---
license: cc-by-nc-4.0
datasets:
- openbmb/VisRAG-Ret-Train-Synthetic-data
- openbmb/VisRAG-Ret-Train-In-domain-data
- Metric-AI/rag_docmatix_100k
- vidore/colpali_train_set
- llamaindex/vdr-multilingual-train
- Metric-AI/tabfquad_train_set
language:
- en
- fr
- es
- it
- de
base_model:
- Qwen/Qwen2.5-VL-7B-Instruct
tags:
- vidore
- multimodal_embedding
- multilingual_embedding
- Text-to-Visual Document (T→VD) retrieval
library_name: peft
pipeline_tag: visual-document-retrieval
---
# ColQwen2.5-7b-multilingual-v1.0: Multilingual Visual Retriever based on Qwen2.5-VL-7B-Instruct with ColBERT strategy
## Ranked #1 on the Vidore benchmark (as of February 11, 2025). The reported scores are on the [Vidore Leaderboard](https://huggingface.co/spaces/vidore/vidore-leaderboard).
### This is the base version trained on 4xA100 80GB with per_device_batch_size=64 and gradient_accumulation_steps=2 for 5 epoch.
ColQwen is a model based on a novel model architecture and training strategy based on Vision Language Models (VLMs) to efficiently index documents from their visual features.
It is a [Qwen2.5-VL-3B](https://huggingface.co/Qwen/Qwen2.5-VL-3B-Instruct) extension that generates [ColBERT](https://arxiv.org/abs/2004.12832)- style multi-vector representations of text and images.
It was introduced in the paper [ColPali: Efficient Document Retrieval with Vision Language Models](https://arxiv.org/abs/2407.01449) and first released in [this repository](https://github.com/ManuelFay/colpali)
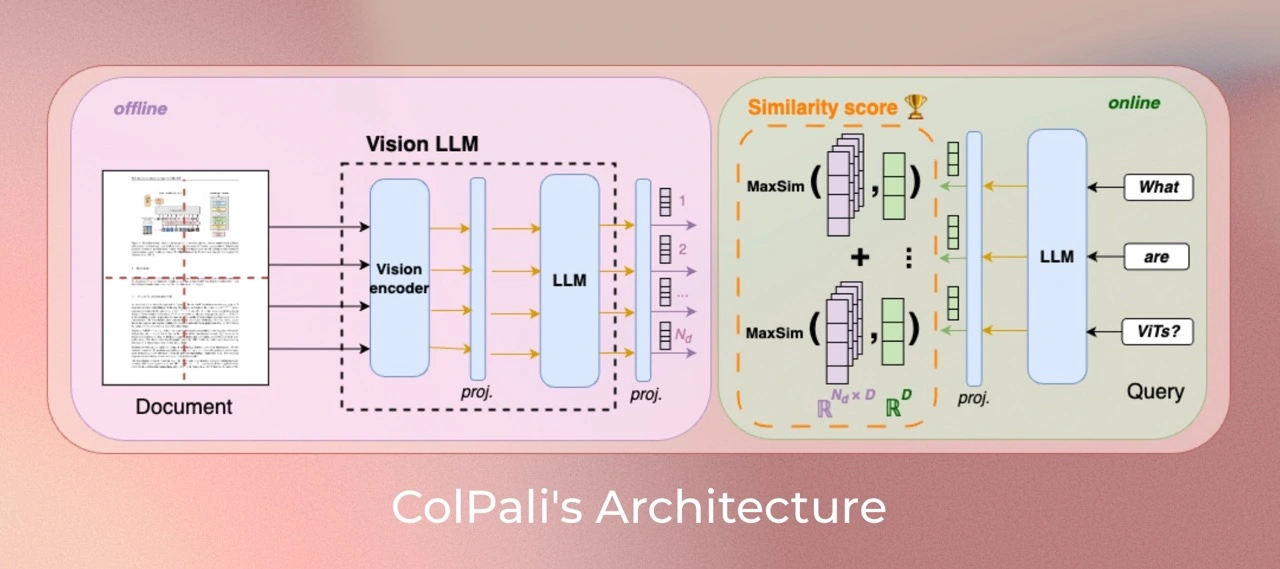
## Version specificity
This model takes dynamic image resolutions in input and does not resize them, changing their aspect ratio as in ColPali.
