {
- PyObject *__pyx_temp[3] = {__pyx_t_7, __pyx_t_6, __pyx_v_bytesitem};
- __pyx_t_1 = __Pyx_PyCFunction_FastCall(__pyx_t_5, __pyx_temp+1-__pyx_t_8, 2+__pyx_t_8); if (unlikely(!__pyx_t_1)) __PYX_ERR(1, 493, __pyx_L3_error)
- __Pyx_XDECREF(__pyx_t_7); __pyx_t_7 = 0;
- __Pyx_GOTREF(__pyx_t_1);
- __Pyx_DECREF(__pyx_t_6); __pyx_t_6 = 0;
- } else
- #endif
- {
- __pyx_t_9 = PyTuple_New(2+__pyx_t_8); if (unlikely(!__pyx_t_9)) __PYX_ERR(1, 493, __pyx_L3_error)
- __Pyx_GOTREF(__pyx_t_9);
- if (__pyx_t_7) {
- __Pyx_GIVEREF(__pyx_t_7); PyTuple_SET_ITEM(__pyx_t_9, 0, __pyx_t_7); __pyx_t_7 = NULL;
- }
- __Pyx_GIVEREF(__pyx_t_6);
- PyTuple_SET_ITEM(__pyx_t_9, 0+__pyx_t_8, __pyx_t_6);
- __Pyx_INCREF(__pyx_v_bytesitem);
- __Pyx_GIVEREF(__pyx_v_bytesitem);
- PyTuple_SET_ITEM(__pyx_t_9, 1+__pyx_t_8, __pyx_v_bytesitem);
- __pyx_t_6 = 0;
- __pyx_t_1 = __Pyx_PyObject_Call(__pyx_t_5, __pyx_t_9, NULL); if (unlikely(!__pyx_t_1)) __PYX_ERR(1, 493, __pyx_L3_error)
- __Pyx_GOTREF(__pyx_t_1);
- __Pyx_DECREF(__pyx_t_9); __pyx_t_9 = 0;
- }
- __Pyx_DECREF(__pyx_t_5); __pyx_t_5 = 0;
- __pyx_v_result = __pyx_t_1;
- __pyx_t_1 = 0;
-
- /* "View.MemoryView":492
- *
- * bytesitem = itemp[:self.view.itemsize]
- * try: # <<<<<<<<<<<<<<
- * result = struct.unpack(self.view.format, bytesitem)
- * except struct.error:
- */
- }
-
- /* "View.MemoryView":497
- * raise ValueError("Unable to convert item to object")
- * else:
- * if len(self.view.format) == 1: # <<<<<<<<<<<<<<
- * return result[0]
- * return result
- */
- /*else:*/ {
- __pyx_t_10 = strlen(__pyx_v_self->view.format);
- __pyx_t_11 = ((__pyx_t_10 == 1) != 0);
- if (__pyx_t_11) {
-
- /* "View.MemoryView":498
- * else:
- * if len(self.view.format) == 1:
- * return result[0] # <<<<<<<<<<<<<<
- * return result
- *
- */
- __Pyx_XDECREF(__pyx_r);
- __pyx_t_1 = __Pyx_GetItemInt(__pyx_v_result, 0, long, 1, __Pyx_PyInt_From_long, 0, 0, 1); if (unlikely(!__pyx_t_1)) __PYX_ERR(1, 498, __pyx_L5_except_error)
- __Pyx_GOTREF(__pyx_t_1);
- __pyx_r = __pyx_t_1;
- __pyx_t_1 = 0;
- goto __pyx_L6_except_return;
-
- /* "View.MemoryView":497
- * raise ValueError("Unable to convert item to object")
- * else:
- * if len(self.view.format) == 1: # <<<<<<<<<<<<<<
- * return result[0]
- * return result
- */
- }
-
- /* "View.MemoryView":499
- * if len(self.view.format) == 1:
- * return result[0]
- * return result # <<<<<<<<<<<<<<
- *
- * cdef assign_item_from_object(self, char *itemp, object value):
- */
- __Pyx_XDECREF(__pyx_r);
- __Pyx_INCREF(__pyx_v_result);
- __pyx_r = __pyx_v_result;
- goto __pyx_L6_except_return;
- }
- __pyx_L3_error:;
- __Pyx_XDECREF(__pyx_t_1); __pyx_t_1 = 0;
- __Pyx_XDECREF(__pyx_t_5); __pyx_t_5 = 0;
- __Pyx_XDECREF(__pyx_t_6); __pyx_t_6 = 0;
- __Pyx_XDECREF(__pyx_t_7); __pyx_t_7 = 0;
- __Pyx_XDECREF(__pyx_t_9); __pyx_t_9 = 0;
-
- /* "View.MemoryView":494
- * try:
- * result = struct.unpack(self.view.format, bytesitem)
- * except struct.error: # <<<<<<<<<<<<<<
- * raise ValueError("Unable to convert item to object")
- * else:
- */
- __Pyx_ErrFetch(&__pyx_t_1, &__pyx_t_5, &__pyx_t_9);
- __pyx_t_6 = __Pyx_PyObject_GetAttrStr(__pyx_v_struct, __pyx_n_s_error); if (unlikely(!__pyx_t_6)) __PYX_ERR(1, 494, __pyx_L5_except_error)
- __Pyx_GOTREF(__pyx_t_6);
- __pyx_t_8 = __Pyx_PyErr_GivenExceptionMatches(__pyx_t_1, __pyx_t_6);
- __Pyx_DECREF(__pyx_t_6); __pyx_t_6 = 0;
- __Pyx_ErrRestore(__pyx_t_1, __pyx_t_5, __pyx_t_9);
- __pyx_t_1 = 0; __pyx_t_5 = 0; __pyx_t_9 = 0;
- if (__pyx_t_8) {
- __Pyx_AddTraceback("View.MemoryView.memoryview.convert_item_to_object", __pyx_clineno, __pyx_lineno, __pyx_filename);
- if (__Pyx_GetException(&__pyx_t_9, &__pyx_t_5, &__pyx_t_1) < 0) __PYX_ERR(1, 494, __pyx_L5_except_error)
- __Pyx_GOTREF(__pyx_t_9);
- __Pyx_GOTREF(__pyx_t_5);
- __Pyx_GOTREF(__pyx_t_1);
-
- /* "View.MemoryView":495
- * result = struct.unpack(self.view.format, bytesitem)
- * except struct.error:
- * raise ValueError("Unable to convert item to object") # <<<<<<<<<<<<<<
- * else:
- * if len(self.view.format) == 1:
- */
- __pyx_t_6 = __Pyx_PyObject_Call(__pyx_builtin_ValueError, __pyx_tuple__10, NULL); if (unlikely(!__pyx_t_6)) __PYX_ERR(1, 495, __pyx_L5_except_error)
- __Pyx_GOTREF(__pyx_t_6);
- __Pyx_Raise(__pyx_t_6, 0, 0, 0);
- __Pyx_DECREF(__pyx_t_6); __pyx_t_6 = 0;
- __PYX_ERR(1, 495, __pyx_L5_except_error)
- }
- goto __pyx_L5_except_error;
- __pyx_L5_except_error:;
-
- /* "View.MemoryView":492
- *
- * bytesitem = itemp[:self.view.itemsize]
- * try: # <<<<<<<<<<<<<<
- * result = struct.unpack(self.view.format, bytesitem)
- * except struct.error:
- */
- __Pyx_XGIVEREF(__pyx_t_2);
- __Pyx_XGIVEREF(__pyx_t_3);
- __Pyx_XGIVEREF(__pyx_t_4);
- __Pyx_ExceptionReset(__pyx_t_2, __pyx_t_3, __pyx_t_4);
- goto __pyx_L1_error;
- __pyx_L6_except_return:;
- __Pyx_XGIVEREF(__pyx_t_2);
- __Pyx_XGIVEREF(__pyx_t_3);
- __Pyx_XGIVEREF(__pyx_t_4);
- __Pyx_ExceptionReset(__pyx_t_2, __pyx_t_3, __pyx_t_4);
- goto __pyx_L0;
- }
-
- /* "View.MemoryView":485
- * self.assign_item_from_object(itemp, value)
- *
- * cdef convert_item_to_object(self, char *itemp): # <<<<<<<<<<<<<<
- * """Only used if instantiated manually by the user, or if Cython doesn't
- * know how to convert the type"""
- */
-
- /* function exit code */
- __pyx_L1_error:;
- __Pyx_XDECREF(__pyx_t_1);
- __Pyx_XDECREF(__pyx_t_5);
- __Pyx_XDECREF(__pyx_t_6);
- __Pyx_XDECREF(__pyx_t_7);
- __Pyx_XDECREF(__pyx_t_9);
- __Pyx_AddTraceback("View.MemoryView.memoryview.convert_item_to_object", __pyx_clineno, __pyx_lineno, __pyx_filename);
- __pyx_r = 0;
- __pyx_L0:;
- __Pyx_XDECREF(__pyx_v_struct);
- __Pyx_XDECREF(__pyx_v_bytesitem);
- __Pyx_XDECREF(__pyx_v_result);
- __Pyx_XGIVEREF(__pyx_r);
- __Pyx_RefNannyFinishContext();
- return __pyx_r;
-}
-
-/* "View.MemoryView":501
- * return result
- *
- * cdef assign_item_from_object(self, char *itemp, object value): # <<<<<<<<<<<<<<
- * """Only used if instantiated manually by the user, or if Cython doesn't
- * know how to convert the type"""
- */
-
-static PyObject *__pyx_memoryview_assign_item_from_object(struct __pyx_memoryview_obj *__pyx_v_self, char *__pyx_v_itemp, PyObject *__pyx_v_value) {
- PyObject *__pyx_v_struct = NULL;
- char __pyx_v_c;
- PyObject *__pyx_v_bytesvalue = 0;
- Py_ssize_t __pyx_v_i;
- PyObject *__pyx_r = NULL;
- __Pyx_RefNannyDeclarations
- PyObject *__pyx_t_1 = NULL;
- int __pyx_t_2;
- int __pyx_t_3;
- PyObject *__pyx_t_4 = NULL;
- PyObject *__pyx_t_5 = NULL;
- PyObject *__pyx_t_6 = NULL;
- int __pyx_t_7;
- PyObject *__pyx_t_8 = NULL;
- Py_ssize_t __pyx_t_9;
- PyObject *__pyx_t_10 = NULL;
- char *__pyx_t_11;
- char *__pyx_t_12;
- char *__pyx_t_13;
- char *__pyx_t_14;
- int __pyx_lineno = 0;
- const char *__pyx_filename = NULL;
- int __pyx_clineno = 0;
- __Pyx_RefNannySetupContext("assign_item_from_object", 0);
-
- /* "View.MemoryView":504
- * """Only used if instantiated manually by the user, or if Cython doesn't
- * know how to convert the type"""
- * import struct # <<<<<<<<<<<<<<
- * cdef char c
- * cdef bytes bytesvalue
- */
- __pyx_t_1 = __Pyx_Import(__pyx_n_s_struct, 0, 0); if (unlikely(!__pyx_t_1)) __PYX_ERR(1, 504, __pyx_L1_error)
- __Pyx_GOTREF(__pyx_t_1);
- __pyx_v_struct = __pyx_t_1;
- __pyx_t_1 = 0;
-
- /* "View.MemoryView":509
- * cdef Py_ssize_t i
- *
- * if isinstance(value, tuple): # <<<<<<<<<<<<<<
- * bytesvalue = struct.pack(self.view.format, *value)
- * else:
- */
- __pyx_t_2 = PyTuple_Check(__pyx_v_value);
- __pyx_t_3 = (__pyx_t_2 != 0);
- if (__pyx_t_3) {
-
- /* "View.MemoryView":510
- *
- * if isinstance(value, tuple):
- * bytesvalue = struct.pack(self.view.format, *value) # <<<<<<<<<<<<<<
- * else:
- * bytesvalue = struct.pack(self.view.format, value)
- */
- __pyx_t_1 = __Pyx_PyObject_GetAttrStr(__pyx_v_struct, __pyx_n_s_pack); if (unlikely(!__pyx_t_1)) __PYX_ERR(1, 510, __pyx_L1_error)
- __Pyx_GOTREF(__pyx_t_1);
- __pyx_t_4 = __Pyx_PyBytes_FromString(__pyx_v_self->view.format); if (unlikely(!__pyx_t_4)) __PYX_ERR(1, 510, __pyx_L1_error)
- __Pyx_GOTREF(__pyx_t_4);
- __pyx_t_5 = PyTuple_New(1); if (unlikely(!__pyx_t_5)) __PYX_ERR(1, 510, __pyx_L1_error)
- __Pyx_GOTREF(__pyx_t_5);
- __Pyx_GIVEREF(__pyx_t_4);
- PyTuple_SET_ITEM(__pyx_t_5, 0, __pyx_t_4);
- __pyx_t_4 = 0;
- __pyx_t_4 = __Pyx_PySequence_Tuple(__pyx_v_value); if (unlikely(!__pyx_t_4)) __PYX_ERR(1, 510, __pyx_L1_error)
- __Pyx_GOTREF(__pyx_t_4);
- __pyx_t_6 = PyNumber_Add(__pyx_t_5, __pyx_t_4); if (unlikely(!__pyx_t_6)) __PYX_ERR(1, 510, __pyx_L1_error)
- __Pyx_GOTREF(__pyx_t_6);
- __Pyx_DECREF(__pyx_t_5); __pyx_t_5 = 0;
- __Pyx_DECREF(__pyx_t_4); __pyx_t_4 = 0;
- __pyx_t_4 = __Pyx_PyObject_Call(__pyx_t_1, __pyx_t_6, NULL); if (unlikely(!__pyx_t_4)) __PYX_ERR(1, 510, __pyx_L1_error)
- __Pyx_GOTREF(__pyx_t_4);
- __Pyx_DECREF(__pyx_t_1); __pyx_t_1 = 0;
- __Pyx_DECREF(__pyx_t_6); __pyx_t_6 = 0;
- if (!(likely(PyBytes_CheckExact(__pyx_t_4))||((__pyx_t_4) == Py_None)||(PyErr_Format(PyExc_TypeError, "Expected %.16s, got %.200s", "bytes", Py_TYPE(__pyx_t_4)->tp_name), 0))) __PYX_ERR(1, 510, __pyx_L1_error)
- __pyx_v_bytesvalue = ((PyObject*)__pyx_t_4);
- __pyx_t_4 = 0;
-
- /* "View.MemoryView":509
- * cdef Py_ssize_t i
- *
- * if isinstance(value, tuple): # <<<<<<<<<<<<<<
- * bytesvalue = struct.pack(self.view.format, *value)
- * else:
- */
- goto __pyx_L3;
- }
-
- /* "View.MemoryView":512
- * bytesvalue = struct.pack(self.view.format, *value)
- * else:
- * bytesvalue = struct.pack(self.view.format, value) # <<<<<<<<<<<<<<
- *
- * for i, c in enumerate(bytesvalue):
- */
- /*else*/ {
- __pyx_t_6 = __Pyx_PyObject_GetAttrStr(__pyx_v_struct, __pyx_n_s_pack); if (unlikely(!__pyx_t_6)) __PYX_ERR(1, 512, __pyx_L1_error)
- __Pyx_GOTREF(__pyx_t_6);
- __pyx_t_1 = __Pyx_PyBytes_FromString(__pyx_v_self->view.format); if (unlikely(!__pyx_t_1)) __PYX_ERR(1, 512, __pyx_L1_error)
- __Pyx_GOTREF(__pyx_t_1);
- __pyx_t_5 = NULL;
- __pyx_t_7 = 0;
- if (CYTHON_UNPACK_METHODS && likely(PyMethod_Check(__pyx_t_6))) {
- __pyx_t_5 = PyMethod_GET_SELF(__pyx_t_6);
- if (likely(__pyx_t_5)) {
- PyObject* function = PyMethod_GET_FUNCTION(__pyx_t_6);
- __Pyx_INCREF(__pyx_t_5);
- __Pyx_INCREF(function);
- __Pyx_DECREF_SET(__pyx_t_6, function);
- __pyx_t_7 = 1;
- }
- }
- #if CYTHON_FAST_PYCALL
- if (PyFunction_Check(__pyx_t_6)) {
- PyObject *__pyx_temp[3] = {__pyx_t_5, __pyx_t_1, __pyx_v_value};
- __pyx_t_4 = __Pyx_PyFunction_FastCall(__pyx_t_6, __pyx_temp+1-__pyx_t_7, 2+__pyx_t_7); if (unlikely(!__pyx_t_4)) __PYX_ERR(1, 512, __pyx_L1_error)
- __Pyx_XDECREF(__pyx_t_5); __pyx_t_5 = 0;
- __Pyx_GOTREF(__pyx_t_4);
- __Pyx_DECREF(__pyx_t_1); __pyx_t_1 = 0;
- } else
- #endif
- #if CYTHON_FAST_PYCCALL
- if (__Pyx_PyFastCFunction_Check(__pyx_t_6)) {
- PyObject *__pyx_temp[3] = {__pyx_t_5, __pyx_t_1, __pyx_v_value};
- __pyx_t_4 = __Pyx_PyCFunction_FastCall(__pyx_t_6, __pyx_temp+1-__pyx_t_7, 2+__pyx_t_7); if (unlikely(!__pyx_t_4)) __PYX_ERR(1, 512, __pyx_L1_error)
- __Pyx_XDECREF(__pyx_t_5); __pyx_t_5 = 0;
- __Pyx_GOTREF(__pyx_t_4);
- __Pyx_DECREF(__pyx_t_1); __pyx_t_1 = 0;
- } else
- #endif
- {
- __pyx_t_8 = PyTuple_New(2+__pyx_t_7); if (unlikely(!__pyx_t_8)) __PYX_ERR(1, 512, __pyx_L1_error)
- __Pyx_GOTREF(__pyx_t_8);
- if (__pyx_t_5) {
- __Pyx_GIVEREF(__pyx_t_5); PyTuple_SET_ITEM(__pyx_t_8, 0, __pyx_t_5); __pyx_t_5 = NULL;
- }
- __Pyx_GIVEREF(__pyx_t_1);
- PyTuple_SET_ITEM(__pyx_t_8, 0+__pyx_t_7, __pyx_t_1);
- __Pyx_INCREF(__pyx_v_value);
- __Pyx_GIVEREF(__pyx_v_value);
- PyTuple_SET_ITEM(__pyx_t_8, 1+__pyx_t_7, __pyx_v_value);
- __pyx_t_1 = 0;
- __pyx_t_4 = __Pyx_PyObject_Call(__pyx_t_6, __pyx_t_8, NULL); if (unlikely(!__pyx_t_4)) __PYX_ERR(1, 512, __pyx_L1_error)
- __Pyx_GOTREF(__pyx_t_4);
- __Pyx_DECREF(__pyx_t_8); __pyx_t_8 = 0;
- }
- __Pyx_DECREF(__pyx_t_6); __pyx_t_6 = 0;
- if (!(likely(PyBytes_CheckExact(__pyx_t_4))||((__pyx_t_4) == Py_None)||(PyErr_Format(PyExc_TypeError, "Expected %.16s, got %.200s", "bytes", Py_TYPE(__pyx_t_4)->tp_name), 0))) __PYX_ERR(1, 512, __pyx_L1_error)
- __pyx_v_bytesvalue = ((PyObject*)__pyx_t_4);
- __pyx_t_4 = 0;
- }
- __pyx_L3:;
-
- /* "View.MemoryView":514
- * bytesvalue = struct.pack(self.view.format, value)
- *
- * for i, c in enumerate(bytesvalue): # <<<<<<<<<<<<<<
- * itemp[i] = c
- *
- */
- __pyx_t_9 = 0;
- if (unlikely(__pyx_v_bytesvalue == Py_None)) {
- PyErr_SetString(PyExc_TypeError, "'NoneType' is not iterable");
- __PYX_ERR(1, 514, __pyx_L1_error)
- }
- __Pyx_INCREF(__pyx_v_bytesvalue);
- __pyx_t_10 = __pyx_v_bytesvalue;
- __pyx_t_12 = PyBytes_AS_STRING(__pyx_t_10);
- __pyx_t_13 = (__pyx_t_12 + PyBytes_GET_SIZE(__pyx_t_10));
- for (__pyx_t_14 = __pyx_t_12; __pyx_t_14 < __pyx_t_13; __pyx_t_14++) {
- __pyx_t_11 = __pyx_t_14;
- __pyx_v_c = (__pyx_t_11[0]);
-
- /* "View.MemoryView":515
- *
- * for i, c in enumerate(bytesvalue):
- * itemp[i] = c # <<<<<<<<<<<<<<
- *
- * @cname('getbuffer')
- */
- __pyx_v_i = __pyx_t_9;
-
- /* "View.MemoryView":514
- * bytesvalue = struct.pack(self.view.format, value)
- *
- * for i, c in enumerate(bytesvalue): # <<<<<<<<<<<<<<
- * itemp[i] = c
- *
- */
- __pyx_t_9 = (__pyx_t_9 + 1);
-
- /* "View.MemoryView":515
- *
- * for i, c in enumerate(bytesvalue):
- * itemp[i] = c # <<<<<<<<<<<<<<
- *
- * @cname('getbuffer')
- */
- (__pyx_v_itemp[__pyx_v_i]) = __pyx_v_c;
- }
- __Pyx_DECREF(__pyx_t_10); __pyx_t_10 = 0;
-
- /* "View.MemoryView":501
- * return result
- *
- * cdef assign_item_from_object(self, char *itemp, object value): # <<<<<<<<<<<<<<
- * """Only used if instantiated manually by the user, or if Cython doesn't
- * know how to convert the type"""
- */
-
- /* function exit code */
- __pyx_r = Py_None; __Pyx_INCREF(Py_None);
- goto __pyx_L0;
- __pyx_L1_error:;
- __Pyx_XDECREF(__pyx_t_1);
- __Pyx_XDECREF(__pyx_t_4);
- __Pyx_XDECREF(__pyx_t_5);
- __Pyx_XDECREF(__pyx_t_6);
- __Pyx_XDECREF(__pyx_t_8);
- __Pyx_XDECREF(__pyx_t_10);
- __Pyx_AddTraceback("View.MemoryView.memoryview.assign_item_from_object", __pyx_clineno, __pyx_lineno, __pyx_filename);
- __pyx_r = 0;
- __pyx_L0:;
- __Pyx_XDECREF(__pyx_v_struct);
- __Pyx_XDECREF(__pyx_v_bytesvalue);
- __Pyx_XGIVEREF(__pyx_r);
- __Pyx_RefNannyFinishContext();
- return __pyx_r;
-}
-
-/* "View.MemoryView":518
- *
- * @cname('getbuffer')
- * def __getbuffer__(self, Py_buffer *info, int flags): # <<<<<<<<<<<<<<
- * if flags & PyBUF_WRITABLE and self.view.readonly:
- * raise ValueError("Cannot create writable memory view from read-only memoryview")
- */
-
-/* Python wrapper */
-static CYTHON_UNUSED int __pyx_memoryview_getbuffer(PyObject *__pyx_v_self, Py_buffer *__pyx_v_info, int __pyx_v_flags); /*proto*/
-static CYTHON_UNUSED int __pyx_memoryview_getbuffer(PyObject *__pyx_v_self, Py_buffer *__pyx_v_info, int __pyx_v_flags) {
- int __pyx_r;
- __Pyx_RefNannyDeclarations
- __Pyx_RefNannySetupContext("__getbuffer__ (wrapper)", 0);
- __pyx_r = __pyx_memoryview___pyx_pf_15View_dot_MemoryView_10memoryview_8__getbuffer__(((struct __pyx_memoryview_obj *)__pyx_v_self), ((Py_buffer *)__pyx_v_info), ((int)__pyx_v_flags));
-
- /* function exit code */
- __Pyx_RefNannyFinishContext();
- return __pyx_r;
-}
-
-static int __pyx_memoryview___pyx_pf_15View_dot_MemoryView_10memoryview_8__getbuffer__(struct __pyx_memoryview_obj *__pyx_v_self, Py_buffer *__pyx_v_info, int __pyx_v_flags) {
- int __pyx_r;
- __Pyx_RefNannyDeclarations
- int __pyx_t_1;
- int __pyx_t_2;
- PyObject *__pyx_t_3 = NULL;
- Py_ssize_t *__pyx_t_4;
- char *__pyx_t_5;
- void *__pyx_t_6;
- int __pyx_t_7;
- Py_ssize_t __pyx_t_8;
- int __pyx_lineno = 0;
- const char *__pyx_filename = NULL;
- int __pyx_clineno = 0;
- if (__pyx_v_info == NULL) {
- PyErr_SetString(PyExc_BufferError, "PyObject_GetBuffer: view==NULL argument is obsolete");
- return -1;
- }
- __Pyx_RefNannySetupContext("__getbuffer__", 0);
- __pyx_v_info->obj = Py_None; __Pyx_INCREF(Py_None);
- __Pyx_GIVEREF(__pyx_v_info->obj);
-
- /* "View.MemoryView":519
- * @cname('getbuffer')
- * def __getbuffer__(self, Py_buffer *info, int flags):
- * if flags & PyBUF_WRITABLE and self.view.readonly: # <<<<<<<<<<<<<<
- * raise ValueError("Cannot create writable memory view from read-only memoryview")
- *
- */
- __pyx_t_2 = ((__pyx_v_flags & PyBUF_WRITABLE) != 0);
- if (__pyx_t_2) {
- } else {
- __pyx_t_1 = __pyx_t_2;
- goto __pyx_L4_bool_binop_done;
- }
- __pyx_t_2 = (__pyx_v_self->view.readonly != 0);
- __pyx_t_1 = __pyx_t_2;
- __pyx_L4_bool_binop_done:;
- if (unlikely(__pyx_t_1)) {
-
- /* "View.MemoryView":520
- * def __getbuffer__(self, Py_buffer *info, int flags):
- * if flags & PyBUF_WRITABLE and self.view.readonly:
- * raise ValueError("Cannot create writable memory view from read-only memoryview") # <<<<<<<<<<<<<<
- *
- * if flags & PyBUF_ND:
- */
- __pyx_t_3 = __Pyx_PyObject_Call(__pyx_builtin_ValueError, __pyx_tuple__11, NULL); if (unlikely(!__pyx_t_3)) __PYX_ERR(1, 520, __pyx_L1_error)
- __Pyx_GOTREF(__pyx_t_3);
- __Pyx_Raise(__pyx_t_3, 0, 0, 0);
- __Pyx_DECREF(__pyx_t_3); __pyx_t_3 = 0;
- __PYX_ERR(1, 520, __pyx_L1_error)
-
- /* "View.MemoryView":519
- * @cname('getbuffer')
- * def __getbuffer__(self, Py_buffer *info, int flags):
- * if flags & PyBUF_WRITABLE and self.view.readonly: # <<<<<<<<<<<<<<
- * raise ValueError("Cannot create writable memory view from read-only memoryview")
- *
- */
- }
-
- /* "View.MemoryView":522
- * raise ValueError("Cannot create writable memory view from read-only memoryview")
- *
- * if flags & PyBUF_ND: # <<<<<<<<<<<<<<
- * info.shape = self.view.shape
- * else:
- */
- __pyx_t_1 = ((__pyx_v_flags & PyBUF_ND) != 0);
- if (__pyx_t_1) {
-
- /* "View.MemoryView":523
- *
- * if flags & PyBUF_ND:
- * info.shape = self.view.shape # <<<<<<<<<<<<<<
- * else:
- * info.shape = NULL
- */
- __pyx_t_4 = __pyx_v_self->view.shape;
- __pyx_v_info->shape = __pyx_t_4;
-
- /* "View.MemoryView":522
- * raise ValueError("Cannot create writable memory view from read-only memoryview")
- *
- * if flags & PyBUF_ND: # <<<<<<<<<<<<<<
- * info.shape = self.view.shape
- * else:
- */
- goto __pyx_L6;
- }
-
- /* "View.MemoryView":525
- * info.shape = self.view.shape
- * else:
- * info.shape = NULL # <<<<<<<<<<<<<<
- *
- * if flags & PyBUF_STRIDES:
- */
- /*else*/ {
- __pyx_v_info->shape = NULL;
- }
- __pyx_L6:;
-
- /* "View.MemoryView":527
- * info.shape = NULL
- *
- * if flags & PyBUF_STRIDES: # <<<<<<<<<<<<<<
- * info.strides = self.view.strides
- * else:
- */
- __pyx_t_1 = ((__pyx_v_flags & PyBUF_STRIDES) != 0);
- if (__pyx_t_1) {
-
- /* "View.MemoryView":528
- *
- * if flags & PyBUF_STRIDES:
- * info.strides = self.view.strides # <<<<<<<<<<<<<<
- * else:
- * info.strides = NULL
- */
- __pyx_t_4 = __pyx_v_self->view.strides;
- __pyx_v_info->strides = __pyx_t_4;
-
- /* "View.MemoryView":527
- * info.shape = NULL
- *
- * if flags & PyBUF_STRIDES: # <<<<<<<<<<<<<<
- * info.strides = self.view.strides
- * else:
- */
- goto __pyx_L7;
- }
-
- /* "View.MemoryView":530
- * info.strides = self.view.strides
- * else:
- * info.strides = NULL # <<<<<<<<<<<<<<
- *
- * if flags & PyBUF_INDIRECT:
- */
- /*else*/ {
- __pyx_v_info->strides = NULL;
- }
- __pyx_L7:;
-
- /* "View.MemoryView":532
- * info.strides = NULL
- *
- * if flags & PyBUF_INDIRECT: # <<<<<<<<<<<<<<
- * info.suboffsets = self.view.suboffsets
- * else:
- */
- __pyx_t_1 = ((__pyx_v_flags & PyBUF_INDIRECT) != 0);
- if (__pyx_t_1) {
-
- /* "View.MemoryView":533
- *
- * if flags & PyBUF_INDIRECT:
- * info.suboffsets = self.view.suboffsets # <<<<<<<<<<<<<<
- * else:
- * info.suboffsets = NULL
- */
- __pyx_t_4 = __pyx_v_self->view.suboffsets;
- __pyx_v_info->suboffsets = __pyx_t_4;
-
- /* "View.MemoryView":532
- * info.strides = NULL
- *
- * if flags & PyBUF_INDIRECT: # <<<<<<<<<<<<<<
- * info.suboffsets = self.view.suboffsets
- * else:
- */
- goto __pyx_L8;
- }
-
- /* "View.MemoryView":535
- * info.suboffsets = self.view.suboffsets
- * else:
- * info.suboffsets = NULL # <<<<<<<<<<<<<<
- *
- * if flags & PyBUF_FORMAT:
- */
- /*else*/ {
- __pyx_v_info->suboffsets = NULL;
- }
- __pyx_L8:;
-
- /* "View.MemoryView":537
- * info.suboffsets = NULL
- *
- * if flags & PyBUF_FORMAT: # <<<<<<<<<<<<<<
- * info.format = self.view.format
- * else:
- */
- __pyx_t_1 = ((__pyx_v_flags & PyBUF_FORMAT) != 0);
- if (__pyx_t_1) {
-
- /* "View.MemoryView":538
- *
- * if flags & PyBUF_FORMAT:
- * info.format = self.view.format # <<<<<<<<<<<<<<
- * else:
- * info.format = NULL
- */
- __pyx_t_5 = __pyx_v_self->view.format;
- __pyx_v_info->format = __pyx_t_5;
-
- /* "View.MemoryView":537
- * info.suboffsets = NULL
- *
- * if flags & PyBUF_FORMAT: # <<<<<<<<<<<<<<
- * info.format = self.view.format
- * else:
- */
- goto __pyx_L9;
- }
-
- /* "View.MemoryView":540
- * info.format = self.view.format
- * else:
- * info.format = NULL # <<<<<<<<<<<<<<
- *
- * info.buf = self.view.buf
- */
- /*else*/ {
- __pyx_v_info->format = NULL;
- }
- __pyx_L9:;
-
- /* "View.MemoryView":542
- * info.format = NULL
- *
- * info.buf = self.view.buf # <<<<<<<<<<<<<<
- * info.ndim = self.view.ndim
- * info.itemsize = self.view.itemsize
- */
- __pyx_t_6 = __pyx_v_self->view.buf;
- __pyx_v_info->buf = __pyx_t_6;
-
- /* "View.MemoryView":543
- *
- * info.buf = self.view.buf
- * info.ndim = self.view.ndim # <<<<<<<<<<<<<<
- * info.itemsize = self.view.itemsize
- * info.len = self.view.len
- */
- __pyx_t_7 = __pyx_v_self->view.ndim;
- __pyx_v_info->ndim = __pyx_t_7;
-
- /* "View.MemoryView":544
- * info.buf = self.view.buf
- * info.ndim = self.view.ndim
- * info.itemsize = self.view.itemsize # <<<<<<<<<<<<<<
- * info.len = self.view.len
- * info.readonly = self.view.readonly
- */
- __pyx_t_8 = __pyx_v_self->view.itemsize;
- __pyx_v_info->itemsize = __pyx_t_8;
-
- /* "View.MemoryView":545
- * info.ndim = self.view.ndim
- * info.itemsize = self.view.itemsize
- * info.len = self.view.len # <<<<<<<<<<<<<<
- * info.readonly = self.view.readonly
- * info.obj = self
- */
- __pyx_t_8 = __pyx_v_self->view.len;
- __pyx_v_info->len = __pyx_t_8;
-
- /* "View.MemoryView":546
- * info.itemsize = self.view.itemsize
- * info.len = self.view.len
- * info.readonly = self.view.readonly # <<<<<<<<<<<<<<
- * info.obj = self
- *
- */
- __pyx_t_1 = __pyx_v_self->view.readonly;
- __pyx_v_info->readonly = __pyx_t_1;
-
- /* "View.MemoryView":547
- * info.len = self.view.len
- * info.readonly = self.view.readonly
- * info.obj = self # <<<<<<<<<<<<<<
- *
- * __pyx_getbuffer = capsule( &__pyx_memoryview_getbuffer, "getbuffer(obj, view, flags)")
- */
- __Pyx_INCREF(((PyObject *)__pyx_v_self));
- __Pyx_GIVEREF(((PyObject *)__pyx_v_self));
- __Pyx_GOTREF(__pyx_v_info->obj);
- __Pyx_DECREF(__pyx_v_info->obj);
- __pyx_v_info->obj = ((PyObject *)__pyx_v_self);
-
- /* "View.MemoryView":518
- *
- * @cname('getbuffer')
- * def __getbuffer__(self, Py_buffer *info, int flags): # <<<<<<<<<<<<<<
- * if flags & PyBUF_WRITABLE and self.view.readonly:
- * raise ValueError("Cannot create writable memory view from read-only memoryview")
- */
-
- /* function exit code */
- __pyx_r = 0;
- goto __pyx_L0;
- __pyx_L1_error:;
- __Pyx_XDECREF(__pyx_t_3);
- __Pyx_AddTraceback("View.MemoryView.memoryview.__getbuffer__", __pyx_clineno, __pyx_lineno, __pyx_filename);
- __pyx_r = -1;
- if (__pyx_v_info->obj != NULL) {
- __Pyx_GOTREF(__pyx_v_info->obj);
- __Pyx_DECREF(__pyx_v_info->obj); __pyx_v_info->obj = 0;
- }
- goto __pyx_L2;
- __pyx_L0:;
- if (__pyx_v_info->obj == Py_None) {
- __Pyx_GOTREF(__pyx_v_info->obj);
- __Pyx_DECREF(__pyx_v_info->obj); __pyx_v_info->obj = 0;
- }
- __pyx_L2:;
- __Pyx_RefNannyFinishContext();
- return __pyx_r;
-}
-
-/* "View.MemoryView":553
- *
- * @property
- * def T(self): # <<<<<<<<<<<<<<
- * cdef _memoryviewslice result = memoryview_copy(self)
- * transpose_memslice(&result.from_slice)
- */
-
-/* Python wrapper */
-static PyObject *__pyx_pw_15View_dot_MemoryView_10memoryview_1T_1__get__(PyObject *__pyx_v_self); /*proto*/
-static PyObject *__pyx_pw_15View_dot_MemoryView_10memoryview_1T_1__get__(PyObject *__pyx_v_self) {
- PyObject *__pyx_r = 0;
- __Pyx_RefNannyDeclarations
- __Pyx_RefNannySetupContext("__get__ (wrapper)", 0);
- __pyx_r = __pyx_pf_15View_dot_MemoryView_10memoryview_1T___get__(((struct __pyx_memoryview_obj *)__pyx_v_self));
-
- /* function exit code */
- __Pyx_RefNannyFinishContext();
- return __pyx_r;
-}
-
-static PyObject *__pyx_pf_15View_dot_MemoryView_10memoryview_1T___get__(struct __pyx_memoryview_obj *__pyx_v_self) {
- struct __pyx_memoryviewslice_obj *__pyx_v_result = 0;
- PyObject *__pyx_r = NULL;
- __Pyx_RefNannyDeclarations
- PyObject *__pyx_t_1 = NULL;
- int __pyx_t_2;
- int __pyx_lineno = 0;
- const char *__pyx_filename = NULL;
- int __pyx_clineno = 0;
- __Pyx_RefNannySetupContext("__get__", 0);
-
- /* "View.MemoryView":554
- * @property
- * def T(self):
- * cdef _memoryviewslice result = memoryview_copy(self) # <<<<<<<<<<<<<<
- * transpose_memslice(&result.from_slice)
- * return result
- */
- __pyx_t_1 = __pyx_memoryview_copy_object(__pyx_v_self); if (unlikely(!__pyx_t_1)) __PYX_ERR(1, 554, __pyx_L1_error)
- __Pyx_GOTREF(__pyx_t_1);
- if (!(likely(((__pyx_t_1) == Py_None) || likely(__Pyx_TypeTest(__pyx_t_1, __pyx_memoryviewslice_type))))) __PYX_ERR(1, 554, __pyx_L1_error)
- __pyx_v_result = ((struct __pyx_memoryviewslice_obj *)__pyx_t_1);
- __pyx_t_1 = 0;
-
- /* "View.MemoryView":555
- * def T(self):
- * cdef _memoryviewslice result = memoryview_copy(self)
- * transpose_memslice(&result.from_slice) # <<<<<<<<<<<<<<
- * return result
- *
- */
- __pyx_t_2 = __pyx_memslice_transpose((&__pyx_v_result->from_slice)); if (unlikely(__pyx_t_2 == ((int)0))) __PYX_ERR(1, 555, __pyx_L1_error)
-
- /* "View.MemoryView":556
- * cdef _memoryviewslice result = memoryview_copy(self)
- * transpose_memslice(&result.from_slice)
- * return result # <<<<<<<<<<<<<<
- *
- * @property
- */
- __Pyx_XDECREF(__pyx_r);
- __Pyx_INCREF(((PyObject *)__pyx_v_result));
- __pyx_r = ((PyObject *)__pyx_v_result);
- goto __pyx_L0;
-
- /* "View.MemoryView":553
- *
- * @property
- * def T(self): # <<<<<<<<<<<<<<
- * cdef _memoryviewslice result = memoryview_copy(self)
- * transpose_memslice(&result.from_slice)
- */
-
- /* function exit code */
- __pyx_L1_error:;
- __Pyx_XDECREF(__pyx_t_1);
- __Pyx_AddTraceback("View.MemoryView.memoryview.T.__get__", __pyx_clineno, __pyx_lineno, __pyx_filename);
- __pyx_r = NULL;
- __pyx_L0:;
- __Pyx_XDECREF((PyObject *)__pyx_v_result);
- __Pyx_XGIVEREF(__pyx_r);
- __Pyx_RefNannyFinishContext();
- return __pyx_r;
-}
-
-/* "View.MemoryView":559
- *
- * @property
- * def base(self): # <<<<<<<<<<<<<<
- * return self.obj
- *
- */
-
-/* Python wrapper */
-static PyObject *__pyx_pw_15View_dot_MemoryView_10memoryview_4base_1__get__(PyObject *__pyx_v_self); /*proto*/
-static PyObject *__pyx_pw_15View_dot_MemoryView_10memoryview_4base_1__get__(PyObject *__pyx_v_self) {
- PyObject *__pyx_r = 0;
- __Pyx_RefNannyDeclarations
- __Pyx_RefNannySetupContext("__get__ (wrapper)", 0);
- __pyx_r = __pyx_pf_15View_dot_MemoryView_10memoryview_4base___get__(((struct __pyx_memoryview_obj *)__pyx_v_self));
-
- /* function exit code */
- __Pyx_RefNannyFinishContext();
- return __pyx_r;
-}
-
-static PyObject *__pyx_pf_15View_dot_MemoryView_10memoryview_4base___get__(struct __pyx_memoryview_obj *__pyx_v_self) {
- PyObject *__pyx_r = NULL;
- __Pyx_RefNannyDeclarations
- __Pyx_RefNannySetupContext("__get__", 0);
-
- /* "View.MemoryView":560
- * @property
- * def base(self):
- * return self.obj # <<<<<<<<<<<<<<
- *
- * @property
- */
- __Pyx_XDECREF(__pyx_r);
- __Pyx_INCREF(__pyx_v_self->obj);
- __pyx_r = __pyx_v_self->obj;
- goto __pyx_L0;
-
- /* "View.MemoryView":559
- *
- * @property
- * def base(self): # <<<<<<<<<<<<<<
- * return self.obj
- *
- */
-
- /* function exit code */
- __pyx_L0:;
- __Pyx_XGIVEREF(__pyx_r);
- __Pyx_RefNannyFinishContext();
- return __pyx_r;
-}
-
-/* "View.MemoryView":563
- *
- * @property
- * def shape(self): # <<<<<<<<<<<<<<
- * return tuple([length for length in self.view.shape[:self.view.ndim]])
- *
- */
-
-/* Python wrapper */
-static PyObject *__pyx_pw_15View_dot_MemoryView_10memoryview_5shape_1__get__(PyObject *__pyx_v_self); /*proto*/
-static PyObject *__pyx_pw_15View_dot_MemoryView_10memoryview_5shape_1__get__(PyObject *__pyx_v_self) {
- PyObject *__pyx_r = 0;
- __Pyx_RefNannyDeclarations
- __Pyx_RefNannySetupContext("__get__ (wrapper)", 0);
- __pyx_r = __pyx_pf_15View_dot_MemoryView_10memoryview_5shape___get__(((struct __pyx_memoryview_obj *)__pyx_v_self));
-
- /* function exit code */
- __Pyx_RefNannyFinishContext();
- return __pyx_r;
-}
-
-static PyObject *__pyx_pf_15View_dot_MemoryView_10memoryview_5shape___get__(struct __pyx_memoryview_obj *__pyx_v_self) {
- Py_ssize_t __pyx_v_length;
- PyObject *__pyx_r = NULL;
- __Pyx_RefNannyDeclarations
- PyObject *__pyx_t_1 = NULL;
- Py_ssize_t *__pyx_t_2;
- Py_ssize_t *__pyx_t_3;
- Py_ssize_t *__pyx_t_4;
- PyObject *__pyx_t_5 = NULL;
- int __pyx_lineno = 0;
- const char *__pyx_filename = NULL;
- int __pyx_clineno = 0;
- __Pyx_RefNannySetupContext("__get__", 0);
-
- /* "View.MemoryView":564
- * @property
- * def shape(self):
- * return tuple([length for length in self.view.shape[:self.view.ndim]]) # <<<<<<<<<<<<<<
- *
- * @property
- */
- __Pyx_XDECREF(__pyx_r);
- __pyx_t_1 = PyList_New(0); if (unlikely(!__pyx_t_1)) __PYX_ERR(1, 564, __pyx_L1_error)
- __Pyx_GOTREF(__pyx_t_1);
- __pyx_t_3 = (__pyx_v_self->view.shape + __pyx_v_self->view.ndim);
- for (__pyx_t_4 = __pyx_v_self->view.shape; __pyx_t_4 < __pyx_t_3; __pyx_t_4++) {
- __pyx_t_2 = __pyx_t_4;
- __pyx_v_length = (__pyx_t_2[0]);
- __pyx_t_5 = PyInt_FromSsize_t(__pyx_v_length); if (unlikely(!__pyx_t_5)) __PYX_ERR(1, 564, __pyx_L1_error)
- __Pyx_GOTREF(__pyx_t_5);
- if (unlikely(__Pyx_ListComp_Append(__pyx_t_1, (PyObject*)__pyx_t_5))) __PYX_ERR(1, 564, __pyx_L1_error)
- __Pyx_DECREF(__pyx_t_5); __pyx_t_5 = 0;
- }
- __pyx_t_5 = PyList_AsTuple(((PyObject*)__pyx_t_1)); if (unlikely(!__pyx_t_5)) __PYX_ERR(1, 564, __pyx_L1_error)
- __Pyx_GOTREF(__pyx_t_5);
- __Pyx_DECREF(__pyx_t_1); __pyx_t_1 = 0;
- __pyx_r = __pyx_t_5;
- __pyx_t_5 = 0;
- goto __pyx_L0;
-
- /* "View.MemoryView":563
- *
- * @property
- * def shape(self): # <<<<<<<<<<<<<<
