Update README.md
Browse files
README.md
CHANGED
@@ -1,23 +1,264 @@
|
|
|
|
|
|
|
|
|
|
|
|
|
|
|
|
|
|
|
|
|
|
|
|
|
|
|
|
|
|
|
|
|
|
|
|
|
|
|
|
|
|
|
|
|
|
|
|
|
|
|
|
|
|
|
|
|
|
|
|
|
|
|
|
|
|
|
|
|
|
|
|
|
|
|
|
|
|
|
|
|
|
|
|
|
|
|
|
|
|
|
|
|
|
|
|
|
|
|
|
|
|
|
|
|
|
|
|
|
|
|
|
|
|
|
|
|
|
|
|
|
|
|
|
|
|
|
|
|
|
|
|
|
|
|
|
|
|
|
|
|
|
|
|
|
|
|
|
|
|
|
|
|
|
|
|
|
|
|
|
|
|
|
|
|
|
|
|
|
|
|
|
|
|
|
|
|
|
|
|
|
|
|
|
|
|
|
|
|
|
|
|
|
|
|
|
|
|
|
|
|
|
|
|
|
|
|
|
|
|
|
|
|
|
|
|
|
|
|
|
|
|
|
|
|
|
|
|
|
|
|
|
|
|
|
|
|
|
|
|
|
|
|
|
|
|
|
|
|
|
|
|
|
|
|
|
|
|
|
|
|
|
|
|
|
|
|
|
|
|
|
|
|
|
|
|
|
|
|
|
|
|
|
|
|
|
|
|
|
|
|
|
|
|
|
|
|
|
|
|
|
|
|
|
|
|
|
|
|
|
|
|
|
|
|
|
|
|
|
|
|
|
|
|
|
|
|
|
|
|
|
|
|
|
|
|
|
|
|
|
|
|
|
|
|
|
|
|
|
|
|
|
|
|
|
|
|
|
|
|
|
|
|
|
|
|
|
|
|
|
|
|
|
|
|
|
|
|
|
|
|
|
|
|
|
|
|
|
|
|
|
|
|
|
|
|
|
|
|
|
|
|
|
|
|
|
|
|
|
|
|
|
|
|
|
|
|
|
|
|
|
|
|
|
|
|
|
|
|
|
|
|
|
|
|
|
|
|
|
|
|
|
|
|
|
|
|
|
|
|
|
|
|
|
|
|
|
|
|
|
|
|
|
|
|
|
|
|
|
|
|
|
|
|
|
|
|
|
|
|
|
|
1 |
---
|
2 |
-
|
3 |
-
|
4 |
-
|
5 |
-
|
6 |
-
|
7 |
-
- qwen2
|
8 |
-
- trl
|
9 |
-
- sft
|
10 |
-
license: apache-2.0
|
11 |
-
language:
|
12 |
-
- en
|
13 |
---
|
14 |
|
15 |
-
|
16 |
|
17 |
-
|
18 |
-
- **License:** apache-2.0
|
19 |
-
- **Finetuned from model :** MasterControlAIML/DeepSeek-R1-Strategy-Qwen-2.5-1.5b-Unstructured-To-Structured
|
20 |
|
21 |
-
|
|
|
|
|
|
|
|
|
|
|
|
|
|
|
|
|
|
|
22 |
|
23 |
-
|
|
|
1 |
+
Below is an improved version of the README in Markdown format. You can copy and paste the following text into your README file.
|
2 |
+
|
3 |
+
---
|
4 |
+
|
5 |
+
# MasterControlAIML R1-Qwen2.5-1.5b SFT R1 JSON Unstructured-To-Structured LoRA Model
|
6 |
+
|
7 |
+
[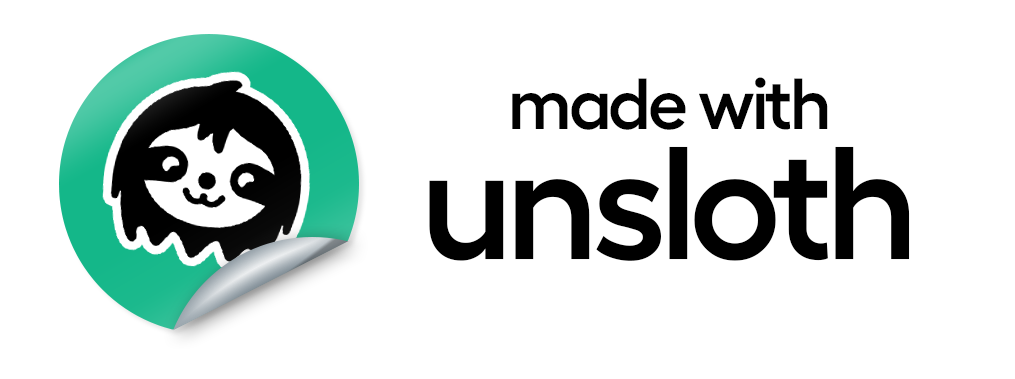](https://github.com/unslothai/unsloth)
|
8 |
+
|
9 |
+
This repository provides a fine-tuned Qwen2 model optimized for transforming unstructured text into structured JSON outputs according to a predefined schema. The model is finetuned from the base model **MasterControlAIML/DeepSeek-R1-Strategy-Qwen-2.5-1.5b-Unstructured-To-Structured** and leverages LoRA techniques for efficient adaptation.
