# Stable Video Diffusion
[[open-in-colab]]
[Stable Video Diffusion (SVD)](https://huggingface.co/papers/2311.15127)은 입력 이미지에 맞춰 2~4초 분량의 고해상도(576x1024) 비디오를 생성할 수 있는 강력한 image-to-video 생성 모델입니다.
이 가이드에서는 SVD를 사용하여 이미지에서 짧은 동영상을 생성하는 방법을 설명합니다.
시작하기 전에 다음 라이브러리가 설치되어 있는지 확인하세요:
```py
!pip install -q -U diffusers transformers accelerate
```
이 모델에는 [SVD](https://huggingface.co/stabilityai/stable-video-diffusion-img2vid)와 [SVD-XT](https://huggingface.co/stabilityai/stable-video-diffusion-img2vid-xt) 두 가지 종류가 있습니다. SVD 체크포인트는 14개의 프레임을 생성하도록 학습되었고, SVD-XT 체크포인트는 25개의 프레임을 생성하도록 파인튜닝되었습니다.
이 가이드에서는 SVD-XT 체크포인트를 사용합니다.
```python
import torch
from diffusers import StableVideoDiffusionPipeline
from diffusers.utils import load_image, export_to_video
pipe = StableVideoDiffusionPipeline.from_pretrained(
"stabilityai/stable-video-diffusion-img2vid-xt", torch_dtype=torch.float16, variant="fp16"
)
pipe.enable_model_cpu_offload()
# Conditioning 이미지 불러오기
image = load_image("https://huggingface.co/datasets/huggingface/documentation-images/resolve/main/diffusers/svd/rocket.png")
image = image.resize((1024, 576))
generator = torch.manual_seed(42)
frames = pipe(image, decode_chunk_size=8, generator=generator).frames[0]
export_to_video(frames, "generated.mp4", fps=7)
```
"source image of a rocket"
"generated video from source image"
## torch.compile
UNet을 [컴파일](../optimization/torch2.0#torchcompile)하면 메모리 사용량이 살짝 증가하지만, 20~25%의 속도 향상을 얻을 수 있습니다.
```diff
- pipe.enable_model_cpu_offload()
+ pipe.to("cuda")
+ pipe.unet = torch.compile(pipe.unet, mode="reduce-overhead", fullgraph=True)
```
## 메모리 사용량 줄이기
비디오 생성은 기본적으로 배치 크기가 큰 text-to-image 생성과 유사하게 'num_frames'를 한 번에 생성하기 때문에 메모리 사용량이 매우 높습니다. 메모리 사용량을 줄이기 위해 추론 속도와 메모리 사용량을 절충하는 여러 가지 옵션이 있습니다:
- 모델 오프로링 활성화: 파이프라인의 각 구성 요소가 더 이상 필요하지 않을 때 CPU로 오프로드됩니다.
- Feed-forward chunking 활성화: feed-forward 레이어가 배치 크기가 큰 단일 feed-forward를 실행하는 대신 루프로 반복해서 실행됩니다.
- `decode_chunk_size` 감소: VAE가 프레임들을 한꺼번에 디코딩하는 대신 chunk 단위로 디코딩합니다. `decode_chunk_size=1`을 설정하면 한 번에 한 프레임씩 디코딩하고 최소한의 메모리만 사용하지만(GPU 메모리에 따라 이 값을 조정하는 것이 좋습니다), 동영상에 약간의 깜박임이 발생할 수 있습니다.
```diff
- pipe.enable_model_cpu_offload()
- frames = pipe(image, decode_chunk_size=8, generator=generator).frames[0]
+ pipe.enable_model_cpu_offload()
+ pipe.unet.enable_forward_chunking()
+ frames = pipe(image, decode_chunk_size=2, generator=generator, num_frames=25).frames[0]
```
이러한 모든 방법들을 사용하면 메모리 사용량이 8GAM VRAM보다 적을 것입니다.
## Micro-conditioning
Stable Diffusion Video는 또한 이미지 conditoning 외에도 micro-conditioning을 허용하므로 생성된 비디오를 더 잘 제어할 수 있습니다:
- `fps`: 생성된 비디오의 초당 프레임 수입니다.
- `motion_bucket_id`: 생성된 동영상에 사용할 모션 버킷 아이디입니다. 생성된 동영상의 모션을 제어하는 데 사용할 수 있습니다. 모션 버킷 아이디를 늘리면 생성되는 동영상의 모션이 증가합니다.
- `noise_aug_strength`: Conditioning 이미지에 추가되는 노이즈의 양입니다. 값이 클수록 비디오가 conditioning 이미지와 덜 유사해집니다. 이 값을 높이면 생성된 비디오의 움직임도 증가합니다.
예를 들어, 모션이 더 많은 동영상을 생성하려면 `motion_bucket_id` 및 `noise_aug_strength` micro-conditioning 파라미터를 사용합니다:
```python
import torch
from diffusers import StableVideoDiffusionPipeline
from diffusers.utils import load_image, export_to_video
pipe = StableVideoDiffusionPipeline.from_pretrained(
"stabilityai/stable-video-diffusion-img2vid-xt", torch_dtype=torch.float16, variant="fp16"
)
pipe.enable_model_cpu_offload()
# Conditioning 이미지 불러오기
image = load_image("https://huggingface.co/datasets/huggingface/documentation-images/resolve/main/diffusers/svd/rocket.png")
image = image.resize((1024, 576))
generator = torch.manual_seed(42)
frames = pipe(image, decode_chunk_size=8, generator=generator, motion_bucket_id=180, noise_aug_strength=0.1).frames[0]
export_to_video(frames, "generated.mp4", fps=7)
```
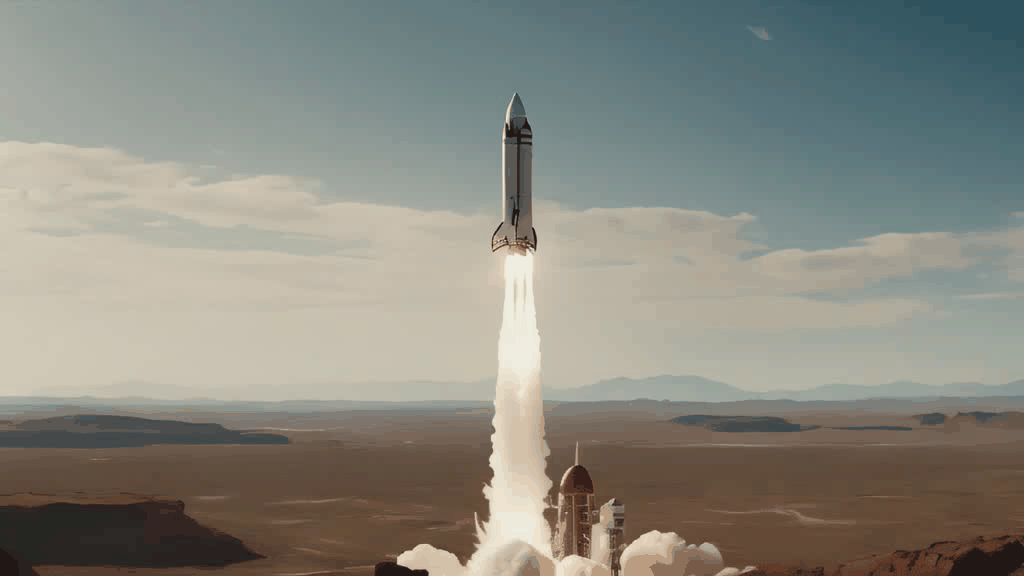