- * return tuple([length for length in self.view.shape[:self.view.ndim]])
- *
- */
-
- /* function exit code */
- __pyx_L1_error:;
- __Pyx_XDECREF(__pyx_t_1);
- __Pyx_XDECREF(__pyx_t_5);
- __Pyx_AddTraceback("View.MemoryView.memoryview.shape.__get__", __pyx_clineno, __pyx_lineno, __pyx_filename);
- __pyx_r = NULL;
- __pyx_L0:;
- __Pyx_XGIVEREF(__pyx_r);
- __Pyx_RefNannyFinishContext();
- return __pyx_r;
-}
-
-/* "View.MemoryView":567
- *
- * @property
- * def strides(self): # <<<<<<<<<<<<<<
- * if self.view.strides == NULL:
- *
- */
-
-/* Python wrapper */
-static PyObject *__pyx_pw_15View_dot_MemoryView_10memoryview_7strides_1__get__(PyObject *__pyx_v_self); /*proto*/
-static PyObject *__pyx_pw_15View_dot_MemoryView_10memoryview_7strides_1__get__(PyObject *__pyx_v_self) {
- PyObject *__pyx_r = 0;
- __Pyx_RefNannyDeclarations
- __Pyx_RefNannySetupContext("__get__ (wrapper)", 0);
- __pyx_r = __pyx_pf_15View_dot_MemoryView_10memoryview_7strides___get__(((struct __pyx_memoryview_obj *)__pyx_v_self));
-
- /* function exit code */
- __Pyx_RefNannyFinishContext();
- return __pyx_r;
-}
-
-static PyObject *__pyx_pf_15View_dot_MemoryView_10memoryview_7strides___get__(struct __pyx_memoryview_obj *__pyx_v_self) {
- Py_ssize_t __pyx_v_stride;
- PyObject *__pyx_r = NULL;
- __Pyx_RefNannyDeclarations
- int __pyx_t_1;
- PyObject *__pyx_t_2 = NULL;
- Py_ssize_t *__pyx_t_3;
- Py_ssize_t *__pyx_t_4;
- Py_ssize_t *__pyx_t_5;
- PyObject *__pyx_t_6 = NULL;
- int __pyx_lineno = 0;
- const char *__pyx_filename = NULL;
- int __pyx_clineno = 0;
- __Pyx_RefNannySetupContext("__get__", 0);
-
- /* "View.MemoryView":568
- * @property
- * def strides(self):
- * if self.view.strides == NULL: # <<<<<<<<<<<<<<
- *
- * raise ValueError("Buffer view does not expose strides")
- */
- __pyx_t_1 = ((__pyx_v_self->view.strides == NULL) != 0);
- if (unlikely(__pyx_t_1)) {
-
- /* "View.MemoryView":570
- * if self.view.strides == NULL:
- *
- * raise ValueError("Buffer view does not expose strides") # <<<<<<<<<<<<<<
- *
- * return tuple([stride for stride in self.view.strides[:self.view.ndim]])
- */
- __pyx_t_2 = __Pyx_PyObject_Call(__pyx_builtin_ValueError, __pyx_tuple__12, NULL); if (unlikely(!__pyx_t_2)) __PYX_ERR(1, 570, __pyx_L1_error)
- __Pyx_GOTREF(__pyx_t_2);
- __Pyx_Raise(__pyx_t_2, 0, 0, 0);
- __Pyx_DECREF(__pyx_t_2); __pyx_t_2 = 0;
- __PYX_ERR(1, 570, __pyx_L1_error)
-
- /* "View.MemoryView":568
- * @property
- * def strides(self):
- * if self.view.strides == NULL: # <<<<<<<<<<<<<<
- *
- * raise ValueError("Buffer view does not expose strides")
- */
- }
-
- /* "View.MemoryView":572
- * raise ValueError("Buffer view does not expose strides")
- *
- * return tuple([stride for stride in self.view.strides[:self.view.ndim]]) # <<<<<<<<<<<<<<
- *
- * @property
- */
- __Pyx_XDECREF(__pyx_r);
- __pyx_t_2 = PyList_New(0); if (unlikely(!__pyx_t_2)) __PYX_ERR(1, 572, __pyx_L1_error)
- __Pyx_GOTREF(__pyx_t_2);
- __pyx_t_4 = (__pyx_v_self->view.strides + __pyx_v_self->view.ndim);
- for (__pyx_t_5 = __pyx_v_self->view.strides; __pyx_t_5 < __pyx_t_4; __pyx_t_5++) {
- __pyx_t_3 = __pyx_t_5;
- __pyx_v_stride = (__pyx_t_3[0]);
- __pyx_t_6 = PyInt_FromSsize_t(__pyx_v_stride); if (unlikely(!__pyx_t_6)) __PYX_ERR(1, 572, __pyx_L1_error)
- __Pyx_GOTREF(__pyx_t_6);
- if (unlikely(__Pyx_ListComp_Append(__pyx_t_2, (PyObject*)__pyx_t_6))) __PYX_ERR(1, 572, __pyx_L1_error)
- __Pyx_DECREF(__pyx_t_6); __pyx_t_6 = 0;
- }
- __pyx_t_6 = PyList_AsTuple(((PyObject*)__pyx_t_2)); if (unlikely(!__pyx_t_6)) __PYX_ERR(1, 572, __pyx_L1_error)
- __Pyx_GOTREF(__pyx_t_6);
- __Pyx_DECREF(__pyx_t_2); __pyx_t_2 = 0;
- __pyx_r = __pyx_t_6;
- __pyx_t_6 = 0;
- goto __pyx_L0;
-
- /* "View.MemoryView":567
- *
- * @property
- * def strides(self): # <<<<<<<<<<<<<<
- * if self.view.strides == NULL:
- *
- */
-
- /* function exit code */
- __pyx_L1_error:;
- __Pyx_XDECREF(__pyx_t_2);
- __Pyx_XDECREF(__pyx_t_6);
- __Pyx_AddTraceback("View.MemoryView.memoryview.strides.__get__", __pyx_clineno, __pyx_lineno, __pyx_filename);
- __pyx_r = NULL;
- __pyx_L0:;
- __Pyx_XGIVEREF(__pyx_r);
- __Pyx_RefNannyFinishContext();
- return __pyx_r;
-}
-
-/* "View.MemoryView":575
- *
- * @property
- * def suboffsets(self): # <<<<<<<<<<<<<<
- * if self.view.suboffsets == NULL:
- * return (-1,) * self.view.ndim
- */
-
-/* Python wrapper */
-static PyObject *__pyx_pw_15View_dot_MemoryView_10memoryview_10suboffsets_1__get__(PyObject *__pyx_v_self); /*proto*/
-static PyObject *__pyx_pw_15View_dot_MemoryView_10memoryview_10suboffsets_1__get__(PyObject *__pyx_v_self) {
- PyObject *__pyx_r = 0;
- __Pyx_RefNannyDeclarations
- __Pyx_RefNannySetupContext("__get__ (wrapper)", 0);
- __pyx_r = __pyx_pf_15View_dot_MemoryView_10memoryview_10suboffsets___get__(((struct __pyx_memoryview_obj *)__pyx_v_self));
-
- /* function exit code */
- __Pyx_RefNannyFinishContext();
- return __pyx_r;
-}
-
-static PyObject *__pyx_pf_15View_dot_MemoryView_10memoryview_10suboffsets___get__(struct __pyx_memoryview_obj *__pyx_v_self) {
- Py_ssize_t __pyx_v_suboffset;
- PyObject *__pyx_r = NULL;
- __Pyx_RefNannyDeclarations
- int __pyx_t_1;
- PyObject *__pyx_t_2 = NULL;
- PyObject *__pyx_t_3 = NULL;
- Py_ssize_t *__pyx_t_4;
- Py_ssize_t *__pyx_t_5;
- Py_ssize_t *__pyx_t_6;
- int __pyx_lineno = 0;
- const char *__pyx_filename = NULL;
- int __pyx_clineno = 0;
- __Pyx_RefNannySetupContext("__get__", 0);
-
- /* "View.MemoryView":576
- * @property
- * def suboffsets(self):
- * if self.view.suboffsets == NULL: # <<<<<<<<<<<<<<
- * return (-1,) * self.view.ndim
- *
- */
- __pyx_t_1 = ((__pyx_v_self->view.suboffsets == NULL) != 0);
- if (__pyx_t_1) {
-
- /* "View.MemoryView":577
- * def suboffsets(self):
- * if self.view.suboffsets == NULL:
- * return (-1,) * self.view.ndim # <<<<<<<<<<<<<<
- *
- * return tuple([suboffset for suboffset in self.view.suboffsets[:self.view.ndim]])
- */
- __Pyx_XDECREF(__pyx_r);
- __pyx_t_2 = __Pyx_PyInt_From_int(__pyx_v_self->view.ndim); if (unlikely(!__pyx_t_2)) __PYX_ERR(1, 577, __pyx_L1_error)
- __Pyx_GOTREF(__pyx_t_2);
- __pyx_t_3 = PyNumber_Multiply(__pyx_tuple__13, __pyx_t_2); if (unlikely(!__pyx_t_3)) __PYX_ERR(1, 577, __pyx_L1_error)
- __Pyx_GOTREF(__pyx_t_3);
- __Pyx_DECREF(__pyx_t_2); __pyx_t_2 = 0;
- __pyx_r = __pyx_t_3;
- __pyx_t_3 = 0;
- goto __pyx_L0;
-
- /* "View.MemoryView":576
- * @property
- * def suboffsets(self):
- * if self.view.suboffsets == NULL: # <<<<<<<<<<<<<<
- * return (-1,) * self.view.ndim
- *
- */
- }
-
- /* "View.MemoryView":579
- * return (-1,) * self.view.ndim
- *
- * return tuple([suboffset for suboffset in self.view.suboffsets[:self.view.ndim]]) # <<<<<<<<<<<<<<
- *
- * @property
- */
- __Pyx_XDECREF(__pyx_r);
- __pyx_t_3 = PyList_New(0); if (unlikely(!__pyx_t_3)) __PYX_ERR(1, 579, __pyx_L1_error)
- __Pyx_GOTREF(__pyx_t_3);
- __pyx_t_5 = (__pyx_v_self->view.suboffsets + __pyx_v_self->view.ndim);
- for (__pyx_t_6 = __pyx_v_self->view.suboffsets; __pyx_t_6 < __pyx_t_5; __pyx_t_6++) {
- __pyx_t_4 = __pyx_t_6;
- __pyx_v_suboffset = (__pyx_t_4[0]);
- __pyx_t_2 = PyInt_FromSsize_t(__pyx_v_suboffset); if (unlikely(!__pyx_t_2)) __PYX_ERR(1, 579, __pyx_L1_error)
- __Pyx_GOTREF(__pyx_t_2);
- if (unlikely(__Pyx_ListComp_Append(__pyx_t_3, (PyObject*)__pyx_t_2))) __PYX_ERR(1, 579, __pyx_L1_error)
- __Pyx_DECREF(__pyx_t_2); __pyx_t_2 = 0;
- }
- __pyx_t_2 = PyList_AsTuple(((PyObject*)__pyx_t_3)); if (unlikely(!__pyx_t_2)) __PYX_ERR(1, 579, __pyx_L1_error)
- __Pyx_GOTREF(__pyx_t_2);
- __Pyx_DECREF(__pyx_t_3); __pyx_t_3 = 0;
- __pyx_r = __pyx_t_2;
- __pyx_t_2 = 0;
- goto __pyx_L0;
-
- /* "View.MemoryView":575
- *
- * @property
- * def suboffsets(self): # <<<<<<<<<<<<<<
- * if self.view.suboffsets == NULL:
- * return (-1,) * self.view.ndim
- */
-
- /* function exit code */
- __pyx_L1_error:;
- __Pyx_XDECREF(__pyx_t_2);
- __Pyx_XDECREF(__pyx_t_3);
- __Pyx_AddTraceback("View.MemoryView.memoryview.suboffsets.__get__", __pyx_clineno, __pyx_lineno, __pyx_filename);
- __pyx_r = NULL;
- __pyx_L0:;
- __Pyx_XGIVEREF(__pyx_r);
- __Pyx_RefNannyFinishContext();
- return __pyx_r;
-}
-
-/* "View.MemoryView":582
- *
- * @property
- * def ndim(self): # <<<<<<<<<<<<<<
- * return self.view.ndim
- *
- */
-
-/* Python wrapper */
-static PyObject *__pyx_pw_15View_dot_MemoryView_10memoryview_4ndim_1__get__(PyObject *__pyx_v_self); /*proto*/
-static PyObject *__pyx_pw_15View_dot_MemoryView_10memoryview_4ndim_1__get__(PyObject *__pyx_v_self) {
- PyObject *__pyx_r = 0;
- __Pyx_RefNannyDeclarations
- __Pyx_RefNannySetupContext("__get__ (wrapper)", 0);
- __pyx_r = __pyx_pf_15View_dot_MemoryView_10memoryview_4ndim___get__(((struct __pyx_memoryview_obj *)__pyx_v_self));
-
- /* function exit code */
- __Pyx_RefNannyFinishContext();
- return __pyx_r;
-}
-
-static PyObject *__pyx_pf_15View_dot_MemoryView_10memoryview_4ndim___get__(struct __pyx_memoryview_obj *__pyx_v_self) {
- PyObject *__pyx_r = NULL;
- __Pyx_RefNannyDeclarations
- PyObject *__pyx_t_1 = NULL;
- int __pyx_lineno = 0;
- const char *__pyx_filename = NULL;
- int __pyx_clineno = 0;
- __Pyx_RefNannySetupContext("__get__", 0);
-
- /* "View.MemoryView":583
- * @property
- * def ndim(self):
- * return self.view.ndim # <<<<<<<<<<<<<<
- *
- * @property
- */
- __Pyx_XDECREF(__pyx_r);
- __pyx_t_1 = __Pyx_PyInt_From_int(__pyx_v_self->view.ndim); if (unlikely(!__pyx_t_1)) __PYX_ERR(1, 583, __pyx_L1_error)
- __Pyx_GOTREF(__pyx_t_1);
- __pyx_r = __pyx_t_1;
- __pyx_t_1 = 0;
- goto __pyx_L0;
-
- /* "View.MemoryView":582
- *
- * @property
- * def ndim(self): # <<<<<<<<<<<<<<
- * return self.view.ndim
- *
- */
-
- /* function exit code */
- __pyx_L1_error:;
- __Pyx_XDECREF(__pyx_t_1);
- __Pyx_AddTraceback("View.MemoryView.memoryview.ndim.__get__", __pyx_clineno, __pyx_lineno, __pyx_filename);
- __pyx_r = NULL;
- __pyx_L0:;
- __Pyx_XGIVEREF(__pyx_r);
- __Pyx_RefNannyFinishContext();
- return __pyx_r;
-}
-
-/* "View.MemoryView":586
- *
- * @property
- * def itemsize(self): # <<<<<<<<<<<<<<
- * return self.view.itemsize
- *
- */
-
-/* Python wrapper */
-static PyObject *__pyx_pw_15View_dot_MemoryView_10memoryview_8itemsize_1__get__(PyObject *__pyx_v_self); /*proto*/
-static PyObject *__pyx_pw_15View_dot_MemoryView_10memoryview_8itemsize_1__get__(PyObject *__pyx_v_self) {
- PyObject *__pyx_r = 0;
- __Pyx_RefNannyDeclarations
- __Pyx_RefNannySetupContext("__get__ (wrapper)", 0);
- __pyx_r = __pyx_pf_15View_dot_MemoryView_10memoryview_8itemsize___get__(((struct __pyx_memoryview_obj *)__pyx_v_self));
-
- /* function exit code */
- __Pyx_RefNannyFinishContext();
- return __pyx_r;
-}
-
-static PyObject *__pyx_pf_15View_dot_MemoryView_10memoryview_8itemsize___get__(struct __pyx_memoryview_obj *__pyx_v_self) {
- PyObject *__pyx_r = NULL;
- __Pyx_RefNannyDeclarations
- PyObject *__pyx_t_1 = NULL;
- int __pyx_lineno = 0;
- const char *__pyx_filename = NULL;
- int __pyx_clineno = 0;
- __Pyx_RefNannySetupContext("__get__", 0);
-
- /* "View.MemoryView":587
- * @property
- * def itemsize(self):
- * return self.view.itemsize # <<<<<<<<<<<<<<
- *
- * @property
- */
- __Pyx_XDECREF(__pyx_r);
- __pyx_t_1 = PyInt_FromSsize_t(__pyx_v_self->view.itemsize); if (unlikely(!__pyx_t_1)) __PYX_ERR(1, 587, __pyx_L1_error)
- __Pyx_GOTREF(__pyx_t_1);
- __pyx_r = __pyx_t_1;
- __pyx_t_1 = 0;
- goto __pyx_L0;
-
- /* "View.MemoryView":586
- *
- * @property
- * def itemsize(self): # <<<<<<<<<<<<<<
- * return self.view.itemsize
- *
- */
-
- /* function exit code */
- __pyx_L1_error:;
- __Pyx_XDECREF(__pyx_t_1);
- __Pyx_AddTraceback("View.MemoryView.memoryview.itemsize.__get__", __pyx_clineno, __pyx_lineno, __pyx_filename);
- __pyx_r = NULL;
- __pyx_L0:;
- __Pyx_XGIVEREF(__pyx_r);
- __Pyx_RefNannyFinishContext();
- return __pyx_r;
-}
-
-/* "View.MemoryView":590
- *
- * @property
- * def nbytes(self): # <<<<<<<<<<<<<<
- * return self.size * self.view.itemsize
- *
- */
-
-/* Python wrapper */
-static PyObject *__pyx_pw_15View_dot_MemoryView_10memoryview_6nbytes_1__get__(PyObject *__pyx_v_self); /*proto*/
-static PyObject *__pyx_pw_15View_dot_MemoryView_10memoryview_6nbytes_1__get__(PyObject *__pyx_v_self) {
- PyObject *__pyx_r = 0;
- __Pyx_RefNannyDeclarations
- __Pyx_RefNannySetupContext("__get__ (wrapper)", 0);
- __pyx_r = __pyx_pf_15View_dot_MemoryView_10memoryview_6nbytes___get__(((struct __pyx_memoryview_obj *)__pyx_v_self));
-
- /* function exit code */
- __Pyx_RefNannyFinishContext();
- return __pyx_r;
-}
-
-static PyObject *__pyx_pf_15View_dot_MemoryView_10memoryview_6nbytes___get__(struct __pyx_memoryview_obj *__pyx_v_self) {
- PyObject *__pyx_r = NULL;
- __Pyx_RefNannyDeclarations
- PyObject *__pyx_t_1 = NULL;
- PyObject *__pyx_t_2 = NULL;
- PyObject *__pyx_t_3 = NULL;
- int __pyx_lineno = 0;
- const char *__pyx_filename = NULL;
- int __pyx_clineno = 0;
- __Pyx_RefNannySetupContext("__get__", 0);
-
- /* "View.MemoryView":591
- * @property
- * def nbytes(self):
- * return self.size * self.view.itemsize # <<<<<<<<<<<<<<
- *
- * @property
- */
- __Pyx_XDECREF(__pyx_r);
- __pyx_t_1 = __Pyx_PyObject_GetAttrStr(((PyObject *)__pyx_v_self), __pyx_n_s_size); if (unlikely(!__pyx_t_1)) __PYX_ERR(1, 591, __pyx_L1_error)
- __Pyx_GOTREF(__pyx_t_1);
- __pyx_t_2 = PyInt_FromSsize_t(__pyx_v_self->view.itemsize); if (unlikely(!__pyx_t_2)) __PYX_ERR(1, 591, __pyx_L1_error)
- __Pyx_GOTREF(__pyx_t_2);
- __pyx_t_3 = PyNumber_Multiply(__pyx_t_1, __pyx_t_2); if (unlikely(!__pyx_t_3)) __PYX_ERR(1, 591, __pyx_L1_error)
- __Pyx_GOTREF(__pyx_t_3);
- __Pyx_DECREF(__pyx_t_1); __pyx_t_1 = 0;
- __Pyx_DECREF(__pyx_t_2); __pyx_t_2 = 0;
- __pyx_r = __pyx_t_3;
- __pyx_t_3 = 0;
- goto __pyx_L0;
-
- /* "View.MemoryView":590
- *
- * @property
- * def nbytes(self): # <<<<<<<<<<<<<<
- * return self.size * self.view.itemsize
- *
- */
-
- /* function exit code */
- __pyx_L1_error:;
- __Pyx_XDECREF(__pyx_t_1);
- __Pyx_XDECREF(__pyx_t_2);
- __Pyx_XDECREF(__pyx_t_3);
- __Pyx_AddTraceback("View.MemoryView.memoryview.nbytes.__get__", __pyx_clineno, __pyx_lineno, __pyx_filename);
- __pyx_r = NULL;
- __pyx_L0:;
- __Pyx_XGIVEREF(__pyx_r);
- __Pyx_RefNannyFinishContext();
- return __pyx_r;
-}
-
-/* "View.MemoryView":594
- *
- * @property
- * def size(self): # <<<<<<<<<<<<<<
- * if self._size is None:
- * result = 1
- */
-
-/* Python wrapper */
-static PyObject *__pyx_pw_15View_dot_MemoryView_10memoryview_4size_1__get__(PyObject *__pyx_v_self); /*proto*/
-static PyObject *__pyx_pw_15View_dot_MemoryView_10memoryview_4size_1__get__(PyObject *__pyx_v_self) {
- PyObject *__pyx_r = 0;
- __Pyx_RefNannyDeclarations
- __Pyx_RefNannySetupContext("__get__ (wrapper)", 0);
- __pyx_r = __pyx_pf_15View_dot_MemoryView_10memoryview_4size___get__(((struct __pyx_memoryview_obj *)__pyx_v_self));
-
- /* function exit code */
- __Pyx_RefNannyFinishContext();
- return __pyx_r;
-}
-
-static PyObject *__pyx_pf_15View_dot_MemoryView_10memoryview_4size___get__(struct __pyx_memoryview_obj *__pyx_v_self) {
- PyObject *__pyx_v_result = NULL;
- PyObject *__pyx_v_length = NULL;
- PyObject *__pyx_r = NULL;
- __Pyx_RefNannyDeclarations
- int __pyx_t_1;
- int __pyx_t_2;
- Py_ssize_t *__pyx_t_3;
- Py_ssize_t *__pyx_t_4;
- Py_ssize_t *__pyx_t_5;
- PyObject *__pyx_t_6 = NULL;
- int __pyx_lineno = 0;
- const char *__pyx_filename = NULL;
- int __pyx_clineno = 0;
- __Pyx_RefNannySetupContext("__get__", 0);
-
- /* "View.MemoryView":595
- * @property
- * def size(self):
- * if self._size is None: # <<<<<<<<<<<<<<
- * result = 1
- *
- */
- __pyx_t_1 = (__pyx_v_self->_size == Py_None);
- __pyx_t_2 = (__pyx_t_1 != 0);
- if (__pyx_t_2) {
-
- /* "View.MemoryView":596
- * def size(self):
- * if self._size is None:
- * result = 1 # <<<<<<<<<<<<<<
- *
- * for length in self.view.shape[:self.view.ndim]:
- */
- __Pyx_INCREF(__pyx_int_1);
- __pyx_v_result = __pyx_int_1;
-
- /* "View.MemoryView":598
- * result = 1
- *
- * for length in self.view.shape[:self.view.ndim]: # <<<<<<<<<<<<<<
- * result *= length
- *
- */
- __pyx_t_4 = (__pyx_v_self->view.shape + __pyx_v_self->view.ndim);
- for (__pyx_t_5 = __pyx_v_self->view.shape; __pyx_t_5 < __pyx_t_4; __pyx_t_5++) {
- __pyx_t_3 = __pyx_t_5;
- __pyx_t_6 = PyInt_FromSsize_t((__pyx_t_3[0])); if (unlikely(!__pyx_t_6)) __PYX_ERR(1, 598, __pyx_L1_error)
- __Pyx_GOTREF(__pyx_t_6);
- __Pyx_XDECREF_SET(__pyx_v_length, __pyx_t_6);
- __pyx_t_6 = 0;
-
- /* "View.MemoryView":599
- *
- * for length in self.view.shape[:self.view.ndim]:
- * result *= length # <<<<<<<<<<<<<<
- *
- * self._size = result
- */
- __pyx_t_6 = PyNumber_InPlaceMultiply(__pyx_v_result, __pyx_v_length); if (unlikely(!__pyx_t_6)) __PYX_ERR(1, 599, __pyx_L1_error)
- __Pyx_GOTREF(__pyx_t_6);
- __Pyx_DECREF_SET(__pyx_v_result, __pyx_t_6);
- __pyx_t_6 = 0;
- }
-
- /* "View.MemoryView":601
- * result *= length
- *
- * self._size = result # <<<<<<<<<<<<<<
- *
- * return self._size
- */
- __Pyx_INCREF(__pyx_v_result);
- __Pyx_GIVEREF(__pyx_v_result);
- __Pyx_GOTREF(__pyx_v_self->_size);
- __Pyx_DECREF(__pyx_v_self->_size);
- __pyx_v_self->_size = __pyx_v_result;
-
- /* "View.MemoryView":595
- * @property
- * def size(self):
- * if self._size is None: # <<<<<<<<<<<<<<
- * result = 1
- *
- */
- }
-
- /* "View.MemoryView":603
- * self._size = result
- *
- * return self._size # <<<<<<<<<<<<<<
- *
- * def __len__(self):
- */
- __Pyx_XDECREF(__pyx_r);
- __Pyx_INCREF(__pyx_v_self->_size);
- __pyx_r = __pyx_v_self->_size;
- goto __pyx_L0;
-
- /* "View.MemoryView":594
- *
- * @property
- * def size(self): # <<<<<<<<<<<<<<
- * if self._size is None:
- * result = 1
- */
-
- /* function exit code */
- __pyx_L1_error:;
- __Pyx_XDECREF(__pyx_t_6);
- __Pyx_AddTraceback("View.MemoryView.memoryview.size.__get__", __pyx_clineno, __pyx_lineno, __pyx_filename);
- __pyx_r = NULL;
- __pyx_L0:;
- __Pyx_XDECREF(__pyx_v_result);
- __Pyx_XDECREF(__pyx_v_length);
- __Pyx_XGIVEREF(__pyx_r);
- __Pyx_RefNannyFinishContext();
- return __pyx_r;
-}
-
-/* "View.MemoryView":605
- * return self._size
- *
- * def __len__(self): # <<<<<<<<<<<<<<
- * if self.view.ndim >= 1:
- * return self.view.shape[0]
- */
-
-/* Python wrapper */
-static Py_ssize_t __pyx_memoryview___len__(PyObject *__pyx_v_self); /*proto*/
-static Py_ssize_t __pyx_memoryview___len__(PyObject *__pyx_v_self) {
- Py_ssize_t __pyx_r;
- __Pyx_RefNannyDeclarations
- __Pyx_RefNannySetupContext("__len__ (wrapper)", 0);
- __pyx_r = __pyx_memoryview___pyx_pf_15View_dot_MemoryView_10memoryview_10__len__(((struct __pyx_memoryview_obj *)__pyx_v_self));
-
- /* function exit code */
- __Pyx_RefNannyFinishContext();
- return __pyx_r;
-}
-
-static Py_ssize_t __pyx_memoryview___pyx_pf_15View_dot_MemoryView_10memoryview_10__len__(struct __pyx_memoryview_obj *__pyx_v_self) {
- Py_ssize_t __pyx_r;
- __Pyx_RefNannyDeclarations
- int __pyx_t_1;
- __Pyx_RefNannySetupContext("__len__", 0);
-
- /* "View.MemoryView":606
- *
- * def __len__(self):
- * if self.view.ndim >= 1: # <<<<<<<<<<<<<<
- * return self.view.shape[0]
- *
- */
- __pyx_t_1 = ((__pyx_v_self->view.ndim >= 1) != 0);
- if (__pyx_t_1) {
-
- /* "View.MemoryView":607
- * def __len__(self):
- * if self.view.ndim >= 1:
- * return self.view.shape[0] # <<<<<<<<<<<<<<
- *
- * return 0
- */
- __pyx_r = (__pyx_v_self->view.shape[0]);
- goto __pyx_L0;
-
- /* "View.MemoryView":606
- *
- * def __len__(self):
- * if self.view.ndim >= 1: # <<<<<<<<<<<<<<
- * return self.view.shape[0]
- *
- */
- }
-
- /* "View.MemoryView":609
- * return self.view.shape[0]
- *
- * return 0 # <<<<<<<<<<<<<<
- *
- * def __repr__(self):
- */
- __pyx_r = 0;
- goto __pyx_L0;
-
- /* "View.MemoryView":605
- * return self._size
- *
- * def __len__(self): # <<<<<<<<<<<<<<
- * if self.view.ndim >= 1:
- * return self.view.shape[0]
- */
-
- /* function exit code */
- __pyx_L0:;
- __Pyx_RefNannyFinishContext();
- return __pyx_r;
-}
-
-/* "View.MemoryView":611
- * return 0
- *
- * def __repr__(self): # <<<<<<<<<<<<<<
- * return "" % (self.base.__class__.__name__,
- * id(self))
- */
-
-/* Python wrapper */
-static PyObject *__pyx_memoryview___repr__(PyObject *__pyx_v_self); /*proto*/
-static PyObject *__pyx_memoryview___repr__(PyObject *__pyx_v_self) {
- PyObject *__pyx_r = 0;
- __Pyx_RefNannyDeclarations
- __Pyx_RefNannySetupContext("__repr__ (wrapper)", 0);
- __pyx_r = __pyx_memoryview___pyx_pf_15View_dot_MemoryView_10memoryview_12__repr__(((struct __pyx_memoryview_obj *)__pyx_v_self));
-
- /* function exit code */
- __Pyx_RefNannyFinishContext();
- return __pyx_r;
-}
-
-static PyObject *__pyx_memoryview___pyx_pf_15View_dot_MemoryView_10memoryview_12__repr__(struct __pyx_memoryview_obj *__pyx_v_self) {
- PyObject *__pyx_r = NULL;
- __Pyx_RefNannyDeclarations
- PyObject *__pyx_t_1 = NULL;
- PyObject *__pyx_t_2 = NULL;
- PyObject *__pyx_t_3 = NULL;
- int __pyx_lineno = 0;
- const char *__pyx_filename = NULL;
- int __pyx_clineno = 0;
- __Pyx_RefNannySetupContext("__repr__", 0);
-
- /* "View.MemoryView":612
- *
- * def __repr__(self):
- * return "" % (self.base.__class__.__name__, # <<<<<<<<<<<<<<
- * id(self))
- *
- */
- __Pyx_XDECREF(__pyx_r);
- __pyx_t_1 = __Pyx_PyObject_GetAttrStr(((PyObject *)__pyx_v_self), __pyx_n_s_base); if (unlikely(!__pyx_t_1)) __PYX_ERR(1, 612, __pyx_L1_error)
- __Pyx_GOTREF(__pyx_t_1);
- __pyx_t_2 = __Pyx_PyObject_GetAttrStr(__pyx_t_1, __pyx_n_s_class); if (unlikely(!__pyx_t_2)) __PYX_ERR(1, 612, __pyx_L1_error)
- __Pyx_GOTREF(__pyx_t_2);
- __Pyx_DECREF(__pyx_t_1); __pyx_t_1 = 0;
- __pyx_t_1 = __Pyx_PyObject_GetAttrStr(__pyx_t_2, __pyx_n_s_name_2); if (unlikely(!__pyx_t_1)) __PYX_ERR(1, 612, __pyx_L1_error)
- __Pyx_GOTREF(__pyx_t_1);
- __Pyx_DECREF(__pyx_t_2); __pyx_t_2 = 0;
-
- /* "View.MemoryView":613
- * def __repr__(self):
- * return "" % (self.base.__class__.__name__,
- * id(self)) # <<<<<<<<<<<<<<
- *
- * def __str__(self):
- */
- __pyx_t_2 = __Pyx_PyObject_CallOneArg(__pyx_builtin_id, ((PyObject *)__pyx_v_self)); if (unlikely(!__pyx_t_2)) __PYX_ERR(1, 613, __pyx_L1_error)
- __Pyx_GOTREF(__pyx_t_2);
-
- /* "View.MemoryView":612
- *
- * def __repr__(self):
- * return "" % (self.base.__class__.__name__, # <<<<<<<<<<<<<<
- * id(self))
- *
- */
- __pyx_t_3 = PyTuple_New(2); if (unlikely(!__pyx_t_3)) __PYX_ERR(1, 612, __pyx_L1_error)
- __Pyx_GOTREF(__pyx_t_3);
- __Pyx_GIVEREF(__pyx_t_1);
- PyTuple_SET_ITEM(__pyx_t_3, 0, __pyx_t_1);
- __Pyx_GIVEREF(__pyx_t_2);
- PyTuple_SET_ITEM(__pyx_t_3, 1, __pyx_t_2);
- __pyx_t_1 = 0;
- __pyx_t_2 = 0;
- __pyx_t_2 = __Pyx_PyString_Format(__pyx_kp_s_MemoryView_of_r_at_0x_x, __pyx_t_3); if (unlikely(!__pyx_t_2)) __PYX_ERR(1, 612, __pyx_L1_error)
- __Pyx_GOTREF(__pyx_t_2);
- __Pyx_DECREF(__pyx_t_3); __pyx_t_3 = 0;
- __pyx_r = __pyx_t_2;
- __pyx_t_2 = 0;
- goto __pyx_L0;
-
- /* "View.MemoryView":611
- * return 0
- *
- * def __repr__(self): # <<<<<<<<<<<<<<
- * return "" % (self.base.__class__.__name__,
- * id(self))
- */
-
- /* function exit code */
- __pyx_L1_error:;
- __Pyx_XDECREF(__pyx_t_1);
- __Pyx_XDECREF(__pyx_t_2);
- __Pyx_XDECREF(__pyx_t_3);
- __Pyx_AddTraceback("View.MemoryView.memoryview.__repr__", __pyx_clineno, __pyx_lineno, __pyx_filename);
- __pyx_r = NULL;
- __pyx_L0:;
- __Pyx_XGIVEREF(__pyx_r);
- __Pyx_RefNannyFinishContext();
- return __pyx_r;
-}
-
-/* "View.MemoryView":615
- * id(self))
- *
- * def __str__(self): # <<<<<<<<<<<<<<
- * return "" % (self.base.__class__.__name__,)
- *
- */
-
-/* Python wrapper */
-static PyObject *__pyx_memoryview___str__(PyObject *__pyx_v_self); /*proto*/
-static PyObject *__pyx_memoryview___str__(PyObject *__pyx_v_self) {
- PyObject *__pyx_r = 0;
- __Pyx_RefNannyDeclarations
- __Pyx_RefNannySetupContext("__str__ (wrapper)", 0);
- __pyx_r = __pyx_memoryview___pyx_pf_15View_dot_MemoryView_10memoryview_14__str__(((struct __pyx_memoryview_obj *)__pyx_v_self));
-
- /* function exit code */
- __Pyx_RefNannyFinishContext();
- return __pyx_r;
-}
-
-static PyObject *__pyx_memoryview___pyx_pf_15View_dot_MemoryView_10memoryview_14__str__(struct __pyx_memoryview_obj *__pyx_v_self) {
- PyObject *__pyx_r = NULL;
- __Pyx_RefNannyDeclarations
- PyObject *__pyx_t_1 = NULL;
- PyObject *__pyx_t_2 = NULL;
- int __pyx_lineno = 0;
- const char *__pyx_filename = NULL;
- int __pyx_clineno = 0;
- __Pyx_RefNannySetupContext("__str__", 0);
-
- /* "View.MemoryView":616
- *
- * def __str__(self):
- * return "" % (self.base.__class__.__name__,) # <<<<<<<<<<<<<<
- *
- *
- */
- __Pyx_XDECREF(__pyx_r);
- __pyx_t_1 = __Pyx_PyObject_GetAttrStr(((PyObject *)__pyx_v_self), __pyx_n_s_base); if (unlikely(!__pyx_t_1)) __PYX_ERR(1, 616, __pyx_L1_error)
- __Pyx_GOTREF(__pyx_t_1);
- __pyx_t_2 = __Pyx_PyObject_GetAttrStr(__pyx_t_1, __pyx_n_s_class); if (unlikely(!__pyx_t_2)) __PYX_ERR(1, 616, __pyx_L1_error)
- __Pyx_GOTREF(__pyx_t_2);
- __Pyx_DECREF(__pyx_t_1); __pyx_t_1 = 0;
- __pyx_t_1 = __Pyx_PyObject_GetAttrStr(__pyx_t_2, __pyx_n_s_name_2); if (unlikely(!__pyx_t_1)) __PYX_ERR(1, 616, __pyx_L1_error)
- __Pyx_GOTREF(__pyx_t_1);
- __Pyx_DECREF(__pyx_t_2); __pyx_t_2 = 0;
- __pyx_t_2 = PyTuple_New(1); if (unlikely(!__pyx_t_2)) __PYX_ERR(1, 616, __pyx_L1_error)
- __Pyx_GOTREF(__pyx_t_2);
- __Pyx_GIVEREF(__pyx_t_1);
- PyTuple_SET_ITEM(__pyx_t_2, 0, __pyx_t_1);
- __pyx_t_1 = 0;
- __pyx_t_1 = __Pyx_PyString_Format(__pyx_kp_s_MemoryView_of_r_object, __pyx_t_2); if (unlikely(!__pyx_t_1)) __PYX_ERR(1, 616, __pyx_L1_error)
- __Pyx_GOTREF(__pyx_t_1);
- __Pyx_DECREF(__pyx_t_2); __pyx_t_2 = 0;
- __pyx_r = __pyx_t_1;
- __pyx_t_1 = 0;
- goto __pyx_L0;
-
- /* "View.MemoryView":615
- * id(self))
- *
- * def __str__(self): # <<<<<<<<<<<<<<
- * return "" % (self.base.__class__.__name__,)
- *
- */
-
- /* function exit code */
- __pyx_L1_error:;
- __Pyx_XDECREF(__pyx_t_1);
- __Pyx_XDECREF(__pyx_t_2);
- __Pyx_AddTraceback("View.MemoryView.memoryview.__str__", __pyx_clineno, __pyx_lineno, __pyx_filename);
- __pyx_r = NULL;
- __pyx_L0:;
- __Pyx_XGIVEREF(__pyx_r);
- __Pyx_RefNannyFinishContext();
- return __pyx_r;
-}
-
-/* "View.MemoryView":619
- *
- *
- * def is_c_contig(self): # <<<<<<<<<<<<<<
- * cdef __Pyx_memviewslice *mslice
- * cdef __Pyx_memviewslice tmp
- */
-
-/* Python wrapper */
-static PyObject *__pyx_memoryview_is_c_contig(PyObject *__pyx_v_self, CYTHON_UNUSED PyObject *unused); /*proto*/
-static PyObject *__pyx_memoryview_is_c_contig(PyObject *__pyx_v_self, CYTHON_UNUSED PyObject *unused) {
- PyObject *__pyx_r = 0;
- __Pyx_RefNannyDeclarations
- __Pyx_RefNannySetupContext("is_c_contig (wrapper)", 0);
- __pyx_r = __pyx_memoryview___pyx_pf_15View_dot_MemoryView_10memoryview_16is_c_contig(((struct __pyx_memoryview_obj *)__pyx_v_self));
-
- /* function exit code */
- __Pyx_RefNannyFinishContext();
- return __pyx_r;
-}
-
-static PyObject *__pyx_memoryview___pyx_pf_15View_dot_MemoryView_10memoryview_16is_c_contig(struct __pyx_memoryview_obj *__pyx_v_self) {
- __Pyx_memviewslice *__pyx_v_mslice;
- __Pyx_memviewslice __pyx_v_tmp;
- PyObject *__pyx_r = NULL;
- __Pyx_RefNannyDeclarations
- __Pyx_memviewslice *__pyx_t_1;
- PyObject *__pyx_t_2 = NULL;
- int __pyx_lineno = 0;
- const char *__pyx_filename = NULL;
- int __pyx_clineno = 0;
- __Pyx_RefNannySetupContext("is_c_contig", 0);
-
- /* "View.MemoryView":622
- * cdef __Pyx_memviewslice *mslice
- * cdef __Pyx_memviewslice tmp
- * mslice = get_slice_from_memview(self, &tmp) # <<<<<<<<<<<<<<
- * return slice_is_contig(mslice[0], 'C', self.view.ndim)
- *
- */
- __pyx_t_1 = __pyx_memoryview_get_slice_from_memoryview(__pyx_v_self, (&__pyx_v_tmp)); if (unlikely(__pyx_t_1 == ((__Pyx_memviewslice *)NULL))) __PYX_ERR(1, 622, __pyx_L1_error)
- __pyx_v_mslice = __pyx_t_1;
-
- /* "View.MemoryView":623
- * cdef __Pyx_memviewslice tmp
- * mslice = get_slice_from_memview(self, &tmp)
- * return slice_is_contig(mslice[0], 'C', self.view.ndim) # <<<<<<<<<<<<<<
- *
- * def is_f_contig(self):
- */
- __Pyx_XDECREF(__pyx_r);
- __pyx_t_2 = __Pyx_PyBool_FromLong(__pyx_memviewslice_is_contig((__pyx_v_mslice[0]), 'C', __pyx_v_self->view.ndim)); if (unlikely(!__pyx_t_2)) __PYX_ERR(1, 623, __pyx_L1_error)
- __Pyx_GOTREF(__pyx_t_2);
- __pyx_r = __pyx_t_2;
- __pyx_t_2 = 0;
- goto __pyx_L0;
-
- /* "View.MemoryView":619
- *
- *
- * def is_c_contig(self): # <<<<<<<<<<<<<<
- * cdef __Pyx_memviewslice *mslice
- * cdef __Pyx_memviewslice tmp
- */
-
- /* function exit code */
- __pyx_L1_error:;
- __Pyx_XDECREF(__pyx_t_2);
- __Pyx_AddTraceback("View.MemoryView.memoryview.is_c_contig", __pyx_clineno, __pyx_lineno, __pyx_filename);
- __pyx_r = NULL;
- __pyx_L0:;
- __Pyx_XGIVEREF(__pyx_r);
- __Pyx_RefNannyFinishContext();
- return __pyx_r;
-}
-
-/* "View.MemoryView":625
- * return slice_is_contig(mslice[0], 'C', self.view.ndim)
- *
- * def is_f_contig(self): # <<<<<<<<<<<<<<
- * cdef __Pyx_memviewslice *mslice
- * cdef __Pyx_memviewslice tmp
- */
-
-/* Python wrapper */
-static PyObject *__pyx_memoryview_is_f_contig(PyObject *__pyx_v_self, CYTHON_UNUSED PyObject *unused); /*proto*/
-static PyObject *__pyx_memoryview_is_f_contig(PyObject *__pyx_v_self, CYTHON_UNUSED PyObject *unused) {
- PyObject *__pyx_r = 0;
- __Pyx_RefNannyDeclarations
- __Pyx_RefNannySetupContext("is_f_contig (wrapper)", 0);
- __pyx_r = __pyx_memoryview___pyx_pf_15View_dot_MemoryView_10memoryview_18is_f_contig(((struct __pyx_memoryview_obj *)__pyx_v_self));
-
- /* function exit code */
- __Pyx_RefNannyFinishContext();
- return __pyx_r;
-}
-
-static PyObject *__pyx_memoryview___pyx_pf_15View_dot_MemoryView_10memoryview_18is_f_contig(struct __pyx_memoryview_obj *__pyx_v_self) {
- __Pyx_memviewslice *__pyx_v_mslice;
- __Pyx_memviewslice __pyx_v_tmp;
- PyObject *__pyx_r = NULL;
- __Pyx_RefNannyDeclarations
- __Pyx_memviewslice *__pyx_t_1;
- PyObject *__pyx_t_2 = NULL;
- int __pyx_lineno = 0;
- const char *__pyx_filename = NULL;
- int __pyx_clineno = 0;
- __Pyx_RefNannySetupContext("is_f_contig", 0);
-
- /* "View.MemoryView":628
- * cdef __Pyx_memviewslice *mslice
- * cdef __Pyx_memviewslice tmp
- * mslice = get_slice_from_memview(self, &tmp) # <<<<<<<<<<<<<<
- * return slice_is_contig(mslice[0], 'F', self.view.ndim)
- *
- */
- __pyx_t_1 = __pyx_memoryview_get_slice_from_memoryview(__pyx_v_self, (&__pyx_v_tmp)); if (unlikely(__pyx_t_1 == ((__Pyx_memviewslice *)NULL))) __PYX_ERR(1, 628, __pyx_L1_error)
- __pyx_v_mslice = __pyx_t_1;