|
10 |
+
|
11 |
+
> **Key Highlights:**
|
12 |
+
>
|
13 |
+
> - **Developed by:** [bhaviktheslider](https://github.com/bhaviktheslider)
|
14 |
+
> - **License:** [Apache-2.0](LICENSE)
|
15 |
+
> - **Finetuned from:** `MasterControlAIML/DeepSeek-R1-Strategy-Qwen-2.5-1.5b-Unstructured-To-Structured`
|
16 |
+
> - **Accelerated Training:** Achieved 2x faster training using [Unsloth](https://github.com/unslothai/unsloth) and Hugging Face's TRL library.
|
17 |
+
|
18 |
+
---
|
19 |
+
|
20 |
+
## Table of Contents
|
21 |
+
|
22 |
+
- [Overview](#overview)
|
23 |
+
- [Features](#features)
|
24 |
+
- [Installation](#installation)
|
25 |
+
- [Quick Start](#quick-start)
|
26 |
+
- [Using Unsloth for Fast Inference](#using-unsloth-for-fast-inference)
|
27 |
+
- [Using Transformers for Inference](#using-transformers-for-inference)
|
28 |
+
- [Advanced Example with LangChain Prompt](#advanced-example-with-langchain-prompt)
|
29 |
+
- [Contributing](#contributing)
|
30 |
+
- [License](#license)
|
31 |
+
- [Acknowledgments](#acknowledgments)
|
32 |
+
|
33 |
+
---
|
34 |
+
|
35 |
+
## Overview
|
36 |
+
|
37 |
+
This model is tailored for tasks where mapping unstructured text (e.g., manuals, QA documents) into a structured JSON format is required. It supports hierarchical data extraction based on a given JSON Schema, ensuring that the generated outputs follow the exact structure and rules defined by the schema.
|
38 |
+
|
39 |
+
---
|
40 |
+
|
41 |
+
## Features
|
42 |
+
|
43 |
+
- **Efficient Inference:** Utilizes the [Unsloth](https://github.com/unslothai/unsloth) library for fast model inference.
|
44 |
+
- **Structured Output:** Maps text inputs into a strict JSON schema with hierarchical relationships.
|
45 |
+
- **Flexible Integration:** Example code snippets show how to use both the Unsloth API and Hugging Face’s Transformers.
|
46 |
+
- **Advanced Prompting:** Includes an example of using LangChain prompt templates for detailed instruction-driven output.
|
47 |
+
|
48 |
+
---
|
49 |
+
|
50 |
+
## Installation
|
51 |
+
|
52 |
+
### Prerequisites
|
53 |
+
|
54 |
+
- **Python:** 3.8+
|
55 |
+
- **PyTorch:** (Preferably with CUDA support)
|
56 |
+
- **Required Libraries:** `transformers`, `torch`, `unsloth`, `langchain` (for advanced usage)
|
57 |
+
|
58 |
+
### Installation Command
|
59 |
+
|
60 |
+
Install the required Python packages with:
|
61 |
+
|
62 |
+
```bash
|
63 |
+
pip install torch transformers unsloth langchain
|
64 |
+
```
|
65 |
+
|
66 |
+
---
|
67 |
+
|
68 |
+
## Quick Start
|
69 |
+
|
70 |
+
### Using Unsloth for Fast Inference
|
71 |
+
|
72 |
+
The Unsloth library allows you to quickly load and run inference with the model. Below is a basic example:
|
73 |
+
|
74 |
+
```python
|
75 |
+
from unsloth import FastLanguageModel
|
76 |
+
import torch
|
77 |
+
|
78 |
+
# Specify the model name
|
79 |
+
MODEL = "MasterControlAIML/R1-Qwen2.5-1.5b-SFT-R1-JSON-Unstructured-To-Structured-lora"
|
80 |
+
|
81 |
+
# Load the model and tokenizer
|
82 |
+
model, tokenizer = FastLanguageModel.from_pretrained(
|
83 |
+
model_name=MODEL,
|
84 |
+
max_seq_length=2048,
|
85 |
+
dtype=None,
|
86 |
+
load_in_4bit=False,
|
87 |
+
)
|
88 |
+
|
89 |
+
# Prepare the model for inference
|
90 |
+
FastLanguageModel.for_inference(model)
|
91 |
+
|
92 |
+
# Define a prompt template
|
93 |
+
ALPACA_PROMPT = """
|
94 |
+
Below is an instruction that describes a task, paired with an input that provides further context. Write a response that appropriately completes the request.