Maximal resolution is set so that 768 image patches are created at most. Experiments show clear improvements with larger amounts of image patches, at the cost of memory requirements.
This version is trained with `colpali-engine==0.3.7`.
## Data
- **Synthetic data**: Selected and preprocessed from the `openbmb/VisRAG-Ret-Train-Synthetic-data` dataset.
- **In-domain VQA dataset**: Drawn from `openbmb/VisRAG-Ret-Train-In-domain-data`.
- **Docmatix dataset**: Extracted from the `Metric-AI/rag_docmatix_100k` dataset.
- **Colpali dataset**: Taken from `vidore/colpali_train_set`.
- **Multilingual dataset**: Taken from `llamaindex/vdr-multilingual-train`.
## Model Training
### Parameters
We train models use low-rank adapters ([LoRA](https://arxiv.org/abs/2106.09685))
with `alpha=128` and `r=128` on the transformer layers from the language model,
as well as the final randomly initialized projection layer, and use a `paged_adamw_8bit` optimizer.
We train on an 4xA100 GPU setup with distributed data parallelism (via accelerate), a learning rate of 2e-4 with linear decay with 1% warmup steps, batch size per device is 128, gradient accumulation steps are 2, in `bfloat16` format
## Installation
Make sure `colpali-engine` is installed from source or with a version superior to 0.3.1.
`transformers` version must be > 4.45.0.
### ColPali
```bash
pip install git+https://github.com/illuin-tech/colpali
```
or
```bash
pip install git+https://github.com/illuin-tech/colpali@colqwen2_5
```
### Qwen2.5
The code of Qwen2.5-VL has been in the latest Hugging face transformers and we advise you to build from source with command:
```
pip install git+https://github.com/huggingface/transformers accelerate
```
or you might encounter the following error:
```
KeyError: 'qwen2_5_vl'
```
## Usage
```python
import torch
from PIL import Image
from colpali_engine.models import ColQwen2_5, ColQwen2_5_Processor
model = ColQwen2_5.from_pretrained(
"Metric-AI/colqwen2.5-3b-multilingual",
torch_dtype=torch.bfloat16,
device_map="cuda:0", # or "mps" if on Apple Silicon
).eval()
processor = ColQwen2_5_Processor.from_pretrained("Metric-AI/colqwen2.5-3b-multilingual")
# Your inputs
images = [
Image.new("RGB", (32, 32), color="white"),
Image.new("RGB", (16, 16), color="black"),
]
queries = [
"Is attention really all you need?",
"What is the amount of bananas farmed in Salvador?",
]
# Process the inputs
batch_images = processor.process_images(images).to(model.device)
batch_queries = processor.process_queries(queries).to(model.device)
# Forward pass
with torch.no_grad():
image_embeddings = model(**batch_images)
query_embeddings = model(**batch_queries)
scores = processor.score_multi_vector(query_embeddings, image_embeddings)
```
## Limitations
- **Focus**: The model primarily focuses on PDF-type documents and high-ressources languages, potentially limiting its generalization to other document types or less represented languages.
- **Support**: The model relies on multi-vector retreiving derived from the ColBERT late interaction mechanism, which may require engineering efforts to adapt to widely used vector retrieval frameworks that lack native multi-vector support.
## License
ColQwen2.5's vision language backbone model (Qwen2.5-VL) is under `apache2.0` license. The adapters attached to the model are under MIT license.
## Citation
If you use this models from this organization in your research, please cite the original paper as follows:
```bibtex
@misc{faysse2024colpaliefficientdocumentretrieval,
title={ColPali: Efficient Document Retrieval with Vision Language Models},
author={Manuel Faysse and Hugues Sibille and Tony Wu and Bilel Omrani and Gautier Viaud and Céline Hudelot and Pierre Colombo},
year={2024},
eprint={2407.01449},
archivePrefix={arXiv},
primaryClass={cs.IR},
url={https://arxiv.org/abs/2407.01449},
}
```
- **Developed by:** [Metric AI Research Lab](https://metric.am/)