-
- /* "View.MemoryView":629
- * cdef __Pyx_memviewslice tmp
- * mslice = get_slice_from_memview(self, &tmp)
- * return slice_is_contig(mslice[0], 'F', self.view.ndim) # <<<<<<<<<<<<<<
- *
- * def copy(self):
- */
- __Pyx_XDECREF(__pyx_r);
- __pyx_t_2 = __Pyx_PyBool_FromLong(__pyx_memviewslice_is_contig((__pyx_v_mslice[0]), 'F', __pyx_v_self->view.ndim)); if (unlikely(!__pyx_t_2)) __PYX_ERR(1, 629, __pyx_L1_error)
- __Pyx_GOTREF(__pyx_t_2);
- __pyx_r = __pyx_t_2;
- __pyx_t_2 = 0;
- goto __pyx_L0;
-
- /* "View.MemoryView":625
- * return slice_is_contig(mslice[0], 'C', self.view.ndim)
- *
- * def is_f_contig(self): # <<<<<<<<<<<<<<
- * cdef __Pyx_memviewslice *mslice
- * cdef __Pyx_memviewslice tmp
- */
-
- /* function exit code */
- __pyx_L1_error:;
- __Pyx_XDECREF(__pyx_t_2);
- __Pyx_AddTraceback("View.MemoryView.memoryview.is_f_contig", __pyx_clineno, __pyx_lineno, __pyx_filename);
- __pyx_r = NULL;
- __pyx_L0:;
- __Pyx_XGIVEREF(__pyx_r);
- __Pyx_RefNannyFinishContext();
- return __pyx_r;
-}
-
-/* "View.MemoryView":631
- * return slice_is_contig(mslice[0], 'F', self.view.ndim)
- *
- * def copy(self): # <<<<<<<<<<<<<<
- * cdef __Pyx_memviewslice mslice
- * cdef int flags = self.flags & ~PyBUF_F_CONTIGUOUS
- */
-
-/* Python wrapper */
-static PyObject *__pyx_memoryview_copy(PyObject *__pyx_v_self, CYTHON_UNUSED PyObject *unused); /*proto*/
-static PyObject *__pyx_memoryview_copy(PyObject *__pyx_v_self, CYTHON_UNUSED PyObject *unused) {
- PyObject *__pyx_r = 0;
- __Pyx_RefNannyDeclarations
- __Pyx_RefNannySetupContext("copy (wrapper)", 0);
- __pyx_r = __pyx_memoryview___pyx_pf_15View_dot_MemoryView_10memoryview_20copy(((struct __pyx_memoryview_obj *)__pyx_v_self));
-
- /* function exit code */
- __Pyx_RefNannyFinishContext();
- return __pyx_r;
-}
-
-static PyObject *__pyx_memoryview___pyx_pf_15View_dot_MemoryView_10memoryview_20copy(struct __pyx_memoryview_obj *__pyx_v_self) {
- __Pyx_memviewslice __pyx_v_mslice;
- int __pyx_v_flags;
- PyObject *__pyx_r = NULL;
- __Pyx_RefNannyDeclarations
- __Pyx_memviewslice __pyx_t_1;
- PyObject *__pyx_t_2 = NULL;
- int __pyx_lineno = 0;
- const char *__pyx_filename = NULL;
- int __pyx_clineno = 0;
- __Pyx_RefNannySetupContext("copy", 0);
-
- /* "View.MemoryView":633
- * def copy(self):
- * cdef __Pyx_memviewslice mslice
- * cdef int flags = self.flags & ~PyBUF_F_CONTIGUOUS # <<<<<<<<<<<<<<
- *
- * slice_copy(self, &mslice)
- */
- __pyx_v_flags = (__pyx_v_self->flags & (~PyBUF_F_CONTIGUOUS));
-
- /* "View.MemoryView":635
- * cdef int flags = self.flags & ~PyBUF_F_CONTIGUOUS
- *
- * slice_copy(self, &mslice) # <<<<<<<<<<<<<<
- * mslice = slice_copy_contig(&mslice, "c", self.view.ndim,
- * self.view.itemsize,
- */
- __pyx_memoryview_slice_copy(__pyx_v_self, (&__pyx_v_mslice));
-
- /* "View.MemoryView":636
- *
- * slice_copy(self, &mslice)
- * mslice = slice_copy_contig(&mslice, "c", self.view.ndim, # <<<<<<<<<<<<<<
- * self.view.itemsize,
- * flags|PyBUF_C_CONTIGUOUS,
- */
- __pyx_t_1 = __pyx_memoryview_copy_new_contig((&__pyx_v_mslice), ((char *)"c"), __pyx_v_self->view.ndim, __pyx_v_self->view.itemsize, (__pyx_v_flags | PyBUF_C_CONTIGUOUS), __pyx_v_self->dtype_is_object); if (unlikely(PyErr_Occurred())) __PYX_ERR(1, 636, __pyx_L1_error)
- __pyx_v_mslice = __pyx_t_1;
-
- /* "View.MemoryView":641
- * self.dtype_is_object)
- *
- * return memoryview_copy_from_slice(self, &mslice) # <<<<<<<<<<<<<<
- *
- * def copy_fortran(self):
- */
- __Pyx_XDECREF(__pyx_r);
- __pyx_t_2 = __pyx_memoryview_copy_object_from_slice(__pyx_v_self, (&__pyx_v_mslice)); if (unlikely(!__pyx_t_2)) __PYX_ERR(1, 641, __pyx_L1_error)
- __Pyx_GOTREF(__pyx_t_2);
- __pyx_r = __pyx_t_2;
- __pyx_t_2 = 0;
- goto __pyx_L0;
-
- /* "View.MemoryView":631
- * return slice_is_contig(mslice[0], 'F', self.view.ndim)
- *
- * def copy(self): # <<<<<<<<<<<<<<
- * cdef __Pyx_memviewslice mslice
- * cdef int flags = self.flags & ~PyBUF_F_CONTIGUOUS
- */
-
- /* function exit code */
- __pyx_L1_error:;
- __Pyx_XDECREF(__pyx_t_2);
- __Pyx_AddTraceback("View.MemoryView.memoryview.copy", __pyx_clineno, __pyx_lineno, __pyx_filename);
- __pyx_r = NULL;
- __pyx_L0:;
- __Pyx_XGIVEREF(__pyx_r);
- __Pyx_RefNannyFinishContext();
- return __pyx_r;
-}
-
-/* "View.MemoryView":643
- * return memoryview_copy_from_slice(self, &mslice)
- *
- * def copy_fortran(self): # <<<<<<<<<<<<<<
- * cdef __Pyx_memviewslice src, dst
- * cdef int flags = self.flags & ~PyBUF_C_CONTIGUOUS
- */
-
-/* Python wrapper */
-static PyObject *__pyx_memoryview_copy_fortran(PyObject *__pyx_v_self, CYTHON_UNUSED PyObject *unused); /*proto*/
-static PyObject *__pyx_memoryview_copy_fortran(PyObject *__pyx_v_self, CYTHON_UNUSED PyObject *unused) {
- PyObject *__pyx_r = 0;
- __Pyx_RefNannyDeclarations
- __Pyx_RefNannySetupContext("copy_fortran (wrapper)", 0);
- __pyx_r = __pyx_memoryview___pyx_pf_15View_dot_MemoryView_10memoryview_22copy_fortran(((struct __pyx_memoryview_obj *)__pyx_v_self));
-
- /* function exit code */
- __Pyx_RefNannyFinishContext();
- return __pyx_r;
-}
-
-static PyObject *__pyx_memoryview___pyx_pf_15View_dot_MemoryView_10memoryview_22copy_fortran(struct __pyx_memoryview_obj *__pyx_v_self) {
- __Pyx_memviewslice __pyx_v_src;
- __Pyx_memviewslice __pyx_v_dst;
- int __pyx_v_flags;
- PyObject *__pyx_r = NULL;
- __Pyx_RefNannyDeclarations
- __Pyx_memviewslice __pyx_t_1;
- PyObject *__pyx_t_2 = NULL;
- int __pyx_lineno = 0;
- const char *__pyx_filename = NULL;
- int __pyx_clineno = 0;
- __Pyx_RefNannySetupContext("copy_fortran", 0);
-
- /* "View.MemoryView":645
- * def copy_fortran(self):
- * cdef __Pyx_memviewslice src, dst
- * cdef int flags = self.flags & ~PyBUF_C_CONTIGUOUS # <<<<<<<<<<<<<<
- *
- * slice_copy(self, &src)
- */
- __pyx_v_flags = (__pyx_v_self->flags & (~PyBUF_C_CONTIGUOUS));
-
- /* "View.MemoryView":647
- * cdef int flags = self.flags & ~PyBUF_C_CONTIGUOUS
- *
- * slice_copy(self, &src) # <<<<<<<<<<<<<<
- * dst = slice_copy_contig(&src, "fortran", self.view.ndim,
- * self.view.itemsize,
- */
- __pyx_memoryview_slice_copy(__pyx_v_self, (&__pyx_v_src));
-
- /* "View.MemoryView":648
- *
- * slice_copy(self, &src)
- * dst = slice_copy_contig(&src, "fortran", self.view.ndim, # <<<<<<<<<<<<<<
- * self.view.itemsize,
- * flags|PyBUF_F_CONTIGUOUS,
- */
- __pyx_t_1 = __pyx_memoryview_copy_new_contig((&__pyx_v_src), ((char *)"fortran"), __pyx_v_self->view.ndim, __pyx_v_self->view.itemsize, (__pyx_v_flags | PyBUF_F_CONTIGUOUS), __pyx_v_self->dtype_is_object); if (unlikely(PyErr_Occurred())) __PYX_ERR(1, 648, __pyx_L1_error)
- __pyx_v_dst = __pyx_t_1;
-
- /* "View.MemoryView":653
- * self.dtype_is_object)
- *
- * return memoryview_copy_from_slice(self, &dst) # <<<<<<<<<<<<<<
- *
- *
- */
- __Pyx_XDECREF(__pyx_r);
- __pyx_t_2 = __pyx_memoryview_copy_object_from_slice(__pyx_v_self, (&__pyx_v_dst)); if (unlikely(!__pyx_t_2)) __PYX_ERR(1, 653, __pyx_L1_error)
- __Pyx_GOTREF(__pyx_t_2);
- __pyx_r = __pyx_t_2;
- __pyx_t_2 = 0;
- goto __pyx_L0;
-
- /* "View.MemoryView":643
- * return memoryview_copy_from_slice(self, &mslice)
- *
- * def copy_fortran(self): # <<<<<<<<<<<<<<
- * cdef __Pyx_memviewslice src, dst
- * cdef int flags = self.flags & ~PyBUF_C_CONTIGUOUS
- */
-
- /* function exit code */
- __pyx_L1_error:;
- __Pyx_XDECREF(__pyx_t_2);
- __Pyx_AddTraceback("View.MemoryView.memoryview.copy_fortran", __pyx_clineno, __pyx_lineno, __pyx_filename);
- __pyx_r = NULL;
- __pyx_L0:;
- __Pyx_XGIVEREF(__pyx_r);
- __Pyx_RefNannyFinishContext();
- return __pyx_r;
-}
-
-/* "(tree fragment)":1
- * def __reduce_cython__(self): # <<<<<<<<<<<<<<
- * raise TypeError("no default __reduce__ due to non-trivial __cinit__")
- * def __setstate_cython__(self, __pyx_state):
- */
-
-/* Python wrapper */
-static PyObject *__pyx_pw___pyx_memoryview_1__reduce_cython__(PyObject *__pyx_v_self, CYTHON_UNUSED PyObject *unused); /*proto*/
-static PyObject *__pyx_pw___pyx_memoryview_1__reduce_cython__(PyObject *__pyx_v_self, CYTHON_UNUSED PyObject *unused) {
- PyObject *__pyx_r = 0;
- __Pyx_RefNannyDeclarations
- __Pyx_RefNannySetupContext("__reduce_cython__ (wrapper)", 0);
- __pyx_r = __pyx_pf___pyx_memoryview___reduce_cython__(((struct __pyx_memoryview_obj *)__pyx_v_self));
-
- /* function exit code */
- __Pyx_RefNannyFinishContext();
- return __pyx_r;
-}
-
-static PyObject *__pyx_pf___pyx_memoryview___reduce_cython__(CYTHON_UNUSED struct __pyx_memoryview_obj *__pyx_v_self) {
- PyObject *__pyx_r = NULL;
- __Pyx_RefNannyDeclarations
- PyObject *__pyx_t_1 = NULL;
- int __pyx_lineno = 0;
- const char *__pyx_filename = NULL;
- int __pyx_clineno = 0;
- __Pyx_RefNannySetupContext("__reduce_cython__", 0);
-
- /* "(tree fragment)":2
- * def __reduce_cython__(self):
- * raise TypeError("no default __reduce__ due to non-trivial __cinit__") # <<<<<<<<<<<<<<
- * def __setstate_cython__(self, __pyx_state):
- * raise TypeError("no default __reduce__ due to non-trivial __cinit__")
- */
- __pyx_t_1 = __Pyx_PyObject_Call(__pyx_builtin_TypeError, __pyx_tuple__14, NULL); if (unlikely(!__pyx_t_1)) __PYX_ERR(1, 2, __pyx_L1_error)
- __Pyx_GOTREF(__pyx_t_1);
- __Pyx_Raise(__pyx_t_1, 0, 0, 0);
- __Pyx_DECREF(__pyx_t_1); __pyx_t_1 = 0;
- __PYX_ERR(1, 2, __pyx_L1_error)
-
- /* "(tree fragment)":1
- * def __reduce_cython__(self): # <<<<<<<<<<<<<<
- * raise TypeError("no default __reduce__ due to non-trivial __cinit__")
- * def __setstate_cython__(self, __pyx_state):
- */
-
- /* function exit code */
- __pyx_L1_error:;
- __Pyx_XDECREF(__pyx_t_1);
- __Pyx_AddTraceback("View.MemoryView.memoryview.__reduce_cython__", __pyx_clineno, __pyx_lineno, __pyx_filename);
- __pyx_r = NULL;
- __Pyx_XGIVEREF(__pyx_r);
- __Pyx_RefNannyFinishContext();
- return __pyx_r;
-}
-
-/* "(tree fragment)":3
- * def __reduce_cython__(self):
- * raise TypeError("no default __reduce__ due to non-trivial __cinit__")
- * def __setstate_cython__(self, __pyx_state): # <<<<<<<<<<<<<<
- * raise TypeError("no default __reduce__ due to non-trivial __cinit__")
- */
-
-/* Python wrapper */
-static PyObject *__pyx_pw___pyx_memoryview_3__setstate_cython__(PyObject *__pyx_v_self, PyObject *__pyx_v___pyx_state); /*proto*/
-static PyObject *__pyx_pw___pyx_memoryview_3__setstate_cython__(PyObject *__pyx_v_self, PyObject *__pyx_v___pyx_state) {
- PyObject *__pyx_r = 0;
- __Pyx_RefNannyDeclarations
- __Pyx_RefNannySetupContext("__setstate_cython__ (wrapper)", 0);
- __pyx_r = __pyx_pf___pyx_memoryview_2__setstate_cython__(((struct __pyx_memoryview_obj *)__pyx_v_self), ((PyObject *)__pyx_v___pyx_state));
-
- /* function exit code */
- __Pyx_RefNannyFinishContext();
- return __pyx_r;
-}
-
-static PyObject *__pyx_pf___pyx_memoryview_2__setstate_cython__(CYTHON_UNUSED struct __pyx_memoryview_obj *__pyx_v_self, CYTHON_UNUSED PyObject *__pyx_v___pyx_state) {
- PyObject *__pyx_r = NULL;
- __Pyx_RefNannyDeclarations
- PyObject *__pyx_t_1 = NULL;
- int __pyx_lineno = 0;
- const char *__pyx_filename = NULL;
- int __pyx_clineno = 0;
- __Pyx_RefNannySetupContext("__setstate_cython__", 0);
-
- /* "(tree fragment)":4
- * raise TypeError("no default __reduce__ due to non-trivial __cinit__")
- * def __setstate_cython__(self, __pyx_state):
- * raise TypeError("no default __reduce__ due to non-trivial __cinit__") # <<<<<<<<<<<<<<
- */
- __pyx_t_1 = __Pyx_PyObject_Call(__pyx_builtin_TypeError, __pyx_tuple__15, NULL); if (unlikely(!__pyx_t_1)) __PYX_ERR(1, 4, __pyx_L1_error)
- __Pyx_GOTREF(__pyx_t_1);
- __Pyx_Raise(__pyx_t_1, 0, 0, 0);
- __Pyx_DECREF(__pyx_t_1); __pyx_t_1 = 0;
- __PYX_ERR(1, 4, __pyx_L1_error)
-
- /* "(tree fragment)":3
- * def __reduce_cython__(self):
- * raise TypeError("no default __reduce__ due to non-trivial __cinit__")
- * def __setstate_cython__(self, __pyx_state): # <<<<<<<<<<<<<<
- * raise TypeError("no default __reduce__ due to non-trivial __cinit__")
- */
-
- /* function exit code */
- __pyx_L1_error:;
- __Pyx_XDECREF(__pyx_t_1);
- __Pyx_AddTraceback("View.MemoryView.memoryview.__setstate_cython__", __pyx_clineno, __pyx_lineno, __pyx_filename);
- __pyx_r = NULL;
- __Pyx_XGIVEREF(__pyx_r);
- __Pyx_RefNannyFinishContext();
- return __pyx_r;
-}
-
-/* "View.MemoryView":657
- *
- * @cname('__pyx_memoryview_new')
- * cdef memoryview_cwrapper(object o, int flags, bint dtype_is_object, __Pyx_TypeInfo *typeinfo): # <<<<<<<<<<<<<<
- * cdef memoryview result = memoryview(o, flags, dtype_is_object)
- * result.typeinfo = typeinfo
- */
-
-static PyObject *__pyx_memoryview_new(PyObject *__pyx_v_o, int __pyx_v_flags, int __pyx_v_dtype_is_object, __Pyx_TypeInfo *__pyx_v_typeinfo) {
- struct __pyx_memoryview_obj *__pyx_v_result = 0;
- PyObject *__pyx_r = NULL;
- __Pyx_RefNannyDeclarations
- PyObject *__pyx_t_1 = NULL;
- PyObject *__pyx_t_2 = NULL;
- PyObject *__pyx_t_3 = NULL;
- int __pyx_lineno = 0;
- const char *__pyx_filename = NULL;
- int __pyx_clineno = 0;
- __Pyx_RefNannySetupContext("memoryview_cwrapper", 0);
-
- /* "View.MemoryView":658
- * @cname('__pyx_memoryview_new')
- * cdef memoryview_cwrapper(object o, int flags, bint dtype_is_object, __Pyx_TypeInfo *typeinfo):
- * cdef memoryview result = memoryview(o, flags, dtype_is_object) # <<<<<<<<<<<<<<
- * result.typeinfo = typeinfo
- * return result
- */
- __pyx_t_1 = __Pyx_PyInt_From_int(__pyx_v_flags); if (unlikely(!__pyx_t_1)) __PYX_ERR(1, 658, __pyx_L1_error)
- __Pyx_GOTREF(__pyx_t_1);
- __pyx_t_2 = __Pyx_PyBool_FromLong(__pyx_v_dtype_is_object); if (unlikely(!__pyx_t_2)) __PYX_ERR(1, 658, __pyx_L1_error)
- __Pyx_GOTREF(__pyx_t_2);
- __pyx_t_3 = PyTuple_New(3); if (unlikely(!__pyx_t_3)) __PYX_ERR(1, 658, __pyx_L1_error)
- __Pyx_GOTREF(__pyx_t_3);
- __Pyx_INCREF(__pyx_v_o);
- __Pyx_GIVEREF(__pyx_v_o);
- PyTuple_SET_ITEM(__pyx_t_3, 0, __pyx_v_o);
- __Pyx_GIVEREF(__pyx_t_1);
- PyTuple_SET_ITEM(__pyx_t_3, 1, __pyx_t_1);
- __Pyx_GIVEREF(__pyx_t_2);
- PyTuple_SET_ITEM(__pyx_t_3, 2, __pyx_t_2);
- __pyx_t_1 = 0;
- __pyx_t_2 = 0;
- __pyx_t_2 = __Pyx_PyObject_Call(((PyObject *)__pyx_memoryview_type), __pyx_t_3, NULL); if (unlikely(!__pyx_t_2)) __PYX_ERR(1, 658, __pyx_L1_error)
- __Pyx_GOTREF(__pyx_t_2);
- __Pyx_DECREF(__pyx_t_3); __pyx_t_3 = 0;
- __pyx_v_result = ((struct __pyx_memoryview_obj *)__pyx_t_2);
- __pyx_t_2 = 0;
-
- /* "View.MemoryView":659
- * cdef memoryview_cwrapper(object o, int flags, bint dtype_is_object, __Pyx_TypeInfo *typeinfo):
- * cdef memoryview result = memoryview(o, flags, dtype_is_object)
- * result.typeinfo = typeinfo # <<<<<<<<<<<<<<
- * return result
- *
- */
- __pyx_v_result->typeinfo = __pyx_v_typeinfo;
-
- /* "View.MemoryView":660
- * cdef memoryview result = memoryview(o, flags, dtype_is_object)
- * result.typeinfo = typeinfo
- * return result # <<<<<<<<<<<<<<
- *
- * @cname('__pyx_memoryview_check')
- */
- __Pyx_XDECREF(__pyx_r);
- __Pyx_INCREF(((PyObject *)__pyx_v_result));
- __pyx_r = ((PyObject *)__pyx_v_result);
- goto __pyx_L0;
-
- /* "View.MemoryView":657
- *
- * @cname('__pyx_memoryview_new')
- * cdef memoryview_cwrapper(object o, int flags, bint dtype_is_object, __Pyx_TypeInfo *typeinfo): # <<<<<<<<<<<<<<
- * cdef memoryview result = memoryview(o, flags, dtype_is_object)
- * result.typeinfo = typeinfo
- */
-
- /* function exit code */
- __pyx_L1_error:;
- __Pyx_XDECREF(__pyx_t_1);
- __Pyx_XDECREF(__pyx_t_2);
- __Pyx_XDECREF(__pyx_t_3);
- __Pyx_AddTraceback("View.MemoryView.memoryview_cwrapper", __pyx_clineno, __pyx_lineno, __pyx_filename);
- __pyx_r = 0;
- __pyx_L0:;
- __Pyx_XDECREF((PyObject *)__pyx_v_result);
- __Pyx_XGIVEREF(__pyx_r);
- __Pyx_RefNannyFinishContext();
- return __pyx_r;
-}
-
-/* "View.MemoryView":663
- *
- * @cname('__pyx_memoryview_check')
- * cdef inline bint memoryview_check(object o): # <<<<<<<<<<<<<<
- * return isinstance(o, memoryview)
- *
- */
-
-static CYTHON_INLINE int __pyx_memoryview_check(PyObject *__pyx_v_o) {
- int __pyx_r;
- __Pyx_RefNannyDeclarations
- int __pyx_t_1;
- __Pyx_RefNannySetupContext("memoryview_check", 0);
-
- /* "View.MemoryView":664
- * @cname('__pyx_memoryview_check')
- * cdef inline bint memoryview_check(object o):
- * return isinstance(o, memoryview) # <<<<<<<<<<<<<<
- *
- * cdef tuple _unellipsify(object index, int ndim):
- */
- __pyx_t_1 = __Pyx_TypeCheck(__pyx_v_o, __pyx_memoryview_type);
- __pyx_r = __pyx_t_1;
- goto __pyx_L0;
-
- /* "View.MemoryView":663
- *
- * @cname('__pyx_memoryview_check')
- * cdef inline bint memoryview_check(object o): # <<<<<<<<<<<<<<
- * return isinstance(o, memoryview)
- *
- */
-
- /* function exit code */
- __pyx_L0:;
- __Pyx_RefNannyFinishContext();
- return __pyx_r;
-}
-
-/* "View.MemoryView":666
- * return isinstance(o, memoryview)
- *
- * cdef tuple _unellipsify(object index, int ndim): # <<<<<<<<<<<<<<
- * """
- * Replace all ellipses with full slices and fill incomplete indices with
- */
-
-static PyObject *_unellipsify(PyObject *__pyx_v_index, int __pyx_v_ndim) {
- PyObject *__pyx_v_tup = NULL;
- PyObject *__pyx_v_result = NULL;
- int __pyx_v_have_slices;
- int __pyx_v_seen_ellipsis;
- CYTHON_UNUSED PyObject *__pyx_v_idx = NULL;
- PyObject *__pyx_v_item = NULL;
- Py_ssize_t __pyx_v_nslices;
- PyObject *__pyx_r = NULL;
- __Pyx_RefNannyDeclarations
- int __pyx_t_1;
- int __pyx_t_2;
- PyObject *__pyx_t_3 = NULL;
- PyObject *__pyx_t_4 = NULL;
- Py_ssize_t __pyx_t_5;
- PyObject *(*__pyx_t_6)(PyObject *);
- PyObject *__pyx_t_7 = NULL;
- Py_ssize_t __pyx_t_8;
- int __pyx_t_9;
- int __pyx_t_10;
- PyObject *__pyx_t_11 = NULL;
- int __pyx_lineno = 0;
- const char *__pyx_filename = NULL;
- int __pyx_clineno = 0;
- __Pyx_RefNannySetupContext("_unellipsify", 0);
-
- /* "View.MemoryView":671
- * full slices.
- * """
- * if not isinstance(index, tuple): # <<<<<<<<<<<<<<
- * tup = (index,)
- * else:
- */
- __pyx_t_1 = PyTuple_Check(__pyx_v_index);
- __pyx_t_2 = ((!(__pyx_t_1 != 0)) != 0);
- if (__pyx_t_2) {
-
- /* "View.MemoryView":672
- * """
- * if not isinstance(index, tuple):
- * tup = (index,) # <<<<<<<<<<<<<<
- * else:
- * tup = index
- */
- __pyx_t_3 = PyTuple_New(1); if (unlikely(!__pyx_t_3)) __PYX_ERR(1, 672, __pyx_L1_error)
- __Pyx_GOTREF(__pyx_t_3);
- __Pyx_INCREF(__pyx_v_index);
- __Pyx_GIVEREF(__pyx_v_index);
- PyTuple_SET_ITEM(__pyx_t_3, 0, __pyx_v_index);
- __pyx_v_tup = __pyx_t_3;
- __pyx_t_3 = 0;
-
- /* "View.MemoryView":671
- * full slices.
- * """
- * if not isinstance(index, tuple): # <<<<<<<<<<<<<<
- * tup = (index,)
- * else:
- */
- goto __pyx_L3;
- }
-
- /* "View.MemoryView":674
- * tup = (index,)
- * else:
- * tup = index # <<<<<<<<<<<<<<
- *
- * result = []
- */
- /*else*/ {
- __Pyx_INCREF(__pyx_v_index);
- __pyx_v_tup = __pyx_v_index;
- }
- __pyx_L3:;
-
- /* "View.MemoryView":676
- * tup = index
- *
- * result = [] # <<<<<<<<<<<<<<
- * have_slices = False
- * seen_ellipsis = False
- */
- __pyx_t_3 = PyList_New(0); if (unlikely(!__pyx_t_3)) __PYX_ERR(1, 676, __pyx_L1_error)
- __Pyx_GOTREF(__pyx_t_3);
- __pyx_v_result = ((PyObject*)__pyx_t_3);
- __pyx_t_3 = 0;
-
- /* "View.MemoryView":677
- *
- * result = []
- * have_slices = False # <<<<<<<<<<<<<<
- * seen_ellipsis = False
- * for idx, item in enumerate(tup):
- */
- __pyx_v_have_slices = 0;
-
- /* "View.MemoryView":678
- * result = []
- * have_slices = False
- * seen_ellipsis = False # <<<<<<<<<<<<<<
- * for idx, item in enumerate(tup):
- * if item is Ellipsis:
- */
- __pyx_v_seen_ellipsis = 0;
-
- /* "View.MemoryView":679
- * have_slices = False
- * seen_ellipsis = False
- * for idx, item in enumerate(tup): # <<<<<<<<<<<<<<
- * if item is Ellipsis:
- * if not seen_ellipsis:
- */
- __Pyx_INCREF(__pyx_int_0);
- __pyx_t_3 = __pyx_int_0;
- if (likely(PyList_CheckExact(__pyx_v_tup)) || PyTuple_CheckExact(__pyx_v_tup)) {
- __pyx_t_4 = __pyx_v_tup; __Pyx_INCREF(__pyx_t_4); __pyx_t_5 = 0;
- __pyx_t_6 = NULL;
- } else {
- __pyx_t_5 = -1; __pyx_t_4 = PyObject_GetIter(__pyx_v_tup); if (unlikely(!__pyx_t_4)) __PYX_ERR(1, 679, __pyx_L1_error)
- __Pyx_GOTREF(__pyx_t_4);
- __pyx_t_6 = Py_TYPE(__pyx_t_4)->tp_iternext; if (unlikely(!__pyx_t_6)) __PYX_ERR(1, 679, __pyx_L1_error)
- }
- for (;;) {
- if (likely(!__pyx_t_6)) {
- if (likely(PyList_CheckExact(__pyx_t_4))) {
- if (__pyx_t_5 >= PyList_GET_SIZE(__pyx_t_4)) break;
- #if CYTHON_ASSUME_SAFE_MACROS && !CYTHON_AVOID_BORROWED_REFS
- __pyx_t_7 = PyList_GET_ITEM(__pyx_t_4, __pyx_t_5); __Pyx_INCREF(__pyx_t_7); __pyx_t_5++; if (unlikely(0 < 0)) __PYX_ERR(1, 679, __pyx_L1_error)
- #else
- __pyx_t_7 = PySequence_ITEM(__pyx_t_4, __pyx_t_5); __pyx_t_5++; if (unlikely(!__pyx_t_7)) __PYX_ERR(1, 679, __pyx_L1_error)
- __Pyx_GOTREF(__pyx_t_7);
- #endif
- } else {
- if (__pyx_t_5 >= PyTuple_GET_SIZE(__pyx_t_4)) break;
- #if CYTHON_ASSUME_SAFE_MACROS && !CYTHON_AVOID_BORROWED_REFS
- __pyx_t_7 = PyTuple_GET_ITEM(__pyx_t_4, __pyx_t_5); __Pyx_INCREF(__pyx_t_7); __pyx_t_5++; if (unlikely(0 < 0)) __PYX_ERR(1, 679, __pyx_L1_error)
- #else
- __pyx_t_7 = PySequence_ITEM(__pyx_t_4, __pyx_t_5); __pyx_t_5++; if (unlikely(!__pyx_t_7)) __PYX_ERR(1, 679, __pyx_L1_error)
- __Pyx_GOTREF(__pyx_t_7);
- #endif
- }
- } else {
- __pyx_t_7 = __pyx_t_6(__pyx_t_4);
- if (unlikely(!__pyx_t_7)) {
- PyObject* exc_type = PyErr_Occurred();
- if (exc_type) {
- if (likely(__Pyx_PyErr_GivenExceptionMatches(exc_type, PyExc_StopIteration))) PyErr_Clear();
- else __PYX_ERR(1, 679, __pyx_L1_error)
- }
- break;
- }
- __Pyx_GOTREF(__pyx_t_7);
- }
- __Pyx_XDECREF_SET(__pyx_v_item, __pyx_t_7);
- __pyx_t_7 = 0;
- __Pyx_INCREF(__pyx_t_3);
- __Pyx_XDECREF_SET(__pyx_v_idx, __pyx_t_3);
- __pyx_t_7 = __Pyx_PyInt_AddObjC(__pyx_t_3, __pyx_int_1, 1, 0, 0); if (unlikely(!__pyx_t_7)) __PYX_ERR(1, 679, __pyx_L1_error)
- __Pyx_GOTREF(__pyx_t_7);
- __Pyx_DECREF(__pyx_t_3);
- __pyx_t_3 = __pyx_t_7;
- __pyx_t_7 = 0;
-
- /* "View.MemoryView":680
- * seen_ellipsis = False
- * for idx, item in enumerate(tup):
- * if item is Ellipsis: # <<<<<<<<<<<<<<
- * if not seen_ellipsis:
- * result.extend([slice(None)] * (ndim - len(tup) + 1))
- */
- __pyx_t_2 = (__pyx_v_item == __pyx_builtin_Ellipsis);
- __pyx_t_1 = (__pyx_t_2 != 0);
- if (__pyx_t_1) {
-
- /* "View.MemoryView":681
- * for idx, item in enumerate(tup):
- * if item is Ellipsis:
- * if not seen_ellipsis: # <<<<<<<<<<<<<<
- * result.extend([slice(None)] * (ndim - len(tup) + 1))
- * seen_ellipsis = True
- */
- __pyx_t_1 = ((!(__pyx_v_seen_ellipsis != 0)) != 0);
- if (__pyx_t_1) {
-
- /* "View.MemoryView":682
- * if item is Ellipsis:
- * if not seen_ellipsis:
- * result.extend([slice(None)] * (ndim - len(tup) + 1)) # <<<<<<<<<<<<<<
- * seen_ellipsis = True
- * else:
- */
- __pyx_t_8 = PyObject_Length(__pyx_v_tup); if (unlikely(__pyx_t_8 == ((Py_ssize_t)-1))) __PYX_ERR(1, 682, __pyx_L1_error)
- __pyx_t_7 = PyList_New(1 * ((((__pyx_v_ndim - __pyx_t_8) + 1)<0) ? 0:((__pyx_v_ndim - __pyx_t_8) + 1))); if (unlikely(!__pyx_t_7)) __PYX_ERR(1, 682, __pyx_L1_error)
- __Pyx_GOTREF(__pyx_t_7);
- { Py_ssize_t __pyx_temp;
- for (__pyx_temp=0; __pyx_temp < ((__pyx_v_ndim - __pyx_t_8) + 1); __pyx_temp++) {
- __Pyx_INCREF(__pyx_slice__16);
- __Pyx_GIVEREF(__pyx_slice__16);
- PyList_SET_ITEM(__pyx_t_7, __pyx_temp, __pyx_slice__16);
- }
- }
- __pyx_t_9 = __Pyx_PyList_Extend(__pyx_v_result, __pyx_t_7); if (unlikely(__pyx_t_9 == ((int)-1))) __PYX_ERR(1, 682, __pyx_L1_error)
- __Pyx_DECREF(__pyx_t_7); __pyx_t_7 = 0;
-
- /* "View.MemoryView":683
- * if not seen_ellipsis:
- * result.extend([slice(None)] * (ndim - len(tup) + 1))
- * seen_ellipsis = True # <<<<<<<<<<<<<<
- * else:
- * result.append(slice(None))
- */
- __pyx_v_seen_ellipsis = 1;
-
- /* "View.MemoryView":681
- * for idx, item in enumerate(tup):
- * if item is Ellipsis:
- * if not seen_ellipsis: # <<<<<<<<<<<<<<
- * result.extend([slice(None)] * (ndim - len(tup) + 1))
- * seen_ellipsis = True
- */
- goto __pyx_L7;
- }
-
- /* "View.MemoryView":685
- * seen_ellipsis = True
- * else:
- * result.append(slice(None)) # <<<<<<<<<<<<<<
- * have_slices = True
- * else:
- */
- /*else*/ {
- __pyx_t_9 = __Pyx_PyList_Append(__pyx_v_result, __pyx_slice__16); if (unlikely(__pyx_t_9 == ((int)-1))) __PYX_ERR(1, 685, __pyx_L1_error)
- }
- __pyx_L7:;
-
- /* "View.MemoryView":686
- * else:
- * result.append(slice(None))
- * have_slices = True # <<<<<<<<<<<<<<
- * else:
- * if not isinstance(item, slice) and not PyIndex_Check(item):
- */
- __pyx_v_have_slices = 1;
-
- /* "View.MemoryView":680
- * seen_ellipsis = False
- * for idx, item in enumerate(tup):
- * if item is Ellipsis: # <<<<<<<<<<<<<<
- * if not seen_ellipsis:
- * result.extend([slice(None)] * (ndim - len(tup) + 1))
- */
- goto __pyx_L6;
- }
-
- /* "View.MemoryView":688
- * have_slices = True
- * else:
- * if not isinstance(item, slice) and not PyIndex_Check(item): # <<<<<<<<<<<<<<
- * raise TypeError("Cannot index with type '%s'" % type(item))
- *
- */
- /*else*/ {
- __pyx_t_2 = PySlice_Check(__pyx_v_item);
- __pyx_t_10 = ((!(__pyx_t_2 != 0)) != 0);
- if (__pyx_t_10) {
- } else {
- __pyx_t_1 = __pyx_t_10;
- goto __pyx_L9_bool_binop_done;
- }
- __pyx_t_10 = ((!(PyIndex_Check(__pyx_v_item) != 0)) != 0);
- __pyx_t_1 = __pyx_t_10;
- __pyx_L9_bool_binop_done:;
- if (unlikely(__pyx_t_1)) {
-
- /* "View.MemoryView":689
- * else:
- * if not isinstance(item, slice) and not PyIndex_Check(item):
- * raise TypeError("Cannot index with type '%s'" % type(item)) # <<<<<<<<<<<<<<
- *
- * have_slices = have_slices or isinstance(item, slice)
- */
- __pyx_t_7 = __Pyx_PyString_FormatSafe(__pyx_kp_s_Cannot_index_with_type_s, ((PyObject *)Py_TYPE(__pyx_v_item))); if (unlikely(!__pyx_t_7)) __PYX_ERR(1, 689, __pyx_L1_error)
- __Pyx_GOTREF(__pyx_t_7);
- __pyx_t_11 = __Pyx_PyObject_CallOneArg(__pyx_builtin_TypeError, __pyx_t_7); if (unlikely(!__pyx_t_11)) __PYX_ERR(1, 689, __pyx_L1_error)
- __Pyx_GOTREF(__pyx_t_11);
- __Pyx_DECREF(__pyx_t_7); __pyx_t_7 = 0;
- __Pyx_Raise(__pyx_t_11, 0, 0, 0);
- __Pyx_DECREF(__pyx_t_11); __pyx_t_11 = 0;
- __PYX_ERR(1, 689, __pyx_L1_error)
-
- /* "View.MemoryView":688
- * have_slices = True
- * else:
- * if not isinstance(item, slice) and not PyIndex_Check(item): # <<<<<<<<<<<<<<
- * raise TypeError("Cannot index with type '%s'" % type(item))
- *
- */
- }
-
- /* "View.MemoryView":691
- * raise TypeError("Cannot index with type '%s'" % type(item))
- *
- * have_slices = have_slices or isinstance(item, slice) # <<<<<<<<<<<<<<
- * result.append(item)
- *
- */
- __pyx_t_10 = (__pyx_v_have_slices != 0);
- if (!__pyx_t_10) {
- } else {
- __pyx_t_1 = __pyx_t_10;
- goto __pyx_L11_bool_binop_done;
- }
- __pyx_t_10 = PySlice_Check(__pyx_v_item);
- __pyx_t_2 = (__pyx_t_10 != 0);
- __pyx_t_1 = __pyx_t_2;
- __pyx_L11_bool_binop_done:;
- __pyx_v_have_slices = __pyx_t_1;
-
- /* "View.MemoryView":692
- *
- * have_slices = have_slices or isinstance(item, slice)
- * result.append(item) # <<<<<<<<<<<<<<
- *
- * nslices = ndim - len(result)
- */
- __pyx_t_9 = __Pyx_PyList_Append(__pyx_v_result, __pyx_v_item); if (unlikely(__pyx_t_9 == ((int)-1))) __PYX_ERR(1, 692, __pyx_L1_error)
- }
- __pyx_L6:;
-
- /* "View.MemoryView":679
- * have_slices = False
- * seen_ellipsis = False
- * for idx, item in enumerate(tup): # <<<<<<<<<<<<<<
- * if item is Ellipsis:
- * if not seen_ellipsis:
- */
- }
- __Pyx_DECREF(__pyx_t_4); __pyx_t_4 = 0;
- __Pyx_DECREF(__pyx_t_3); __pyx_t_3 = 0;
-
- /* "View.MemoryView":694
- * result.append(item)
- *
- * nslices = ndim - len(result) # <<<<<<<<<<<<<<
- * if nslices:
- * result.extend([slice(None)] * nslices)
- */
- __pyx_t_5 = PyList_GET_SIZE(__pyx_v_result); if (unlikely(__pyx_t_5 == ((Py_ssize_t)-1))) __PYX_ERR(1, 694, __pyx_L1_error)
- __pyx_v_nslices = (__pyx_v_ndim - __pyx_t_5);
-
- /* "View.MemoryView":695
- *
- * nslices = ndim - len(result)
- * if nslices: # <<<<<<<<<<<<<<
- * result.extend([slice(None)] * nslices)
- *
- */
- __pyx_t_1 = (__pyx_v_nslices != 0);
- if (__pyx_t_1) {
-
- /* "View.MemoryView":696
- * nslices = ndim - len(result)
- * if nslices:
- * result.extend([slice(None)] * nslices) # <<<<<<<<<<<<<<
- *
- * return have_slices or nslices, tuple(result)
- */
- __pyx_t_3 = PyList_New(1 * ((__pyx_v_nslices<0) ? 0:__pyx_v_nslices)); if (unlikely(!__pyx_t_3)) __PYX_ERR(1, 696, __pyx_L1_error)
- __Pyx_GOTREF(__pyx_t_3);
- { Py_ssize_t __pyx_temp;
- for (__pyx_temp=0; __pyx_temp < __pyx_v_nslices; __pyx_temp++) {
- __Pyx_INCREF(__pyx_slice__16);
- __Pyx_GIVEREF(__pyx_slice__16);
- PyList_SET_ITEM(__pyx_t_3, __pyx_temp, __pyx_slice__16);
- }
- }
- __pyx_t_9 = __Pyx_PyList_Extend(__pyx_v_result, __pyx_t_3); if (unlikely(__pyx_t_9 == ((int)-1))) __PYX_ERR(1, 696, __pyx_L1_error)
- __Pyx_DECREF(__pyx_t_3); __pyx_t_3 = 0;
-
- /* "View.MemoryView":695
- *
- * nslices = ndim - len(result)
- * if nslices: # <<<<<<<<<<<<<<
- * result.extend([slice(None)] * nslices)
- *
- */
- }
-
- /* "View.MemoryView":698
- * result.extend([slice(None)] * nslices)
- *
- * return have_slices or nslices, tuple(result) # <<<<<<<<<<<<<<
- *
- * cdef assert_direct_dimensions(Py_ssize_t *suboffsets, int ndim):
- */
- __Pyx_XDECREF(__pyx_r);
- if (!__pyx_v_have_slices) {
- } else {
- __pyx_t_4 = __Pyx_PyBool_FromLong(__pyx_v_have_slices); if (unlikely(!__pyx_t_4)) __PYX_ERR(1, 698, __pyx_L1_error)
- __Pyx_GOTREF(__pyx_t_4);
- __pyx_t_3 = __pyx_t_4;
- __pyx_t_4 = 0;
- goto __pyx_L14_bool_binop_done;
- }
- __pyx_t_4 = PyInt_FromSsize_t(__pyx_v_nslices); if (unlikely(!__pyx_t_4)) __PYX_ERR(1, 698, __pyx_L1_error)
- __Pyx_GOTREF(__pyx_t_4);
- __pyx_t_3 = __pyx_t_4;
- __pyx_t_4 = 0;
- __pyx_L14_bool_binop_done:;
- __pyx_t_4 = PyList_AsTuple(__pyx_v_result); if (unlikely(!__pyx_t_4)) __PYX_ERR(1, 698, __pyx_L1_error)
- __Pyx_GOTREF(__pyx_t_4);
- __pyx_t_11 = PyTuple_New(2); if (unlikely(!__pyx_t_11)) __PYX_ERR(1, 698, __pyx_L1_error)
- __Pyx_GOTREF(__pyx_t_11);
- __Pyx_GIVEREF(__pyx_t_3);
- PyTuple_SET_ITEM(__pyx_t_11, 0, __pyx_t_3);
- __Pyx_GIVEREF(__pyx_t_4);
- PyTuple_SET_ITEM(__pyx_t_11, 1, __pyx_t_4);
- __pyx_t_3 = 0;
- __pyx_t_4 = 0;
- __pyx_r = ((PyObject*)__pyx_t_11);
- __pyx_t_11 = 0;
- goto __pyx_L0;
-
- /* "View.MemoryView":666
- * return isinstance(o, memoryview)
- *
- * cdef tuple _unellipsify(object index, int ndim): # <<<<<<<<<<<<<<
- * """
- * Replace all ellipses with full slices and fill incomplete indices with
- */
-
- /* function exit code */