|
95 |
+
### Instruction:
|
96 |
+
{}
|
97 |
+
### Response:
|
98 |
+
{}
|
99 |
+
"""
|
100 |
+
|
101 |
+
# Example: Create input and generate output
|
102 |
+
instruction = "Provide a summary of the Quality Assurance Manual."
|
103 |
+
prompt = ALPACA_PROMPT.format(instruction, "")
|
104 |
+
inputs = tokenizer([prompt], return_tensors="pt").to("cuda")
|
105 |
+
output = model.generate(**inputs, max_new_tokens=2000)
|
106 |
+
|
107 |
+
# Decode and print the generated text
|
108 |
+
print(tokenizer.batch_decode(output, skip_special_tokens=True)[0])
|
109 |
+
```
|
110 |
+
|
111 |
+
---
|
112 |
+
|
113 |
+
### Using Transformers for Inference
|
114 |
+
|
115 |
+
If you prefer to use Hugging Face's Transformers directly, here’s an alternative example:
|
116 |
+
|
117 |
+
```python
|
118 |
+
from transformers import AutoTokenizer, AutoModelForCausalLM, TextStreamer
|
119 |
+
import torch
|
120 |
+
|
121 |
+
MODEL = "MasterControlAIML/R1-Qwen2.5-1.5b-SFT-R1-JSON-Unstructured-To-Structured-lora"
|
122 |
+
|
123 |
+
# Initialize tokenizer and model
|
124 |
+
tokenizer = AutoTokenizer.from_pretrained(MODEL)
|
125 |
+
model = AutoModelForCausalLM.from_pretrained(MODEL, torch_dtype=torch.float16, device_map="auto")
|
126 |
+
|
127 |
+
ALPACA_PROMPT = """
|
128 |
+
Below is an instruction that describes a task, paired with an input that provides further context. Write a response that appropriately completes the request.
|
129 |
+
### Instruction:
|
130 |
+
{}
|
131 |
+
### Response:
|
132 |
+
{}
|
133 |
+
"""
|
134 |
+
|
135 |
+
# Define your text input
|
136 |
+
TEXT = "Provide a detailed explanation of the QA processes in manufacturing."
|
137 |
+
prompt = ALPACA_PROMPT.format(TEXT, "")
|
138 |
+
inputs = tokenizer([prompt], return_tensors="pt").to("cuda")
|
139 |
+
text_streamer = TextStreamer(tokenizer)
|
140 |
+
|
141 |
+
# Generate output with specific generation parameters
|
142 |
+
with torch.no_grad():
|
143 |
+
output_ids = model.generate(
|
144 |
+
input_ids=inputs["input_ids"],
|
145 |
+
attention_mask=inputs["attention_mask"],
|
146 |
+
max_new_tokens=2000,
|
147 |
+
temperature=0.7,
|
148 |
+
top_p=0.9,
|
149 |
+
repetition_penalty=1.1,
|
150 |
+
streamer=text_streamer,
|
151 |
+
pad_token_id=tokenizer.pad_token_id,
|
152 |
+
)
|
153 |
+
|
154 |
+
# Print the decoded output
|
155 |
+
print(tokenizer.decode(output_ids[0], skip_special_tokens=True))
|
156 |
+
```
|
157 |
+
|
158 |
+
---
|
159 |
+
|
160 |
+
### Advanced Example with LangChain Prompt
|
161 |
+
|
162 |
+
For advanced users, the repository includes an example that integrates with LangChain to map hierarchical text data into a JSON schema. This example uses a prompt template to instruct the model on how to generate an output that includes both the JSON object (`<answer>`) and the reasoning behind the mapping decisions (`<think>`).
|
163 |
+
|
164 |
+
```python
|
165 |
+
from langchain_core.prompts import PromptTemplate
|
166 |
+
|
167 |
+
SYSTEM_PROMPT = """
|
168 |
+
### Role:
|
169 |
+
You are an expert data extractor specializing in mapping hierarchical text data into a given JSON Schema.
|
170 |
+
|
171 |
+
### DATA INPUT:
|
172 |
+
- **Text:** ```{TEXT}```
|
173 |
+
- **Blank JSON Schema:** ```{SCHEMA}```
|
174 |
+
|
175 |
+
### TASK REQUIREMENT:
|
176 |
+
1. Analyze the given text and map all relevant information strictly into the provided JSON Schema.
|
177 |
+
2. Provide your output in **two mandatory sections**:
|
178 |
+
- **`<answer>`:** The filled JSON object
|
179 |
+
- **`<think>`:** Reasoning for the mapping decisions
|
180 |
+
|
181 |
+
### OUTPUT STRUCTURE:
|
182 |
+
```
|
183 |
+
<think> /* Explanation of mapping logic */ </think>
|
184 |
+
<answer> /* Completed JSON Object */ </answer>
|
185 |
+
```
|
186 |
+
|
187 |
+
### STRICT RULES FOR GENERATING OUTPUT:
|
188 |
+
1. **Both Tags Required:**
|
189 |
+
- Always provide both the `<think>` and `<answer>` sections.