- __pyx_L1_error:;
- __Pyx_XDECREF(__pyx_t_3);
- __Pyx_XDECREF(__pyx_t_4);
- __Pyx_XDECREF(__pyx_t_7);
- __Pyx_XDECREF(__pyx_t_11);
- __Pyx_AddTraceback("View.MemoryView._unellipsify", __pyx_clineno, __pyx_lineno, __pyx_filename);
- __pyx_r = 0;
- __pyx_L0:;
- __Pyx_XDECREF(__pyx_v_tup);
- __Pyx_XDECREF(__pyx_v_result);
- __Pyx_XDECREF(__pyx_v_idx);
- __Pyx_XDECREF(__pyx_v_item);
- __Pyx_XGIVEREF(__pyx_r);
- __Pyx_RefNannyFinishContext();
- return __pyx_r;
-}
-
-/* "View.MemoryView":700
- * return have_slices or nslices, tuple(result)
- *
- * cdef assert_direct_dimensions(Py_ssize_t *suboffsets, int ndim): # <<<<<<<<<<<<<<
- * for suboffset in suboffsets[:ndim]:
- * if suboffset >= 0:
- */
-
-static PyObject *assert_direct_dimensions(Py_ssize_t *__pyx_v_suboffsets, int __pyx_v_ndim) {
- Py_ssize_t __pyx_v_suboffset;
- PyObject *__pyx_r = NULL;
- __Pyx_RefNannyDeclarations
- Py_ssize_t *__pyx_t_1;
- Py_ssize_t *__pyx_t_2;
- Py_ssize_t *__pyx_t_3;
- int __pyx_t_4;
- PyObject *__pyx_t_5 = NULL;
- int __pyx_lineno = 0;
- const char *__pyx_filename = NULL;
- int __pyx_clineno = 0;
- __Pyx_RefNannySetupContext("assert_direct_dimensions", 0);
-
- /* "View.MemoryView":701
- *
- * cdef assert_direct_dimensions(Py_ssize_t *suboffsets, int ndim):
- * for suboffset in suboffsets[:ndim]: # <<<<<<<<<<<<<<
- * if suboffset >= 0:
- * raise ValueError("Indirect dimensions not supported")
- */
- __pyx_t_2 = (__pyx_v_suboffsets + __pyx_v_ndim);
- for (__pyx_t_3 = __pyx_v_suboffsets; __pyx_t_3 < __pyx_t_2; __pyx_t_3++) {
- __pyx_t_1 = __pyx_t_3;
- __pyx_v_suboffset = (__pyx_t_1[0]);
-
- /* "View.MemoryView":702
- * cdef assert_direct_dimensions(Py_ssize_t *suboffsets, int ndim):
- * for suboffset in suboffsets[:ndim]:
- * if suboffset >= 0: # <<<<<<<<<<<<<<
- * raise ValueError("Indirect dimensions not supported")
- *
- */
- __pyx_t_4 = ((__pyx_v_suboffset >= 0) != 0);
- if (unlikely(__pyx_t_4)) {
-
- /* "View.MemoryView":703
- * for suboffset in suboffsets[:ndim]:
- * if suboffset >= 0:
- * raise ValueError("Indirect dimensions not supported") # <<<<<<<<<<<<<<
- *
- *
- */
- __pyx_t_5 = __Pyx_PyObject_Call(__pyx_builtin_ValueError, __pyx_tuple__17, NULL); if (unlikely(!__pyx_t_5)) __PYX_ERR(1, 703, __pyx_L1_error)
- __Pyx_GOTREF(__pyx_t_5);
- __Pyx_Raise(__pyx_t_5, 0, 0, 0);
- __Pyx_DECREF(__pyx_t_5); __pyx_t_5 = 0;
- __PYX_ERR(1, 703, __pyx_L1_error)
-
- /* "View.MemoryView":702
- * cdef assert_direct_dimensions(Py_ssize_t *suboffsets, int ndim):
- * for suboffset in suboffsets[:ndim]:
- * if suboffset >= 0: # <<<<<<<<<<<<<<
- * raise ValueError("Indirect dimensions not supported")
- *
- */
- }
- }
-
- /* "View.MemoryView":700
- * return have_slices or nslices, tuple(result)
- *
- * cdef assert_direct_dimensions(Py_ssize_t *suboffsets, int ndim): # <<<<<<<<<<<<<<
- * for suboffset in suboffsets[:ndim]:
- * if suboffset >= 0:
- */
-
- /* function exit code */
- __pyx_r = Py_None; __Pyx_INCREF(Py_None);
- goto __pyx_L0;
- __pyx_L1_error:;
- __Pyx_XDECREF(__pyx_t_5);
- __Pyx_AddTraceback("View.MemoryView.assert_direct_dimensions", __pyx_clineno, __pyx_lineno, __pyx_filename);
- __pyx_r = 0;
- __pyx_L0:;
- __Pyx_XGIVEREF(__pyx_r);
- __Pyx_RefNannyFinishContext();
- return __pyx_r;
-}
-
-/* "View.MemoryView":710
- *
- * @cname('__pyx_memview_slice')
- * cdef memoryview memview_slice(memoryview memview, object indices): # <<<<<<<<<<<<<<
- * cdef int new_ndim = 0, suboffset_dim = -1, dim
- * cdef bint negative_step
- */
-
-static struct __pyx_memoryview_obj *__pyx_memview_slice(struct __pyx_memoryview_obj *__pyx_v_memview, PyObject *__pyx_v_indices) {
- int __pyx_v_new_ndim;
- int __pyx_v_suboffset_dim;
- int __pyx_v_dim;
- __Pyx_memviewslice __pyx_v_src;
- __Pyx_memviewslice __pyx_v_dst;
- __Pyx_memviewslice *__pyx_v_p_src;
- struct __pyx_memoryviewslice_obj *__pyx_v_memviewsliceobj = 0;
- __Pyx_memviewslice *__pyx_v_p_dst;
- int *__pyx_v_p_suboffset_dim;
- Py_ssize_t __pyx_v_start;
- Py_ssize_t __pyx_v_stop;
- Py_ssize_t __pyx_v_step;
- int __pyx_v_have_start;
- int __pyx_v_have_stop;
- int __pyx_v_have_step;
- PyObject *__pyx_v_index = NULL;
- struct __pyx_memoryview_obj *__pyx_r = NULL;
- __Pyx_RefNannyDeclarations
- int __pyx_t_1;
- int __pyx_t_2;
- PyObject *__pyx_t_3 = NULL;
- struct __pyx_memoryview_obj *__pyx_t_4;
- char *__pyx_t_5;
- int __pyx_t_6;
- Py_ssize_t __pyx_t_7;
- PyObject *(*__pyx_t_8)(PyObject *);
- PyObject *__pyx_t_9 = NULL;
- Py_ssize_t __pyx_t_10;
- int __pyx_t_11;
- Py_ssize_t __pyx_t_12;
- int __pyx_lineno = 0;
- const char *__pyx_filename = NULL;
- int __pyx_clineno = 0;
- __Pyx_RefNannySetupContext("memview_slice", 0);
-
- /* "View.MemoryView":711
- * @cname('__pyx_memview_slice')
- * cdef memoryview memview_slice(memoryview memview, object indices):
- * cdef int new_ndim = 0, suboffset_dim = -1, dim # <<<<<<<<<<<<<<
- * cdef bint negative_step
- * cdef __Pyx_memviewslice src, dst
- */
- __pyx_v_new_ndim = 0;
- __pyx_v_suboffset_dim = -1;
-
- /* "View.MemoryView":718
- *
- *
- * memset(&dst, 0, sizeof(dst)) # <<<<<<<<<<<<<<
- *
- * cdef _memoryviewslice memviewsliceobj
- */
- (void)(memset((&__pyx_v_dst), 0, (sizeof(__pyx_v_dst))));
-
- /* "View.MemoryView":722
- * cdef _memoryviewslice memviewsliceobj
- *
- * assert memview.view.ndim > 0 # <<<<<<<<<<<<<<
- *
- * if isinstance(memview, _memoryviewslice):
- */
- #ifndef CYTHON_WITHOUT_ASSERTIONS
- if (unlikely(!Py_OptimizeFlag)) {
- if (unlikely(!((__pyx_v_memview->view.ndim > 0) != 0))) {
- PyErr_SetNone(PyExc_AssertionError);
- __PYX_ERR(1, 722, __pyx_L1_error)
- }
- }
- #endif
-
- /* "View.MemoryView":724
- * assert memview.view.ndim > 0
- *
- * if isinstance(memview, _memoryviewslice): # <<<<<<<<<<<<<<
- * memviewsliceobj = memview
- * p_src = &memviewsliceobj.from_slice
- */
- __pyx_t_1 = __Pyx_TypeCheck(((PyObject *)__pyx_v_memview), __pyx_memoryviewslice_type);
- __pyx_t_2 = (__pyx_t_1 != 0);
- if (__pyx_t_2) {
-
- /* "View.MemoryView":725
- *
- * if isinstance(memview, _memoryviewslice):
- * memviewsliceobj = memview # <<<<<<<<<<<<<<
- * p_src = &memviewsliceobj.from_slice
- * else:
- */
- if (!(likely(((((PyObject *)__pyx_v_memview)) == Py_None) || likely(__Pyx_TypeTest(((PyObject *)__pyx_v_memview), __pyx_memoryviewslice_type))))) __PYX_ERR(1, 725, __pyx_L1_error)
- __pyx_t_3 = ((PyObject *)__pyx_v_memview);
- __Pyx_INCREF(__pyx_t_3);
- __pyx_v_memviewsliceobj = ((struct __pyx_memoryviewslice_obj *)__pyx_t_3);
- __pyx_t_3 = 0;
-
- /* "View.MemoryView":726
- * if isinstance(memview, _memoryviewslice):
- * memviewsliceobj = memview
- * p_src = &memviewsliceobj.from_slice # <<<<<<<<<<<<<<
- * else:
- * slice_copy(memview, &src)
- */
- __pyx_v_p_src = (&__pyx_v_memviewsliceobj->from_slice);
-
- /* "View.MemoryView":724
- * assert memview.view.ndim > 0
- *
- * if isinstance(memview, _memoryviewslice): # <<<<<<<<<<<<<<
- * memviewsliceobj = memview
- * p_src = &memviewsliceobj.from_slice
- */
- goto __pyx_L3;
- }
-
- /* "View.MemoryView":728
- * p_src = &memviewsliceobj.from_slice
- * else:
- * slice_copy(memview, &src) # <<<<<<<<<<<<<<
- * p_src = &src
- *
- */
- /*else*/ {
- __pyx_memoryview_slice_copy(__pyx_v_memview, (&__pyx_v_src));
-
- /* "View.MemoryView":729
- * else:
- * slice_copy(memview, &src)
- * p_src = &src # <<<<<<<<<<<<<<
- *
- *
- */
- __pyx_v_p_src = (&__pyx_v_src);
- }
- __pyx_L3:;
-
- /* "View.MemoryView":735
- *
- *
- * dst.memview = p_src.memview # <<<<<<<<<<<<<<
- * dst.data = p_src.data
- *
- */
- __pyx_t_4 = __pyx_v_p_src->memview;
- __pyx_v_dst.memview = __pyx_t_4;
-
- /* "View.MemoryView":736
- *
- * dst.memview = p_src.memview
- * dst.data = p_src.data # <<<<<<<<<<<<<<
- *
- *
- */
- __pyx_t_5 = __pyx_v_p_src->data;
- __pyx_v_dst.data = __pyx_t_5;
-
- /* "View.MemoryView":741
- *
- *
- * cdef __Pyx_memviewslice *p_dst = &dst # <<<<<<<<<<<<<<
- * cdef int *p_suboffset_dim = &suboffset_dim
- * cdef Py_ssize_t start, stop, step
- */
- __pyx_v_p_dst = (&__pyx_v_dst);
-
- /* "View.MemoryView":742
- *
- * cdef __Pyx_memviewslice *p_dst = &dst
- * cdef int *p_suboffset_dim = &suboffset_dim # <<<<<<<<<<<<<<
- * cdef Py_ssize_t start, stop, step
- * cdef bint have_start, have_stop, have_step
- */
- __pyx_v_p_suboffset_dim = (&__pyx_v_suboffset_dim);
-
- /* "View.MemoryView":746
- * cdef bint have_start, have_stop, have_step
- *
- * for dim, index in enumerate(indices): # <<<<<<<<<<<<<<
- * if PyIndex_Check(index):
- * slice_memviewslice(
- */
- __pyx_t_6 = 0;
- if (likely(PyList_CheckExact(__pyx_v_indices)) || PyTuple_CheckExact(__pyx_v_indices)) {
- __pyx_t_3 = __pyx_v_indices; __Pyx_INCREF(__pyx_t_3); __pyx_t_7 = 0;
- __pyx_t_8 = NULL;
- } else {
- __pyx_t_7 = -1; __pyx_t_3 = PyObject_GetIter(__pyx_v_indices); if (unlikely(!__pyx_t_3)) __PYX_ERR(1, 746, __pyx_L1_error)
- __Pyx_GOTREF(__pyx_t_3);
- __pyx_t_8 = Py_TYPE(__pyx_t_3)->tp_iternext; if (unlikely(!__pyx_t_8)) __PYX_ERR(1, 746, __pyx_L1_error)
- }
- for (;;) {
- if (likely(!__pyx_t_8)) {
- if (likely(PyList_CheckExact(__pyx_t_3))) {
- if (__pyx_t_7 >= PyList_GET_SIZE(__pyx_t_3)) break;
- #if CYTHON_ASSUME_SAFE_MACROS && !CYTHON_AVOID_BORROWED_REFS
- __pyx_t_9 = PyList_GET_ITEM(__pyx_t_3, __pyx_t_7); __Pyx_INCREF(__pyx_t_9); __pyx_t_7++; if (unlikely(0 < 0)) __PYX_ERR(1, 746, __pyx_L1_error)
- #else
- __pyx_t_9 = PySequence_ITEM(__pyx_t_3, __pyx_t_7); __pyx_t_7++; if (unlikely(!__pyx_t_9)) __PYX_ERR(1, 746, __pyx_L1_error)
- __Pyx_GOTREF(__pyx_t_9);
- #endif
- } else {
- if (__pyx_t_7 >= PyTuple_GET_SIZE(__pyx_t_3)) break;
- #if CYTHON_ASSUME_SAFE_MACROS && !CYTHON_AVOID_BORROWED_REFS
- __pyx_t_9 = PyTuple_GET_ITEM(__pyx_t_3, __pyx_t_7); __Pyx_INCREF(__pyx_t_9); __pyx_t_7++; if (unlikely(0 < 0)) __PYX_ERR(1, 746, __pyx_L1_error)
- #else
- __pyx_t_9 = PySequence_ITEM(__pyx_t_3, __pyx_t_7); __pyx_t_7++; if (unlikely(!__pyx_t_9)) __PYX_ERR(1, 746, __pyx_L1_error)
- __Pyx_GOTREF(__pyx_t_9);
- #endif
- }
- } else {
- __pyx_t_9 = __pyx_t_8(__pyx_t_3);
- if (unlikely(!__pyx_t_9)) {
- PyObject* exc_type = PyErr_Occurred();
- if (exc_type) {
- if (likely(__Pyx_PyErr_GivenExceptionMatches(exc_type, PyExc_StopIteration))) PyErr_Clear();
- else __PYX_ERR(1, 746, __pyx_L1_error)
- }
- break;
- }
- __Pyx_GOTREF(__pyx_t_9);
- }
- __Pyx_XDECREF_SET(__pyx_v_index, __pyx_t_9);
- __pyx_t_9 = 0;
- __pyx_v_dim = __pyx_t_6;
- __pyx_t_6 = (__pyx_t_6 + 1);
-
- /* "View.MemoryView":747
- *
- * for dim, index in enumerate(indices):
- * if PyIndex_Check(index): # <<<<<<<<<<<<<<
- * slice_memviewslice(
- * p_dst, p_src.shape[dim], p_src.strides[dim], p_src.suboffsets[dim],
- */
- __pyx_t_2 = (PyIndex_Check(__pyx_v_index) != 0);
- if (__pyx_t_2) {
-
- /* "View.MemoryView":751
- * p_dst, p_src.shape[dim], p_src.strides[dim], p_src.suboffsets[dim],
- * dim, new_ndim, p_suboffset_dim,
- * index, 0, 0, # start, stop, step # <<<<<<<<<<<<<<
- * 0, 0, 0, # have_{start,stop,step}
- * False)
- */
- __pyx_t_10 = __Pyx_PyIndex_AsSsize_t(__pyx_v_index); if (unlikely((__pyx_t_10 == (Py_ssize_t)-1) && PyErr_Occurred())) __PYX_ERR(1, 751, __pyx_L1_error)
-
- /* "View.MemoryView":748
- * for dim, index in enumerate(indices):
- * if PyIndex_Check(index):
- * slice_memviewslice( # <<<<<<<<<<<<<<
- * p_dst, p_src.shape[dim], p_src.strides[dim], p_src.suboffsets[dim],
- * dim, new_ndim, p_suboffset_dim,
- */
- __pyx_t_11 = __pyx_memoryview_slice_memviewslice(__pyx_v_p_dst, (__pyx_v_p_src->shape[__pyx_v_dim]), (__pyx_v_p_src->strides[__pyx_v_dim]), (__pyx_v_p_src->suboffsets[__pyx_v_dim]), __pyx_v_dim, __pyx_v_new_ndim, __pyx_v_p_suboffset_dim, __pyx_t_10, 0, 0, 0, 0, 0, 0); if (unlikely(__pyx_t_11 == ((int)-1))) __PYX_ERR(1, 748, __pyx_L1_error)
-
- /* "View.MemoryView":747
- *
- * for dim, index in enumerate(indices):
- * if PyIndex_Check(index): # <<<<<<<<<<<<<<
- * slice_memviewslice(
- * p_dst, p_src.shape[dim], p_src.strides[dim], p_src.suboffsets[dim],
- */
- goto __pyx_L6;
- }
-
- /* "View.MemoryView":754
- * 0, 0, 0, # have_{start,stop,step}
- * False)
- * elif index is None: # <<<<<<<<<<<<<<
- * p_dst.shape[new_ndim] = 1
- * p_dst.strides[new_ndim] = 0
- */
- __pyx_t_2 = (__pyx_v_index == Py_None);
- __pyx_t_1 = (__pyx_t_2 != 0);
- if (__pyx_t_1) {
-
- /* "View.MemoryView":755
- * False)
- * elif index is None:
- * p_dst.shape[new_ndim] = 1 # <<<<<<<<<<<<<<
- * p_dst.strides[new_ndim] = 0
- * p_dst.suboffsets[new_ndim] = -1
- */
- (__pyx_v_p_dst->shape[__pyx_v_new_ndim]) = 1;
-
- /* "View.MemoryView":756
- * elif index is None:
- * p_dst.shape[new_ndim] = 1
- * p_dst.strides[new_ndim] = 0 # <<<<<<<<<<<<<<
- * p_dst.suboffsets[new_ndim] = -1
- * new_ndim += 1
- */
- (__pyx_v_p_dst->strides[__pyx_v_new_ndim]) = 0;
-
- /* "View.MemoryView":757
- * p_dst.shape[new_ndim] = 1
- * p_dst.strides[new_ndim] = 0
- * p_dst.suboffsets[new_ndim] = -1 # <<<<<<<<<<<<<<
- * new_ndim += 1
- * else:
- */
- (__pyx_v_p_dst->suboffsets[__pyx_v_new_ndim]) = -1L;
-
- /* "View.MemoryView":758
- * p_dst.strides[new_ndim] = 0
- * p_dst.suboffsets[new_ndim] = -1
- * new_ndim += 1 # <<<<<<<<<<<<<<
- * else:
- * start = index.start or 0
- */
- __pyx_v_new_ndim = (__pyx_v_new_ndim + 1);
-
- /* "View.MemoryView":754
- * 0, 0, 0, # have_{start,stop,step}
- * False)
- * elif index is None: # <<<<<<<<<<<<<<
- * p_dst.shape[new_ndim] = 1
- * p_dst.strides[new_ndim] = 0
- */
- goto __pyx_L6;
- }
-
- /* "View.MemoryView":760
- * new_ndim += 1
- * else:
- * start = index.start or 0 # <<<<<<<<<<<<<<
- * stop = index.stop or 0
- * step = index.step or 0
- */
- /*else*/ {
- __pyx_t_9 = __Pyx_PyObject_GetAttrStr(__pyx_v_index, __pyx_n_s_start); if (unlikely(!__pyx_t_9)) __PYX_ERR(1, 760, __pyx_L1_error)
- __Pyx_GOTREF(__pyx_t_9);
- __pyx_t_1 = __Pyx_PyObject_IsTrue(__pyx_t_9); if (unlikely(__pyx_t_1 < 0)) __PYX_ERR(1, 760, __pyx_L1_error)
- if (!__pyx_t_1) {
- __Pyx_DECREF(__pyx_t_9); __pyx_t_9 = 0;
- } else {
- __pyx_t_12 = __Pyx_PyIndex_AsSsize_t(__pyx_t_9); if (unlikely((__pyx_t_12 == (Py_ssize_t)-1) && PyErr_Occurred())) __PYX_ERR(1, 760, __pyx_L1_error)
- __pyx_t_10 = __pyx_t_12;
- __Pyx_DECREF(__pyx_t_9); __pyx_t_9 = 0;
- goto __pyx_L7_bool_binop_done;
- }
- __pyx_t_10 = 0;
- __pyx_L7_bool_binop_done:;
- __pyx_v_start = __pyx_t_10;
-
- /* "View.MemoryView":761
- * else:
- * start = index.start or 0
- * stop = index.stop or 0 # <<<<<<<<<<<<<<
- * step = index.step or 0
- *
- */
- __pyx_t_9 = __Pyx_PyObject_GetAttrStr(__pyx_v_index, __pyx_n_s_stop); if (unlikely(!__pyx_t_9)) __PYX_ERR(1, 761, __pyx_L1_error)
- __Pyx_GOTREF(__pyx_t_9);
- __pyx_t_1 = __Pyx_PyObject_IsTrue(__pyx_t_9); if (unlikely(__pyx_t_1 < 0)) __PYX_ERR(1, 761, __pyx_L1_error)
- if (!__pyx_t_1) {
- __Pyx_DECREF(__pyx_t_9); __pyx_t_9 = 0;
- } else {
- __pyx_t_12 = __Pyx_PyIndex_AsSsize_t(__pyx_t_9); if (unlikely((__pyx_t_12 == (Py_ssize_t)-1) && PyErr_Occurred())) __PYX_ERR(1, 761, __pyx_L1_error)
- __pyx_t_10 = __pyx_t_12;
- __Pyx_DECREF(__pyx_t_9); __pyx_t_9 = 0;
- goto __pyx_L9_bool_binop_done;
- }
- __pyx_t_10 = 0;
- __pyx_L9_bool_binop_done:;
- __pyx_v_stop = __pyx_t_10;
-
- /* "View.MemoryView":762
- * start = index.start or 0
- * stop = index.stop or 0
- * step = index.step or 0 # <<<<<<<<<<<<<<
- *
- * have_start = index.start is not None
- */
- __pyx_t_9 = __Pyx_PyObject_GetAttrStr(__pyx_v_index, __pyx_n_s_step); if (unlikely(!__pyx_t_9)) __PYX_ERR(1, 762, __pyx_L1_error)
- __Pyx_GOTREF(__pyx_t_9);
- __pyx_t_1 = __Pyx_PyObject_IsTrue(__pyx_t_9); if (unlikely(__pyx_t_1 < 0)) __PYX_ERR(1, 762, __pyx_L1_error)
- if (!__pyx_t_1) {
- __Pyx_DECREF(__pyx_t_9); __pyx_t_9 = 0;
- } else {
- __pyx_t_12 = __Pyx_PyIndex_AsSsize_t(__pyx_t_9); if (unlikely((__pyx_t_12 == (Py_ssize_t)-1) && PyErr_Occurred())) __PYX_ERR(1, 762, __pyx_L1_error)
- __pyx_t_10 = __pyx_t_12;
- __Pyx_DECREF(__pyx_t_9); __pyx_t_9 = 0;
- goto __pyx_L11_bool_binop_done;
- }
- __pyx_t_10 = 0;
- __pyx_L11_bool_binop_done:;
- __pyx_v_step = __pyx_t_10;
-
- /* "View.MemoryView":764
- * step = index.step or 0
- *
- * have_start = index.start is not None # <<<<<<<<<<<<<<
- * have_stop = index.stop is not None
- * have_step = index.step is not None
- */
- __pyx_t_9 = __Pyx_PyObject_GetAttrStr(__pyx_v_index, __pyx_n_s_start); if (unlikely(!__pyx_t_9)) __PYX_ERR(1, 764, __pyx_L1_error)
- __Pyx_GOTREF(__pyx_t_9);
- __pyx_t_1 = (__pyx_t_9 != Py_None);
- __Pyx_DECREF(__pyx_t_9); __pyx_t_9 = 0;
- __pyx_v_have_start = __pyx_t_1;
-
- /* "View.MemoryView":765
- *
- * have_start = index.start is not None
- * have_stop = index.stop is not None # <<<<<<<<<<<<<<
- * have_step = index.step is not None
- *
- */
- __pyx_t_9 = __Pyx_PyObject_GetAttrStr(__pyx_v_index, __pyx_n_s_stop); if (unlikely(!__pyx_t_9)) __PYX_ERR(1, 765, __pyx_L1_error)
- __Pyx_GOTREF(__pyx_t_9);
- __pyx_t_1 = (__pyx_t_9 != Py_None);
- __Pyx_DECREF(__pyx_t_9); __pyx_t_9 = 0;
- __pyx_v_have_stop = __pyx_t_1;
-
- /* "View.MemoryView":766
- * have_start = index.start is not None
- * have_stop = index.stop is not None
- * have_step = index.step is not None # <<<<<<<<<<<<<<
- *
- * slice_memviewslice(
- */
- __pyx_t_9 = __Pyx_PyObject_GetAttrStr(__pyx_v_index, __pyx_n_s_step); if (unlikely(!__pyx_t_9)) __PYX_ERR(1, 766, __pyx_L1_error)
- __Pyx_GOTREF(__pyx_t_9);
- __pyx_t_1 = (__pyx_t_9 != Py_None);
- __Pyx_DECREF(__pyx_t_9); __pyx_t_9 = 0;
- __pyx_v_have_step = __pyx_t_1;
-
- /* "View.MemoryView":768
- * have_step = index.step is not None
- *
- * slice_memviewslice( # <<<<<<<<<<<<<<
- * p_dst, p_src.shape[dim], p_src.strides[dim], p_src.suboffsets[dim],
- * dim, new_ndim, p_suboffset_dim,
- */
- __pyx_t_11 = __pyx_memoryview_slice_memviewslice(__pyx_v_p_dst, (__pyx_v_p_src->shape[__pyx_v_dim]), (__pyx_v_p_src->strides[__pyx_v_dim]), (__pyx_v_p_src->suboffsets[__pyx_v_dim]), __pyx_v_dim, __pyx_v_new_ndim, __pyx_v_p_suboffset_dim, __pyx_v_start, __pyx_v_stop, __pyx_v_step, __pyx_v_have_start, __pyx_v_have_stop, __pyx_v_have_step, 1); if (unlikely(__pyx_t_11 == ((int)-1))) __PYX_ERR(1, 768, __pyx_L1_error)
-
- /* "View.MemoryView":774
- * have_start, have_stop, have_step,
- * True)
- * new_ndim += 1 # <<<<<<<<<<<<<<
- *
- * if isinstance(memview, _memoryviewslice):
- */
- __pyx_v_new_ndim = (__pyx_v_new_ndim + 1);
- }
- __pyx_L6:;
-
- /* "View.MemoryView":746
- * cdef bint have_start, have_stop, have_step
- *
- * for dim, index in enumerate(indices): # <<<<<<<<<<<<<<
- * if PyIndex_Check(index):
- * slice_memviewslice(
- */
- }
- __Pyx_DECREF(__pyx_t_3); __pyx_t_3 = 0;
-
- /* "View.MemoryView":776
- * new_ndim += 1
- *
- * if isinstance(memview, _memoryviewslice): # <<<<<<<<<<<<<<
- * return memoryview_fromslice(dst, new_ndim,
- * memviewsliceobj.to_object_func,
- */
- __pyx_t_1 = __Pyx_TypeCheck(((PyObject *)__pyx_v_memview), __pyx_memoryviewslice_type);
- __pyx_t_2 = (__pyx_t_1 != 0);
- if (__pyx_t_2) {
-
- /* "View.MemoryView":777
- *
- * if isinstance(memview, _memoryviewslice):
- * return memoryview_fromslice(dst, new_ndim, # <<<<<<<<<<<<<<
- * memviewsliceobj.to_object_func,
- * memviewsliceobj.to_dtype_func,
- */
- __Pyx_XDECREF(((PyObject *)__pyx_r));
-
- /* "View.MemoryView":778
- * if isinstance(memview, _memoryviewslice):
- * return memoryview_fromslice(dst, new_ndim,
- * memviewsliceobj.to_object_func, # <<<<<<<<<<<<<<
- * memviewsliceobj.to_dtype_func,
- * memview.dtype_is_object)
- */
- if (unlikely(!__pyx_v_memviewsliceobj)) { __Pyx_RaiseUnboundLocalError("memviewsliceobj"); __PYX_ERR(1, 778, __pyx_L1_error) }
-
- /* "View.MemoryView":779
- * return memoryview_fromslice(dst, new_ndim,
- * memviewsliceobj.to_object_func,
- * memviewsliceobj.to_dtype_func, # <<<<<<<<<<<<<<
- * memview.dtype_is_object)
- * else:
- */
- if (unlikely(!__pyx_v_memviewsliceobj)) { __Pyx_RaiseUnboundLocalError("memviewsliceobj"); __PYX_ERR(1, 779, __pyx_L1_error) }
-
- /* "View.MemoryView":777
- *
- * if isinstance(memview, _memoryviewslice):
- * return memoryview_fromslice(dst, new_ndim, # <<<<<<<<<<<<<<
- * memviewsliceobj.to_object_func,
- * memviewsliceobj.to_dtype_func,
- */
- __pyx_t_3 = __pyx_memoryview_fromslice(__pyx_v_dst, __pyx_v_new_ndim, __pyx_v_memviewsliceobj->to_object_func, __pyx_v_memviewsliceobj->to_dtype_func, __pyx_v_memview->dtype_is_object); if (unlikely(!__pyx_t_3)) __PYX_ERR(1, 777, __pyx_L1_error)
- __Pyx_GOTREF(__pyx_t_3);
- if (!(likely(((__pyx_t_3) == Py_None) || likely(__Pyx_TypeTest(__pyx_t_3, __pyx_memoryview_type))))) __PYX_ERR(1, 777, __pyx_L1_error)
- __pyx_r = ((struct __pyx_memoryview_obj *)__pyx_t_3);
- __pyx_t_3 = 0;
- goto __pyx_L0;
-
- /* "View.MemoryView":776
- * new_ndim += 1
- *
- * if isinstance(memview, _memoryviewslice): # <<<<<<<<<<<<<<
- * return memoryview_fromslice(dst, new_ndim,
- * memviewsliceobj.to_object_func,
- */
- }
-
- /* "View.MemoryView":782
- * memview.dtype_is_object)
- * else:
- * return memoryview_fromslice(dst, new_ndim, NULL, NULL, # <<<<<<<<<<<<<<
- * memview.dtype_is_object)
- *
- */
- /*else*/ {
- __Pyx_XDECREF(((PyObject *)__pyx_r));
-
- /* "View.MemoryView":783
- * else:
- * return memoryview_fromslice(dst, new_ndim, NULL, NULL,
- * memview.dtype_is_object) # <<<<<<<<<<<<<<
- *
- *
- */
- __pyx_t_3 = __pyx_memoryview_fromslice(__pyx_v_dst, __pyx_v_new_ndim, NULL, NULL, __pyx_v_memview->dtype_is_object); if (unlikely(!__pyx_t_3)) __PYX_ERR(1, 782, __pyx_L1_error)
- __Pyx_GOTREF(__pyx_t_3);
-
- /* "View.MemoryView":782
- * memview.dtype_is_object)
- * else:
- * return memoryview_fromslice(dst, new_ndim, NULL, NULL, # <<<<<<<<<<<<<<
- * memview.dtype_is_object)
- *
- */
- if (!(likely(((__pyx_t_3) == Py_None) || likely(__Pyx_TypeTest(__pyx_t_3, __pyx_memoryview_type))))) __PYX_ERR(1, 782, __pyx_L1_error)
- __pyx_r = ((struct __pyx_memoryview_obj *)__pyx_t_3);
- __pyx_t_3 = 0;
- goto __pyx_L0;
- }
-
- /* "View.MemoryView":710
- *
- * @cname('__pyx_memview_slice')
- * cdef memoryview memview_slice(memoryview memview, object indices): # <<<<<<<<<<<<<<
- * cdef int new_ndim = 0, suboffset_dim = -1, dim
- * cdef bint negative_step
- */
-
- /* function exit code */
- __pyx_L1_error:;
- __Pyx_XDECREF(__pyx_t_3);
- __Pyx_XDECREF(__pyx_t_9);
- __Pyx_AddTraceback("View.MemoryView.memview_slice", __pyx_clineno, __pyx_lineno, __pyx_filename);
- __pyx_r = 0;
- __pyx_L0:;
- __Pyx_XDECREF((PyObject *)__pyx_v_memviewsliceobj);
- __Pyx_XDECREF(__pyx_v_index);
- __Pyx_XGIVEREF((PyObject *)__pyx_r);
- __Pyx_RefNannyFinishContext();
- return __pyx_r;
-}
-
-/* "View.MemoryView":807
- *
- * @cname('__pyx_memoryview_slice_memviewslice')
- * cdef int slice_memviewslice( # <<<<<<<<<<<<<<
- * __Pyx_memviewslice *dst,
- * Py_ssize_t shape, Py_ssize_t stride, Py_ssize_t suboffset,
- */
-
-static int __pyx_memoryview_slice_memviewslice(__Pyx_memviewslice *__pyx_v_dst, Py_ssize_t __pyx_v_shape, Py_ssize_t __pyx_v_stride, Py_ssize_t __pyx_v_suboffset, int __pyx_v_dim, int __pyx_v_new_ndim, int *__pyx_v_suboffset_dim, Py_ssize_t __pyx_v_start, Py_ssize_t __pyx_v_stop, Py_ssize_t __pyx_v_step, int __pyx_v_have_start, int __pyx_v_have_stop, int __pyx_v_have_step, int __pyx_v_is_slice) {
- Py_ssize_t __pyx_v_new_shape;
- int __pyx_v_negative_step;
- int __pyx_r;
- int __pyx_t_1;
- int __pyx_t_2;
- int __pyx_t_3;
- int __pyx_lineno = 0;
- const char *__pyx_filename = NULL;
- int __pyx_clineno = 0;
-
- /* "View.MemoryView":827
- * cdef bint negative_step
- *
- * if not is_slice: # <<<<<<<<<<<<<<
- *
- * if start < 0:
- */
- __pyx_t_1 = ((!(__pyx_v_is_slice != 0)) != 0);
- if (__pyx_t_1) {
-
- /* "View.MemoryView":829
- * if not is_slice:
- *
- * if start < 0: # <<<<<<<<<<<<<<
- * start += shape
- * if not 0 <= start < shape:
- */
- __pyx_t_1 = ((__pyx_v_start < 0) != 0);
- if (__pyx_t_1) {
-
- /* "View.MemoryView":830
- *
- * if start < 0:
- * start += shape # <<<<<<<<<<<<<<
- * if not 0 <= start < shape:
- * _err_dim(IndexError, "Index out of bounds (axis %d)", dim)
- */
- __pyx_v_start = (__pyx_v_start + __pyx_v_shape);
-
- /* "View.MemoryView":829
- * if not is_slice:
- *
- * if start < 0: # <<<<<<<<<<<<<<
- * start += shape
- * if not 0 <= start < shape:
- */
- }
-
- /* "View.MemoryView":831
- * if start < 0:
- * start += shape
- * if not 0 <= start < shape: # <<<<<<<<<<<<<<
- * _err_dim(IndexError, "Index out of bounds (axis %d)", dim)
- * else:
- */
- __pyx_t_1 = (0 <= __pyx_v_start);
- if (__pyx_t_1) {
- __pyx_t_1 = (__pyx_v_start < __pyx_v_shape);
- }
- __pyx_t_2 = ((!(__pyx_t_1 != 0)) != 0);
- if (__pyx_t_2) {
-
- /* "View.MemoryView":832
- * start += shape
- * if not 0 <= start < shape:
- * _err_dim(IndexError, "Index out of bounds (axis %d)", dim) # <<<<<<<<<<<<<<
- * else:
- *
- */
- __pyx_t_3 = __pyx_memoryview_err_dim(__pyx_builtin_IndexError, ((char *)"Index out of bounds (axis %d)"), __pyx_v_dim); if (unlikely(__pyx_t_3 == ((int)-1))) __PYX_ERR(1, 832, __pyx_L1_error)
-
- /* "View.MemoryView":831
- * if start < 0:
- * start += shape
- * if not 0 <= start < shape: # <<<<<<<<<<<<<<
- * _err_dim(IndexError, "Index out of bounds (axis %d)", dim)
- * else:
- */
- }
-
- /* "View.MemoryView":827
- * cdef bint negative_step
- *
- * if not is_slice: # <<<<<<<<<<<<<<
- *
- * if start < 0:
- */
- goto __pyx_L3;
- }
-
- /* "View.MemoryView":835
- * else:
- *
- * negative_step = have_step != 0 and step < 0 # <<<<<<<<<<<<<<
- *
- * if have_step and step == 0:
- */
- /*else*/ {
- __pyx_t_1 = ((__pyx_v_have_step != 0) != 0);
- if (__pyx_t_1) {
- } else {
- __pyx_t_2 = __pyx_t_1;
- goto __pyx_L6_bool_binop_done;
- }
- __pyx_t_1 = ((__pyx_v_step < 0) != 0);
- __pyx_t_2 = __pyx_t_1;
- __pyx_L6_bool_binop_done:;
- __pyx_v_negative_step = __pyx_t_2;
-
- /* "View.MemoryView":837
- * negative_step = have_step != 0 and step < 0
- *
- * if have_step and step == 0: # <<<<<<<<<<<<<<
- * _err_dim(ValueError, "Step may not be zero (axis %d)", dim)
- *
- */
- __pyx_t_1 = (__pyx_v_have_step != 0);
- if (__pyx_t_1) {
- } else {
- __pyx_t_2 = __pyx_t_1;
- goto __pyx_L9_bool_binop_done;
- }
- __pyx_t_1 = ((__pyx_v_step == 0) != 0);
- __pyx_t_2 = __pyx_t_1;
- __pyx_L9_bool_binop_done:;
- if (__pyx_t_2) {
-
- /* "View.MemoryView":838
- *
- * if have_step and step == 0:
- * _err_dim(ValueError, "Step may not be zero (axis %d)", dim) # <<<<<<<<<<<<<<
- *
- *
- */
- __pyx_t_3 = __pyx_memoryview_err_dim(__pyx_builtin_ValueError, ((char *)"Step may not be zero (axis %d)"), __pyx_v_dim); if (unlikely(__pyx_t_3 == ((int)-1))) __PYX_ERR(1, 838, __pyx_L1_error)
-
- /* "View.MemoryView":837
- * negative_step = have_step != 0 and step < 0
- *
- * if have_step and step == 0: # <<<<<<<<<<<<<<
- * _err_dim(ValueError, "Step may not be zero (axis %d)", dim)
- *
- */
- }
-
- /* "View.MemoryView":841
- *
- *
- * if have_start: # <<<<<<<<<<<<<<
- * if start < 0:
- * start += shape
- */
- __pyx_t_2 = (__pyx_v_have_start != 0);
- if (__pyx_t_2) {
-
- /* "View.MemoryView":842
- *
- * if have_start:
- * if start < 0: # <<<<<<<<<<<<<<
- * start += shape
- * if start < 0:
- */
- __pyx_t_2 = ((__pyx_v_start < 0) != 0);
- if (__pyx_t_2) {
-
- /* "View.MemoryView":843
- * if have_start:
- * if start < 0:
- * start += shape # <<<<<<<<<<<<<<
- * if start < 0:
- * start = 0
- */
- __pyx_v_start = (__pyx_v_start + __pyx_v_shape);
-
- /* "View.MemoryView":844
- * if start < 0:
- * start += shape
- * if start < 0: # <<<<<<<<<<<<<<
- * start = 0
- * elif start >= shape:
- */
- __pyx_t_2 = ((__pyx_v_start < 0) != 0);
- if (__pyx_t_2) {
-
- /* "View.MemoryView":845
- * start += shape
- * if start < 0:
- * start = 0 # <<<<<<<<<<<<<<
- * elif start >= shape:
- * if negative_step:
- */
- __pyx_v_start = 0;
-
- /* "View.MemoryView":844
- * if start < 0:
- * start += shape
- * if start < 0: # <<<<<<<<<<<<<<
- * start = 0
- * elif start >= shape:
- */
- }
-
- /* "View.MemoryView":842
- *
- * if have_start:
- * if start < 0: # <<<<<<<<<<<<<<
- * start += shape
- * if start < 0:
- */
- goto __pyx_L12;
- }
-
- /* "View.MemoryView":846
- * if start < 0:
- * start = 0
- * elif start >= shape: # <<<<<<<<<<<<<<
- * if negative_step:
- * start = shape - 1
- */
- __pyx_t_2 = ((__pyx_v_start >= __pyx_v_shape) != 0);
- if (__pyx_t_2) {
-
- /* "View.MemoryView":847
- * start = 0
- * elif start >= shape:
- * if negative_step: # <<<<<<<<<<<<<<
- * start = shape - 1
- * else:
- */
- __pyx_t_2 = (__pyx_v_negative_step != 0);
- if (__pyx_t_2) {
-
- /* "View.MemoryView":848
- * elif start >= shape:
- * if negative_step:
- * start = shape - 1 # <<<<<<<<<<<<<<
- * else:
- * start = shape
- */
- __pyx_v_start = (__pyx_v_shape - 1);
-
- /* "View.MemoryView":847
- * start = 0
- * elif start >= shape:
- * if negative_step: # <<<<<<<<<<<<<<
- * start = shape - 1
- * else:
- */
- goto __pyx_L14;
- }
-
- /* "View.MemoryView":850
- * start = shape - 1
- * else:
- * start = shape # <<<<<<<<<<<<<<
- * else:
- * if negative_step:
- */
- /*else*/ {
- __pyx_v_start = __pyx_v_shape;
- }
- __pyx_L14:;
-
- /* "View.MemoryView":846
- * if start < 0:
- * start = 0
- * elif start >= shape: # <<<<<<<<<<<<<<
- * if negative_step:
- * start = shape - 1
- */
- }
- __pyx_L12:;
-
- /* "View.MemoryView":841
- *
- *
- * if have_start: # <<<<<<<<<<<<<<
- * if start < 0:
- * start += shape
- */
- goto __pyx_L11;
- }
-
- /* "View.MemoryView":852
- * start = shape
- * else:
- * if negative_step: # <<<<<<<<<<<<<<
- * start = shape - 1
- * else:
- */
- /*else*/ {
- __pyx_t_2 = (__pyx_v_negative_step != 0);
- if (__pyx_t_2) {
-
- /* "View.MemoryView":853
- * else:
- * if negative_step:
- * start = shape - 1 # <<<<<<<<<<<<<<
- * else:
- * start = 0
- */
- __pyx_v_start = (__pyx_v_shape - 1);
-
- /* "View.MemoryView":852
- * start = shape
- * else:
- * if negative_step: # <<<<<<<<<<<<<<
- * start = shape - 1
- * else:
- */
- goto __pyx_L15;
- }
-
- /* "View.MemoryView":855
- * start = shape - 1
- * else:
- * start = 0 # <<<<<<<<<<<<<<
- *
- * if have_stop:
- */
- /*else*/ {
- __pyx_v_start = 0;
- }
- __pyx_L15:;
- }
- __pyx_L11:;
-
- /* "View.MemoryView":857
- * start = 0
- *
- * if have_stop: # <<<<<<<<<<<<<<
- * if stop < 0:
- * stop += shape
- */
- __pyx_t_2 = (__pyx_v_have_stop != 0);
- if (__pyx_t_2) {
-
- /* "View.MemoryView":858
- *
- * if have_stop:
- * if stop < 0: # <<<<<<<<<<<<<<
- * stop += shape
- * if stop < 0:
- */
- __pyx_t_2 = ((__pyx_v_stop < 0) != 0);
- if (__pyx_t_2) {
-
- /* "View.MemoryView":859
- * if have_stop:
- * if stop < 0:
- * stop += shape # <<<<<<<<<<<<<<
- * if stop < 0:
- * stop = 0
- */
- __pyx_v_stop = (__pyx_v_stop + __pyx_v_shape);
-
- /* "View.MemoryView":860
- * if stop < 0:
- * stop += shape
- * if stop < 0: # <<<<<<<<<<<<<<
- * stop = 0
- * elif stop > shape:
- */
- __pyx_t_2 = ((__pyx_v_stop < 0) != 0);
- if (__pyx_t_2) {
-
- /* "View.MemoryView":861
- * stop += shape
- * if stop < 0:
- * stop = 0 # <<<<<<<<<<<<<<
- * elif stop > shape:
- * stop = shape
- */
- __pyx_v_stop = 0;
-
- /* "View.MemoryView":860
- * if stop < 0:
- * stop += shape
- * if stop < 0: # <<<<<<<<<<<<<<
- * stop = 0
- * elif stop > shape:
- */
- }
-
- /* "View.MemoryView":858
- *
- * if have_stop:
- * if stop < 0: # <<<<<<<<<<<<<<
- * stop += shape
- * if stop < 0:
- */
- goto __pyx_L17;
- }
-
- /* "View.MemoryView":862
- * if stop < 0:
- * stop = 0
- * elif stop > shape: # <<<<<<<<<<<<<<
- * stop = shape
- * else:
- */
- __pyx_t_2 = ((__pyx_v_stop > __pyx_v_shape) != 0);
- if (__pyx_t_2) {
-
- /* "View.MemoryView":863
- * stop = 0
- * elif stop > shape:
- * stop = shape # <<<<<<<<<<<<<<
- * else:
- * if negative_step:
- */
- __pyx_v_stop = __pyx_v_shape;
-
- /* "View.MemoryView":862
- * if stop < 0:
- * stop = 0
- * elif stop > shape: # <<<<<<<<<<<<<<
- * stop = shape
- * else:
- */
- }
- __pyx_L17:;
-
- /* "View.MemoryView":857
- * start = 0
- *
- * if have_stop: # <<<<<<<<<<<<<<
- * if stop < 0:
- * stop += shape
- */
- goto __pyx_L16;
- }
-
- /* "View.MemoryView":865
- * stop = shape
- * else:
- * if negative_step: # <<<<<<<<<<<<<<
- * stop = -1
- * else:
- */
- /*else*/ {
- __pyx_t_2 = (__pyx_v_negative_step != 0);
- if (__pyx_t_2) {
-
- /* "View.MemoryView":866
- * else:
- * if negative_step:
- * stop = -1 # <<<<<<<<<<<<<<
- * else:
- * stop = shape
- */
- __pyx_v_stop = -1L;
-
- /* "View.MemoryView":865
- * stop = shape
- * else:
- * if negative_step: # <<<<<<<<<<<<<<
- * stop = -1
- * else:
- */
- goto __pyx_L19;
- }
-
- /* "View.MemoryView":868
- * stop = -1
- * else:
- * stop = shape # <<<<<<<<<<<<<<
- *
- * if not have_step:
- */
- /*else*/ {
- __pyx_v_stop = __pyx_v_shape;
- }
- __pyx_L19:;
- }
- __pyx_L16:;
-
- /* "View.MemoryView":870
- * stop = shape
- *
- * if not have_step: # <<<<<<<<<<<<<<
- * step = 1
- *
- */
- __pyx_t_2 = ((!(__pyx_v_have_step != 0)) != 0);
- if (__pyx_t_2) {
-
- /* "View.MemoryView":871
- *
- * if not have_step:
- * step = 1 # <<<<<<<<<<<<<<
- *
- *
- */
- __pyx_v_step = 1;
-
- /* "View.MemoryView":870
- * stop = shape
- *
- * if not have_step: # <<<<<<<<<<<<<<
- * step = 1
- *
- */
- }
-
- /* "View.MemoryView":875
- *
- * with cython.cdivision(True):
- * new_shape = (stop - start) // step # <<<<<<<<<<<<<<
- *
- * if (stop - start) - step * new_shape:
- */
- __pyx_v_new_shape = ((__pyx_v_stop - __pyx_v_start) / __pyx_v_step);
-
- /* "View.MemoryView":877
- * new_shape = (stop - start) // step
- *
- * if (stop - start) - step * new_shape: # <<<<<<<<<<<<<<
- * new_shape += 1
- *
- */
- __pyx_t_2 = (((__pyx_v_stop - __pyx_v_start) - (__pyx_v_step * __pyx_v_new_shape)) != 0);
- if (__pyx_t_2) {
-
- /* "View.MemoryView":878
- *
- * if (stop - start) - step * new_shape:
- * new_shape += 1 # <<<<<<<<<<<<<<
- *
- * if new_shape < 0:
- */
- __pyx_v_new_shape = (__pyx_v_new_shape + 1);
-
- /* "View.MemoryView":877
- * new_shape = (stop - start) // step
- *
- * if (stop - start) - step * new_shape: # <<<<<<<<<<<<<<
- * new_shape += 1
- *
- */
- }
-
- /* "View.MemoryView":880
- * new_shape += 1
- *
- * if new_shape < 0: # <<<<<<<<<<<<<<
- * new_shape = 0
- *
- */
- __pyx_t_2 = ((__pyx_v_new_shape < 0) != 0);
- if (__pyx_t_2) {
-
- /* "View.MemoryView":881
- *
- * if new_shape < 0:
- * new_shape = 0 # <<<<<<<<<<<<<<
- *
- *
- */
- __pyx_v_new_shape = 0;
-
- /* "View.MemoryView":880
- * new_shape += 1
- *
- * if new_shape < 0: # <<<<<<<<<<<<<<
- * new_shape = 0
- *
- */
- }
-
- /* "View.MemoryView":884
- *
- *
- * dst.strides[new_ndim] = stride * step # <<<<<<<<<<<<<<
- * dst.shape[new_ndim] = new_shape
- * dst.suboffsets[new_ndim] = suboffset
- */
- (__pyx_v_dst->strides[__pyx_v_new_ndim]) = (__pyx_v_stride * __pyx_v_step);
-
- /* "View.MemoryView":885
- *
- * dst.strides[new_ndim] = stride * step
- * dst.shape[new_ndim] = new_shape # <<<<<<<<<<<<<<
- * dst.suboffsets[new_ndim] = suboffset
- *
- */
- (__pyx_v_dst->shape[__pyx_v_new_ndim]) = __pyx_v_new_shape;
-
- /* "View.MemoryView":886
- * dst.strides[new_ndim] = stride * step
- * dst.shape[new_ndim] = new_shape
- * dst.suboffsets[new_ndim] = suboffset # <<<<<<<<<<<<<<
- *
- *
- */
- (__pyx_v_dst->suboffsets[__pyx_v_new_ndim]) = __pyx_v_suboffset;
- }
- __pyx_L3:;
-
- /* "View.MemoryView":889
- *
- *
- * if suboffset_dim[0] < 0: # <<<<<<<<<<<<<<
- * dst.data += start * stride
- * else:
- */
- __pyx_t_2 = (((__pyx_v_suboffset_dim[0]) < 0) != 0);
- if (__pyx_t_2) {
-
- /* "View.MemoryView":890
- *
- * if suboffset_dim[0] < 0:
- * dst.data += start * stride # <<<<<<<<<<<<<<
- * else:
- * dst.suboffsets[suboffset_dim[0]] += start * stride
- */
- __pyx_v_dst->data = (__pyx_v_dst->data + (__pyx_v_start * __pyx_v_stride));
-
- /* "View.MemoryView":889
- *
- *
- * if suboffset_dim[0] < 0: # <<<<<<<<<<<<<<
- * dst.data += start * stride
- * else:
- */
- goto __pyx_L23;
- }
-
- /* "View.MemoryView":892
- * dst.data += start * stride
- * else:
- * dst.suboffsets[suboffset_dim[0]] += start * stride # <<<<<<<<<<<<<<
- *
- * if suboffset >= 0:
- */
- /*else*/ {
- __pyx_t_3 = (__pyx_v_suboffset_dim[0]);
- (__pyx_v_dst->suboffsets[__pyx_t_3]) = ((__pyx_v_dst->suboffsets[__pyx_t_3]) + (__pyx_v_start * __pyx_v_stride));
- }
- __pyx_L23:;
-
- /* "View.MemoryView":894
- * dst.suboffsets[suboffset_dim[0]] += start * stride
- *
- * if suboffset >= 0: # <<<<<<<<<<<<<<
- * if not is_slice:
- * if new_ndim == 0:
- */
- __pyx_t_2 = ((__pyx_v_suboffset >= 0) != 0);
- if (__pyx_t_2) {
-
- /* "View.MemoryView":895
- *
- * if suboffset >= 0:
- * if not is_slice: # <<<<<<<<<<<<<<
- * if new_ndim == 0:
- * dst.data = ( dst.data)[0] + suboffset
- */
- __pyx_t_2 = ((!(__pyx_v_is_slice != 0)) != 0);
- if (__pyx_t_2) {
-
- /* "View.MemoryView":896
- * if suboffset >= 0:
- * if not is_slice:
- * if new_ndim == 0: # <<<<<<<<<<<<<<
- * dst.data = ( dst.data)[0] + suboffset
- * else:
- */
- __pyx_t_2 = ((__pyx_v_new_ndim == 0) != 0);
- if (__pyx_t_2) {
-
- /* "View.MemoryView":897
- * if not is_slice:
- * if new_ndim == 0:
- * dst.data = ( dst.data)[0] + suboffset # <<<<<<<<<<<<<<
- * else:
- * _err_dim(IndexError, "All dimensions preceding dimension %d "
- */
- __pyx_v_dst->data = ((((char **)__pyx_v_dst->data)[0]) + __pyx_v_suboffset);
-
- /* "View.MemoryView":896
- * if suboffset >= 0:
- * if not is_slice:
- * if new_ndim == 0: # <<<<<<<<<<<<<<
- * dst.data = ( dst.data)[0] + suboffset
- * else:
- */
- goto __pyx_L26;
- }
-
- /* "View.MemoryView":899
- * dst.data = ( dst.data)[0] + suboffset
- * else:
- * _err_dim(IndexError, "All dimensions preceding dimension %d " # <<<<<<<<<<<<<<
- * "must be indexed and not sliced", dim)
- * else:
- */
- /*else*/ {
-
- /* "View.MemoryView":900
- * else:
- * _err_dim(IndexError, "All dimensions preceding dimension %d "
- * "must be indexed and not sliced", dim) # <<<<<<<<<<<<<<
- * else:
- * suboffset_dim[0] = new_ndim
- */
- __pyx_t_3 = __pyx_memoryview_err_dim(__pyx_builtin_IndexError, ((char *)"All dimensions preceding dimension %d must be indexed and not sliced"), __pyx_v_dim); if (unlikely(__pyx_t_3 == ((int)-1))) __PYX_ERR(1, 899, __pyx_L1_error)
- }
- __pyx_L26:;
-
- /* "View.MemoryView":895
- *
- * if suboffset >= 0:
- * if not is_slice: # <<<<<<<<<<<<<<
- * if new_ndim == 0:
- * dst.data = ( dst.data)[0] + suboffset
- */
- goto __pyx_L25;
- }
-
- /* "View.MemoryView":902
- * "must be indexed and not sliced", dim)
- * else:
- * suboffset_dim[0] = new_ndim # <<<<<<<<<<<<<<
- *
- * return 0
- */
- /*else*/ {
- (__pyx_v_suboffset_dim[0]) = __pyx_v_new_ndim;
- }
- __pyx_L25:;
-
- /* "View.MemoryView":894
- * dst.suboffsets[suboffset_dim[0]] += start * stride
- *
- * if suboffset >= 0: # <<<<<<<<<<<<<<
- * if not is_slice:
- * if new_ndim == 0:
- */
- }
-
- /* "View.MemoryView":904
- * suboffset_dim[0] = new_ndim
- *
- * return 0 # <<<<<<<<<<<<<<
- *
- *
- */
- __pyx_r = 0;
- goto __pyx_L0;
-
- /* "View.MemoryView":807
- *
- * @cname('__pyx_memoryview_slice_memviewslice')
- * cdef int slice_memviewslice( # <<<<<<<<<<<<<<
- * __Pyx_memviewslice *dst,
- * Py_ssize_t shape, Py_ssize_t stride, Py_ssize_t suboffset,
- */
-
- /* function exit code */
- __pyx_L1_error:;
- {
- #ifdef WITH_THREAD
- PyGILState_STATE __pyx_gilstate_save = __Pyx_PyGILState_Ensure();
- #endif
- __Pyx_AddTraceback("View.MemoryView.slice_memviewslice", __pyx_clineno, __pyx_lineno, __pyx_filename);
- #ifdef WITH_THREAD
- __Pyx_PyGILState_Release(__pyx_gilstate_save);
- #endif
- }
- __pyx_r = -1;
- __pyx_L0:;
- return __pyx_r;
-}
-
-/* "View.MemoryView":910
- *
- * @cname('__pyx_pybuffer_index')
- * cdef char *pybuffer_index(Py_buffer *view, char *bufp, Py_ssize_t index, # <<<<<<<<<<<<<<
- * Py_ssize_t dim) except NULL:
- * cdef Py_ssize_t shape, stride, suboffset = -1
- */
-
-static char *__pyx_pybuffer_index(Py_buffer *__pyx_v_view, char *__pyx_v_bufp, Py_ssize_t __pyx_v_index, Py_ssize_t __pyx_v_dim) {
- Py_ssize_t __pyx_v_shape;
- Py_ssize_t __pyx_v_stride;
- Py_ssize_t __pyx_v_suboffset;
- Py_ssize_t __pyx_v_itemsize;
- char *__pyx_v_resultp;
- char *__pyx_r;
- __Pyx_RefNannyDeclarations
- Py_ssize_t __pyx_t_1;
- int __pyx_t_2;
- PyObject *__pyx_t_3 = NULL;
- PyObject *__pyx_t_4 = NULL;
- int __pyx_lineno = 0;
- const char *__pyx_filename = NULL;
- int __pyx_clineno = 0;
- __Pyx_RefNannySetupContext("pybuffer_index", 0);
-
- /* "View.MemoryView":912
- * cdef char *pybuffer_index(Py_buffer *view, char *bufp, Py_ssize_t index,
- * Py_ssize_t dim) except NULL:
- * cdef Py_ssize_t shape, stride, suboffset = -1 # <<<<<<<<<<<<<<
- * cdef Py_ssize_t itemsize = view.itemsize
- * cdef char *resultp
- */
- __pyx_v_suboffset = -1L;
-
- /* "View.MemoryView":913
- * Py_ssize_t dim) except NULL:
- * cdef Py_ssize_t shape, stride, suboffset = -1
- * cdef Py_ssize_t itemsize = view.itemsize # <<<<<<<<<<<<<<
- * cdef char *resultp
- *
- */
- __pyx_t_1 = __pyx_v_view->itemsize;
- __pyx_v_itemsize = __pyx_t_1;
-
- /* "View.MemoryView":916
- * cdef char *resultp
- *
- * if view.ndim == 0: # <<<<<<<<<<<<<<
- * shape = view.len / itemsize
- * stride = itemsize
- */
- __pyx_t_2 = ((__pyx_v_view->ndim == 0) != 0);
- if (__pyx_t_2) {
-
- /* "View.MemoryView":917
- *
- * if view.ndim == 0:
- * shape = view.len / itemsize # <<<<<<<<<<<<<<
- * stride = itemsize
- * else:
- */
- if (unlikely(__pyx_v_itemsize == 0)) {
- PyErr_SetString(PyExc_ZeroDivisionError, "integer division or modulo by zero");
- __PYX_ERR(1, 917, __pyx_L1_error)
- }
- else if (sizeof(Py_ssize_t) == sizeof(long) && (!(((Py_ssize_t)-1) > 0)) && unlikely(__pyx_v_itemsize == (Py_ssize_t)-1) && unlikely(UNARY_NEG_WOULD_OVERFLOW(__pyx_v_view->len))) {
- PyErr_SetString(PyExc_OverflowError, "value too large to perform division");
- __PYX_ERR(1, 917, __pyx_L1_error)
- }
- __pyx_v_shape = __Pyx_div_Py_ssize_t(__pyx_v_view->len, __pyx_v_itemsize);
-
- /* "View.MemoryView":918
- * if view.ndim == 0:
- * shape = view.len / itemsize
- * stride = itemsize # <<<<<<<<<<<<<<
- * else:
- * shape = view.shape[dim]
- */
- __pyx_v_stride = __pyx_v_itemsize;
-
- /* "View.MemoryView":916
- * cdef char *resultp
- *
- * if view.ndim == 0: # <<<<<<<<<<<<<<
- * shape = view.len / itemsize
- * stride = itemsize
- */
- goto __pyx_L3;
- }
-
- /* "View.MemoryView":920
- * stride = itemsize
- * else:
- * shape = view.shape[dim] # <<<<<<<<<<<<<<
- * stride = view.strides[dim]
- * if view.suboffsets != NULL:
- */
- /*else*/ {
- __pyx_v_shape = (__pyx_v_view->shape[__pyx_v_dim]);
-
- /* "View.MemoryView":921
- * else:
- * shape = view.shape[dim]
- * stride = view.strides[dim] # <<<<<<<<<<<<<<
- * if view.suboffsets != NULL:
- * suboffset = view.suboffsets[dim]
- */
- __pyx_v_stride = (__pyx_v_view->strides[__pyx_v_dim]);
-
- /* "View.MemoryView":922
- * shape = view.shape[dim]
- * stride = view.strides[dim]
- * if view.suboffsets != NULL: # <<<<<<<<<<<<<<
- * suboffset = view.suboffsets[dim]
- *
- */
- __pyx_t_2 = ((__pyx_v_view->suboffsets != NULL) != 0);
- if (__pyx_t_2) {
-
- /* "View.MemoryView":923
- * stride = view.strides[dim]
- * if view.suboffsets != NULL:
- * suboffset = view.suboffsets[dim] # <<<<<<<<<<<<<<
- *
- * if index < 0:
- */
- __pyx_v_suboffset = (__pyx_v_view->suboffsets[__pyx_v_dim]);
-
- /* "View.MemoryView":922
- * shape = view.shape[dim]
- * stride = view.strides[dim]
- * if view.suboffsets != NULL: # <<<<<<<<<<<<<<
- * suboffset = view.suboffsets[dim]
- *
- */
- }
- }
- __pyx_L3:;
-
- /* "View.MemoryView":925
- * suboffset = view.suboffsets[dim]
- *
- * if index < 0: # <<<<<<<<<<<<<<
- * index += view.shape[dim]
- * if index < 0:
- */
- __pyx_t_2 = ((__pyx_v_index < 0) != 0);
- if (__pyx_t_2) {
-
- /* "View.MemoryView":926
- *
- * if index < 0:
- * index += view.shape[dim] # <<<<<<<<<<<<<<
- * if index < 0:
- * raise IndexError("Out of bounds on buffer access (axis %d)" % dim)
- */
- __pyx_v_index = (__pyx_v_index + (__pyx_v_view->shape[__pyx_v_dim]));
-
- /* "View.MemoryView":927
- * if index < 0:
- * index += view.shape[dim]
- * if index < 0: # <<<<<<<<<<<<<<
- * raise IndexError("Out of bounds on buffer access (axis %d)" % dim)
- *
- */
- __pyx_t_2 = ((__pyx_v_index < 0) != 0);
- if (unlikely(__pyx_t_2)) {
-
- /* "View.MemoryView":928
- * index += view.shape[dim]
- * if index < 0:
- * raise IndexError("Out of bounds on buffer access (axis %d)" % dim) # <<<<<<<<<<<<<<
- *
- * if index >= shape:
- */
- __pyx_t_3 = PyInt_FromSsize_t(__pyx_v_dim); if (unlikely(!__pyx_t_3)) __PYX_ERR(1, 928, __pyx_L1_error)
- __Pyx_GOTREF(__pyx_t_3);
- __pyx_t_4 = __Pyx_PyString_Format(__pyx_kp_s_Out_of_bounds_on_buffer_access_a, __pyx_t_3); if (unlikely(!__pyx_t_4)) __PYX_ERR(1, 928, __pyx_L1_error)
- __Pyx_GOTREF(__pyx_t_4);
- __Pyx_DECREF(__pyx_t_3); __pyx_t_3 = 0;
- __pyx_t_3 = __Pyx_PyObject_CallOneArg(__pyx_builtin_IndexError, __pyx_t_4); if (unlikely(!__pyx_t_3)) __PYX_ERR(1, 928, __pyx_L1_error)
- __Pyx_GOTREF(__pyx_t_3);
- __Pyx_DECREF(__pyx_t_4); __pyx_t_4 = 0;
- __Pyx_Raise(__pyx_t_3, 0, 0, 0);
- __Pyx_DECREF(__pyx_t_3); __pyx_t_3 = 0;
- __PYX_ERR(1, 928, __pyx_L1_error)
-
- /* "View.MemoryView":927
- * if index < 0:
- * index += view.shape[dim]
- * if index < 0: # <<<<<<<<<<<<<<
- * raise IndexError("Out of bounds on buffer access (axis %d)" % dim)
- *
- */
- }
-
- /* "View.MemoryView":925
- * suboffset = view.suboffsets[dim]
- *
- * if index < 0: # <<<<<<<<<<<<<<
- * index += view.shape[dim]
- * if index < 0:
- */
- }
-
- /* "View.MemoryView":930
- * raise IndexError("Out of bounds on buffer access (axis %d)" % dim)
- *
- * if index >= shape: # <<<<<<<<<<<<<<
- * raise IndexError("Out of bounds on buffer access (axis %d)" % dim)
- *
- */
- __pyx_t_2 = ((__pyx_v_index >= __pyx_v_shape) != 0);
- if (unlikely(__pyx_t_2)) {
-
- /* "View.MemoryView":931
- *
- * if index >= shape:
- * raise IndexError("Out of bounds on buffer access (axis %d)" % dim) # <<<<<<<<<<<<<<
- *
- * resultp = bufp + index * stride
- */
- __pyx_t_3 = PyInt_FromSsize_t(__pyx_v_dim); if (unlikely(!__pyx_t_3)) __PYX_ERR(1, 931, __pyx_L1_error)
- __Pyx_GOTREF(__pyx_t_3);
- __pyx_t_4 = __Pyx_PyString_Format(__pyx_kp_s_Out_of_bounds_on_buffer_access_a, __pyx_t_3); if (unlikely(!__pyx_t_4)) __PYX_ERR(1, 931, __pyx_L1_error)
- __Pyx_GOTREF(__pyx_t_4);
- __Pyx_DECREF(__pyx_t_3); __pyx_t_3 = 0;
- __pyx_t_3 = __Pyx_PyObject_CallOneArg(__pyx_builtin_IndexError, __pyx_t_4); if (unlikely(!__pyx_t_3)) __PYX_ERR(1, 931, __pyx_L1_error)
- __Pyx_GOTREF(__pyx_t_3);
- __Pyx_DECREF(__pyx_t_4); __pyx_t_4 = 0;
- __Pyx_Raise(__pyx_t_3, 0, 0, 0);
- __Pyx_DECREF(__pyx_t_3); __pyx_t_3 = 0;
- __PYX_ERR(1, 931, __pyx_L1_error)
-
- /* "View.MemoryView":930
- * raise IndexError("Out of bounds on buffer access (axis %d)" % dim)
- *
- * if index >= shape: # <<<<<<<<<<<<<<
- * raise IndexError("Out of bounds on buffer access (axis %d)" % dim)
- *
- */
- }
-
- /* "View.MemoryView":933
- * raise IndexError("Out of bounds on buffer access (axis %d)" % dim)
- *
- * resultp = bufp + index * stride # <<<<<<<<<<<<<<
- * if suboffset >= 0:
- * resultp = ( resultp)[0] + suboffset
- */
- __pyx_v_resultp = (__pyx_v_bufp + (__pyx_v_index * __pyx_v_stride));
-
- /* "View.MemoryView":934
- *
- * resultp = bufp + index * stride
- * if suboffset >= 0: # <<<<<<<<<<<<<<
- * resultp = ( resultp)[0] + suboffset
- *
- */
- __pyx_t_2 = ((__pyx_v_suboffset >= 0) != 0);
- if (__pyx_t_2) {
-
- /* "View.MemoryView":935
- * resultp = bufp + index * stride
- * if suboffset >= 0:
- * resultp = ( resultp)[0] + suboffset # <<<<<<<<<<<<<<
- *
- * return resultp
- */
- __pyx_v_resultp = ((((char **)__pyx_v_resultp)[0]) + __pyx_v_suboffset);
-
- /* "View.MemoryView":934
- *
- * resultp = bufp + index * stride
- * if suboffset >= 0: # <<<<<<<<<<<<<<
- * resultp = ( resultp)[0] + suboffset
- *
- */
- }
-
- /* "View.MemoryView":937
- * resultp = ( resultp)[0] + suboffset
- *
- * return resultp # <<<<<<<<<<<<<<
- *
- *
- */
- __pyx_r = __pyx_v_resultp;
- goto __pyx_L0;
-
- /* "View.MemoryView":910
- *
- * @cname('__pyx_pybuffer_index')
- * cdef char *pybuffer_index(Py_buffer *view, char *bufp, Py_ssize_t index, # <<<<<<<<<<<<<<
- * Py_ssize_t dim) except NULL:
- * cdef Py_ssize_t shape, stride, suboffset = -1
- */
-
- /* function exit code */
- __pyx_L1_error:;
- __Pyx_XDECREF(__pyx_t_3);
- __Pyx_XDECREF(__pyx_t_4);
- __Pyx_AddTraceback("View.MemoryView.pybuffer_index", __pyx_clineno, __pyx_lineno, __pyx_filename);
- __pyx_r = NULL;
- __pyx_L0:;
- __Pyx_RefNannyFinishContext();
- return __pyx_r;
-}
-
-/* "View.MemoryView":943
- *
- * @cname('__pyx_memslice_transpose')
- * cdef int transpose_memslice(__Pyx_memviewslice *memslice) nogil except 0: # <<<<<<<<<<<<<<
- * cdef int ndim = memslice.memview.view.ndim
- *
- */
-
-static int __pyx_memslice_transpose(__Pyx_memviewslice *__pyx_v_memslice) {
- int __pyx_v_ndim;
- Py_ssize_t *__pyx_v_shape;
- Py_ssize_t *__pyx_v_strides;
- int __pyx_v_i;
- int __pyx_v_j;
- int __pyx_r;
- int __pyx_t_1;
- Py_ssize_t *__pyx_t_2;
- long __pyx_t_3;
- long __pyx_t_4;
- Py_ssize_t __pyx_t_5;
- Py_ssize_t __pyx_t_6;
- int __pyx_t_7;
- int __pyx_t_8;
- int __pyx_t_9;
- int __pyx_lineno = 0;
- const char *__pyx_filename = NULL;
- int __pyx_clineno = 0;
-
- /* "View.MemoryView":944
- * @cname('__pyx_memslice_transpose')
- * cdef int transpose_memslice(__Pyx_memviewslice *memslice) nogil except 0:
- * cdef int ndim = memslice.memview.view.ndim # <<<<<<<<<<<<<<
- *
- * cdef Py_ssize_t *shape = memslice.shape
- */
- __pyx_t_1 = __pyx_v_memslice->memview->view.ndim;
- __pyx_v_ndim = __pyx_t_1;
-
- /* "View.MemoryView":946
- * cdef int ndim = memslice.memview.view.ndim
- *
- * cdef Py_ssize_t *shape = memslice.shape # <<<<<<<<<<<<<<
- * cdef Py_ssize_t *strides = memslice.strides
- *
- */
- __pyx_t_2 = __pyx_v_memslice->shape;
- __pyx_v_shape = __pyx_t_2;
-
- /* "View.MemoryView":947
- *
- * cdef Py_ssize_t *shape = memslice.shape
- * cdef Py_ssize_t *strides = memslice.strides # <<<<<<<<<<<<<<
- *
- *
- */
- __pyx_t_2 = __pyx_v_memslice->strides;
- __pyx_v_strides = __pyx_t_2;
-
- /* "View.MemoryView":951
- *
- * cdef int i, j
- * for i in range(ndim / 2): # <<<<<<<<<<<<<<
- * j = ndim - 1 - i
- * strides[i], strides[j] = strides[j], strides[i]
- */
- __pyx_t_3 = __Pyx_div_long(__pyx_v_ndim, 2);
- __pyx_t_4 = __pyx_t_3;
- for (__pyx_t_1 = 0; __pyx_t_1 < __pyx_t_4; __pyx_t_1+=1) {
- __pyx_v_i = __pyx_t_1;
-
- /* "View.MemoryView":952
- * cdef int i, j
- * for i in range(ndim / 2):
- * j = ndim - 1 - i # <<<<<<<<<<<<<<
- * strides[i], strides[j] = strides[j], strides[i]
- * shape[i], shape[j] = shape[j], shape[i]
- */
- __pyx_v_j = ((__pyx_v_ndim - 1) - __pyx_v_i);
-
- /* "View.MemoryView":953
- * for i in range(ndim / 2):
- * j = ndim - 1 - i
- * strides[i], strides[j] = strides[j], strides[i] # <<<<<<<<<<<<<<
- * shape[i], shape[j] = shape[j], shape[i]
- *
- */
- __pyx_t_5 = (__pyx_v_strides[__pyx_v_j]);
- __pyx_t_6 = (__pyx_v_strides[__pyx_v_i]);
- (__pyx_v_strides[__pyx_v_i]) = __pyx_t_5;
- (__pyx_v_strides[__pyx_v_j]) = __pyx_t_6;
-
- /* "View.MemoryView":954
- * j = ndim - 1 - i
- * strides[i], strides[j] = strides[j], strides[i]
- * shape[i], shape[j] = shape[j], shape[i] # <<<<<<<<<<<<<<
- *
- * if memslice.suboffsets[i] >= 0 or memslice.suboffsets[j] >= 0:
- */
- __pyx_t_6 = (__pyx_v_shape[__pyx_v_j]);
- __pyx_t_5 = (__pyx_v_shape[__pyx_v_i]);
- (__pyx_v_shape[__pyx_v_i]) = __pyx_t_6;
- (__pyx_v_shape[__pyx_v_j]) = __pyx_t_5;
-
- /* "View.MemoryView":956
- * shape[i], shape[j] = shape[j], shape[i]
- *
- * if memslice.suboffsets[i] >= 0 or memslice.suboffsets[j] >= 0: # <<<<<<<<<<<<<<
- * _err(ValueError, "Cannot transpose memoryview with indirect dimensions")
- *
- */
- __pyx_t_8 = (((__pyx_v_memslice->suboffsets[__pyx_v_i]) >= 0) != 0);
- if (!__pyx_t_8) {
- } else {
- __pyx_t_7 = __pyx_t_8;
- goto __pyx_L6_bool_binop_done;
- }
- __pyx_t_8 = (((__pyx_v_memslice->suboffsets[__pyx_v_j]) >= 0) != 0);
- __pyx_t_7 = __pyx_t_8;
- __pyx_L6_bool_binop_done:;
- if (__pyx_t_7) {
-
- /* "View.MemoryView":957
- *
- * if memslice.suboffsets[i] >= 0 or memslice.suboffsets[j] >= 0:
- * _err(ValueError, "Cannot transpose memoryview with indirect dimensions") # <<<<<<<<<<<<<<
- *
- * return 1
- */
- __pyx_t_9 = __pyx_memoryview_err(__pyx_builtin_ValueError, ((char *)"Cannot transpose memoryview with indirect dimensions")); if (unlikely(__pyx_t_9 == ((int)-1))) __PYX_ERR(1, 957, __pyx_L1_error)
-
- /* "View.MemoryView":956
- * shape[i], shape[j] = shape[j], shape[i]
- *
- * if memslice.suboffsets[i] >= 0 or memslice.suboffsets[j] >= 0: # <<<<<<<<<<<<<<
- * _err(ValueError, "Cannot transpose memoryview with indirect dimensions")
- *
- */
- }
- }
-
- /* "View.MemoryView":959
- * _err(ValueError, "Cannot transpose memoryview with indirect dimensions")
- *
- * return 1 # <<<<<<<<<<<<<<
- *
- *
- */
- __pyx_r = 1;
- goto __pyx_L0;
-
- /* "View.MemoryView":943
- *
- * @cname('__pyx_memslice_transpose')
- * cdef int transpose_memslice(__Pyx_memviewslice *memslice) nogil except 0: # <<<<<<<<<<<<<<
- * cdef int ndim = memslice.memview.view.ndim
- *
- */
-
- /* function exit code */
- __pyx_L1_error:;
- {
- #ifdef WITH_THREAD
- PyGILState_STATE __pyx_gilstate_save = __Pyx_PyGILState_Ensure();
- #endif
- __Pyx_AddTraceback("View.MemoryView.transpose_memslice", __pyx_clineno, __pyx_lineno, __pyx_filename);
- #ifdef WITH_THREAD
- __Pyx_PyGILState_Release(__pyx_gilstate_save);
- #endif
- }
- __pyx_r = 0;
- __pyx_L0:;
- return __pyx_r;
-}
-
-/* "View.MemoryView":976
- * cdef int (*to_dtype_func)(char *, object) except 0
- *
- * def __dealloc__(self): # <<<<<<<<<<<<<<
- * __PYX_XDEC_MEMVIEW(&self.from_slice, 1)
- *
- */
-
-/* Python wrapper */
-static void __pyx_memoryviewslice___dealloc__(PyObject *__pyx_v_self); /*proto*/
-static void __pyx_memoryviewslice___dealloc__(PyObject *__pyx_v_self) {
- __Pyx_RefNannyDeclarations
- __Pyx_RefNannySetupContext("__dealloc__ (wrapper)", 0);
- __pyx_memoryviewslice___pyx_pf_15View_dot_MemoryView_16_memoryviewslice___dealloc__(((struct __pyx_memoryviewslice_obj *)__pyx_v_self));
-
- /* function exit code */
- __Pyx_RefNannyFinishContext();
-}
-
-static void __pyx_memoryviewslice___pyx_pf_15View_dot_MemoryView_16_memoryviewslice___dealloc__(struct __pyx_memoryviewslice_obj *__pyx_v_self) {
- __Pyx_RefNannyDeclarations
- __Pyx_RefNannySetupContext("__dealloc__", 0);
-
- /* "View.MemoryView":977
- *
- * def __dealloc__(self):
- * __PYX_XDEC_MEMVIEW(&self.from_slice, 1) # <<<<<<<<<<<<<<
- *
- * cdef convert_item_to_object(self, char *itemp):
- */
- __PYX_XDEC_MEMVIEW((&__pyx_v_self->from_slice), 1);
-
- /* "View.MemoryView":976
- * cdef int (*to_dtype_func)(char *, object) except 0
- *
- * def __dealloc__(self): # <<<<<<<<<<<<<<
- * __PYX_XDEC_MEMVIEW(&self.from_slice, 1)
- *
- */
-
- /* function exit code */
- __Pyx_RefNannyFinishContext();
-}
-
-/* "View.MemoryView":979
- * __PYX_XDEC_MEMVIEW(&self.from_slice, 1)
- *
- * cdef convert_item_to_object(self, char *itemp): # <<<<<<<<<<<<<<
- * if self.to_object_func != NULL:
- * return self.to_object_func(itemp)
- */
-
-static PyObject *__pyx_memoryviewslice_convert_item_to_object(struct __pyx_memoryviewslice_obj *__pyx_v_self, char *__pyx_v_itemp) {
- PyObject *__pyx_r = NULL;
- __Pyx_RefNannyDeclarations
- int __pyx_t_1;
- PyObject *__pyx_t_2 = NULL;
- int __pyx_lineno = 0;
- const char *__pyx_filename = NULL;
- int __pyx_clineno = 0;
- __Pyx_RefNannySetupContext("convert_item_to_object", 0);
-
- /* "View.MemoryView":980
- *
- * cdef convert_item_to_object(self, char *itemp):
- * if self.to_object_func != NULL: # <<<<<<<<<<<<<<
- * return self.to_object_func(itemp)
- * else:
- */
- __pyx_t_1 = ((__pyx_v_self->to_object_func != NULL) != 0);
- if (__pyx_t_1) {
-
- /* "View.MemoryView":981
- * cdef convert_item_to_object(self, char *itemp):
- * if self.to_object_func != NULL:
- * return self.to_object_func(itemp) # <<<<<<<<<<<<<<
- * else:
- * return memoryview.convert_item_to_object(self, itemp)
- */
- __Pyx_XDECREF(__pyx_r);
- __pyx_t_2 = __pyx_v_self->to_object_func(__pyx_v_itemp); if (unlikely(!__pyx_t_2)) __PYX_ERR(1, 981, __pyx_L1_error)
- __Pyx_GOTREF(__pyx_t_2);
- __pyx_r = __pyx_t_2;
- __pyx_t_2 = 0;
- goto __pyx_L0;
-
- /* "View.MemoryView":980
- *
- * cdef convert_item_to_object(self, char *itemp):
- * if self.to_object_func != NULL: # <<<<<<<<<<<<<<
- * return self.to_object_func(itemp)
- * else:
- */
- }
-
- /* "View.MemoryView":983
- * return self.to_object_func(itemp)
- * else:
- * return memoryview.convert_item_to_object(self, itemp) # <<<<<<<<<<<<<<
- *
- * cdef assign_item_from_object(self, char *itemp, object value):
- */
- /*else*/ {
- __Pyx_XDECREF(__pyx_r);
- __pyx_t_2 = __pyx_memoryview_convert_item_to_object(((struct __pyx_memoryview_obj *)__pyx_v_self), __pyx_v_itemp); if (unlikely(!__pyx_t_2)) __PYX_ERR(1, 983, __pyx_L1_error)
- __Pyx_GOTREF(__pyx_t_2);
- __pyx_r = __pyx_t_2;
- __pyx_t_2 = 0;
- goto __pyx_L0;
- }
-
- /* "View.MemoryView":979
- * __PYX_XDEC_MEMVIEW(&self.from_slice, 1)
- *
- * cdef convert_item_to_object(self, char *itemp): # <<<<<<<<<<<<<<
- * if self.to_object_func != NULL:
- * return self.to_object_func(itemp)
- */
-
- /* function exit code */
- __pyx_L1_error:;
- __Pyx_XDECREF(__pyx_t_2);
- __Pyx_AddTraceback("View.MemoryView._memoryviewslice.convert_item_to_object", __pyx_clineno, __pyx_lineno, __pyx_filename);
- __pyx_r = 0;
- __pyx_L0:;
- __Pyx_XGIVEREF(__pyx_r);
- __Pyx_RefNannyFinishContext();
- return __pyx_r;
-}
-
-/* "View.MemoryView":985
- * return memoryview.convert_item_to_object(self, itemp)
- *
- * cdef assign_item_from_object(self, char *itemp, object value): # <<<<<<<<<<<<<<
- * if self.to_dtype_func != NULL:
- * self.to_dtype_func(itemp, value)
- */
-
-static PyObject *__pyx_memoryviewslice_assign_item_from_object(struct __pyx_memoryviewslice_obj *__pyx_v_self, char *__pyx_v_itemp, PyObject *__pyx_v_value) {
- PyObject *__pyx_r = NULL;
- __Pyx_RefNannyDeclarations
- int __pyx_t_1;
- int __pyx_t_2;
- PyObject *__pyx_t_3 = NULL;
- int __pyx_lineno = 0;
- const char *__pyx_filename = NULL;
- int __pyx_clineno = 0;
- __Pyx_RefNannySetupContext("assign_item_from_object", 0);
-
- /* "View.MemoryView":986
- *
- * cdef assign_item_from_object(self, char *itemp, object value):
- * if self.to_dtype_func != NULL: # <<<<<<<<<<<<<<
- * self.to_dtype_func(itemp, value)
- * else:
- */
- __pyx_t_1 = ((__pyx_v_self->to_dtype_func != NULL) != 0);
- if (__pyx_t_1) {
-
- /* "View.MemoryView":987
- * cdef assign_item_from_object(self, char *itemp, object value):
- * if self.to_dtype_func != NULL:
- * self.to_dtype_func(itemp, value) # <<<<<<<<<<<<<<
- * else:
- * memoryview.assign_item_from_object(self, itemp, value)
- */
- __pyx_t_2 = __pyx_v_self->to_dtype_func(__pyx_v_itemp, __pyx_v_value); if (unlikely(__pyx_t_2 == ((int)0))) __PYX_ERR(1, 987, __pyx_L1_error)
-
- /* "View.MemoryView":986
- *
- * cdef assign_item_from_object(self, char *itemp, object value):
- * if self.to_dtype_func != NULL: # <<<<<<<<<<<<<<
- * self.to_dtype_func(itemp, value)
- * else:
- */
- goto __pyx_L3;
- }
-
- /* "View.MemoryView":989
- * self.to_dtype_func(itemp, value)
- * else:
- * memoryview.assign_item_from_object(self, itemp, value) # <<<<<<<<<<<<<<
- *
- * @property
- */
- /*else*/ {
- __pyx_t_3 = __pyx_memoryview_assign_item_from_object(((struct __pyx_memoryview_obj *)__pyx_v_self), __pyx_v_itemp, __pyx_v_value); if (unlikely(!__pyx_t_3)) __PYX_ERR(1, 989, __pyx_L1_error)
- __Pyx_GOTREF(__pyx_t_3);
- __Pyx_DECREF(__pyx_t_3); __pyx_t_3 = 0;
- }
- __pyx_L3:;
-
- /* "View.MemoryView":985
- * return memoryview.convert_item_to_object(self, itemp)
- *
- * cdef assign_item_from_object(self, char *itemp, object value): # <<<<<<<<<<<<<<
- * if self.to_dtype_func != NULL:
- * self.to_dtype_func(itemp, value)
- */
-
- /* function exit code */
- __pyx_r = Py_None; __Pyx_INCREF(Py_None);
- goto __pyx_L0;
- __pyx_L1_error:;
- __Pyx_XDECREF(__pyx_t_3);
- __Pyx_AddTraceback("View.MemoryView._memoryviewslice.assign_item_from_object", __pyx_clineno, __pyx_lineno, __pyx_filename);
- __pyx_r = 0;
- __pyx_L0:;
- __Pyx_XGIVEREF(__pyx_r);
- __Pyx_RefNannyFinishContext();
- return __pyx_r;
-}
-
-/* "View.MemoryView":992
- *
- * @property
- * def base(self): # <<<<<<<<<<<<<<
- * return self.from_object
- *
- */
-
-/* Python wrapper */
-static PyObject *__pyx_pw_15View_dot_MemoryView_16_memoryviewslice_4base_1__get__(PyObject *__pyx_v_self); /*proto*/
-static PyObject *__pyx_pw_15View_dot_MemoryView_16_memoryviewslice_4base_1__get__(PyObject *__pyx_v_self) {
- PyObject *__pyx_r = 0;
- __Pyx_RefNannyDeclarations
- __Pyx_RefNannySetupContext("__get__ (wrapper)", 0);