|
190 |
+
- If reasoning is minimal, state: "Direct mapping from text to schema."
|
191 |
+
2. **JSON Schema Mapping:**
|
192 |
+
- Strictly map the text data to the given JSON Schema without modification or omissions.
|
193 |
+
3. **Hierarchy Preservation:**
|
194 |
+
- Maintain proper parent-child relationships and follow the schema's hierarchical structure.
|
195 |
+
4. **Correct Mapping of Attributes:**
|
196 |
+
- Map key attributes, including `id`, `idc`, `idx`, `level_type`, and `component_type`.
|
197 |
+
5. **JSON Format Compliance:**
|
198 |
+
- Escape quotes, replace newlines with `\\n`, avoid trailing commas, and use double quotes exclusively.
|
199 |
+
6. **Step-by-Step Reasoning:**
|
200 |
+
- Explain your reasoning within the `<think>` tag.
|
201 |
+
|
202 |
+
### IMPORTANT:
|
203 |
+
If either the `<think>` or `<answer>` tags is missing, the response will be considered incomplete.
|
204 |
+
"""
|
205 |
+
|
206 |
+
# Create a prompt template with LangChain
|
207 |
+
system_prompt_template = PromptTemplate(template=SYSTEM_PROMPT, input_variables=["TEXT", "SCHEMA"])
|
208 |
+
|
209 |
+
# Format the prompt with your text and JSON schema
|
210 |
+
system_prompt_str = system_prompt_template.format(
|
211 |
+
TEXT="Your detailed text input here...",
|
212 |
+
SCHEMA="""{
|
213 |
+
"type": "object",
|
214 |
+
"properties": {
|
215 |
+
"id": {"type": "string", "description": "Unique identifier."},
|
216 |
+
"title": {"type": "string", "description": "Section title."},
|
217 |
+
"level": {"type": "integer", "description": "Hierarchy level."},
|
218 |
+
"level_type": {"type": "string", "enum": ["ROOT", "SECTION", "SUBSECTION", "DETAIL_N"], "description": "Hierarchy type."},
|
219 |
+
"component": {
|
220 |
+
"type": "array",
|
221 |
+
"items": {
|
222 |
+
"type": "object",
|
223 |
+
"properties": {
|
224 |
+
"idc": {"type": "integer", "description": "Component ID."},
|
225 |
+
"component_type": {"type": "string", "enum": ["PARAGRAPH", "TABLE", "CALCULATION", "CHECKBOX"], "description": "Component type."},
|
226 |
+
"metadata": {"type": "string", "description": "Additional metadata."},
|
227 |
+
"properties": {"type": "object"}
|
228 |
+
},
|
229 |
+
"required": ["idc", "component_type", "metadata", "properties"]
|
230 |
+
}
|
231 |
+
},
|
232 |
+
"children": {"type": "array", "items": {}}
|
233 |
+
},
|
234 |
+
"required": ["id", "title", "level", "level_type", "component", "children"]
|
235 |
+
}"""
|
236 |
+
)
|
237 |
+
|
238 |
+
# Use the system prompt with your inference code as shown in previous examples.
|
239 |
+
```
|
240 |
+
|
241 |
---
|
242 |
+
|
243 |
+
## Contributing
|
244 |
+
|
245 |
+
Contributions, bug reports, and feature requests are welcome! Please open an issue or submit a pull request if you would like to contribute to this project.
|
246 |
+
|
|
|
|
|
|
|
|
|
|
|
|
|
247 |
---
|
248 |
|
249 |
+
## License
|
250 |
|
251 |
+
This project is licensed under the [Apache-2.0 License](LICENSE).
|
|
|
|
|
252 |
|
253 |
+
---
|
254 |
+
|
255 |
+
## Acknowledgments
|
256 |
+
|
257 |
+
- **Unsloth:** For providing fast model inference capabilities. ([GitHub](https://github.com/unslothai/unsloth))
|
258 |
+
- **Hugging Face:** For the [Transformers](https://github.com/huggingface/transformers) and [TRL](https://github.com/huggingface/trl) libraries.
|
259 |
+
- **LangChain:** For advanced prompt management and integration.
|
260 |
+
- And, of course, thanks to the community and contributors who helped shape this project.
|
261 |
+
|
262 |
+
---
|
263 |
|
264 |
+
Enjoy using the model, and happy coding!
|