- __pyx_r = __pyx_pf_15View_dot_MemoryView_16_memoryviewslice_4base___get__(((struct __pyx_memoryviewslice_obj *)__pyx_v_self));
-
- /* function exit code */
- __Pyx_RefNannyFinishContext();
- return __pyx_r;
-}
-
-static PyObject *__pyx_pf_15View_dot_MemoryView_16_memoryviewslice_4base___get__(struct __pyx_memoryviewslice_obj *__pyx_v_self) {
- PyObject *__pyx_r = NULL;
- __Pyx_RefNannyDeclarations
- __Pyx_RefNannySetupContext("__get__", 0);
-
- /* "View.MemoryView":993
- * @property
- * def base(self):
- * return self.from_object # <<<<<<<<<<<<<<
- *
- * __pyx_getbuffer = capsule( &__pyx_memoryview_getbuffer, "getbuffer(obj, view, flags)")
- */
- __Pyx_XDECREF(__pyx_r);
- __Pyx_INCREF(__pyx_v_self->from_object);
- __pyx_r = __pyx_v_self->from_object;
- goto __pyx_L0;
-
- /* "View.MemoryView":992
- *
- * @property
- * def base(self): # <<<<<<<<<<<<<<
- * return self.from_object
- *
- */
-
- /* function exit code */
- __pyx_L0:;
- __Pyx_XGIVEREF(__pyx_r);
- __Pyx_RefNannyFinishContext();
- return __pyx_r;
-}
-
-/* "(tree fragment)":1
- * def __reduce_cython__(self): # <<<<<<<<<<<<<<
- * raise TypeError("no default __reduce__ due to non-trivial __cinit__")
- * def __setstate_cython__(self, __pyx_state):
- */
-
-/* Python wrapper */
-static PyObject *__pyx_pw___pyx_memoryviewslice_1__reduce_cython__(PyObject *__pyx_v_self, CYTHON_UNUSED PyObject *unused); /*proto*/
-static PyObject *__pyx_pw___pyx_memoryviewslice_1__reduce_cython__(PyObject *__pyx_v_self, CYTHON_UNUSED PyObject *unused) {
- PyObject *__pyx_r = 0;
- __Pyx_RefNannyDeclarations
- __Pyx_RefNannySetupContext("__reduce_cython__ (wrapper)", 0);
- __pyx_r = __pyx_pf___pyx_memoryviewslice___reduce_cython__(((struct __pyx_memoryviewslice_obj *)__pyx_v_self));
-
- /* function exit code */
- __Pyx_RefNannyFinishContext();
- return __pyx_r;
-}
-
-static PyObject *__pyx_pf___pyx_memoryviewslice___reduce_cython__(CYTHON_UNUSED struct __pyx_memoryviewslice_obj *__pyx_v_self) {
- PyObject *__pyx_r = NULL;
- __Pyx_RefNannyDeclarations
- PyObject *__pyx_t_1 = NULL;
- int __pyx_lineno = 0;
- const char *__pyx_filename = NULL;
- int __pyx_clineno = 0;
- __Pyx_RefNannySetupContext("__reduce_cython__", 0);
-
- /* "(tree fragment)":2
- * def __reduce_cython__(self):
- * raise TypeError("no default __reduce__ due to non-trivial __cinit__") # <<<<<<<<<<<<<<
- * def __setstate_cython__(self, __pyx_state):
- * raise TypeError("no default __reduce__ due to non-trivial __cinit__")
- */
- __pyx_t_1 = __Pyx_PyObject_Call(__pyx_builtin_TypeError, __pyx_tuple__18, NULL); if (unlikely(!__pyx_t_1)) __PYX_ERR(1, 2, __pyx_L1_error)
- __Pyx_GOTREF(__pyx_t_1);
- __Pyx_Raise(__pyx_t_1, 0, 0, 0);
- __Pyx_DECREF(__pyx_t_1); __pyx_t_1 = 0;
- __PYX_ERR(1, 2, __pyx_L1_error)
-
- /* "(tree fragment)":1
- * def __reduce_cython__(self): # <<<<<<<<<<<<<<
- * raise TypeError("no default __reduce__ due to non-trivial __cinit__")
- * def __setstate_cython__(self, __pyx_state):
- */
-
- /* function exit code */
- __pyx_L1_error:;
- __Pyx_XDECREF(__pyx_t_1);
- __Pyx_AddTraceback("View.MemoryView._memoryviewslice.__reduce_cython__", __pyx_clineno, __pyx_lineno, __pyx_filename);
- __pyx_r = NULL;
- __Pyx_XGIVEREF(__pyx_r);
- __Pyx_RefNannyFinishContext();
- return __pyx_r;
-}
-
-/* "(tree fragment)":3
- * def __reduce_cython__(self):
- * raise TypeError("no default __reduce__ due to non-trivial __cinit__")
- * def __setstate_cython__(self, __pyx_state): # <<<<<<<<<<<<<<
- * raise TypeError("no default __reduce__ due to non-trivial __cinit__")
- */
-
-/* Python wrapper */
-static PyObject *__pyx_pw___pyx_memoryviewslice_3__setstate_cython__(PyObject *__pyx_v_self, PyObject *__pyx_v___pyx_state); /*proto*/
-static PyObject *__pyx_pw___pyx_memoryviewslice_3__setstate_cython__(PyObject *__pyx_v_self, PyObject *__pyx_v___pyx_state) {
- PyObject *__pyx_r = 0;
- __Pyx_RefNannyDeclarations
- __Pyx_RefNannySetupContext("__setstate_cython__ (wrapper)", 0);
- __pyx_r = __pyx_pf___pyx_memoryviewslice_2__setstate_cython__(((struct __pyx_memoryviewslice_obj *)__pyx_v_self), ((PyObject *)__pyx_v___pyx_state));
-
- /* function exit code */
- __Pyx_RefNannyFinishContext();
- return __pyx_r;
-}
-
-static PyObject *__pyx_pf___pyx_memoryviewslice_2__setstate_cython__(CYTHON_UNUSED struct __pyx_memoryviewslice_obj *__pyx_v_self, CYTHON_UNUSED PyObject *__pyx_v___pyx_state) {
- PyObject *__pyx_r = NULL;
- __Pyx_RefNannyDeclarations
- PyObject *__pyx_t_1 = NULL;
- int __pyx_lineno = 0;
- const char *__pyx_filename = NULL;
- int __pyx_clineno = 0;
- __Pyx_RefNannySetupContext("__setstate_cython__", 0);
-
- /* "(tree fragment)":4
- * raise TypeError("no default __reduce__ due to non-trivial __cinit__")
- * def __setstate_cython__(self, __pyx_state):
- * raise TypeError("no default __reduce__ due to non-trivial __cinit__") # <<<<<<<<<<<<<<
- */
- __pyx_t_1 = __Pyx_PyObject_Call(__pyx_builtin_TypeError, __pyx_tuple__19, NULL); if (unlikely(!__pyx_t_1)) __PYX_ERR(1, 4, __pyx_L1_error)
- __Pyx_GOTREF(__pyx_t_1);
- __Pyx_Raise(__pyx_t_1, 0, 0, 0);
- __Pyx_DECREF(__pyx_t_1); __pyx_t_1 = 0;
- __PYX_ERR(1, 4, __pyx_L1_error)
-
- /* "(tree fragment)":3
- * def __reduce_cython__(self):
- * raise TypeError("no default __reduce__ due to non-trivial __cinit__")
- * def __setstate_cython__(self, __pyx_state): # <<<<<<<<<<<<<<
- * raise TypeError("no default __reduce__ due to non-trivial __cinit__")
- */
-
- /* function exit code */
- __pyx_L1_error:;
- __Pyx_XDECREF(__pyx_t_1);
- __Pyx_AddTraceback("View.MemoryView._memoryviewslice.__setstate_cython__", __pyx_clineno, __pyx_lineno, __pyx_filename);
- __pyx_r = NULL;
- __Pyx_XGIVEREF(__pyx_r);
- __Pyx_RefNannyFinishContext();
- return __pyx_r;
-}
-
-/* "View.MemoryView":999
- *
- * @cname('__pyx_memoryview_fromslice')
- * cdef memoryview_fromslice(__Pyx_memviewslice memviewslice, # <<<<<<<<<<<<<<
- * int ndim,
- * object (*to_object_func)(char *),
- */
-
-static PyObject *__pyx_memoryview_fromslice(__Pyx_memviewslice __pyx_v_memviewslice, int __pyx_v_ndim, PyObject *(*__pyx_v_to_object_func)(char *), int (*__pyx_v_to_dtype_func)(char *, PyObject *), int __pyx_v_dtype_is_object) {
- struct __pyx_memoryviewslice_obj *__pyx_v_result = 0;
- Py_ssize_t __pyx_v_suboffset;
- PyObject *__pyx_v_length = NULL;
- PyObject *__pyx_r = NULL;
- __Pyx_RefNannyDeclarations
- int __pyx_t_1;
- PyObject *__pyx_t_2 = NULL;
- PyObject *__pyx_t_3 = NULL;
- __Pyx_TypeInfo *__pyx_t_4;
- Py_buffer __pyx_t_5;
- Py_ssize_t *__pyx_t_6;
- Py_ssize_t *__pyx_t_7;
- Py_ssize_t *__pyx_t_8;
- Py_ssize_t __pyx_t_9;
- int __pyx_lineno = 0;
- const char *__pyx_filename = NULL;
- int __pyx_clineno = 0;
- __Pyx_RefNannySetupContext("memoryview_fromslice", 0);
-
- /* "View.MemoryView":1007
- * cdef _memoryviewslice result
- *
- * if memviewslice.memview == Py_None: # <<<<<<<<<<<<<<
- * return None
- *
- */
- __pyx_t_1 = ((((PyObject *)__pyx_v_memviewslice.memview) == Py_None) != 0);
- if (__pyx_t_1) {
-
- /* "View.MemoryView":1008
- *
- * if memviewslice.memview == Py_None:
- * return None # <<<<<<<<<<<<<<
- *
- *
- */
- __Pyx_XDECREF(__pyx_r);
- __pyx_r = Py_None; __Pyx_INCREF(Py_None);
- goto __pyx_L0;
-
- /* "View.MemoryView":1007
- * cdef _memoryviewslice result
- *
- * if memviewslice.memview == Py_None: # <<<<<<<<<<<<<<
- * return None
- *
- */
- }
-
- /* "View.MemoryView":1013
- *
- *
- * result = _memoryviewslice(None, 0, dtype_is_object) # <<<<<<<<<<<<<<
- *
- * result.from_slice = memviewslice
- */
- __pyx_t_2 = __Pyx_PyBool_FromLong(__pyx_v_dtype_is_object); if (unlikely(!__pyx_t_2)) __PYX_ERR(1, 1013, __pyx_L1_error)
- __Pyx_GOTREF(__pyx_t_2);
- __pyx_t_3 = PyTuple_New(3); if (unlikely(!__pyx_t_3)) __PYX_ERR(1, 1013, __pyx_L1_error)
- __Pyx_GOTREF(__pyx_t_3);
- __Pyx_INCREF(Py_None);
- __Pyx_GIVEREF(Py_None);
- PyTuple_SET_ITEM(__pyx_t_3, 0, Py_None);
- __Pyx_INCREF(__pyx_int_0);
- __Pyx_GIVEREF(__pyx_int_0);
- PyTuple_SET_ITEM(__pyx_t_3, 1, __pyx_int_0);
- __Pyx_GIVEREF(__pyx_t_2);
- PyTuple_SET_ITEM(__pyx_t_3, 2, __pyx_t_2);
- __pyx_t_2 = 0;
- __pyx_t_2 = __Pyx_PyObject_Call(((PyObject *)__pyx_memoryviewslice_type), __pyx_t_3, NULL); if (unlikely(!__pyx_t_2)) __PYX_ERR(1, 1013, __pyx_L1_error)
- __Pyx_GOTREF(__pyx_t_2);
- __Pyx_DECREF(__pyx_t_3); __pyx_t_3 = 0;
- __pyx_v_result = ((struct __pyx_memoryviewslice_obj *)__pyx_t_2);
- __pyx_t_2 = 0;
-
- /* "View.MemoryView":1015
- * result = _memoryviewslice(None, 0, dtype_is_object)
- *
- * result.from_slice = memviewslice # <<<<<<<<<<<<<<
- * __PYX_INC_MEMVIEW(&memviewslice, 1)
- *
- */
- __pyx_v_result->from_slice = __pyx_v_memviewslice;
-
- /* "View.MemoryView":1016
- *
- * result.from_slice = memviewslice
- * __PYX_INC_MEMVIEW(&memviewslice, 1) # <<<<<<<<<<<<<<
- *
- * result.from_object = ( memviewslice.memview).base
- */
- __PYX_INC_MEMVIEW((&__pyx_v_memviewslice), 1);
-
- /* "View.MemoryView":1018
- * __PYX_INC_MEMVIEW(&memviewslice, 1)
- *
- * result.from_object = ( memviewslice.memview).base # <<<<<<<<<<<<<<
- * result.typeinfo = memviewslice.memview.typeinfo
- *
- */
- __pyx_t_2 = __Pyx_PyObject_GetAttrStr(((PyObject *)__pyx_v_memviewslice.memview), __pyx_n_s_base); if (unlikely(!__pyx_t_2)) __PYX_ERR(1, 1018, __pyx_L1_error)
- __Pyx_GOTREF(__pyx_t_2);
- __Pyx_GIVEREF(__pyx_t_2);
- __Pyx_GOTREF(__pyx_v_result->from_object);
- __Pyx_DECREF(__pyx_v_result->from_object);
- __pyx_v_result->from_object = __pyx_t_2;
- __pyx_t_2 = 0;
-
- /* "View.MemoryView":1019
- *
- * result.from_object = ( memviewslice.memview).base
- * result.typeinfo = memviewslice.memview.typeinfo # <<<<<<<<<<<<<<
- *
- * result.view = memviewslice.memview.view
- */
- __pyx_t_4 = __pyx_v_memviewslice.memview->typeinfo;
- __pyx_v_result->__pyx_base.typeinfo = __pyx_t_4;
-
- /* "View.MemoryView":1021
- * result.typeinfo = memviewslice.memview.typeinfo
- *
- * result.view = memviewslice.memview.view # <<<<<<<<<<<<<<
- * result.view.buf = memviewslice.data
- * result.view.ndim = ndim
- */
- __pyx_t_5 = __pyx_v_memviewslice.memview->view;
- __pyx_v_result->__pyx_base.view = __pyx_t_5;
-
- /* "View.MemoryView":1022
- *
- * result.view = memviewslice.memview.view
- * result.view.buf = memviewslice.data # <<<<<<<<<<<<<<
- * result.view.ndim = ndim
- * (<__pyx_buffer *> &result.view).obj = Py_None
- */
- __pyx_v_result->__pyx_base.view.buf = ((void *)__pyx_v_memviewslice.data);
-
- /* "View.MemoryView":1023
- * result.view = memviewslice.memview.view
- * result.view.buf = memviewslice.data
- * result.view.ndim = ndim # <<<<<<<<<<<<<<
- * (<__pyx_buffer *> &result.view).obj = Py_None
- * Py_INCREF(Py_None)
- */
- __pyx_v_result->__pyx_base.view.ndim = __pyx_v_ndim;
-
- /* "View.MemoryView":1024
- * result.view.buf = memviewslice.data
- * result.view.ndim = ndim
- * (<__pyx_buffer *> &result.view).obj = Py_None # <<<<<<<<<<<<<<
- * Py_INCREF(Py_None)
- *
- */
- ((Py_buffer *)(&__pyx_v_result->__pyx_base.view))->obj = Py_None;
-
- /* "View.MemoryView":1025
- * result.view.ndim = ndim
- * (<__pyx_buffer *> &result.view).obj = Py_None
- * Py_INCREF(Py_None) # <<<<<<<<<<<<<<
- *
- * if (memviewslice.memview).flags & PyBUF_WRITABLE:
- */
- Py_INCREF(Py_None);
-
- /* "View.MemoryView":1027
- * Py_INCREF(Py_None)
- *
- * if (memviewslice.memview).flags & PyBUF_WRITABLE: # <<<<<<<<<<<<<<
- * result.flags = PyBUF_RECORDS
- * else:
- */
- __pyx_t_1 = ((((struct __pyx_memoryview_obj *)__pyx_v_memviewslice.memview)->flags & PyBUF_WRITABLE) != 0);
- if (__pyx_t_1) {
-
- /* "View.MemoryView":1028
- *
- * if (memviewslice.memview).flags & PyBUF_WRITABLE:
- * result.flags = PyBUF_RECORDS # <<<<<<<<<<<<<<
- * else:
- * result.flags = PyBUF_RECORDS_RO
- */
- __pyx_v_result->__pyx_base.flags = PyBUF_RECORDS;
-
- /* "View.MemoryView":1027
- * Py_INCREF(Py_None)
- *
- * if (memviewslice.memview).flags & PyBUF_WRITABLE: # <<<<<<<<<<<<<<
- * result.flags = PyBUF_RECORDS
- * else:
- */
- goto __pyx_L4;
- }
-
- /* "View.MemoryView":1030
- * result.flags = PyBUF_RECORDS
- * else:
- * result.flags = PyBUF_RECORDS_RO # <<<<<<<<<<<<<<
- *
- * result.view.shape = result.from_slice.shape
- */
- /*else*/ {
- __pyx_v_result->__pyx_base.flags = PyBUF_RECORDS_RO;
- }
- __pyx_L4:;
-
- /* "View.MemoryView":1032
- * result.flags = PyBUF_RECORDS_RO
- *
- * result.view.shape = result.from_slice.shape # <<<<<<<<<<<<<<
- * result.view.strides = result.from_slice.strides
- *
- */
- __pyx_v_result->__pyx_base.view.shape = ((Py_ssize_t *)__pyx_v_result->from_slice.shape);
-
- /* "View.MemoryView":1033
- *
- * result.view.shape = result.from_slice.shape
- * result.view.strides = result.from_slice.strides # <<<<<<<<<<<<<<
- *
- *
- */
- __pyx_v_result->__pyx_base.view.strides = ((Py_ssize_t *)__pyx_v_result->from_slice.strides);
-
- /* "View.MemoryView":1036
- *
- *
- * result.view.suboffsets = NULL # <<<<<<<<<<<<<<
- * for suboffset in result.from_slice.suboffsets[:ndim]:
- * if suboffset >= 0:
- */
- __pyx_v_result->__pyx_base.view.suboffsets = NULL;
-
- /* "View.MemoryView":1037
- *
- * result.view.suboffsets = NULL
- * for suboffset in result.from_slice.suboffsets[:ndim]: # <<<<<<<<<<<<<<
- * if suboffset >= 0:
- * result.view.suboffsets = result.from_slice.suboffsets
- */
- __pyx_t_7 = (__pyx_v_result->from_slice.suboffsets + __pyx_v_ndim);
- for (__pyx_t_8 = __pyx_v_result->from_slice.suboffsets; __pyx_t_8 < __pyx_t_7; __pyx_t_8++) {
- __pyx_t_6 = __pyx_t_8;
- __pyx_v_suboffset = (__pyx_t_6[0]);
-
- /* "View.MemoryView":1038
- * result.view.suboffsets = NULL
- * for suboffset in result.from_slice.suboffsets[:ndim]:
- * if suboffset >= 0: # <<<<<<<<<<<<<<
- * result.view.suboffsets = result.from_slice.suboffsets
- * break
- */
- __pyx_t_1 = ((__pyx_v_suboffset >= 0) != 0);
- if (__pyx_t_1) {
-
- /* "View.MemoryView":1039
- * for suboffset in result.from_slice.suboffsets[:ndim]:
- * if suboffset >= 0:
- * result.view.suboffsets = result.from_slice.suboffsets # <<<<<<<<<<<<<<
- * break
- *
- */
- __pyx_v_result->__pyx_base.view.suboffsets = ((Py_ssize_t *)__pyx_v_result->from_slice.suboffsets);
-
- /* "View.MemoryView":1040
- * if suboffset >= 0:
- * result.view.suboffsets = result.from_slice.suboffsets
- * break # <<<<<<<<<<<<<<
- *
- * result.view.len = result.view.itemsize
- */
- goto __pyx_L6_break;
-
- /* "View.MemoryView":1038
- * result.view.suboffsets = NULL
- * for suboffset in result.from_slice.suboffsets[:ndim]:
- * if suboffset >= 0: # <<<<<<<<<<<<<<
- * result.view.suboffsets = result.from_slice.suboffsets
- * break
- */
- }
- }
- __pyx_L6_break:;
-
- /* "View.MemoryView":1042
- * break
- *
- * result.view.len = result.view.itemsize # <<<<<<<<<<<<<<
- * for length in result.view.shape[:ndim]:
- * result.view.len *= length
- */
- __pyx_t_9 = __pyx_v_result->__pyx_base.view.itemsize;
- __pyx_v_result->__pyx_base.view.len = __pyx_t_9;
-
- /* "View.MemoryView":1043
- *
- * result.view.len = result.view.itemsize
- * for length in result.view.shape[:ndim]: # <<<<<<<<<<<<<<
- * result.view.len *= length
- *
- */
- __pyx_t_7 = (__pyx_v_result->__pyx_base.view.shape + __pyx_v_ndim);
- for (__pyx_t_8 = __pyx_v_result->__pyx_base.view.shape; __pyx_t_8 < __pyx_t_7; __pyx_t_8++) {
- __pyx_t_6 = __pyx_t_8;
- __pyx_t_2 = PyInt_FromSsize_t((__pyx_t_6[0])); if (unlikely(!__pyx_t_2)) __PYX_ERR(1, 1043, __pyx_L1_error)
- __Pyx_GOTREF(__pyx_t_2);
- __Pyx_XDECREF_SET(__pyx_v_length, __pyx_t_2);
- __pyx_t_2 = 0;
-
- /* "View.MemoryView":1044
- * result.view.len = result.view.itemsize
- * for length in result.view.shape[:ndim]:
- * result.view.len *= length # <<<<<<<<<<<<<<
- *
- * result.to_object_func = to_object_func
- */
- __pyx_t_2 = PyInt_FromSsize_t(__pyx_v_result->__pyx_base.view.len); if (unlikely(!__pyx_t_2)) __PYX_ERR(1, 1044, __pyx_L1_error)
- __Pyx_GOTREF(__pyx_t_2);
- __pyx_t_3 = PyNumber_InPlaceMultiply(__pyx_t_2, __pyx_v_length); if (unlikely(!__pyx_t_3)) __PYX_ERR(1, 1044, __pyx_L1_error)
- __Pyx_GOTREF(__pyx_t_3);
- __Pyx_DECREF(__pyx_t_2); __pyx_t_2 = 0;
- __pyx_t_9 = __Pyx_PyIndex_AsSsize_t(__pyx_t_3); if (unlikely((__pyx_t_9 == (Py_ssize_t)-1) && PyErr_Occurred())) __PYX_ERR(1, 1044, __pyx_L1_error)
- __Pyx_DECREF(__pyx_t_3); __pyx_t_3 = 0;
- __pyx_v_result->__pyx_base.view.len = __pyx_t_9;
- }
-
- /* "View.MemoryView":1046
- * result.view.len *= length
- *
- * result.to_object_func = to_object_func # <<<<<<<<<<<<<<
- * result.to_dtype_func = to_dtype_func
- *
- */
- __pyx_v_result->to_object_func = __pyx_v_to_object_func;
-
- /* "View.MemoryView":1047
- *
- * result.to_object_func = to_object_func
- * result.to_dtype_func = to_dtype_func # <<<<<<<<<<<<<<
- *
- * return result
- */
- __pyx_v_result->to_dtype_func = __pyx_v_to_dtype_func;
-
- /* "View.MemoryView":1049
- * result.to_dtype_func = to_dtype_func
- *
- * return result # <<<<<<<<<<<<<<
- *
- * @cname('__pyx_memoryview_get_slice_from_memoryview')
- */
- __Pyx_XDECREF(__pyx_r);
- __Pyx_INCREF(((PyObject *)__pyx_v_result));
- __pyx_r = ((PyObject *)__pyx_v_result);
- goto __pyx_L0;
-
- /* "View.MemoryView":999
- *
- * @cname('__pyx_memoryview_fromslice')
- * cdef memoryview_fromslice(__Pyx_memviewslice memviewslice, # <<<<<<<<<<<<<<
- * int ndim,
- * object (*to_object_func)(char *),
- */
-
- /* function exit code */
- __pyx_L1_error:;
- __Pyx_XDECREF(__pyx_t_2);
- __Pyx_XDECREF(__pyx_t_3);
- __Pyx_AddTraceback("View.MemoryView.memoryview_fromslice", __pyx_clineno, __pyx_lineno, __pyx_filename);
- __pyx_r = 0;
- __pyx_L0:;
- __Pyx_XDECREF((PyObject *)__pyx_v_result);
- __Pyx_XDECREF(__pyx_v_length);
- __Pyx_XGIVEREF(__pyx_r);
- __Pyx_RefNannyFinishContext();
- return __pyx_r;
-}
-
-/* "View.MemoryView":1052
- *
- * @cname('__pyx_memoryview_get_slice_from_memoryview')
- * cdef __Pyx_memviewslice *get_slice_from_memview(memoryview memview, # <<<<<<<<<<<<<<
- * __Pyx_memviewslice *mslice) except NULL:
- * cdef _memoryviewslice obj
- */
-
-static __Pyx_memviewslice *__pyx_memoryview_get_slice_from_memoryview(struct __pyx_memoryview_obj *__pyx_v_memview, __Pyx_memviewslice *__pyx_v_mslice) {
- struct __pyx_memoryviewslice_obj *__pyx_v_obj = 0;
- __Pyx_memviewslice *__pyx_r;
- __Pyx_RefNannyDeclarations
- int __pyx_t_1;
- int __pyx_t_2;
- PyObject *__pyx_t_3 = NULL;
- int __pyx_lineno = 0;
- const char *__pyx_filename = NULL;
- int __pyx_clineno = 0;
- __Pyx_RefNannySetupContext("get_slice_from_memview", 0);
-
- /* "View.MemoryView":1055
- * __Pyx_memviewslice *mslice) except NULL:
- * cdef _memoryviewslice obj
- * if isinstance(memview, _memoryviewslice): # <<<<<<<<<<<<<<
- * obj = memview
- * return &obj.from_slice
- */
- __pyx_t_1 = __Pyx_TypeCheck(((PyObject *)__pyx_v_memview), __pyx_memoryviewslice_type);
- __pyx_t_2 = (__pyx_t_1 != 0);
- if (__pyx_t_2) {
-
- /* "View.MemoryView":1056
- * cdef _memoryviewslice obj
- * if isinstance(memview, _memoryviewslice):
- * obj = memview # <<<<<<<<<<<<<<
- * return &obj.from_slice
- * else:
- */
- if (!(likely(((((PyObject *)__pyx_v_memview)) == Py_None) || likely(__Pyx_TypeTest(((PyObject *)__pyx_v_memview), __pyx_memoryviewslice_type))))) __PYX_ERR(1, 1056, __pyx_L1_error)
- __pyx_t_3 = ((PyObject *)__pyx_v_memview);
- __Pyx_INCREF(__pyx_t_3);
- __pyx_v_obj = ((struct __pyx_memoryviewslice_obj *)__pyx_t_3);
- __pyx_t_3 = 0;
-
- /* "View.MemoryView":1057
- * if isinstance(memview, _memoryviewslice):
- * obj = memview
- * return &obj.from_slice # <<<<<<<<<<<<<<
- * else:
- * slice_copy(memview, mslice)
- */
- __pyx_r = (&__pyx_v_obj->from_slice);
- goto __pyx_L0;
-
- /* "View.MemoryView":1055
- * __Pyx_memviewslice *mslice) except NULL:
- * cdef _memoryviewslice obj
- * if isinstance(memview, _memoryviewslice): # <<<<<<<<<<<<<<
- * obj = memview
- * return &obj.from_slice
- */
- }
-
- /* "View.MemoryView":1059
- * return &obj.from_slice
- * else:
- * slice_copy(memview, mslice) # <<<<<<<<<<<<<<
- * return mslice
- *
- */
- /*else*/ {
- __pyx_memoryview_slice_copy(__pyx_v_memview, __pyx_v_mslice);
-
- /* "View.MemoryView":1060
- * else:
- * slice_copy(memview, mslice)
- * return mslice # <<<<<<<<<<<<<<
- *
- * @cname('__pyx_memoryview_slice_copy')
- */
- __pyx_r = __pyx_v_mslice;
- goto __pyx_L0;
- }
-
- /* "View.MemoryView":1052
- *
- * @cname('__pyx_memoryview_get_slice_from_memoryview')
- * cdef __Pyx_memviewslice *get_slice_from_memview(memoryview memview, # <<<<<<<<<<<<<<
- * __Pyx_memviewslice *mslice) except NULL:
- * cdef _memoryviewslice obj
- */
-
- /* function exit code */
- __pyx_L1_error:;
- __Pyx_XDECREF(__pyx_t_3);
- __Pyx_AddTraceback("View.MemoryView.get_slice_from_memview", __pyx_clineno, __pyx_lineno, __pyx_filename);
- __pyx_r = NULL;
- __pyx_L0:;
- __Pyx_XDECREF((PyObject *)__pyx_v_obj);
- __Pyx_RefNannyFinishContext();
- return __pyx_r;
-}
-
-/* "View.MemoryView":1063
- *
- * @cname('__pyx_memoryview_slice_copy')
- * cdef void slice_copy(memoryview memview, __Pyx_memviewslice *dst): # <<<<<<<<<<<<<<
- * cdef int dim
- * cdef (Py_ssize_t*) shape, strides, suboffsets
- */
-
-static void __pyx_memoryview_slice_copy(struct __pyx_memoryview_obj *__pyx_v_memview, __Pyx_memviewslice *__pyx_v_dst) {
- int __pyx_v_dim;
- Py_ssize_t *__pyx_v_shape;
- Py_ssize_t *__pyx_v_strides;
- Py_ssize_t *__pyx_v_suboffsets;
- __Pyx_RefNannyDeclarations
- Py_ssize_t *__pyx_t_1;
- int __pyx_t_2;
- int __pyx_t_3;
- int __pyx_t_4;
- Py_ssize_t __pyx_t_5;
- __Pyx_RefNannySetupContext("slice_copy", 0);
-
- /* "View.MemoryView":1067
- * cdef (Py_ssize_t*) shape, strides, suboffsets
- *
- * shape = memview.view.shape # <<<<<<<<<<<<<<
- * strides = memview.view.strides
- * suboffsets = memview.view.suboffsets
- */
- __pyx_t_1 = __pyx_v_memview->view.shape;
- __pyx_v_shape = __pyx_t_1;
-
- /* "View.MemoryView":1068
- *
- * shape = memview.view.shape
- * strides = memview.view.strides # <<<<<<<<<<<<<<
- * suboffsets = memview.view.suboffsets
- *
- */
- __pyx_t_1 = __pyx_v_memview->view.strides;
- __pyx_v_strides = __pyx_t_1;
-
- /* "View.MemoryView":1069
- * shape = memview.view.shape
- * strides = memview.view.strides
- * suboffsets = memview.view.suboffsets # <<<<<<<<<<<<<<
- *
- * dst.memview = <__pyx_memoryview *> memview
- */
- __pyx_t_1 = __pyx_v_memview->view.suboffsets;
- __pyx_v_suboffsets = __pyx_t_1;
-
- /* "View.MemoryView":1071
- * suboffsets = memview.view.suboffsets
- *
- * dst.memview = <__pyx_memoryview *> memview # <<<<<<<<<<<<<<
- * dst.data = memview.view.buf
- *
- */
- __pyx_v_dst->memview = ((struct __pyx_memoryview_obj *)__pyx_v_memview);
-
- /* "View.MemoryView":1072
- *
- * dst.memview = <__pyx_memoryview *> memview
- * dst.data = memview.view.buf # <<<<<<<<<<<<<<
- *
- * for dim in range(memview.view.ndim):
- */
- __pyx_v_dst->data = ((char *)__pyx_v_memview->view.buf);
-
- /* "View.MemoryView":1074
- * dst.data = memview.view.buf
- *
- * for dim in range(memview.view.ndim): # <<<<<<<<<<<<<<
- * dst.shape[dim] = shape[dim]
- * dst.strides[dim] = strides[dim]
- */
- __pyx_t_2 = __pyx_v_memview->view.ndim;
- __pyx_t_3 = __pyx_t_2;
- for (__pyx_t_4 = 0; __pyx_t_4 < __pyx_t_3; __pyx_t_4+=1) {
- __pyx_v_dim = __pyx_t_4;
-
- /* "View.MemoryView":1075
- *
- * for dim in range(memview.view.ndim):
- * dst.shape[dim] = shape[dim] # <<<<<<<<<<<<<<
- * dst.strides[dim] = strides[dim]
- * dst.suboffsets[dim] = suboffsets[dim] if suboffsets else -1
- */
- (__pyx_v_dst->shape[__pyx_v_dim]) = (__pyx_v_shape[__pyx_v_dim]);
-
- /* "View.MemoryView":1076
- * for dim in range(memview.view.ndim):
- * dst.shape[dim] = shape[dim]
- * dst.strides[dim] = strides[dim] # <<<<<<<<<<<<<<
- * dst.suboffsets[dim] = suboffsets[dim] if suboffsets else -1
- *
- */
- (__pyx_v_dst->strides[__pyx_v_dim]) = (__pyx_v_strides[__pyx_v_dim]);
-
- /* "View.MemoryView":1077
- * dst.shape[dim] = shape[dim]
- * dst.strides[dim] = strides[dim]
- * dst.suboffsets[dim] = suboffsets[dim] if suboffsets else -1 # <<<<<<<<<<<<<<
- *
- * @cname('__pyx_memoryview_copy_object')
- */
- if ((__pyx_v_suboffsets != 0)) {
- __pyx_t_5 = (__pyx_v_suboffsets[__pyx_v_dim]);
- } else {
- __pyx_t_5 = -1L;
- }
- (__pyx_v_dst->suboffsets[__pyx_v_dim]) = __pyx_t_5;
- }
-
- /* "View.MemoryView":1063
- *
- * @cname('__pyx_memoryview_slice_copy')
- * cdef void slice_copy(memoryview memview, __Pyx_memviewslice *dst): # <<<<<<<<<<<<<<
- * cdef int dim
- * cdef (Py_ssize_t*) shape, strides, suboffsets
- */
-
- /* function exit code */
- __Pyx_RefNannyFinishContext();
-}
-
-/* "View.MemoryView":1080
- *
- * @cname('__pyx_memoryview_copy_object')
- * cdef memoryview_copy(memoryview memview): # <<<<<<<<<<<<<<
- * "Create a new memoryview object"
- * cdef __Pyx_memviewslice memviewslice
- */
-
-static PyObject *__pyx_memoryview_copy_object(struct __pyx_memoryview_obj *__pyx_v_memview) {
- __Pyx_memviewslice __pyx_v_memviewslice;
- PyObject *__pyx_r = NULL;
- __Pyx_RefNannyDeclarations
- PyObject *__pyx_t_1 = NULL;
- int __pyx_lineno = 0;
- const char *__pyx_filename = NULL;
- int __pyx_clineno = 0;
- __Pyx_RefNannySetupContext("memoryview_copy", 0);
-
- /* "View.MemoryView":1083
- * "Create a new memoryview object"
- * cdef __Pyx_memviewslice memviewslice
- * slice_copy(memview, &memviewslice) # <<<<<<<<<<<<<<
- * return memoryview_copy_from_slice(memview, &memviewslice)
- *
- */
- __pyx_memoryview_slice_copy(__pyx_v_memview, (&__pyx_v_memviewslice));
-
- /* "View.MemoryView":1084
- * cdef __Pyx_memviewslice memviewslice
- * slice_copy(memview, &memviewslice)
- * return memoryview_copy_from_slice(memview, &memviewslice) # <<<<<<<<<<<<<<
- *
- * @cname('__pyx_memoryview_copy_object_from_slice')
- */
- __Pyx_XDECREF(__pyx_r);
- __pyx_t_1 = __pyx_memoryview_copy_object_from_slice(__pyx_v_memview, (&__pyx_v_memviewslice)); if (unlikely(!__pyx_t_1)) __PYX_ERR(1, 1084, __pyx_L1_error)
- __Pyx_GOTREF(__pyx_t_1);
- __pyx_r = __pyx_t_1;
- __pyx_t_1 = 0;
- goto __pyx_L0;
-
- /* "View.MemoryView":1080
- *
- * @cname('__pyx_memoryview_copy_object')
- * cdef memoryview_copy(memoryview memview): # <<<<<<<<<<<<<<
- * "Create a new memoryview object"
- * cdef __Pyx_memviewslice memviewslice
- */
-
- /* function exit code */
- __pyx_L1_error:;
- __Pyx_XDECREF(__pyx_t_1);
- __Pyx_AddTraceback("View.MemoryView.memoryview_copy", __pyx_clineno, __pyx_lineno, __pyx_filename);
- __pyx_r = 0;
- __pyx_L0:;
- __Pyx_XGIVEREF(__pyx_r);
- __Pyx_RefNannyFinishContext();
- return __pyx_r;
-}
-
-/* "View.MemoryView":1087
- *
- * @cname('__pyx_memoryview_copy_object_from_slice')
- * cdef memoryview_copy_from_slice(memoryview memview, __Pyx_memviewslice *memviewslice): # <<<<<<<<<<<<<<
- * """
- * Create a new memoryview object from a given memoryview object and slice.
- */
-
-static PyObject *__pyx_memoryview_copy_object_from_slice(struct __pyx_memoryview_obj *__pyx_v_memview, __Pyx_memviewslice *__pyx_v_memviewslice) {
- PyObject *(*__pyx_v_to_object_func)(char *);
- int (*__pyx_v_to_dtype_func)(char *, PyObject *);
- PyObject *__pyx_r = NULL;
- __Pyx_RefNannyDeclarations
- int __pyx_t_1;
- int __pyx_t_2;
- PyObject *(*__pyx_t_3)(char *);
- int (*__pyx_t_4)(char *, PyObject *);
- PyObject *__pyx_t_5 = NULL;
- int __pyx_lineno = 0;
- const char *__pyx_filename = NULL;
- int __pyx_clineno = 0;
- __Pyx_RefNannySetupContext("memoryview_copy_from_slice", 0);
-
- /* "View.MemoryView":1094
- * cdef int (*to_dtype_func)(char *, object) except 0
- *
- * if isinstance(memview, _memoryviewslice): # <<<<<<<<<<<<<<
- * to_object_func = (<_memoryviewslice> memview).to_object_func
- * to_dtype_func = (<_memoryviewslice> memview).to_dtype_func
- */
- __pyx_t_1 = __Pyx_TypeCheck(((PyObject *)__pyx_v_memview), __pyx_memoryviewslice_type);
- __pyx_t_2 = (__pyx_t_1 != 0);
- if (__pyx_t_2) {
-
- /* "View.MemoryView":1095
- *
- * if isinstance(memview, _memoryviewslice):
- * to_object_func = (<_memoryviewslice> memview).to_object_func # <<<<<<<<<<<<<<
- * to_dtype_func = (<_memoryviewslice> memview).to_dtype_func
- * else:
- */
- __pyx_t_3 = ((struct __pyx_memoryviewslice_obj *)__pyx_v_memview)->to_object_func;
- __pyx_v_to_object_func = __pyx_t_3;
-
- /* "View.MemoryView":1096
- * if isinstance(memview, _memoryviewslice):
- * to_object_func = (<_memoryviewslice> memview).to_object_func
- * to_dtype_func = (<_memoryviewslice> memview).to_dtype_func # <<<<<<<<<<<<<<
- * else:
- * to_object_func = NULL
- */
- __pyx_t_4 = ((struct __pyx_memoryviewslice_obj *)__pyx_v_memview)->to_dtype_func;
- __pyx_v_to_dtype_func = __pyx_t_4;
-
- /* "View.MemoryView":1094
- * cdef int (*to_dtype_func)(char *, object) except 0
- *
- * if isinstance(memview, _memoryviewslice): # <<<<<<<<<<<<<<
- * to_object_func = (<_memoryviewslice> memview).to_object_func
- * to_dtype_func = (<_memoryviewslice> memview).to_dtype_func
- */
- goto __pyx_L3;
- }
-
- /* "View.MemoryView":1098
- * to_dtype_func = (<_memoryviewslice> memview).to_dtype_func
- * else:
- * to_object_func = NULL # <<<<<<<<<<<<<<
- * to_dtype_func = NULL
- *
- */
- /*else*/ {
- __pyx_v_to_object_func = NULL;
-
- /* "View.MemoryView":1099
- * else:
- * to_object_func = NULL
- * to_dtype_func = NULL # <<<<<<<<<<<<<<
- *
- * return memoryview_fromslice(memviewslice[0], memview.view.ndim,
- */
- __pyx_v_to_dtype_func = NULL;
- }
- __pyx_L3:;
-
- /* "View.MemoryView":1101
- * to_dtype_func = NULL
- *
- * return memoryview_fromslice(memviewslice[0], memview.view.ndim, # <<<<<<<<<<<<<<
- * to_object_func, to_dtype_func,
- * memview.dtype_is_object)
- */
- __Pyx_XDECREF(__pyx_r);
-
- /* "View.MemoryView":1103
- * return memoryview_fromslice(memviewslice[0], memview.view.ndim,
- * to_object_func, to_dtype_func,
- * memview.dtype_is_object) # <<<<<<<<<<<<<<
- *
- *
- */
- __pyx_t_5 = __pyx_memoryview_fromslice((__pyx_v_memviewslice[0]), __pyx_v_memview->view.ndim, __pyx_v_to_object_func, __pyx_v_to_dtype_func, __pyx_v_memview->dtype_is_object); if (unlikely(!__pyx_t_5)) __PYX_ERR(1, 1101, __pyx_L1_error)
- __Pyx_GOTREF(__pyx_t_5);
- __pyx_r = __pyx_t_5;
- __pyx_t_5 = 0;
- goto __pyx_L0;
-
- /* "View.MemoryView":1087
- *
- * @cname('__pyx_memoryview_copy_object_from_slice')
- * cdef memoryview_copy_from_slice(memoryview memview, __Pyx_memviewslice *memviewslice): # <<<<<<<<<<<<<<
- * """
- * Create a new memoryview object from a given memoryview object and slice.
- */
-
- /* function exit code */
- __pyx_L1_error:;
- __Pyx_XDECREF(__pyx_t_5);
- __Pyx_AddTraceback("View.MemoryView.memoryview_copy_from_slice", __pyx_clineno, __pyx_lineno, __pyx_filename);
- __pyx_r = 0;
- __pyx_L0:;
- __Pyx_XGIVEREF(__pyx_r);
- __Pyx_RefNannyFinishContext();
- return __pyx_r;
-}
-
-/* "View.MemoryView":1109
- *
- *
- * cdef Py_ssize_t abs_py_ssize_t(Py_ssize_t arg) nogil: # <<<<<<<<<<<<<<
- * if arg < 0:
- * return -arg
- */
-
-static Py_ssize_t abs_py_ssize_t(Py_ssize_t __pyx_v_arg) {
- Py_ssize_t __pyx_r;
- int __pyx_t_1;
-
- /* "View.MemoryView":1110
- *
- * cdef Py_ssize_t abs_py_ssize_t(Py_ssize_t arg) nogil:
- * if arg < 0: # <<<<<<<<<<<<<<
- * return -arg
- * else:
- */
- __pyx_t_1 = ((__pyx_v_arg < 0) != 0);
- if (__pyx_t_1) {
-
- /* "View.MemoryView":1111
- * cdef Py_ssize_t abs_py_ssize_t(Py_ssize_t arg) nogil:
- * if arg < 0:
- * return -arg # <<<<<<<<<<<<<<
- * else:
- * return arg
- */
- __pyx_r = (-__pyx_v_arg);
- goto __pyx_L0;
-
- /* "View.MemoryView":1110
- *
- * cdef Py_ssize_t abs_py_ssize_t(Py_ssize_t arg) nogil:
- * if arg < 0: # <<<<<<<<<<<<<<
- * return -arg
- * else:
- */
- }
-
- /* "View.MemoryView":1113
- * return -arg
- * else:
- * return arg # <<<<<<<<<<<<<<
- *
- * @cname('__pyx_get_best_slice_order')
- */
- /*else*/ {
- __pyx_r = __pyx_v_arg;
- goto __pyx_L0;
- }
-
- /* "View.MemoryView":1109
- *
- *
- * cdef Py_ssize_t abs_py_ssize_t(Py_ssize_t arg) nogil: # <<<<<<<<<<<<<<
- * if arg < 0:
- * return -arg
- */
-
- /* function exit code */
- __pyx_L0:;
- return __pyx_r;
-}
-
-/* "View.MemoryView":1116
- *
- * @cname('__pyx_get_best_slice_order')
- * cdef char get_best_order(__Pyx_memviewslice *mslice, int ndim) nogil: # <<<<<<<<<<<<<<
- * """
- * Figure out the best memory access order for a given slice.
- */
-
-static char __pyx_get_best_slice_order(__Pyx_memviewslice *__pyx_v_mslice, int __pyx_v_ndim) {
- int __pyx_v_i;
- Py_ssize_t __pyx_v_c_stride;
- Py_ssize_t __pyx_v_f_stride;
- char __pyx_r;
- int __pyx_t_1;
- int __pyx_t_2;
- int __pyx_t_3;
- int __pyx_t_4;
-
- /* "View.MemoryView":1121
- * """
- * cdef int i
- * cdef Py_ssize_t c_stride = 0 # <<<<<<<<<<<<<<
- * cdef Py_ssize_t f_stride = 0
- *
- */
- __pyx_v_c_stride = 0;
-
- /* "View.MemoryView":1122
- * cdef int i
- * cdef Py_ssize_t c_stride = 0
- * cdef Py_ssize_t f_stride = 0 # <<<<<<<<<<<<<<
- *
- * for i in range(ndim - 1, -1, -1):
- */
- __pyx_v_f_stride = 0;
-
- /* "View.MemoryView":1124
- * cdef Py_ssize_t f_stride = 0
- *
- * for i in range(ndim - 1, -1, -1): # <<<<<<<<<<<<<<
- * if mslice.shape[i] > 1:
- * c_stride = mslice.strides[i]
- */
- for (__pyx_t_1 = (__pyx_v_ndim - 1); __pyx_t_1 > -1; __pyx_t_1-=1) {
- __pyx_v_i = __pyx_t_1;
-
- /* "View.MemoryView":1125
- *
- * for i in range(ndim - 1, -1, -1):
- * if mslice.shape[i] > 1: # <<<<<<<<<<<<<<
- * c_stride = mslice.strides[i]
- * break
- */
- __pyx_t_2 = (((__pyx_v_mslice->shape[__pyx_v_i]) > 1) != 0);
- if (__pyx_t_2) {
-
- /* "View.MemoryView":1126
- * for i in range(ndim - 1, -1, -1):
- * if mslice.shape[i] > 1:
- * c_stride = mslice.strides[i] # <<<<<<<<<<<<<<
- * break
- *
- */
- __pyx_v_c_stride = (__pyx_v_mslice->strides[__pyx_v_i]);
-
- /* "View.MemoryView":1127
- * if mslice.shape[i] > 1:
- * c_stride = mslice.strides[i]
- * break # <<<<<<<<<<<<<<
- *
- * for i in range(ndim):
- */
- goto __pyx_L4_break;
-
- /* "View.MemoryView":1125
- *
- * for i in range(ndim - 1, -1, -1):
- * if mslice.shape[i] > 1: # <<<<<<<<<<<<<<
- * c_stride = mslice.strides[i]
- * break
- */
- }
- }
- __pyx_L4_break:;
-
- /* "View.MemoryView":1129
- * break
- *
- * for i in range(ndim): # <<<<<<<<<<<<<<
- * if mslice.shape[i] > 1:
- * f_stride = mslice.strides[i]
- */
- __pyx_t_1 = __pyx_v_ndim;
- __pyx_t_3 = __pyx_t_1;
- for (__pyx_t_4 = 0; __pyx_t_4 < __pyx_t_3; __pyx_t_4+=1) {
- __pyx_v_i = __pyx_t_4;
-
- /* "View.MemoryView":1130
- *
- * for i in range(ndim):
- * if mslice.shape[i] > 1: # <<<<<<<<<<<<<<
- * f_stride = mslice.strides[i]
- * break
- */
- __pyx_t_2 = (((__pyx_v_mslice->shape[__pyx_v_i]) > 1) != 0);
- if (__pyx_t_2) {
-
- /* "View.MemoryView":1131
- * for i in range(ndim):
- * if mslice.shape[i] > 1:
- * f_stride = mslice.strides[i] # <<<<<<<<<<<<<<
- * break
- *
- */
- __pyx_v_f_stride = (__pyx_v_mslice->strides[__pyx_v_i]);
-
- /* "View.MemoryView":1132
- * if mslice.shape[i] > 1:
- * f_stride = mslice.strides[i]
- * break # <<<<<<<<<<<<<<
- *
- * if abs_py_ssize_t(c_stride) <= abs_py_ssize_t(f_stride):
- */
- goto __pyx_L7_break;
-
- /* "View.MemoryView":1130
- *
- * for i in range(ndim):
- * if mslice.shape[i] > 1: # <<<<<<<<<<<<<<
- * f_stride = mslice.strides[i]
- * break
- */
- }
- }
- __pyx_L7_break:;
-
- /* "View.MemoryView":1134
- * break
- *
- * if abs_py_ssize_t(c_stride) <= abs_py_ssize_t(f_stride): # <<<<<<<<<<<<<<
- * return 'C'
- * else:
- */
- __pyx_t_2 = ((abs_py_ssize_t(__pyx_v_c_stride) <= abs_py_ssize_t(__pyx_v_f_stride)) != 0);
- if (__pyx_t_2) {
-
- /* "View.MemoryView":1135
- *
- * if abs_py_ssize_t(c_stride) <= abs_py_ssize_t(f_stride):
- * return 'C' # <<<<<<<<<<<<<<
- * else:
- * return 'F'
- */
- __pyx_r = 'C';
- goto __pyx_L0;
-
- /* "View.MemoryView":1134
- * break
- *
- * if abs_py_ssize_t(c_stride) <= abs_py_ssize_t(f_stride): # <<<<<<<<<<<<<<
- * return 'C'
- * else:
- */
- }
-
- /* "View.MemoryView":1137
- * return 'C'
- * else:
- * return 'F' # <<<<<<<<<<<<<<
- *
- * @cython.cdivision(True)
- */
- /*else*/ {
- __pyx_r = 'F';
- goto __pyx_L0;
- }
-
- /* "View.MemoryView":1116
- *
- * @cname('__pyx_get_best_slice_order')
- * cdef char get_best_order(__Pyx_memviewslice *mslice, int ndim) nogil: # <<<<<<<<<<<<<<
- * """
- * Figure out the best memory access order for a given slice.
- */
-
- /* function exit code */
- __pyx_L0:;
- return __pyx_r;
-}
-
-/* "View.MemoryView":1140
- *
- * @cython.cdivision(True)
- * cdef void _copy_strided_to_strided(char *src_data, Py_ssize_t *src_strides, # <<<<<<<<<<<<<<
- * char *dst_data, Py_ssize_t *dst_strides,
- * Py_ssize_t *src_shape, Py_ssize_t *dst_shape,
- */
-
-static void _copy_strided_to_strided(char *__pyx_v_src_data, Py_ssize_t *__pyx_v_src_strides, char *__pyx_v_dst_data, Py_ssize_t *__pyx_v_dst_strides, Py_ssize_t *__pyx_v_src_shape, Py_ssize_t *__pyx_v_dst_shape, int __pyx_v_ndim, size_t __pyx_v_itemsize) {
- CYTHON_UNUSED Py_ssize_t __pyx_v_i;
- CYTHON_UNUSED Py_ssize_t __pyx_v_src_extent;
- Py_ssize_t __pyx_v_dst_extent;
- Py_ssize_t __pyx_v_src_stride;
- Py_ssize_t __pyx_v_dst_stride;
- int __pyx_t_1;
- int __pyx_t_2;
- int __pyx_t_3;
- Py_ssize_t __pyx_t_4;
- Py_ssize_t __pyx_t_5;
- Py_ssize_t __pyx_t_6;
-
- /* "View.MemoryView":1147
- *
- * cdef Py_ssize_t i
- * cdef Py_ssize_t src_extent = src_shape[0] # <<<<<<<<<<<<<<
- * cdef Py_ssize_t dst_extent = dst_shape[0]
- * cdef Py_ssize_t src_stride = src_strides[0]
- */
- __pyx_v_src_extent = (__pyx_v_src_shape[0]);
-
- /* "View.MemoryView":1148
- * cdef Py_ssize_t i
- * cdef Py_ssize_t src_extent = src_shape[0]
- * cdef Py_ssize_t dst_extent = dst_shape[0] # <<<<<<<<<<<<<<
- * cdef Py_ssize_t src_stride = src_strides[0]
- * cdef Py_ssize_t dst_stride = dst_strides[0]
- */
- __pyx_v_dst_extent = (__pyx_v_dst_shape[0]);
-
- /* "View.MemoryView":1149
- * cdef Py_ssize_t src_extent = src_shape[0]
- * cdef Py_ssize_t dst_extent = dst_shape[0]
- * cdef Py_ssize_t src_stride = src_strides[0] # <<<<<<<<<<<<<<
- * cdef Py_ssize_t dst_stride = dst_strides[0]
- *
- */
- __pyx_v_src_stride = (__pyx_v_src_strides[0]);
-
- /* "View.MemoryView":1150
- * cdef Py_ssize_t dst_extent = dst_shape[0]
- * cdef Py_ssize_t src_stride = src_strides[0]
- * cdef Py_ssize_t dst_stride = dst_strides[0] # <<<<<<<<<<<<<<
- *
- * if ndim == 1:
- */
- __pyx_v_dst_stride = (__pyx_v_dst_strides[0]);
-
- /* "View.MemoryView":1152
- * cdef Py_ssize_t dst_stride = dst_strides[0]
- *
- * if ndim == 1: # <<<<<<<<<<<<<<
- * if (src_stride > 0 and dst_stride > 0 and
- * src_stride == itemsize == dst_stride):
- */
- __pyx_t_1 = ((__pyx_v_ndim == 1) != 0);
- if (__pyx_t_1) {
-
- /* "View.MemoryView":1153
- *
- * if ndim == 1:
- * if (src_stride > 0 and dst_stride > 0 and # <<<<<<<<<<<<<<
- * src_stride == itemsize == dst_stride):
- * memcpy(dst_data, src_data, itemsize * dst_extent)
- */
- __pyx_t_2 = ((__pyx_v_src_stride > 0) != 0);
- if (__pyx_t_2) {
- } else {
- __pyx_t_1 = __pyx_t_2;
- goto __pyx_L5_bool_binop_done;
- }
- __pyx_t_2 = ((__pyx_v_dst_stride > 0) != 0);
- if (__pyx_t_2) {
- } else {
- __pyx_t_1 = __pyx_t_2;
- goto __pyx_L5_bool_binop_done;
- }
-
- /* "View.MemoryView":1154
- * if ndim == 1:
- * if (src_stride > 0 and dst_stride > 0 and
- * src_stride == itemsize == dst_stride): # <<<<<<<<<<<<<<
- * memcpy(dst_data, src_data, itemsize * dst_extent)
- * else:
- */
- __pyx_t_2 = (((size_t)__pyx_v_src_stride) == __pyx_v_itemsize);
- if (__pyx_t_2) {
- __pyx_t_2 = (__pyx_v_itemsize == ((size_t)__pyx_v_dst_stride));
- }
- __pyx_t_3 = (__pyx_t_2 != 0);
- __pyx_t_1 = __pyx_t_3;
- __pyx_L5_bool_binop_done:;
-
- /* "View.MemoryView":1153
- *
- * if ndim == 1:
- * if (src_stride > 0 and dst_stride > 0 and # <<<<<<<<<<<<<<
- * src_stride == itemsize == dst_stride):
- * memcpy(dst_data, src_data, itemsize * dst_extent)
- */
- if (__pyx_t_1) {
-
- /* "View.MemoryView":1155
- * if (src_stride > 0 and dst_stride > 0 and
- * src_stride == itemsize == dst_stride):
- * memcpy(dst_data, src_data, itemsize * dst_extent) # <<<<<<<<<<<<<<
- * else:
- * for i in range(dst_extent):
- */
- (void)(memcpy(__pyx_v_dst_data, __pyx_v_src_data, (__pyx_v_itemsize * __pyx_v_dst_extent)));
-
- /* "View.MemoryView":1153
- *
- * if ndim == 1:
- * if (src_stride > 0 and dst_stride > 0 and # <<<<<<<<<<<<<<
- * src_stride == itemsize == dst_stride):
- * memcpy(dst_data, src_data, itemsize * dst_extent)
- */
- goto __pyx_L4;
- }
-
- /* "View.MemoryView":1157
- * memcpy(dst_data, src_data, itemsize * dst_extent)
- * else:
- * for i in range(dst_extent): # <<<<<<<<<<<<<<
- * memcpy(dst_data, src_data, itemsize)
- * src_data += src_stride
- */
- /*else*/ {
- __pyx_t_4 = __pyx_v_dst_extent;
- __pyx_t_5 = __pyx_t_4;
- for (__pyx_t_6 = 0; __pyx_t_6 < __pyx_t_5; __pyx_t_6+=1) {
- __pyx_v_i = __pyx_t_6;
-
- /* "View.MemoryView":1158
- * else:
- * for i in range(dst_extent):
- * memcpy(dst_data, src_data, itemsize) # <<<<<<<<<<<<<<
- * src_data += src_stride
- * dst_data += dst_stride
- */
- (void)(memcpy(__pyx_v_dst_data, __pyx_v_src_data, __pyx_v_itemsize));
-
- /* "View.MemoryView":1159
- * for i in range(dst_extent):
- * memcpy(dst_data, src_data, itemsize)
- * src_data += src_stride # <<<<<<<<<<<<<<
- * dst_data += dst_stride
- * else:
- */
- __pyx_v_src_data = (__pyx_v_src_data + __pyx_v_src_stride);
-
- /* "View.MemoryView":1160
- * memcpy(dst_data, src_data, itemsize)
- * src_data += src_stride
- * dst_data += dst_stride # <<<<<<<<<<<<<<
- * else:
- * for i in range(dst_extent):
- */
- __pyx_v_dst_data = (__pyx_v_dst_data + __pyx_v_dst_stride);
- }
- }
- __pyx_L4:;
-
- /* "View.MemoryView":1152
- * cdef Py_ssize_t dst_stride = dst_strides[0]
- *
- * if ndim == 1: # <<<<<<<<<<<<<<
- * if (src_stride > 0 and dst_stride > 0 and
- * src_stride == itemsize == dst_stride):
- */
- goto __pyx_L3;
- }
-
- /* "View.MemoryView":1162
- * dst_data += dst_stride
- * else:
- * for i in range(dst_extent): # <<<<<<<<<<<<<<
- * _copy_strided_to_strided(src_data, src_strides + 1,
- * dst_data, dst_strides + 1,
- */
- /*else*/ {
- __pyx_t_4 = __pyx_v_dst_extent;
- __pyx_t_5 = __pyx_t_4;
- for (__pyx_t_6 = 0; __pyx_t_6 < __pyx_t_5; __pyx_t_6+=1) {
- __pyx_v_i = __pyx_t_6;
-
- /* "View.MemoryView":1163
- * else:
- * for i in range(dst_extent):
- * _copy_strided_to_strided(src_data, src_strides + 1, # <<<<<<<<<<<<<<
- * dst_data, dst_strides + 1,
- * src_shape + 1, dst_shape + 1,
- */
- _copy_strided_to_strided(__pyx_v_src_data, (__pyx_v_src_strides + 1), __pyx_v_dst_data, (__pyx_v_dst_strides + 1), (__pyx_v_src_shape + 1), (__pyx_v_dst_shape + 1), (__pyx_v_ndim - 1), __pyx_v_itemsize);
-
- /* "View.MemoryView":1167
- * src_shape + 1, dst_shape + 1,
- * ndim - 1, itemsize)
- * src_data += src_stride # <<<<<<<<<<<<<<
- * dst_data += dst_stride
- *
- */
- __pyx_v_src_data = (__pyx_v_src_data + __pyx_v_src_stride);
-
- /* "View.MemoryView":1168
- * ndim - 1, itemsize)
- * src_data += src_stride
- * dst_data += dst_stride # <<<<<<<<<<<<<<
- *
- * cdef void copy_strided_to_strided(__Pyx_memviewslice *src,
- */
- __pyx_v_dst_data = (__pyx_v_dst_data + __pyx_v_dst_stride);
- }
- }
- __pyx_L3:;
-
- /* "View.MemoryView":1140
- *
- * @cython.cdivision(True)
- * cdef void _copy_strided_to_strided(char *src_data, Py_ssize_t *src_strides, # <<<<<<<<<<<<<<
- * char *dst_data, Py_ssize_t *dst_strides,
- * Py_ssize_t *src_shape, Py_ssize_t *dst_shape,
- */
-
- /* function exit code */
-}
-
-/* "View.MemoryView":1170
- * dst_data += dst_stride
- *
- * cdef void copy_strided_to_strided(__Pyx_memviewslice *src, # <<<<<<<<<<<<<<
- * __Pyx_memviewslice *dst,
- * int ndim, size_t itemsize) nogil:
- */
-
-static void copy_strided_to_strided(__Pyx_memviewslice *__pyx_v_src, __Pyx_memviewslice *__pyx_v_dst, int __pyx_v_ndim, size_t __pyx_v_itemsize) {
-
- /* "View.MemoryView":1173
- * __Pyx_memviewslice *dst,
- * int ndim, size_t itemsize) nogil:
- * _copy_strided_to_strided(src.data, src.strides, dst.data, dst.strides, # <<<<<<<<<<<<<<
- * src.shape, dst.shape, ndim, itemsize)
- *
- */
- _copy_strided_to_strided(__pyx_v_src->data, __pyx_v_src->strides, __pyx_v_dst->data, __pyx_v_dst->strides, __pyx_v_src->shape, __pyx_v_dst->shape, __pyx_v_ndim, __pyx_v_itemsize);
-
- /* "View.MemoryView":1170
- * dst_data += dst_stride
- *
- * cdef void copy_strided_to_strided(__Pyx_memviewslice *src, # <<<<<<<<<<<<<<
- * __Pyx_memviewslice *dst,
- * int ndim, size_t itemsize) nogil:
- */
-
- /* function exit code */
-}
-
-/* "View.MemoryView":1177
- *
- * @cname('__pyx_memoryview_slice_get_size')
- * cdef Py_ssize_t slice_get_size(__Pyx_memviewslice *src, int ndim) nogil: # <<<<<<<<<<<<<<
- * "Return the size of the memory occupied by the slice in number of bytes"
- * cdef Py_ssize_t shape, size = src.memview.view.itemsize
- */
-
-static Py_ssize_t __pyx_memoryview_slice_get_size(__Pyx_memviewslice *__pyx_v_src, int __pyx_v_ndim) {
- Py_ssize_t __pyx_v_shape;
- Py_ssize_t __pyx_v_size;
- Py_ssize_t __pyx_r;
- Py_ssize_t __pyx_t_1;
- Py_ssize_t *__pyx_t_2;
- Py_ssize_t *__pyx_t_3;
- Py_ssize_t *__pyx_t_4;
-
- /* "View.MemoryView":1179
- * cdef Py_ssize_t slice_get_size(__Pyx_memviewslice *src, int ndim) nogil:
- * "Return the size of the memory occupied by the slice in number of bytes"
- * cdef Py_ssize_t shape, size = src.memview.view.itemsize # <<<<<<<<<<<<<<
- *
- * for shape in src.shape[:ndim]:
- */
- __pyx_t_1 = __pyx_v_src->memview->view.itemsize;
- __pyx_v_size = __pyx_t_1;
-
- /* "View.MemoryView":1181
- * cdef Py_ssize_t shape, size = src.memview.view.itemsize
- *
- * for shape in src.shape[:ndim]: # <<<<<<<<<<<<<<
- * size *= shape
- *
- */
- __pyx_t_3 = (__pyx_v_src->shape + __pyx_v_ndim);
- for (__pyx_t_4 = __pyx_v_src->shape; __pyx_t_4 < __pyx_t_3; __pyx_t_4++) {
- __pyx_t_2 = __pyx_t_4;
- __pyx_v_shape = (__pyx_t_2[0]);
-
- /* "View.MemoryView":1182
- *
- * for shape in src.shape[:ndim]:
- * size *= shape # <<<<<<<<<<<<<<
- *
- * return size
- */
- __pyx_v_size = (__pyx_v_size * __pyx_v_shape);
- }
-
- /* "View.MemoryView":1184
- * size *= shape
- *
- * return size # <<<<<<<<<<<<<<
- *
- * @cname('__pyx_fill_contig_strides_array')
- */
- __pyx_r = __pyx_v_size;
- goto __pyx_L0;
-
- /* "View.MemoryView":1177
- *
- * @cname('__pyx_memoryview_slice_get_size')
- * cdef Py_ssize_t slice_get_size(__Pyx_memviewslice *src, int ndim) nogil: # <<<<<<<<<<<<<<
- * "Return the size of the memory occupied by the slice in number of bytes"
- * cdef Py_ssize_t shape, size = src.memview.view.itemsize
- */
-
- /* function exit code */
- __pyx_L0:;
- return __pyx_r;
-}
-
-/* "View.MemoryView":1187
- *
- * @cname('__pyx_fill_contig_strides_array')
- * cdef Py_ssize_t fill_contig_strides_array( # <<<<<<<<<<<<<<
- * Py_ssize_t *shape, Py_ssize_t *strides, Py_ssize_t stride,
- * int ndim, char order) nogil:
- */
-
-static Py_ssize_t __pyx_fill_contig_strides_array(Py_ssize_t *__pyx_v_shape, Py_ssize_t *__pyx_v_strides, Py_ssize_t __pyx_v_stride, int __pyx_v_ndim, char __pyx_v_order) {
- int __pyx_v_idx;
- Py_ssize_t __pyx_r;
- int __pyx_t_1;
- int __pyx_t_2;
- int __pyx_t_3;
- int __pyx_t_4;
-
- /* "View.MemoryView":1196
- * cdef int idx
- *
- * if order == 'F': # <<<<<<<<<<<<<<
- * for idx in range(ndim):
- * strides[idx] = stride
- */
- __pyx_t_1 = ((__pyx_v_order == 'F') != 0);
- if (__pyx_t_1) {
-
- /* "View.MemoryView":1197
- *
- * if order == 'F':
- * for idx in range(ndim): # <<<<<<<<<<<<<<
- * strides[idx] = stride
- * stride *= shape[idx]
- */
- __pyx_t_2 = __pyx_v_ndim;
- __pyx_t_3 = __pyx_t_2;
- for (__pyx_t_4 = 0; __pyx_t_4 < __pyx_t_3; __pyx_t_4+=1) {
- __pyx_v_idx = __pyx_t_4;
-
- /* "View.MemoryView":1198
- * if order == 'F':
- * for idx in range(ndim):
- * strides[idx] = stride # <<<<<<<<<<<<<<
- * stride *= shape[idx]
- * else:
- */
- (__pyx_v_strides[__pyx_v_idx]) = __pyx_v_stride;
-
- /* "View.MemoryView":1199
- * for idx in range(ndim):
- * strides[idx] = stride
- * stride *= shape[idx] # <<<<<<<<<<<<<<
- * else:
- * for idx in range(ndim - 1, -1, -1):
- */
- __pyx_v_stride = (__pyx_v_stride * (__pyx_v_shape[__pyx_v_idx]));
- }
-
- /* "View.MemoryView":1196
- * cdef int idx
- *
- * if order == 'F': # <<<<<<<<<<<<<<
- * for idx in range(ndim):
- * strides[idx] = stride
- */
- goto __pyx_L3;
- }
-
- /* "View.MemoryView":1201
- * stride *= shape[idx]
- * else:
- * for idx in range(ndim - 1, -1, -1): # <<<<<<<<<<<<<<
- * strides[idx] = stride
- * stride *= shape[idx]
- */
- /*else*/ {
- for (__pyx_t_2 = (__pyx_v_ndim - 1); __pyx_t_2 > -1; __pyx_t_2-=1) {
- __pyx_v_idx = __pyx_t_2;
-
- /* "View.MemoryView":1202
- * else:
- * for idx in range(ndim - 1, -1, -1):
- * strides[idx] = stride # <<<<<<<<<<<<<<
- * stride *= shape[idx]
- *
- */
- (__pyx_v_strides[__pyx_v_idx]) = __pyx_v_stride;
-
- /* "View.MemoryView":1203
- * for idx in range(ndim - 1, -1, -1):
- * strides[idx] = stride
- * stride *= shape[idx] # <<<<<<<<<<<<<<
- *
- * return stride
- */
- __pyx_v_stride = (__pyx_v_stride * (__pyx_v_shape[__pyx_v_idx]));
- }
- }
- __pyx_L3:;
-
- /* "View.MemoryView":1205
- * stride *= shape[idx]
- *
- * return stride # <<<<<<<<<<<<<<
- *
- * @cname('__pyx_memoryview_copy_data_to_temp')
- */
- __pyx_r = __pyx_v_stride;
- goto __pyx_L0;
-
- /* "View.MemoryView":1187
- *
- * @cname('__pyx_fill_contig_strides_array')
- * cdef Py_ssize_t fill_contig_strides_array( # <<<<<<<<<<<<<<
- * Py_ssize_t *shape, Py_ssize_t *strides, Py_ssize_t stride,
- * int ndim, char order) nogil:
- */
-
- /* function exit code */
- __pyx_L0:;
- return __pyx_r;
-}
-
-/* "View.MemoryView":1208
- *
- * @cname('__pyx_memoryview_copy_data_to_temp')
- * cdef void *copy_data_to_temp(__Pyx_memviewslice *src, # <<<<<<<<<<<<<<
- * __Pyx_memviewslice *tmpslice,
- * char order,
- */
-
-static void *__pyx_memoryview_copy_data_to_temp(__Pyx_memviewslice *__pyx_v_src, __Pyx_memviewslice *__pyx_v_tmpslice, char __pyx_v_order, int __pyx_v_ndim) {
- int __pyx_v_i;
- void *__pyx_v_result;
- size_t __pyx_v_itemsize;
- size_t __pyx_v_size;
- void *__pyx_r;
- Py_ssize_t __pyx_t_1;
- int __pyx_t_2;
- int __pyx_t_3;
- struct __pyx_memoryview_obj *__pyx_t_4;
- int __pyx_t_5;
- int __pyx_t_6;
- int __pyx_lineno = 0;
- const char *__pyx_filename = NULL;
- int __pyx_clineno = 0;
-
- /* "View.MemoryView":1219
- * cdef void *result
- *
- * cdef size_t itemsize = src.memview.view.itemsize # <<<<<<<<<<<<<<
- * cdef size_t size = slice_get_size(src, ndim)
- *
- */
- __pyx_t_1 = __pyx_v_src->memview->view.itemsize;
- __pyx_v_itemsize = __pyx_t_1;
-
- /* "View.MemoryView":1220
- *
- * cdef size_t itemsize = src.memview.view.itemsize
- * cdef size_t size = slice_get_size(src, ndim) # <<<<<<<<<<<<<<
- *
- * result = malloc(size)
- */
- __pyx_v_size = __pyx_memoryview_slice_get_size(__pyx_v_src, __pyx_v_ndim);
-
- /* "View.MemoryView":1222
- * cdef size_t size = slice_get_size(src, ndim)
- *
- * result = malloc(size) # <<<<<<<<<<<<<<
- * if not result:
- * _err(MemoryError, NULL)
- */
- __pyx_v_result = malloc(__pyx_v_size);
-
- /* "View.MemoryView":1223
- *
- * result = malloc(size)
- * if not result: # <<<<<<<<<<<<<<
- * _err(MemoryError, NULL)
- *
- */
- __pyx_t_2 = ((!(__pyx_v_result != 0)) != 0);
- if (__pyx_t_2) {
-
- /* "View.MemoryView":1224
- * result = malloc(size)
- * if not result:
- * _err(MemoryError, NULL) # <<<<<<<<<<<<<<
- *
- *
- */
- __pyx_t_3 = __pyx_memoryview_err(__pyx_builtin_MemoryError, NULL); if (unlikely(__pyx_t_3 == ((int)-1))) __PYX_ERR(1, 1224, __pyx_L1_error)
-
- /* "View.MemoryView":1223
- *
- * result = malloc(size)
- * if not result: # <<<<<<<<<<<<<<
- * _err(MemoryError, NULL)
- *
- */
- }
-
- /* "View.MemoryView":1227
- *
- *
- * tmpslice.data = result # <<<<<<<<<<<<<<
- * tmpslice.memview = src.memview
- * for i in range(ndim):
- */
- __pyx_v_tmpslice->data = ((char *)__pyx_v_result);
-
- /* "View.MemoryView":1228
- *
- * tmpslice.data = result
- * tmpslice.memview = src.memview # <<<<<<<<<<<<<<
- * for i in range(ndim):
- * tmpslice.shape[i] = src.shape[i]
- */
- __pyx_t_4 = __pyx_v_src->memview;
- __pyx_v_tmpslice->memview = __pyx_t_4;
-
- /* "View.MemoryView":1229
- * tmpslice.data = result
- * tmpslice.memview = src.memview
- * for i in range(ndim): # <<<<<<<<<<<<<<
- * tmpslice.shape[i] = src.shape[i]
- * tmpslice.suboffsets[i] = -1
- */
- __pyx_t_3 = __pyx_v_ndim;
- __pyx_t_5 = __pyx_t_3;
- for (__pyx_t_6 = 0; __pyx_t_6 < __pyx_t_5; __pyx_t_6+=1) {
- __pyx_v_i = __pyx_t_6;
-
- /* "View.MemoryView":1230
- * tmpslice.memview = src.memview
- * for i in range(ndim):
- * tmpslice.shape[i] = src.shape[i] # <<<<<<<<<<<<<<
- * tmpslice.suboffsets[i] = -1
- *
- */
- (__pyx_v_tmpslice->shape[__pyx_v_i]) = (__pyx_v_src->shape[__pyx_v_i]);
-
- /* "View.MemoryView":1231
- * for i in range(ndim):
- * tmpslice.shape[i] = src.shape[i]
- * tmpslice.suboffsets[i] = -1 # <<<<<<<<<<<<<<
- *
- * fill_contig_strides_array(&tmpslice.shape[0], &tmpslice.strides[0], itemsize,
- */
- (__pyx_v_tmpslice->suboffsets[__pyx_v_i]) = -1L;
- }
-
- /* "View.MemoryView":1233
- * tmpslice.suboffsets[i] = -1
- *
- * fill_contig_strides_array(&tmpslice.shape[0], &tmpslice.strides[0], itemsize, # <<<<<<<<<<<<<<
- * ndim, order)
- *
- */
- (void)(__pyx_fill_contig_strides_array((&(__pyx_v_tmpslice->shape[0])), (&(__pyx_v_tmpslice->strides[0])), __pyx_v_itemsize, __pyx_v_ndim, __pyx_v_order));
-
- /* "View.MemoryView":1237
- *
- *
- * for i in range(ndim): # <<<<<<<<<<<<<<
- * if tmpslice.shape[i] == 1:
- * tmpslice.strides[i] = 0
- */
- __pyx_t_3 = __pyx_v_ndim;
- __pyx_t_5 = __pyx_t_3;
- for (__pyx_t_6 = 0; __pyx_t_6 < __pyx_t_5; __pyx_t_6+=1) {
- __pyx_v_i = __pyx_t_6;
-
- /* "View.MemoryView":1238
- *
- * for i in range(ndim):
- * if tmpslice.shape[i] == 1: # <<<<<<<<<<<<<<
- * tmpslice.strides[i] = 0
- *
- */
- __pyx_t_2 = (((__pyx_v_tmpslice->shape[__pyx_v_i]) == 1) != 0);
- if (__pyx_t_2) {
-
- /* "View.MemoryView":1239
- * for i in range(ndim):
- * if tmpslice.shape[i] == 1:
- * tmpslice.strides[i] = 0 # <<<<<<<<<<<<<<
- *
- * if slice_is_contig(src[0], order, ndim):
- */
- (__pyx_v_tmpslice->strides[__pyx_v_i]) = 0;
-
- /* "View.MemoryView":1238
- *
- * for i in range(ndim):
- * if tmpslice.shape[i] == 1: # <<<<<<<<<<<<<<
- * tmpslice.strides[i] = 0
- *
- */
- }
- }
-
- /* "View.MemoryView":1241
- * tmpslice.strides[i] = 0
- *
- * if slice_is_contig(src[0], order, ndim): # <<<<<<<<<<<<<<
- * memcpy(result, src.data, size)
- * else:
- */
- __pyx_t_2 = (__pyx_memviewslice_is_contig((__pyx_v_src[0]), __pyx_v_order, __pyx_v_ndim) != 0);
- if (__pyx_t_2) {
-
- /* "View.MemoryView":1242
- *
- * if slice_is_contig(src[0], order, ndim):
- * memcpy(result, src.data, size) # <<<<<<<<<<<<<<
- * else:
- * copy_strided_to_strided(src, tmpslice, ndim, itemsize)
- */
- (void)(memcpy(__pyx_v_result, __pyx_v_src->data, __pyx_v_size));
-
- /* "View.MemoryView":1241
- * tmpslice.strides[i] = 0
- *
- * if slice_is_contig(src[0], order, ndim): # <<<<<<<<<<<<<<
- * memcpy(result, src.data, size)
- * else:
- */
- goto __pyx_L9;
- }
-
- /* "View.MemoryView":1244
- * memcpy(result, src.data, size)
- * else:
- * copy_strided_to_strided(src, tmpslice, ndim, itemsize) # <<<<<<<<<<<<<<
- *
- * return result
- */
- /*else*/ {
- copy_strided_to_strided(__pyx_v_src, __pyx_v_tmpslice, __pyx_v_ndim, __pyx_v_itemsize);
- }
- __pyx_L9:;
-
- /* "View.MemoryView":1246
- * copy_strided_to_strided(src, tmpslice, ndim, itemsize)
- *
- * return result # <<<<<<<<<<<<<<
- *
- *
- */
- __pyx_r = __pyx_v_result;
- goto __pyx_L0;
-
- /* "View.MemoryView":1208
- *
- * @cname('__pyx_memoryview_copy_data_to_temp')
- * cdef void *copy_data_to_temp(__Pyx_memviewslice *src, # <<<<<<<<<<<<<<
- * __Pyx_memviewslice *tmpslice,
- * char order,
- */
-
- /* function exit code */
- __pyx_L1_error:;
- {
- #ifdef WITH_THREAD
- PyGILState_STATE __pyx_gilstate_save = __Pyx_PyGILState_Ensure();
- #endif
- __Pyx_AddTraceback("View.MemoryView.copy_data_to_temp", __pyx_clineno, __pyx_lineno, __pyx_filename);
- #ifdef WITH_THREAD
- __Pyx_PyGILState_Release(__pyx_gilstate_save);
- #endif
- }
- __pyx_r = NULL;
- __pyx_L0:;
- return __pyx_r;
-}
-
-/* "View.MemoryView":1251
- *
- * @cname('__pyx_memoryview_err_extents')
- * cdef int _err_extents(int i, Py_ssize_t extent1, # <<<<<<<<<<<<<<
- * Py_ssize_t extent2) except -1 with gil:
- * raise ValueError("got differing extents in dimension %d (got %d and %d)" %
- */
-
-static int __pyx_memoryview_err_extents(int __pyx_v_i, Py_ssize_t __pyx_v_extent1, Py_ssize_t __pyx_v_extent2) {
- int __pyx_r;
- __Pyx_RefNannyDeclarations
- PyObject *__pyx_t_1 = NULL;
- PyObject *__pyx_t_2 = NULL;
- PyObject *__pyx_t_3 = NULL;
- PyObject *__pyx_t_4 = NULL;
- int __pyx_lineno = 0;
- const char *__pyx_filename = NULL;
- int __pyx_clineno = 0;
- #ifdef WITH_THREAD
- PyGILState_STATE __pyx_gilstate_save = __Pyx_PyGILState_Ensure();
- #endif
- __Pyx_RefNannySetupContext("_err_extents", 0);
-
- /* "View.MemoryView":1254
- * Py_ssize_t extent2) except -1 with gil:
- * raise ValueError("got differing extents in dimension %d (got %d and %d)" %
- * (i, extent1, extent2)) # <<<<<<<<<<<<<<
- *
- * @cname('__pyx_memoryview_err_dim')
- */
- __pyx_t_1 = __Pyx_PyInt_From_int(__pyx_v_i); if (unlikely(!__pyx_t_1)) __PYX_ERR(1, 1254, __pyx_L1_error)
- __Pyx_GOTREF(__pyx_t_1);
- __pyx_t_2 = PyInt_FromSsize_t(__pyx_v_extent1); if (unlikely(!__pyx_t_2)) __PYX_ERR(1, 1254, __pyx_L1_error)
- __Pyx_GOTREF(__pyx_t_2);
- __pyx_t_3 = PyInt_FromSsize_t(__pyx_v_extent2); if (unlikely(!__pyx_t_3)) __PYX_ERR(1, 1254, __pyx_L1_error)
- __Pyx_GOTREF(__pyx_t_3);
- __pyx_t_4 = PyTuple_New(3); if (unlikely(!__pyx_t_4)) __PYX_ERR(1, 1254, __pyx_L1_error)
- __Pyx_GOTREF(__pyx_t_4);
- __Pyx_GIVEREF(__pyx_t_1);
- PyTuple_SET_ITEM(__pyx_t_4, 0, __pyx_t_1);
- __Pyx_GIVEREF(__pyx_t_2);
- PyTuple_SET_ITEM(__pyx_t_4, 1, __pyx_t_2);
- __Pyx_GIVEREF(__pyx_t_3);
- PyTuple_SET_ITEM(__pyx_t_4, 2, __pyx_t_3);
- __pyx_t_1 = 0;
- __pyx_t_2 = 0;
- __pyx_t_3 = 0;
-
- /* "View.MemoryView":1253
- * cdef int _err_extents(int i, Py_ssize_t extent1,
- * Py_ssize_t extent2) except -1 with gil:
- * raise ValueError("got differing extents in dimension %d (got %d and %d)" % # <<<<<<<<<<<<<<
- * (i, extent1, extent2))
- *
- */
- __pyx_t_3 = __Pyx_PyString_Format(__pyx_kp_s_got_differing_extents_in_dimensi, __pyx_t_4); if (unlikely(!__pyx_t_3)) __PYX_ERR(1, 1253, __pyx_L1_error)
- __Pyx_GOTREF(__pyx_t_3);
- __Pyx_DECREF(__pyx_t_4); __pyx_t_4 = 0;
- __pyx_t_4 = __Pyx_PyObject_CallOneArg(__pyx_builtin_ValueError, __pyx_t_3); if (unlikely(!__pyx_t_4)) __PYX_ERR(1, 1253, __pyx_L1_error)
- __Pyx_GOTREF(__pyx_t_4);
- __Pyx_DECREF(__pyx_t_3); __pyx_t_3 = 0;
- __Pyx_Raise(__pyx_t_4, 0, 0, 0);
- __Pyx_DECREF(__pyx_t_4); __pyx_t_4 = 0;
- __PYX_ERR(1, 1253, __pyx_L1_error)
-
- /* "View.MemoryView":1251
- *
- * @cname('__pyx_memoryview_err_extents')
- * cdef int _err_extents(int i, Py_ssize_t extent1, # <<<<<<<<<<<<<<
- * Py_ssize_t extent2) except -1 with gil:
- * raise ValueError("got differing extents in dimension %d (got %d and %d)" %
- */
-
- /* function exit code */
- __pyx_L1_error:;
- __Pyx_XDECREF(__pyx_t_1);
- __Pyx_XDECREF(__pyx_t_2);
- __Pyx_XDECREF(__pyx_t_3);
- __Pyx_XDECREF(__pyx_t_4);
- __Pyx_AddTraceback("View.MemoryView._err_extents", __pyx_clineno, __pyx_lineno, __pyx_filename);
- __pyx_r = -1;
- __Pyx_RefNannyFinishContext();
- #ifdef WITH_THREAD
- __Pyx_PyGILState_Release(__pyx_gilstate_save);
- #endif
- return __pyx_r;
-}
-
-/* "View.MemoryView":1257
- *
- * @cname('__pyx_memoryview_err_dim')
- * cdef int _err_dim(object error, char *msg, int dim) except -1 with gil: # <<<<<<<<<<<<<<
- * raise error(msg.decode('ascii') % dim)
- *
- */
-
-static int __pyx_memoryview_err_dim(PyObject *__pyx_v_error, char *__pyx_v_msg, int __pyx_v_dim) {
- int __pyx_r;
- __Pyx_RefNannyDeclarations
- PyObject *__pyx_t_1 = NULL;
- PyObject *__pyx_t_2 = NULL;
- PyObject *__pyx_t_3 = NULL;
- PyObject *__pyx_t_4 = NULL;
- int __pyx_lineno = 0;
- const char *__pyx_filename = NULL;
- int __pyx_clineno = 0;
- #ifdef WITH_THREAD
- PyGILState_STATE __pyx_gilstate_save = __Pyx_PyGILState_Ensure();
- #endif
- __Pyx_RefNannySetupContext("_err_dim", 0);
- __Pyx_INCREF(__pyx_v_error);
-
- /* "View.MemoryView":1258
- * @cname('__pyx_memoryview_err_dim')
- * cdef int _err_dim(object error, char *msg, int dim) except -1 with gil:
- * raise error(msg.decode('ascii') % dim) # <<<<<<<<<<<<<<
- *
- * @cname('__pyx_memoryview_err')
- */
- __pyx_t_2 = __Pyx_decode_c_string(__pyx_v_msg, 0, strlen(__pyx_v_msg), NULL, NULL, PyUnicode_DecodeASCII); if (unlikely(!__pyx_t_2)) __PYX_ERR(1, 1258, __pyx_L1_error)
- __Pyx_GOTREF(__pyx_t_2);
- __pyx_t_3 = __Pyx_PyInt_From_int(__pyx_v_dim); if (unlikely(!__pyx_t_3)) __PYX_ERR(1, 1258, __pyx_L1_error)
- __Pyx_GOTREF(__pyx_t_3);
- __pyx_t_4 = PyUnicode_Format(__pyx_t_2, __pyx_t_3); if (unlikely(!__pyx_t_4)) __PYX_ERR(1, 1258, __pyx_L1_error)
- __Pyx_GOTREF(__pyx_t_4);
- __Pyx_DECREF(__pyx_t_2); __pyx_t_2 = 0;
- __Pyx_DECREF(__pyx_t_3); __pyx_t_3 = 0;
- __Pyx_INCREF(__pyx_v_error);
- __pyx_t_3 = __pyx_v_error; __pyx_t_2 = NULL;
- if (CYTHON_UNPACK_METHODS && unlikely(PyMethod_Check(__pyx_t_3))) {
- __pyx_t_2 = PyMethod_GET_SELF(__pyx_t_3);
- if (likely(__pyx_t_2)) {
- PyObject* function = PyMethod_GET_FUNCTION(__pyx_t_3);
- __Pyx_INCREF(__pyx_t_2);
- __Pyx_INCREF(function);
- __Pyx_DECREF_SET(__pyx_t_3, function);
- }
- }
- __pyx_t_1 = (__pyx_t_2) ? __Pyx_PyObject_Call2Args(__pyx_t_3, __pyx_t_2, __pyx_t_4) : __Pyx_PyObject_CallOneArg(__pyx_t_3, __pyx_t_4);
- __Pyx_XDECREF(__pyx_t_2); __pyx_t_2 = 0;
- __Pyx_DECREF(__pyx_t_4); __pyx_t_4 = 0;
- if (unlikely(!__pyx_t_1)) __PYX_ERR(1, 1258, __pyx_L1_error)
- __Pyx_GOTREF(__pyx_t_1);
- __Pyx_DECREF(__pyx_t_3); __pyx_t_3 = 0;
- __Pyx_Raise(__pyx_t_1, 0, 0, 0);
- __Pyx_DECREF(__pyx_t_1); __pyx_t_1 = 0;
- __PYX_ERR(1, 1258, __pyx_L1_error)
-
- /* "View.MemoryView":1257
- *
- * @cname('__pyx_memoryview_err_dim')
- * cdef int _err_dim(object error, char *msg, int dim) except -1 with gil: # <<<<<<<<<<<<<<
- * raise error(msg.decode('ascii') % dim)
- *
- */
-
- /* function exit code */
- __pyx_L1_error:;
- __Pyx_XDECREF(__pyx_t_1);
- __Pyx_XDECREF(__pyx_t_2);
- __Pyx_XDECREF(__pyx_t_3);
- __Pyx_XDECREF(__pyx_t_4);
- __Pyx_AddTraceback("View.MemoryView._err_dim", __pyx_clineno, __pyx_lineno, __pyx_filename);
- __pyx_r = -1;
- __Pyx_XDECREF(__pyx_v_error);
- __Pyx_RefNannyFinishContext();
- #ifdef WITH_THREAD
- __Pyx_PyGILState_Release(__pyx_gilstate_save);
- #endif
- return __pyx_r;
-}
-
-/* "View.MemoryView":1261
- *
- * @cname('__pyx_memoryview_err')
- * cdef int _err(object error, char *msg) except -1 with gil: # <<<<<<<<<<<<<<
- * if msg != NULL:
- * raise error(msg.decode('ascii'))
- */
-
-static int __pyx_memoryview_err(PyObject *__pyx_v_error, char *__pyx_v_msg) {
- int __pyx_r;
- __Pyx_RefNannyDeclarations
- int __pyx_t_1;
- PyObject *__pyx_t_2 = NULL;
- PyObject *__pyx_t_3 = NULL;
- PyObject *__pyx_t_4 = NULL;
- PyObject *__pyx_t_5 = NULL;
- int __pyx_lineno = 0;
- const char *__pyx_filename = NULL;
- int __pyx_clineno = 0;
- #ifdef WITH_THREAD
- PyGILState_STATE __pyx_gilstate_save = __Pyx_PyGILState_Ensure();
- #endif
- __Pyx_RefNannySetupContext("_err", 0);
- __Pyx_INCREF(__pyx_v_error);
-
- /* "View.MemoryView":1262
- * @cname('__pyx_memoryview_err')
- * cdef int _err(object error, char *msg) except -1 with gil:
- * if msg != NULL: # <<<<<<<<<<<<<<
- * raise error(msg.decode('ascii'))
- * else:
- */
- __pyx_t_1 = ((__pyx_v_msg != NULL) != 0);
- if (unlikely(__pyx_t_1)) {
-
- /* "View.MemoryView":1263
- * cdef int _err(object error, char *msg) except -1 with gil:
- * if msg != NULL:
- * raise error(msg.decode('ascii')) # <<<<<<<<<<<<<<
- * else:
- * raise error
- */
- __pyx_t_3 = __Pyx_decode_c_string(__pyx_v_msg, 0, strlen(__pyx_v_msg), NULL, NULL, PyUnicode_DecodeASCII); if (unlikely(!__pyx_t_3)) __PYX_ERR(1, 1263, __pyx_L1_error)
- __Pyx_GOTREF(__pyx_t_3);
- __Pyx_INCREF(__pyx_v_error);
- __pyx_t_4 = __pyx_v_error; __pyx_t_5 = NULL;
- if (CYTHON_UNPACK_METHODS && unlikely(PyMethod_Check(__pyx_t_4))) {
- __pyx_t_5 = PyMethod_GET_SELF(__pyx_t_4);
- if (likely(__pyx_t_5)) {
- PyObject* function = PyMethod_GET_FUNCTION(__pyx_t_4);
- __Pyx_INCREF(__pyx_t_5);
- __Pyx_INCREF(function);
- __Pyx_DECREF_SET(__pyx_t_4, function);
- }
- }
- __pyx_t_2 = (__pyx_t_5) ? __Pyx_PyObject_Call2Args(__pyx_t_4, __pyx_t_5, __pyx_t_3) : __Pyx_PyObject_CallOneArg(__pyx_t_4, __pyx_t_3);
- __Pyx_XDECREF(__pyx_t_5); __pyx_t_5 = 0;
- __Pyx_DECREF(__pyx_t_3); __pyx_t_3 = 0;
- if (unlikely(!__pyx_t_2)) __PYX_ERR(1, 1263, __pyx_L1_error)
- __Pyx_GOTREF(__pyx_t_2);
- __Pyx_DECREF(__pyx_t_4); __pyx_t_4 = 0;
- __Pyx_Raise(__pyx_t_2, 0, 0, 0);
- __Pyx_DECREF(__pyx_t_2); __pyx_t_2 = 0;
- __PYX_ERR(1, 1263, __pyx_L1_error)
-
- /* "View.MemoryView":1262
- * @cname('__pyx_memoryview_err')
- * cdef int _err(object error, char *msg) except -1 with gil:
- * if msg != NULL: # <<<<<<<<<<<<<<
- * raise error(msg.decode('ascii'))
- * else:
- */
- }
-
- /* "View.MemoryView":1265
- * raise error(msg.decode('ascii'))
- * else:
- * raise error # <<<<<<<<<<<<<<
- *
- * @cname('__pyx_memoryview_copy_contents')
- */
- /*else*/ {
- __Pyx_Raise(__pyx_v_error, 0, 0, 0);
- __PYX_ERR(1, 1265, __pyx_L1_error)
- }
-
- /* "View.MemoryView":1261
- *
- * @cname('__pyx_memoryview_err')
- * cdef int _err(object error, char *msg) except -1 with gil: # <<<<<<<<<<<<<<
- * if msg != NULL:
- * raise error(msg.decode('ascii'))
- */
-
- /* function exit code */
- __pyx_L1_error:;
- __Pyx_XDECREF(__pyx_t_2);
- __Pyx_XDECREF(__pyx_t_3);
- __Pyx_XDECREF(__pyx_t_4);
- __Pyx_XDECREF(__pyx_t_5);
- __Pyx_AddTraceback("View.MemoryView._err", __pyx_clineno, __pyx_lineno, __pyx_filename);
- __pyx_r = -1;
- __Pyx_XDECREF(__pyx_v_error);
- __Pyx_RefNannyFinishContext();
- #ifdef WITH_THREAD
- __Pyx_PyGILState_Release(__pyx_gilstate_save);
- #endif
- return __pyx_r;
-}
-
-/* "View.MemoryView":1268
- *
- * @cname('__pyx_memoryview_copy_contents')
- * cdef int memoryview_copy_contents(__Pyx_memviewslice src, # <<<<<<<<<<<<<<
- * __Pyx_memviewslice dst,
- * int src_ndim, int dst_ndim,
- */
-
-static int __pyx_memoryview_copy_contents(__Pyx_memviewslice __pyx_v_src, __Pyx_memviewslice __pyx_v_dst, int __pyx_v_src_ndim, int __pyx_v_dst_ndim, int __pyx_v_dtype_is_object) {
- void *__pyx_v_tmpdata;
- size_t __pyx_v_itemsize;
- int __pyx_v_i;
- char __pyx_v_order;
- int __pyx_v_broadcasting;
- int __pyx_v_direct_copy;
- __Pyx_memviewslice __pyx_v_tmp;
- int __pyx_v_ndim;
- int __pyx_r;
- Py_ssize_t __pyx_t_1;
- int __pyx_t_2;
- int __pyx_t_3;
- int __pyx_t_4;
- int __pyx_t_5;
- int __pyx_t_6;
- void *__pyx_t_7;
- int __pyx_t_8;
- int __pyx_lineno = 0;
- const char *__pyx_filename = NULL;
- int __pyx_clineno = 0;
-
- /* "View.MemoryView":1276
- * Check for overlapping memory and verify the shapes.
- * """
- * cdef void *tmpdata = NULL # <<<<<<<<<<<<<<
- * cdef size_t itemsize = src.memview.view.itemsize
- * cdef int i
- */
- __pyx_v_tmpdata = NULL;
-
- /* "View.MemoryView":1277
- * """
- * cdef void *tmpdata = NULL
- * cdef size_t itemsize = src.memview.view.itemsize # <<<<<<<<<<<<<<
- * cdef int i
- * cdef char order = get_best_order(&src, src_ndim)
- */
- __pyx_t_1 = __pyx_v_src.memview->view.itemsize;
- __pyx_v_itemsize = __pyx_t_1;
-
- /* "View.MemoryView":1279
- * cdef size_t itemsize = src.memview.view.itemsize
- * cdef int i
- * cdef char order = get_best_order(&src, src_ndim) # <<<<<<<<<<<<<<
- * cdef bint broadcasting = False
- * cdef bint direct_copy = False
- */
- __pyx_v_order = __pyx_get_best_slice_order((&__pyx_v_src), __pyx_v_src_ndim);
-
- /* "View.MemoryView":1280
- * cdef int i
- * cdef char order = get_best_order(&src, src_ndim)
- * cdef bint broadcasting = False # <<<<<<<<<<<<<<
- * cdef bint direct_copy = False
- * cdef __Pyx_memviewslice tmp
- */
- __pyx_v_broadcasting = 0;
-
- /* "View.MemoryView":1281
- * cdef char order = get_best_order(&src, src_ndim)
- * cdef bint broadcasting = False
- * cdef bint direct_copy = False # <<<<<<<<<<<<<<
- * cdef __Pyx_memviewslice tmp
- *
- */
- __pyx_v_direct_copy = 0;
-
- /* "View.MemoryView":1284
- * cdef __Pyx_memviewslice tmp
- *
- * if src_ndim < dst_ndim: # <<<<<<<<<<<<<<
- * broadcast_leading(&src, src_ndim, dst_ndim)
- * elif dst_ndim < src_ndim:
- */
- __pyx_t_2 = ((__pyx_v_src_ndim < __pyx_v_dst_ndim) != 0);
- if (__pyx_t_2) {
-
- /* "View.MemoryView":1285
- *
- * if src_ndim < dst_ndim:
- * broadcast_leading(&src, src_ndim, dst_ndim) # <<<<<<<<<<<<<<
- * elif dst_ndim < src_ndim:
- * broadcast_leading(&dst, dst_ndim, src_ndim)
- */
- __pyx_memoryview_broadcast_leading((&__pyx_v_src), __pyx_v_src_ndim, __pyx_v_dst_ndim);
-
- /* "View.MemoryView":1284
- * cdef __Pyx_memviewslice tmp
- *
- * if src_ndim < dst_ndim: # <<<<<<<<<<<<<<
- * broadcast_leading(&src, src_ndim, dst_ndim)
- * elif dst_ndim < src_ndim:
- */
- goto __pyx_L3;
- }
-
- /* "View.MemoryView":1286
- * if src_ndim < dst_ndim:
- * broadcast_leading(&src, src_ndim, dst_ndim)
- * elif dst_ndim < src_ndim: # <<<<<<<<<<<<<<
- * broadcast_leading(&dst, dst_ndim, src_ndim)
- *
- */
- __pyx_t_2 = ((__pyx_v_dst_ndim < __pyx_v_src_ndim) != 0);
- if (__pyx_t_2) {
-
- /* "View.MemoryView":1287
- * broadcast_leading(&src, src_ndim, dst_ndim)
- * elif dst_ndim < src_ndim:
- * broadcast_leading(&dst, dst_ndim, src_ndim) # <<<<<<<<<<<<<<
- *
- * cdef int ndim = max(src_ndim, dst_ndim)
- */
- __pyx_memoryview_broadcast_leading((&__pyx_v_dst), __pyx_v_dst_ndim, __pyx_v_src_ndim);
-
- /* "View.MemoryView":1286
- * if src_ndim < dst_ndim:
- * broadcast_leading(&src, src_ndim, dst_ndim)
- * elif dst_ndim < src_ndim: # <<<<<<<<<<<<<<
- * broadcast_leading(&dst, dst_ndim, src_ndim)
- *
- */
- }
- __pyx_L3:;
-
- /* "View.MemoryView":1289
- * broadcast_leading(&dst, dst_ndim, src_ndim)
- *
- * cdef int ndim = max(src_ndim, dst_ndim) # <<<<<<<<<<<<<<
- *
- * for i in range(ndim):
- */
- __pyx_t_3 = __pyx_v_dst_ndim;
- __pyx_t_4 = __pyx_v_src_ndim;
- if (((__pyx_t_3 > __pyx_t_4) != 0)) {
- __pyx_t_5 = __pyx_t_3;
- } else {
- __pyx_t_5 = __pyx_t_4;
- }
- __pyx_v_ndim = __pyx_t_5;
-
- /* "View.MemoryView":1291
- * cdef int ndim = max(src_ndim, dst_ndim)
- *
- * for i in range(ndim): # <<<<<<<<<<<<<<
- * if src.shape[i] != dst.shape[i]:
- * if src.shape[i] == 1:
- */
- __pyx_t_5 = __pyx_v_ndim;
- __pyx_t_3 = __pyx_t_5;
- for (__pyx_t_4 = 0; __pyx_t_4 < __pyx_t_3; __pyx_t_4+=1) {
- __pyx_v_i = __pyx_t_4;
-
- /* "View.MemoryView":1292
- *
- * for i in range(ndim):
- * if src.shape[i] != dst.shape[i]: # <<<<<<<<<<<<<<
- * if src.shape[i] == 1:
- * broadcasting = True
- */
- __pyx_t_2 = (((__pyx_v_src.shape[__pyx_v_i]) != (__pyx_v_dst.shape[__pyx_v_i])) != 0);
- if (__pyx_t_2) {
-
- /* "View.MemoryView":1293
- * for i in range(ndim):
- * if src.shape[i] != dst.shape[i]:
- * if src.shape[i] == 1: # <<<<<<<<<<<<<<
- * broadcasting = True
- * src.strides[i] = 0
- */
- __pyx_t_2 = (((__pyx_v_src.shape[__pyx_v_i]) == 1) != 0);
- if (__pyx_t_2) {
-
- /* "View.MemoryView":1294
- * if src.shape[i] != dst.shape[i]:
- * if src.shape[i] == 1:
- * broadcasting = True # <<<<<<<<<<<<<<
- * src.strides[i] = 0
- * else:
- */
- __pyx_v_broadcasting = 1;
-
- /* "View.MemoryView":1295
- * if src.shape[i] == 1:
- * broadcasting = True
- * src.strides[i] = 0 # <<<<<<<<<<<<<<
- * else:
- * _err_extents(i, dst.shape[i], src.shape[i])
- */
- (__pyx_v_src.strides[__pyx_v_i]) = 0;
-
- /* "View.MemoryView":1293
- * for i in range(ndim):
- * if src.shape[i] != dst.shape[i]:
- * if src.shape[i] == 1: # <<<<<<<<<<<<<<
- * broadcasting = True
- * src.strides[i] = 0
- */
- goto __pyx_L7;
- }
-
- /* "View.MemoryView":1297
- * src.strides[i] = 0
- * else:
- * _err_extents(i, dst.shape[i], src.shape[i]) # <<<<<<<<<<<<<<
- *
- * if src.suboffsets[i] >= 0:
- */
- /*else*/ {
- __pyx_t_6 = __pyx_memoryview_err_extents(__pyx_v_i, (__pyx_v_dst.shape[__pyx_v_i]), (__pyx_v_src.shape[__pyx_v_i])); if (unlikely(__pyx_t_6 == ((int)-1))) __PYX_ERR(1, 1297, __pyx_L1_error)
- }
- __pyx_L7:;
-
- /* "View.MemoryView":1292
- *
- * for i in range(ndim):
- * if src.shape[i] != dst.shape[i]: # <<<<<<<<<<<<<<
- * if src.shape[i] == 1:
- * broadcasting = True
- */
- }
-
- /* "View.MemoryView":1299
- * _err_extents(i, dst.shape[i], src.shape[i])
- *
- * if src.suboffsets[i] >= 0: # <<<<<<<<<<<<<<
- * _err_dim(ValueError, "Dimension %d is not direct", i)
- *
- */
- __pyx_t_2 = (((__pyx_v_src.suboffsets[__pyx_v_i]) >= 0) != 0);
- if (__pyx_t_2) {
-
- /* "View.MemoryView":1300
- *
- * if src.suboffsets[i] >= 0:
- * _err_dim(ValueError, "Dimension %d is not direct", i) # <<<<<<<<<<<<<<
- *
- * if slices_overlap(&src, &dst, ndim, itemsize):
- */
- __pyx_t_6 = __pyx_memoryview_err_dim(__pyx_builtin_ValueError, ((char *)"Dimension %d is not direct"), __pyx_v_i); if (unlikely(__pyx_t_6 == ((int)-1))) __PYX_ERR(1, 1300, __pyx_L1_error)
-
- /* "View.MemoryView":1299
- * _err_extents(i, dst.shape[i], src.shape[i])
- *
- * if src.suboffsets[i] >= 0: # <<<<<<<<<<<<<<
- * _err_dim(ValueError, "Dimension %d is not direct", i)
- *
- */
- }
- }
-
- /* "View.MemoryView":1302
- * _err_dim(ValueError, "Dimension %d is not direct", i)
- *
- * if slices_overlap(&src, &dst, ndim, itemsize): # <<<<<<<<<<<<<<
- *
- * if not slice_is_contig(src, order, ndim):
- */
- __pyx_t_2 = (__pyx_slices_overlap((&__pyx_v_src), (&__pyx_v_dst), __pyx_v_ndim, __pyx_v_itemsize) != 0);
- if (__pyx_t_2) {
-
- /* "View.MemoryView":1304
- * if slices_overlap(&src, &dst, ndim, itemsize):
- *
- * if not slice_is_contig(src, order, ndim): # <<<<<<<<<<<<<<
- * order = get_best_order(&dst, ndim)
- *
- */
- __pyx_t_2 = ((!(__pyx_memviewslice_is_contig(__pyx_v_src, __pyx_v_order, __pyx_v_ndim) != 0)) != 0);
- if (__pyx_t_2) {
-
- /* "View.MemoryView":1305
- *
- * if not slice_is_contig(src, order, ndim):
- * order = get_best_order(&dst, ndim) # <<<<<<<<<<<<<<
- *
- * tmpdata = copy_data_to_temp(&src, &tmp, order, ndim)
- */
- __pyx_v_order = __pyx_get_best_slice_order((&__pyx_v_dst), __pyx_v_ndim);
-
- /* "View.MemoryView":1304
- * if slices_overlap(&src, &dst, ndim, itemsize):
- *
- * if not slice_is_contig(src, order, ndim): # <<<<<<<<<<<<<<
- * order = get_best_order(&dst, ndim)
- *
- */
- }
-
- /* "View.MemoryView":1307
- * order = get_best_order(&dst, ndim)
- *
- * tmpdata = copy_data_to_temp(&src, &tmp, order, ndim) # <<<<<<<<<<<<<<
- * src = tmp
- *
- */
- __pyx_t_7 = __pyx_memoryview_copy_data_to_temp((&__pyx_v_src), (&__pyx_v_tmp), __pyx_v_order, __pyx_v_ndim); if (unlikely(__pyx_t_7 == ((void *)NULL))) __PYX_ERR(1, 1307, __pyx_L1_error)
- __pyx_v_tmpdata = __pyx_t_7;
-
- /* "View.MemoryView":1308
- *
- * tmpdata = copy_data_to_temp(&src, &tmp, order, ndim)
- * src = tmp # <<<<<<<<<<<<<<
- *
- * if not broadcasting:
- */
- __pyx_v_src = __pyx_v_tmp;
-
- /* "View.MemoryView":1302
- * _err_dim(ValueError, "Dimension %d is not direct", i)
- *
- * if slices_overlap(&src, &dst, ndim, itemsize): # <<<<<<<<<<<<<<
- *
- * if not slice_is_contig(src, order, ndim):
- */
- }
-
- /* "View.MemoryView":1310
- * src = tmp
- *
- * if not broadcasting: # <<<<<<<<<<<<<<
- *
- *
- */
- __pyx_t_2 = ((!(__pyx_v_broadcasting != 0)) != 0);
- if (__pyx_t_2) {
-
- /* "View.MemoryView":1313
- *
- *
- * if slice_is_contig(src, 'C', ndim): # <<<<<<<<<<<<<<
- * direct_copy = slice_is_contig(dst, 'C', ndim)
- * elif slice_is_contig(src, 'F', ndim):
- */
- __pyx_t_2 = (__pyx_memviewslice_is_contig(__pyx_v_src, 'C', __pyx_v_ndim) != 0);
- if (__pyx_t_2) {
-
- /* "View.MemoryView":1314
- *
- * if slice_is_contig(src, 'C', ndim):
- * direct_copy = slice_is_contig(dst, 'C', ndim) # <<<<<<<<<<<<<<
- * elif slice_is_contig(src, 'F', ndim):
- * direct_copy = slice_is_contig(dst, 'F', ndim)
- */
- __pyx_v_direct_copy = __pyx_memviewslice_is_contig(__pyx_v_dst, 'C', __pyx_v_ndim);
-
- /* "View.MemoryView":1313
- *
- *
- * if slice_is_contig(src, 'C', ndim): # <<<<<<<<<<<<<<
- * direct_copy = slice_is_contig(dst, 'C', ndim)
- * elif slice_is_contig(src, 'F', ndim):
- */
- goto __pyx_L12;
- }
-
- /* "View.MemoryView":1315
- * if slice_is_contig(src, 'C', ndim):
- * direct_copy = slice_is_contig(dst, 'C', ndim)
- * elif slice_is_contig(src, 'F', ndim): # <<<<<<<<<<<<<<
- * direct_copy = slice_is_contig(dst, 'F', ndim)
- *
- */
- __pyx_t_2 = (__pyx_memviewslice_is_contig(__pyx_v_src, 'F', __pyx_v_ndim) != 0);
- if (__pyx_t_2) {
-
- /* "View.MemoryView":1316
- * direct_copy = slice_is_contig(dst, 'C', ndim)
- * elif slice_is_contig(src, 'F', ndim):
- * direct_copy = slice_is_contig(dst, 'F', ndim) # <<<<<<<<<<<<<<
- *
- * if direct_copy:
- */
- __pyx_v_direct_copy = __pyx_memviewslice_is_contig(__pyx_v_dst, 'F', __pyx_v_ndim);
-
- /* "View.MemoryView":1315
- * if slice_is_contig(src, 'C', ndim):
- * direct_copy = slice_is_contig(dst, 'C', ndim)
- * elif slice_is_contig(src, 'F', ndim): # <<<<<<<<<<<<<<
- * direct_copy = slice_is_contig(dst, 'F', ndim)
- *
- */
- }
- __pyx_L12:;
-
- /* "View.MemoryView":1318
- * direct_copy = slice_is_contig(dst, 'F', ndim)
- *
- * if direct_copy: # <<<<<<<<<<<<<<
- *
- * refcount_copying(&dst, dtype_is_object, ndim, False)
- */
- __pyx_t_2 = (__pyx_v_direct_copy != 0);
- if (__pyx_t_2) {
-
- /* "View.MemoryView":1320
- * if direct_copy:
- *
- * refcount_copying(&dst, dtype_is_object, ndim, False) # <<<<<<<<<<<<<<
- * memcpy(dst.data, src.data, slice_get_size(&src, ndim))
- * refcount_copying(&dst, dtype_is_object, ndim, True)
- */
- __pyx_memoryview_refcount_copying((&__pyx_v_dst), __pyx_v_dtype_is_object, __pyx_v_ndim, 0);
-
- /* "View.MemoryView":1321
- *
- * refcount_copying(&dst, dtype_is_object, ndim, False)
- * memcpy(dst.data, src.data, slice_get_size(&src, ndim)) # <<<<<<<<<<<<<<
- * refcount_copying(&dst, dtype_is_object, ndim, True)
- * free(tmpdata)
- */
- (void)(memcpy(__pyx_v_dst.data, __pyx_v_src.data, __pyx_memoryview_slice_get_size((&__pyx_v_src), __pyx_v_ndim)));
-
- /* "View.MemoryView":1322
- * refcount_copying(&dst, dtype_is_object, ndim, False)
- * memcpy(dst.data, src.data, slice_get_size(&src, ndim))
- * refcount_copying(&dst, dtype_is_object, ndim, True) # <<<<<<<<<<<<<<
- * free(tmpdata)
- * return 0
- */
- __pyx_memoryview_refcount_copying((&__pyx_v_dst), __pyx_v_dtype_is_object, __pyx_v_ndim, 1);
-
- /* "View.MemoryView":1323
- * memcpy(dst.data, src.data, slice_get_size(&src, ndim))
- * refcount_copying(&dst, dtype_is_object, ndim, True)
- * free(tmpdata) # <<<<<<<<<<<<<<
- * return 0
- *
- */
- free(__pyx_v_tmpdata);
-
- /* "View.MemoryView":1324
- * refcount_copying(&dst, dtype_is_object, ndim, True)
- * free(tmpdata)
- * return 0 # <<<<<<<<<<<<<<
- *
- * if order == 'F' == get_best_order(&dst, ndim):
- */
- __pyx_r = 0;
- goto __pyx_L0;
-
- /* "View.MemoryView":1318
- * direct_copy = slice_is_contig(dst, 'F', ndim)
- *
- * if direct_copy: # <<<<<<<<<<<<<<
- *
- * refcount_copying(&dst, dtype_is_object, ndim, False)
- */
- }
-
- /* "View.MemoryView":1310
- * src = tmp
- *
- * if not broadcasting: # <<<<<<<<<<<<<<
- *
- *
- */
- }
-
- /* "View.MemoryView":1326
- * return 0
- *
- * if order == 'F' == get_best_order(&dst, ndim): # <<<<<<<<<<<<<<
- *
- *
- */
- __pyx_t_2 = (__pyx_v_order == 'F');
- if (__pyx_t_2) {
- __pyx_t_2 = ('F' == __pyx_get_best_slice_order((&__pyx_v_dst), __pyx_v_ndim));
- }
- __pyx_t_8 = (__pyx_t_2 != 0);
- if (__pyx_t_8) {
-
- /* "View.MemoryView":1329
- *
- *
- * transpose_memslice(&src) # <<<<<<<<<<<<<<
- * transpose_memslice(&dst)
- *
- */
- __pyx_t_5 = __pyx_memslice_transpose((&__pyx_v_src)); if (unlikely(__pyx_t_5 == ((int)0))) __PYX_ERR(1, 1329, __pyx_L1_error)
-
- /* "View.MemoryView":1330
- *
- * transpose_memslice(&src)
- * transpose_memslice(&dst) # <<<<<<<<<<<<<<
- *
- * refcount_copying(&dst, dtype_is_object, ndim, False)
- */
- __pyx_t_5 = __pyx_memslice_transpose((&__pyx_v_dst)); if (unlikely(__pyx_t_5 == ((int)0))) __PYX_ERR(1, 1330, __pyx_L1_error)
-
- /* "View.MemoryView":1326
- * return 0
- *
- * if order == 'F' == get_best_order(&dst, ndim): # <<<<<<<<<<<<<<
- *
- *
- */
- }
-
- /* "View.MemoryView":1332
- * transpose_memslice(&dst)
- *
- * refcount_copying(&dst, dtype_is_object, ndim, False) # <<<<<<<<<<<<<<
- * copy_strided_to_strided(&src, &dst, ndim, itemsize)
- * refcount_copying(&dst, dtype_is_object, ndim, True)
- */
- __pyx_memoryview_refcount_copying((&__pyx_v_dst), __pyx_v_dtype_is_object, __pyx_v_ndim, 0);
-
- /* "View.MemoryView":1333
- *
- * refcount_copying(&dst, dtype_is_object, ndim, False)
- * copy_strided_to_strided(&src, &dst, ndim, itemsize) # <<<<<<<<<<<<<<
- * refcount_copying(&dst, dtype_is_object, ndim, True)
- *
- */
- copy_strided_to_strided((&__pyx_v_src), (&__pyx_v_dst), __pyx_v_ndim, __pyx_v_itemsize);
-
- /* "View.MemoryView":1334
- * refcount_copying(&dst, dtype_is_object, ndim, False)
- * copy_strided_to_strided(&src, &dst, ndim, itemsize)
- * refcount_copying(&dst, dtype_is_object, ndim, True) # <<<<<<<<<<<<<<
- *
- * free(tmpdata)
- */
- __pyx_memoryview_refcount_copying((&__pyx_v_dst), __pyx_v_dtype_is_object, __pyx_v_ndim, 1);
-
- /* "View.MemoryView":1336
- * refcount_copying(&dst, dtype_is_object, ndim, True)
- *
- * free(tmpdata) # <<<<<<<<<<<<<<
- * return 0
- *
- */
- free(__pyx_v_tmpdata);
-
- /* "View.MemoryView":1337
- *
- * free(tmpdata)
- * return 0 # <<<<<<<<<<<<<<
- *
- * @cname('__pyx_memoryview_broadcast_leading')
- */
- __pyx_r = 0;
- goto __pyx_L0;
-
- /* "View.MemoryView":1268
- *
- * @cname('__pyx_memoryview_copy_contents')
- * cdef int memoryview_copy_contents(__Pyx_memviewslice src, # <<<<<<<<<<<<<<
- * __Pyx_memviewslice dst,
- * int src_ndim, int dst_ndim,
- */
-
- /* function exit code */
- __pyx_L1_error:;
- {
- #ifdef WITH_THREAD
- PyGILState_STATE __pyx_gilstate_save = __Pyx_PyGILState_Ensure();
- #endif
- __Pyx_AddTraceback("View.MemoryView.memoryview_copy_contents", __pyx_clineno, __pyx_lineno, __pyx_filename);
- #ifdef WITH_THREAD
- __Pyx_PyGILState_Release(__pyx_gilstate_save);
- #endif
- }
- __pyx_r = -1;
- __pyx_L0:;
- return __pyx_r;
-}
-
-/* "View.MemoryView":1340
- *
- * @cname('__pyx_memoryview_broadcast_leading')
- * cdef void broadcast_leading(__Pyx_memviewslice *mslice, # <<<<<<<<<<<<<<
- * int ndim,
- * int ndim_other) nogil:
- */
-
-static void __pyx_memoryview_broadcast_leading(__Pyx_memviewslice *__pyx_v_mslice, int __pyx_v_ndim, int __pyx_v_ndim_other) {
- int __pyx_v_i;
- int __pyx_v_offset;
- int __pyx_t_1;
- int __pyx_t_2;
- int __pyx_t_3;
-
- /* "View.MemoryView":1344
- * int ndim_other) nogil:
- * cdef int i
- * cdef int offset = ndim_other - ndim # <<<<<<<<<<<<<<
- *
- * for i in range(ndim - 1, -1, -1):
- */
- __pyx_v_offset = (__pyx_v_ndim_other - __pyx_v_ndim);
-
- /* "View.MemoryView":1346
- * cdef int offset = ndim_other - ndim
- *
- * for i in range(ndim - 1, -1, -1): # <<<<<<<<<<<<<<
- * mslice.shape[i + offset] = mslice.shape[i]
- * mslice.strides[i + offset] = mslice.strides[i]
- */
- for (__pyx_t_1 = (__pyx_v_ndim - 1); __pyx_t_1 > -1; __pyx_t_1-=1) {
- __pyx_v_i = __pyx_t_1;
-
- /* "View.MemoryView":1347
- *
- * for i in range(ndim - 1, -1, -1):
- * mslice.shape[i + offset] = mslice.shape[i] # <<<<<<<<<<<<<<
- * mslice.strides[i + offset] = mslice.strides[i]
- * mslice.suboffsets[i + offset] = mslice.suboffsets[i]
- */
- (__pyx_v_mslice->shape[(__pyx_v_i + __pyx_v_offset)]) = (__pyx_v_mslice->shape[__pyx_v_i]);
-
- /* "View.MemoryView":1348
- * for i in range(ndim - 1, -1, -1):
- * mslice.shape[i + offset] = mslice.shape[i]
- * mslice.strides[i + offset] = mslice.strides[i] # <<<<<<<<<<<<<<
- * mslice.suboffsets[i + offset] = mslice.suboffsets[i]
- *
- */
- (__pyx_v_mslice->strides[(__pyx_v_i + __pyx_v_offset)]) = (__pyx_v_mslice->strides[__pyx_v_i]);
-
- /* "View.MemoryView":1349
- * mslice.shape[i + offset] = mslice.shape[i]
- * mslice.strides[i + offset] = mslice.strides[i]
- * mslice.suboffsets[i + offset] = mslice.suboffsets[i] # <<<<<<<<<<<<<<
- *
- * for i in range(offset):
- */
- (__pyx_v_mslice->suboffsets[(__pyx_v_i + __pyx_v_offset)]) = (__pyx_v_mslice->suboffsets[__pyx_v_i]);
- }
-
- /* "View.MemoryView":1351
- * mslice.suboffsets[i + offset] = mslice.suboffsets[i]
- *
- * for i in range(offset): # <<<<<<<<<<<<<<
- * mslice.shape[i] = 1
- * mslice.strides[i] = mslice.strides[0]
- */
- __pyx_t_1 = __pyx_v_offset;
- __pyx_t_2 = __pyx_t_1;
- for (__pyx_t_3 = 0; __pyx_t_3 < __pyx_t_2; __pyx_t_3+=1) {
- __pyx_v_i = __pyx_t_3;
-
- /* "View.MemoryView":1352
- *
- * for i in range(offset):
- * mslice.shape[i] = 1 # <<<<<<<<<<<<<<
- * mslice.strides[i] = mslice.strides[0]
- * mslice.suboffsets[i] = -1
- */
- (__pyx_v_mslice->shape[__pyx_v_i]) = 1;
-
- /* "View.MemoryView":1353
- * for i in range(offset):
- * mslice.shape[i] = 1
- * mslice.strides[i] = mslice.strides[0] # <<<<<<<<<<<<<<
- * mslice.suboffsets[i] = -1
- *
- */
- (__pyx_v_mslice->strides[__pyx_v_i]) = (__pyx_v_mslice->strides[0]);
-
- /* "View.MemoryView":1354
- * mslice.shape[i] = 1
- * mslice.strides[i] = mslice.strides[0]
- * mslice.suboffsets[i] = -1 # <<<<<<<<<<<<<<
- *
- *
- */
- (__pyx_v_mslice->suboffsets[__pyx_v_i]) = -1L;
- }
-
- /* "View.MemoryView":1340
- *
- * @cname('__pyx_memoryview_broadcast_leading')
- * cdef void broadcast_leading(__Pyx_memviewslice *mslice, # <<<<<<<<<<<<<<
- * int ndim,
- * int ndim_other) nogil:
- */
-
- /* function exit code */
-}
-
-/* "View.MemoryView":1362
- *
- * @cname('__pyx_memoryview_refcount_copying')
- * cdef void refcount_copying(__Pyx_memviewslice *dst, bint dtype_is_object, # <<<<<<<<<<<<<<
- * int ndim, bint inc) nogil:
- *
- */
-
-static void __pyx_memoryview_refcount_copying(__Pyx_memviewslice *__pyx_v_dst, int __pyx_v_dtype_is_object, int __pyx_v_ndim, int __pyx_v_inc) {
- int __pyx_t_1;
-
- /* "View.MemoryView":1366
- *
- *
- * if dtype_is_object: # <<<<<<<<<<<<<<
- * refcount_objects_in_slice_with_gil(dst.data, dst.shape,
- * dst.strides, ndim, inc)
- */
- __pyx_t_1 = (__pyx_v_dtype_is_object != 0);
- if (__pyx_t_1) {
-
- /* "View.MemoryView":1367
- *
- * if dtype_is_object:
- * refcount_objects_in_slice_with_gil(dst.data, dst.shape, # <<<<<<<<<<<<<<
- * dst.strides, ndim, inc)
- *
- */
- __pyx_memoryview_refcount_objects_in_slice_with_gil(__pyx_v_dst->data, __pyx_v_dst->shape, __pyx_v_dst->strides, __pyx_v_ndim, __pyx_v_inc);
-
- /* "View.MemoryView":1366
- *
- *
- * if dtype_is_object: # <<<<<<<<<<<<<<
- * refcount_objects_in_slice_with_gil(dst.data, dst.shape,
- * dst.strides, ndim, inc)
- */
- }
-
- /* "View.MemoryView":1362
- *
- * @cname('__pyx_memoryview_refcount_copying')
- * cdef void refcount_copying(__Pyx_memviewslice *dst, bint dtype_is_object, # <<<<<<<<<<<<<<
- * int ndim, bint inc) nogil:
- *
- */
-
- /* function exit code */
-}
-
-/* "View.MemoryView":1371
- *
- * @cname('__pyx_memoryview_refcount_objects_in_slice_with_gil')
- * cdef void refcount_objects_in_slice_with_gil(char *data, Py_ssize_t *shape, # <<<<<<<<<<<<<<
- * Py_ssize_t *strides, int ndim,
- * bint inc) with gil:
- */
-
-static void __pyx_memoryview_refcount_objects_in_slice_with_gil(char *__pyx_v_data, Py_ssize_t *__pyx_v_shape, Py_ssize_t *__pyx_v_strides, int __pyx_v_ndim, int __pyx_v_inc) {
- __Pyx_RefNannyDeclarations
- #ifdef WITH_THREAD
- PyGILState_STATE __pyx_gilstate_save = __Pyx_PyGILState_Ensure();
- #endif
- __Pyx_RefNannySetupContext("refcount_objects_in_slice_with_gil", 0);
-
- /* "View.MemoryView":1374
- * Py_ssize_t *strides, int ndim,
- * bint inc) with gil:
- * refcount_objects_in_slice(data, shape, strides, ndim, inc) # <<<<<<<<<<<<<<
- *
- * @cname('__pyx_memoryview_refcount_objects_in_slice')
- */
- __pyx_memoryview_refcount_objects_in_slice(__pyx_v_data, __pyx_v_shape, __pyx_v_strides, __pyx_v_ndim, __pyx_v_inc);
-
- /* "View.MemoryView":1371
- *
- * @cname('__pyx_memoryview_refcount_objects_in_slice_with_gil')
- * cdef void refcount_objects_in_slice_with_gil(char *data, Py_ssize_t *shape, # <<<<<<<<<<<<<<
- * Py_ssize_t *strides, int ndim,
- * bint inc) with gil:
- */
-
- /* function exit code */
- __Pyx_RefNannyFinishContext();
- #ifdef WITH_THREAD
- __Pyx_PyGILState_Release(__pyx_gilstate_save);
- #endif
-}
-
-/* "View.MemoryView":1377
- *
- * @cname('__pyx_memoryview_refcount_objects_in_slice')
- * cdef void refcount_objects_in_slice(char *data, Py_ssize_t *shape, # <<<<<<<<<<<<<<
- * Py_ssize_t *strides, int ndim, bint inc):
- * cdef Py_ssize_t i
- */
-
-static void __pyx_memoryview_refcount_objects_in_slice(char *__pyx_v_data, Py_ssize_t *__pyx_v_shape, Py_ssize_t *__pyx_v_strides, int __pyx_v_ndim, int __pyx_v_inc) {
- CYTHON_UNUSED Py_ssize_t __pyx_v_i;
- __Pyx_RefNannyDeclarations
- Py_ssize_t __pyx_t_1;
- Py_ssize_t __pyx_t_2;
- Py_ssize_t __pyx_t_3;
- int __pyx_t_4;
- __Pyx_RefNannySetupContext("refcount_objects_in_slice", 0);
-
- /* "View.MemoryView":1381
- * cdef Py_ssize_t i
- *
- * for i in range(shape[0]): # <<<<<<<<<<<<<<
- * if ndim == 1:
- * if inc:
- */
- __pyx_t_1 = (__pyx_v_shape[0]);
- __pyx_t_2 = __pyx_t_1;
- for (__pyx_t_3 = 0; __pyx_t_3 < __pyx_t_2; __pyx_t_3+=1) {
- __pyx_v_i = __pyx_t_3;
-
- /* "View.MemoryView":1382
- *
- * for i in range(shape[0]):
- * if ndim == 1: # <<<<<<<<<<<<<<
- * if inc:
- * Py_INCREF(( data)[0])
- */
- __pyx_t_4 = ((__pyx_v_ndim == 1) != 0);
- if (__pyx_t_4) {
-
- /* "View.MemoryView":1383
- * for i in range(shape[0]):
- * if ndim == 1:
- * if inc: # <<<<<<<<<<<<<<
- * Py_INCREF(( data)[0])
- * else:
- */
- __pyx_t_4 = (__pyx_v_inc != 0);
- if (__pyx_t_4) {
-
- /* "View.MemoryView":1384
- * if ndim == 1:
- * if inc:
- * Py_INCREF(( data)[0]) # <<<<<<<<<<<<<<
- * else:
- * Py_DECREF(( data)[0])
- */
- Py_INCREF((((PyObject **)__pyx_v_data)[0]));
-
- /* "View.MemoryView":1383
- * for i in range(shape[0]):
- * if ndim == 1:
- * if inc: # <<<<<<<<<<<<<<
- * Py_INCREF(( data)[0])
- * else:
- */
- goto __pyx_L6;
- }
-
- /* "View.MemoryView":1386
- * Py_INCREF(( data)[0])
- * else:
- * Py_DECREF(( data)[0]) # <<<<<<<<<<<<<<
- * else:
- * refcount_objects_in_slice(data, shape + 1, strides + 1,
- */
- /*else*/ {
- Py_DECREF((((PyObject **)__pyx_v_data)[0]));
- }
- __pyx_L6:;
-
- /* "View.MemoryView":1382
- *
- * for i in range(shape[0]):
- * if ndim == 1: # <<<<<<<<<<<<<<
- * if inc:
- * Py_INCREF(( data)[0])
- */
- goto __pyx_L5;
- }
-
- /* "View.MemoryView":1388
- * Py_DECREF((